file_path
stringlengths 21
202
| content
stringlengths 19
1.02M
| size
int64 19
1.02M
| lang
stringclasses 8
values | avg_line_length
float64 5.88
100
| max_line_length
int64 12
993
| alphanum_fraction
float64 0.27
0.93
|
---|---|---|---|---|---|---|
MomentFactory/Omniverse-Lidar-Live-Synthetic-Data/source/extensions/mf.ov.lidar_live_synth/config/extension.toml | [package]
version = "0.2.0"
title = "MF Lidar live synthetic data"
description = "Send real-time Lidar synthetic point cloud data from Omniverse to third party software."
category = "Graph"
keywords = ["lidar", "UDP", "omnigraph", "Graph", "Node", "OmniGraph", "synthetic", "realtime"]
preview_image = "data/preview.png"
icon = "data/icon.png"
changelog = "docs/CHANGELOG.md"
readme = "docs/README.md"
authors = ["Moment Factory","Frederic Lestage","Steven Beliveau"]
repository = "https://github.com/MomentFactory/Omniverse-Lidar-extension"
[dependencies]
"omni.graph" = {}
[[python.module]]
name = "mf.ov.lidar_live_synth"
[[native.plugin]]
path = "bin/*.plugin"
[documentation]
pages = [
"docs/README.md",
"docs/CHANGELOG.md",
]
[package.target]
kit = ["105.1"]
[package.writeTarget]
kit = true
python = false
| 829 | TOML | 22.055555 | 103 | 0.694813 |
MomentFactory/Omniverse-Lidar-Live-Synthetic-Data/source/extensions/mf.ov.lidar_live_synth/plugins/mf.ov.lidar_live_synth/LidarLiveSyntheticDataExtension.cpp | #define CARB_EXPORTS
#include <carb/PluginUtils.h>
#include <omni/ext/IExt.h>
#include <omni/graph/core/IGraphRegistry.h>
#include <omni/graph/core/ogn/Database.h>
#include <omni/graph/core/ogn/Registration.h>
// Standard plugin definitions required by Carbonite.
const struct carb::PluginImplDesc pluginImplDesc = { "mf.ov.lidar_live_synth.plugin",
"MF Lidar live synthetic data.", "MF",
carb::PluginHotReload::eEnabled, "dev" };
// These interface dependencies are required by all OmniGraph node types
CARB_PLUGIN_IMPL_DEPS(omni::graph::core::IGraphRegistry,
omni::fabric::IPath,
omni::fabric::IToken)
// This macro sets up the information required to register your node type definitions with OmniGraph
DECLARE_OGN_NODES()
namespace mf
{
namespace ov
{
namespace lidar_live_synth
{
class LidarLiveSyntheticDataExtension : public omni::ext::IExt
{
public:
void onStartup(const char* extId) override
{
// This macro walks the list of pending node type definitions and registers them with OmniGraph
INITIALIZE_OGN_NODES()
}
void onShutdown() override
{
// This macro walks the list of registered node type definitions and deregisters all of them. This is required
// for hot reload to work.
RELEASE_OGN_NODES()
}
private:
};
}
}
}
CARB_PLUGIN_IMPL(pluginImplDesc, mf::ov::lidar_live_synth::LidarLiveSyntheticDataExtension)
void fillInterface(mf::ov::lidar_live_synth::LidarLiveSyntheticDataExtension& iface)
{
}
| 1,622 | C++ | 26.982758 | 118 | 0.676326 |
MomentFactory/Omniverse-Lidar-Live-Synthetic-Data/source/extensions/mf.ov.lidar_live_synth/plugins/nodes/OgnBeamToOusterUDPNode.cpp | #include <OgnBeamToOusterUDPNodeDatabase.h>
#include <chrono>
#define WIN32_LEAN_AND_MEAN
#define _WINSOCK_DEPRECATED_NO_WARNINGS
#ifdef _WIN32
#include <Winsock2.h>
#else
#include <arpa/inet.h>
#include <netdb.h>
#include <netinet/in.h>
#include <sys/select.h>
#include <sys/socket.h>
#include <sys/types.h>
#define SOCKET int
#define INVALID_SOCKET (SOCKET)(~0)
#define SOCKET_ERROR (-1)
#define closesocket close
#define SOCKADDR sockaddr
#endif
namespace mf {
namespace ov {
namespace lidar_live_synth {
static const int kColumnsPerPacket = 16;
static const float kPi = 3.14159265359f;
static const float kTwoPi = kPi * 2.0f;
static const float kDegToRad = kTwoPi / 360.0f;
static const int kOusterNumRotAngles = 90112;
static const float kOusterNumRotAnglesOverTwoPi = kOusterNumRotAngles / kTwoPi;
class OgnBeamToOusterUDPNode
{
int m_frameId{ 0 };
#pragma pack(push,4) // Force packing in 4-byte packs (Words)
struct OusterChannelDataBlock
{
unsigned int rangemm;
unsigned short reflectivity;
unsigned short signal_photons;
unsigned short noise_photons;
unsigned short unused;
OusterChannelDataBlock()
: rangemm(0)
, reflectivity(0)
, signal_photons(0)
, noise_photons(0)
, unused(0)
{}
};
template <int NUMROWS>
struct OusterAzimuthBlock
{
unsigned long long timeStamp; // Word 0,1
unsigned short measurementId; // Word 2[0:15]
unsigned short frameId; // Word 2[16:31]
unsigned int encoderCount; // Word 3
OusterChannelDataBlock channelDataBlock[NUMROWS]; // Word [4:195] in groups of 3
unsigned int azimuthDataBlockStatus; // word 196
OusterAzimuthBlock()
: timeStamp(0)
, measurementId(0)
, frameId(0)
, encoderCount(0)
, channelDataBlock{}
, azimuthDataBlockStatus(0)
{}
};
template <int NUMROWS>
struct OusterDataPacket
{
OusterAzimuthBlock<NUMROWS> block[16]; // Each packet consists of 16 azimuth blocks
OusterDataPacket()
:block{}
{}
};
#pragma pack(pop)
class OgnBeamToOusterUDPNodeSocket
{
public:
OgnBeamToOusterUDPNodeSocket()
: SendSocket(INVALID_SOCKET)
, isBroadcastSocket(false)
{}
virtual ~OgnBeamToOusterUDPNodeSocket()
{
if (SendSocket != INVALID_SOCKET)
{
closesocket(SendSocket);
}
}
bool prepare(OgnBeamToOusterUDPNodeDatabase& db)
{
if (isBroadcastSocket != db.inputs.broadcast())
{
closesocket(SendSocket);
SendSocket = INVALID_SOCKET;
}
if (SendSocket == INVALID_SOCKET)
{
SendSocket = socket(AF_INET, SOCK_DGRAM, IPPROTO_UDP);
if (SendSocket == INVALID_SOCKET)
{
db.logError("Error in OgnBeamToOusterUDPNode opening socket : %d", SendSocket);
return false;
}
if (db.inputs.broadcast())
{
char broadcast = 1;
int iResult = setsockopt(SendSocket, SOL_SOCKET, SO_BROADCAST, &broadcast, sizeof(broadcast));
if (!iResult)
{
closesocket(SendSocket);
SendSocket = INVALID_SOCKET;
db.logError("Error in OgnBeamToOusterUDPNode setting socket options : %d", iResult);
return false;
}
}
isBroadcastSocket = db.inputs.broadcast();
}
RecvAddr.sin_family = AF_INET;
RecvAddr.sin_port = htons(db.inputs.port());
std::string ipAddress = db.inputs.ip_address();
RecvAddr.sin_addr.s_addr = inet_addr(ipAddress.data());
return true;
}
template <int NUMROWS>
bool send(const OusterDataPacket<NUMROWS>& packet, OgnBeamToOusterUDPNodeDatabase& db)
{
int iResult = sendto(SendSocket, reinterpret_cast<const char*>(&packet), sizeof(packet), 0, (SOCKADDR*)&RecvAddr, sizeof(RecvAddr));
if (iResult == SOCKET_ERROR)
{
db.logError("Error in OgnBeamToOusterUDPNode sending data on socket : %d", iResult);
return false;
}
return true;
}
private:
SOCKET SendSocket;
sockaddr_in RecvAddr;
bool isBroadcastSocket;
};
OgnBeamToOusterUDPNodeSocket m_ognBeamToOusterUDPNodeSocket;
template<int NUMROWS>
static bool computeForSize(OgnBeamToOusterUDPNodeDatabase& db)
{
auto& state = db.internalState<OgnBeamToOusterUDPNode>();
const auto& linearDepthData = db.inputs.linearDepthData();
const int& numCols = db.inputs.numCols();
const float& azimuthStart = db.inputs.azimuthRange()[0] + kTwoPi + kTwoPi;
const float& horizontalStepInRads = -1.0f * db.inputs.horizontalResolution() * kDegToRad;
const int& frameId = state.m_frameId % 65536;
try
{
if (!state.m_ognBeamToOusterUDPNodeSocket.prepare(db))
{
return false;
}
int measurementId = 0;
OusterDataPacket<NUMROWS> packet;
int currentChunkColumn = 0;
// We need to send data in ascending angle (encoder_count) order
// Data is in right-to-left order, we need to iterate left-to-right
// We also need to start at the middle (center) of the data which is encoderCount 0
int colEndIndex = (numCols - 1) / 2;
int colStartIndex = colEndIndex + numCols;
for (int tempColIndex = colStartIndex; tempColIndex > colEndIndex; tempColIndex--)
{
int colIndex = tempColIndex % numCols;
// This assumes consistent input data across azimuthRange, horizontalResolution, numCols, numRows and linearDepthData size
int currentEncoderCount = int((azimuthStart + horizontalStepInRads * tempColIndex) * kOusterNumRotAnglesOverTwoPi);
if (currentEncoderCount < 0 || currentEncoderCount >= kOusterNumRotAngles)
{
db.logError("currentEncoderCount must be between 0 and %d, not %d", kOusterNumRotAngles, currentEncoderCount);
return false;
}
// If previous chunk is complete, start new one
if (currentChunkColumn == kColumnsPerPacket)
{
state.m_ognBeamToOusterUDPNodeSocket.send<NUMROWS>(packet, db);
packet = OusterDataPacket<NUMROWS>();
currentChunkColumn = 0;
}
packet.block[currentChunkColumn].timeStamp =
std::chrono::duration_cast<std::chrono::nanoseconds>(std::chrono::system_clock::now().time_since_epoch()).count();
packet.block[currentChunkColumn].measurementId = measurementId;
packet.block[currentChunkColumn].frameId = frameId;
packet.block[currentChunkColumn].encoderCount = currentEncoderCount;
measurementId = (measurementId + 1) % 65536;
int colIndexStart = colIndex * NUMROWS;
for (int rowIndex = 0; rowIndex < NUMROWS; rowIndex++)
{
packet.block[currentChunkColumn].channelDataBlock[rowIndex].rangemm = (int)(linearDepthData[colIndexStart + rowIndex] * 1000.0f);
packet.block[currentChunkColumn].channelDataBlock[rowIndex].signal_photons = 0xFFFF; //0xFFFF means valid
}
packet.block[currentChunkColumn].azimuthDataBlockStatus = 0xFFFFFFFF; //0xFFFFFFFF means valid
currentChunkColumn++;
}
if (currentChunkColumn != 0)
{
for (int extraColumnIndex = currentChunkColumn; extraColumnIndex < kColumnsPerPacket; extraColumnIndex++)
{
packet.block[extraColumnIndex].timeStamp =
std::chrono::duration_cast<std::chrono::nanoseconds>(std::chrono::system_clock::now().time_since_epoch()).count();
packet.block[extraColumnIndex].measurementId = measurementId;
packet.block[extraColumnIndex].frameId = frameId;
packet.block[extraColumnIndex].encoderCount = kOusterNumRotAngles;
}
state.m_ognBeamToOusterUDPNodeSocket.send<NUMROWS>(packet, db);
}
}
catch (...)
{
db.logError("Error in OgnBeamToOusterUDPNode::compute");
return false;
}
state.m_frameId++;
// Always enable the output execution
db.outputs.execOut() = omni::graph::core::ExecutionAttributeState::kExecutionAttributeStateEnabled;
// Even if inputs were edge cases like empty arrays, correct outputs mean success
return true;
}
public:
static bool compute(OgnBeamToOusterUDPNodeDatabase& db)
{
// TODO: why is state declared here
// auto& state = db.internalState<OgnBeamToOusterUDPNode>();
const int& numRows = db.inputs.numRows();
switch (numRows)
{
case 16:
return computeForSize<16>(db);
break;
case 32:
return computeForSize<32>(db);
break;
case 64:
return computeForSize<64>(db);
break;
case 128:
return computeForSize<128>(db);
break;
}
db.logError("Row count must be either 16, 32, 64 or 128, not %d", numRows);
return false;
}
};
// This macro provides the information necessary to OmniGraph that lets it automatically register and deregister
// your node type definition.
REGISTER_OGN_NODE()
}
}
}
| 10,237 | C++ | 32.348534 | 149 | 0.58142 |
MomentFactory/Omniverse-Lidar-Live-Synthetic-Data/source/extensions/mf.ov.lidar_live_synth/docs/CHANGELOG.md | # Changelog
## [0.2.0] - 2023-12-20
### Modified
- Adapted for compatibility with kit 105
- Enhanced documentation
### Added
- Linux support thanks to [@Samahu](https://github.com/Samahu)'s PR on Github
## [0.1.3] - 2023-08-30
### Changed
- Version bump for registry publishing
## [0.1.2] - 2023-08-30
### Added
- New example with more Lidars
### Modified
- Now comes as C++ for maximum performance.
## [0.1.1] - 2023-05-09
### Added
- Documentation
### Modified
- Name of the Node
- Icon
## [0.1.0] - 2023-05-09
### Added
- Action Graph Node that sends Isaac Lidar Point Cloud data in UDP
| 603 | Markdown | 14.894736 | 77 | 0.658375 |
MomentFactory/Omniverse-Lidar-Live-Synthetic-Data/source/extensions/mf.ov.lidar_live_synth/docs/README.md | # MF Lidar live synthetic data [mf.ov.lidar_live_synth]
Adds an Action Graph Node ("Generic/Beam to Ouster UDP") to send Isaac beam data via the Ouster(tm) UDP procotol.
This allows any third party software implementing Ouster(tm) lidars to be connected to simulated sensors instead of physical sensors.
Developped for kit 105.1 and currently working only in Isaac Sim.
This extensions provides pre-built binaries for Windows and Linux x86_64.
You may want to compile from the [source code](https://github.com/MomentFactory/Omniverse-Lidar-Live-Synthetic-Data) | 562 | Markdown | 69.374991 | 133 | 0.798932 |
openhackathons-org/End-to-End-AI-for-Science/CONTRIBUTING.md | Contributing
------------
Please use the following guidelines when contributing to this project.
Before contributing significant changes, please begin a discussion of the desired changes via a GitHub Issue to prevent doing unnecessary or overlapping work.
## License
The preferred license for source code contributed to this project is the Apache License 2.0 (https://www.apache.org/licenses/LICENSE-2.0) and for documentation, including Jupyter notebooks and text documentation, is the Creative Commons Attribution 4.0 International (CC BY 4.0) (https://creativecommons.org/licenses/by/4.0/). Contributions under other, compatible licenses will be considered on a case-by-case basis.
## Styling
Please use the following style guidelines when making contributions.
### Source Code
* Tab indentation, no spaces
* To the extent possible, variable names should be descriptive
* Code should be documentation with detail like what function does and returns making the code readable. The code should also have proper license at the beginning of the file.
* The following file extensions should be used appropriately:
* Python = .py
### Jupyter Notebooks & Markdown
* When they appear inline with the text; directive names, clauses, function or subroutine names, variable names, file names, commands and command-line arguments should appear between two backticks.
* Code blocks should begin with three backticks and either 'python' or 'yaml' to enable appropriate source formatting and end with three backticks.
* Leave an empty line before and after the codeblock.
Emphasis, including quotes made for emphasis and introduction of new terms should be highlighted between a single pair of asterisks
* A level 1 heading should appear at the top of the notebook as the title of the notebook.
* A horizontal rule should appear between sections that begin with a level 2 heading.
Please refer to the following template for jupyter notebook styling in the github repository:misc/jupyter_lab_template
## Contributing Labs/Modules
### Directory stucture for Github
Before starting to work on new lab it is important to follow the recommended git structure as shown below to avoid reformatting.
Each lab will have following files/directories consisting of training material for the lab.
* jupyter_notebook folder: Consists of jupyter notebooks and its corresponding images.
* source_code folder: Source codes are stored in a separate directory because sometime not all clusters may support jupyter notebooks. During such bootcamps, we should be able to use the source codes directly from this directory. Source code folder may optionally contain Makefile especially for HPC labs.
* presentations: Consists of presentations for the labs ( pdf format is preferred )
* Dockerfile and Singularity: Each lab should have both Docker and Singularity recipes.
The lab optionally may also add custom license in case of any deviation from the top level directory license ( Apache 2.0 ). The base of the module contains individual subdirectory containing versions of the module for languages respectively(C/C++/Fortran…). Each of these directories should contain a directory for individual language translation provided (English, for instance). Each lab translation and programming language combination should have a solutions directory containing correct solutions
Additionally there are two folders "experimental" and "archived" for labs covering features which are in early access phase ( not stable ) or deprecated features repectively.
### Git Branching
Adding a new feature/lab will follow a forking workflow. Which means a feature branch development will happen on a forked repo which later gets merged into our original project (OpenHackathons.org) repository.

The 5 main steps depicted in image above are as follows:
1. Fork: To create a new lab/feature the repository must be forked. Fork will create a snapshot of repository at the time it was forked. Any new feature/lab that will be developed should be based on the develop branch of the repository.
2. Clone: Developer can than clone this new repository to local machine
Create Feature Branch: Create a new branch with a feature name in which your changes will be done. Recommend naming convention of feature branch is naming convention for branch: <feature_name>. The new changes that developer makes can be added, committed and pushed
3. Push: After the changes are committed, the developer pushes the changes to the remote branch. Push command helps the local changes to github repository
4. Pull: Submit a pull request. Upon receiving pull request a Hackathon team reviewer/owner will review the changes and upon accepting it can be merged into the develop branch of GpuHacakthons.org
Git Branch details are as follows:
* main branch: Consists of the stable branch.
* origin/main to be the main branch where the source code of HEAD always reflects a production-ready state
* Merge request is possible through: develop branch
* develop branch: branched from master branch
* Must branch from: main branch
* Must merge back into: main branch
* It is the main development branch where the source code of HEAD always reflects a state with the latest delivered development changes for the next release.
* When the source code in the develop branch reaches a stable point and is ready to be released, all of the changes should be merged back into master somehow and then tagged with a release number
* All feature development should happen by forking and branching from develop branch only.
| 5,650 | Markdown | 76.410958 | 502 | 0.80354 |
openhackathons-org/End-to-End-AI-for-Science/workspace/python/source_code/dataset.py | # Copyright (c) 2023, NVIDIA CORPORATION. All rights reserved.
#
# Permission is hereby granted, free of charge, to any person obtaining
# a copy of this software and associated documentation files (the
# "Software"), to deal in the Software without restriction, including
# without limitation the rights to use, copy, modify, merge, publish,
# distribute, sublicense, and/or sell copies of the Software, and to
# permit persons to whom the Software is furnished to do so, subject to
# the following conditions:
#
# The above copyright notice and this permission notice shall be
# included in all copies or substantial portions of the Software.
#
# THE SOFTWARE IS PROVIDED "AS IS", WITHOUT WARRANTY OF ANY KIND,
# EXPRESS OR IMPLIED, INCLUDING BUT NOT LIMITED TO THE WARRANTIES OF
# MERCHANTABILITY, FITNESS FOR A PARTICULAR PURPOSE AND
# NONINFRINGEMENT. IN NO EVENT SHALL THE AUTHORS OR COPYRIGHT HOLDERS BE
# LIABLE FOR ANY CLAIM, DAMAGES OR OTHER LIABILITY, WHETHER IN AN ACTION
# OF CONTRACT, TORT OR OTHERWISE, ARISING FROM, OUT OF OR IN CONNECTION
# WITH THE SOFTWARE OR THE USE OR OTHER DEALINGS IN THE SOFTWARE.
import gdown
import os
## FCN Dataset
url = 'https://drive.google.com/uc?id=1mSN6eLqPYEo9d9pBjSGzQ-ocLd8itP0P&export=download'
output = str(os.path.realpath(os.path.dirname(__file__)))+ '/fourcastnet/dataset.zip'
gdown.cached_download(url, output, quiet=False,proxy=None,postprocess=gdown.extractall)
os.remove(output)
## FCN Pre-trained
url = 'https://drive.google.com/uc?id=1oSkK69LGP3DfU2tlH5iaejOh94VNsMDu&export=download'
output = str(os.path.realpath(os.path.dirname(__file__)))+ '/../jupyter_notebook/FourCastNet/pre_trained.zip'
gdown.cached_download(url, output, quiet=False,proxy=None,postprocess=gdown.extractall)
os.remove(output)
## NS Data
url = 'https://drive.google.com/uc?id=1IXEGbM3NOO6Dig1sxG1stHubwb09-D2N&export=download'
output = str(os.path.realpath(os.path.dirname(__file__)))+ '/navier_stokes/dataset.zip'
gdown.cached_download(url, output, quiet=False,proxy=None,postprocess=gdown.extractall)
os.remove(output)
## FCN for Omniverse-P1
url = 'https://drive.google.com/uc?id=16YqSnstqoSJdgBzerbzYIkYagwS12lK3&export=download'
output = str(os.path.realpath(os.path.dirname(__file__)))+ '/FCN.zip'
gdown.cached_download(url, output, quiet=False,proxy=None,postprocess=gdown.extractall)
os.remove(output)
## FCN for Omniverse-P2
url = 'https://drive.google.com/uc?id=1lSSx8eKfqCcHAbDvXTeUMoZGHfVQe-HG&export=download'
output = str(os.path.realpath(os.path.dirname(__file__)))+ '/FCN/dataset.zip'
gdown.cached_download(url, output, quiet=False,proxy=None,postprocess=gdown.extractall)
os.remove(output)
## Download and Install Omniverse
url = 'https://drive.google.com/uc?id=1DugS2IbHhBPyCE-EuZczLHBZnlnFViIm&export=download'
output = str(os.path.realpath(os.path.dirname(__file__)))+'/ov.zip'
gdown.cached_download(url, output, quiet=False,proxy=None,postprocess=gdown.extractall)
os.remove(output)
| 2,958 | Python | 46.725806 | 110 | 0.772481 |
openhackathons-org/End-to-End-AI-for-Science/workspace/python/source_code/spring_mass/spring_mass_solver.py | # Copyright (c) 2023, NVIDIA CORPORATION. All rights reserved.
#
# Permission is hereby granted, free of charge, to any person obtaining
# a copy of this software and associated documentation files (the
# "Software"), to deal in the Software without restriction, including
# without limitation the rights to use, copy, modify, merge, publish,
# distribute, sublicense, and/or sell copies of the Software, and to
# permit persons to whom the Software is furnished to do so, subject to
# the following conditions:
#
# The above copyright notice and this permission notice shall be
# included in all copies or substantial portions of the Software.
#
# THE SOFTWARE IS PROVIDED "AS IS", WITHOUT WARRANTY OF ANY KIND,
# EXPRESS OR IMPLIED, INCLUDING BUT NOT LIMITED TO THE WARRANTIES OF
# MERCHANTABILITY, FITNESS FOR A PARTICULAR PURPOSE AND
# NONINFRINGEMENT. IN NO EVENT SHALL THE AUTHORS OR COPYRIGHT HOLDERS BE
# LIABLE FOR ANY CLAIM, DAMAGES OR OTHER LIABILITY, WHETHER IN AN ACTION
# OF CONTRACT, TORT OR OTHERWISE, ARISING FROM, OUT OF OR IN CONNECTION
# WITH THE SOFTWARE OR THE USE OR OTHER DEALINGS IN THE SOFTWARE.
import numpy as np
from sympy import Symbol, Eq
import modulus
from modulus.sym.hydra import ModulusConfig, instantiate_arch
from modulus.sym.solver import Solver
from modulus.sym.domain import Domain
from modulus.sym.geometry.primitives_1d import Point1D
from modulus.sym.geometry import Parameterization
from modulus.sym.domain.constraint import (
PointwiseBoundaryConstraint,
PointwiseBoundaryConstraint,
)
from modulus.sym.domain.validator import PointwiseValidator
from modulus.sym.key import Key
from modulus.sym.node import Node
from spring_mass_ode import SpringMass
@modulus.sym.main(config_path="conf", config_name="config")
def run(cfg: ModulusConfig) -> None:
# make list of nodes to unroll graph on
sm = SpringMass(k=(2, 1, 1, 2), m=(1, 1, 1))
sm_net = instantiate_arch(
input_keys=[Key("t")],
output_keys=[Key("x1"), Key("x2"), Key("x3")],
cfg=cfg.arch.fully_connected,
)
nodes = sm.make_nodes() + [
sm_net.make_node(name="spring_mass_network", jit=cfg.jit)
]
# add constraints to solver
# make geometry
geo = Point1D(0)
t_max = 10.0
t_symbol = Symbol("t")
x = Symbol("x")
time_range = {t_symbol: (0, t_max)}
# make domain
domain = Domain()
# initial conditions
IC = PointwiseBoundaryConstraint(
nodes=nodes,
geometry=geo,
outvar={"x1": 1.0, "x2": 0, "x3": 0, "x1__t": 0, "x2__t": 0, "x3__t": 0},
batch_size=cfg.batch_size.IC,
lambda_weighting={
"x1": 1.0,
"x2": 1.0,
"x3": 1.0,
"x1__t": 1.0,
"x2__t": 1.0,
"x3__t": 1.0,
},
parameterization=Parameterization({t_symbol: 0}),
)
domain.add_constraint(IC, name="IC")
# solve over given time period
interior = PointwiseBoundaryConstraint(
nodes=nodes,
geometry=geo,
outvar={"ode_x1": 0.0, "ode_x2": 0.0, "ode_x3": 0.0},
batch_size=cfg.batch_size.interior,
parameterization=Parameterization(time_range),
)
domain.add_constraint(interior, "interior")
# add validation data
deltaT = 0.001
t = np.arange(0, t_max, deltaT)
t = np.expand_dims(t, axis=-1)
invar_numpy = {"t": t}
outvar_numpy = {
"x1": (1 / 6) * np.cos(t)
+ (1 / 2) * np.cos(np.sqrt(3) * t)
+ (1 / 3) * np.cos(2 * t),
"x2": (2 / 6) * np.cos(t)
+ (0 / 2) * np.cos(np.sqrt(3) * t)
- (1 / 3) * np.cos(2 * t),
"x3": (1 / 6) * np.cos(t)
- (1 / 2) * np.cos(np.sqrt(3) * t)
+ (1 / 3) * np.cos(2 * t),
}
validator = PointwiseValidator(
nodes=nodes, invar=invar_numpy, true_outvar=outvar_numpy, batch_size=1024
)
domain.add_validator(validator)
# make solver
slv = Solver(cfg, domain)
# start solver
slv.solve()
if __name__ == "__main__":
run()
| 4,033 | Python | 31.532258 | 81 | 0.631044 |
openhackathons-org/End-to-End-AI-for-Science/workspace/python/source_code/spring_mass/spring_mass_ode.py | # Copyright (c) 2023, NVIDIA CORPORATION. All rights reserved.
#
# Permission is hereby granted, free of charge, to any person obtaining
# a copy of this software and associated documentation files (the
# "Software"), to deal in the Software without restriction, including
# without limitation the rights to use, copy, modify, merge, publish,
# distribute, sublicense, and/or sell copies of the Software, and to
# permit persons to whom the Software is furnished to do so, subject to
# the following conditions:
#
# The above copyright notice and this permission notice shall be
# included in all copies or substantial portions of the Software.
#
# THE SOFTWARE IS PROVIDED "AS IS", WITHOUT WARRANTY OF ANY KIND,
# EXPRESS OR IMPLIED, INCLUDING BUT NOT LIMITED TO THE WARRANTIES OF
# MERCHANTABILITY, FITNESS FOR A PARTICULAR PURPOSE AND
# NONINFRINGEMENT. IN NO EVENT SHALL THE AUTHORS OR COPYRIGHT HOLDERS BE
# LIABLE FOR ANY CLAIM, DAMAGES OR OTHER LIABILITY, WHETHER IN AN ACTION
# OF CONTRACT, TORT OR OTHERWISE, ARISING FROM, OUT OF OR IN CONNECTION
# WITH THE SOFTWARE OR THE USE OR OTHER DEALINGS IN THE SOFTWARE.
from sympy import Symbol, Function, Number
from modulus.sym.eq.pde import PDE
class SpringMass(PDE):
name = "SpringMass"
def __init__(self, k=(2, 1, 1, 2), m=(1, 1, 1)):
self.k = k
self.m = m
k1 = k[0]
k2 = k[1]
k3 = k[2]
k4 = k[3]
m1 = m[0]
m2 = m[1]
m3 = m[2]
t = Symbol("t")
input_variables = {"t": t}
x1 = Function("x1")(*input_variables)
x2 = Function("x2")(*input_variables)
x3 = Function("x3")(*input_variables)
if type(k1) is str:
k1 = Function(k1)(*input_variables)
elif type(k1) in [float, int]:
k1 = Number(k1)
if type(k2) is str:
k2 = Function(k2)(*input_variables)
elif type(k2) in [float, int]:
k2 = Number(k2)
if type(k3) is str:
k3 = Function(k3)(*input_variables)
elif type(k3) in [float, int]:
k3 = Number(k3)
if type(k4) is str:
k4 = Function(k4)(*input_variables)
elif type(k4) in [float, int]:
k4 = Number(k4)
if type(m1) is str:
m1 = Function(m1)(*input_variables)
elif type(m1) in [float, int]:
m1 = Number(m1)
if type(m2) is str:
m2 = Function(m2)(*input_variables)
elif type(m2) in [float, int]:
m2 = Number(m2)
if type(m3) is str:
m3 = Function(m3)(*input_variables)
elif type(m3) in [float, int]:
m3 = Number(m3)
self.equations = {}
self.equations["ode_x1"] = m1 * (x1.diff(t)).diff(t) + k1 * x1 - k2 * (x2 - x1)
self.equations["ode_x2"] = (
m2 * (x2.diff(t)).diff(t) + k2 * (x2 - x1) - k3 * (x3 - x2)
)
self.equations["ode_x3"] = m3 * (x3.diff(t)).diff(t) + k3 * (x3 - x2) + k4 * x3
| 2,999 | Python | 34.294117 | 87 | 0.585195 |
openhackathons-org/End-to-End-AI-for-Science/workspace/python/source_code/spring_mass/spring_mass_inverse.py | # Copyright (c) 2023, NVIDIA CORPORATION. All rights reserved.
#
# Permission is hereby granted, free of charge, to any person obtaining
# a copy of this software and associated documentation files (the
# "Software"), to deal in the Software without restriction, including
# without limitation the rights to use, copy, modify, merge, publish,
# distribute, sublicense, and/or sell copies of the Software, and to
# permit persons to whom the Software is furnished to do so, subject to
# the following conditions:
#
# The above copyright notice and this permission notice shall be
# included in all copies or substantial portions of the Software.
#
# THE SOFTWARE IS PROVIDED "AS IS", WITHOUT WARRANTY OF ANY KIND,
# EXPRESS OR IMPLIED, INCLUDING BUT NOT LIMITED TO THE WARRANTIES OF
# MERCHANTABILITY, FITNESS FOR A PARTICULAR PURPOSE AND
# NONINFRINGEMENT. IN NO EVENT SHALL THE AUTHORS OR COPYRIGHT HOLDERS BE
# LIABLE FOR ANY CLAIM, DAMAGES OR OTHER LIABILITY, WHETHER IN AN ACTION
# OF CONTRACT, TORT OR OTHERWISE, ARISING FROM, OUT OF OR IN CONNECTION
# WITH THE SOFTWARE OR THE USE OR OTHER DEALINGS IN THE SOFTWARE.
import torch
import numpy as np
from sympy import Symbol, Eq
import modulus
from modulus.sym.hydra import ModulusConfig, instantiate_arch
from modulus.sym.solver import Solver
from modulus.sym.domain import Domain
from modulus.sym.geometry.primitives_1d import Point1D
from modulus.sym.geometry import Parameterization
from modulus.sym.domain.constraint import (
PointwiseBoundaryConstraint,
PointwiseConstraint,
)
from modulus.sym.domain.validator import PointwiseValidator
from modulus.sym.domain.monitor import PointwiseMonitor
from modulus.sym.key import Key
from modulus.sym.node import Node
from spring_mass_ode import SpringMass
@modulus.sym.main(config_path="conf", config_name="config_inverse")
def run(cfg: ModulusConfig) -> None:
# prepare data
t_max = 10.0
deltaT = 0.01
t = np.arange(0, t_max, deltaT)
t = np.expand_dims(t, axis=-1)
invar_numpy = {"t": t}
outvar_numpy = {
"x1": (1 / 6) * np.cos(t)
+ (1 / 2) * np.cos(np.sqrt(3) * t)
+ (1 / 3) * np.cos(2 * t),
"x2": (2 / 6) * np.cos(t)
+ (0 / 2) * np.cos(np.sqrt(3) * t)
- (1 / 3) * np.cos(2 * t),
"x3": (1 / 6) * np.cos(t)
- (1 / 2) * np.cos(np.sqrt(3) * t)
+ (1 / 3) * np.cos(2 * t),
}
outvar_numpy.update({"ode_x1": np.full_like(invar_numpy["t"], 0)})
outvar_numpy.update({"ode_x2": np.full_like(invar_numpy["t"], 0)})
outvar_numpy.update({"ode_x3": np.full_like(invar_numpy["t"], 0)})
# make list of nodes to unroll graph on
sm = SpringMass(k=(2, 1, 1, "k4"), m=("m1", 1, 1))
sm_net = instantiate_arch(
input_keys=[Key("t")],
output_keys=[Key("x1"), Key("x2"), Key("x3")],
cfg=cfg.arch.fully_connected,
)
invert_net = instantiate_arch(
input_keys=[Key("t")],
output_keys=[Key("m1"), Key("k4")],
cfg=cfg.arch.fully_connected,
)
nodes = (
sm.make_nodes(
detach_names=[
"x1",
"x1__t",
"x1__t__t",
"x2",
"x2__t",
"x2__t__t",
"x3",
"x3__t",
"x3__t__t",
]
)
+ [sm_net.make_node(name="spring_mass_network", jit=cfg.jit)]
+ [invert_net.make_node(name="invert_network", jit=cfg.jit)]
)
# add constraints to solver
# make geometry
geo = Point1D(0)
t_symbol = Symbol("t")
x = Symbol("x")
time_range = {t_symbol: (0, t_max)}
# make domain
domain = Domain()
# initial conditions
IC = PointwiseBoundaryConstraint(
nodes=nodes,
geometry=geo,
outvar={"x1": 1.0, "x2": 0, "x3": 0, "x1__t": 0, "x2__t": 0, "x3__t": 0},
batch_size=cfg.batch_size.IC,
lambda_weighting={
"x1": 1.0,
"x2": 1.0,
"x3": 1.0,
"x1__t": 1.0,
"x2__t": 1.0,
"x3__t": 1.0,
},
parameterization=Parameterization({t_symbol: 0}),
)
domain.add_constraint(IC, name="IC")
# data and pdes
data = PointwiseConstraint.from_numpy(
nodes=nodes,
invar=invar_numpy,
outvar=outvar_numpy,
batch_size=cfg.batch_size.data,
)
domain.add_constraint(data, name="Data")
# add monitors
monitor = PointwiseMonitor(
invar_numpy,
output_names=["m1"],
metrics={"mean_m1": lambda var: torch.mean(var["m1"])},
nodes=nodes,
)
domain.add_monitor(monitor)
monitor = PointwiseMonitor(
invar_numpy,
output_names=["k4"],
metrics={"mean_k4": lambda var: torch.mean(var["k4"])},
nodes=nodes,
)
domain.add_monitor(monitor)
# make solver
slv = Solver(cfg, domain)
# start solver
slv.solve()
if __name__ == "__main__":
run()
| 4,988 | Python | 29.796296 | 81 | 0.591419 |
openhackathons-org/End-to-End-AI-for-Science/workspace/python/source_code/spring_mass/conf/config_inverse.yaml | defaults :
- modulus_default
- arch:
- fully_connected
- scheduler: tf_exponential_lr
- optimizer: adam
- loss: sum
- _self_
arch:
fully_connected:
layer_size: 256
save_filetypes : "vtk,npz"
scheduler:
decay_rate: 0.95
decay_steps: 100
training:
rec_results_freq: 1000
max_steps : 10000
batch_size:
IC: 10
data: 1000
| 364 | YAML | 12.518518 | 32 | 0.634615 |
openhackathons-org/End-to-End-AI-for-Science/workspace/python/source_code/projectile/projectile.py | # Copyright (c) 2023, NVIDIA CORPORATION. All rights reserved.
#
# Permission is hereby granted, free of charge, to any person obtaining
# a copy of this software and associated documentation files (the
# "Software"), to deal in the Software without restriction, including
# without limitation the rights to use, copy, modify, merge, publish,
# distribute, sublicense, and/or sell copies of the Software, and to
# permit persons to whom the Software is furnished to do so, subject to
# the following conditions:
#
# The above copyright notice and this permission notice shall be
# included in all copies or substantial portions of the Software.
#
# THE SOFTWARE IS PROVIDED "AS IS", WITHOUT WARRANTY OF ANY KIND,
# EXPRESS OR IMPLIED, INCLUDING BUT NOT LIMITED TO THE WARRANTIES OF
# MERCHANTABILITY, FITNESS FOR A PARTICULAR PURPOSE AND
# NONINFRINGEMENT. IN NO EVENT SHALL THE AUTHORS OR COPYRIGHT HOLDERS BE
# LIABLE FOR ANY CLAIM, DAMAGES OR OTHER LIABILITY, WHETHER IN AN ACTION
# OF CONTRACT, TORT OR OTHERWISE, ARISING FROM, OUT OF OR IN CONNECTION
# WITH THE SOFTWARE OR THE USE OR OTHER DEALINGS IN THE SOFTWARE.
import numpy as np
from sympy import Symbol, sin, cos, pi, Eq
import torch
import modulus
from modulus.sym.hydra import instantiate_arch, ModulusConfig
from modulus.sym.solver import Solver
from modulus.sym.domain import Domain
from modulus.sym.geometry.primitives_1d import Line1D,Point1D
from modulus.sym.geometry.primitives_2d import Rectangle
from modulus.sym.domain.constraint import (
PointwiseBoundaryConstraint,
PointwiseInteriorConstraint,
)
from modulus.sym.domain.inferencer import PointwiseInferencer
from modulus.sym.domain.validator import PointwiseValidator
from modulus.sym.key import Key
from modulus.sym.node import Node
from projectile_eqn import ProjectileEquation
from modulus.sym.utils.io import (
csv_to_dict,
ValidatorPlotter,
InferencerPlotter,
)
@modulus.sym.main(config_path="conf", config_name="config")
def run(cfg: ModulusConfig) -> None:
#Creating Nodes and Domain
pe = ProjectileEquation()
projectile_net = instantiate_arch(
input_keys=[Key("t")],
output_keys=[Key("x"),Key("y")],
cfg=cfg.arch.fully_connected,
)
nodes = pe.make_nodes() + [projectile_net.make_node(name="projectile_network")]
x, y, t = Symbol("x"), Symbol("y"), Symbol("t")
#Creating Geometry and adding constraint
geo = Point1D(0)
#make domain
projectile_domain = Domain()
#add constraint to solver
v_o = 40.0
theta = np.pi/3
time_range = {t :(0.0,5.0)}
#initial condition
# Set boundary to be only left boundary
IC = PointwiseBoundaryConstraint(
nodes = nodes,
geometry = geo,
outvar = {"x": 0.0,"y":0.0, "x__t":v_o*cos(theta), "y__t":v_o*sin(theta)},
batch_size = cfg.batch_size.initial_x,
parameterization = {t:0.0}
)
projectile_domain.add_constraint(IC,"IC")
#interior
interior = PointwiseBoundaryConstraint(
nodes = nodes,
geometry = geo,
outvar = {"ode_x":0.0,"ode_y":-9.81},
batch_size = cfg.batch_size.interior,
parameterization = time_range,
)
projectile_domain.add_constraint(interior,"interior")
# Setup validator
delta_T = 0.01
t_val = np.arange(0.,5.,delta_T)
T_val = np.expand_dims(t_val.flatten(), axis = -1)
X_val = v_o*np.cos(theta)*T_val
Y_val = v_o*np.sin(theta)*T_val - 0.5*9.81*(T_val**2)
invar_numpy = {"t": T_val}
outvar_numpy = {"x":X_val, "y": Y_val}
validator = PointwiseValidator(
nodes=nodes,
invar=invar_numpy,
true_outvar=outvar_numpy,
batch_size=128,
plotter = ValidatorPlotter(),
)
projectile_domain.add_validator(validator)
# Setup Inferencer
t_infe = np.arange(0,8,0.001)
T_infe = np.expand_dims(t_infe.flatten(), axis = -1)
invar_infe = {"t":T_infe}
grid_inference = PointwiseInferencer(
nodes=nodes,
invar=invar_infe,
output_names=["x","y"],
batch_size=128,
plotter=InferencerPlotter(),
)
projectile_domain.add_inferencer(grid_inference, "inferencer_data")
#make solver
slv = Solver(cfg, projectile_domain)
#start solve
slv.solve()
if __name__ == "__main__":
run()
| 4,482 | Python | 25.370588 | 86 | 0.657073 |
openhackathons-org/End-to-End-AI-for-Science/workspace/python/source_code/projectile/conf/config.yaml | defaults :
- modulus_default
- arch:
- fully_connected
- scheduler: tf_exponential_lr
- optimizer: adam
- loss: sum
- _self_
save_filetypes : "vtk,npz"
scheduler:
decay_rate: 0.95
decay_steps: 100
training:
rec_validation_freq: 1000
rec_inference_freq: 2000
rec_monitor_freq: 1000
rec_constraint_freq: 2000
max_steps : 5000
batch_size:
initial_x: 100
interior: 1000
graph:
func_arch: true
cuda_graphs: True
cuda_graph_warmup: 20 | 479 | YAML | 14 | 32 | 0.670146 |
openhackathons-org/End-to-End-AI-for-Science/workspace/python/source_code/fourcastnet/fourcastnet.py | # Copyright (c) 2023, NVIDIA CORPORATION. All rights reserved.
#
# Permission is hereby granted, free of charge, to any person obtaining
# a copy of this software and associated documentation files (the
# "Software"), to deal in the Software without restriction, including
# without limitation the rights to use, copy, modify, merge, publish,
# distribute, sublicense, and/or sell copies of the Software, and to
# permit persons to whom the Software is furnished to do so, subject to
# the following conditions:
#
# The above copyright notice and this permission notice shall be
# included in all copies or substantial portions of the Software.
#
# THE SOFTWARE IS PROVIDED "AS IS", WITHOUT WARRANTY OF ANY KIND,
# EXPRESS OR IMPLIED, INCLUDING BUT NOT LIMITED TO THE WARRANTIES OF
# MERCHANTABILITY, FITNESS FOR A PARTICULAR PURPOSE AND
# NONINFRINGEMENT. IN NO EVENT SHALL THE AUTHORS OR COPYRIGHT HOLDERS BE
# LIABLE FOR ANY CLAIM, DAMAGES OR OTHER LIABILITY, WHETHER IN AN ACTION
# OF CONTRACT, TORT OR OTHERWISE, ARISING FROM, OUT OF OR IN CONNECTION
# WITH THE SOFTWARE OR THE USE OR OTHER DEALINGS IN THE SOFTWARE.
# Script to train Fourcastnet on ERA5
# Ref: https://arxiv.org/abs/2202.11214
import modulus
from modulus.sym.hydra.config import ModulusConfig
from modulus.sym.key import Key
from modulus.sym.domain import Domain
from modulus.sym.domain.constraint import SupervisedGridConstraint
from modulus.sym.domain.validator import GridValidator
from modulus.sym.solver import Solver
from modulus.sym.utils.io import GridValidatorPlotter
from src.dataset import ERA5HDF5GridDataset
from src.fourcastnet import FourcastNetArch
from src.loss import LpLoss
@modulus.sym.main(config_path="conf", config_name="config_FCN")
def run(cfg: ModulusConfig) -> None:
# load training/ test data
channels = list(range(cfg.custom.n_channels))
train_dataset = ERA5HDF5GridDataset(
cfg.custom.training_data_path,
chans=channels,
tstep=cfg.custom.tstep,
n_tsteps=cfg.custom.n_tsteps,
patch_size=cfg.arch.afno.patch_size,
)
test_dataset = ERA5HDF5GridDataset(
cfg.custom.test_data_path,
chans=channels,
tstep=cfg.custom.tstep,
n_tsteps=cfg.custom.n_tsteps,
patch_size=cfg.arch.afno.patch_size,
n_samples_per_year=20,
)
# define input/output keys
input_keys = [Key(k, size=train_dataset.nchans) for k in train_dataset.invar_keys]
output_keys = [Key(k, size=train_dataset.nchans) for k in train_dataset.outvar_keys]
# make list of nodes to unroll graph on
model = FourcastNetArch(
input_keys=input_keys,
output_keys=output_keys,
img_shape=test_dataset.img_shape,
patch_size=cfg.arch.afno.patch_size,
embed_dim=cfg.arch.afno.embed_dim,
depth=cfg.arch.afno.depth,
num_blocks=cfg.arch.afno.num_blocks,
)
nodes = [model.make_node(name="FCN")]
# make domain
domain = Domain()
# add constraints to domain
supervised = SupervisedGridConstraint(
nodes=nodes,
dataset=train_dataset,
batch_size=cfg.batch_size.grid,
loss=LpLoss(),
num_workers=cfg.custom.num_workers.grid,
)
domain.add_constraint(supervised, "supervised")
# add validator
val = GridValidator(
nodes,
dataset=test_dataset,
batch_size=cfg.batch_size.validation,
plotter=GridValidatorPlotter(n_examples=5),
num_workers=cfg.custom.num_workers.validation,
)
domain.add_validator(val, "test")
# make solver
slv = Solver(cfg, domain)
# start solver
slv.solve()
if __name__ == "__main__":
run()
| 3,688 | Python | 33.157407 | 88 | 0.706345 |
openhackathons-org/End-to-End-AI-for-Science/workspace/python/source_code/fourcastnet/inferencer.py | # Copyright (c) 2023, NVIDIA CORPORATION. All rights reserved.
#
# Permission is hereby granted, free of charge, to any person obtaining
# a copy of this software and associated documentation files (the
# "Software"), to deal in the Software without restriction, including
# without limitation the rights to use, copy, modify, merge, publish,
# distribute, sublicense, and/or sell copies of the Software, and to
# permit persons to whom the Software is furnished to do so, subject to
# the following conditions:
#
# The above copyright notice and this permission notice shall be
# included in all copies or substantial portions of the Software.
#
# THE SOFTWARE IS PROVIDED "AS IS", WITHOUT WARRANTY OF ANY KIND,
# EXPRESS OR IMPLIED, INCLUDING BUT NOT LIMITED TO THE WARRANTIES OF
# MERCHANTABILITY, FITNESS FOR A PARTICULAR PURPOSE AND
# NONINFRINGEMENT. IN NO EVENT SHALL THE AUTHORS OR COPYRIGHT HOLDERS BE
# LIABLE FOR ANY CLAIM, DAMAGES OR OTHER LIABILITY, WHETHER IN AN ACTION
# OF CONTRACT, TORT OR OTHERWISE, ARISING FROM, OUT OF OR IN CONNECTION
# WITH THE SOFTWARE OR THE USE OR OTHER DEALINGS IN THE SOFTWARE.
#"Script to carry out Fourcastnet inference"
import omegaconf
import torch
import logging
import numpy as np
from torch.utils.data import DataLoader, Sampler
from modulus.sym.hydra import to_absolute_path
from modulus.sym.key import Key
from modulus.sym.distributed.manager import DistributedManager
from src.dataset import ERA5HDF5GridDataset
from src.fourcastnet import FourcastNetArch
from src.metrics import Metrics
logging.basicConfig(format="[%(levelname)s] - %(message)s", level=logging.INFO)
var_key_dict = {
0: "u10",
1: "v10",
2: "t2m",
3: "sp",
4: "msl",
5: "t850",
6: "u1000",
7: "v1000",
8: "z1000",
9: "u850",
10: "v850",
11: "z850",
12: "u500",
13: "v500",
14: "z500",
15: "t500",
16: "z50",
17: "r500",
18: "r850",
19: "tcwv",
}
def to_device(tensor_dict):
return {
key: torch.as_tensor(value, dtype=torch.float32, device=device)
for key, value in tensor_dict.items()
}
class SubsetSequentialBatchSampler(Sampler):
"""Custom subset sequential batch sampler for inferencer"""
def __init__(self, subset):
self.subset = subset
def __iter__(self):
for i in self.subset:
yield [i] # batch size of 1
def __len__(self):
return len(self.subset)
# load configuration
cfg = omegaconf.OmegaConf.load("conf/config_FCN.yaml")
model_path = to_absolute_path("fcn_era5.pth")
# get device
device = DistributedManager().device
# load test data
test_dataset = ERA5HDF5GridDataset(
cfg.custom.test_data_path, # Test data location e.g. /era5/20var/test
chans=list(range(cfg.custom.n_channels)),
tstep=cfg.custom.tstep,
n_tsteps=1, # set to one for inference
patch_size=cfg.arch.afno.patch_size,
)
m = Metrics(
test_dataset.img_shape,
clim_mean_path="/data/stats/time_means.npy", # Path to climate mean
device=device
)
# define input/output keys
input_keys = [Key(k, size=test_dataset.nchans) for k in test_dataset.invar_keys]
output_keys = [Key(k, size=test_dataset.nchans) for k in test_dataset.outvar_keys]
# create model
model = FourcastNetArch(
input_keys=input_keys,
output_keys=output_keys,
img_shape=test_dataset.img_shape,
patch_size=cfg.arch.afno.patch_size,
embed_dim=cfg.arch.afno.embed_dim,
depth=cfg.arch.afno.depth,
num_blocks=cfg.arch.afno.num_blocks,
)
# load parameters
model.load_state_dict(torch.load(model_path))
model.to(device)
logging.info(f"Loaded model {model_path}")
# define subsets of dataset to run inference
nics = 180 # Number of 2 day correl time samples
nsteps = 25
last = len(test_dataset) - 1 - nsteps * cfg.custom.tstep
# Variable dictionary
acc_recursive = {key: [] for key in var_key_dict.values()}
rmse_recursive = {key: [] for key in var_key_dict.values()}
# Normalization stats
mu = torch.tensor(test_dataset.mu[0]).to(device) # shape [C, 1, 1]
sd = torch.tensor(test_dataset.sd[0]).to(device) # shape [C, 1, 1]
# run inference
with torch.no_grad():
for ic in range(0, min([8 * nics + 1, last])):
subset = cfg.custom.tstep * np.arange(nsteps) + ic
if (ic + 1) % 8 == 0 or (ic + 1) % 36 == 0 or ic == 0:
logging.info(f"Running IC at step {ic}")
# get dataloader
dataloader = DataLoader(
dataset=test_dataset,
batch_sampler=SubsetSequentialBatchSampler(subset),
pin_memory=True,
num_workers=1,
worker_init_fn=test_dataset.worker_init_fn,
)
acc_error = torch.zeros(nsteps, test_dataset.nchans)
rmse_error = torch.zeros(nsteps, test_dataset.nchans)
for tstep, (invar, true_outvar, _) in enumerate(dataloader):
if tstep % 10 == 0:
logging.info(f"ic: {ic} tstep: {tstep}/{nsteps}")
# place tensors on device
invar = to_device(invar)
true_outvar = to_device(true_outvar)
# 1. single step inference
pred_outvar_single = model(invar)
pred_single = sd * pred_outvar_single["x_t1"][0]
# 2. recursive inference
if tstep == 0:
pred_outvar_recursive = model(invar)
else:
pred_outvar_recursive = model(
{"x_t0": pred_outvar_recursive["x_t1"]}
)
# get unormalised target / prediction
true = sd * true_outvar["x_t1"][0]
pred_recursive = sd * pred_outvar_recursive["x_t1"][0]
# Calc metrics
rmse_error[tstep] = m.weighted_rmse(pred_recursive, true).detach().cpu()
acc_error[tstep] = m.weighted_acc(pred_recursive, true).detach().cpu()
# Save fields into dictionary
if (ic + 1) % 8 == 0 or (ic + 1) % 36 == 0 or ic == 0:
for i, fld in var_key_dict.items():
# Fields with 9 day (36) dc time
if fld == "z500" or fld == "t2m" or fld == "t850":
if (ic + 1) % 36 == 0 or ic == 0:
acc_recursive[fld].append(acc_error[:, i].numpy())
rmse_recursive[fld].append(rmse_error[:, i].numpy())
# Rest have regular 2 day (8) dc time
else:
if (ic + 1) % 8 == 0 or ic == 0:
acc_recursive[fld].append(acc_error[:, i].numpy())
rmse_recursive[fld].append(rmse_error[:, i].numpy())
# Field stacking
for var_dict in [acc_recursive, rmse_recursive]:
for key, value in var_dict.items():
print(f"{len(value)} samples for field {key}")
var_dict[key] = np.stack(value, axis=0)
np.save("rmse_recursive", rmse_recursive)
np.save("acc_recursive", acc_recursive)
| 7,069 | Python | 33.827586 | 88 | 0.610553 |
openhackathons-org/End-to-End-AI-for-Science/workspace/python/source_code/fourcastnet/src/fourcastnet.py | # Copyright (c) 2023, NVIDIA CORPORATION. All rights reserved.
#
# Permission is hereby granted, free of charge, to any person obtaining
# a copy of this software and associated documentation files (the
# "Software"), to deal in the Software without restriction, including
# without limitation the rights to use, copy, modify, merge, publish,
# distribute, sublicense, and/or sell copies of the Software, and to
# permit persons to whom the Software is furnished to do so, subject to
# the following conditions:
#
# The above copyright notice and this permission notice shall be
# included in all copies or substantial portions of the Software.
#
# THE SOFTWARE IS PROVIDED "AS IS", WITHOUT WARRANTY OF ANY KIND,
# EXPRESS OR IMPLIED, INCLUDING BUT NOT LIMITED TO THE WARRANTIES OF
# MERCHANTABILITY, FITNESS FOR A PARTICULAR PURPOSE AND
# NONINFRINGEMENT. IN NO EVENT SHALL THE AUTHORS OR COPYRIGHT HOLDERS BE
# LIABLE FOR ANY CLAIM, DAMAGES OR OTHER LIABILITY, WHETHER IN AN ACTION
# OF CONTRACT, TORT OR OTHERWISE, ARISING FROM, OUT OF OR IN CONNECTION
# WITH THE SOFTWARE OR THE USE OR OTHER DEALINGS IN THE SOFTWARE.
"""Defines the FCN architecture"""
import logging
import torch
from torch import Tensor
from typing import List, Tuple, Dict
from modulus.sym.models.afno.afno import AFNONet
from modulus.sym.models.arch import Arch
from modulus.sym.key import Key
class FourcastNetArch(Arch):
"Defines the FourcastNet architecture"
def __init__(
self,
input_keys: List[Key],
output_keys: List[Key],
img_shape: Tuple[int, int],
detach_keys: List[Key] = [],
patch_size: int = 16,
embed_dim: int = 256,
depth: int = 4,
num_blocks: int = 4,
) -> None:
"""Fourcastnet model. This is a simple wrapper for Modulus' AFNO model.
The only difference is that FourcastNet needs multi-step training. This class
allows the model to auto-regressively predict multiple timesteps
Parameters (Same as AFNO)
----------
input_keys : List[Key]
Input key list. The key dimension size should equal the variables channel dim.
output_keys : List[Key]
Output key list. The key dimension size should equal the variables channel dim.
img_shape : Tuple[int, int]
Input image dimensions (height, width)
detach_keys : List[Key], optional
List of keys to detach gradients, by default []
patch_size : int, optional
Size of image patchs, by default 16
embed_dim : int, optional
Embedded channel size, by default 256
depth : int, optional
Number of AFNO layers, by default 4
num_blocks : int, optional
Number of blocks in the frequency weight matrices, by default 4
"""
super().__init__(
input_keys=input_keys,
output_keys=output_keys,
detach_keys=detach_keys,
)
# get number of timesteps steps to unroll
assert (
len(self.input_keys) == 1
), "Error, FourcastNet only accepts one input variable (x_t0)"
self.n_tsteps = len(self.output_keys)
logging.info(f"Unrolling FourcastNet over {self.n_tsteps} timesteps")
# get number of input/output channels
in_channels = self.input_keys[0].size
out_channels = self.output_keys[0].size
# intialise AFNO kernel
self._impl = AFNONet(
in_channels=in_channels,
out_channels=out_channels,
patch_size=(patch_size, patch_size),
img_size=img_shape,
embed_dim=embed_dim,
depth=depth,
num_blocks=num_blocks,
)
def forward(self, in_vars: Dict[str, Tensor]) -> Dict[str, Tensor]:
# prepare input tensor
x = self.prepare_input(
input_variables=in_vars,
mask=self.input_key_dict.keys(),
detach_dict=self.detach_key_dict,
dim=1,
input_scales=self.input_scales,
)
# unroll model over multiple timesteps
ys = []
for t in range(self.n_tsteps):
x = self._impl(x)
ys.append(x)
y = torch.cat(ys, dim=1)
# prepare output dict
return self.prepare_output(
output_tensor=y,
output_var=self.output_key_dict,
dim=1,
output_scales=self.output_scales,
)
| 4,496 | Python | 35.560975 | 91 | 0.630338 |
openhackathons-org/End-to-End-AI-for-Science/workspace/python/source_code/fourcastnet/src/metrics.py | # Copyright (c) 2023, NVIDIA CORPORATION. All rights reserved.
#
# Permission is hereby granted, free of charge, to any person obtaining
# a copy of this software and associated documentation files (the
# "Software"), to deal in the Software without restriction, including
# without limitation the rights to use, copy, modify, merge, publish,
# distribute, sublicense, and/or sell copies of the Software, and to
# permit persons to whom the Software is furnished to do so, subject to
# the following conditions:
#
# The above copyright notice and this permission notice shall be
# included in all copies or substantial portions of the Software.
#
# THE SOFTWARE IS PROVIDED "AS IS", WITHOUT WARRANTY OF ANY KIND,
# EXPRESS OR IMPLIED, INCLUDING BUT NOT LIMITED TO THE WARRANTIES OF
# MERCHANTABILITY, FITNESS FOR A PARTICULAR PURPOSE AND
# NONINFRINGEMENT. IN NO EVENT SHALL THE AUTHORS OR COPYRIGHT HOLDERS BE
# LIABLE FOR ANY CLAIM, DAMAGES OR OTHER LIABILITY, WHETHER IN AN ACTION
# OF CONTRACT, TORT OR OTHERWISE, ARISING FROM, OUT OF OR IN CONNECTION
# WITH THE SOFTWARE OR THE USE OR OTHER DEALINGS IN THE SOFTWARE.
import torch
import numpy as np
from typing import Tuple
class Metrics:
"""Class used for computing performance related metrics. Expects predictions /
targets to be of shape [C, H, W] where H is latitude dimension and W is longitude
dimension. Metrics are computed for each channel separately.
Parameters
----------
img_shape : Tuple[int]
Shape of input image (resolution for fourcastnet)
clim_mean_path : str, optional
Path to total climate mean data, needed for ACC. By default "/era5/stats/time_means.npy"
device : torch.device, optional
Pytorch device model is on, by default 'cpu'
"""
def __init__(
self,
img_shape: Tuple[int],
clim_mean_path: str = "/era5/stats/time_means.npy",
device: torch.device = "cpu",
):
self.img_shape = tuple(img_shape)
self.device = device
# Load climate mean value
self.clim_mean = torch.as_tensor(np.load(clim_mean_path))
# compute latitude weighting
nlat = img_shape[0]
lat = torch.linspace(90, -90, nlat)
lat_weight = torch.cos(torch.pi * (lat / 180))
lat_weight = nlat * lat_weight / lat_weight.sum()
self.lat_weight = lat_weight.view(1, nlat, 1)
# place on device
if self.device is not None:
self.lat_weight = self.lat_weight.to(self.device)
self.clim_mean = self.clim_mean.to(self.device)
def _check_shape(self, *args):
# checks for shape [C, H, W]
for x in args:
assert x.ndim == 3
assert tuple(x.shape[1:]) == self.img_shape
def weighted_acc(self, pred: torch.Tensor, target: torch.Tensor) -> torch.Tensor:
"""Computes the anomaly correlation coefficient (ACC). The ACC calculation is
weighted based on the latitude.
Parameters
----------
pred : torch.Tensor
[C, H, W] Predicted tensor
target : torch.Tensor
[C, H, W] Target tensor
Returns
-------
torch.Tensor
[C] ACC values for each channel
"""
self._check_shape(pred, target)
# subtract climate means
(n_chans, img_x, img_y) = pred.shape
clim_mean = self.clim_mean[0, 0:n_chans, 0:img_x]
pred_hat = pred - clim_mean
target_hat = target - clim_mean
# Weighted mean
pred_bar = torch.sum(
self.lat_weight * pred_hat, dim=(1, 2), keepdim=True
) / torch.sum(
self.lat_weight * torch.ones_like(pred_hat), dim=(1, 2), keepdim=True
)
target_bar = torch.sum(
self.lat_weight * target_hat, dim=(1, 2), keepdim=True
) / torch.sum(
self.lat_weight * torch.ones_like(target_hat), dim=(1, 2), keepdim=True
)
pred_diff = pred_hat - pred_bar
target_diff = target_hat - target_bar
# compute weighted acc
# Ref: https://www.atmos.albany.edu/daes/atmclasses/atm401/spring_2016/ppts_pdfs/ECMWF_ACC_definition.pdf
p1 = torch.sum(self.lat_weight * pred_diff * target_diff, dim=(1, 2))
p2 = torch.sum(self.lat_weight * pred_diff * pred_diff, dim=(1, 2))
p3 = torch.sum(self.lat_weight * target_diff * target_diff, dim=(1, 2))
m = p1 / torch.sqrt(p2 * p3)
return m
def weighted_rmse(self, pred: torch.Tensor, target: torch.Tensor) -> torch.Tensor:
"""Computes RMSE weighted based on latitude
Parameters
----------
pred : torch.Tensor
[C, H, W] Predicted tensor
target : torch.Tensor
[C, H, W] Target tensor
Returns
-------
torch.Tensor
[C] Weighted RSME values for each channel
"""
self._check_shape(pred, target)
# compute weighted rmse
m = torch.sqrt(torch.mean(self.lat_weight * (pred - target) ** 2, dim=(1, 2)))
return m
| 5,098 | Python | 34.657342 | 113 | 0.616712 |
openhackathons-org/End-to-End-AI-for-Science/workspace/python/source_code/fourcastnet/src/dataset.py | # Copyright (c) 2023, NVIDIA CORPORATION. All rights reserved.
#
# Permission is hereby granted, free of charge, to any person obtaining
# a copy of this software and associated documentation files (the
# "Software"), to deal in the Software without restriction, including
# without limitation the rights to use, copy, modify, merge, publish,
# distribute, sublicense, and/or sell copies of the Software, and to
# permit persons to whom the Software is furnished to do so, subject to
# the following conditions:
#
# The above copyright notice and this permission notice shall be
# included in all copies or substantial portions of the Software.
#
# THE SOFTWARE IS PROVIDED "AS IS", WITHOUT WARRANTY OF ANY KIND,
# EXPRESS OR IMPLIED, INCLUDING BUT NOT LIMITED TO THE WARRANTIES OF
# MERCHANTABILITY, FITNESS FOR A PARTICULAR PURPOSE AND
# NONINFRINGEMENT. IN NO EVENT SHALL THE AUTHORS OR COPYRIGHT HOLDERS BE
# LIABLE FOR ANY CLAIM, DAMAGES OR OTHER LIABILITY, WHETHER IN AN ACTION
# OF CONTRACT, TORT OR OTHERWISE, ARISING FROM, OUT OF OR IN CONNECTION
# WITH THE SOFTWARE OR THE USE OR OTHER DEALINGS IN THE SOFTWARE.
import h5py
import logging
import numpy as np
from typing import List
from pathlib import Path
from modulus.sym.hydra import to_absolute_path
from modulus.sym.dataset import Dataset
class ERA5HDF5GridDataset(Dataset):
"""Lazy-loading ERA5 dataset.
Parameters
----------
data_dir : str
Directory where ERA5 data is stored
chans : List[int]
Defines which ERA5 variables to load
tstep : int
Defines the size of the timestep between the input and output variables
n_tsteps : int, optional
Defines how many timesteps are included in the output variables
Default is 1
patch_size : int, optional
If specified, crops input and output variables so image dimensions are
divisible by patch_size
Default is None
n_samples_per_year : int, optional
If specified, randomly selects n_samples_per_year samples from each year
rather than all of the samples per year
Default is None
stats_dir : str, optional
Directory to test data statistic numpy files that have the global mean and variance
"""
def __init__(
self,
data_dir: str,
chans: List[int],
tstep: int = 1,
n_tsteps: int = 1,
patch_size: int = None,
n_samples_per_year: int = None,
stats_dir: str = None,
):
self.data_dir = Path(to_absolute_path(data_dir))
print(self.data_dir)
self.chans = chans
self.nchans = len(self.chans)
self.tstep = tstep
self.n_tsteps = n_tsteps
self.patch_size = patch_size
self.n_samples_per_year = n_samples_per_year
if stats_dir is None:
self.stats_dir = self.data_dir.parent / "stats"
# check root directory exists
assert (
self.data_dir.is_dir()
), f"Error, data directory {self.data_dir} does not exist"
assert (
self.stats_dir.is_dir()
), f"Error, stats directory {self.stats_dir} does not exist"
# get all input data files
self.data_paths = sorted(self.data_dir.glob("??????.h5"))
for data_path in self.data_paths:
logging.info(f"ERA5 file found: {data_path}")
self.n_years = len(self.data_paths)
logging.info(f"Number of months: {self.n_years}")
# get total number of examples and image shape from the first file,
# assuming other files have exactly the same format.
logging.info(f"Getting file stats from {self.data_paths[0]}")
with h5py.File(self.data_paths[0], "r") as f:
self.n_samples_per_year_all = f["fields"].shape[0]
self.img_shape = f["fields"].shape[2:]
logging.info(f"Number of channels available: {f['fields'].shape[1]}")
# get example indices to use
if self.n_samples_per_year is None:
self.n_samples_per_year = self.n_samples_per_year_all
self.samples = [
np.arange(self.n_samples_per_year) for _ in range(self.n_years)
]
else:
if self.n_samples_per_year > self.n_samples_per_year_all:
raise ValueError(
f"n_samples_per_year ({self.n_samples_per_year}) > number of samples available ({self.n_samples_per_year_all})!"
)
self.samples = [
np.random.choice(
np.arange(self.n_samples_per_year_all),
self.n_samples_per_year,
replace=False,
)
for _ in range(self.n_years)
]
logging.info(f"Number of samples/month: {self.n_samples_per_year}")
# get total length
self.length = self.n_years * self.n_samples_per_year
# adjust image shape if patch_size defined
if self.patch_size is not None:
self.img_shape = [s - s % self.patch_size for s in self.img_shape]
logging.info(f"Input image shape: {self.img_shape}")
# load normalisation values
# has shape [1, C, 1, 1]
self.mu = np.load(self.stats_dir / "global_means.npy")[:, self.chans]
# has shape [1, C, 1, 1]
self.sd = np.load(self.stats_dir / "global_stds.npy")[:, self.chans]
assert (
self.mu.shape == self.sd.shape == (1, self.nchans, 1, 1)
), "Error, normalisation arrays have wrong shape"
def worker_init_fn(self, iworker):
super().worker_init_fn(iworker)
# open all year files at once on worker thread
self.data_files = [h5py.File(path, "r") for path in self.data_paths]
@property
def invar_keys(self):
return ["x_t0"]
@property
def outvar_keys(self):
return [f"x_t{(i+1)*self.tstep}" for i in range(self.n_tsteps)]
def __getitem__(self, idx):
# get local indices from global index
year_idx = int(idx / self.n_samples_per_year)
local_idx = int(idx % self.n_samples_per_year)
in_idx = self.samples[year_idx][local_idx]
# get output indices
out_idxs = []
for i in range(self.n_tsteps):
out_idx = in_idx + (i + 1) * self.tstep
# if at end of dataset, just learn identity instead
if out_idx > (self.n_samples_per_year_all - 1):
out_idx = in_idx
out_idxs.append(out_idx)
# get data
xs = []
for idx in [in_idx] + out_idxs:
# get array
# has shape [C, H, W]
x = self.data_files[year_idx]["fields"][idx, self.chans]
assert x.ndim == 3, f"Expected 3 dimensions, but got {x.shape}"
# apply input / output normalisation (broadcasted operation)
x = (x - self.mu[0]) / self.sd[0]
# crop data if needed
if self.patch_size is not None:
x = x[..., : self.img_shape[0], : self.img_shape[1]]
xs.append(x)
# convert to tensor dicts
invar = {"x_t0": xs[0]}
outvar = {f"x_t{(i+1)*self.tstep}": x for i, x in enumerate(xs[1:])}
invar = Dataset._to_tensor_dict(invar)
outvar = Dataset._to_tensor_dict(outvar)
# TODO: get rid to lambda weighting
lambda_weighting = Dataset._to_tensor_dict(
{k: np.ones_like(v) for k, v in outvar.items()}
)
# lambda_weighting = Dataset._to_tensor_dict(
# {k: np.array([1]) for k, v in outvar.items()}
# )
return invar, outvar, lambda_weighting
def __len__(self):
return self.length
| 7,719 | Python | 36.294686 | 132 | 0.598523 |
openhackathons-org/End-to-End-AI-for-Science/workspace/python/source_code/fourcastnet/src/loss.py | # Copyright (c) 2023, NVIDIA CORPORATION. All rights reserved.
#
# Permission is hereby granted, free of charge, to any person obtaining
# a copy of this software and associated documentation files (the
# "Software"), to deal in the Software without restriction, including
# without limitation the rights to use, copy, modify, merge, publish,
# distribute, sublicense, and/or sell copies of the Software, and to
# permit persons to whom the Software is furnished to do so, subject to
# the following conditions:
#
# The above copyright notice and this permission notice shall be
# included in all copies or substantial portions of the Software.
#
# THE SOFTWARE IS PROVIDED "AS IS", WITHOUT WARRANTY OF ANY KIND,
# EXPRESS OR IMPLIED, INCLUDING BUT NOT LIMITED TO THE WARRANTIES OF
# MERCHANTABILITY, FITNESS FOR A PARTICULAR PURPOSE AND
# NONINFRINGEMENT. IN NO EVENT SHALL THE AUTHORS OR COPYRIGHT HOLDERS BE
# LIABLE FOR ANY CLAIM, DAMAGES OR OTHER LIABILITY, WHETHER IN AN ACTION
# OF CONTRACT, TORT OR OTHERWISE, ARISING FROM, OUT OF OR IN CONNECTION
# WITH THE SOFTWARE OR THE USE OR OTHER DEALINGS IN THE SOFTWARE.
import torch
from typing import Dict
Tensor = torch.Tensor
class LpLoss(torch.nn.Module):
def __init__(
self,
d: float = 2.0,
p: float = 2.0,
):
"""Relative Lp loss normalized seperately in the batch dimension.
Expects inputs of the shape [B, C, ...]
Parameters
----------
p : float, optional
Norm power, by default 2.0
"""
super(LpLoss, self).__init__()
# Dimension and Lp-norm type are postive
assert p > 0.0
self.p = p
def _rel(self, x: torch.Tensor, y: torch.Tensor) -> float:
num_examples = x.size()[0]
xv = x.reshape(num_examples, -1)
yv = y.reshape(num_examples, -1)
diff_norms = torch.linalg.norm(xv - yv, ord=self.p, dim=1)
y_norms = torch.linalg.norm(yv, ord=self.p, dim=1)
return torch.mean(diff_norms / y_norms)
def forward(
self,
invar: Dict[str, Tensor],
pred_outvar: Dict[str, Tensor],
true_outvar: Dict[str, Tensor],
lambda_weighting: Dict[str, Tensor],
step: int,
) -> Dict[str, float]:
losses = {}
for key, value in pred_outvar.items():
losses[key] = self._rel(pred_outvar[key], true_outvar[key])
return losses
| 2,433 | Python | 33.28169 | 73 | 0.648993 |
openhackathons-org/End-to-End-AI-for-Science/workspace/python/source_code/fourcastnet/conf/config_FCN.yaml | defaults :
- modulus_default
- arch:
- afno
- scheduler: cosine_annealing
- optimizer: adam
- loss: sum
- _self_
arch:
afno:
patch_size: 8
embed_dim: 512
depth: 10
num_blocks: 8
optimizer:
lr: 0.0005
scheduler:
T_max: 80000
custom:
n_channels: 20
tstep: 1
n_tsteps: 1
training_data_path: "/workspace/python/source_code/fourcastnet/data/train" # Training dataset path here
test_data_path: "/workspace/python/source_code/fourcastnet/data/test" # Test dataset path here
num_workers:
grid: 4
validation: 4
tag:
batch_size:
grid: 1
validation: 1
training:
amp: true
rec_constraint_freq: 10000
rec_results_freq : 1000
save_network_freq: 1000
print_stats_freq: 100
summary_freq: 1000
max_steps : 71000
| 787 | YAML | 15.765957 | 105 | 0.662008 |
openhackathons-org/End-to-End-AI-for-Science/workspace/python/source_code/diffusion_1d/plot_results.py | # Copyright (c) 2023, NVIDIA CORPORATION. All rights reserved.
#
# Permission is hereby granted, free of charge, to any person obtaining
# a copy of this software and associated documentation files (the
# "Software"), to deal in the Software without restriction, including
# without limitation the rights to use, copy, modify, merge, publish,
# distribute, sublicense, and/or sell copies of the Software, and to
# permit persons to whom the Software is furnished to do so, subject to
# the following conditions:
#
# The above copyright notice and this permission notice shall be
# included in all copies or substantial portions of the Software.
#
# THE SOFTWARE IS PROVIDED "AS IS", WITHOUT WARRANTY OF ANY KIND,
# EXPRESS OR IMPLIED, INCLUDING BUT NOT LIMITED TO THE WARRANTIES OF
# MERCHANTABILITY, FITNESS FOR A PARTICULAR PURPOSE AND
# NONINFRINGEMENT. IN NO EVENT SHALL THE AUTHORS OR COPYRIGHT HOLDERS BE
# LIABLE FOR ANY CLAIM, DAMAGES OR OTHER LIABILITY, WHETHER IN AN ACTION
# OF CONTRACT, TORT OR OTHERWISE, ARISING FROM, OUT OF OR IN CONNECTION
# WITH THE SOFTWARE OR THE USE OR OTHER DEALINGS IN THE SOFTWARE.
import numpy as np
import matplotlib.pyplot as plt
network_dir = "./outputs/diffusion_bar/validators/"
data_1 = np.load(network_dir + "Val1.npz", allow_pickle=True)
data_2 = np.load(network_dir + "Val2.npz", allow_pickle=True)
data_1 = np.atleast_1d(data_1.f.arr_0)[0]
data_2 = np.atleast_1d(data_2.f.arr_0)[0]
plt.plot(data_1["x"][:, 0], data_1["pred_u_1"][:, 0], "--", label="u_1_pred")
plt.plot(data_2["x"][:, 0], data_2["pred_u_2"][:, 0], "--", label="u_2_pred")
plt.plot(data_1["x"][:, 0], data_1["true_u_1"][:, 0], label="u_1_true")
plt.plot(data_2["x"][:, 0], data_2["true_u_2"][:, 0], label="u_2_true")
plt.legend()
plt.savefig("image_diffusion_problem_bootcamp")
| 1,801 | Python | 46.421051 | 77 | 0.716824 |
openhackathons-org/End-to-End-AI-for-Science/workspace/python/source_code/diffusion_1d/diffusion_bar.py | # Copyright (c) 2023, NVIDIA CORPORATION. All rights reserved.
#
# Permission is hereby granted, free of charge, to any person obtaining
# a copy of this software and associated documentation files (the
# "Software"), to deal in the Software without restriction, including
# without limitation the rights to use, copy, modify, merge, publish,
# distribute, sublicense, and/or sell copies of the Software, and to
# permit persons to whom the Software is furnished to do so, subject to
# the following conditions:
#
# The above copyright notice and this permission notice shall be
# included in all copies or substantial portions of the Software.
#
# THE SOFTWARE IS PROVIDED "AS IS", WITHOUT WARRANTY OF ANY KIND,
# EXPRESS OR IMPLIED, INCLUDING BUT NOT LIMITED TO THE WARRANTIES OF
# MERCHANTABILITY, FITNESS FOR A PARTICULAR PURPOSE AND
# NONINFRINGEMENT. IN NO EVENT SHALL THE AUTHORS OR COPYRIGHT HOLDERS BE
# LIABLE FOR ANY CLAIM, DAMAGES OR OTHER LIABILITY, WHETHER IN AN ACTION
# OF CONTRACT, TORT OR OTHERWISE, ARISING FROM, OUT OF OR IN CONNECTION
# WITH THE SOFTWARE OR THE USE OR OTHER DEALINGS IN THE SOFTWARE.
import torch
import numpy as np
from sympy import Symbol, Eq, Function, Number
import modulus
from modulus.sym.hydra import instantiate_arch , ModulusConfig
from modulus.sym.solver import Solver
from modulus.sym.domain import Domain
from modulus.sym.geometry.primitives_1d import Line1D
from modulus.sym.domain.constraint import (
PointwiseBoundaryConstraint,
PointwiseInteriorConstraint,
)
from modulus.sym.domain.validator import PointwiseValidator
from modulus.sym.domain.monitor import PointwiseMonitor
from modulus.sym.key import Key
from modulus.sym.node import Node
from modulus.sym.eq.pde import PDE
# params for domain
L1 = Line1D(0, 1)
L2 = Line1D(1, 2)
D1 = 1e1
D2 = 1e-1
Tc = 100
Ta = 0
Tb = (Tc + (D1 / D2) * Ta) / (1 + (D1 / D2))
print(Ta)
print(Tb)
print(Tc)
class Diffusion(PDE):
name = "Diffusion"
def __init__(self, T="T", D="D", Q=0, dim=3, time=True):
# set params
self.T = T
self.dim = dim
self.time = time
# coordinates
x, y, z = Symbol("x"), Symbol("y"), Symbol("z")
# time
t = Symbol("t")
# make input variables
input_variables = {"x": x, "y": y, "z": z, "t": t}
if self.dim == 1:
input_variables.pop("y")
input_variables.pop("z")
elif self.dim == 2:
input_variables.pop("z")
if not self.time:
input_variables.pop("t")
# Temperature
assert type(T) == str, "T needs to be string"
T = Function(T)(*input_variables)
# Diffusivity
if type(D) is str:
D = Function(D)(*input_variables)
elif type(D) in [float, int]:
D = Number(D)
# Source
if type(Q) is str:
Q = Function(Q)(*input_variables)
elif type(Q) in [float, int]:
Q = Number(Q)
# set equations
self.equations = {}
self.equations["diffusion_" + self.T] = (
T.diff(t)
- (D * T.diff(x)).diff(x)
- (D * T.diff(y)).diff(y)
- (D * T.diff(z)).diff(z)
- Q
)
class DiffusionInterface(PDE):
name = "DiffusionInterface"
def __init__(self, T_1, T_2, D_1, D_2, dim=3, time=True):
# set params
self.T_1 = T_1
self.T_2 = T_2
self.dim = dim
self.time = time
# coordinates
x, y, z = Symbol("x"), Symbol("y"), Symbol("z")
normal_x, normal_y, normal_z = (
Symbol("normal_x"),
Symbol("normal_y"),
Symbol("normal_z"),
)
# time
t = Symbol("t")
# make input variables
input_variables = {"x": x, "y": y, "z": z, "t": t}
if self.dim == 1:
input_variables.pop("y")
input_variables.pop("z")
elif self.dim == 2:
input_variables.pop("z")
if not self.time:
input_variables.pop("t")
# Diffusivity
if type(D_1) is str:
D_1 = Function(D_1)(*input_variables)
elif type(D_1) in [float, int]:
D_1 = Number(D_1)
if type(D_2) is str:
D_2 = Function(D_2)(*input_variables)
elif type(D_2) in [float, int]:
D_2 = Number(D_2)
# variables to match the boundary conditions (example Temperature)
T_1 = Function(T_1)(*input_variables)
T_2 = Function(T_2)(*input_variables)
# set equations
self.equations = {}
self.equations["diffusion_interface_dirichlet_" + self.T_1 + "_" + self.T_2] = (
T_1 - T_2
)
flux_1 = D_1 * (
normal_x * T_1.diff(x) + normal_y * T_1.diff(y) + normal_z * T_1.diff(z)
)
flux_2 = D_2 * (
normal_x * T_2.diff(x) + normal_y * T_2.diff(y) + normal_z * T_2.diff(z)
)
self.equations["diffusion_interface_neumann_" + self.T_1 + "_" + self.T_2] = (
flux_1 - flux_2
)
@modulus.sym.main(config_path="conf", config_name="config")
def run(cfg: ModulusConfig) -> None:
# make list of nodes to unroll graph on
diff_u1 = Diffusion(T="u_1", D=D1, dim=1, time=False)
diff_u2 = Diffusion(T="u_2", D=D2, dim=1, time=False)
diff_in = DiffusionInterface("u_1", "u_2", D1, D2, dim=1, time=False)
diff_net_u_1 = instantiate_arch(
input_keys=[Key("x")],
output_keys=[Key("u_1")],
cfg=cfg.arch.fully_connected,
)
diff_net_u_2 = instantiate_arch(
input_keys=[Key("x")],
output_keys=[Key("u_2")],
cfg=cfg.arch.fully_connected,
)
nodes = (
diff_u1.make_nodes()
+ diff_u2.make_nodes()
+ diff_in.make_nodes()
+ [diff_net_u_1.make_node(name="u1_network", jit=cfg.jit)]
+ [diff_net_u_2.make_node(name="u2_network", jit=cfg.jit)]
)
# make domain add constraints to the solver
domain = Domain()
# sympy variables
x = Symbol("x")
# right hand side (x = 2) Pt c
rhs = PointwiseBoundaryConstraint(
nodes=nodes,
geometry=L2,
outvar={"u_2": Tc},
batch_size=cfg.batch_size.rhs,
criteria=Eq(x, 2),
)
domain.add_constraint(rhs, "right_hand_side")
# left hand side (x = 0) Pt a
lhs = PointwiseBoundaryConstraint(
nodes=nodes,
geometry=L1,
outvar={"u_1": Ta},
batch_size=cfg.batch_size.lhs,
criteria=Eq(x, 0),
)
domain.add_constraint(lhs, "left_hand_side")
# interface 1-2
interface = PointwiseBoundaryConstraint(
nodes=nodes,
geometry=L1,
outvar={
"diffusion_interface_dirichlet_u_1_u_2": 0,
"diffusion_interface_neumann_u_1_u_2": 0,
},
batch_size=cfg.batch_size.interface,
criteria=Eq(x, 1),
)
domain.add_constraint(interface, "interface")
# interior 1
interior_u1 = PointwiseInteriorConstraint(
nodes=nodes,
geometry=L1,
outvar={"diffusion_u_1": 0},
bounds={x: (0, 1)},
batch_size=cfg.batch_size.interior_u1,
)
domain.add_constraint(interior_u1, "interior_u1")
# interior 2
interior_u2 = PointwiseInteriorConstraint(
nodes=nodes,
geometry=L2,
outvar={"diffusion_u_2": 0},
bounds={x: (1, 2)},
batch_size=cfg.batch_size.interior_u2,
)
domain.add_constraint(interior_u2, "interior_u2")
# validation data
x = np.expand_dims(np.linspace(0, 1, 100), axis=-1)
u_1 = x * Tb + (1 - x) * Ta
invar_numpy = {"x": x}
outvar_numpy = {"u_1": u_1}
val = PointwiseValidator(nodes=nodes,invar=invar_numpy, true_outvar=outvar_numpy)
domain.add_validator(val, name="Val1")
# make validation data line 2
x = np.expand_dims(np.linspace(1, 2, 100), axis=-1)
u_2 = (x - 1) * Tc + (2 - x) * Tb
invar_numpy = {"x": x}
outvar_numpy = {"u_2": u_2}
val = PointwiseValidator(nodes=nodes, invar=invar_numpy, true_outvar=outvar_numpy)
domain.add_validator(val, name="Val2")
# make monitors
invar_numpy = {"x": [[1.0]]}
monitor = PointwiseMonitor(
invar_numpy,
output_names=["u_1__x"],
metrics={"flux_u1": lambda var: torch.mean(var["u_1__x"])},
nodes=nodes,
requires_grad=True,
)
domain.add_monitor(monitor)
monitor = PointwiseMonitor(
invar_numpy,
output_names=["u_2__x"],
metrics={"flux_u2": lambda var: torch.mean(var["u_2__x"])},
nodes=nodes,
requires_grad=True,
)
domain.add_monitor(monitor)
# make solver
slv = Solver(cfg, domain)
# start solver
slv.solve()
if __name__ == "__main__":
run()
| 8,835 | Python | 28.065789 | 88 | 0.572835 |
openhackathons-org/End-to-End-AI-for-Science/workspace/python/source_code/diffusion_1d/conf/config.yaml | defaults :
- modulus_default
- arch:
- fully_connected
- scheduler: tf_exponential_lr
- optimizer: adam
- loss: sum
- _self_
arch:
fully_connected:
layer_size: 256
save_filetypes : "vtk,npz"
scheduler:
decay_rate: 0.95
decay_steps: 100
optimizer:
lr : 1e-4
training:
rec_results_freq: 1000
max_steps : 5000
batch_size:
rhs: 2
lhs: 2
interface: 2
interior_u1: 200
interior_u2: 200
| 437 | YAML | 12.272727 | 32 | 0.631579 |
openhackathons-org/End-to-End-AI-for-Science/workspace/python/source_code/darcy/darcy_FNO_lazy.py | # Copyright (c) 2023, NVIDIA CORPORATION. All rights reserved.
#
# Permission is hereby granted, free of charge, to any person obtaining
# a copy of this software and associated documentation files (the
# "Software"), to deal in the Software without restriction, including
# without limitation the rights to use, copy, modify, merge, publish,
# distribute, sublicense, and/or sell copies of the Software, and to
# permit persons to whom the Software is furnished to do so, subject to
# the following conditions:
#
# The above copyright notice and this permission notice shall be
# included in all copies or substantial portions of the Software.
#
# THE SOFTWARE IS PROVIDED "AS IS", WITHOUT WARRANTY OF ANY KIND,
# EXPRESS OR IMPLIED, INCLUDING BUT NOT LIMITED TO THE WARRANTIES OF
# MERCHANTABILITY, FITNESS FOR A PARTICULAR PURPOSE AND
# NONINFRINGEMENT. IN NO EVENT SHALL THE AUTHORS OR COPYRIGHT HOLDERS BE
# LIABLE FOR ANY CLAIM, DAMAGES OR OTHER LIABILITY, WHETHER IN AN ACTION
# OF CONTRACT, TORT OR OTHERWISE, ARISING FROM, OUT OF OR IN CONNECTION
# WITH THE SOFTWARE OR THE USE OR OTHER DEALINGS IN THE SOFTWARE.
import modulus
from modulus.sym.hydra import to_absolute_path, instantiate_arch, ModulusConfig
from modulus.sym.key import Key
from modulus.sym.solver import Solver
from modulus.sym.domain import Domain
from modulus.sym.domain.constraint import SupervisedGridConstraint
from modulus.sym.domain.validator import GridValidator
from modulus.sym.dataset import HDF5GridDataset
from modulus.sym.utils.io.plotter import GridValidatorPlotter
from utilities import download_FNO_dataset
@modulus.sym.main(config_path="conf", config_name="config_FNO")
def run(cfg: ModulusConfig) -> None:
# load training/ test data
input_keys = [Key("coeff", scale=(7.48360e00, 4.49996e00))]
output_keys = [Key("sol", scale=(5.74634e-03, 3.88433e-03))]
download_FNO_dataset("Darcy_241", outdir="datasets/")
train_path = to_absolute_path(
"datasets/Darcy_241/piececonst_r241_N1024_smooth1.hdf5"
)
test_path = to_absolute_path(
"datasets/Darcy_241/piececonst_r241_N1024_smooth2.hdf5"
)
# make datasets
train_dataset = HDF5GridDataset(
train_path, invar_keys=["coeff"], outvar_keys=["sol"], n_examples=1000
)
test_dataset = HDF5GridDataset(
test_path, invar_keys=["coeff"], outvar_keys=["sol"], n_examples=100
)
# make list of nodes to unroll graph on
decoder_net = instantiate_arch(
cfg=cfg.arch.decoder,
output_keys=output_keys,
)
fno = instantiate_arch(
cfg=cfg.arch.fno,
input_keys=input_keys,
decoder_net=decoder_net,
)
nodes = [fno.make_node('fno')]
# make domain
domain = Domain()
# add constraints to domain
supervised = SupervisedGridConstraint(
nodes=nodes,
dataset=train_dataset,
batch_size=cfg.batch_size.grid,
num_workers=4, # number of parallel data loaders
)
domain.add_constraint(supervised, "supervised")
# add validator
val = GridValidator(
nodes,
dataset=test_dataset,
batch_size=cfg.batch_size.validation,
plotter=GridValidatorPlotter(n_examples=5),
)
domain.add_validator(val, "test")
# make solver
slv = Solver(cfg, domain)
# start solver
slv.solve()
if __name__ == "__main__":
run()
| 3,392 | Python | 32.264706 | 79 | 0.704009 |
openhackathons-org/End-to-End-AI-for-Science/workspace/python/source_code/darcy/utilities.py | # Copyright (c) 2023, NVIDIA CORPORATION. All rights reserved.
#
# Permission is hereby granted, free of charge, to any person obtaining
# a copy of this software and associated documentation files (the
# "Software"), to deal in the Software without restriction, including
# without limitation the rights to use, copy, modify, merge, publish,
# distribute, sublicense, and/or sell copies of the Software, and to
# permit persons to whom the Software is furnished to do so, subject to
# the following conditions:
#
# The above copyright notice and this permission notice shall be
# included in all copies or substantial portions of the Software.
#
# THE SOFTWARE IS PROVIDED "AS IS", WITHOUT WARRANTY OF ANY KIND,
# EXPRESS OR IMPLIED, INCLUDING BUT NOT LIMITED TO THE WARRANTIES OF
# MERCHANTABILITY, FITNESS FOR A PARTICULAR PURPOSE AND
# NONINFRINGEMENT. IN NO EVENT SHALL THE AUTHORS OR COPYRIGHT HOLDERS BE
# LIABLE FOR ANY CLAIM, DAMAGES OR OTHER LIABILITY, WHETHER IN AN ACTION
# OF CONTRACT, TORT OR OTHERWISE, ARISING FROM, OUT OF OR IN CONNECTION
# WITH THE SOFTWARE OR THE USE OR OTHER DEALINGS IN THE SOFTWARE.
import os
import zipfile
try:
import gdown
except:
gdown = None
import scipy.io
import numpy as np
import h5py
from modulus.sym.hydra import to_absolute_path
# list of FNO dataset url ids on drive: https://drive.google.com/drive/folders/1UnbQh2WWc6knEHbLn-ZaXrKUZhp7pjt-
_FNO_datatsets_ids = {
"Darcy_241": "1ViDqN7nc_VCnMackiXv_d7CHZANAFKzV",
"Darcy_421": "1Z1uxG9R8AdAGJprG5STcphysjm56_0Jf",
}
_FNO_dataset_names = {
"Darcy_241": (
"piececonst_r241_N1024_smooth1.hdf5",
"piececonst_r241_N1024_smooth2.hdf5",
),
"Darcy_421": (
"piececonst_r421_N1024_smooth1.hdf5",
"piececonst_r421_N1024_smooth2.hdf5",
),
}
def load_FNO_dataset(path, input_keys, output_keys, n_examples=None):
"Loads a FNO dataset"
if not path.endswith(".hdf5"):
raise Exception(
".hdf5 file required: please use utilities.preprocess_FNO_mat to convert .mat file"
)
# load data
path = to_absolute_path(path)
data = h5py.File(path, "r")
_ks = [k for k in data.keys() if not k.startswith("__")]
print(f"loaded: {path}\navaliable keys: {_ks}")
# parse data
invar, outvar = dict(), dict()
for d, keys in [(invar, input_keys), (outvar, output_keys)]:
for k in keys:
# get data
x = data[k] # N, C, H, W
# cut examples out
if n_examples is not None:
x = x[:n_examples]
# print out normalisation values
print(f"selected key: {k}, mean: {x.mean():.5e}, std: {x.std():.5e}")
d[k] = x
del data
return (invar, outvar)
def download_FNO_dataset(name, outdir="datasets/"):
"Tries to download FNO dataset from drive"
if name not in _FNO_datatsets_ids:
raise Exception(
f"Error: FNO dataset {name} not recognised, select one from {list(_FNO_datatsets_ids.keys())}"
)
id = _FNO_datatsets_ids[name]
outdir = to_absolute_path(outdir) + "/"
namedir = f"{outdir}{name}/"
# skip if already exists
exists = True
for file_name in _FNO_dataset_names[name]:
if not os.path.isfile(namedir + file_name):
exists = False
break
if exists:
return
print(f"FNO dataset {name} not detected, downloading dataset")
# Make sure we have gdown installed
if gdown is None:
raise ModuleNotFoundError("gdown package is required to download the dataset!")
# get output directory
os.makedirs(namedir, exist_ok=True)
# download dataset
zippath = f"{outdir}{name}.zip"
_download_file_from_google_drive(id, zippath)
# unzip
with zipfile.ZipFile(zippath, "r") as f:
f.extractall(namedir)
os.remove(zippath)
# preprocess files
for file in os.listdir(namedir):
if file.endswith(".mat"):
matpath = f"{namedir}{file}"
preprocess_FNO_mat(matpath)
os.remove(matpath)
def _download_file_from_google_drive(id, path):
"Downloads a file from google drive"
# use gdown library to download file
gdown.download(id=id, output=path)
def preprocess_FNO_mat(path):
"Convert a FNO .mat file to a hdf5 file, adding extra dimension to data arrays"
assert path.endswith(".mat")
data = scipy.io.loadmat(path)
ks = [k for k in data.keys() if not k.startswith("__")]
with h5py.File(path[:-4] + ".hdf5", "w") as f:
for k in ks:
x = np.expand_dims(data[k], axis=1) # N, C, H, W
f.create_dataset(
k, data=x, dtype="float32"
) # note h5 files larger than .mat because no compression used
| 4,794 | Python | 30.339869 | 112 | 0.646016 |
openhackathons-org/End-to-End-AI-for-Science/workspace/python/source_code/darcy/ops.py | # Copyright (c) 2023, NVIDIA CORPORATION. All rights reserved.
#
# Permission is hereby granted, free of charge, to any person obtaining
# a copy of this software and associated documentation files (the
# "Software"), to deal in the Software without restriction, including
# without limitation the rights to use, copy, modify, merge, publish,
# distribute, sublicense, and/or sell copies of the Software, and to
# permit persons to whom the Software is furnished to do so, subject to
# the following conditions:
#
# The above copyright notice and this permission notice shall be
# included in all copies or substantial portions of the Software.
#
# THE SOFTWARE IS PROVIDED "AS IS", WITHOUT WARRANTY OF ANY KIND,
# EXPRESS OR IMPLIED, INCLUDING BUT NOT LIMITED TO THE WARRANTIES OF
# MERCHANTABILITY, FITNESS FOR A PARTICULAR PURPOSE AND
# NONINFRINGEMENT. IN NO EVENT SHALL THE AUTHORS OR COPYRIGHT HOLDERS BE
# LIABLE FOR ANY CLAIM, DAMAGES OR OTHER LIABILITY, WHETHER IN AN ACTION
# OF CONTRACT, TORT OR OTHERWISE, ARISING FROM, OUT OF OR IN CONNECTION
# WITH THE SOFTWARE OR THE USE OR OTHER DEALINGS IN THE SOFTWARE.
import torch
import torch.nn.functional as F
def dx(inpt, dx, channel, dim, order=1, padding="zeros"):
"Compute first order numerical derivatives of input tensor"
var = inpt[:, channel : channel + 1, :, :]
# get filter
if order == 1:
ddx1D = torch.Tensor(
[
-0.5,
0.0,
0.5,
]
).to(inpt.device)
elif order == 3:
ddx1D = torch.Tensor(
[
-1.0 / 60.0,
3.0 / 20.0,
-3.0 / 4.0,
0.0,
3.0 / 4.0,
-3.0 / 20.0,
1.0 / 60.0,
]
).to(inpt.device)
ddx3D = torch.reshape(ddx1D, shape=[1, 1] + dim * [1] + [-1] + (1 - dim) * [1])
# apply convolution
if padding == "zeros":
var = F.pad(var, 4 * [(ddx1D.shape[0] - 1) // 2], "constant", 0)
elif padding == "replication":
var = F.pad(var, 4 * [(ddx1D.shape[0] - 1) // 2], "replicate")
output = F.conv2d(var, ddx3D, padding="valid")
output = (1.0 / dx) * output
if dim == 0:
output = output[:, :, :, (ddx1D.shape[0] - 1) // 2 : -(ddx1D.shape[0] - 1) // 2]
elif dim == 1:
output = output[:, :, (ddx1D.shape[0] - 1) // 2 : -(ddx1D.shape[0] - 1) // 2, :]
return output
def ddx(inpt, dx, channel, dim, order=1, padding="zeros"):
"Compute second order numerical derivatives of input tensor"
var = inpt[:, channel : channel + 1, :, :]
# get filter
if order == 1:
ddx1D = torch.Tensor(
[
1.0,
-2.0,
1.0,
]
).to(inpt.device)
elif order == 3:
ddx1D = torch.Tensor(
[
1.0 / 90.0,
-3.0 / 20.0,
3.0 / 2.0,
-49.0 / 18.0,
3.0 / 2.0,
-3.0 / 20.0,
1.0 / 90.0,
]
).to(inpt.device)
ddx3D = torch.reshape(ddx1D, shape=[1, 1] + dim * [1] + [-1] + (1 - dim) * [1])
# apply convolution
if padding == "zeros":
var = F.pad(var, 4 * [(ddx1D.shape[0] - 1) // 2], "constant", 0)
elif padding == "replication":
var = F.pad(var, 4 * [(ddx1D.shape[0] - 1) // 2], "replicate")
output = F.conv2d(var, ddx3D, padding="valid")
output = (1.0 / dx ** 2) * output
if dim == 0:
output = output[:, :, :, (ddx1D.shape[0] - 1) // 2 : -(ddx1D.shape[0] - 1) // 2]
elif dim == 1:
output = output[:, :, (ddx1D.shape[0] - 1) // 2 : -(ddx1D.shape[0] - 1) // 2, :]
return output
| 3,754 | Python | 33.136363 | 88 | 0.531167 |
openhackathons-org/End-to-End-AI-for-Science/workspace/python/source_code/darcy/conf/config_FNO-Backup.yaml | defaults :
- modulus_default
- arch:
- fno
- scheduler: tf_exponential_lr
- optimizer: adam
- loss: sum
- _self_
jit: false
arch:
fno:
dimension: 2
nr_fno_layers: 4
fno_layer_size: 32
fno_modes: 12
padding: 9
output_fc_layer_sizes:
- 128
scheduler:
decay_rate: 0.95
decay_steps: 1000
training:
rec_results_freq : 1000
max_steps : 10000
batch_size:
grid: 32
validation: 32 | 443 | YAML | 12.875 | 32 | 0.604966 |
openhackathons-org/End-to-End-AI-for-Science/workspace/python/source_code/darcy/conf/config_AFNO.yaml | defaults :
- modulus_default
- arch:
- afno
- scheduler: tf_exponential_lr
- optimizer: adam
- loss: sum
- _self_
arch:
afno:
patch_size: 16
embed_dim: 256
depth: 4
num_blocks: 8
scheduler:
decay_rate: 0.95
decay_steps: 1000
training:
rec_results_freq : 1000
max_steps : 10000
batch_size:
grid: 32
validation: 32
| 369 | YAML | 12.214285 | 32 | 0.609756 |
openhackathons-org/End-to-End-AI-for-Science/workspace/python/source_code/darcy/conf/config_FNO.yaml | defaults :
- modulus_default
- /arch/[email protected]
- /arch/[email protected]
- scheduler: tf_exponential_lr
- optimizer: adam
- loss: sum
- _self_
arch:
decoder:
input_keys: [z, 32]
output_keys: sol
nr_layers: 1
layer_size: 32
fno:
input_keys: coeff
dimension: 2
nr_fno_layers: 4
fno_modes: 12
padding: 9
scheduler:
decay_rate: 0.95
decay_steps: 1000
training:
rec_results_freq : 1000
max_steps : 10000
batch_size:
grid: 32
validation: 32 | 532 | YAML | 14.67647 | 47 | 0.633459 |
openhackathons-org/End-to-End-AI-for-Science/workspace/python/source_code/navier_stokes/navier_stokes.py | # Copyright (c) 2023, NVIDIA CORPORATION. All rights reserved.
#
# Permission is hereby granted, free of charge, to any person obtaining
# a copy of this software and associated documentation files (the
# "Software"), to deal in the Software without restriction, including
# without limitation the rights to use, copy, modify, merge, publish,
# distribute, sublicense, and/or sell copies of the Software, and to
# permit persons to whom the Software is furnished to do so, subject to
# the following conditions:
#
# The above copyright notice and this permission notice shall be
# included in all copies or substantial portions of the Software.
#
# THE SOFTWARE IS PROVIDED "AS IS", WITHOUT WARRANTY OF ANY KIND,
# EXPRESS OR IMPLIED, INCLUDING BUT NOT LIMITED TO THE WARRANTIES OF
# MERCHANTABILITY, FITNESS FOR A PARTICULAR PURPOSE AND
# NONINFRINGEMENT. IN NO EVENT SHALL THE AUTHORS OR COPYRIGHT HOLDERS BE
# LIABLE FOR ANY CLAIM, DAMAGES OR OTHER LIABILITY, WHETHER IN AN ACTION
# OF CONTRACT, TORT OR OTHERWISE, ARISING FROM, OUT OF OR IN CONNECTION
# WITH THE SOFTWARE OR THE USE OR OTHER DEALINGS IN THE SOFTWARE.
import numpy as np
import os
from sympy import Symbol, Eq, Abs, sin, cos
import modulus
from modulus.sym.hydra import to_absolute_path, instantiate_arch, ModulusConfig
from modulus.sym.eq.pdes.navier_stokes import NavierStokes
from modulus.sym.geometry.primitives_2d import Rectangle as rect
from modulus.sym.models.fully_connected import FullyConnectedArch
from modulus.sym.key import Key
from modulus.sym.node import Node
from modulus.sym.solver import Solver
from modulus.sym.domain import Domain
from modulus.sym.domain.constraint import (
PointwiseConstraint,
PointwiseInteriorConstraint,
)
from modulus.sym.domain.inferencer import PointVTKInferencer
from modulus.sym.utils.io import (
VTKUniformGrid,
)
def read_wf_data(velocity_scale,pressure_scale):
path = "/workspace/python/source_code/navier_stokes/data_lat.npy"
print(path)
ic = np.load(path).astype(np.float32)
Pa_to_kgm3 = 0.10197
mesh_y, mesh_x = np.meshgrid(
np.linspace(-0.720, 0.719, ic[0].shape[0]),
np.linspace(-0.720, 0.719, ic[0].shape[1]),
indexing="ij",
)
invar = {}
invar["x"] = np.expand_dims(mesh_x.astype(np.float32).flatten(),axis=-1)
invar["y"] = np.expand_dims(mesh_y.astype(np.float32).flatten(),axis=-1)
invar["t"] = np.full_like(invar["x"], 0)
outvar = {}
outvar["u"] = np.expand_dims((ic[0]/velocity_scale).flatten(),axis=-1)
outvar["v"] = np.expand_dims((ic[1]/velocity_scale).flatten(),axis=-1)
outvar["p"] = np.expand_dims((ic[2]*Pa_to_kgm3/pressure_scale).flatten(),axis=-1)
return invar, outvar
@modulus.sym.main(config_path="conf", config_name="config")
def run(cfg: ModulusConfig) -> None:
# define sympy variables to parametrize domain curves
x, y = Symbol("x"), Symbol("y")
# make geometry for problem
length = (-0.720, 0.720)
height = (-0.720, 0.720)
box_bounds = {x: length, y: height}
# define geometry
rec = rect(
(length[0], height[0]),
(length[1], height[1])
)
# Scaling and Nondimensionalizing the Problem
#############
# Real Params
#############
fluid_kinematic_viscosity = 1.655e-5 # m**2/s
fluid_density = 1.1614 # kg/m**3
fluid_specific_heat = 1005 # J/(kg K)
fluid_conductivity = 0.0261 # W/(m K)
################
# Non dim params for normalisation
################
# Diameter of Earth : 12742000 m over range of 1.440
length_scale = 12742000/1.440
# 60 hrs to 1 timestep- every inference frame is a 6 hour prediction (s)
time_scale = 60*60*60
# Calcuale velocity & pressure scale
velocity_scale = length_scale / time_scale # m/s
pressure_scale = fluid_density * ((length_scale / time_scale) ** 2) # kg / (m s**2)
# Density scale
density_scale = 1.1614 # kg/m3
##############################
# Nondimensionalization Params for NavierStokes fn
##############################
# fluid params
nd_fluid_kinematic_viscosity = fluid_kinematic_viscosity / (
length_scale ** 2 / time_scale
)
nd_fluid_density = fluid_density / density_scale
# time window parameters
time_window_size = 1.0
t_symbol = Symbol("t")
time_range = {t_symbol: (0, time_window_size)}
# make navier stokes equations
ns = NavierStokes(nu=nd_fluid_kinematic_viscosity, rho=nd_fluid_density, dim=2, time=True)
# make network
flow_net = FullyConnectedArch(
input_keys=[Key("x"), Key("y"), Key("t")],
output_keys=[Key("u"), Key("v"), Key("p")],
periodicity={"x": length, "y" : height},
layer_size=256,
)
# make nodes to unroll graph on
nodes = ns.make_nodes() + [flow_net.make_node(name="flow_net")]
# make initial condition domain
navier = Domain("navier_stokes")
# make initial condition
ic_invar,ic_outvar = read_wf_data(velocity_scale,pressure_scale)
ic = PointwiseConstraint.from_numpy(
nodes,
ic_invar,
ic_outvar,
batch_size=cfg.batch_size.initial_condition,
)
navier.add_constraint(ic, name="ic")
# make interior constraint
interior = PointwiseInteriorConstraint(
nodes=nodes,
geometry=rec,
outvar={"continuity": 0, "momentum_x": 0, "momentum_y": 0},
bounds=box_bounds,
batch_size=cfg.batch_size.interior,
parameterization=time_range,
)
navier.add_constraint(interior, name="interior")
# add inference data for time slices
for i, specific_time in enumerate(np.linspace(0, time_window_size, 10)):
vtk_obj = VTKUniformGrid(
bounds=[(-0.720, 0.720), (-0.360, 0.360)],
npoints=[1440,720],
export_map={"u": ["u", "v"], "p": ["p"]},
)
grid_inference = PointVTKInferencer(
vtk_obj=vtk_obj,
nodes=nodes,
input_vtk_map={"x": "x", "y": "y"},
output_names=["u", "v", "p"],
requires_grad=False,
invar={"t": np.full([720 *1440, 1], specific_time)},
batch_size=100000,
)
navier.add_inferencer(grid_inference, name="time_slice_" + str(i).zfill(4))
slv = Solver(cfg, navier)
# start solver
slv.solve()
if __name__ == "__main__":
run()
| 6,473 | Python | 33.43617 | 94 | 0.631083 |
openhackathons-org/End-to-End-AI-for-Science/workspace/python/source_code/navier_stokes/conf/config.yaml | defaults :
- modulus_default
- arch:
- fully_connected
- scheduler: tf_exponential_lr
- optimizer: adam
- loss: sum
- _self_
save_filetypes : "vtk,npz"
scheduler:
decay_rate: 0.95
decay_steps: 3000
training:
rec_results_freq : 1000
rec_constraint_freq: 5000
max_steps : 110000
batch_size:
initial_condition: 2048
interior: 2048
| 364 | YAML | 14.208333 | 32 | 0.67033 |
metaiintw/build-an-avatar-with-ASR-TTS-Transformer-Omniverse-Audio2Face/README.md | # Build an avatar with ASR, Sentence-transformer, Similarity Search, TTS and Omniverse Audio2Face
## Project Description
I'll show you how I used several Python packages and NVIDIA's Omniverse Audio2Face to quickly implement an avatar that can answer questions defined in a knowledge set or FAQ.
## Demo
[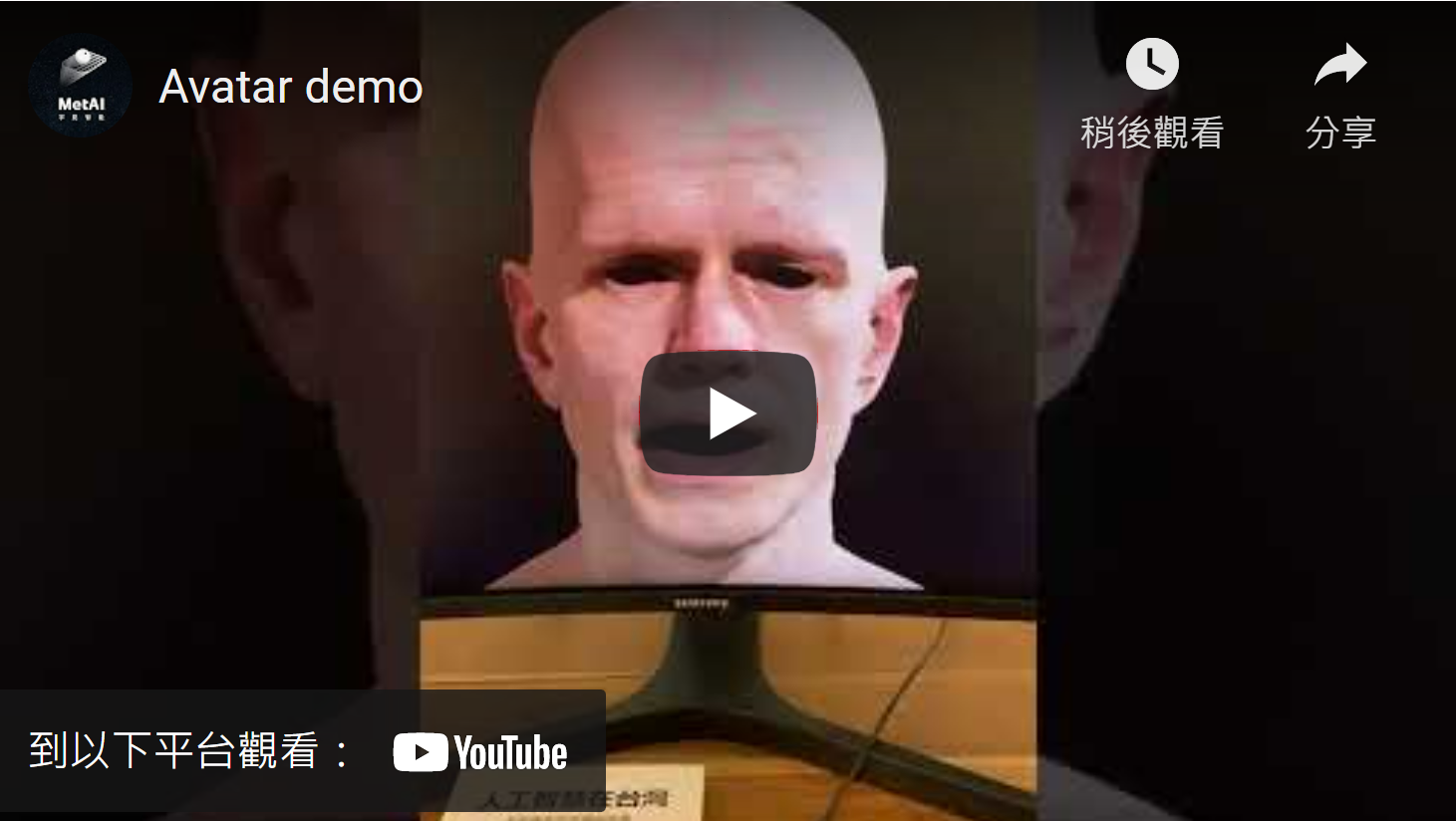](http://www.youtube.com/watch?v=G_c94cGIKgs "Video Title")
## How It Works

#### ***Automatic Speech Recognition, ASR***
Upon receiving user's request, the [SpeechRecognition API](https://pypi.org/project/SpeechRecognition/) records the frequencies and sound waves from user's voice and translates them into text.
#### ***Language Understanding***
[Sentence-Transformer](https://www.sbert.net/) is for state-of-the-art sentence, text and image embeddings that can encode input questions into feature vectors. The feature vectors represent entire sentences and their semantic information, this helps the machine in understanding the context, intention, and other nuances in the entire text.
We’ll conduct a similarity search, comparing a user input question to a list of FAQs and return the most likely answers by [Facebook’s Similarity Search API](https://ai.facebook.com/tools/faiss/).
#### ***Text To Speech***
The avatar's voice is fully synthesized by the [Gtts API](https://pypi.org/project/gTTS/), which turns text into natural-sounding speech. The synthesized voice is also used to drive the avatar's facial animation.
#### ***Omniverse Audio2Face***

Omniverse Audio2Face is an application brings our avatars to life. With [Omniverse Audio2Face](https://www.nvidia.com/en-us/omniverse/apps/audio2face/), anyone can now create realistic facial expressions and emotions to match any voice-over track. The technology feeds the audio input into a pre-trained Deep Neural Network, based on NVIDIA and the output of the network drives the facial animation of 3D characters in real-time.
## System Requirements
| Element | Minimum Specifications |
| ---- | ---- |
| OS Supported | Windows 10 64-bit (Version 1909 and above) |
| CPU | Intel I7, AMD Ryzen 2.5GHz or greater |
| CPU Cores | 4 or higher |
| RAM | 16 GB or higher |
| Storage | 500 Gb SSD or higher |
| GPU | Any RTX GPU |
| VRAM | 6 GB or higher |
| Min. Video Driver Version | See latest drivers [here](https://developer.nvidia.com/omniverse/driver) |
## How to Install and Run the Project
Before you begin, you'll need to clone the repository with the template code used in this repo. Open your Terminal app and find a directory where you'd like to store the code. Run this command to clone the GitHub App template repository:
```
$ git clone https://github.com/metaiintw/build-an-avatar-with-ASR-TTS-Transformer-Omniverse-Audio2Face.git
```
#### Creating an environment from an environment. yml file
Make sure Anaconda is installed on your local machine. Use the following command to install packages included in requirements.yml:
```
$ conda env create -f /path/to/requirements.yml
```
#### Download and Install Omniverse Launcher
[NVIDIA Omniverse](https://docs.omniverse.nvidia.com/prod_install-guide/prod_install-guide.html) is a development platform for 3D simulation and design collaboration, it is free for individual, you can download Omniverse Launcher [here](https://www.nvidia.com/en-us/omniverse/download/).
I also recommend you to watch this [video tutorial](https://www.youtube.com/watch?v=Ol-bCNBgyFw), which guides you through the installation process.
|  |
|:--:|
| *Omniverse Launcher* |
#### Install Omniverse Audio2Face
|  |
|:--:|
| *Omniverse apps* |
Once you got Omniverse Launcher installed, you can immediate access to all the apps, including [Omniverse Audio2Face](https://www.nvidia.com/en-us/omniverse/apps/audio2face/). Next, simply install Omniverse Audio2Face and you're good to go.
|  |
|:--:|
| *Omniverse Audio2Face* |
#### Omniverse Audio2Face setup
To get our Python program interacts with Omniverse Audio2Face, you should use streaming audio player that allows developers to stream audio data from an external source or applications via the gRPC protocol.
|  |
|:--:|
| *streaming audio player allows developers to stream audio data from an external source* |
This [tutorial](https://www.youtube.com/watch?v=qKhPwdcOG_w&t=17s) showcases how to create an audio player and connect it to the audio2face instance using the omnigraph editor.
#### Bring Your Avatar to life
Now we're ready to bring our avatar to life, simply enter the following commands into your terminal.
```
$ cd path_to_the_project_folder
$ conda activate avatar
$ jupyter lab
```
Execute the .ipynb notebook file named ***1.Creating_a_simple_avatar.ipynb***, start building your first avatar!
|  |
|:--:|
| *1.Creating_a_simple_avatar.ipynb* |
## Creators
**Renton Hsu**
- [Linkedin](https://www.linkedin.com/in/renton-hsu-bba5a0102)
- [Facebook](https://www.facebook.com/renton.hsu/)
| 5,296 | Markdown | 53.608247 | 429 | 0.749056 |
metaiintw/build-an-avatar-with-ASR-TTS-Transformer-Omniverse-Audio2Face/1.Create_A_Simple_Avatar/requirements.yml | name: avatar666
channels:
- conda-forge
- pytorch-nightly
dependencies:
- argon2-cffi=21.3.0
- argon2-cffi-bindings=21.2.0
- ca-certificates=2020.10.14
- charset-normalizer=2.0.4
- huggingface_hub=0.5.1
- importlib-metadata=4.11.3
- intel-openmp=2021.4.0
- libfaiss-avx2=1.7.2
- lz4-c=1.9.3=h2bbff1b_1
- m2w64-gcc-libgfortran=5.3.0
- m2w64-gcc-libs=5.3.0=7
- m2w64-gcc-libs-core=5.3.0=7
- m2w64-gmp=6.1.0
- m2w64-libwinpthread-git=5.0.0.4634.697f757=2
- matplotlib-inline=0.1.2
- mkl-service=2.4.0
- msys2-conda-epoch=20160418
- nest-asyncio=1.5.5
- numpy-base=1.21.5
- prompt-toolkit=3.0.20
- python-dateutil=2.8.2
- python-fastjsonschema=2.15.1
- pytorch-mutex=1.0
- scikit-learn=1.0.2
- sentence-transformers=2.2.0
- typing-extensions=4.1.1
- websocket-client=0.58.0
- ffmpeg-python=0.2.0
- pyaudio=0.2.11
- faiss=1.7.2
- pip:
# Package that is only on PyPI
- jupyter
- notebook
- jupyterlab
- grpcio
- pandas
- faiss
- pydub
- soundfile
- google
- protobuf==3.20.0
- gtts
- librosa==0.7.2
- numba==0.48
- SpeechRecognition | 1,184 | YAML | 22.235294 | 48 | 0.614865 |
eliabntt/animated_human_SMPL_to_USD/generate_sequence.py | import json
import os
import humangenerator
import bpy
import humangenerator as hgen
import argparse
import ipdb
import sys
import yaml
parser = argparse.ArgumentParser()
parser.add_argument("--dataset", help="Dataset from which you want to generate data")
parser.add_argument("--output_dir", help="Path to where the data should be saved")
parser.add_argument("--samples_dir", help="Paths where the data is stored")
parser.add_argument("--last_sample",
help="Last sample processed, this must be the FULL name of the folder (e.g. 00001). This WILL be processed",
default="")
parser.add_argument("--parent_path", help="Path containing the subfolders for the datasets (with the pkl models)",
default="")
parser.add_argument("--sample_id", help="ID of the sample, if emtpy process all", default="all")
parser.add_argument("--with_cache", help="Write \"False\" if generating blendshapes", default="True")
parser.add_argument("--suppress_out", help="Write \"False\" if output in console", default="False")
parser.add_argument("--write_verts", help="Write \"True\" if you want to write verts info in the pkl", default="False")
parser.add_argument("--frame", help="The n-th frame to generate. Default all", default="all")
parser.add_argument("--config_file", help="json file containing the configuration", default="")
parser.add_argument("--exp_name",
help="The name of the \"experiment\" of the dataset. By default the name of the samples_dir folder",
default="")
# structure should be `parent_path/[surreal/datageneration/smpl_data,body_models/{smplh,dmpls}]`
args = parser.parse_args()
with open(os.path.join("humangenerator", "avail_datasets.yaml"), 'r') as stream:
data_loaded = yaml.safe_load(stream)
avail_datasets = data_loaded["datasets"]
processor = None
if avail_datasets == [] or args.dataset not in avail_datasets:
if not avail_datasets:
print("No avail dataset. Check file")
else:
print(f"Sought dataset is not yet avail. The avail ones are {avail_datasets}")
exit(-1)
else:
print(f"Processing {args.dataset} data")
found = (args.last_sample == "")
try:
WITH_CACHE = (False if args.with_cache == "False" else True)
parent_path = args.parent_path
smpl_body_list = []
# Init SMPL models
smpl_path = os.path.join(parent_path, "surreal", "datageneration", "smpl_data")
smpl_models = {
'f': hgen.SMPLModel(os.path.join(smpl_path, 'smpl', 'models', 'basicModel_f_lbs_10_207_0_v1.0.0.pkl')),
'm': hgen.SMPLModel(os.path.join(smpl_path, 'smpl', 'models', 'basicModel_m_lbs_10_207_0_v1.0.0.pkl')),
}
if args.frame != "all":
try:
frame = int(args.frame)
except:
print("Error converting frame to int, considering the WHOLE sequence")
frame = None
else:
frame = None
print("Whole sequence considered")
print("This will export only the whole sequence")
hgen.init()
# Parse args
PATH_SAMPLES = args.samples_dir
if args.exp_name == "":
exp_name = os.path.split(PATH_SAMPLES)[-1]
else:
exp_name = args.exp_name
PATH_OUT = os.path.join(args.output_dir, exp_name)
if not os.path.exists(PATH_OUT):
os.makedirs(PATH_OUT)
if args.config_file == "":
config = {}
else:
if os.path.exists(args.config_file):
with open(args.config_file, "r") as f:
config = json.load(f)
else:
raise Exception("The taxonomy file could not be found: {}".format(args.config_file))
processor, PATH_SAMPLES = hgen.get_processor(args.dataset, parent_path, WITH_CACHE, PATH_OUT, PATH_SAMPLES,
smpl_models, args.write_verts.lower() == "false", config)
sample_id = args.sample_id
if sample_id != "all":
print("Processing single sample")
# Check if sample exists
if not os.path.isdir(os.path.join(PATH_SAMPLES, sample_id)):
print("Specified sample does not exist")
exit(-1)
else:
sample_id = [sample_id]
else:
print("Processing all samples")
sample_id = os.listdir(PATH_SAMPLES)
if not sample_id:
print("No subfolder found")
exit(-1)
if len(smpl_body_list) == 0:
smpl_body_list = processor.generator.load_SMPLs_objects()
found = (args.last_sample == "")
sample_id.sort()
clean_cnt = 1
for sample in sample_id:
if not found:
if sample == args.last_sample:
found = True
else:
continue
if clean_cnt % 100 == 0:
clean_cnt = 0
hgen.init()
smpl_body_list = processor.generator.load_SMPLs_objects()
clean_cnt += 1
print("------------------------------")
print(f"Processing {sample}")
isdone = False
count = 0
while (not isdone and count <= 5):
hgen.deselect()
if len(sample_id) > 1:
hgen.clean_mesh_and_textures(
exclude=['Material_0', 'Material_1', 'Armature_0', 'Armature_1', 'body_0', 'body_1'])
print("Scene cleaned!\n\n")
count += 1
path_sample = os.path.join(PATH_OUT, sample + ('_with_cache' if WITH_CACHE else ''))
if not os.path.exists(path_sample):
os.makedirs(path_sample)
with open(os.path.join(path_sample, f"out_{count}.txt"), "w") as file_out, open(
os.path.join(path_sample, f"err_{count}.txt"), "w") as file_err:
# file logging
try:
if args.suppress_out == "True":
sys.stdout = file_out
sys.stderr = file_err
res = processor.process_sample(sample, frame, smpl_body_list)
if res:
print("Exported!")
else:
raise Exception("Unknown error")
isdone = True
except:
import traceback
sys.stderr.write('error\n')
sys.stderr.write(traceback.format_exc())
print(f"Failed -- going with try {count}\n\n")
finally:
sys.stderr.flush()
sys.stdout.flush()
sys.stdout = sys.__stdout__
sys.stderr = sys.__stderr__
except:
import traceback
sys.stderr.write('error\n')
sys.stderr.write(traceback.format_exc())
sys.stdout.flush()
sys.stderr.flush()
sys.stdout = sys.__stdout__
sys.stderr = sys.__stderr__
print('error')
print(traceback.format_exc())
extype, value, tb = sys.exc_info()
ipdb.post_mortem(tb)
| 6,955 | Python | 35.610526 | 128 | 0.570669 |
eliabntt/animated_human_SMPL_to_USD/start_blend_debug.py | import bpy
import sys
import ipdb
import os
from pathlib import Path
from bl_ui.space_text import TEXT_MT_editor_menus
repo_root_directory = os.path.join(os.path.dirname(__file__), ".")
sys.path.append(repo_root_directory)
argv = sys.argv[sys.argv.index("--") + 1:]
bpy.context.window.workspace = bpy.data.workspaces["Scripting"]
bpy.context.view_layer.update()
if argv[0].endswith(".py"):
print(f"Loading: {os.path.join(os.path.dirname(os.path.abspath(__file__)), argv[0])}")
text = bpy.data.texts.load(os.path.join(os.path.dirname(os.path.abspath(__file__)), argv[0]))
sys.argv = argv[:]
print(f"New argv: {sys.argv}")
else:
print("First argument should be the script file")
exit(-1)
# Declare operator that runs the blender proc script
class RunHumanGeneratorOperator(bpy.types.Operator):
bl_idname = "wm.run_humangenerator"
bl_label = "Run Human Generator"
bl_description = "This operator runs the loaded HumanGenerator script and also makes sure to unload all modules before starting."
bl_options = {"REGISTER"}
def execute(self, context):
# Delete all loaded models inside src/, as they are cached inside blender
for module in list(sys.modules.keys()):
if module.startswith("humangenerator"):
del sys.modules[module]
# Make sure the parent of the humangenerator folder is in sys.path
import_path = os.path.abspath(os.path.join(os.path.dirname(__file__), "."))
if import_path not in sys.path:
sys.path.append(import_path)
# Run the script
try:
bpy.ops.text.run_script()
except RuntimeError:
# Skip irrelevant error messages (The relevant stacktrace+error has already been printed at this point)
pass
return {"FINISHED"}
bpy.utils.register_class(RunHumanGeneratorOperator)
def draw(self, context):
layout = self.layout
st = context.space_data
text = st.text
is_syntax_highlight_supported = st.is_syntax_highlight_supported()
layout.template_header()
TEXT_MT_editor_menus.draw_collapsible(context, layout)
if text and text.is_modified:
row = layout.row(align=True)
row.alert = True
row.operator("text.resolve_conflict", text="", icon='HELP')
layout.separator_spacer()
row = layout.row(align=True)
row.template_ID(st, "text", new="text.new",
unlink="text.unlink", open="text.open")
if text:
is_osl = text.name.endswith((".osl", ".osl"))
if is_osl:
row.operator("node.shader_script_update",
text="", icon='FILE_REFRESH')
else:
row = layout.row()
row.active = is_syntax_highlight_supported
# The following line has changed compared to the orignal code, it starts our operator instead of text.run_script
row.operator("wm.run_humangenerator", text="Run")
layout.separator_spacer()
row = layout.row(align=True)
row.prop(st, "show_line_numbers", text="")
row.prop(st, "show_word_wrap", text="")
syntax = row.row(align=True)
syntax.active = is_syntax_highlight_supported
syntax.prop(st, "show_syntax_highlight", text="")
# Set our draw function as the default draw function for text area headers
bpy.types.TEXT_HT_header.draw = draw
# Put text into scripting tool
for area in bpy.data.workspaces["Scripting"].screens[0].areas.values():
if area.type == 'TEXT_EDITOR':
area.spaces.active.text = text | 3,540 | Python | 34.767676 | 133 | 0.652825 |
eliabntt/animated_human_SMPL_to_USD/convert_fbx.py | import json
import os
import humangenerator
import bpy
import humangenerator as hgen
import argparse
import ipdb
import sys
import yaml
parser = argparse.ArgumentParser()
parser.add_argument("--fbx", help="Path to the fbx file")
parser.add_argument("--output_dir", help="Path to where the data should be saved")
parser.add_argument("--temp_dir", help="Path to where the data should be temporary saved")
parser.add_argument("--usd", help="True if export usd is necessary, default to false", default="False")
args = parser.parse_args()
out_dir = args.output_dir
if not os.path.exists(out_dir):
os.makedirs(out_dir)
fbx = args.fbx
for o in bpy.context.scene.objects:
o.select_set(True)
# Call the operator only once
bpy.ops.object.delete()
with open(os.path.join(out_dir, f"out.txt"), "w") as file_out, open(
os.path.join(out_dir, f"err.txt"), "w") as file_err:
try:
sys.stdout = file_out
sys.stderr = file_err
bpy.ops.import_scene.fbx(filepath=fbx)
filepath=os.path.join(out_dir,os.path.basename(fbx[:-4])+".usd")
temp_filepath = os.path.join(args.temp_dir,os.path.basename(fbx[:-4])+".usd")
hgen.export_data(temp_path, out_dir, os.path.basename(fbx[:-4]), False, None, {}, {}, False, args.usd.lower() == "true")
bpy.ops.object.select_all(action='SELECT')
bpy.ops.object.delete()
succeed = True
except:
import traceback
sys.stderr.write('error\n')
sys.stderr.write(traceback.format_exc())
finally:
sys.stdout.flush()
sys.stderr.flush()
sys.stdout = sys.__stdout__
sys.stderr = sys.__stderr__ | 1,653 | Python | 30.807692 | 128 | 0.655777 |
eliabntt/animated_human_SMPL_to_USD/notes.md | Installation instructions
From the `generate_people` folder
```
mkdir data_folder
cd data_folder
git clone https://github.com/gulvarol/surreact surreal
```
- Download the following two fbx files for SMPL for Maya from https://smpl.is.tue.mpg.de/ using your credentials. Please comply with their license. The files are `basicModel_f_lbs_10_207_0_v1.0.2.fbx` and `basicModel_m_lbs_10_207_0_v1.0.2.fbx` and can be downloaded with this [link](https://download.is.tue.mpg.de/download.php?domain=smpl&sfile=SMPL_maya.zip). Place them in `.../surreal/datageneration/smpl_data`.
- download this [pkl](https://raw.githubusercontent.com/gulvarol/surreal/master/datageneration/pkl/segm_per_v_overlap.pkl) and place it in `.../surreal/datageneration/smpl_data`
- get [SMPL_python_v.1.0.0](https://download.is.tue.mpg.de/download.php?domain=smpl&sfile=SMPL_python_v.1.0.0.zip). Extract the basicModel\_[m,f]\_lbs\_10\_207\_0\_v1.0.0.pkl. Place those two files in `.../surreal/datageneration/smpl_data/smpl/models/basicModel_{f,m}_lbs_10_207_0_v1.0.0.pkl`. Run `mv basicmodel_m_lbs_10_207_0_v1.0.0.pkl basicModel_m_lbs_10_207_0_v1.0.0.pkl`
- `cp .../surreal/datageneration/misc/prepare_smpl_data/extract_J_regressors.py .../surreal/datageneration/smpl_data/smpl/`
- run `python3 extract_J_regressor.py`
## Surreal Textures
- Accept surreal terms and get an account (you will need username and password to download textures)
- get the download script https://github.com/gulvarol/surreal/blob/master/download/download_smpl_data.sh and place it somewhere you like
let's call this location "loc"
- download this file https://github.com/gulvarol/surreal/blob/master/download/files/files_smpl_data.txt
and place it "loc/files/files_smpl_data.txt"(alongside the fbx models)
essentially you have ./loc/{script,files/files_smpl_data.txt}
- call the download script with `./download_smpl_data.sh /yourpath/surreal/datageneration/smpl_data username_surreal pw_surreal`
_____
At this point you should have
smpl_data/basicModel_{f,m}_lbs_10_207_0_v1.0.2.fbx
smpl_data/smpl/models/basicModel_{f,m}_lbs_10_207_0_v1.0.0.pkl
smpl_data/segm_per_v_overlap.pkl
smpl_data/joint_regressors.pkl
_____
## For AMASS
- create a `body_models` folder in `data_folder`
- create inside `smplh` and `dmpls` folders
- download [dmpls](https://download.is.tue.mpg.de/download.php?domain=smpl&sfile=dmpls.tar.xz) (DMPLs compatibile with SMPL) and [smplh](https://mano.is.tue.mpg.de/download.php) and get `Extended SMPLH model for AMASS` (accepting the respective licenses) there.
NOTE:
If exporting WITH cache, the hand movement will be complete, if exporting WITHOUT cache it will not as the basic model for blendshapes is the SMPL model WITHOUT hand. It shouldn't be too difficult to adapt the code to your needs eventually.
TESTED ONLY WITH CMU DATA | 2,822 | Markdown | 56.612244 | 414 | 0.759036 |
eliabntt/animated_human_SMPL_to_USD/README.md | # Human animations to USD
## This repository is part of the [GRADE](https://eliabntt.github.io/GRADE-RR/home) project
### This was tested on Windows, using Omniverse suggested Drivers and CUDA version.
The goal of this code is to show how you can convert any SMPL-based animation to a USD-based animation.
The script is capable of managing mesh caches and skeletal animations. It can export point-sequence based animations and skeletal-based animations.
### Installation instructions
Install blender connector from the Omniverse launcher. This code was tested with versions 3.4.0-usd.101.0 (main branch). For the paper work we used 3.1.0-usd.100.1.10.
Some limitations of 3.1.0-usd.100.1.10:
- you might need to use the mesh cache modifier instead of the blendshape. There is a _minimal_ difference that arise when loading the animation in Omniverse's products.
- keep textures with absolute paths. You can replace them whenever you want afterwards with our tool [USD_text_replace](https://github.com/eliabntt/GRADE-RR/tree/main/scripts/process_paths)
Install the necessary *dependencies*. Locate the blender installation path and run `python.exe -m pip install ipdb pyquaternion scipy torch pyyaml chumpy`.
e.g. In my case `C:\User\ebonetto\AppData\Local\ov\pkg\blender-3.4.0-usd.101.0\Release\3.4\python\bin\python.exe -m pip install ipdb pyquaternion scipy torch pyyaml chumpy`
Additionally, you need to follow [this]() to fill up the installation missing files that we cannot redistribute because of licensing.
### Already Supported datasets and HowTo expand
We are already supporting two datasets. [Cloth3D](https://chalearnlap.cvc.uab.cat/dataset/38/description/) and [AMASS](https://amass.is.tue.mpg.de/).
If you want to add a different dataset for AMASS you need to add it to the `data_folder/taxonomy.json` file
### Run the code
*From the cloned repository main folder*
`\AppData\Local\ov\pkg\blender-3.4.0-usd.101.0\Release\blender.exe --python-use-system-env --python-exit-code 0 --python start_blend_debug.py -- generate_sequence.py --dataset ... --output_dir ... --samples_dir ... --last_sample ... --parent_path ... --sample_id ...`
The parameters are explained in the code or self-explaining.
`dataset` can be either `[cloth3d, amass]`. With `amass` a necessary configuration file needs to be included (e.g. `--config_file this_repo\humangenerator\amass.json`). We provide a sample config [here](https://github.com/eliabntt/generate_people/blob/main/humangenerator/amass.json).
Note that AMASS will process the folder directly (by querying subfolders) differently than Cloth3D for which you need to give the main parent folder (eg. `cloth3d/train_t1`).
`sample_id` if is an ID it will process that ID otherwise you can set it to all or leave it empty and it will process the whole set of data.
`last_sample` is used in case `sample_id` is empty and will be used to signal where to restart the processing.
If running multiple generations the code will automatically periodically _clean_ the whole simulation environment including textures and materials to avoid crashing.
- Cloth3D single sample example `--python-use-system-env --python-exit-code 0 --python start_blend_debug.py -- generate_sequence.py --dataset cloth3d --output_dir outdir --samples_dir cloth3d\train --last_sample 01056 --parent_path D:\generate_people\data_folder\ --sample_id 01056`
- AMASS `--python-use-system-env --python-exit-code 0 --python start_blend_debug.py -- generate_sequence.py --dataset amass --output_dir D:\cloth3d\exported_usd --samples_dir D:\AMASS\CMU\ --parent_path D:\Cloth3D_to_usd\data_folder\ --config_file D:\Cloth3D_to_usd\humangenerator\amass.json`
### How does it work
The texture of the person is random. In the Cloth3D case the chosen ones are the ones with underwears, with AMASS the ones with clothes.
You have the possibility of exporting the SMPL information, the vertex info, the USD file, the STL trace of the animation and much more.
You can also suppress the output from the shell. However, the exporter in USD forcibly write directly to stdout. I have found no redirect strategy that works.
The system will replicate the input folder structure in the output folder.
You can also select a single frame.
You are encouraged to extend this and create pull requests.
Cloth3D clothes are loaded and exported as MeshCaches.
For the human animations you can chose.
### How to edit
You can create your own processor by creating a new class [here](https://github.com/eliabntt/generate_people/tree/main/humangenerator), adding your dataset name [here](https://github.com/eliabntt/generate_people/blob/main/humangenerator/avail_datasets.yaml) and write the else [here](https://github.com/eliabntt/generate_people/blob/main/humangenerator/generator.py#L17).
In practice you need to write your own python `dataset_gen.py`.
That file needs to have a `process_sample` method which will be then called by the main script.
Within `process_sample` you want to take care either of the sample (CLOTH3D) or of the whole folder (AMASS). Your choice.
We see the processing from the loading of the animation to writing data.
In the main script then there is a call to `get_processor` that returns `processor, PATH_SAMPLES`, `processor` is the instance of the class you just created.
Few lines below you find `res = processor.process_sample(sample, frame, smpl_body_list)`.
### Some notes
The exported USDs will have 24 fps as default. We did not investigate this much. You can change this by using the usd converter to text and change the 4th line to 30 fps. This value will influence how the mesh will be loaded into the simulation by the scripts used in GRADE.
In our work we did NOT change this value.
_______
### LICENSING
For licensing information, please refer to the main repository located [here](https://github.com/eliabntt/GRADE-RR/).
__________
### CITATION
If you find this work useful please cite our work based on [this](https://github.com/eliabntt/GRADE-RR#citation) information
__________
### Acknowledgment
Code based on
- [blenderproc](https://github.com/DLR-RM/BlenderProc/)
- [amass](https://amass.is.tue.mpg.de/)
- [Cloth3D starter kit](http://158.109.8.102/CLOTH3D/StarterKit.zip)
- [surreact](https://github.com/gulvarol/surreact) and [surreal](https://github.com/gulvarol/surreal)
| 6,354 | Markdown | 58.95283 | 371 | 0.765974 |
eliabntt/animated_human_SMPL_to_USD/LICENSE.md | For licensing information please refer to the main repository of the project located [here](https://github.com/eliabntt/GRADE-RR/). The same terms and conditions apply.
| 169 | Markdown | 83.999958 | 168 | 0.798817 |
eliabntt/animated_human_SMPL_to_USD/data_folder/smpl/smpl_np.py | import sys
import numpy as np
import pickle
class SMPLModel():
def __init__(self, model_path):
"""
SMPL model.
Parameter:
---------
model_path: Path to the SMPL model parameters, pre-processed by
`preprocess.py`.
"""
with open(model_path, 'rb') as f:
if sys.version_info[0] == 2:
params = pickle.load(f) # Python 2.x
elif sys.version_info[0] == 3:
params = pickle.load(f, encoding='latin1') # Python 3.x
self.J_regressor = params['J_regressor']
self.weights = params['weights']
self.posedirs = params['posedirs']
self.v_template = params['v_template']
self.shapedirs = params['shapedirs']
self.faces = params['f']
self.kintree_table = params['kintree_table']
id_to_col = {
self.kintree_table[1, i]: i for i in range(self.kintree_table.shape[1])
}
self.parent = {
i: id_to_col[self.kintree_table[0, i]]
for i in range(1, self.kintree_table.shape[1])
}
self.pose_shape = [24, 3]
self.beta_shape = [10]
self.trans_shape = [3]
self.pose = np.zeros(self.pose_shape)
self.beta = np.zeros(self.beta_shape)
self.trans = np.zeros(self.trans_shape)
self.verts = None
self.J = None
self.R = None
self.update()
def set_params(self, pose=None, beta=None, trans=None):
"""
Set pose, shape, and/or translation parameters of SMPL model. Verices of the
model will be updated and returned.
Parameters:
---------
pose: Also known as 'theta', a [24,3] matrix indicating child joint rotation
relative to parent joint. For root joint it's global orientation.
Represented in a axis-angle format.
beta: Parameter for model shape. A vector of shape [10]. Coefficients for
PCA component. Only 10 components were released by MPI.
trans: Global translation of shape [3].
Return:
------
Updated vertices.
"""
if pose is not None:
self.pose = pose
if beta is not None:
self.beta = beta
if trans is not None:
self.trans = trans
self.update()
return self.verts, self.J
def update(self):
"""
Called automatically when parameters are updated.
"""
# how beta affect body shape
v_shaped = self.shapedirs.dot(self.beta) + self.v_template
# joints location
self.J = self.J_regressor.dot(v_shaped)
pose_cube = self.pose.reshape((-1, 1, 3))
# rotation matrix for each joint
self.R = self.rodrigues(pose_cube)
I_cube = np.broadcast_to(
np.expand_dims(np.eye(3), axis=0),
(self.R.shape[0]-1, 3, 3)
)
lrotmin = (self.R[1:] - I_cube).ravel()
# how pose affect body shape in zero pose
v_posed = v_shaped + self.posedirs.dot(lrotmin)
# world transformation of each joint
G = np.empty((self.kintree_table.shape[1], 4, 4))
G[0] = self.with_zeros(np.hstack((self.R[0], self.J[0, :].reshape([3, 1]))))
for i in range(1, self.kintree_table.shape[1]):
G[i] = G[self.parent[i]].dot(
self.with_zeros(
np.hstack(
[self.R[i],((self.J[i, :]-self.J[self.parent[i],:]).reshape([3,1]))]
)
)
)
G = G - self.pack(
np.matmul(
G,
np.hstack([self.J, np.zeros([24, 1])]).reshape([24, 4, 1])
)
)
# transformation of each vertex
T = np.tensordot(self.weights, G, axes=[[1], [0]])
rest_shape_h = np.hstack((v_posed, np.ones([v_posed.shape[0], 1])))
v = np.matmul(T, rest_shape_h.reshape([-1, 4, 1])).reshape([-1, 4])[:, :3]
self.verts = v + self.trans.reshape([1, 3])
def rodrigues(self, r):
"""
Rodrigues' rotation formula that turns axis-angle vector into rotation
matrix in a batch-ed manner.
Parameter:
----------
r: Axis-angle rotation vector of shape [batch_size, 1, 3].
Return:
-------
Rotation matrix of shape [batch_size, 3, 3].
"""
theta = np.linalg.norm(r, axis=(1, 2), keepdims=True)
# avoid zero divide
theta = np.maximum(theta, np.finfo(np.float64).tiny)
r_hat = r / theta
cos = np.cos(theta)
z_stick = np.zeros(theta.shape[0])
m = np.dstack([
z_stick, -r_hat[:, 0, 2], r_hat[:, 0, 1],
r_hat[:, 0, 2], z_stick, -r_hat[:, 0, 0],
-r_hat[:, 0, 1], r_hat[:, 0, 0], z_stick]
).reshape([-1, 3, 3])
i_cube = np.broadcast_to(
np.expand_dims(np.eye(3), axis=0),
[theta.shape[0], 3, 3]
)
A = np.transpose(r_hat, axes=[0, 2, 1])
B = r_hat
dot = np.matmul(A, B)
R = cos * i_cube + (1 - cos) * dot + np.sin(theta) * m
return R
def with_zeros(self, x):
"""
Append a [0, 0, 0, 1] vector to a [3, 4] matrix.
Parameter:
---------
x: Matrix to be appended.
Return:
------
Matrix after appending of shape [4,4]
"""
return np.vstack((x, np.array([[0.0, 0.0, 0.0, 1.0]])))
def pack(self, x):
"""
Append zero matrices of shape [4, 3] to vectors of [4, 1] shape in a batched
manner.
Parameter:
----------
x: Matrices to be appended of shape [batch_size, 4, 1]
Return:
------
Matrix of shape [batch_size, 4, 4] after appending.
"""
return np.dstack((np.zeros((x.shape[0], 4, 3)), x)) | 5,242 | Python | 27.037433 | 80 | 0.571347 |
eliabntt/animated_human_SMPL_to_USD/humangenerator/cloth3d_gen.py | from humangenerator.util.blender_util import *
import bpy
from .util.cloth3d_util import loadInfo, bodyCache, loadGarment
import humangenerator as hgen
from pathlib import Path
class cloth3d:
def __init__(self, parent_path, with_cache, path_out, path_samples, smpl_models, write_verts):
from humangenerator.generator import generator
# temporary usd export path, we cannot directly write in mounted network drives sometimes
temp_path = os.path.join(parent_path, 'usd_exports')
# surreal path for textures
smpl_path = os.path.join(parent_path, "surreal", "datageneration", "smpl_data")
self.generator = generator(smpl_path)
self.with_cache = with_cache
self.path_out = path_out
self.path_samples = path_samples
self.smpl = smpl_models
self.temp_path = temp_path
self.write_verts = (write_verts == "True")
def animateSMPL(self, sample, smpl_ob, info, j):
if self.with_cache:
bodyCache(self.path_cache, sample, info, smpl_ob.ob, self.smpl)
# generate blendshapes + trans
s = info['shape']
smpl_ob.reset_joint_positions(s, bpy.data.scenes["Scene"])
if len(info['poses'].shape) > 1:
N = info['poses'].shape[1]
else:
sys.stderr.write('Error animation is ONLY ONE FRAME \n')
N = 1
for i in range(N):
if N > 1:
p = info['poses'][:, i]
t = info['trans'][:, i].reshape((3,)) - j[0]
else:
p = info['poses'][:]
t = info['trans'][:].reshape((3,)) - j[0]
bpy.data.scenes["Scene"].frame_set(i)
smpl_ob.apply_trans_pose_shape(t, p, s, i, with_blendshapes=not self.with_cache)
def generate_SMPLbody_animation(self, sample, info, gender, index):
print("Generate Animation..")
if len(info['poses'].shape) > 1:
p = info['poses'][:, 0].reshape((24, 3))
t = info['trans'][:, 0].reshape((3,))
else:
p = info['poses'][:].reshape((24, 3))
t = info['trans'][:].reshape((3,))
s = info['shape']
v, j = self.smpl[gender].set_params(pose=p, beta=s, trans=t)
cloth_img_name = self.generator.pick_skin_texture(gender=gender, clothing_option="grey")
img = bpy.data.materials[f'Material_{index}'].node_tree.nodes["Image Texture"]
img.image = bpy.data.images.load(cloth_img_name)
material = bpy.data.materials[f'Material_{index}']
self.smpl_body_list[index].refine_SMPL(material, j, info['zrot'])
self.animateSMPL(sample, self.smpl_body_list[index], info, j)
# Smooth
bpy.ops.object.shade_smooth()
def loadCloth3DSequence(self, sample: str, info: dict, frame: int = None):
if len(info['poses'].shape) > 1:
bpy.context.scene.frame_end = info['poses'].shape[-1] - 1
else:
bpy.context.scene.frame_end = 1
bpy.ops.object.select_all(action='DESELECT')
# delete current garments
for obj in bpy.data.objects.values():
if 'body' not in obj.name.lower() and 'armature' not in obj.name.lower():
obj.select_set(True)
bpy.ops.object.delete()
# Load new garments
for garment in info['outfit']:
loadGarment(self.path_samples, self.path_cache, sample, garment, info)
for obj in bpy.data.objects.values():
obj.select_set(False)
gender = 'm' if info['gender'] else 'f'
index = 0 if info['gender'] else 1
self.generate_SMPLbody_animation(sample, info, gender, index)
bpy.context.view_layer.objects.active = bpy.data.objects[f'Armature_{index}']
arm_obj = bpy.data.objects[f'Armature_{index}']
bpy.context.scene.frame_current = bpy.context.scene.frame_start
for obj in bpy.data.objects.values():
if 'body' not in obj.name.lower() and 'armature' not in obj.name.lower():
obj.select_set(True)
obj.parent = arm_obj
obj.rotation_euler = [0, 0, 0]
obj.select_set(False)
for obj in bpy.data.objects.values():
if 'armature' not in obj.name.lower() and 'body' not in obj.name.lower():
obj.select_set(True)
else:
if str(index) in obj.name:
obj.select_set(True)
if frame != None and frame >= 0 and frame <= bpy.context.scene.frame_end:
bpy.context.scene.frame_current = frame
def process_sample(self, sample: str, frame: int, smpl_body_list):
# load info
info = loadInfo(os.path.join(self.path_samples, sample, 'info.mat'))
self.smpl_body_list = smpl_body_list
subfolder_name = Path(sample).stem + ('_with_cache' if self.with_cache else '')
self.path_cache = hgen.create_outfolder_structure(self.path_out, subfolder_name, self.with_cache)
if frame is None:
self.loadCloth3DSequence(sample, info)
else:
self.loadCloth3DSequence(sample, info, frame)
bpy.ops.wm.save_as_mainfile(filepath=os.path.join(self.path_out, subfolder_name, subfolder_name + ".blend"))
return hgen.export_data(self.temp_path, self.path_out, Path(sample).stem, self.with_cache, frame, info, info['zrot'], self.write_verts) | 5,429 | Python | 42.095238 | 143 | 0.589796 |
eliabntt/animated_human_SMPL_to_USD/humangenerator/__init__.py | import os
import sys
# check the python version, only python 3.X is allowed:
if sys.version_info.major < 3:
raise Exception("HumanGenerator requires at least python 3.X to run.")
sys.path.remove(os.path.abspath(os.path.join(os.path.dirname(__file__), "..")))
from .util.blender_util import *
from data_folder.smpl.smpl_np import SMPLModel
from .generator import *
| 370 | Python | 29.916664 | 79 | 0.737838 |
eliabntt/animated_human_SMPL_to_USD/humangenerator/generator.py | import os
from random import choice
import bpy
from .util.smplutils import SMPL_Body, rotate_vector
from .cloth3d_gen import *
from .amass_gen import *
from .util.blender_util import export_stl_data, write_pkl_data, write_usd
# import amass_gen
def get_processor(dataset, parent_path, with_cache, path_out, path_samples, smpl_models, write_verts, config={}):
if dataset == "cloth3d":
return cloth3d(parent_path, with_cache, path_out, path_samples, smpl_models, write_verts), path_samples
if dataset == "amass": # todo fixme
tmp_obj = amass(parent_path, with_cache, path_out, path_samples, smpl_models, write_verts, config)
return tmp_obj, path_samples
raise Exception("NOT A VALID DATASET")
def export_data(temp_path, path_out, sample, with_cache, frame, info, orient, write_verts, usd=True):
try:
if usd:
write_usd(temp_path, path_out, sample + ('_with_cache' if with_cache else ''), with_cache,
True if frame == None else False, 0 if frame == None else frame)
for obj in bpy.data.objects.values():
if "body" in obj.name.lower() and obj.select_get():
ob = obj
elif "armature" in obj.name.lower() and obj.select_get():
arm_ob = obj
export_stl_data(path_out, sample + ('_with_cache' if with_cache else ''),
[ob for ob in bpy.data.objects if ob.select_get()], orient)
write_pkl_data(path_out, sample + ('_with_cache' if with_cache else ''), arm_ob, ob, info, write_verts=write_verts)
except:
return False
return True
def create_outfolder_structure(path_out, subfolder_name, with_cache):
if (with_cache):
path_cache = os.path.join(path_out, subfolder_name, 'view_cache')
if not os.path.exists(path_cache):
os.makedirs(path_cache)
else:
path_cache = os.path.join(path_out, subfolder_name, 'view_cache')
if not os.path.exists(path_cache):
os.makedirs(path_cache)
return path_cache
class generator:
def __init__(self, smpl_path, write_verts=False):
self.SMPL_PATH = smpl_path
def pick_skin_texture(self, split_name='all', clothing_option="grey", gender="m"):
if gender == "f":
with open(
os.path.join(self.SMPL_PATH, "textures", "female_{}.txt".format(split_name))
) as f:
txt_paths = f.read().splitlines()
else:
with open(
os.path.join(self.SMPL_PATH, "textures", "male_{}.txt".format(split_name))
) as f:
txt_paths = f.read().splitlines()
# if using only one source of clothing
if clothing_option == "nongrey":
txt_paths = [k for k in txt_paths if "nongrey" in k]
elif clothing_option == "grey":
txt_paths = [k for k in txt_paths if "nongrey" not in k]
elif clothing_option == "same":
# Orig
txt_paths = ["textures/male/nongrey_male_0244.jpg"]
elif clothing_option == "all":
txt_paths = [k for k in txt_paths]
# random clothing texture
cloth_img_name = choice(txt_paths)
cloth_img_name = os.path.join(self.SMPL_PATH, cloth_img_name)
print("Picked skin texture: {}".format(cloth_img_name))
return cloth_img_name
def create_material_SMPL(self, gender="m", person_no=0, clothing_option="grey", split_name="all"):
print("Creating SMPL texture material")
cloth_img_name = self.pick_skin_texture(split_name, clothing_option, gender)
material = bpy.data.materials.new(name=f"Material_{person_no}")
material.use_nodes = True
# Add nodes
tree = material.node_tree
nodes = tree.nodes
# Principled BSDf
bsdf = nodes['Principled BSDF']
# Image
img = nodes.new('ShaderNodeTexImage')
img.image = bpy.data.images.load(cloth_img_name)
# Links
tree.links.new(img.outputs[0], bsdf.inputs[0])
return material
def load_SMPLs_objects(self):
# create the material for SMPL
material = self.create_material_SMPL("m", 0)
print("Male Material Created")
smpl_body_list = []
# create the SMPL_Body object
smpl_body_list.append(
SMPL_Body(self.SMPL_PATH, material, 0, "male", person_no=0)
)
print("Male created")
material = self.create_material_SMPL("f", 1)
print("Female material created")
smpl_body_list.append(
SMPL_Body(self.SMPL_PATH, material, 0, "female", person_no=1)
)
print("Female created")
return smpl_body_list
| 4,735 | Python | 38.140496 | 123 | 0.597043 |
eliabntt/animated_human_SMPL_to_USD/humangenerator/amass_gen.py | from pathlib import Path
from humangenerator.util.blender_util import *
import bpy
from .util.amass_util import loadInfo, bodyCache, _load_parametric_body_model, _get_supported_mocap_datasets, \
_get_sequence_path
import humangenerator as hgen
class amass:
def __init__(self, parent_path, with_cache, path_out, path_samples, smpl_models, write_verts, config):
# temporary usd export path, we cannot directly write in mounted network drives sometimes
temp_path = os.path.join(parent_path, 'usd_exports')
# surreal path for textures
smpl_path = os.path.join(parent_path, "surreal", "datageneration", "smpl_data")
from humangenerator.generator import generator
self.generator = generator(smpl_path)
self.with_cache = with_cache
self.path_out = path_out
self.path_samples = path_samples
self.smpl = smpl_models
self.sub_dataset_id = config['sub_dataset_id']
self.num_betas = config['num_betas']
self.num_dmpls = config['num_dmpls']
self.subject_ids = config['subject_ids'].split()
self.write_verts = (write_verts == "True")
self.temp_path = temp_path
self.body_model_m, self.faces_m = _load_parametric_body_model(parent_path, "male", self.num_betas,
self.num_dmpls)
self.body_model_f, self.faces_f = _load_parametric_body_model(parent_path, "female", self.num_betas,
self.num_dmpls)
taxonomy_file_path = os.path.join(parent_path, "taxonomy.json")
self.supported_datasets = _get_supported_mocap_datasets(taxonomy_file_path, path_samples)
def animateSMPL(self, sample, smpl_ob, info, body_model):
if self.with_cache:
bodyCache(self.path_cache, sample, info, smpl_ob.ob, body_model, self.num_betas, self.num_dmpls)
# generate blendshapes + trans
s = info['betas'][:10]
smpl_ob.reset_joint_positions(s, bpy.data.scenes["Scene"])
for i in range(info['poses'].shape[0]):
p = np.append(info['poses'][i][:66].reshape(-1, 3), [[0, 0, 0], [0, 0, 0]], 0)
t = info['trans'][i].reshape((3,))
bpy.data.scenes["Scene"].frame_set(i)
smpl_ob.apply_trans_pose_shape(t, p, s, i, with_blendshapes=not self.with_cache)
def generate_SMPLbody_animation(self, sample, info, gender, index, body_model):
print("Generate Animation..")
orient = info['poses'][0, :3][2]
p = np.append(info['poses'][0][:66].reshape(-1, 3), [[0, 0, 0], [0, 0, 0]], 0)
t = info['trans'][0].reshape((3,))
s = info['betas'][:10]
v, j = self.smpl[gender].set_params(pose=p, beta=s, trans=t)
cloth_img_name = self.generator.pick_skin_texture(gender=gender, clothing_option="all")
img = bpy.data.materials[f'Material_{index}'].node_tree.nodes["Image Texture"]
img.image = bpy.data.images.load(cloth_img_name)
material = bpy.data.materials[f'Material_{index}']
self.smpl_body_list[index].refine_SMPL(material, j, orient) # info['zrot']
self.animateSMPL(sample, self.smpl_body_list[index], info, body_model)
# Smooth
bpy.ops.object.shade_smooth()
def loadAmassSequence(self, sample: str, info: dict, body_model, frame: int = None):
bpy.context.scene.frame_end = info['poses'].shape[0] - 1
bpy.ops.object.select_all(action='DESELECT')
# delete current garments
for obj in bpy.data.objects.values():
if 'body' not in obj.name.lower() and 'armature' not in obj.name.lower():
obj.select_set(True)
bpy.ops.object.delete()
for obj in bpy.data.objects.values():
obj.select_set(False)
gender = 'm' if info['gender'] == 'male' else 'f'
index = 0 if info['gender'] == 'male' else 1
self.generate_SMPLbody_animation(sample, info, gender, index, body_model)
bpy.context.view_layer.objects.active = bpy.data.objects[f'Armature_{index}']
arm_obj = bpy.data.objects[f'Armature_{index}']
bpy.context.scene.frame_current = bpy.context.scene.frame_start
for obj in bpy.data.objects.values():
if 'body' not in obj.name.lower() and 'armature' not in obj.name.lower():
obj.select_set(True)
obj.parent = arm_obj
obj.rotation_euler = [0, 0, 0]
obj.select_set(False)
for obj in bpy.data.objects.values():
if 'armature' not in obj.name.lower() and 'body' not in obj.name.lower():
obj.select_set(True)
else:
if str(index) in obj.name:
obj.select_set(True)
if frame != None and frame >= 0 and frame <= bpy.context.scene.frame_end:
bpy.context.scene.frame_current = frame
def process_sample(self, sample: str, frame: int, smpl_body_list):
# load info
if sample in self.subject_ids:
for subject_id in os.listdir(os.path.join(self.path_samples, sample)):
sequence_path, main_path = _get_sequence_path(self.supported_datasets, self.sub_dataset_id, sample,
subject_id)
info = loadInfo(sequence_path)
self.smpl_body_list = smpl_body_list
subfolder_name = Path(subject_id).stem + ('_with_cache' if self.with_cache else '')
self.path_cache = hgen.create_outfolder_structure(self.path_out, subfolder_name, self.with_cache)
if frame is None:
self.loadAmassSequence(sample, info, self.body_model_m if info["gender"] == "male" else self.body_model_f)
else:
self.loadAmassSequence(sample, info, self.body_model_m if info["gender"] == "male" else self.body_model_f,
frame)
bpy.ops.wm.save_as_mainfile(filepath=os.path.join(self.path_out, subfolder_name, subfolder_name + ".blend"))
my_l = list(info.keys())
new_info = {}
for i in my_l:
new_info[i] = info[i]
hgen.export_data(self.temp_path, self.path_out, Path(subject_id).stem, self.with_cache, frame, new_info,
info['poses'][0, :3][2], self.write_verts)
return True | 6,545 | Python | 47.488889 | 126 | 0.579221 |
eliabntt/animated_human_SMPL_to_USD/humangenerator/util/amass_util.py | import numpy as np
import glob
import os
import random
from .IO import readPC2, writePC2
import bpy, sys, torch
from .blender_util import mesh_cache
from typing import Tuple
def bodyCache(path_cache, sample, info, ob, body_model, num_betas, num_dmpls):
print("Processing Body Cache")
pc2_path = os.path.join(path_cache, sample + '.pc2')
V = np.zeros((info['poses'].shape[1], 6890, 3), np.float32)
bdata = info
time_length = len(bdata['trans'])
comp_device = torch.device("cuda" if torch.cuda.is_available() else "cpu")
body_params = {
'root_orient': torch.Tensor(bdata['poses'][:, :3]).to(comp_device), # controls the global root orientation
'pose_body': torch.Tensor(bdata['poses'][:, 3:66]).to(comp_device), # controls the body
'pose_hand': torch.Tensor(bdata['poses'][:, 66:]).to(comp_device), # controls the finger articulation
'trans': torch.Tensor(bdata['trans']).to(comp_device), # controls the global body position
'betas': torch.Tensor(np.repeat(bdata['betas'][:num_betas][np.newaxis], repeats=time_length, axis=0)).to(
comp_device), # controls the body shape. Body shape is static
'dmpls': torch.Tensor(bdata['dmpls'][:, :num_dmpls]).to(comp_device) # controls soft tissue dynamics
}
body_trans_root = body_model(
**{k: v for k, v in body_params.items() if k in ['pose_body', 'betas', 'pose_hand', 'dmpls',
'trans', 'root_orient']})
if not os.path.isfile(pc2_path):
V = body_trans_root.v.data.cpu().numpy()
print("Writing PC2 file...")
writePC2(pc2_path, V)
else:
V = readPC2(pc2_path)['V']
if V.shape[1] != len(ob.data.vertices):
sys.stderr.write("ERROR IN THE VERTEX COUNT FOR THE BODY!!!!!")
sys.stderr.flush()
mesh_cache(ob, pc2_path)
bpy.ops.object.shade_smooth()
return body_trans_root
def loadInfo(sequence_path):
if os.path.exists(sequence_path):
# load AMASS dataset sequence file which contains the coefficients for the whole motion sequence
sequence_body_data = np.load(sequence_path)
# get the number of supported frames
return sequence_body_data
else:
raise Exception(
"Invalid sequence/subject category identifiers, please choose a "
"valid one. Used path: {}".format(sequence_path))
def _get_sequence_path(supported_mocap_datasets: dict, used_sub_dataset_id: str, used_subject_id: str, used_sequence_id: str) -> [str, str]:
""" Extract pose and shape parameters corresponding to the requested pose from the database to be processed by the parametric model
:param supported_mocap_datasets: A dict which maps sub dataset names to their paths.
:param used_sub_dataset_id: Identifier for the sub dataset, the dataset which the human pose object should be extracted from.
:param used_subject_id: Type of motion from which the pose should be extracted, this is dataset dependent parameter.
:param used_sequence_id: Sequence id in the dataset, sequences are the motion recorded to represent certain action.
:return: tuple of arrays contains the parameters. Type: tuple
"""
# check if the sub_dataset is supported
if used_sub_dataset_id in supported_mocap_datasets:
# get path from dictionary
sub_dataset_path = supported_mocap_datasets[used_sub_dataset_id]
# concatenate path to specific
if not used_subject_id:
# if none was selected
possible_subject_ids = glob.glob(os.path.join(sub_dataset_path, "*"))
possible_subject_ids.sort()
if len(possible_subject_ids) > 0:
used_subject_id_str = os.path.basename(random.choice(possible_subject_ids))
else:
raise Exception("No subjects found in folder: {}".format(sub_dataset_path))
else:
try:
used_subject_id_str = "{:02d}".format(int(used_subject_id))
except:
used_subject_id_str = used_subject_id
subject_path = os.path.join(sub_dataset_path, used_subject_id_str)
sequence_path = os.path.join(subject_path, used_sequence_id)
return sequence_path, subject_path
else:
raise Exception(
"The requested mocap dataset is not yest supported, please choose anothe one from the following "
"supported datasets: {}".format([key for key, value in supported_mocap_datasets.items()]))
def _load_parametric_body_model(data_path: str, used_body_model_gender: str, num_betas: int,
num_dmpls: int) -> Tuple["BodyModel", np.array]:
""" loads the parametric model that is used to generate the mesh object
:return: parametric model. Type: tuple.
"""
import torch
from human_body_prior.body_model.body_model import BodyModel
bm_path = os.path.join(data_path, 'body_models', 'smplh', used_body_model_gender, 'model.npz') # body model
dmpl_path = os.path.join(data_path, 'body_models', 'dmpls', used_body_model_gender, 'model.npz') # deformation model
if not os.path.exists(bm_path) or not os.path.exists(dmpl_path):
raise Exception("Parametric Body model doesn't exist, please follow download instructions section in AMASS Example")
comp_device = torch.device("cuda" if torch.cuda.is_available() else "cpu")
body_model = BodyModel(bm_path=bm_path, num_betas=num_betas, num_dmpls=num_dmpls, path_dmpl=dmpl_path).to(comp_device)
faces = body_model.f.detach().cpu().numpy()
return body_model, faces
def _get_supported_mocap_datasets(taxonomy_file_path: str, data_path: str) -> dict:
""" get latest updated list from taxonomoy json file about the supported mocap datasets supported in the loader module and update.supported_mocap_datasets list
:param taxonomy_file_path: path to taxomomy.json file which contains the supported datasets and their respective paths. Type: string.
:param data_path: path to the AMASS dataset root folder. Type: string.
"""
import json
# dictionary contains mocap dataset name and path to its sub folder within the main dataset, dictionary will
# be filled from taxonomy.json file which indicates the supported datastests
supported_mocap_datasets = {}
if os.path.exists(taxonomy_file_path):
with open(taxonomy_file_path, "r") as f:
loaded_data = json.load(f)
for block in loaded_data:
if "sub_data_id" in block:
sub_dataset_id = block["sub_data_id"]
supported_mocap_datasets[sub_dataset_id] = os.path.join(data_path, block["path"])
else:
raise Exception("The taxonomy file could not be found: {}".format(taxonomy_file_path))
return supported_mocap_datasets | 6,996 | Python | 50.448529 | 163 | 0.646512 |
eliabntt/animated_human_SMPL_to_USD/humangenerator/util/cloth3d_util.py | import numpy as np
import scipy.io as sio
from math import cos, sin
from .blender_util import readOBJ, createBPYObj, setMaterial, mesh_cache, convert_meshcache
import os, sys
from .IO import readPC2, writePC2
import bpy
def loadInfo(path: str):
'''
this function should be called instead of direct sio.loadmat
as it cures the problem of not properly recovering python dictionaries
from mat files. It calls the function check keys to cure all entries
which are still mat-objects
'''
data = sio.loadmat(path, struct_as_record=False, squeeze_me=True)
del data['__globals__']
del data['__header__']
del data['__version__']
return _check_keys(data)
def _check_keys(dict):
'''
checks if entries in dictionary are mat-objects. If yes
todict is called to change them to nested dictionaries
'''
for key in dict:
if isinstance(dict[key], sio.matlab.mio5_params.mat_struct):
dict[key] = _todict(dict[key])
return dict
def _todict(matobj):
'''
A recursive function which constructs from matobjects nested dictionaries
'''
dict = {}
for strg in matobj._fieldnames:
elem = matobj.__dict__[strg]
if isinstance(elem, sio.matlab.mio5_params.mat_struct):
dict[strg] = _todict(elem)
elif isinstance(elem, np.ndarray) and np.any([isinstance(item, sio.matlab.mio5_params.mat_struct) for item in elem]):
dict[strg] = [None] * len(elem)
for i,item in enumerate(elem):
if isinstance(item, sio.matlab.mio5_params.mat_struct):
dict[strg][i] = _todict(item)
else:
dict[strg][i] = item
else:
dict[strg] = elem
return dict
# Computes matrix of rotation around z-axis for 'zrot' radians
def zRotMatrix(zrot):
c, s = cos(zrot), sin(zrot)
return np.array([[c, -s, 0],
[s, c, 0],
[0, 0, 1]], np.float32)
""" CAMERA """
def intrinsic():
RES_X = 640
RES_Y = 480
f_mm = 50 # blender default
sensor_w_mm = 36 # blender default
sensor_h_mm = sensor_w_mm * RES_Y / RES_X
fx_px = f_mm * RES_X / sensor_w_mm;
fy_px = f_mm * RES_Y / sensor_h_mm;
u = RES_X / 2;
v = RES_Y / 2;
return np.array([[fx_px, 0, u],
[0, fy_px, v],
[0, 0, 1]], np.float32)
def extrinsic(camLoc):
R_w2bc = np.array([[0, 1, 0],
[0, 0, 1],
[1, 0, 0]], np.float32)
T_w2bc = -1 * R_w2bc.dot(camLoc)
R_bc2cv = np.array([[1, 0, 0],
[0, -1, 0],
[0, 0, -1]], np.float32)
R_w2cv = R_bc2cv.dot(R_w2bc)
T_w2cv = R_bc2cv.dot(T_w2bc)
return np.concatenate((R_w2cv, T_w2cv[:,None]), axis=1)
def proj(camLoc):
return intrinsic().dot(extrinsic(camLoc))
"""
Mesh to UV map
Computes correspondences between 3D mesh and UV map
NOTE: 3D mesh vertices can have multiple correspondences with UV vertices
"""
def mesh2UV(F, Ft):
m2uv = {v: set() for f in F for v in f}
for f, ft in zip(F, Ft):
for v, vt in zip(f, ft):
m2uv[v].add(vt)
# m2uv = {k:list(v) for k,v in m2uv.items()}
return m2uv
# Maps UV coordinates to texture space (pixel)
IMG_SIZE = 2048 # all image textures have this squared size
def uv_to_pixel(vt):
px = vt * IMG_SIZE # scale to image plane
px %= IMG_SIZE # wrap to [0, IMG_SIZE]
# Note that Blender graphic engines invert vertical axis
return int(px[0]), int(IMG_SIZE - px[1]) # texel X, texel Y
def loadGarment(path_sample, path_cache, sample, garment, info):
print("Processing Garment Cache")
print(f"Loading {garment}")
texture = info['outfit'][garment]['texture']
# Read OBJ file and create BPY object
V, F, Vt, Ft = readOBJ(os.path.join(path_sample, sample, garment + '.obj'))
ob = createBPYObj(V, F, Vt, Ft, name=sample + '_' + garment)
# z-rot
ob.rotation_euler[2] = info['zrot']
# Convert cache PC16 to PC2
pc2_path = os.path.join(path_cache,
sample + '_' + garment + '.pc2'
)
if not os.path.isfile(pc2_path):
# Convert PC16 to PC2 (and move to view_cache folder)
# Add trans to vertex locations
pc16_path = os.path.join(path_sample, sample, garment + '.pc16')
V = readPC2(pc16_path, True)['V']
for i in range(V.shape[0]):
sys.stdout.write('\r' + str(i + 1) + '/' + str(V.shape[0]))
sys.stdout.flush()
if V.shape[0] > 1:
V[i] += info['trans'][:, i][None]
else:
V[i] += info['trans'][:][None]
writePC2(pc2_path, V)
else:
V = readPC2(pc2_path)['V']
if V.shape[1] != len(ob.data.vertices):
sys.stderr.write("ERROR IN THE VERTEX COUNT!!!!!")
sys.stderr.flush()
mesh_cache(ob, pc2_path)
# necessary to have this in the old version of the code with the old omni-blender
# convert_meshcache(bpy.ops.object)
# Set material
setMaterial(path_sample, ob, sample, garment, texture)
# Smooth
bpy.ops.object.shade_smooth()
print(f"\nLoaded {garment}.\n")
def bodyCache(path_cache, sample, info, ob, smpl):
print("Processing Body Cache")
pc2_path = os.path.join(path_cache, sample + '.pc2')
if not os.path.isfile(pc2_path):
# Compute body sequence
print("Computing body sequence...")
print("")
gender = 'm' if info['gender'] else 'f'
if len(info['poses'].shape)>1:
N = info['poses'].shape[1]
else:
N = 1
V = np.zeros((N, 6890, 3), np.float32)
for i in range(N):
sys.stdout.write('\r' + str(i + 1) + '/' + str(N))
sys.stdout.flush()
s = info['shape']
if N == 1:
p = info['poses'][:].reshape((24, 3))
t = info['trans'][:].reshape((3,))
else:
p = info['poses'][:, i].reshape((24, 3))
t = info['trans'][:, i].reshape((3,))
v, j = smpl[gender].set_params(pose=p, beta=s, trans=t)
V[i] = v - j[0:1]
print("")
print("Writing PC2 file...")
writePC2(pc2_path, V)
else:
V = readPC2(pc2_path)['V']
if V.shape[1] != len(ob.data.vertices):
sys.stderr.write("ERROR IN THE VERTEX COUNT FOR THE BODY!!!!!")
sys.stderr.flush()
mesh_cache(ob, pc2_path)
bpy.ops.object.shade_smooth() | 6,626 | Python | 32.469697 | 125 | 0.551313 |
eliabntt/animated_human_SMPL_to_USD/humangenerator/util/smplutils.py | import bpy
from bpy_extras.object_utils import world_to_camera_view
from mathutils import Matrix, Quaternion
import numpy as np
import pickle as pkl
import os
import math
from pyquaternion import Quaternion
# computes rotation matrix through Rodrigues formula as in cv2.Rodrigues
def Rodrigues(rotvec):
theta = np.linalg.norm(rotvec)
r = (rotvec / theta).reshape(3, 1) if theta > 0.0 else rotvec
cost = np.cos(theta)
mat = np.asarray([[0, -r[2], r[1]], [r[2], 0, -r[0]], [-r[1], r[0], 0]])
return cost * np.eye(3) + (1 - cost) * r.dot(r.T) + np.sin(theta) * mat
# transformation between pose and blendshapes
def rodrigues2bshapes(pose):
rod_rots = np.asarray(pose).reshape(24, 3)
mat_rots = [Rodrigues(rod_rot) for rod_rot in rod_rots]
bshapes = np.concatenate(
[(mat_rot - np.eye(3)).ravel() for mat_rot in mat_rots[1:]]
)
return mat_rots, bshapes
def rotate_vector(vector, axis, angle):
"""
Rotate a vector around an axis by an angle.
"""
q = Quaternion(axis=axis, angle=angle)
return q.rotate(vector)
class SMPL_Body:
def __init__(self, smpl_data_folder, material, j, gender="female", person_no=0, zrot=0):
# load fbx model
bpy.ops.import_scene.fbx(
filepath=os.path.join(
smpl_data_folder,
"basicModel_{}_lbs_10_207_0_v1.0.2.fbx".format(gender[0]),
),
axis_forward="Y",
axis_up="Z",
global_scale=100,
)
J_regressors = pkl.load(
open(os.path.join(smpl_data_folder, "joint_regressors.pkl"), "rb")
)
# 24 x 6890 regressor from vertices to joints
self.joint_regressor = J_regressors["J_regressor_{}".format(gender)]
self.j = j
armature_name = "Armature_{}".format(person_no)
bpy.context.active_object.name = armature_name
self.gender_name = "{}_avg".format(gender[0])
self.obj_name = "body_{:d}".format(person_no)
bpy.data.objects[armature_name].children[0].name = self.obj_name
# not the default self.gender_name because each time fbx is loaded it adds some suffix
self.ob = bpy.data.objects[self.obj_name]
# Rename the armature
self.ob.data.use_auto_smooth = False # autosmooth creates artifacts
# assign the existing spherical harmonics material
self.ob.active_material = bpy.data.materials["Material_{}".format(person_no)]
bpy.ops.object.origin_set(type='GEOMETRY_ORIGIN', center='MEDIAN')
# clear existing animation data
# self.ob.shape_key_clear()
self.ob.data.shape_keys.animation_data_clear()
self.arm_ob = bpy.data.objects[armature_name]
self.arm_ob.animation_data_clear()
self.setState0()
# self.ob.select = True # blender < 2.8x
self.ob.select_set(True)
# bpy.context.scene.objects.active = self.ob # blender < 2.8x
bpy.context.view_layer.objects.active = self.ob
self.smpl_data_folder = smpl_data_folder
self.materials = self.create_segmentation(material, smpl_data_folder)
# unblocking both the pose and the blendshape limits
for k in self.ob.data.shape_keys.key_blocks.keys():
self.ob.data.shape_keys.key_blocks[k].slider_min = -100
self.ob.data.shape_keys.key_blocks[k].slider_max = 100
# bpy.context.scene.objects.active = self.arm_ob # blender < 2.8x
bpy.context.view_layer.objects.active = self.arm_ob
# order
self.part_match = {
"root": "root",
"bone_00": "Pelvis",
"bone_01": "L_Hip",
"bone_02": "R_Hip",
"bone_03": "Spine1",
"bone_04": "L_Knee",
"bone_05": "R_Knee",
"bone_06": "Spine2",
"bone_07": "L_Ankle",
"bone_08": "R_Ankle",
"bone_09": "Spine3",
"bone_10": "L_Foot",
"bone_11": "R_Foot",
"bone_12": "Neck",
"bone_13": "L_Collar",
"bone_14": "R_Collar",
"bone_15": "Head",
"bone_16": "L_Shoulder",
"bone_17": "R_Shoulder",
"bone_18": "L_Elbow",
"bone_19": "R_Elbow",
"bone_20": "L_Wrist",
"bone_21": "R_Wrist",
"bone_22": "L_Hand",
"bone_23": "R_Hand",
}
def refine_SMPL(self, material, j, zrot):
self.j = j
self.arm_ob.rotation_euler = [0, 0, zrot]
self.ob.data.shape_keys.animation_data_clear()
self.arm_ob.animation_data_clear()
self.ob.select_set(True)
bpy.ops.object.origin_set(type='GEOMETRY_ORIGIN', center='MEDIAN')
# bpy.context.scene.objects.active = self.ob # blender < 2.8x
bpy.context.view_layer.objects.active = self.ob
self.materials = self.create_segmentation(material, self.smpl_data_folder)
for k in self.ob.data.shape_keys.key_blocks.keys():
self.ob.data.shape_keys.key_blocks[k].slider_min = -10
self.ob.data.shape_keys.key_blocks[k].slider_max = 10
# bpy.context.scene.objects.active = self.arm_ob # blender < 2.8x
bpy.context.view_layer.objects.active = self.arm_ob
def setState0(self):
for ob in bpy.data.objects.values():
# ob.select = False # blender < 2.8x
ob.select_set(False)
# bpy.context.scene.objects.active = None # blender < 2.8x
bpy.context.view_layer.objects.active = None
# create one material per part as defined in a pickle with the segmentation
# this is useful to render the segmentation in a material pass
def create_segmentation(self, material, smpl_path):
print("Creating materials segmentation")
sorted_parts = [
"hips",
"leftUpLeg",
"rightUpLeg",
"spine",
"leftLeg",
"rightLeg",
"spine1",
"leftFoot",
"rightFoot",
"spine2",
"leftToeBase",
"rightToeBase",
"neck",
"leftShoulder",
"rightShoulder",
"head",
"leftArm",
"rightArm",
"leftForeArm",
"rightForeArm",
"leftHand",
"rightHand",
"leftHandIndex1",
"rightHandIndex1",
]
part2num = {part: (ipart + 1) for ipart, part in enumerate(sorted_parts)}
materials = {}
vgroups = {}
with open(os.path.join(smpl_path,"segm_per_v_overlap.pkl"), "rb") as f:
vsegm = pkl.load(f)
if len(self.ob.material_slots) <= 1:
bpy.ops.object.material_slot_remove()
parts = sorted(vsegm.keys())
existing = False
cnt = 0
for part in parts:
vs = vsegm[part]
# vgroups[part] = self.ob.vertex_groups.new(part) # blender < 2.8x
if part not in self.ob.vertex_groups:
vgroups[part] = self.ob.vertex_groups.new(name=part)
vgroups[part].add(vs, 1.0, "ADD")
else:
existing = True
bpy.ops.object.vertex_group_set_active(group=part)
materials[part] = material.copy()
materials[part].pass_index = part2num[part]
if not existing:
bpy.ops.object.material_slot_add()
self.ob.material_slots[-1].material = materials[part]
bpy.ops.object.mode_set(mode="EDIT")
bpy.ops.mesh.select_all(action="DESELECT")
bpy.ops.object.vertex_group_select()
bpy.ops.object.material_slot_assign()
bpy.ops.object.mode_set(mode="OBJECT")
else:
self.ob.material_slots[cnt].material = materials[part]
cnt += 1
for scene_material in bpy.data.materials:
if not scene_material.users and len(scene_material.name) != len(material.name):
bpy.data.materials.remove(scene_material)
return materials
def quaternion_multiply(self, quaternion1, quaternion0):
w0, x0, y0, z0 = quaternion0
w1, x1, y1, z1 = quaternion1
return np.array([-x1 * x0 - y1 * y0 - z1 * z0 + w1 * w0,
x1 * w0 + y1 * z0 - z1 * y0 + w1 * x0,
-x1 * z0 + y1 * w0 + z1 * x0 + w1 * y0,
x1 * y0 - y1 * x0 + z1 * w0 + w1 * z0], dtype=np.float64)
def euler_from_quaternion(self, quat):
"""
Convert a quaternion into euler angles (roll, pitch, yaw)
roll is rotation around x in radians (counterclockwise)
pitch is rotation around y in radians (counterclockwise)
yaw is rotation around z in radians (counterclockwise)
"""
w,x,y,z = quat
t0 = +2.0 * (w * x + y * z)
t1 = +1.0 - 2.0 * (x * x + y * y)
roll_x = math.atan2(t0, t1)
t2 = +2.0 * (w * y - z * x)
t2 = +1.0 if t2 > +1.0 else t2
t2 = -1.0 if t2 < -1.0 else t2
pitch_y = math.asin(t2)
t3 = +2.0 * (w * z + x * y)
t4 = +1.0 - 2.0 * (y * y + z * z)
yaw_z = math.atan2(t3, t4)
return roll_x*180/3.1415, pitch_y*180/3.1415, yaw_z*180/3.1415 # in radians
def apply_trans_pose_shape(self, trans, pose, shape, frame=None, with_blendshapes = True):
"""
Apply trans pose and shape to character
"""
# transform pose into rotation matrices (for pose) and pose blendshapes
mrots, bsh = rodrigues2bshapes(pose)
# set the location of the first bone to the translation parameter
mytrans = [0,0,0]
mytrans[2] = trans[2]
mytrans[1] = trans[1]
mytrans[0] = trans[0]
self.arm_ob.pose.bones[self.gender_name + "_Pelvis"].location = mytrans
if frame is not None:
self.arm_ob.pose.bones[self.gender_name + "_root"].keyframe_insert(
"location", frame=frame
)
self.arm_ob.pose.bones[self.gender_name + "_root"].keyframe_insert(
"rotation_quaternion", frame=frame
)
# set the pose of each bone to the quaternion specified by pose
for ibone, mrot in enumerate(mrots):
bone = self.arm_ob.pose.bones[
self.gender_name + "_" + self.part_match["bone_{:02d}".format(ibone)]
]
bone.rotation_quaternion = Matrix(mrot).to_quaternion()
if frame is not None:
bone.keyframe_insert("rotation_quaternion", frame=frame)
bone.keyframe_insert("location", frame=frame)
# apply pose blendshapes
if with_blendshapes:
for ibshape, bshape in enumerate(bsh):
self.ob.data.shape_keys.key_blocks[
"Pose{:03d}".format(ibshape)
].value = bshape
if frame is not None:
self.ob.data.shape_keys.key_blocks[
"Pose{:03d}".format(ibshape)
].keyframe_insert("value", index=-1, frame=frame)
# apply shape blendshapes
for ibshape, shape_elem in enumerate(shape):
self.ob.data.shape_keys.key_blocks[
"Shape{:03d}".format(ibshape)
].value = shape_elem
if frame is not None:
self.ob.data.shape_keys.key_blocks[
"Shape{:03d}".format(ibshape)
].keyframe_insert("value", index=-1, frame=frame)
else:
mod = self.ob.modifiers.get('Armature')
if mod is not None: self.ob.modifiers.remove(mod)
def reset_joint_positions(self, shape, scene):
orig_trans = np.asarray(
self.arm_ob.pose.bones[self.gender_name + "_Pelvis"].location
).copy()
# zero the pose and trans to obtain joint positions in zero pose
self.apply_trans_pose_shape(orig_trans, np.zeros(72), shape)
bpy.ops.wm.memory_statistics()
depsgraph = bpy.context.evaluated_depsgraph_get()
me = self.ob.evaluated_get(depsgraph).to_mesh()
num_vertices = len(me.vertices) # 6890
reg_vs = np.empty((num_vertices, 3))
for iiv in range(num_vertices):
reg_vs[iiv] = me.vertices[iiv].co
# bpy.data.meshes.remove(me) # blender < 2.8x
self.ob.evaluated_get(depsgraph).to_mesh_clear()
# regress joint positions in rest pose
joint_xyz = self.j
# adapt joint positions in rest pose
# self.arm_ob.hide = False
# Added this line
# bpy.context.scene.objects.active = self.arm_ob # blender < 2.8x
bpy.context.view_layer.objects.active = self.arm_ob
bpy.ops.object.mode_set(mode="EDIT")
# self.arm_ob.hide = True
for ibone in range(24):
bb = self.arm_ob.data.edit_bones[
self.gender_name + "_" + self.part_match["bone_{:02d}".format(ibone)]
]
bboffset = bb.tail - bb.head
bb.head = joint_xyz[ibone]
bb.tail = bb.head + bboffset
bpy.ops.object.mode_set(mode="OBJECT") | 13,308 | Python | 37.915205 | 94 | 0.550646 |
eliabntt/animated_human_SMPL_to_USD/humangenerator/util/blender_util.py | import os
import bpy
from humangenerator.util.IO import readOBJ, readPC2, writePC2
import numpy as np
import bmesh
import sys
import pickle as pkl
import shutil
import random
PI = 3.14159
""" Scene """
def init():
clean()
# scene
return scene()
def clean():
for collection in dir(bpy.data):
data_structure = getattr(bpy.data, collection)
# Check that it is a data collection
if isinstance(data_structure, bpy.types.bpy_prop_collection) and hasattr(data_structure,
"remove") and collection not in [
"texts"]:
# Go over all entities in that collection
for block in data_structure:
# Remove everything besides the default scene
if not isinstance(block, bpy.types.Scene) or block.name != "Scene":
data_structure.remove(block)
def clean_mesh_and_textures(exclude=[]):
# ensure everything is lowered
exclude = [i.lower() for i in exclude]
for block in bpy.data.objects:
if block.users == 0 or block.name.lower() not in exclude:
bpy.data.objects.remove(block)
for block in bpy.data.meshes:
if block.users == 0:
bpy.data.meshes.remove(block)
for block in bpy.data.materials:
if block.users == 0 and block.name.lower() not in exclude:
bpy.data.materials.remove(block)
for block in bpy.data.textures:
if block.users == 0:
bpy.data.textures.remove(block)
for block in bpy.data.images:
bpy.data.images.remove(block)
for block in bpy.data.shape_keys:
if block.users == 0:
bpy.data.textures.remove(block)
for block in bpy.data.actions:
if block.users == 0:
bpy.data.actions.remove(block)
def scene():
scene = bpy.data.scenes["Scene"]
scene.render.engine = "CYCLES"
# bpy.data.materials['Material'].use_nodes = True
scene.cycles.shading_system = True
scene.use_nodes = True
scene.render.film_transparent = True
scene.frame_current = 0
scene.render.fps = 30
scene.render.resolution_x = 640
scene.render.resolution_y = 480
return scene
""" BPY obj manipulation """
def select(ob, only=True):
if type(ob) is str: ob = bpy.data.objects[ob]
if only: deselect()
ob.select_set(True)
bpy.context.view_layer.objects.active = ob
return ob
def deselect():
for obj in bpy.data.objects.values():
obj.select_set(False)
bpy.context.view_layer.objects.active = None
def delete(ob):
select(ob)
bpy.ops.object.delete()
def createBPYObj(V, F, Vt=None, Ft=None, name='new_obj'):
# Create obj
mesh = bpy.data.meshes.new('mesh')
ob = bpy.data.objects.new(name, mesh)
# Add to collection
bpy.context.collection.objects.link(ob)
select(ob)
mesh = bpy.context.object.data
bm = bmesh.new()
# Vertices
for v in V:
bm.verts.new(v)
bm.verts.ensure_lookup_table()
# Faces
for f in F:
v = [bm.verts[i] for i in f]
bm.faces.new(v)
bm.to_mesh(mesh)
bm.free()
# UV Map
if not Vt is None:
# Create UV layer
ob.data.uv_layers.new()
# Assign UV coords
iloop = 0
for f in Ft:
for i in f:
ob.data.uv_layers['UVMap'].data[iloop].uv = Vt[i]
iloop += 1
return ob
def convert_meshcache(ob: bpy.ops.object, offset=0):
# Converts a MeshCache or Cloth modifiers to ShapeKeys
bpy.context.scene.frame_current = bpy.context.scene.frame_start
for frame in range(bpy.context.scene.frame_end + 1):
bpy.context.scene.frame_current = frame
# for alembic files converted to PC2 and loaded as MeshCache
bpy.ops.object.modifier_apply_as_shapekey(keep_modifier=True, modifier="MeshCache")
# loop through shapekeys and add as keyframe per frame
# https://blender.stackexchange.com/q/149045/87258
bpy.context.scene.frame_current = bpy.context.scene.frame_start
for frame in range(bpy.context.scene.frame_end + 1):
bpy.context.scene.frame_current = frame
shapekey = bpy.data.shape_keys[-1]
for i, keyblock in enumerate(shapekey.key_blocks):
if keyblock.name != "Basis":
curr = i - 1
if curr != frame:
keyblock.value = 0
keyblock.keyframe_insert("value", frame=frame)
else:
keyblock.value = 1
keyblock.keyframe_insert("value", frame=frame)
bpy.ops.object.modifier_remove(modifier="MeshCache")
def setMaterial(path_sample, ob, sample, garment, texture):
mat = bpy.data.materials.new(name=sample + '_' + garment + '_Material')
mat.use_nodes = True
ob.data.materials.append(mat)
if texture['type'] == 'color':
mat.node_tree.nodes['Principled BSDF'].inputs[0].default_value = texture['data'].tolist() + [1]
elif texture['type'] == 'pattern':
# Read pattern
img_path = os.path.join(path_sample, sample, garment + '.png')
# Add nodes
tree = mat.node_tree
nodes = tree.nodes
# Principled BSDf
bsdf = nodes['Principled BSDF']
# Image
img = nodes.new('ShaderNodeTexImage')
try:
img.image = bpy.data.images.load(img_path)
# Links
tree.links.new(img.outputs[0], bsdf.inputs[0])
except:
mat.node_tree.nodes['Principled BSDF'].inputs[0].default_value = [random.random(), random.random(),
random.random(), 1]
""" Modifiers """
def mesh_cache(ob, cache, scale=1):
ob = select(ob)
bpy.ops.object.modifier_add(type='MESH_CACHE')
ob.modifiers['MeshCache'].cache_format = 'PC2'
ob.modifiers['MeshCache'].filepath = cache
ob.modifiers['MeshCache'].frame_scale = scale
def write_usd(temppath, filepath, filename, with_cache, export_animation=True, sf=0, ef=-1, frame_step=1):
outpath = os.path.join(filepath, filename)
filepath = os.path.join(filepath, filename, filename + ".usd")
if ef == -1:
ef = bpy.context.scene.frame_end
print(f"\nExporting usd to {filepath}\n")
print(f"With blendshapes = {not with_cache}")
bpy.ops.wm.usd_export(filepath=os.path.join(temppath, filename + ".usd"),
filemode=8, display_type='DEFAULT', sort_method='DEFAULT',
selected_objects_only=True, visible_objects_only=True, export_animation=export_animation,
export_hair=True, export_vertices=True, export_vertex_colors=True,
export_vertex_groups=True, export_face_maps=True, export_uvmaps=True, export_normals=True,
export_transforms=True, export_materials=True, export_meshes=True, export_lights=True,
export_cameras=False, export_blendshapes=(not with_cache),
export_curves=True, export_particles=True, export_armatures=True, use_instancing=False,
evaluation_mode='VIEWPORT', default_prim_path=f"/body_{filename}",
root_prim_path=f"/body_{filename}", material_prim_path=f"/body_{filename}/materials",
generate_cycles_shaders=False, generate_preview_surface=True, generate_mdl=True,
convert_uv_to_st=True, convert_orientation=True,
convert_to_cm=True, export_global_forward_selection='Y', export_global_up_selection='Z',
export_child_particles=False,
export_as_overs=False, merge_transform_and_shape=False, export_custom_properties=True,
add_properties_namespace=False, export_identity_transforms=False,
apply_subdiv=True, author_blender_name=True, vertex_data_as_face_varying=False,
frame_step=frame_step, start=sf, end=ef, override_shutter=False,
init_scene_frame_range=True, export_textures=True, relative_paths=True,
light_intensity_scale=1,
convert_light_to_nits=True, scale_light_radius=True, convert_world_material=True,
fix_skel_root=True, xform_op_mode='SRT')
shutil.move(os.path.join(temppath, filename + ".usd"), filepath)
shutil.move(os.path.join(temppath, "textures"), os.path.join(outpath, "textures"))
def export_stl_data(filepath, filename, lobs, zrot):
context = bpy.context
dg = context.evaluated_depsgraph_get()
scene = context.scene
coll = context.collection
step = 5
for ob in lobs:
if ob.type != 'MESH':
print(ob.name)
print(ob.type)
ob.select_set(False)
continue
bpy.context.view_layer.objects.active = ob
rings = []
me = ob.data
nverts = len(me.vertices)
nedges = len(me.edges)
bm = bmesh.new()
f = scene.frame_start
while f <= scene.frame_end:
scene.frame_set(f)
bm.from_object(ob, dg, cage=True)
bmesh.ops.remove_doubles(bm, verts=bm.verts, dist=0.02)
# bmesh.ops.transform(bm, verts=bm.verts[:], matrix=ob.matrix_world)
f += step
rings.append(bm.edges[:])
print("Frames processeds, going to do rings")
# build from rings
next = rings.pop()
while rings:
ring = rings.pop()
bmesh.ops.bridge_loops(bm, edges=ring + next)
next = ring
rme = bpy.data.meshes.new("Rib")
bm.to_mesh(rme)
copy = bpy.data.objects.new("Rib", rme)
coll.objects.link(copy)
print("DONE" + ob.name)
for ob in bpy.data.objects:
if 'Rib' in ob.name:
ob.select_set(True)
bpy.context.view_layer.objects.active = ob
else:
ob.select_set(False)
bpy.ops.object.join()
ob = bpy.context.view_layer.objects.active
ob.select_set(True)
ob.rotation_euler = [0, 0, zrot]
bpy.ops.export_mesh.stl(filepath=os.path.join(filepath, filename, filename + ".stl"), check_existing=True,
use_selection=True, global_scale=1, ascii=False, use_mesh_modifiers=False, batch_mode='OFF',
axis_forward='Y', axis_up='Z')
bpy.ops.object.delete()
def write_pkl_data(filepath, filename, arm_ob, ob, info, frame_step=1, write_verts=False):
bpy.context.scene.frame_current = bpy.context.scene.frame_start
N = int((bpy.context.scene.frame_end - bpy.context.scene.frame_start + 1) / frame_step)
n_bones = len(arm_ob.pose.bones) - 1
n_verts = len(ob.data.vertices)
if write_verts:
d = {
'frame': [],
'bones': np.zeros((N, n_bones, 3), np.float32),
'info': info,
'verts': np.zeros((N, n_verts, 3), np.float32),
'sf': bpy.context.scene.frame_start,
'ef': bpy.context.scene.frame_end + 1,
'nframes': frame_step
}
else:
d = {
'frame': [],
'bones': np.zeros((N, n_bones, 3), np.float32),
'info': info,
'sf': bpy.context.scene.frame_start,
'ef': bpy.context.scene.frame_end + 1,
'nframes': frame_step
}
select(ob)
dg = bpy.context.evaluated_depsgraph_get()
cnt = 0
for f in range(bpy.context.scene.frame_start, bpy.context.scene.frame_end + 1):
sys.stdout.write('\r' + str(f) + '/' + str(N * frame_step))
sys.stdout.flush()
bpy.context.scene.frame_current = f
bpy.context.view_layer.update()
d['frame'].append(f)
select(ob)
tmp = ob.evaluated_get(dg)
me = tmp.to_mesh()
if write_verts:
d['verts'][cnt] = np.reshape([ob.matrix_world @ v.co for v in me.vertices], (n_verts, 3))
select(arm_ob)
d['bones'][cnt] = np.reshape([arm_ob.matrix_world @ bone.head for bone in arm_ob.pose.bones[1:]], (n_bones, 3))
cnt += 1
if not os.path.exists(os.path.join(filepath, filename)):
os.makedirs(os.path.join(filepath, filename))
filepath = os.path.join(filepath, filename, filename + ".pkl")
out = open(filepath, 'wb')
pkl.dump(d, out)
out.close() | 12,339 | Python | 35.081871 | 120 | 0.593322 |
eliabntt/animated_human_SMPL_to_USD/humangenerator/util/IO.py | import os
import numpy as np
from struct import pack, unpack
"""
Reads OBJ files
Only handles vertices, faces and UV maps
Input:
- file: path to .obj file
Outputs:
- V: 3D vertices
- F: 3D faces
- Vt: UV vertices
- Ft: UV faces
Correspondence between mesh and UV map is implicit in F to Ft correspondences
If no UV map data in .obj file, it shall return Vt=None and Ft=None
"""
def readOBJ(file):
V, Vt, F, Ft = [], [], [], []
with open(file, 'r') as f:
T = f.readlines()
for t in T:
# 3D vertex
if t.startswith('v '):
v = [float(n) for n in t.replace('v ','').split(' ')]
V += [v]
# UV vertex
elif t.startswith('vt '):
v = [float(n) for n in t.replace('vt ','').split(' ')]
Vt += [v]
# Face
elif t.startswith('f '):
idx = [n.split('/') for n in t.replace('f ','').split(' ')]
f = [int(n[0]) - 1 for n in idx]
F += [f]
# UV face
if '/' in t:
f = [int(n[1]) - 1 for n in idx]
Ft += [f]
V = np.array(V, np.float32)
Vt = np.array(Vt, np.float32)
if Ft: assert len(F) == len(Ft), 'Inconsistent .obj file, mesh and UV map do not have the same number of faces'
else: Vt, Ft = None, None
return V, F, Vt, Ft
"""
Writes OBJ files
Only handles vertices, faces and UV maps
Inputs:
- file: path to .obj file (overwrites if exists)
- V: 3D vertices
- F: 3D faces
- Vt: UV vertices
- Ft: UV faces
Correspondence between mesh and UV map is implicit in F to Ft correspondences
If no UV map data as input, it will write only 3D data in .obj file
"""
def writeOBJ(file, V, F, Vt=None, Ft=None):
if not Vt is None:
assert len(F) == len(Ft), 'Inconsistent data, mesh and UV map do not have the same number of faces'
with open(file, 'w') as file:
# Vertices
for v in V:
line = 'v ' + ' '.join([str(_) for _ in v]) + '\n'
file.write(line)
# UV verts
if not Vt is None:
for v in Vt:
line = 'vt ' + ' '.join([str(_) for _ in v]) + '\n'
file.write(line)
# 3D Faces / UV faces
if Ft:
F = [[str(i+1)+'/'+str(j+1) for i,j in zip(f,ft)] for f,ft in zip(F,Ft)]
else:
F = [[str(i + 1) for i in f] for f in F]
for f in F:
line = 'f ' + ' '.join(f) + '\n'
file.write(line)
"""
Reads PC2 files, and proposed format PC16 files
Inputs:
- file: path to .pc2/.pc16 file
- float16: False for PC2 files, True for PC16
Output:
- data: dictionary with .pc2/.pc16 file data
NOTE: 16-bit floats lose precision with high values (positive or negative),
we do not recommend using this format for data outside range [-2, 2]
"""
def readPC2(file, float16=False):
# assert file.endswith('.pc2') and not float16 or file.endswith('.pc16') and float16, 'File format not consistent with specified input format'
data = {}
bytes = 2 if float16 else 4
dtype = np.float16 if float16 else np.float32
with open(file, 'rb') as f:
# Header
data['sign'] = f.read(12)
# data['version'] = int.from_bytes(f.read(4), 'little')
data['version'] = unpack('<i', f.read(4))[0]
# Num points
# data['nPoints'] = int.from_bytes(f.read(4), 'little')
data['nPoints'] = unpack('<i', f.read(4))[0]
# Start frame
data['startFrame'] = unpack('f', f.read(4))
# Sample rate
data['sampleRate'] = unpack('f', f.read(4))
# Number of samples
# data['nSamples'] = int.from_bytes(f.read(4), 'little')
data['nSamples'] = unpack('<i', f.read(4))[0]
# Animation data
size = data['nPoints']*data['nSamples']*3*bytes
data['V'] = np.frombuffer(f.read(size), dtype=dtype).astype(np.float32)
data['V'] = data['V'].reshape(data['nSamples'], data['nPoints'], 3)
return data
"""
Reads an specific frame of PC2/PC16 files
Inputs:
- file: path to .pc2/.pc16 file
- frame: number of the frame to read
- float16: False for PC2 files, True for PC16
Output:
- T: mesh vertex data at specified frame
"""
def readPC2Frame(file, frame, float16=False):
assert file.endswith('.pc2') and not float16 or file.endswith('.pc16') and float16, 'File format not consistent with specified input format'
assert frame >= 0 and isinstance(frame,int), 'Frame must be a positive integer'
bytes = 2 if float16 else 4
dtype = np.float16 if float16 else np.float32
with open(file,'rb') as f:
# Num points
f.seek(16)
# nPoints = int.from_bytes(f.read(4), 'little')
nPoints = unpack('<i', f.read(4))[0]
# Number of samples
f.seek(28)
# nSamples = int.from_bytes(f.read(4), 'little')
nSamples = unpack('<i', f.read(4))[0]
if frame > nSamples:
print("Frame index outside size")
print("\tN. frame: " + str(frame))
print("\tN. samples: " + str(nSamples))
return
# Read frame
size = nPoints * 3 * bytes
f.seek(size * frame, 1) # offset from current '1'
T = np.frombuffer(f.read(size), dtype=dtype).astype(np.float32)
return T.reshape(nPoints, 3)
"""
Writes PC2 and PC16 files
Inputs:
- file: path to file (overwrites if exists)
- V: 3D animation data as a three dimensional array (N. Frames x N. Vertices x 3)
- float16: False for writing as PC2 file, True for PC16
This function assumes 'startFrame' to be 0 and 'sampleRate' to be 1
NOTE: 16-bit floats lose precision with high values (positive or negative),
we do not recommend using this format for data outside range [-2, 2]
"""
def writePC2(file, V, float16=False):
assert file.endswith('.pc2') and not float16 or file.endswith('.pc16') and float16, 'File format not consistent with specified input format'
if float16: V = V.astype(np.float16)
else: V = V.astype(np.float32)
with open(file, 'wb') as f:
# Create the header
headerFormat='<12siiffi'
headerStr = pack(headerFormat, b'POINTCACHE2\0',
1, V.shape[1], 0, 1, V.shape[0])
f.write(headerStr)
# Write vertices
f.write(V.tobytes())
"""
Reads proposed compressed file format for mesh topology.
Inputs:
- fname: name of the file to read
Outputs:
- F: faces of the mesh, as triangles
"""
def readFaceBIN(fname):
if '.' in os.path.basename(fname) and not fname.endswith('.bin'):
print("File name extension should be '.bin'")
return
elif not '.' in os.path.basename(fname): fname += '.bin'
with open(fname, 'rb') as f:
F = np.frombuffer(f.read(), dtype=np.uint16).astype(np.int32)
return F.reshape((-1,3))
"""
Compress mesh topology into uint16 (Note that this imposes a maximum of 65,536 vertices).
Writes this data into the specified file.
Inputs:
- fname: name of the file to be created (provide NO extension)
- F: faces. MUST be an Nx3 array
"""
def writeFaceBIN(fname, F):
assert type(F) is np.ndarray, "Make sure faces is an Nx3 NumPy array"
assert len(F.shape) == 2 and F.shape[1] == 3, "Faces have the wron shape (should be Nx3)"
if '.' in os.path.basename(fname) and not fname.endswith('.bin'):
print("File name extension should be '.bin'")
return
elif not '.' in os.path.basename(fname): fname += '.bin'
F = F.astype(np.uint16)
with open(fname, 'wb') as f:
f.write(F.tobytes()) | 6,824 | Python | 31.971014 | 143 | 0.652989 |
eliabntt/GRADE-RR/TipsAndTricks.md | ## Tips and tricks
### Main concepts
The simulation has various components.
1. `kit` or `SimulationApp` used to access the engine itself. Essentially, the base engine over which everything works [link](https://docs.omniverse.nvidia.com/py/isaacsim/source/extensions/omni.isaac.kit/docs/index.html). You can't do much with this. It's always the first call, prior to loading isaac/omni components (even python import calls).
2. The simulation context. This class provide functions that take care of many time-related events such as perform a physics or a render step for instance. It also includes an instance of PhysicsContext which takes care of many physics related settings such as setting physics dt, solver type..etc [link](https://docs.omniverse.nvidia.com/py/isaacsim/source/extensions/omni.isaac.core/docs/index.html?highlight=context#module-omni.isaac.core.simulation_context).
3. The `stage` object. Accessed with `omni.usd.get_context().get_stage()`. Used to access all the simulations objects and their properties, e.g. through `prim = stage.GetPrimAtPath('/whatever')`.
4. `omni` is generally available, independently in which piece of code you are. Thus using 3. or `omni.kit.app.get_app()` you should be able to access everything you need without passing objects around.
You'll work mainly with 2 and 3. All functions have autocomplete capabilities, just place a breakpoint and walk your way through them.
If you hover over some properties in the UI sometimes a helper dialog will give you the name of the property. Otherwise, the `prim.GetPropertyNames()` will give you the available properties.
In general, the prims and their properties are accessed through a prim tree. Sometimes, some properties, are accessible only if the prim is accessed as a specific kind (e.g. a mesh [link](https://github.com/eliabntt/GRADE-RR/blob/7d9cb9a3d75d57628adacb9b9f969909d7663f3d/simulator/smpl_and_bbox.py#L206)) or under specific additional keywords (e.g. the physics collision [link](https://github.com/eliabntt/GRADE-RR/blob/7d9cb9a3d75d57628adacb9b9f969909d7663f3d/simulator/utils/misc_utils.py#L275)). Unfortunately, there is no easy way to figure this out due to the symbiosys between Isaac and USD.
NOTE that some functions are highly customized (e.g. `set_drone_joints_init_loc`)! This is thought to help you out set up your _custom_ simulation, and not an off the shelf solution!
### Clear properties
For each object that you load you should call `clear_properties(path)` [link](https://github.com/eliabntt/GRADE-RR/blob/7d9cb9a3d75d57628adacb9b9f969909d7663f3d/simulator/utils/misc_utils.py#L414). This will ensure that the translate, rotate and scale operations are attached to the objects as expected.
### Viewports and cameras
Each rendering component will have a viewport associated to that. In our testing we found out that switching cameras related to a viewport (to reduce memory consumption) may lead to memory leakage and other problems. What we suggest is to have one viewport for every thing that you want to render, be that a full-res camera or a ROS-camera. If you want both high-res and low-res images, we suggest to have two viewports. It will slow down things (as rendering will be slower), but it will be easier to manage.
### Try out before deployment
You can easily try out code in the script tool of the main simulation.
In short you want to open the stage, open the script toolbox, and try out your snippets there. Remember that it is not possible to render/update the simulation in this case. If you need the physics, stop and play the simulation using the corresponding buttons. You will probably need the `stage` object (see point 3 above), and your own code. Remember that you need to import any additional module.
Some commands can also be seen copied and used using the [Command Tool](https://docs.omniverse.nvidia.com/isaacsim/latest/ext_omni_kit_commands.html) util.
<details closed>

</details closed>
### Time
For robotics applications the time is goverened by the physics time. On the other hand, the default step of the simulation in Isaac is governed by the rendering. The easy way to solve this is to manually publish the clock as shown in the tutorials, and keep the timeline tool under "control".
The timeline tool is what controls the animations. You can access that using
```
timeline = setup_timeline(config) # config containing some additional options
# or
timeline = omni.timeline.get_timeline_interface()
```
And perform various operations such as
```
timeline.set_current_time(0)
timeline.get_current_time()
timeline.forward/backward_one_frame()
timeline.play()
timeline.stop()
```
`timeline.set_auto_update(False)` is used to stop the timeline advancing every rendering call. The timeline must be `playing` for the physics, clock etc, to work correctly. Thus, in theory, it would be possible to set the update to false, forward the timeline step before rendering whenever necessary, and the problem would be solved. *However, this is apparently not working in the current version of Isaac Sim. Thus, in the `sleeping()` function [link](https://github.com/eliabntt/GRADE-RR/blob/7d9cb9a3d75d57628adacb9b9f969909d7663f3d/simulator/utils/simulation_utils.py#L149) we constantly reset the time to the current time so that the rendering is correct. Also, continuously call `play` and `stop` might cause problems.*
### Simulation rendering, manual interaction, and UI
The simulation app UI will be refreshed ONLY when you do a rendering call.
For stepping the physics and rendering you have different options:
1. `kit.update()` will step both the physics and the rendering
2. `simulation_context.render()` will do only a SINGLE rendering step
3. `simulation_context.step()` will do both a rendering and physics step. Not always working
4. `simulation_context.step(render=False)` will do a physics step
5. `omni.kit.app.get_app().update()` as `kit.update()`, but accessible if you do not have access to the kit object itself.
My suggestion is to always work with a combination of `simulation_context.render()/step(render=False)` and to stick to that.
If needed, you will be able to interact with the application only when fast enough rendering calls are made. Sometimes, it is necessary to also step the physics to see the effects of your actions. A quick way to do this is to:
1. enter debug mode in python
2. run a loop such as
```
for _ in range(1000):
simulation_context.step(render=False)
simulation_context.render()
```
#### *The rendering calls are NOT blocking. This means that every time you render it will do that for either 1) a fixed amount of time in case of RTX rendering, or 2) a single step for path tracing rendering. This has been solved by us through the `sleeping` function in the `simulation_utils.py`.*
#### *The visual information on the application is updated after the SECOND render call.*
### Save the GT information
The process is to either save stuff from the main simulation loop, or to use the synthetic recorder extension.
In the latter case you can use directly what we provide in `isaac_internals/exts/omni.isaac.synthetic_recorder/omni/isaac/synthetic_recorder/extension_custom.py` and expand it alongside with `isaac_internals/exts/omni.isaac.synthetic_utils/omni/isaac/synthetic_utils/writers/numpy.py` and `isaac_internals/exts/omni.isaac.synthetic_utils/omni/isaac/synthetic_utils/syntheticdata.py` code.
Then you can create a recorder directly in your code using:
```
from omni.isaac.synthetic_recorder import extension_custom
my_recorder = extension_custom.MyRecorder()
my_recorder.on_startup() # necessary call
_settings = my_recorder.get_default_settings()
_settings["rgb"]["enabled"] = True # inspect and extend this dictionary
my_recorder.set_single_settings(_settings)
my_recorder._dir_name = os.path.join(out_path)
my_recorder._enable_record = True # set to false to disable
my_recorder.skip_cameras = 0 # number of viewports to skip
# do stuff
my_recorder._update() # write data if enabled
```
This will create the desired data for EACH viewport.
A shorter version is by using
```
recorder = recorder_setup(recorder_setup(_recorder_settings, out_path, enabled, skip_cameras)
recorder._update()
```
Skip cameras is used to let the system know how many viewports it need to skip when saving the data itself.
Multiple recorders can be set in place. They will all cycle through all the viewports, unless you change the code yourself.
All data can be also accessed in the main simulation loop. Some examples are the vertices, or the lidar information (see the replay experiment script).
Potentially, you could also get as output of the recorder `_update()` call all the information, edit, and publish them as ROS messages.
### Save the motion vector
This is not possible during rendering itself. To save it you need to manually render (for the second time), wait for the data to be produced, and then save the motion vector itself. See the repeating experiment tool for an example on how to do that [here](https://github.com/eliabntt/GRADE-RR/blob/7d9cb9a3d75d57628adacb9b9f969909d7663f3d/simulator/replay_experiment.py#L391-L392). Note that the motion vector can be only visualized by the default Isaac installation and not saved (see [here](https://docs.omniverse.nvidia.com/py/isaacsim/source/extensions/omni.isaac.sensor/docs/index.html#module-omni.isaac.sensor.scripts.camera)). Thus, we cannot ensure correctness.
### Traverse the stage
To traverse all the prims in the stage you can simply run `for prim in stage.Traverse(): ...`
### Enable/Disable collisions
Add colliders [here](https://github.com/eliabntt/GRADE-RR/blob/7d9cb9a3d75d57628adacb9b9f969909d7663f3d/simulator/utils/misc_utils.py#L125) See [here](https://github.com/eliabntt/GRADE-RR/blob/7d9cb9a3d75d57628adacb9b9f969909d7663f3d/simulator/utils/misc_utils.py#L106)
### Hide objects from the viewport
Change visibility of a list of objects [here](https://github.com/eliabntt/GRADE-RR/blob/7d9cb9a3d75d57628adacb9b9f969909d7663f3d/simulator/utils/misc_utils.py#L513)
### Postprocess the data
Please check our dedicated repository [here](https://github.com/robot-perception-group/GRADE_tools).
### Colorize the saved data
Simply run `python scripts/colorize.py --viewport_folder main_folder_with_npy_files`.
Check our code [here](https://github.com/eliabntt/GRADE-RR/blob/main/scripts/colorize.py), you can save images, images and videos, and decide which kind of data you want.
### Get skeletal, vertices, and SMPL information while correcting bounding boxes
Look [here](https://github.com/eliabntt/GRADE-RR/blob/main/simulator/smpl_and_bbox.py). This is mainly tuned for our data. However, it can be easily expanded to your own dataset. In short, for skeleton you need to open the prim as `AnimationSchema.SkelJoint(prim).GetJoint()` [here](https://github.com/eliabntt/GRADE-RR/blob/7d9cb9a3d75d57628adacb9b9f969909d7663f3d/simulator/smpl_and_bbox.py#L192), for the vertices use `points = UsdGeom.PointBased(prim)`[here](https://github.com/eliabntt/GRADE-RR/blob/7d9cb9a3d75d57628adacb9b9f969909d7663f3d/simulator/smpl_and_bbox.py#L206). Using the latters, you can get the bounding boxes.
### Edit directly USD files
Check the tutorial [here](https://github.com/eliabntt/GRADE-RR/blob/37ee985abccc6239bec7f22241c49da0acc5402c/EDIT_USDS.md). This will help you convert USD to txt files for easy file processing.
### How to move/control the camera/robot
You have several possibilities with and without ROS, with and without physics. Check them out [here](https://github.com/eliabntt/GRADE-RR/blob/37ee985abccc6239bec7f22241c49da0acc5402c/MOVEMENT.md)
### Possible missing textures/wrong paths
When loading humans or environments (or anything else) it may be necessar for you to edit the paths of the shaders, especially when moving between Windows and Linux.
To do that you can use the [`change_shader_path`](https://github.com/eliabntt/GRADE-RR/blob/main/simulator/utils/misc_utils.py#L62) or the [correct paths](https://github.com/eliabntt/GRADE-RR/tree/main/scripts/process_paths) scripts.
Otherwise, you can simply process the text files as explained [here](https://github.com/eliabntt/GRADE-RR/blob/main/EDIT_USDS.md).
### Segmentation <-> instance
Instance segmentation files will save also the mappings between classes. An example on how to do the mapping and process those file is [here](https://github.com/robot-perception-group/GRADE-eval/blob/main/mapping_and_visualization/convert_classes.py).
### Shapenet and GSO
For the objects please download at least some assets from ShapeNetv2 or GSO websites. Paths should be `../gso/folders_of_the_objects` and `../shapenet/synsetIds/...`. For ShapeNet please also add `../shapenet/v1_csv/all_of_the_synset_csvs`. Our code will convert locally in `../gso/exported_usd` and `../shapenet/local-converted-USD`. Clearly, you can pre-process everything and use only the USDs afterwards (to save space). All the code is on `simulator/utils/object_utils.py`.
### Project pixels to world
Look at `scripts/pixel_to_world.py`
### Bag processing
Average stats `scripts/average_rosbag.py`
Filter and compress `scripts/filter_compress.sh`
### Automatically generate data
See `scripts/bash_process.zsh` using `screen`
| 13,343 | Markdown | 71.129729 | 727 | 0.781908 |
eliabntt/GRADE-RR/HOWTO.md | ## Requirements and basic software installation
Please check the [requirements](https://docs.omniverse.nvidia.com/app_isaacsim/app_isaacsim/requirements.html) on the official page.
Then download the omniverse launcher and install Nucleus, Cache, and Isaac Sim.
From now on, we will assume that you installed Isaac Sim within a `ISAAC_FOLDER`. Default location is `~/.local/share/ov/pkg/isaac-version/`.
Clone this repository. You can clone this wherever you prefer. For simplicity, we usually download it within the `isaac` folder.
However, by using global paths you should be able to run this code anywhere in your PC.
_Note_ Isaac will have its own python installation, if you need packages and you run software within the Isaac python executable remember that. To do so, you usually do something like
```
cd $ISAAC_FOLDER
./python.sh -m pip install ...
# or
./python.sh python_file.py
```
We have some dependencies which are not installed by default. To install them run `sh req.sh $ISAAC_FOLDER`. (This will simply use the main Isaac `python.sh` to install everything via `pip`).
Independently on where you cloned the repository you need to run
`sh cp_local_to_different_folder.sh $CLONE_FOLDER $ISAAC_FOLDER`
This will copy the edited files from $1 (source) to the $2 (destination). You can use it in reverse (from Isaac to repo), or with any couple of folders.
## Misc
A general note: every script has been more or less commented and almost each piece of code should be self-explanatory. If you don't find it like that please **open an issue**.
I worked on this mainly alone so the code is far from perfect, super-modular, or anything like that. But together we can make it better.
Thus, we welcome any contribution that you might have. Include coding style, comments, additions, or better strategies that you want to propose (of course after you have published your paper).
## How to start the simulation
To launch Isaac you can run `./isaac-sim.sh` from the main installation folder to launch the simulator. It is suggested to do this once before starting any other coding activity.
To control the simulation with your own code the general process is `./python.sh python_script args`. In `args` you can specify either python arguments arguments for the Isaac simulator itself (e.g. ` --/renderer/enabled='iray'`).
The functions that we use in our scripts are all contained in the `simulator/utils` folder. An explanation of each one of the function is given in their comment, while a brief overview is given [here](https://github.com/eliabntt/GRADE-RR/blob/main/simulator/utils/UTILS.md).
## Main concept
Our programs all follow the same structure.
- load the basic kit and start the initial simulation
- load a basic environment with some settings pre-applied (some config changes cannot be made with the code itself)
- load libraries and settings
- load your main environment
- edit the environment
- load the robots
- attach sensors to the robot
- correct the camera fov (bug in Isaac that changes it)
- [optional] load and place humans, objects and animate objects
- setup information recorder
- loop the simulation and publish/write the information when necessary
Every aspect can be personalized or adapted. The basic environment could be your final one, the humans/animations can be present or placed in a different way, robot can have your set of sensors or your own publishing rate.
Our code is thought in such a way that each robot is loaded pre-fixed with the `my_robot_` name, and this applies to each topic that is published from that robot. The exception lies in the `tf` topic, for which we will have a publisher for each robot. Data can be published in ROS and saved as npy files. If you want both, with the former using a lowres camera and the latter an high res camera you should first load all the robots, and then call `add_npy_cameras` adjusting the skipped camera of your `recorder`. See the [tips](https://github.com/eliabntt/GRADE-RR/blob/main/TipsAndTricks.md) readme for more insights.
## Your first code
[Here](https://github.com/eliabntt/GRADE-RR/blob/main/simulator/first_run.py) is a first example showing how to launch the simulation, load a basic environment, and perform some basic actions.
The workflow will always be the same. Import general modules, create the `SimulationApp`, import the IsaacSim related stuff, and proceed. Please, look at the comments in the code directly. Brief explanations are also given below.
## Going past your first code
Before adventuring here, please be sure to download our sample [world]() and [animated assets](). Those scripts will be incremental (i.e. based on the previous one). Please open all the downloaded USDs once at least to be sure that textures and everything else is correctly loaded.
We marked _Optional_ what can be skipped in future iterations of _your_ code, but still, please go through them. They will go step by step from the general environment to the animated house.
**Beore launching any simulation that need ros you need to start `roscore` if using ROS preferably with sim time set to true (`rosparam set use_sim_time true`)**
In these codes, we consider our provided sampled world, the animated assets, and the drone provided with this repository. For the objects, you will find a note in the corresponding tutorial details. Additional samples (our used code, adapted from v2021), will be added in the next section.
##### Still WIP, need to add links and make sure that the code works. But most of it should work rn.
- Using a config file, adding your own "world", and a robot [here](https://github.com/eliabntt/GRADE-RR/blob/main/simulator/world_and_robot.py).
<details closed>
- To create a robot you can either import our `usds/drone_2022.usd` or `usds/robotino.usd`, use your own URDF [link](https://docs.omniverse.nvidia.com/isaacsim/latest/ext_omni_isaac_urdf.html), create your own USD (add a mesh and attach some joints to it, [link](https://docs.omniverse.nvidia.com/isaacsim/latest/tutorial_gui_simple_robot.html)), or use one of the already available models. For now, the USD file is enough.
- The world can be either empty (thus you can skip loading), just with static objects, or with pre-placed animated objects (as in the zebra case). The world needs to be placed into a subfolder, e.g. `worlds/Savana/...`. Inside, you could (not mandatory) have:
- `npy` file with the limits of the environment
- `stl` file with the 3D occupancy of the environment
If you do NOT have those, just disable the flags in the config file (see last point of this list). Otherwise, they will be used as shown [here](https://github.com/eliabntt/GRADE-RR/blob/455891d5021009695a5da13c4feda0ceb258d476/simulator/utils/environment_utils.py).
- You will also see how to add colliders to the environment, how to generate a 2D occupancy map, how to use the meters per unit, how to move the robot before starting the simulation (by moving the joints).
- Launch this with `./python.sh simulator/world_and_robot.py --config="/your_full_path/simulator/world_and_robot.yaml" --fix_env=Something`. `--config` is mandatory, `--fix_env` will tell to the system to select the `Something` world from the `world` environments folder, e.g. `Sample_house`
</details closed>
- [Optional] Fix the rendering engine, add and publish some ROS components to the robot itself [here](https://github.com/eliabntt/GRADE-RR/blob/main/simulator/robot_with_ros.py).
<details closed>
- You will see how to add the clock to the simulation. Thanks to how we define it [here](https://github.com/eliabntt/GRADE-RR/blob/455891d5021009695a5da13c4feda0ceb258d476/simulator/utils/robot_utils.py#L274) the clock will tick with pysics steps, but will need to be manually published.
- Our phylosophy is to manually publish ROS messages for better flexibility
- We will show both how to add single components, or a batch of them, i.e. through custom "add all sensors" functions as we have done [here](https://github.com/eliabntt/GRADE-RR/blob/7d9cb9a3d75d57628adacb9b9f969909d7663f3d/simulator/utils/robot_utils.py#L557).
- How to publish data (either manually with ROS messages or using the internal Isaac Components)
- You can then fix the rendering engine (path vs raytracing), and get to know the `sleeping` function
- Place a breakpoint somewhere and try to manually render the environment while the timeline is playing (not using sleeping). Note how the rendering will advance the timeline of more than what you want. This does not affect the physics, but will affect the animations. Keep this in mind. See [here](https://github.com/eliabntt/GRADE-RR/blob/6e42652201509ed7ad95624d9a551e24fe5ce03c/TipsAndTricks.md#L38) for more details.
- Launch this with `./python.sh simulator/robot_with_ros.py --config="/your_full_path/simulator/robot_with_ros.yaml" --fix_env=Something`. `--config` is mandatory, `--fix_env` will tell to the system to select the `Something` world from the `world` environments folder, e.g. `Sample_house`
</details closed>
- [Optional] Add animated people, additional objects, and animate those while solving the timeline problem [here]().
<details closed>
- You can get a sample human from [here](). Soon, we will upload our collection. Since then, you can follow our other repository [here](https://github.com/eliabntt/animated_human_SMPL_to_USD) to convert your SMPL models to USD. The preferred folder structure is `main/dataset/ID`, you will provide the `main` folder to allow the randomizer to work.
- You can either place the models manually into your world beforehand (see the zebra case), use pre-fixed (or random) locations, or use a placement technique. Our placement technique will be explored in the additional scripts since it requires setting up the catkin workspace as well.
- For the objects please download at least some assets from ShapeNetv2 or GSO websites. If not, please comment out that part of the code, or adapt it to your own assets. We think the GSO part can be made general quite easily. Paths should be `../gso/folders_of_the_objects` and `../shapenet/synsetIds/...`. For ShapeNet please also add `../shapenet/v1_csv/all_of_the_synset_csvs`. In the config add the `gso` and the `shapenet` folders. Additional options are there.
- The animation will use the timeline interface see [here](https://github.com/eliabntt/GRADE-RR/blob/064c1b888727c6faa191f88519184dc272a8b950/simulator/utils/objects_utils.py#L135).
- The objects loading code is [here](https://github.com/eliabntt/GRADE-RR/blob/064c1b888727c6faa191f88519184dc272a8b950/simulator/utils/objects_utils.py), for both shapenet and google scanned objects. You can see how the conversion works [here](https://github.com/eliabntt/GRADE-RR/blob/064c1b888727c6faa191f88519184dc272a8b950/simulator/utils/objects_utils.py#L65). The system will automatically save the converted USD for backup and to avoid re-conversion.
- Launch this with `./python.sh simulator/people_and_objects.py --config="/your_full_path/simulator/humans_and_objects.yaml" --fix_env=Something`. `--config` is mandatory, `--fix_env` will tell to the system to select the `Something` world from the `world` environments folder, e.g. `Sample_house`
</details closed>
- [Optional] Launch your own SIL from within your own simulation script, add some randomization (e.g. lights, textures etc) and save GT data [link]()
## Additional scripts
### Correct data and smpl_and_bbox
[This](https://github.com/eliabntt/GRADE-RR/blob/7d9cb9a3d75d57628adacb9b9f969909d7663f3d/simulator/smpl_and_bbox.py) and [this](https://github.com/eliabntt/GRADE-RR/blob/7d9cb9a3d75d57628adacb9b9f969909d7663f3d/simulator/correct_data.py) show how to access low-level information of the meshes, how it is possible to correct the 3DBbox and pose incorrect information.
### Zebra data generation and Animation Sequences
[This](https://github.com/eliabntt/GRADE-RR/blob/7d9cb9a3d75d57628adacb9b9f969909d7663f3d/simulator/zebra_datagen.py) is the code that we used to generate the data for the Zebra paper. Unfortunately, we cannot share the USDs of the environments, whith the exception of the Savanna one, due to licensing limitations.
You can however explore how to access low level animation sequences [link](https://github.com/eliabntt/GRADE-RR/blob/455891d5021009695a5da13c4feda0ceb258d476/simulator/utils/zebra_utils.py#L136) and how we managed to generate our data for the [Synthetic Data-based Detection of Zebras in Drone Imagery paper](https://arxiv.org/abs/2305.00432). Run it with `./python.sh GRADE-RR/simulator/zebra_datagen.py --/renderer/enabled='rtx,iray' --config='configs/config_zebra_datagen.yaml' --headless=False --fix_env=Savana`
### Replay experiment
[This](https://github.com/eliabntt/GRADE-RR/blob/7d9cb9a3d75d57628adacb9b9f969909d7663f3d/simulator/replay_experiment.py) is a very useful piece of code. You can use this to replay any previously recorded experiment, modify the robot (or the scene conditions) and record new data. You can replay the experiment in two modalities, namely using teleport or by physically interpolating the trajectory. Note that the latter is subject to some drift due to the interpolation of the data itself.
<details closed>
Please run
```
./python.sh GRADE-RR/simulator/replay_experiment.py --experiment_folder FOLDER
```
to do so.
In our code we show how to create a new stereo camera, save previously unsaved data, save motion-vector, and create a LiDAR sensor.
You need some information to be able to repeat an experiment. Namely, the joint positions. We load those [from the rosbags](https://github.com/eliabntt/GRADE-RR/blob/7d9cb9a3d75d57628adacb9b9f969909d7663f3d/simulator/replay_experiment.py#L177), although you can access them from the GT pose arrays.
</details closed>
### Paper autonomous indoor exploration (humans, objects, SIL, active SLAM etc)
### Multi robot management
## Known issues
1. ros clock might have some delay in publishing. This implies that you need to sleep the simulation every time that component gets triggered. Other component behave consistently based on our tests. Alternatively, you can post-process the data as shown in [here](https://github.com/robot-perception-group/GRADE-eval)
2. BBOX3D are wrong for moving objects. The script [here](https://github.com/eliabntt/GRADE-RR/blob/main/simulator/correct_data.py#L267) show a way to solve this.
3. Pose information is wrong for some moving objects. The code [here](https://github.com/eliabntt/GRADE-RR/blob/main/simulator/correct_data.py#L224) will solve this.
4. Collisions for dynamic objects are not computed most of the times due to PhysX limitations. This is addressed by the new LiDAR-RTX of the new Isaac Sim version. However, its management is not intuitive.
5. The rendering is not blocking. Multiple calls (especially for path tracing) are necessary. Thus, this usually disrupt the motion-vector data. A possible workaround is to do two rendering steps and save the motion-vector data, and then finish rendering to save the rgb information. See [here](https://github.com/eliabntt/GRADE-RR/blob/main/simulator/replay_experiment.py#L390) an example on how to do that. Note that a rendering call is done just after the clocking.
6. In the v2022 it is not possible to set indipendent vfov of the cameras. It will take the hfov and use the AR to have a "correct" vfov.
7. In the v2022 the internal PD control for the joints will NOT work using position setpoints. Also, the maximum velocity set is not considered.
8. In the v2022 the timeline gets updated automatically even if you do not want it. You need to keep track of the ctime and constantly re-update it to correctly generate the data you want. | 15,825 | Markdown | 96.691357 | 619 | 0.776114 |
eliabntt/GRADE-RR/SAMPLES.md | # Our Samples
Still WIP.
We have several showcase examples (all located in the simulator folder).
Each one of the python files has its own configuration yaml file. More details will be given below for each file
To follow these tutorials, we suggest that either you download one of our example environments [here]() and human animations [here]() or you use our code [SMPL to USD](https://github.com/eliabntt/animated_human_SMPL_to_USD) and [Blender to USD](https://github.com/eliabntt/Front3D_to_USD) to create your own assets.
We also suggest that you pre-install the [drone](https://github.com/eliabntt/ros_isaac_drone) control and placement repository.
This is necessary to be able to use our placement strategy, control the drone with our custom 6DOF controller, or use FUEL with IsaacSim.
Each simulation file will power up the environment, load the assets and manage the saving based on the loaded configuration file.
The scripts are the following:
1. `FUEL_indoor_simulation` this is the code that we used to generate the dataset.
4. `irotate_simulation` this is the code that we used to simulate [iRotate](https://github.com/eliabntt/irotate_active_slam), our active SLAM method, with Isaac Sim. This is very similar to 1 and 2, despite using an initial location but shwos how you can manage different robot with practically the same code.
5. `multi_robot_sim` simulate multi robots, a bit hardcoded but generalizable. This simulate two drones and a ground robot. The two drones will be controlled independently with two FUEL sessions, while the ground robot is controlled with iRotate.
6. `savana_simulation` to show how we created the Savana with the Zebras. Animated animals are pre-positioned within the environment. The robot is controlled through joint waypoints. **THIS DOES NOT WORK in v2022.2.1 DUE TO ISAACSIM BUGS**
**Each config needs to be updated with your own paths**
___
## Paper(ros) simulation
Install ROS and create a `catkin_ws` and install [this](https://github.com/eliabntt/ros_isaac_drone).
The default location for this installation is `$HOME` (`/home/user/catkin_ws`).
The repo above will install
1. `FUEL`, our chosen exploration manager
2. `mav_comm` and `mav_control_rw` which are used to control the robot and get velocity commands to follow the path generated by `FUEL`
3. `custom_6dof_joint_controller` which is the bridge between the position/velocity commands and the joint velocities expected by IsaacSim
4. `moveit_based_collision_checker_and_placement` which is needed to do the placement of the "objects"
The [README](https://github.com/eliabntt/ros_isaac_drone/blob/main/README.md) already explicate the dependencies.
If you install it in a different location _update `setup_python_env.sh:2`_ with your new location.
Remember that you can also `source ... --extend` to source different environments in cascade.
At this point, assuming you are locate in the ISAAC folder you can run
```
./python.sh GRADE-RR/simulator/paper_simulation.py --config="/GLOBAL/GRADE-RR/simulator/configs/config_paper.yaml"
```
*BASH PROCESSING*
If you want to run everything (including the exploration visualization and the rosbag recorder) the `bash_process.zsh` file is what you are looking for.
That file is what we used to streamline the generation and process in batches. In the config file you can easily chose which sensor to use.
Similarly
```
./python.sh GRADE-RR/simulator/simulator_ros.py --config="/GLOBAL/simulator/configs/config.yaml"
```
would work. Note that in this case you need to edit both the configs and the code otherwise the robot will not move.
_______
## iRotate simulation
Download and install the iRotate package [here](https://github.com/eliabntt/irotate_active_slam/tree/isaac) from the Isaac branch.
This simulation by default does NOT use animated objects. You can see how one can have a blueprint and quickly edit it based on its own convenience.
_update `setup_python_env.sh:2`_ with your catkin workspace location.
Before launching the simulation you need to open a terminal and run `python[3] irotate_specific/republish_tf.py`
Also, run `irotate` as explained in the repo. A set of commands could be:
```
roslaunch robotino_simulations world.launch
roslaunch robotino_simulations rtabmap.launch delete:=-d
roslaunch active_slam active_node.launch
roslaunch robotino_mpc robotino_mpc.launch
```
Note that we launch the FSM later on.
With iRotate we usually let the robot start from `0,0,0` and `yaw=0`. If you change this, like with the previous work, you need to change the ekfs accordingly.
The transform `world->map` is constant. `map->odom` is done by `rtabmap`. `odom->base_link` is done from the ekfs.
Isaac is setted up to publish the tfs to the `/tf2` topic. Step 4 is necessary to publish everything back to the `/tf` cleaned up of the ground truth estimation.
The custom joint controller has been updated. You need to be sure you are running the one from irotate repository.
Thus, we need either to build everything in the same workspace or use `source ... --extend` if you are using two workspaces.
You can eventually change the scripts to have it working how you want.
You can launch an rviz visualization with `rviz -d irotate_specific irotate.rviz`
```
./python.sh GRADE-RR/simulator/irotate_simulation.py --config="/GLOBAL/GRADE-RR/simulator/configs/config_irotate.yaml"
```
Once the simulation is running, you can launch `roslaunch robotino_fsm robotino_fsm.launch kind:=2 only_last_set:=false pre_fix:=true mid_optimizer:=true weighted_avg:=true robot_odom:=/odometry/filtered cam_odom:=/camera/odometry/filtered`
Note how the topics are stilll without `/my_robot_x`. This should be changed in the EKF formulation.
_______
## Multi robot
For this you need both the irotate repository and the original paper repository. The code will launch first the irotate robot and then two drones. You need to include both workspaces in `setup_python_env.sh:2` using first the _ros_isaac_drone_ and then the _irotate_ workspace (use the `--extend` keyword).
You can follow a similar procedure like the one above to launch `iRotate`.
To run the main simulation
```
./python.sh GRADE-RR/simulator/multi_robot_sim.py --config="/GLOBAL/GRADE-RR/simulator/configs/config_multi_robot.yaml"
```
This piece of code show you how multiple robots can be loaded and controlled, how the configuration file can be expanded (e.g. only iRotate's robot has an initial location) and how everything can be customized.
_______
## Savana - not working on 2022
Is another simple scenario since everything is managed internally. The animations are already placed within the environment and the robot has pre-defined waypoints. The FSM is internal to the main source code which can be launched with
```
./python.sh GRADE-RR/simulator/savana_simulation.py --config="/GLOBAL/GRADE-RR/simulator/configs/config_savana.yaml"
```
| 6,912 | Markdown | 57.092436 | 315 | 0.774306 |
eliabntt/GRADE-RR/PARAMS.md | # Which parameters are available and how to edit them
Note: we try our best to update this consistently, but there might be some misalignment, this should be normal also considering different code runs. For example the "init_loc" parameter can be either a number or a list, depending on which version of the code you are running.
The main simulation parameters are the last ones in this page.
_____
### Python params for simulator/smpl_and_bbox
- `experiment_folder`, mandatory, the experiment folder with the USD file and the info file
- `body` When true process the bodies
- `garments` When true process the garments
- `base_path` Human prim base path, i.e. filtering prim paths
- `headless` Whether run this headless or not
- `write` Whether to write results
- `fast` Whether to write only the axis-aligned box or the oriented one, if False, the program will be slow
- `both` Whether to write both vertex types -- preference in code is both
- `only_exp` Whether to export only the experiment (considering the reverse strategy) or the whole sequences
- `get_skel` Whether to get/include the skeleton info
- `skel_root` This is a recognizable last part of the root of the skeleton prim, in our case _avg_root It will process ONLY the path of which the last part is this root
_____
### Python params for scripts/colorize
- `viewport_folder` mandatory, the "viewport" folder where npy data is saved
- `img_id` if negative process the whole sequence, otherwise just the img_id
- `save_imgs` if save images
- `save_video` if save videos (will produce two videos using ffmpeg)
- `always_update_map` if always updating the instance mapping. default to false using the first one. Useful if you toggle objects
- `semantics` if generate semantic
- `output_dir` output directory
_____
### Params for scripts/process_paths
- `config_file` configuration file with options to normalize the path, and critical keys that will be used in the main folder. You want to change these to your paths
- `input` input USDA file. Check the main readme on how to convert the USD file and convert back or use the .sh script
- `output_name` output USDA file
- `output_dir` output dir
_____
### Python params for the main simulator/[*simulation.py, multi_robot_sim.py] files
All the parameters are optional with the exception of the config file.
If you remove something from the config file please be sure that is not used in the code. Otherwise, it will crash.
- `config_file` mandatory, the yaml file with mosst of the params
- `headless` if it's true the visualization is turned off
- `rtx_mode` if it's true the simulation will launch with the RTX rendering (faster), else the PathTracing is used
- `record` if it's true it will write to disk
- `debug_vis` if it's true it will loop visualization and ros camera publishing
- `neverending` if it's true it will continue forever the main loop
- `fix_env` you can set this to the _name_ of the sub-folder containing the environment that you want to load
### Simulation Config YAML params -- these are described for how they are used. You can easily edit and modiy their behavior (e.g. `human_path` can be your only human asset)
***"Mandatory" params***
- `env_path`: folder containing the subfolders of the environments (`env_path/env1, env_path/env2, ...`). You can randomly chose or use `fix_env` to specify an environment.
- `human_path`: folder that contains the subfolders of the human animated assets, separated in the various datasets (`human_path/dataset1/animationX`,`human_path/dataset2/animationX`)
- `base_env_path`: global path of the basic environment (the background). NOTE: some configs cannot be changed from the code
- `usd_robot_path`: global path of the USD of the robot. Can be an array as for multi_robot case
- `robot_mesh_path`: global path of the mesh of the robot. Can be an array as for multi_robot case
- `out_folder`: path of the output folder in which we will save the ROS logs, and the map
- `out_folder_npy`: path of the output folder in which we will save the groundtruth from the simulator code (not the rosbags)
- `num_robots`: number of robots
- `_recorder_settings`: what to save or what not to save. Note that some things are not implemented. I strongly suggest to NOT save colorize data. Motion-vectors can be saved with the strategy shown in replay experiment
- `fps` the fps of the simulation
- `physics_hz`: NOTE THAT THIS IS THE RATE OF CLOCK AND IMU
- `render_hz`: LEAVE IT EQUAL TO PHYSICS HZ
- `env_prim_path` IsaacSim internal path for the prim of the environment
- `robot_base_prim_path` same thing for the robot (the number of the robot is the postfix)
- `is_iRotate` whether the robot is Robotino or not, this changes the launched ROS and some settings. Note that this can be a vector, and can be expanded to different robots
***THE OPTIONAL PARAMETER NEED TO BE EXPLICITLY ADDRESSED IN THE CODE. This wants to be a reference to search code and understand what is going on***
***Depending on usage params - experiment***
- `experiment_length`: camera frames length of the experiment (`seconds * camera_fps`), will be overridden by `neverending`. In the savana experiment this has not been used (experiment ends when last waypoint reached)
- `num_humans`: depends on your usage, can be fixed, minimum number or whatever you want
- `[robot,npy]_sensor_size` camera sensor size for robot and npy data. Can be equal. Npy not necessary if not loaded.
- `bootstrap_exploration`: seconds to boostrap the simulation before starting from time 0 (min(abs(this_value), 1/(physics_hz/ratio_camera)). It sets the time negative and cicle through physics and rendering.
- `reverse_strategy`: timeline reverse strategy based on the loaded animation lengths. Possibilities are [min, max, avg, half, none], works only with animated sequences. It makes the timeline going backward/forward based on this. It will roll back the simulation timeline (not the time, just the animation). This uses the animation length (see `paper_simulation.py`)
- `anim_exp_len` an alternative of `reverse_strategy`, rewinding the simulation after this many frames
- `clean_base_env` whether to remove some things from the base environment loaded at the beginning.
- `reload_references` whether to reload the references of the assets or not. Sometimes it might be necessary (seems solved in the newer versions)
- `generate_map` whether to generate the occupancy map or not. Works only if stls are loaded. Suggest to use only with limited environments (it takes a while to add collisions).
***Depending on usage params - robot movement***
- `autonomous`: true -> use FUEL, false -> use random goals (not fully tested), this is applicable only to the main paper simulation, used with autonomous=True. just to show it.
- `use_robot_traj`: Whether to use or not a predefined traj *not physics enabled*
- `use_joint_traj`: Whether to use or not joint trajecotry *physics enabled*. This cannot be true at the same time of robot_traj.
- `robot_traj`: The trajectory remember that movement will be linear and instantaneous. No acceleration or anything. This implies no odom, nor IMU data. If you want those, please add the same trajectory to a joint publisher.
- `init_loc`: initial location for the robot (the elements can be vectors as in the multi-robot case)
***Depending on usage params - humans***
- `max_distance_human_ground`: max distance from human to ground to be consider to force the first frame grounding of animations
- `allow_collision`: max number of collisions allowed between the stl of the human and the stl of the environment
- `human_base_prim_path` for the humans (the number of the human is the postfix)
- `[max,min]_human_anim_len`: [Max,Min]imum human animation to be considered.
***Depending on usage params - objects***
- `obstacles`: increase those numbers to load shapenet or google objects (or any other objects)
- `google_obj_folder`: google_scanned_objects folder. Structure is `folder/exported_usd` and `folder/assets`
- `google_obj_shortlist`: shortlist some objects, not fully tested
- `shapenet_local_dir`: local dir of ShapeNet *suggestion is to download this beforehand*
- `shapenet_username`: if want to download on the fly. Last time I tried it was not working anymore.
- `shapenet_password`: if want to download on the fly. Last time I tried it was not working anymore.
- `synsetId`: shortlist some objects, not fully tested
- `modelId`: shortlist some objects, not fully tested
***Depending on usage params - simulation***
- `ratio_[tf,odom,camera,...]`: physics_hz/ratio_tf = tf publish hz
- `_random_light`
- `intensity` If intensity needs to be changed
- `color` If color needs to be changed
- `intensity_interval`
- `during_experiment` Change color/intensity during the experiment
- `n-frames` if during experiment True switch the color of the light
- `smooth` NOT IMPLEMENTED
- `_random_roughness` Roughness/reflectance of the materials
- `enabled` If enabled
- `intensity_interval`
***Depending on usage params - others***
- `only_placement` if the placement strategy should be the only ROS thing launched. Following a similar strategy all ROS can be disabled.
- `use_stl` wheter to load the STLs of env/humans or not. This will have repercussion but gives the possibility to avoid generating/loading the STL files. | 9,347 | Markdown | 75.62295 | 366 | 0.758853 |
eliabntt/GRADE-RR/EDIT_USDS.md | If you desire to edit an usd file offline for whatever reason there is an easy way to do it.
`.usd` files are binary files. However, they can be converted to text file easily.
Just go to the official [USD](https://github.com/PixarAnimationStudios/USD) repository and install it in your system.
Then you can run the following:
`usdcat -o text_version.usda binary_version.usd`
to obtain the text file of your `usd`.
With that you can edit all the paths and many other things (e.g. keyframe information).
This may or may not be convenient depending on the use case.
Both are loadable by the system. However, if you wish to convert back to binary format you can run the same command again with
`usdcat -o binary_version.usd text_version.usda`.
Alternatively check out our `scripts/process_paths` folder. It will be automatic and easily adaptable to your use case.
| 867 | Markdown | 47.22222 | 126 | 0.775087 |
eliabntt/GRADE-RR/MOVEMENT.md | # Movement control
There are two main aspects: ROS and Physics.
You can control the movement with or without ROS and with or without Physics.
In general, including ROS implies having physics.
With "With ROS" we mean that ROS is involved in the input. Clearly, you can always publish ROS information from the simulation.
When using joints, the system will essentially always use physics in some way. Clearly, as in Gazebo, you can disable gravity, collisions etc to your convenience.
An important thing to remember is that by being physics enabled implies that joints will be affected by the mass of the object to which those are attached. Clearly, even with teleport this might be true. In practice, not being physically enabled requires you to disable gravity of the object and collisions. To disable gravity, do so by changing the property (if exists) of the asset, similarly to what we do for the collision [here](https://github.com/eliabntt/GRADE-RR/blob/7d9cb9a3d75d57628adacb9b9f969909d7663f3d/simulator/utils/misc_utils.py#L106). With the exception of options 2 and 4 (which are pretty much equivalent) of the _Without ROS_ case.
### With ROS
1. Attach a joint publisher/listener to the robot ([link](https://github.com/eliabntt/GRADE-RR/blob/7d9cb9a3d75d57628adacb9b9f969909d7663f3d/simulator/utils/robot_utils.py#L233)) and directly publish a `joint_commands` ROS message either on your own [link](https://docs.ros.org/en/melodic/api/control_msgs/html/msg/JointJog.html), using our 6DOF joint controller [link](https://github.com/eliabntt/custom_6dof_joint_controller), through MoveIt (see the [tutorial](https://docs.omniverse.nvidia.com/isaacsim/latest/tutorial_ros_moveit.html)).
2. Use an embedded controller provided by IsaacSim and publish `cmd_vel` commands dependending on your use case.
3. Use ROS to publish setpoints in some ways, listen to the topic within the simulation loop, and fall back to the "without ROS" section.
### Without ROS
1. Move the robot by sending _joint_ position/velocity setpoints directly from IsaacSim. This will output physics and will abide the settings that you use for your joints (mass, force...). The implementations within IsaacSim is through a PD control. An example of this has been implemented [here](https://github.com/eliabntt/GRADE-RR/blob/7d9cb9a3d75d57628adacb9b9f969909d7663f3d/simulator/utils/robot_utils.py#L760). Although the implementation there is different (it assume a set of predefined checkpoints) the concept is the same. To learn about stiffness and damping, please check [this](https://forums.developer.nvidia.com/t/stiffness-damping-setting-in-joint-drive/213916) or [this](https://docs.omniverse.nvidia.com/isaacsim/latest/ext_omni_isaac_motion_generation.html). Note that this has some issues in the 2022.2.1 version of the engine. Clearly, using this you can write your own controller.
<details closed>
```python
import omni.kit.commands
from pxr import Sdf
omni.kit.commands.execute('ChangeProperty',
prop_path=Sdf.Path('/World/robot/.../PrismaticJoint.drive:linear:physics:targetPosition'),
value=10,
prev=0)
```
```python
import omni.kit.commands
from pxr import Sdf
omni.kit.commands.execute('ChangeProperty',
prop_path=Sdf.Path('/World/Cone/PrismaticJoint.drive:linear:physics:targetVelocity'),
value=10,
prev=0.0)
```
</details closed>
2. Use a strategy like the one we use for the [flying objects](https://github.com/eliabntt/GRADE-RR/blob/7d9cb9a3d75d57628adacb9b9f969909d7663f3d/simulator/utils/objects_utils.py#L191) adding [translation](https://github.com/eliabntt/GRADE-RR/blob/7d9cb9a3d75d57628adacb9b9f969909d7663f3d/simulator/utils/misc_utils.py#L288) and [rotation](https://github.com/eliabntt/GRADE-RR/blob/7d9cb9a3d75d57628adacb9b9f969909d7663f3d/simulator/utils/misc_utils.py#304) animations (also scale is possible). However, this does NOT include physics, collision or anything similar whatsoever. In this case the trajectory is followed blindly and interpolated based on your settings.
3. Use [teleporting](https://github.com/eliabntt/GRADE-RR/blob/7d9cb9a3d75d57628adacb9b9f969909d7663f3d/simulator/utils/misc_utils.py#L497). For this see [replay experiment](https://github.com/eliabntt/GRADE-RR/blob/main/simulator/replay_experiment.py) code. Note that running the physics steps will imply that the robot will be affected by physics (e.g. collisions, gravity etc)
4. Create a spline, animation sequence, or whatever and saving that to the USD file itself. Once loaded, the robot will behave as an animated object. Again, this won't follow physics low. It will still be affected by physics (accel, velocities) but not to collisions, gravity, etc. See [here](https://docs.omniverse.nvidia.com/extensions/latest/ext_animation-timeline.html) and related resources. Similar to #2.
5. Directly set joint status as done in [replay experiment](https://github.com/eliabntt/GRADE-RR/blob/7d9cb9a3d75d57628adacb9b9f969909d7663f3d/simulator/replay_experiment.py#L348) from within the simulation itself, although this is quite similar to do #1. | 5,063 | Markdown | 94.547168 | 904 | 0.796563 |
eliabntt/GRADE-RR/README.md | # GRADE-RR or how to Generate Realistic Animated Dynamic Environments for Robotics Research
### Note that while we used the v2021 for the paper, that version is now deprecated. I will work only on v2022+
GRADE is a system I developed to seamlessly manage the Isaac Sim simulation software to Generate Realistic Animated Dynamic Environments for Robotics Research

This will help you in:
1. managing the simulation
2. load, place, animate assets
3. load and control any robot --- with or without ROS, with or without physics
4. get sensor readings from such robots, saving *ground truth* or *noisy* data
5. customize your workflow
6. postprocess the data --- add noise, reorganize the bags, prep the data for DL models...
7. repeat any experiment --- *this includes recording new sensor, getting new data, changing the conditions and repair the data while working in realistically looking environments and in a physics enabled simulator.*
Each step of the pipeline can be easily customized, expanded or removed from your workflow.
If you want more information check out the [paper](https://arxiv.org/abs/2303.04466) or our [website](https://eliabntt.github.io/grade-rr).
_______
## Useful related repositories (that couldn't fit this page)
1. The tools to process the data, add noise to the rosbags or during the simulation, to evaluate the SLAM methods, generate training data can be found [here](https://github.com/robot-perception-group/GRADE_tools)
2. The code to convert SMPL-based animations to USD files is [here](https://github.com/eliabntt/animated_human_SMPL_to_USD). Use this if you want to convert AMASS animated SMPL models, the Cloth3D dataset, or any other dataset that you might have that contains skeletal animations. If you use something different than SMPL (or some of its variations), you will need to extend this code.
3. To convert any environment from Blender to USD and generate some accompanying data use [this](https://github.com/eliabntt/Front3D_to_USD). This has a special focus in indoor environmets and Front3D. Based on BlenderProc. You can use this tool also to convert ANY fbx or other file.
4. The tools we used to autonomously explore the environments during the data generation is [here](https://github.com/eliabntt/ros_isaac_drone), using RotorS, FUEL, our custom 6DOF controller, etc.
5. The modified version of DynaSLAM working with Python3 and using `detectron2` is [here](https://github.com/eliabntt/DynaSLAM)
6. `custom_6dof_joint_controller` is the bridge between the position/velocity commands and the joint velocities expected by IsaacSim. This will allow you to control any robot within the simulation environment. [Link here](https://github.com/eliabntt/custom_6dof_joint_controller/tree/main).
7. `moveit_based_collision_checker_and_placement` our Move-it based placement strategy. [Link here](https://github.com/eliabntt/moveit_based_collision_checker_and_placement/tree/main)
______
## Our projects
### Active SLAM, indoor scenes data collection, and dynamic SLAM
With this framework in conjuction with our [people generator](https://github.com/eliabntt/animated_human_SMPL_to_USD), [environment exporter](https://github.com/eliabntt/Front3D_to_USD) and [control framework](https://github.com/eliabntt/ros_isaac_drone) (which can control virtually anything thanks to our expandable [custom 6DOF joint controller](https://github.com/eliabntt/custom_6dof_joint_controller)), we generated an extensive dataset of indoor animated scenes.
The data generated has been then post-processed and evaluated with our set of [tools](https://github.com/robot-perception-group/GRADE_tools) against popular SLAM libraries, and used to test the realism your synthetic data.
With those tests we showed how many of these methods cannot recover from failures, and have highly degraded performance in dynamic environments even during very short sequences(60 seconds).
### In the wild Zebras observed by drones
We used the teleport capabilities of the system to generate both an **outdoor synthetic Zebra** datasets. The details are in the corresponding [Zebra](https://arxiv.org/abs/2305.00432) paper. The goal was to try to bridge the gap between simulation and reality and demonstrate that we can avoid tedious tasks such as precise data annotation.
Using a variety of environments from Unreal Engine and a freely available zebra model we were able to generate data realistic enough to obtain models trained from *scratch* that reached >90% accuracy on real world data.
_______
### Folder structure
<details closed>
<summary>A folder structure summary with comments of what is inside each folder</summary>
```bash
├── cp_local_to_diff_folder.sh # update code from/to isaac folder
├── irotate_specific # specific files used for simulate irotate in isaac sim and instructions
│ └── ...
├── isaac_internals # edited isaac files
│ ├── apps
│ │ └── omni.isaac.sim.python.kit # pre-load some additional extensions and disable a moveit (so that we can load the one from the system)
│ ├── kit # solve some bugs in the synthetic data processing
│ ├── exts
│ │ ├── omni.isaac.shapenet # slightly modified loader
│ │ ├── omni.isaac.synthetic_recorder # custom recorder extension that allows more control
│ │ └── omni.isaac.synthetic_utils # minor edits
│ └── setup_python_env.sh # source the ros environment and show how to source multiple ones
├── kill.sh # script to kill the whole simulation
├── req.sh # requirements file
├── scripts # useful scripts and additional accompanying stuff
│ └── ...
├── simulator # main simulator folder, each main file will have it's own description
│ ├── configs # yaml configuration files
│ ├── utils # utils loaded and used by the main files
│ └── ...
├── meshes # folder containing meshes
└── usds # usds files
```
</details closed>
___________________
## HowToS, Installation, Tips, and Known issues
The system, contrary to Gazebo, is not straightforward. This is the price you have to pay to be able to access low level APIs and have more control. We highly encourage thorugh readings of the documentation, of the tips section, and for you to get acquainted to the utils that we have organized (perhaps badly, open a pull request please).
[Install, StartUp, Issues](https://github.com/eliabntt/GRADE-RR/blob/main/HOWTO.md)
[Tips](https://github.com/eliabntt/GRADE-RR/blob/main/TipsAndTricks.md) --- highly encouraged reading!
To [generate people based on SMPL](https://github.com/eliabntt/animated_human_SMPL_to_USD), [convert environments/objects from Front3D or other files beforehand](https://github.com/eliabntt/Front3D_to_USD) and see a possible [control framework](https://github.com/eliabntt/ros_isaac_drone) (which can act thanks to our [custom 6DOF joint controller](https://github.com/eliabntt/custom_6dof_joint_controller)), please check our other repositories.
Additional scripts are provided [here](https://github.com/eliabntt/GRADE-RR/blob/main/scripts). Those can be used to process paths, get statistics of the rosbags, colorize the data filter and compress rosbags, transform the pixels to world coordinates etc.
A brief description of the utils libraries used in our code is [here](https://github.com/eliabntt/GRADE-RR/blob/main/simulator/utils/UTILS.md).
_____
## Isaac's edited files details
<details closed>
<summary>We had to edit some of the files to have more flexibility and solve some bugs. Here are reported details</summary>
Edited files are inside `isaac_internals`. The edited ones are the one that are copied by the `cp_local..` script. As per Isaac requirements, we had to include all the licenses and other files. Note that these might be outdated w.r.t. your current installation.
- _synthetic\_recorder_ created a custom extension to save our data, and offset the number of cameras. In that way we can save high-resolution images to the disk, while providing ROS smaller images. We found this faster than resizing images afterwards and caused less "issues".
- _synthetic\_utils_ we edited the `numpy.py` and the `syntheticdata.py` to save more data and have more flexibility. What is still missing (our bad) is the vertical fov of the camera, which is not directly exposed by Isaac Sim.
- In `setup_python_env.sh` we had to prevent the loading of `$SCRIPT_DIR/exts/omni.isaac.motion_planning/bin` (you can find it commented at the very end of line 8), to be able to run the system version of `move_base`. That module could be necessary for some of the Isaac extensions or configurations. Please be aware of this.
- `apps/omni.isaac.sim.python.kit` will load a couple of additional necessary extensions
- `isaac_internals/kit/extscore/omni.syntheticdata` will simply solve some bugs related to out of bounds and processing errors
</details closed>
______
## Download data
The data will be available in our [data repository](https://github.com/eliabntt/GRADE_data/).
__________
## Citations
You acknowledge that the Data & Software is a valuable scientific resource and agree to appropriately reference the following paper in any publication making use of the Data & Software.
Citation:
```
@misc{bonetto2023grade,
doi = {10.48550/ARXIV.2303.04466},
url = {https://arxiv.org/abs/2303.04466},
author = {Bonetto, Elia and Xu, Chenghao and Ahmad, Aamir},
title = {GRADE: Generating Realistic Animated Dynamic Environments for Robotics Research},
publisher = {arXiv},
year = {2023},
copyright = {arXiv.org perpetual, non-exclusive license}
}
```
Additionally:
- If you use any Data and/or Software related to zebras(animal) detection from drone imagery reference the following paper in any publication as well
```
@INPROCEEDINGS{10256293,
author={Bonetto, Elia and Ahmad, Aamir},
booktitle={2023 European Conference on Mobile Robots (ECMR)},
title={Synthetic Data-Based Detection of Zebras in Drone Imagery},
year={2023},
volume={},
number={},
pages={1-8},
doi={10.1109/ECMR59166.2023.10256293}}
}
```
- If you use any Data and/or Software related to our Dyanmic SLAM evaluations
```
@inproceedings{bonetto2023dynamicSLAM,
title={{S}imulation of {D}ynamic {E}nvironments for {SLAM}},
author={Elia Bonetto and Chenghao Xu and Aamir Ahmad},
booktitle={ICRA2023 Workshop on Active Methods in Autonomous Navigation},
year={2023},
url={https://arxiv.org/abs/2305.04286},
month = jun,
month_numeric = {6}
}
```
- If you use any Data and/or Software related to the tasks of detection/segmentation of humans in dynamic environments.
```
@inproceedings{bonetto2023learning,
title={Learning from synthetic data generated with {GRADE}},
author={Elia Bonetto and Chenghao Xu and Aamir Ahmad},
booktitle={ICRA2023 Workshop on Pretraining for Robotics (PT4R)},
year={2023},
url={https://openreview.net/forum?id=SUIOuV2y-Ce},
month = jun,
month_numeric = {6}
}
```
____________
## LICENSE
By downloading and/or using the Data & Software (including downloading, cloning, installing, and any other use of the corresponding github repository), you acknowledge that you have read these terms and conditions, understand them, and agree to be bound by them. If you do not agree with these terms and conditions, you must not download and/or use the Data & Software. Any infringement of the terms of this agreement will automatically terminate your rights under this License. Please read the [licensing](https://github.com/eliabntt/GRADE-RR/blob/main/LICENSE.md) agreement prior to any use of our Data or Software.
Accompanying software, such as, but not limited to, the one from Isaac Sim, is licensed according to their specific term of use.
If you use data/software from other projects such as, but not limited to, TUM RGB-D, 3D-Front, 3D-Future, ... it is your responsibility to follow their licensing terms, whose you implicitly agree.
If you have questions regarding the license, please contact the [[email protected]](mailto:[email protected]).
______
## Thanks
I would like to thank the amazing [NVIDIA support](http://forums.developer.nvidia.com) for their quick response times and precise answers.
[Chenghao Xu](http://kyle-xu-001.github.io/) for helping in testing and refining the evaluation scripts. [Aamir Ahmad](aamirahmad.de) for his supervision.
| 12,526 | Markdown | 61.949748 | 617 | 0.749481 |
eliabntt/GRADE-RR/LICENSE.md | # Data & Software Copyright License for non-commercial scientific research purposes
Please read carefully the following terms and conditions and any accompanying documentation before you download and/or use GRADE data, models, and software, (the "Data & Software"), including synthetic images and videos, SMPL and SMPL-X parameters, 3D body and clothing meshes, 2D textures, and scripts. By downloading and/or using the Data & Software (including downloading, cloning, installing, and any other use of the corresponding code repository), you acknowledge that you have read these terms and conditions, understand them, and agree to be bound by them. If you do not agree with these terms and conditions, you must not download and/or use the Data & Software. Any infringement of the terms of this agreement will automatically terminate your rights under this License
## Ownership / Licensees
The Data & Software and the associated materials have been developed at the Max Planck Institute for Intelligent Systems (hereinafter "MPI").
Any copyright or patent right is owned by and proprietary material of the Max-Planck-Gesellschaft zur Förderung der Wissenschaften e.V. (hereinafter “MPG”; MPI and MPG hereinafter collectively “Max-Planck”) hereinafter the “Licensor”.
## License Grant
Licensor grants you (Licensee) personally a single-user, non-exclusive, non-transferable, free of charge right:
To install the Data & Software on computers owned, leased or otherwise controlled by you and/or your organization;
To use the Data & Software for the sole purpose of performing non-commercial scientific research, non-commercial education, or non-commercial artistic projects;
Any other use, in particular any use for commercial, pornographic, military, or surveillance, purposes is prohibited. This includes, without limitation, incorporation in a commercial product, use in a commercial service, or production of other artifacts for commercial purposes. The Data & Software may not be used to create fake, libelous, misleading, or defamatory content of any kind excluding analyses in peer-reviewed scientific research. The Data & Software may not be reproduced, modified and/or made available in any form to any third party without Max-Planck’s prior written permission.
The Data & Software may not be used for pornographic purposes or to generate pornographic material whether commercial or not. This license also prohibits the use of the Data & Software to train methods/algorithms/neural networks/etc. for commercial, pornographic, military, surveillance, or defamatory use of any kind. By downloading the Data & Software, you agree not to reverse engineer it.
## No Distribution
The Data & Software and the license herein granted shall not be copied, shared, distributed, re-sold, offered for re-sale, transferre d or sub-licensed in whole or in part except that you may make one copy for archive purposes only.
## Disclaimer of Representations and Warranties
You expressly acknowledge and agree that the Data & Software results from basic research, is provided “AS IS”, may contain errors, and that any use of the Data & Software is at your sole risk. LICENSOR MAKES NO REPRESENTATIONS OR WARRANTIES OF ANY KIND CONCERNING THE DATA & SOFTWARE, NEITHER EXPRESS NOR IMPLIED, AND THE ABSENCE OF ANY LEGAL OR ACTUAL DEFECTS, WHETHER DISCOVERABLE OR NOT. Specifically, and not to limit the foregoing, licensor makes no representations or warranties (i) regarding the merchantability or fitness for a particular purpose of the Data & Software, (ii) that the use of the Data & Software will not infringe any patents, copyrights or other intellectual property rights of a third party, and (iii) that the use of the Data & Software will not cause any damage of any kind to you or a third party.
## Limitation of Liability
Because this Data & Software License Agreement qualifies as a donation, according to Section 521 of the German Civil Code (Bürgerliches Gesetzbuch – BGB) Licensor as a donor is liable for intent and gross negligence only. If the Licensor fraudulently conceals a legal or material defect, they are obliged to compensate the Licensee for the resulting damage.
Licensor shall be liable for loss of data only up to the amount of typical recovery costs which would have arisen had proper and regular data backup measures been taken. For the avoidance of doubt Licensor shall be liable in accordance with the German Product Liability Act in the event of product liability. The foregoing applies also to Licensor’s legal representatives or assistants in performance. Any further liability shall be excluded.
Patent claims generated through the usage of the Data & Software cannot be directed towards the copyright holders.
The Data & Software is provided in the state of development the licensor defines. If modified or extended by Licensee, the Licensor makes no claims about the fitness of the Data & Software and is not responsible for any problems such modifications cause.
## No Maintenance Services
You understand and agree that Licensor is under no obligation to provide either maintenance services, update services, notices of latent defects, or corrections of defects with regard to the Data & Software. Licensor nevertheless reserves the right to update, modify, or discontinue the Data & Software at any time.
Defects of the Data & Software must be notified in writing to the Licensor with a comprehensible description of the error symptoms. The notification of the defect should enable the reproduction of the error. The Licensee is encouraged to communicate any use, results, modification, or publication.
## Publications using the Data & Software
You acknowledge that the Data & Software is a valuable scientific resource and agree to appropriately reference the following paper in any publication making use of the Data & Software.
Citation:
```
@misc{bonetto2023grade,
doi = {10.48550/ARXIV.2303.04466},
url = {https://arxiv.org/abs/2303.04466},
author = {Bonetto, Elia and Xu, Chenghao and Ahmad, Aamir},
title = {GRADE: Generating Realistic Animated Dynamic Environments for Robotics Research},
publisher = {arXiv},
year = {2023},
copyright = {arXiv.org perpetual, non-exclusive license}
}
```
Additionally:
- If you use any Data and/or Software related to zebras(animal) detection from drone imagery reference the following paper in any publication as well
```
@inproceedings{bonetto2023synthetic,
title={Synthetic Data-based Detection of Zebras in Drone Imagery},
author={Elia Bonetto and Aamir Ahmad},
year={2023},
month = sep,
month_numeric = {9},
publisher = {IEEE},
url = {https://arxiv.org/abs/2305.00432},
booktitle = {2023 European Conference on Mobile Robots (ECMR 2023)},
note={To appear}
}
```
- If you use any Data and/or Software related to our Dyanmic SLAM evaluations
```
@inproceedings{bonetto2023dynamicSLAM,
title={{S}imulation of {D}ynamic {E}nvironments for {SLAM}},
author={Elia Bonetto and Chenghao Xu and Aamir Ahmad},
booktitle={ICRA2023 Workshop on Active Methods in Autonomous Navigation},
year={2023},
url={https://arxiv.org/abs/2305.04286},
month = jun,
month_numeric = {6}
}
```
- If you use any Data and/or Software related to the tasks of detection/segmentation of humans in dynamic environments.
```
@inproceedings{bonetto2023learning,
title={Learning from synthetic data generated with {GRADE}},
author={Elia Bonetto and Chenghao Xu and Aamir Ahmad},
booktitle={ICRA2023 Workshop on Pretraining for Robotics (PT4R)},
year={2023},
url={https://openreview.net/forum?id=SUIOuV2y-Ce},
month = jun,
month_numeric = {6}
}
```
## Commercial licensing opportunities
For commercial use of the Data & Software, please send emails to [email protected]
This Agreement shall be governed by the laws of the Federal Republic of Germany except for the UN Sales Convention.
| 8,214 | Markdown | 74.366972 | 826 | 0.758461 |
eliabntt/GRADE-RR/additional_scripts/average_rosbag.py | """
This is the code used to get the average acc speed and dynamic frames for the GRADE paper.
You need some experiment folders.
This code will use the bags files in those folder.
Please change the folders as desired (first loop in the code, first two lines).
We also suppose that you have the instance images to compute the percentage of dynamic frames.
"""
import rosbag
import sys
import numpy as np
import os
# loop through all the bags in the folder
folders = []
folders.append("/ps/project/irotate/GRADE-paper/Test.bak2/d94ecc9f-10f6-4f6d-b49f-1ed841f86772")
folders.append("/ps/project/irotate/GRADE-paper/Test.bak2/d8c14dd6-d794-46d5-aa59-01d3552828c7")
folders.append("/ps/project/irotate/GRADE-paper/Test.bak2/b13a4874-00a4-49a5-aa2d-e22d7d864b56")
folders.append("/ps/project/irotate/GRADE-paper/Test.bak2/75bf66e8-acb0-4f27-842d-1945ad42f9de")
folders.append("/ps/project/irotate/GRADE-paper/Test.bak2/53bfe530-122d-42cb-a1f4-453e6a2a617f")
folders.append("/ps/project/irotate/GRADE-paper/Test.bak2/23aae785-c0bc-4645-9e64-fdea78c42e2d")
folders.append("/ps/project/irotate/GRADE-paper/Test.bak2/b0a9c3c3-d470-45ea-82c6-ac529b6882ea")
folders.append("/ps/project/irotate/GRADE-paper/Test.bak2/12e463c1-4993-4ea8-9cbf-54ba9403e5f8")
names = ["d94ecc9f-10f6-4f6d-b49f-1ed841f86772","d8c14dd6-d794-46d5-aa59-01d3552828c7","b13a4874-00a4-49a5-aa2d-e22d7d864b56","75bf66e8-acb0-4f27-842d-1945ad42f9de","53bfe530-122d-42cb-a1f4-453e6a2a617f","23aae785-c0bc-4645-9e64-fdea78c42e2d","b0a9c3c3-d470-45ea-82c6-ac529b6882ea","12e463c1-4993-4ea8-9cbf-54ba9403e5f8"]
import pandas as pd
df = pd.DataFrame(columns=['name','speed','acc','dynamic_frames','dynamic_frames_avg_coverage'])
for folder in folders:
bag_folder = os.path.join(folder, "reindex_bags")
bags = []
for bag in os.listdir(bag_folder):
if bag.endswith(".bag"):
for n in names:
if n in bag:
bags.append(bag)
break
# sort bags according to the number
bags = sorted(bags, key=lambda x: int(x.split("_")[1].split(".")[0]))
avg_speed = [] # avg absolute speed per axis
avg_acc = [] # avg absolute acc per axis
for bagname in bags:
print(bagname)
# open the bag
bag = rosbag.Bag(os.path.join(bag_folder, bagname))
old_t = None
# loop through all the topics
for topic, msg, t in bag.read_messages(topics=['/my_robot_0/odom']):
# if the topic is the one we want
if topic == "/my_robot_0/odom":
# get the data
data_lin = np.array([msg.twist.twist.linear.x, msg.twist.twist.linear.y, msg.twist.twist.linear.z])
data_ang = np.array([msg.twist.twist.angular.x, msg.twist.twist.angular.y, msg.twist.twist.angular.z])
# get the speed
avg_speed.append([np.abs(data_lin[0]), np.abs(data_lin[1]), np.abs(data_lin[2]), np.abs(data_ang[0]), np.abs(data_ang[1]), np.abs(data_ang[2])])
# get the acceleration by using the difference between the current and the previous time
if old_t is None:
old_speed = [data_lin[0], data_lin[1], data_lin[2], data_ang[0], data_ang[1], data_ang[2]]
old_t = t
else:
# get the difference between the current and the previous time
dt = (t - old_t).to_sec()
# get the acceleration
avg_acc.append(np.abs(np.array(
[(data_lin[0] - old_speed[0]) / dt, (data_lin[1] - old_speed[1]) / dt, (data_lin[2] - old_speed[2]) / dt,
(data_ang[0] - old_speed[3]) / dt, (data_ang[1] - old_speed[4]) / dt, (data_ang[2] - old_speed[5]) / dt])))
# update the old speed and time
old_speed = [data_lin[0], data_lin[1], data_lin[2], data_ang[0], data_ang[1], data_ang[2]]
old_t = t
bag.close()
df = pd.concat([df, pd.DataFrame([[bagname[:-6], np.round(np.mean(avg_speed, axis=0),3), np.round(np.mean(avg_acc, axis=0),3), 0, 0]],
columns=df.columns)])
folders = []
folders.append("/ps/project/irotate/DE_few_obs_cam0_horiz/d94ecc9f-10f6-4f6d-b49f-1ed841f86772")
folders.append("/ps/project/irotate/DE_few_obs_cam0_horiz/d8c14dd6-d794-46d5-aa59-01d3552828c7")
folders.append("/ps/project/irotate/DE_cam0_horiz/b13a4874-00a4-49a5-aa2d-e22d7d864b56")
folders.append("/ps/project/irotate/DE_cam1/75bf66e8-acb0-4f27-842d-1945ad42f9de")
folders.append("/ps/project/irotate/DE_few_obs_cam1/53bfe530-122d-42cb-a1f4-453e6a2a617f")
folders.append("/ps/project/irotate/DE_lot_obs_cam0/23aae785-c0bc-4645-9e64-fdea78c42e2d")
import cv2
for folder in folders:
dynamic_images = 0
dynamic_coverage = 0
masks = os.path.join(folder, "Viewport0_occluded/instance")
for mask in os.listdir(masks):
if mask.endswith(".npy"):
f = np.load(os.path.join(masks, mask), allow_pickle=True)
classes = []
for item in f[1]:
if item[3] == "human" or item[3] == "google" or item[3] == "shapenet":
classes.append(item[0])
""" opencv reshape f[0] to (640, 480) """
img = cv2.resize(f[0].astype(np.uint16), (640, 480), interpolation=cv2.INTER_NEAREST)
out = np.isin(img, classes)
"""count the number of elements of img that are equal to an element of classes"""
if len(out[out==True]) > 0:
dynamic_coverage += len(out[out==True]) / img.size
dynamic_images += 1
df.loc[df["name"] == folder.split("/")[-1], "dynamic_frames"] = dynamic_images
df.loc[df["name"] == folder.split("/")[-1], "dynamic_frames_avg_coverage"] = round(dynamic_coverage / dynamic_images*100,2)
# print dataframe as latex table
print(df.to_latex(index=False))
df.to_pickle("dynamic_frames.pkl") | 5,411 | Python | 46.060869 | 321 | 0.697653 |
eliabntt/GRADE-RR/additional_scripts/colorize.py | """
Use this code to colorize the generated data.
The code is thought to colorize all the data, create videos, and fix the vertical fov issue.
Please check the arguments to understand how to use it.
Please set the corresponding data_enabled to False if you do not want to colorize some kind of data (eg. depth_enabled)
"""
import math
import argparse
import colorsys
import confuse
import copy
import cv2
import ipdb
import numpy as np
import os
import pickle as pkl
import random
from PIL import Image, ImageDraw
def project_pinhole(points, view_proj_matrix):
"""
Project 3D points to 2D camera view using a pinhole camera model.
Args:
points (numpy.ndarray): Array of points in world frame of shape (num_points, 3).
viewport (omni.kit.viewport._viewport.IViewportWindow): Viewport from which to retrieve/create sensor.
Returns:
(numpy.ndarray): Image-space points of shape (num_points, 3)
"""
homo = np.pad(points, ((0, 0), (0, 1)), constant_values=1.0)
tf_points = np.dot(homo, view_proj_matrix)
tf_points = tf_points / (tf_points[..., -1:])
tf_points[..., :2] = 0.5 * (tf_points[..., :2] + 1)
return tf_points[..., :3]
def random_colours(N, enable_random=True, num_channels=3):
"""
Generate random colors.
Generate visually distinct colours by linearly spacing the hue
channel in HSV space and then convert to RGB space.
"""
start = 0
if enable_random:
random.seed(10)
start = random.random()
hues = [(start + i / N) % 1.0 for i in range(N)]
colours = [list(colorsys.hsv_to_rgb(h, 0.9, 1.0)) for i, h in enumerate(hues)]
if num_channels == 4:
for color in colours:
color.append(1.0)
if enable_random:
random.shuffle(colours)
return colours
def colorize_bboxes(bboxes_2d_data, rgb, num_channels=4):
""" Colorizes 2D bounding box data for visualization.
Args:
bboxes_2d_data (numpy.ndarray): 2D bounding box data from the sensor.
rgb (numpy.ndarray): RGB data from the sensor to embed bounding box.
num_channels (int): Specify number of channels i.e. 3 or 4.
"""
obj_name_list = []
rgb_img = Image.fromarray(rgb).convert("RGBA")
rgb_img2 = Image.fromarray(rgb)
overlay = Image.new("RGBA", rgb_img.size, (0, 0, 0, 0))
rgb_img_draw = ImageDraw.Draw(overlay)
rgb_img_draw2 = ImageDraw.Draw(rgb_img2)
for bbox_2d in bboxes_2d_data:
obj_name_list.append(bbox_2d[1])
obj_name_list_np = np.unique(np.array(obj_name_list))
color_list = random_colours(len(obj_name_list_np.tolist()), True, num_channels)
for bbox_2d in bboxes_2d_data:
index = np.where(obj_name_list_np == bbox_2d[1])[0][0]
bbox_color = color_list[index]
outline = (int(255 * bbox_color[0]), int(255 * bbox_color[1]), int(255 * bbox_color[2]))
if num_channels == 4:
outline = (
int(255 * bbox_color[0]),
int(255 * bbox_color[1]),
int(255 * bbox_color[2]),
int(255 * bbox_color[3]),
)
fill = (
int(255 * bbox_color[0]),
int(255 * bbox_color[1]),
int(255 * bbox_color[2]),
int(0.25 * 255),
)
rgb_img_draw.rectangle([(bbox_2d[6], bbox_2d[7]), (bbox_2d[8], bbox_2d[9])], fill=fill, outline=outline, width=3)
rgb_img_draw2.rectangle([(bbox_2d[6], bbox_2d[7]), (bbox_2d[8], bbox_2d[9])], outline=outline, width=3)
bboxes_2d_rgb = Image.alpha_composite(rgb_img, overlay)
bboxes_2d_rgb = np.array(bboxes_2d_rgb)
bboxes_2d_rgb2 = np.array(rgb_img2)
bboxes_2d_rgb3 = np.array(Image.alpha_composite(rgb_img2.convert("RGBA"), overlay))
return bboxes_2d_rgb3 # , bboxes_2d_rgb2 #only boxes
def colorize_depth(depth_image):
"""
It takes a depth image, normalizes it, and then maps it to a color image
:param depth_image: The depth image to be colorized
:return: The colorized depth image.
"""
height, width = depth_image.shape[:2]
colorized_image = np.zeros((height, width, 4))
depth_image *= 100
depth_image = np.reciprocal(depth_image)
depth_image[depth_image == 0.0] = 1e-5
depth_image = np.clip(depth_image, 0, 255)
depth_image -= np.min(depth_image)
if np.max(depth_image) > 0:
depth_image /= np.max(depth_image) + 1e-8
colorized_image[:, :, 0] = depth_image
colorized_image[:, :, 1] = depth_image
colorized_image[:, :, 2] = depth_image
colorized_image[:, :, 3] = 1
colorized_image = (colorized_image * 255).astype(np.uint8)
return colorized_image
def colorize_semantic_from_instance(instance_image, instance_mappings, sem = False):
"""
It takes the instance image and the instance mappings and returns a colorized image
:param instance_image: the instance image from the instance segmentation
:param instance_mappings: a list of dictionaries, each of which has the following keys:
"""
if len(instance_mappings) == 0:
segmentation_image = np.zeros_like(instance_image)
segmentation_ids = np.unique(segmentation_image)
num_colours = len(segmentation_ids)
# This is to avoid generating lots of colours for semantic classes not in frame
lut = np.array([segmentation_ids, list(range(num_colours))])
re_instanced = lut[1, np.searchsorted(lut[0, :], segmentation_image)]
colours = np.array([[0.0] * 4] + random_colours(num_colours))
else:
semantic_instances = {}
changed = np.zeros(instance_image.shape)
for im in instance_mappings[::-1]:
semantic_instances.setdefault(im["semanticId"], []).extend(im["instanceIds"])
changed[instance_image == im["uniqueId"]] = max(im["instanceIds"])
instance_image = changed.astype(np.uint32)
max_semantic_instance_id = np.max([max(il) for _, il in semantic_instances.items()])
max_instance_id = instance_image.max()
lut = np.zeros(max(max_semantic_instance_id, max_instance_id) + 1, dtype=np.uint32)
if sem:
for i, (_, il) in enumerate(semantic_instances.items()):
lut[np.array(il)] = i + 1 # +1 to differentiate from background
re_instanced = np.take(lut, instance_image)
colours = np.array([[0.0] * 3] + random_colours(len(semantic_instances)))
else:
colours = np.array([[0.0] * 3] + random_colours(len(lut)))
re_instanced = instance_image
rgb = np.zeros((re_instanced.shape[0], re_instanced.shape[1], 3))
for i in range(len(colours)):
rgb[re_instanced == i] = colours[i]
rgb = rgb * 255
return rgb.astype(np.uint8)
def colorize_bboxes_3d(bboxes_3d_corners, rgb):
"""
> It takes a list of 3D bounding boxes and a RGB image, and returns the RGB image with the 3D bounding boxes drawn on it
:param bboxes_3d_corners: in the local camera frame
:param rgb: the image
:return: the image with the bounding boxes drawn on it.
"""
height, width = rgb.shape[:2]
# FILTER BOXES
mask_uv = ~np.any(np.all(bboxes_3d_corners < 0, axis=1), axis=1) & ~np.any(
np.all(bboxes_3d_corners > 1, axis=1), axis=1
)
mask_z = np.all(np.all(bboxes_3d_corners[..., 2:] >= 0, axis=1), axis=1) & np.all(
np.all(bboxes_3d_corners[..., 2:] <= 1, axis=1), axis=1
)
bboxes_3d_corners = bboxes_3d_corners[mask_uv & mask_z]
bboxes_3d_corners = bboxes_3d_corners[..., :2].reshape(-1, 8, 2) * np.array([[width, height]])
face_idx_list = [[0, 1, 3, 2], [4, 5, 7, 6], [2, 3, 7, 6], [0, 1, 5, 4], [0, 2, 6, 4], [1, 3, 7, 5]]
colours = random_colours(len(face_idx_list))
master_overlay_img = Image.new("RGBA", (width, height), (0, 0, 0, 0))
for face_idxs, colour in zip(face_idx_list, colours):
overlay = Image.new("RGBA", (width, height))
draw = ImageDraw.Draw(overlay)
colour = [int(c * 255) for c in colour]
for p in bboxes_3d_corners:
draw.polygon([tuple(xy) for xy in p[face_idxs]], fill=tuple([*colour[:3], 120]))
draw.line([tuple(xy) for xy in p[face_idxs]], width=3, fill=tuple(colour))
master_overlay_img = Image.alpha_composite(master_overlay_img, overlay)
rgb_img = Image.fromarray(rgb).convert("RGBA")
rgb_img = Image.alpha_composite(rgb_img, master_overlay_img)
return np.asarray(rgb_img)
def colorize_normals(normals):
"""
It takes a 3-channel array of normals, and returns a 4-channel array of normals with the background pixels set to
transparent
:param normals: a numpy array of shape (H, W, 3) containing the surface normals
:return: the normals of the image.
"""
background_mask = np.sum(normals, axis=-1) == 0.0
# normalize from [-1, 1] to [0, 255]
normals = (normals + 1.0) / 2 * 255
# Set background alpha to 0.
normals = np.pad(normals, ((0, 0), (0, 0), (0, 1)), constant_values=255)
normals[background_mask, 3] = 0.
return normals.astype(np.uint8)
def colorize_motion_vector(data):
"""Convert motion vector into colored image. The conversion is done by mapping
3D direction vector to HLS space, then converted to RGB.
Args:
data (numpy.array): data returned by the annotator of shape (H, W, 4).
Return:
(np.array): Data converted to uint8 RGBA image.
"""
r, theta, phi = _cartesian_to_spherical(data[:, :, :3])
phi += np.pi
theta_degree = theta * 180 / np.pi
phi_degree = phi * 180 / np.pi
h = phi_degree / 360
l = theta_degree / 180
r = cv2.normalize(r, None, 0, 1, cv2.NORM_MINMAX)
pixels = np.dstack((h * 180, l * 255, r * 255)).astype(np.uint8)
rgb = cv2.cvtColor(pixels, cv2.COLOR_HLS2RGB)
return rgb
def _cartesian_to_spherical(xyz):
"""
It takes a 3D Cartesian coordinate and returns the corresponding spherical coordinates
:param xyz: the 3D coordinates of the points in the image
"""
h, w = xyz.shape[0], xyz.shape[1]
xyz = xyz.reshape(-1, 3)
xy = xyz[:, 0] ** 2 + xyz[:, 1] ** 2
r = np.sqrt(xy + xyz[:, 2] ** 2)
theta = np.arctan2(np.sqrt(xy), xyz[:, 2]) # for elevation angle defined from Z-axis down
phi = np.arctan2(xyz[:, 1], xyz[:, 0]) # for elevation angle defined from XY-plane up
return r.reshape(h, w), theta.reshape(h, w), phi.reshape(h, w)
def boolean_string(s):
"""
It takes a string and returns a boolean
:param s: the string to convert
:return: The boolean value of the string.
"""
if s.lower() not in {'false', 'true'}:
raise ValueError('Not a valid boolean string')
return s.lower() == 'true'
parser = argparse.ArgumentParser(description="Colorize data")
parser.add_argument("--viewport_folder", type=str)
parser.add_argument("--img_id", type=str, default="-1")
parser.add_argument("--save_imgs", type=boolean_string, default=True)
parser.add_argument("--save_video", type=boolean_string, default=False)
parser.add_argument("--always_update_map", type=boolean_string, default=False)
parser.add_argument("--semantics", type=boolean_string, default=False)
parser.add_argument("--convert_depth", type=boolean_string, default=True) # used to better visualize inverse depth
parser.add_argument("--corrected_bbox_folder", type=str, default="")
parser.add_argument("--vertical_aperture", type=float, default=2.32)
parser.add_argument("--change_aperture", type=boolean_string, default=False)
parser.add_argument("--output_dir", type=str)
args, unknown = parser.parse_known_args()
config = confuse.Configuration("ColorizeData", __name__)
config.set_args(args)
minid = 1
maxid = 1801
isdigit = False
try:
int(config["img_id"].get())
isdigit = True
except:
isdigit = False
if isdigit:
img_id = int(config["img_id"].get())
if img_id <= -1:
print("Processing all images")
else:
minid = img_id
maxid = img_id + 1
ids = [i for i in range(minid, maxid)]
else:
ids = [config["img_id"].get()]
vertical_aperture = config["vertical_aperture"].get()
change_aperture = config["change_aperture"].get()
viewport = config["viewport_folder"].get()
subfolders = os.listdir(config["viewport_folder"].get())
depth_enabled = "depth" in subfolders
depthLinear_enabled = "depthLinear" in subfolders
normals_enabled = "normals" in subfolders
bbox2d_enabled = "bbox_2d_tight" in subfolders
bbox3d_enabled = "bbox_3d" in subfolders # todo these need to be fixed
instance_enabled = "instance" in subfolders
sem_enabled = "instance" in subfolders and config["semantics"].get()
motion_enabled = "motion-vector" in subfolders
always_update_map = config["always_update_map"].get()
save_video = config["save_video"].get()
save_img = config["save_imgs"].get()
if save_video or save_img:
outdir = config["output_dir"].get()
if not os.path.exists(config["output_dir"].get()):
os.makedirs(outdir)
if config["corrected_bbox_folder"].get() != "":
corrected_bbox_folder = config["corrected_bbox_folder"].get()
else:
corrected_bbox_folder = None
old_instance_map = None
vrgb, vdepth, vdepthLinear, vnormals, vbbox2d, vbbox3d, vinstance, vmotion, vsem = [], [], [], [], [], [], [], [], []
for i in ids:
rgb = cv2.imread(os.path.join(viewport, "rgb", f"{i}.png"))
if save_img:
cv2.imwrite(os.path.join(outdir, f"rgb_{i}.png"), rgb)
if save_video:
vrgb.append(os.path.join(outdir, f"rgb_{i}.png"))
if depthLinear_enabled:
depth = np.load(os.path.join(viewport, "depthLinear", f"{i}.npy"))
depth = colorize_depth(depth)
if save_img:
cv2.imwrite(os.path.join(outdir, f"depthLinear_{i}.png"), depth)
if save_video:
vdepthLinear.append(os.path.join(outdir, f"depthLinear_{i}.png"))
if depth_enabled:
depth = np.load(os.path.join(viewport, "depth", f"{i}.npy"))
if config["convert_depth"].get():
depth = 1/depth
depth = colorize_depth(depth)
if save_img:
cv2.imwrite(os.path.join(outdir, f"depth_{i}.png"), depth)
if save_video:
vdepth.append(os.path.join(outdir, f"depth_{i}.png"))
if normals_enabled:
normals = np.load(os.path.join(viewport, "normals", f"{i}.npy"))
normals = colorize_normals(normals)
if save_img:
cv2.imwrite(os.path.join(outdir, f"normals_{i}.png"), normals)
if save_video:
vnormals.append(os.path.join(outdir, f"normals_{i}.png"))
if bbox2d_enabled:
bbox2d = np.load(os.path.join(viewport, "bbox_2d_tight", f"{i}.npy"), allow_pickle=True)
rgb_data = copy.deepcopy(rgb)
bbox2d = colorize_bboxes(bbox2d, rgb_data)
if save_img:
cv2.imwrite(os.path.join(outdir, f"bbox2d_{i}.png"), bbox2d)
if save_video:
vbbox2d.append(os.path.join(outdir, f"bbox2d_{i}.png"))
if bbox3d_enabled:
bbox3d = np.load(os.path.join(viewport, "bbox_3d", f"{i}.npy"), allow_pickle=True)
viewport_mat = np.load(os.path.join(viewport, "camera", f"{i}.npy"), allow_pickle=True)
view_mat = viewport_mat.item()["view_projection_matrix"]
pose_mat = viewport_mat.item()["pose"]
if change_aperture:
viewproj_mat = np.dot(pose_mat, view_mat)
vertical_aperture = vertical_aperture
vfov = 2 * math.atan(vertical_aperture / (2 * viewport_mat.item()["focal_length"]))
viewproj_mat[1,1] = 1 / math.tan(vfov / 2)
viewproj_mat = np.dot(np.linalg.inv(pose_mat), viewproj_mat)
corners = project_pinhole(bbox3d["corners"].reshape(-1, 3), viewproj_mat)
corners = corners.reshape(-1, 8, 3)
rgb_data = copy.deepcopy(rgb)
e = []
for idx,bb in enumerate(bbox3d):
if bb['semanticLabel'] in ['zebra','human','google','shapenet']:
e.append(corners[idx])
if corrected_bbox_folder is not None:
corrected_bbox = np.load(os.path.join(corrected_bbox_folder, f"{i}.npy"), allow_pickle=True)
corrected_bbox = corrected_bbox.item()
for idx, bb in enumerate(bbox3d):
if bb[1] in corrected_bbox['bbox3d']:
print(f"Correcting bbox3d for {bb[1]}")
# if corrected_bbox['bbox3d'] is dictionary
if isinstance(corrected_bbox['bbox3d'][bb[1]], dict):
bbox3d[idx]["corners"] = corrected_bbox['bbox3d'][bb[1]]["oriented"] / 0.01
else:
bbox3d[idx]["corners"] = corrected_bbox['bbox3d'][bb[1]] / 0.01
bbox3d = colorize_bboxes_3d(np.array(e), rgb_data)
if save_img:
cv2.imwrite(os.path.join(outdir, f"bbox3d_{i}.png"), bbox3d)
if save_video:
vbbox3d.append(os.path.join(outdir, f"bbox3d_{i}.png"))
if instance_enabled:
instance = np.load(os.path.join(viewport, "instance", f"{i}.npy"), allow_pickle=True)
if old_instance_map is None or always_update_map:
old_instance_map = copy.deepcopy(instance[1])
instance[1] = copy.deepcopy(old_instance_map)
instance_img = colorize_semantic_from_instance(instance[0], instance[1])
if save_img:
cv2.imwrite(os.path.join(outdir, f"instance_{i}.png"), instance_img)
if save_video:
vinstance.append(os.path.join(outdir, f"instance_{i}.png"))
if sem_enabled:
sem = colorize_semantic_from_instance(instance[0], instance[1], sem=True)
if save_img:
cv2.imwrite(os.path.join(outdir, f"sem_{i}.png"), sem)
if save_video:
vsem.append(os.path.join(outdir, f"sem_{i}.png"))
if motion_enabled:
motion = np.load(os.path.join(viewport, "motion-vector", f"{i}.npy"), allow_pickle=True)
motion = colorize_motion_vector(motion)
if save_img:
cv2.imwrite(os.path.join(outdir, f"motion_{i}.png"), motion)
if save_video:
vmotion.append(os.path.join(outdir, f"motion_{i}.png"))
if save_video:
height, width, layers = rgb.shape
for v in zip([vrgb, vdepth, vdepthLinear, vnormals, vbbox2d, vbbox3d, vinstance, vmotion, vsem],
["rgb", "depth", "depthLinear", "normals", "bbox2d", "bbox3d", "instance", "motion", "sem"]):
if len(v[0]) > 0:
video = cv2.VideoWriter(os.path.join(outdir, f"{v[1]}.mp4"), cv2.VideoWriter_fourcc(*"mp4v"), 30, (width, height))
for img_path in v[0]:
img = cv2.imread(img_path)
if img.shape[2] < 3:
img = cv2.cvtColor(img, cv2.COLOR_GRAY2BGR)
video.write(img[:, :, :3])
video.release()
os.system("ffmpeg -i " + os.path.join(outdir, f"{v[1]}.mp4") + " -vcodec libx264 -y " + os.path.join(outdir,
f"{v[1]}_conv.mp4"))
| 17,948 | Python | 37.027542 | 127 | 0.651159 |
eliabntt/GRADE-RR/additional_scripts/pixel_to_world.py | """
This code serve as an example to project the points from the pixel coordinates to the world coordinates.
You need the camera pose and projection matrix, as well as clearly the pixel depth.
Those are available in the viewport folder, for example:
Viewport0/camera
Viewport0/depth (or depthLinear)
You will load the camera viewport_mat from the camera folder.
This dictionary will have the view projection matrix and the global camera pose
They use a near/far clipping plane model, and not a focal length model.
At the end of the file you can also check how to use the focal length model, but you need to know the focal length of the camera
"""
viewport_mat = np.load(os.path.join(viewport, 'camera',f'{i}.npy'), allow_pickle=True)
# in Isaac view_projection is np.dot(view_matrix, proj_matrix)
# view_matrix is local to world, i.e. the inverse of the pose matrix
# the proj_matrix use the near far clipping plane model
# a = -1.0 / np.tan(np.radians(fov / 2))
# b = -a * aspect_ratio
# c = z_far / (z_far - z_near)
# d = z_near * z_far / (z_far - z_near)
# Construct the camera projection matrix
# projection_matrix = np.array([
# [a, 0.0, 0.0, 0.0],
# [0.0, b, 0.0, 0.0],
# [0.0, 0.0, c, 1.0],
# [0.0, 0.0, d, 0.0]
# ])
view_mat = viewport_mat.item()["view_projection_matrix"]
pose_mat = viewport_mat.item()["pose"]
inv_VP = np.linalg.inv(view_mat)
pixel_x = ....
pixel_y = ....
pixel_d = ....
width = viewport_mat['resolution']['width']
width = viewport_mat['resolution']['height']
F = viewport_mat['clipping_range'][1]
N = viewport_mat['clipping_range'][0]
W = -pixel_d
ndc_x = (2 * pixel_x) / width - 1
ndc_y = 1 - (2 * pixel_y) / height
Z = ( (W*F/(F-N)) + N*F/(F-N) )/(W)
xyz = np.array([ndc_x, ndc_y, Z, 1]) * W
xyz = np.dot(xyz, inv_VP)
# alternatively consider that a = -fx, b = fy, cx = widht / 2, cy = height /2
# and that the pose_mat has the translation in the last ROW (in unit coordinates, so mind the scale)
tmp = np.dot(pose_mat, view_mat)
fx = -tmp[0,0]
fy = tmp[1,1]
cx = width / 2
cy = height / 2
x = (px - cx) * d / fx
y = (py - cy) * d / fy
pt = [x,y,z,1]
xyz = np.dot(cpose.T, pt)[:3]
| 2,131 | Python | 33.387096 | 128 | 0.656969 |
eliabntt/GRADE-RR/additional_scripts/check_folders.py | """
Use this to check if all the files/folders are there
"""
import os
import ipdb
mainpath = "/ps/project/irotate/"
folders = ["DE_lot_obs_cam0"]
tocheck = ["bbox_2d_loose","bbox_2d_tight","bbox_3d","camera","depthLinear","instance","poses","rgb"]
for mainfolder in folders:
for folder in os.listdir(os.path.join(mainpath, mainfolder)):
for subfolder in [os.path.join(mainpath, mainfolder, folder, "Viewport0"), os.path.join(mainpath, mainfolder, folder, "Viewport0_occluded")]:
print(subfolder)
data = os.listdir(subfolder)
if len(data) > len(tocheck):
print("More than expected folders")
print(subfolder)
ipdb.set_trace()
if len(data) < len(tocheck):
print("Less than expected folders")
print(subfolder)
ipdb.set_trace()
for f in data:
if f not in tocheck:
continue
if len(os.listdir(os.path.join(subfolder, f))) != 1801:
print("Not enough files in folder")
print(os.path.join(subfolder, f))
ipdb.set_trace()
| 991 | Python | 29.060605 | 143 | 0.672048 |
eliabntt/GRADE-RR/additional_scripts/process_paths/parser_config.yaml | cc_path: "../.." # set your cc_texture path
prefix_cc: ""
front3d_path: "../.." # set your global 3d_front path
prefix_front3d: ""
cloth3d_path: "../../.."
prefix_cloth3d: ""
surreal_path: "../.."
prefix_surreal: ""
normpath: True
| 235 | YAML | 15.857142 | 53 | 0.604255 |
eliabntt/GRADE-RR/additional_scripts/process_paths/change_paths.py | import argparse
import confuse
import os
def change_path(c_line, prefix, my_cc_path, match_str, normpath, remove_prefix=True):
if remove_prefix:
offset = len(match_str)
else:
offset = -1
path = os.path.join(my_cc_path + c_line[c_line.find(match_str) + offset:])
if normpath:
path = os.path.normpath(path[:path.rfind("@")].replace('\\',"/")) + path[path.rfind("@"):]
new_path = c_line[:c_line.find("@") + 1] + prefix + path
return new_path
parser = argparse.ArgumentParser(description="USD reference changer")
parser.add_argument("--config_file", type=str, default="parser_config.yaml")
parser.add_argument("--input", type=str)
parser.add_argument("--output_name", type=str, default="")
parser.add_argument("--output_dir", type=str, default="")
args, unknown = parser.parse_known_args()
config = confuse.Configuration("USDRefChanger", __name__)
config.set_file(args.config_file)
config.set_args(args)
filename = config["input"].get()
output_loc = config["output_dir"].get()
if output_loc == "":
output_loc = os.path.dirname(config["input"].get())
out_name = config["output_name"].get()
if out_name == "":
out_name = os.path.basename(config["input"].get())[:-4] + "_proc.usda"
else:
if out_name[-4:] != "usda":
out_name += ".usda"
out_file_path = os.path.join(output_loc, out_name)
prefix_cc = config["prefix_cc"].get()
my_cc_path = config["cc_path"].get()
prefix_3dfront = config["prefix_front3d"].get()
my_front_path = config["front3d_path"].get()
prefix_cloth3d = config["prefix_cloth3d"].get()
my_cloth_path = config["cloth3d_path"].get()
prefix_surreal = config["prefix_surreal"].get()
my_surr_path = config["surreal_path"].get()
normpath = config["normpath"].get()
with open(out_file_path, "w") as o_file, open(filename, "r") as i_file:
lines = i_file.readlines()
for line in lines:
c_line = line
if ".png" in line or ".jpg" in line or ".jpeg" in line or ".tga" in line or ".tif" in line or ".bmp" in line and "cc_textures" not in line:
# remove 3D-FUTURE-model
if "3D-FUTURE-model" in line:
# import ipdb; ipdb.set_trace()
c_line = line.replace("3D-FUTURE-model/", "")
if "cc_textures" not in line: # and "../../" in line:
# import ipdb; ipdb.set_trace()
# add after ../../ 3D-FUTURE-model
l_index = c_line.find("../../")
c_line = c_line[:l_index+6] + "3D-FUTURE-model/" + c_line[l_index+6:]
if "opacity_constant" in line or "reflection_roughness_constant" in line or "metallic_constant" in line:
tmp = c_line.split(" ")
tmp[-1] = tmp[-1].replace("\n", "")
if "int" in tmp:
tmp[tmp.index("int")] = "float"
if float(tmp[-1]) == 0:
tmp[-1] = str(0.00001)
try:
tmp[-1] = str(format(float(tmp[-1])))
except:
import ipdb; ipdb.set_trace()
c_line = " ".join(tmp)+"\n"
elif "cc_textures" in line:
c_line = change_path(c_line, prefix_cc, my_cc_path, "cc_textures", normpath, remove_prefix=False)
elif "3DFRONT" in line or "3D-FUTURE" in line:
if "future" in line.lower():
c_line = change_path(c_line, prefix_3dfront, my_front_path, "3D-FUTURE-model", normpath)
else:
import ipdb;
ipdb.set_trace()
c_line = change_path(c_line, prefix_3dfront, my_front_path, "3DFRONT", normpath)
elif "cloth3d" in line:
c_line = change_path(c_line, prefix_cloth3d, my_cloth_path, "cloth_3d", normpath)
elif "surreal" in line:
c_line = change_path(c_line, prefix_surreal, my_surr_path, "surreal", normpath)
o_file.write(c_line)
| 3,457 | Python | 34.285714 | 141 | 0.647961 |
eliabntt/GRADE-RR/additional_scripts/process_paths/README.md | Requirements:
Please install the following packages:
[OpenUSD](https://github.com/PixarAnimationStudios/OpenUSD)
These files are useful to automatically change some text in the USD files.
In short you will edit the `change_paths` script to your desire using python and the `parser_config.yaml` config file.
Then you can run `process_paths.sh` to process the USD file.
The processing work as follow: USD -> convert to USDA -> process -> convert back to USD
| 462 | Markdown | 34.615382 | 118 | 0.774892 |
eliabntt/GRADE-RR/simulator/people_and_objects.py | import argparse
import time
import os
import numpy as np
# base_env_path and other settings are in the config file
out_dir = "" # set this to a temporary empty dir
from omni.isaac.kit import SimulationApp
def boolean_string(s):
if s.lower() not in {'false', 'true'}:
raise ValueError('Not a valid boolean string')
return s.lower() == 'true'
parser = argparse.ArgumentParser(description="Your second IsaacSim run")
parser.add_argument("--headless", type=boolean_string, default=True, help="Wheter to run it in headless mode or not")
parser.add_argument("--rtx_mode", type=boolean_string, default=False, help="Use rtx when True, use path tracing when False")
parser.add_argument("--config_file", type=str, default="config.yaml")
parser.add_argument("--fix_env", type=str, default="",
help="leave it empty to have a random env, fix it to use a fixed one. Useful for loop processing")
args, unknown = parser.parse_known_args()
config = confuse.Configuration("world_and_robot", __name__)
config.set_file(args.config_file)
config.set_args(args)
CONFIG = {"display_options": 3286, "width": 1280, "height": 720, "headless": config["headless"].get()}
kit = SimulationApp(launch_config=CONFIG, experience=f"{os.environ['EXP_PATH']}/omni.isaac.sim.python.kit")
omni.usd.get_context().open_stage(config["base_env_path"].get(), None)
kit.update()
kit.update()
print("Loading stage...")
while is_stage_loading():
kit.update()
print("Loading Complete")
context = omni.usd.get_context()
stage = context.get_stage()
meters_per_unit = config["meters_per_unit"].get()
simulation_context = SimulationContext(physics_dt=1.0 / config["physics_hz"].get(), rendering_dt=1.0 / config["render_hz"].get(), stage_units_in_meters=meters_per_unit, backend='torch')
simulation_context.initialize_physics()
physx_interface = omni.physx.acquire_physx_interface()
physx_interface.start_simulation()
print("Adding ROS clock, you can check with rostopic echo /clock")
_clock_graph = add_clock()
simulation_context.play()
for _ in range(10):
simulation_context.step()
og.Controller.evaluate_sync(_clock_graph)
simulation_context.stop()
import utils.misc_utils
from utils.misc_utils import *
from utils.robot_utils import *
from utils.simulation_utils import *
from utils.objects_utils import *
from utils.human_utils import *
simulation_environment_setup(need_ros = True)
if base_world_path != "":
from utils.environment_utils import *
print("Loading environment...")
environment = environment(config, meters_per_unit=meters_per_unit)
env_prim_path = environment.load_and_center(config["env_prim_path"].get())
process_semantics(config["env_prim_path"].get())
print("Visualization...")
for _ in range(1000):
simulation_context.render()
simulation_context.step(render=False)
print("Environment loading done...")
add_colliders(env_prim_path)
print("Colliders added..")
simulation_context.play()
x, y, z = 0, 0, 0
if out_dir != "":
environment.generate_map(out_dir, origin=[x,y,z])
print("Map generated..")
simulation_context.stop()
ros_transform_components = []
camera_list = []
viewport_list = []
camera_pose, camera_pose_pub = [], []
imus,imu_pubs = [], []
lidars = []
odoms, odom_pubs = [], []
from omni.isaac.sensor import _sensor
_is = _sensor.acquire_imu_sensor_interface()
old_h_ape, old_v_ape = [], []
_dc = dynamic_control_interface()
print("Loading robots..")
robot_base_prim_path = config["robot_base_prim_path"].get()
usd_robot_path = str(config["usd_robot_path"].get())
for n in range(config["num_robots"].get()):
import_robot(robot_base_prim_path, n, usd_robot_path)
x, y, z, yaw = np.random.randint(-100,100,4)
set_drone_joints_init_loc(f"{robot_base_prim_path}{n}",
[x / meters_per_unit, y / meters_per_unit, z / meters_per_unit],
[0, 0, np.deg2rad(yaw)],
upper_zlim = z * 2,
lower_zlim = -z * 2
)
print("Adding ROS components")
add_ros_components(robot_base_prim_path, n, ros_transform_components, camera_list, viewport_list,
camera_pose, camera_pose_pub, imu_pubs, imus,
odoms, odom_pubs, lidars,
[], config, old_h_ape, old_v_ape, _is, simulation_context, _clock, irotate=False)
kit.update()
timeline = setup_timeline(config) # setup the timeline before adding anything animated
print("Loading people")
n = 0
human_base_prim_path = config["human_base_prim_path"].get()
while n < config["num_humans"].get():
folder = rng.choice(human_folders)
random_name = rng.choice(os.listdir(os.path.join(human_export_folder, folder)))
asset_path = os.path.join(human_export_folder, folder, random_name, random_name + ".usd")
print("Loading human {} from {}".format(random_name, folder))
tmp_pkl = pkl.load(open(os.path.join(human_export_folder, folder, random_name, random_name + ".pkl"), 'rb'))
used_ob_stl_paths.append(os.path.join(human_export_folder, folder, random_name, random_name + ".stl"))
load_human(human_base_prim_path, n, asset_path)
stl_path = os.path.join(human_export_folder, folder, random_name, random_name + ".stl")
x = np.random.randint(environment.env_limits_shifted[0], environment.env_limits_shifted[3])
y = np.random.randint(environment.env_limits_shifted[1], environment.env_limits_shifted[4])
z = 0
yaw = np.random.randint(0,360)
# position the mesh
set_translate(stage.GetPrimAtPath(f"{human_base_prim_path}{n}"),
[x / meters_per_unit, y / meters_per_unit, z / meters_per_unit])
set_scale(stage.GetPrimAtPath(f"{human_base_prim_path}{n}"), 1 / meters_per_unit)
set_rotate(stage.GetPrimAtPath(f"{human_base_prim_path}{n}"), [0, 0, np.deg2rad(yaw)])
n += 1
print("Load objects")
google_ob_used, shapenet_ob_used = load_objects(config, environment, np.random.default_rng(), [], 1/meters_per_unit)
if (config["rtx_mode"].get()):
set_raytracing_settings(config["physics_hz"].get())
else:
set_pathtracing_settings(config["physics_hz"].get())
print("Note that the rendering is now blocking until finished")
for i in range(100):
print(f"Iteration {i}/100", end="\r")
sleeping(simulation_context, viewport_list, raytracing=config["rtx_mode"].get())
# deselect all objects
omni.usd.get_context().get_selection().clear_selected_prim_paths()
omni.usd.get_context().get_selection().set_selected_prim_paths([], False)
timeline.set_current_time(0)
timeline.set_auto_update(False) # this no longer works as expected.
# Theoretically, once this is set and the timeline plays, rendering will not advance the timeline
# this is no longer the case. Thus, keep track of the ctime (as we do within sleeping function)
# the simulation context can be kept stopped, but that will prevent physics and time to advance.
# https://forums.developer.nvidia.com/t/the-timeline-set-auto-update-false-no-longer-works/253504/10
simulation_context.play()
for i in range(2000):
simulation_context.step(render=False)
og.Controller.evaluate_sync(_clock)
time.sleep(0.2)
simulation_context.render()
# publish IMU
print("Publishing IMU...")
pub_imu(_is, imu_pubs, robot_imu_frames, meters_per_unit)
if i % ratio_joints == 0:
for js in joint_states:
og.Controller.set(og.Controller.attribute(f"{js}/OnImpulseEvent.state:enableImpulse"), True)
if i % ratio_tf:
for tf in tf_trees:
og.Controller.set(og.Controller.attribute(f"{tf}/OnImpulseEvent.state:enableImpulse"), True)
if simulation_step % ratio_odom == 0:
c_pose, _ = pub_odom(odoms, odom_pubs, _dc, meters_per_unit)
pub_cam_pose(camera_pose, camera_pose_pub, _dc, meters_per_unit)
if simulation_step % ratio_camera == 0:
# The RTX LiDAR is still a fuzzy component. The "normal" LiDAR is more stable, but won't see non-colliding objects
for lidar in lidars:
og.Controller.attribute(lidar+".inputs:step").set(1)
ctime = timeline.get_current_time()
simulation_context.render()
timeline.set_current_time(ctime)
for lidar in lidars:
og.Controller.attribute(lidar+".inputs:step").set(0)
pub_and_write_images(simulation_context, viewport_list, ros_camera_list, raytracing) # clearly not writing anything here
timeline.forward_one_frame() # advancing the timeline
simulation_context.stop()
try:
kit.close()
except:
pass
| 8,219 | Python | 37.411215 | 185 | 0.709089 |
eliabntt/GRADE-RR/simulator/smpl_and_bbox.py | import argparse
import carb
import confuse
import ipdb
import math
import numpy as np
import os
import roslaunch
import rospy
import scipy.spatial.transform as tf
import sys
import time
import traceback
import trimesh
import yaml
from omni.isaac.kit import SimulationApp
from time import sleep
from omni.syntheticdata import sensors, helpers as sensors, generic_helper_lib
def get_obj_pose(time):
"""Get pose of all objects with a semantic label.
"""
stage = omni.usd.get_context().get_stage()
mappings = generic_helper_lib.get_instance_mappings()
pose = []
for m in mappings:
prim_path = m[1]
prim = stage.GetPrimAtPath(prim_path)
prim_tf = omni.usd.get_world_transform_matrix(prim, time)
pose.append((str(prim_path), m[2], str(m[3]), np.array(prim_tf)))
return pose
def boolean_string(s):
if s.lower() not in {'false', 'true'}:
raise ValueError('Not a valid boolean string')
return s.lower() == 'true'
"""
Exported information will have the shape of
[[prim_asset_path, bbox] [prim_asset_path,skel] [prim_asset_path, init_tf, init_rot]]
prim_asset_path is string of the asset in the simulation.
It will be processed in order so expect groups of human,cloth --- possibly reversed
All is output in WORLD frame. Please check the notes regarding projection in camera frame.
bbox will be of shape (ef, 8, 3) if only one bbox is saved or (ef, 2, 8, 3) if both are saved
ef will be either the last animated frame (given the simulated environment) or the last frame of the animations + 1
if you need to access the bbox of the mesh after that just use [-1]
skel is the smpl skeleton info
use the flags below to export only the skeleton, only the garments or only the body or any combination
init_rot is the same of the info file
init_tf is equal, except that here we account for the small vertical translation that is added to meshes very close to the ground
-- this was a bug during the data generation which actually has very little influence (< 0.1 cm in vertical displacement)
-- the design choice was to save the placement value and then have always a way to recover the eventual vertical displacement which is anyway based on a rule (check human_utils.py:move_humans_to_ground)
everything is in meters
NOTE: We start writing images from timeline.frame = 1 (1/fps) since the "forward_timeline" call has been placed _before_ the publishing
"""
try:
parser = argparse.ArgumentParser(description="Get Bounding Boxes")
parser.add_argument("--experiment_folder", type=str,
help="The experiment folder with the USD file and the info file")
parser.add_argument("--body", type=boolean_string, default=True, help="When true process the bodies")
parser.add_argument("--garments", type=boolean_string, default=True, help="When true process the garments")
parser.add_argument("--base_path", type=str, default="my_human_", help="Human prim base path")
parser.add_argument("--headless", type=boolean_string, default=False, help="Whether run this headless or not")
parser.add_argument("--write", type=boolean_string, default=True, help="Whether to write results")
parser.add_argument("--both", type=boolean_string, default=False,
help="Whether to write both vertex types -- preference in code is both - fast - slow")
parser.add_argument("--fast", type=boolean_string, default=True,
help="Whether to write only the axis-aligned box or the oriented one")
parser.add_argument("--only_exp", type=boolean_string, default=True,
help="Whether to export only the experiment (considering the reverse strategy) or the whole sequences")
parser.add_argument("--get_skel", type=boolean_string, default=True, help="Whether to include the skeleton info")
parser.add_argument("--skel_root", type=str, default="avg_root",
help="This is a recognizable last part of the root of the skeleton prim, in our case _avg_root "
+ "It will process ONLY the path of which the last part is this root")
parser.add_argument("--correct_poses", type=boolean_string, default=False)
args, unknown = parser.parse_known_args()
config = confuse.Configuration("BoundingBoxes", __name__)
config.set_args(args)
exp_info = np.load(os.path.join(config["experiment_folder"].get(), "experiment_info.npy"), allow_pickle=True)
exp_info = exp_info.item()
CONFIG = {"display_options": 3286, "width": 1280, "height": 720, "headless": config["headless"].get()}
kit = SimulationApp(launch_config=CONFIG, experience=f"{os.environ['EXP_PATH']}/omni.isaac.sim.python.kit")
import utils.misc_utils
from utils.misc_utils import *
from utils.robot_utils import *
from utils.simulation_utils import *
from utils.objects_utils import *
from utils.environment_utils import *
from utils.human_utils import *
simulation_environment_setup()
local_file_prefix = "my-computer://"
omni.usd.get_context().open_stage(local_file_prefix + config["experiment_folder"].get() + "/loaded_stage.usd", None)
kit.update()
kit.update()
print("Loading stage...")
while is_stage_loading():
kit.update()
print("Loading Complete")
context = omni.usd.get_context()
stage = context.get_stage()
set_stage_up_axis("Z")
simulation_context = SimulationContext(physics_dt=1.0 / exp_info["config"]["physics_hz"].get(),
rendering_dt=1.0 / exp_info["config"]["render_hz"].get(),
stage_units_in_meters=0.01)
simulation_context.start_simulation()
meters_per_unit = UsdGeom.GetStageMetersPerUnit(stage)
set_raytracing_settings(exp_info["config"]["physics_hz"].get())
timeline = setup_timeline(exp_info["config"])
base_path = config["base_path"].get()
fast, both, slow = False, False, False
if config["both"].get():
both = True
elif config["fast"].get():
fast = True
else:
slow = True
get_skel = config["get_skel"]
only_exp = config["only_exp"].get()
humans_info = exp_info["humans"]
write = config["write"].get()
if write:
results = []
stime = time.time()
helper_list_global = []
helper_list_skel = []
skel_root = config["skel_root"].get()
smpl_info_path = ""
for prim in stage.Traverse():
prim_path = str(prim.GetPath()).lower()
if base_path in prim_path:
if (get_skel and skel_root in prim_path and prim_path[:prim_path.find(skel_root)] not in helper_list_skel) or \
(str(prim.GetTypeName()).lower() == "mesh" and "points" in prim.GetPropertyNames()):
print(f"Processing {prim}")
parent = prim.GetParent()
refs = omni.usd.get_composed_references_from_prim(parent)
while len(refs) == 0:
parent = parent.GetParent()
refs = omni.usd.get_composed_references_from_prim(parent)
human_global_path = str(omni.usd.get_composed_references_from_prim(parent)[0][0].assetPath)
human_global_path = human_global_path[len(local_file_prefix):]
index = humans_info['folders'].index(human_global_path[:-3] + "stl")
init_tf = np.array(parent.GetAttribute("xformOp:translate").Get())
init_rot = parent.GetAttribute("xformOp:orient").Get()
init_rot = np.array([init_rot.GetImaginary()[0], init_rot.GetImaginary()[1], init_rot.GetImaginary()[2],
init_rot.GetReal()])
init_rot_mat = tf.Rotation.from_quat(init_rot).as_matrix()
if write and str(parent.GetPath()) not in helper_list_global:
results.append([str(parent.GetPath()), init_tf, init_rot])
helper_list_global.append(str(parent.GetPath()))
if human_global_path[:-3] + "pkl" != smpl_info_path:
smpl_info_path = human_global_path[:-3] + "pkl"
smpl_anim_info = pkl.load(open(smpl_info_path, 'rb'))
smpl_info = smpl_anim_info["info"]
r = smpl_info["zrot"]
rot_mat = tf.Rotation.from_euler('z', r).as_matrix()
ef = int(math.ceil(smpl_anim_info["ef"] * exp_info["config"]["fps"].get() / 24))
if only_exp:
ef = min(ef, int(math.ceil(
exp_info["config"]["experiment_length"].get() / exp_info['reversing_timeline_ratio'])))
if (get_skel and skel_root in prim_path):
helper_list_skel.append(prim_path[:prim_path.find(skel_root)])
skeleton, joint_token = AnimationSchema.SkelJoint(prim).GetJoint()
skel_cache = UsdSkel.Cache()
skel_query = skel_cache.GetSkelQuery(UsdSkel.Skeleton(skeleton.GetPrim()))
xfCache = UsdGeom.XformCache()
skeleton_info = np.empty((ef, 3), dtype=object)
for i in range(0, ef):
xfCache.SetTime(i)
transforms = skel_query.ComputeJointWorldTransforms(xfCache)
translates, rotations, scales = UsdSkel.DecomposeTransforms(transforms)
skeleton_info[i] = [np.array(translates) * meters_per_unit, np.array(rotations),
np.array(scales) * meters_per_unit]
if write:
results.append([str(prim.GetPath()), np.array(skeleton_info)])
else:
points = UsdGeom.PointBased(prim)
if both:
bounds = np.zeros((ef, 2, 8, 3))
else:
bounds = np.zeros((ef, 8, 3))
for i in range(0, ef):
points_in_mesh = points.ComputePointsAtTime(i, Usd.TimeCode(i))
points_in_mesh = np.array(points_in_mesh)
# bound = points.ComputeWorldBound(i, "default")
# for j in range(8):
# print(bound.ComputeAlignedRange().GetCorner(j))
points_in_mesh = ((points_in_mesh @ rot_mat.T @ init_rot_mat.T) + init_tf * meters_per_unit)
# normals = prim.GetAttribute("normals").Get(i)
# normals = np.array(normals)
mymesh = trimesh.PointCloud(points_in_mesh)
if fast:
temp_bounds = mymesh.bounding_box.vertices
elif slow:
temp_bounds = mymesh.bounding_box_oriented.vertices
elif both:
temp_bounds = [mymesh.bounding_box.vertices, mymesh.bounding_box_oriented.vertices]
bounds[i] = temp_bounds
if write:
results.append([str(prim.GetPath()), bounds])
results = np.array(results, dtype=object)
print(f"etime {time.time() - stime}")
if write:
np.save(os.path.join(config["experiment_folder"].get(), "bboxes.npy"), results)
except:
extype, value, tb = sys.exc_info()
traceback.print_exc()
import ipdb
ipdb.set_trace()
finally:
simulation_context.stop()
try:
kit.close()
except:
pass
| 10,213 | Python | 39.693227 | 202 | 0.684911 |
eliabntt/GRADE-RR/simulator/replay_experiment.py | import argparse
import carb
import confuse
import cv2
import ipdb
import math
import numpy as np
import os
import rosbag
import roslaunch
import rospy
import scipy.spatial.transform as tf
import sys
import time
import traceback
import trimesh
import yaml
from omni.isaac.kit import SimulationApp
from time import sleep
def boolean_string(s):
if s.lower() not in {'false', 'true'}:
raise ValueError('Not a valid boolean string')
return s.lower() == 'true'
"""
Suppose you want a stereo camera
And to have optical flow
And LiDAR (not fully supported yet) of the experiments.
This is a way in which you can re-process your info and get the results.
Suggestion: teleport is much more precise (sub mm difference). Working with velocities is fisy
This code is a bit hard-coded as it is a demonstration code.
"""
try:
parser = argparse.ArgumentParser(description="Get Bounding Boxes")
parser.add_argument("--experiment_folder", type=str,
help="The experiment folder with the USD file and the info file")
parser.add_argument("--headless", type=boolean_string, default=False, help="Whether run this headless or not")
parser.add_argument("--write", type=boolean_string, default=False, help="Whether to write new cameras results")
parser.add_argument("--write_flow", type=boolean_string, default=False, help="Whether to write optical flow")
parser.add_argument("--write_normals", type=boolean_string, default=False, help="Whether to write normals")
parser.add_argument("--use_teleport", type=boolean_string, default=False,
help="Whether to use teleport or force joint vel, both have adv and disadv")
parser.add_argument("--use_reindex", type=boolean_string, default=False, help="Whether to use reindexed bags")
parser.add_argument("--bag_basename", type=str, default="7659a6c9-9fc7-4be5-bc93-5b202ff2a22b")
parser.add_argument("--out_folder_npy", type=str, default='additional_data')
parser.add_argument("--bag_subpath", type=str, default="")
args, unknown = parser.parse_known_args()
config = confuse.Configuration("NewSensor", __name__)
config.set_args(args)
exp_info = np.load(os.path.join(config["experiment_folder"].get(), "experiment_info.npy"), allow_pickle=True)
exp_info = exp_info.item()
poses_path = os.path.join(config["experiment_folder"].get(), "Viewport0", "camera")
write_flow = config["write_flow"].get()
write_normals = config["write_normals"].get()
write = config["write"].get()
CONFIG = {"display_options": 3286, "width": 1280, "height": 720, "headless": config["headless"].get()}
kit = SimulationApp(launch_config=CONFIG, experience=f"{os.environ['EXP_PATH']}/omni.isaac.sim.python.kit")
import utils.misc_utils
from utils.misc_utils import *
from utils.robot_utils import *
from utils.simulation_utils import *
from utils.objects_utils import *
from utils.environment_utils import *
from utils.human_utils import *
simulation_environment_setup()
rospy.init_node("new_sensor_publisher", anonymous=True, disable_signals=True, log_level=rospy.ERROR)
local_file_prefix = "my-computer://"
omni.usd.get_context().open_stage(local_file_prefix + config["experiment_folder"].get() + "/loaded_stage.usd", None)
kit.update()
kit.update()
print("Loading stage...")
while is_stage_loading():
kit.update()
print("Loading Complete")
context = omni.usd.get_context()
stage = context.get_stage()
simulation_context = SimulationContext(physics_dt=1.0 / exp_info["config"]["physics_hz"].get(),
rendering_dt=1.0 / exp_info["config"]["render_hz"].get(),
stage_units_in_meters=0.01)
simulation_context.initialize_physics()
meters_per_unit = UsdGeom.GetStageMetersPerUnit(stage)
set_raytracing_settings(exp_info["config"]["physics_hz"].get())
timeline = setup_timeline(exp_info["config"])
reversing_timeline_ratio = exp_info['reversing_timeline_ratio']
experiment_length = exp_info['config']['experiment_length'].get()
ratio_camera = exp_info['config']['ratio_camera'].get()
cnt_reversal = 1
simulation_context.stop()
### here we add the new camera to the robot. It will be located 5 cm to the right w.r.t. the original one
old_h_ape = []
old_v_ape = []
viewport_window_list = []
ros_camera_list = []
# omni.kit.commands.execute('CopyPrim',
# path_from='/my_robot_0/camera_link/Camera',
# path_to='/my_robot_0/camera_link/Camera_stereo',
# exclusive_select=False)
# set_translate(stage.GetPrimAtPath('/my_robot_0/camera_link/Camera_stereo'), [1, 0, 0])
# component, viewport = add_camera_and_viewport("/my_robot_0/camera_link",
# exp_info["config"]["robot_sensor_size"].get(), old_h_ape, old_v_ape,
# simulation_context, 0, 0, camera_path="Camera_stereo")
# cam_outputs = control_camera(viewport, simulation_context)
# ros_camera_list.append([0, component, cam_outputs])
# viewport_window_list.append(viewport)
# omni.kit.commands.execute('CopyPrim',
# path_from='/my_robot_0/camera_link/Camera_npy',
# path_to='/my_robot_0/camera_link/Camera_npy_stereo',
# exclusive_select=False)
#
# set_translate(stage.GetPrimAtPath('/my_robot_0/camera_link/Camera_npy_stereo'), [1, 0, 0])
# viewport_npy, _ = create_viewport("/my_robot_0/camera_link/Camera_npy_stereo", config["headless"].get(),
# 0, exp_info["config"]["npy_sensor_size"].get(), old_h_ape, old_v_ape, simulation_context)
# viewport_window_list.append(viewport_npy)
viewport_npy, _ = create_viewport("/my_robot_0/camera_link/Camera_npy", config["headless"].get(),
0, exp_info["config"]["npy_sensor_size"].get(), old_h_ape, old_v_ape, simulation_context)
viewport_window_list.append(viewport_npy)
is_rtx = exp_info["config"]["rtx_mode"].get()
if is_rtx:
set_raytracing_settings(exp_info["config"]["physics_hz"].get())
else:
set_pathtracing_settings(exp_info["config"]["physics_hz"].get())
simulation_context.play()
for _ in range(5): simulation_context.render()
old_v_ape = [2.32] * len(old_v_ape) # todo this is harcoded
for index, cam in enumerate(viewport_window_list):
simulation_context.step(render=False)
simulation_context.render()
camera = stage.GetPrimAtPath(cam.get_active_camera())
camera.GetAttribute("horizontalAperture").Set(old_h_ape[index])
camera.GetAttribute("verticalAperture").Set(old_v_ape[index])
simulation_context.stop()
_clock_graph = add_clock() # add ROS clock
og.Controller.evaluate_sync(_clock_graph)
# add a new sensor
lidars = []
# sensor = add_lidar(f"/my_robot_0/yaw_link", [0, 0, -.1], [0, 0, 0], is_3d=True, is_2d=False)
# lidars.append(sensor)
kit.update()
cnt_tf = -1
use_teleport = config["use_teleport"].get()
use_reindex = config["use_reindex"].get()
id_bag = 0
bag_path = os.path.join(config["experiment_folder"].get(), config['bag_subpath'].get(),
f"{config['bag_basename'].get()}_{id_bag}.bag")
joint_order = ['x_joint', 'y_joint', 'z_joint', 'roll_joint', 'pitch_joint', 'yaw_joint']
joint_position = []
joint_velocity = []
joint_time = []
robot_pose = []
started = use_reindex
while os.path.exists(bag_path):
bag = rosbag.Bag(bag_path)
for topic, msg, t in bag.read_messages(
topics=["/my_robot_0/joint_states", "/my_robot_0/odom", "/starting_experiment"]):
if not started:
if topic == "/starting_experiment":
started = True
continue
else:
continue
if 'joint' in topic:
joint_position.append(msg.position)
joint_velocity.append(msg.velocity)
joint_time.append(msg.header.stamp)
else:
robot_pose.append([msg.pose.pose.position, msg.pose.pose.orientation])
id_bag += 1
bag_path = os.path.join(config["experiment_folder"].get(), config['bag_subpath'].get(),
f"{config['bag_basename'].get()}_{id_bag}.bag")
if len(joint_position) == 0:
print("No bag found")
sys.exit(-1)
ratio_tf = exp_info['config']['ratio_tf'].get()
init_x, init_y, init_z, init_roll, init_pitch, init_yaw = get_robot_joint_init_loc('/my_robot_0')
init_pos = np.array([init_x, init_y, init_z])
init_rot = np.array([init_roll, init_pitch, init_yaw])
change_collision_at_path(False,paths=['/my_robot_0/camera_link/Cube.physics:collisionEnabled','/my_robot_0/yaw_link/visuals.physics:collisionEnabled'])
kit.update()
set_drone_joints_init_loc('/my_robot_0', [0, 0, 0], [0,0,0], 300, lower_zlim=0) # todo use actual limit from simulation
kit.update()
simulation_context.play()
for _ in range(5):
simulation_context.step(render=False)
simulation_context.render()
timeline.set_auto_update(False)
timeline.set_current_time(min(- 1 / (exp_info['config']["physics_hz"].get() / ratio_camera),
-abs(exp_info['config']["bootstrap_exploration"].get())))
simulation_step = int(timeline.get_current_time() * exp_info['config']["physics_hz"].get()) - 1
out_dir_npy = os.path.join(config['experiment_folder'].get(), config['out_folder_npy'].get())
if write_flow:
_tmp = extension_custom.MyRecorder()
_tmp.on_startup()
_settings = _tmp.get_default_settings()
_settings["rgb"]["enabled"] = False
_settings["motion-vector"]["enabled"] = write_flow
_settings["motion-vector"]["colorize"] = False
_settings["motion-vector"]["npy"] = True
my_recorder_flow = recorder_setup(_settings, out_dir_npy, True, 0)
my_recorder_flow._enable_record = False
if write_normals:
_tmp = extension_custom.MyRecorder()
_tmp.on_startup()
_settings = _tmp.get_default_settings()
_settings["rgb"]["enabled"] = True
_settings["normals"]["enabled"] = write_normals
_settings["motion-vector"]["colorize"] = False
_settings["motion-vector"]["npy"] = True
my_recorder_normals = recorder_setup(_settings, out_dir_npy, True, 0)
my_recorder_normals._enable_record = False
if write:
_tmp = exp_info['config']['_recorder_settings'].get()
_tmp["depth"]["enabled"] = False
_tmp["depthLinear"]["enabled"] = False
_tmp["semantic"]["enabled"] = False
_tmp["normals"]["enabled"] = False
_tmp["bbox_2d_loose"]["enabled"] = False
_tmp["bbox_2d_tight"]["enabled"] = False
_tmp["bbox_3d"]["enabled"] = False
my_recorder = recorder_setup(_tmp, out_dir_npy, True, 0)
my_recorder._enable_record = False
# how to hide dynamic content
dynamicprims = []
for prim in stage.Traverse():
if 'my_human' in str(prim.GetPath()).lower():
dynamicprims.append(prim)
for prim in stage.GetPrimAtPath("/World").GetChildren()[6:]:
dynamicprims.append(prim)
toggle_dynamic_objects(dynamicprims, False)
forward = True
while kit.is_running():
simulation_step += 1
if simulation_step == 0:
_dc = dynamic_control_interface()
handle = _dc.get_rigid_body('/my_robot_0/yaw_link')
if not use_teleport:
art = _dc.get_articulation('/my_robot_0')
joints = []
_dc.wake_up_articulation(art)
for joint in joint_order:
joints.append(_dc.find_articulation_dof(art, joint))
change_collision_at_path(True,paths=['/my_robot_0/camera_link/Cube.physics:collisionEnabled','/my_robot_0/yaw_link/visuals.physics:collisionEnabled'])
og.Controller.evaluate_sync(_clock_graph)
# since the first image generated is at time=1/30, we add 7/240
prev_time = timeline.get_current_time() + 7 / 240 * (simulation_step == 0)
timeline.set_current_time(prev_time)
simulation_step += 8
sleeping(simulation_context, viewport_window_list, is_rtx)
try:
if write:
my_recorder._update()
my_recorder._enable_record = True
if write_flow:
my_recorder_flow._update()
my_recorder_flow._enable_record = True
if write_normals:
my_recorder_normals._update()
my_recorder_normals._enable_record = True
except:
sleeping(simulation_context, viewport_window_list, is_rtx)
if write:
my_recorder._update()
my_recorder._enable_record = True
if write_flow:
my_recorder_flow._update()
my_recorder_flow._enable_record = True
if write_normals:
my_recorder_normals._update()
my_recorder_normals._enable_record = True
simulation_context.render()
simulation_context.render()
timeline.set_current_time(prev_time)
if simulation_step < 0:
simulation_context.step(render=False)
if (simulation_step % ratio_camera == 0):
timeline.forward_one_frame()
continue
if use_teleport:
if simulation_step % ratio_tf == 0:
cnt_tf += 1
teleport("/my_robot_0", np.array(joint_position[cnt_tf][:3]) / meters_per_unit + init_pos
, tf.Rotation.from_euler('XYZ', joint_position[cnt_tf][3:] + init_rot).as_quat())
if (simulation_step % (ratio_tf * 2) == 0): # odm is published half the rate of the tf
myp = _dc.get_rigid_body_pose(handle)
print(
f"pose diff {np.array(_dc.get_rigid_body_pose(handle).p) / 100 - np.array([robot_pose[int(cnt_tf / 2)][0].x, robot_pose[int(cnt_tf / 2)][0].y, robot_pose[int(cnt_tf / 2)][0].z])}")
else:
vel = np.array(joint_velocity[
cnt_tf]) # or average position between the two, or use the IMU to interpolate also which has 240 hz
pos = (np.array(joint_position[cnt_tf][:3]) + vel[:3] * 1 / 240) / meters_per_unit + init_pos
ori = (np.array(joint_position[cnt_tf][3:]) + vel[3:] * 1 / 240) + init_rot
teleport("/my_robot_0", pos, tf.Rotation.from_euler('XYZ', ori).as_quat())
else:
_dc.wake_up_articulation(art)
if simulation_step % ratio_tf == 0:
cnt_tf += 1
vel = np.array(joint_velocity[cnt_tf])
next_vel = vel
if cnt_tf < len(joint_position) - 1:
next_vel = np.array(joint_velocity[cnt_tf + 1])
if cnt_tf == 0:
pos = np.append(np.array(joint_position[cnt_tf][:3]) / meters_per_unit + init_pos - vel[:3] * 1 / 240,
joint_position[cnt_tf][3:] + init_rot - vel[3:] * 1 / 240)
for idx, joint in enumerate(joints):
_dc.set_dof_position(joint, pos[idx] * (-1 if idx == 1 else 1))
cvel = (vel + next_vel) / 2
cvel[:3] = cvel[:3] / meters_per_unit
_dc.set_articulation_dof_velocity_targets(art, list(cvel))
for idx, joint in enumerate(joints):
_dc.set_dof_velocity(joint, cvel[idx] * (-1 if idx == 1 else 1))
if (simulation_step % (ratio_tf * 2) == 0):
myp = _dc.get_rigid_body_pose(handle)
print(
f"pose diff {np.array(_dc.get_rigid_body_pose(handle).p) / 100 - np.array([robot_pose[int(cnt_tf / 2)][0].x, robot_pose[int(cnt_tf / 2)][0].y, robot_pose[int(cnt_tf / 2)][0].z])}")
if simulation_step % 8 == 0:
# tmp = np.load(
# f'/ps/project/irotate/GRADE_DATA/DE/7659a6c9-9fc7-4be5-bc93-5b202ff2a22b/Viewport0/camera/{int(simulation_step/8)}.npy',
# allow_pickle=True).item()
prim_tf = omni.usd.get_world_transform_matrix(stage.GetPrimAtPath('/my_robot_0/camera_link/Camera'))
# in v2022 this is the only viable option to control time since timeline.set_auto_update=False is not working
timeline.set_current_time(prev_time + 1 / 240 * (1 if forward else -1))
prev_time = timeline.get_current_time()
simulation_context.step(render=False)
simulation_context.render()
print("Clocking...")
# NOTE THAT THIS MIGHT GET CONFUSING -- reindexing/retiming is needed for sure. Tests need to be careful!
og.Controller.evaluate_sync(_clock_graph)
if simulation_step == 0:
og.Controller.evaluate_sync(_clock_graph)
time.sleep(0.2)
if simulation_step % ratio_camera == 0:
if (simulation_step + ratio_camera) / ratio_camera < (experiment_length / reversing_timeline_ratio) * (
cnt_reversal):
forward = True
else:
if (simulation_step + ratio_camera) / ratio_camera >= ((experiment_length - 1) / reversing_timeline_ratio) * (
cnt_reversal + 1) or \
(timeline.get_current_time() - 1 / timeline.get_time_codes_per_seconds()) < 0:
cnt_reversal += 2
forward = True
else:
forward = False
if write_flow:
if my_recorder_flow._enable_record:
simulation_context.render()
my_recorder_flow._counter += 1
time.sleep(1.5) # this seems necessary
my_recorder_flow._update()
# you have two ways to proceed here. the sleeping performs just the rendering and then you manually toggle the recorder below
# otherwise use pub_and_write_images which automatically calls it if necessary. In the latter case, remember to increase the counter
sleeping(simulation_context, viewport_window_list, is_rtx)
# if write:
# if my_recorder._enable_record:
# my_recorder._counter += 1
# pub_and_write_images(simulation_context, viewport_window_list, ros_camera_list, is_rtx, my_recorder)
if write:
if my_recorder._enable_record:
my_recorder._counter += 1
my_recorder._update()
if write_normals:
if my_recorder_normals._enable_record:
my_recorder_normals._counter += 1
my_recorder_normals._update()
# new sensor here -- imagine 30 fps -- in that case I need to publish
# if you need sensors in the middle you need to interpolate
# using IMU and TF readings
# you can access those from the rosbags
# note you might need to work with the timeline times if the rate that you want is different
# if simulation_step % ratio_camera == 0:
# for lidar in lidars:
# og.Controller.attribute(lidar + ".inputs:step").set(1)
# ctime = timeline.get_current_time()
# simulation_context.render()
# # point_cloud = og.Controller().node("/Render/PostProcess/SDGPipeline/RenderProduct_Replicator_RtxSensorCpuIsaacComputeRTXLidarPointCloud").get_attribute("outputs:pointCloudData").get()
# # laser_scan = og.Controller().node("/Render/PostProcess/SDGPipeline/RenderProduct_Replicator_RtxSensorCpuIsaacComputeRTXLidarFlatScan").get_attribute("outputs:linearDepthData").get()
# timeline.set_current_time(ctime)
# for lidar in lidars:
# og.Controller.attribute(lidar+".inputs:step").set(0)
if simulation_step % ratio_camera == 0 and simulation_step / ratio_camera == experiment_length:
print("End of experiment!!!")
simulation_context.pause()
break
except:
extype, value, tb = sys.exc_info()
traceback.print_exc()
import ipdb
ipdb.set_trace()
finally:
simulation_context.stop()
try:
kit.close()
except:
pass
| 18,401 | Python | 40.26009 | 190 | 0.668062 |
eliabntt/GRADE-RR/simulator/zebra_datagen.py | import argparse
import carb
import confuse
import ipdb
import numpy as np
import os
import sys
import time
import traceback
import yaml
from omni.isaac.kit import SimulationApp
from time import sleep
def boolean_string(s):
if s.lower() not in {'false', 'true'}:
raise ValueError('Not a valid boolean string')
return s.lower() == 'true'
def compute_points(skel_root_path, prim, ef, stage):
usdSkelRoot = UsdSkel.Root.Get(stage, skel_root_path)
UsdSkel.BakeSkinning(usdSkelRoot, Gf.Interval(0, ef))
prim = UsdGeom.PointBased(prim)
xformCache = UsdGeom.XformCache()
final_points = np.zeros((ef, len(prim.GetPointsAttr().Get()), 3))
for prim in Usd.PrimRange(usdSkelRoot.GetPrim()):
if prim.GetTypeName() != "Mesh":
continue
localToWorld = xformCache.GetLocalToWorldTransform(prim)
for time in range(ef):
points = UsdGeom.Mesh(prim).GetPointsAttr().Get(time)
for index in range(len(points)):
points[index] = localToWorld.Transform(points[index])
points = np.array(points)
final_points[time] = points
return final_points
def randomize_floor_position(floor_data, floor_translation, scale, meters_per_unit, env_name, rng):
floor_points = np.zeros((len(floor_data), 3))
if env_name == "Windmills":
yaw = np.deg2rad(-155)
rot = np.array([[np.cos(yaw), -np.sin(yaw), 0], [np.sin(yaw), np.cos(yaw), 0], [0, 0, 1]])
floor_translation = np.matmul(floor_translation, rot)
if env_name == "L_Terrain":
meters_per_unit = 1
for i in range(len(floor_data)):
floor_points[i, 0] = floor_data[i][0] * scale[0] * meters_per_unit + floor_translation[0] * meters_per_unit
floor_points[i, 1] = floor_data[i][1] * scale[1] * meters_per_unit + floor_translation[1] * meters_per_unit
floor_points[i, 2] = floor_data[i][2] * scale[2] * meters_per_unit + floor_translation[2] * meters_per_unit
if env_name == "L_Terrain":
meters_per_unit = 0.01
max_floor_x = max(floor_points[:, 0])
min_floor_x = min(floor_points[:, 0])
max_floor_y = max(floor_points[:, 1])
min_floor_y = min(floor_points[:, 1])
if env_name == "Windmills":
min_floor_x = -112
max_floor_x = 161
min_floor_y = -209
max_floor_y = 63
rows = np.where((floor_points[:, 0] > min_floor_x) & (floor_points[:, 0] < max_floor_x) & (floor_points[:, 1] > min_floor_y) & (floor_points[:, 1] < max_floor_y))[0]
floor_points = floor_points[rows]
rows = []
while (len(rows) == 0):
size_x = rng.integers(40, 120)
size_y = rng.integers(40, 120)
# get all floor_points within a size x size square randomly centered
min_x = rng.uniform(min(floor_points[:, 0]), max(floor_points[:, 0]))
max_x = min_x + min(size_x, max(floor_points[:, 0]) - min(floor_points[:, 0]))
while max_x > max(floor_points[:, 0]):
min_x = rng.uniform(min(floor_points[:, 0]), max(floor_points[:, 0]))
max_x = min_x + min(size_x, max(floor_points[:, 0]) - min(floor_points[:, 0]))
min_y = rng.uniform(min(floor_points[:, 1]), max(floor_points[:, 1]))
max_y = min_y + min(size_y, max(floor_points[:, 1]) - min(floor_points[:, 1]))
while max_y > max(floor_points[:, 1]):
min_y = rng.uniform(min(floor_points[:, 1]), max(floor_points[:, 1]))
max_y = min_y + min(size_y, max(floor_points[:, 1]) - min(floor_points[:, 1]))
# FIXME this is just an approximation which MAY NOT WORK ALWAYS!
rows = np.where((min_x <= floor_points[:,0]) & (floor_points[:,0] <= max_x) & (floor_points[:,1]<=max_y) & (floor_points[:,1]>= min_y))[0]
floor_points = floor_points[rows]
shape = (len(np.unique(floor_points[:, 0])), -1, 3)
floor_points = floor_points.reshape(shape)
if (floor_points[0, 1, 0] - floor_points[0, 0, 0]) > 1:
zoom_factor = int(floor_points[0, 1, 0] - floor_points[0, 0, 0])
import scipy.ndimage.interpolation as interpolation
floor_points = interpolation.zoom(floor_points, (zoom_factor, zoom_factor, 1))
return floor_points, max_floor_x, min_floor_x, max_floor_y, min_floor_y
try:
parser = argparse.ArgumentParser(description="Dynamic Worlds Simulator")
parser.add_argument("--config_file", type=str, default="config.yaml")
parser.add_argument("--headless", type=boolean_string, default=True, help="Wheter to run it in headless mode or not")
parser.add_argument("--rtx_mode", type=boolean_string, default=False,
help="Use rtx when True, use path tracing when False")
parser.add_argument("--record", type=boolean_string, default=False, help="Writing data to the disk")
parser.add_argument("--debug_vis", type=boolean_string, default=False,
help="When true continuosly loop the rendering")
parser.add_argument("--neverending", type=boolean_string, default=False, help="Never stop the main loop")
parser.add_argument("--fix_env", type=str, default="",
help="leave it empty to have a random env, fix it to use a fixed one. Useful for loop processing")
args, unknown = parser.parse_known_args()
config = confuse.Configuration("DynamicWorlds", __name__)
config.set_file(args.config_file)
config.set_args(args)
can_start = True
CONFIG = {"display_options": 3286, "width": 1280, "height": 720, "headless": config["headless"].get()}
kit = SimulationApp(launch_config=CONFIG, experience=f"{os.environ['EXP_PATH']}/omni.isaac.sim.python.kit")
# Cannot move before SimApp is launched
import utils.misc_utils
from utils.misc_utils import *
from utils.robot_utils import *
from utils.simulation_utils import *
from utils.environment_utils import *
from pxr import UsdGeom, UsdLux, Gf, Vt, UsdPhysics, PhysxSchema, Usd, UsdShade, Sdf, UsdSkel
simulation_environment_setup(need_ros=False)
all_env_names = ["Bliss", "Forest", "Grasslands", "Iceland", "L_Terrain", "Meadow",
"Moorlands", "Nature_1", 'Nature_2', "Savana", "Windmills", "Woodland"]
ground_area_name = ["Landscape_1", "Landscape_1", "Landscape_1", "Landscape_0", "Terrain_5", "Landscape_0",
"Landscape_2", "Ground", "Ground", "Landscape_1", "Landscape_0", "Landscape_1"]
need_sky = [True] * len(all_env_names)
env_id = all_env_names.index(config["fix_env"].get())
rng = np.random.default_rng()
rng_state = np.random.get_state()
local_file_prefix = ""
# setup environment variables
environment = environment(config, rng, local_file_prefix)
out_dir = os.path.join(config['out_folder'].get(), environment.env_name)
out_dir_npy = os.path.join(config['out_folder_npy'].get(), environment.env_name)
if not os.path.exists(out_dir):
os.makedirs(out_dir)
omni.usd.get_context().open_stage(local_file_prefix + config["base_env_path"].get(), None)
# Wait two frames so that stage starts loading
kit.update()
kit.update()
print("Loading stage...")
while is_stage_loading():
kit.update()
print("Loading Complete")
context = omni.usd.get_context()
stage = context.get_stage()
set_stage_up_axis("Z")
omni.kit.commands.execute("DeletePrimsCommand", paths=["/World/GroundPlane"])
# do this AFTER loading the world
simulation_context = SimulationContext(physics_dt=1.0 / config["physics_hz"].get(),
rendering_dt=1.0 / config["render_hz"].get(),
stage_units_in_meters=0.01)
simulation_context.initialize_physics()
simulation_context.play()
simulation_context.stop()
kit.update()
meters_per_unit = 0.01
# use rtx while setting up!
set_raytracing_settings(config["physics_hz"].get())
env_prim_path = environment.load_and_center(config["env_prim_path"].get())
process_semantics(config["env_prim_path"].get(), "World")
if all_env_names[env_id] == "L_Terrain":
set_scale(stage.GetPrimAtPath(f"/World/home"), 100)
while is_stage_loading():
kit.update()
floor_data = stage.GetPrimAtPath(f"/World/home/{ground_area_name[env_id]}/{ground_area_name[env_id]}").GetProperty(
'points').Get()
floor_translation = np.array(stage.GetPrimAtPath(f"/World/home/{ground_area_name[env_id]}").GetProperty(
'xformOp:translate').Get())
scale = np.array(stage.GetPrimAtPath(f"/World/home/{ground_area_name[env_id]}").GetProperty("xformOp:scale").Get())
# i need to consider that z has a bounding box and that the position is on the top corner
for _ in range(50):
simulation_context.render()
floor_points, max_floor_x, min_floor_x, max_floor_y, min_floor_y = randomize_floor_position(floor_data,
floor_translation, scale,
meters_per_unit, all_env_names[env_id], rng)
add_semantics(stage.GetPrimAtPath("/World/home"), "world")
# set timeline of the experiment
timeline = setup_timeline(config)
viewport_window_list = []
dynamic_prims = []
first = True
simulation_context.stop()
simulation_context.play()
for _ in range(10):
simulation_context.step()
_dc = dynamic_control_interface()
print("Loading robots..")
robot_base_prim_path = config["robot_base_prim_path"].get()
usd_robot_path = str(config["usd_robot_path"].get())
old_h_ap = []
old_v_ap = []
simulation_context.stop()
for n in range(config["num_robots"].get()):
import_robot(robot_base_prim_path, n, usd_robot_path, local_file_prefix)
change_prim_collision(False, robot_base_prim_path + str(n))
set_drone_joints_init_loc(robot_base_prim_path + str(n), [0, 0, 0], [0, 0, 0], 10e15)
kit.update()
for n in range(config["num_robots"].get()):
add_npy_viewport(viewport_window_list, robot_base_prim_path, n, old_h_ap, old_v_ap, config,simulation_context, tot_num_ros_cam=0)
kit.update()
for _ in range(5):
simulation_context.render()
for index, cam in enumerate(viewport_window_list):
camera = stage.GetPrimAtPath(cam.get_active_camera())
camera.GetAttribute("horizontalAperture").Set(old_h_ap[index])
camera.GetAttribute("verticalAperture").Set(old_v_ap[index])
print("Loading robot complete")
print("Loading zebras..")
zebra_anims_loc = config["zebra_anims_loc"].get()
# get a list of .usd file in the folder
import glob
zebra_files = glob.glob(f"{zebra_anims_loc}/*.usd")
from utils.zebra_utils import *
from omni.kit.window.sequencer.scripts import sequencer_drop_controller
_, sequence = omni.kit.commands.execute("SequencerCreateSequenceCommand")
sequence_path = sequence.GetPrim().GetPath()
kit.update()
zebra_anim_names = ["Attack", "Attack01", "Attack02", "Eating", "Gallop", "Hit_Back", "Hit_Front", "Hit_Left",
"Hit_Right", "Idle", "Idle2", "Idle3", "Idle4", "Jump", "Tarsus", "Trot", "Walkback"]
zebra_seq_lengths = [27, 54, 32, 133, 12, 15, 17, 20, 15, 48, 72, 119, 201, 43, 29, 24, 27]
zebra_mesh_paths = [
"Attack/Zebra_motions/African_Animal___Zebra/_Object_Pivot_Node_/Object_6/Object_45/Zebra_SHP2_0_Zebra_Mat_0_001",
"Attack01/Zebra_motions/African_Animal___Zebra/_Object_Pivot_Node_/Object_6/Object_45/Zebra_SHP2_0_Zebra_Mat_0_001",
"Attack02/Zebra_motions/African_Animal___Zebra/_Object_Pivot_Node_/Object_6/Object_45/Zebra_SHP2_0_Zebra_Mat_0_001",
"Eating/Zebra_motions/African_Animal___Zebra/_Object_Pivot_Node_/Object_6/Object_45/Zebra_SHP2_0_Zebra_Mat_0",
"Gallop/Zebra_motions/African_Animal___Zebra/_Object_Pivot_Node_/Object_6/Object_45/Zebra_SHP2_0_Zebra_Mat_0",
"Hit_Back/Zebra_motions/African_Animal___Zebra/_Object_Pivot_Node_/Object_6/Object_45/Zebra_SHP2_0_Zebra_Mat_0",
"Hit_Front/Zebra_motions/African_Animal___Zebra/_Object_Pivot_Node_/Object_6/Object_45/Zebra_SHP2_0_Zebra_Mat_0",
"Hit_Left/Zebra_motions/African_Animal___Zebra/_Object_Pivot_Node_/Object_6/Object_45/Zebra_SHP2_0_Zebra_Mat_0",
"Hit_Right/Zebra_motions/African_Animal___Zebra/_Object_Pivot_Node_/Object_6/Object_45/Zebra_SHP2_0_Zebra_Mat_0",
"Idle/Zebra_motions/African_Animal___Zebra/_Object_Pivot_Node_/Object_6/Object_45/Zebra_SHP2_0_Zebra_Mat_0",
"Idle2/Zebra_motions/African_Animal___Zebra/_Object_Pivot_Node_/Object_6/Object_45/Zebra_SHP2_0_Zebra_Mat_0",
"Idle3/Zebra_motions/African_Animal___Zebra/_Object_Pivot_Node_/Object_6/Object_45/Zebra_SHP2_0_Zebra_Mat_0",
"Idle4/Zebra_motions/African_Animal___Zebra/_Object_Pivot_Node_/Object_6/Object_45/Zebra_SHP2_0_Zebra_Mat_0",
"Jump/Zebra_motions/African_Animal___Zebra/_Object_Pivot_Node_/Object_6/Object_45/Zebra_SHP2_0_Zebra_Mat_0",
"Tarsus/Zebra_motions/African_Animal___Zebra/_Object_Pivot_Node_/Object_6/Object_45/Zebra_SHP2_0_Zebra_Mat_0",
"Trot/Zebra_motions/African_Animal___Zebra/_Object_Pivot_Node_/Object_6/Object_45/Zebra_SHP2_0_Zebra_Mat_0",
"Walkback/Zebra_motions/African_Animal___Zebra/_Object_Pivot_Node_/Object_6/Object_45/Zebra_SHP2_0_Zebra_Mat_0"]
zebra_info = {}
for i, v in enumerate(zebra_anim_names):
zebra_info[v] = {"path": zebra_mesh_paths[i], "length": zebra_seq_lengths[i], "mesh_path": zebra_mesh_paths[i]}
for zebra_file in zebra_files:
if not os.path.exists(zebra_file[:-4] + "_points.npy"):
zebra_name = zebra_file.split("/")[-1].split(".")[0]
zebra_index = zebra_anim_names.index(zebra_name)
zebra_path = load_zebra("/zebra_", zebra_index, zebra_file)
kit.update()
kit.update()
zebra_name = zebra_file.split("/")[-1].split(".")[0]
zebra_index = zebra_anim_names.index(zebra_name)
prim = stage.GetPrimAtPath(zebra_path + zebra_mesh_paths[zebra_index][len(zebra_name):])
skel_root_path = zebra_path + "/Zebra_motions/African_Animal___Zebra/_Object_Pivot_Node_/Object_6"
points = compute_points(skel_root_path, prim, zebra_seq_lengths[zebra_index], stage) * meters_per_unit
np.save(zebra_file[:-4] + "_points.npy", points)
zebra_info[zebra_name]["points"] = points
omni.kit.commands.execute("DeletePrimsCommand", paths=[zebra_path])
else:
zebra_name = zebra_file.split("/")[-1].split(".")[0]
zebra_index = zebra_anim_names.index(zebra_name)
zebra_info[zebra_name]["points"] = np.load(zebra_file[:-4] + "_points.npy")
max_anim_length = max(zebra_seq_lengths)
# IT IS OF CRUCIAL IMPORTANCE THAT AFTER THIS POINT THE RENDER GETS DONE WITH THE SLEEPING CALL! OTHERWISE PATH TRACING SPP WILL GET RUINED
if (config["rtx_mode"].get()):
set_raytracing_settings(config["physics_hz"].get())
else:
set_pathtracing_settings(config["physics_hz"].get())
omni.usd.get_context().get_selection().set_selected_prim_paths([], False)
for _ in range(5):
simulation_context.step(render=False)
sleeping(simulation_context, viewport_window_list, config["rtx_mode"].get())
timeline.set_current_time(0)
simulation_step = 0 # this is NOT the frame, this is the "step" (related to physics_hz)
my_recorder = recorder_setup(config['_recorder_settings'].get(), out_dir_npy, config['record'].get(), 0)
timeline.set_current_time(0) # set to 0 to be sure that the first frame is recorded
timeline.set_auto_update(False)
# two times, this will ensure that totalSpp is reached
sleeping(simulation_context, viewport_window_list, config["rtx_mode"].get())
sleeping(simulation_context, viewport_window_list, config["rtx_mode"].get())
my_recorder._enable_record = False
exp_len = config["anim_exp_len"].get()
my_recorder._enable_record = False
sleeping(simulation_context, viewport_window_list, config["rtx_mode"].get())
if config["rtx_mode"].get():
my_recorder._update()
hidden_position = [min_floor_x / meters_per_unit, min_floor_y / meters_per_unit, -10e5]
all_zebras = preload_all_zebras(config, rng, zebra_files, zebra_info, simulation_context, sequencer_drop_controller,
max_anim_length, hidden_position)
substep = 3
simulation_context.play()
import ipdb; ipdb.set_trace()
while kit.is_running():
if simulation_step > 0:
for zebra in all_zebras:
set_translate(stage.GetPrimAtPath(zebra), list(hidden_position))
floor_points, max_floor_x, min_floor_x, max_floor_y, min_floor_y = randomize_floor_position(floor_data,
floor_translation,
scale,
meters_per_unit,
all_env_names[env_id], rng)
frame_info = place_zebras(all_zebras, rng, floor_points, meters_per_unit, hidden_position, config, max_anim_length,
zebra_info)
for c_substep in range(substep):
average_zebra_x = 0
average_zebra_y = 0
average_zebra_z = 0
max_zebra_x = -1e10
max_zebra_y = -1e10
min_zebra_x = 1e10
min_zebra_y = 1e10
counter = 0
for prim in frame_info:
if "zebra" in prim:
average_zebra_x += frame_info[prim]["position"][0]
average_zebra_y += frame_info[prim]["position"][1]
average_zebra_z += frame_info[prim]["position"][2]
max_zebra_x = max(max_zebra_x, frame_info[prim]["position"][0])
max_zebra_y = max(max_zebra_y, frame_info[prim]["position"][1])
min_zebra_x = min(min_zebra_x, frame_info[prim]["position"][0])
min_zebra_y = min(min_zebra_y, frame_info[prim]["position"][1])
counter += 1
average_zebra_x /= counter
average_zebra_y /= counter
average_zebra_z /= counter
delta_x = max_zebra_x - min_zebra_x
delta_y = max_zebra_y - min_zebra_y
used_x = []
used_y = []
used_z = []
for n in range(config["num_robots"].get()):
safe = False
while not safe:
# -100 + 100
random_x = rng.uniform(average_zebra_x - delta_x/2 - 5, average_zebra_x + delta_x/2 + 5)
# keep random_x within max_floor_x min_floor_x
random_x = max(random_x, min_floor_x)
random_x = min(random_x, max_floor_x)
random_y = rng.uniform(average_zebra_y - delta_y/2 -5, average_zebra_y + delta_y/2 + 5)
# keep random_y within max_floor_y min_floor_y
random_y = max(random_y, min_floor_y)
random_y = min(random_y, max_floor_y)
random_z = rng.uniform(average_zebra_z + 5, average_zebra_z + 20)
if len(used_x) > 0:
for i in range(len(used_x)):
safe = True
if np.sqrt((used_x[i] - random_x) ** 2 + (used_y[i] - random_y) ** 2 + (used_z[i] - random_z) ** 2) < .5:
safe = False
break
else:
safe = True
if safe:
used_x.append(random_x)
used_y.append(random_y)
used_z.append(random_z)
# get angle between robot and average_zebra
angle = np.arctan2(average_zebra_y - random_y, average_zebra_x - random_x)
# randomize yaw +- 30 degrees
yaw = rng.uniform(-np.pi / 6, np.pi / 6) + angle
# randomize yaw +- 15 degrees
yaw = rng.uniform(-np.pi / 12, np.pi / 12) + angle
# get pitch + 15 degrees (camera already pitched)
# with a weight based on the average zebra location
pitch = - np.arctan2(average_zebra_z - random_z, np.sqrt(
(average_zebra_x - random_x) ** 2 + (average_zebra_y - random_y) ** 2))
# roll minimal -10, 10 degrees
roll = rng.uniform(-np.pi / 18, np.pi / 18)
rot = Rotation.from_euler('xyz', [roll, pitch, yaw])
teleport(robot_base_prim_path + str(n),
[random_x / meters_per_unit, random_y / meters_per_unit, random_z / meters_per_unit],
rot.as_quat())
frame_info[f"{robot_base_prim_path}{n}"] = {"position": [random_x, random_y, random_z],
"rotation": [roll, pitch, yaw]}
simulation_context.step(render=False)
simulation_context.step(render=False)
for _ in range(3):
simulation_context.step(render=False)
simulation_context.render()
sleep(0.5)
# two frames with the same animation point
# todo fix the time
import ipdb;
ipdb.set_trace()
timeline.set_current_time(max_anim_length / timeline.get_time_codes_per_seconds())
if need_sky[env_id]:
# with probability 0.9 during day hours
stage.GetPrimAtPath("/World/Looks/SkyMaterial/Shader").GetAttribute("inputs:SunPositionFromTOD").Set(True)
if rng.uniform() < 0.9:
stage.GetPrimAtPath("/World/Looks/SkyMaterial/Shader").GetAttribute("inputs:TimeOfDay").Set(
rng.uniform(5, 20))
else:
if rng.uniform() < 0.5:
stage.GetPrimAtPath("/World/Looks/SkyMaterial/Shader").GetAttribute("inputs:TimeOfDay").Set(
rng.uniform(0, 5))
else:
stage.GetPrimAtPath("/World/Looks/SkyMaterial/Shader").GetAttribute("inputs:TimeOfDay").Set(
rng.uniform(20, 24))
print("Publishing cameras...")
my_recorder._enable_record = True
frame_info["step"] = simulation_step
frame_info["substep"] = c_substep
pub_try_cnt = 0
success_pub = False
while not success_pub and pub_try_cnt < 3:
try:
pub_and_write_images(simulation_context, viewport_window_list, [],
config["rtx_mode"].get(), my_recorder)
success_pub = True
except:
print("Error publishing camera")
pub_try_cnt += 1
import ipdb; ipdb.set_trace()
# simulation_context.stop()
# simulation_context.play()
sleep(0.5)
simulation_context.render()
simulation_context.render()
if not success_pub:
frame_info["error"] = True
else:
frame_info["error"] = False
np.save(out_dir_npy + f"/frame_{simulation_step}_{c_substep}.npy", frame_info)
simulation_context.stop()
# clips = [f"/World/Sequence{k}{k}_Clip" for k in frame_info.keys() if k.startswith("/zebra")]
# remove targets from clips
# for clip in clips:
# relationship = stage.GetPrimAtPath(clip).GetProperty("animation")
# relationship.RemoveTarget(relationship.GetTargets()[0])
# relationship = stage.GetPrimAtPath(clip).GetProperty("assetPrim")
# asset = relationship.GetTargets()[0]
# relationship.RemoveTarget(asset)
# omni.kit.commands.execute("DeletePrimsCommand",
# paths=clips)
# omni.kit.commands.execute("DeletePrimsCommand",
# paths=
# [f"/World/Sequence{k}" for k in frame_info.keys() if k.startswith("/zebra")])
# omni.kit.commands.execute("DeletePrimsCommand", paths=[k for k in frame_info.keys() if k.startswith("/zebra")])
timeline.set_current_time(0)
my_recorder._counter += 1
simulation_step += 1
if simulation_step >= exp_len:
break
except:
extype, value, tb = sys.exc_info()
traceback.print_exc()
ipdb.post_mortem(tb)
finally:
simulation_context.stop()
try:
kit.close()
except:
pass
| 22,410 | Python | 40.88972 | 167 | 0.65328 |
eliabntt/GRADE-RR/simulator/FUEL_indoor_simulation.py | import argparse
import carb
import confuse
import ipdb
import numpy as np
import os
import roslaunch
import rospy
import sys
import time
import traceback
import yaml
from omni.isaac.kit import SimulationApp
from time import sleep
def boolean_string(s):
if s.lower() not in {'false', 'true'}:
raise ValueError('Not a valid boolean string')
return s.lower() == 'true'
try:
parser = argparse.ArgumentParser(description="Dynamic Worlds Simulator")
parser.add_argument("--config_file", type=str, default="config.yaml")
parser.add_argument("--headless", type=boolean_string, default=True, help="Wheter to run it in headless mode or not")
parser.add_argument("--rtx_mode", type=boolean_string, default=False,
help="Use rtx when True, use path tracing when False")
parser.add_argument("--record", type=boolean_string, default=True, help="Writing data to the disk")
parser.add_argument("--debug_vis", type=boolean_string, default=False,
help="When true continuosly loop the rendering")
parser.add_argument("--neverending", type=boolean_string, default=False, help="Never stop the main loop")
parser.add_argument("--fix_env", type=str, default="",
help="leave it empty to have a random env, fix it to use a fixed one. Useful for loop processing")
args, unknown = parser.parse_known_args()
config = confuse.Configuration("DynamicWorlds", __name__)
config.set_file(args.config_file)
config.set_args(args)
os.environ["SHAPENET_LOCAL_DIR"] = config["shapenet_local_dir"].get()
experiment_length = config["experiment_length"].get()
can_start = True
CONFIG = {"display_options": 3286, "width": 1280, "height": 720, "headless": config["headless"].get()}
kit = SimulationApp(launch_config=CONFIG, experience=f"{os.environ['EXP_PATH']}/omni.isaac.sim.python.kit")
# Cannot move before SimApp is launched
import utils.misc_utils
from utils.misc_utils import *
from utils.robot_utils import *
from utils.simulation_utils import *
from utils.objects_utils import *
from utils.environment_utils import *
from utils.human_utils import *
def monitor_movement(msg, args):
global second_start
global last_check_time
global c_pose
global old_pose
global rng
global env_prim_path
wait_time = rospy.Duration(1)
index, environment = args[0], args[1]
if second_start and rospy.Time.now() > last_check_time + wait_time:
last_check_time = rospy.Time.now()
diff_x = abs(old_pose[index][0] - c_pose[index][0]) ** 2
diff_y = abs(old_pose[index][1] - c_pose[index][1]) ** 2
diff_z = abs(old_pose[index][2] - c_pose[index][2]) ** 2
dist = (diff_x + diff_y + diff_z) ** 0.5
if (dist) < 0.1:
my_pose = PoseStamped()
if (rng.uniform() > .9):
x, y, z, yaw = position_object(environment, type=0)
x = x[0]
y = y[0]
z = z[0]
yaw = yaw[0] + rng.uniform(0, 2 * np.pi)
else:
yaw = get_robot_yaw(c_pose[index][0], c_pose[index][1], c_pose[index][2],
environment.env_mesh, environment.shifts)
x = c_pose[index][0] + 0.2 * np.cos(yaw)
y = c_pose[index][1] + 0.2 * np.sin(yaw)
z = c_pose[index][2]
yaw += rng.uniform(0, 2 * np.pi)
my_pose.pose.position.x = x
my_pose.pose.position.y = y
my_pose.pose.position.z = z
rot = np.array(yaw) * 180 / np.pi
quat = (
Gf.Rotation(Gf.Vec3d.XAxis(), 0)
* Gf.Rotation(Gf.Vec3d.YAxis(), 0)
* Gf.Rotation(Gf.Vec3d.ZAxis(), rot)
).GetQuat()
my_pose.pose.orientation.x = quat.imaginary[0]
my_pose.pose.orientation.y = quat.imaginary[1]
my_pose.pose.orientation.z = quat.imaginary[2]
my_pose.pose.orientation.w = quat.real
print(
f"Publishing random goal since robot {index} stuck [{x},{y},{z}, {yaw} ({yaw * 180 / 3.14})].")
my_pose.header.frame_id = "world"
my_pose.header.stamp = rospy.Time.now()
movement_monitor_pubs[index].publish(my_pose)
if (dist) < 0.05:
set_colliders(env_prim_path, True)
else:
old_pose[index] = c_pose[index]
set_colliders(env_prim_path, True)
def autostart_exploration(msg, index):
global first_start
global second_start
global can_start
global can_change_second_start
global last_pub_time
if (msg.data == "PUB_FIRST_360"):
can_change_second_start = True
wait_time = rospy.Duration(0, 500000000) if second_start else rospy.Duration(1)
if (msg.data == "WAIT_TRIGGER" or (
msg.data == "PUB_360" and not second_start) and rospy.Time.now() > last_pub_time + wait_time):
if can_start:
if not first_start:
first_start = True
elif can_change_second_start:
second_start = True
print("Exploration will start at the end of this movement")
default_pose = PoseStamped()
default_pose.header.frame_id = "world"
default_pose.header.stamp = rospy.Time.now()
start_explorer_pubs[index].publish(default_pose)
last_pub_time = rospy.Time.now()
def publish_random_goal(msg, args):
global last_pub_time
global first_start
global second_start
global can_start
global can_change_second_start
index, environment = args[0], args[1]
if (msg.data == "PUB_FIRST_360"):
can_change_second_start = True
if (msg.data == "WAIT_TRIGGER" or (
msg.data == "PUB_360" and not second_start) and rospy.Time.now() > last_pub_time + rospy.Duration(0,
500000000)):
if can_start:
if not first_start:
first_start = True
elif can_change_second_start:
second_start = True
my_pose = PoseStamped()
x, y, z, yaw = position_object(environment, type=0)
my_pose.pose.position.x = x[0]
my_pose.pose.position.y = y[0]
my_pose.pose.position.z = z[0]
rot = np.array(yaw[0]) * 180 / np.pi
quat = (
Gf.Rotation(Gf.Vec3d.XAxis(), 0)
* Gf.Rotation(Gf.Vec3d.YAxis(), 0)
* Gf.Rotation(Gf.Vec3d.ZAxis(), rot)
).GetQuat()
my_pose.pose.orientation.x = quat.imaginary[0]
my_pose.pose.orientation.y = quat.imaginary[1]
my_pose.pose.orientation.z = quat.imaginary[2]
my_pose.pose.orientation.w = quat.real
print(f"Publishing random goal [{x[0]},{y[0]},{z[0]}, {yaw[0]} ({yaw[0] * 180 / 3.14})] for robot {index}")
my_pose.header.frame_id = "fixing_manual"
my_pose.header.stamp = rospy.Time.now()
send_waypoint_pubs[index].publish(my_pose)
last_pub_time = rospy.Time.now()
simulation_environment_setup()
# set timeline of the experiment
timeline = setup_timeline(config)
rospy.init_node("my_isaac_ros_app", anonymous=True, disable_signals=True, log_level=rospy.ERROR)
starting_pub = rospy.Publisher('starting_experiment', String)
rng = np.random.default_rng()
rng_state = np.random.get_state()
local_file_prefix = "" # if something is broken try my-computer://
# setup environment variables
meters_per_unit = config["meters_per_unit"].get()
environment = environment(config, rng, local_file_prefix, meters_per_unit)
uuid = roslaunch.rlutil.get_or_generate_uuid(None, False)
out_dir = os.path.join(config['out_folder'].get(), environment.env_name)
out_dir_npy = os.path.join(config['out_folder_npy'].get(), environment.env_name)
if not os.path.exists(out_dir):
os.makedirs(out_dir)
os.environ["ROS_LOG_DIR"] = out_dir
roslaunch.configure_logging(uuid)
launch_files = ros_launchers_setup(roslaunch, environment.env_limits_shifted, config)
parent = roslaunch.parent.ROSLaunchParent(uuid, launch_files, force_log=True)
omni.usd.get_context().open_stage(local_file_prefix + config["base_env_path"].get(), None)
# Wait two frames so that stage starts loading
kit.update()
kit.update()
print("Loading stage...")
while is_stage_loading():
kit.update()
print("Loading Complete")
context = omni.usd.get_context()
stage = context.get_stage()
set_stage_up_axis("Z")
# do this AFTER loading the world
simulation_context = SimulationContext(physics_dt=1.0 / config["physics_hz"].get(),
rendering_dt=1.0 / config["render_hz"].get(),
stage_units_in_meters=meters_per_unit, backend='torch')
simulation_context.initialize_physics()
physx_interface = omni.physx.acquire_physx_interface()
physx_interface.start_simulation()
_clock_graph = add_clock() # add ROS clock
simulation_context.play()
for _ in range(10):
simulation_context.step()
og.Controller.evaluate_sync(_clock_graph)
last_pub_time = rospy.Time.now()
simulation_context.stop()
# fixme IDK why this is necessary sometimes
try:
parent.start()
except:
print("Failed to start roslaunch, retry")
try:
parent.start()
except:
print("Failed to start roslaunch, exit")
exit(1)
print("ros node launched")
kit.update()
# use rtx while setting up!
set_raytracing_settings(config["physics_hz"].get())
env_prim_path = environment.load_and_center(config["env_prim_path"].get())
process_semantics(config["env_prim_path"].get())
randomize_and_fix_lights(config["_random_light"].get(), rng, env_prim_path, environment.env_limits[-1] - 0.2,
meters_per_unit, is_rtx=config["rtx_mode"].get())
randomize_roughness(config["_random_roughness"].get(), rng, env_prim_path)
ros_camera_list = []
ros_transform_components = [] # list of tf and joint components, one (of each) for each robot
viewport_window_list = []
dynamic_prims = []
imus_handle_list = []
robot_odom_frames = []
robot_imu_frames = []
camera_pose_frames = []
imu_pubs = []
odom_pubs = []
cam_pose_pubs = []
first = True
simulation_context.play()
for _ in range(100):
og.Controller.evaluate_sync(_clock_graph)
simulation_context.step()
last_pub_time = rospy.Time.now()
simulation_context.stop()
print("Generating map...")
if add_colliders(env_prim_path):
simulation_context.play()
x, y, z, yaw = position_object(environment, type=3)
environment.generate_map(out_dir, origin=[x[0], y[0], 0])
for _ in range(10):
simulation_context.step()
timeline.set_current_time(0) # set to 0 to be sure that the first frame is recorded
else:
simulation_context.play()
for _ in range(10):
simulation_context.step()
print("Error generating collisions", file=sys.stderr)
simulation_context.play()
_dc = dynamic_control_interface()
print("Loading robots..")
from omni.isaac.sensor import _sensor
_is = _sensor.acquire_imu_sensor_interface()
robot_base_prim_path = config["robot_base_prim_path"].get()
usd_robot_path = str(config["usd_robot_path"].get())
c_pose = []
old_pose = []
old_h_ap = []
old_v_ap = []
lidars = []
simulation_context.stop()
for n in range(config["num_robots"].get()):
import_robot(robot_base_prim_path, n, usd_robot_path, local_file_prefix)
x, y, z, yaw = get_valid_robot_location(environment, first)
set_drone_joints_init_loc(f"{robot_base_prim_path}{n}", [x / meters_per_unit, y / meters_per_unit, z / meters_per_unit], [0,0,yaw],
(environment.env_limits[5]) / meters_per_unit, 0.3/meters_per_unit, irotate=config["is_iRotate"].get())
c_pose.append([x, y, z])
old_pose.append([x, y, z])
# todo make a comment about this and the number of cameras
add_ros_components(robot_base_prim_path, n, ros_transform_components, ros_camera_list, viewport_window_list,
camera_pose_frames, cam_pose_pubs, imu_pubs, robot_imu_frames,
robot_odom_frames, odom_pubs, lidars,
dynamic_prims, config, old_h_ap, old_v_ap, _is, simulation_context, _clock_graph)
kit.update()
first = False
for n in range(config["num_robots"].get()):
add_npy_viewport(viewport_window_list, robot_base_prim_path, n, old_h_ap, old_v_ap, config, simulation_context,
config["num_robots"].get() * 1)
for _ in range(50):
simulation_context.render()
print("Loading robot complete")
print("WARNING: CAMERA APERTURE MANUAL SET NO LONGER WORKS, NEEDS TO BE FIXED BY NVIDIA!!!!")
time.sleep(5)
# # legacy code
# for index, cam in enumerate(viewport_window_list):
# camera = stage.GetPrimAtPath(cam.get_active_camera())
# camera.GetAttribute("horizontalAperture").Set(old_h_ap[index])
# camera.GetAttribute("verticalAperture").Set(old_v_ap[index])
print("Starting FSM - setting up topics...")
start_explorer_pubs = []
send_waypoint_pubs = []
movement_monitor_pubs = []
for index, _ in enumerate(robot_odom_frames):
print("Waiting for fsm to start for robot {}".format(index))
my_topic = f"{robot_base_prim_path}{index}/exploration_node/fsm_exploration/state"
if config["autonomous"].get():
rospy.Subscriber(my_topic, String, callback=autostart_exploration, callback_args=index)
start_explorer_pubs.append(
rospy.Publisher(f"{robot_base_prim_path}{index}/traj_start_trigger", PoseStamped, queue_size=10))
else:
rospy.Subscriber(my_topic, String, callback=publish_random_goal, callback_args=(index, environment))
send_waypoint_pubs.append(
rospy.Publisher(f"{robot_base_prim_path}{index}/exploration_node/manual_goal", PoseStamped,
queue_size=10))
rospy.Subscriber(my_topic, String, callback=monitor_movement, callback_args=(index, environment))
movement_monitor_pubs.append(
rospy.Publisher(f"{robot_base_prim_path}{index}/command/pose", PoseStamped, queue_size=10))
print("fsm management for robot {} setted up".format(index))
print("FSM setted up")
print("Loading humans..")
my_humans = []
my_humans_heights = []
human_export_folder = config["human_path"].get()
human_folders = os.listdir(human_export_folder)
tot_area = 0
areas = []
initial_dynamics = len(dynamic_prims)
used_ob_stl_paths = []
## todo cycle to complete area, need to update the service probably
n = 0
human_anim_len = []
added_prims = []
human_base_prim_path = config["human_base_prim_path"].get()
while n < rng.integers(7, 1 + max(7, config["num_humans"].get())):
anim_len = 0
# the animation needs to be shorter than config["max_anim_len"].get() and longer than 0/min_len
while anim_len < max(config["min_human_anim_len"].get(), 0) or anim_len > config["max_human_anim_len"].get():
folder = rng.choice(human_folders)
while "old_textures" in folder:
folder = rng.choice(human_folders)
random_name = rng.choice(os.listdir(os.path.join(human_export_folder, folder)))
asset_path = local_file_prefix + os.path.join(human_export_folder, folder, random_name,
random_name + ".usd")
tmp_pkl = pkl.load(open(os.path.join(human_export_folder, folder, random_name, random_name + ".pkl"), 'rb'))
anim_len = tmp_pkl['ef']
print("Loading human {} from {}".format(random_name, folder))
used_ob_stl_paths.append(os.path.join(human_export_folder, folder, random_name, random_name + ".stl"))
human_anim_len.append(tmp_pkl['ef'])
if "verts" in tmp_pkl.keys():
my_humans_heights.append(tmp_pkl['verts'][:, :, 2])
else:
my_humans_heights.append(None)
my_humans.append(random_name)
load_human(human_base_prim_path, n, asset_path, dynamic_prims, added_prims)
stl_path = os.path.join(human_export_folder, folder, random_name, random_name + ".stl")
this_mesh = mesh.Mesh.from_file(stl_path)
areas.append((this_mesh.x.max() - this_mesh.x.min()) * (this_mesh.y.max() - this_mesh.y.min()))
tot_area += areas[-1]
n += 1
x, y, z, yaw = position_object(environment, type=1, objects=my_humans, ob_stl_paths=used_ob_stl_paths, max_collisions=int(config["allow_collision"].get()))
to_be_removed = []
human_prim_list = []
body_origins = []
for n, human in enumerate(my_humans):
if z[n] < 0:
to_be_removed.append(n)
tot_area -= areas[n]
else:
set_translate(stage.GetPrimAtPath(f"{human_base_prim_path}{n}"),
[x[n] / meters_per_unit, y[n] / meters_per_unit, z[n] / meters_per_unit])
set_scale(stage.GetPrimAtPath(f"{human_base_prim_path}{n}"), 1 / meters_per_unit)
set_rotate(stage.GetPrimAtPath(f"{human_base_prim_path}{n}"), [0, 0, yaw[n]])
human_prim_list.append(f"{human_base_prim_path}{n}")
body_origins.append([x[n], y[n], z[n], yaw[n]])
if len(to_be_removed) > 0:
print("Removing humans that are out of the environment")
to_be_removed.reverse()
cumsum = np.cumsum(added_prims)
for n in to_be_removed:
my_humans.pop(n)
used_ob_stl_paths.pop(n)
my_humans_heights.pop(n)
for _ in range(added_prims[n]):
if n > 0:
dynamic_prims.pop(cumsum[n - 1] + initial_dynamics)
else:
dynamic_prims.pop(initial_dynamics)
human_anim_len.pop(n)
omni.kit.commands.execute("DeletePrimsCommand", paths=[f"{human_base_prim_path}{n}" for n in to_be_removed])
print("Loading human complete")
google_ob_used, shapenet_ob_used = load_objects(config, environment, rng, dynamic_prims, 1/meters_per_unit)
# IT IS OF CRUCIAL IMPORTANCE THAT AFTER THIS POINT THE RENDER GETS DONE WITH THE SLEEPING CALL! OTHERWISE PATH TRACING SPP WILL GET RUINED
if (config["rtx_mode"].get()):
set_raytracing_settings(config["physics_hz"].get())
else:
set_pathtracing_settings(config["physics_hz"].get())
omni.usd.get_context().get_selection().clear_selected_prim_paths()
omni.usd.get_context().get_selection().set_selected_prim_paths([], False)
for _ in range(5):
simulation_context.step(render=False)
sleeping(simulation_context, viewport_window_list, raytracing=config["rtx_mode"].get())
timeline.set_current_time(0)
simulation_step = 0 # this is NOT the frame, this is the "step" (related to physics_hz)
my_recorder = recorder_setup(config['_recorder_settings'].get(), out_dir_npy, config['record'].get(), skip_cameras=1)
simulation_context.stop()
timeline.set_current_time(0) # set to 0 to be sure that the first frame is recorded
timeline.set_auto_update(False)
for _ in range(5):
kit.update()
simulation_context.play()
timeline.set_auto_update(False)
first_start = False
second_start = False
can_change_second_start = False
# two times, this will ensure that totalSpp is reached
sleeping(simulation_context, viewport_window_list, raytracing=config["rtx_mode"].get())
sleeping(simulation_context, viewport_window_list, raytracing=config["rtx_mode"].get())
last_pub_time = rospy.Time.now()
last_check_time = rospy.Time.now()
if config['debug_vis'].get():
cnt = 0
while 1:
cnt += 1
if cnt % 10000 == 0:
import ipdb
ipdb.set_trace()
print("Debug vis")
sleeping(simulation_context, viewport_window_list, raytracing=config["rtx_mode"].get())
reversing_timeline_ratio = compute_timeline_ratio(human_anim_len, config["reverse_strategy"].get(),
experiment_length)
print(f"The reversing ratio is {reversing_timeline_ratio}.\n"
f"This implies that that every {experiment_length / reversing_timeline_ratio} frames we reverse the animations")
cnt_reversal = 1
# example
# exp length: 600, ratio: 4
# forward 0-150, 151-300 backward, 300-450 forward, 450-600 backward (so 4 slots)
# exp length: 1200, ratio: 4
# forward 0-300, 301-600 backward, 601-900 forward, 901-1200 backward (so 4 slots)
ratio_camera = config["ratio_camera"].get()
ratio_odom = config["ratio_odom"].get()
ratio_tf = config["ratio_tf"].get()
starting_to_pub = False
my_recorder._enable_record = False
status = True
while kit.is_running():
# NOTE EVERYTHING THAT NEEDS TO BE RENDERED NEEDS TO BE MOVED AFTER THE TIMELINE UPDATE CONSISTENTLY
if can_start:
last_check_time = rospy.Time.now()
if second_start:
if config['record'].get():
sleeping(simulation_context, viewport_window_list, raytracing=config["rtx_mode"].get())
my_recorder._update()
sleeping(simulation_context, viewport_window_list, raytracing=config["rtx_mode"].get())
starting_to_pub = True
timeline.set_current_time(min(- 1 / (config["physics_hz"].get() / ratio_camera),
-abs(config["bootstrap_exploration"].get())))
simulation_step = int(timeline.get_current_time() * config["physics_hz"].get()) - 1
# reset_physics(timeline, simulation_context)
print("Bootstrap started")
can_start = False
simulation_step += 1
if starting_to_pub and simulation_step == 0:
timeline.set_current_time(0)
# reset_physics(timeline, simulation_context)
move_humans_to_ground(my_humans_heights, human_prim_list, simulation_step / ratio_camera, meters_per_unit,
config["max_distance_human_ground"].get())
print("Starting recording NOW!")
msg = String("starting")
starting_pub.publish(msg)
starting_to_pub = False
time.sleep(0.5)
if config['record'].get():
my_recorder._enable_record = True
last_check_time = rospy.Time.now()
if (config["_random_light"].get()["during_experiment"]):
if (simulation_step % config["_random_light"].get()["n-frames"] == 0):
# fixme todo smooth change, idea get max-min and time window
randomize_and_fix_lights(config["_random_light"].get(), rng, env_prim_path, environment.env_limits[-1],
environment.meters_per_unit, is_rtx=config["rtx_mode"].get())
# step the physics
simulation_context.step(render=False)
# get the current time in ROS
print("Clocking...")
og.Controller.evaluate_sync(_clock_graph)
time.sleep(0.1)
ctime = timeline.get_current_time()
simulation_context.render()
timeline.set_current_time(ctime)
# publish IMU
print("Publishing IMU...")
pub_imu(_is, imu_pubs, robot_imu_frames, meters_per_unit)
# publish joint status (ca 120 Hz)
if simulation_step % ratio_tf == 0:
print("Publishing joint/tf status...")
for component in ros_transform_components:
og.Controller.set(og.Controller.attribute(f"{component}/OnImpulseEvent.state:enableImpulse"), True)
# publish odometry (60 hz)
if simulation_step % ratio_odom == 0:
print("Publishing odometry...")
c_pose, _ = pub_odom(robot_odom_frames, odom_pubs, _dc, meters_per_unit)
pub_cam_pose(camera_pose_frames, cam_pose_pubs, _dc, meters_per_unit)
# we consider ratio_camera to forward the animation.
# If you want it different ratio_animation < ratio_camera to avoid
# two frames with the same animation point
if second_start:
if simulation_step % ratio_camera == 0:
if my_recorder._enable_record:
# update the image counter externally so that we can use it in the recorder and all images have the same index
my_recorder._counter += 1
if simulation_step / ratio_camera < (experiment_length / reversing_timeline_ratio) * (
cnt_reversal):
timeline.forward_one_frame()
else:
if simulation_step / ratio_camera >= ((experiment_length - 1) / reversing_timeline_ratio) * (
cnt_reversal + 1) or \
(timeline.get_current_time() - 1 / timeline.get_time_codes_per_seconds()) < 0:
cnt_reversal += 2
timeline.forward_one_frame()
else:
timeline.rewind_one_frame()
if simulation_step % ratio_camera == 0:
for lidar in lidars:
og.Controller.attribute(lidar+".inputs:step").set(1)
ctime = timeline.get_current_time()
simulation_context.render()
timeline.set_current_time(ctime)
for lidar in lidars:
og.Controller.attribute(lidar+".inputs:step").set(0)
# publish camera (30 hz)
if simulation_step % ratio_camera == 0:
ctime = timeline.get_current_time()
print("Publishing cameras...")
# FIRST ONE WRITTEN IS AT 1/30 on the timeline
pub_and_write_images(simulation_context, viewport_window_list, ros_camera_list, config["rtx_mode"].get(), my_recorder, second_start)
timeline.set_current_time(ctime)
if simulation_step % ratio_camera == 0 and simulation_step / ratio_camera == experiment_length \
and not config["neverending"].get():
print("End of experiment!!!")
simulation_context.pause()
if my_recorder.data_writer is not None:
my_recorder.data_writer.stop_threads()
timeline.set_current_time(0)
context.save_as_stage(os.path.join(out_dir, "loaded_stage.usd"))
experiment_info = {}
experiment_info["config"] = config
experiment_info["reversing_timeline_ratio"] = reversing_timeline_ratio
experiment_info["humans"] = {}
experiment_info["humans"]["ids"] = my_humans
experiment_info["humans"]["folders"] = used_ob_stl_paths
experiment_info["humans"]["origins"] = body_origins # x y z yaw
experiment_info["google_obs"] = google_ob_used
experiment_info["shapenet_obs"] = shapenet_ob_used
experiment_info["environment"] = {}
experiment_info["environment"]["id"] = environment.env_name
experiment_info["environment"]["folder"] = environment.env_path
experiment_info["environment"]["shifts"] = environment.shifts
experiment_info["rng_state"] = rng_state
np.save(os.path.join(out_dir, "experiment_info.npy"), experiment_info)
break
except:
extype, value, tb = sys.exc_info()
traceback.print_exc()
ipdb.post_mortem(tb)
finally:
for pub in odom_pubs:
pub.unregister()
for pub in imu_pubs:
pub.unregister()
for pub in cam_pose_pubs:
pub.unregister()
for pub in start_explorer_pubs:
pub.unregister()
for pub in send_waypoint_pubs:
pub.unregister()
parent.shutdown()
rospy.signal_shutdown("my_simulation complete")
simulation_context.stop()
try:
kit.close()
except:
pass
| 25,227 | Python | 37.457317 | 156 | 0.674793 |
eliabntt/GRADE-RR/simulator/robot_with_ros.py | import argparse
import time
import os
import numpy as np
# base_env_path and other settings are in the config file
out_dir = "" # set this to a temporary empty dir
from omni.isaac.kit import SimulationApp
def boolean_string(s):
if s.lower() not in {'false', 'true'}:
raise ValueError('Not a valid boolean string')
return s.lower() == 'true'
parser = argparse.ArgumentParser(description="Your second IsaacSim run")
parser.add_argument("--headless", type=boolean_string, default=True, help="Wheter to run it in headless mode or not")
parser.add_argument("--rtx_mode", type=boolean_string, default=False, help="Use rtx when True, use path tracing when False")
parser.add_argument("--config_file", type=str, default="config.yaml")
parser.add_argument("--fix_env", type=str, default="",
help="leave it empty to have a random env, fix it to use a fixed one. Useful for loop processing")
args, unknown = parser.parse_known_args()
config = confuse.Configuration("world_and_robot", __name__)
config.set_file(args.config_file)
config.set_args(args)
CONFIG = {"display_options": 3286, "width": 1280, "height": 720, "headless": config["headless"].get()}
kit = SimulationApp(launch_config=CONFIG, experience=f"{os.environ['EXP_PATH']}/omni.isaac.sim.python.kit")
omni.usd.get_context().open_stage(config["base_env_path"].get(), None)
kit.update()
kit.update()
print("Loading stage...")
while is_stage_loading():
kit.update()
print("Loading Complete")
context = omni.usd.get_context()
stage = context.get_stage()
meters_per_unit = config["meters_per_unit"].get()
simulation_context = SimulationContext(physics_dt=1.0 / config["physics_hz"].get(), rendering_dt=1.0 / config["render_hz"].get(), stage_units_in_meters=meters_per_unit, backend='torch')
simulation_context.initialize_physics()
physx_interface = omni.physx.acquire_physx_interface()
physx_interface.start_simulation()
print("Adding ROS clock, you can check with rostopic echo /clock")
_clock_graph = add_clock() # add ROS clock
simulation_context.play()
for _ in range(10):
simulation_context.step() # remember that this step also the physics
og.Controller.evaluate_sync(_clock_graph)
simulation_context.stop()
import utils.misc_utils
from utils.misc_utils import *
from utils.robot_utils import *
from utils.simulation_utils import *
simulation_environment_setup(need_ros = True)
if base_world_path != "":
from utils.environment_utils import *
print("Loading environment...")
environment = environment(config, meters_per_unit=meters_per_unit)
env_prim_path = environment.load_and_center(config["env_prim_path"].get())
process_semantics(config["env_prim_path"].get())
print("Visualization...")
for _ in range(1000):
simulation_context.render()
simulation_context.step(render=False)
print("Environment loading done...")
add_colliders(env_prim_path)
print("Colliders added..")
simulation_context.play()
x, y, z = 0, 0, 0
if out_dir != "":
environment.generate_map(out_dir, origin=[x,y,z])
print("Map generated..")
simulation_context.stop()
# prepare some containers
joint_states = []
tf_trees = []
camera_list = []
viewport_list = []
camera_pose, camera_pose_pub = [], []
imus,imu_pubs = [], []
lidars = []
odoms, odom_pubs = [], []
# get the interface to add imu sensors
from omni.isaac.sensor import _sensor
_is = _sensor.acquire_imu_sensor_interface()
# these are kept because the aperture is resetted based on the h aperture by IsaacSim.
# In v2021 this could have been reverted. In v2022 not.
old_h_ape, old_v_ape = [], []
# get the interface to access dynamics of the assets
_dc = dynamic_control_interface()
print("Loading robots..")
robot_base_prim_path = config["robot_base_prim_path"].get()
usd_robot_path = str(config["usd_robot_path"].get())
for n in range(config["num_robots"].get()):
import_robot(robot_base_prim_path, n, usd_robot_path)
x, y, z, yaw = np.random.randint(-100,100,4)
set_drone_joints_init_loc(f"{robot_base_prim_path}{n}",
[x / meters_per_unit, y / meters_per_unit, z / meters_per_unit],
[0, 0, np.deg2rad(yaw)],
upper_zlim = z * 2,
lower_zlim = -z * 2
)
print("Adding ROS components")
joint_states.append(add_joint_state(f"{robot_base_prim_path}{n}"))
tf_trees.append(add_pose_tree(f"{robot_base_prim_path}{n}"))
# create the viewport, the camera component
component, viewport = add_camera_and_viewport(f"{robot_base_prim_path}{n}/camera_link",
config["robot_sensor_size"].get(),
old_h_ape, old_v_ape, simulation_context,
0, n, cam_per_robot=1) # cam index is useful if you want multiple cameras
cam_outputs = control_camera(viewport, simulation_context)
camera_list.append([n + 0, component, cam_outputs])
viewport_list.append(viewport)
omni.kit.app.get_app().update()
camera_pose.append(f"{robot_base_prim_path}{n}/camera_link")
camera_pose_pub.append(rospy.Publisher(f"{robot_base_prim_path}{n}/camera/pose", PoseStamped, queue_size=10))
setup_imu_sensor(_is, config, f"{robot_base_prim_path}{n}/imu_link")
imu_pubs.append(rospy.Publisher(f"{robot_base_prim_path}{n}/imu_body", Imu, queue_size=10))
imus.append(f"{robot_base_prim_path}{n}/imu_link")
odoms.append(f"{robot_base_prim_path}{n}/yaw_link")
odom_pubs.append(rospy.Publisher(f"{robot_base_prim_path}{n}/odom", Odometry, queue_size=10))
sensor = add_lidar(f"{robot_base_prim_path}{n}/yaw_link", [0, 0, -.1], [0, 0, 0], is_3d=True, is_2d=True)
lidars.append(sensor)
# alternatively
# add_ros_components(robot_base_prim_path, n, ros_transform_components, camera_list, viewport_list,
# camera_pose, camera_pose_pub, imu_pubs, imus,
# odoms, odom_pubs, lidars,
# [], config, old_h_ape, old_v_ape, _is, simulation_context, _clock, irotate=False):
print("Loading robots done")
# set some settings for the rendering
if (config["rtx_mode"].get()):
set_raytracing_settings(config["physics_hz"].get())
else:
set_pathtracing_settings(config["physics_hz"].get())
print("Note that the rendering is now blocking until finished")
for i in range(100):
print(f"Iteration {i}/100", end="\r")
sleeping(simulation_context, viewport_list, raytracing=config["rtx_mode"].get())
# deselect all objects
omni.usd.get_context().get_selection().clear_selected_prim_paths()
omni.usd.get_context().get_selection().set_selected_prim_paths([], False)
simulation_context.play()
for i in range(2000):
simulation_context.step(render=False)
og.Controller.evaluate_sync(_clock)
time.sleep(0.2)
simulation_context.render()
# publish IMU
print("Publishing IMU...")
pub_imu(_is, imu_pubs, robot_imu_frames, meters_per_unit)
if i % ratio_joints == 0:
for js in joint_states:
og.Controller.set(og.Controller.attribute(f"{js}/OnImpulseEvent.state:enableImpulse"), True)
if i % ratio_tf:
for tf in tf_trees:
og.Controller.set(og.Controller.attribute(f"{tf}/OnImpulseEvent.state:enableImpulse"), True)
if simulation_step % ratio_odom == 0:
c_pose, _ = pub_odom(odoms, odom_pubs, _dc, meters_per_unit)
pub_cam_pose(camera_pose, camera_pose_pub, _dc, meters_per_unit)
if simulation_step % ratio_camera == 0:
# The RTX LiDAR is still a fuzzy component. The "normal" LiDAR is more stable, but won't see non-colliding objects
for lidar in lidars:
og.Controller.attribute(lidar+".inputs:step").set(1)
ctime = timeline.get_current_time()
simulation_context.render()
timeline.set_current_time(ctime)
for lidar in lidars:
og.Controller.attribute(lidar+".inputs:step").set(0)
pub_and_write_images(simulation_context, viewport_list, ros_camera_list, raytracing) # clearly not writing anything here
simulation_context.stop()
try:
kit.close()
except:
pass
| 7,858 | Python | 36.966183 | 185 | 0.693688 |
eliabntt/GRADE-RR/simulator/world_and_robot.py | import argparse
# base_env_path and other settings are in the config file
out_dir = "" # set this to a temporary empty dir
from omni.isaac.kit import SimulationApp
def boolean_string(s):
if s.lower() not in {'false', 'true'}:
raise ValueError('Not a valid boolean string')
return s.lower() == 'true'
parser = argparse.ArgumentParser(description="Your second IsaacSim run")
parser.add_argument("--headless", type=boolean_string, default=True, help="Wheter to run it in headless mode or not")
parser.add_argument("--rtx_mode", type=boolean_string, default=False, help="Use rtx when True, use path tracing when False")
# new options
parser.add_argument("--config_file", type=str, default="config.yaml")
parser.add_argument("--fix_env", type=str, default="",
help="leave it empty to have a random env, fix it to use a fixed one. Useful for loop processing")
args, unknown = parser.parse_known_args()
config = confuse.Configuration("world_and_robot", __name__)
# load the config file specified
config.set_file(args.config_file)
config.set_args(args)
CONFIG = {"display_options": 3286, "width": 1280, "height": 720, "headless": config["headless"].get()}
kit = SimulationApp(launch_config=CONFIG, experience=f"{os.environ['EXP_PATH']}/omni.isaac.sim.python.kit")
omni.usd.get_context().open_stage(config["base_env_path"].get(), None)
kit.update()
kit.update()
print("Loading stage...")
while is_stage_loading():
kit.update()
print("Loading Complete")
context = omni.usd.get_context()
stage = context.get_stage()
meters_per_unit = config["meters_per_unit"].get()
simulation_context = SimulationContext(physics_dt=1.0 / config["physics_hz"].get(), rendering_dt=1.0 / config["render_hz"].get(), stage_units_in_meters=meters_per_unit, backend='torch')
simulation_context.initialize_physics()
physx_interface = omni.physx.acquire_physx_interface()
physx_interface.start_simulation()
for _ in range(100):
simulation_context.render()
simulation_context.step(render=False)
import utils.misc_utils
from utils.misc_utils import *
from utils.robot_utils import *
from utils.simulation_utils import *
simulation_environment_setup(need_ros = False) # enable some extensions, check if ros is running automatically
if base_world_path != "":
from utils.environment_utils import *
print("Loading environment...")
environment = environment(config, meters_per_unit=meters_per_unit) # setup the class
env_prim_path = environment.load_and_center(config["env_prim_path"].get()) # actually load the env
process_semantics(config["env_prim_path"].get()) # add semantic information based either on label you provide, or looking into fields of the objcets. This applies semantic to all childs
print("Visualization...")
for _ in range(1000):
simulation_context.render()
simulation_context.step(render=False)
print("Environment loading done...")
print("Add colliders to the environment, if the environment is big this could take ages..")
add_colliders(env_prim_path) # add colliders to the environment
print("Colliders added..")
print("For the next step please check out the code and set x, y, z manually to test them out..")
print()
ipdb.set_trace()
simulation_context.play()
x, y, z = 0, 0, 0
if out_dir == "":
print("Change out_dir")
environment.generate_map(out_dir, origin=[x,y,z])
print("Map generated..")
simulation_context.stop()
print("Loading robots..")
robot_base_prim_path = config["robot_base_prim_path"].get()
usd_robot_path = str(config["usd_robot_path"].get())
for n in range(config["num_robots"].get()):
import_robot(robot_base_prim_path, n, usd_robot_path)
x, y, z, yaw = np.random.randint(-100,100,4)
set_drone_joints_init_loc(f"{robot_base_prim_path}{n}",
[x / meters_per_unit, y / meters_per_unit, z / meters_per_unit],
[0, 0, np.deg2rad(yaw)],
upper_zlim = z * 2,
lower_zlim = -z * 2
)
print("Loading robots done")
simulation_context.play()
for _ in range(2000):
simulation_context.render()
simulation_context.step(render=False)
simulation_context.stop()
try:
kit.close()
except:
pass
| 4,102 | Python | 35.633928 | 186 | 0.714529 |
eliabntt/GRADE-RR/simulator/irotate_simulation.py | import argparse
import carb
import confuse
import ipdb
import numpy as np
import os
import roslaunch
import rospy
import sys
import time
import traceback
import yaml
from omni.isaac.kit import SimulationApp
from time import sleep
def boolean_string(s):
if s.lower() not in {'false', 'true'}:
raise ValueError('Not a valid boolean string')
return s.lower() == 'true'
try:
parser = argparse.ArgumentParser(description="Dynamic Worlds Simulator")
parser.add_argument("--config_file", type=str, default="config.yaml")
parser.add_argument("--headless", type=boolean_string, default=True, help="Wheter to run it in headless mode or not")
parser.add_argument("--rtx_mode", type=boolean_string, default=False,
help="Use rtx when True, use path tracing when False")
parser.add_argument("--record", type=boolean_string, default=True, help="Writing data to the disk")
parser.add_argument("--debug_vis", type=boolean_string, default=False,
help="When true continuosly loop the rendering")
parser.add_argument("--neverending", type=boolean_string, default=False, help="Never stop the main loop")
parser.add_argument("--fix_env", type=str, default="",
help="leave it empty to have a random env, fix it to use a fixed one. Useful for loop processing")
args, unknown = parser.parse_known_args()
config = confuse.Configuration("DynamicWorlds", __name__)
config.set_file(args.config_file)
config.set_args(args)
experiment_length = config["experiment_length"].get()
can_start = True
CONFIG = {"display_options": 3286, "width": 1280, "height": 720, "headless": config["headless"].get()}
kit = SimulationApp(launch_config=CONFIG, experience=f"{os.environ['EXP_PATH']}/omni.isaac.sim.python.kit")
# Cannot move before SimApp is launched
import utils.misc_utils
from utils.misc_utils import *
from utils.robot_utils import *
from utils.simulation_utils import *
from utils.environment_utils import *
simulation_environment_setup()
rospy.init_node("my_isaac_ros_app", anonymous=True, disable_signals=True, log_level=rospy.ERROR)
starting_pub = rospy.Publisher('starting_experiment', String)
rng = np.random.default_rng()
rng_state = np.random.get_state()
local_file_prefix = "my-computer://"
# setup environment variables
environment = environment(config, rng, local_file_prefix)
uuid = roslaunch.rlutil.get_or_generate_uuid(None, False)
out_dir = os.path.join(config['out_folder'].get(), environment.env_name)
out_dir_npy = os.path.join(config['out_folder_npy'].get(), environment.env_name)
if not os.path.exists(out_dir):
os.makedirs(out_dir)
os.environ["ROS_LOG_DIR"] = out_dir
roslaunch.configure_logging(uuid)
launch_files = ros_launchers_setup(roslaunch, environment.env_limits_shifted, config)
parent = roslaunch.parent.ROSLaunchParent(uuid, launch_files, force_log=True)
omni.usd.get_context().open_stage(local_file_prefix + config["base_env_path"].get(), None)
# Wait two frames so that stage starts loading
kit.update()
kit.update()
print("Loading stage...")
while is_stage_loading():
kit.update()
print("Loading Complete")
context = omni.usd.get_context()
stage = context.get_stage()
set_stage_up_axis("Z")
# do this AFTER loading the world
simulation_context = SimulationContext(physics_dt=1.0 / config["physics_hz"].get(),
rendering_dt=1.0 / config["render_hz"].get(),
stage_units_in_meters=0.01)
simulation_context.start_simulation()
add_clock() # add ROS clock
simulation_context.play()
for _ in range(100):
omni.kit.commands.execute("RosBridgeTickComponent", path="/ROS_Clock")
simulation_context.step()
last_pub_time = rospy.Time.now()
simulation_context.stop()
# fixme IDK why this is necessary sometimes
try:
parent.start()
except:
print("Failed to start roslaunch, retry")
try:
parent.start()
except:
print("Failed to start roslaunch, exit")
exit(1)
print("ros node launched")
kit.update()
meters_per_unit = UsdGeom.GetStageMetersPerUnit(stage)
# use rtx while setting up!
set_raytracing_settings(config["physics_hz"].get())
env_prim_path = environment.load_and_center(config["env_prim_path"].get())
process_semantics(config["env_prim_path"].get())
randomize_and_fix_lights(config["_random_light"].get(), rng, env_prim_path, environment.env_limits[-1] - 0.2,
environment.meters_per_unit, is_rtx=config["rtx_mode"].get())
randomize_roughness(config["_random_roughness"].get(), rng, env_prim_path)
# set timeline of the experiment
timeline = setup_timeline(config)
ros_camera_list = []
ros_transform_components = [] # list of tf and joint components, one (of each) for each robot
viewport_window_list = []
dynamic_prims = []
imus_handle_list = []
robot_odom_frames = []
robot_imu_frames = []
camera_pose_frames = []
imu_pubs = []
odom_pubs = []
cam_pose_pubs = []
camera_odom_pubs = []
camera_odom_frames = []
lidar_components = []
first = True
imu_sensor, imu_props = setup_imu_sensor(config)
simulation_context.play()
for _ in range(100):
omni.kit.commands.execute("RosBridgeTickComponent", path="/ROS_Clock")
simulation_context.step()
last_pub_time = rospy.Time.now()
simulation_context.stop()
print("Generating map...")
if add_colliders(env_prim_path):
simulation_context.play()
x, y, z, yaw = position_object(environment, type=3)
environment.generate_map(out_dir, origin=[x[0], y[0], 0])
for _ in range(10):
simulation_context.step()
timeline.set_current_time(0) # set to 0 to be sure that the first frame is recorded
else:
simulation_context.play()
for _ in range(10):
simulation_context.step()
print("Error generating collisions", file=sys.stderr)
simulation_context.play()
_dc = dynamic_control_interface()
print("Loading robots..")
robot_base_prim_path = config["robot_base_prim_path"].get()
usd_robot_path = str(config["usd_robot_path"].get())
c_pose = []
old_pose = []
old_h_ap = []
old_v_ap = []
for n in range(config["num_robots"].get()):
simulation_context.stop()
import_robot(robot_base_prim_path, n, usd_robot_path, local_file_prefix)
x, y, z, yaw = 0, 0, 0, 0
simulation_context.stop()
set_drone_joints_init_loc(f"{robot_base_prim_path}{n}", [x / meters_per_unit, y / meters_per_unit, z / meters_per_unit],
[0, 0, yaw],
(environment.env_limits[5]) / meters_per_unit, irotate=config["is_iRotate"].get())
c_pose.append([x, y, z])
old_pose.append([x, y, z])
kit.update()
simulation_context.play()
kit.update()
add_ros_components(robot_base_prim_path, n, ros_transform_components, ros_camera_list, viewport_window_list,
camera_pose_frames, cam_pose_pubs, imus_handle_list, imu_pubs, robot_imu_frames,
robot_odom_frames, odom_pubs,
dynamic_prims, config, imu_sensor, imu_props, old_h_ap, old_v_ap, config["is_iRotate"].get())
add_irotate_ros_components(camera_odom_frames, camera_odom_pubs, lidar_components, robot_base_prim_path, n)
kit.update()
first = False
for n in range(config["num_robots"].get()):
add_npy_viewport(viewport_window_list, robot_base_prim_path, n, old_h_ap, old_v_ap, config,
config["num_robots"].get() * 1)
kit.update()
for _ in range(50):
simulation_context.render()
print("Loading robot complete")
for index, cam in enumerate(viewport_window_list):
camera = stage.GetPrimAtPath(cam.get_active_camera())
camera.GetAttribute("horizontalAperture").Set(old_h_ap[index])
camera.GetAttribute("verticalAperture").Set(old_v_ap[index])
# setup manual ticks for all components (just to be sure)
# IMU not necessary as it is NOT a ROS component itself
for component in ros_camera_list:
omni.kit.commands.execute("RosBridgeTickComponent", path=str(component.GetPath()))
for component in ros_transform_components:
omni.kit.commands.execute("RosBridgeTickComponent", path=str(component.GetPath()))
# IT IS OF CRUCIAL IMPORTANCE THAT AFTER THIS POINT THE RENDER GETS DONE WITH THE SLEEPING CALL! OTHERWISE PATH TRACING SPP WILL GET RUINED
if (config["rtx_mode"].get()):
set_raytracing_settings(config["physics_hz"].get())
else:
set_pathtracing_settings(config["physics_hz"].get())
omni.usd.get_context().get_selection().set_selected_prim_paths([], False)
simulation_context.stop()
simulation_context.play()
for _ in range(5):
simulation_context.step(render=False)
sleeping(simulation_context, viewport_window_list, raytracing=config["rtx_mode"].get())
timeline.set_current_time(0)
simulation_step = 0 # this is NOT the frame, this is the "step" (related to physics_hz)
my_recorder = recorder_setup(config['_recorder_settings'].get(), out_dir_npy, config['record'].get())
timeline.set_current_time(0) # set to 0 to be sure that the first frame is recorded
timeline.set_auto_update(False)
omni.kit.commands.execute("RosBridgeUseSimTime", use_sim_time=True)
omni.kit.commands.execute("RosBridgeUsePhysicsStepSimTime", use_physics_step_sim_time=True)
# two times, this will ensure that totalSpp is reached
sleeping(simulation_context, viewport_window_list, raytracing=config["rtx_mode"].get())
sleeping(simulation_context, viewport_window_list, raytracing=config["rtx_mode"].get())
last_pub_time = rospy.Time.now()
last_check_time = rospy.Time.now()
if config['debug_vis'].get():
cnt = 0
while 1:
cnt += 1
if cnt % 10000 == 0:
import ipdb
ipdb.set_trace()
print("DEBUGGING VIS")
sleeping(simulation_context, viewport_window_list, raytracing=config["rtx_mode"].get())
for i, cam in enumerate(ros_camera_list):
omni.kit.commands.execute("RosBridgeTickComponent", path=str(cam.GetPath()))
reversing_timeline_ratio = 1
print(
f"The reversing ratio is {reversing_timeline_ratio}.\n"
f"This implies that that every {experiment_length / reversing_timeline_ratio} frames we reverse the animations")
cnt_reversal = 1
ratio_camera = config["ratio_camera"].get()
ratio_odom = config["ratio_odom"].get()
ratio_tf = config["ratio_tf"].get()
starting_to_pub = False
my_recorder._enable_record = False
second_start = False
while kit.is_running():
if can_start:
last_check_time = rospy.Time.now()
if config['record'].get():
sleeping(simulation_context, viewport_window_list, raytracing=config["rtx_mode"].get())
my_recorder._update()
sleeping(simulation_context, viewport_window_list, raytracing=config["rtx_mode"].get())
starting_to_pub = True
timeline.set_current_time(min(- 1 / (config["physics_hz"].get() / ratio_camera),
-abs(config["bootstrap_exploration"].get())))
simulation_step = int(timeline.get_current_time() * config["physics_hz"].get()) - 1
print("Bootstrap started")
can_start = False
second_start = True
simulation_step += 1
if starting_to_pub and simulation_step == 0:
print("Starting recording NOW!")
msg = String("starting")
starting_pub.publish(msg)
starting_to_pub = False
time.sleep(0.5)
if config['record'].get():
my_recorder._enable_record = True
last_check_time = rospy.Time.now()
if (config["_random_light"].get()["during_experiment"]):
if (simulation_step % config["_random_light"].get()["n-frames"] == 0):
# fixme todo smooth change, idea get max-min and time window
randomize_and_fix_lights(config["_random_light"].get(), rng, env_prim_path, environment.env_limits[-1],
environment.meters_per_unit, is_rtx=config["rtx_mode"].get())
# step the physics
simulation_context.step(render=False)
# get the current time in ROS
print("Clocking...")
omni.kit.commands.execute("RosBridgeTickComponent", path="/ROS_Clock")
time.sleep(0.2)
# publish IMU
print("Publishing IMU...")
pub_imu(imus_handle_list, imu_sensor, imu_pubs, robot_imu_frames, meters_per_unit)
# publish joint status (ca 120 Hz)
if simulation_step % ratio_tf == 0:
print("Publishing joint/tf status...")
for component in ros_transform_components:
omni.kit.commands.execute("RosBridgeTickComponent", path=str(component.GetPath()))
# publish odometry (60 hz)
if simulation_step % ratio_odom == 0:
print("Publishing odometry...")
pub_cam_pose(camera_pose_frames, cam_pose_pubs, _dc, meters_per_unit)
c_pose, _ = pub_odom(camera_odom_frames, camera_odom_pubs, _dc, meters_per_unit, robot_odom_frames)
c_pose, _ = pub_odom(robot_odom_frames, odom_pubs, _dc, meters_per_unit)
for component in lidar_components:
omni.kit.commands.execute("RosBridgeTickComponent", path=str(component.GetPath()))
# we consider ratio_camera to forward the animation.
# If you want it different ratio_animation < ratio_camera to avoid
# two frames with the same animation point
if simulation_step % ratio_camera == 0:
if my_recorder._enable_record:
# update the image counter externally so that we can use it in the recorder and all images have the same index
my_recorder._counter += 1
if simulation_step / ratio_camera < (experiment_length / reversing_timeline_ratio) * (
cnt_reversal):
timeline.forward_one_frame()
else:
if simulation_step / ratio_camera >= ((experiment_length - 1) / reversing_timeline_ratio) * (
cnt_reversal + 1) or \
(timeline.get_current_time() - 1 / timeline.get_time_codes_per_seconds()) < 0:
cnt_reversal += 2
timeline.forward_one_frame()
else:
timeline.rewind_one_frame()
# publish camera (30 hz)
if simulation_step % ratio_camera == 0:
print("Publishing cameras...")
# getting skel pose for each joint
# get_skeleton_info(meters_per_unit, body_origins, body_list)
# FIRST ONE WRITTEN IS AT 1/30 on the timeline
pub_and_write_images(simulation_context, viewport_window_list,
ros_camera_list, config["rtx_mode"].get(),
my_recorder, second_start)
if simulation_step % ratio_camera == 0 and simulation_step / ratio_camera == experiment_length \
and not config["neverending"].get():
print("End of experiment!!!")
simulation_context.pause()
if my_recorder.data_writer is not None:
my_recorder.data_writer.stop_threads()
timeline.set_current_time(0)
context.save_as_stage(os.path.join(out_dir, "loaded_stage.usd"))
experiment_info = {}
experiment_info["config"] = config
experiment_info["reversing_timeline_ratio"] = reversing_timeline_ratio
experiment_info["environment"] = {}
experiment_info["environment"]["id"] = environment.env_name
experiment_info["environment"]["folder"] = environment.env_path
experiment_info["environment"]["shifts"] = environment.shifts
experiment_info["rng_state"] = rng_state
np.save(os.path.join(out_dir, "experiment_info.npy"), experiment_info)
break
except:
extype, value, tb = sys.exc_info()
traceback.print_exc()
# ipdb.post_mortem(tb)
finally:
for pub in odom_pubs:
pub.unregister()
for pub in imu_pubs:
pub.unregister()
for pub in cam_pose_pubs:
pub.unregister()
parent.shutdown()
rospy.signal_shutdown("my_simulation complete")
simulation_context.stop()
try:
kit.close()
except:
pass
| 15,217 | Python | 36.761787 | 143 | 0.693829 |
eliabntt/GRADE-RR/simulator/multi_robot_sim.py | import argparse
import carb
import confuse
import ipdb
import numpy as np
import os
import roslaunch
import rospy
import sys
import time
import traceback
import yaml
from omni.isaac.kit import SimulationApp
from time import sleep
def boolean_string(s):
if s.lower() not in {'false', 'true'}:
raise ValueError('Not a valid boolean string')
return s.lower() == 'true'
try:
parser = argparse.ArgumentParser(description="Dynamic Worlds Simulator")
parser.add_argument("--config_file", type=str, default="config.yaml")
parser.add_argument("--headless", type=boolean_string, default=True, help="Wheter to run it in headless mode or not")
parser.add_argument("--rtx_mode", type=boolean_string, default=False,
help="Use rtx when True, use path tracing when False")
parser.add_argument("--record", type=boolean_string, default=True, help="Writing data to the disk")
parser.add_argument("--debug_vis", type=boolean_string, default=False,
help="When true continuosly loop the rendering")
parser.add_argument("--neverending", type=boolean_string, default=False, help="Never stop the main loop")
parser.add_argument("--fix_env", type=str, default="",
help="leave it empty to have a random env, fix it to use a fixed one. Useful for loop processing")
args, unknown = parser.parse_known_args()
config = confuse.Configuration("DynamicWorlds", __name__)
config.set_file(args.config_file)
config.set_args(args)
os.environ["SHAPENET_LOCAL_DIR"] = config["shapenet_local_dir"].get()
experiment_length = config["experiment_length"].get()
can_start = True
CONFIG = {"display_options": 3286, "width": 1280, "height": 720, "headless": config["headless"].get()}
kit = SimulationApp(launch_config=CONFIG, experience=f"{os.environ['EXP_PATH']}/omni.isaac.sim.python.kit")
# Cannot move before SimApp is launched
import utils.misc_utils
from utils.misc_utils import *
from utils.robot_utils import *
from utils.simulation_utils import *
from utils.objects_utils import *
from utils.environment_utils import *
from utils.human_utils import *
def monitor_movement(msg, args):
global second_start
global last_check_time
global c_pose
global old_pose
global rng
global env_prim_path
wait_time = rospy.Duration(1)
index, environment = args[0], args[1]
if second_start and rospy.Time.now() > last_check_time + wait_time:
last_check_time = rospy.Time.now()
diff_x = abs(old_pose[index][0] - c_pose[index][0]) ** 2
diff_y = abs(old_pose[index][1] - c_pose[index][1]) ** 2
diff_z = abs(old_pose[index][2] - c_pose[index][2]) ** 2
dist = (diff_x + diff_y + diff_z) ** 0.5
if (dist) < 0.1:
my_pose = PoseStamped()
if (rng.uniform() > .9):
x, y, z, yaw = position_object(environment, type=0)
x = x[0]
y = y[0]
z = z[0]
yaw = yaw[0] + rng.uniform(0, 2 * np.pi)
else:
yaw = get_robot_yaw(c_pose[index][0], c_pose[index][1], c_pose[index][2],
environment.env_mesh, environment.shifts)
x = c_pose[index][0] + 0.2 * np.cos(yaw)
y = c_pose[index][1] + 0.2 * np.sin(yaw)
z = c_pose[index][2]
yaw += rng.uniform(0, 2 * np.pi)
my_pose.pose.position.x = x
my_pose.pose.position.y = y
my_pose.pose.position.z = z
rot = np.array(yaw) * 180 / np.pi
quat = (
Gf.Rotation(Gf.Vec3d.XAxis(), 0)
* Gf.Rotation(Gf.Vec3d.YAxis(), 0)
* Gf.Rotation(Gf.Vec3d.ZAxis(), rot)
).GetQuat()
my_pose.pose.orientation.x = quat.imaginary[0]
my_pose.pose.orientation.y = quat.imaginary[1]
my_pose.pose.orientation.z = quat.imaginary[2]
my_pose.pose.orientation.w = quat.real
print(
f"Publishing random goal since robot {index} stuck [{x},{y},{z}, {yaw} ({yaw * 180 / 3.14})].")
my_pose.header.frame_id = "world"
my_pose.header.stamp = rospy.Time.now()
movement_monitor_pubs[index].publish(my_pose)
if (dist) < 0.05:
set_colliders(env_prim_path, True)
else:
old_pose[index] = c_pose[index]
set_colliders(env_prim_path, True)
def autostart_exploration(msg, index):
global first_start
global second_start
global can_start
global can_change_second_start
global last_pub_time
if (msg.data == "PUB_FIRST_360"):
can_change_second_start = True
wait_time = rospy.Duration(0, 500000000) if second_start else rospy.Duration(1)
if (msg.data == "WAIT_TRIGGER" or (
msg.data == "PUB_360" and not second_start) and rospy.Time.now() > last_pub_time + wait_time):
if can_start:
if not first_start:
first_start = True
elif can_change_second_start:
second_start = True
print("Exploration will start at the end of this movement")
default_pose = PoseStamped()
default_pose.header.frame_id = "world"
default_pose.header.stamp = rospy.Time.now()
start_explorer_pubs[index].publish(default_pose)
last_pub_time = rospy.Time.now()
def publish_random_goal(msg, args):
global last_pub_time
global first_start
global second_start
global can_start
global can_change_second_start
index, environment = args[0], args[1]
if (msg.data == "PUB_FIRST_360"):
can_change_second_start = True
if (msg.data == "WAIT_TRIGGER" or (
msg.data == "PUB_360" and not second_start) and rospy.Time.now() > last_pub_time + rospy.Duration(0,
500000000)):
if can_start:
if not first_start:
first_start = True
elif can_change_second_start:
second_start = True
my_pose = PoseStamped()
x, y, z, yaw = position_object(environment, type=0)
my_pose.pose.position.x = x[0]
my_pose.pose.position.y = y[0]
my_pose.pose.position.z = z[0]
rot = np.array(yaw[0]) * 180 / np.pi
quat = (
Gf.Rotation(Gf.Vec3d.XAxis(), 0)
* Gf.Rotation(Gf.Vec3d.YAxis(), 0)
* Gf.Rotation(Gf.Vec3d.ZAxis(), rot)
).GetQuat()
my_pose.pose.orientation.x = quat.imaginary[0]
my_pose.pose.orientation.y = quat.imaginary[1]
my_pose.pose.orientation.z = quat.imaginary[2]
my_pose.pose.orientation.w = quat.real
print(f"Publishing random goal [{x[0]},{y[0]},{z[0]}, {yaw[0]} ({yaw[0] * 180 / 3.14})] for robot {index}")
my_pose.header.frame_id = "fixing_manual"
my_pose.header.stamp = rospy.Time.now()
send_waypoint_pubs[index].publish(my_pose)
last_pub_time = rospy.Time.now()
simulation_environment_setup()
rospy.init_node("my_isaac_ros_app", anonymous=True, disable_signals=True, log_level=rospy.ERROR)
starting_pub = rospy.Publisher('starting_experiment', String)
rng = np.random.default_rng()
rng_state = np.random.get_state()
local_file_prefix = "my-computer://"
# setup environment variables
environment = environment(config, rng, local_file_prefix)
uuid = roslaunch.rlutil.get_or_generate_uuid(None, False)
out_dir = os.path.join(config['out_folder'].get(), environment.env_name)
out_dir_npy = os.path.join(config['out_folder_npy'].get(), environment.env_name)
if not os.path.exists(out_dir):
os.makedirs(out_dir)
os.environ["ROS_LOG_DIR"] = out_dir
roslaunch.configure_logging(uuid)
launch_files = ros_launchers_setup(roslaunch, environment.env_limits_shifted, config)
parent = roslaunch.parent.ROSLaunchParent(uuid, launch_files, force_log=True)
omni.usd.get_context().open_stage(local_file_prefix + config["base_env_path"].get(), None)
# Wait two frames so that stage starts loading
kit.update()
kit.update()
print("Loading stage...")
while is_stage_loading():
kit.update()
print("Loading Complete")
context = omni.usd.get_context()
stage = context.get_stage()
set_stage_up_axis("Z")
# do this AFTER loading the world
simulation_context = SimulationContext(physics_dt=1.0 / config["physics_hz"].get(),
rendering_dt=1.0 / config["render_hz"].get(),
stage_units_in_meters=0.01)
simulation_context.start_simulation()
add_clock() # add ROS clock
simulation_context.play()
for _ in range(100):
omni.kit.commands.execute("RosBridgeTickComponent", path="/ROS_Clock")
simulation_context.step()
last_pub_time = rospy.Time.now()
simulation_context.stop()
# fixme IDK why this is necessary sometimes
try:
parent.start()
except:
print("Failed to start roslaunch, retry")
try:
parent.start()
except:
print("Failed to start roslaunch, exit")
exit(1)
print("ros node launched")
kit.update()
meters_per_unit = UsdGeom.GetStageMetersPerUnit(stage)
# use rtx while setting up!
set_raytracing_settings(config["physics_hz"].get())
env_prim_path = environment.load_and_center(config["env_prim_path"].get())
process_semantics(config["env_prim_path"].get())
randomize_and_fix_lights(config["_random_light"].get(), rng, env_prim_path, environment.env_limits[-1] - 0.2,
environment.meters_per_unit, is_rtx=config["rtx_mode"].get())
randomize_roughness(config["_random_roughness"].get(), rng, env_prim_path)
# set timeline of the experiment
timeline = setup_timeline(config)
ros_camera_list = []
ros_transform_components = [] # list of tf and joint components, one (of each) for each robot
viewport_window_list = []
dynamic_prims = []
imus_handle_list = []
robot_odom_frames = []
robot_imu_frames = []
camera_pose_frames = []
imu_pubs = []
odom_pubs = []
cam_pose_pubs = []
irotate_cam_odom_pubs = []
irotate_cam_odom_frames = []
irotate_differential_odom_frames = []
lidar_components = []
first = True
imu_sensor, imu_props = setup_imu_sensor(config)
simulation_context.play()
for _ in range(100):
omni.kit.commands.execute("RosBridgeTickComponent", path="/ROS_Clock")
simulation_context.step()
last_pub_time = rospy.Time.now()
simulation_context.stop()
print("Generating map...")
if add_colliders(env_prim_path):
simulation_context.play()
x, y, z, yaw = position_object(environment, type=3)
environment.generate_map(out_dir, origin=[x[0], y[0], 0])
for _ in range(10):
simulation_context.step()
timeline.set_current_time(0) # set to 0 to be sure that the first frame is recorded
else:
simulation_context.play()
for _ in range(10):
simulation_context.step()
print("Error generating collisions", file=sys.stderr)
simulation_context.play()
_dc = dynamic_control_interface()
print("Loading robots..")
robot_base_prim_path = config["robot_base_prim_path"].get()
usd_robot_path = [str(i) for i in config["usd_robot_path"].get()]
c_pose = []
old_pose = []
old_h_ap = []
old_v_ap = []
is_irotate = np.array(config["is_iRotate"].get())
for n in range(config["num_robots"].get()):
simulation_context.stop()
import_robot(robot_base_prim_path, n, usd_robot_path[n], local_file_prefix)
if is_irotate[n]:
x, y, z, yaw = 0, 0, 0, 0
else:
x, y, z, yaw = get_valid_robot_location(environment, first)
simulation_context.stop()
set_drone_joints_init_loc(f"{robot_base_prim_path}{n}", [x / meters_per_unit, y / meters_per_unit, z / meters_per_unit],
[0, 0, yaw],
(environment.env_limits[5]) / meters_per_unit, 0.3/meters_per_unit, is_irotate[n])
c_pose.append([x, y, z])
old_pose.append([x, y, z])
kit.update()
simulation_context.play()
kit.update()
add_ros_components(robot_base_prim_path, n, ros_transform_components, ros_camera_list, viewport_window_list,
camera_pose_frames, cam_pose_pubs, imus_handle_list, imu_pubs, robot_imu_frames,
robot_odom_frames, odom_pubs,
dynamic_prims, config, imu_sensor, imu_props, old_h_ap, old_v_ap, is_irotate[n])
if is_irotate[n]:
add_irotate_ros_components(irotate_cam_odom_frames, irotate_cam_odom_pubs, lidar_components, robot_base_prim_path,
n)
irotate_differential_odom_frames.append(robot_odom_frames[-1])
kit.update()
first = False
for n in range(config["num_robots"].get()):
add_npy_viewport(viewport_window_list, robot_base_prim_path, n, old_h_ap, old_v_ap, config, config["num_robots"].get()*1)
for _ in range(50):
simulation_context.render()
print("Loading robot complete")
for index, cam in enumerate(viewport_window_list):
camera = stage.GetPrimAtPath(cam.get_active_camera())
camera.GetAttribute("horizontalAperture").Set(old_h_ap[index])
camera.GetAttribute("verticalAperture").Set(old_v_ap[index])
# IMU not necessary as it is NOT a ROS component itself
for component in ros_camera_list:
omni.kit.commands.execute("RosBridgeTickComponent", path=str(component.GetPath()))
for component in ros_transform_components:
omni.kit.commands.execute("RosBridgeTickComponent", path=str(component.GetPath()))
print("Starting FSM - setting up topics...")
start_explorer_pubs = []
send_waypoint_pubs = []
movement_monitor_pubs = []
for index, _ in enumerate(robot_odom_frames):
print("Waiting for fsm to start for robot {}".format(index))
my_topic = f"{robot_base_prim_path}{index}/exploration_node/fsm_exploration/state"
if config["autonomous"].get():
rospy.Subscriber(my_topic, String, callback=autostart_exploration, callback_args=index)
start_explorer_pubs.append(
rospy.Publisher(f"{robot_base_prim_path}{index}/traj_start_trigger", PoseStamped, queue_size=10))
else:
rospy.Subscriber(my_topic, String, callback=publish_random_goal, callback_args=(index, environment))
send_waypoint_pubs.append(
rospy.Publisher(f"{robot_base_prim_path}{index}/exploration_node/manual_goal", PoseStamped,
queue_size=10))
rospy.Subscriber(my_topic, String, callback=monitor_movement, callback_args=(index, environment))
movement_monitor_pubs.append(
rospy.Publisher(f"{robot_base_prim_path}{index}/command/pose", PoseStamped, queue_size=10))
print("fsm management for robot {} setted up".format(index))
print("FSM setted up")
print("Loading humans..")
my_humans = []
my_humans_heights = []
human_export_folder = config["human_path"].get()
human_folders = os.listdir(human_export_folder)
tot_area = 0
areas = []
initial_dynamics = len(dynamic_prims)
used_ob_stl_paths = []
## todo cycle to complete area, need to update the service probably
n = 0
human_anim_len = []
added_prims = []
human_base_prim_path = config["human_base_prim_path"].get()
n_humans_loading = rng.integers(7, 1 + max(7, config["num_humans"].get()))
while n < n_humans_loading:
anim_len = 0
# the animation needs to be shorter than config["max_anim_len"].get() and longer than 0/min_len
while anim_len < max(config["min_human_anim_len"].get(), 0) or anim_len > config["max_human_anim_len"].get():
folder = rng.choice(human_folders)
random_name = rng.choice(os.listdir(os.path.join(human_export_folder, folder)))
asset_path = local_file_prefix + os.path.join(human_export_folder, folder, random_name,
random_name + ".usd")
tmp_pkl = pkl.load(open(os.path.join(human_export_folder, folder, random_name, random_name + ".pkl"), 'rb'))
anim_len = tmp_pkl['ef']
print("Loading human {} from {}".format(random_name, folder))
used_ob_stl_paths.append(os.path.join(human_export_folder, folder, random_name, random_name + ".stl"))
human_anim_len.append(tmp_pkl['ef'])
if "verts" in tmp_pkl.keys():
my_humans_heights.append(tmp_pkl['verts'][:, :, 2])
else:
my_humans_heights.append(None)
my_humans.append(random_name)
load_human(human_base_prim_path, n, asset_path, dynamic_prims, added_prims)
stl_path = os.path.join(human_export_folder, folder, random_name, random_name + ".stl")
this_mesh = mesh.Mesh.from_file(stl_path)
areas.append((this_mesh.x.max() - this_mesh.x.min()) * (this_mesh.y.max() - this_mesh.y.min()))
tot_area += areas[-1]
# if not config["use_area"].get():
n += 1
# if env_area / area_polygon * 100 > config["area_percentage"].get():
# break
x, y, z, yaw = position_object(environment, type=1, objects=my_humans, ob_stl_paths=used_ob_stl_paths,
max_collisions=int(config["allow_collision"].get()))
to_be_removed = []
human_prim_list = []
body_origins = []
for n, human in enumerate(my_humans):
if z[n] < 0:
to_be_removed.append(n)
tot_area -= areas[n]
else:
set_translate(stage.GetPrimAtPath(f"{human_base_prim_path}{n}"),
[x[n] / meters_per_unit, y[n] / meters_per_unit, z[n] / meters_per_unit])
set_rotate(stage.GetPrimAtPath(f"{human_base_prim_path}{n}"), [0, 0, yaw[n]])
human_prim_list.append(f"{human_base_prim_path}{n}")
body_origins.append([x[n], y[n], z[n], yaw[n]])
if len(to_be_removed) > 0:
print("Removing humans that are out of the environment")
to_be_removed.reverse()
cumsum = np.cumsum(added_prims)
for n in to_be_removed:
my_humans.pop(n)
used_ob_stl_paths.pop(n)
my_humans_heights.pop(n)
for _ in range(added_prims[n]):
if n > 0:
dynamic_prims.pop(cumsum[n - 1] + initial_dynamics)
else:
dynamic_prims.pop(initial_dynamics)
human_anim_len.pop(n)
omni.kit.commands.execute("DeletePrimsCommand", paths=[f"{human_base_prim_path}{n}" for n in to_be_removed])
print("Loading human complete")
google_ob_used, shapenet_ob_used = load_objects(config, environment, rng, dynamic_prims)
# IT IS OF CRUCIAL IMPORTANCE THAT AFTER THIS POINT THE RENDER GETS DONE WITH THE SLEEPING CALL! OTHERWISE PATH TRACING SPP WILL GET RUINED
if (config["rtx_mode"].get()):
set_raytracing_settings(config["physics_hz"].get())
else:
set_pathtracing_settings(config["physics_hz"].get())
omni.usd.get_context().get_selection().set_selected_prim_paths([], False)
simulation_context.stop()
simulation_context.play()
for _ in range(5):
simulation_context.step(render=False)
sleeping(simulation_context, viewport_window_list, raytracing=config["rtx_mode"].get())
timeline.set_current_time(0)
simulation_step = 0 # this is NOT the frame, this is the "step" (related to physics_hz)
my_recorder = recorder_setup(config['_recorder_settings'].get(), out_dir_npy, config['record'].get(),
config["num_robots"].get() * 1)
timeline.set_current_time(0) # set to 0 to be sure that the first frame is recorded
timeline.set_auto_update(False)
first_start = False
second_start = False
can_change_second_start = False
omni.kit.commands.execute("RosBridgeUseSimTime", use_sim_time=True)
omni.kit.commands.execute("RosBridgeUsePhysicsStepSimTime", use_physics_step_sim_time=True)
# two times, this will ensure that totalSpp is reached
sleeping(simulation_context, viewport_window_list, raytracing=config["rtx_mode"].get())
sleeping(simulation_context, viewport_window_list, raytracing=config["rtx_mode"].get())
last_pub_time = rospy.Time.now()
last_check_time = rospy.Time.now()
if config['debug_vis'].get():
cnt = 0
while 1:
cnt += 1
if cnt % 10000 == 0:
import ipdb
ipdb.set_trace()
print("DEBUGGING VIS")
sleeping(simulation_context, viewport_window_list, raytracing=config["rtx_mode"].get())
for i, cam in enumerate(ros_camera_list):
omni.kit.commands.execute("RosBridgeTickComponent", path=str(cam.GetPath()))
reversing_timeline_ratio = compute_timeline_ratio(human_anim_len, config["reverse_strategy"].get(),
experiment_length)
print(
f"The reversing ratio is {reversing_timeline_ratio}.\n"
f"This implies that that every {experiment_length / reversing_timeline_ratio} frames we reverse the animations")
cnt_reversal = 1
ratio_camera = config["ratio_camera"].get()
ratio_odom = config["ratio_odom"].get()
ratio_tf = config["ratio_tf"].get()
starting_to_pub = False
my_recorder._enable_record = False
while kit.is_running():
if can_start:
last_check_time = rospy.Time.now()
if second_start:
if config['record'].get():
sleeping(simulation_context, viewport_window_list, raytracing=config["rtx_mode"].get())
my_recorder._update()
sleeping(simulation_context, viewport_window_list, raytracing=config["rtx_mode"].get())
starting_to_pub = True
timeline.set_current_time(min(- 1 / (config["physics_hz"].get() / ratio_camera),
-abs(config["bootstrap_exploration"].get())))
simulation_step = int(timeline.get_current_time() * config["physics_hz"].get()) - 1
print("Bootstrap started")
can_start = False
simulation_step += 1
if starting_to_pub and simulation_step == 0:
move_humans_to_ground(my_humans_heights, human_prim_list, simulation_step / ratio_camera, meters_per_unit,
config["max_distance_human_ground"].get())
print("Starting recording NOW!")
msg = String("starting")
starting_pub.publish(msg)
starting_to_pub = False
time.sleep(0.5)
if config['record'].get():
my_recorder._enable_record = True
last_check_time = rospy.Time.now()
if (config["_random_light"].get()["during_experiment"]):
if (simulation_step % config["_random_light"].get()["n-frames"] == 0):
# fixme todo smooth change, idea get max-min and time window
randomize_and_fix_lights(config["_random_light"].get(), rng, env_prim_path, environment.env_limits[-1],
environment.meters_per_unit, is_rtx=config["rtx_mode"].get())
# step the physics
simulation_context.step(render=False)
# get the current time in ROS
print("Clocking...")
omni.kit.commands.execute("RosBridgeTickComponent", path="/ROS_Clock")
time.sleep(0.2)
# publish IMU
print("Publishing IMU...")
pub_imu(imus_handle_list, imu_sensor, imu_pubs, robot_imu_frames, meters_per_unit)
# publish joint status (ca 120 Hz)
if simulation_step % ratio_tf == 0:
print("Publishing joint/tf status...")
for component in ros_transform_components:
omni.kit.commands.execute("RosBridgeTickComponent", path=str(component.GetPath()))
# publish odometry (60 hz)
if simulation_step % ratio_odom == 0:
print("Publishing odometry...")
c_pose, _ = pub_odom(robot_odom_frames, odom_pubs, _dc, meters_per_unit)
pub_cam_pose(camera_pose_frames, cam_pose_pubs, _dc, meters_per_unit)
_, _ = pub_odom(irotate_cam_odom_frames, irotate_cam_odom_pubs, _dc, meters_per_unit,
irotate_differential_odom_frames)
for component in lidar_components:
omni.kit.commands.execute("RosBridgeTickComponent", path=str(component.GetPath()))
# we consider ratio_camera to forward the animation.
# If you want it different ratio_animation < ratio_camera to avoid
# two frames with the same animation point
if second_start:
if simulation_step % ratio_camera == 0:
if my_recorder._enable_record:
# update the image counter externally so that we can use it in the recorder and all images have the same index
my_recorder._counter += 1
if simulation_step / ratio_camera < (experiment_length / reversing_timeline_ratio) * (
cnt_reversal):
timeline.forward_one_frame()
else:
if simulation_step / ratio_camera >= ((experiment_length - 1) / reversing_timeline_ratio) * (
cnt_reversal + 1) or \
(timeline.get_current_time() - 1 / timeline.get_time_codes_per_seconds()) < 0:
cnt_reversal += 2
timeline.forward_one_frame()
else:
timeline.rewind_one_frame()
# publish camera (30 hz)
if simulation_step % ratio_camera == 0:
print("Publishing cameras...")
# getting skel pose for each joint
# get_skeleton_info(meters_per_unit, body_origins, body_list)
# FIRST ONE WRITTEN IS AT 1/30 on the timeline
pub_and_write_images(simulation_context, viewport_window_list,
ros_camera_list, config["rtx_mode"].get(),
my_recorder, second_start)
if simulation_step % ratio_camera == 0 and simulation_step / ratio_camera == experiment_length \
and not config["neverending"].get():
print("End of experiment!!!")
simulation_context.pause()
if my_recorder.data_writer is not None:
my_recorder.data_writer.stop_threads()
timeline.set_current_time(0)
context.save_as_stage(os.path.join(out_dir, "loaded_stage.usd"))
experiment_info = {}
experiment_info["config"] = config
experiment_info["reversing_timeline_ratio"] = reversing_timeline_ratio
experiment_info["humans"] = {}
experiment_info["humans"]["ids"] = my_humans
experiment_info["humans"]["folders"] = used_ob_stl_paths
experiment_info["humans"]["origins"] = body_origins # x y z yaw
experiment_info["google_obs"] = google_ob_used
experiment_info["shapenet_obs"] = shapenet_ob_used
experiment_info["environment"] = {}
experiment_info["environment"]["id"] = environment.env_name
experiment_info["environment"]["folder"] = environment.env_path
experiment_info["environment"]["shifts"] = environment.shifts
experiment_info["rng_state"] = rng_state
np.save(os.path.join(out_dir, "experiment_info.npy"), experiment_info)
break
except:
extype, value, tb = sys.exc_info()
traceback.print_exc()
# ipdb.post_mortem(tb)
finally:
for pub in odom_pubs:
pub.unregister()
for pub in imu_pubs:
pub.unregister()
for pub in cam_pose_pubs:
pub.unregister()
for pub in start_explorer_pubs:
pub.unregister()
for pub in send_waypoint_pubs:
pub.unregister()
parent.shutdown()
rospy.signal_shutdown("my_simulation complete")
simulation_context.stop()
try:
kit.close()
except:
pass
| 26,681 | Python | 39.550152 | 144 | 0.639331 |
eliabntt/GRADE-RR/simulator/first_run.py | import argparse
base_environment_path = "" # please edit this e.g. GRADE-RR/usds/env_base.usd
# necessary import
from omni.isaac.kit import SimulationApp
# simply use this to correctly parse booleans
def boolean_string(s):
if s.lower() not in {'false', 'true'}:
raise ValueError('Not a valid boolean string')
return s.lower() == 'true'
parser = argparse.ArgumentParser(description="Your first IsaacSim run")
parser.add_argument("--headless", type=boolean_string, default=True, help="Wheter to run it in headless mode or not")
parser.add_argument("--rtx_mode", type=boolean_string, default=False, help="Use rtx when True, use path tracing when False")
args, unknown = parser.parse_known_args()
config = confuse.Configuration("first_run", __name__)
config.set_args(args)
# create a kit object which is your Simulation App
CONFIG = {"display_options": 3286, "width": 1280, "height": 720, "headless": config["headless"].get()}
kit = SimulationApp(launch_config=CONFIG, experience=f"{os.environ['EXP_PATH']}/omni.isaac.sim.python.kit")
# !!! you can ONLY load Isaac modules AFTER this point !!!
# after here you can do everything that you desire
# first step is usually opening a basic stage, perhaps with some assets already in as the sky
omni.usd.get_context().open_stage(base_environment_path, None)
# Wait two frames so that stage starts loading
kit.update()
kit.update()
print("Loading stage...")
while is_stage_loading():
kit.update()
print("Loading Complete")
context = omni.usd.get_context()
stage = context.get_stage() # used to access the elements of the simulation
simulation_context = SimulationContext(physics_dt=1.0 / 60, rendering_dt=1.0 / 60, stage_units_in_meters=0.01, backend='torch')
simulation_context.initialize_physics()
physx_interface = omni.physx.acquire_physx_interface()
physx_interface.start_simulation()
for _ in range(100):
simulation_context.render()
simulation_context.step(render=False)
try:
kit.close()
except:
pass
| 1,978 | Python | 33.719298 | 127 | 0.73913 |
eliabntt/GRADE-RR/simulator/savana_simulation.py | import carb
import rospy
from omni.isaac.kit import SimulationApp
import argparse
import os
import time
import numpy as np
import roslaunch
from time import sleep
import yaml
import confuse
import ipdb, traceback, sys
def boolean_string(s):
if s.lower() not in {'false', 'true'}:
raise ValueError('Not a valid boolean string')
return s.lower() == 'true'
try:
parser = argparse.ArgumentParser(description="Dynamic Worlds Simulator")
parser.add_argument("--config_file", type=str, default="config.yaml")
parser.add_argument("--headless", type=boolean_string, default=True, help="Wheter to run it in headless mode or not")
parser.add_argument("--rtx_mode", type=boolean_string, default=False, help="Use rtx when True, use path tracing when False")
parser.add_argument("--record", type=boolean_string, default=True, help="Writing data to the disk")
parser.add_argument("--debug_vis", type=boolean_string, default=False, help="When true continuosly loop the rendering")
parser.add_argument("--neverending", type=boolean_string, default=False, help="Never stop the main loop")
parser.add_argument("--fix_env", type=str, default="",
help="leave it empty to have a random env, fix it to use a fixed one. Useful for loop processing")
args, unknown = parser.parse_known_args()
config = confuse.Configuration("DynamicWorlds", __name__)
config.set_file(args.config_file)
config.set_args(args)
can_start = True
CONFIG = {"display_options": 3286, "width": 1280, "height": 720, "headless": config["headless"].get()}
kit = SimulationApp(launch_config=CONFIG, experience=f"{os.environ['EXP_PATH']}/omni.isaac.sim.python.kit")
# Cannot move before SimApp is launched
import utils.misc_utils
from utils.misc_utils import *
from utils.robot_utils import *
from utils.simulation_utils import *
from utils.environment_utils import *
simulation_environment_setup()
# set timeline of the experiment
timeline = setup_timeline(config)
rospy.init_node("my_isaac_ros_app", anonymous=True, disable_signals=True, log_level=rospy.ERROR)
starting_pub = rospy.Publisher('starting_experiment', String)
rng = np.random.default_rng()
rng_state = np.random.get_state()
local_file_prefix = ""
# setup environment variables
meters_per_unit = config["meters_per_unit"].get()
environment = environment(config, rng, local_file_prefix, meters_per_unit)
out_dir = os.path.join(config['out_folder'].get(), environment.env_name)
out_dir_npy = os.path.join(config['out_folder_npy'].get(), environment.env_name)
if not os.path.exists(out_dir):
os.makedirs(out_dir)
os.environ["ROS_LOG_DIR"] = out_dir
omni.usd.get_context().open_stage(local_file_prefix + config["base_env_path"].get(), None)
# Wait two frames so that stage starts loading
kit.update()
kit.update()
print("Loading stage...")
while is_stage_loading():
kit.update()
print("Loading Complete")
context = omni.usd.get_context()
stage = context.get_stage()
set_stage_up_axis("Z")
if config["clean_base_env"].get():
omni.kit.commands.execute("DeletePrimsCommand", paths=["/World/GroundPlane"])
# do this AFTER loading the world
simulation_context = SimulationContext(physics_dt=1.0 / config["physics_hz"].get(),
rendering_dt=1.0 / config["render_hz"].get(),
stage_units_in_meters=0.01)
simulation_context.initialize_physics()
physx_interface = omni.physx.acquire_physx_interface()
physx_interface.start_simulation()
_clock_graph = add_clock() # add ROS clock
simulation_context.play()
for _ in range(10):
simulation_context.step()
og.Controller.evaluate_sync(_clock_graph)
last_pub_time = rospy.Time.now()
simulation_context.stop()
kit.update()
# use rtx while setting up!
set_raytracing_settings(config["physics_hz"].get())
env_prim_path = environment.load_and_center(config["env_prim_path"].get())
process_semantics(config["env_prim_path"].get())
ros_camera_list = []
ros_transform_components = [] # list of tf and joint components, one (of each) for each robot
viewport_window_list = []
dynamic_prims = []
imus_handle_list = []
robot_odom_frames = []
robot_imu_frames = []
camera_pose_frames = []
imu_pubs = []
odom_pubs = []
cam_pose_pubs = []
simulation_context.play()
for _ in range(100):
og.Controller.evaluate_sync(_clock_graph)
simulation_context.step()
print("Loading robots..")
from omni.isaac.sensor import _sensor
_is = _sensor.acquire_imu_sensor_interface()
_dc = dynamic_control_interface()
robot_base_prim_path = config["robot_base_prim_path"].get()
usd_robot_path = str(config["usd_robot_path"].get())
old_h_ap = []
old_v_ap = []
robot_init_loc = []
robot_init_ang = []
simulation_context.stop()
for n in range(config["num_robots"].get()):
import_robot(robot_base_prim_path, n, usd_robot_path, local_file_prefix)
if config["init_loc"].get()["use"]:
# assuming we go here
x = config["init_loc"].get()["x"][n]
y = config["init_loc"].get()["y"][n]
z = config["init_loc"].get()["z"][n]
yaw = np.deg2rad(config["init_loc"].get()["yaw"][n])
roll = np.deg2rad(config["init_loc"].get()["roll"][n])
pitch = np.deg2rad(config["init_loc"].get()["pitch"][n])
robot_init_loc.append([x,y,z])
robot_init_ang.append([roll, pitch, yaw])
set_drone_joints_init_loc(f"{robot_base_prim_path}{n}", [x / meters_per_unit, y / meters_per_unit, z / meters_per_unit], [roll, pitch, yaw],
(environment.env_limits[5]) / meters_per_unit)
add_ros_components(robot_base_prim_path, n, ros_transform_components, ros_camera_list, viewport_window_list,
camera_pose_frames, cam_pose_pubs, imu_pubs, robot_imu_frames,
robot_odom_frames, odom_pubs, None, #lidars = None
dynamic_prims, config, old_h_ap, old_v_ap, _is, simulation_context, _clock_graph)
kit.update()
if config["use_robot_traj"].get():
add_robot_traj(f"{robot_base_prim_path}{n}",config,meters_per_unit,timeline.get_time_codes_per_seconds())
for n in range(config["num_robots"].get()):
add_npy_viewport(viewport_window_list, robot_base_prim_path, n, old_h_ap, old_v_ap, config, simulation_context,
config["num_robots"].get())
for _ in range(50):
simulation_context.render()
print("Loading robot complete")
print("WARNING: CAMERA APERTURE MANUAL SET NO LONGER WORKS, NEEDS TO BE FIXED BY NVIDIA!!!!")
time.sleep(5)
for index, cam in enumerate(viewport_window_list):
camera = stage.GetPrimAtPath(cam.get_active_camera())
camera.GetAttribute("horizontalAperture").Set(old_h_ap[index])
camera.GetAttribute("verticalAperture").Set(old_v_ap[index])
# IT IS OF CRUCIAL IMPORTANCE THAT AFTER THIS POINT THE RENDER GETS DONE WITH THE SLEEPING CALL! OTHERWISE PATH TRACING SPP WILL GET RUINED
if (config["rtx_mode"].get()):
set_raytracing_settings(config["physics_hz"].get())
else:
set_pathtracing_settings(config["physics_hz"].get())
omni.usd.get_context().get_selection().set_selected_prim_paths([], False)
simulation_context.stop()
simulation_context.play()
for _ in range(5):
simulation_context.step(render=False)
sleeping(simulation_context, viewport_window_list, config["rtx_mode"].get())
timeline.set_current_time(0)
simulation_step = 0 # this is NOT the frame, this is the "step" (related to physics_hz)
my_recorder = recorder_setup(config['_recorder_settings'].get(), out_dir_npy, config['record'].get())
timeline.set_current_time(0) # set to 0 to be sure that the first frame is recorded
timeline.set_auto_update(False)
# two times, this will ensure that totalSpp is reached
sleeping(simulation_context, viewport_window_list, config["rtx_mode"].get())
sleeping(simulation_context, viewport_window_list, config["rtx_mode"].get())
if config['debug_vis'].get():
cnt = 0
while 1:
cnt += 1
if cnt % 10000 == 0:
import ipdb
ipdb.set_trace()
print("DEBUGGING VIS")
simulation_context.step(render=False)
simulation_context.step(render=True)
sleeping(simulation_context, viewport_window_list, config["rtx_mode"].get())
for i, cam in enumerate(ros_camera_list):
omni.kit.commands.execute("RosBridgeTickComponent", path=str(cam.GetPath()))
ratio_camera = config["ratio_camera"].get()
ratio_odom = config["ratio_odom"].get()
ratio_tf = config["ratio_tf"].get()
starting_to_pub = False
my_recorder._enable_record = False
forward = True
goal_list = []
exp_len = config["anim_exp_len"].get()
if not config["use_robot_traj"].get() and config["use_joint_traj"].get():
for elem in config["robot_traj"].get():
goal_list.append([elem["pose"]["x"], elem["pose"]["y"], elem["pose"]["z"],
elem["pose"]["roll"], elem["pose"]["pitch"], elem["pose"]["yaw"]])
while kit.is_running():
if can_start:
if config['record'].get():
# reload_references("/World/home")
sleeping(simulation_context, viewport_window_list, config["rtx_mode"].get())
my_recorder._update()
sleeping(simulation_context, viewport_window_list, config["rtx_mode"].get())
starting_to_pub = True
timeline.set_current_time(min(- 1 / (config["physics_hz"].get() / ratio_camera),
-abs(config["bootstrap_exploration"].get())))
simulation_step = int(timeline.get_current_time() * config["physics_hz"].get()) - 1
print("Bootstrap started")
can_start = False
simulation_step += 1
if starting_to_pub and simulation_step == 0:
print("Starting recording NOW!")
msg = String("starting")
starting_pub.publish(msg)
time.sleep(0.5)
starting_to_pub = False
if config['record'].get():
my_recorder._enable_record = True
# step the physics
simulation_context.step(render=False)
# get the current time in ROS
print("Clocking...")
og.Controller.evaluate_sync(_clock_graph)
ctime = timeline.get_current_time()
simulation_context.render()
timeline.set_current_time(ctime)
# publish IMU
print("Publishing IMU...")
pub_imu(_is, imu_pubs, robot_imu_frames, meters_per_unit)
# publish joint status (ca 120 Hz)
if simulation_step % ratio_tf == 0:
print("Publishing joint/tf status...")
for component in ros_transform_components:
og.Controller.set(og.Controller.attribute(f"{component}/OnImpulseEvent.state:enableImpulse"), True)
# publish odometry (60 hz)
if simulation_step % ratio_odom == 0:
print("Publishing odometry...")
c_pose, c_angle = pub_odom(robot_odom_frames, odom_pubs, _dc, meters_per_unit)
pub_cam_pose(camera_pose_frames, cam_pose_pubs, _dc, meters_per_unit)
if config["use_joint_traj"].get():
if len(goal_list)>0 and simulation_step >= 0:
# this needs to be expanded to multiple robots
goal_list = check_pose_and_goals(robot_init_loc[0], robot_init_ang[0], c_pose[0], c_angle[0], "/my_robot_0", goal_list, meters_per_unit, simulation_step == 0)
if len(goal_list)==0:
break
# we consider ratio_camera to forward the animation.
# If you want it different ratio_animation < ratio_camera to avoid
# two frames with the same animation point
if simulation_step % ratio_camera == 0:
if my_recorder._enable_record:
# update the image counter externally so that we can use it in the recorder and all images have the same index
my_recorder._counter += 1
if (simulation_step > 0 and (simulation_step / ratio_camera + 1) % exp_len == 0):
forward = not forward
if (timeline.get_current_time() - 1 / timeline.get_time_codes_per_seconds()<0):
forward = True
if forward:
timeline.forward_one_frame()
else:
timeline.rewind_one_frame()
# publish camera (30 hz)
if simulation_step % ratio_camera == 0:
ctime = timeline.get_current_time()
print("Publishing cameras...")
pub_and_write_images(simulation_context, viewport_window_list,
ros_camera_list, config["rtx_mode"].get(), my_recorder)
timeline.set_current_time(ctime)
except:
extype, value, tb = sys.exc_info()
traceback.print_exc()
# ipdb.post_mortem(tb)
finally:
for pub in odom_pubs:
pub.unregister()
for pub in imu_pubs:
pub.unregister()
for pub in cam_pose_pubs:
pub.unregister()
parent.shutdown()
rospy.signal_shutdown("my_simulation complete")
simulation_context.stop()
try:
kit.close()
except:
pass
| 13,742 | Python | 39.780415 | 174 | 0.613375 |
eliabntt/GRADE-RR/simulator/configs/config_multi_robot.yaml | # GENERAL NOTE the paths need to be ABSOLUTE!
env_path: "/media/ebonetto/WindowsData/Demo_GRADE"
use_stl: True # this disable stl loading thus placement AND robot heading
human_path: "/ps/project/irotate/cloth3d/exported_usd/"
base_env_path: "/home/ebonetto/.local/share/ov/pkg/isaac_sim-2021.2.1/isaac_sim_manager/usds/empty.usd"
clean_base_env: False
only_placement: False
robot_mesh_path: ["/home/ebonetto/.local/share/ov/pkg/isaac_sim-2021.2.1/isaac_sim_manager/meshes/robotino.dae",
"/home/ebonetto/.local/share/ov/pkg/isaac_sim-2021.2.1/isaac_sim_manager/meshes/drone.dae","/home/ebonetto/.local/share/ov/pkg/isaac_sim-2021.2.1/isaac_sim_manager/meshes/drone.dae"]
usd_robot_path: ["/home/ebonetto/.local/share/ov/pkg/isaac_sim-2021.2.1/multi_robot/usds/robotino.usd",
"/home/ebonetto/.local/share/ov/pkg/isaac_sim-2021.2.1/isaac_sim_manager/usds/drone_paper.usd",
"/home/ebonetto/.local/share/ov/pkg/isaac_sim-2021.2.1/isaac_sim_manager/usds/drone_paper.usd"]
out_folder: "/media/ebonetto/WindowsData/exp_out2"
out_folder_npy: "/media/ebonetto/WindowsData/exp_out2"
#out_folder: "/home/ebonetto/exp_out"
fps: 30
num_robots: 3
num_humans: 40
max_distance_human_ground: 0.1 # max distance from human to ground to be consider to force the first frame grounding of animations
allow_collision: 200
experiment_length: 3600 # camera frames length
autonomous: True # true -> FUEL, false -> random goals
obstacles: {
"shapenet": 0,
"google": 0
}
physics_hz: 240 # NOTE THAT THIS IS THE RATE OF CLOCK AND IMU
render_hz: 240 # LEAVE IT EQUAL TO PHYSICS HZ
ratio_tf: 2 # physics_hz/ratio_tf = tf publish hz
ratio_odom: 4 # physics_hz/ratio_odom = odom publish hz
ratio_camera: 8 # physics_hz/ratio_cam = imgs publish hz
bootstrap_exploration: 1 # seconds to boostrap exploration (min(abs(this_value), 1/(physics_hz/ratio_camera))
reverse_strategy: "avg" # in [min, max, avg, half, none], works only with animated sequences
robot_sensor_size: [ 640, 480 ]
npy_sensor_size: [ 640, 480]
_random_light: { "intensity": True,
"color": True,
"intensity_interval": [ 600, 4500 ], # [min, max], for rtx
"during_experiment": False,
"n-frames": 24,
"smooth": False }
_random_roughness: {
"enabled": True,
"intensity_interval": [ 0.1, 0.9 ]
}
env_prim_path: "/World/home"
robot_base_prim_path: "/my_robot_"
human_base_prim_path: "/my_human_"
max_human_anim_len: 500 # max human anim length to be considered in frames
min_human_anim_len: 50 # min human anim length to be loaded in frames
_recorder_settings: {
"rgb": { "enabled": True },
"depth": { "enabled": False, "colorize": False, "npy": True },
"depthLinear": { "enabled": True, "colorize": False, "npy": True },
"instance": { "enabled": True, "colorize": False, "npy": True, "mappings": True },
"semantic": { "enabled": False, "colorize": False, "npy": True, "mappings": True },
"bbox_2d_tight": { "enabled": True, "colorize": False, "npy": True },
"bbox_2d_loose": { "enabled": True, "colorize": False, "npy": True },
"normals": { "enabled": False, "colorize": False, "npy": True },
"motion-vector": { "enabled": False, "colorize": True, "npy": True },
"bbox_3d": { "enabled": True, "colorize": False, "npy": True },
"camera": { "enabled": True, "colorize": True, "npy": True },
"poses": { "enabled": True, "colorize": True, "npy": True },
}
google_obj_folder: "/ps/project/irotate/google_scanned_objects"
google_obj_shortlist: ""
shapenet_local_dir: "/ps/project/irotate/ShapeNet"
shapenet_username: ""
shapenet_password: ""
synsetId: "random"
modelId: "random"
is_iRotate: [True, False, False] | 3,691 | YAML | 50.277777 | 182 | 0.678136 |
eliabntt/GRADE-RR/simulator/configs/config_paper.yaml | # GENERAL NOTE the paths need to be ABSOLUTE!
env_path: "/ps/project/irotate/3DFRONT/USD-exports"
use_stl: True # this disable stl loading thus placement AND initial robot heading
use_npy: True # use limits of the environment saved in the npy file located in the same folder of the environment
meters_per_unit: 0.01
human_path: "/ps/project/irotate/cloth3d/exported_usd/"
base_env_path: "/media/ebonetto/WindowsData/ov/isaac_sim-2022.2.1/GRADE-RR/usds/env_base.usd"
reload_references: False
generate_map: True
clean_base_env: False
only_placement: False
robot_mesh_path: "/media/ebonetto/WindowsData/ov/isaac_sim-2022.2.1/GRADE-RR/meshes/drone.dae"
usd_robot_path: "/media/ebonetto/WindowsData/ov/isaac_sim-2022.2.1/GRADE-RR/usds/drone_2022.usd"
out_folder: "/media/ebonetto/WindowsData/exp_out2/"
out_folder_npy: "/media/ebonetto/WindowsData/exp_out2/"
fps: 30
num_robots: 1
num_humans: 40
max_distance_human_ground: 0.1 # max distance from human to ground to be consider to force the first frame grounding of animations
allow_collision: 200
experiment_length: 1800 # camera frames length
autonomous: True # true -> FUEL, false -> random goals
obstacles: {
"shapenet": 0, # 5, 10
"google": 1
}
physics_hz: 240 # NOTE THAT THIS IS THE RATE OF CLOCK AND IMU
render_hz: 240 # LEAVE IT EQUAL TO PHYSICS HZ
ratio_tf: 2 # physics_hz/ratio_tf = tf publish hz
ratio_odom: 4 # physics_hz/ratio_odom = odom publish hz
ratio_camera: 8 # physics_hz/ratio_cam = imgs publish hz
bootstrap_exploration: 1 # seconds to boostrap exploration (min(abs(this_value), 1/(physics_hz/ratio_camera))
reverse_strategy: "avg" # in [min, max, avg, half, none], works only with animated sequences
robot_sensor_size: [ 640, 480 ]
npy_sensor_size: [ 1920, 1080]
_random_light: { "intensity": True,
"color": True,
"intensity_interval": [ 600, 4500 ], # [min, max], for rtx
"during_experiment": False,
"n-frames": 24,
"smooth": False }
_random_roughness: {
"enabled": True,
"intensity_interval": [ 0.1, 0.9 ]
}
env_prim_path: "/World/home"
robot_base_prim_path: "/my_robot_"
human_base_prim_path: "/my_human_"
max_human_anim_len: 500 # max human anim length to be considered in frames
min_human_anim_len: 50 # min human anim length to be loaded in frames
_recorder_settings: {
"rgb": { "enabled": True },
"depth": { "enabled": False, "colorize": False, "npy": True },
"depthLinear": { "enabled": True, "colorize": False, "npy": True },
"instance": { "enabled": True, "colorize": False, "npy": True, "mappings": True },
"semantic": { "enabled": False, "colorize": False, "npy": True, "mappings": True },
"bbox_2d_tight": { "enabled": True, "colorize": False, "npy": True },
"bbox_2d_loose": { "enabled": True, "colorize": False, "npy": True },
"normals": { "enabled": False, "colorize": False, "npy": True },
"motion-vector": { "enabled": False, "colorize": True, "npy": True },
"bbox_3d": { "enabled": True, "colorize": False, "npy": True },
"camera": { "enabled": True, "colorize": True, "npy": True },
"poses": { "enabled": True, "colorize": True, "npy": True },
}
google_obj_folder: "/ps/project/irotate/google_scanned_objects"
google_obj_shortlist: ""
shapenet_local_dir: "/ps/project/irotate/ShapeNet"
shapenet_username: ""
shapenet_password: ""
synsetId: "random"
modelId: "random"
is_iRotate: False
| 3,391 | YAML | 45.465753 | 130 | 0.6821 |
eliabntt/GRADE-RR/simulator/configs/config_savana.yaml | # GENERAL NOTE the paths need to be ABSOLUTE!
env_path: "/media/ebonetto/WindowsData/Demo_GRADE"
use_stl: False # this disable stl loading thus placement AND robot heading
base_env_path: "/media/ebonetto/WindowsData/ov/isaac_sim-2022.2.1/GRADE-RR/usds/env_base.usd"
clean_base_env: True
only_placement: True
robot_mesh_path: "/media/ebonetto/WindowsData/ov/isaac_sim-2022.2.1/GRADE-RR/meshes/drone.dae"
usd_robot_path: "/media/ebonetto/WindowsData/ov/isaac_sim-2022.2.1/GRADE-RR/usds/drone_2022.usd"
out_folder: "/media/ebonetto/WindowsData/exp_out2022"
out_folder_npy: "/media/ebonetto/WindowsData/exp_out2022"
fps: 30
num_robots: 1
anim_exp_len: 450 # after how many frames we should roll back the animations
physics_hz: 240 # NOTE THAT THIS IS THE RATE OF CLOCK AND IMU
render_hz: 240 # LEAVE IT EQUAL TO PHYSICS HZ
ratio_tf: 2 # physics_hz/ratio_tf = tf publish hz
ratio_odom: 4 # physics_hz/ratio_odom = odom publish hz
ratio_camera: 8 # physics_hz/ratio_cam = imgs publish hz
bootstrap_exploration: 1 # seconds to boostrap exploration (min(abs(this_value), 1/(physics_hz/ratio_camera))
robot_sensor_size: [ 640, 480 ]
npy_sensor_size: [ 1920,1080]
env_prim_path: "/World/home"
robot_base_prim_path: "/my_robot_"
use_npy: True
meters_per_unit: 0.01
_recorder_settings: {
"rgb": { "enabled": True },
"depth": { "enabled": True, "colorize": False, "npy": True },
"depthLinear": { "enabled": True, "colorize": False, "npy": True },
"instance": { "enabled": True, "colorize": False, "npy": True, "mappings": True },
"semantic": { "enabled": False, "colorize": False, "npy": True, "mappings": True },
"bbox_2d_tight": { "enabled": True, "colorize": False, "npy": True },
"bbox_2d_loose": { "enabled": True, "colorize": False, "npy": True },
"normals": { "enabled": False, "colorize": False, "npy": True },
"motion-vector": { "enabled": False, "colorize": True, "npy": True },
"bbox_3d": { "enabled": True, "colorize": False, "npy": True },
"camera": { "enabled": True, "colorize": True, "npy": True },
"poses": { "enabled": True, "colorize": True, "npy": True },
}
# the following cannot be both true at the same time
# if so, only the robot traj will be executed
# if both false we assume an external source is publishing something to your robot (in our case on /my_robot_0/joint_commands)
use_robot_traj: False # this is an absolute value. Note that the main root link and the actual position of the robot may differ based on the initial shift(which remains constant)
use_joint_traj: True # this is a relative value w.r.t. the starting location
robot_traj: # remember that movement will be linear and instantaneous. No acceleration or anything. This implies no odom, nor IMU data. If you want those, please add the same trajectory to a joint publisher.
- {pose: {x: 0, y: 0, z: 0, roll: 0, pitch: 0, yaw: 0}, time: 0}
- {pose: {x: -16.61, y: 4.4, z: 6.55, roll: 20, pitch: -10, yaw: 15}, time: 2}
- {pose: {x: -58.83, y: 11.00, z: -2.67, roll: 4, pitch: -22, yaw: 60}, time: 3}
- {pose: {x: 56.38, y: -55.85, z: 45.23, roll: -10, pitch: 30, yaw: 120}, time: 7}
- {pose: {x: -20.95, y: -37.64, z: -4.46, roll: 10, pitch: 50, yaw: 240}, time: 10}
- {pose: {x: 0, y: 0, z: 0, roll: 0, pitch: 0, yaw: 0}, time: 0}
init_loc: {
"use": [True],
"x": [26.35],
"y": [241.43],
"z": [8.57],
"yaw": [271],
"roll": [0],
"pitch": [0]
} | 3,373 | YAML | 54.311475 | 207 | 0.664987 |
eliabntt/GRADE-RR/simulator/configs/humans_and_objects.yaml | # GENERAL NOTE the paths need to be ABSOLUTE!
physics_hz: 240 # the size of a single physics step
render_hz: 240 # not influencing anything for now
base_env_path: "" # the base environment, e.g. GRADE-RR/usds/env_base.usd
env_path: "" # the parent folder that contains your environments, in subfolders
use_stl: True #
use_npy: True #
meters_per_unit: 0.01 # how many meters are in one single unit in the simulation. In this case x=1 will be 1 cm.
usd_robot_path: "" # the usd path of the robot, e.g. GRADE-RR/usds/drone_2022.usd
num_robots: 1 # how many robots we want to load
robot_sensor_size: [640,480]
ratio_joints: 2
ratio_tf: 2 # physics_hz/ratio_tf = tf publish hz
ratio_odom: 4 # physics_hz/ratio_odom = odom publish hz
ratio_camera: 8 # physics_hz/ratio_cam = imgs publish hz
# prefixes where to load the prims
env_prim_path: "/World/home"
robot_base_prim_path: "/my_robot_" # 0, 1, 2 ... num_robots
obstacles: {
"shapenet": 0, # 5, 10
"google": 1
}
human_base_prim_path: "/my_human_"
max_human_anim_len: 500 # max human anim length to be considered in frames
min_human_anim_len: 50 # min human anim length to be loaded in frames
google_obj_folder: "" # the main folder of the google_scanned_objects models
google_obj_shortlist: "" # check out here https://github.com/eliabntt/GRADE-RR/blob/064c1b888727c6faa191f88519184dc272a8b950/simulator/utils/objects_utils.py#L55
shapenet_local_dir: "" # the main folder of the shapenet predownloaded models
shapenet_username: "" # leave empty, or find a way to download from the website dynamically
shapenet_password: ""
synsetId: "random" # check out here https://github.com/eliabntt/GRADE-RR/blob/064c1b888727c6faa191f88519184dc272a8b950/simulator/utils/objects_utils.py#L22
modelId: "random" | 1,756 | YAML | 42.924999 | 161 | 0.742027 |
eliabntt/GRADE-RR/simulator/configs/world_and_robot.yaml | # GENERAL NOTE the paths need to be ABSOLUTE!
physics_hz: 240 # the size of a single physics step
render_hz: 240 # not influencing anything for now
base_env_path: "" # the base environment, e.g. GRADE-RR/usds/env_base.usd
env_path: "" # the parent folder that contains your environments, in subfolders
use_stl: True #
use_npy: True #
meters_per_unit: 0.01 # how many meters are in one single unit in the simulation. In this case x=1 will be 1 cm.
usd_robot_path: "" # the usd path of the robot, e.g. GRADE-RR/usds/drone_2022.usd
num_robots: 1 # how many robots we want to load
# prefixes where to load the prims
env_prim_path: "/World/home"
robot_base_prim_path: "/my_robot_" # 0, 1, 2 ... num_robots | 706 | YAML | 38.277776 | 112 | 0.715297 |
eliabntt/GRADE-RR/simulator/configs/config.yaml | # GENERAL NOTE the paths need to be ABSOLUTE!
env_path: "3DFRONT/USD-exports"
#env_path: "/media/ebonetto/WindowsData/Demo_GRADE"
use_stl: True # this disable stl loading thus placement AND robot heading
human_path: "cloth3d/exported_usd/"
base_env_path: "../usds/env_base.usd"
meters_per_unit: 1
clean_base_env: False
robot_mesh_path: "../meshes/drone.dae"
usd_robot_path: "../usds/drone.usd"
out_folder: "exp_out"
out_folder_npy: "exp_out"
num_robots: 1
num_humans: 40 # min 5, if 0 change the main code
max_distance_human_ground: 0.1 # max distance from human to ground to be consider to force the first frame grounding of animations
allow_collision: 200
experiment_length: 1800 # camera frames length
autonomous: True # true -> FUEL, false -> random goals
obstacles: {
"shapenet": 0,
"google": 0
}
physics_hz: 240 # NOTE THAT THIS IS THE RATE OF CLOCK AND IMU
render_hz: 240 # LEAVE IT EQUAL TO PHYSICS HZ
ratio_tf: 2 # physics_hz/ratio_tf = tf publish hz
ratio_odom: 4 # physics_hz/ratio_odom = odom publish hz
ratio_camera: 8 # physics_hz/ratio_cam = imgs publish hz
bootstrap_exploration: 1 # seconds to boostrap exploration (min(abs(this_value), 1/(physics_hz/ratio_camera))
reverse_strategy: "avg" # in [min, max, avg, half, none], works only with animated sequences
robot_sensor_size: [ 640, 480 ]
npy_sensor_size: [ 1920, 1080]
_random_light: { "intensity": True,
"color": True,
"intensity_interval": [ 600, 4500 ], # [min, max], for rtx
"during_experiment": False,
"n-frames": 24,
"smooth": False }
_random_roughness: {
"enabled": True,
"intensity_interval": [ 0.1, 0.9 ]
}
env_prim_path: "/World/home"
robot_base_prim_path: "/my_robot_"
human_base_prim_path: "/my_human_"
max_human_anim_len: 500 # max human anim length to be considered in frames
min_human_anim_len: 50 # min human anim length to be loaded in frames
_recorder_settings: {
"rgb": { "enabled": True },
"depth": { "enabled": True, "colorize": False, "npy": True },
"depthLinear": { "enabled": True, "colorize": False, "npy": True },
"instance": { "enabled": True, "colorize": False, "npy": True, "mappings": True },
"semantic": { "enabled": True, "colorize": False, "npy": True, "mappings": True },
"bbox_2d_tight": { "enabled": True, "colorize": False, "npy": True },
"bbox_2d_loose": { "enabled": True, "colorize": False, "npy": True },
"normals": { "enabled": True, "colorize": False, "npy": True },
"motion-vector": { "enabled": False, "colorize": False, "npy": False },
"bbox_3d": { "enabled": True, "colorize": False, "npy": True },
"camera": { "enabled": True, "colorize": True, "npy": True },
"poses": { "enabled": True, "colorize": True, "npy": True },
}
google_obj_folder: "google_scanned_objects"
google_obj_shortlist: ""
shapenet_local_dir: "ShapeNet"
shapenet_username: ""
shapenet_password: ""
synsetId: "random"
modelId: "random"
is_iRotate: False
# the following cannot be both true at the same time
# if so, only the robot traj will be executed
# if both false we assume an external source is publishing something to your robot (in our case on /my_robot_0/joint_commands)
use_robot_traj: False # this is an absolute value. Note that the main root link and the actual position of the robot may differ based on the initial shift(which remains constant)
use_joint_traj: False # this is a relative value w.r.t. the starting location
robot_traj: # remember that movement will be linear and instantaneous. No acceleration or anything. This implies no odom, nor IMU data. If you want those, please add the same trajectory to a joint publisher.
- {pose: {x: 0, y: 0, z: 0, roll: 0, pitch: 0, yaw: 0}, time: 0}
- {pose: {x: -16.61, y: 4.4, z: 6.55, roll: 20, pitch: -15, yaw: 15}, time: 2}
- {pose: {x: -58.83, y: 11.00, z: -2.67, roll: 4, pitch: -27, yaw: 60}, time: 3}
- {pose: {x: 56.38, y: -55.85, z: 45.23, roll: -10, pitch: 40, yaw: 120}, time: 7}
- {pose: {x: -20.95, y: -37.64, z: -4.46, roll: 27, pitch: 15, yaw: 240}, time: 10}
init_loc: {
"use": False,
"x": 26.35,
"y": 241.43,
"z": 8.57,
"yaw": 271,
"roll": 0,
"pitch": 0
} | 4,164 | YAML | 45.797752 | 208 | 0.657541 |
eliabntt/GRADE-RR/simulator/configs/config_zebra_datagen.yaml | # GENERAL NOTE the paths need to be ABSOLUTE!
env_path: "/media/ebonetto/WindowsData/Zebra_envs/Usable"
use_stl: False # this disable stl loading thus placement AND robot heading
use_npy: False
base_env_path: "/media/ebonetto/WindowsData/ov/isaac_sim-2022.2.1/GRADE-RR/usds/env_base.usd"
zebra_anims_loc: "/media/ebonetto/WindowsData/Zebra_anims"
randomize_sky: True # only if allowed
robot_mesh_path: "/media/ebonetto/WindowsData/ov/isaac_sim-2021.2.1/GRADE-RR/meshes/drone.dae"
usd_robot_path: "/media/ebonetto/WindowsData/ov/isaac_sim-2022.2.1/GRADE-RR/usds/drone_2022.usd"
out_folder: "/media/ebonetto/WindowsData/zebra_out_close"
out_folder_npy: "/media/ebonetto/WindowsData/zebra_out_close"
fps: 30
num_robots: 3
experiment_length: 220
anim_exp_len: 200
physics_hz: 240 # NOTE THAT THIS IS THE RATE OF CLOCK AND IMU
render_hz: 240 # LEAVE IT EQUAL TO PHYSICS HZ
npy_sensor_size: [1920, 1080]
env_prim_path: "/World/home"
robot_base_prim_path: "/my_robot_"
_recorder_settings: {
"rgb": { "enabled": True },
"depth": { "enabled": False, "colorize": False, "npy": True },
"depthLinear": { "enabled": True, "colorize": False, "npy": True },
"instance": { "enabled": True, "colorize": False, "npy": True, "mappings": True },
"semantic": { "enabled": False, "colorize": False, "npy": True, "mappings": True },
"bbox_2d_tight": { "enabled": True, "colorize": False, "npy": True },
"bbox_2d_loose": { "enabled": True, "colorize": False, "npy": True },
"normals": { "enabled": False, "colorize": False, "npy": True },
"motion-vector": { "enabled": False, "colorize": True, "npy": True },
"bbox_3d": { "enabled": True, "colorize": False, "npy": True },
"camera": { "enabled": True, "colorize": True, "npy": True },
"poses": { "enabled": True, "colorize": True, "npy": True },
}
min_number_zebras: 2
max_number_zebras: 5
| 1,840 | YAML | 48.756755 | 96 | 0.681522 |
eliabntt/GRADE-RR/simulator/configs/config_irotate.yaml | # GENERAL NOTE the paths need to be ABSOLUTE!
env_path: "/media/ebonetto/WindowsData/Demo_GRADE"
use_stl: True # this disable stl loading thus placement AND robot heading
base_env_path: "/home/ebonetto/Desktop/empty.usd"
clean_base_env: False
only_placement: False
robot_mesh_path: "/home/ebonetto/.local/share/ov/pkg/isaac_sim-2021.2.1/main_repo/meshes/robotino.dae"
usd_robot_path: "/home/ebonetto/.local/share/ov/pkg/isaac_sim-2021.2.1/main_repo/usds/robotino.usd"
out_folder: "/media/ebonetto/WindowsData/exp_out2/"
out_folder_npy: "/media/ebonetto/WindowsData/exp_out2/"
fps: 30
num_robots: 1
experiment_length: 180 # camera frames length
autonomous: True # true -> FUEL, false -> random goals
physics_hz: 240 # NOTE THAT THIS IS THE RATE OF CLOCK AND IMU
render_hz: 240 # LEAVE IT EQUAL TO PHYSICS HZ
ratio_tf: 2 # physics_hz/ratio_tf = tf publish hz
ratio_odom: 4 # physics_hz/ratio_odom = odom publish hz
ratio_camera: 8 # physics_hz/ratio_cam = imgs publish hz
bootstrap_exploration: 1 # seconds to boostrap exploration (min(abs(this_value), 1/(physics_hz/ratio_camera))
robot_sensor_size: [ 640, 480 ]
npy_sensor_size: [ 1920, 1080]
_random_light: { "intensity": True,
"color": True,
"intensity_interval": [ 600, 4500 ], # [min, max], for rtx
"during_experiment": False,
"n-frames": 24,
"smooth": False }
_random_roughness: {
"enabled": True,
"intensity_interval": [ 0.1, 0.9 ]
}
env_prim_path: "/World/home"
robot_base_prim_path: "/my_robot_"
_recorder_settings: {
"rgb": { "enabled": True },
"depth": { "enabled": False, "colorize": False, "npy": True },
"depthLinear": { "enabled": True, "colorize": False, "npy": True },
"instance": { "enabled": True, "colorize": False, "npy": True, "mappings": True },
"semantic": { "enabled": False, "colorize": False, "npy": True, "mappings": True },
"bbox_2d_tight": { "enabled": True, "colorize": False, "npy": True },
"bbox_2d_loose": { "enabled": True, "colorize": False, "npy": True },
"normals": { "enabled": True, "colorize": False, "npy": True },
"motion-vector": { "enabled": False, "colorize": True, "npy": True },
"bbox_3d": { "enabled": True, "colorize": False, "npy": True },
"camera": { "enabled": True, "colorize": True, "npy": True },
"poses": { "enabled": True, "colorize": True, "npy": True },
}
is_iRotate: True | 2,394 | YAML | 47.87755 | 109 | 0.651211 |
eliabntt/GRADE-RR/simulator/configs/robot_with_ros.yaml | # GENERAL NOTE the paths need to be ABSOLUTE!
physics_hz: 240 # the size of a single physics step
render_hz: 240 # not influencing anything for now
base_env_path: "" # the base environment, e.g. GRADE-RR/usds/env_base.usd
env_path: "" # the parent folder that contains your environments, in subfolders
use_stl: True #
use_npy: True #
meters_per_unit: 0.01 # how many meters are in one single unit in the simulation. In this case x=1 will be 1 cm.
usd_robot_path: "" # the usd path of the robot, e.g. GRADE-RR/usds/drone_2022.usd
num_robots: 1 # how many robots we want to load
robot_sensor_size: [640,480]
ratio_joints: 2
ratio_tf: 2 # physics_hz/ratio_tf = tf publish hz
ratio_odom: 4 # physics_hz/ratio_odom = odom publish hz
ratio_camera: 8 # physics_hz/ratio_cam = imgs publish hz
# prefixes where to load the prims
env_prim_path: "/World/home"
robot_base_prim_path: "/my_robot_" # 0, 1, 2 ... num_robots
| 916 | YAML | 35.679999 | 112 | 0.716157 |
eliabntt/GRADE-RR/simulator/utils/robot_utils.py | import utils.misc_utils
from omni.isaac.core.utils.prims import set_targets
from scipy.spatial.transform import Rotation
from utils.misc_utils import *
from omni.isaac.core.utils.render_product import create_hydra_texture
def create_odom_message(_dc, robot_body_ptr, handle, meters_per_unit):
"""
Create odometry message for the robot_body_ptr.
Converts the readings from the IsaacSim unit to the mps when necessary
Gets the current rostime
header frame us "WORLD" and the child frame is from the "handle"
"""
lin_vel = _dc.get_rigid_body_local_linear_velocity(robot_body_ptr)
ang_vel = _dc.get_rigid_body_angular_velocity(robot_body_ptr)
pose = _dc.get_rigid_body_pose(robot_body_ptr)
odom_msg = Odometry()
odom_msg.header.frame_id = "world"
odom_msg.header.stamp = rospy.Time.now()
odom_msg.child_frame_id = handle[1:] if handle.startswith("/") else handle
odom_msg.pose.pose.position.x = pose.p.x * meters_per_unit
odom_msg.pose.pose.position.y = pose.p.y * meters_per_unit
odom_msg.pose.pose.position.z = pose.p.z * meters_per_unit
odom_msg.pose.pose.orientation.x = pose.r.x
odom_msg.pose.pose.orientation.y = pose.r.y
odom_msg.pose.pose.orientation.z = pose.r.z
odom_msg.pose.pose.orientation.w = pose.r.w
odom_msg.twist.twist.linear.x = lin_vel.x * meters_per_unit
odom_msg.twist.twist.linear.y = lin_vel.y * meters_per_unit
odom_msg.twist.twist.linear.z = lin_vel.z * meters_per_unit
odom_msg.twist.twist.angular.x = ang_vel.x
odom_msg.twist.twist.angular.y = ang_vel.y
odom_msg.twist.twist.angular.z = ang_vel.z
p_cov = np.array([0.0] * 36).reshape(6, 6)
p_cov[0:2, 0:2] = 0.00
p_cov[5, 5] = 0.00
odom_msg.pose.covariance = tuple(p_cov.ravel().tolist())
odom_msg.twist.covariance = tuple(p_cov.ravel().tolist())
return odom_msg
def create_diff_odom_message(_dc, robot_body_ptr, handle, meters_per_unit, base_body_ptr, base_handle):
"""
Create odometry message for the robot_body_ptr.
Converts the readings from the IsaacSim unit to the mps when necessary
Gets the current rostime
header frame us "WORLD" and the child frame is from the "handle"
"""
lin_vel = _dc.get_rigid_body_local_linear_velocity(robot_body_ptr)
ang_vel = _dc.get_rigid_body_angular_velocity(robot_body_ptr)
pose = _dc.get_rigid_body_pose(robot_body_ptr)
base_lin_vel = _dc.get_rigid_body_local_linear_velocity(base_body_ptr)
base_ang_vel = _dc.get_rigid_body_angular_velocity(base_body_ptr)
base_pose = _dc.get_rigid_body_pose(base_body_ptr)
odom_msg = Odometry()
odom_msg.header.frame_id = base_handle
odom_msg.header.stamp = rospy.Time.now()
odom_msg.child_frame_id = handle[1:] if handle.startswith("/") else handle
odom_msg.pose.pose.position.x = (pose.p.x - base_pose.p.x) * meters_per_unit
odom_msg.pose.pose.position.y = (pose.p.y - base_pose.p.y) * meters_per_unit
odom_msg.pose.pose.position.z = (pose.p.z - base_pose.p.z) * meters_per_unit
q1 = Quaternion(base_pose.r.w, base_pose.r.x, base_pose.r.y, base_pose.r.z)
q2 = Quaternion(pose.r.w, pose.r.x, pose.r.y, pose.r.z)
q = q1.conjugate * q2
odom_msg.pose.pose.orientation.x = q.x
odom_msg.pose.pose.orientation.y = q.y
odom_msg.pose.pose.orientation.z = q.z
odom_msg.pose.pose.orientation.w = q.w
odom_msg.twist.twist.linear.x = (lin_vel.x - base_lin_vel.x) * meters_per_unit
odom_msg.twist.twist.linear.y = (lin_vel.y - base_lin_vel.y) * meters_per_unit
odom_msg.twist.twist.linear.z = (lin_vel.z - base_lin_vel.z) * meters_per_unit
odom_msg.twist.twist.angular.x = (ang_vel.x - base_ang_vel.x)
odom_msg.twist.twist.angular.y = (ang_vel.y - base_ang_vel.y)
odom_msg.twist.twist.angular.z = (ang_vel.z - base_ang_vel.z)
p_cov = np.array([0.0] * 36).reshape(6, 6)
p_cov[0:2, 0:2] = 0.00
p_cov[5, 5] = 0.00
odom_msg.pose.covariance = tuple(p_cov.ravel().tolist())
odom_msg.twist.covariance = tuple(p_cov.ravel().tolist())
return odom_msg
def create_camera_pose_message(_dc, camera_body_ptr, handle, meters_per_unit):
"""
Similar to the odom, but it's just for a pose message, in this case for the camera
"""
pose = _dc.get_rigid_body_pose(camera_body_ptr)
camera_pose = PoseStamped()
camera_pose.header.frame_id = "world"
camera_pose.header.stamp = rospy.Time.now()
camera_pose.pose.position.x = pose.p.x * meters_per_unit
camera_pose.pose.position.y = pose.p.y * meters_per_unit
camera_pose.pose.position.z = pose.p.z * meters_per_unit
camera_pose.pose.orientation.x = pose.r.x
camera_pose.pose.orientation.y = pose.r.y
camera_pose.pose.orientation.z = pose.r.z
camera_pose.pose.orientation.w = pose.r.w
return camera_pose
def add_pose_tree(path: str, irotate: bool=False):
"""
Add the tf publisher to the desired path.
This path should be the robot itself.
Each robot has a pose tree.
"""
if path.startswith("/"):
path = path[1:]
og.Controller.edit(
{"graph_path": f"/{path}/TFActionGraph", "evaluator_name": "execution"},
{
og.Controller.Keys.CREATE_NODES: [
("ReadSimTime", "omni.isaac.core_nodes.IsaacReadSimulationTime"),
("OnImpulseEvent", "omni.graph.action.OnImpulseEvent"),
("PublishTF", "omni.isaac.ros_bridge.ROS1PublishTransformTree"),
],
og.Controller.Keys.CONNECT: [
("OnImpulseEvent.outputs:execOut", "PublishTF.inputs:execIn"),
("ReadSimTime.outputs:simulationTime", "PublishTF.inputs:timeStamp"),
],
og.Controller.Keys.SET_VALUES: [
("PublishTF.inputs:nodeNamespace", f"/{path}"),
]
},
)
# fixme
if irotate:
omni.kit.commands.execute('ChangeProperty',
prop_path=Sdf.Path('/my_robot_0/ROS_PoseTree.poseTreePubTopic'),
value='/tf2',
prev='/tf')
set_target_prims(primPath=f"/{path}/TFActionGraph/PublishTF", inputName="inputs:targetPrims",
targetPrimPaths=[f"/{path}"])
return f"/{path}/TFActionGraph"
def add_camera_and_viewport(path: str, resolution: list, old_h_ape, old_v_ape, sc, index=0,
robot_index=0, cam_per_robot=1, camera_path="Camera"):
"""
The function create first the ROSBridge Camera and then the corresponding viewport.
index is the number of the camera for the given robot.
headless is a boolean that indicates if the simulation is headless or not (i.e. create a visual viewport or not).
robot_index correspond to the n-th robot in the scene.
"""
resolution = tuple(resolution)
camera_path = path + f"/{camera_path}"
index = robot_index * cam_per_robot + index
stage = omni.usd.get_context().get_stage()
camera = stage.GetPrimAtPath(camera_path)
old_h_ape.append(camera.GetAttribute("horizontalAperture").Get())
old_v_ape.append(camera.GetAttribute("verticalAperture").Get())
viewport_name = "Viewport" + (f" {index + 1}" if str(index + 1) != "0" and str(index + 1) != "1" else "")
sc.step()
keys = og.Controller.Keys
(camera_graph, _, _, _) = og.Controller.edit(
{
"graph_path": f"{path}/ROSCamera_{index}_Graph",
"evaluator_name": "push",
"pipeline_stage": og.GraphPipelineStage.GRAPH_PIPELINE_STAGE_ONDEMAND,
},
{
keys.CREATE_NODES: [
("OnTick", "omni.graph.action.OnTick"),
("createViewport", "omni.isaac.core_nodes.IsaacCreateViewport"),
("setViewportResolution", "omni.isaac.core_nodes.IsaacSetViewportResolution"),
("getRenderProduct", "omni.isaac.core_nodes.IsaacGetViewportRenderProduct"),
("setCamera", "omni.isaac.core_nodes.IsaacSetCameraOnRenderProduct"),
("cameraHelperRgb", "omni.isaac.ros_bridge.ROS1CameraHelper"),
("cameraHelperInfo", "omni.isaac.ros_bridge.ROS1CameraHelper"),
("cameraHelperDepth", "omni.isaac.ros_bridge.ROS1CameraHelper"),
],
keys.CONNECT: [
("OnTick.outputs:tick", "createViewport.inputs:execIn"),
("createViewport.outputs:execOut", "getRenderProduct.inputs:execIn"),
("createViewport.outputs:viewport", "getRenderProduct.inputs:viewport"),
("createViewport.outputs:execOut", "setViewportResolution.inputs:execIn"),
("createViewport.outputs:viewport", "setViewportResolution.inputs:viewport"),
("getRenderProduct.outputs:execOut", "setCamera.inputs:execIn"),
("getRenderProduct.outputs:renderProductPath", "setCamera.inputs:renderProductPath"),
("setCamera.outputs:execOut", "cameraHelperRgb.inputs:execIn"),
("setCamera.outputs:execOut", "cameraHelperInfo.inputs:execIn"),
("setCamera.outputs:execOut", "cameraHelperDepth.inputs:execIn"),
("getRenderProduct.outputs:renderProductPath", "cameraHelperRgb.inputs:renderProductPath"),
("getRenderProduct.outputs:renderProductPath", "cameraHelperInfo.inputs:renderProductPath"),
("getRenderProduct.outputs:renderProductPath", "cameraHelperDepth.inputs:renderProductPath"),
],
og.Controller.Keys.SET_VALUES: [
("createViewport.inputs:viewportId", index),
("setViewportResolution.inputs:height", int(resolution[1])),
("setViewportResolution.inputs:width", int(resolution[0])),
("cameraHelperRgb.inputs:frameId", path[1:]),
("cameraHelperRgb.inputs:topicName", path + f"/{index}/rgb/image_raw"),
("cameraHelperRgb.inputs:type", "rgb"),
("cameraHelperDepth.inputs:frameId", path[1:]),
("cameraHelperDepth.inputs:topicName", path + f"/{index}/depth/image_raw"),
("cameraHelperDepth.inputs:type", "depth"),
("cameraHelperInfo.inputs:frameId", path[1:]),
("cameraHelperInfo.inputs:topicName", path + f"/{index}/camera_info"),
("cameraHelperInfo.inputs:type", "camera_info"),
],
},
)
set_targets(
prim=omni.usd.get_context().get_stage().GetPrimAtPath(f"{path}/ROSCamera_{index}_Graph/setCamera"),
attribute="inputs:cameraPrim",
target_prim_paths=[camera_path],
)
og.Controller.evaluate_sync(camera_graph)
for _ in range(5):
sc.step()
omni.kit.app.get_app().update()
viewport_handle = [x for x in omni.kit.viewport.window.get_viewport_window_instances()][-1].viewport_api
viewport_handle.set_texture_resolution((resolution[0], resolution[1]))
for _ in range(5):
sc.step()
omni.kit.app.get_app().update()
return camera_graph.get_path_to_graph(), viewport_handle
def add_joint_state(path: str):
if path.startswith("/"):
path = path[1:]
og.Controller.edit(
{"graph_path": f"/{path}/JointActionGraph", "evaluator_name": "execution"},
{
og.Controller.Keys.CREATE_NODES: [
("ReadSimTime", "omni.isaac.core_nodes.IsaacReadSimulationTime"),
("OnImpulseEvent", "omni.graph.action.OnImpulseEvent"),
("PublishJointState", "omni.isaac.ros_bridge.ROS1PublishJointState"),
("SubscribeJointState", "omni.isaac.ros_bridge.ROS1SubscribeJointState"),
("ArticulationController", "omni.isaac.core_nodes.IsaacArticulationController"),
],
og.Controller.Keys.CONNECT: [
("OnImpulseEvent.outputs:execOut", "PublishJointState.inputs:execIn"),
("OnImpulseEvent.outputs:execOut", "SubscribeJointState.inputs:execIn"),
("OnImpulseEvent.outputs:execOut", "ArticulationController.inputs:execIn"),
("ReadSimTime.outputs:simulationTime", "PublishJointState.inputs:timeStamp"),
("SubscribeJointState.outputs:jointNames", "ArticulationController.inputs:jointNames"),
("SubscribeJointState.outputs:positionCommand", "ArticulationController.inputs:positionCommand"),
("SubscribeJointState.outputs:velocityCommand", "ArticulationController.inputs:velocityCommand"),
("SubscribeJointState.outputs:effortCommand", "ArticulationController.inputs:effortCommand"),
],
og.Controller.Keys.SET_VALUES: [
# Providing path to Articulation Controller node
# Providing the robot path is equivalent to setting the targetPrim in Articulation Controller node
("ArticulationController.inputs:usePath", True),
("ArticulationController.inputs:robotPath", "/" + path),
# Assigning topic names to clock publishers
("PublishJointState.inputs:topicName", "/" + path + "/joint_states"),
("SubscribeJointState.inputs:topicName", "/" + path + "/joint_commands"),
],
},
)
# set_target_prims(primPath=f"/{path}/JointActionGraph/SubscribeJointState", targetPrimPaths=[f"/{path}"])
set_target_prims(primPath=f"/{path}/JointActionGraph/PublishJointState", targetPrimPaths=[f"/{path}"])
return f"/{path}/JointActionGraph"
def add_clock():
(_clock_graph, _, _, _) = og.Controller.edit(
{"graph_path": "/ClockActionGraph", "evaluator_name": "push",
"pipeline_stage": og.GraphPipelineStage.GRAPH_PIPELINE_STAGE_ONDEMAND, },
{
og.Controller.Keys.CREATE_NODES: [
("ReadSimTime", "omni.isaac.core_nodes.IsaacReadSimulationTime"),
("OnTick", "omni.graph.action.OnTick"),
("PublishManualClock", "omni.isaac.ros_bridge.ROS1PublishClock"),
],
og.Controller.Keys.CONNECT: [
# Connecting execution of OnImpulseEvent node to PublishManualClock so it will only publish when an impulse event is triggered
("OnTick.outputs:tick", "PublishManualClock.inputs:execIn"),
# Connecting simulationTime data of ReadSimTime to the clock publisher nodes
("ReadSimTime.outputs:simulationTime", "PublishManualClock.inputs:timeStamp"),
],
og.Controller.Keys.SET_VALUES: [
# Assigning topic names to clock publishers
("PublishManualClock.inputs:topicName", "/clock"),
],
},
)
return _clock_graph
def get_robot_yaw(x, y, z, env_mesh, shifts):
"""
Checks the best robot yaw angle for the given position.
Cast rays from the robot position to the environment mesh and returns the angle
It considers 36 rays.
For each ray we compute the distance to the nearest point on the mesh.
If the distance is infinite, it gets interpolated.
We compute a rolling window sum (with a window size of 4 rays) of the distances.
Return the best yaw angle in RADIANS.
"""
checking_steps = 36
angles = [[np.cos(np.pi * 2.0 / checking_steps * c_step), np.sin(np.pi * 2.0 / checking_steps * c_step), 0] for
c_step in range(checking_steps)]
positions = [[x + shifts[0], y + shifts[1], z + shifts[2]] for _ in range(checking_steps)]
checking_rays = trimesh.proximity.longest_ray(env_mesh, positions, angles)
checking_rays[checking_rays < 0] = 0
nans, x = inf_helper(checking_rays)
checking_rays[nans] = np.interp(x(nans), x(~nans), checking_rays[~nans])
checking_rays[checking_rays > 8] = 8
rolling_rays = int(40 / (360 / checking_steps))
checking_rays = np.append(checking_rays, checking_rays[:rolling_rays - 1])
checking_rays = np.convolve(checking_rays, np.ones(rolling_rays, dtype=int), 'valid') / rolling_rays
return (np.argmax(checking_rays) + rolling_rays / 2) * 2 * np.pi / checking_steps
def get_vp_list():
from omni.kit.viewport.window import get_viewport_window_instances
return [x for x in get_viewport_window_instances()]
def create_viewport(camera_path, is_headless, index, resolution, old_h_ape, old_v_ape, sc):
"""
The function create the viewport for the given camera.
Creates an handle, a viewport and the window position/size if the system is not headless.
"""
stage = omni.usd.get_context().get_stage()
camera = stage.GetPrimAtPath(camera_path)
old_h_ape.append(camera.GetAttribute("horizontalAperture").Get())
old_v_ape.append(camera.GetAttribute("verticalAperture").Get())
index += 1 # omniverse starts from 1
viewport_name = "Viewport" + (f" {index}" if str(index) != "0" and str(index) != "1" else "")
viewport = omni.kit.viewport.utility.get_active_viewport_window(window_name=viewport_name)
viewport_handle = omni.kit.viewport.utility.get_viewport_from_window_name(viewport_name)
if not viewport_handle:
viewport = omni.kit.viewport.utility.create_viewport_window(name=viewport_name)
viewport_handle = omni.kit.viewport.utility.get_viewport_from_window_name(viewport.name)
if not is_headless:
viewport.setPosition(1000, 400)
viewport.height, viewport.width = 300, 300
viewport_handle.set_active_camera(camera_path)
for _ in range(10):
sc.step()
viewport_handle.set_texture_resolution((resolution[0], resolution[1]))
sc.step()
return viewport_handle, viewport.name
def ros_launchers_setup(roslaunch, env_limits_shifted, config):
"""
Setup the ros launchers for the simulation.
We need an exploration manager for every robot, and a collision checking service to place the objects.
"""
roslaunch_files = []
roslaunch_args = []
launch_files = []
print("launching ros nodes...")
if not config["only_placement"].get():
for i in range(config["num_robots"].get()):
# TODO hack to be compatible with the old version
if type(config["is_iRotate"].get()) == list:
is_irotate = config["is_iRotate"].get()[i]
else:
is_irotate = config["is_iRotate"].get()
if not is_irotate:
cli_args1 = ["exploration_manager", "my_exploration.launch",
# cli_args1 = ["/home/ebonetto/catkin_ws/src/FUEL/fuel_planner/exploration_manager/launch/my_exploration.launch",
"box_min_x:={:.4f}".format(env_limits_shifted[0] - 0.2),
"box_min_y:={:.4f}".format(env_limits_shifted[1] - 0.2),
"box_min_z:={:.4f}".format(env_limits_shifted[2]),
"box_max_x:={:.4f}".format(env_limits_shifted[3] + 0.2),
"box_max_y:={:.4f}".format(env_limits_shifted[4] + 0.2),
"box_max_z:={:.4f}".format(min(3, env_limits_shifted[5] - 0.1)),
f"mav_name:={config['robot_base_prim_path'].get()}{i}"]
roslaunch_files.append(roslaunch.rlutil.resolve_launch_arguments(cli_args1)[0])
roslaunch_args.append(cli_args1[2:])
launch_files.append((roslaunch_files[-1], roslaunch_args[-1]))
else:
cli_args1 = ["custom_joint_controller_ros_irotate", "publish_joint_commands_node.launch",
"position_limit_x:={:.4f}".format(env_limits_shifted[3] + 0.2),
"position_limit_y:={:.4f}".format(env_limits_shifted[4] + 0.2),
"position_limit_z:={:.4f}".format(3),
"robot_id:=1", "frame_id:='base'"]
roslaunch_files.append(roslaunch.rlutil.resolve_launch_arguments(cli_args1)[0])
roslaunch_args.append(cli_args1[2:])
launch_files.append((roslaunch_files[-1], roslaunch_args[-1]))
# TODO hack because we pre-cache the robot mesh
if type(config["robot_mesh_path"].get()) == list:
mesh_path = config["robot_mesh_path"].get()[0]
else:
mesh_path = config["robot_mesh_path"].get()
cli_args2 = ["collision_check", "collision_check.launch",
"robot_mesh_path:={}".format(mesh_path)]
roslaunch_file2 = roslaunch.rlutil.resolve_launch_arguments(cli_args2)[0]
roslaunch_args2 = cli_args2[2:]
launch_files.append((roslaunch_file2, roslaunch_args2))
return launch_files
def create_imu_message(frame, last_reading, meters_per_unit):
"""
Create the IMU message from the last reading.
"""
imu_msg = Imu()
imu_msg.header.frame_id = frame[1:] if frame.startswith("/") else frame
imu_msg.header.stamp = rospy.Time.now()
imu_msg.angular_velocity.x = last_reading.ang_vel_x
imu_msg.angular_velocity.y = last_reading.ang_vel_y
imu_msg.angular_velocity.z = last_reading.ang_vel_z
imu_msg.linear_acceleration.x = last_reading.lin_acc_x * meters_per_unit * meters_per_unit
imu_msg.linear_acceleration.y = last_reading.lin_acc_y * meters_per_unit * meters_per_unit
imu_msg.linear_acceleration.z = last_reading.lin_acc_z * meters_per_unit * meters_per_unit
imu_msg.angular_velocity_covariance = [0, 0, 0, 0, 0, 0, 0, 0, 0]
imu_msg.linear_acceleration_covariance = [0, 0, 0, 0, 0, 0, 0, 0, 0]
return imu_msg
def setup_imu_sensor(_is, config, imu_sensor_path):
"""
Setup the IMU sensor config.
Keep in mind that this is relative to the parent body, so any transform the parent has is already reflected.
"""
add_imu_sensor, sensor = omni.kit.commands.execute(
"IsaacSensorCreateImuSensor",
path="/imu_sensor",
parent=imu_sensor_path,
sensor_period=1 / config["physics_hz"].get(),
orientation=Gf.Quatd(1, 0, 0, 0),
visualize=False,
)
if not add_imu_sensor:
raise Exception("Failed to add IMU sensor")
return sensor
def pub_imu(_is, imu_pubs, robot_imu_frames, meters_per_unit):
"""
Simple message publisher
"""
for index, handle in enumerate(robot_imu_frames):
last_reading = _is.get_sensor_sim_reading(handle + "/imu_sensor")
imu_pubs[index].publish(create_imu_message(handle, last_reading, meters_per_unit))
def pub_cam_pose(camera_pose_frames, cam_pose_pubs, _dc, meters_per_unit):
"""
Simple message publisher
"""
for index, handle in enumerate(camera_pose_frames):
camera_body_ptr = _dc.get_rigid_body(handle)
cam_pose_pubs[index].publish(create_camera_pose_message(_dc, camera_body_ptr, handle, meters_per_unit))
def pub_odom(robot_odom_frames, odom_pubs, _dc, meters_per_unit, diff_odom_frames=[]):
"""
Simple message publisher
"""
odoms = []
angles = []
if len(diff_odom_frames) == 0:
for index, handle in enumerate(robot_odom_frames):
robot_body_ptr = _dc.get_rigid_body(handle)
odom = create_odom_message(_dc, robot_body_ptr, handle, meters_per_unit)
odoms.append([odom.pose.pose.position.x, odom.pose.pose.position.y, odom.pose.pose.position.z])
angles.append(Rotation.from_quat(
[odom.pose.pose.orientation.x, odom.pose.pose.orientation.y, odom.pose.pose.orientation.z,
odom.pose.pose.orientation.w]).as_euler("XYZ"))
odom_pubs[index].publish(odom)
else:
for index, handle in enumerate(robot_odom_frames):
robot_body_ptr = _dc.get_rigid_body(handle)
diff_body_ptr = _dc.get_rigid_body(diff_odom_frames[index])
diff_handle = diff_odom_frames[index][1:] if diff_odom_frames[index].startswith("/") else diff_odom_frames[
index]
odom = create_diff_odom_message(_dc, robot_body_ptr, handle, meters_per_unit, diff_body_ptr, diff_handle)
odoms.append([odom.pose.pose.position.x, odom.pose.pose.position.y, odom.pose.pose.position.z])
angles.append(Rotation.from_quat(
[odom.pose.pose.orientation.x, odom.pose.pose.orientation.y, odom.pose.pose.orientation.z,
odom.pose.pose.orientation.w]).as_euler("XYZ"))
odom_pubs[index].publish(odom)
return odoms, angles
def import_robot(robot_base_prim_path, n, usd_robot_path, local_file_prefix=''):
"""
Add the robot to the stage.
Add semantics.
"""
stage = omni.usd.get_context().get_stage()
res, _ = omni.kit.commands.execute("CreateReferenceCommand",
usd_context=omni.usd.get_context(),
path_to=f"{robot_base_prim_path}{n}",
asset_path=local_file_prefix + usd_robot_path,
instanceable=False)
if res:
clear_properties(f"{robot_base_prim_path}{n}")
add_semantics(stage.GetPrimAtPath(f"{robot_base_prim_path}{n}"), "robot")
else:
raise Exception("Failed to import robot")
def get_valid_robot_location(environment, first):
"""
Query the service to place the robot in a free space AND compute an initial good yaw.
"""
x, y, z, _ = position_object(environment, type=0, reset=first)
# robot is nearly circular so I do not have to worry about collisionsif environment.env_mesh != None:
if environment.env_mesh != None:
yaw = get_robot_yaw(x[0], y[0], z[0], environment.env_mesh, environment.shifts)
print(f"Initial yaw: {yaw}")
return x[0], y[0], z[0], yaw
def control_camera(viewport, sc):
sc.step()
if viewport is not None:
import omni.syntheticdata._syntheticdata as sd
stage = omni.usd.get_context().get_stage()
# Required for editing the SDGPipeline graph which exists in the Session Layer
with Usd.EditContext(stage, stage.GetSessionLayer()):
# Get name of rendervar for RGB sensor type
rv_rgb = omni.syntheticdata.SyntheticData.convert_sensor_type_to_rendervar(sd.SensorType.Rgb.name)
# Get path to IsaacSimulationGate node in RGB pipeline
rgb_camera_gate_path = omni.syntheticdata.SyntheticData._get_node_path(
rv_rgb + "IsaacSimulationGate", viewport.get_render_product_path()
)
# Get name of rendervar for DistanceToImagePlane sensor type
rv_depth = omni.syntheticdata.SyntheticData.convert_sensor_type_to_rendervar(
sd.SensorType.DistanceToImagePlane.name)
# Get path to IsaacSimulationGate node in Depth pipeline
depth_camera_gate_path = omni.syntheticdata.SyntheticData._get_node_path(
rv_depth + "IsaacSimulationGate", viewport.get_render_product_path()
)
# Get path to IsaacSimulationGate node in CameraInfo pipeline
camera_info_gate_path = omni.syntheticdata.SyntheticData._get_node_path(
"PostProcessDispatch" + "IsaacSimulationGate", viewport.get_render_product_path()
)
return rgb_camera_gate_path, depth_camera_gate_path, camera_info_gate_path
def add_ros_components(robot_base_prim_path, n, ros_transform_components, ros_camera_list, viewport_window_list,
camera_pose_frames, cam_pose_pubs, imu_pubs, robot_imu_frames,
robot_odom_frames, odom_pubs, lidars,
dynamic_prims, config, old_h_ape, old_v_ape, _is, simulation_context, _clock, irotate=False):
"""
Add the ROS components to the robot.
This is done because we need different topics for each robot.
Components added:
- joint_states (publisher and subscriber)
- tf broadcaster
- camera
- camera pose
- imu
- odom
When necessary we create also the corresponding publisher (whenever the RosBridge component is not available).
Publishers created:
- imu
- odom
- camera pose
"""
ros_transform_components.append(add_joint_state(f"{robot_base_prim_path}{n}"))
ros_transform_components.append(add_pose_tree(f"{robot_base_prim_path}{n}", irotate))
# create camera
component, viewport = add_camera_and_viewport(f"{robot_base_prim_path}{n}/camera_link",
config["robot_sensor_size"].get(),
old_h_ape, old_v_ape, simulation_context,
0, n, cam_per_robot=1) # cam index is useful if you want multiple cameras
cam_outputs = control_camera(viewport, simulation_context)
ros_camera_list.append([n + 0, component, cam_outputs])
viewport_window_list.append(viewport)
# component, viewport = add_camera_and_viewport(f"{robot_base_prim_path}{n}/camera_link",
# config["robot_sensor_size"].get(),
# old_h_ape, old_v_ape, simulation_context,
# 1, n, cam_per_robot=2) # cam index is useful if you want multiple cameras
# cam_outputs = control_camera(viewport, simulation_context)
# ros_camera_list.append([n + 1, component, cam_outputs])
# viewport_window_list.append(viewport)
omni.kit.app.get_app().update()
# append camera pose frame (we need only one) and pubs
camera_pose_frames.append(f"{robot_base_prim_path}{n}/camera_link")
cam_pose_pubs.append(rospy.Publisher(f"{robot_base_prim_path}{n}/camera/pose", PoseStamped, queue_size=10))
for _ in range(10):
og.Controller.set(og.Controller.attribute(f"{ros_transform_components[-1]}/OnImpulseEvent.state:enableImpulse"),
True)
og.Controller.set(og.Controller.attribute(f"{ros_transform_components[-2]}/OnImpulseEvent.state:enableImpulse"),
True)
og.Controller.evaluate_sync(_clock)
simulation_context.step()
# attach IMU sensor to the robot
if irotate:
setup_imu_sensor(_is, config, f"{robot_base_prim_path}{n}/imu_link")
imu_pubs.append(rospy.Publisher(f"{robot_base_prim_path}{n}/imu_cam", Imu, queue_size=10))
robot_imu_frames.append(f"{robot_base_prim_path}{n}/imu_link")
setup_imu_sensor(_is, config, f"{robot_base_prim_path}{n}/base_link")
imu_pubs.append(rospy.Publisher(f"{robot_base_prim_path}{n}/imu_body", Imu, queue_size=10))
robot_imu_frames.append(f"{robot_base_prim_path}{n}/base_link")
robot_odom_frames.append(f"{robot_base_prim_path}{n}/base_link")
else:
setup_imu_sensor(_is, config, f"{robot_base_prim_path}{n}/imu_link")
imu_pubs.append(rospy.Publisher(f"{robot_base_prim_path}{n}/imu_body", Imu, queue_size=10))
robot_imu_frames.append(f"{robot_base_prim_path}{n}/imu_link")
setup_imu_sensor(_is, config, f"{robot_base_prim_path}{n}/camera_link")
imu_pubs.append(rospy.Publisher(f"{robot_base_prim_path}{n}/imu_camera", Imu, queue_size=10))
robot_imu_frames.append(f"{robot_base_prim_path}{n}/camera_link")
robot_odom_frames.append(f"{robot_base_prim_path}{n}/yaw_link")
odom_pubs.append(rospy.Publisher(f"{robot_base_prim_path}{n}/odom", Odometry, queue_size=10))
stage = omni.usd.get_context().get_stage()
dynamic_prims.append(stage.GetPrimAtPath(f"{robot_base_prim_path}{n}"))
if lidars:
stage = omni.usd.get_context().get_stage()
dynamic_prims.append(stage.GetPrimAtPath(f"{robot_base_prim_path}{n}"))
sensor = add_lidar(f"{robot_base_prim_path}{n}/yaw_link", [0, 0, -.1], [0, 0, 0], is_3d=True, is_2d=True)
lidars.append(sensor)
def get_robot_joint_init_loc(name):
"""
It gets the initial location of the robot's joints
:param name: The name of the robot
:return: The initial location of the robot's joints.
"""
stage = omni.usd.get_context().get_stage()
x = UsdPhysics.Joint.Get(stage, name + '/base_link/x_joint').GetLocalPos0Attr().Get()[0]
y = UsdPhysics.Joint.Get(stage, name + '/x_link/y_joint').GetLocalPos0Attr().Get()[1]
z = UsdPhysics.Joint.Get(stage, name + '/y_link/z_joint').GetLocalPos0Attr().Get()[2]
roll = UsdPhysics.RevoluteJoint.Get(stage, name + '/z_link/roll_joint').GetLocalRot0Attr().Get()
roll = Rotation.from_quat([roll.imaginary[0], roll.imaginary[1], roll.imaginary[2], roll.real]).as_euler('XYZ')[0]
pitch = UsdPhysics.RevoluteJoint.Get(stage, name + '/roll_link/pitch_joint').GetLocalRot0Attr().Get()
pitch = Rotation.from_quat([pitch.imaginary[0], pitch.imaginary[1], pitch.imaginary[2], pitch.real]).as_euler('XYZ')[
1]
yaw = UsdPhysics.RevoluteJoint.Get(stage, name + '/pitch_link/yaw_joint').GetLocalRot0Attr().Get()
yaw = Rotation.from_quat([yaw.imaginary[0], yaw.imaginary[1], yaw.imaginary[2], yaw.real]).as_euler('XYZ')[2]
return x, y, z, roll, pitch, yaw
def set_drone_joints_init_loc(name: str, pos: [], orientation: [], upper_zlim: float=100, lower_zlim: float=0, irotate=False):
"""
Move the drone to the specified location by acting on the JOINTS.
PLEASE NOTE: the intial joint position published by joint_states will be 0,0,0 strangely. #IsaacBug
The joints should be named as follows:
- base_link/x_joint
- x_link/y_joint
- y_link/z_joint
- z_link/roll_joint
- roll_link/pitch_joint
- pitch_link/yaw_joint
name: the name of the robot (e.g. "my_robot_0", the prim path)
pos: the position of the robot (x,y,z)
orientation: the orientation of the robot (roll,pitch,yaw), in rad
upper_zlim: the z limit of the robot (z)
irotate: if True, the joints considered are the iRotate ones
"""
x, y, z = pos
upper_zlim = max(upper_zlim, z)
roll, pitch, yaw = orientation
stage = omni.usd.get_context().get_stage()
if irotate:
UsdPhysics.Joint.Get(stage, name + '/x_link/x_joint').GetLocalPos0Attr().Set(Gf.Vec3f(x, 0, 0))
UsdPhysics.Joint.Get(stage, name + '/y_link/y_joint').GetLocalPos0Attr().Set(Gf.Vec3f(0, y, 0))
yaw = np.rad2deg(yaw)
quat = (
Gf.Rotation(Gf.Vec3d.XAxis(), 0)
* Gf.Rotation(Gf.Vec3d.YAxis(), 0)
* Gf.Rotation(Gf.Vec3d.ZAxis(), yaw)
)
UsdPhysics.RevoluteJoint.Get(stage, name + '/yaw_link/yaw_joint').GetLocalRot1Attr().Set(Gf.Quatf(quat.GetQuat()))
else:
UsdPhysics.Joint.Get(stage, name + '/base_link/x_joint').GetLocalPos0Attr().Set(Gf.Vec3f(x, 0, 0))
UsdPhysics.Joint.Get(stage, name + '/x_link/y_joint').GetLocalPos0Attr().Set(Gf.Vec3f(0, y, 0))
UsdPhysics.Joint.Get(stage, name + '/y_link/z_joint').GetLocalPos0Attr().Set(Gf.Vec3f(0, 0, z))
stage.GetPrimAtPath(name + '/y_link/z_joint').GetAttribute('physics:lowerLimit').Set(-z + lower_zlim)
stage.GetPrimAtPath(name + '/y_link/z_joint').GetAttribute('physics:upperLimit').Set(upper_zlim - z)
roll = np.rad2deg(roll)
quat = (
Gf.Rotation(Gf.Vec3d.XAxis(), roll)
* Gf.Rotation(Gf.Vec3d.YAxis(), 0)
* Gf.Rotation(Gf.Vec3d.ZAxis(), 0)
)
UsdPhysics.RevoluteJoint.Get(stage, name + '/z_link/roll_joint').GetLocalRot0Attr().Set(Gf.Quatf(quat.GetQuat()))
pitch = np.rad2deg(pitch)
quat = (
Gf.Rotation(Gf.Vec3d.XAxis(), pitch)
* Gf.Rotation(Gf.Vec3d.YAxis(), 0)
* Gf.Rotation(Gf.Vec3d.ZAxis(), 90)
)
UsdPhysics.RevoluteJoint.Get(stage, name + '/roll_link/pitch_joint').GetLocalRot0Attr().Set(
Gf.Quatf(quat.GetQuat()))
yaw = np.rad2deg(yaw)
quat = (
Gf.Rotation(Gf.Vec3d.XAxis(), 0)
* Gf.Rotation(Gf.Vec3d.YAxis(), 0)
* Gf.Rotation(Gf.Vec3d.ZAxis(), yaw)
)
UsdPhysics.RevoluteJoint.Get(stage, name + '/pitch_link/yaw_joint').GetLocalRot0Attr().Set(Gf.Quatf(quat.GetQuat()))
def add_robot_traj(path: str, config, meters_per_unit, time_codes_per_second):
"""
It adds a translation and rotation animation to the given path,
using the given configuration, meters per unit, and time codes per second
:param path: The path to the USD stage
:type path: str
:param config: The configuration file that contains the robot trajectory
:param meters_per_unit: The scale of the scene
:param time_codes_per_second: This is the number of time codes per second. This is the same as the frame rate of the
animation
"""
clear_properties(path)
for entry in config["robot_traj"].get():
add_translate_anim(path, Gf.Vec3d(entry["pose"]["x"] / meters_per_unit, entry["pose"]["y"] / meters_per_unit,
entry["pose"]["z"] / meters_per_unit),
entry["time"] * time_codes_per_second)
add_rotation_anim(path, Gf.Vec3d(entry["pose"]["roll"], entry["pose"]["pitch"], entry["pose"]["yaw"]),
entry["time"] * time_codes_per_second, use_double=True)
def diff_angle(alpha, beta):
dist = (alpha - beta + np.pi + 2 * np.pi) % (2 * np.pi) - np.pi
return dist
# assume position control
def check_pose_and_goals(init_loc, init_angle, c_pose, c_angle, path, goal_list, meters_per_unit, first):
"""
It sets the target position of the joints to the next goal in the list
:param init_loc: the initial location of the robot
:param init_angle: the initial orientation of the robot
:param c_pose: current pose of the robot
:param c_angle: current angle of the robot
:param path: the path to the robot in the simulation
:param goal_list: a list of goals, each goal is a list of 6 elements: x, y, z, roll, pitch, yaw
:param meters_per_unit: This is the scale of the robot
:param first: whether this is the first time the function is called
:return: The goal list is being returned.
"""
dist_roll = abs(diff_angle(np.deg2rad(goal_list[0][3]), diff_angle(c_angle[0], init_angle[0])))
dist_pitch = abs(diff_angle(np.deg2rad(goal_list[0][4]), diff_angle(c_angle[1], init_angle[1])))
dist_yaw = abs(diff_angle(np.deg2rad(goal_list[0][5]), diff_angle(c_angle[2], init_angle[2])))
sum_dist = dist_roll + dist_pitch + dist_yaw
if not first and \
(np.linalg.norm(np.array([goal_list[0][0], goal_list[0][1], goal_list[0][2]]) - np.array(c_pose) + np.array(
init_loc[0:3])) > 0.8 \
or sum_dist > 0.6):
return goal_list
if not first:
goal_list.pop(0)
if len(goal_list) == 0:
return []
omni.kit.commands.execute('ChangeProperty',
prop_path=Sdf.Path(f'{path}/base_link/x_joint.drive:linear:physics:stiffness'),
value=1200.0,
prev=0.0)
omni.kit.commands.execute('ChangeProperty',
prop_path=Sdf.Path(f'{path}/base_link/x_joint.drive:linear:physics:damping'),
value=1000.0,
prev=0.0)
omni.kit.commands.execute('ChangeProperty',
prop_path=Sdf.Path(f'{path}/base_link/x_joint.drive:linear:physics:maxForce'),
value=500.0,
prev=0.0)
omni.kit.commands.execute('ChangeProperty',
prop_path=Sdf.Path(f'{path}/base_link/x_joint.physxJoint:maxJointVelocity'),
value=200.0, # cm/s
prev=0.0)
omni.kit.commands.execute('ChangeProperty',
prop_path=Sdf.Path(f'{path}/base_link/x_joint.drive:linear:physics:targetPosition'),
value=(goal_list[0][0]) / meters_per_unit,
prev=0.0)
omni.kit.commands.execute('ChangeProperty',
prop_path=Sdf.Path(f'{path}/x_link/y_joint.drive:linear:physics:stiffness'),
value=1200.0,
prev=0.0)
omni.kit.commands.execute('ChangeProperty',
prop_path=Sdf.Path(f'{path}/x_link/y_joint.drive:linear:physics:damping'),
value=1000.0,
prev=0.0)
omni.kit.commands.execute('ChangeProperty',
prop_path=Sdf.Path(f'{path}/x_link/y_joint.drive:linear:physics:maxForce'),
value=500.0,
prev=0.0)
omni.kit.commands.execute('ChangeProperty',
prop_path=Sdf.Path(f'{path}/x_link/y_joint.physxJoint:maxJointVelocity'),
value=200.0,
prev=0.0)
omni.kit.commands.execute('ChangeProperty',
prop_path=Sdf.Path(f'{path}/x_link/y_joint.drive:linear:physics:targetPosition'),
value=(goal_list[0][1]) / meters_per_unit,
prev=0.0)
omni.kit.commands.execute('ChangeProperty',
prop_path=Sdf.Path(f'{path}/y_link/z_joint.drive:linear:physics:stiffness'),
value=1200.0,
prev=0.0)
omni.kit.commands.execute('ChangeProperty',
prop_path=Sdf.Path(f'{path}/y_link/z_joint.drive:linear:physics:damping'),
value=1000.0,
prev=0.0)
omni.kit.commands.execute('ChangeProperty',
prop_path=Sdf.Path(f'{path}/y_link/z_joint.drive:linear:physics:maxForce'),
value=500.0,
prev=0.0)
omni.kit.commands.execute('ChangeProperty',
prop_path=Sdf.Path(f'{path}/y_link/z_joint.physxJoint:maxJointVelocity'),
value=200.0,
prev=0.0)
omni.kit.commands.execute('ChangeProperty',
prop_path=Sdf.Path(f'{path}/y_link/z_joint.drive:linear:physics:targetPosition'),
value=(goal_list[0][2]) / meters_per_unit,
prev=0.0)
omni.kit.commands.execute('ChangeProperty',
prop_path=Sdf.Path(f'{path}/z_link/roll_joint.drive:angular:physics:stiffness'),
value=1200.0,
prev=0.0)
omni.kit.commands.execute('ChangeProperty',
prop_path=Sdf.Path(f'{path}/z_link/roll_joint.drive:angular:physics:damping'),
value=1000.0,
prev=0.0)
omni.kit.commands.execute('ChangeProperty',
prop_path=Sdf.Path(f'{path}/z_link/roll_joint.drive:angular:physics:maxForce'),
value=300.0,
prev=0.0)
omni.kit.commands.execute('ChangeProperty',
prop_path=Sdf.Path(f'{path}/z_link/roll_joint.physxJoint:maxJointVelocity'),
value=0.2,
prev=0.0)
omni.kit.commands.execute('ChangeProperty',
prop_path=Sdf.Path(f'{path}/z_link/roll_joint.drive:angular:physics:targetPosition'),
value=(goal_list[0][3]),
prev=0.0)
omni.kit.commands.execute('ChangeProperty',
prop_path=Sdf.Path(f'{path}/roll_link/pitch_joint.drive:angular:physics:stiffness'),
value=1200.0,
prev=0.0)
omni.kit.commands.execute('ChangeProperty',
prop_path=Sdf.Path(f'{path}/roll_link/pitch_joint.drive:angular:physics:damping'),
value=1000.0,
prev=0.0)
omni.kit.commands.execute('ChangeProperty',
prop_path=Sdf.Path(f'{path}/roll_link/pitch_joint.drive:angular:physics:maxForce'),
value=300.0,
prev=0.0)
omni.kit.commands.execute('ChangeProperty',
prop_path=Sdf.Path(f'{path}/roll_link/pitch_joint.physxJoint:maxJointVelocity'),
value=0.2,
prev=0.0)
omni.kit.commands.execute('ChangeProperty',
prop_path=Sdf.Path(f'{path}/roll_link/pitch_joint.drive:angular:physics:targetPosition'),
value=(goal_list[0][4]),
prev=0.0)
omni.kit.commands.execute('ChangeProperty',
prop_path=Sdf.Path(f'{path}/pitch_link/yaw_joint.drive:angular:physics:stiffness'),
value=1200.0,
prev=0.0)
omni.kit.commands.execute('ChangeProperty',
prop_path=Sdf.Path(f'{path}/pitch_link/yaw_joint.drive:angular:physics:damping'),
value=1000.0,
prev=0.0)
omni.kit.commands.execute('ChangeProperty',
prop_path=Sdf.Path(f'{path}/pitch_link/yaw_joint.drive:angular:physics:maxForce'),
value=300.0,
prev=0.0)
omni.kit.commands.execute('ChangeProperty',
prop_path=Sdf.Path(f'{path}/pitch_link/yaw_joint.physxJoint:maxJointVelocity'),
value=1.3,
prev=0.0)
omni.kit.commands.execute('ChangeProperty',
prop_path=Sdf.Path(f'{path}/pitch_link/yaw_joint.drive:angular:physics:targetPosition'),
value=(goal_list[0][5]),
prev=0.0)
return goal_list
def add_irotate_ros_components(camera_odom_frames, camera_odom_pubs, lidar_components, robot_base_prim_path, n):
"""
Add the irotate-specific ros-components to the robot.
"""
camera_odom_frames.append(f"{robot_base_prim_path}{n}/cameraholder_link")
camera_odom_pubs.append(rospy.Publisher(f"{robot_base_prim_path}{n}/camera_odom", Odometry, queue_size=10))
lidar_components.append(add_lidar(f"{robot_base_prim_path}{n}/lasersensor_link"), is_2d = True, is_3d=False)
def add_lidar(path, translation=[0, 0, 0], orientation=[0, 0, 0], is_2d=True, is_3d=False, degrees=True):
# drive sim applies 0.5,-0.5,-0.5,w(-0.5), we have to apply the reverse
base_or = tf.Rotation.from_quat([0.5, -0.5, -0.5, -0.5])
orientation = tf.Rotation.from_euler('XYZ', orientation, degrees=degrees)
orientation = (base_or * orientation).as_quat()
success, sensor = omni.kit.commands.execute(
"IsaacSensorCreateRtxLidar",
path="/RTX_Lidar",
parent=path,
config="Example_Rotary",
translation=(translation[0], translation[1], translation[2]),
orientation=Gf.Quatd(orientation[3], orientation[0], orientation[1], orientation[2]), # Gf.Quatd is w,i,j,k
)
omni.kit.app.get_app().update()
omni.kit.app.get_app().update()
omni.kit.app.get_app().update()
render_product_path = rep.create.render_product(sensor.GetPath().pathString, resolution=(1, 1))
# _, render_product_path = create_hydra_texture([1, 1], sensor.GetPath().pathString)
omni.kit.app.get_app().update()
omni.kit.app.get_app().update()
# add the lidar to the graph
# config is isaac_sim-2022.2.1/exts/omni.sensors.nv.lidar/data/Example_Rotary.json
if is_3d:
writer = rep.writers.get("RtxLidar" + "ROS1PublishPointCloud")
writer.initialize(topicName=f"{path}/lidar/point_cloud", frameId=path[1:])
writer.attach([render_product_path])
if is_2d:
writer = rep.writers.get("RtxLidar" + "ROS1PublishLaserScan")
writer.initialize(topicName=f"{path}/lidar/laser_scan", frameId=path[1:], rotationRate=100,
horizontalFov=360, depthRange=[0.1,10000], horizontalResolution=0.1)
writer.attach([render_product_path])
# todo for lidar one can change directly /Render/PostProcess/SDGPipeline/RenderProduct_Isaac_RtxSensorCpuIsaacComputeRTXLidarFlatScan
# but NOT for the 3d lidar
# todo theoretically I can avoid returning anything making just sure that I render at each loop
return omni.syntheticdata.SyntheticData._get_node_path(
"PostProcessDispatch" + "IsaacSimulationGate", render_product_path
)
def add_npy_viewport(viewport_window_list, robot_base_prim_path, n, old_h_ape, old_v_ape, config, sc,
tot_num_ros_cam=1):
viewport_npy, _ = create_viewport(f"{robot_base_prim_path}{n}/camera_link/Camera_npy", config["headless"].get(),
tot_num_ros_cam + 1 * n, config["npy_sensor_size"].get(), old_h_ape, old_v_ape, sc)
viewport_window_list.append(viewport_npy)
def change_joint_limit(joint: str, limit):
omni.kit.commands.execute('ChangeProperty',
prop_path=Sdf.Path(f'{joint}'),
value=(limit),
prev=0.0)
| 45,348 | Python | 45.086382 | 135 | 0.661066 |
eliabntt/GRADE-RR/simulator/utils/environment_utils.py | """
Use this class to load the environment and the relative information.
The init function should be used to load the environment.
It will get the environment from a given folder and create the necessary support variables.
"""
from omni.isaac.occupancy_map import _occupancy_map
from omni.isaac.occupancy_map.scripts.utils import update_location, compute_coordinates, generate_image
import utils.misc_utils
from utils.misc_utils import *
class environment:
def __init__(self, config, rng = np.random.default_rng(), local_file_prefix = '', meters_per_unit=0.01):
self.get_environment(config, rng, local_file_prefix)
self.meters_per_unit = meters_per_unit
def set_meters_per_unit(self, meters_per_unit):
self.meters_per_unit = meters_per_unit
def get_environment(self, config, rng: np.random.default_rng, local_file_prefix: str):
"""
If the name is not specified the environment will be taken at random using the rng.
Based on the config one can decide if
1. loading the stl of the environment
2. loading the environment limits with the npy file [note that this is preferable, otherwise default values will be used]
3. Using the limits the system will compute the necessary translations to center the environment in 0,0,0
config: the configuration processed by the main algorithm
rng: global rng
local_file_prefix: necessary to access the local storage from isaacsim
"""
self.env_usd_export_folder = config["env_path"].get()
if config["fix_env"].get() != "":
self.env_name = config["fix_env"].get()
else:
self.env_name = rng.choice([f for f in os.listdir(self.env_usd_export_folder) if not f.startswith('.')])
self.env_path = local_file_prefix + os.path.join(self.env_usd_export_folder, self.env_name, self.env_name + ".usd")
if config["use_stl"].get():
self.env_stl_path = os.path.join(self.env_usd_export_folder, self.env_name, self.env_name + ".stl")
self.env_mesh = trimesh.load(os.path.join(self.env_usd_export_folder, self.env_name, self.env_name + ".stl"))
else:
self.env_stl_path = None
self.env_mesh = None
if config["use_npy"].get():
self.env_info = np.load(os.path.join(self.env_usd_export_folder, self.env_name, self.env_name + ".npy"),
allow_pickle=True)
self.env_info = self.env_info.tolist()
else:
self.env_info = [0, 0, 0, 0, 0, 0, np.array([[-1000, -1000], [-1000, 1000], [1000, 1000], [1000, -1000]])]
self.env_limits = self.env_info[0:6]
self.shifts = [(self.env_limits[0] + self.env_limits[3]) / 2, (self.env_limits[1] + self.env_limits[4]) / 2,
self.env_limits[2]]
self.env_limits_shifted = [self.env_limits[i] - self.shifts[i % 3] for i, _ in enumerate(self.env_limits)]
self.area_polygon = get_area(self.env_info[6])
self.env_polygon = [Point(i[0], i[1], 0) for i in self.env_info[-1]]
def generate_map(self, out_path: str, zlim=[0, 1], cell_size = 0.05, origin=[0, 0, 0]):
"""
WARNING: HACK! ALL UNKNWON ARE WHITE!
Generates a map for the environment and save it to the out_path location in the disk.
First it searches for a non colliding location.
Then it creates a map of the environment.
We ovverride the unknown color to be "white" (i.e. free) as the system map unknown unreachable areas.
out_path: the folder where to save the map
z_limit: height to consider for projection
cell_size: size of a single cell in the map (cm)
origin: computed origin. Must be a free cell
"""
bound = int(
max(abs(self.env_limits_shifted[0]) + abs(self.env_limits_shifted[3]),
abs(self.env_limits_shifted[1]) + abs(self.env_limits_shifted[4])) / self.meters_per_unit * 1.5)
_om = _occupancy_map.acquire_occupancy_map_interface()
lower_bound = [-bound, -bound, zlim[0]/ self.meters_per_unit]
lower_bound = np.array(lower_bound) - np.array(origin) / self.meters_per_unit
upper_bound = [bound, bound, zlim[1]/ self.meters_per_unit *.8]
upper_bound = np.array(upper_bound) - np.array(origin) / self.meters_per_unit
center = np.array(origin) / self.meters_per_unit
center[2] += 0.1 / self.meters_per_unit # 10 cm above the floor
update_location(_om, center, lower_bound, upper_bound)
_om.set_cell_size(cell_size/self.meters_per_unit)
_om.generate()
image_buffer = generate_image(_om, [0, 0, 0, 255], [255, 255, 255, 255], [255, 255, 255, 255])
dims = _om.get_dimensions()
_im = Image.frombytes("RGBA", (dims.x, dims.y), bytes(image_buffer))
image_width = _im.width
image_height = _im.height
size = [0, 0, 0]
size[0] = image_width * cell_size
size[1] = image_height * cell_size
scale_to_meters = 1.0 / self.meters_per_unit
default_image_name = os.path.join(out_path, "map.png")
top_left, top_right, bottom_left, bottom_right, image_coords = compute_coordinates(_om, cell_size)
ros_yaml_file_text = "image: " + default_image_name
ros_yaml_file_text += f"\nresolution: {float(cell_size / scale_to_meters)}"
ros_yaml_file_text += (
f"\norigin: [{float(bottom_left[0] / scale_to_meters)}, {float(bottom_left[1] / scale_to_meters)}, 0.0000]"
)
ros_yaml_file_text += "\nnegate: 0"
ros_yaml_file_text += f"\noccupied_thresh: {0.65}"
ros_yaml_file_text += "\nfree_thresh: 0.196"
_im.save(default_image_name)
with open(default_image_name[:-3] + "yaml", 'w') as f:
f.write(ros_yaml_file_text)
center = lower_bound
center[2] = -100000000.0
update_location(_om, center, [0, 0, 0], [0, 0, 0])
_om.generate()
# disable_extension('omni.isaac.occupancy_map')
def load_and_center(self, prim_path: str = "/World/home", correct_paths_req: bool = False, push_in_floor: bool = False):
"""
Load the environment from the usd path env_path
Center it wrt the world coordinate frames
The environment is loaded at prim_path
prim_path: path that the environment should have in the prim tree
correct_paths_req: if True, corrects the paths of the assets in the environment
push_in_floor: if True, pushes the environment in the floor a bit. Useful for thin meshes that sometimes are not correctly visualized (flickering)
"""
stage = omni.usd.get_context().get_stage()
print("loading environment {}".format(self.env_name))
# from omni.isaac.core.utils.nucleus import find_nucleus_server
# result, nucleus_server = find_nucleus_server()
res, _ = omni.kit.commands.execute('CreateReferenceCommand',
usd_context=omni.usd.get_context(),
path_to=prim_path,
asset_path=self.env_path,
# asset_path= nucleus_server + "/Isaac/Environments/Simple_Warehouse/warehouse.usd",
instanceable=True)
if res:
clear_properties(prim_path)
if correct_paths_req:
print("Correcting paths... --- note that you might want to change utils/misc_utils.py:correct_paths")
try:
correct_paths(prim_path)
except:
print("Failed to correct paths for {}".format(prim_path))
time.sleep(10)
else:
print("Not correcting paths --- check that all textures are visibile and the reflection maps are correct")
# center the home in the middle of the environment
set_translate(stage.GetPrimAtPath(prim_path), list(- np.array(self.shifts) / self.meters_per_unit))
for child in stage.GetPrimAtPath(prim_path).GetAllChildren():
if "xform" == child.GetTypeName().lower():
clear_properties(str(child.GetPath()))
if push_in_floor and "floor" not in str(child.GetPath()).lower():
myold = child.GetProperty('xformOp:translate').Get()
myold = [myold[0], myold[1], myold[2] - 0.04]
set_translate(child, list(np.array(myold)))
return prim_path
else:
raise Exception("Failed to load environment {}".format(self.env_name))
| 7,793 | Python | 45.118343 | 148 | 0.674451 |
eliabntt/GRADE-RR/simulator/utils/UTILS.md | ## Environment utils
Used to manage the environment.
With these functions you can load and center the environment, create a 2D occupancy map (only if the collisions are turned on), and center the environment.
This is where you want to act if you want to remove the centering of the environment, create a different kind of occupancy, or do something specific while loading.
Nothing super-fancy here.
## Human utils
Human management functions.
Can load the human, correct the paths of the assets if necessary, move it to the ground. Just showcasing some functions.
## Misc utils
Used as a collage library.
There are tools to add semantic information, change the path of the textures, add colliders (or unset them), randomize lights and roughness of the materials, add translate and rotate animations, the service to position objects (that works with ROS), tools to rotate/translate objects, teleport the prim.
This is the main file you want to edit for example if you want to change the position strategy. Our position strategy uses FCL library from MoveIt and check collisios between two STL meshes. The system is done in a way in which it caches the environment and the robot stls at the beginning. We have different placement strategies for different objects (eg. humans, robot, and objects have different rules).
## Objects utils
Used to load the objects in the simulation. It will automatically convert the objects to the USD format to cache it. The objects are converted in local directories located in the GSO/shapenet folders. Semantic and collisions can be added to objects using thee utilities. Everything can be expanded easily by adding new object types.
The `shapenet` and `google_scanned_objects` folders are set up at runtime for example through the `os.environ["SHAPENET_LOCAL_DIR"]`.
## Robot utils
Various ways to create messages, add sensors, differentiate between poses (`create_diff_odom_message`), create viewports and publish stuff. Moreover, you want to use this to load your robot, set its initial joint locations and manage the trajectory. In general each component is loaded with auto publishing false and need to be automatically ticked or published. Some things like the odometry do not have a specific sensor but you can publish all the data that you want.
Edit if you need new sensors, publish different data, or remove sensors. You would also like to clean the code or add noise to the data directly here.
## Simulation utils
Mainly used for configuration settings (enalbe/disable extensions, change raytracing/pathtracing options), check that nucleus is powered up and ros is working, and manage the timeline.
| 2,661 | Markdown | 65.549998 | 470 | 0.796317 |
eliabntt/GRADE-RR/simulator/utils/zebra_utils.py | import utils.misc_utils
from omni.kit.sequencer.usd import SequenceSchema, usd_sequencer
from utils.misc_utils import *
def load_zebra(zebra_base_prim_path, n, asset_path):
stage = omni.usd.get_context().get_stage()
res, _ = omni.kit.commands.execute("CreateReferenceCommand",
usd_context=omni.usd.get_context(),
path_to=f"{zebra_base_prim_path}{n}",
asset_path=asset_path,
instanceable=False)
clear_properties(f"{zebra_base_prim_path}{n}")
return f"{zebra_base_prim_path}{n}"
def place_zebras(frame_info, rng, floor_points, meters_per_unit, hidden_position, config, max_anim_len, zebra_info):
stage = omni.usd.get_context().get_stage()
# create bool array as big as floor_points
occupied = np.zeros((floor_points.shape[0]-2, floor_points.shape[1]-2), dtype=bool)
deleted_zebras = []
out_frame_info = {}
min_number_zebras = config["min_number_zebras"].get()
max_number_zebras = config["max_number_zebras"].get()
selected_zebras = rng.choice(list(frame_info.keys()), size=int(rng.uniform(min_number_zebras, max_number_zebras)),
replace=False)
for zebra in selected_zebras:
out_frame_info[zebra] = frame_info[zebra].copy()
out_frame_info[zebra] = randomize_frame(out_frame_info[zebra], rng, max_anim_len, zebra_info)
# process the box and extract xmin xmax ymin ymax
box = np.array(out_frame_info[zebra]["box"])
xmin = np.min(box[:, 0])
xmax = np.max(box[:, 0])
ymin = np.min(box[:, 1])
ymax = np.max(box[:, 1])
# box is the 2D box
box = np.array([[xmin, ymin], [xmax, ymin], [xmax, ymax], [xmin, ymax]])
# random yaw rotation of the box
yaw = rng.uniform(0, 2 * np.pi)
# create a rotation matrix
rot = np.array([[np.cos(yaw), -np.sin(yaw)], [np.sin(yaw), np.cos(yaw)]])
# rotate the box
box = np.matmul(box, rot)
positioned = False
newbox = []
# get intermediate int points
for i in range(4):
p1 = np.round(box[i]).astype(int)
p2 = np.round(box[(i + 1) % 4]).astype(int)
# compute all int numbers between p1 and p2
dx = p2[0] - p1[0]
dy = p2[1] - p1[1]
if dx == 0:
x = p1[0]
y = np.arange(min(p1[1], p2[1]), max(p1[1], p2[1]) + 1 if max(p1[1], p2[1]) >= 0 else -1)
for j in range(len(y)):
newbox.append([x, y[j]])
elif dy == 0:
x = np.arange(min(p1[0], p2[0]), max(p1[0], p2[0]) + 1 if max(p1[0], p2[0]) >= 0 else -1)
y = p1[1]
for j in range(len(x)):
newbox.append([x[j], y])
elif dx == 0 and dy == 0:
newbox.append([p1[0], p1[1]])
else:
x = np.arange(min(p1[0], p2[0]), max(p1[0], p2[0]) + 1 if max(p1[0], p2[0]) >= 0 else -1)
y = p1[1] + (x - p1[0]) * dy / dx
for j in range(len(x)):
newbox.append([x[j], y[j]])
newbox = np.unique(np.array(newbox).astype(int), axis=0).astype(int)
for _ in range(100):
# get a random location in occupied -- this will be my center
center = np.array([rng.integers(0, occupied.shape[1]), rng.integers(0, occupied.shape[0])])
# check if all the cells covered by the box in occupied are free -- not only the boundaries
collision = False
for x_coor, y_coor in newbox:
try:
if occupied[center[0] - y_coor, center[1] + x_coor]:
collision = True
break
except IndexError:
collision = True
break
if collision:
break
if not collision:
tmp_floor_points = []
newcenter = np.array([center[0] + 1, center[1] + 1])
# if there is no collision, set the cells covered by the box to occupied
for x_coor, y_coor in newbox:
occupied[center[0] - y_coor, center[1] + x_coor] = True
# get the corresponding floor point given the center and x_coor and col
# NOTE THAT Y IS OPPOSITE SIGN
tmp_floor_points.append(floor_points[newcenter[0] - y_coor, newcenter[1] + x_coor])
# set the position of the zebra to the center
loc = np.mean(tmp_floor_points, axis=0) / meters_per_unit
loc = np.array(floor_points[newcenter[0], newcenter[1]]) / meters_per_unit
set_translate(stage.GetPrimAtPath(zebra), list(loc))
# set the rotation of the zebra to the roll, pitch, yaw
# lower_point = np.min(tmp_floor_points, axis=0)
# upper_point = np.max(tmp_floor_points, axis=0)
# vector = np.array(upper_point) - np.array(lower_point)
# compute roll pitch and yaw of vector
# roll, pitch, yaw = Rotation.from_rotvec(vector).as_euler("XYZ")
# transform = Rotation.from_matrix(
# trimesh.PointCloud(tmp_floor_points).bounding_box_oriented.transform[:3, :3]).as_euler("XYZ")
out_frame_info[zebra]["position"] = loc * meters_per_unit
out_frame_info[zebra]["rotation"] = [0, 0, yaw]
out_frame_info[zebra]["center"] = newcenter
out_frame_info[zebra]["box"] = box
set_rotate(stage.GetPrimAtPath(zebra), [0, 0, yaw]) # todo refine this to account for terrain
positioned = True
break
if not positioned:
print("Could not position zebra", zebra)
# delete the zebra
deleted_zebras.append(zebra)
set_translate(stage.GetPrimAtPath(zebra), list(hidden_position))
for zebra in deleted_zebras:
del out_frame_info[zebra]
return out_frame_info
def randomize_frame(zebra, rng, max_anim_len, zebra_info):
stage = omni.usd.get_context().get_stage()
zebra_path = zebra["path"]
scale = rng.integers(40, 100)
set_scale(stage.GetPrimAtPath(zebra_path), scale)
zebra_name = zebra["name"]
prim = stage.GetPrimAtPath(f"/World/Sequence{zebra_path}{zebra_path}_Clip")
anim_len = zebra_info[zebra_name]["length"]
timeslot = max_anim_len - rng.integers(0, anim_len)
prim.GetAttribute("startTime").Set(Sdf.TimeCode(timeslot * 1.0))
prim.GetAttribute("endTime").Set(
Sdf.TimeCode(float(max(timeslot + zebra_info[zebra_name]["length"], max_anim_len))))
points_in_mesh = zebra_info[zebra_name]["points"][max_anim_len - timeslot] * scale / 100
zebra = {"name": zebra_name, "time": timeslot, "used_frame": max_anim_len - timeslot + 1,
"scale": scale, "box": trimesh.PointCloud(points_in_mesh).bounding_box.vertices,
"path": zebra_path}
return zebra
def preload_all_zebras(config, rng, zebra_files, zebra_info, simulation_context, sequencer_drop_controller, max_anim_len,
hidden_position):
stage = omni.usd.get_context().get_stage()
# load a random number of zebras between min_number_zebra and max_number_zebra
num_zebras = config["max_number_zebras"].get()
frame_info = {}
for n in range(num_zebras):
# load a random zebra
zebra_file = rng.choice(zebra_files)
# load the zebra
zebra_path = load_zebra("/zebra_", n, zebra_file)
scale = rng.integers(40, 100)
set_scale(stage.GetPrimAtPath(zebra_path), scale)
zebra_name = zebra_file.split("/")[-1].split(".")[0]
add_semantics(stage.GetPrimAtPath(zebra_path), "zebra")
timeslot = max_anim_len - rng.integers(0, zebra_info[zebra_name]["length"])
sequencer_drop_controller.sequencer_drop(stage.GetPrimAtPath("/World/Sequence"), zebra_path, float(timeslot))
prim = stage.GetPrimAtPath(f"/World/Sequence{zebra_path}{zebra_path}_Clip")
prim.GetAttribute("startTime").Set(Sdf.TimeCode(timeslot * 1.0))
prim.GetAttribute("endTime").Set(
Sdf.TimeCode(float(max(timeslot + zebra_info[zebra_name]["length"], max_anim_len))))
points_in_mesh = zebra_info[zebra_name]["points"][max_anim_len - timeslot] * scale / 100
frame_info[zebra_path] = {"name": zebra_name, "time": timeslot, "used_frame": max_anim_len - timeslot + 1,
"scale": scale, "box": trimesh.PointCloud(points_in_mesh).bounding_box.vertices,
"path": zebra_path}
simulation_context.step(render=False)
simulation_context.render()
set_translate(stage.GetPrimAtPath(zebra_path), hidden_position)
return frame_info
| 7,881 | Python | 40.052083 | 121 | 0.642431 |
eliabntt/GRADE-RR/simulator/utils/misc_utils.py | import asyncio
import carb
import ipdb
import json
import ntpath
import numpy as np
import os
import pickle as pkl
from PIL import Image
from pyquaternion import Quaternion
import scipy.spatial.transform as tf
from stl import mesh
import time
import trimesh
from typing import Dict, Optional, Union
# ros
import rospy, rosgraph
from geometry_msgs.msg import PoseStamped, Point
from nav_msgs.msg import Odometry
from sensor_msgs.msg import Imu
from std_msgs.msg import String
# omni
import omni.isaac.shapenet as shapenet
import omni.kit
from omni.isaac import RangeSensorSchema
from omni.isaac.core import SimulationContext, PhysicsContext
import omni.replicator.core as rep
from omni.isaac.core.prims import XFormPrim
from omni.isaac.core.utils.carb import set_carb_setting
from omni.isaac.core.utils.extensions import enable_extension, disable_extension
from omni.isaac.core.utils.stage import is_stage_loading, set_stage_up_axis
from omni.isaac.dynamic_control import _dynamic_control
import omni.isaac.IsaacSensorSchema as IsaacSensorSchema
from omni.isaac.synthetic_recorder import extension_custom
from omni.physxcommands import SetStaticColliderCommand, RemoveStaticColliderCommand
from pxr import UsdGeom, Gf, Usd, UsdSkel, AnimationSchema, Semantics, UsdPhysics, Sdf, UsdShade
from pxr.Usd import Prim
# 2022 edits
import omni.graph.core as og
from omni.isaac.core_nodes.scripts.utils import set_target_prims
def add_semantics(prim: Prim, semantic_label: str):
"""
Adds semantic labels to the prim.
prim: the prim to add the semantic label to
semantic_label: the semantic label to add
"""
if not prim.HasAPI(Semantics.SemanticsAPI):
sem = Semantics.SemanticsAPI.Apply(prim, "Semantics")
sem.CreateSemanticTypeAttr()
sem.CreateSemanticDataAttr()
else:
sem = Semantics.SemanticsAPI.Get(prim, "Semantics")
sem.GetSemanticTypeAttr().Set("class")
sem.GetSemanticDataAttr().Set(str(semantic_label))
def correct_paths(parent_name: str):
"""
Helper function to correct the paths of the world's materials (as they come from Windows).
parent_name: the prim path of the father.
"""
stage = omni.usd.get_context().get_stage()
for prim in stage.Traverse():
shader_path = prim.GetPath()
if parent_name.lower() in str(shader_path).lower():
if prim.GetTypeName().lower() == "mesh":
prim.GetProperty('doubleSided').Set(False)
if prim.GetTypeName().lower() == "shader":
try:
change_shader_path(shader_path)
except:
print(f"Error changing shader of in {shader_path}")
time.sleep(5)
def change_shader_path(shader_path: str):
"""
Changes the shader path of the material.
material_path: the prim path to the material collection (e.g. "/World/my_robot_0/materials, /World/home/materials")
"""
stage = omni.usd.get_context().get_stage()
shader = stage.GetPrimAtPath(shader_path)
if 'inputs:diffuse_texture' in shader.GetPropertyNames():
old_path = str(shader.GetAttribute('inputs:diffuse_texture').Get().resolvedPath)
new_path = old_path.replace("@", "")
# print(f"Changing path {old_path}")
if "something" in old_path or "P:" in old_path:
new_path = old_path.replace(ntpath.sep, os.sep).replace('P:/', '').replace("@", "")
elif "somethingelse" in old_path.lower():
splitted = old_path.split(ntpath.sep)
tmp_path = ""
for i in splitted:
tmp_path += i + ntpath.sep
if "something" in i:
break
tmp_path = tmp_path.replace(ntpath.sep, os.sep)
new_path = old_path.replace(ntpath.sep, os.sep).replace(tmp_path, '').replace(
"@", "")
shader.GetAttribute('inputs:diffuse_texture').Set(new_path)
if 'inputs:reflectionroughness_texture' in shader.GetPropertyNames():
old_path = str(shader.GetAttribute('inputs:reflectionroughness_texture').Get().resolvedPath)
new_path = old_path.replace("@", "")
# print(f"Changing path {old_path}")
if "something" in old_path or "P:" in old_path:
new_path = old_path.replace(ntpath.sep, os.sep).replace('P:/', '').replace("@", "")
elif "somethingelse" in old_path.lower():
splitted = old_path.split(ntpath.sep)
tmp_path = ""
for i in splitted:
tmp_path += i + ntpath.sep
if "something" in i:
break
tmp_path = tmp_path.replace(ntpath.sep, os.sep)
new_path = old_path.replace(ntpath.sep, os.sep).replace(tmp_path, '').replace(
"@", "")
shader.GetAttribute('inputs:reflectionroughness_texture').Set(new_path)
def set_colliders(path_main_asset: str, value: bool):
"""
It takes a path to a main asset, and a boolean value, and sets the physics:collisionEnabled attribute to the boolean
value for all children of the main asset. This effectively enable or disable collisions.
:param path_main_asset: The path to the main asset in the USD file
:type path_main_asset: str
:param value: bool
:type value: bool
"""
stage = omni.usd.get_context().get_stage()
for j in stage.GetPrimAtPath(path_main_asset).GetAllChildren():
for i in j.GetAllChildren():
if "physics:collisionEnabled" in i.GetPropertyNames():
if i.GetProperty("physics:collisionEnabled").Get() == value:
continue
i.GetProperty("physics:collisionEnabled").Set(value)
def add_colliders(path_main_asset: str):
"""
Adds the colliders to the main asset. This allows the object to have collisions or not (if supported).
Return True if the colliders were added, False otherwise.
path_main_asset: the path of the prim asset whose childs need to be processed
"""
stage = omni.usd.get_context().get_stage()
fres = True
for prim in stage.Traverse():
prim_path = prim.GetPath()
if path_main_asset.lower() in str(prim_path).lower():
if prim.GetTypeName().lower() == "mesh" or prim.GetTypeName().lower() == "xform":
res, _ = SetStaticColliderCommand.execute(str(prim.GetPath()))
fres = res and fres
return fres
def process_semantics(parent_name: str, name_to_label: str = None):
"""
Processes the semantics of the world.
In case the name_to_label is specified (not coming from Front3D), it will be set to the name_to_label param.
parent_name: the prim path of the father.
label: the eventual label to give to the set of assets
"""
for prim in omni.usd.get_context().get_stage().Traverse():
primpath = prim.GetPath()
if parent_name.lower() in str(primpath).lower():
if prim.GetTypeName().lower() == "mesh" or prim.GetTypeName().lower() == "xform":
if name_to_label == None:
# tmp = prim.GetAttribute('userProperties:category_id')
tmp = prim.GetAttribute('userProperties:semantic')
if tmp.Get() != None:
add_semantics(prim, str(tmp.Get()))
else:
add_semantics(prim, name_to_label)
def randomize_and_fix_lights(config: dict, rng: np.random.default_rng, parent_name: str, z_lim, meters_per_unit,
is_rtx: bool = False):
"""
Randomize the lights within an environment
config: the configuration dict with the parameters and enabled/disabled config for intensity/color
rng: global rng
parent_name: parent whose childs need to be considered to change the lights
"""
stage = omni.usd.get_context().get_stage()
if not (config["intensity"] or config["color"]):
return
min_int = config.get("intensity_interval", 0.0)[0]
max_int = config.get("intensity_interval", 1.0)[1]
for prim in stage.Traverse():
path = prim.GetPath()
if parent_name.lower() in str(path).lower():
if "light" in prim.GetTypeName().lower():
if "environment" in str(path).lower():
continue
if config["intensity"]:
prim.GetAttribute('intensity').Set(rng.uniform(low=min_int, high=max_int))
if config["color"]:
col = rng.random(size=3)
prim.GetAttribute('color').Set(Gf.Vec3f(col[0], col[1], col[2]))
if not is_rtx:
prim.GetAttribute('diffuse').Set(4)
prim.GetAttribute('specular').Set(4)
# FIXME no actual check I'm not moving other stuff. but this should work based on the "existance" of segmentation info and that lights on its own does not have a translation attribute
z_lamp = omni.usd.get_world_transform_matrix(prim)[3, 2] * meters_per_unit
if z_lamp > z_lim - 0.08:
diff = z_lamp - z_lim - 0.08
while not prim.HasAttribute('xformOp:translate'):
prim = prim.GetParent()
# while (not "semantic:Semantics:params:semanticData" in parent.GetPropertyNames()):
# parent = parent.GetParent()
p_lamp = prim.GetAttribute('xformOp:translate').Get()
p_lamp[2] -= diff
prim.GetAttribute('xformOp:translate').Set(p_lamp)
# move the light if it is too high
def randomize_roughness(config: dict, rng: np.random.default_rng, parent_name: str):
"""
Randomize the roughness (reflectivity) of assets within an environment
config: the configuration dict with the parameters and enabled/disabled config for intensity/color
rng: global rng
parent_name: parent whose childs need to be considered to change the lights
"""
stage = omni.usd.get_context().get_stage()
if not (config["enabled"]):
return
min_int = config.get("intensity_interval", 0.0)[0]
max_int = config.get("intensity_interval", 1.0)[1]
for prim in stage.Traverse():
path = prim.GetPath()
if parent_name.lower() in str(path).lower():
if prim.GetTypeName().lower() == "material" or prim.GetTypeName().lower() == "shader":
if "inputs:RoughnessMin" in prim.GetPropertyNames():
val = rng.uniform(low=min_int, high=max_int)
prim.GetAttribute('inputs:RoughnessMin').Set(val)
prim.GetAttribute('inputs:RoughnessMax').Set(val)
def get_area(polygon):
"""
Computes the area of a polygon.
"""
x = polygon[:, 0]
y = polygon[:, 1]
return .5 * np.absolute(np.dot(x, np.roll(y, 1)) - np.dot(y, np.roll(x, 1)))
def change_prim_collision(enable, prim_path):
for j in omni.usd.get_context().get_stage().Traverse():
if str(j.GetPath()).startswith(prim_path):
if 'physics:collisionEnabled' in j.GetPropertyNames():
omni.kit.commands.execute('ChangeProperty',
prop_path=Sdf.Path(str(j.GetPath())+'.physics:collisionEnabled'),
value=enable,
prev=None)
def change_collision_at_path(enable, paths=['/my_robot_0/camera_link/Cube.physics:collisionEnabled','/my_robot_0/yaw_link/visuals.physics:collisionEnabled']):
"""
It enables or disables collisions for the paths
:param enable: True or False
"""
for path in paths:
omni.kit.commands.execute('ChangeProperty',
prop_path=Sdf.Path(path),
value=enable,
prev=None)
def add_translate_anim(prim_path: str, pos: Gf.Vec3d, time: float = 0.0):
"""
Add a goal location at a given timecode. The object will EVENTUALLY move there with a smooth movement.
prim_path: the path of the asset to be moved
pos: the final position
time: the time in FRAME
"""
omni.kit.commands.execute('ChangePropertyCommand',
prop_path=prim_path + '.xformOp:translate',
value=pos,
prev=Gf.Vec3d(0, 0, 0),
type_to_create_if_not_exist=UsdGeom.XformOp.TypeTranslate,
timecode=Usd.TimeCode(time))
def add_rotation_anim(prim_path: str, rot: list, time: float = 0.0, use_double=False):
"""
Add a goal rotation at a given timecode. The object will EVENTUALLY move there with a smooth movement.
EXPECT ROT IN RAD!
prim_path: the path of the asset to be moved
rot: the final position
time: the time in FRAME
"""
rot = np.array(rot) * 180 / np.pi
quat = (
Gf.Rotation(Gf.Vec3d.XAxis(), rot[0])
* Gf.Rotation(Gf.Vec3d.YAxis(), rot[1])
* Gf.Rotation(Gf.Vec3d.ZAxis(), rot[2])
)
omni.kit.commands.execute('ChangePropertyCommand',
prop_path=prim_path + ".xformOp:orient",
value=Gf.Quatf(quat.GetQuat()) if not use_double else Gf.Quatd(quat.GetQuat()),
prev=Gf.Quatf(0, 0, 0, 1) if not use_double else Gf.Quatd(0, 0, 0, 1),
type_to_create_if_not_exist=UsdGeom.XformOp.TypeOrient,
timecode=Usd.TimeCode(time))
def inf_helper(y: np.array):
"""Helper to handle indices and logical indices of NaNs.
Input:
- y, 1d numpy array with possible NaNs
Output:
- nans, logical indices of NaNs
- index, a function, with signature indices= index(logical_indices),
to convert logical indices of NaNs to 'equivalent' indices
"""
return np.isinf(y), lambda z: z.nonzero()[0]
def position_object(environment, type: int, objects: list = [], ob_stl_paths: list = [], reset: bool = False,
max_collisions: int = 200):
"""
type = 0 -> camera z_lim = [0.8 - 1.8] using camera stl
type = 1 -> humans z_lim = [0 - 0] using human stl
type = 2 -> shapenet z_lim = [0 - 1.8] using camera stl
type = 3 -> origin z_lim = [0 - 0] using camera stl
note: when min == max we apply a small offset to the max to address shifts in the z-axis to allow small collisions.
However, the result will be still published at the wanted height.
envionment: the environment object
type: see above
objects: the list of objects to be placed
ob_stl_paths: the corresponding stls
reset: if the collision checker need to be resetted forcefully
"""
# thih import will work if you compile our https://github.com/eliabntt/moveit_based_collision_checker_and_placement/tree/main
# and you add the source catkin command to isaac_X_X/setup_python_env.sh
from collision_check.srv import *
if environment.env_stl_path == None:
print(
"No stl is being loaded for the environment, please pre-fix all objects locations or implement your own strategy")
environment.env_stl_path = ""
print("Wait for service")
rospy.wait_for_service("/fake/collision_checker/check")
print("Service loaded")
try:
check_collision = rospy.ServiceProxy("/fake/collision_checker/check", collision_check_srv)
req = collision_check_srvRequest()
req.env_stl_path = environment.env_stl_path
req.env_polygon = environment.env_polygon
req.reset = reset
if type == 1:
for ob in objects:
req.ob_names.append(ob)
req.ob_stl_paths = ob_stl_paths
req.is_cam = True if type != 1 else False
min_z = (0.8 + environment.env_limits[2]) if type == 0 else environment.env_limits[2]
max_z = environment.env_limits[2] if (type == 1 or type == 3) else min(1.8 + environment.env_limits[2],
environment.env_limits[5] - 0.5)
if type == 4:
min_z = environment.env_limits[2]
max_z = environment.env_limits[2]
has_forced_z = -1
if min_z == max_z:
max_z += 0.5
has_forced_z = min_z
req.min_limits = [environment.env_limits[0] + 0.5, environment.env_limits[1] + 0.5, min_z]
req.max_limits = [environment.env_limits[3] - 0.5, environment.env_limits[4] - 0.5, max_z]
req.limit_collision = 0 if type != 1 else max_collisions
req.forced_z = has_forced_z
res = check_collision.call(req)
if has_forced_z != -1:
res.z = [min(has_forced_z, z) for z in res.z]
return np.array(res.x) - environment.shifts[0], np.array(res.y) - environment.shifts[1], np.array(res.z) - \
environment.shifts[2], res.yaw
except rospy.ServiceException as e:
print("Service call failed: %s" % e)
return [-1] * len(objects), [-1] * len(objects), [-1] * len(objects), [0] * len(objects)
def set_scale(prim: Prim, scale: float = 1.0):
"""
Set the scale of a Prim
prim: the prim
scale: the scale
"""
prop_names = prim.GetPropertyNames()
if "xformOp:scale" not in prop_names:
xformable = UsdGeom.Xformable(prim)
xform_op_scale = xformable.AddXformOp(UsdGeom.XformOp.TypeScale, UsdGeom.XformOp.PrecisionDouble, "")
else:
xform_op_scale = UsdGeom.XformOp(prim.GetAttribute("xformOp:scale"))
xform_op_scale.Set(Gf.Vec3d([scale, scale, scale]))
def clear_properties(path: str):
"""
The function clears all the POSE properties of the given prim.
This is to ensure a consistent way of setting those properties for different objects.
This should be called with ALL loaded objects so that we have consistent xformOp:trans/Orient
"""
current_position, current_orientation = XFormPrim(path).get_world_pose()
def set_translate(prim: Prim, new_loc: list):
"""
prim: must be prim type, the prim to be moved
new_loc: list [x-y-z] for the single prim
"""
properties = prim.GetPropertyNames()
if "xformOp:translate" in properties:
translate_attr = prim.GetAttribute("xformOp:translate")
translate_attr.Set(Gf.Vec3d(new_loc))
elif "xformOp:transform" in properties:
transform_attr = prim.GetAttribute("xformOp:transform")
matrix = prim.GetAttribute("xformOp:transform").Get()
matrix.SetTranslateOnly(Gf.Vec3d(new_loc))
transform_attr.Set(matrix)
else:
xform = UsdGeom.Xformable(prim)
xform_op = xform.AddXformOp(UsdGeom.XformOp.TypeTranslate, UsdGeom.XformOp.PrecisionDouble, "")
xform_op.Set(Gf.Vec3d(new_loc))
def set_rotate(prim: XFormPrim, rot: list):
"""
expects rot in rad
prim: The prim to be rotated
rot: roll-pitch-yaw in RAD
"""
properties = prim.GetPropertyNames()
rot = np.array(rot) * 180 / np.pi
quat = (
Gf.Rotation(Gf.Vec3d.XAxis(), rot[0])
* Gf.Rotation(Gf.Vec3d.YAxis(), rot[1])
* Gf.Rotation(Gf.Vec3d.ZAxis(), rot[2])
)
if "xformOp:orient" in properties:
rotation = prim.GetAttribute("xformOp:orient")
rotation.Set(Gf.Quatd(quat.GetQuat()))
else:
xform = UsdGeom.Xformable(prim)
xform_op = xform.AddXformOp(UsdGeom.XformOp.TypeOrient, UsdGeom.XformOp.PrecisionDouble, "")
xform_op.Set(Gf.Quatd(quat.GetQuat()))
def dynamic_control_interface():
"""
This is placed here as the extension is not loaded in the main script.
"""
return _dynamic_control.acquire_dynamic_control_interface()
def reload_references(path):
"""
It reloads all the references and payloads of a given prim
:param path: The path to the prim you want to reload references for
"""
stage = omni.usd.get_context().get_stage()
prim_list = []
for j in stage.GetPrimAtPath(path).GetAllChildren():
prim_list.append(j)
layers = set()
for prim in prim_list:
for (ref, intro_layer) in omni.usd.get_composed_references_from_prim(prim):
layer = Sdf.Find(intro_layer.ComputeAbsolutePath(ref.assetPath)) if ref.assetPath else None
if layer:
layers.add(layer)
for (ref, intro_layer) in omni.usd.get_composed_payloads_from_prim(prim):
layer = Sdf.Find(intro_layer.ComputeAbsolutePath(ref.assetPath)) if ref.assetPath else None
if layer:
layers.add(layer)
for l in layers:
l.Reload(force=True)
def teleport(path, loc, rot):
"""
It teleports the object at the given path to the given location and rotation
:param path: The path to the object you want to teleport
:param loc: (x, y, z)
:param rot: (x, y, z, w)
"""
omni.kit.commands.execute(
"IsaacSimTeleportPrim",
prim_path=path,
translation=(loc[0], loc[1], loc[2]),
rotation=(rot[0], rot[1], rot[2], rot[3]),
)
def toggle_dynamic_objects(dynamic_prims: list, status: bool):
"""
It toggles the visibility of the dynamic objects in the scene
:param dynamic_prims: a list of prims that you want to toggle
:type dynamic_prims: list
"""
# print("Toggling environment...")
for _ in range(3):
for prim in dynamic_prims:
imageable = UsdGeom.Imageable(prim)
if status:
imageable.MakeVisible()
else:
imageable.MakeInvisible()
imageable = []
def reset_physics(timeline, simulation_context):
timeline.stop()
simulation_context.reset()
timeline.play() | 20,439 | Python | 39 | 191 | 0.658056 |
eliabntt/GRADE-RR/simulator/utils/simulation_utils.py | import time
import utils.misc_utils
from utils.misc_utils import *
GRAPH_PATH = "/Render/PostProcess/SDGPipeline"
def set_common_stage_properties(rate):
"""
Note: some properties as of now can only be set with the general environment USD file.
"""
_desired_render_settings: Dict[str, Union[bool, int]] = {
"/app/asyncRendering": False,
"/app/renderer/skipWhileMinimized": False,
"/app/renderer/sleepMsOnFocus": 0,
"/app/renderer/sleepMsOutOfFocus": 0,
"/app/runLoops/main/rateLimitEnabled": True,
"/app/runLoops/main/rateLimitFrequency": rate,
"/persistent/simulation/minFrameRate": rate,
"/app/runLoops/main/rateLimitUseBusyLoop": True,
"/app/runLoops/rendering_0/rateLimitEnabled": True,
"/app/viewport/showSettingMenu": True,
"/app/viewport/showCameraMenu": True,
"/app/viewport/showRendererMenu": True,
"/app/viewport/showHideMenu": True,
"/app/viewport/showLayerMenu": True,
"/app/viewport/grid/showOrigin": False,
"/app/viewport/grid/enabled": False, ## this does not work
"/persistent/app/viewport/grid/lineWidth": 0,
"/rtx/multiThreading/enabled": True,
"/app/asyncRenderingLowLatency": False,
# "/persistent/app/captureFrame/viewport": True,
}
for setting_key, desired_value in _desired_render_settings.items():
set_carb_setting(carb.settings.get_settings(), setting_key, desired_value)
def simulation_environment_setup(need_ros = True):
"""
Enable the necessary extensions that will be used within the simulation
"""
enable_extension("omni.isaac.ros_bridge")
enable_extension("omni.isaac.physics_inspector")
enable_extension("omni.isaac.physics_utilities")
enable_extension("omni.anim.skelJoint")
enable_extension("omni.kit.window.sequencer")
enable_extension("omni.isaac.dynamic_control")
enable_extension("omni.isaac.shapenet")
enable_extension("semantics.schema.editor")
enable_extension("omni.hydra.iray")
enable_extension("omni.iray.settings.core")
enable_extension('omni.isaac.occupancy_map')
enable_extension('omni.isaac.shapenet')
enable_extension('omni.isaac.range_sensor')
disable_extension('omni.isaac.sun_study')
enable_extension('omni.isaac.core_nodes')
enable_extension('omni.isaac.sensor')
# Necessary ONLY if using NUCLEUS
# Locate /Isaac folder on nucleus server to load sample
from omni.isaac.core.utils.nucleus import get_assets_root_path
nucleus_server = get_assets_root_path()
if nucleus_server is None:
carb.log_error("Could not find nucleus server with /Isaac folder, exiting")
exit()
if need_ros:
if not rosgraph.is_master_online():
carb.log_error("Please run roscore before executing this script")
exit()
def set_raytracing_settings(physics_hz):
set_common_stage_properties(physics_hz)
settings = carb.settings.get_settings()
settings.set("/app/hydraEngine/waitIdle", True)
settings.set_string("/rtx/rendermode", "RayTracing")
settings.set_int('/rtx/post/aa/op', 2)
def set_pathtracing_settings(physics_hz):
set_common_stage_properties(physics_hz)
settings = carb.settings.get_settings()
settings.set_string("/rtx/rendermode", "PathTracing")
settings.set_int('/rtx/post/aa/op', 1)
# settings.set_int('/rtx/multiThreading/enabled', True)
# settings.set_bool('/rtx/multiThreading/enabled', True)
settings.set_int('/rtx/post/histogram/filterType', 1)
settings.set_int('/rtx/post/histogram/tau', 100)
settings.set_float('/rtx/post/histogram/minEV', 2)
settings.set_float('/rtx/post/histogram/maxEV', 50)
settings.set_bool('/rtx/post/histogram/enabaled', True)
settings.set_int('/rtx/post/tonemap/filmIso', 100) # 400
settings.set_int('/rtx/post/tonemap/cameraShutter', 30)
settings.set_int('/rtx/post/tonemap/fStop', 4)
settings.set_int("/rtx/pathtracing/maxBounces", 6) # 6
settings.set_int("/rtx/pathtracing/maxSpecularAndTransmissionBounces", 6)
# settings.set_int("/rtx/pathtracing/maxDiffuseBounces", 10)
settings.set_int("/rtx/pathtracing/spp", 1)
settings.set_int("/rtx/pathtracing/totalSpp", 64)
settings.set_int("/rtx/pathtracing/clampSpp", 64)
settings.set_int("/rtx/pathtracing/cached/enabled", False)
settings.set_bool("/rtx/pathtracing/cached/enabled", False)
settings.set_int("/rtx/pathtracing/lightcache/cached/enabled", False)
settings.set_bool("/rtx/pathtracing/lightcache/cached/enabled", False)
settings.set("/app/hydraEngine/waitIdle", False)
def compute_timeline_ratio(human_anim_len, reverse_strategy, experiment_length):
"""
based on the reverse strategy compute how the system should roll back animations
This might be counter-productive in some instances
"""
if len(human_anim_len) == 0:
return 1
if reverse_strategy == "avg":
return float(experiment_length) / (sum(human_anim_len) / len(human_anim_len))
elif reverse_strategy == "min":
return float(experiment_length) / min(human_anim_len)
elif reverse_strategy == "max":
return float(experiment_length) / max(human_anim_len)
elif reverse_strategy == "half":
return 2
elif reverse_strategy == "none":
return 1
else:
return 1
def pub_and_write_images(simulation_context, viewport_window_list, ros_camera_list, raytracing, my_recorder=None, enable_recorder=True):
sleeping(simulation_context, viewport_window_list, raytracing)
ctime = omni.timeline.get_timeline_interface().get_current_time()
for i, cam, outs in ros_camera_list:
print(f"Publishing camera {cam}...")
for output in outs:
og.Controller.attribute(output+ ".inputs:step").set(1)
simulation_context.render()
for i, cam, outs in ros_camera_list:
for output in outs:
og.Controller.attribute(output+ ".inputs:step").set(0)
omni.timeline.get_timeline_interface().set_current_time(ctime)
if my_recorder and my_recorder._enable_record and enable_recorder:
my_recorder._update()
print("Writing")
def sleeping(simulation_context, viewport_window_list, raytracing, totalSpp=64, spp=1):
"""
Sleeps the simulation to be sure that the whole frame has been rendered and updated.
First we render a couple of frames.
In rtx mode we need to wait the fps of the viewport to be reached.
In pathtracing mode we need to do "/rtx/pathtracing/spp" rendering steps.
e.g.
carb.settings.get_settings().get("/rtx/pathtracing/totalSpp")
carb.settings.get_settings().get("/rtx/pathtracing/spp")
"""
# todo is there a better way? I don"t think so, this is variable
# fixme making sure timeline does not advance
timeline = omni.timeline.get_timeline_interface()
mytime = timeline.get_current_time()
if raytracing:
sleep_time = 0
start = time.time()
for _ in range(100):
for vp in viewport_window_list:
if vp.fps == 0: continue
sleep_time = max(1 / vp.fps * 1.1, sleep_time)
if sleep_time != 0 and time.time() - start > sleep_time * 2: # overly cautious
break
simulation_context.render()
timeline.set_current_time(mytime)
else:
cnt = totalSpp
increase = spp
while cnt >= 0:
simulation_context.render()
timeline.set_current_time(mytime)
cnt -= increase
simulation_context.render()
timeline.set_current_time(mytime)
simulation_context.render()
timeline.set_current_time(mytime)
time.sleep(0.2)
def recorder_setup(_recorder_settings, out_path, enabled, skip_cameras=1):
my_recorder = extension_custom.MyRecorder()
my_recorder.on_startup()
my_recorder.set_single_settings(_recorder_settings)
my_recorder._dir_name = os.path.join(out_path)
my_recorder._enable_record = enabled
my_recorder.skip_cameras = skip_cameras
return my_recorder
def setup_timeline(config):
"""
It sets up the timeline to have a start time of 0.0, an end time of the experiment length * 2, and a time code per
second of the fps
:param config: a dictionary of parameters that are used to configure the experiment
:return: timeline
"""
timeline = omni.timeline.get_timeline_interface()
timeline.set_start_time(0.0)
if "fps" not in config:
fps = 30
else:
fps = config['fps'].get()
if "experiment_length" in config:
timeline.set_end_time(config["experiment_length"].get() * 2 / fps) # *2 to have room
else:
print("No experiment length found, setting it to 3600")
timeline.set_end_time(3600 / fps)
timeline.set_time_codes_per_second(fps)
return timeline
| 8,162 | Python | 35.936651 | 136 | 0.735849 |
eliabntt/GRADE-RR/simulator/utils/human_utils.py | import utils.misc_utils
from utils.misc_utils import *
def move_humans_to_ground(my_humans_heights: list, body_lists: list, frame: float, meters_per_unit: float,
max_height: float):
"""
Function to keep the human at ground level (0 for now, but can be elaborated)
my_human_heights: list of [animation_frames, [vertices, z_loc]]. For every frame of the animation, for every vertex, the z loc
body_lists: Using to access the prim, list of prim paths
frame: the simulation frame we are in (float or int will get a cast to int)
meters_per_unit: meter per unit of distance in the simulation
"""
stage = omni.usd.get_context().get_stage()
for index, height in enumerate(my_humans_heights):
z_min = None
if height is None:
context = omni.usd.get_context()
stage = context.get_stage()
prim = stage.GetPrimAtPath(body_lists[index])
for i in prim.GetAllChildren():
if "armature" in str(i.GetPath()).lower():
prim = i
for i in prim.GetAllChildren():
if "body" in str(i.GetPath()).lower():
prim = i
for i in prim.GetAllChildren():
if "mesh" in str(i.GetPath()).lower():
prim = i
l = prim.GetPropertyNames()
if "points" in l:
k = prim.GetAttribute("points").Get()
if k is not None:
k = np.array(k)
z_min = min(k[:, 2])
else:
z_min = min(height[int(min(max(frame - 1, 0), len(height) - 1))]) / meters_per_unit
if z_min is None:
continue
if z_min < max_height:
loc = stage.GetPrimAtPath(body_lists[index]).GetProperty('xformOp:translate').Get()
loc = [loc[0], loc[1], loc[2] - z_min]
set_translate(stage.GetPrimAtPath(body_lists[index]), loc)
def load_human(human_base_prim_path, n, asset_path, dynamic_prims=[], added_prims=[], correct_texture_paths=False):
"""
Load the human based on the usd path and add it to the dynamic prims list
Follow prim naming convention /human_base_prim_path+n
Add also the semantic with the label "human"
human_base_prim_path: the base path to which we add the n of the n-th human as per the prim path
n: the number of the human
asset_path: the path of the ussd of the human
dynamic_prims: the list of dynamic prims in the world. Only the body, and the clothes will be added (not the armature) as separate objects
added_prims: the list of the number of prims added to the world
correct_texture_paths: if True, correct the texture paths to the correct path
"""
stage = omni.usd.get_context().get_stage()
res, _ = omni.kit.commands.execute("CreateReferenceCommand",
usd_context=omni.usd.get_context(),
path_to=f"{human_base_prim_path}{n}",
asset_path=asset_path,
instanceable=False)
cnt = 0
if res:
for child in stage.GetPrimAtPath(f"{human_base_prim_path}{n}").GetAllChildren():
if "armature" in child.GetName().lower():
for sub_child in child.GetAllChildren():
if "armature" not in sub_child.GetName().lower():
dynamic_prims.append(sub_child)
cnt += 1
added_prims.append(cnt)
clear_properties(f"{human_base_prim_path}{n}")
if correct_texture_paths:
print("Correcting texture paths, you might want to change utils/misc_utils.py:correct_paths")
correct_paths(f"{human_base_prim_path}{n}")
else:
print("Not correcting texture paths, you might want to check the textures")
process_semantics(f"{human_base_prim_path}{n}", "human")
else:
raise Exception(f"Failed to load human {n} from {asset_path}")
| 3,553 | Python | 41.309523 | 139 | 0.663102 |
eliabntt/GRADE-RR/simulator/utils/objects_utils.py | import utils.misc_utils
from utils.misc_utils import *
mtl_created_list = []
def setup_shapenet(username, password, csv_location):
global database
shapenet.settings.ShapenetSettings()
if not os.path.exists(csv_location):
logged_in = shapenet.login.save_v1_csvs(username, password, csv_location)
database = shapenet.globals.get_database()
return database
def load_object(rng=np.random.default_rng(), obj_name="shapenet", config=None, scale=1):
if obj_name == "shapenet":
return load_shapenet_object(rng, config, scale)
elif obj_name == "google":
return load_google_obj(rng, config, scale)
def load_shapenet_object(rng=np.random.default_rng(), config=None, scale=1):
"""
It loads a random object from the ShapeNet database
:param rng: a random number generator. If you don't have one, you can use np.random.default_rng()
:param config: a dictionary of parameters that can be set by the user
:param scale: The scale of the object, defaults to 1 (optional)
:return: The path to the object and the synsetId and modelId of the object.
"""
global database
scale /= 100
synsetId = rng.choice(list(database)) if config["synsetId"].get() == "random" else config["synsetId"].get()
modelId = rng.choice(list(database[synsetId])) if config["modelId"].get() == "random" else config["modelId"].get()
_settings = carb.settings.get_settings()
prim = shapenet.shape.addShapePrim(_settings.get("/isaac/shapenet/omniverseServer"), synsetId, modelId,
Gf.Vec3d(0, 0, 0),
Gf.Rotation(Gf.Vec3d(1, 0, 0), 0),
scale, True, True)
if type(prim) == str:
raise Exception(prim)
return str(prim.GetPath()), [synsetId, modelId]
def load_google_obj(rng=np.random.default_rng(), config=None, scale = 1):
"""
It loads a random Google 3D asset from the Google Scanned Object, converts it to USD, and then creates a reference to it
in the current stage
:param rng: a random number generator
:param config: a dictionary of the config file
:return: The prim path of the asset and the name of the asset
"""
google_obj_folder = config['google_obj_folder'].get()
if config['google_obj_shortlist'].get() == "":
asset = rng.choice(os.listdir(google_obj_folder))
else:
with (open(config['google_obj_shortlist'].get(), 'r')) as f:
asset = rng.choice(f.read().splitlines())
if not os.path.exists(f"{google_obj_folder}/exported_usd/{asset}/"):
os.makedirs(f"{google_obj_folder}/exported_usd/{asset}/")
usd_asset_path = f"{google_obj_folder}/exported_usd/{asset}/{asset}.usd"
obj_asset_path = f"{google_obj_folder}/{asset}/meshes/model.obj"
print(f"Converting {obj_asset_path} to {usd_asset_path}")
if not os.path.exists(usd_asset_path):
success = asyncio.new_event_loop().run_until_complete(convert_google_obj(obj_asset_path, usd_asset_path))
if not success:
raise Exception("Failed to convert obj to usd")
stage = omni.usd.get_context().get_stage()
prim_path = str(stage.GetDefaultPrim().GetPath()) + "/" + asset
insta_count = 0
prim_path_len = len(prim_path)
while stage.GetPrimAtPath(prim_path):
insta_count += 1
prim_path = f"{prim_path[:prim_path_len]}_{insta_count}"
omni.kit.commands.execute('CreateReferenceCommand',
usd_context=omni.usd.get_context(),
path_to=prim_path,
asset_path=usd_asset_path,
instanceable=True)
texture_list = os.listdir(f"{google_obj_folder}/{asset}/materials/textures")[0]
# shader = UsdShade.Shader(stage.GetPrimAtPath(f"{prim_path}/Looks/material_0/material_0"))
# shader.CreateInput("diffuse_texture", Sdf.ValueTypeNames.Asset)
# omni.kit.commands.execute('ChangePropertyCommand',
# prop_path=f'{prim_path}/Looks/material_0/material_0.inputs:diffuse_texture',
# value=f"{google_obj_folder}/{asset}/materials/textures/{texture_list}",
# prev=None)
global mtl_created_list
omni.kit.commands.execute(
"CreateAndBindMdlMaterialFromLibrary",
mdl_name="OmniPBR.mdl",
mtl_name=f"{asset}",
mtl_created_list=mtl_created_list,
)
mtl_prim = stage.GetPrimAtPath(mtl_created_list[0])
omni.usd.create_material_input(
mtl_prim,
"diffuse_texture",
"my-computer://" + texture_list, # my-computer seems necessary
Sdf.ValueTypeNames.Asset,
)
obj_shade = UsdShade.Material(mtl_prim)
for child in stage.GetPrimAtPath(prim_path).GetAllChildren():
if child.GetTypeName().lower() == "xform":
for subchild in child.GetAllChildren():
if subchild.GetTypeName().lower() == "mesh":
UsdShade.MaterialBindingAPI(subchild).Bind(obj_shade, UsdShade.Tokens.strongerThanDescendants)
set_scale(stage.GetPrimAtPath(prim_path), scale)
return str(prim_path), asset
async def convert_google_obj(in_path, out_path):
"""
It converts a Google 3D model to a format that can be used in Omni
:param in_path: The path to the file you want to convert
:param out_path: The path to the output file
:return: A boolean value.
"""
import omni.kit.asset_converter as assetimport
context = omni.kit.asset_converter.AssetConverterContext()
converter_manager = omni.kit.asset_converter.get_instance()
context.embed_textures = False
task = converter_manager.create_converter_task(in_path, out_path, None, context)
success = await task.wait_until_finished()
return success
def load_objects(config, environment, rng, dynamic_prims, scale):
"""
Load objects in the environment
Config should contain `config["obstacles"]` with the various considered keys.
In our case those are shapenet and google(scanned_objects)
In config we define the # of objects for each class.
If the import fails the system tries to load it from another class.
For now we do not generate positions that are collision free, so the objects will go through obstacles/humans/camera.
config: the config dictionary
environment: the environment object
rng: the global rng
dynamic_prims: the list of dynamic prims that will be used in the main thread
"""
stage = omni.usd.get_context().get_stage()
shapenet_obs = config["obstacles"]["shapenet"].get()
google_obs = config["obstacles"]["google"].get()
num_obstacles = shapenet_obs + google_obs
loc = ''
google_obs_used = []
shapenet_obs_used = []
meters_per_unit = environment.meters_per_unit
if (num_obstacles > 0):
print("Loading obstacles..")
for n in range(num_obstacles):
print("Loading obstacle {}".format(n))
# set random valid location, use "camera"
x, y, z, yaw = position_object(environment, type=2)
if google_obs > 0:
ob_type = "google"
google_obs -= 1
else:
ob_type = "shapenet"
if loc == '':
loc = shapenet.globals.get_local_shape_loc()
print("Location is {}".format(loc))
csv_location = loc + "/v1_csv/"
database = setup_shapenet(config["shapenet_username"].get(), config["shapenet_password"].get(), csv_location)
if database is None:
print("Error loading database, resort to google")
ob_type = "google"
shapenet_obs -= 1
try:
my_shape, shape_infos = load_object(rng, ob_type, config, scale)
except:
print("Error loading object, try with the other type")
try:
my_shape, shape_infos = load_object(rng, "google" if ob_type == "shapenet" else "shapenet", config, scale)
except:
print("Error loading object, giving up")
continue
google_obs_used.append(shape_infos) if ob_type == "google" else shapenet_obs_used.append(shape_infos)
print(f"{my_shape} loaded.. pose and adding animation")
clear_properties(my_shape)
add_translate_anim(my_shape, Gf.Vec3d(x[0] / meters_per_unit, y[0] / meters_per_unit, z[0] / meters_per_unit))
add_rotation_anim(my_shape,
Gf.Vec3d(rng.uniform(0, 2 * np.pi), rng.uniform(0, 2 * np.pi), rng.uniform(0, 2 * np.pi)))
dynamic_prims.append(stage.GetPrimAtPath(my_shape))
num_keys = rng.choice(range(1, config["experiment_length"].get()), rng.integers(1, 10)).astype(float)
num_keys.sort()
for key in num_keys:
key *= 1
x, y, z, yaw = position_object(environment, type=2)
add_translate_anim(my_shape, Gf.Vec3d(x[0] / meters_per_unit, y[0] / meters_per_unit, z[0] / meters_per_unit),
key)
add_rotation_anim(my_shape, Gf.Vec3d(rng.uniform(0, 360), rng.uniform(0, 360), rng.uniform(0, 360)),
key)
if ob_type == "google":
add_colliders(my_shape)
add_semantics(stage.GetPrimAtPath(my_shape), ob_type)
print("Loading obstacle complete")
return google_obs_used, shapenet_obs_used
| 8,654 | Python | 40.018957 | 121 | 0.681303 |
eliabntt/GRADE-RR/irotate_specific/republish_tf.py | #!/usr/bin/env python
import rospy
import ipdb
import random
from tf2_msgs.msg import TFMessage
import copy
def callback(data, pub):
data_to_pub = TFMessage()
data_to_pub.transforms = copy.copy(data.transforms)
cnt = 0
for i, d in enumerate(data.transforms):
if "x_link" in d.child_frame_id or "y_link" in d.child_frame_id or "yaw_link" in d.child_frame_id or "base_link" in d.child_frame_id or "cameraholder_link" in d.child_frame_id:
data_to_pub.transforms.pop(i - cnt)
cnt += 1
pub.publish(data_to_pub)
return
def listener():
rospy.init_node('tf_republisher')
pub = rospy.Publisher("tf", TFMessage, queue_size=1)
rospy.Subscriber("/tf2", TFMessage, callback, callback_args=(pub))
rospy.spin()
if __name__ == '__main__':
listener()
| 816 | Python | 29.259258 | 187 | 0.650735 |
eliabntt/GRADE-RR/isaac_internals/exts/omni.isaac.synthetic_utils/PACKAGE-LICENSES/omni.isaac.synthetic_utils-LICENSE.md | Copyright (c) 2020, NVIDIA CORPORATION. All rights reserved.
NVIDIA CORPORATION and its licensors retain all intellectual property
and proprietary rights in and to this software, related documentation
and any modifications thereto. Any use, reproduction, disclosure or
distribution of this software and related documentation without an express
license agreement from NVIDIA CORPORATION is strictly prohibited. | 412 | Markdown | 57.999992 | 74 | 0.839806 |
eliabntt/GRADE-RR/isaac_internals/exts/omni.isaac.synthetic_utils/config/extension.toml | [core]
reloadable = true
order = 0
[package]
version = "0.4.3"
category = "Simulation"
title = "Isaac Sim Synthetic Data Utilities"
description = "Utility functions for generating synthetic data"
authors = ["NVIDIA"]
repository = ""
keywords = ["isaac", "synthetic", "utils"]
changelog = "docs/CHANGELOG.md"
readme = "docs/README.md"
icon = "data/icon.png"
writeTarget.kit = true
[dependencies]
"omni.kit.uiapp" = {}
"omni.syntheticdata" = {}
"omni.kit.pip_archive" = {} # pulls in pillow
"omni.isaac.core" = {}
"omni.isaac.core_archive" = {}
[[python.module]]
name = "omni.isaac.synthetic_utils"
[[python.module]]
name = "omni.isaac.synthetic_utils.tests"
[[test]]
dependencies = [
"omni.hydra.rtx", # "omni.hydra.pxr", Can we run and pass with Storm ?
"omni.kit.viewport.utility",
"omni.kit.viewport.window",
"omni.physx",
"omni.kit.primitive.mesh",
"omni.kit.material.library",
] | 934 | TOML | 22.374999 | 96 | 0.6606 |
eliabntt/GRADE-RR/isaac_internals/exts/omni.isaac.synthetic_utils/omni/isaac/synthetic_utils/syntheticdata.py | # Copyright (c) 2020-2021, NVIDIA CORPORATION. All rights reserved.
#
# NVIDIA CORPORATION and its licensors retain all intellectual property
# and proprietary rights in and to this software, related documentation
# and any modifications thereto. Any use, reproduction, disclosure or
# distribution of this software and related documentation without an express
# license agreement from NVIDIA CORPORATION is strictly prohibited.
"""Helper class for obtaining groundtruth data from OmniKit.
Support provided for RGB, Depth, Bounding Box (2D Tight, 2D Loose, 3D),
segmentation (instance and semantic), and camera parameters.
Typical usage example:
kit = OmniKitHelper() # Start omniverse kit
sd_helper = SyntheticDataHelper()
gt = sd_helper.get_groundtruth(['rgb', 'depth', 'boundingBox2DTight'], viewport)
"""
import math
import time
import typing
import asyncio
import carb
import omni
import numpy as np
import builtins
from pxr import Usd
class SyntheticDataHelper:
def __init__(self):
self.app = omni.kit.app.get_app_interface()
ext_manager = self.app.get_extension_manager()
ext_manager.set_extension_enabled("omni.syntheticdata", True)
from omni.syntheticdata import sensors, helpers
import omni.syntheticdata._syntheticdata as sd # Must be imported after getting app interface
self.sd = sd
self.sd_interface = self.sd.acquire_syntheticdata_interface()
self.carb_settings = carb.settings.acquire_settings_interface()
self.sensor_helper_lib = sensors
self.generic_helper_lib = helpers
self.sensor_helpers = {
"rgb": sensors.get_rgb,
"depth": sensors.get_distance_to_image_plane,
"depthLinear": sensors.get_distance_to_camera,
"instanceSegmentation": sensors.get_instance_segmentation,
"semanticSegmentation": sensors.get_semantic_segmentation,
"boundingBox2DTight": sensors.get_bounding_box_2d_tight,
"boundingBox2DLoose": sensors.get_bounding_box_2d_loose,
"boundingBox3D": sensors.get_bounding_box_3d,
"motion-vector": sensors.get_motion_vector,
"normals": sensors.get_normals,
"camera": self.get_camera_params,
"pose": self.get_pose,
"occlusion": sensors.get_occlusion,
}
self.sensor_types = {
"rgb": self.sd.SensorType.Rgb,
"depth": self.sd.SensorType.DistanceToImagePlane,
"depthLinear": self.sd.SensorType.DistanceToCamera,
"instanceSegmentation": self.sd.SensorType.InstanceSegmentation,
"semanticSegmentation": self.sd.SensorType.SemanticSegmentation,
"boundingBox2DTight": self.sd.SensorType.BoundingBox2DTight,
"boundingBox2DLoose": self.sd.SensorType.BoundingBox2DLoose,
"boundingBox3D": self.sd.SensorType.BoundingBox3D,
"occlusion": self.sd.SensorType.Occlusion,
"motion-vector": self.sd.SensorType.MotionVector,
"normals": self.sd.SensorType.Normal,
}
self.sensor_state = {s: False for s in list(self.sensor_helpers.keys())}
def get_camera_params(self, viewport):
"""Get active camera intrinsic and extrinsic parameters.
Returns:
A dict of the active camera's parameters.
pose (numpy.ndarray): camera position in world coordinates,
fov (float): horizontal field of view in radians
focal_length (float)
horizontal_aperture (float)
view_projection_matrix (numpy.ndarray(dtype=float64, shape=(4, 4)))
resolution (dict): resolution as a dict with 'width' and 'height'.
clipping_range (tuple(float, float)): Near and Far clipping values.
"""
stage = omni.usd.get_context().get_stage()
prim = stage.GetPrimAtPath(viewport.get_active_camera())
prim_tf = omni.usd.get_world_transform_matrix(prim)
current_time = omni.timeline.get_timeline_interface().get_current_time()
view_params = self.generic_helper_lib.get_view_params(viewport)
hfov = 2 * math.atan(view_params["horizontal_aperture"] / (2 * view_params["focal_length"]))
vfov = prim.GetAttribute('verticalAperture').Get()
view_proj_mat = self.generic_helper_lib.get_view_proj_mat(view_params)
return {
"pose": np.array(prim_tf),
"hfov": hfov,
"vfov": vfov,
"ctime": current_time,
"focal_length": view_params["focal_length"],
"horizontal_aperture": view_params["horizontal_aperture"],
"view_projection_matrix": view_proj_mat,
"resolution": {"width": view_params["width"], "height": view_params["height"]},
"clipping_range": view_params["clipping_range"],
}
def get_pose(self):
"""Get pose of all objects with a semantic label.
"""
stage = omni.usd.get_context().get_stage()
mappings = self.generic_helper_lib.get_instance_mappings()
pose = []
timeline = omni.timeline.get_timeline_interface()
time = timeline.get_current_time() * timeline.get_time_codes_per_seconds()
time = Usd.TimeCode(time)
for m in mappings:
prim_path = m[1]
prim = stage.GetPrimAtPath(prim_path)
prim_tf = omni.usd.get_world_transform_matrix(prim, time)
pose.append((str(prim_path), m[2], str(m[3]), np.array(prim_tf)))
return pose
def initialize(self, sensor_names, viewport_api):
"""Initialize sensors in the list provided.
Args:
viewport_api (Any): Viewport from which to retrieve/create sensor.
sensor_types (list of omni.syntheticdata._syntheticdata.SensorType): List of sensor types to initialize.
"""
for sensor_name in sensor_names:
if sensor_name != "camera" and sensor_name != "pose":
self.sensor_helper_lib.enable_sensors(viewport_api, [self.sensor_types[sensor_name]])
if builtins.ISAAC_LAUNCHED_FROM_JUPYTER:
data = []
while data == []:
self.app.update()
data = self.sensor_helpers[sensor_name](viewport_api)
else:
future = asyncio.ensure_future(self.sensor_helper_lib.next_sensor_data_async(viewport_api))
while not future.done():
self.app.update()
self.app.update()
async def initialize_async(self, sensor_names, viewport_api):
"""Initialize sensors in the list provided. Async version
Args:
viewport_api (Any): Viewport from which to retrieve/create sensor.
sensor_types (list of omni.syntheticdata._syntheticdata.SensorType): List of sensor types to initialize.
"""
for sensor_name in sensor_names:
if sensor_name != "camera" and sensor_name != "pose":
await self.sensor_helper_lib.initialize_async(viewport_api, [self.sensor_types[sensor_name]])
await self.sensor_helper_lib.next_sensor_data_async(viewport_api)
pass
def get_groundtruth(self, sensor_names, viewport_api, verify_sensor_init=True, wait_for_sensor_data=0.1):
"""Get groundtruth from specified gt_sensors.
Args:
sensor_names (list): List of strings of sensor names. Valid sensors names: rgb, depth,
instanceSegmentation, semanticSegmentation, boundingBox2DTight,
boundingBox2DLoose, boundingBox3D, camera
viewport_api (Any): Viewport from which to retrieve/create sensor.
verify_sensor_init (bool): Additional check to verify creation and initialization of sensors.
wait_for_sensor_data (float): Additional time to sleep before returning ground truth so are correctly filled. Default is 0.1 seconds
Returns:
Dict of sensor outputs
"""
if wait_for_sensor_data > 0:
time.sleep(wait_for_sensor_data)
# Create and initialize sensors
if verify_sensor_init:
loop = asyncio.get_event_loop()
if loop and loop.is_running():
carb.log_warn("Set verify_sensor_init to false if running with asyncio")
pass
else:
self.initialize(sensor_names, viewport_api)
gt = {}
sensor_state = {}
# Process non-RT-only sensors
for sensor in sensor_names:
if sensor not in ["camera", "pose"]:
if sensor == "instanceSegmentation":
gt[sensor] = self.sensor_helpers[sensor](viewport_api, parsed=True, return_mapping=True)
elif sensor == "boundingBox3D":
gt[sensor] = self.sensor_helpers[sensor](viewport_api, parsed=True, return_corners=True)
else:
gt[sensor] = self.sensor_helpers[sensor](viewport_api)
self.sensor_helper_lib.create_or_retrieve_sensor(viewport_api, self.sensor_types[sensor])
# sensors are always initialized after they are created
sensor_state[sensor] = True
elif sensor == "pose":
sensor_state[sensor] = True
gt[sensor] = self.sensor_helpers[sensor]()
else:
sensor_state[sensor] = True
gt[sensor] = self.sensor_helpers[sensor](viewport_api)
gt["state"] = sensor_state
return gt
def get_semantic_ids(self, semantic_data: list = [[]]) -> typing.List[int]:
"""Returns unique id's for a semantic image
Args:
semantic_data (list, optional): Semantic Image. Defaults to [[]].
Returns:
typing.List[int]: List of unique semantic IDs in image
"""
return list(np.unique(semantic_data))
def get_semantic_id_map(self, semantic_labels: list = []) -> dict:
"""
Get map of semantic ID from label
"""
output = {}
if len(semantic_labels) > 0:
for label in semantic_labels:
idx = self.sd_interface.get_semantic_segmentation_id_from_data("class", label)
output[label] = idx
return output
def get_semantic_label_map(self, semantic_ids: list = []) -> dict:
"""
Get map of semantic label from ID
"""
output = {}
if len(semantic_ids) > 0:
for idx in semantic_ids:
label = self.sd_interface.get_semantic_segmentation_data_from_id(idx)
output[idx] = label
return output
def get_mapped_semantic_data(
self, semantic_data: list = [[]], user_semantic_label_map: dict = {}, remap_using_base_class=False
) -> dict:
"""Map semantic segmentation data to IDs specified by user
Usage:
gt = get_groundtruth()
user_semantic_label_map ={"cone":4, "cylinder":5, "cube":6}
mapped_data = get_mapped_semantic_data(gt["semanticSegmentation"], user_semantic_label_map)
Args:
semantic_data (list, optional): Raw semantic image. Defaults to [[]].
user_semantic_label_map (dict, optional): Dictionary of label to id pairs. Defaults to {}.
remap_using_base_class (bool, optional): If multiple class labels are found, use the topmost one. Defaults to False.
Returns:
dict: [description]
"""
semantic_data_np = np.array(semantic_data)
unique_semantic_ids = list(np.unique(semantic_data_np))
unique_semantic_labels_map = self.get_semantic_label_map(unique_semantic_ids)
for unique_id, unique_label in unique_semantic_labels_map.items():
label = unique_label
if remap_using_base_class:
label = unique_label.split(":")[-1]
if label in user_semantic_label_map:
semantic_data_np[np.where(semantic_data == unique_id)] = user_semantic_label_map[label]
return semantic_data_np.tolist()
| 10,852 | Python | 37.214789 | 145 | 0.69084 |
eliabntt/GRADE-RR/isaac_internals/exts/omni.isaac.synthetic_utils/omni/isaac/synthetic_utils/visualization.py | # Copyright (c) 2020-2021, NVIDIA CORPORATION. All rights reserved.
#
# NVIDIA CORPORATION and its licensors retain all intellectual property
# and proprietary rights in and to this software, related documentation
# and any modifications thereto. Any use, reproduction, disclosure or
# distribution of this software and related documentation without an express
# license agreement from NVIDIA CORPORATION is strictly prohibited.
import struct
import random
import colorsys
import numpy as np
from PIL import Image, ImageDraw
def random_colours(N, enable_random=True, num_channels=3):
"""
Generate random colors.
Generate visually distinct colours by linearly spacing the hue
channel in HSV space and then convert to RGB space.
"""
start = 0
if enable_random:
random.seed(10)
start = random.random()
hues = [(start + i / N) % 1.0 for i in range(N)]
colours = [list(colorsys.hsv_to_rgb(h, 0.9, 1.0)) for i, h in enumerate(hues)]
if num_channels == 4:
for color in colours:
color.append(1.0)
if enable_random:
random.shuffle(colours)
return colours
def plot_boxes(ax, bboxes, labels=None, colours=None, label_size=10):
import matplotlib.pyplot as plt
if colours is None:
colours = random_colours(len(bboxes))
if labels is None:
labels = [""] * len(bboxes)
for bb, label, colour in zip(bboxes, labels, colours):
maxint = 2 ** (struct.Struct("i").size * 8 - 1) - 1
# if a bbox is not visible, do not draw
if bb[0] != maxint and bb[1] != maxint:
x = bb[0]
y = bb[1]
w = bb[2] - x
h = bb[3] - y
box = plt.Rectangle((x, y), w, h, fill=False, edgecolor=colour)
ax.add_patch(box)
if label:
font = {"family": "sans-serif", "color": colour, "size": label_size}
ax.text(bb[0], bb[1], label, fontdict=font)
def colorize_depth(depth_image, width, height, num_channels=3):
""" Colorizes depth data for visualization.
Args:
depth_image (numpy.ndarray): Depth data from the sensor.
width (int): Width of the viewport.
height (int): Height of the viewport.
num_channels (int): Specify number of channels i.e. 3 or 4.
"""
colorized_image = np.zeros((height, width, num_channels))
depth_image[depth_image == 0.0] = 1e-5
depth_image = np.clip(depth_image, 0, 255)
depth_image -= np.min(depth_image)
depth_image /= np.max(depth_image) - np.min(depth_image)
colorized_image[:, :, 0] = depth_image
colorized_image[:, :, 1] = depth_image
colorized_image[:, :, 2] = depth_image
if num_channels == 4:
colorized_image[:, :, 3] = 1
colorized_image = (colorized_image * 255).astype(int)
return colorized_image
def colorize_segmentation(segmentation_image, width, height, num_channels=3, num_colors=None):
""" Colorizes segmentation data for visualization.
Args:
segmentation_image (numpy.ndarray): Segmentation data from the sensor.
width (int): Width of the viewport.
height (int): Height of the viewport.
num_channels (int): Specify number of channels i.e. 3 or 4.
num_colors (int): Specify number of colors for consistency across frames.
"""
segmentation_mappings = segmentation_image[:, :, 0]
segmentation_list = np.unique(segmentation_mappings)
if num_colors is None:
num_colors = np.max(segmentation_list) + 1
color_pixels = random_colours(num_colors, True, num_channels)
color_pixels = [[color_pixel[i] * 255 for i in range(num_channels)] for color_pixel in color_pixels]
segmentation_masks = np.zeros((len(segmentation_list), *segmentation_mappings.shape), dtype=np.bool)
index_list = []
for index, segmentation_id in enumerate(segmentation_list):
segmentation_masks[index] = segmentation_mappings == segmentation_id
index_list.append(segmentation_id)
color_image = np.zeros((height, width, num_channels), dtype=np.uint8)
for index, mask, colour in zip(index_list, segmentation_masks, color_pixels):
color_image[mask] = color_pixels[index] if index > 0 else 0
return color_image
def colorize_bboxes(bboxes_2d_data, bboxes_2d_rgb, num_channels=3):
""" Colorizes 2D bounding box data for visualization.
Args:
bboxes_2d_data (numpy.ndarray): 2D bounding box data from the sensor.
bboxes_2d_rgb (numpy.ndarray): RGB data from the sensor to embed bounding box.
num_channels (int): Specify number of channels i.e. 3 or 4.
"""
semantic_id_list = []
bbox_2d_list = []
rgb_img = Image.fromarray(bboxes_2d_rgb)
rgb_img_draw = ImageDraw.Draw(rgb_img)
for bbox_2d in bboxes_2d_data:
if bbox_2d[5] > 0:
semantic_id_list.append(bbox_2d[1])
bbox_2d_list.append(bbox_2d)
semantic_id_list_np = np.unique(np.array(semantic_id_list))
color_list = random_colours(len(semantic_id_list_np.tolist()), True, num_channels)
for bbox_2d in bbox_2d_list:
index = np.where(semantic_id_list_np == bbox_2d[1])[0][0]
bbox_color = color_list[index]
outline = (int(255 * bbox_color[0]), int(255 * bbox_color[1]), int(255 * bbox_color[2]))
if num_channels == 4:
outline = (
int(255 * bbox_color[0]),
int(255 * bbox_color[1]),
int(255 * bbox_color[2]),
int(255 * bbox_color[3]),
)
rgb_img_draw.rectangle([(bbox_2d[6], bbox_2d[7]), (bbox_2d[8], bbox_2d[9])], outline=outline, width=2)
bboxes_2d_rgb = np.array(rgb_img)
return bboxes_2d_rgb
| 5,785 | Python | 39.461538 | 110 | 0.626102 |
eliabntt/GRADE-RR/isaac_internals/exts/omni.isaac.synthetic_utils/omni/isaac/synthetic_utils/tests/test_synthetic_utils.py | # Copyright (c) 2018-2021, NVIDIA CORPORATION. All rights reserved.
#
# NVIDIA CORPORATION and its licensors retain all intellectual property
# and proprietary rights in and to this software, related documentation
# and any modifications thereto. Any use, reproduction, disclosure or
# distribution of this software and related documentation without an express
# license agreement from NVIDIA CORPORATION is strictly prohibited.
#
# NOTE:
# omni.kit.test - std python's unittest module with additional wrapping to add suport for async/await tests
# For most things refer to unittest docs: https://docs.python.org/3/library/unittest.html
from omni.isaac.core.utils.viewports import set_camera_view
import omni.kit.test
import omni.kit.commands
import carb
import carb.tokens
import copy
import os
import asyncio
import numpy as np
from pxr import Gf, UsdGeom, UsdPhysics
import random
# Import extension python module we are testing with absolute import path, as if we are external user (other extension)
from omni.isaac.synthetic_utils import SyntheticDataHelper
from omni.isaac.synthetic_utils.writers import NumpyWriter
from omni.isaac.synthetic_utils.writers import KittiWriter
from omni.syntheticdata.tests.utils import add_semantics
from omni.isaac.core.utils.physics import simulate_async
from omni.isaac.core.utils.nucleus import get_assets_root_path
from omni.isaac.core.utils.semantics import add_update_semantics
from omni.isaac.core.utils.extensions import get_extension_path_from_name
from omni.isaac.core.utils.stage import set_stage_up_axis
from omni.isaac.core import PhysicsContext
from omni.physx.scripts.physicsUtils import add_ground_plane
from omni.kit.viewport.utility import get_active_viewport
# Having a test class dervived from omni.kit.test.AsyncTestCase declared on the root of module will make it auto-discoverable by omni.kit.test
class TestSyntheticUtils(omni.kit.test.AsyncTestCase):
# Before running each test
async def setUp(self):
await omni.usd.get_context().new_stage_async()
await omni.kit.app.get_app().next_update_async()
self._physics_rate = 60
set_stage_up_axis("z")
PhysicsContext(physics_dt=1.0 / self._physics_rate)
self._time_step = 1.0 / self._physics_rate
carb.settings.get_settings().set_int("/app/runLoops/main/rateLimitFrequency", int(self._physics_rate))
carb.settings.get_settings().set_bool("/app/runLoops/main/rateLimitEnabled", True)
carb.settings.get_settings().set_int("/persistent/simulation/minFrameRate", int(self._physics_rate))
carb.settings.get_settings().set("/app/asyncRendering", False)
carb.settings.get_settings().set("/app/hydraEngine/waitIdle", True)
carb.settings.get_settings().set("/rtx/hydra/enableSemanticSchema", True)
await omni.kit.app.get_app().next_update_async()
# Start Simulation and wait
self._timeline = omni.timeline.get_timeline_interface()
self._viewport_api = get_active_viewport()
self._usd_context = omni.usd.get_context()
self._sd_helper = SyntheticDataHelper()
self._synthetic_utils_path = get_extension_path_from_name("omni.isaac.synthetic_utils")
self._stage = self._usd_context.get_stage()
self._camera_path = "/Camera"
camera = self._stage.DefinePrim(self._camera_path, "Camera")
self._viewport_api.set_active_camera(self._camera_path)
pass
# After running each test
async def tearDown(self):
await omni.kit.app.get_app().next_update_async()
self._timeline.stop()
while omni.usd.get_context().get_stage_loading_status()[2] > 0:
print("tearDown, assets still loading, waiting to finish...")
await omni.kit.app.get_app().next_update_async()
await omni.kit.app.get_app().next_update_async()
pass
async def initialize_sensors(self):
# Initialize syntheticdata sensors
await omni.kit.app.get_app().next_update_async()
await self._sd_helper.initialize_async(
[
"rgb",
"depth",
"instanceSegmentation",
"semanticSegmentation",
"boundingBox2DTight",
"boundingBox2DLoose",
"boundingBox3D",
],
self._viewport_api,
)
await omni.kit.app.get_app().next_update_async()
# Acquire a copy of the ground truth.
def get_groundtruth(self):
gt = self._sd_helper.get_groundtruth(
[
"rgb",
"depthLinear",
"boundingBox2DTight",
"boundingBox2DLoose",
"instanceSegmentation",
"semanticSegmentation",
"boundingBox3D",
"camera",
"pose",
],
self._viewport_api,
verify_sensor_init=False,
)
return copy.deepcopy(gt)
async def load_robot_scene(self):
assets_root_path = get_assets_root_path()
if assets_root_path is None:
carb.log_error("Could not find Isaac Sim assets folder")
return
robot_usd = assets_root_path + "/Isaac/Robots/Carter/carter_v1.usd"
add_ground_plane(self._stage, "/physics/groundPlane", "Z", 1000.0, Gf.Vec3f(0.0, 0, -0.25), Gf.Vec3f(1.0))
# setup high-level robot prim
self.prim = self._stage.DefinePrim("/robot", "Xform")
self.prim.GetReferences().AddReference(robot_usd)
add_semantics(self.prim, "robot")
rot_mat = Gf.Matrix3d(Gf.Rotation((0, 0, 1), 90))
omni.kit.commands.execute(
"TransformPrimCommand",
path=self.prim.GetPath(),
old_transform_matrix=None,
new_transform_matrix=Gf.Matrix4d().SetRotate(rot_mat).SetTranslateOnly(Gf.Vec3d(0, -0.64, 0)),
)
# setup scene camera
set_camera_view([3.00, 3.0, 3.00], [0, -0.64, 0], self._camera_path, self._viewport_api)
await self.initialize_sensors()
# Unit test for sensor groundtruth
async def test_groundtruth(self):
await self.load_robot_scene()
self._timeline.play()
await omni.kit.app.get_app().next_update_async()
await simulate_async(1.0)
await omni.syntheticdata.sensors.next_sensor_data_async(self._viewport_api)
gt = self.get_groundtruth()
# Validate Depth groundtruth
gt_depth = gt["depthLinear"]
self.assertAlmostEqual(np.min(gt_depth), 5.11157, delta=0.1)
self.assertAlmostEqual(np.max(gt_depth), 7.4313293, delta=0.1)
# Validate 2D BBox groundtruth
gt_bbox2d = gt["boundingBox2DTight"]
self.assertEqual(len(gt_bbox2d), 1)
self.assertAlmostEqual(gt_bbox2d[0][6], 432, delta=2)
self.assertAlmostEqual(gt_bbox2d[0][7], 138, delta=2)
self.assertAlmostEqual(gt_bbox2d[0][8], 844, delta=2)
self.assertAlmostEqual(gt_bbox2d[0][9], 542, delta=2)
# Validate semantic segmentation groundtruth - 0 (unlabeled) and 1 (robot)
gt_semantic = gt["semanticSegmentation"]
self.assertEqual(len(np.unique(gt_semantic)), 2)
user_semantic_label_map = {"robot": 4, "cylinder": 5, "cube": 6}
mapped_data = self._sd_helper.get_mapped_semantic_data(gt_semantic, user_semantic_label_map, True)
unique_data = np.unique(mapped_data)
self.assertEqual(unique_data[0], 0)
self.assertEqual(unique_data[1], 4)
# Validate 3D BBox groundtruth
gt_bbox3d = gt["boundingBox3D"]
self.assertEqual(len(gt_bbox3d), 1)
self.assertAlmostEqual(gt_bbox3d[0][6], -0.43041847, delta=0.01)
self.assertAlmostEqual(gt_bbox3d[0][7], -0.31312422, delta=0.01)
self.assertAlmostEqual(gt_bbox3d[0][8], -0.25173292, delta=0.01)
self.assertAlmostEqual(gt_bbox3d[0][9], 0.24220554, delta=0.01)
self.assertAlmostEqual(gt_bbox3d[0][10], 0.3131649, delta=0.01)
self.assertAlmostEqual(gt_bbox3d[0][11], 0.4119104, delta=0.01)
# Validate camera groundtruth - position, fov, focal length, aperature
gt_camera = gt["camera"]
gt_camera_trans = gt_camera["pose"][3, :3]
self.assertAlmostEqual(gt_camera_trans[0], 3.000, delta=0.001)
self.assertAlmostEqual(gt_camera_trans[1], 3.000, delta=0.001)
self.assertAlmostEqual(gt_camera_trans[2], 3.000, delta=0.001)
self.assertEqual(gt_camera["resolution"]["width"], 1280)
self.assertEqual(gt_camera["resolution"]["height"], 720)
self.assertAlmostEqual(gt_camera["fov"], 0.4131223226073451, 1e-5)
self.assertAlmostEqual(gt_camera["focal_length"], 50.0, 1e-5)
self.assertAlmostEqual(gt_camera["horizontal_aperture"], 20.954999923706055, 1e-2)
# Validate pose groundtruth - prim path, semantic label, position
gt_pose = gt["pose"]
self.assertEqual(len(gt_pose), 1)
self.assertEqual(gt_pose[0][0], "/robot")
self.assertEqual(gt_pose[0][2], "robot")
gt_pose_trans = (gt_pose[0])[3][3, :3]
self.assertAlmostEqual(gt_pose_trans[0], 0.0, delta=0.001)
self.assertAlmostEqual(gt_pose_trans[1], -0.640, delta=0.001)
self.assertAlmostEqual(gt_pose_trans[2], 0.0, delta=0.001)
pass
# Unit test for data writer
async def test_writer(self):
await self.load_robot_scene()
self._timeline.play()
await omni.kit.app.get_app().next_update_async()
await simulate_async(1.0)
await omni.kit.app.get_app().next_update_async()
viewport_window = omni.kit.viewport.utility.get_active_viewport_window()
# Setting up config for writer
sensor_settings = {}
sensor_settings_viewport = {"rgb": {"enabled": True}}
viewport_name = viewport_window.title
sensor_settings[viewport_name] = copy.deepcopy(sensor_settings_viewport)
# Initialize data writer
output_folder = os.getcwd() + "/output"
data_writer = NumpyWriter(output_folder, 4, 100, sensor_settings)
data_writer.start_threads()
# Get rgb groundtruth
gt = self._sd_helper.get_groundtruth(["rgb"], self._viewport_api, verify_sensor_init=False)
# Write rgb groundtruth
image_id = 1
groundtruth = {"METADATA": {"image_id": str(image_id), "viewport_name": viewport_name}, "DATA": {}}
groundtruth["DATA"]["RGB"] = gt["rgb"]
data_writer.q.put(groundtruth)
# Validate output file
output_file_path = os.path.join(output_folder, viewport_name, "rgb", str(image_id) + ".png")
data_writer.stop_threads()
await asyncio.sleep(0.1)
self.assertEqual(os.path.isfile(output_file_path), True)
pass
# Unit test for data writer
async def test_kitti_writer(self):
await self.load_robot_scene()
self._timeline.play()
await omni.kit.app.get_app().next_update_async()
await simulate_async(1.0)
await omni.kit.app.get_app().next_update_async()
viewport_window = omni.kit.viewport.utility.get_active_viewport_window()
# Setting up config for writer
sensor_settings = {}
sensor_settings_viewport = {"rgb": {"enabled": True}}
viewport_name = viewport_window.title
sensor_settings[viewport_name] = copy.deepcopy(sensor_settings_viewport)
# Initialize data writer
output_folder_tight = os.getcwd() + "/kitti_tight"
output_folder_loose = os.getcwd() + "/kitti_loose"
data_writer_tight = KittiWriter(
output_folder_tight, 4, 100, train_size=1, classes="robot", bbox_type="BBOX2DTIGHT"
)
data_writer_tight.start_threads()
data_writer_loose = KittiWriter(
output_folder_loose, 4, 100, train_size=1, classes="robot", bbox_type="BBOX2DLOOSE"
)
data_writer_loose.start_threads()
# Get rgb groundtruth
gt = self._sd_helper.get_groundtruth(
["rgb", "boundingBox2DTight", "boundingBox2DLoose"], self._viewport_api, verify_sensor_init=False
)
# Write rgb groundtruth
image_id = 0
groundtruth = {
"METADATA": {
"image_id": str(image_id),
"viewport_name": viewport_name,
"BBOX2DTIGHT": {},
"BBOX2DLOOSE": {},
},
"DATA": {},
}
image = gt["rgb"]
groundtruth["DATA"]["RGB"] = image
groundtruth["DATA"]["BBOX2DTIGHT"] = gt["boundingBox2DTight"]
groundtruth["METADATA"]["BBOX2DTIGHT"]["WIDTH"] = image.shape[1]
groundtruth["METADATA"]["BBOX2DTIGHT"]["HEIGHT"] = image.shape[0]
groundtruth["DATA"]["BBOX2DLOOSE"] = gt["boundingBox2DLoose"]
groundtruth["METADATA"]["BBOX2DLOOSE"]["WIDTH"] = image.shape[1]
groundtruth["METADATA"]["BBOX2DLOOSE"]["HEIGHT"] = image.shape[0]
for f in range(2):
groundtruth["METADATA"]["image_id"] = image_id
data_writer_tight.q.put(copy.deepcopy(groundtruth))
data_writer_loose.q.put(copy.deepcopy(groundtruth))
image_id = image_id + 1
# Validate output file
data_writer_tight.stop_threads()
data_writer_loose.stop_threads()
await asyncio.sleep(0.1)
for output_folder in [output_folder_tight, output_folder_loose]:
self.assertEqual(os.path.isfile(os.path.join(output_folder + "/training/image_2", str(0) + ".png")), True)
self.assertEqual(os.path.isfile(os.path.join(output_folder + "/training/label_2", str(0) + ".txt")), True)
self.assertEqual(os.path.isfile(os.path.join(output_folder + "/testing/image_2", str(1) + ".png")), True)
pass
# create a cube.
async def add_cube(self, path, size, offset):
cubeGeom = UsdGeom.Cube.Define(self._stage, path)
cubePrim = self._stage.GetPrimAtPath(path)
# use add_semantics to set its class to Cube
add_semantics(cubePrim, "cube")
cubeGeom.CreateSizeAttr(size)
cubeGeom.ClearXformOpOrder()
cubeGeom.AddTranslateOp().Set(offset)
await omni.kit.app.get_app().next_update_async()
UsdPhysics.CollisionAPI.Apply(cubePrim)
return cubePrim, cubeGeom
# create a scene with a cube.
async def load_cube_scene(self):
# ensure we are done with all of scene setup.
await omni.kit.app.get_app().next_update_async()
# check units
meters_per_unit = UsdGeom.GetStageMetersPerUnit(self._stage)
add_ground_plane(self._stage, "/physics/groundPlane", "Z", 1000.0, Gf.Vec3f(0.0, 0, -25), Gf.Vec3f(1.0))
# Add a cube at a "close" location
self.cube_location = Gf.Vec3f(-300.0, 0.0, 50.0)
self.cube, self.cube_geom = await self.add_cube("/World/Cube", 100.0, self.cube_location)
# setup scene camera
set_camera_view([1000, 1000, 1000], [0, 0, 0], self._camera_path, self._viewport_api)
await self.initialize_sensors()
# Unit test for sensor groundtruth
async def frame_lag_test(self, move):
# start the scene
# wait for update
move(Gf.Vec3f(random.random() * 100, random.random() * 100, random.random() * 100))
await omni.syntheticdata.sensors.next_sensor_data_async(self._viewport_api)
# grab ground truth
gt1 = self.get_groundtruth()
# move the cube
move(Gf.Vec3f(random.random() * 100, random.random() * 100, random.random() * 100))
# wait for update
await omni.syntheticdata.sensors.next_sensor_data_async(self._viewport_api)
# grab ground truth
gt2 = self.get_groundtruth()
await omni.syntheticdata.sensors.next_sensor_data_async(self._viewport_api)
gt3 = self.get_groundtruth()
# ensure segmentation is identical
gt_seg1 = gt1["semanticSegmentation"]
gt_seg2 = gt2["semanticSegmentation"]
self.assertEqual(len(np.unique(gt_seg1)), len(np.unique(gt_seg2)))
# the cube 3d bboxes should be different after update
gt_box3d1 = gt1["boundingBox3D"]
gt_box3d2 = gt2["boundingBox3D"]
gt_box3d3 = gt3["boundingBox3D"]
# check the list size
self.assertEqual(len(gt_box3d1), len(gt_box3d2))
# check the corners, they should/must move to pass the test.
self.assertNotEqual(gt_box3d1["corners"].tolist(), gt_box3d2["corners"].tolist())
# Should be no change between these two frames
self.assertEqual(gt_box3d2["corners"].tolist(), gt_box3d3["corners"].tolist())
await omni.syntheticdata.sensors.next_sensor_data_async(self._viewport_api)
# stop the scene
pass
# Test lag by executing a command
async def test_oneframelag_kitcommand(self):
await self.load_cube_scene()
def set_prim_pose(location):
omni.kit.commands.execute(
"TransformPrimCommand",
path=self.cube.GetPath(),
old_transform_matrix=None,
new_transform_matrix=Gf.Matrix4d()
.SetRotate(Gf.Matrix3d(Gf.Rotation((0, 0, 1), 90)))
.SetTranslateOnly(Gf.Vec3d(location)),
)
for frame in range(50):
await self.frame_lag_test(set_prim_pose)
pass
# Test lag using a USD prim.
async def test_oneframelag_usdprim(self):
await self.load_cube_scene()
def set_prim_pose(location):
properties = self.cube.GetPropertyNames()
if "xformOp:translate" in properties:
translate_attr = self.cube.GetAttribute("xformOp:translate")
translate_attr.Set(location)
for frame in range(50):
await self.frame_lag_test(set_prim_pose)
pass
async def test_remap_semantics(self):
set_camera_view([1000, 1000, 1000], [0, 0, 0], self._camera_path, self._viewport_api)
usd_path = self._synthetic_utils_path + "/data/usd/tests/nested_semantics.usd"
self.prim = self._stage.DefinePrim("/test_nested", "Xform")
self.prim.GetReferences().AddReference(usd_path)
await omni.kit.app.get_app().next_update_async()
await self.initialize_sensors()
gt = self.get_groundtruth()
ids = self._sd_helper.get_semantic_ids(gt["semanticSegmentation"])
labels = self._sd_helper.get_semantic_label_map(ids)
# make sure remapping with remap_using_base_class True should work even if we don't have nested classes
mapped_id_a = self._sd_helper.get_semantic_ids(
self._sd_helper.get_mapped_semantic_data(
gt["semanticSegmentation"], {"red": 1, "green": 10, "blue": 100}, remap_using_base_class=True
)
)
mapped_id_b = self._sd_helper.get_semantic_ids(
self._sd_helper.get_mapped_semantic_data(
gt["semanticSegmentation"], {"red": 1, "green": 10, "blue": 100}, remap_using_base_class=False
)
)
# if labels aren't nested, they should remain the same
unique_data_a = np.unique(mapped_id_a).tolist()
unique_data_b = np.unique(mapped_id_b).tolist()
self.assertListEqual(unique_data_a, unique_data_b)
self.assertEqual(unique_data_a[0], 0)
self.assertEqual(unique_data_a[1], 1)
self.assertEqual(unique_data_a[2], 10)
self.assertEqual(unique_data_a[3], 100)
async def test_nested_semantics(self):
set_camera_view([1000, 1000, 1000], [0, 0, 0], self._camera_path, self._viewport_api)
usd_path = self._synthetic_utils_path + "/data/usd/tests/nested_semantics.usd"
self.prim = self._stage.DefinePrim("/test_nested", "Xform")
add_update_semantics(self.prim, "combined")
self.prim.GetReferences().AddReference(usd_path)
await omni.kit.app.get_app().next_update_async()
await self.initialize_sensors()
gt = self.get_groundtruth()
ids = self._sd_helper.get_semantic_ids(gt["semanticSegmentation"])
labels = self._sd_helper.get_semantic_label_map(ids)
mapped_id_a = self._sd_helper.get_semantic_ids(
self._sd_helper.get_mapped_semantic_data(
gt["semanticSegmentation"], {"combined": 99}, remap_using_base_class=True
)
)
mapped_id_b = self._sd_helper.get_semantic_ids(
self._sd_helper.get_mapped_semantic_data(
gt["semanticSegmentation"], {"combined": 99}, remap_using_base_class=False
)
)
unique_data_a = np.unique(mapped_id_a).tolist()
unique_data_b = np.unique(mapped_id_b).tolist()
self.assertEqual(unique_data_a[0], 0)
self.assertEqual(unique_data_a[1], 99)
# remap_using_base_class false should result in the mapping not changing
self.assertEqual(unique_data_b[0], 0)
self.assertEqual(unique_data_b[1], 1)
self.assertEqual(unique_data_b[2], 2)
self.assertEqual(unique_data_b[3], 3)
| 21,136 | Python | 43.876858 | 142 | 0.629731 |
eliabntt/GRADE-RR/isaac_internals/exts/omni.isaac.synthetic_utils/omni/isaac/synthetic_utils/writers/numpy.py | # Copyright (c) 2020-2021, NVIDIA CORPORATION. All rights reserved.
#
# NVIDIA CORPORATION and its licensors retain all intellectual property
# and proprietary rights in and to this software, related documentation
# and any modifications thereto. Any use, reproduction, disclosure or
# distribution of this software and related documentation without an express
# license agreement from NVIDIA CORPORATION is strictly prohibited.
"""Helper class for writing groundtruth data offline in numpy format.
"""
import copy
import omni
import os
import numpy as np
from PIL import Image
from .base import BaseWriter
from omni.isaac.core.utils.viewports import get_viewport_names
class NumpyWriter(BaseWriter):
def __init__(self, data_dir, num_worker_threads, max_queue_size=500, sensor_settings=None):
BaseWriter.__init__(self, data_dir, num_worker_threads, max_queue_size)
from omni.isaac.synthetic_utils import visualization
self.visualization = visualization
self.create_output_folders(sensor_settings)
def worker(self):
"""Processes task from queue. Each tasks contains groundtruth data and metadata which is used to transform the output and write it to disk."""
while True:
groundtruth = self.q.get()
if groundtruth is None:
break
filename = groundtruth["METADATA"]["image_id"]
viewport_name = groundtruth["METADATA"]["viewport_name"]
for gt_type, data in groundtruth["DATA"].items():
if gt_type == "RGB":
self.save_image(viewport_name, gt_type, data, filename)
elif gt_type == "DEPTH":
if groundtruth["METADATA"]["DEPTH"]["NPY"]:
self.depth_folder = self.data_dir + "/" + str(viewport_name) + "/depth/"
np.save(self.depth_folder + filename + ".npy", data)
if groundtruth["METADATA"]["DEPTH"]["COLORIZE"]:
self.save_image(viewport_name, gt_type, data, filename)
elif gt_type == "DEPTHLINEAR":
if groundtruth["METADATA"]["DEPTHLINEAR"]["NPY"]:
self.depthLinear_folder = self.data_dir + "/" + str(viewport_name) + "/depthLinear/"
np.save(self.depthLinear_folder + filename + ".npy", data)
if groundtruth["METADATA"]["DEPTHLINEAR"]["COLORIZE"]:
self.save_image(viewport_name, gt_type, data, filename)
elif gt_type == "INSTANCE":
self.save_segmentation(
viewport_name,
gt_type,
data,
filename,
groundtruth["METADATA"]["INSTANCE"]["WIDTH"],
groundtruth["METADATA"]["INSTANCE"]["HEIGHT"],
groundtruth["METADATA"]["INSTANCE"]["COLORIZE"],
groundtruth["METADATA"]["INSTANCE"]["MAPPINGS"],
groundtruth["METADATA"]["INSTANCE"]["NPY"],
)
elif gt_type == "SEMANTIC":
self.save_segmentation(
viewport_name,
gt_type,
data,
filename,
groundtruth["METADATA"]["SEMANTIC"]["WIDTH"],
groundtruth["METADATA"]["SEMANTIC"]["HEIGHT"],
groundtruth["METADATA"]["SEMANTIC"]["COLORIZE"],
groundtruth["METADATA"]["SEMANTIC"]["MAPPINGS"],
groundtruth["METADATA"]["SEMANTIC"]["NPY"],
)
elif gt_type in ["BBOX2DTIGHT", "BBOX2DLOOSE"]:
self.save_bbox(
viewport_name,
gt_type,
data,
filename,
groundtruth["METADATA"][gt_type]["COLORIZE"],
groundtruth["DATA"]["RGB"],
groundtruth["METADATA"][gt_type]["NPY"],
)
elif gt_type in ["BBOX3D"]:
self.save_bbox(
viewport_name,
gt_type,
data,
filename,
groundtruth["METADATA"][gt_type]["COLORIZE"],
groundtruth["METADATA"]["BBOX3D_IMAGE"],
groundtruth["METADATA"][gt_type]["NPY"],
)
elif gt_type in ["MOTIONVECTOR"]:
self.save_motion(
viewport_name,
gt_type,
data,
filename,
groundtruth["METADATA"][gt_type]["COLORIZE"],
groundtruth["DATA"]["RGB"],
groundtruth["METADATA"][gt_type]["NPY"],
)
elif gt_type == "CAMERA":
self.camera_folder = self.data_dir + "/" + str(viewport_name) + "/camera/"
np.save(self.camera_folder + filename + ".npy", data)
elif gt_type == "POSES":
self.poses_folder = self.data_dir + "/" + str(viewport_name) + "/poses/"
np.save(self.poses_folder + filename + ".npy", data)
elif gt_type == "NORMALS":
self.normals_folder = self.data_dir + "/" + str(viewport_name) + "/normals/"
np.save(self.normals_folder + filename + ".npy", data)
else:
raise NotImplementedError
self.q.task_done()
def save_motion(
self, viewport_name, data_type, data, filename, width=1280, height=720, display_rgb=True, save_npy=True
):
self.motion_folder = self.data_dir + "/" + str(viewport_name) + "/motion-vector/"
if save_npy:
np.save(self.motion_folder + filename + ".npy", data)
def save_segmentation(
self, viewport_name, data_type, data, filename, width=1280, height=720, display_rgb=True, mappings=True,
save_npy=True):
self.instance_folder = self.data_dir + "/" + str(viewport_name) + "/instance/"
self.semantic_folder = self.data_dir + "/" + str(viewport_name) + "/semantic/"
# Save ground truth data locally as npy
if not mappings:
data = data[0]
if data_type == "INSTANCE" and save_npy:
np.save(self.instance_folder + filename + ".npy", data)
if data_type == "SEMANTIC" and save_npy:
np.save(self.semantic_folder + filename + ".npy", data)
if mappings:
data = data[0]
if display_rgb:
image_data = np.frombuffer(data, dtype=np.uint8).reshape(*data.shape, -1)
num_colors = 50 if data_type == "SEMANTIC" else None
color_image = self.visualization.colorize_segmentation(image_data, width, height, 3, num_colors)
# color_image = visualize.colorize_instance(image_data)
color_image_rgb = Image.fromarray(color_image, "RGB")
if data_type == "INSTANCE":
color_image_rgb.save(f"{self.instance_folder}/{filename}.png")
if data_type == "SEMANTIC":
color_image_rgb.save(f"{self.semantic_folder}/{filename}.png")
def save_image(self, viewport_name, img_type, image_data, filename):
self.rgb_folder = self.data_dir + "/" + str(viewport_name) + "/rgb/"
self.depth_folder = self.data_dir + "/" + str(viewport_name) + "/depth/"
self.depthLinear_folder = self.data_dir + "/" + str(viewport_name) + "/depthLinear/"
if img_type == "RGB":
# Save ground truth data locally as png
rgb_img = Image.fromarray(image_data, "RGBA")
rgb_img.save(f"{self.rgb_folder}/{filename}.png")
elif img_type == "DEPTH" or img_type == "DEPTHLINEAR":
# Convert linear depth to inverse depth for better visualization
image_data = image_data * 100
# Save ground truth data locally as png
image_data[image_data == 0.0] = 1e-5
image_data = np.clip(image_data, 0, 255)
image_data -= np.min(image_data)
if np.max(image_data) > 0:
image_data /= np.max(image_data)
depth_img = Image.fromarray((image_data * 255.0).astype(np.uint8))
if img_type == "DEPTH":
depth_img.save(f"{self.depth_folder}/{filename}.png")
if img_type == "DEPTHLINEAR":
depth_img.save(f"{self.depthLinear_folder}/{filename}.png")
def save_bbox(self, viewport_name, data_type, data, filename, display_rgb=True, rgb_data=None, save_npy=True):
self.bbox_2d_tight_folder = self.data_dir + "/" + str(viewport_name) + "/bbox_2d_tight/"
self.bbox_2d_loose_folder = self.data_dir + "/" + str(viewport_name) + "/bbox_2d_loose/"
self.bbox_3d_folder = self.data_dir + "/" + str(viewport_name) + "/bbox_3d/"
# Save ground truth data locally as npy
if data_type == "BBOX2DTIGHT" and save_npy:
np.save(self.bbox_2d_tight_folder + filename + ".npy", data)
if data_type == "BBOX2DLOOSE" and save_npy:
np.save(self.bbox_2d_loose_folder + filename + ".npy", data)
if data_type == "BBOX3D" and save_npy:
np.save(self.bbox_3d_folder + filename + ".npy", data)
if display_rgb and rgb_data is not None:
if "2D" in data_type:
color_image = self.visualization.colorize_bboxes(data, rgb_data)
color_image_rgb = Image.fromarray(color_image, "RGBA")
if data_type == "BBOX2DTIGHT":
color_image_rgb.save(f"{self.bbox_2d_tight_folder}/{filename}.png")
if data_type == "BBOX2DLOOSE":
color_image_rgb.save(f"{self.bbox_2d_loose_folder}/{filename}.png")
if "3D" in data_type:
rgb_img = Image.fromarray(rgb_data, "RGBA")
rgb_img.save(f"{self.bbox_3d_folder}/{filename}.png")
def create_output_folders(self, sensor_settings=None):
"""Checks if the sensor output folder corresponding to each viewport is created. If not, it creates them."""
if not os.path.exists(self.data_dir):
os.mkdir(self.data_dir)
if sensor_settings is None:
sensor_settings = dict()
viewport_names = get_viewport_names()
sensor_settings_viewport = {
"rgb": {"enabled": True},
"depth": {"enabled": True, "colorize": True, "npy": True},
"depthLinear": {"enabled": True, "colorize": True, "npy": True},
"instance": {"enabled": True, "colorize": True, "npy": True},
"semantic": {"enabled": True, "colorize": True, "npy": True},
"bbox_2d_tight": {"enabled": True, "colorize": True, "npy": True},
"bbox_2d_loose": {"enabled": True, "colorize": True, "npy": True},
"camera": {"enabled": True, "npy": True},
"poses": {"enabled": True, "npy": True},
"motion-vector": {"enabled": True, "npy": True, "colorize": True},
"bbox_3d": {"enabled": True, "npy": True, "colorize": True},
"normals": {"enabled": True, "npy": True, "colorize": True},
}
for name in viewport_names:
sensor_settings[name] = copy.deepcopy(sensor_settings_viewport)
for viewport_name in sensor_settings:
viewport_folder = self.data_dir + "/" + str(viewport_name)
if not os.path.exists(viewport_folder):
os.mkdir(viewport_folder)
for sensor_name in sensor_settings[viewport_name]:
if sensor_settings[viewport_name][sensor_name]["enabled"]:
sensor_folder = self.data_dir + "/" + str(viewport_name) + "/" + str(sensor_name)
if not os.path.exists(sensor_folder):
os.mkdir(sensor_folder)
| 12,152 | Python | 51.83913 | 150 | 0.534398 |
eliabntt/GRADE-RR/isaac_internals/exts/omni.isaac.synthetic_utils/omni/isaac/synthetic_utils/writers/base.py | # Copyright (c) 2020-2021, NVIDIA CORPORATION. All rights reserved.
#
# NVIDIA CORPORATION and its licensors retain all intellectual property
# and proprietary rights in and to this software, related documentation
# and any modifications thereto. Any use, reproduction, disclosure or
# distribution of this software and related documentation without an express
# license agreement from NVIDIA CORPORATION is strictly prohibited.
"""Base class for writing groundtruth data offline.
"""
import atexit
import queue
import threading
class BaseWriter:
def __init__(self, data_dir, num_worker_threads, max_queue_size=500):
atexit.register(self.stop_threads)
# Threading for multiple scenes
self.num_worker_threads = num_worker_threads
# Initialize queue with a specified size
self.q = queue.Queue(max_queue_size)
self.data_dir = data_dir
self.threads = []
def start_threads(self):
"""Start worker threads."""
for _ in range(self.num_worker_threads):
t = threading.Thread(target=self.worker, daemon=True)
t.start()
self.threads.append(t)
def stop_threads(self):
"""Waits for all tasks to be completed before stopping worker threads."""
print("Finish writing data...")
# Block until all tasks are done
self.q.join()
print("Done.")
def worker(self):
"""Processes task from queue. Each tasks contains groundtruth data and metadata which is used to transform the output and write it to disk."""
pass
| 1,581 | Python | 32.659574 | 150 | 0.679317 |
Subsets and Splits
No community queries yet
The top public SQL queries from the community will appear here once available.