repo_id
stringlengths 15
86
| file_path
stringlengths 28
180
| content
stringlengths 1
1.75M
| __index_level_0__
int64 0
0
|
---|---|---|---|
hf_public_repos/transformers/docs/source | hf_public_repos/transformers/docs/source/ja/accelerate.md | <!--Copyright 2022 The HuggingFace Team. All rights reserved.
Licensed under the Apache License, Version 2.0 (the "License"); you may not use this file except in compliance with
the License. You may obtain a copy of the License at
http://www.apache.org/licenses/LICENSE-2.0
Unless required by applicable law or agreed to in writing, software distributed under the License is distributed on
an "AS IS" BASIS, WITHOUT WARRANTIES OR CONDITIONS OF ANY KIND, either express or implied. See the License for the
specific language governing permissions and limitations under the License.
โ ๏ธ Note that this file is in Markdown but contain specific syntax for our doc-builder (similar to MDX) that may not be
rendered properly in your Markdown viewer.
-->
# ๐ค Accelerate ใ็จใใๅๆฃๅญฆ็ฟ
ใขใใซใๅคงใใใชใใซใคใใฆใ้ใใใใใผใใฆใงใขใงใใๅคงใใชใขใใซใ่จ็ทดใใ่จ็ทด้ๅบฆใๅคงๅน
ใซไธๆใใใใใใฎๆนๆณใจใใฆไธฆๅๅฆ็ใๆตฎไธใใฆใใพใใใ1ๅฐใฎใใทใณใซ่คๆฐใฎGPUใใใฃใฆใใ่คๆฐใฎใใทใณใซใพใใใ่คๆฐใฎGPUใใใฃใฆใใใใใใใฟใคใใฎๅๆฃๅฆ็ใปใใใขใใไธใงใฆใผใถใผใ็ฐกๅใซ ๐ค Transformers ใขใใซใ่จ็ทดใงใใใใใซใ Hugging Face ใงใฏ [๐ค Accelerate](https://huggingface.co/docs/accelerate) ใฉใคใใฉใชใไฝๆใใพใใใใใฎใใฅใผใใชใขใซใงใฏใPyTorch ใฎ่จ็ทดใซใผใใใซในใฟใใคใบใใฆใๅๆฃๅฆ็็ฐๅขใงใฎ่จ็ทดใๅฏ่ฝใซใใๆนๆณใซใคใใฆๅญฆใณใพใใ
## ใปใใใขใใ
ใฏใใใซ ๐ค Accelerate ใใคใณในใใผใซใใพใใใ:
```bash
pip install accelerate
```
ใใใใใคใณใใผใใใฆ [`~accelerate.Accelerator`] ใชใใธใงใฏใใไฝๆใใพใใใใ[`~accelerate.Accelerator`] ใฏๅๆฃๅฆ็ใปใใใขใใใ่ชๅ็ใซๆคๅบใใ่จ็ทดใฎใใใซๅฟ
่ฆใชๅ
จใฆใฎใณใณใใผใใณใใๅๆๅใใพใใใขใใซใใใใคในใซๆ็คบ็ใซ้
็ฝฎใใๅฟ
่ฆใฏใใใพใใใ
```py
>>> from accelerate import Accelerator
>>> accelerator = Accelerator()
```
## Accelerate ใใๆบๅใใใพใใใ
ๆฌกใซใ้ข้ฃใใๅ
จใฆใฎ่จ็ทดใชใใธใงใฏใใ [`~accelerate.Accelerator.prepare`] ใกใฝใใใซๆธกใใพใใใใใซใฏใ่จ็ทดใจ่ฉไพกใใใใใฎDataloaderใใขใใซใoptimizer ใๅซใพใใพใ:
```py
>>> train_dataloader, eval_dataloader, model, optimizer = accelerator.prepare(
... train_dataloader, eval_dataloader, model, optimizer
... )
```
## Backward
ๆๅพใซ่จ็ทดใซใผใๅ
ใฎ `loss.backward()` ใ ๐ค Accelerate ใฎ [`~accelerate.Accelerator.backward`] ใกใฝใใใง็ฝฎใๆใใพใ๏ผ
```py
>>> for epoch in range(num_epochs):
... for batch in train_dataloader:
... outputs = model(**batch)
... loss = outputs.loss
... accelerator.backward(loss)
... optimizer.step()
... lr_scheduler.step()
... optimizer.zero_grad()
... progress_bar.update(1)
```
ไปฅไธใฎใณใผใใง็ขบ่ชใงใใ้ใใ่จ็ทดใซใผใใซ4่กใฎใณใผใใ่ฟฝๅ ใใใ ใใงๅๆฃๅญฆ็ฟใๅฏ่ฝใงใ๏ผ
```diff
+ from accelerate import Accelerator
from transformers import AdamW, AutoModelForSequenceClassification, get_scheduler
+ accelerator = Accelerator()
model = AutoModelForSequenceClassification.from_pretrained(checkpoint, num_labels=2)
optimizer = AdamW(model.parameters(), lr=3e-5)
- device = torch.device("cuda") if torch.cuda.is_available() else torch.device("cpu")
- model.to(device)
+ train_dataloader, eval_dataloader, model, optimizer = accelerator.prepare(
+ train_dataloader, eval_dataloader, model, optimizer
+ )
num_epochs = 3
num_training_steps = num_epochs * len(train_dataloader)
lr_scheduler = get_scheduler(
"linear",
optimizer=optimizer,
num_warmup_steps=0,
num_training_steps=num_training_steps
)
progress_bar = tqdm(range(num_training_steps))
model.train()
for epoch in range(num_epochs):
for batch in train_dataloader:
- batch = {k: v.to(device) for k, v in batch.items()}
outputs = model(**batch)
loss = outputs.loss
- loss.backward()
+ accelerator.backward(loss)
optimizer.step()
lr_scheduler.step()
optimizer.zero_grad()
progress_bar.update(1)
```
## ่จ็ทดใใ
้ข้ฃใใใณใผใใ่ฟฝๅ ใใใใในใฏใชใใใพใใฏ Colaboratory ใชใฉใฎใใผใใใใฏใง่จ็ทดใ้ๅงใใพใใ
### ในใฏใชใใใง่จ็ทดใใ
ในใฏใชใใใใ่จ็ทดใใใฆใใๅ ดๅใฏใ่จญๅฎใใกใคใซใไฝๆใปไฟๅญใใใใใซไปฅไธใฎใณใใณใใๅฎ่กใใฆใใ ใใ:
```bash
accelerate config
```
ใใใฆๆฌกใฎใใใซใใฆ่จ็ทดใ้ๅงใใพใ:
```bash
accelerate launch train.py
```
### ใใผใใใใฏใง่จ็ทดใใ
Colaboratory ใฎ TPU ใฎๅฉ็จใใ่ใใฎๅ ดๅใ๐ค Accelerate ใฏใใผใใใใฏไธใงๅฎ่กใใใใจใใงใใพใใ่จ็ทดใซๅฟ
่ฆใชๅ
จใฆใฎใณใผใใ้ขๆฐใซๅซใใ[`~accelerate.notebook_launcher`] ใซๆธกใใฆใใ ใใ:
```py
>>> from accelerate import notebook_launcher
>>> notebook_launcher(training_function)
```
๐ค Accelerate ใจ่ฑๅฏใชๆฉ่ฝใซใคใใฆใใฃใจ็ฅใใใๆนใฏ[ใใญใฅใกใณใ](https://huggingface.co/docs/accelerate)ใๅ็
งใใฆใใ ใใใ
| 0 |
hf_public_repos/transformers/docs/source | hf_public_repos/transformers/docs/source/ko/autoclass_tutorial.md | <!--Copyright 2022 The HuggingFace Team. All rights reserved.
Licensed under the Apache License, Version 2.0 (the "License"); you may not use this file except in compliance with
the License. You may obtain a copy of the License at
http://www.apache.org/licenses/LICENSE-2.0
Unless required by applicable law or agreed to in writing, software distributed under the License is distributed on
an "AS IS" BASIS, WITHOUT WARRANTIES OR CONDITIONS OF ANY KIND, either express or implied. See the License for the
specific language governing permissions and limitations under the License.
โ ๏ธ Note that this file is in Markdown but contain specific syntax for our doc-builder (similar to MDX) that may not be
rendered properly in your Markdown viewer.
-->
# AutoClass๋ก ์ฌ์ ํ์ต๋ ์ธ์คํด์ค ๋ก๋[[load-pretrained-instances-with-an-autoclass]]
ํธ๋์คํฌ๋จธ ์ํคํ
์ฒ๊ฐ ๋งค์ฐ ๋ค์ํ๊ธฐ ๋๋ฌธ์ ์ฒดํฌํฌ์ธํธ์ ๋ง๋ ์ํคํ
์ฒ๋ฅผ ์์ฑํ๋ ๊ฒ์ด ์ด๋ ค์ธ ์ ์์ต๋๋ค. ๋ผ์ด๋ธ๋ฌ๋ฆฌ๋ฅผ ์ฝ๊ณ ๊ฐ๋จํ๋ฉฐ ์ ์ฐํ๊ฒ ์ฌ์ฉํ๊ธฐ ์ํ Transformer ํต์ฌ ์ฒ ํ์ ์ผํ์ผ๋ก, `AutoClass`๋ ์ฃผ์ด์ง ์ฒดํฌํฌ์ธํธ์์ ์ฌ๋ฐ๋ฅธ ์ํคํ
์ฒ๋ฅผ ์๋์ผ๋ก ์ถ๋ก ํ์ฌ ๋ก๋ํฉ๋๋ค. `from_pretrained()` ๋ฉ์๋๋ฅผ ์ฌ์ฉํ๋ฉด ๋ชจ๋ ์ํคํ
์ฒ์ ๋ํด ์ฌ์ ํ์ต๋ ๋ชจ๋ธ์ ๋น ๋ฅด๊ฒ ๋ก๋ํ ์ ์์ผ๋ฏ๋ก ๋ชจ๋ธ์ ์ฒ์๋ถํฐ ํ์ตํ๋ ๋ฐ ์๊ฐ๊ณผ ๋ฆฌ์์ค๋ฅผ ํฌ์
ํ ํ์๊ฐ ์์ต๋๋ค.
์ฒดํฌํฌ์ธํธ์ ๊ตฌ์ ๋ฐ์ง ์๋ ์ฝ๋๋ฅผ ์์ฑํ๋ค๋ ๊ฒ์ ์ฝ๋๊ฐ ํ ์ฒดํฌํฌ์ธํธ์์ ์๋ํ๋ฉด ์ํคํ
์ฒ๊ฐ ๋ค๋ฅด๋๋ผ๋ ๋ค๋ฅธ ์ฒดํฌํฌ์ธํธ(์ ์ฌํ ์์
์ ๋ํด ํ์ต๋ ๊ฒฝ์ฐ)์์๋ ์๋ํ๋ค๋ ๊ฒ์ ์๋ฏธํฉ๋๋ค.
<Tip>
์ํคํ
์ฒ๋ ๋ชจ๋ธ์ ๊ณจ๊ฒฉ์ ์๋ฏธํ๋ฉฐ ์ฒดํฌํฌ์ธํธ๋ ์ฃผ์ด์ง ์ํคํ
์ฒ์ ๋ํ ๊ฐ์ค์น์
๋๋ค. ์๋ฅผ ๋ค์ด, [BERT](https://huggingface.co/bert-base-uncased)๋ ์ํคํ
์ฒ์ด๊ณ , `bert-base-uncased`๋ ์ฒดํฌํฌ์ธํธ์
๋๋ค. ๋ชจ๋ธ์ ์ํคํ
์ฒ ๋๋ ์ฒดํฌํฌ์ธํธ๋ฅผ ์๋ฏธํ ์ ์๋ ์ผ๋ฐ์ ์ธ ์ฉ์ด์
๋๋ค.
</Tip>
์ด ํํ ๋ฆฌ์ผ์์๋ ๋ค์์ ํ์ตํฉ๋๋ค:
* ์ฌ์ ํ์ต๋ ํ ํฌ๋์ด์ ๋ก๋ํ๊ธฐ.
* ์ฌ์ ํ์ต๋ ์ด๋ฏธ์ง ํ๋ก์ธ์ ๋ก๋ํ๊ธฐ.
* ์ฌ์ ํ์ต๋ ํน์ง ์ถ์ถ๊ธฐ ๋ก๋ํ๊ธฐ.
* ์ฌ์ ํ๋ จ๋ ํ๋ก์ธ์ ๋ก๋ํ๊ธฐ.
* ์ฌ์ ํ์ต๋ ๋ชจ๋ธ ๋ก๋ํ๊ธฐ.
## AutoTokenizer[[autotokenizer]]
๊ฑฐ์ ๋ชจ๋ NLP ์์
์ ํ ํฌ๋์ด์ ๋ก ์์๋ฉ๋๋ค. ํ ํฌ๋์ด์ ๋ ์ฌ์ฉ์์ ์
๋ ฅ์ ๋ชจ๋ธ์์ ์ฒ๋ฆฌํ ์ ์๋ ํ์์ผ๋ก ๋ณํํฉ๋๋ค.
[`AutoTokenizer.from_pretrained`]๋ก ํ ํฌ๋์ด์ ๋ฅผ ๋ก๋ํฉ๋๋ค:
```py
>>> from transformers import AutoTokenizer
>>> tokenizer = AutoTokenizer.from_pretrained("bert-base-uncased")
```
๊ทธ๋ฆฌ๊ณ ์๋์ ๊ฐ์ด ์
๋ ฅ์ ํ ํฐํํฉ๋๋ค:
```py
>>> sequence = "In a hole in the ground there lived a hobbit."
>>> print(tokenizer(sequence))
{'input_ids': [101, 1999, 1037, 4920, 1999, 1996, 2598, 2045, 2973, 1037, 7570, 10322, 4183, 1012, 102],
'token_type_ids': [0, 0, 0, 0, 0, 0, 0, 0, 0, 0, 0, 0, 0, 0, 0],
'attention_mask': [1, 1, 1, 1, 1, 1, 1, 1, 1, 1, 1, 1, 1, 1, 1]}
```
## AutoImageProcessor[[autoimageprocessor]]
๋น์ ์์
์ ๊ฒฝ์ฐ ์ด๋ฏธ์ง ํ๋ก์ธ์๊ฐ ์ด๋ฏธ์ง๋ฅผ ์ฌ๋ฐ๋ฅธ ์
๋ ฅ ํ์์ผ๋ก ์ฒ๋ฆฌํฉ๋๋ค.
```py
>>> from transformers import AutoImageProcessor
>>> image_processor = AutoImageProcessor.from_pretrained("google/vit-base-patch16-224")
```
## AutoFeatureExtractor[[autofeatureextractor]]
์ค๋์ค ์์
์ ๊ฒฝ์ฐ ํน์ง ์ถ์ถ๊ธฐ๊ฐ ์ค๋์ค ์ ํธ๋ฅผ ์ฌ๋ฐ๋ฅธ ์
๋ ฅ ํ์์ผ๋ก ์ฒ๋ฆฌํฉ๋๋ค.
[`AutoFeatureExtractor.from_pretrained`]๋ก ํน์ง ์ถ์ถ๊ธฐ๋ฅผ ๋ก๋ํฉ๋๋ค:
```py
>>> from transformers import AutoFeatureExtractor
>>> feature_extractor = AutoFeatureExtractor.from_pretrained(
... "ehcalabres/wav2vec2-lg-xlsr-en-speech-emotion-recognition"
... )
```
## AutoProcessor[[autoprocessor]]
๋ฉํฐ๋ชจ๋ฌ ์์
์๋ ๋ ๊ฐ์ง ์ ํ์ ์ ์ฒ๋ฆฌ ๋๊ตฌ๋ฅผ ๊ฒฐํฉํ ํ๋ก์ธ์๊ฐ ํ์ํฉ๋๋ค. ์๋ฅผ ๋ค์ด LayoutLMV2 ๋ชจ๋ธ์๋ ์ด๋ฏธ์ง๋ฅผ ์ฒ๋ฆฌํ๋ ์ด๋ฏธ์ง ํ๋ก์ธ์์ ํ
์คํธ๋ฅผ ์ฒ๋ฆฌํ๋ ํ ํฌ๋์ด์ ๊ฐ ํ์ํ๋ฉฐ, ํ๋ก์ธ์๋ ์ด ๋ ๊ฐ์ง๋ฅผ ๊ฒฐํฉํฉ๋๋ค.
[`AutoProcessor.from_pretrained()`]๋ก ํ๋ก์ธ์๋ฅผ ๋ก๋ํฉ๋๋ค:
```py
>>> from transformers import AutoProcessor
>>> processor = AutoProcessor.from_pretrained("microsoft/layoutlmv2-base-uncased")
```
## AutoModel[[automodel]]
<frameworkcontent>
<pt>
๋ง์ง๋ง์ผ๋ก AutoModelForํด๋์ค๋ฅผ ์ฌ์ฉํ๋ฉด ์ฃผ์ด์ง ์์
์ ๋ํด ๋ฏธ๋ฆฌ ํ์ต๋ ๋ชจ๋ธ์ ๋ก๋ํ ์ ์์ต๋๋ค (์ฌ์ฉ ๊ฐ๋ฅํ ์์
์ ์ ์ฒด ๋ชฉ๋ก์ [์ฌ๊ธฐ](model_doc/auto)๋ฅผ ์ฐธ์กฐํ์ธ์). ์๋ฅผ ๋ค์ด, [`AutoModelForSequenceClassification.from_pretrained`]๋ฅผ ์ฌ์ฉํ์ฌ ์ํ์ค ๋ถ๋ฅ์ฉ ๋ชจ๋ธ์ ๋ก๋ํ ์ ์์ต๋๋ค:
```py
>>> from transformers import AutoModelForSequenceClassification
>>> model = AutoModelForSequenceClassification.from_pretrained("distilbert-base-uncased")
```
๋์ผํ ์ฒดํฌํฌ์ธํธ๋ฅผ ์ฝ๊ฒ ์ฌ์ฌ์ฉํ์ฌ ๋ค๋ฅธ ์์
์ ์ํคํ
์ฒ๋ฅผ ๋ก๋ํ ์ ์์ต๋๋ค:
```py
>>> from transformers import AutoModelForTokenClassification
>>> model = AutoModelForTokenClassification.from_pretrained("distilbert-base-uncased")
```
<Tip warning={true}>
PyTorch๋ชจ๋ธ์ ๊ฒฝ์ฐ `from_pretrained()` ๋ฉ์๋๋ ๋ด๋ถ์ ์ผ๋ก ํผํด์ ์ฌ์ฉํ์ฌ ์์ ํ์ง ์์ ๊ฒ์ผ๋ก ์๋ ค์ง `torch.load()`๋ฅผ ์ฌ์ฉํฉ๋๋ค.
์ผ๋ฐ์ ์ผ๋ก ์ ๋ขฐํ ์ ์๋ ์์ค์์ ๊ฐ์ ธ์๊ฑฐ๋ ๋ณ์กฐ๋์์ ์ ์๋ ๋ชจ๋ธ์ ๋ก๋ํ์ง ๋ง์ธ์. ํ๊น
ํ์ด์ค ํ๋ธ์์ ํธ์คํ
๋๋ ๊ณต๊ฐ ๋ชจ๋ธ์ ๊ฒฝ์ฐ ์ด๋ฌํ ๋ณด์ ์ํ์ด ๋ถ๋ถ์ ์ผ๋ก ์ํ๋๋ฉฐ, ๊ฐ ์ปค๋ฐ ์ ๋ฉ์จ์ด๋ฅผ [๊ฒ์ฌํฉ๋๋ค](https://huggingface.co/docs/hub/security-malware). GPG๋ฅผ ์ฌ์ฉํด ์๋ช
๋ [์ปค๋ฐ ๊ฒ์ฆ](https://huggingface.co/docs/hub/security-gpg#signing-commits-with-gpg)๊ณผ ๊ฐ์ ๋ชจ๋ฒ์ฌ๋ก๋ [๋ฌธ์](https://huggingface.co/docs/hub/security)๋ฅผ ์ฐธ์กฐํ์ธ์.
ํ
์ํ๋ก์ฐ์ Flax ์ฒดํฌํฌ์ธํธ๋ ์ํฅ์ ๋ฐ์ง ์์ผ๋ฉฐ, `from_pretrained`๋ฉ์๋์ `from_tf` ์ `from_flax` ํค์๋ ๊ฐ๋ณ ์ธ์๋ฅผ ์ฌ์ฉํ์ฌ ์ด ๋ฌธ์ ๋ฅผ ์ฐํํ ์ ์์ต๋๋ค.
</Tip>
์ผ๋ฐ์ ์ผ๋ก AutoTokenizer ํด๋์ค์ AutoModelFor ํด๋์ค๋ฅผ ์ฌ์ฉํ์ฌ ๋ฏธ๋ฆฌ ํ์ต๋ ๋ชจ๋ธ ์ธ์คํด์ค๋ฅผ ๋ก๋ํ๋ ๊ฒ์ด ์ข์ต๋๋ค. ์ด๋ ๊ฒ ํ๋ฉด ๋งค๋ฒ ์ฌ๋ฐ๋ฅธ ์ํคํ
์ฒ๋ฅผ ๋ก๋ํ ์ ์์ต๋๋ค. ๋ค์ [ํํ ๋ฆฌ์ผ](preprocessing)์์๋ ์๋กญ๊ฒ ๋ก๋ํ ํ ํฌ๋์ด์ , ์ด๋ฏธ์ง ํ๋ก์ธ์, ํน์ง ์ถ์ถ๊ธฐ๋ฅผ ์ฌ์ฉํ์ฌ ๋ฏธ์ธ ํ๋์ฉ ๋ฐ์ดํฐ ์ธํธ๋ฅผ ์ ์ฒ๋ฆฌํ๋ ๋ฐฉ๋ฒ์ ๋ํด ์์๋ด
๋๋ค.
</pt>
<tf>
๋ง์ง๋ง์ผ๋ก `TFAutoModelFor` ํด๋์ค๋ฅผ ์ฌ์ฉํ๋ฉด ์ฃผ์ด์ง ์์
์ ๋ํด ์ฌ์ ํ๋ จ๋ ๋ชจ๋ธ์ ๋ก๋ํ ์ ์์ต๋๋ค. (์ฌ์ฉ ๊ฐ๋ฅํ ์์
์ ์ ์ฒด ๋ชฉ๋ก์ [์ฌ๊ธฐ](model_doc/auto)๋ฅผ ์ฐธ์กฐํ์ธ์. ์๋ฅผ ๋ค์ด, [`TFAutoModelForSequenceClassification.from_pretrained`]๋ก ์ํ์ค ๋ถ๋ฅ๋ฅผ ์ํ ๋ชจ๋ธ์ ๋ก๋ํฉ๋๋ค:
```py
>>> from transformers import TFAutoModelForSequenceClassification
>>> model = TFAutoModelForSequenceClassification.from_pretrained("distilbert-base-uncased")
```
์ฝ๊ฒ ๋์ผํ ์ฒดํฌํฌ์ธํธ๋ฅผ ์ฌ์ฌ์ฉํ์ฌ ๋ค๋ฅธ ์์
์ ์ํคํ
์ฒ๋ฅผ ๋ก๋ํ ์ ์์ต๋๋ค:
```py
>>> from transformers import TFAutoModelForTokenClassification
>>> model = TFAutoModelForTokenClassification.from_pretrained("distilbert-base-uncased")
```
์ผ๋ฐ์ ์ผ๋ก, `AutoTokenizer`ํด๋์ค์ `TFAutoModelFor` ํด๋์ค๋ฅผ ์ฌ์ฉํ์ฌ ๋ฏธ๋ฆฌ ํ์ต๋ ๋ชจ๋ธ ์ธ์คํด์ค๋ฅผ ๋ก๋ํ๋ ๊ฒ์ด ์ข์ต๋๋ค. ์ด๋ ๊ฒ ํ๋ฉด ๋งค๋ฒ ์ฌ๋ฐ๋ฅธ ์ํคํ
์ฒ๋ฅผ ๋ก๋ํ ์ ์์ต๋๋ค. ๋ค์ [ํํ ๋ฆฌ์ผ](preprocessing)์์๋ ์๋กญ๊ฒ ๋ก๋ํ ํ ํฌ๋์ด์ , ์ด๋ฏธ์ง ํ๋ก์ธ์, ํน์ง ์ถ์ถ๊ธฐ๋ฅผ ์ฌ์ฉํ์ฌ ๋ฏธ์ธ ํ๋์ฉ ๋ฐ์ดํฐ ์ธํธ๋ฅผ ์ ์ฒ๋ฆฌํ๋ ๋ฐฉ๋ฒ์ ๋ํด ์์๋ด
๋๋ค.
</tf>
</frameworkcontent>
| 0 |
hf_public_repos/transformers/docs/source | hf_public_repos/transformers/docs/source/ko/torchscript.md | <!--Copyright 2022 The HuggingFace Team. All rights reserved.
Licensed under the Apache License, Version 2.0 (the "License"); you may not use this file except in compliance with
the License. You may obtain a copy of the License at
http://www.apache.org/licenses/LICENSE-2.0
Unless required by applicable law or agreed to in writing, software distributed under the License is distributed on
an "AS IS" BASIS, WITHOUT WARRANTIES OR CONDITIONS OF ANY KIND, either express or implied. See the License for the
specific language governing permissions and limitations under the License.
โ ๏ธ Note that this file is in Markdown but contain specific syntax for our doc-builder (similar to MDX) that may not be
rendered properly in your Markdown viewer.
-->
# TorchScript๋ก ๋ด๋ณด๋ด๊ธฐ[[export-to-torchscript]]
<Tip>
TorchScript๋ฅผ ํ์ฉํ ์คํ์ ์์ง ์ด๊ธฐ ๋จ๊ณ๋ก, ๊ฐ๋ณ์ ์ธ ์
๋ ฅ ํฌ๊ธฐ ๋ชจ๋ธ๋ค์ ํตํด ๊ทธ ๊ธฐ๋ฅ์ฑ์ ๊ณ์ ํ๊ตฌํ๊ณ ์์ต๋๋ค.
์ด ๊ธฐ๋ฅ์ ์ ํฌ๊ฐ ๊ด์ฌ์ ๋๊ณ ์๋ ๋ถ์ผ ์ค ํ๋์ด๋ฉฐ,
์์ผ๋ก ์ถ์๋ ๋ฒ์ ์์ ๋ ๋ง์ ์ฝ๋ ์์ , ๋ ์ ์ฐํ ๊ตฌํ, ๊ทธ๋ฆฌ๊ณ Python ๊ธฐ๋ฐ ์ฝ๋์ ์ปดํ์ผ๋ TorchScript๋ฅผ ๋น๊ตํ๋ ๋ฒค์น๋งํฌ๋ฅผ ๋ฑ์ ํตํด ๋ถ์์ ์ฌํํ ์์ ์
๋๋ค.
</Tip>
[TorchScript ๋ฌธ์](https://pytorch.org/docs/stable/jit.html)์์๋ ์ด๋ ๊ฒ ๋งํฉ๋๋ค.
> TorchScript๋ PyTorch ์ฝ๋์์ ์ง๋ ฌํ ๋ฐ ์ต์ ํ ๊ฐ๋ฅํ ๋ชจ๋ธ์ ์์ฑํ๋ ๋ฐฉ๋ฒ์
๋๋ค.
[JIT๊ณผ TRACE](https://pytorch.org/docs/stable/jit.html)๋ ๊ฐ๋ฐ์๊ฐ ๋ชจ๋ธ์ ๋ด๋ณด๋ด์ ํจ์จ ์งํฅ์ ์ธ C++ ํ๋ก๊ทธ๋จ๊ณผ ๊ฐ์ ๋ค๋ฅธ ํ๋ก๊ทธ๋จ์์ ์ฌ์ฌ์ฉํ ์ ์๋๋ก ํ๋ PyTorch ๋ชจ๋์
๋๋ค.
PyTorch ๊ธฐ๋ฐ Python ํ๋ก๊ทธ๋จ๊ณผ ๋ค๋ฅธ ํ๊ฒฝ์์ ๋ชจ๋ธ์ ์ฌ์ฌ์ฉํ ์ ์๋๋ก, ๐ค Transformers ๋ชจ๋ธ์ TorchScript๋ก ๋ด๋ณด๋ผ ์ ์๋ ์ธํฐํ์ด์ค๋ฅผ ์ ๊ณตํฉ๋๋ค.
์ด ๋ฌธ์์์๋ TorchScript๋ฅผ ์ฌ์ฉํ์ฌ ๋ชจ๋ธ์ ๋ด๋ณด๋ด๊ณ ์ฌ์ฉํ๋ ๋ฐฉ๋ฒ์ ์ค๋ช
ํฉ๋๋ค.
๋ชจ๋ธ์ ๋ด๋ณด๋ด๋ ค๋ฉด ๋ ๊ฐ์ง๊ฐ ํ์ํฉ๋๋ค:
- `torchscript` ํ๋๊ทธ๋ก ๋ชจ๋ธ ์ธ์คํด์คํ
- ๋๋ฏธ ์
๋ ฅ์ ์ฌ์ฉํ ์์ ํ(forward pass)
์ด ํ์ ์กฐ๊ฑด๋ค์ ์๋์ ์์ธํ ์ค๋ช
๋ ๊ฒ์ฒ๋ผ ๊ฐ๋ฐ์๋ค์ด ์ฃผ์ํด์ผ ํ ์ฌ๋ฌ ์ฌํญ๋ค์ ์๋ฏธํฉ๋๋ค.
## TorchScript ํ๋๊ทธ์ ๋ฌถ์ธ ๊ฐ์ค์น(tied weights)[[torchscript-flag-and-tied-weights]]
`torchscript` ํ๋๊ทธ๊ฐ ํ์ํ ์ด์ ๋ ๋๋ถ๋ถ์ ๐ค Transformers ์ธ์ด ๋ชจ๋ธ์์ `Embedding` ๋ ์ด์ด์ `Decoding` ๋ ์ด์ด ๊ฐ์ ๋ฌถ์ธ ๊ฐ์ค์น(tied weights)๊ฐ ์กด์ฌํ๊ธฐ ๋๋ฌธ์
๋๋ค.
TorchScript๋ ๋ฌถ์ธ ๊ฐ์ค์น๋ฅผ ๊ฐ์ง ๋ชจ๋ธ์ ๋ด๋ณด๋ผ ์ ์์ผ๋ฏ๋ก, ๋ฏธ๋ฆฌ ๊ฐ์ค์น๋ฅผ ํ๊ณ ๋ณต์ ํด์ผ ํฉ๋๋ค.
`torchscript` ํ๋๊ทธ๋ก ์ธ์คํด์คํ๋ ๋ชจ๋ธ์ `Embedding` ๋ ์ด์ด์ `Decoding` ๋ ์ด์ด๊ฐ ๋ถ๋ฆฌ๋์ด ์์ผ๋ฏ๋ก ์ดํ์ ํ๋ จํด์๋ ์ ๋ฉ๋๋ค.
ํ๋ จ์ ํ๊ฒ ๋๋ฉด ๋ ๋ ์ด์ด ๊ฐ ๋๊ธฐํ๊ฐ ํด์ ๋์ด ์์์น ๋ชปํ ๊ฒฐ๊ณผ๊ฐ ๋ฐ์ํ ์ ์์ต๋๋ค.
์ธ์ด ๋ชจ๋ธ ํค๋๋ฅผ ๊ฐ์ง ์์ ๋ชจ๋ธ์ ๊ฐ์ค์น๊ฐ ๋ฌถ์ฌ ์์ง ์์์ ์ด ๋ฌธ์ ๊ฐ ๋ฐ์ํ์ง ์์ต๋๋ค.
์ด๋ฌํ ๋ชจ๋ธ๋ค์ `torchscript` ํ๋๊ทธ ์์ด ์์ ํ๊ฒ ๋ด๋ณด๋ผ ์ ์์ต๋๋ค.
## ๋๋ฏธ ์
๋ ฅ๊ณผ ํ์ค ๊ธธ์ด[[dummy-inputs-and-standard-lengths]]
๋๋ฏธ ์
๋ ฅ(dummy inputs)์ ๋ชจ๋ธ์ ์์ ํ(forward pass)์ ์ฌ์ฉ๋ฉ๋๋ค.
์
๋ ฅ ๊ฐ์ด ๋ ์ด์ด๋ฅผ ํตํด ์ ํ๋๋ ๋์, PyTorch๋ ๊ฐ ํ
์์์ ์คํ๋ ๋ค๋ฅธ ์ฐ์ฐ์ ์ถ์ ํฉ๋๋ค.
์ด๋ฌํ ๊ธฐ๋ก๋ ์ฐ์ฐ์ ๋ชจ๋ธ์ *์ถ์ (trace)*์ ์์ฑํ๋ ๋ฐ ์ฌ์ฉ๋ฉ๋๋ค.
์ถ์ ์ ์
๋ ฅ์ ์ฐจ์์ ๊ธฐ์ค์ผ๋ก ์์ฑ๋ฉ๋๋ค.
๋ฐ๋ผ์ ๋๋ฏธ ์
๋ ฅ์ ์ฐจ์์ ์ ํ๋์ด, ๋ค๋ฅธ ์ํ์ค ๊ธธ์ด๋ ๋ฐฐ์น ํฌ๊ธฐ์์๋ ์๋ํ์ง ์์ต๋๋ค.
๋ค๋ฅธ ํฌ๊ธฐ๋ก ์๋ํ ๊ฒฝ์ฐ ๋ค์๊ณผ ๊ฐ์ ์ค๋ฅ๊ฐ ๋ฐ์ํฉ๋๋ค:
```
`The expanded size of the tensor (3) must match the existing size (7) at non-singleton dimension 2`
```
์ถ๋ก ์ค ๋ชจ๋ธ์ ๊ณต๊ธ๋ ๊ฐ์ฅ ํฐ ์
๋ ฅ๋งํผ ํฐ ๋๋ฏธ ์
๋ ฅ ํฌ๊ธฐ๋ก ๋ชจ๋ธ์ ์ถ์ ํ๋ ๊ฒ์ด ์ข์ต๋๋ค.
ํจ๋ฉ์ ๋๋ฝ๋ ๊ฐ์ ์ฑ์ฐ๋ ๋ฐ ๋์์ด ๋ ์ ์์ต๋๋ค.
๊ทธ๋ฌ๋ ๋ชจ๋ธ์ด ๋ ํฐ ์
๋ ฅ ํฌ๊ธฐ๋ก ์ถ์ ๋๊ธฐ ๋๋ฌธ์, ํ๋ ฌ์ ์ฐจ์์ด ์ปค์ง๊ณ ๊ณ์ฐ๋์ด ๋ง์์ง๋๋ค.
๋ค์ํ ์ํ์ค ๊ธธ์ด ๋ชจ๋ธ์ ๋ด๋ณด๋ผ ๋๋ ๊ฐ ์
๋ ฅ์ ๋ํด ์ํ๋๋ ์ด ์ฐ์ฐ ํ์์ ์ฃผ์ํ๊ณ ์ฑ๋ฅ์ ์ฃผ์ ๊น๊ฒ ํ์ธํ์ธ์.
## Python์์ TorchScript ์ฌ์ฉํ๊ธฐ[[using-torchscript-in-python]]
์ด ์น์
์์๋ ๋ชจ๋ธ์ ์ ์ฅํ๊ณ ๊ฐ์ ธ์ค๋ ๋ฐฉ๋ฒ, ์ถ์ ์ ์ฌ์ฉํ์ฌ ์ถ๋ก ํ๋ ๋ฐฉ๋ฒ์ ๋ณด์ฌ์ค๋๋ค.
### ๋ชจ๋ธ ์ ์ฅํ๊ธฐ[[saving-a-model]]
`BertModel`์ TorchScript๋ก ๋ด๋ณด๋ด๋ ค๋ฉด `BertConfig` ํด๋์ค์์ `BertModel`์ ์ธ์คํด์คํํ ๋ค์, `traced_bert.pt`๋ผ๋ ํ์ผ๋ช
์ผ๋ก ๋์คํฌ์ ์ ์ฅํ๋ฉด ๋ฉ๋๋ค.
```python
from transformers import BertModel, BertTokenizer, BertConfig
import torch
enc = BertTokenizer.from_pretrained("bert-base-uncased")
# ์
๋ ฅ ํ
์คํธ ํ ํฐํํ๊ธฐ
text = "[CLS] Who was Jim Henson ? [SEP] Jim Henson was a puppeteer [SEP]"
tokenized_text = enc.tokenize(text)
# ์
๋ ฅ ํ ํฐ ์ค ํ๋๋ฅผ ๋ง์คํนํ๊ธฐ
masked_index = 8
tokenized_text[masked_index] = "[MASK]"
indexed_tokens = enc.convert_tokens_to_ids(tokenized_text)
segments_ids = [0, 0, 0, 0, 0, 0, 0, 1, 1, 1, 1, 1, 1, 1]
# ๋๋ฏธ ์
๋ ฅ ๋ง๋ค๊ธฐ
tokens_tensor = torch.tensor([indexed_tokens])
segments_tensors = torch.tensor([segments_ids])
dummy_input = [tokens_tensor, segments_tensors]
# torchscript ํ๋๊ทธ๋ก ๋ชจ๋ธ ์ด๊ธฐํํ๊ธฐ
# ์ด ๋ชจ๋ธ์ LM ํค๋๊ฐ ์์ผ๋ฏ๋ก ํ์ํ์ง ์์ง๋ง, ํ๋๊ทธ๋ฅผ True๋ก ์ค์ ํฉ๋๋ค.
config = BertConfig(
vocab_size_or_config_json_file=32000,
hidden_size=768,
num_hidden_layers=12,
num_attention_heads=12,
intermediate_size=3072,
torchscript=True,
)
# ๋ชจ๋ธ์ ์ธ์คํดํธํํ๊ธฐ
model = BertModel(config)
# ๋ชจ๋ธ์ ํ๊ฐ ๋ชจ๋๋ก ๋์ด์ผ ํฉ๋๋ค.
model.eval()
# ๋ง์ฝ *from_pretrained*๋ฅผ ์ฌ์ฉํ์ฌ ๋ชจ๋ธ์ ์ธ์คํด์คํํ๋ ๊ฒฝ์ฐ, TorchScript ํ๋๊ทธ๋ฅผ ์ฝ๊ฒ ์ค์ ํ ์ ์์ต๋๋ค
model = BertModel.from_pretrained("bert-base-uncased", torchscript=True)
# ์ถ์ ์์ฑํ๊ธฐ
traced_model = torch.jit.trace(model, [tokens_tensor, segments_tensors])
torch.jit.save(traced_model, "traced_bert.pt")
```
### ๋ชจ๋ธ ๊ฐ์ ธ์ค๊ธฐ[[loading-a-model]]
์ด์ ์ด์ ์ ์ ์ฅํ `BertModel`, ์ฆ `traced_bert.pt`๋ฅผ ๋์คํฌ์์ ๊ฐ์ ธ์ค๊ณ , ์ด์ ์ ์ด๊ธฐํํ `dummy_input`์์ ์ฌ์ฉํ ์ ์์ต๋๋ค.
```python
loaded_model = torch.jit.load("traced_bert.pt")
loaded_model.eval()
all_encoder_layers, pooled_output = loaded_model(*dummy_input)
```
### ์ถ์ ๋ ๋ชจ๋ธ์ ์ฌ์ฉํ์ฌ ์ถ๋ก ํ๊ธฐ[[using-a-traced-model-for-inference]]
`__call__` ์ด์ค ์ธ๋์ค์ฝ์ด(dunder) ๋ฉ์๋๋ฅผ ์ฌ์ฉํ์ฌ ์ถ๋ก ์ ์ถ์ ๋ ๋ชจ๋ธ์ ์ฌ์ฉํ์ธ์:
```python
traced_model(tokens_tensor, segments_tensors)
```
## Neuron SDK๋ก Hugging Face TorchScript ๋ชจ๋ธ์ AWS์ ๋ฐฐํฌํ๊ธฐ[[deploy-hugging-face-torchscript-models-to-aws-with-the-neuron-sdk]]
AWS๊ฐ ํด๋ผ์ฐ๋์์ ์ ๋น์ฉ, ๊ณ ์ฑ๋ฅ ๋จธ์ ๋ฌ๋ ์ถ๋ก ์ ์ํ [Amazon EC2 Inf1](https://aws.amazon.com/ec2/instance-types/inf1/) ์ธ์คํด์ค ์ ํ๊ตฐ์ ์ถ์ํ์ต๋๋ค.
Inf1 ์ธ์คํด์ค๋ ๋ฅ๋ฌ๋ ์ถ๋ก ์ํฌ๋ก๋์ ํนํ๋ ๋ง์ถค ํ๋์จ์ด ๊ฐ์๊ธฐ์ธ AWS Inferentia ์นฉ์ผ๋ก ๊ตฌ๋๋ฉ๋๋ค.
[AWS Neuron](https://awsdocs-neuron.readthedocs-hosted.com/en/latest/#)์ Inferentia๋ฅผ ์ํ SDK๋ก, Inf1์ ๋ฐฐํฌํ๊ธฐ ์ํ transformers ๋ชจ๋ธ ์ถ์ ๋ฐ ์ต์ ํ๋ฅผ ์ง์ํฉ๋๋ค.
Neuron SDK๋ ๋ค์๊ณผ ๊ฐ์ ๊ธฐ๋ฅ์ ์ ๊ณตํฉ๋๋ค:
1. ์ฝ๋ ํ ์ค๋ง ๋ณ๊ฒฝํ๋ฉด ํด๋ผ์ฐ๋ ์ถ๋ก ๋ฅผ ์ํด TorchScript ๋ชจ๋ธ์ ์ถ์ ํ๊ณ ์ต์ ํํ ์ ์๋ ์ฌ์ด API
2. ์ฆ์ ์ฌ์ฉ ๊ฐ๋ฅํ ์ฑ๋ฅ ์ต์ ํ๋ก [๋น์ฉ ํจ์จ ํฅ์](https://awsdocs-neuron.readthedocs-hosted.com/en/latest/neuron-guide/benchmark/>)
3. [PyTorch](https://awsdocs-neuron.readthedocs-hosted.com/en/latest/src/examples/pytorch/bert_tutorial/tutorial_pretrained_bert.html) ๋๋ [TensorFlow](https://awsdocs-neuron.readthedocs-hosted.com/en/latest/src/examples/tensorflow/huggingface_bert/huggingface_bert.html)๋ก ๊ตฌ์ถ๋ Hugging Face transformers ๋ชจ๋ธ ์ง์
### ์์ฌ์ [[implications]]
[BERT (Bidirectional Encoder Representations from Transformers)](https://huggingface.co/docs/transformers/main/model_doc/bert) ์ํคํ
์ฒ ๋๋ ๊ทธ ๋ณํ์ธ [distilBERT](https://huggingface.co/docs/transformers/main/model_doc/distilbert) ๋ฐ [roBERTa](https://huggingface.co/docs/transformers/main/model_doc/roberta)๋ฅผ ๊ธฐ๋ฐ์ผ๋ก ํ Transformers ๋ชจ๋ธ์ ์ถ์ถ ๊ธฐ๋ฐ ์ง์์๋ต, ์ํ์ค ๋ถ๋ฅ ๋ฐ ํ ํฐ ๋ถ๋ฅ์ ๊ฐ์ ๋น์์ฑ ์์
์ Inf1์์ ์ต์์ ์ฑ๋ฅ์ ๋ณด์
๋๋ค.
๊ทธ๋ฌ๋ ํ
์คํธ ์์ฑ ์์
๋ [AWS Neuron MarianMT ํํ ๋ฆฌ์ผ](https://awsdocs-neuron.readthedocs-hosted.com/en/latest/src/examples/pytorch/transformers-marianmt.html)์ ๋ฐ๋ผ Inf1์์ ์คํ๋๋๋ก ์กฐ์ ํ ์ ์์ต๋๋ค.
Inferentia์์ ๋ฐ๋ก ๋ณํํ ์ ์๋ ๋ชจ๋ธ์ ๋ํ ์์ธํ ์ ๋ณด๋ Neuron ๋ฌธ์์ [Model Architecture Fit](https://awsdocs-neuron.readthedocs-hosted.com/en/latest/neuron-guide/models/models-inferentia.html#models-inferentia) ์น์
์์ ํ์ธํ ์ ์์ต๋๋ค.
### ์ข
์์ฑ[[dependencies]]
AWS Neuron์ ์ฌ์ฉํ์ฌ ๋ชจ๋ธ์ ๋ณํํ๋ ค๋ฉด [Neuron SDK ํ๊ฒฝ](https://awsdocs-neuron.readthedocs-hosted.com/en/latest/neuron-guide/neuron-frameworks/pytorch-neuron/index.html#installation-guide)์ด ํ์ํฉ๋๋ค.
์ด๋ [AWS Deep Learning AMI](https://docs.aws.amazon.com/dlami/latest/devguide/tutorial-inferentia-launching.html)์ ๋ฏธ๋ฆฌ ๊ตฌ์ฑ๋์ด ์์ต๋๋ค.
### AWS Neuron์ผ๋ก ๋ชจ๋ธ ๋ณํํ๊ธฐ[[converting-a-model-for-aws-neuron]]
`BertModel`์ ์ถ์ ํ๋ ค๋ฉด, [Python์์ TorchScript ์ฌ์ฉํ๊ธฐ](torchscript#using-torchscript-in-python)์์์ ๋์ผํ ์ฝ๋๋ฅผ ์ฌ์ฉํด์ AWS NEURON์ฉ ๋ชจ๋ธ์ ๋ณํํฉ๋๋ค.
`torch.neuron` ํ๋ ์์ํฌ ์ต์คํ
์
์ ๊ฐ์ ธ์ Python API๋ฅผ ํตํด Neuron SDK์ ๊ตฌ์ฑ ์์์ ์ ๊ทผํฉ๋๋ค:
```python
from transformers import BertModel, BertTokenizer, BertConfig
import torch
import torch.neuron
```
๋ค์ ์ค๋ง ์์ ํ๋ฉด ๋ฉ๋๋ค:
```diff
- torch.jit.trace(model, [tokens_tensor, segments_tensors])
+ torch.neuron.trace(model, [token_tensor, segments_tensors])
```
์ด๋ก์จ Neuron SDK๊ฐ ๋ชจ๋ธ์ ์ถ์ ํ๊ณ Inf1 ์ธ์คํด์ค์ ์ต์ ํํ ์ ์๊ฒ ๋ฉ๋๋ค.
AWS Neuron SDK์ ๊ธฐ๋ฅ, ๋๊ตฌ, ์์ ํํ ๋ฆฌ์ผ ๋ฐ ์ต์ ์
๋ฐ์ดํธ์ ๋ํด ์์ธํ ์์๋ณด๋ ค๋ฉด [AWS NeuronSDK ๋ฌธ์](https://awsdocs-neuron.readthedocs-hosted.com/en/latest/index.html)๋ฅผ ์ฐธ์กฐํ์ธ์.
| 0 |
hf_public_repos/transformers/docs/source | hf_public_repos/transformers/docs/source/ko/task_summary.md | <!--Copyright 2020 The HuggingFace Team. All rights reserved.
Licensed under the Apache License, Version 2.0 (the "License"); you may not use this file except in compliance with
the License. You may obtain a copy of the License at
http://www.apache.org/licenses/LICENSE-2.0
Unless required by applicable law or agreed to in writing, software distributed under the License is distributed on
an "AS IS" BASIS, WITHOUT WARRANTIES OR CONDITIONS OF ANY KIND, either express or implied. See the License for the
specific language governing permissions and limitations under the License.
โ ๏ธ Note that this file is in Markdown but contain specific syntax for our doc-builder (similar to MDX) that may not be
rendered properly in your Markdown viewer.
-->
# ๐ค Transformers๋ก ํ ์ ์๋ ๊ฒ[[what__transformers_can_do]]
๐ค Transformers๋ ์์ฐ์ด์ฒ๋ฆฌ(NLP), ์ปดํจํฐ ๋น์ , ์ค๋์ค ๋ฐ ์์ฑ ์ฒ๋ฆฌ ์์
์ ๋ํ ์ฌ์ ํ๋ จ๋ ์ต์ฒจ๋จ ๋ชจ๋ธ ๋ผ์ด๋ธ๋ฌ๋ฆฌ์
๋๋ค.
์ด ๋ผ์ด๋ธ๋ฌ๋ฆฌ๋ ํธ๋์คํฌ๋จธ ๋ชจ๋ธ๋ฟ๋ง ์๋๋ผ ์ปดํจํฐ ๋น์ ์์
์ ์ํ ํ๋์ ์ธ ํฉ์ฑ๊ณฑ ์ ๊ฒฝ๋ง๊ณผ ๊ฐ์ ํธ๋์คํฌ๋จธ๊ฐ ์๋ ๋ชจ๋ธ๋ ํฌํจํ๊ณ ์์ต๋๋ค.
์ค๋งํธํฐ, ์ฑ, ํ
๋ ๋น์ ๊ณผ ๊ฐ์ ์ค๋๋ ๊ฐ์ฅ ์ธ๊ธฐ ์๋ ์๋น์ ์ ํ์ ์ดํด๋ณด๋ฉด, ๋ฅ๋ฌ๋ ๊ธฐ์ ์ด ๊ทธ ๋ค์ ์ฌ์ฉ๋๊ณ ์์ ํ๋ฅ ์ด ๋์ต๋๋ค.
์ค๋งํธํฐ์ผ๋ก ์ดฌ์ํ ์ฌ์ง์์ ๋ฐฐ๊ฒฝ ๊ฐ์ฒด๋ฅผ ์ ๊ฑฐํ๊ณ ์ถ๋ค๋ฉด ์ด๋ป๊ฒ ํ ๊น์? ์ด๋ ํ๋ํฑ ์ธ๊ทธ๋ฉํ
์ด์
์์
์ ์์
๋๋ค(์์ง ์ด๊ฒ ๋ฌด์์ธ์ง ๋ชจ๋ฅธ๋ค๋ฉด, ๋ค์ ์น์
์์ ์ค๋ช
ํ๊ฒ ์ต๋๋ค!).
์ด ํ์ด์ง๋ ๋ค์ํ ์์ฑ ๋ฐ ์ค๋์ค, ์ปดํจํฐ ๋น์ , NLP ์์
์ ๐ค Transformers ๋ผ์ด๋ธ๋ฌ๋ฆฌ๋ฅผ ํ์ฉํ์ฌ ๋ค๋ฃจ๋ ๊ฐ๋จํ ์์ ๋ฅผ 3์ค์ ์ฝ๋๋ก ์ ๊ณตํฉ๋๋ค.
## ์ค๋์ค[[audio]]
์์ฑ ๋ฐ ์ค๋์ค ์ฒ๋ฆฌ ์์
์ ๋ค๋ฅธ ๋ชจ๋ฌ๋ฆฌํฐ์ ์ฝ๊ฐ ๋ค๋ฆ
๋๋ค. ์ด๋ ์ฃผ๋ก ์ค๋์ค๊ฐ ์ฐ์์ ์ธ ์ ํธ๋ก ์
๋ ฅ๋๊ธฐ ๋๋ฌธ์
๋๋ค.
ํ
์คํธ์ ๋ฌ๋ฆฌ ์๋ณธ ์ค๋์ค ํํ(waveform)์ ๋ฌธ์ฅ์ด ๋จ์ด๋ก ๋๋ ์ง๋ ๊ฒ์ฒ๋ผ ๊น๋ํ๊ฒ ์ด์ฐ์ ์ธ ๋ฌถ์์ผ๋ก ๋๋ ์ ์์ต๋๋ค.
์ด๋ฅผ ๊ทน๋ณตํ๊ธฐ ์ํด ์๋ณธ ์ค๋์ค ์ ํธ๋ ์ผ์ ํ ๊ฐ๊ฒฉ์ผ๋ก ์ํ๋ง๋ฉ๋๋ค. ํด๋น ๊ฐ๊ฒฉ ๋ด์์ ๋ ๋ง์ ์ํ์ ์ทจํ ๊ฒฝ์ฐ ์ํ๋ง๋ฅ ์ด ๋์์ง๋ฉฐ, ์ค๋์ค๋ ์๋ณธ ์ค๋์ค ์์ค์ ๋ ๊ฐ๊น์์ง๋๋ค.
๊ณผ๊ฑฐ์ ์ ๊ทผ ๋ฐฉ์์ ์ค๋์ค์์ ์ ์ฉํ ํน์ง์ ์ถ์ถํ๊ธฐ ์ํด ์ค๋์ค๋ฅผ ์ ์ฒ๋ฆฌํ๋ ๊ฒ์ด์์ต๋๋ค.
ํ์ง๋ง ํ์ฌ๋ ์๋ณธ ์ค๋์ค ํํ์ ํน์ฑ ์ธ์ฝ๋์ ์ง์ ๋ฃ์ด์ ์ค๋์ค ํํ(representation)์ ์ถ์ถํ๋ ๊ฒ์ด ๋ ์ผ๋ฐ์ ์
๋๋ค.
์ด๋ ๊ฒ ํ๋ฉด ์ ์ฒ๋ฆฌ ๋จ๊ณ๊ฐ ๋จ์ํด์ง๊ณ ๋ชจ๋ธ์ด ๊ฐ์ฅ ์ค์ํ ํน์ง์ ํ์ตํ ์ ์์ต๋๋ค.
### ์ค๋์ค ๋ถ๋ฅ[[audio_classification]]
์ค๋์ค ๋ถ๋ฅ๋ ์ค๋์ค ๋ฐ์ดํฐ์ ๋ฏธ๋ฆฌ ์ ์๋ ํด๋์ค ์งํฉ์ ๋ ์ด๋ธ์ ์ง์ ํ๋ ์์
์
๋๋ค. ์ด๋ ๋ง์ ๊ตฌ์ฒด์ ์ธ ์์ฉ ํ๋ก๊ทธ๋จ์ ํฌํจํ ๋์ ๋ฒ์ฃผ์
๋๋ค.
์ผ๋ถ ์์๋ ๋ค์๊ณผ ๊ฐ์ต๋๋ค:
* ์ํฅ ์ฅ๋ฉด ๋ถ๋ฅ: ์ค๋์ค์ ์ฅ๋ฉด ๋ ์ด๋ธ("์ฌ๋ฌด์ค", "ํด๋ณ", "๊ฒฝ๊ธฐ์ฅ")์ ์ง์ ํฉ๋๋ค.
* ์ํฅ ์ด๋ฒคํธ ๊ฐ์ง: ์ค๋์ค์ ์๋ฆฌ ์ด๋ฒคํธ ๋ ์ด๋ธ("์ฐจ ๊ฒฝ์ ", "๊ณ ๋ ์ธ์์๋ฆฌ", "์ ๋ฆฌ ํ์")์ ์ง์ ํฉ๋๋ค.
* ํ๊น
: ์ฌ๋ฌ ๊ฐ์ง ์๋ฆฌ(์ ์ง์ ๊ท, ํ์์์์ ํ์ ์๋ณ)๊ฐ ํฌํจ๋ ์ค๋์ค์ ๋ ์ด๋ธ์ ์ง์ ํฉ๋๋ค.
* ์์
๋ถ๋ฅ: ์์
์ ์ฅ๋ฅด ๋ ์ด๋ธ("๋ฉํ", "ํํฉ", "์ปจํธ๋ฆฌ")์ ์ง์ ํฉ๋๋ค.
```py
>>> from transformers import pipeline
>>> classifier = pipeline(task="audio-classification", model="superb/hubert-base-superb-er")
>>> preds = classifier("https://huggingface.co/datasets/Narsil/asr_dummy/resolve/main/mlk.flac")
>>> preds = [{"score": round(pred["score"], 4), "label": pred["label"]} for pred in preds]
>>> preds
[{'score': 0.4532, 'label': 'hap'},
{'score': 0.3622, 'label': 'sad'},
{'score': 0.0943, 'label': 'neu'},
{'score': 0.0903, 'label': 'ang'}]
```
### ์๋ ์์ฑ ์ธ์[[automatic_speech_recognition]]
์๋ ์์ฑ ์ธ์(ASR)์ ์์ฑ์ ํ
์คํธ๋ก ๋ณํํ๋ ์์
์
๋๋ค.
์์ฑ์ ์ธ๊ฐ์ ์์ฐ์ค๋ฌ์ด ์์ฌ์ํต ํํ์ด๊ธฐ ๋๋ฌธ์ ASR์ ๊ฐ์ฅ ์ผ๋ฐ์ ์ธ ์ค๋์ค ์์
์ค ํ๋์
๋๋ค.
์ค๋๋ ASR ์์คํ
์ ์คํผ์ปค, ์ ํ ๋ฐ ์๋์ฐจ์ ๊ฐ์ "์ค๋งํธ" ๊ธฐ์ ์ ํ์ ๋ด์ฅ๋์ด ์์ต๋๋ค.
์ฐ๋ฆฌ๋ ๊ฐ์ ๋น์์๊ฒ ์์
์ฌ์, ์๋ฆผ ์ค์ ๋ฐ ๋ ์จ ์ ๋ณด๋ฅผ ์์ฒญํ ์ ์์ต๋๋ค.
ํ์ง๋ง ํธ๋์คํฌ๋จธ ์ํคํ
์ฒ๊ฐ ํด๊ฒฐํ๋ ๋ฐ ๋์์ ์ค ํต์ฌ ๋์ ๊ณผ์ ์ค ํ๋๋ ์์ด ๋ฐ์ดํฐ ์์ด ์ ์ ์ธ์ด(low-resource language)์ ๋ํ ๊ฒ์
๋๋ค. ๋๋์ ์์ฑ ๋ฐ์ดํฐ๋ก ์ฌ์ ํ๋ จํ ํ ๋ฐ์ดํฐ ์์ด ์ ์ ์ธ์ด์์ ๋ ์ด๋ธ์ด ์ง์ ๋ ์์ฑ ๋ฐ์ดํฐ 1์๊ฐ๋ง์ผ๋ก ๋ชจ๋ธ์ ๋ฏธ์ธ ์กฐ์ ํ๋ฉด ์ด์ ์ 100๋ฐฐ ๋ง์ ๋ ์ด๋ธ์ด ์ง์ ๋ ๋ฐ์ดํฐ๋ก ํ๋ จ๋ ASR ์์คํ
๋ณด๋ค ํจ์ฌ ๋ ๋์ ํ์ง์ ๊ฒฐ๊ณผ๋ฅผ ์ป์ ์ ์์ต๋๋ค.
```py
>>> from transformers import pipeline
>>> transcriber = pipeline(task="automatic-speech-recognition", model="openai/whisper-small")
>>> transcriber("https://huggingface.co/datasets/Narsil/asr_dummy/resolve/main/mlk.flac")
{'text': ' I have a dream that one day this nation will rise up and live out the true meaning of its creed.'}
```
## ์ปดํจํฐ ๋น์ [[computer_vision]]
์ปดํจํฐ ๋น์ ์์
์ค ๊ฐ์ฅ ์ด๊ธฐ์ ์ฑ๊ณต์ ์ธ ์์
์ค ํ๋๋ [ํฉ์ฑ๊ณฑ ์ ๊ฒฝ๋ง(CNN)](glossary#convolution)์ ์ฌ์ฉํ์ฌ ์ฐํธ๋ฒํธ ์ซ์ ์ด๋ฏธ์ง๋ฅผ ์ธ์ํ๋ ๊ฒ์ด์์ต๋๋ค. ์ด๋ฏธ์ง๋ ํฝ์
๋ก ๊ตฌ์ฑ๋์ด ์์ผ๋ฉฐ ๊ฐ ํฝ์
์ ์ซ์ ๊ฐ์ผ๋ก ํํ๋ฉ๋๋ค. ์ด๋ก์จ ์ด๋ฏธ์ง๋ฅผ ํฝ์
๊ฐ์ ํ๋ ฌ๋ก ๋ํ๋ด๋ ๊ฒ์ด ์ฌ์์ง๋๋ค. ํน์ ํ ํฝ์
๊ฐ์ ์กฐํฉ์ ์ด๋ฏธ์ง์ ์์์ ์๋ฏธํฉ๋๋ค.
์ปดํจํฐ ๋น์ ์์
์ ์ผ๋ฐ์ ์ผ๋ก ๋ค์ ๋ ๊ฐ์ง ๋ฐฉ๋ฒ์ผ๋ก ์ ๊ทผ ๊ฐ๋ฅํฉ๋๋ค:
1. ํฉ์ฑ๊ณฑ์ ์ฌ์ฉํ์ฌ ์ด๋ฏธ์ง์ ๋ฎ์ ์์ค ํน์ง์์ ๋์ ์์ค์ ์ถ์์ ์ธ ์์๊น์ง ๊ณ์ธต์ ์ผ๋ก ํ์ตํฉ๋๋ค.
2. ์ด๋ฏธ์ง๋ฅผ ํจ์น๋ก ๋๋๊ณ ํธ๋์คํฌ๋จธ๋ฅผ ์ฌ์ฉํ์ฌ ์ ์ง์ ์ผ๋ก ๊ฐ ์ด๋ฏธ์ง ํจ์น๊ฐ ์๋ก ์ด๋ ํ ๋ฐฉ์์ผ๋ก ์ฐ๊ด๋์ด ์ด๋ฏธ์ง๋ฅผ ํ์ฑํ๋์ง ํ์ตํฉ๋๋ค. `CNN`์์ ์ ํธํ๋ ์ํฅ์ ์ ๊ทผ๋ฒ๊ณผ๋ ๋ฌ๋ฆฌ, ์ด ๋ฐฉ์์ ํ๋ฆฟํ ์ด๋ฏธ์ง๋ก ์ด์์ ๊ทธ๋ฆฌ๊ณ ์ ์ง์ ์ผ๋ก ์ ๋ช
ํ ์ด๋ฏธ์ง๋ก ๋ง๋ค์ด๊ฐ๋ ๊ฒ๊ณผ ์ ์ฌํฉ๋๋ค.
### ์ด๋ฏธ์ง ๋ถ๋ฅ[[image_classification]]
์ด๋ฏธ์ง ๋ถ๋ฅ๋ ํ ๊ฐ์ ์ ์ฒด ์ด๋ฏธ์ง์ ๋ฏธ๋ฆฌ ์ ์๋ ํด๋์ค ์งํฉ์ ๋ ์ด๋ธ์ ์ง์ ํ๋ ์์
์
๋๋ค.
๋๋ถ๋ถ์ ๋ถ๋ฅ ์์
๊ณผ ๋ง์ฐฌ๊ฐ์ง๋ก, ์ด๋ฏธ์ง ๋ถ๋ฅ์๋ ๋ค์ํ ์ค์ฉ์ ์ธ ์ฉ๋๊ฐ ์์ผ๋ฉฐ, ์ผ๋ถ ์์๋ ๋ค์๊ณผ ๊ฐ์ต๋๋ค:
* ์๋ฃ: ์ง๋ณ์ ๊ฐ์งํ๊ฑฐ๋ ํ์ ๊ฑด๊ฐ์ ๋ชจ๋ํฐ๋งํ๊ธฐ ์ํด ์๋ฃ ์ด๋ฏธ์ง์ ๋ ์ด๋ธ์ ์ง์ ํฉ๋๋ค.
* ํ๊ฒฝ: ์์ฑ ์ด๋ฏธ์ง๋ฅผ ๋ถ๋ฅํ์ฌ ์ฐ๋ฆผ ๋ฒ์ฑ๋ฅผ ๊ฐ์ํ๊ณ ์ผ์ ์ง์ญ ๊ด๋ฆฌ๋ฅผ ์ํ ์ ๋ณด๋ฅผ ์ ๊ณตํ๊ฑฐ๋ ์ฐ๋ถ์ ๊ฐ์งํฉ๋๋ค.
* ๋์
: ์๋ฌผ ์ด๋ฏธ์ง๋ฅผ ๋ถ๋ฅํ์ฌ ์๋ฌผ ๊ฑด๊ฐ์ ํ์ธํ๊ฑฐ๋ ์์ฑ ์ด๋ฏธ์ง๋ฅผ ๋ถ๋ฅํ์ฌ ํ ์ง ์ด์ฉ ๊ด์ฐฐ์ ์ฌ์ฉํฉ๋๋ค.
* ์ํํ: ๋๋ฌผ์ด๋ ์๋ฌผ ์ข
์ด๋ฏธ์ง๋ฅผ ๋ถ๋ฅํ์ฌ ์ผ์ ๋๋ฌผ ๊ฐ์ฒด๊ตฐ์ ์กฐ์ฌํ๊ฑฐ๋ ๋ฉธ์ข
์๊ธฐ์ ์ฒํ ์ข
์ ์ถ์ ํฉ๋๋ค.
```py
>>> from transformers import pipeline
>>> classifier = pipeline(task="image-classification")
>>> preds = classifier(
... "https://huggingface.co/datasets/huggingface/documentation-images/resolve/main/pipeline-cat-chonk.jpeg"
... )
>>> preds = [{"score": round(pred["score"], 4), "label": pred["label"]} for pred in preds]
>>> print(*preds, sep="\n")
{'score': 0.4335, 'label': 'lynx, catamount'}
{'score': 0.0348, 'label': 'cougar, puma, catamount, mountain lion, painter, panther, Felis concolor'}
{'score': 0.0324, 'label': 'snow leopard, ounce, Panthera uncia'}
{'score': 0.0239, 'label': 'Egyptian cat'}
{'score': 0.0229, 'label': 'tiger cat'}
```
### ๊ฐ์ฒด ํ์ง[[object_detection]]
์ด๋ฏธ์ง ๋ถ๋ฅ์ ๋ฌ๋ฆฌ ๊ฐ์ฒด ํ์ง๋ ์ด๋ฏธ์ง ๋ด์์ ์ฌ๋ฌ ๊ฐ์ฒด๋ฅผ ์๋ณํ๊ณ ๋ฐ์ด๋ฉ ๋ฐ์ค๋ก ์ ์๋ ๊ฐ์ฒด์ ์์น๋ฅผ ํ์
ํฉ๋๋ค.
๊ฐ์ฒด ํ์ง์ ๋ช ๊ฐ์ง ์์ฉ ์์๋ ๋ค์๊ณผ ๊ฐ์ต๋๋ค:
* ์์จ ์ฃผํ ์ฐจ๋: ๋ค๋ฅธ ์ฐจ๋, ๋ณดํ์ ๋ฐ ์ ํธ๋ฑ๊ณผ ๊ฐ์ ์ผ์์ ์ธ ๊ตํต ๊ฐ์ฒด๋ฅผ ๊ฐ์งํฉ๋๋ค.
* ์๊ฒฉ ๊ฐ์ง: ์ฌ๋ ๋ชจ๋ํฐ๋ง, ๋์ ๊ณํ ๋ฐ ๊ธฐ์ ์์ธก ๋ฑ์ ์ํํฉ๋๋ค.
* ๊ฒฐํจ ํ์ง: ๊ฑด๋ฌผ์ ๊ท ์ด์ด๋ ๊ตฌ์กฐ์ ์์, ์ ์กฐ ๊ฒฐํจ ๋ฑ์ ํ์งํฉ๋๋ค.
```py
>>> from transformers import pipeline
>>> detector = pipeline(task="object-detection")
>>> preds = detector(
... "https://huggingface.co/datasets/huggingface/documentation-images/resolve/main/pipeline-cat-chonk.jpeg"
... )
>>> preds = [{"score": round(pred["score"], 4), "label": pred["label"], "box": pred["box"]} for pred in preds]
>>> preds
[{'score': 0.9865,
'label': 'cat',
'box': {'xmin': 178, 'ymin': 154, 'xmax': 882, 'ymax': 598}}]
```
### ์ด๋ฏธ์ง ๋ถํ [[image_segmentation]]
์ด๋ฏธ์ง ๋ถํ ์ ํฝ์
์ฐจ์์ ์์
์ผ๋ก, ์ด๋ฏธ์ง ๋ด์ ๋ชจ๋ ํฝ์
์ ํด๋์ค์ ํ ๋นํฉ๋๋ค. ์ด๋ ๊ฐ์ฒด ํ์ง์ ๋ค๋ฆ
๋๋ค. ๊ฐ์ฒด ํ์ง๋ ๋ฐ์ด๋ฉ ๋ฐ์ค๋ฅผ ์ฌ์ฉํ์ฌ ์ด๋ฏธ์ง ๋ด์ ๊ฐ์ฒด๋ฅผ ๋ ์ด๋ธ๋งํ๊ณ ์์ธกํ๋ ๋ฐ๋ฉด, ๋ถํ ์ ๋ ์ธ๋ถํ๋ ์์
์
๋๋ค. ๋ถํ ์ ํฝ์
์์ค์์ ๊ฐ์ฒด๋ฅผ ๊ฐ์งํ ์ ์์ต๋๋ค.
์ด๋ฏธ์ง ๋ถํ ์๋ ์ฌ๋ฌ ์ ํ์ด ์์ต๋๋ค:
* ์ธ์คํด์ค ๋ถํ : ๊ฐ์ฒด์ ํด๋์ค๋ฅผ ๋ ์ด๋ธ๋งํ๋ ๊ฒ ์ธ์๋, ๊ฐ์ฒด์ ๊ฐ ๊ตฌ๋ถ๋ ์ธ์คํด์ค์๋ ๋ ์ด๋ธ์ ์ง์ ํฉ๋๋ค ("๊ฐ-1", "๊ฐ-2" ๋ฑ).
* ํ๋ํฑ ๋ถํ : ์๋ฏธ์ ๋ถํ ๊ณผ ์ธ์คํด์ค ๋ถํ ์ ์กฐํฉ์
๋๋ค. ๊ฐ ํฝ์
์ ์๋ฏธ์ ํด๋์ค๋ก ๋ ์ด๋ธ๋งํ๋ **๋์์** ๊ฐ์ฒด์ ๊ฐ๊ฐ ๊ตฌ๋ถ๋ ์ธ์คํด์ค๋ก๋ ๋ ์ด๋ธ์ ์ง์ ํฉ๋๋ค.
๋ถํ ์์
์ ์์จ ์ฃผํ ์ฐจ๋์์ ์ ์ฉํ๋ฉฐ, ์ฃผ๋ณ ํ๊ฒฝ์ ํฝ์
์์ค ์ง๋๋ฅผ ์์ฑํ์ฌ ๋ณดํ์์ ๋ค๋ฅธ ์ฐจ๋ ์ฃผ๋ณ์์ ์์ ํ๊ฒ ํ์ํ ์ ์์ต๋๋ค. ๋ํ ์๋ฃ ์์์์๋ ์ ์ฉํฉ๋๋ค. ๋ถํ ์์
์ด ํฝ์
์์ค์์ ๊ฐ์ฒด๋ฅผ ๊ฐ์งํ ์ ์๊ธฐ ๋๋ฌธ์ ๋น์ ์์ ์ธ ์ธํฌ๋ ์ฅ๊ธฐ์ ํน์ง์ ์๋ณํ๋ ๋ฐ ๋์์ด ๋ ์ ์์ต๋๋ค. ์ด๋ฏธ์ง ๋ถํ ์ ์๋ฅ ๊ฐ์ ์์ฐฉ์ด๋ ์นด๋ฉ๋ผ๋ฅผ ํตํด ์ค์ ์ธ๊ณ์ ๊ฐ์ ๊ฐ์ฒด๋ฅผ ๋ง์์ ์ฆ๊ฐ ํ์ค ๊ฒฝํ์ ๋ง๋๋ ๋ฑ ์ ์ ์๊ฑฐ๋ ๋ถ์ผ์์๋ ์ฌ์ฉ๋ ์ ์์ต๋๋ค.
```py
>>> from transformers import pipeline
>>> segmenter = pipeline(task="image-segmentation")
>>> preds = segmenter(
... "https://huggingface.co/datasets/huggingface/documentation-images/resolve/main/pipeline-cat-chonk.jpeg"
... )
>>> preds = [{"score": round(pred["score"], 4), "label": pred["label"]} for pred in preds]
>>> print(*preds, sep="\n")
{'score': 0.9879, 'label': 'LABEL_184'}
{'score': 0.9973, 'label': 'snow'}
{'score': 0.9972, 'label': 'cat'}
```
### ๊น์ด ์ถ์ [[depth_estimation]]
๊น์ด ์ถ์ ์ ์นด๋ฉ๋ผ๋ก๋ถํฐ ์ด๋ฏธ์ง ๋ด๋ถ์ ๊ฐ ํฝ์
์ ๊ฑฐ๋ฆฌ๋ฅผ ์์ธกํฉ๋๋ค. ์ด ์ปดํจํฐ ๋น์ ์์
์ ํนํ ์ฅ๋ฉด ์ดํด์ ์ฌ๊ตฌ์ฑ์ ์ค์ํฉ๋๋ค. ์๋ฅผ ๋ค์ด, ์์จ ์ฃผํ ์ฐจ๋์ ๋ณดํ์, ๊ตํต ํ์งํ ๋ฐ ๋ค๋ฅธ ์ฐจ๋๊ณผ ๊ฐ์ ๊ฐ์ฒด์์ ๊ฑฐ๋ฆฌ๋ฅผ ์ดํดํ์ฌ ์ฅ์ ๋ฌผ๊ณผ ์ถฉ๋์ ํผํด์ผ ํฉ๋๋ค. ๊น์ด ์ ๋ณด๋ ๋ํ 2D ์ด๋ฏธ์ง์์ 3D ํํ์ ๊ตฌ์ฑํ๋ ๋ฐ ๋์์ด ๋๋ฉฐ ์๋ฌผํ์ ๊ตฌ์กฐ๋ ๊ฑด๋ฌผ์ ๊ณ ํ์ง 3D ํํ์ ์์ฑํ๋ ๋ฐ ์ฌ์ฉ๋ ์ ์์ต๋๋ค.
๊น์ด ์ถ์ ์๋ ๋ ๊ฐ์ง ์ ๊ทผ ๋ฐฉ์์ด ์์ต๋๋ค:
* ์คํ
๋ ์ค: ์ฝ๊ฐ ๋ค๋ฅธ ๊ฐ๋์์ ์ดฌ์๋ ๋์ผํ ์ด๋ฏธ์ง ๋ ์ฅ์ ๋น๊ตํ์ฌ ๊น์ด๋ฅผ ์ถ์ ํฉ๋๋ค.
* ๋จ์: ๋จ์ผ ์ด๋ฏธ์ง์์ ๊น์ด๋ฅผ ์ถ์ ํฉ๋๋ค.
```py
>>> from transformers import pipeline
>>> depth_estimator = pipeline(task="depth-estimation")
>>> preds = depth_estimator(
... "https://huggingface.co/datasets/huggingface/documentation-images/resolve/main/pipeline-cat-chonk.jpeg"
... )
```
## ์์ฐ์ด์ฒ๋ฆฌ[[natural_language_processing]]
ํ
์คํธ๋ ์ธ๊ฐ์ด ์์ฌ ์ํตํ๋ ์์ฐ์ค๋ฌ์ด ๋ฐฉ์ ์ค ํ๋์ด๊ธฐ ๋๋ฌธ์ ์์ฐ์ด์ฒ๋ฆฌ ์ญ์ ๊ฐ์ฅ ์ผ๋ฐ์ ์ธ ์์
์ ํ ์ค ํ๋์
๋๋ค. ๋ชจ๋ธ์ด ์ธ์ํ๋ ํ์์ผ๋ก ํ
์คํธ๋ฅผ ๋ณํํ๋ ค๋ฉด ํ ํฐํํด์ผ ํฉ๋๋ค. ์ด๋ ํ
์คํธ ์ํ์ค๋ฅผ ๊ฐ๋ณ ๋จ์ด ๋๋ ํ์ ๋จ์ด(ํ ํฐ)๋ก ๋ถํ ํ ๋ค์ ์ด๋ฌํ ํ ํฐ์ ์ซ์๋ก ๋ณํํ๋ ๊ฒ์ ์๋ฏธํฉ๋๋ค. ๊ฒฐ๊ณผ์ ์ผ๋ก ํ
์คํธ ์ํ์ค๋ฅผ ์ซ์ ์ํ์ค๋ก ํํํ ์ ์์ผ๋ฉฐ, ์ซ์ ์ํ์ค๋ฅผ ๋ค์ํ ์์ฐ์ด์ฒ๋ฆฌ ์์
์ ํด๊ฒฐํ๊ธฐ ์ํ ๋ชจ๋ธ์ ์
๋ ฅํ ์ ์์ต๋๋ค!
### ํ
์คํธ ๋ถ๋ฅ[[text_classification]]
๋ค๋ฅธ ๋ชจ๋ฌ๋ฆฌํฐ์์์ ๋ถ๋ฅ ์์
๊ณผ ๋ง์ฐฌ๊ฐ์ง๋ก ํ
์คํธ ๋ถ๋ฅ๋ ๋ฏธ๋ฆฌ ์ ์๋ ํด๋์ค ์งํฉ์์ ํ
์คํธ ์ํ์ค(๋ฌธ์ฅ ์์ค, ๋จ๋ฝ ๋๋ ๋ฌธ์ ๋ฑ)์ ๋ ์ด๋ธ์ ์ง์ ํฉ๋๋ค. ํ
์คํธ ๋ถ๋ฅ์๋ ๋ค์ํ ์ค์ฉ์ ์ธ ์์ฉ ์ฌ๋ก๊ฐ ์์ผ๋ฉฐ, ์ผ๋ถ ์์๋ ๋ค์๊ณผ ๊ฐ์ต๋๋ค:
* ๊ฐ์ฑ ๋ถ์: ํ
์คํธ๋ฅผ `๊ธ์ ` ๋๋ `๋ถ์ `๊ณผ ๊ฐ์ ์ด๋ค ๊ทน์ฑ์ ๋ฐ๋ผ ๋ ์ด๋ธ๋งํ์ฌ ์ ์น, ๊ธ์ต, ๋ง์ผํ
๊ณผ ๊ฐ์ ๋ถ์ผ์์ ์์ฌ ๊ฒฐ์ ์ ์ ๋ณด๋ฅผ ์ ๊ณตํ๊ณ ์ง์ํ ์ ์์ต๋๋ค.
* ์ฝํ
์ธ ๋ถ๋ฅ: ํ
์คํธ๋ฅผ ์ฃผ์ ์ ๋ฐ๋ผ ๋ ์ด๋ธ๋ง(๋ ์จ, ์คํฌ์ธ , ๊ธ์ต ๋ฑ)ํ์ฌ ๋ด์ค ๋ฐ ์์
๋ฏธ๋์ด ํผ๋์์ ์ ๋ณด๋ฅผ ๊ตฌ์ฑํ๊ณ ํํฐ๋งํ๋ ๋ฐ ๋์์ด ๋ ์ ์์ต๋๋ค.
```py
>>> from transformers import pipeline
>>> classifier = pipeline(task="sentiment-analysis")
>>> preds = classifier("Hugging Face is the best thing since sliced bread!")
>>> preds = [{"score": round(pred["score"], 4), "label": pred["label"]} for pred in preds]
>>> preds
[{'score': 0.9991, 'label': 'POSITIVE'}]
```
### ํ ํฐ ๋ถ๋ฅ[[token_classification]]
๋ชจ๋ ์์ฐ์ด์ฒ๋ฆฌ ์์
์์๋ ํ
์คํธ๊ฐ ๊ฐ๋ณ ๋จ์ด๋ ํ์ ๋จ์ด๋ก ๋ถ๋ฆฌ๋์ด ์ ์ฒ๋ฆฌ๋ฉ๋๋ค. ๋ถ๋ฆฌ๋ ๋จ์ด๋ฅผ [ํ ํฐ](/glossary#token)์ด๋ผ๊ณ ํฉ๋๋ค. ํ ํฐ ๋ถ๋ฅ๋ ๊ฐ ํ ํฐ์ ๋ฏธ๋ฆฌ ์ ์๋ ํด๋์ค ์งํฉ์ ๋ ์ด๋ธ์ ํ ๋นํฉ๋๋ค.
ํ ํฐ ๋ถ๋ฅ์ ๋ ๊ฐ์ง ์ผ๋ฐ์ ์ธ ์ ํ์ ๋ค์๊ณผ ๊ฐ์ต๋๋ค:
* ๊ฐ์ฒด๋ช
์ธ์ (NER): ํ ํฐ์ ์กฐ์ง, ์ธ๋ฌผ, ์์น ๋๋ ๋ ์ง์ ๊ฐ์ ๊ฐ์ฒด ๋ฒ์ฃผ์ ๋ฐ๋ผ ๋ ์ด๋ธ๋งํฉ๋๋ค. NER์ ํนํ ์ ์ ์ฒดํ์ ์ธ ํ๊ฒฝ์์ ์ ์ ์, ๋จ๋ฐฑ์ง ๋ฐ ์ฝ๋ฌผ ์ด๋ฆ์ ๋ ์ด๋ธ์ ์ง์ ํ๋ ๋ฐ ๋๋ฆฌ ์ฌ์ฉ๋ฉ๋๋ค.
* ํ์ฌ ํ๊น
(POS): ๋ช
์ฌ, ๋์ฌ, ํ์ฉ์ฌ์ ๊ฐ์ ํ์ฌ์ ๋ฐ๋ผ ํ ํฐ์ ๋ ์ด๋ธ์ ํ ๋นํฉ๋๋ค. POS๋ ๋ฒ์ญ ์์คํ
์ด ๋์ผํ ๋จ์ด๊ฐ ๋ฌธ๋ฒ์ ์ผ๋ก ์ด๋ป๊ฒ ๋ค๋ฅธ์ง ์ดํดํ๋ ๋ฐ ๋์์ด ๋ฉ๋๋ค (๋ช
์ฌ๋ก ์ฌ์ฉ๋๋ "bank(์ํ)"๊ณผ ๋์ฌ๋ก ์ฌ์ฉ๋๋ "bank(์๊ธ์ ์์นํ๋ค)"๊ณผ ๊ฐ์ ๊ฒฝ์ฐ).
```py
>>> from transformers import pipeline
>>> classifier = pipeline(task="ner")
>>> preds = classifier("Hugging Face is a French company based in New York City.")
>>> preds = [
... {
... "entity": pred["entity"],
... "score": round(pred["score"], 4),
... "index": pred["index"],
... "word": pred["word"],
... "start": pred["start"],
... "end": pred["end"],
... }
... for pred in preds
... ]
>>> print(*preds, sep="\n")
{'entity': 'I-ORG', 'score': 0.9968, 'index': 1, 'word': 'Hu', 'start': 0, 'end': 2}
{'entity': 'I-ORG', 'score': 0.9293, 'index': 2, 'word': '##gging', 'start': 2, 'end': 7}
{'entity': 'I-ORG', 'score': 0.9763, 'index': 3, 'word': 'Face', 'start': 8, 'end': 12}
{'entity': 'I-MISC', 'score': 0.9983, 'index': 6, 'word': 'French', 'start': 18, 'end': 24}
{'entity': 'I-LOC', 'score': 0.999, 'index': 10, 'word': 'New', 'start': 42, 'end': 45}
{'entity': 'I-LOC', 'score': 0.9987, 'index': 11, 'word': 'York', 'start': 46, 'end': 50}
{'entity': 'I-LOC', 'score': 0.9992, 'index': 12, 'word': 'City', 'start': 51, 'end': 55}
```
### ์ง์์๋ต[[question_answering]]
์ง์์๋ต์ ๋ ํ๋์ ํ ํฐ ์ฐจ์์ ์์
์ผ๋ก, ๋ฌธ๋งฅ์ด ์์ ๋(๊ฐ๋ฐฉํ ๋๋ฉ์ธ)์ ๋ฌธ๋งฅ์ด ์์ ๋(ํ์ํ ๋๋ฉ์ธ) ์ง๋ฌธ์ ๋ํ ๋ต๋ณ์ ๋ฐํํฉ๋๋ค. ์ด ์์
์ ๊ฐ์ ๋น์์๊ฒ ์๋น์ด ์์
์ค์ธ์ง์ ๊ฐ์ ์ง๋ฌธ์ ํ ๋๋ง๋ค ๋ฐ์ํ ์ ์์ต๋๋ค. ๊ณ ๊ฐ ์ง์ ๋๋ ๊ธฐ์ ์ง์์ ์ ๊ณตํ๊ฑฐ๋ ๊ฒ์ ์์ง์ด ์์ฒญํ ์ ๋ณด๋ฅผ ๊ฒ์ํ๋ ๋ฐ ๋์์ ์ค ์ ์์ต๋๋ค.
์ง๋ฌธ ๋ต๋ณ์๋ ์ผ๋ฐ์ ์ผ๋ก ๋ ๊ฐ์ง ์ ํ์ด ์์ต๋๋ค:
* ์ถ์ถํ: ์ง๋ฌธ๊ณผ ๋ฌธ๋งฅ์ด ์ฃผ์ด์ก์ ๋, ๋ชจ๋ธ์ด ์ฃผ์ด์ง ๋ฌธ๋งฅ์ ์ผ๋ถ์์ ๊ฐ์ ธ์จ ํ
์คํธ์ ๋ฒ์๋ฅผ ๋ต๋ณ์ผ๋ก ํฉ๋๋ค.
* ์์ฑํ: ์ง๋ฌธ๊ณผ ๋ฌธ๋งฅ์ด ์ฃผ์ด์ก์ ๋, ์ฃผ์ด์ง ๋ฌธ๋งฅ์ ํตํด ๋ต๋ณ์ ์์ฑํฉ๋๋ค. ์ด ์ ๊ทผ ๋ฐฉ์์ [`QuestionAnsweringPipeline`] ๋์ [`Text2TextGenerationPipeline`]์ ํตํด ์ฒ๋ฆฌ๋ฉ๋๋ค.
```py
>>> from transformers import pipeline
>>> question_answerer = pipeline(task="question-answering")
>>> preds = question_answerer(
... question="What is the name of the repository?",
... context="The name of the repository is huggingface/transformers",
... )
>>> print(
... f"score: {round(preds['score'], 4)}, start: {preds['start']}, end: {preds['end']}, answer: {preds['answer']}"
... )
score: 0.9327, start: 30, end: 54, answer: huggingface/transformers
```
### ์์ฝ[[summarization]]
์์ฝ์ ์๋ณธ ๋ฌธ์์ ์๋ฏธ๋ฅผ ์ต๋ํ ๋ณด์กดํ๋ฉด์ ๊ธด ๋ฌธ์๋ฅผ ์งง์ ๋ฌธ์๋ก ๋ง๋๋ ์์
์
๋๋ค. ์์ฝ์ `sequence-to-sequence` ์์
์
๋๋ค. ์
๋ ฅ๋ณด๋ค ์งง์ ํ
์คํธ ์ํ์ค๋ฅผ ์ถ๋ ฅํฉ๋๋ค. ์์ฝ ์์
์ ๋
์๊ฐ ์ฅ๋ฌธ ๋ฌธ์๋ค์ ์ฃผ์ ํฌ์ธํธ๋ฅผ ๋น ๋ฅด๊ฒ ์ดํดํ๋ ๋ฐ ๋์์ ์ค ์ ์์ต๋๋ค. ์
๋ฒ์, ๋ฒ๋ฅ ๋ฐ ๊ธ์ต ๋ฌธ์, ํนํ ๋ฐ ๊ณผํ ๋
ผ๋ฌธ์ ์์ฝ ์์
์ด ๋
์์ ์๊ฐ์ ์ ์ฝํ๊ณ ๋
์ ๋ณด์กฐ ๋๊ตฌ๋ก ์ฌ์ฉ๋ ์ ์๋ ๋ช ๊ฐ์ง ์์์
๋๋ค.
์ง๋ฌธ ๋ต๋ณ๊ณผ ๋ง์ฐฌ๊ฐ์ง๋ก ์์ฝ์๋ ๋ ๊ฐ์ง ์ ํ์ด ์์ต๋๋ค:
* ์ถ์ถํ: ์๋ณธ ํ
์คํธ์์ ๊ฐ์ฅ ์ค์ํ ๋ฌธ์ฅ์ ์๋ณํ๊ณ ์ถ์ถํฉ๋๋ค.
* ์์ฑํ: ์๋ณธ ํ
์คํธ์์ ๋ชฉํ ์์ฝ์ ์์ฑํฉ๋๋ค. ์
๋ ฅ ๋ฌธ์์ ์๋ ์๋ก์ด ๋จ์ด๋ฅผ ํฌํจํ ์๋ ์์ต๋๋ค. [`SummarizationPipeline`]์ ์์ฑํ ์ ๊ทผ ๋ฐฉ์์ ์ฌ์ฉํฉ๋๋ค.
```py
>>> from transformers import pipeline
>>> summarizer = pipeline(task="summarization")
>>> summarizer(
... "In this work, we presented the Transformer, the first sequence transduction model based entirely on attention, replacing the recurrent layers most commonly used in encoder-decoder architectures with multi-headed self-attention. For translation tasks, the Transformer can be trained significantly faster than architectures based on recurrent or convolutional layers. On both WMT 2014 English-to-German and WMT 2014 English-to-French translation tasks, we achieve a new state of the art. In the former task our best model outperforms even all previously reported ensembles."
... )
[{'summary_text': ' The Transformer is the first sequence transduction model based entirely on attention . It replaces the recurrent layers most commonly used in encoder-decoder architectures with multi-headed self-attention . For translation tasks, the Transformer can be trained significantly faster than architectures based on recurrent or convolutional layers .'}]
```
### ๋ฒ์ญ[[translation]]
๋ฒ์ญ์ ํ ์ธ์ด๋ก ๋ ํ
์คํธ ์ํ์ค๋ฅผ ๋ค๋ฅธ ์ธ์ด๋ก ๋ณํํ๋ ์์
์
๋๋ค. ์ด๋ ์๋ก ๋ค๋ฅธ ๋ฐฐ๊ฒฝ์ ๊ฐ์ง ์ฌ๋๋ค์ด ์๋ก ์ํตํ๋ ๋ฐ ๋์์ ์ฃผ๋ ์ค์ํ ์ญํ ์ ํฉ๋๋ค. ๋ ๋์ ๋์ค์๊ฒ ์ฝํ
์ธ ๋ฅผ ๋ฒ์ญํ์ฌ ์ ๋ฌํ๊ฑฐ๋, ์๋ก์ด ์ธ์ด๋ฅผ ๋ฐฐ์ฐ๋ ๋ฐ ๋์์ด ๋๋ ํ์ต ๋๊ตฌ๊ฐ ๋ ์๋ ์์ต๋๋ค. ์์ฝ๊ณผ ๋ง์ฐฌ๊ฐ์ง๋ก, ๋ฒ์ญ์ `sequence-to-sequence` ์์
์
๋๋ค. ์ฆ, ๋ชจ๋ธ์ ์
๋ ฅ ์ํ์ค๋ฅผ ๋ฐ์์ ์ถ๋ ฅ์ด ๋๋ ๋ชฉํ ์ํ์ค๋ฅผ ๋ฐํํฉ๋๋ค.
์ด๊ธฐ์ ๋ฒ์ญ ๋ชจ๋ธ์ ๋๋ถ๋ถ ๋จ์ผ ์ธ์ด๋ก ์ด๋ฃจ์ด์ ธ ์์์ง๋ง, ์ต๊ทผ์๋ ๋ง์ ์ธ์ด ์ ๊ฐ์ ๋ฒ์ญ์ ์ํํ ์ ์๋ ๋ค์ค ์ธ์ด ๋ชจ๋ธ์ ๋ํ ๊ด์ฌ์ด ๋์์ง๊ณ ์์ต๋๋ค.
```py
>>> from transformers import pipeline
>>> text = "translate English to French: Hugging Face is a community-based open-source platform for machine learning."
>>> translator = pipeline(task="translation", model="t5-small")
>>> translator(text)
[{'translation_text': "Hugging Face est une tribune communautaire de l'apprentissage des machines."}]
```
### ์ธ์ด ๋ชจ๋ธ๋ง[[language_modeling]]
์ธ์ด ๋ชจ๋ธ๋ง์ ํ
์คํธ ์ํ์ค์์ ๋จ์ด๋ฅผ ์์ธกํ๋ ์์
์
๋๋ค. ์ฌ์ ํ๋ จ๋ ์ธ์ด ๋ชจ๋ธ์ ๋ง์ ๋ค๋ฅธ ํ์ ์์
์ ๋ฐ๋ผ ๋ฏธ์ธ ์กฐ์ ๋ ์ ์๊ธฐ ๋๋ฌธ์ ๋งค์ฐ ์ธ๊ธฐ ์๋ ์์ฐ์ด์ฒ๋ฆฌ ์์
์ด ๋์์ต๋๋ค. ์ต๊ทผ์๋ ์ ๋ก ์ท(zero-shot) ๋๋ ํจ ์ท(few-shot) ํ์ต์ด ๊ฐ๋ฅํ ๋๊ท๋ชจ ์ธ์ด ๋ชจ๋ธ(Large Language Models, LLM)์ ๋ํ ๋ง์ ๊ด์ฌ์ด ๋ฐ์ํ๊ณ ์์ต๋๋ค. ์ด๋ ๋ชจ๋ธ์ด ๋ช
์์ ์ผ๋ก ํ๋ จ๋์ง ์์ ์์
๋ ํด๊ฒฐํ ์ ์๋ค๋ ๊ฒ์ ์๋ฏธํฉ๋๋ค! ์ธ์ด ๋ชจ๋ธ์ ์ ์ฐฝํ๊ณ ์ค๋๋ ฅ ์๋ ํ
์คํธ๋ฅผ ์์ฑํ๋ ๋ฐ ์ฌ์ฉ๋ ์ ์์ง๋ง, ํ
์คํธ๊ฐ ํญ์ ์ ํํ์ง๋ ์์ ์ ์์ผ๋ฏ๋ก ์ฃผ์๊ฐ ํ์ํฉ๋๋ค.
์ธ์ด ๋ชจ๋ธ๋ง์๋ ๋ ๊ฐ์ง ์ ํ์ด ์์ต๋๋ค:
* ์ธ๊ณผ์ ์ธ์ด ๋ชจ๋ธ๋ง: ์ด ๋ชจ๋ธ์ ๋ชฉ์ ์ ์ํ์ค์์ ๋ค์ ํ ํฐ์ ์์ธกํ๋ ๊ฒ์ด๋ฉฐ, ๋ฏธ๋ ํ ํฐ์ด ๋ง์คํน ๋ฉ๋๋ค.
```py
>>> from transformers import pipeline
>>> prompt = "Hugging Face is a community-based open-source platform for machine learning."
>>> generator = pipeline(task="text-generation")
>>> generator(prompt) # doctest: +SKIP
```
* ๋ง์คํน๋ ์ธ์ด ๋ชจ๋ธ๋ง: ์ด ๋ชจ๋ธ์ ๋ชฉ์ ์ ์ํ์ค ๋ด์ ๋ง์คํน๋ ํ ํฐ์ ์์ธกํ๋ ๊ฒ์ด๋ฉฐ, ์ํ์ค ๋ด์ ๋ชจ๋ ํ ํฐ์ ๋ํ ์ ๊ทผ์ด ์ ๊ณต๋ฉ๋๋ค.
```py
>>> text = "Hugging Face is a community-based open-source <mask> for machine learning."
>>> fill_mask = pipeline(task="fill-mask")
>>> preds = fill_mask(text, top_k=1)
>>> preds = [
... {
... "score": round(pred["score"], 4),
... "token": pred["token"],
... "token_str": pred["token_str"],
... "sequence": pred["sequence"],
... }
... for pred in preds
... ]
>>> preds
[{'score': 0.2236,
'token': 1761,
'token_str': ' platform',
'sequence': 'Hugging Face is a community-based open-source platform for machine learning.'}]
```
์ด ํ์ด์ง๋ฅผ ํตํด ๊ฐ ๋ชจ๋ฌ๋ฆฌํฐ์ ๋ค์ํ ์์
์ ํ๊ณผ ๊ฐ ์์
์ ์ค์ฉ์ ์ค์์ฑ์ ๋ํด ์ถ๊ฐ์ ์ธ ๋ฐฐ๊ฒฝ ์ ๋ณด๋ฅผ ์ป์ผ์
จ๊ธฐ๋ฅผ ๋ฐ๋๋๋ค. ๋ค์ [์น์
](tasks_explained)์์๋ ๐ค Transformer๊ฐ ์ด๋ฌํ ์์
์ ํด๊ฒฐํ๋ **๋ฐฉ๋ฒ**์ ๋ํด ์์๋ณด์ค ์ ์์ต๋๋ค. | 0 |
hf_public_repos/transformers/docs/source | hf_public_repos/transformers/docs/source/ko/custom_models.md | <!--Copyright 2020 The HuggingFace Team. All rights reserved.
Licensed under the Apache License, Version 2.0 (the "License"); you may not use this file except in compliance with
the License. You may obtain a copy of the License at
http://www.apache.org/licenses/LICENSE-2.0
Unless required by applicable law or agreed to in writing, software distributed under the License is distributed on
an "AS IS" BASIS, WITHOUT WARRANTIES OR CONDITIONS OF ANY KIND, either express or implied. See the License for the
specific language governing permissions and limitations under the License.
โ ๏ธ Note that this file is in Markdown but contain specific syntax for our doc-builder (similar to MDX) that may not be
rendered properly in your Markdown viewer.
-->
# ์ฌ์ฉ์ ์ ์ ๋ชจ๋ธ ๊ณต์ ํ๊ธฐ[[sharing-custom-models]]
๐ค Transformers ๋ผ์ด๋ธ๋ฌ๋ฆฌ๋ ์ฝ๊ฒ ํ์ฅํ ์ ์๋๋ก ์ค๊ณ๋์์ต๋๋ค.
๋ชจ๋ ๋ชจ๋ธ์ ์ถ์ํ ์์ด ์ ์ฅ์์ ์ง์ ๋ ํ์ ํด๋์ ์์ ํ ์ฝ๋ฉ๋์ด ์์ผ๋ฏ๋ก, ์์ฝ๊ฒ ๋ชจ๋ธ๋ง ํ์ผ์ ๋ณต์ฌํ๊ณ ํ์์ ๋ฐ๋ผ ์กฐ์ ํ ์ ์์ต๋๋ค.
์์ ํ ์๋ก์ด ๋ชจ๋ธ์ ๋ง๋๋ ๊ฒฝ์ฐ์๋ ์ฒ์๋ถํฐ ์์ํ๋ ๊ฒ์ด ๋ ์ฌ์ธ ์ ์์ต๋๋ค.
์ด ํํ ๋ฆฌ์ผ์์๋ Transformers ๋ด์์ ์ฌ์ฉํ ์ ์๋๋ก ์ฌ์ฉ์ ์ ์ ๋ชจ๋ธ๊ณผ ๊ตฌ์ฑ์ ์์ฑํ๋ ๋ฐฉ๋ฒ๊ณผ
๐ค Transformers ๋ผ์ด๋ธ๋ฌ๋ฆฌ์ ์๋ ๊ฒฝ์ฐ์๋ ๋๊ตฌ๋ ์ฌ์ฉํ ์ ์๋๋ก (์์กด์ฑ๊ณผ ํจ๊ป) ์ปค๋ฎค๋ํฐ์ ๊ณต์ ํ๋ ๋ฐฉ๋ฒ์ ๋ฐฐ์ธ ์ ์์ต๋๋ค.
[timm ๋ผ์ด๋ธ๋ฌ๋ฆฌ](https://github.com/rwightman/pytorch-image-models)์ ResNet ํด๋์ค๋ฅผ [`PreTrainedModel`]๋ก ๋ํํ ResNet ๋ชจ๋ธ์ ์๋ก ๋ชจ๋ ๊ฒ์ ์ค๋ช
ํฉ๋๋ค.
## ์ฌ์ฉ์ ์ ์ ๊ตฌ์ฑ ์์ฑํ๊ธฐ[[writing-a-custom-configuration]]
๋ชจ๋ธ์ ๋ค์ด๊ฐ๊ธฐ ์ ์ ๋จผ์ ๊ตฌ์ฑ์ ์์ฑํด๋ณด๋๋ก ํ๊ฒ ์ต๋๋ค.
๋ชจ๋ธ์ `configuration`์ ๋ชจ๋ธ์ ๋ง๋ค๊ธฐ ์ํด ํ์ํ ๋ชจ๋ ์ค์ํ ๊ฒ๋ค์ ํฌํจํ๊ณ ์๋ ๊ฐ์ฒด์
๋๋ค.
๋ค์ ์น์
์์ ๋ณผ ์ ์๋ฏ์ด, ๋ชจ๋ธ์ `config`๋ฅผ ์ฌ์ฉํด์๋ง ์ด๊ธฐํํ ์ ์๊ธฐ ๋๋ฌธ์ ์๋ฒฝํ ๊ตฌ์ฑ์ด ํ์ํฉ๋๋ค.
์๋ ์์์์๋ ResNet ํด๋์ค์ ์ธ์(argument)๋ฅผ ์กฐ์ ํด๋ณด๊ฒ ์ต๋๋ค.
๋ค๋ฅธ ๊ตฌ์ฑ์ ๊ฐ๋ฅํ ResNet ์ค ๋ค๋ฅธ ์ ํ์ ์ ๊ณตํฉ๋๋ค.
๊ทธ๋ฐ ๋ค์ ๋ช ๊ฐ์ง ์ ํจ์ฑ์ ํ์ธํ ํ ํด๋น ์ธ์๋ฅผ ์ ์ฅํฉ๋๋ค.
```python
from transformers import PretrainedConfig
from typing import List
class ResnetConfig(PretrainedConfig):
model_type = "resnet"
def __init__(
self,
block_type="bottleneck",
layers: List[int] = [3, 4, 6, 3],
num_classes: int = 1000,
input_channels: int = 3,
cardinality: int = 1,
base_width: int = 64,
stem_width: int = 64,
stem_type: str = "",
avg_down: bool = False,
**kwargs,
):
if block_type not in ["basic", "bottleneck"]:
raise ValueError(f"`block_type` must be 'basic' or bottleneck', got {block_type}.")
if stem_type not in ["", "deep", "deep-tiered"]:
raise ValueError(f"`stem_type` must be '', 'deep' or 'deep-tiered', got {stem_type}.")
self.block_type = block_type
self.layers = layers
self.num_classes = num_classes
self.input_channels = input_channels
self.cardinality = cardinality
self.base_width = base_width
self.stem_width = stem_width
self.stem_type = stem_type
self.avg_down = avg_down
super().__init__(**kwargs)
```
์ฌ์ฉ์ ์ ์ `configuration`์ ์์ฑํ ๋ ๊ธฐ์ตํด์ผ ํ ์ธ ๊ฐ์ง ์ค์ํ ์ฌํญ์ ๋ค์๊ณผ ๊ฐ์ต๋๋ค:
- `PretrainedConfig`์ ์์ํด์ผ ํฉ๋๋ค.
- `PretrainedConfig`์ `__init__`์ ๋ชจ๋ kwargs๋ฅผ ํ์ฉํด์ผ ํ๊ณ ,
- ์ด๋ฌํ `kwargs`๋ ์์ ํด๋์ค `__init__`์ ์ ๋ฌ๋์ด์ผ ํฉ๋๋ค.
์์์ ๐ค Transformers ๋ผ์ด๋ธ๋ฌ๋ฆฌ์์ ๋ชจ๋ ๊ธฐ๋ฅ์ ๊ฐ์ ธ์ค๋ ๊ฒ์
๋๋ค.
์ด๋ฌํ ์ ์ผ๋ก๋ถํฐ ๋น๋กฏ๋๋ ๋ ๊ฐ์ง ์ ์ฝ ์กฐ๊ฑด์ `PretrainedConfig`์ ์ค์ ํ๋ ๊ฒ๋ณด๋ค ๋ ๋ง์ ํ๋๊ฐ ์์ต๋๋ค.
`from_pretrained` ๋ฉ์๋๋ก ๊ตฌ์ฑ์ ๋ค์ ๋ก๋ํ ๋ ํด๋น ํ๋๋ ๊ตฌ์ฑ์์ ์๋ฝํ ํ ์์ ํด๋์ค๋ก ๋ณด๋ด์ผ ํฉ๋๋ค.
๋ชจ๋ธ์ auto ํด๋์ค์ ๋ฑ๋กํ์ง ์๋ ํ, `configuration`์์ `model_type`์ ์ ์(์ฌ๊ธฐ์ `model_type="resnet"`)ํ๋ ๊ฒ์ ํ์ ์ฌํญ์ด ์๋๋๋ค (๋ง์ง๋ง ์น์
์ฐธ์กฐ).
์ด๋ ๊ฒ ํ๋ฉด ๋ผ์ด๋ธ๋ฌ๋ฆฌ์ ๋ค๋ฅธ ๋ชจ๋ธ ๊ตฌ์ฑ๊ณผ ๋ง์ฐฌ๊ฐ์ง๋ก ๊ตฌ์ฑ์ ์ฝ๊ฒ ๋ง๋ค๊ณ ์ ์ฅํ ์ ์์ต๋๋ค.
๋ค์์ resnet50d ๊ตฌ์ฑ์ ์์ฑํ๊ณ ์ ์ฅํ๋ ๋ฐฉ๋ฒ์
๋๋ค:
```py
resnet50d_config = ResnetConfig(block_type="bottleneck", stem_width=32, stem_type="deep", avg_down=True)
resnet50d_config.save_pretrained("custom-resnet")
```
์ด๋ ๊ฒ ํ๋ฉด `custom-resnet` ํด๋ ์์ `config.json`์ด๋ผ๋ ํ์ผ์ด ์ ์ฅ๋ฉ๋๋ค.
๊ทธ๋ฐ ๋ค์ `from_pretrained` ๋ฉ์๋๋ฅผ ์ฌ์ฉํ์ฌ ๊ตฌ์ฑ์ ๋ค์ ๋ก๋ํ ์ ์์ต๋๋ค.
```py
resnet50d_config = ResnetConfig.from_pretrained("custom-resnet")
```
๊ตฌ์ฑ์ Hub์ ์ง์ ์
๋ก๋ํ๊ธฐ ์ํด [`PretrainedConfig`] ํด๋์ค์ [`~PretrainedConfig.push_to_hub`]์ ๊ฐ์ ๋ค๋ฅธ ๋ฉ์๋๋ฅผ ์ฌ์ฉํ ์ ์์ต๋๋ค.
## ์ฌ์ฉ์ ์ ์ ๋ชจ๋ธ ์์ฑํ๊ธฐ[[writing-a-custom-model]]
์ด์ ResNet ๊ตฌ์ฑ์ด ์์ผ๋ฏ๋ก ๋ชจ๋ธ์ ์์ฑํ ์ ์์ต๋๋ค.
์ค์ ๋ก๋ ๋ ๊ฐ๋ฅผ ์์ฑํ ๊ฒ์
๋๋ค. ํ๋๋ ์ด๋ฏธ์ง ๋ฐฐ์น์์ hidden features๋ฅผ ์ถ์ถํ๋ ๊ฒ([`BertModel`]๊ณผ ๊ฐ์ด), ๋ค๋ฅธ ํ๋๋ ์ด๋ฏธ์ง ๋ถ๋ฅ์ ์ ํฉํ ๊ฒ์
๋๋ค([`BertForSequenceClassification`]๊ณผ ๊ฐ์ด).
์ด์ ์ ์ธ๊ธํ๋ฏ์ด ์ด ์์ ์์๋ ๋จ์ํ๊ฒ ํ๊ธฐ ์ํด ๋ชจ๋ธ์ ๋์จํ ๋ํผ(loose wrapper)๋ง ์์ฑํ ๊ฒ์
๋๋ค.
์ด ํด๋์ค๋ฅผ ์์ฑํ๊ธฐ ์ ์ ๋ธ๋ก ์ ํ๊ณผ ์ค์ ๋ธ๋ก ํด๋์ค ๊ฐ์ ๋งคํ ์์
๋ง ํ๋ฉด ๋ฉ๋๋ค.
๊ทธ๋ฐ ๋ค์ `ResNet` ํด๋์ค๋ก ์ ๋ฌ๋์ด `configuration`์ ํตํด ๋ชจ๋ธ์ด ์ ์ธ๋ฉ๋๋ค:
```py
from transformers import PreTrainedModel
from timm.models.resnet import BasicBlock, Bottleneck, ResNet
from .configuration_resnet import ResnetConfig
BLOCK_MAPPING = {"basic": BasicBlock, "bottleneck": Bottleneck}
class ResnetModel(PreTrainedModel):
config_class = ResnetConfig
def __init__(self, config):
super().__init__(config)
block_layer = BLOCK_MAPPING[config.block_type]
self.model = ResNet(
block_layer,
config.layers,
num_classes=config.num_classes,
in_chans=config.input_channels,
cardinality=config.cardinality,
base_width=config.base_width,
stem_width=config.stem_width,
stem_type=config.stem_type,
avg_down=config.avg_down,
)
def forward(self, tensor):
return self.model.forward_features(tensor)
```
์ด๋ฏธ์ง ๋ถ๋ฅ ๋ชจ๋ธ์ ๋ง๋ค๊ธฐ ์ํด์๋ forward ๋ฉ์๋๋ง ๋ณ๊ฒฝํ๋ฉด ๋ฉ๋๋ค:
```py
import torch
class ResnetModelForImageClassification(PreTrainedModel):
config_class = ResnetConfig
def __init__(self, config):
super().__init__(config)
block_layer = BLOCK_MAPPING[config.block_type]
self.model = ResNet(
block_layer,
config.layers,
num_classes=config.num_classes,
in_chans=config.input_channels,
cardinality=config.cardinality,
base_width=config.base_width,
stem_width=config.stem_width,
stem_type=config.stem_type,
avg_down=config.avg_down,
)
def forward(self, tensor, labels=None):
logits = self.model(tensor)
if labels is not None:
loss = torch.nn.cross_entropy(logits, labels)
return {"loss": loss, "logits": logits}
return {"logits": logits}
```
๋ ๊ฒฝ์ฐ ๋ชจ๋ `PreTrainedModel`๋ฅผ ์์๋ฐ๊ณ , `config`๋ฅผ ํตํด ์์ ํด๋์ค ์ด๊ธฐํ๋ฅผ ํธ์ถํ๋ค๋ ์ ์ ๊ธฐ์ตํ์ธ์ (์ผ๋ฐ์ ์ธ `torch.nn.Module`์ ์์ฑํ ๋์ ๋น์ทํจ).
๋ชจ๋ธ์ auto ํด๋์ค์ ๋ฑ๋กํ๊ณ ์ถ์ ๊ฒฝ์ฐ์๋ `config_class`๋ฅผ ์ค์ ํ๋ ๋ถ๋ถ์ด ํ์์
๋๋ค (๋ง์ง๋ง ์น์
์ฐธ์กฐ).
<Tip>
๋ผ์ด๋ธ๋ฌ๋ฆฌ์ ์กด์ฌํ๋ ๋ชจ๋ธ๊ณผ ๊ต์ฅํ ์ ์ฌํ๋ค๋ฉด, ๋ชจ๋ธ์ ์์ฑํ ๋ ๊ตฌ์ฑ์ ์ฐธ์กฐํด ์ฌ์ฌ์ฉํ ์ ์์ต๋๋ค.
</Tip>
์ํ๋ ๊ฒ์ ๋ชจ๋ธ์ด ๋ฐํํ๋๋ก ํ ์ ์์ง๋ง, `ResnetModelForImageClassification`์์ ํ๋ ๊ฒ ์ฒ๋ผ
๋ ์ด๋ธ์ ํต๊ณผ์์ผฐ์ ๋ ์์ค๊ณผ ํจ๊ป ์ฌ์ ํํ๋ก ๋ฐํํ๋ ๊ฒ์ด [`Trainer`] ํด๋์ค ๋ด์์ ์ง์ ๋ชจ๋ธ์ ์ฌ์ฉํ๊ธฐ์ ์ ์ฉํฉ๋๋ค.
์์ ๋ง์ ํ์ต ๋ฃจํ ๋๋ ๋ค๋ฅธ ํ์ต ๋ผ์ด๋ธ๋ฌ๋ฆฌ๋ฅผ ์ฌ์ฉํ ๊ณํ์ด๋ผ๋ฉด ๋ค๋ฅธ ์ถ๋ ฅ ํ์์ ์ฌ์ฉํด๋ ์ข์ต๋๋ค.
์ด์ ๋ชจ๋ธ ํด๋์ค๊ฐ ์์ผ๋ฏ๋ก ํ๋ ์์ฑํด ๋ณด๊ฒ ์ต๋๋ค:
```py
resnet50d = ResnetModelForImageClassification(resnet50d_config)
```
๋ค์ ๋งํ์ง๋ง, [`~PreTrainedModel.save_pretrained`]๋๋ [`~PreTrainedModel.push_to_hub`]์ฒ๋ผ [`PreTrainedModel`]์ ์ํ๋ ๋ชจ๋ ๋ฉ์๋๋ฅผ ์ฌ์ฉํ ์ ์์ต๋๋ค.
๋ค์ ์น์
์์ ๋ ๋ฒ์งธ ๋ฉ์๋๋ฅผ ์ฌ์ฉํด ๋ชจ๋ธ ์ฝ๋์ ๋ชจ๋ธ ๊ฐ์ค์น๋ฅผ ์
๋ก๋ํ๋ ๋ฐฉ๋ฒ์ ์ดํด๋ณด๊ฒ ์ต๋๋ค.
๋จผ์ , ๋ชจ๋ธ ๋ด๋ถ์ ์ฌ์ ํ๋ จ๋ ๊ฐ์ค์น๋ฅผ ๋ก๋ํด ๋ณด๊ฒ ์ต๋๋ค.
์ด ์์ ๋ฅผ ํ์ฉํ ๋๋, ์ฌ์ฉ์ ์ ์ ๋ชจ๋ธ์ ์์ ๋ง์ ๋ฐ์ดํฐ๋ก ํ์ต์ํฌ ๊ฒ์
๋๋ค.
์ด ํํ ๋ฆฌ์ผ์์๋ ๋น ๋ฅด๊ฒ ์งํํ๊ธฐ ์ํด ์ฌ์ ํ๋ จ๋ resnet50d๋ฅผ ์ฌ์ฉํ๊ฒ ์ต๋๋ค.
์๋ ๋ชจ๋ธ์ resnet50d์ ๋ํผ์ด๊ธฐ ๋๋ฌธ์, ๊ฐ์ค์น๋ฅผ ์ฝ๊ฒ ๋ก๋ํ ์ ์์ต๋๋ค.
```py
import timm
pretrained_model = timm.create_model("resnet50d", pretrained=True)
resnet50d.model.load_state_dict(pretrained_model.state_dict())
```
์ด์ [`~PreTrainedModel.save_pretrained`] ๋๋ [`~PreTrainedModel.push_to_hub`]๋ฅผ ์ฌ์ฉํ ๋ ๋ชจ๋ธ ์ฝ๋๊ฐ ์ ์ฅ๋๋์ง ํ์ธํด๋ด
์๋ค.
## Hub๋ก ์ฝ๋ ์
๋ก๋ํ๊ธฐ[[sending-the-code-to-the-hub]]
<Tip warning={true}>
์ด API๋ ์คํ์ ์ด๋ฉฐ ๋ค์ ๋ฆด๋ฆฌ์ค์์ ์ฝ๊ฐ์ ๋ณ๊ฒฝ ์ฌํญ์ด ์์ ์ ์์ต๋๋ค.
</Tip>
๋จผ์ ๋ชจ๋ธ์ด `.py` ํ์ผ์ ์์ ํ ์ ์๋์ด ์๋์ง ํ์ธํ์ธ์.
๋ชจ๋ ํ์ผ์ด ๋์ผํ ์์
๊ฒฝ๋ก์ ์๊ธฐ ๋๋ฌธ์ ์๋๊ฒฝ๋ก ์ํฌํธ(relative import)์ ์์กดํ ์ ์์ต๋๋ค (transformers์์๋ ์ด ๊ธฐ๋ฅ์ ๋ํ ํ์ ๋ชจ๋์ ์ง์ํ์ง ์์ต๋๋ค).
์ด ์์์์๋ ์์
๊ฒฝ๋ก ์์ `resnet_model`์์ `modeling_resnet.py` ํ์ผ๊ณผ `configuration_resnet.py` ํ์ผ์ ์ ์ํฉ๋๋ค.
๊ตฌ์ฑ ํ์ผ์๋ `ResnetConfig`์ ๋ํ ์ฝ๋๊ฐ ์๊ณ ๋ชจ๋ธ๋ง ํ์ผ์๋ `ResnetModel` ๋ฐ `ResnetModelForImageClassification`์ ๋ํ ์ฝ๋๊ฐ ์์ต๋๋ค.
```
.
โโโ resnet_model
โโโ __init__.py
โโโ configuration_resnet.py
โโโ modeling_resnet.py
```
Python์ด `resnet_model`์ ๋ชจ๋๋ก ์ฌ์ฉํ ์ ์๋๋ก ๊ฐ์งํ๋ ๋ชฉ์ ์ด๊ธฐ ๋๋ฌธ์ `__init__.py`๋ ๋น์ด ์์ ์ ์์ต๋๋ค.
<Tip warning={true}>
๋ผ์ด๋ธ๋ฌ๋ฆฌ์์ ๋ชจ๋ธ๋ง ํ์ผ์ ๋ณต์ฌํ๋ ๊ฒฝ์ฐ,
๋ชจ๋ ํ์ผ ์๋จ์ ์๋ ์๋ ๊ฒฝ๋ก ์ํฌํธ(relative import) ๋ถ๋ถ์ `transformers` ํจํค์ง์์ ์ํฌํธ ํ๋๋ก ๋ณ๊ฒฝํด์ผ ํฉ๋๋ค.
</Tip>
๊ธฐ์กด ๊ตฌ์ฑ์ด๋ ๋ชจ๋ธ์ ์ฌ์ฌ์ฉ(๋๋ ์๋ธ ํด๋์คํ)ํ ์ ์์ต๋๋ค.
์ปค๋ฎค๋ํฐ์ ๋ชจ๋ธ์ ๊ณต์ ํ๊ธฐ ์ํด์๋ ๋ค์ ๋จ๊ณ๋ฅผ ๋ฐ๋ผ์ผ ํฉ๋๋ค:
๋จผ์ , ์๋ก ๋ง๋ ํ์ผ์ ResNet ๋ชจ๋ธ๊ณผ ๊ตฌ์ฑ์ ์ํฌํธํฉ๋๋ค:
```py
from resnet_model.configuration_resnet import ResnetConfig
from resnet_model.modeling_resnet import ResnetModel, ResnetModelForImageClassification
```
๋ค์์ผ๋ก `save_pretrained` ๋ฉ์๋๋ฅผ ์ฌ์ฉํด ํด๋น ๊ฐ์ฒด์ ์ฝ๋ ํ์ผ์ ๋ณต์ฌํ๊ณ ,
๋ณต์ฌํ ํ์ผ์ Auto ํด๋์ค๋ก ๋ฑ๋กํ๊ณ (๋ชจ๋ธ์ธ ๊ฒฝ์ฐ) ์คํํฉ๋๋ค:
```py
ResnetConfig.register_for_auto_class()
ResnetModel.register_for_auto_class("AutoModel")
ResnetModelForImageClassification.register_for_auto_class("AutoModelForImageClassification")
```
`configuration`์ ๋ํ auto ํด๋์ค๋ฅผ ์ง์ ํ ํ์๋ ์์ง๋ง(`configuration` ๊ด๋ จ auto ํด๋์ค๋ AutoConfig ํด๋์ค ํ๋๋ง ์์), ๋ชจ๋ธ์ ๊ฒฝ์ฐ์๋ ์ง์ ํด์ผ ํฉ๋๋ค.
์ฌ์ฉ์ ์ง์ ๋ชจ๋ธ์ ๋ค์ํ ์์
์ ์ ํฉํ ์ ์์ผ๋ฏ๋ก, ๋ชจ๋ธ์ ๋ง๋ auto ํด๋์ค๋ฅผ ์ง์ ํด์ผ ํฉ๋๋ค.
๋ค์์ผ๋ก, ์ด์ ์ ์์
ํ๋ ๊ฒ๊ณผ ๋ง์ฐฌ๊ฐ์ง๋ก ๊ตฌ์ฑ๊ณผ ๋ชจ๋ธ์ ์์ฑํฉ๋๋ค:
```py
resnet50d_config = ResnetConfig(block_type="bottleneck", stem_width=32, stem_type="deep", avg_down=True)
resnet50d = ResnetModelForImageClassification(resnet50d_config)
pretrained_model = timm.create_model("resnet50d", pretrained=True)
resnet50d.model.load_state_dict(pretrained_model.state_dict())
```
์ด์ ๋ชจ๋ธ์ Hub๋ก ์
๋ก๋ํ๊ธฐ ์ํด ๋ก๊ทธ์ธ ์ํ์ธ์ง ํ์ธํ์ธ์.
ํฐ๋ฏธ๋์์ ๋ค์ ์ฝ๋๋ฅผ ์คํํด ํ์ธํ ์ ์์ต๋๋ค:
```bash
huggingface-cli login
```
์ฃผํผํฐ ๋
ธํธ๋ถ์ ๊ฒฝ์ฐ์๋ ๋ค์๊ณผ ๊ฐ์ต๋๋ค:
```py
from huggingface_hub import notebook_login
notebook_login()
```
๊ทธ๋ฐ ๋ค์ ์ด๋ ๊ฒ ์์ ์ ๋ค์์คํ์ด์ค(๋๋ ์์ ์ด ์ํ ์กฐ์ง)์ ์
๋ก๋ํ ์ ์์ต๋๋ค:
```py
resnet50d.push_to_hub("custom-resnet50d")
```
On top of the modeling weights and the configuration in json format, this also copied the modeling and
configuration `.py` files in the folder `custom-resnet50d` and uploaded the result to the Hub. You can check the result
in this [model repo](https://huggingface.co/sgugger/custom-resnet50d).
json ํ์์ ๋ชจ๋ธ๋ง ๊ฐ์ค์น์ ๊ตฌ์ฑ ์ธ์๋ `custom-resnet50d` ํด๋ ์์ ๋ชจ๋ธ๋ง๊ณผ ๊ตฌ์ฑ `.py` ํ์ผ์ ๋ณต์ฌํํด Hub์ ์
๋ก๋ํฉ๋๋ค.
[๋ชจ๋ธ ์ ์ฅ์](https://huggingface.co/sgugger/custom-resnet50d)์์ ๊ฒฐ๊ณผ๋ฅผ ํ์ธํ ์ ์์ต๋๋ค.
[sharing tutorial](model_sharing) ๋ฌธ์์ `push_to_hub` ๋ฉ์๋์์ ์์ธํ ๋ด์ฉ์ ํ์ธํ ์ ์์ต๋๋ค.
## ์ฌ์ฉ์ ์ ์ ์ฝ๋๋ก ๋ชจ๋ธ ์ฌ์ฉํ๊ธฐ[[using-a-model-with-custom-code]]
auto ํด๋์ค์ `from_pretrained` ๋ฉ์๋๋ฅผ ์ฌ์ฉํ์ฌ ์ฌ์ฉ์ ์ง์ ์ฝ๋ ํ์ผ๊ณผ ํจ๊ป ๋ชจ๋ ๊ตฌ์ฑ, ๋ชจ๋ธ, ํ ํฌ๋์ด์ ๋ฅผ ์ฌ์ฉํ ์ ์์ต๋๋ค.
Hub์ ์
๋ก๋๋ ๋ชจ๋ ํ์ผ ๋ฐ ์ฝ๋๋ ๋ฉ์จ์ด๊ฐ ์๋์ง ๊ฒ์ฌ๋์ง๋ง (์์ธํ ๋ด์ฉ์ [Hub ๋ณด์](https://huggingface.co/docs/hub/security#malware-scanning) ์ค๋ช
์ฐธ์กฐ),
์์ ์ ์ปดํจํฐ์์ ๋ชจ๋ธ ์ฝ๋์ ์์ฑ์๊ฐ ์
์ฑ ์ฝ๋๋ฅผ ์คํํ์ง ์๋์ง ํ์ธํด์ผ ํฉ๋๋ค.
์ฌ์ฉ์ ์ ์ ์ฝ๋๋ก ๋ชจ๋ธ์ ์ฌ์ฉํ๋ ค๋ฉด `trust_remote_code=True`๋ก ์ค์ ํ์ธ์:
```py
from transformers import AutoModelForImageClassification
model = AutoModelForImageClassification.from_pretrained("sgugger/custom-resnet50d", trust_remote_code=True)
```
๋ชจ๋ธ ์์ฑ์๊ฐ ์
์์ ์ผ๋ก ์ฝ๋๋ฅผ ์
๋ฐ์ดํธํ์ง ์์๋ค๋ ์ ์ ํ์ธํ๊ธฐ ์ํด, ์ปค๋ฐ ํด์(commit hash)๋ฅผ `revision`์ผ๋ก ์ ๋ฌํ๋ ๊ฒ๋ ๊ฐ๋ ฅํ ๊ถ์ฅ๋ฉ๋๋ค (๋ชจ๋ธ ์์ฑ์๋ฅผ ์์ ํ ์ ๋ขฐํ์ง ์๋ ๊ฒฝ์ฐ).
```py
commit_hash = "ed94a7c6247d8aedce4647f00f20de6875b5b292"
model = AutoModelForImageClassification.from_pretrained(
"sgugger/custom-resnet50d", trust_remote_code=True, revision=commit_hash
)
```
Hub์์ ๋ชจ๋ธ ์ ์ฅ์์ ์ปค๋ฐ ๊ธฐ๋ก์ ์ฐพ์๋ณผ ๋, ๋ชจ๋ ์ปค๋ฐ์ ์ปค๋ฐ ํด์๋ฅผ ์ฝ๊ฒ ๋ณต์ฌํ ์ ์๋ ๋ฒํผ์ด ์์ต๋๋ค.
## ์ฌ์ฉ์ ์ ์ ์ฝ๋๋ก ๋ง๋ ๋ชจ๋ธ์ auto ํด๋์ค๋ก ๋ฑ๋กํ๊ธฐ[[registering-a-model-with-custom-code-to-the-auto-classes]]
๐ค Transformers๋ฅผ ์์ํ๋ ๋ผ์ด๋ธ๋ฌ๋ฆฌ๋ฅผ ์์ฑํ๋ ๊ฒฝ์ฐ ์ฌ์ฉ์ ์ ์ ๋ชจ๋ธ์ auto ํด๋์ค์ ์ถ๊ฐํ ์ ์์ต๋๋ค.
์ฌ์ฉ์ ์ ์ ๋ชจ๋ธ์ ์ฌ์ฉํ๊ธฐ ์ํด ํด๋น ๋ผ์ด๋ธ๋ฌ๋ฆฌ๋ฅผ ์ํฌํธํด์ผ ํ๊ธฐ ๋๋ฌธ์, ์ด๋ Hub๋ก ์ฝ๋๋ฅผ ์
๋ก๋ํ๋ ๊ฒ๊ณผ ๋ค๋ฆ
๋๋ค (Hub์์ ์๋์ ์ผ๋ก ๋ชจ๋ธ ์ฝ๋๋ฅผ ๋ค์ด๋ก๋ ํ๋ ๊ฒ๊ณผ ๋ฐ๋).
๊ตฌ์ฑ์ ๊ธฐ์กด ๋ชจ๋ธ ์ ํ๊ณผ ๋ค๋ฅธ `model_type` ์์ฑ์ด ์๊ณ ๋ชจ๋ธ ํด๋์ค์ ์ฌ๋ฐ๋ฅธ `config_class` ์์ฑ์ด ์๋ ํ,
๋ค์๊ณผ ๊ฐ์ด auto ํด๋์ค์ ์ถ๊ฐํ ์ ์์ต๋๋ค:
```py
from transformers import AutoConfig, AutoModel, AutoModelForImageClassification
AutoConfig.register("resnet", ResnetConfig)
AutoModel.register(ResnetConfig, ResnetModel)
AutoModelForImageClassification.register(ResnetConfig, ResnetModelForImageClassification)
```
์ฌ์ฉ์ ์ ์ ๊ตฌ์ฑ์ [`AutoConfig`]์ ๋ฑ๋กํ ๋ ์ฌ์ฉ๋๋ ์ฒซ ๋ฒ์งธ ์ธ์๋ ์ฌ์ฉ์ ์ ์ ๊ตฌ์ฑ์ `model_type`๊ณผ ์ผ์นํด์ผ ํฉ๋๋ค.
๋ํ, ์ฌ์ฉ์ ์ ์ ๋ชจ๋ธ์ auto ํด๋์ค์ ๋ฑ๋กํ ๋ ์ฌ์ฉ๋๋ ์ฒซ ๋ฒ์งธ ์ธ์๋ ํด๋น ๋ชจ๋ธ์ `config_class`์ ์ผ์นํด์ผ ํฉ๋๋ค. | 0 |
hf_public_repos/transformers/docs/source | hf_public_repos/transformers/docs/source/ko/perf_hardware.md | <!---
Copyright 2022 The HuggingFace Team. All rights reserved.
Licensed under the Apache License, Version 2.0 (the "License");
you may not use this file except in compliance with the License.
You may obtain a copy of the License at
http://www.apache.org/licenses/LICENSE-2.0
Unless required by applicable law or agreed to in writing, software
distributed under the License is distributed on an "AS IS" BASIS,
WITHOUT WARRANTIES OR CONDITIONS OF ANY KIND, either express or implied.
See the License for the specific language governing permissions and
limitations under the License.
โ ๏ธ Note that this file is in Markdown but contain specific syntax for our doc-builder (similar to MDX) that may not be
rendered properly in your Markdown viewer.
-->
# ํ๋ จ์ฉ ์ฌ์ฉ์ ๋ง์ถคํ ํ๋์จ์ด [[custom-hardware-for-training]]
๋ชจ๋ธ ํ๋ จ๊ณผ ์ถ๋ก ์ ์ฌ์ฉํ๋ ํ๋์จ์ด๋ ์ฑ๋ฅ์ ํฐ ์ํฅ์ ๋ฏธ์น ์ ์์ต๋๋ค. GPU์ ๋ํด ์์ธํ ์์๋ณด๋ ค๋ฉด, Tim Dettmer์ ํ๋ฅญํ ๋ธ๋ก๊ทธ ํฌ์คํธ๋ฅผ ํ์ธํด๋ณด์ธ์. [๋ธ๋ก๊ทธ ํฌ์คํธ ๋งํฌ](https://timdettmers.com/2020/09/07/which-gpu-for-deep-learning/) (์์ด๋ก ์์ฑ๋จ).
GPU ์ค์ ์ ๋ํ ์ค์ฉ์ ์ธ ์กฐ์ธ์ ์ดํด๋ณด๊ฒ ์ต๋๋ค.
## GPU [[gpu]]
๋ ํฐ ๋ชจ๋ธ์ ํ๋ จ์ํฌ ๋๋ ๊ธฐ๋ณธ์ ์ผ๋ก ์ธ ๊ฐ์ง ์ต์
์ด ์์ต๋๋ค:
- ๋ ํฐ GPU
- ๋ ๋ง์ GPU
- ๋ ๋ง์ CPU ๋ฐ NVMe ([DeepSpeed-Infinity](../en/main_classes/deepspeed#nvme-support)๋ฅผ ํตํ ์คํ๋ก๋(offload))
์ฐ์ , ํ๋์ GPU๋ง ์ฌ์ฉํ๋ ๊ฒฝ์ฐ๋ถํฐ ์์ํด๋ด
์๋ค.
### ์ ์ ๊ณต๊ธ๊ณผ ๋๊ฐ [[power-and-cooling]]
๋น์ผ ๊ณ ์ฑ๋ฅ GPU๋ฅผ ๊ตฌ๋งคํ ๊ฒฝ์ฐ, ์ฌ๋ฐ๋ฅธ ์ ์ ๊ณต๊ธ๊ณผ ์ถฉ๋ถํ ๋๊ฐ์ ์ ๊ณตํด์ผ ํฉ๋๋ค.
**์ ์ ๊ณต๊ธ**:
์ผ๋ถ ๊ณ ์ฑ๋ฅ ์๋น์์ฉ GPU๋ 2๊ฐ ํน์ ๊ฐ๋๊ฐ๋ค 3๊ฐ์ PCI-E 8ํ ์ ์ ์์ผ์ด ์์ต๋๋ค. ์นด๋์ ์๋ ์์ผ ์๋งํผ ๋
๋ฆฝ์ ์ธ 12V PCI-E 8ํ ์ผ์ด๋ธ์ด ์ฐ๊ฒฐ๋์ด ์๋์ง ํ์ธํ์ธ์. ๊ฐ์ ์ผ์ด๋ธ์ ํ์ชฝ ๋์ ์๋ 2๊ฐ์ ์คํ๋ฆฟ(๋๋ ํผ๊ทธํ
์ผ(pigtail) ์ผ์ด๋ธ)์ ์ฌ์ฉํ์ง ๋ง์ธ์. ์ฆ, GPU์ 2๊ฐ์ ์์ผ์ด ์๋ค๋ฉด, PSU(์ ์ ๊ณต๊ธ ์ฅ์น)์์ ์นด๋๋ก ์ฐ๊ฒฐ๋๋ 2๊ฐ์ PCI-E 8ํ ์ผ์ด๋ธ์ด ํ์ํ๋ฉฐ, ๋์ 2๊ฐ์ PCI-E 8ํ ์ปค๋ฅํฐ๊ฐ ์๋ ์ผ์ด๋ธ์ด ํ์ํ์ง ์์ต๋๋ค! ๊ทธ๋ ์ง ์์ผ๋ฉด ์นด๋์ ์ ์ฒด ์ฑ๋ฅ์ ์ ๋๋ก ๋ฐํํ์ง ๋ชปํ ์ ์์ต๋๋ค.
๊ฐ๊ฐ์ PCI-E 8ํ ์ ์ ์ผ์ด๋ธ์ PSU ์ชฝ์ 12V ๋ ์ผ์ ์ฐ๊ฒฐ๋์ด์ผ ํ๋ฉฐ ์ต๋ 150W์ ์ ๋ ฅ์ ๊ณต๊ธํ ์ ์์ต๋๋ค.
์ผ๋ถ ๋ค๋ฅธ GPU๋ PCI-E 12ํ ์ปค๋ฅํฐ๋ฅผ ์ฌ์ฉํ๋ฉฐ, ์ด๋ฌํ ์ปค๋ฅํฐ๋ ์ต๋ 500W-600W์ ์ ๋ ฅ์ ๊ณต๊ธํ ์ ์์ต๋๋ค.
์ ๊ฐํ GPU๋ 6ํ ์ปค๋ฅํฐ๋ฅผ ์ฌ์ฉํ๋ฉฐ, ์ต๋ 75W์ ์ ๋ ฅ์ ๊ณต๊ธํฉ๋๋ค.
๋ํ GPU๊ฐ ์์ ์ ์ธ ์ ์์ ๋ฐ์ ์ ์๋๋ก ๊ณ ๊ธ PSU๋ฅผ ์ ํํด์ผ ํฉ๋๋ค. ์ผ๋ถ ์ ํ์ง์ PSU๋ GPU๊ฐ ์ต๊ณ ์ฑ๋ฅ์ผ๋ก ๋์ํ๊ธฐ ์ํด ํ์ํ ์ ์์ ์์ ์ ์ผ๋ก ๊ณต๊ธํ์ง ๋ชปํ ์ ์์ต๋๋ค.
๋ฌผ๋ก , PSU๋ GPU์ ์ ์์ ๊ณต๊ธํ๊ธฐ์ ์ถฉ๋ถํ ์ฌ๋ถ์ ์ ๋ ฅ ์ฉ๋์ ๊ฐ์ ธ์ผ ํฉ๋๋ค.
**๋๊ฐ**:
GPU๊ฐ ๊ณผ์ด๋๋ฉด ์ฑ๋ฅ์ด ์ ํ๋๊ณ ์ต๋ ์ฑ๋ฅ์ ๋ฐํํ์ง ๋ชปํ ์ ์์ผ๋ฉฐ, ๋๋ฌด ๋จ๊ฑฐ์์ง๋ฉด ์ค์ง๋ ์ ์์ต๋๋ค.
GPU๊ฐ ๊ณผ์ด๋ ๋ ์ ํํ ์ ์ ์จ๋๋ฅผ ์๊ธฐ ์ด๋ ค์ฐ๋, ์๋ง๋ +80โ ๋ฏธ๋ง์ด๋ฉด ์ข์ง๋ง ๋ ๋ฎ์์๋ก ์ข์ต๋๋ค. 70โ-75โ ์ ๋๊ฐ ํ๋ฅญํ ์จ๋ ๋ฒ์์
๋๋ค. ์ฑ๋ฅ ์ ํ๊ฐ ๋ฐ์ํ๊ธฐ ์์ํ๋ ์จ๋๋ ๋๋ต 84โ-90โ ์ ๋์ผ ๊ฒ์
๋๋ค. ํ์ง๋ง ์ฑ๋ฅ ์ ํ ์ด์ธ์๋ ์ง์์ ์ผ๋ก ๋งค์ฐ ๋์ ์จ๋๋ GPU ์๋ช
์ ๋จ์ถ์ํฌ ์ ์์ต๋๋ค.
์ด์ด์, ์ฌ๋ฌ ๊ฐ์ GPU๋ฅผ ์ฌ์ฉํ ๋ ๊ฐ์ฅ ์ค์ํ ์ธก๋ฉด ์ค ํ๋์ธ GPU ๊ฐ ์ฐ๊ฒฐ ๋ฐฉ์์ ์ดํด๋ณด๊ฒ ์ต๋๋ค.
### ๋ค์ค GPU ์ฐ๊ฒฐ ๋ฐฉ์ [[multigpu-connectivity]]
๋ค์ค GPU๋ฅผ ์ฌ์ฉํ๋ ๊ฒฝ์ฐ GPU ๊ฐ์ ์ฐ๊ฒฐ ๋ฐฉ์์ ์ ์ฒด ํ๋ จ ์๊ฐ์ ํฐ ์ํฅ์ ๋ฏธ์น ์ ์์ต๋๋ค. ๋ง์ฝ GPU๊ฐ ๋์ผํ ๋ฌผ๋ฆฌ์ ๋
ธ๋์ ์์ ๊ฒฝ์ฐ, ๋ค์๊ณผ ๊ฐ์ด ํ์ธํ ์ ์์ต๋๋ค:
```
nvidia-smi topo -m
```
๋ง์ฝ NVLink๋ก ์ฐ๊ฒฐ๋ ๋์ผ GPU ํ๊ฒฝ์ด๋ผ๋ฉด, ๋ค์๊ณผ ๊ฐ์ ๊ฒฐ๊ณผ๋ฅผ ํ์ธํ ์ ์์ต๋๋ค:
```
GPU0 GPU1 CPU Affinity NUMA Affinity
GPU0 X NV2 0-23 N/A
GPU1 NV2 X 0-23 N/A
```
NVLink๋ฅผ ์ง์ํ์ง ์๋ ๋ค๋ฅธ ํ๊ฒฝ์ ๊ฒฝ์ฐ์๋ ๋ค์๊ณผ ๊ฐ์ ๊ฒฐ๊ณผ๋ฅผ ํ์ธํ ์ ์์ต๋๋ค:
```
GPU0 GPU1 CPU Affinity NUMA Affinity
GPU0 X PHB 0-11 N/A
GPU1 PHB X 0-11 N/A
```
์ด ๊ฒฐ๊ณผ์๋ ๋ค์๊ณผ ๊ฐ์ ๋ฒ๋ก๊ฐ ํฌํจ๋์ด ์์ต๋๋ค:
```
X = Self
SYS = Connection traversing PCIe as well as the SMP interconnect between NUMA nodes (e.g., QPI/UPI)
NODE = Connection traversing PCIe as well as the interconnect between PCIe Host Bridges within a NUMA node
PHB = Connection traversing PCIe as well as a PCIe Host Bridge (typically the CPU)
PXB = Connection traversing multiple PCIe bridges (without traversing the PCIe Host Bridge)
PIX = Connection traversing at most a single PCIe bridge
NV# = Connection traversing a bonded set of # NVLinks
```
๋ฐ๋ผ์ ์ฒซ ๋ฒ์งธ ๊ฒฐ๊ณผ์ `NV2`๋ GPU๊ฐ 2๊ฐ์ NVLink๋ก ์ฐ๊ฒฐ๋์ด ์๋ค๋ ๊ฒ์ ๋ํ๋ด๊ณ , ๋ ๋ฒ์งธ ๊ฒฐ๊ณผ์ `PHB`๋ ์ผ๋ฐ์ ์ธ ์๋น์์ฉ PCIe+๋ธ๋ฆฟ์ง ์ค์ ์ ๊ฐ์ง๊ณ ์๋ค๋ ๊ฒ์ ๋ํ๋
๋๋ค.
์ค์ ์์ ์ด๋ค ์ ํ์ ์ฐ๊ฒฐ ๋ฐฉ์์ ๊ฐ์ง๊ณ ์๋์ง ํ์ธํ์ธ์. ์ผ๋ถ ์ฐ๊ฒฐ ๋ฐฉ์์ GPU ๊ฐ ํต์ ์ ๋ ๋น ๋ฅด๊ฒ ๋ง๋ค ์ ์์ผ๋ฉฐ(NVLink์ ๊ฐ์ด), ์ด๋ค ์ฐ๊ฒฐ ๋ฐฉ์์ ๋ ๋๋ฆฌ๊ฒ ๋ง๋ค ์ ์์ต๋๋ค(PHB์ ๊ฐ์ด).
์ฌ์ฉํ๋ ํ์ฅ์ฑ ์๋ฃจ์
์ ์ข
๋ฅ์ ๋ฐ๋ผ ์ฐ๊ฒฐ ์๋๊ฐ ์ฃผ์ํ ์ํฅ์ ๋ฏธ์น ์๋ ์๊ณ ๋ฏธ๋ฏธํ ์ํฅ์ ๋ฏธ์น ์๋ ์์ต๋๋ค. DDP์ ๊ฐ์ด GPU๊ฐ ๊ฑฐ์ ๋๊ธฐํํ์ง ์์๋ ๋๋ ๊ฒฝ์ฐ, ์ฐ๊ฒฐ ์๋๊ฐ ๋๋ ค๋ ํฐ ์ํฅ์ ๋ฐ์ง ์์ต๋๋ค. ๋ฐ๋ฉด ZeRO-DP์ ๊ฐ์ด GPU๊ฐ ํต์ ์ด ๋ง์ด ํ์ํ ๊ฒฝ์ฐ, ๋ ๋น ๋ฅธ ํ๋ จ์ ์ํด์๋ ๋ ๋น ๋ฅธ ์ฐ๊ฒฐ ์๋๊ฐ ์ค์ํฉ๋๋ค.
#### NVLink [[nvlink]]
[NVLink](https://en.wikipedia.org/wiki/NVLink)๋ Nvidia์์ ๊ฐ๋ฐํ ์ ์ ๊ธฐ๋ฐ์ ์ง๋ ฌ ๋ค์ค ๋ ์ธ ๊ทผ๊ฑฐ๋ฆฌ ํต์ ๋งํฌ์
๋๋ค.
์๋ก์ด ์ธ๋์ NVLink๋ ๋ ๋น ๋ฅธ ๋์ญํญ์ ์ ๊ณตํฉ๋๋ค. [Nvidia Ampere GA102 GPU Architecture](https://www.nvidia.com/content/dam/en-zz/Solutions/geforce/ampere/pdf/NVIDIA-ampere-GA102-GPU-Architecture-Whitepaper-V1.pdf)์์ ์๋์ ๊ฐ์ ์ ๋ณด๋ฅผ ํ์ธํ์ค ์ ์์ต๋๋ค:
> 3์ธ๋ NVLinkยฎ
> GA102 GPU๋ 4๊ฐ์ x4 ๋งํฌ๋ฅผ ํฌํจํ๋ NVIDIA์ 3์ธ๋ NVLink ์ธํฐํ์ด์ค๋ฅผ ํ์ฉํ๋ฉฐ,
> ๊ฐ ๋งํฌ๋ ๋ ๊ฐ์ GPU ๊ฐ์ ๊ฐ ๋ฐฉํฅ์ผ๋ก ์ด๋น 14.0625GB์ ๋์ญํญ์ ์ ๊ณตํฉ๋๋ค.
> 4๊ฐ์ ๋งํฌ๋ ๊ฐ ๋ฐฉํฅ์ ์ด๋น 56.25GB์ ๋์ญํญ์ ์ ๊ณตํ๋ฉฐ, ๋ ๊ฐ์ GPU ๊ฐ์๋ ์ด๋น 112.5GB์ ์ด ๋์ญํญ์ ์ ๊ณตํฉ๋๋ค.
> ๋ ๊ฐ์ RTX 3090 GPU๋ฅผ NVLink๋ฅผ ์ฌ์ฉํด SLI๋ก ์ฐ๊ฒฐํ ์ ์์ต๋๋ค.
> (3-Way ๋ฐ 4-Way SLI ๊ตฌ์ฑ์ ์ง์๋์ง ์์์ ์ ์ํ์ธ์.)
๋ฐ๋ผ์ `nvidia-smi topo -m`์ ๊ฒฐ๊ณผ์์ `NVX`์ ๊ฐ์ด ๋์์๋ก ๋ ์ข์ต๋๋ค. ์ธ๋๋ GPU ์ํคํ
์ฒ์ ๋ฐ๋ผ ๋ค๋ฅผ ์ ์์ต๋๋ค.
๊ทธ๋ ๋ค๋ฉด, gpt2๋ฅผ ์์ wikitext ์ํ๋ก ํ์ต์ํค๋ ์์ ๋ฅผ ํตํด, NVLink๊ฐ ํ๋ จ์ ์ด๋ค ์ํฅ์ ๋ฏธ์น๋์ง ์ดํด๋ณด๊ฒ ์ต๋๋ค.
๊ฒฐ๊ณผ๋ ๋ค์๊ณผ ๊ฐ์ต๋๋ค:
| NVlink | Time |
| ----- | ---: |
| Y | 101s |
| N | 131s |
NVLink ์ฌ์ฉ ์ ํ๋ จ์ด ์ฝ 23% ๋ ๋น ๋ฅด๊ฒ ์๋ฃ๋จ์ ํ์ธํ ์ ์์ต๋๋ค. ๋ ๋ฒ์งธ ๋ฒค์น๋งํฌ์์๋ `NCCL_P2P_DISABLE=1`์ ์ฌ์ฉํ์ฌ NVLink๋ฅผ ์ฌ์ฉํ์ง ์๋๋ก ์ค์ ํ์ต๋๋ค.
์ ์ฒด ๋ฒค์น๋งํฌ ์ฝ๋์ ๊ฒฐ๊ณผ๋ ๋ค์๊ณผ ๊ฐ์ต๋๋ค:
```bash
# DDP w/ NVLink
rm -r /tmp/test-clm; CUDA_VISIBLE_DEVICES=0,1 python -m torch.distributed.launch \
--nproc_per_node 2 examples/pytorch/language-modeling/run_clm.py --model_name_or_path gpt2 \
--dataset_name wikitext --dataset_config_name wikitext-2-raw-v1 --do_train \
--output_dir /tmp/test-clm --per_device_train_batch_size 4 --max_steps 200
{'train_runtime': 101.9003, 'train_samples_per_second': 1.963, 'epoch': 0.69}
# DDP w/o NVLink
rm -r /tmp/test-clm; CUDA_VISIBLE_DEVICES=0,1 NCCL_P2P_DISABLE=1 python -m torch.distributed.launch \
--nproc_per_node 2 examples/pytorch/language-modeling/run_clm.py --model_name_or_path gpt2 \
--dataset_name wikitext --dataset_config_name wikitext-2-raw-v1 --do_train
--output_dir /tmp/test-clm --per_device_train_batch_size 4 --max_steps 200
{'train_runtime': 131.4367, 'train_samples_per_second': 1.522, 'epoch': 0.69}
```
ํ๋์จ์ด: ๊ฐ๊ฐ 2๊ฐ์ TITAN RTX 24GB + 2๊ฐ์ NVLink (`NV2` in `nvidia-smi topo -m`)
์ํํธ์จ์ด: `pytorch-1.8-to-be` + `cuda-11.0` / `transformers==4.3.0.dev0`
| 0 |
hf_public_repos/transformers/docs/source | hf_public_repos/transformers/docs/source/ko/pad_truncation.md | <!--Copyright 2022 The HuggingFace Team. All rights reserved.
Licensed under the Apache License, Version 2.0 (the "License"); you may not use this file except in compliance with
the License. You may obtain a copy of the License at
http://www.apache.org/licenses/LICENSE-2.0
Unless required by applicable law or agreed to in writing, software distributed under the License is distributed on
an "AS IS" BASIS, WITHOUT WARRANTIES OR CONDITIONS OF ANY KIND, either express or implied. See the License for the
specific language governing permissions and limitations under the License.
โ ๏ธ Note that this file is in Markdown but contain specific syntax for our doc-builder (similar to MDX) that may not be
rendered properly in your Markdown viewer.
-->
# ํจ๋ฉ๊ณผ ์๋ผ๋ด๊ธฐ[[padding-and-truncation]]
๋ฐฐ์น ์
๋ ฅ์ ๊ธธ์ด๊ฐ ๋ค๋ฅธ ๊ฒฝ์ฐ๊ฐ ๋ง์์ ๊ณ ์ ํฌ๊ธฐ ํ
์๋ก ๋ณํํ ์ ์์ต๋๋ค. ํจ๋ฉ๊ณผ ์๋ผ๋ด๊ธฐ๋ ๋ค์ํ ๊ธธ์ด์ ๋ฐฐ์น์์ ์ง์ฌ๊ฐํ ํ
์๋ฅผ ์์ฑํ ์ ์๋๋ก ์ด ๋ฌธ์ ๋ฅผ ํด๊ฒฐํ๋ ์ ๋ต์
๋๋ค. ํจ๋ฉ์ ํน์ํ **ํจ๋ฉ ํ ํฐ**์ ์ถ๊ฐํ์ฌ ์งง์ ์ํ์ค๊ฐ ๋ฐฐ์น์์ ๊ฐ์ฅ ๊ธด ์ํ์ค ๋๋ ๋ชจ๋ธ์์ ํ์ฉํ๋ ์ต๋ ๊ธธ์ด์ ๋์ผํ ๊ธธ์ด๋ฅผ ๊ฐ๋๋ก ํฉ๋๋ค. ์๋ผ๋ด๊ธฐ๋ ๊ธด ์ํ์ค๋ฅผ ์๋ผ๋ด์ด ํจ๋ฉ๊ณผ ๋ค๋ฅธ ๋ฐฉ์์ผ๋ก ์ํ์ค์ ๊ธธ์ด๋ฅผ ๋์ผํ๊ฒ ํฉ๋๋ค.
๋๋ถ๋ถ์ ๊ฒฝ์ฐ ๋ฐฐ์น์ ๊ฐ์ฅ ๊ธด ์ํ์ค์ ๊ธธ์ด๋ก ํจ๋ฉํ๊ณ ๋ชจ๋ธ์ด ํ์ฉํ ์ ์๋ ์ต๋ ๊ธธ์ด๋ก ์๋ผ๋ด๋ ๊ฒ์ด ์ ์๋ํฉ๋๋ค. ๊ทธ๋ฌ๋ ํ์ํ๋ค๋ฉด API๊ฐ ์ง์ํ๋ ๋ ๋ง์ ์ ๋ต์ ์ฌ์ฉํ ์ ์์ต๋๋ค. ํ์ํ ์ธ์๋ `padding`, `truncation`, `max_length` ์ธ ๊ฐ์ง์
๋๋ค.
`padding` ์ธ์๋ ํจ๋ฉ์ ์ ์ดํฉ๋๋ค. ๋ถ๋ฆฌ์ธ ๋๋ ๋ฌธ์์ด์ผ ์ ์์ต๋๋ค:
- `True` ๋๋ `'longest'`: ๋ฐฐ์น์์ ๊ฐ์ฅ ๊ธด ์ํ์ค๋ก ํจ๋ฉํฉ๋๋ค(๋จ์ผ ์ํ์ค๋ง ์ ๊ณตํ๋ ๊ฒฝ์ฐ ํจ๋ฉ์ด ์ ์ฉ๋์ง ์์ต๋๋ค).
- `'max_length'`: `max_length` ์ธ์๊ฐ ์ง์ ํ ๊ธธ์ด๋ก ํจ๋ฉํ๊ฑฐ๋, `max_length`๊ฐ ์ ๊ณต๋์ง ์์ ๊ฒฝ์ฐ(`max_length=None`) ๋ชจ๋ธ์์ ํ์ฉ๋๋ ์ต๋ ๊ธธ์ด๋ก ํจ๋ฉํฉ๋๋ค. ๋จ์ผ ์ํ์ค๋ง ์ ๊ณตํ๋ ๊ฒฝ์ฐ์๋ ํจ๋ฉ์ด ์ ์ฉ๋ฉ๋๋ค.
- `False` ๋๋ `'do_not_pad'`: ํจ๋ฉ์ด ์ ์ฉ๋์ง ์์ต๋๋ค. ์ด๊ฒ์ด ๊ธฐ๋ณธ ๋์์
๋๋ค.
`truncation` ์ธ์๋ ์๋ผ๋ผ ๋ฐฉ๋ฒ์ ์ ํฉ๋๋ค. ๋ถ๋ฆฌ์ธ ๋๋ ๋ฌธ์์ด์ผ ์ ์์ต๋๋ค:
- `True` ๋๋ `longest_first`: `max_length` ์ธ์๊ฐ ์ง์ ํ ์ต๋ ๊ธธ์ด๋ก ์๋ผ๋ด๊ฑฐ๋,
`max_length`๊ฐ ์ ๊ณต๋์ง ์์ ๊ฒฝ์ฐ(`max_length=None`) ๋ชจ๋ธ์์ ํ์ฉ๋๋ ์ต๋ ๊ธธ์ด๋ก ์๋ผ๋
๋๋ค.
์ํ์ค ์์์ ๊ฐ์ฅ ๊ธด ์ํ์ค์ ํ ํฐ์ ์ ์ ํ ๊ธธ์ด์ ๋๋ฌํ ๋๊น์ง ํ๋์ฉ ์ ๊ฑฐํฉ๋๋ค.
- `'only_second'`: `max_length` ์ธ์๊ฐ ์ง์ ํ ์ต๋ ๊ธธ์ด๋ก ์๋ผ๋ด๊ฑฐ๋,
`max_length`๊ฐ ์ ๊ณต๋์ง ์์ ๊ฒฝ์ฐ(`max_length=None`) ๋ชจ๋ธ์์ ํ์ฉ๋๋ ์ต๋ ๊ธธ์ด๋ก ์๋ผ๋
๋๋ค.
์ํ์ค ์(๋๋ ์ํ์ค ์์ ๋ฐฐ์น)๊ฐ ์ ๊ณต๋ ๊ฒฝ์ฐ ์์ ๋ ๋ฒ์งธ ๋ฌธ์ฅ๋ง ์๋ผ๋
๋๋ค.
- `'only_first'`: `max_length` ์ธ์๊ฐ ์ง์ ํ ์ต๋ ๊ธธ์ด๋ก ์๋ผ๋ด๊ฑฐ๋,
`max_length`๊ฐ ์ ๊ณต๋์ง ์์ ๊ฒฝ์ฐ(`max_length=None`) ๋ชจ๋ธ์์ ํ์ฉ๋๋ ์ต๋ ๊ธธ์ด๋ก ์๋ผ๋
๋๋ค.
์ํ์ค ์(๋๋ ์ํ์ค ์์ ๋ฐฐ์น)๊ฐ ์ ๊ณต๋ ๊ฒฝ์ฐ ์์ ์ฒซ ๋ฒ์งธ ๋ฌธ์ฅ๋ง ์๋ผ๋
๋๋ค.
- `False` ๋๋ `'do_not_truncate'`: ์๋ผ๋ด๊ธฐ๋ฅผ ์ ์ฉํ์ง ์์ต๋๋ค. ์ด๊ฒ์ด ๊ธฐ๋ณธ ๋์์
๋๋ค.
`max_length` ์ธ์๋ ํจ๋ฉ ๋ฐ ์๋ผ๋ด๊ธฐ๋ฅผ ์ ์ฉํ ๊ธธ์ด๋ฅผ ์ ์ดํฉ๋๋ค. ์ด ์ธ์๋ ์ ์ ๋๋ `None`์ผ ์ ์์ผ๋ฉฐ, `None`์ผ ๊ฒฝ์ฐ ๋ชจ๋ธ์ด ํ์ฉํ ์ ์๋ ์ต๋ ๊ธธ์ด๋ก ๊ธฐ๋ณธ๊ฐ์ด ์ค์ ๋ฉ๋๋ค. ๋ชจ๋ธ์ ํน์ ํ ์ต๋ ์
๋ ฅ ๊ธธ์ด๊ฐ ์๋ ๊ฒฝ์ฐ `max_length`์ ๋ํ ์๋ผ๋ด๊ธฐ ๋๋ ํจ๋ฉ์ด ๋นํ์ฑํ๋ฉ๋๋ค.
๋ค์ ํ์๋ ํจ๋ฉ ๋ฐ ์๋ผ๋ด๊ธฐ๋ฅผ ์ค์ ํ๋ ๊ถ์ฅ ๋ฐฉ๋ฒ์ด ์์ฝ๋์ด ์์ต๋๋ค.
์
๋ ฅ์ผ๋ก ์ํ์ค ์์ ์ฌ์ฉํ๋ ๊ฒฝ์ฐ, ๋ค์ ์์ ์์ `truncation=True`๋ฅผ `['only_first', 'only_second', 'longest_first']`์์ ์ ํํ `STRATEGY`, ์ฆ `truncation='only_second'` ๋๋ `truncation='longest_first'`๋ก ๋ฐ๊พธ๋ฉด ์์ ์ค๋ช
ํ ๋๋ก ์์ ๋ ์ํ์ค๊ฐ ์๋ฆฌ๋ ๋ฐฉ์์ ์ ์ดํ ์ ์์ต๋๋ค.
| ์๋ผ๋ด๊ธฐ | ํจ๋ฉ | ์ฌ์ฉ ๋ฐฉ๋ฒ |
|--------------------------------------|-----------------------------------|------------------------------------------------------------------------------------------|
| ์๋ผ๋ด๊ธฐ ์์ | ํจ๋ฉ ์์ | `tokenizer(batch_sentences)` |
| | ๋ฐฐ์น ๋ด ์ต๋ ๊ธธ์ด๋ก ํจ๋ฉ | `tokenizer(batch_sentences, padding=True)` ๋๋ |
| | | `tokenizer(batch_sentences, padding='longest')` |
| | ๋ชจ๋ธ์ ์ต๋ ์
๋ ฅ ๊ธธ์ด๋ก ํจ๋ฉ | `tokenizer(batch_sentences, padding='max_length')` |
| | ํน์ ๊ธธ์ด๋ก ํจ๋ฉ | `tokenizer(batch_sentences, padding='max_length', max_length=42)` |
| | ๋ค์ํ ๊ธธ์ด๋ก ํจ๋ฉ | `tokenizer(batch_sentences, padding=True, pad_to_multiple_of=8) |
| ๋ชจ๋ธ์ ์ต๋ ์
๋ ฅ ๊ธธ์ด๋ก ์๋ผ๋ด๊ธฐ | ํจ๋ฉ ์์ | `tokenizer(batch_sentences, truncation=True)` ๋๋ |
| | | `tokenizer(batch_sentences, truncation=STRATEGY)` |
| | ๋ฐฐ์น ๋ด ์ต๋ ๊ธธ์ด๋ก ํจ๋ฉ | `tokenizer(batch_sentences, padding=True, truncation=True)` ๋๋ |
| | | `tokenizer(batch_sentences, padding=True, truncation=STRATEGY)` |
| | ๋ชจ๋ธ์ ์ต๋ ์
๋ ฅ ๊ธธ์ด๋ก ํจ๋ฉ | `tokenizer(batch_sentences, padding='max_length', truncation=True)` ๋๋ |
| | | `tokenizer(batch_sentences, padding='max_length', truncation=STRATEGY)` |
| | ํน์ ๊ธธ์ด๋ก ํจ๋ฉ | ์ฌ์ฉ ๋ถ๊ฐ |
| ํน์ ๊ธธ์ด๋ก ์๋ผ๋ด๊ธฐ | ํจ๋ฉ ์์ | `tokenizer(batch_sentences, truncation=True, max_length=42)` ๋๋ |
| | | `tokenizer(batch_sentences, truncation=STRATEGY, max_length=42)` |
| | ๋ฐฐ์น ๋ด ์ต๋ ๊ธธ์ด๋ก ํจ๋ฉ | `tokenizer(batch_sentences, padding=True, truncation=True, max_length=42)` ๋๋ |
| | | `tokenizer(batch_sentences, padding=True, truncation=STRATEGY, max_length=42)` |
| | ๋ชจ๋ธ์ ์ต๋ ์
๋ ฅ ๊ธธ์ด๋ก ํจ๋ฉ | ์ฌ์ฉ ๋ถ๊ฐ |
| | ํน์ ๊ธธ์ด๋ก ํจ๋ฉ | `tokenizer(batch_sentences, padding='max_length', truncation=True, max_length=42)` ๋๋ |
| | | `tokenizer(batch_sentences, padding='max_length', truncation=STRATEGY, max_length=42)` |
| 0 |
hf_public_repos/transformers/docs/source | hf_public_repos/transformers/docs/source/ko/attention.md | <!--Copyright 2023 The HuggingFace Team. All rights reserved.
Licensed under the Apache License, Version 2.0 (the "License"); you may not use this file except in compliance with
the License. You may obtain a copy of the License at
http://www.apache.org/licenses/LICENSE-2.0
Unless required by applicable law or agreed to in writing, software distributed under the License is distributed on
an "AS IS" BASIS, WITHOUT WARRANTIES OR CONDITIONS OF ANY KIND, either express or implied. See the License for the
specific language governing permissions and limitations under the License.
โ ๏ธ Note that this file is in Markdown but contain specific syntax for our doc-builder (similar to MDX) that may not be
rendered properly in your Markdown viewer.
-->
# ์ดํ
์
๋ฉ์ปค๋์ฆ[[attention_mechanisms]]
๋๋ถ๋ถ์ ํธ๋์คํฌ๋จธ ๋ชจ๋ธ์ ์ ๋ฐฉํ๋ ฌ์ธ ์ ์ฒด ์ดํ
์
์ ์ฌ์ฉํฉ๋๋ค.
ํ์ง๋ง ์ด๋ ๊ธด ํ
์คํธ๋ฅผ ๋ค๋ฃฐ ๋๋ ํฐ ๊ณ์ฐ ๋ณ๋ชฉ ํ์์ ์ ๋ฐํ ์ ์์ต๋๋ค.
`Longformer`์ `Reformer`๋ ํ๋ จ ์๋๋ฅผ ๋์ด๊ธฐ ์ํด ์ดํ
์
ํ๋ ฌ์ ํฌ์ ๋ฒ์ ์ ์ฌ์ฉํ์ฌ ํจ์จ์ ๋์ด๋ ค๋ ๋ชจ๋ธ์
๋๋ค.
## LSH ์ดํ
์
[[lsh_attention]]
[Reformer](#reformer)๋ LSH(Locality Sensitive Hashing) ์ดํ
์
์ ์ฌ์ฉํฉ๋๋ค. softmax(QK^t)์์๋ ํ๋ ฌ QK^t์ (softmax ์ฐจ์์์) ๊ฐ์ฅ ํฐ ์์๋ค๋ง ์ ์ฉํ ๊ธฐ์ฌ๋ฅผ ํ ๊ฒ์
๋๋ค.
๋ฐ๋ผ์ ๊ฐ๊ฐ์ ์ฟผ๋ฆฌ q์ ๋ํด, q์ ๊ฐ๊น์ด ํค k๋ง ๊ณ ๋ คํ ์ ์์ต๋๋ค. ํด์ ํจ์๋ q์ k๊ฐ ๊ฐ๊น์ด์ง ์ฌ๋ถ๋ฅผ ๊ฒฐ์ ํ๋ ๋ฐ ์ฌ์ฉ๋ฉ๋๋ค.
์ดํ
์
๋ง์คํฌ๋ ํ์ฌ ํ ํฐ์ ๋ง์คํนํ์ฌ ๋ณ๊ฒฝ๋ฉ๋๋ค. ์ด ๋ ์ฒซ ๋ฒ์งธ ์์น์ ํ ํฐ์ ์ ์ธํฉ๋๋ค. ์๋ํ๋ฉด ์ฟผ๋ฆฌ์ ํค๊ฐ ๋์ผํ ๊ฐ์ ๊ฐ๊ฒ ๋๊ธฐ ๋๋ฌธ์
๋๋ค(์๋ก ๋งค์ฐ ์ ์ฌํจ).
ํด์๋ ์ฝ๊ฐ์ ๋ฌด์์์ฑ์ ๊ฐ์ง ์ ์์ผ๋ฏ๋ก, ์ค์ ๋ก๋ ์ฌ๋ฌ ๊ฐ์ ํด์ ํจ์๊ฐ ์ฌ์ฉ๋๊ณ (`n_rounds` ๋งค๊ฐ๋ณ์์ ์ํด ๊ฒฐ์ ๋จ) ๊ทธ ํ์ ํ๊ท ๊ฐ์ ์ทจํ๊ฒ ๋ฉ๋๋ค.
## ์ง์ญ ์ดํ
์
[[local_attention]]
[Longformer](#longformer)๋ ์ง์ญ ์ดํ
์
์ ์ฌ์ฉํฉ๋๋ค. ์ข
์ข
ํน์ ํ ํฐ์ ๋ํด ์ง์ญ ์ปจํ
์คํธ(์: ์ผ์ชฝ๊ณผ ์ค๋ฅธ์ชฝ์ ์๋ ๋ ๊ฐ์ ํ ํฐ์ ๋ฌด์์ธ๊ฐ์?)๋ง์ผ๋ก๋ ์์
์ ์ํํ๋๋ฐ ์ถฉ๋ถํฉ๋๋ค.
๋ํ ์์ ์ฐฝ(window)์ ๊ฐ์ง ์ดํ
์
๋ ์ด์ด๋ฅผ ์์์ผ๋ก์จ ๋ง์ง๋ง ๋ ์ด์ด๋ ์ฐฝ ๋ด์ ํ ํฐ๋ฟ๋ง ์๋๋ผ ๋ ๋ง์ ์์ ํ ํฐ์ ๋ํ ์์ฉ ์์ญ(receptive field)์ ๊ฐ๊ฒ ๋์ด ์ ์ฒด ๋ฌธ์ฅ์ ํํ์ ๊ตฌ์ถํ ์ ์์ต๋๋ค.
์ฌ์ ์ ์ ํ๋ ์ผ๋ถ ์
๋ ฅ ํ ํฐ๋ค์ ์ ์ญ ์ดํ
์
์ ๋ฐ์ต๋๋ค. ์ด ๋ช ๊ฐ์ ํ ํฐ์ ๋ํด์๋ ์ดํ
์
ํ๋ ฌ์ด ๋ชจ๋ ํ ํฐ์ ์ ๊ทผํ ์ ์์ผ๋ฉฐ, ์ด ๊ณผ์ ์ ๋์นญ์ ์ผ๋ก ์ด๋ฃจ์ด์ง๋๋ค.
๋ค๋ฅธ ๋ชจ๋ ํ ํฐ๋ค์ ๋ก์ปฌ ์ฐฝ ๋ด์ ํ ํฐ๋ค์ ๋ํด ํด๋น ํน์ ํ ํฐ๋ค์๋ ์ ๊ทผํ ์ ์์ต๋๋ค. ์ด๋ ๋
ผ๋ฌธ์ Figure 2d์์ ๋ํ๋๋ฉฐ, ์๋์ ์ํ ์ดํ
์
๋ง์คํฌ๊ฐ ์ ์๋์ด ์์ต๋๋ค:
<div class="flex justify-center">
<img scale="50 %" align="center" src="https://huggingface.co/datasets/huggingface/documentation-images/resolve/main/local_attention_mask.png"/>
</div>
์ ์ ํ๋ผ๋ฏธํฐ์ ์ดํ
์
ํ๋ ฌ์ ์ฌ์ฉํ๋ฉด ๋ชจ๋ธ์ด ๋ ํฐ ์ํ์ค ์
๋ ฅ ๊ธธ์ด๋ฅผ ๊ฐ์ง ์ ์์ต๋๋ค.
## ๋ค๋ฅธ ๋ฐฉ๋ฒ๋ค[[other_tricks]]
### ์ถ๋ณ ์์น ์ธ์ฝ๋ฉ[[axial_positional_encodings]]
[Reformer](#reformer)๋ ์ถ๋ณ ์์น ์ธ์ฝ๋ฉ(axial positional encodings)์ ์ฌ์ฉํฉ๋๋ค. ๊ธฐ์กด์ ํธ๋์คํฌ๋จธ ๋ชจ๋ธ์์๋ ์์น ์ธ์ฝ๋ฉ ํ๋ ฌ E๋ ํฌ๊ธฐ๊ฐ \\(l \times d\\)์ธ ํ๋ ฌ์ด๋ฉฐ,
์ฌ๊ธฐ์ \\(l\\)์ ์ํ์ค ๊ธธ์ด(sequence length)์ด๊ณ \\(d\\)๋ ์จ๊ฒจ์ง ์ํ(hidden state)์ ์ฐจ์์
๋๋ค. ๋งค์ฐ ๊ธด ํ
์คํธ์ ๊ฒฝ์ฐ, ์ด ํ๋ ฌ์ ๋งค์ฐ ํฌ๋ฉฐ GPU ์์์ ๊ณต๊ฐ์ ๋ง์ด ์ฐจ์งํ ์ ์์ต๋๋ค.
์ด๋ฅผ ์ํํ๊ธฐ ์ํด, ์ถ๋ณ ์์น ์ธ์ฝ๋ฉ์ ํฐ ํ๋ ฌ E๋ฅผ ๋ ๊ฐ์ ์์ ํ๋ ฌ E1๊ณผ E2๋ก ๋ถํดํฉ๋๋ค. ์ด๋ E1์ ํฌ๊ธฐ๋ \\(l_{1} \times d_{1}\\)์ด๊ณ , E2์ ํฌ๊ธฐ๋ \\(l_{2} \times d_{2}\\)์
๋๋ค.
์ด๋ \\(l_{1} \times l_{2} = l\\)์ด๊ณ \\(d_{1} + d_{2} = d\\)(๊ธธ์ด์ ๋ํ ๊ณฑ์
์ฐ์ฐ์ ์ฌ์ฉํ๋ฉด ํจ์ฌ ์์์ง๋๋ค). E์ ์๊ฐ ๋จ๊ณ j์ ๋ํ ์๋ฒ ๋ฉ์ E1์์ ์๊ฐ ๋จ๊ณ \\(j \% l1\\)์ ์๋ฒ ๋ฉ๊ณผ E2์์ ์๊ฐ ๋จ๊ณ \\(j // l1\\)์ ์๋ฒ ๋ฉ์ ์ฐ๊ฒฐํ์ฌ ์ป์ต๋๋ค. | 0 |
hf_public_repos/transformers/docs/source | hf_public_repos/transformers/docs/source/ko/quicktour.md | <!--Copyright 2022 The HuggingFace Team. All rights reserved.
Licensed under the Apache License, Version 2.0 (the "License"); you may not use this file except in compliance with
the License. You may obtain a copy of the License at
http://www.apache.org/licenses/LICENSE-2.0
Unless required by applicable law or agreed to in writing, software distributed under the License is distributed on
an "AS IS" BASIS, WITHOUT WARRANTIES OR CONDITIONS OF ANY KIND, either express or implied. See the License for the
specific language governing permissions and limitations under the License.
โ ๏ธ Note that this file is in Markdown but contain specific syntax for our doc-builder (similar to MDX) that may not be
rendered properly in your Markdown viewer.
-->
# ๋๋ฌ๋ณด๊ธฐ [[quick-tour]]
[[open-in-colab]]
๐ค Transformers๋ฅผ ์์ํด๋ณด์ธ์! ๊ฐ๋ฐํด๋ณธ ์ ์ด ์๋๋ผ๋ ์ฝ๊ฒ ์ฝ์ ์ ์๋๋ก ์ฐ์ธ ์ด ๊ธ์ [`pipeline`](./main_classes/pipelines)์ ์ฌ์ฉํ์ฌ ์ถ๋ก ํ๊ณ , ์ฌ์ ํ์ต๋ ๋ชจ๋ธ๊ณผ ์ ์ฒ๋ฆฌ๊ธฐ๋ฅผ [AutoClass](./model_doc/auto)๋ก ๋ก๋ํ๊ณ , PyTorch ๋๋ TensorFlow๋ก ๋ชจ๋ธ์ ๋น ๋ฅด๊ฒ ํ์ต์ํค๋ ๋ฐฉ๋ฒ์ ์๊ฐํด ๋๋ฆด ๊ฒ์
๋๋ค. ๋ณธ ๊ฐ์ด๋์์ ์๊ฐ๋๋ ๊ฐ๋
์ (ํนํ ์ด๋ณด์์ ๊ด์ ์ผ๋ก) ๋ ์น์ ํ๊ฒ ์ ํ๊ณ ์ถ๋ค๋ฉด, ํํ ๋ฆฌ์ผ์ด๋ [์ฝ์ค](https://huggingface.co/course/chapter1/1)๋ฅผ ์ฐธ์กฐํ๊ธฐ๋ฅผ ๊ถ์ฅํฉ๋๋ค.
์์ํ๊ธฐ ์ ์ ํ์ํ ๋ผ์ด๋ธ๋ฌ๋ฆฌ๊ฐ ๋ชจ๋ ์ค์น๋์ด ์๋์ง ํ์ธํ์ธ์:
```bash
!pip install transformers datasets
```
๋ํ ์ ํธํ๋ ๋จธ์ ๋ฌ๋ ํ๋ ์์ํฌ๋ฅผ ์ค์นํด์ผ ํฉ๋๋ค:
<frameworkcontent>
<pt>
```bash
pip install torch
```
</pt>
<tf>
```bash
pip install tensorflow
```
</tf>
</frameworkcontent>
## ํ์ดํ๋ผ์ธ [[pipeline]]
<Youtube id="tiZFewofSLM"/>
[`pipeline`](./main_classes/pipelines)์ ์ฌ์ ํ๋ จ๋ ๋ชจ๋ธ๋ก ์ถ๋ก ํ๊ธฐ์ ๊ฐ์ฅ ์ฝ๊ณ ๋น ๋ฅธ ๋ฐฉ๋ฒ์
๋๋ค. [`pipeline`]์ ์ฌ๋ฌ ๋ชจ๋ฌ๋ฆฌํฐ์์ ๋ค์ํ ๊ณผ์
์ ์ฝ๊ฒ ์ฒ๋ฆฌํ ์ ์์ผ๋ฉฐ, ์๋ ํ์ ํ์๋ ๋ช ๊ฐ์ง ๊ณผ์
์ ๊ธฐ๋ณธ์ ์ผ๋ก ์ง์ํฉ๋๋ค:
<Tip>
์ฌ์ฉ ๊ฐ๋ฅํ ์์
์ ์ ์ฒด ๋ชฉ๋ก์ [Pipelines API ์ฐธ์กฐ](./main_classes/pipelines)๋ฅผ ํ์ธํ์ธ์.
</Tip>
| **ํ์คํฌ** | **์ค๋ช
** | **๋ชจ๋ฌ๋ฆฌํฐ** | **ํ์ดํ๋ผ์ธ ID** |
|-----------------|----------------------------------------------------------------------|------------------|-----------------------------------------------|
| ํ
์คํธ ๋ถ๋ฅ | ํ
์คํธ์ ์๋ง์ ๋ ์ด๋ธ ๋ถ์ด๊ธฐ | ์์ฐ์ด ์ฒ๋ฆฌ(NLP) | pipeline(task="sentiment-analysis") |
| ํ
์คํธ ์์ฑ | ์ฃผ์ด์ง ๋ฌธ์์ด ์
๋ ฅ๊ณผ ์ด์ด์ง๋ ํ
์คํธ ์์ฑํ๊ธฐ | ์์ฐ์ด ์ฒ๋ฆฌ(NLP) | pipeline(task="text-generation") |
| ๊ฐ์ฒด๋ช
์ธ์ | ๋ฌธ์์ด์ ๊ฐ ํ ํฐ๋ง๋ค ์๋ง์ ๋ ์ด๋ธ ๋ถ์ด๊ธฐ (์ธ๋ฌผ, ์กฐ์ง, ์ฅ์ ๋ฑ๋ฑ) | ์์ฐ์ด ์ฒ๋ฆฌ(NLP) | pipeline(task="ner") |
| ์ง์์๋ต | ์ฃผ์ด์ง ๋ฌธ๋งฅ๊ณผ ์ง๋ฌธ์ ๋ฐ๋ผ ์ฌ๋ฐ๋ฅธ ๋๋ตํ๊ธฐ | ์์ฐ์ด ์ฒ๋ฆฌ(NLP) | pipeline(task="question-answering") |
| ๋น์นธ ์ฑ์ฐ๊ธฐ | ๋ฌธ์์ด์ ๋น์นธ์ ์๋ง์ ํ ํฐ ๋ง์ถ๊ธฐ | ์์ฐ์ด ์ฒ๋ฆฌ(NLP) | pipeline(task="fill-mask") |
| ์์ฝ | ํ
์คํธ๋ ๋ฌธ์๋ฅผ ์์ฝํ๊ธฐ | ์์ฐ์ด ์ฒ๋ฆฌ(NLP) | pipeline(task="summarization") |
| ๋ฒ์ญ | ํ
์คํธ๋ฅผ ํ ์ธ์ด์์ ๋ค๋ฅธ ์ธ์ด๋ก ๋ฒ์ญํ๊ธฐ | ์์ฐ์ด ์ฒ๋ฆฌ(NLP) | pipeline(task="translation") |
| ์ด๋ฏธ์ง ๋ถ๋ฅ | ์ด๋ฏธ์ง์ ์๋ง์ ๋ ์ด๋ธ ๋ถ์ด๊ธฐ | ์ปดํจํฐ ๋น์ (CV) | pipeline(task="image-classification") |
| ์ด๋ฏธ์ง ๋ถํ | ์ด๋ฏธ์ง์ ํฝ์
๋ง๋ค ๋ ์ด๋ธ ๋ถ์ด๊ธฐ(์๋งจํฑ, ํ๋ํฑ ๋ฐ ์ธ์คํด์ค ๋ถํ ํฌํจ) | ์ปดํจํฐ ๋น์ (CV) | pipeline(task="image-segmentation") |
| ๊ฐ์ฒด ํ์ง | ์ด๋ฏธ์ง ์ ๊ฐ์ฒด์ ๊ฒฝ๊ณ ์์๋ฅผ ๊ทธ๋ฆฌ๊ณ ํด๋์ค๋ฅผ ์์ธกํ๊ธฐ | ์ปดํจํฐ ๋น์ (CV) | pipeline(task="object-detection") |
| ์ค๋์ค ๋ถ๋ฅ | ์ค๋์ค ํ์ผ์ ์๋ง์ ๋ ์ด๋ธ ๋ถ์ด๊ธฐ | ์ค๋์ค | pipeline(task="audio-classification") |
| ์๋ ์์ฑ ์ธ์ | ์ค๋์ค ํ์ผ ์ ์์ฑ์ ํ
์คํธ๋ก ๋ฐ๊พธ๊ธฐ | ์ค๋์ค | pipeline(task="automatic-speech-recognition") |
| ์๊ฐ ์ง์์๋ต | ์ฃผ์ด์ง ์ด๋ฏธ์ง์ ์ง๋ฌธ์ ๋ํด ์ฌ๋ฐ๋ฅด๊ฒ ๋๋ตํ๊ธฐ | ๋ฉํฐ๋ชจ๋ฌ | pipeline(task="vqa") |
| ๋ฌธ์ ์ง์์๋ต | ์ฃผ์ด์ง ๋ฌธ์์ ์ง๋ฌธ์ ๋ํด ์ฌ๋ฐ๋ฅด๊ฒ ๋๋ตํ๊ธฐ | ๋ฉํฐ๋ชจ๋ฌ | pipeline(task="document-question-answering") |
| ์ด๋ฏธ์ง ์บก์
๋ฌ๊ธฐ | ์ฃผ์ด์ง ์ด๋ฏธ์ง์ ์บก์
์์ฑํ๊ธฐ | ๋ฉํฐ๋ชจ๋ฌ | pipeline(task="image-to-text") |
๋จผ์ [`pipeline`]์ ์ธ์คํด์ค๋ฅผ ์์ฑํ๊ณ ์ฌ์ฉํ ์์
์ ์ง์ ํฉ๋๋ค. ์ด ๊ฐ์ด๋์์๋ ๊ฐ์ ๋ถ์์ ์ํด [`pipeline`]์ ์ฌ์ฉํ๋ ์์ ๋ฅผ ๋ณด์ฌ๋๋ฆฌ๊ฒ ์ต๋๋ค:
```py
>>> from transformers import pipeline
>>> classifier = pipeline("sentiment-analysis")
```
[`pipeline`]์ ๊ฐ์ ๋ถ์์ ์ํ [์ฌ์ ํ๋ จ๋ ๋ชจ๋ธ](https://huggingface.co/distilbert-base-uncased-finetuned-sst-2-english)๊ณผ ํ ํฌ๋์ด์ ๋ฅผ ์๋์ผ๋ก ๋ค์ด๋ก๋ํ๊ณ ์บ์ํฉ๋๋ค. ์ด์ `classifier`๋ฅผ ๋์ ํ
์คํธ์ ์ฌ์ฉํ ์ ์์ต๋๋ค:
```py
>>> classifier("We are very happy to show you the ๐ค Transformers library.")
[{'label': 'POSITIVE', 'score': 0.9998}]
```
๋ง์ฝ ์
๋ ฅ์ด ์ฌ๋ฌ ๊ฐ ์๋ ๊ฒฝ์ฐ, ์
๋ ฅ์ ๋ฆฌ์คํธ๋ก [`pipeline`]์ ์ ๋ฌํ์ฌ, ์ฌ์ ํ๋ จ๋ ๋ชจ๋ธ์ ์ถ๋ ฅ์ ๋์
๋๋ฆฌ๋ก ์ด๋ฃจ์ด์ง ๋ฆฌ์คํธ ํํ๋ก ๋ฐ์ ์ ์์ต๋๋ค:
```py
>>> results = classifier(["We are very happy to show you the ๐ค Transformers library.", "We hope you don't hate it."])
>>> for result in results:
... print(f"label: {result['label']}, with score: {round(result['score'], 4)}")
label: POSITIVE, with score: 0.9998
label: NEGATIVE, with score: 0.5309
```
[`pipeline`]์ ์ฃผ์ด์ง ๊ณผ์
์ ๊ด๊ณ์์ด ๋ฐ์ดํฐ์
์ ๋ถ๋ฅผ ์ํํ ์๋ ์์ต๋๋ค. ์ด ์์ ์์๋ ์๋ ์์ฑ ์ธ์์ ๊ณผ์
์ผ๋ก ์ ํํด ๋ณด๊ฒ ์ต๋๋ค:
```py
>>> import torch
>>> from transformers import pipeline
>>> speech_recognizer = pipeline("automatic-speech-recognition", model="facebook/wav2vec2-base-960h")
```
๋ฐ์ดํฐ์
์ ๋ก๋ํ ์ฐจ๋ก์
๋๋ค. (์์ธํ ๋ด์ฉ์ ๐ค Datasets [์์ํ๊ธฐ](https://huggingface.co/docs/datasets/quickstart#audio)์ ์ฐธ์กฐํ์ธ์) ์ฌ๊ธฐ์์๋ [MInDS-14](https://huggingface.co/datasets/PolyAI/minds14) ๋ฐ์ดํฐ์
์ ๋ก๋ํ๊ฒ ์ต๋๋ค:
```py
>>> from datasets import load_dataset, Audio
>>> dataset = load_dataset("PolyAI/minds14", name="en-US", split="train") # doctest: +IGNORE_RESULT
```
๋ฐ์ดํฐ์
์ ์ํ๋ง ๋ ์ดํธ๊ฐ ๊ธฐ์กด ๋ชจ๋ธ์ธ [`facebook/wav2vec2-base-960h`](https://huggingface.co/facebook/wav2vec2-base-960h)์ ํ๋ จ ๋น์ ์ํ๋ง ๋ ์ดํธ์ ์ผ์นํ๋์ง ํ์ธํด์ผ ํฉ๋๋ค:
```py
>>> dataset = dataset.cast_column("audio", Audio(sampling_rate=speech_recognizer.feature_extractor.sampling_rate))
```
`"audio"` ์ด์ ํธ์ถํ๋ฉด ์๋์ผ๋ก ์ค๋์ค ํ์ผ์ ๊ฐ์ ธ์์ ๋ฆฌ์ํ๋งํฉ๋๋ค. ์ฒซ 4๊ฐ ์ํ์์ ์์ ์จ์ด๋ธํผ ๋ฐฐ์ด์ ์ถ์ถํ๊ณ ํ์ดํ๋ผ์ธ์ ๋ฆฌ์คํธ๋ก ์ ๋ฌํ์ธ์:
```py
>>> result = speech_recognizer(dataset[:4]["audio"])
>>> print([d["text"] for d in result])
['I WOULD LIKE TO SET UP A JOINT ACCOUNT WITH MY PARTNER HOW DO I PROCEED WITH DOING THAT', "FONDERING HOW I'D SET UP A JOIN TO HELL T WITH MY WIFE AND WHERE THE AP MIGHT BE", "I I'D LIKE TOY SET UP A JOINT ACCOUNT WITH MY PARTNER I'M NOT SEEING THE OPTION TO DO IT ON THE APSO I CALLED IN TO GET SOME HELP CAN I JUST DO IT OVER THE PHONE WITH YOU AND GIVE YOU THE INFORMATION OR SHOULD I DO IT IN THE AP AN I'M MISSING SOMETHING UQUETTE HAD PREFERRED TO JUST DO IT OVER THE PHONE OF POSSIBLE THINGS", 'HOW DO I FURN A JOINA COUT']
```
์์ฑ์ด๋ ๋น์ ๊ณผ ๊ฐ์ด ์
๋ ฅ์ด ํฐ ๋๊ท๋ชจ ๋ฐ์ดํฐ์
์ ๊ฒฝ์ฐ, ๋ชจ๋ ์
๋ ฅ์ ๋ฉ๋ชจ๋ฆฌ์ ๋ก๋ํ๋ ค๋ฉด ๋ฆฌ์คํธ ๋์ ์ ๋๋ ์ดํฐ ํํ๋ก ์ ๋ฌํด์ผ ํฉ๋๋ค. ์์ธํ ๋ด์ฉ์ [Pipelines API ์ฐธ์กฐ](./main_classes/pipelines)๋ฅผ ํ์ธํ์ธ์.
### ํ์ดํ๋ผ์ธ์์ ๋ค๋ฅธ ๋ชจ๋ธ๊ณผ ํ ํฌ๋์ด์ ์ฌ์ฉํ๊ธฐ [[use-another-model-and-tokenizer-in-the-pipeline]]
[`pipeline`]์ [Hub](https://huggingface.co/models)์ ๋ชจ๋ ๋ชจ๋ธ์ ์ฌ์ฉํ ์ ์๊ธฐ ๋๋ฌธ์, [`pipeline`]์ ๋ค๋ฅธ ์ฉ๋์ ๋ง๊ฒ ์ฝ๊ฒ ์์ ํ ์ ์์ต๋๋ค. ์๋ฅผ ๋ค์ด, ํ๋์ค์ด ํ
์คํธ๋ฅผ ์ฒ๋ฆฌํ ์ ์๋ ๋ชจ๋ธ์ ์ฌ์ฉํ๊ธฐ ์ํด์ Hub์ ํ๊ทธ๋ฅผ ์ฌ์ฉํ์ฌ ์ ์ ํ ๋ชจ๋ธ์ ํํฐ๋งํ๋ฉด ๋ฉ๋๋ค. ํํฐ๋ง๋ ๊ฒฐ๊ณผ์ ์์ ํญ๋ชฉ์ผ๋ก๋ ํ๋์ค์ด ํ
์คํธ์ ์ฌ์ฉํ ์ ์๋ ๋ค๊ตญ์ด [BERT ๋ชจ๋ธ](https://huggingface.co/nlptown/bert-base-multilingual-uncased-sentiment)์ด ๋ฐํ๋ฉ๋๋ค:
```py
>>> model_name = "nlptown/bert-base-multilingual-uncased-sentiment"
```
<frameworkcontent>
<pt>
[`AutoModelForSequenceClassification`]๊ณผ [`AutoTokenizer`]๋ฅผ ์ฌ์ฉํ์ฌ ์ฌ์ ํ๋ จ๋ ๋ชจ๋ธ๊ณผ ๊ด๋ จ๋ ํ ํฌ๋์ด์ ๋ฅผ ๋ก๋ํ์ธ์ (๋ค์ ์น์
์์ [`AutoClass`]์ ๋ํด ๋ ์์ธํ ์์๋ณด๊ฒ ์ต๋๋ค):
```py
>>> from transformers import AutoTokenizer, AutoModelForSequenceClassification
>>> model = AutoModelForSequenceClassification.from_pretrained(model_name)
>>> tokenizer = AutoTokenizer.from_pretrained(model_name)
```
</pt>
<tf>
[`TFAutoModelForSequenceClassification`]๊ณผ [`AutoTokenizer`]๋ฅผ ์ฌ์ฉํ์ฌ ์ฌ์ ํ๋ จ๋ ๋ชจ๋ธ๊ณผ ๊ด๋ จ๋ ํ ํฌ๋์ด์ ๋ฅผ ๋ก๋ํ์ธ์ (๋ค์ ์น์
์์ [`TFAutoClass`]์ ๋ํด ๋ ์์ธํ ์์๋ณด๊ฒ ์ต๋๋ค):
```py
>>> from transformers import AutoTokenizer, TFAutoModelForSequenceClassification
>>> model = TFAutoModelForSequenceClassification.from_pretrained(model_name)
>>> tokenizer = AutoTokenizer.from_pretrained(model_name)
```
</tf>
</frameworkcontent>
[`pipeline`]์์ ๋ชจ๋ธ๊ณผ ํ ํฌ๋์ด์ ๋ฅผ ์ง์ ํ๋ฉด, ์ด์ `classifier`๋ฅผ ํ๋์ค์ด ํ
์คํธ์ ์ ์ฉํ ์ ์์ต๋๋ค:
```py
>>> classifier = pipeline("sentiment-analysis", model=model, tokenizer=tokenizer)
>>> classifier("Nous sommes trรจs heureux de vous prรฉsenter la bibliothรจque ๐ค Transformers.")
[{'label': '5 stars', 'score': 0.7273}]
```
๋ง๋
ํ ๋ชจ๋ธ์ ์ฐพ์ ์ ์๋ ๊ฒฝ์ฐ ๋ฐ์ดํฐ๋ฅผ ๊ธฐ๋ฐ์ผ๋ก ์ฌ์ ํ๋ จ๋ ๋ชจ๋ธ์ ๋ฏธ์ธ์กฐ์ ํด์ผ ํฉ๋๋ค. ๋ฏธ์ธ์กฐ์ ๋ฐฉ๋ฒ์ ๋ํ ์์ธํ ๋ด์ฉ์ [๋ฏธ์ธ์กฐ์ ํํ ๋ฆฌ์ผ](./training)์ ์ฐธ์กฐํ์ธ์. ์ฌ์ ํ๋ จ๋ ๋ชจ๋ธ์ ๋ฏธ์ธ์กฐ์ ํ ํ์๋ ๋ชจ๋ธ์ Hub์ ์ปค๋ฎค๋ํฐ์ ๊ณต์ ํ์ฌ ๋จธ์ ๋ฌ๋ ๋ฏผ์ฃผํ์ ๊ธฐ์ฌํด์ฃผ์ธ์! ๐ค
## AutoClass [[autoclass]]
<Youtube id="AhChOFRegn4"/>
[`AutoModelForSequenceClassification`]๊ณผ [`AutoTokenizer`] ํด๋์ค๋ ์์์ ๋ค๋ฃฌ [`pipeline`]์ ๊ธฐ๋ฅ์ ๊ตฌํํ๋ ๋ฐ ์ฌ์ฉ๋ฉ๋๋ค. [AutoClass](./model_doc/auto)๋ ์ฌ์ ํ๋ จ๋ ๋ชจ๋ธ์ ์ํคํ
์ฒ๋ฅผ ์ด๋ฆ์ด๋ ๊ฒฝ๋ก์์ ์๋์ผ๋ก ๊ฐ์ ธ์ค๋ '๋ฐ๋ก๊ฐ๊ธฐ'์
๋๋ค. ๊ณผ์
์ ์ ํฉํ `AutoClass`๋ฅผ ์ ํํ๊ณ ํด๋น ์ ์ฒ๋ฆฌ ํด๋์ค๋ฅผ ์ ํํ๊ธฐ๋ง ํ๋ฉด ๋ฉ๋๋ค.
์ด์ ์น์
์ ์์ ๋ก ๋์๊ฐ์ [`pipeline`]์ ๊ฒฐ๊ณผ๋ฅผ `AutoClass`๋ฅผ ํ์ฉํด ๋ณต์ ํ๋ ๋ฐฉ๋ฒ์ ์ดํด๋ณด๊ฒ ์ต๋๋ค.
### AutoTokenizer [[autotokenizer]]
ํ ํฌ๋์ด์ ๋ ํ
์คํธ๋ฅผ ๋ชจ๋ธ์ ์
๋ ฅ์ผ๋ก ์ฌ์ฉํ๊ธฐ ์ํด ์ซ์ ๋ฐฐ์ด ํํ๋ก ์ ์ฒ๋ฆฌํ๋ ์ญํ ์ ๋ด๋นํฉ๋๋ค. ํ ํฐํ ๊ณผ์ ์๋ ๋จ์ด๋ฅผ ์ด๋์์ ๋์์ง, ์ด๋ ์์ค๊น์ง ๋๋์ง์ ๊ฐ์ ์ฌ๋ฌ ๊ท์น๋ค์ด ์์ต๋๋ค (ํ ํฐํ์ ๋ํ ์์ธํ ๋ด์ฉ์ [ํ ํฌ๋์ด์ ์์ฝ](./tokenizer_summary)์ ์ฐธ์กฐํ์ธ์). ๊ฐ์ฅ ์ค์ํ ์ ์ ๋ชจ๋ธ์ด ์ฌ์ ํ๋ จ๋ ๋ชจ๋ธ๊ณผ ๋์ผํ ํ ํฐํ ๊ท์น์ ์ฌ์ฉํ๋๋ก ๋์ผํ ๋ชจ๋ธ ์ด๋ฆ์ผ๋ก ํ ํฌ๋์ด์ ๋ฅผ ์ธ์คํด์คํํด์ผ ํ๋ค๋ ๊ฒ์
๋๋ค.
[`AutoTokenizer`]๋ก ํ ํฌ๋์ด์ ๋ฅผ ๋ก๋ํ์ธ์:
```py
>>> from transformers import AutoTokenizer
>>> model_name = "nlptown/bert-base-multilingual-uncased-sentiment"
>>> tokenizer = AutoTokenizer.from_pretrained(model_name)
```
ํ
์คํธ๋ฅผ ํ ํฌ๋์ด์ ์ ์ ๋ฌํ์ธ์:
```py
>>> encoding = tokenizer("We are very happy to show you the ๐ค Transformers library.")
>>> print(encoding)
{'input_ids': [101, 11312, 10320, 12495, 19308, 10114, 11391, 10855, 10103, 100, 58263, 13299, 119, 102],
'token_type_ids': [0, 0, 0, 0, 0, 0, 0, 0, 0, 0, 0, 0, 0, 0],
'attention_mask': [1, 1, 1, 1, 1, 1, 1, 1, 1, 1, 1, 1, 1, 1]}
```
ํ ํฌ๋์ด์ ๋ ๋ค์์ ํฌํจํ ๋์
๋๋ฆฌ๋ฅผ ๋ฐํํฉ๋๋ค:
* [input_ids](./glossary#input-ids): ํ ํฐ์ ์ซ์ ํํ.
* [attention_mask](.glossary#attention-mask): ์ด๋ค ํ ํฐ์ ์ฃผ์๋ฅผ ๊ธฐ์ธ์ฌ์ผ ํ๋์ง๋ฅผ ๋ํ๋
๋๋ค.
ํ ํฌ๋์ด์ ๋ ์
๋ ฅ์ ๋ฆฌ์คํธ ํํ๋ก๋ ๋ฐ์ ์ ์์ผ๋ฉฐ, ํ
์คํธ๋ฅผ ํจ๋ฉํ๊ณ ์๋ผ๋ด์ด ์ผ์ ํ ๊ธธ์ด์ ๋ฌถ์์ ๋ฐํํ ์๋ ์์ต๋๋ค:
<frameworkcontent>
<pt>
```py
>>> pt_batch = tokenizer(
... ["We are very happy to show you the ๐ค Transformers library.", "We hope you don't hate it."],
... padding=True,
... truncation=True,
... max_length=512,
... return_tensors="pt",
... )
```
</pt>
<tf>
```py
>>> tf_batch = tokenizer(
... ["We are very happy to show you the ๐ค Transformers library.", "We hope you don't hate it."],
... padding=True,
... truncation=True,
... max_length=512,
... return_tensors="tf",
... )
```
</tf>
</frameworkcontent>
<Tip>
[์ ์ฒ๋ฆฌ](./preprocessing) ํํ ๋ฆฌ์ผ์ ์ฐธ์กฐํ์๋ฉด ํ ํฐํ์ ๋ํ ์์ธํ ์ค๋ช
๊ณผ ํจ๊ป ์ด๋ฏธ์ง, ์ค๋์ค์ ๋ฉํฐ๋ชจ๋ฌ ์
๋ ฅ์ ์ ์ฒ๋ฆฌํ๊ธฐ ์ํ [`AutoImageProcessor`]์ [`AutoFeatureExtractor`], [`AutoProcessor`]์ ์ฌ์ฉ๋ฐฉ๋ฒ๋ ์ ์ ์์ต๋๋ค.
</Tip>
### AutoModel [[automodel]]
<frameworkcontent>
<pt>
๐ค Transformers๋ ์ฌ์ ํ๋ จ๋ ์ธ์คํด์ค๋ฅผ ๊ฐ๋จํ๊ณ ํตํฉ๋ ๋ฐฉ๋ฒ์ผ๋ก ๋ก๋ํ ์ ์์ต๋๋ค. ์ฆ, [`AutoTokenizer`]์ฒ๋ผ [`AutoModel`]์ ๋ก๋ํ ์ ์์ต๋๋ค. ์ ์ผํ ์ฐจ์ด์ ์ ๊ณผ์
์ ์๋ง์ [`AutoModel`]์ ์ ํํด์ผ ํ๋ค๋ ์ ์
๋๋ค. ํ
์คํธ (๋๋ ์ํ์ค) ๋ถ๋ฅ์ ๊ฒฝ์ฐ [`AutoModelForSequenceClassification`]์ ๋ก๋ํด์ผ ํฉ๋๋ค:
```py
>>> from transformers import AutoModelForSequenceClassification
>>> model_name = "nlptown/bert-base-multilingual-uncased-sentiment"
>>> pt_model = AutoModelForSequenceClassification.from_pretrained(model_name)
```
<Tip>
[`AutoModel`] ํด๋์ค์์ ์ง์ํ๋ ๊ณผ์
์ ๋ํด์๋ [๊ณผ์
์์ฝ](./task_summary)์ ์ฐธ์กฐํ์ธ์.
</Tip>
์ด์ ์ ์ฒ๋ฆฌ๋ ์
๋ ฅ ๋ฌถ์์ ์ง์ ๋ชจ๋ธ์ ์ ๋ฌํด์ผ ํฉ๋๋ค. ์๋์ฒ๋ผ `**`๋ฅผ ์์ ๋ถ์ฌ ๋์
๋๋ฆฌ๋ฅผ ํ์ด์ฃผ๋ฉด ๋ฉ๋๋ค:
```py
>>> pt_outputs = pt_model(**pt_batch)
```
๋ชจ๋ธ์ ์ต์ข
ํ์ฑํ ํจ์ ์ถ๋ ฅ์ `logits` ์์ฑ์ ๋ด๊ฒจ์์ต๋๋ค. `logits`์ softmax ํจ์๋ฅผ ์ ์ฉํ์ฌ ํ๋ฅ ์ ์ป์ ์ ์์ต๋๋ค:
```py
>>> from torch import nn
>>> pt_predictions = nn.functional.softmax(pt_outputs.logits, dim=-1)
>>> print(pt_predictions)
tensor([[0.0021, 0.0018, 0.0115, 0.2121, 0.7725],
[0.2084, 0.1826, 0.1969, 0.1755, 0.2365]], grad_fn=<SoftmaxBackward0>)
```
</pt>
<tf>
๐ค Transformers๋ ์ฌ์ ํ๋ จ๋ ์ธ์คํด์ค๋ฅผ ๊ฐ๋จํ๊ณ ํตํฉ๋ ๋ฐฉ๋ฒ์ผ๋ก ๋ก๋ํ ์ ์์ต๋๋ค. ์ฆ, [`AutoTokenizer`]์ฒ๋ผ [`TFAutoModel`]์ ๋ก๋ํ ์ ์์ต๋๋ค. ์ ์ผํ ์ฐจ์ด์ ์ ๊ณผ์
์ ์๋ง์ [`TFAutoModel`]์ ์ ํํด์ผ ํ๋ค๋ ์ ์
๋๋ค. ํ
์คํธ (๋๋ ์ํ์ค) ๋ถ๋ฅ์ ๊ฒฝ์ฐ [`TFAutoModelForSequenceClassification`]์ ๋ก๋ํด์ผ ํฉ๋๋ค:
```py
>>> from transformers import TFAutoModelForSequenceClassification
>>> model_name = "nlptown/bert-base-multilingual-uncased-sentiment"
>>> tf_model = TFAutoModelForSequenceClassification.from_pretrained(model_name)
```
<Tip>
[`AutoModel`] ํด๋์ค์์ ์ง์ํ๋ ๊ณผ์
์ ๋ํด์๋ [๊ณผ์
์์ฝ](./task_summary)์ ์ฐธ์กฐํ์ธ์.
</Tip>
์ด์ ์ ์ฒ๋ฆฌ๋ ์
๋ ฅ ๋ฌถ์์ ์ง์ ๋ชจ๋ธ์ ์ ๋ฌํด์ผ ํฉ๋๋ค. ์๋์ฒ๋ผ ๊ทธ๋๋ก ํ
์๋ฅผ ์ ๋ฌํ๋ฉด ๋ฉ๋๋ค:
```py
>>> tf_outputs = tf_model(tf_batch)
```
๋ชจ๋ธ์ ์ต์ข
ํ์ฑํ ํจ์ ์ถ๋ ฅ์ `logits` ์์ฑ์ ๋ด๊ฒจ์์ต๋๋ค. `logits`์ softmax ํจ์๋ฅผ ์ ์ฉํ์ฌ ํ๋ฅ ์ ์ป์ ์ ์์ต๋๋ค:
```py
>>> import tensorflow as tf
>>> tf_predictions = tf.nn.softmax(tf_outputs.logits, axis=-1)
>>> tf_predictions # doctest: +IGNORE_RESULT
```
</tf>
</frameworkcontent>
<Tip>
๋ชจ๋ ๐ค Transformers ๋ชจ๋ธ(PyTorch ๋๋ TensorFlow)์ (softmax์ ๊ฐ์) ์ต์ข
ํ์ฑํ ํจ์ *์ด์ ์* ํ
์๋ฅผ ์ถ๋ ฅํฉ๋๋ค. ์๋ํ๋ฉด ์ต์ข
ํ์ฑํ ํจ์์ ์ถ๋ ฅ์ ์ข
์ข
์์ค ํจ์ ์ถ๋ ฅ๊ณผ ๊ฒฐํฉ๋๊ธฐ ๋๋ฌธ์
๋๋ค. ๋ชจ๋ธ ์ถ๋ ฅ์ ํน์ํ ๋ฐ์ดํฐ ํด๋์ค์ด๋ฏ๋ก IDE์์ ์๋ ์์ฑ๋ฉ๋๋ค. ๋ชจ๋ธ ์ถ๋ ฅ์ ํํ์ด๋ ๋์
๋๋ฆฌ์ฒ๋ผ ๋์ํ๋ฉฐ (์ ์, ์ฌ๋ผ์ด์ค ๋๋ ๋ฌธ์์ด๋ก ์ธ๋ฑ์ฑ ๊ฐ๋ฅ), None์ธ ์์ฑ์ ๋ฌด์๋ฉ๋๋ค.
</Tip>
### ๋ชจ๋ธ ์ ์ฅํ๊ธฐ [[save-a-model]]
<frameworkcontent>
<pt>
๋ฏธ์ธ์กฐ์ ๋ ๋ชจ๋ธ์ ํ ํฌ๋์ด์ ์ ํจ๊ป ์ ์ฅํ๋ ค๋ฉด [`PreTrainedModel.save_pretrained`]๋ฅผ ์ฌ์ฉํ์ธ์:
```py
>>> pt_save_directory = "./pt_save_pretrained"
>>> tokenizer.save_pretrained(pt_save_directory) # doctest: +IGNORE_RESULT
>>> pt_model.save_pretrained(pt_save_directory)
```
๋ชจ๋ธ์ ๋ค์ ์ฌ์ฉํ๋ ค๋ฉด [`PreTrainedModel.from_pretrained`]๋ก ๋ชจ๋ธ์ ๋ค์ ๋ก๋ํ์ธ์:
```py
>>> pt_model = AutoModelForSequenceClassification.from_pretrained("./pt_save_pretrained")
```
</pt>
<tf>
๋ฏธ์ธ์กฐ์ ๋ ๋ชจ๋ธ์ ํ ํฌ๋์ด์ ์ ํจ๊ป ์ ์ฅํ๋ ค๋ฉด [`TFPreTrainedModel.save_pretrained`]๋ฅผ ์ฌ์ฉํ์ธ์:
```py
>>> tf_save_directory = "./tf_save_pretrained"
>>> tokenizer.save_pretrained(tf_save_directory) # doctest: +IGNORE_RESULT
>>> tf_model.save_pretrained(tf_save_directory)
```
๋ชจ๋ธ์ ๋ค์ ์ฌ์ฉํ๋ ค๋ฉด [`TFPreTrainedModel.from_pretrained`]๋ก ๋ชจ๋ธ์ ๋ค์ ๋ก๋ํ์ธ์:
```py
>>> tf_model = TFAutoModelForSequenceClassification.from_pretrained("./tf_save_pretrained")
```
</tf>
</frameworkcontent>
๐ค Transformers์ ๋ฉ์ง ๊ธฐ๋ฅ ์ค ํ๋๋ ๋ชจ๋ธ์ PyTorch ๋๋ TensorFlow ๋ชจ๋ธ๋ก ์ ์ฅํด๋๋ค๊ฐ ๋ค๋ฅธ ํ๋ ์์ํฌ๋ก ๋ค์ ๋ก๋ํ ์ ์๋ ์ ์
๋๋ค. `from_pt` ๋๋ `from_tf` ๋งค๊ฐ๋ณ์๋ฅผ ์ฌ์ฉํ์ฌ ๋ชจ๋ธ์ ํ ํ๋ ์์ํฌ์์ ๋ค๋ฅธ ํ๋ ์์ํฌ๋ก ๋ณํํ ์ ์์ต๋๋ค:
<frameworkcontent>
<pt>
```py
>>> from transformers import AutoModel
>>> tokenizer = AutoTokenizer.from_pretrained(tf_save_directory)
>>> pt_model = AutoModelForSequenceClassification.from_pretrained(tf_save_directory, from_tf=True)
```
</pt>
<tf>
```py
>>> from transformers import TFAutoModel
>>> tokenizer = AutoTokenizer.from_pretrained(pt_save_directory)
>>> tf_model = TFAutoModelForSequenceClassification.from_pretrained(pt_save_directory, from_pt=True)
```
</tf>
</frameworkcontent>
## ์ปค์คํ
๋ชจ๋ธ ๊ตฌ์ถํ๊ธฐ [[custom-model-builds]]
๋ชจ๋ธ์ ๊ตฌ์ฑ ํด๋์ค๋ฅผ ์์ ํ์ฌ ๋ชจ๋ธ์ ๊ตฌ์กฐ๋ฅผ ๋ฐ๊ฟ ์ ์์ต๋๋ค. (์๋์ธต์ด๋ ์ดํ
์
ํค๋์ ์์ ๊ฐ์) ๋ชจ๋ธ์ ์์ฑ์ ๊ตฌ์ฑ์์ ์ง์ ๋๊ธฐ ๋๋ฌธ์
๋๋ค. ์ปค์คํ
๊ตฌ์ฑ ํด๋์ค๋ก ๋ชจ๋ธ์ ๋ง๋ค๋ฉด ์ฒ์๋ถํฐ ์์ํด์ผ ํฉ๋๋ค. ๋ชจ๋ธ ์์ฑ์ ๋ฌด์์๋ก ์ด๊ธฐํ๋๋ฏ๋ก ์๋ฏธ ์๋ ๊ฒฐ๊ณผ๋ฅผ ์ป์ผ๋ ค๋ฉด ๋จผ์ ๋ชจ๋ธ์ ํ๋ จ์์ผ์ผ ํฉ๋๋ค.
๋จผ์ [`AutoConfig`]๋ฅผ ๊ฐ์ ธ์ค๊ณ ์์ ํ๊ณ ์ถ์ ์ฌ์ ํ์ต๋ ๋ชจ๋ธ์ ๋ก๋ํ์ธ์. [`AutoConfig.from_pretrained`] ๋ด๋ถ์์ (์ดํ
์
ํค๋ ์์ ๊ฐ์ด) ๋ณ๊ฒฝํ๋ ค๋ ์์ฑ๋ฅผ ์ง์ ํ ์ ์์ต๋๋ค:
```py
>>> from transformers import AutoConfig
>>> my_config = AutoConfig.from_pretrained("distilbert-base-uncased", n_heads=12)
```
<frameworkcontent>
<pt>
[`AutoModel.from_config`]๋ฅผ ์ฌ์ฉํ์ฌ ๋ฐ๊พผ ๊ตฌ์ฑ๋๋ก ๋ชจ๋ธ์ ์์ฑํ์ธ์:
```py
>>> from transformers import AutoModel
>>> my_model = AutoModel.from_config(my_config)
```
</pt>
<tf>
[`TFAutoModel.from_config`]๋ฅผ ์ฌ์ฉํ์ฌ ๋ฐ๊พผ ๊ตฌ์ฑ๋๋ก ๋ชจ๋ธ์ ์์ฑํ์ธ์:
```py
>>> from transformers import TFAutoModel
>>> my_model = TFAutoModel.from_config(my_config)
```
</tf>
</frameworkcontent>
์ปค์คํ
๊ตฌ์ฑ์ ๋ํ ์์ธํ ๋ด์ฉ์ [์ปค์คํ
์ํคํ
์ฒ ๋ง๋ค๊ธฐ](./create_a_model) ๊ฐ์ด๋๋ฅผ ํ์ธํ์ธ์.
## Trainer - PyTorch์ ์ต์ ํ๋ ํ๋ จ ๋ฃจํ [[trainer-a-pytorch-optimized-training-loop]]
๋ชจ๋ ๋ชจ๋ธ์ [`torch.nn.Module`](https://pytorch.org/docs/stable/nn.html#torch.nn.Module)์ด๋ฏ๋ก ์ผ๋ฐ์ ์ธ ํ๋ จ ๋ฃจํ์์ ์ฌ์ฉํ ์ ์์ต๋๋ค. ์ง์ ํ๋ จ ๋ฃจํ๋ฅผ ์์ฑํ ์๋ ์์ง๋ง, ๐ค Transformers๋ PyTorch๋ฅผ ์ํ [`Trainer`] ํด๋์ค๋ฅผ ์ ๊ณตํฉ๋๋ค. ์ด ํด๋์ค์๋ ๊ธฐ๋ณธ ํ๋ จ ๋ฃจํ๊ฐ ํฌํจ๋์ด ์์ผ๋ฉฐ ๋ถ์ฐ ํ๋ จ, ํผํฉ ์ ๋ฐ๋ ๋ฑ๊ณผ ๊ฐ์ ๊ธฐ๋ฅ์ ์ถ๊ฐ๋ก ์ ๊ณตํฉ๋๋ค.
๊ณผ์
์ ๋ฐ๋ผ ๋ค๋ฅด์ง๋ง ์ผ๋ฐ์ ์ผ๋ก [`Trainer`]์ ๋ค์ ๋งค๊ฐ๋ณ์๋ฅผ ์ ๋ฌํฉ๋๋ค:
1. [`PreTrainedModel`] ๋๋ [`torch.nn.Module`](https://pytorch.org/docs/stable/nn.html#torch.nn.Module)๋ก ์์ํฉ๋๋ค:
```py
>>> from transformers import AutoModelForSequenceClassification
>>> model = AutoModelForSequenceClassification.from_pretrained("distilbert-base-uncased")
```
2. [`TrainingArguments`]๋ ํ์ต๋ฅ , ๋ฐฐ์น ํฌ๊ธฐ, ํ๋ จํ ์ํฌํฌ ์์ ๊ฐ์ ๋ชจ๋ธ ํ์ดํผํ๋ผ๋ฏธํฐ๋ฅผ ํฌํจํฉ๋๋ค. ํ๋ จ ์ธ์๋ฅผ ์ง์ ํ์ง ์์ผ๋ฉด ๊ธฐ๋ณธ๊ฐ์ด ์ฌ์ฉ๋ฉ๋๋ค:
```py
>>> from transformers import TrainingArguments
>>> training_args = TrainingArguments(
... output_dir="path/to/save/folder/",
... learning_rate=2e-5,
... per_device_train_batch_size=8,
... per_device_eval_batch_size=8,
... num_train_epochs=2,
... )
```
3. ํ ํฌ๋์ด์ , ์ด๋ฏธ์ง ํ๋ก์ธ์, ํน์ง ์ถ์ถ๊ธฐ(feature extractor) ๋๋ ํ๋ก์ธ์์ ์ ์ฒ๋ฆฌ ํด๋์ค๋ฅผ ๋ก๋ํ์ธ์:
```py
>>> from transformers import AutoTokenizer
>>> tokenizer = AutoTokenizer.from_pretrained("distilbert-base-uncased")
```
4. ๋ฐ์ดํฐ์
์ ๋ก๋ํ์ธ์:
```py
>>> from datasets import load_dataset
>>> dataset = load_dataset("rotten_tomatoes") # doctest: +IGNORE_RESULT
```
5. ๋ฐ์ดํฐ์
์ ํ ํฐํํ๋ ํจ์๋ฅผ ์์ฑํ์ธ์:
```py
>>> def tokenize_dataset(dataset):
... return tokenizer(dataset["text"])
```
๊ทธ๋ฆฌ๊ณ [`~datasets.Dataset.map`]๋ก ๋ฐ์ดํฐ์
์ ์ฒด์ ์ ์ฉํ์ธ์:
```py
>>> dataset = dataset.map(tokenize_dataset, batched=True)
```
6. [`DataCollatorWithPadding`]์ ์ฌ์ฉํ์ฌ ๋ฐ์ดํฐ์
์ ํ๋ณธ ๋ฌถ์์ ๋ง๋์ธ์:
```py
>>> from transformers import DataCollatorWithPadding
>>> data_collator = DataCollatorWithPadding(tokenizer=tokenizer)
```
์ด์ ์์ ๋ชจ๋ ํด๋์ค๋ฅผ [`Trainer`]๋ก ๋ชจ์ผ์ธ์:
```py
>>> from transformers import Trainer
>>> trainer = Trainer(
... model=model,
... args=training_args,
... train_dataset=dataset["train"],
... eval_dataset=dataset["test"],
... tokenizer=tokenizer,
... data_collator=data_collator,
... ) # doctest: +SKIP
```
์ค๋น๊ฐ ๋์์ผ๋ฉด [`~Trainer.train`]์ ํธ์ถํ์ฌ ํ๋ จ์ ์์ํ์ธ์:
```py
>>> trainer.train() # doctest: +SKIP
```
<Tip>
๋ฒ์ญ์ด๋ ์์ฝ๊ณผ ๊ฐ์ด ์ํ์ค-์ํ์ค ๋ชจ๋ธ์ ์ฌ์ฉํ๋ ๊ณผ์
์๋ [`Seq2SeqTrainer`] ๋ฐ [`Seq2SeqTrainingArguments`] ํด๋์ค๋ฅผ ์ฌ์ฉํ์ธ์.
</Tip>
[`Trainer`] ๋ด์ ๋ฉ์๋๋ฅผ ์๋ธํด๋์คํํ์ฌ ํ๋ จ ๋ฃจํ๋ฅผ ๋ฐ๊ฟ ์๋ ์์ต๋๋ค. ์ด๋ฌ๋ฉด ์์ค ํจ์, ์ตํฐ๋ง์ด์ , ์ค์ผ์ค๋ฌ์ ๊ฐ์ ๊ธฐ๋ฅ ๋ํ ๋ฐ๊ฟ ์ ์๊ฒ ๋ฉ๋๋ค. ๋ณ๊ฒฝ ๊ฐ๋ฅํ ๋ฉ์๋์ ๋ํด์๋ [`Trainer`] ๋ฌธ์๋ฅผ ์ฐธ๊ณ ํ์ธ์.
ํ๋ จ ๋ฃจํ๋ฅผ ์์ ํ๋ ๋ค๋ฅธ ๋ฐฉ๋ฒ์ [Callbacks](./main_classes/callbacks)๋ฅผ ์ฌ์ฉํ๋ ๊ฒ์
๋๋ค. Callbacks๋ก ๋ค๋ฅธ ๋ผ์ด๋ธ๋ฌ๋ฆฌ์ ํตํฉํ๊ณ , ํ๋ จ ๋ฃจํ๋ฅผ ์ฒดํฌํ์ฌ ์งํ ์ํฉ์ ๋ณด๊ณ ๋ฐ๊ฑฐ๋, ํ๋ จ์ ์กฐ๊ธฐ์ ์ค๋จํ ์ ์์ต๋๋ค. Callbacks์ ํ๋ จ ๋ฃจํ ์์ฒด๋ฅผ ๋ฐ๊พธ์ง๋ ์์ต๋๋ค. ์์ค ํจ์์ ๊ฐ์ ๊ฒ์ ๋ฐ๊พธ๋ ค๋ฉด [`Trainer`]๋ฅผ ์๋ธํด๋์คํํด์ผ ํฉ๋๋ค.
## TensorFlow๋ก ํ๋ จ์ํค๊ธฐ [[train-with-tensorflow]]
๋ชจ๋ ๋ชจ๋ธ์ [`tf.keras.Model`](https://www.tensorflow.org/api_docs/python/tf/keras/Model)์ด๋ฏ๋ก [Keras](https://keras.io/) API๋ฅผ ํตํด TensorFlow์์ ํ๋ จ์ํฌ ์ ์์ต๋๋ค. ๐ค Transformers๋ ๋ฐ์ดํฐ์
์ ์ฝ๊ฒ `tf.data.Dataset` ํํ๋ก ์ฝ๊ฒ ๋ก๋ํ ์ ์๋ [`~TFPreTrainedModel.prepare_tf_dataset`] ๋ฉ์๋๋ฅผ ์ ๊ณตํ๊ธฐ ๋๋ฌธ์, Keras์ [`compile`](https://keras.io/api/models/model_training_apis/#compile-method) ๋ฐ [`fit`](https://keras.io/api/models/model_training_apis/#fit-method) ๋ฉ์๋๋ก ๋ฐ๋ก ํ๋ จ์ ์์ํ ์ ์์ต๋๋ค.
1. [`TFPreTrainedModel`] ๋๋ [`tf.keras.Model`](https://www.tensorflow.org/api_docs/python/tf/keras/Model)๋ก ์์ํฉ๋๋ค:
```py
>>> from transformers import TFAutoModelForSequenceClassification
>>> model = TFAutoModelForSequenceClassification.from_pretrained("distilbert-base-uncased")
```
2. ํ ํฌ๋์ด์ , ์ด๋ฏธ์ง ํ๋ก์ธ์, ํน์ง ์ถ์ถ๊ธฐ(feature extractor) ๋๋ ํ๋ก์ธ์์ ๊ฐ์ ์ ์ฒ๋ฆฌ ํด๋์ค๋ฅผ ๋ก๋ํ์ธ์:
```py
>>> from transformers import AutoTokenizer
>>> tokenizer = AutoTokenizer.from_pretrained("distilbert-base-uncased")
```
3. ๋ฐ์ดํฐ์
์ ํ ํฐํํ๋ ํจ์๋ฅผ ์์ฑํ์ธ์:
```py
>>> def tokenize_dataset(dataset):
... return tokenizer(dataset["text"]) # doctest: +SKIP
```
4. [`~datasets.Dataset.map`]์ ์ฌ์ฉํ์ฌ ์ ์ฒด ๋ฐ์ดํฐ์
์ ํ ํฐํ ํจ์๋ฅผ ์ ์ฉํ๊ณ , ๋ฐ์ดํฐ์
๊ณผ ํ ํฌ๋์ด์ ๋ฅผ [`~TFPreTrainedModel.prepare_tf_dataset`]์ ์ ๋ฌํ์ธ์. ๋ฐฐ์น ํฌ๊ธฐ๋ฅผ ๋ณ๊ฒฝํ๊ฑฐ๋ ๋ฐ์ดํฐ์
์ ์์ ์๋ ์์ต๋๋ค:
```py
>>> dataset = dataset.map(tokenize_dataset) # doctest: +SKIP
>>> tf_dataset = model.prepare_tf_dataset(
... dataset["train"], batch_size=16, shuffle=True, tokenizer=tokenizer
... ) # doctest: +SKIP
```
5. ์ค๋น๋์์ผ๋ฉด `compile` ๋ฐ `fit`๋ฅผ ํธ์ถํ์ฌ ํ๋ จ์ ์์ํ์ธ์. ๐ค Transformers์ ๋ชจ๋ ๋ชจ๋ธ์ ๊ณผ์
๊ณผ ๊ด๋ จ๋ ๊ธฐ๋ณธ ์์ค ํจ์๋ฅผ ๊ฐ์ง๊ณ ์์ผ๋ฏ๋ก ๋ช
์์ ์ผ๋ก ์ง์ ํ์ง ์์๋ ๋ฉ๋๋ค:
```py
>>> from tensorflow.keras.optimizers import Adam
>>> model.compile(optimizer=Adam(3e-5)) # No loss argument!
>>> model.fit(tf_dataset) # doctest: +SKIP
```
## ๋ค์ ๋จ๊ณ๋ ๋ฌด์์ธ๊ฐ์? [[whats-next]]
๐ค Transformers ๋๋ฌ๋ณด๊ธฐ๋ฅผ ๋ชจ๋ ์ฝ์ผ์
จ๋ค๋ฉด, ๊ฐ์ด๋๋ฅผ ์ดํด๋ณด๊ณ ๋ ๊ตฌ์ฒด์ ์ธ ๊ฒ์ ์ํํ๋ ๋ฐฉ๋ฒ์ ์์๋ณด์ธ์. ์ด๋ฅผํ
๋ฉด ์ปค์คํ
๋ชจ๋ธ ๊ตฌ์ถํ๋ ๋ฐฉ๋ฒ, ๊ณผ์
์ ์๋ง๊ฒ ๋ชจ๋ธ์ ๋ฏธ์ธ์กฐ์ ํ๋ ๋ฐฉ๋ฒ, ์คํฌ๋ฆฝํธ๋ก ๋ชจ๋ธ ํ๋ จํ๋ ๋ฐฉ๋ฒ ๋ฑ์ด ์์ต๋๋ค. ๐ค Transformers ํต์ฌ ๊ฐ๋
์ ๋ํด ๋ ์์๋ณด๋ ค๋ฉด ์ปคํผ ํ ์ ๋ค๊ณ ๊ฐ๋
๊ฐ์ด๋๋ฅผ ์ดํด๋ณด์ธ์! | 0 |
hf_public_repos/transformers/docs/source | hf_public_repos/transformers/docs/source/ko/run_scripts.md | <!--Copyright 2022 The HuggingFace Team. All rights reserved.
Licensed under the Apache License, Version 2.0 (the "License"); you may not use this file except in compliance with
the License. You may obtain a copy of the License at
http://www.apache.org/licenses/LICENSE-2.0
Unless required by applicable law or agreed to in writing, software distributed under the License is distributed on
an "AS IS" BASIS, WITHOUT WARRANTIES OR CONDITIONS OF ANY KIND, either express or implied. See the License for the
specific language governing permissions and limitations under the License.
โ ๏ธ Note that this file is in Markdown but contain specific syntax for our doc-builder (similar to MDX) that may not be
rendered properly in your Markdown viewer.
-->
# ์คํฌ๋ฆฝํธ๋ก ์คํํ๊ธฐ[[train-with-a-script]]
๐ค Transformers ๋
ธํธ๋ถ๊ณผ ํจ๊ป [PyTorch](https://github.com/huggingface/transformers/tree/main/examples/pytorch), [TensorFlow](https://github.com/huggingface/transformers/tree/main/examples/tensorflow), ๋๋ [JAX/Flax](https://github.com/huggingface/transformers/tree/main/examples/flax)๋ฅผ ์ฌ์ฉํด ํน์ ํ์คํฌ์ ๋ํ ๋ชจ๋ธ์ ํ๋ จํ๋ ๋ฐฉ๋ฒ์ ๋ณด์ฌ์ฃผ๋ ์์ ์คํฌ๋ฆฝํธ๋ ์์ต๋๋ค.
๋ํ [์ฐ๊ตฌ ํ๋ก์ ํธ](https://github.com/huggingface/transformers/tree/main/examples/research_projects) ๋ฐ [๋ ๊ฑฐ์ ์์ ](https://github.com/huggingface/transformers/tree/main/examples/legacy)์์ ๋๋ถ๋ถ ์ปค๋ฎค๋ํฐ์์ ์ ๊ณตํ ์คํฌ๋ฆฝํธ๋ฅผ ์ฐพ์ ์ ์์ต๋๋ค.
์ด๋ฌํ ์คํฌ๋ฆฝํธ๋ ์ ๊ทน์ ์ผ๋ก ์ ์ง ๊ด๋ฆฌ๋์ง ์์ผ๋ฉฐ ์ต์ ๋ฒ์ ์ ๋ผ์ด๋ธ๋ฌ๋ฆฌ์ ํธํ๋์ง ์์ ๊ฐ๋ฅ์ฑ์ด ๋์ ํน์ ๋ฒ์ ์ ๐ค Transformers๋ฅผ ํ์๋ก ํฉ๋๋ค.
์์ ์คํฌ๋ฆฝํธ๊ฐ ๋ชจ๋ ๋ฌธ์ ์์ ๋ฐ๋ก ์๋ํ๋ ๊ฒ์ ์๋๋ฉฐ, ํด๊ฒฐํ๋ ค๋ ๋ฌธ์ ์ ๋ง๊ฒ ์คํฌ๋ฆฝํธ๋ฅผ ๋ณ๊ฒฝํด์ผ ํ ์๋ ์์ต๋๋ค.
์ด๋ฅผ ์ํด ๋๋ถ๋ถ์ ์คํฌ๋ฆฝํธ์๋ ๋ฐ์ดํฐ ์ ์ฒ๋ฆฌ ๋ฐฉ๋ฒ์ด ๋์์์ด ํ์์ ๋ฐ๋ผ ์์ ํ ์ ์์ต๋๋ค.
์์ ์คํฌ๋ฆฝํธ์ ๊ตฌํํ๊ณ ์ถ์ ๊ธฐ๋ฅ์ด ์์ผ๋ฉด pull request๋ฅผ ์ ์ถํ๊ธฐ ์ ์ [ํฌ๋ผ](https://discuss.huggingface.co/) ๋๋ [์ด์](https://github.com/huggingface/transformers/issues)์์ ๋
ผ์ํด ์ฃผ์ธ์.
๋ฒ๊ทธ ์์ ์ ํ์ํ์ง๋ง ๊ฐ๋
์ฑ์ ํฌ์ํ๋ฉด์๊น์ง ๋ ๋ง์ ๊ธฐ๋ฅ์ ์ถ๊ฐํ๋ pull request๋ ๋ณํฉ(merge)ํ์ง ์์ ๊ฐ๋ฅ์ฑ์ด ๋์ต๋๋ค.
์ด ๊ฐ์ด๋์์๋ [PyTorch](https://github.com/huggingface/transformers/tree/main/examples/pytorch/summarization) ๋ฐ [TensorFlow](https://github.com/huggingface/transformers/tree/main/examples/tensorflow/summarization)์์ ์์ฝ ํ๋ จํ๋
์คํฌ๋ฆฝํธ ์์ ๋ฅผ ์คํํ๋ ๋ฐฉ๋ฒ์ ์ค๋ช
ํฉ๋๋ค.
ํน๋ณํ ์ค๋ช
์ด ์๋ ํ ๋ชจ๋ ์์ ๋ ๋ ํ๋ ์์ํฌ ๋ชจ๋์์ ์๋ํ ๊ฒ์ผ๋ก ์์๋ฉ๋๋ค.
## ์ค์ ํ๊ธฐ[[setup]]
์ต์ ๋ฒ์ ์ ์์ ์คํฌ๋ฆฝํธ๋ฅผ ์ฑ๊ณต์ ์ผ๋ก ์คํํ๋ ค๋ฉด ์ ๊ฐ์ ํ๊ฒฝ์์ **์์ค๋ก๋ถํฐ ๐ค Transformers๋ฅผ ์ค์น**ํด์ผ ํฉ๋๋ค:
```bash
git clone https://github.com/huggingface/transformers
cd transformers
pip install .
```
์ด์ ๋ฒ์ ์ ์์ ์คํฌ๋ฆฝํธ๋ฅผ ๋ณด๋ ค๋ฉด ์๋ ํ ๊ธ์ ํด๋ฆญํ์ธ์:
<details>
<summary>์ด์ ๋ฒ์ ์ ๐ค Transformers ์์ </summary>
<ul>
<li><a href="https://github.com/huggingface/transformers/tree/v4.5.1/examples">v4.5.1</a></li>
<li><a href="https://github.com/huggingface/transformers/tree/v4.4.2/examples">v4.4.2</a></li>
<li><a href="https://github.com/huggingface/transformers/tree/v4.3.3/examples">v4.3.3</a></li>
<li><a href="https://github.com/huggingface/transformers/tree/v4.2.2/examples">v4.2.2</a></li>
<li><a href="https://github.com/huggingface/transformers/tree/v4.1.1/examples">v4.1.1</a></li>
<li><a href="https://github.com/huggingface/transformers/tree/v4.0.1/examples">v4.0.1</a></li>
<li><a href="https://github.com/huggingface/transformers/tree/v3.5.1/examples">v3.5.1</a></li>
<li><a href="https://github.com/huggingface/transformers/tree/v3.4.0/examples">v3.4.0</a></li>
<li><a href="https://github.com/huggingface/transformers/tree/v3.3.1/examples">v3.3.1</a></li>
<li><a href="https://github.com/huggingface/transformers/tree/v3.2.0/examples">v3.2.0</a></li>
<li><a href="https://github.com/huggingface/transformers/tree/v3.1.0/examples">v3.1.0</a></li>
<li><a href="https://github.com/huggingface/transformers/tree/v3.0.2/examples">v3.0.2</a></li>
<li><a href="https://github.com/huggingface/transformers/tree/v2.11.0/examples">v2.11.0</a></li>
<li><a href="https://github.com/huggingface/transformers/tree/v2.10.0/examples">v2.10.0</a></li>
<li><a href="https://github.com/huggingface/transformers/tree/v2.9.1/examples">v2.9.1</a></li>
<li><a href="https://github.com/huggingface/transformers/tree/v2.8.0/examples">v2.8.0</a></li>
<li><a href="https://github.com/huggingface/transformers/tree/v2.7.0/examples">v2.7.0</a></li>
<li><a href="https://github.com/huggingface/transformers/tree/v2.6.0/examples">v2.6.0</a></li>
<li><a href="https://github.com/huggingface/transformers/tree/v2.5.1/examples">v2.5.1</a></li>
<li><a href="https://github.com/huggingface/transformers/tree/v2.4.0/examples">v2.4.0</a></li>
<li><a href="https://github.com/huggingface/transformers/tree/v2.3.0/examples">v2.3.0</a></li>
<li><a href="https://github.com/huggingface/transformers/tree/v2.2.0/examples">v2.2.0</a></li>
<li><a href="https://github.com/huggingface/transformers/tree/v2.1.0/examples">v2.1.1</a></li>
<li><a href="https://github.com/huggingface/transformers/tree/v2.0.0/examples">v2.0.0</a></li>
<li><a href="https://github.com/huggingface/transformers/tree/v1.2.0/examples">v1.2.0</a></li>
<li><a href="https://github.com/huggingface/transformers/tree/v1.1.0/examples">v1.1.0</a></li>
<li><a href="https://github.com/huggingface/transformers/tree/v1.0.0/examples">v1.0.0</a></li>
</ul>
</details>
๊ทธ๋ฆฌ๊ณ ๋ค์๊ณผ ๊ฐ์ด ๋ณต์ (clone)ํด์จ ๐ค Transformers ๋ฒ์ ์ ํน์ ๋ฒ์ (์: v3.5.1)์ผ๋ก ์ ํํ์ธ์:
```bash
git checkout tags/v3.5.1
```
์ฌ๋ฐ๋ฅธ ๋ผ์ด๋ธ๋ฌ๋ฆฌ ๋ฒ์ ์ ์ค์ ํ ํ ์ํ๋ ์์ ํด๋๋ก ์ด๋ํ์ฌ ์์ ๋ณ๋ก ๋ผ์ด๋ธ๋ฌ๋ฆฌ์ ๋ํ ์๊ตฌ ์ฌํญ(requirements)์ ์ค์นํฉ๋๋ค:
```bash
pip install -r requirements.txt
```
## ์คํฌ๋ฆฝํธ ์คํํ๊ธฐ[[run-a-script]]
<frameworkcontent>
<pt>
์์ ์คํฌ๋ฆฝํธ๋ ๐ค [Datasets](https://huggingface.co/docs/datasets/) ๋ผ์ด๋ธ๋ฌ๋ฆฌ์์ ๋ฐ์ดํฐ ์ธํธ๋ฅผ ๋ค์ด๋ก๋ํ๊ณ ์ ์ฒ๋ฆฌํฉ๋๋ค.
๊ทธ๋ฐ ๋ค์ ์คํฌ๋ฆฝํธ๋ ์์ฝ ๊ธฐ๋ฅ์ ์ง์ํ๋ ์ํคํ
์ฒ์์ [Trainer](https://huggingface.co/docs/transformers/main_classes/trainer)๋ฅผ ์ฌ์ฉํ์ฌ ๋ฐ์ดํฐ ์ธํธ๋ฅผ ๋ฏธ์ธ ์กฐ์ ํฉ๋๋ค.
๋ค์ ์๋ [CNN/DailyMail](https://huggingface.co/datasets/cnn_dailymail) ๋ฐ์ดํฐ ์ธํธ์์ [T5-small](https://huggingface.co/t5-small)์ ๋ฏธ์ธ ์กฐ์ ํฉ๋๋ค.
T5 ๋ชจ๋ธ์ ํ๋ จ ๋ฐฉ์์ ๋ฐ๋ผ ์ถ๊ฐ `source_prefix` ์ธ์๊ฐ ํ์ํ๋ฉฐ, ์ด ํ๋กฌํํธ๋ ์์ฝ ์์
์์ T5์ ์๋ ค์ค๋๋ค.
```bash
python examples/pytorch/summarization/run_summarization.py \
--model_name_or_path t5-small \
--do_train \
--do_eval \
--dataset_name cnn_dailymail \
--dataset_config "3.0.0" \
--source_prefix "summarize: " \
--output_dir /tmp/tst-summarization \
--per_device_train_batch_size=4 \
--per_device_eval_batch_size=4 \
--overwrite_output_dir \
--predict_with_generate
```
</pt>
<tf>
์์ ์คํฌ๋ฆฝํธ๋ ๐ค [Datasets](https://huggingface.co/docs/datasets/) ๋ผ์ด๋ธ๋ฌ๋ฆฌ์์ ๋ฐ์ดํฐ ์ธํธ๋ฅผ ๋ค์ด๋ก๋ํ๊ณ ์ ์ฒ๋ฆฌํฉ๋๋ค.
๊ทธ๋ฐ ๋ค์ ์คํฌ๋ฆฝํธ๋ ์์ฝ ๊ธฐ๋ฅ์ ์ง์ํ๋ ์ํคํ
์ฒ์์ Keras๋ฅผ ์ฌ์ฉํ์ฌ ๋ฐ์ดํฐ ์ธํธ๋ฅผ ๋ฏธ์ธ ์กฐ์ ํฉ๋๋ค.
๋ค์ ์๋ [CNN/DailyMail](https://huggingface.co/datasets/cnn_dailymail) ๋ฐ์ดํฐ ์ธํธ์์ [T5-small](https://huggingface.co/t5-small)์ ๋ฏธ์ธ ์กฐ์ ํฉ๋๋ค.
T5 ๋ชจ๋ธ์ ํ๋ จ ๋ฐฉ์์ ๋ฐ๋ผ ์ถ๊ฐ `source_prefix` ์ธ์๊ฐ ํ์ํ๋ฉฐ, ์ด ํ๋กฌํํธ๋ ์์ฝ ์์
์์ T5์ ์๋ ค์ค๋๋ค.
```bash
python examples/tensorflow/summarization/run_summarization.py \
--model_name_or_path t5-small \
--dataset_name cnn_dailymail \
--dataset_config "3.0.0" \
--output_dir /tmp/tst-summarization \
--per_device_train_batch_size 8 \
--per_device_eval_batch_size 16 \
--num_train_epochs 3 \
--do_train \
--do_eval
```
</tf>
</frameworkcontent>
## ํผํฉ ์ ๋ฐ๋(mixed precision)๋ก ๋ถ์ฐ ํ๋ จํ๊ธฐ[[distributed-training-and-mixed-precision]]
[Trainer](https://huggingface.co/docs/transformers/main_classes/trainer) ํด๋์ค๋ ๋ถ์ฐ ํ๋ จ๊ณผ ํผํฉ ์ ๋ฐ๋(mixed precision)๋ฅผ ์ง์ํ๋ฏ๋ก ์คํฌ๋ฆฝํธ์์๋ ์ฌ์ฉํ ์ ์์ต๋๋ค.
์ด ๋ ๊ฐ์ง ๊ธฐ๋ฅ์ ๋ชจ๋ ํ์ฑํํ๋ ค๋ฉด ๋ค์ ๋ ๊ฐ์ง๋ฅผ ์ค์ ํด์ผ ํฉ๋๋ค:
- `fp16` ์ธ์๋ฅผ ์ถ๊ฐํด ํผํฉ ์ ๋ฐ๋(mixed precision)๋ฅผ ํ์ฑํํฉ๋๋ค.
- `nproc_per_node` ์ธ์๋ฅผ ์ถ๊ฐํด ์ฌ์ฉํ GPU ๊ฐ์๋ฅผ ์ค์ ํฉ๋๋ค.
```bash
python -m torch.distributed.launch \
--nproc_per_node 8 pytorch/summarization/run_summarization.py \
--fp16 \
--model_name_or_path t5-small \
--do_train \
--do_eval \
--dataset_name cnn_dailymail \
--dataset_config "3.0.0" \
--source_prefix "summarize: " \
--output_dir /tmp/tst-summarization \
--per_device_train_batch_size=4 \
--per_device_eval_batch_size=4 \
--overwrite_output_dir \
--predict_with_generate
```
TensorFlow ์คํฌ๋ฆฝํธ๋ ๋ถ์ฐ ํ๋ จ์ ์ํด [`MirroredStrategy`](https://www.tensorflow.org/guide/distributed_training#mirroredstrategy)๋ฅผ ํ์ฉํ๋ฉฐ, ํ๋ จ ์คํฌ๋ฆฝํธ์ ์ธ์๋ฅผ ์ถ๊ฐํ ํ์๊ฐ ์์ต๋๋ค.
๋ค์ค GPU ํ๊ฒฝ์ด๋ผ๋ฉด, TensorFlow ์คํฌ๋ฆฝํธ๋ ๊ธฐ๋ณธ์ ์ผ๋ก ์ฌ๋ฌ ๊ฐ์ GPU๋ฅผ ์ฌ์ฉํฉ๋๋ค.
## TPU ์์์ ์คํฌ๋ฆฝํธ ์คํํ๊ธฐ[[run-a-script-on-a-tpu]]
<frameworkcontent>
<pt>
Tensor Processing Units (TPUs)๋ ์ฑ๋ฅ์ ๊ฐ์ํํ๊ธฐ ์ํด ํน๋ณํ ์ค๊ณ๋์์ต๋๋ค.
PyTorch๋ [XLA](https://www.tensorflow.org/xla) ๋ฅ๋ฌ๋ ์ปดํ์ผ๋ฌ์ ํจ๊ป TPU๋ฅผ ์ง์ํฉ๋๋ค(์์ธํ ๋ด์ฉ์ [์ฌ๊ธฐ](https://github.com/pytorch/xla/blob/master/README.md) ์ฐธ์กฐ).
TPU๋ฅผ ์ฌ์ฉํ๋ ค๋ฉด `xla_spawn.py` ์คํฌ๋ฆฝํธ๋ฅผ ์คํํ๊ณ `num_cores` ์ธ์๋ฅผ ์ฌ์ฉํ์ฌ ์ฌ์ฉํ๋ ค๋ TPU ์ฝ์ด ์๋ฅผ ์ค์ ํฉ๋๋ค.
```bash
python xla_spawn.py --num_cores 8 \
summarization/run_summarization.py \
--model_name_or_path t5-small \
--do_train \
--do_eval \
--dataset_name cnn_dailymail \
--dataset_config "3.0.0" \
--source_prefix "summarize: " \
--output_dir /tmp/tst-summarization \
--per_device_train_batch_size=4 \
--per_device_eval_batch_size=4 \
--overwrite_output_dir \
--predict_with_generate
```
</pt>
<tf>
Tensor Processing Units (TPUs)๋ ์ฑ๋ฅ์ ๊ฐ์ํํ๊ธฐ ์ํด ํน๋ณํ ์ค๊ณ๋์์ต๋๋ค.
TensorFlow ์คํฌ๋ฆฝํธ๋ TPU๋ฅผ ํ๋ จ์ ์ฌ์ฉํ๊ธฐ ์ํด [`TPUStrategy`](https://www.tensorflow.org/guide/distributed_training#tpustrategy)๋ฅผ ํ์ฉํฉ๋๋ค.
TPU๋ฅผ ์ฌ์ฉํ๋ ค๋ฉด TPU ๋ฆฌ์์ค์ ์ด๋ฆ์ `tpu` ์ธ์์ ์ ๋ฌํฉ๋๋ค.
```bash
python run_summarization.py \
--tpu name_of_tpu_resource \
--model_name_or_path t5-small \
--dataset_name cnn_dailymail \
--dataset_config "3.0.0" \
--output_dir /tmp/tst-summarization \
--per_device_train_batch_size 8 \
--per_device_eval_batch_size 16 \
--num_train_epochs 3 \
--do_train \
--do_eval
```
</tf>
</frameworkcontent>
## ๐ค Accelerate๋ก ์คํฌ๋ฆฝํธ ์คํํ๊ธฐ[[run-a-script-with-accelerate]]
๐ค [Accelerate](https://huggingface.co/docs/accelerate)๋ PyTorch ํ๋ จ ๊ณผ์ ์ ๋ํ ์์ ํ ๊ฐ์์ฑ์ ์ ์งํ๋ฉด์ ์ฌ๋ฌ ์ ํ์ ์ค์ (CPU ์ ์ฉ, ๋ค์ค GPU, TPU)์์ ๋ชจ๋ธ์ ํ๋ จํ ์ ์๋ ํตํฉ ๋ฐฉ๋ฒ์ ์ ๊ณตํ๋ PyTorch ์ ์ฉ ๋ผ์ด๋ธ๋ฌ๋ฆฌ์
๋๋ค.
๐ค Accelerate๊ฐ ์ค์น๋์ด ์๋์ง ํ์ธํ์ธ์:
> ์ฐธ๊ณ : Accelerate๋ ๋น ๋ฅด๊ฒ ๊ฐ๋ฐ ์ค์ด๋ฏ๋ก ์คํฌ๋ฆฝํธ๋ฅผ ์คํํ๋ ค๋ฉด accelerate๋ฅผ ์ค์นํด์ผ ํฉ๋๋ค.
```bash
pip install git+https://github.com/huggingface/accelerate
```
`run_summarization.py` ์คํฌ๋ฆฝํธ ๋์ `run_summarization_no_trainer.py` ์คํฌ๋ฆฝํธ๋ฅผ ์ฌ์ฉํด์ผ ํฉ๋๋ค.
๐ค Accelerate ํด๋์ค๊ฐ ์ง์๋๋ ์คํฌ๋ฆฝํธ๋ ํด๋์ `task_no_trainer.py` ํ์ผ์ด ์์ต๋๋ค.
๋ค์ ๋ช
๋ น์ ์คํํ์ฌ ๊ตฌ์ฑ ํ์ผ์ ์์ฑํ๊ณ ์ ์ฅํฉ๋๋ค:
```bash
accelerate config
```
์ค์ ์ ํ
์คํธํ์ฌ ์ฌ๋ฐ๋ฅด๊ฒ ๊ตฌ์ฑ๋์๋์ง ํ์ธํฉ๋๋ค:
```bash
accelerate test
```
์ด์ ํ๋ จ์ ์์ํ ์ค๋น๊ฐ ๋์์ต๋๋ค:
```bash
accelerate launch run_summarization_no_trainer.py \
--model_name_or_path t5-small \
--dataset_name cnn_dailymail \
--dataset_config "3.0.0" \
--source_prefix "summarize: " \
--output_dir ~/tmp/tst-summarization
```
## ์ฌ์ฉ์ ์ ์ ๋ฐ์ดํฐ ์ธํธ ์ฌ์ฉํ๊ธฐ[[use-a-custom-dataset]]
์์ฝ ์คํฌ๋ฆฝํธ๋ ์ฌ์ฉ์ ์ง์ ๋ฐ์ดํฐ ์ธํธ๊ฐ CSV ๋๋ JSON ํ์ผ์ธ ๊ฒฝ์ฐ ์ง์ํฉ๋๋ค.
์ฌ์ฉ์ ์ง์ ๋ฐ์ดํฐ ์ธํธ๋ฅผ ์ฌ์ฉํ๋ ๊ฒฝ์ฐ์๋ ๋ช ๊ฐ์ง ์ถ๊ฐ ์ธ์๋ฅผ ์ง์ ํด์ผ ํฉ๋๋ค:
- `train_file`๊ณผ `validation_file`์ ํ๋ จ ๋ฐ ๊ฒ์ฆ ํ์ผ์ ๊ฒฝ๋ก๋ฅผ ์ง์ ํฉ๋๋ค.
- `text_column`์ ์์ฝํ ์
๋ ฅ ํ
์คํธ์
๋๋ค.
- `summary_column`์ ์ถ๋ ฅํ ๋์ ํ
์คํธ์
๋๋ค.
์ฌ์ฉ์ ์ง์ ๋ฐ์ดํฐ ์ธํธ๋ฅผ ์ฌ์ฉํ๋ ์์ฝ ์คํฌ๋ฆฝํธ๋ ๋ค์๊ณผ ๊ฐ์ต๋๋ค:
```bash
python examples/pytorch/summarization/run_summarization.py \
--model_name_or_path t5-small \
--do_train \
--do_eval \
--train_file path_to_csv_or_jsonlines_file \
--validation_file path_to_csv_or_jsonlines_file \
--text_column text_column_name \
--summary_column summary_column_name \
--source_prefix "summarize: " \
--output_dir /tmp/tst-summarization \
--overwrite_output_dir \
--per_device_train_batch_size=4 \
--per_device_eval_batch_size=4 \
--predict_with_generate
```
## ์คํฌ๋ฆฝํธ ํ
์คํธํ๊ธฐ[[test-a-script]]
์ ์ฒด ๋ฐ์ดํฐ ์ธํธ๋ฅผ ๋์์ผ๋ก ํ๋ จ์ ์๋ฃํ๋๋ฐ ๊ฝค ์ค๋ ์๊ฐ์ด ๊ฑธ๋ฆฌ๊ธฐ ๋๋ฌธ์, ์์ ๋ฐ์ดํฐ ์ธํธ์์ ๋ชจ๋ ๊ฒ์ด ์์๋๋ก ์คํ๋๋์ง ํ์ธํ๋ ๊ฒ์ด ์ข์ต๋๋ค.
๋ค์ ์ธ์๋ฅผ ์ฌ์ฉํ์ฌ ๋ฐ์ดํฐ ์ธํธ๋ฅผ ์ต๋ ์ํ ์๋ก ์๋ผ๋
๋๋ค:
- `max_train_samples`
- `max_eval_samples`
- `max_predict_samples`
```bash
python examples/pytorch/summarization/run_summarization.py \
--model_name_or_path t5-small \
--max_train_samples 50 \
--max_eval_samples 50 \
--max_predict_samples 50 \
--do_train \
--do_eval \
--dataset_name cnn_dailymail \
--dataset_config "3.0.0" \
--source_prefix "summarize: " \
--output_dir /tmp/tst-summarization \
--per_device_train_batch_size=4 \
--per_device_eval_batch_size=4 \
--overwrite_output_dir \
--predict_with_generate
```
๋ชจ๋ ์์ ์คํฌ๋ฆฝํธ๊ฐ `max_predict_samples` ์ธ์๋ฅผ ์ง์ํ์ง๋ ์์ต๋๋ค.
์คํฌ๋ฆฝํธ๊ฐ ์ด ์ธ์๋ฅผ ์ง์ํ๋์ง ํ์คํ์ง ์์ ๊ฒฝ์ฐ `-h` ์ธ์๋ฅผ ์ถ๊ฐํ์ฌ ํ์ธํ์ธ์:
```bash
examples/pytorch/summarization/run_summarization.py -h
```
## ์ฒดํฌํฌ์ธํธ(checkpoint)์์ ํ๋ จ ์ด์ด์ ํ๊ธฐ[[resume-training-from-checkpoint]]
๋ ๋ค๋ฅธ ์ ์ฉํ ์ต์
์ ์ด์ ์ฒดํฌํฌ์ธํธ์์ ํ๋ จ์ ์ฌ๊ฐํ๋ ๊ฒ์
๋๋ค.
์ด๋ ๊ฒ ํ๋ฉด ํ๋ จ์ด ์ค๋จ๋๋๋ผ๋ ์ฒ์๋ถํฐ ๋ค์ ์์ํ์ง ์๊ณ ์ค๋จํ ๋ถ๋ถ๋ถํฐ ๋ค์ ์์ํ ์ ์์ต๋๋ค.
์ฒดํฌํฌ์ธํธ์์ ํ๋ จ์ ์ฌ๊ฐํ๋ ๋ฐฉ๋ฒ์๋ ๋ ๊ฐ์ง๊ฐ ์์ต๋๋ค.
์ฒซ ๋ฒ์งธ๋ `output_dir previous_output_dir` ์ธ์๋ฅผ ์ฌ์ฉํ์ฌ `output_dir`์ ์ ์ฅ๋ ์ต์ ์ฒดํฌํฌ์ธํธ๋ถํฐ ํ๋ จ์ ์ฌ๊ฐํ๋ ๋ฐฉ๋ฒ์
๋๋ค.
์ด ๊ฒฝ์ฐ `overwrite_output_dir`์ ์ ๊ฑฐํด์ผ ํฉ๋๋ค:
```bash
python examples/pytorch/summarization/run_summarization.py
--model_name_or_path t5-small \
--do_train \
--do_eval \
--dataset_name cnn_dailymail \
--dataset_config "3.0.0" \
--source_prefix "summarize: " \
--output_dir /tmp/tst-summarization \
--per_device_train_batch_size=4 \
--per_device_eval_batch_size=4 \
--output_dir previous_output_dir \
--predict_with_generate
```
๋ ๋ฒ์งธ๋ `resume_from_checkpoint path_to_specific_checkpoint` ์ธ์๋ฅผ ์ฌ์ฉํ์ฌ ํน์ ์ฒดํฌํฌ์ธํธ ํด๋์์ ํ๋ จ์ ์ฌ๊ฐํ๋ ๋ฐฉ๋ฒ์
๋๋ค.
```bash
python examples/pytorch/summarization/run_summarization.py
--model_name_or_path t5-small \
--do_train \
--do_eval \
--dataset_name cnn_dailymail \
--dataset_config "3.0.0" \
--source_prefix "summarize: " \
--output_dir /tmp/tst-summarization \
--per_device_train_batch_size=4 \
--per_device_eval_batch_size=4 \
--overwrite_output_dir \
--resume_from_checkpoint path_to_specific_checkpoint \
--predict_with_generate
```
## ๋ชจ๋ธ ๊ณต์ ํ๊ธฐ[[share-your-model]]
๋ชจ๋ ์คํฌ๋ฆฝํธ๋ ์ต์ข
๋ชจ๋ธ์ [Model Hub](https://huggingface.co/models)์ ์
๋ก๋ํ ์ ์์ต๋๋ค.
์์ํ๊ธฐ ์ ์ Hugging Face์ ๋ก๊ทธ์ธํ๋์ง ํ์ธํ์ธ์:
```bash
huggingface-cli login
```
๊ทธ๋ฐ ๋ค์ ์คํฌ๋ฆฝํธ์ `push_to_hub` ์ธ์๋ฅผ ์ถ๊ฐํฉ๋๋ค.
์ด ์ธ์๋ Hugging Face ์ฌ์ฉ์ ์ด๋ฆ๊ณผ `output_dir`์ ์ง์ ๋ ํด๋ ์ด๋ฆ์ผ๋ก ์ ์ฅ์๋ฅผ ์์ฑํฉ๋๋ค.
์ ์ฅ์์ ํน์ ์ด๋ฆ์ ์ง์ ํ๋ ค๋ฉด `push_to_hub_model_id` ์ธ์๋ฅผ ์ฌ์ฉํ์ฌ ์ถ๊ฐํฉ๋๋ค.
์ ์ฅ์๋ ๋ค์์คํ์ด์ค ์๋์ ์๋์ผ๋ก ๋์ด๋ฉ๋๋ค.
๋ค์ ์๋ ํน์ ์ ์ฅ์ ์ด๋ฆ์ผ๋ก ๋ชจ๋ธ์ ์
๋ก๋ํ๋ ๋ฐฉ๋ฒ์
๋๋ค:
```bash
python examples/pytorch/summarization/run_summarization.py
--model_name_or_path t5-small \
--do_train \
--do_eval \
--dataset_name cnn_dailymail \
--dataset_config "3.0.0" \
--source_prefix "summarize: " \
--push_to_hub \
--push_to_hub_model_id finetuned-t5-cnn_dailymail \
--output_dir /tmp/tst-summarization \
--per_device_train_batch_size=4 \
--per_device_eval_batch_size=4 \
--overwrite_output_dir \
--predict_with_generate
``` | 0 |
hf_public_repos/transformers/docs/source | hf_public_repos/transformers/docs/source/ko/troubleshooting.md | <!---
Copyright 2022 The HuggingFace Team. All rights reserved.
Licensed under the Apache License, Version 2.0 (the "License");
you may not use this file except in compliance with the License.
You may obtain a copy of the License at
http://www.apache.org/licenses/LICENSE-2.0
Unless required by applicable law or agreed to in writing, software
distributed under the License is distributed on an "AS IS" BASIS,
WITHOUT WARRANTIES OR CONDITIONS OF ANY KIND, either express or implied.
See the License for the specific language governing permissions and
limitations under the License.
โ ๏ธ Note that this file is in Markdown but contain specific syntax for our doc-builder (similar to MDX) that may not be
rendered properly in your Markdown viewer.
-->
# ๋ฌธ์ ํด๊ฒฐ[[troubleshoot]]
๋๋๋ก ์ค๋ฅ๊ฐ ๋ฐ์ํ ์ ์์ง๋ง, ์ ํฌ๊ฐ ๋์๋๋ฆฌ๊ฒ ์ต๋๋ค! ์ด ๊ฐ์ด๋๋ ํ์ฌ๊น์ง ํ์ธ๋ ๊ฐ์ฅ ์ผ๋ฐ์ ์ธ ๋ฌธ์ ๋ช ๊ฐ์ง์ ๊ทธ๊ฒ๋ค์ ํด๊ฒฐํ๋ ๋ฐฉ๋ฒ์ ๋ํด ๋ค๋ฃน๋๋ค. ๊ทธ๋ฌ๋ ์ด ๊ฐ์ด๋๋ ๋ชจ๋ ๐ค Transformers ๋ฌธ์ ๋ฅผ ํฌ๊ด์ ์ผ๋ก ๋ค๋ฃจ๊ณ ์์ง ์์ต๋๋ค. ๋ฌธ์ ํด๊ฒฐ์ ๋ ๋ง์ ๋์์ ๋ฐ์ผ๋ ค๋ฉด ๋ค์์ ์๋ํด๋ณด์ธ์:
<Youtube id="S2EEG3JIt2A"/>
1. [ํฌ๋ผ](https://discuss.huggingface.co/)์์ ๋์์ ์์ฒญํ์ธ์. [Beginners](https://discuss.huggingface.co/c/beginners/5) ๋๋ [๐ค Transformers](https://discuss.huggingface.co/c/transformers/9)์ ๊ฐ์ ํน์ ์นดํ
๊ณ ๋ฆฌ์ ์ง๋ฌธ์ ๊ฒ์ํ ์ ์์ต๋๋ค. ์ฌํ ๊ฐ๋ฅํ ์ฝ๋์ ํจ๊ป ์ ์์ ๋ ํฌ๋ผ ๊ฒ์๋ฌผ์ ์์ฑํ์ฌ ์ฌ๋ฌ๋ถ์ ๋ฌธ์ ๊ฐ ํด๊ฒฐ๋ ๊ฐ๋ฅ์ฑ์ ๊ทน๋ํํ์ธ์!
<Youtube id="_PAli-V4wj0"/>
2. ๋ผ์ด๋ธ๋ฌ๋ฆฌ์ ๊ด๋ จ๋ ๋ฒ๊ทธ์ด๋ฉด ๐ค Transformers ์ ์ฅ์์์ [์ด์](https://github.com/huggingface/transformers/issues/new/choose)๋ฅผ ์์ฑํ์ธ์. ๋ฒ๊ทธ์ ๋ํด ์ค๋ช
ํ๋ ์ ๋ณด๋ฅผ ๊ฐ๋ฅํ ๋ง์ด ํฌํจํ๋ ค๊ณ ๋
ธ๋ ฅํ์ฌ, ๋ฌด์์ด ์๋ชป ๋์๋์ง์ ์ด๋ป๊ฒ ์์ ํ ์ ์๋์ง ๋ ์ ํ์
ํ ์ ์๋๋ก ๋์์ฃผ์ธ์.
3. ์ด์ ๋ฒ์ ์ ๐ค Transformers์ ์ฌ์ฉํ๋ ๊ฒฝ์ฐ ์ค์ํ ๋ณ๊ฒฝ ์ฌํญ์ด ๋ฒ์ ์ฌ์ด์ ๋์
๋์๊ธฐ ๋๋ฌธ์ [๋ง์ด๊ทธ๋ ์ด์
](migration) ๊ฐ์ด๋๋ฅผ ํ์ธํ์ธ์.
๋ฌธ์ ํด๊ฒฐ ๋ฐ ๋์ ๋งค๋ด์ผ์ ๋ํ ์์ธํ ๋ด์ฉ์ Hugging Face ๊ฐ์ข์ [8์ฅ](https://huggingface.co/course/chapter8/1?fw=pt)์ ์ฐธ์กฐํ์ธ์.
## ๋ฐฉํ๋ฒฝ ํ๊ฒฝ[[firewalled-environments]]
ํด๋ผ์ฐ๋ ๋ฐ ๋ด๋ถ๋ง(intranet) ์ค์ ์ ์ผ๋ถ GPU ์ธ์คํด์ค๋ ์ธ๋ถ ์ฐ๊ฒฐ์ ๋ํ ๋ฐฉํ๋ฒฝ์ผ๋ก ์ฐจ๋จ๋์ด ์ฐ๊ฒฐ ์ค๋ฅ๊ฐ ๋ฐ์ํ ์ ์์ต๋๋ค. ์คํฌ๋ฆฝํธ๊ฐ ๋ชจ๋ธ ๊ฐ์ค์น๋ ๋ฐ์ดํฐ๋ฅผ ๋ค์ด๋ก๋ํ๋ ค๊ณ ํ ๋, ๋ค์ด๋ก๋๊ฐ ์ค๋จ๋๊ณ ๋ค์ ๋ฉ์์ง์ ํจ๊ป ์๊ฐ ์ด๊ณผ๋ฉ๋๋ค:
```
ValueError: Connection error, and we cannot find the requested files in the cached path.
Please try again or make sure your Internet connection is on.
```
์ด ๊ฒฝ์ฐ์๋ ์ฐ๊ฒฐ ์ค๋ฅ๋ฅผ ํผํ๊ธฐ ์ํด ๐ค Transformers๋ฅผ [์คํ๋ผ์ธ ๋ชจ๋](installation#offline-mode)๋ก ์คํํด์ผ ํฉ๋๋ค.
## CUDA ๋ฉ๋ชจ๋ฆฌ ๋ถ์กฑ(CUDA out of memory)[[cuda-out-of-memory]]
์๋ฐฑ๋ง ๊ฐ์ ๋งค๊ฐ๋ณ์๋ก ๋๊ท๋ชจ ๋ชจ๋ธ์ ํ๋ จํ๋ ๊ฒ์ ์ ์ ํ ํ๋์จ์ด ์์ด ์ด๋ ค์ธ ์ ์์ต๋๋ค. GPU ๋ฉ๋ชจ๋ฆฌ๊ฐ ๋ถ์กฑํ ๊ฒฝ์ฐ ๋ฐ์ํ ์ ์๋ ์ผ๋ฐ์ ์ธ ์ค๋ฅ๋ ๋ค์๊ณผ ๊ฐ์ต๋๋ค:
```
CUDA out of memory. Tried to allocate 256.00 MiB (GPU 0; 11.17 GiB total capacity; 9.70 GiB already allocated; 179.81 MiB free; 9.85 GiB reserved in total by PyTorch)
```
๋ค์์ ๋ฉ๋ชจ๋ฆฌ ์ฌ์ฉ์ ์ค์ด๊ธฐ ์ํด ์๋ํด ๋ณผ ์ ์๋ ๋ช ๊ฐ์ง ์ ์ฌ์ ์ธ ํด๊ฒฐ์ฑ
์
๋๋ค:
- [`TrainingArguments`]์ [`per_device_train_batch_size`](main_classes/trainer#transformers.TrainingArguments.per_device_train_batch_size) ๊ฐ์ ์ค์ด์ธ์.
- [`TrainingArguments`]์ [`gradient_accumulation_steps`](main_classes/trainer#transformers.TrainingArguments.gradient_accumulation_steps)์ ์ ์ฒด ๋ฐฐ์น ํฌ๊ธฐ๋ฅผ ํจ๊ณผ์ ์ผ๋ก ๋๋ฆฌ์ธ์.
<Tip>
๋ฉ๋ชจ๋ฆฌ ์ ์ฝ ๊ธฐ์ ์ ๋ํ ์์ธํ ๋ด์ฉ์ ์ฑ๋ฅ [๊ฐ์ด๋](performance)๋ฅผ ์ฐธ์กฐํ์ธ์.
</Tip>
## ์ ์ฅ๋ TensorFlow ๋ชจ๋ธ์ ๊ฐ์ ธ์ฌ ์ ์์ต๋๋ค(Unable to load a saved TensorFlow model)[[unable-to-load-a-saved-uensorFlow-model]]
TensorFlow์ [model.save](https://www.tensorflow.org/tutorials/keras/save_and_load#save_the_entire_model) ๋ฉ์๋๋ ์ํคํ
์ฒ, ๊ฐ์ค์น, ํ๋ จ ๊ตฌ์ฑ ๋ฑ ์ ์ฒด ๋ชจ๋ธ์ ๋จ์ผ ํ์ผ์ ์ ์ฅํฉ๋๋ค. ๊ทธ๋ฌ๋ ๋ชจ๋ธ ํ์ผ์ ๋ค์ ๊ฐ์ ธ์ฌ ๋ ๐ค Transformers๋ ๋ชจ๋ธ ํ์ผ์ ์๋ ๋ชจ๋ TensorFlow ๊ด๋ จ ๊ฐ์ฒด๋ฅผ ๊ฐ์ ธ์ค์ง ์์ ์ ์๊ธฐ ๋๋ฌธ์ ์ค๋ฅ๊ฐ ๋ฐ์ํ ์ ์์ต๋๋ค. TensorFlow ๋ชจ๋ธ ์ ์ฅ ๋ฐ ๊ฐ์ ธ์ค๊ธฐ ๋ฌธ์ ๋ฅผ ํผํ๋ ค๋ฉด ๋ค์์ ๊ถ์ฅํฉ๋๋ค:
- ๋ชจ๋ธ ๊ฐ์ค์น๋ฅผ `h5` ํ์ผ ํ์ฅ์๋ก [`model.save_weights`](https://www.tensorflow.org/tutorials/keras/save_and_load#save_the_entire_model)๋ก ์ ์ฅํ ๋ค์ [`~TFPreTrainedModel.from_pretrained`]๋ก ๋ชจ๋ธ์ ๋ค์ ๊ฐ์ ธ์ต๋๋ค:
```py
>>> from transformers import TFPreTrainedModel
>>> from tensorflow import keras
>>> model.save_weights("some_folder/tf_model.h5")
>>> model = TFPreTrainedModel.from_pretrained("some_folder")
```
- ๋ชจ๋ธ์ [`~TFPretrainedModel.save_pretrained`]๋ก ์ ์ฅํ๊ณ [`~TFPreTrainedModel.from_pretrained`]๋ก ๋ค์ ๊ฐ์ ธ์ต๋๋ค:
```py
>>> from transformers import TFPreTrainedModel
>>> model.save_pretrained("path_to/model")
>>> model = TFPreTrainedModel.from_pretrained("path_to/model")
```
## ImportError[[importerror]]
ํนํ ์ต์ ๋ชจ๋ธ์ธ ๊ฒฝ์ฐ ๋ง๋ ์ ์๋ ๋ค๋ฅธ ์ผ๋ฐ์ ์ธ ์ค๋ฅ๋ `ImportError`์
๋๋ค:
```
ImportError: cannot import name 'ImageGPTImageProcessor' from 'transformers' (unknown location)
```
์ด๋ฌํ ์ค๋ฅ ์ ํ์ ๊ฒฝ์ฐ ์ต์ ๋ชจ๋ธ์ ์ก์ธ์คํ ์ ์๋๋ก ์ต์ ๋ฒ์ ์ ๐ค Transformers๊ฐ ์ค์น๋์ด ์๋์ง ํ์ธํ์ธ์:
```bash
pip install transformers --upgrade
```
## CUDA error: device-side assert triggered[[cuda-error-deviceside-assert-triggered]]
๋๋๋ก ์ฅ์น ์ฝ๋ ์ค๋ฅ์ ๋ํ ์ผ๋ฐ์ ์ธ CUDA ์ค๋ฅ๊ฐ ๋ฐ์ํ ์ ์์ต๋๋ค.
```
RuntimeError: CUDA error: device-side assert triggered
```
๋ ์์ธํ ์ค๋ฅ ๋ฉ์์ง๋ฅผ ์ป์ผ๋ ค๋ฉด ์ฐ์ ์ฝ๋๋ฅผ CPU์์ ์คํํฉ๋๋ค. ๋ค์ ํ๊ฒฝ ๋ณ์๋ฅผ ์ฝ๋์ ์์ ๋ถ๋ถ์ ์ถ๊ฐํ์ฌ CPU๋ก ์ ํํ์ธ์:
```py
>>> import os
>>> os.environ["CUDA_VISIBLE_DEVICES"] = ""
```
๋ ๋ค๋ฅธ ์ต์
์ GPU์์ ๋ ๋์ ์ญ์ถ์ (traceback)์ ์ป๋ ๊ฒ์
๋๋ค. ๋ค์ ํ๊ฒฝ ๋ณ์๋ฅผ ์ฝ๋์ ์์ ๋ถ๋ถ์ ์ถ๊ฐํ์ฌ ์ญ์ถ์ ์ด ์ค๋ฅ๊ฐ ๋ฐ์ํ ์์ค๋ฅผ ๊ฐ๋ฆฌํค๋๋ก ํ์ธ์:
```py
>>> import os
>>> os.environ["CUDA_LAUNCH_BLOCKING"] = "1"
```
## ํจ๋ฉ ํ ํฐ์ด ๋ง์คํน๋์ง ์์ ๊ฒฝ์ฐ ์๋ชป๋ ์ถ๋ ฅ(Incorrect output when padding tokens aren't masked)[[incorrect-output-when-padding-tokens-arent-masked]]
๊ฒฝ์ฐ์ ๋ฐ๋ผ `input_ids`์ ํจ๋ฉ ํ ํฐ์ด ํฌํจ๋ ๊ฒฝ์ฐ `hidden_state` ์ถ๋ ฅ์ด ์ฌ๋ฐ๋ฅด์ง ์์ ์ ์์ต๋๋ค. ๋ฐ๋ชจ๋ฅผ ์ํด ๋ชจ๋ธ๊ณผ ํ ํฌ๋์ด์ ๋ฅผ ๊ฐ์ ธ์ค์ธ์. ๋ชจ๋ธ์ `pad_token_id`์ ์ก์ธ์คํ์ฌ ํด๋น ๊ฐ์ ํ์ธํ ์ ์์ต๋๋ค. ์ผ๋ถ ๋ชจ๋ธ์ ๊ฒฝ์ฐ `pad_token_id`๊ฐ `None`์ผ ์ ์์ง๋ง ์ธ์ ๋ ์ง ์๋์ผ๋ก ์ค์ ํ ์ ์์ต๋๋ค.
```py
>>> from transformers import AutoModelForSequenceClassification
>>> import torch
>>> model = AutoModelForSequenceClassification.from_pretrained("bert-base-uncased")
>>> model.config.pad_token_id
0
```
๋ค์ ์์ ๋ ํจ๋ฉ ํ ํฐ์ ๋ง์คํนํ์ง ์์ ์ถ๋ ฅ์ ๋ณด์ฌ์ค๋๋ค:
```py
>>> input_ids = torch.tensor([[7592, 2057, 2097, 2393, 9611, 2115], [7592, 0, 0, 0, 0, 0]])
>>> output = model(input_ids)
>>> print(output.logits)
tensor([[ 0.0082, -0.2307],
[ 0.1317, -0.1683]], grad_fn=<AddmmBackward0>)
```
๋ค์์ ๋ ๋ฒ์งธ ์ํ์ค์ ์ค์ ์ถ๋ ฅ์
๋๋ค:
```py
>>> input_ids = torch.tensor([[7592]])
>>> output = model(input_ids)
>>> print(output.logits)
tensor([[-0.1008, -0.4061]], grad_fn=<AddmmBackward0>)
```
๋๋ถ๋ถ์ ๊ฒฝ์ฐ ๋ชจ๋ธ์ `attention_mask`๋ฅผ ์ ๊ณตํ์ฌ ํจ๋ฉ ํ ํฐ์ ๋ฌด์ํด์ผ ์ด๋ฌํ ์กฐ์ฉํ ์ค๋ฅ๋ฅผ ๋ฐฉ์งํ ์ ์์ต๋๋ค. ์ด์ ๋ ๋ฒ์งธ ์ํ์ค์ ์ถ๋ ฅ์ด ์ค์ ์ถ๋ ฅ๊ณผ ์ผ์นํฉ๋๋ค:
<Tip>
์ผ๋ฐ์ ์ผ๋ก ํ ํฌ๋์ด์ ๋ ํน์ ํ ํฌ๋์ด์ ์ ๊ธฐ๋ณธ ๊ฐ์ ๊ธฐ์ค์ผ๋ก ์ฌ์ฉ์์ ๋ํ 'attention_mask'๋ฅผ ๋ง๋ญ๋๋ค.
</Tip>
```py
>>> attention_mask = torch.tensor([[1, 1, 1, 1, 1, 1], [1, 0, 0, 0, 0, 0]])
>>> output = model(input_ids, attention_mask=attention_mask)
>>> print(output.logits)
tensor([[ 0.0082, -0.2307],
[-0.1008, -0.4061]], grad_fn=<AddmmBackward0>)
```
๐ค Transformers๋ ํจ๋ฉ ํ ํฐ์ด ์ ๊ณต๋ ๊ฒฝ์ฐ ํจ๋ฉ ํ ํฐ์ ๋ง์คํนํ๊ธฐ ์ํ `attention_mask`๋ฅผ ์๋์ผ๋ก ์์ฑํ์ง ์์ต๋๋ค. ๊ทธ ์ด์ ๋ ๋ค์๊ณผ ๊ฐ์ต๋๋ค:
- ์ผ๋ถ ๋ชจ๋ธ์๋ ํจ๋ฉ ํ ํฐ์ด ์์ต๋๋ค.
- ์ผ๋ถ ์ฌ์ฉ ์ฌ๋ก์ ๊ฒฝ์ฐ ์ฌ์ฉ์๊ฐ ๋ชจ๋ธ์ด ํจ๋ฉ ํ ํฐ์ ๊ด๋ฆฌํ๊ธฐ๋ฅผ ์ํฉ๋๋ค.
## ValueError: ์ด ์ ํ์ AutoModel์ ๋ํด ์ธ์ํ ์ ์๋ XYZ ๊ตฌ์ฑ ํด๋์ค(ValueError: Unrecognized configuration class XYZ for this kind of AutoModel)[[valueerror-unrecognized-configuration-class-xyz-for-this-kind-of-automodel]]
์ผ๋ฐ์ ์ผ๋ก, ์ฌ์ ํ์ต๋ ๋ชจ๋ธ์ ์ธ์คํด์ค๋ฅผ ๊ฐ์ ธ์ค๊ธฐ ์ํด [`AutoModel`] ํด๋์ค๋ฅผ ์ฌ์ฉํ๋ ๊ฒ์ด ์ข์ต๋๋ค.
์ด ํด๋์ค๋ ๊ตฌ์ฑ์ ๋ฐ๋ผ ์ฃผ์ด์ง ์ฒดํฌํฌ์ธํธ์์ ์ฌ๋ฐ๋ฅธ ์ํคํ
์ฒ๋ฅผ ์๋์ผ๋ก ์ถ๋ก ํ๊ณ ๊ฐ์ ธ์ฌ ์ ์์ต๋๋ค.
๋ชจ๋ธ์ ์ฒดํฌํฌ์ธํธ์์ ๊ฐ์ ธ์ฌ ๋ ์ด `ValueError`๊ฐ ๋ฐ์ํ๋ฉด, ์ด๋ Auto ํด๋์ค๊ฐ ์ฃผ์ด์ง ์ฒดํฌํฌ์ธํธ์ ๊ตฌ์ฑ์์
๊ฐ์ ธ์ค๋ ค๋ ๋ชจ๋ธ ์ ํ๊ณผ ๋งคํ์ ์ฐพ์ ์ ์๋ค๋ ๊ฒ์ ์๋ฏธํฉ๋๋ค. ๊ฐ์ฅ ํํ๊ฒ ๋ฐ์ํ๋ ๊ฒฝ์ฐ๋
์ฒดํฌํฌ์ธํธ๊ฐ ์ฃผ์ด์ง ํ์คํฌ๋ฅผ ์ง์ํ์ง ์์ ๋์
๋๋ค.
์๋ฅผ ๋ค์ด, ๋ค์ ์์ ์์ ์ง์์๋ต์ ๋ํ GPT2๊ฐ ์๊ธฐ ๋๋ฌธ์ ์ค๋ฅ๊ฐ ๋ฐ์ํฉ๋๋ค:
```py
>>> from transformers import AutoProcessor, AutoModelForQuestionAnswering
>>> processor = AutoProcessor.from_pretrained("gpt2-medium")
>>> model = AutoModelForQuestionAnswering.from_pretrained("gpt2-medium")
ValueError: Unrecognized configuration class <class 'transformers.models.gpt2.configuration_gpt2.GPT2Config'> for this kind of AutoModel: AutoModelForQuestionAnswering.
Model type should be one of AlbertConfig, BartConfig, BertConfig, BigBirdConfig, BigBirdPegasusConfig, BloomConfig, ...
```
| 0 |
hf_public_repos/transformers/docs/source | hf_public_repos/transformers/docs/source/ko/index.md | <!--Copyright 2020 The HuggingFace Team. All rights reserved.
Licensed under the Apache License, Version 2.0 (the "License"); you may not use this file except in compliance with
the License. You may obtain a copy of the License at
http://www.apache.org/licenses/LICENSE-2.0
Unless required by applicable law or agreed to in writing, software distributed under the License is distributed on
an "AS IS" BASIS, WITHOUT WARRANTIES OR CONDITIONS OF ANY KIND, either express or implied. See the License for the
specific language governing permissions and limitations under the License.
โ ๏ธ Note that this file is in Markdown but contain specific syntax for our doc-builder (similar to MDX) that may not be
rendered properly in your Markdown viewer.
-->
# ๐ค Transformers
[PyTorch](https://pytorch.org/), [TensorFlow](https://www.tensorflow.org/), [JAX](https://jax.readthedocs.io/en/latest/)๋ฅผ ์ํ ์ต์ฒจ๋จ ๋จธ์ ๋ฌ๋
๐ค Transformers๋ ์ฌ์ ํ์ต๋ ์ต์ฒจ๋จ ๋ชจ๋ธ๋ค์ ์ฝ๊ฒ ๋ค์ด๋ก๋ํ๊ณ ํ๋ จ์ํฌ ์ ์๋ API์ ๋๊ตฌ๋ฅผ ์ ๊ณตํฉ๋๋ค. ์ฌ์ ํ์ต๋ ๋ชจ๋ธ์ ์ฐ๋ฉด ์ปดํจํ
๋น์ฉ๊ณผ ํ์ ๋ฐฐ์ถ๋์ด ์ค๊ณ , ๋ชจ๋ธ์ ์ฒ์๋ถํฐ ํ๋ จ์ํค๋ ๋ฐ ํ์ํ ์๊ฐ๊ณผ ๋ฆฌ์์ค๋ฅผ ์ ์ฝํ ์ ์์ต๋๋ค. ์ ํฌ ๋ชจ๋ธ๋ค์ ๋ค์ํ ๋ถ์ผ์ ํ์คํฌ๋ฅผ ์ง์ํฉ๋๋ค.
๐ **์์ฐ์ด ์ฒ๋ฆฌ**: ํ
์คํธ ๋ถ๋ฅ, ๊ฐ์ฒด๋ช
์ธ์, ์ง์์๋ต, ์ธ์ด ๋ชจ๋ธ๋ง, ์์ฝ, ๋ฒ์ญ, ๊ฐ๊ด์ ์ง์์๋ต, ํ
์คํธ ์์ฑ<br>
๐ผ๏ธ **์ปดํจํฐ ๋น์ **: ์ด๋ฏธ์ง ๋ถ๋ฅ, ๊ฐ์ฒด ํ์ง, ๊ฐ์ฒด ๋ถํ <br>
๐ฃ๏ธ **์ค๋์ค**: ์๋์์ฑ์ธ์, ์ค๋์ค ๋ถ๋ฅ<br>
๐ **๋ฉํฐ๋ชจ๋ฌ**: ํ ์ง์์๋ต, ๊ดํ ๋ฌธ์ ์ธ์ (OCR), ์ค์บํ ๋ฌธ์์์ ์ ๋ณด ์ถ์ถ, ๋น๋์ค ๋ถ๋ฅ, ์๊ฐ ์ง์์๋ต
๐ค Transformers๋ PyTorch, TensorFlow์ JAX ๊ฐ์ ์ํธ์ด์ฉ์ฑ์ ์ง์ํฉ๋๋ค. ์ ์ฐํ๊ฒ ๋ชจ๋ธ์ ๊ฐ ๋จ๊ณ๋ง๋ค ๋ค๋ฅธ ํ๋ ์์ํฌ๋ฅผ ์ฌ์ฉํ ์๋ ์์ต๋๋ค. ์๋ฅผ ๋ค์ด ์ฝ๋ 3์ค๋ง ์จ์ ๋ชจ๋ธ์ ํ๋ จ์ํจ ๋ค์, ๋ค๋ฅธ ํ๋ ์์ํฌ ์์์ ์ถ๋ก ํ ์ ์์ต๋๋ค. ๋ชจ๋ธ์ ์ด์ ํ๊ฒฝ์ ๋ฐฐํฌํ๊ธฐ ์ํด ONNX๋ TorchScript ํ์์ผ๋ก ๋ด๋ณด๋ผ ์๋ ์์ต๋๋ค.
์ปค๋ฎค๋ํฐ์ ์ฐธ์ฌํ์๋ ค๋ฉด [Hub](https://huggingface.co/models), [ํฌ๋ผ](https://discuss.huggingface.co/), [๋์ค์ฝ๋](https://discord.com/invite/JfAtkvEtRb)๋ฅผ ๋ฐฉ๋ฌธํด์ฃผ์ธ์!
## Hugging Face ํ๊ณผ ์ง์ ๋ํํ๊ณ ์ถ์ผ์ ๊ฐ์?[[hugging-face-team]]
<a target="_blank" href="https://huggingface.co/support">
<img alt="HuggingFace Expert Acceleration Program" src="https://cdn-media.huggingface.co/marketing/transformers/new-support-improved.png" style="width: 100%; max-width: 600px; border: 1px solid #eee; border-radius: 4px; box-shadow: 0 1px 2px 0 rgba(0, 0, 0, 0.05);">
</a>
## ์ฝํ
์ธ [[contents]]
์ ํฌ ๊ธฐ์ ๋ฌธ์๋ ํฌ๊ฒ 5๊ฐ ์น์
์ผ๋ก ๋๋ ์ ์์ต๋๋ค:
- **์์ํ๊ธฐ**์์ ๋ผ์ด๋ธ๋ฌ๋ฆฌ๋ฅผ ๊ฐ๋จํ ํ์ด๋ณด๊ณ , ๋ณธ๊ฒฉ์ ์ผ๋ก ๋ฐ์ด๋ค ์ ์๊ฒ ์ค์น ๋ฐฉ๋ฒ์ ์๋ดํฉ๋๋ค.
- **ํํ ๋ฆฌ์ผ**์์ ๋ผ์ด๋ธ๋ฌ๋ฆฌ์ ์ต์ํด์ง ์ ์๋๋ก ์์ธํ๊ณ ๋ ์ฝ๊ฒ ๊ธฐ๋ณธ์ ์ธ ๋ถ๋ถ์ ์๋ดํฉ๋๋ค.
- **How-to ๊ฐ์ด๋**์์ ์ธ์ด ๋ชจ๋ธ๋ง์ ์ํด ์ฌ์ ํ์ต๋ ๋ชจ๋ธ์ ํ์ธ ํ๋ํ๋ ๋ฐฉ๋ฒ์ด๋, ์ง์ ๋ชจ๋ธ์ ์์ฑํ๊ณ ๊ณต์ ํ๋ ๋ฐฉ๋ฒ๊ณผ ๊ฐ์ด ํน์ ๋ชฉํ๋ฅผ ๋ฌ์ฑํ๋ ๋ฐฉ๋ฒ์ ์๋ดํฉ๋๋ค.
- **๊ฐ๋
๊ฐ์ด๋**์์ ๐ค Transformers์ ์ค๊ณ ์ฒ ํ๊ณผ ํจ๊ป ๋ชจ๋ธ์ด๋ ํ์คํฌ ๋ค์ ์จ๊ฒจ์ง ๊ฐ๋
๋ค๊ณผ ์์ด๋์ด๋ฅผ ํ๊ตฌํ๊ณ ์ค๋ช
์ ๋ง๋ถ์
๋๋ค.
- **API**์์ ๋ชจ๋ ํด๋์ค์ ํจ์๋ฅผ ์ค๋ช
ํฉ๋๋ค.
- **๋ฉ์ธ ํด๋์ค**์์ configuration, model, tokenizer, pipeline๊ณผ ๊ฐ์ด ์ ์ผ ์ค์ํ ํด๋์ค๋ค์ ์์ธํ ์ค๋ช
ํฉ๋๋ค.
- **๋ชจ๋ธ**์์ ๋ผ์ด๋ธ๋ฌ๋ฆฌ ์ ๊ตฌํ๋ ๊ฐ ๋ชจ๋ธ๊ณผ ์ฐ๊ด๋ ํด๋์ค์ ํจ์๋ฅผ ์์ธํ ์ค๋ช
ํฉ๋๋ค.
- **๋ด๋ถ ์ ํธ๋ฆฌํฐ**์์ ๋ด๋ถ์ ์ผ๋ก ์ฌ์ฉ๋๋ ์ ํธ๋ฆฌํฐ ํด๋์ค์ ํจ์๋ฅผ ์์ธํ ์ค๋ช
ํฉ๋๋ค.
### ์ง์ ๋ชจ๋ธ[[supported-models]]
<!--This list is updated automatically from the README with _make fix-copies_. Do not update manually! -->
1. **[ALBERT](model_doc/albert)** (from Google Research and the Toyota Technological Institute at Chicago) released with the paper [ALBERT: A Lite BERT for Self-supervised Learning of Language Representations](https://arxiv.org/abs/1909.11942), by Zhenzhong Lan, Mingda Chen, Sebastian Goodman, Kevin Gimpel, Piyush Sharma, Radu Soricut.
1. **[BART](model_doc/bart)** (from Facebook) released with the paper [BART: Denoising Sequence-to-Sequence Pre-training for Natural Language Generation, Translation, and Comprehension](https://arxiv.org/abs/1910.13461) by Mike Lewis, Yinhan Liu, Naman Goyal, Marjan Ghazvininejad, Abdelrahman Mohamed, Omer Levy, Ves Stoyanov and Luke Zettlemoyer.
1. **[BARThez](model_doc/barthez)** (from รcole polytechnique) released with the paper [BARThez: a Skilled Pretrained French Sequence-to-Sequence Model](https://arxiv.org/abs/2010.12321) by Moussa Kamal Eddine, Antoine J.-P. Tixier, Michalis Vazirgiannis.
1. **[BARTpho](model_doc/bartpho)** (from VinAI Research) released with the paper [BARTpho: Pre-trained Sequence-to-Sequence Models for Vietnamese](https://arxiv.org/abs/2109.09701) by Nguyen Luong Tran, Duong Minh Le and Dat Quoc Nguyen.
1. **[BEiT](model_doc/beit)** (from Microsoft) released with the paper [BEiT: BERT Pre-Training of Image Transformers](https://arxiv.org/abs/2106.08254) by Hangbo Bao, Li Dong, Furu Wei.
1. **[BERT](model_doc/bert)** (from Google) released with the paper [BERT: Pre-training of Deep Bidirectional Transformers for Language Understanding](https://arxiv.org/abs/1810.04805) by Jacob Devlin, Ming-Wei Chang, Kenton Lee and Kristina Toutanova.
1. **[BERT For Sequence Generation](model_doc/bert-generation)** (from Google) released with the paper [Leveraging Pre-trained Checkpoints for Sequence Generation Tasks](https://arxiv.org/abs/1907.12461) by Sascha Rothe, Shashi Narayan, Aliaksei Severyn.
1. **[BERTweet](model_doc/bertweet)** (from VinAI Research) released with the paper [BERTweet: A pre-trained language model for English Tweets](https://aclanthology.org/2020.emnlp-demos.2/) by Dat Quoc Nguyen, Thanh Vu and Anh Tuan Nguyen.
1. **[BigBird-Pegasus](model_doc/bigbird_pegasus)** (from Google Research) released with the paper [Big Bird: Transformers for Longer Sequences](https://arxiv.org/abs/2007.14062) by Manzil Zaheer, Guru Guruganesh, Avinava Dubey, Joshua Ainslie, Chris Alberti, Santiago Ontanon, Philip Pham, Anirudh Ravula, Qifan Wang, Li Yang, Amr Ahmed.
1. **[BigBird-RoBERTa](model_doc/big_bird)** (from Google Research) released with the paper [Big Bird: Transformers for Longer Sequences](https://arxiv.org/abs/2007.14062) by Manzil Zaheer, Guru Guruganesh, Avinava Dubey, Joshua Ainslie, Chris Alberti, Santiago Ontanon, Philip Pham, Anirudh Ravula, Qifan Wang, Li Yang, Amr Ahmed.
1. **[Blenderbot](model_doc/blenderbot)** (from Facebook) released with the paper [Recipes for building an open-domain chatbot](https://arxiv.org/abs/2004.13637) by Stephen Roller, Emily Dinan, Naman Goyal, Da Ju, Mary Williamson, Yinhan Liu, Jing Xu, Myle Ott, Kurt Shuster, Eric M. Smith, Y-Lan Boureau, Jason Weston.
1. **[BlenderbotSmall](model_doc/blenderbot-small)** (from Facebook) released with the paper [Recipes for building an open-domain chatbot](https://arxiv.org/abs/2004.13637) by Stephen Roller, Emily Dinan, Naman Goyal, Da Ju, Mary Williamson, Yinhan Liu, Jing Xu, Myle Ott, Kurt Shuster, Eric M. Smith, Y-Lan Boureau, Jason Weston.
1. **[BLOOM](model_doc/bloom)** (from BigScience workshop) released by the [BigScience Workshop](https://bigscience.huggingface.co/).
1. **[BORT](model_doc/bort)** (from Alexa) released with the paper [Optimal Subarchitecture Extraction For BERT](https://arxiv.org/abs/2010.10499) by Adrian de Wynter and Daniel J. Perry.
1. **[ByT5](model_doc/byt5)** (from Google Research) released with the paper [ByT5: Towards a token-free future with pre-trained byte-to-byte models](https://arxiv.org/abs/2105.13626) by Linting Xue, Aditya Barua, Noah Constant, Rami Al-Rfou, Sharan Narang, Mihir Kale, Adam Roberts, Colin Raffel.
1. **[CamemBERT](model_doc/camembert)** (from Inria/Facebook/Sorbonne) released with the paper [CamemBERT: a Tasty French Language Model](https://arxiv.org/abs/1911.03894) by Louis Martin*, Benjamin Muller*, Pedro Javier Ortiz Suรกrez*, Yoann Dupont, Laurent Romary, รric Villemonte de la Clergerie, Djamรฉ Seddah and Benoรฎt Sagot.
1. **[CANINE](model_doc/canine)** (from Google Research) released with the paper [CANINE: Pre-training an Efficient Tokenization-Free Encoder for Language Representation](https://arxiv.org/abs/2103.06874) by Jonathan H. Clark, Dan Garrette, Iulia Turc, John Wieting.
1. **[CLIP](model_doc/clip)** (from OpenAI) released with the paper [Learning Transferable Visual Models From Natural Language Supervision](https://arxiv.org/abs/2103.00020) by Alec Radford, Jong Wook Kim, Chris Hallacy, Aditya Ramesh, Gabriel Goh, Sandhini Agarwal, Girish Sastry, Amanda Askell, Pamela Mishkin, Jack Clark, Gretchen Krueger, Ilya Sutskever.
1. **[CLIPSeg](model_doc/clipseg)** (from University of Gรถttingen) released with the paper [Image Segmentation Using Text and Image Prompts](https://arxiv.org/abs/2112.10003) by Timo Lรผddecke and Alexander Ecker.
1. **[CodeGen](model_doc/codegen)** (from Salesforce) released with the paper [A Conversational Paradigm for Program Synthesis](https://arxiv.org/abs/2203.13474) by Erik Nijkamp, Bo Pang, Hiroaki Hayashi, Lifu Tu, Huan Wang, Yingbo Zhou, Silvio Savarese, Caiming Xiong.
1. **[Conditional DETR](model_doc/conditional_detr)** (from Microsoft Research Asia) released with the paper [Conditional DETR for Fast Training Convergence](https://arxiv.org/abs/2108.06152) by Depu Meng, Xiaokang Chen, Zejia Fan, Gang Zeng, Houqiang Li, Yuhui Yuan, Lei Sun, Jingdong Wang.
1. **[ConvBERT](model_doc/convbert)** (from YituTech) released with the paper [ConvBERT: Improving BERT with Span-based Dynamic Convolution](https://arxiv.org/abs/2008.02496) by Zihang Jiang, Weihao Yu, Daquan Zhou, Yunpeng Chen, Jiashi Feng, Shuicheng Yan.
1. **[ConvNeXT](model_doc/convnext)** (from Facebook AI) released with the paper [A ConvNet for the 2020s](https://arxiv.org/abs/2201.03545) by Zhuang Liu, Hanzi Mao, Chao-Yuan Wu, Christoph Feichtenhofer, Trevor Darrell, Saining Xie.
1. **[ConvNeXTV2](model_doc/convnextv2)** (from Facebook AI) released with the paper [ConvNeXt V2: Co-designing and Scaling ConvNets with Masked Autoencoders](https://arxiv.org/abs/2301.00808) by Sanghyun Woo, Shoubhik Debnath, Ronghang Hu, Xinlei Chen, Zhuang Liu, In So Kweon, Saining Xie.
1. **[CPM](model_doc/cpm)** (from Tsinghua University) released with the paper [CPM: A Large-scale Generative Chinese Pre-trained Language Model](https://arxiv.org/abs/2012.00413) by Zhengyan Zhang, Xu Han, Hao Zhou, Pei Ke, Yuxian Gu, Deming Ye, Yujia Qin, Yusheng Su, Haozhe Ji, Jian Guan, Fanchao Qi, Xiaozhi Wang, Yanan Zheng, Guoyang Zeng, Huanqi Cao, Shengqi Chen, Daixuan Li, Zhenbo Sun, Zhiyuan Liu, Minlie Huang, Wentao Han, Jie Tang, Juanzi Li, Xiaoyan Zhu, Maosong Sun.
1. **[CTRL](model_doc/ctrl)** (from Salesforce) released with the paper [CTRL: A Conditional Transformer Language Model for Controllable Generation](https://arxiv.org/abs/1909.05858) by Nitish Shirish Keskar*, Bryan McCann*, Lav R. Varshney, Caiming Xiong and Richard Socher.
1. **[CvT](model_doc/cvt)** (from Microsoft) released with the paper [CvT: Introducing Convolutions to Vision Transformers](https://arxiv.org/abs/2103.15808) by Haiping Wu, Bin Xiao, Noel Codella, Mengchen Liu, Xiyang Dai, Lu Yuan, Lei Zhang.
1. **[Data2Vec](model_doc/data2vec)** (from Facebook) released with the paper [Data2Vec: A General Framework for Self-supervised Learning in Speech, Vision and Language](https://arxiv.org/abs/2202.03555) by Alexei Baevski, Wei-Ning Hsu, Qiantong Xu, Arun Babu, Jiatao Gu, Michael Auli.
1. **[DeBERTa](model_doc/deberta)** (from Microsoft) released with the paper [DeBERTa: Decoding-enhanced BERT with Disentangled Attention](https://arxiv.org/abs/2006.03654) by Pengcheng He, Xiaodong Liu, Jianfeng Gao, Weizhu Chen.
1. **[DeBERTa-v2](model_doc/deberta-v2)** (from Microsoft) released with the paper [DeBERTa: Decoding-enhanced BERT with Disentangled Attention](https://arxiv.org/abs/2006.03654) by Pengcheng He, Xiaodong Liu, Jianfeng Gao, Weizhu Chen.
1. **[Decision Transformer](model_doc/decision_transformer)** (from Berkeley/Facebook/Google) released with the paper [Decision Transformer: Reinforcement Learning via Sequence Modeling](https://arxiv.org/abs/2106.01345) by Lili Chen, Kevin Lu, Aravind Rajeswaran, Kimin Lee, Aditya Grover, Michael Laskin, Pieter Abbeel, Aravind Srinivas, Igor Mordatch.
1. **[Deformable DETR](model_doc/deformable_detr)** (from SenseTime Research) released with the paper [Deformable DETR: Deformable Transformers for End-to-End Object Detection](https://arxiv.org/abs/2010.04159) by Xizhou Zhu, Weijie Su, Lewei Lu, Bin Li, Xiaogang Wang, Jifeng Dai.
1. **[DeiT](model_doc/deit)** (from Facebook) released with the paper [Training data-efficient image transformers & distillation through attention](https://arxiv.org/abs/2012.12877) by Hugo Touvron, Matthieu Cord, Matthijs Douze, Francisco Massa, Alexandre Sablayrolles, Hervรฉ Jรฉgou.
1. **[DETR](model_doc/detr)** (from Facebook) released with the paper [End-to-End Object Detection with Transformers](https://arxiv.org/abs/2005.12872) by Nicolas Carion, Francisco Massa, Gabriel Synnaeve, Nicolas Usunier, Alexander Kirillov, Sergey Zagoruyko.
1. **[DialoGPT](model_doc/dialogpt)** (from Microsoft Research) released with the paper [DialoGPT: Large-Scale Generative Pre-training for Conversational Response Generation](https://arxiv.org/abs/1911.00536) by Yizhe Zhang, Siqi Sun, Michel Galley, Yen-Chun Chen, Chris Brockett, Xiang Gao, Jianfeng Gao, Jingjing Liu, Bill Dolan.
1. **[DistilBERT](model_doc/distilbert)** (from HuggingFace), released together with the paper [DistilBERT, a distilled version of BERT: smaller, faster, cheaper and lighter](https://arxiv.org/abs/1910.01108) by Victor Sanh, Lysandre Debut and Thomas Wolf. The same method has been applied to compress GPT2 into [DistilGPT2](https://github.com/huggingface/transformers/tree/main/examples/research_projects/distillation), RoBERTa into [DistilRoBERTa](https://github.com/huggingface/transformers/tree/main/examples/research_projects/distillation), Multilingual BERT into [DistilmBERT](https://github.com/huggingface/transformers/tree/main/examples/research_projects/distillation) and a German version of DistilBERT.
1. **[DiT](model_doc/dit)** (from Microsoft Research) released with the paper [DiT: Self-supervised Pre-training for Document Image Transformer](https://arxiv.org/abs/2203.02378) by Junlong Li, Yiheng Xu, Tengchao Lv, Lei Cui, Cha Zhang, Furu Wei.
1. **[Donut](model_doc/donut)** (from NAVER), released together with the paper [OCR-free Document Understanding Transformer](https://arxiv.org/abs/2111.15664) by Geewook Kim, Teakgyu Hong, Moonbin Yim, Jeongyeon Nam, Jinyoung Park, Jinyeong Yim, Wonseok Hwang, Sangdoo Yun, Dongyoon Han, Seunghyun Park.
1. **[DPR](model_doc/dpr)** (from Facebook) released with the paper [Dense Passage Retrieval for Open-Domain Question Answering](https://arxiv.org/abs/2004.04906) by Vladimir Karpukhin, Barlas Oฤuz, Sewon Min, Patrick Lewis, Ledell Wu, Sergey Edunov, Danqi Chen, and Wen-tau Yih.
1. **[DPT](master/model_doc/dpt)** (from Intel Labs) released with the paper [Vision Transformers for Dense Prediction](https://arxiv.org/abs/2103.13413) by Renรฉ Ranftl, Alexey Bochkovskiy, Vladlen Koltun.
1. **[EfficientNet](model_doc/efficientnet)** (from Google Research) released with the paper [EfficientNet: Rethinking Model Scaling for Convolutional Neural Networks](https://arxiv.org/abs/1905.11946) by Mingxing Tan and Quoc V. Le.
1. **[ELECTRA](model_doc/electra)** (from Google Research/Stanford University) released with the paper [ELECTRA: Pre-training text encoders as discriminators rather than generators](https://arxiv.org/abs/2003.10555) by Kevin Clark, Minh-Thang Luong, Quoc V. Le, Christopher D. Manning.
1. **[EncoderDecoder](model_doc/encoder-decoder)** (from Google Research) released with the paper [Leveraging Pre-trained Checkpoints for Sequence Generation Tasks](https://arxiv.org/abs/1907.12461) by Sascha Rothe, Shashi Narayan, Aliaksei Severyn.
1. **[ERNIE](model_doc/ernie)** (from Baidu) released with the paper [ERNIE: Enhanced Representation through Knowledge Integration](https://arxiv.org/abs/1904.09223) by Yu Sun, Shuohuan Wang, Yukun Li, Shikun Feng, Xuyi Chen, Han Zhang, Xin Tian, Danxiang Zhu, Hao Tian, Hua Wu.
1. **[ESM](model_doc/esm)** (from Meta AI) are transformer protein language models. **ESM-1b** was released with the paper [Biological structure and function emerge from scaling unsupervised learning to 250 million protein sequences](https://www.pnas.org/content/118/15/e2016239118) by Alexander Rives, Joshua Meier, Tom Sercu, Siddharth Goyal, Zeming Lin, Jason Liu, Demi Guo, Myle Ott, C. Lawrence Zitnick, Jerry Ma, and Rob Fergus. **ESM-1v** was released with the paper [Language models enable zero-shot prediction of the effects of mutations on protein function](https://doi.org/10.1101/2021.07.09.450648) by Joshua Meier, Roshan Rao, Robert Verkuil, Jason Liu, Tom Sercu and Alexander Rives. **ESM-2 and ESMFold** were released with the paper [Language models of protein sequences at the scale of evolution enable accurate structure prediction](https://doi.org/10.1101/2022.07.20.500902) by Zeming Lin, Halil Akin, Roshan Rao, Brian Hie, Zhongkai Zhu, Wenting Lu, Allan dos Santos Costa, Maryam Fazel-Zarandi, Tom Sercu, Sal Candido, Alexander Rives.
1. **[FLAN-T5](model_doc/flan-t5)** (from Google AI) released in the repository [google-research/t5x](https://github.com/google-research/t5x/blob/main/docs/models.md#flan-t5-checkpoints) by Hyung Won Chung, Le Hou, Shayne Longpre, Barret Zoph, Yi Tay, William Fedus, Eric Li, Xuezhi Wang, Mostafa Dehghani, Siddhartha Brahma, Albert Webson, Shixiang Shane Gu, Zhuyun Dai, Mirac Suzgun, Xinyun Chen, Aakanksha Chowdhery, Sharan Narang, Gaurav Mishra, Adams Yu, Vincent Zhao, Yanping Huang, Andrew Dai, Hongkun Yu, Slav Petrov, Ed H. Chi, Jeff Dean, Jacob Devlin, Adam Roberts, Denny Zhou, Quoc V. Le, and Jason Wei
1. **[FlauBERT](model_doc/flaubert)** (from CNRS) released with the paper [FlauBERT: Unsupervised Language Model Pre-training for French](https://arxiv.org/abs/1912.05372) by Hang Le, Loรฏc Vial, Jibril Frej, Vincent Segonne, Maximin Coavoux, Benjamin Lecouteux, Alexandre Allauzen, Benoรฎt Crabbรฉ, Laurent Besacier, Didier Schwab.
1. **[FLAVA](model_doc/flava)** (from Facebook AI) released with the paper [FLAVA: A Foundational Language And Vision Alignment Model](https://arxiv.org/abs/2112.04482) by Amanpreet Singh, Ronghang Hu, Vedanuj Goswami, Guillaume Couairon, Wojciech Galuba, Marcus Rohrbach, and Douwe Kiela.
1. **[FNet](model_doc/fnet)** (from Google Research) released with the paper [FNet: Mixing Tokens with Fourier Transforms](https://arxiv.org/abs/2105.03824) by James Lee-Thorp, Joshua Ainslie, Ilya Eckstein, Santiago Ontanon.
1. **[Funnel Transformer](model_doc/funnel)** (from CMU/Google Brain) released with the paper [Funnel-Transformer: Filtering out Sequential Redundancy for Efficient Language Processing](https://arxiv.org/abs/2006.03236) by Zihang Dai, Guokun Lai, Yiming Yang, Quoc V. Le.
1. **[GLPN](model_doc/glpn)** (from KAIST) released with the paper [Global-Local Path Networks for Monocular Depth Estimation with Vertical CutDepth](https://arxiv.org/abs/2201.07436) by Doyeon Kim, Woonghyun Ga, Pyungwhan Ahn, Donggyu Joo, Sehwan Chun, Junmo Kim.
1. **[GPT](model_doc/openai-gpt)** (from OpenAI) released with the paper [Improving Language Understanding by Generative Pre-Training](https://blog.openai.com/language-unsupervised/) by Alec Radford, Karthik Narasimhan, Tim Salimans and Ilya Sutskever.
1. **[GPT Neo](model_doc/gpt_neo)** (from EleutherAI) released in the repository [EleutherAI/gpt-neo](https://github.com/EleutherAI/gpt-neo) by Sid Black, Stella Biderman, Leo Gao, Phil Wang and Connor Leahy.
1. **[GPT NeoX](model_doc/gpt_neox)** (from EleutherAI) released with the paper [GPT-NeoX-20B: An Open-Source Autoregressive Language Model](https://arxiv.org/abs/2204.06745) by Sid Black, Stella Biderman, Eric Hallahan, Quentin Anthony, Leo Gao, Laurence Golding, Horace He, Connor Leahy, Kyle McDonell, Jason Phang, Michael Pieler, USVSN Sai Prashanth, Shivanshu Purohit, Laria Reynolds, Jonathan Tow, Ben Wang, Samuel Weinbach
1. **[GPT NeoX Japanese](model_doc/gpt_neox_japanese)** (from ABEJA) released by Shinya Otani, Takayoshi Makabe, Anuj Arora, and Kyo Hattori.
1. **[GPT-2](model_doc/gpt2)** (from OpenAI) released with the paper [Language Models are Unsupervised Multitask Learners](https://blog.openai.com/better-language-models/) by Alec Radford*, Jeffrey Wu*, Rewon Child, David Luan, Dario Amodei** and Ilya Sutskever**.
1. **[GPT-J](model_doc/gptj)** (from EleutherAI) released in the repository [kingoflolz/mesh-transformer-jax](https://github.com/kingoflolz/mesh-transformer-jax/) by Ben Wang and Aran Komatsuzaki.
1. **[GPTSAN-japanese](model_doc/gptsan-japanese)** released in the repository [tanreinama/GPTSAN](https://github.com/tanreinama/GPTSAN/blob/main/report/model.md) by Toshiyuki Sakamoto(tanreinama).
1. **[GroupViT](model_doc/groupvit)** (from UCSD, NVIDIA) released with the paper [GroupViT: Semantic Segmentation Emerges from Text Supervision](https://arxiv.org/abs/2202.11094) by Jiarui Xu, Shalini De Mello, Sifei Liu, Wonmin Byeon, Thomas Breuel, Jan Kautz, Xiaolong Wang.
1. **[Hubert](model_doc/hubert)** (from Facebook) released with the paper [HuBERT: Self-Supervised Speech Representation Learning by Masked Prediction of Hidden Units](https://arxiv.org/abs/2106.07447) by Wei-Ning Hsu, Benjamin Bolte, Yao-Hung Hubert Tsai, Kushal Lakhotia, Ruslan Salakhutdinov, Abdelrahman Mohamed.
1. **[I-BERT](model_doc/ibert)** (from Berkeley) released with the paper [I-BERT: Integer-only BERT Quantization](https://arxiv.org/abs/2101.01321) by Sehoon Kim, Amir Gholami, Zhewei Yao, Michael W. Mahoney, Kurt Keutzer.
1. **[ImageGPT](model_doc/imagegpt)** (from OpenAI) released with the paper [Generative Pretraining from Pixels](https://openai.com/blog/image-gpt/) by Mark Chen, Alec Radford, Rewon Child, Jeffrey Wu, Heewoo Jun, David Luan, Ilya Sutskever.
1. **[Jukebox](model_doc/jukebox)** (from OpenAI) released with the paper [Jukebox: A Generative Model for Music](https://arxiv.org/pdf/2005.00341.pdf) by Prafulla Dhariwal, Heewoo Jun, Christine Payne, Jong Wook Kim, Alec Radford, Ilya Sutskever.
1. **[LayoutLM](model_doc/layoutlm)** (from Microsoft Research Asia) released with the paper [LayoutLM: Pre-training of Text and Layout for Document Image Understanding](https://arxiv.org/abs/1912.13318) by Yiheng Xu, Minghao Li, Lei Cui, Shaohan Huang, Furu Wei, Ming Zhou.
1. **[LayoutLMv2](model_doc/layoutlmv2)** (from Microsoft Research Asia) released with the paper [LayoutLMv2: Multi-modal Pre-training for Visually-Rich Document Understanding](https://arxiv.org/abs/2012.14740) by Yang Xu, Yiheng Xu, Tengchao Lv, Lei Cui, Furu Wei, Guoxin Wang, Yijuan Lu, Dinei Florencio, Cha Zhang, Wanxiang Che, Min Zhang, Lidong Zhou.
1. **[LayoutLMv3](model_doc/layoutlmv3)** (from Microsoft Research Asia) released with the paper [LayoutLMv3: Pre-training for Document AI with Unified Text and Image Masking](https://arxiv.org/abs/2204.08387) by Yupan Huang, Tengchao Lv, Lei Cui, Yutong Lu, Furu Wei.
1. **[LayoutXLM](model_doc/layoutxlm)** (from Microsoft Research Asia) released with the paper [LayoutXLM: Multimodal Pre-training for Multilingual Visually-rich Document Understanding](https://arxiv.org/abs/2104.08836) by Yiheng Xu, Tengchao Lv, Lei Cui, Guoxin Wang, Yijuan Lu, Dinei Florencio, Cha Zhang, Furu Wei.
1. **[LED](model_doc/led)** (from AllenAI) released with the paper [Longformer: The Long-Document Transformer](https://arxiv.org/abs/2004.05150) by Iz Beltagy, Matthew E. Peters, Arman Cohan.
1. **[LeViT](model_doc/levit)** (from Meta AI) released with the paper [LeViT: A Vision Transformer in ConvNet's Clothing for Faster Inference](https://arxiv.org/abs/2104.01136) by Ben Graham, Alaaeldin El-Nouby, Hugo Touvron, Pierre Stock, Armand Joulin, Hervรฉ Jรฉgou, Matthijs Douze.
1. **[LiLT](model_doc/lilt)** (from South China University of Technology) released with the paper [LiLT: A Simple yet Effective Language-Independent Layout Transformer for Structured Document Understanding](https://arxiv.org/abs/2202.13669) by Jiapeng Wang, Lianwen Jin, Kai Ding.
1. **[Longformer](model_doc/longformer)** (from AllenAI) released with the paper [Longformer: The Long-Document Transformer](https://arxiv.org/abs/2004.05150) by Iz Beltagy, Matthew E. Peters, Arman Cohan.
1. **[LongT5](model_doc/longt5)** (from Google AI) released with the paper [LongT5: Efficient Text-To-Text Transformer for Long Sequences](https://arxiv.org/abs/2112.07916) by Mandy Guo, Joshua Ainslie, David Uthus, Santiago Ontanon, Jianmo Ni, Yun-Hsuan Sung, Yinfei Yang.
1. **[LUKE](model_doc/luke)** (from Studio Ousia) released with the paper [LUKE: Deep Contextualized Entity Representations with Entity-aware Self-attention](https://arxiv.org/abs/2010.01057) by Ikuya Yamada, Akari Asai, Hiroyuki Shindo, Hideaki Takeda, Yuji Matsumoto.
1. **[LXMERT](model_doc/lxmert)** (from UNC Chapel Hill) released with the paper [LXMERT: Learning Cross-Modality Encoder Representations from Transformers for Open-Domain Question Answering](https://arxiv.org/abs/1908.07490) by Hao Tan and Mohit Bansal.
1. **[M-CTC-T](model_doc/mctct)** (from Facebook) released with the paper [Pseudo-Labeling For Massively Multilingual Speech Recognition](https://arxiv.org/abs/2111.00161) by Loren Lugosch, Tatiana Likhomanenko, Gabriel Synnaeve, and Ronan Collobert.
1. **[M2M100](model_doc/m2m_100)** (from Facebook) released with the paper [Beyond English-Centric Multilingual Machine Translation](https://arxiv.org/abs/2010.11125) by Angela Fan, Shruti Bhosale, Holger Schwenk, Zhiyi Ma, Ahmed El-Kishky, Siddharth Goyal, Mandeep Baines, Onur Celebi, Guillaume Wenzek, Vishrav Chaudhary, Naman Goyal, Tom Birch, Vitaliy Liptchinsky, Sergey Edunov, Edouard Grave, Michael Auli, Armand Joulin.
1. **[MarianMT](model_doc/marian)** Machine translation models trained using [OPUS](http://opus.nlpl.eu/) data by Jรถrg Tiedemann. The [Marian Framework](https://marian-nmt.github.io/) is being developed by the Microsoft Translator Team.
1. **[MarkupLM](model_doc/markuplm)** (from Microsoft Research Asia) released with the paper [MarkupLM: Pre-training of Text and Markup Language for Visually-rich Document Understanding](https://arxiv.org/abs/2110.08518) by Junlong Li, Yiheng Xu, Lei Cui, Furu Wei.
1. **[Mask2Former](model_doc/mask2former)** (from FAIR and UIUC) released with the paper [Masked-attention Mask Transformer for Universal Image Segmentation](https://arxiv.org/abs/2112.01527) by Bowen Cheng, Ishan Misra, Alexander G. Schwing, Alexander Kirillov, Rohit Girdhar.
1. **[MaskFormer](model_doc/maskformer)** (from Meta and UIUC) released with the paper [Per-Pixel Classification is Not All You Need for Semantic Segmentation](https://arxiv.org/abs/2107.06278) by Bowen Cheng, Alexander G. Schwing, Alexander Kirillov.
1. **[mBART](model_doc/mbart)** (from Facebook) released with the paper [Multilingual Denoising Pre-training for Neural Machine Translation](https://arxiv.org/abs/2001.08210) by Yinhan Liu, Jiatao Gu, Naman Goyal, Xian Li, Sergey Edunov, Marjan Ghazvininejad, Mike Lewis, Luke Zettlemoyer.
1. **[mBART-50](model_doc/mbart)** (from Facebook) released with the paper [Multilingual Translation with Extensible Multilingual Pretraining and Finetuning](https://arxiv.org/abs/2008.00401) by Yuqing Tang, Chau Tran, Xian Li, Peng-Jen Chen, Naman Goyal, Vishrav Chaudhary, Jiatao Gu, Angela Fan.
1. **[Megatron-BERT](model_doc/megatron-bert)** (from NVIDIA) released with the paper [Megatron-LM: Training Multi-Billion Parameter Language Models Using Model Parallelism](https://arxiv.org/abs/1909.08053) by Mohammad Shoeybi, Mostofa Patwary, Raul Puri, Patrick LeGresley, Jared Casper and Bryan Catanzaro.
1. **[Megatron-GPT2](model_doc/megatron_gpt2)** (from NVIDIA) released with the paper [Megatron-LM: Training Multi-Billion Parameter Language Models Using Model Parallelism](https://arxiv.org/abs/1909.08053) by Mohammad Shoeybi, Mostofa Patwary, Raul Puri, Patrick LeGresley, Jared Casper and Bryan Catanzaro.
1. **[mLUKE](model_doc/mluke)** (from Studio Ousia) released with the paper [mLUKE: The Power of Entity Representations in Multilingual Pretrained Language Models](https://arxiv.org/abs/2110.08151) by Ryokan Ri, Ikuya Yamada, and Yoshimasa Tsuruoka.
1. **[MobileBERT](model_doc/mobilebert)** (from CMU/Google Brain) released with the paper [MobileBERT: a Compact Task-Agnostic BERT for Resource-Limited Devices](https://arxiv.org/abs/2004.02984) by Zhiqing Sun, Hongkun Yu, Xiaodan Song, Renjie Liu, Yiming Yang, and Denny Zhou.
1. **[MobileViT](model_doc/mobilevit)** (from Apple) released with the paper [MobileViT: Light-weight, General-purpose, and Mobile-friendly Vision Transformer](https://arxiv.org/abs/2110.02178) by Sachin Mehta and Mohammad Rastegari.
1. **[MPNet](model_doc/mpnet)** (from Microsoft Research) released with the paper [MPNet: Masked and Permuted Pre-training for Language Understanding](https://arxiv.org/abs/2004.09297) by Kaitao Song, Xu Tan, Tao Qin, Jianfeng Lu, Tie-Yan Liu.
1. **[MT5](model_doc/mt5)** (from Google AI) released with the paper [mT5: A massively multilingual pre-trained text-to-text transformer](https://arxiv.org/abs/2010.11934) by Linting Xue, Noah Constant, Adam Roberts, Mihir Kale, Rami Al-Rfou, Aditya Siddhant, Aditya Barua, Colin Raffel.
1. **[MVP](model_doc/mvp)** (from RUC AI Box) released with the paper [MVP: Multi-task Supervised Pre-training for Natural Language Generation](https://arxiv.org/abs/2206.12131) by Tianyi Tang, Junyi Li, Wayne Xin Zhao and Ji-Rong Wen.
1. **[Nezha](model_doc/nezha)** (from Huawei Noahโs Ark Lab) released with the paper [NEZHA: Neural Contextualized Representation for Chinese Language Understanding](https://arxiv.org/abs/1909.00204) by Junqiu Wei, Xiaozhe Ren, Xiaoguang Li, Wenyong Huang, Yi Liao, Yasheng Wang, Jiashu Lin, Xin Jiang, Xiao Chen and Qun Liu.
1. **[NLLB](model_doc/nllb)** (from Meta) released with the paper [No Language Left Behind: Scaling Human-Centered Machine Translation](https://arxiv.org/abs/2207.04672) by the NLLB team.
1. **[Nystrรถmformer](model_doc/nystromformer)** (from the University of Wisconsin - Madison) released with the paper [Nystrรถmformer: A Nystrรถm-Based Algorithm for Approximating Self-Attention](https://arxiv.org/abs/2102.03902) by Yunyang Xiong, Zhanpeng Zeng, Rudrasis Chakraborty, Mingxing Tan, Glenn Fung, Yin Li, Vikas Singh.
1. **[OneFormer](model_doc/oneformer)** (from SHI Labs) released with the paper [OneFormer: One Transformer to Rule Universal Image Segmentation](https://arxiv.org/abs/2211.06220) by Jitesh Jain, Jiachen Li, MangTik Chiu, Ali Hassani, Nikita Orlov, Humphrey Shi.
1. **[OPT](master/model_doc/opt)** (from Meta AI) released with the paper [OPT: Open Pre-trained Transformer Language Models](https://arxiv.org/abs/2205.01068) by Susan Zhang, Stephen Roller, Naman Goyal, Mikel Artetxe, Moya Chen, Shuohui Chen et al.
1. **[OWL-ViT](model_doc/owlvit)** (from Google AI) released with the paper [Simple Open-Vocabulary Object Detection with Vision Transformers](https://arxiv.org/abs/2205.06230) by Matthias Minderer, Alexey Gritsenko, Austin Stone, Maxim Neumann, Dirk Weissenborn, Alexey Dosovitskiy, Aravindh Mahendran, Anurag Arnab, Mostafa Dehghani, Zhuoran Shen, Xiao Wang, Xiaohua Zhai, Thomas Kipf, and Neil Houlsby.
1. **[Pegasus](model_doc/pegasus)** (from Google) released with the paper [PEGASUS: Pre-training with Extracted Gap-sentences for Abstractive Summarization](https://arxiv.org/abs/1912.08777) by Jingqing Zhang, Yao Zhao, Mohammad Saleh and Peter J. Liu.
1. **[PEGASUS-X](model_doc/pegasus_x)** (from Google) released with the paper [Investigating Efficiently Extending Transformers for Long Input Summarization](https://arxiv.org/abs/2208.04347) by Jason Phang, Yao Zhao, and Peter J. Liu.
1. **[Perceiver IO](model_doc/perceiver)** (from Deepmind) released with the paper [Perceiver IO: A General Architecture for Structured Inputs & Outputs](https://arxiv.org/abs/2107.14795) by Andrew Jaegle, Sebastian Borgeaud, Jean-Baptiste Alayrac, Carl Doersch, Catalin Ionescu, David Ding, Skanda Koppula, Daniel Zoran, Andrew Brock, Evan Shelhamer, Olivier Hรฉnaff, Matthew M. Botvinick, Andrew Zisserman, Oriol Vinyals, Joรฃo Carreira.
1. **[PhoBERT](model_doc/phobert)** (from VinAI Research) released with the paper [PhoBERT: Pre-trained language models for Vietnamese](https://www.aclweb.org/anthology/2020.findings-emnlp.92/) by Dat Quoc Nguyen and Anh Tuan Nguyen.
1. **[PLBart](model_doc/plbart)** (from UCLA NLP) released with the paper [Unified Pre-training for Program Understanding and Generation](https://arxiv.org/abs/2103.06333) by Wasi Uddin Ahmad, Saikat Chakraborty, Baishakhi Ray, Kai-Wei Chang.
1. **[PoolFormer](model_doc/poolformer)** (from Sea AI Labs) released with the paper [MetaFormer is Actually What You Need for Vision](https://arxiv.org/abs/2111.11418) by Yu, Weihao and Luo, Mi and Zhou, Pan and Si, Chenyang and Zhou, Yichen and Wang, Xinchao and Feng, Jiashi and Yan, Shuicheng.
1. **[ProphetNet](model_doc/prophetnet)** (from Microsoft Research) released with the paper [ProphetNet: Predicting Future N-gram for Sequence-to-Sequence Pre-training](https://arxiv.org/abs/2001.04063) by Yu Yan, Weizhen Qi, Yeyun Gong, Dayiheng Liu, Nan Duan, Jiusheng Chen, Ruofei Zhang and Ming Zhou.
1. **[QDQBert](model_doc/qdqbert)** (from NVIDIA) released with the paper [Integer Quantization for Deep Learning Inference: Principles and Empirical Evaluation](https://arxiv.org/abs/2004.09602) by Hao Wu, Patrick Judd, Xiaojie Zhang, Mikhail Isaev and Paulius Micikevicius.
1. **[RAG](model_doc/rag)** (from Facebook) released with the paper [Retrieval-Augmented Generation for Knowledge-Intensive NLP Tasks](https://arxiv.org/abs/2005.11401) by Patrick Lewis, Ethan Perez, Aleksandara Piktus, Fabio Petroni, Vladimir Karpukhin, Naman Goyal, Heinrich Kรผttler, Mike Lewis, Wen-tau Yih, Tim Rocktรคschel, Sebastian Riedel, Douwe Kiela.
1. **[REALM](model_doc/realm.html)** (from Google Research) released with the paper [REALM: Retrieval-Augmented Language Model Pre-Training](https://arxiv.org/abs/2002.08909) by Kelvin Guu, Kenton Lee, Zora Tung, Panupong Pasupat and Ming-Wei Chang.
1. **[Reformer](model_doc/reformer)** (from Google Research) released with the paper [Reformer: The Efficient Transformer](https://arxiv.org/abs/2001.04451) by Nikita Kitaev, ลukasz Kaiser, Anselm Levskaya.
1. **[RegNet](model_doc/regnet)** (from META Platforms) released with the paper [Designing Network Design Space](https://arxiv.org/abs/2003.13678) by Ilija Radosavovic, Raj Prateek Kosaraju, Ross Girshick, Kaiming He, Piotr Dollรกr.
1. **[RemBERT](model_doc/rembert)** (from Google Research) released with the paper [Rethinking embedding coupling in pre-trained language models](https://arxiv.org/abs/2010.12821) by Hyung Won Chung, Thibault Fรฉvry, Henry Tsai, M. Johnson, Sebastian Ruder.
1. **[ResNet](model_doc/resnet)** (from Microsoft Research) released with the paper [Deep Residual Learning for Image Recognition](https://arxiv.org/abs/1512.03385) by Kaiming He, Xiangyu Zhang, Shaoqing Ren, Jian Sun.
1. **[RoBERTa](model_doc/roberta)** (from Facebook), released together with the paper [RoBERTa: A Robustly Optimized BERT Pretraining Approach](https://arxiv.org/abs/1907.11692) by Yinhan Liu, Myle Ott, Naman Goyal, Jingfei Du, Mandar Joshi, Danqi Chen, Omer Levy, Mike Lewis, Luke Zettlemoyer, Veselin Stoyanov.
1. **[RoCBert](model_doc/roc_bert)** (from WeChatAI) released with the paper [RoCBert: Robust Chinese Bert with Multimodal Contrastive Pretraining](https://aclanthology.org/2022.acl-long.65.pdf) by HuiSu, WeiweiShi, XiaoyuShen, XiaoZhou, TuoJi, JiaruiFang, JieZhou.
1. **[RoFormer](model_doc/roformer)** (from ZhuiyiTechnology), released together with the paper [RoFormer: Enhanced Transformer with Rotary Position Embedding](https://arxiv.org/abs/2104.09864) by Jianlin Su and Yu Lu and Shengfeng Pan and Bo Wen and Yunfeng Liu.
1. **[SegFormer](model_doc/segformer)** (from NVIDIA) released with the paper [SegFormer: Simple and Efficient Design for Semantic Segmentation with Transformers](https://arxiv.org/abs/2105.15203) by Enze Xie, Wenhai Wang, Zhiding Yu, Anima Anandkumar, Jose M. Alvarez, Ping Luo.
1. **[SEW](model_doc/sew)** (from ASAPP) released with the paper [Performance-Efficiency Trade-offs in Unsupervised Pre-training for Speech Recognition](https://arxiv.org/abs/2109.06870) by Felix Wu, Kwangyoun Kim, Jing Pan, Kyu Han, Kilian Q. Weinberger, Yoav Artzi.
1. **[SEW-D](model_doc/sew_d)** (from ASAPP) released with the paper [Performance-Efficiency Trade-offs in Unsupervised Pre-training for Speech Recognition](https://arxiv.org/abs/2109.06870) by Felix Wu, Kwangyoun Kim, Jing Pan, Kyu Han, Kilian Q. Weinberger, Yoav Artzi.
1. **[SpeechToTextTransformer](model_doc/speech_to_text)** (from Facebook), released together with the paper [fairseq S2T: Fast Speech-to-Text Modeling with fairseq](https://arxiv.org/abs/2010.05171) by Changhan Wang, Yun Tang, Xutai Ma, Anne Wu, Dmytro Okhonko, Juan Pino.
1. **[SpeechToTextTransformer2](model_doc/speech_to_text_2)** (from Facebook), released together with the paper [Large-Scale Self- and Semi-Supervised Learning for Speech Translation](https://arxiv.org/abs/2104.06678) by Changhan Wang, Anne Wu, Juan Pino, Alexei Baevski, Michael Auli, Alexis Conneau.
1. **[Splinter](model_doc/splinter)** (from Tel Aviv University), released together with the paper [Few-Shot Question Answering by Pretraining Span Selection](https://arxiv.org/abs/2101.00438) by Ori Ram, Yuval Kirstain, Jonathan Berant, Amir Globerson, Omer Levy.
1. **[SqueezeBERT](model_doc/squeezebert)** (from Berkeley) released with the paper [SqueezeBERT: What can computer vision teach NLP about efficient neural networks?](https://arxiv.org/abs/2006.11316) by Forrest N. Iandola, Albert E. Shaw, Ravi Krishna, and Kurt W. Keutzer.
1. **[Swin Transformer](model_doc/swin)** (from Microsoft) released with the paper [Swin Transformer: Hierarchical Vision Transformer using Shifted Windows](https://arxiv.org/abs/2103.14030) by Ze Liu, Yutong Lin, Yue Cao, Han Hu, Yixuan Wei, Zheng Zhang, Stephen Lin, Baining Guo.
1. **[Swin Transformer V2](model_doc/swinv2)** (from Microsoft) released with the paper [Swin Transformer V2: Scaling Up Capacity and Resolution](https://arxiv.org/abs/2111.09883) by Ze Liu, Han Hu, Yutong Lin, Zhuliang Yao, Zhenda Xie, Yixuan Wei, Jia Ning, Yue Cao, Zheng Zhang, Li Dong, Furu Wei, Baining Guo.
1. **[T5](model_doc/t5)** (from Google AI) released with the paper [Exploring the Limits of Transfer Learning with a Unified Text-to-Text Transformer](https://arxiv.org/abs/1910.10683) by Colin Raffel and Noam Shazeer and Adam Roberts and Katherine Lee and Sharan Narang and Michael Matena and Yanqi Zhou and Wei Li and Peter J. Liu.
1. **[T5v1.1](model_doc/t5v1.1)** (from Google AI) released in the repository [google-research/text-to-text-transfer-transformer](https://github.com/google-research/text-to-text-transfer-transformer/blob/main/released_checkpoints.md#t511) by Colin Raffel and Noam Shazeer and Adam Roberts and Katherine Lee and Sharan Narang and Michael Matena and Yanqi Zhou and Wei Li and Peter J. Liu.
1. **[Table Transformer](model_doc/table-transformer)** (from Microsoft Research) released with the paper [PubTables-1M: Towards Comprehensive Table Extraction From Unstructured Documents](https://arxiv.org/abs/2110.00061) by Brandon Smock, Rohith Pesala, Robin Abraham.
1. **[TAPAS](model_doc/tapas)** (from Google AI) released with the paper [TAPAS: Weakly Supervised Table Parsing via Pre-training](https://arxiv.org/abs/2004.02349) by Jonathan Herzig, Paweล Krzysztof Nowak, Thomas Mรผller, Francesco Piccinno and Julian Martin Eisenschlos.
1. **[TAPEX](model_doc/tapex)** (from Microsoft Research) released with the paper [TAPEX: Table Pre-training via Learning a Neural SQL Executor](https://arxiv.org/abs/2107.07653) by Qian Liu, Bei Chen, Jiaqi Guo, Morteza Ziyadi, Zeqi Lin, Weizhu Chen, Jian-Guang Lou.
1. **[Time Series Transformer](model_doc/time_series_transformer)** (from HuggingFace).
1. **[Trajectory Transformer](model_doc/trajectory_transformers)** (from the University of California at Berkeley) released with the paper [Offline Reinforcement Learning as One Big Sequence Modeling Problem](https://arxiv.org/abs/2106.02039) by Michael Janner, Qiyang Li, Sergey Levine
1. **[Transformer-XL](model_doc/transfo-xl)** (from Google/CMU) released with the paper [Transformer-XL: Attentive Language Models Beyond a Fixed-Length Context](https://arxiv.org/abs/1901.02860) by Zihang Dai*, Zhilin Yang*, Yiming Yang, Jaime Carbonell, Quoc V. Le, Ruslan Salakhutdinov.
1. **[TrOCR](model_doc/trocr)** (from Microsoft), released together with the paper [TrOCR: Transformer-based Optical Character Recognition with Pre-trained Models](https://arxiv.org/abs/2109.10282) by Minghao Li, Tengchao Lv, Lei Cui, Yijuan Lu, Dinei Florencio, Cha Zhang, Zhoujun Li, Furu Wei.
1. **[UL2](model_doc/ul2)** (from Google Research) released with the paper [Unifying Language Learning Paradigms](https://arxiv.org/abs/2205.05131v1) by Yi Tay, Mostafa Dehghani, Vinh Q. Tran, Xavier Garcia, Dara Bahri, Tal Schuster, Huaixiu Steven Zheng, Neil Houlsby, Donald Metzler
1. **[UniSpeech](model_doc/unispeech)** (from Microsoft Research) released with the paper [UniSpeech: Unified Speech Representation Learning with Labeled and Unlabeled Data](https://arxiv.org/abs/2101.07597) by Chengyi Wang, Yu Wu, Yao Qian, Kenichi Kumatani, Shujie Liu, Furu Wei, Michael Zeng, Xuedong Huang.
1. **[UniSpeechSat](model_doc/unispeech-sat)** (from Microsoft Research) released with the paper [UNISPEECH-SAT: UNIVERSAL SPEECH REPRESENTATION LEARNING WITH SPEAKER AWARE PRE-TRAINING](https://arxiv.org/abs/2110.05752) by Sanyuan Chen, Yu Wu, Chengyi Wang, Zhengyang Chen, Zhuo Chen, Shujie Liu, Jian Wu, Yao Qian, Furu Wei, Jinyu Li, Xiangzhan Yu.
1. **[VAN](model_doc/van)** (from Tsinghua University and Nankai University) released with the paper [Visual Attention Network](https://arxiv.org/abs/2202.09741) by Meng-Hao Guo, Cheng-Ze Lu, Zheng-Ning Liu, Ming-Ming Cheng, Shi-Min Hu.
1. **[VideoMAE](model_doc/videomae)** (from Multimedia Computing Group, Nanjing University) released with the paper [VideoMAE: Masked Autoencoders are Data-Efficient Learners for Self-Supervised Video Pre-Training](https://arxiv.org/abs/2203.12602) by Zhan Tong, Yibing Song, Jue Wang, Limin Wang.
1. **[ViLT](model_doc/vilt)** (from NAVER AI Lab/Kakao Enterprise/Kakao Brain) released with the paper [ViLT: Vision-and-Language Transformer Without Convolution or Region Supervision](https://arxiv.org/abs/2102.03334) by Wonjae Kim, Bokyung Son, Ildoo Kim.
1. **[Vision Transformer (ViT)](model_doc/vit)** (from Google AI) released with the paper [An Image is Worth 16x16 Words: Transformers for Image Recognition at Scale](https://arxiv.org/abs/2010.11929) by Alexey Dosovitskiy, Lucas Beyer, Alexander Kolesnikov, Dirk Weissenborn, Xiaohua Zhai, Thomas Unterthiner, Mostafa Dehghani, Matthias Minderer, Georg Heigold, Sylvain Gelly, Jakob Uszkoreit, Neil Houlsby.
1. **[VisualBERT](model_doc/visual_bert)** (from UCLA NLP) released with the paper [VisualBERT: A Simple and Performant Baseline for Vision and Language](https://arxiv.org/pdf/1908.03557) by Liunian Harold Li, Mark Yatskar, Da Yin, Cho-Jui Hsieh, Kai-Wei Chang.
1. **[ViTMAE](model_doc/vit_mae)** (from Meta AI) released with the paper [Masked Autoencoders Are Scalable Vision Learners](https://arxiv.org/abs/2111.06377) by Kaiming He, Xinlei Chen, Saining Xie, Yanghao Li, Piotr Dollรกr, Ross Girshick.
1. **[ViTMSN](model_doc/vit_msn)** (from Meta AI) released with the paper [Masked Siamese Networks for Label-Efficient Learning](https://arxiv.org/abs/2204.07141) by Mahmoud Assran, Mathilde Caron, Ishan Misra, Piotr Bojanowski, Florian Bordes, Pascal Vincent, Armand Joulin, Michael Rabbat, Nicolas Ballas.
1. **[Wav2Vec2](model_doc/wav2vec2)** (from Facebook AI) released with the paper [wav2vec 2.0: A Framework for Self-Supervised Learning of Speech Representations](https://arxiv.org/abs/2006.11477) by Alexei Baevski, Henry Zhou, Abdelrahman Mohamed, Michael Auli.
1. **[Wav2Vec2-Conformer](model_doc/wav2vec2-conformer)** (from Facebook AI) released with the paper [FAIRSEQ S2T: Fast Speech-to-Text Modeling with FAIRSEQ](https://arxiv.org/abs/2010.05171) by Changhan Wang, Yun Tang, Xutai Ma, Anne Wu, Sravya Popuri, Dmytro Okhonko, Juan Pino.
1. **[Wav2Vec2Phoneme](model_doc/wav2vec2_phoneme)** (from Facebook AI) released with the paper [Simple and Effective Zero-shot Cross-lingual Phoneme Recognition](https://arxiv.org/abs/2109.11680) by Qiantong Xu, Alexei Baevski, Michael Auli.
1. **[WavLM](model_doc/wavlm)** (from Microsoft Research) released with the paper [WavLM: Large-Scale Self-Supervised Pre-Training for Full Stack Speech Processing](https://arxiv.org/abs/2110.13900) by Sanyuan Chen, Chengyi Wang, Zhengyang Chen, Yu Wu, Shujie Liu, Zhuo Chen, Jinyu Li, Naoyuki Kanda, Takuya Yoshioka, Xiong Xiao, Jian Wu, Long Zhou, Shuo Ren, Yanmin Qian, Yao Qian, Jian Wu, Michael Zeng, Furu Wei.
1. **[Whisper](model_doc/whisper)** (from OpenAI) released with the paper [Robust Speech Recognition via Large-Scale Weak Supervision](https://cdn.openai.com/papers/whisper.pdf) by Alec Radford, Jong Wook Kim, Tao Xu, Greg Brockman, Christine McLeavey, Ilya Sutskever.
1. **[X-CLIP](model_doc/xclip)** (from Microsoft Research) released with the paper [Expanding Language-Image Pretrained Models for General Video Recognition](https://arxiv.org/abs/2208.02816) by Bolin Ni, Houwen Peng, Minghao Chen, Songyang Zhang, Gaofeng Meng, Jianlong Fu, Shiming Xiang, Haibin Ling.
1. **[XGLM](model_doc/xglm)** (From Facebook AI) released with the paper [Few-shot Learning with Multilingual Language Models](https://arxiv.org/abs/2112.10668) by Xi Victoria Lin, Todor Mihaylov, Mikel Artetxe, Tianlu Wang, Shuohui Chen, Daniel Simig, Myle Ott, Naman Goyal, Shruti Bhosale, Jingfei Du, Ramakanth Pasunuru, Sam Shleifer, Punit Singh Koura, Vishrav Chaudhary, Brian O'Horo, Jeff Wang, Luke Zettlemoyer, Zornitsa Kozareva, Mona Diab, Veselin Stoyanov, Xian Li.
1. **[XLM](model_doc/xlm)** (from Facebook) released together with the paper [Cross-lingual Language Model Pretraining](https://arxiv.org/abs/1901.07291) by Guillaume Lample and Alexis Conneau.
1. **[XLM-ProphetNet](model_doc/xlm-prophetnet)** (from Microsoft Research) released with the paper [ProphetNet: Predicting Future N-gram for Sequence-to-Sequence Pre-training](https://arxiv.org/abs/2001.04063) by Yu Yan, Weizhen Qi, Yeyun Gong, Dayiheng Liu, Nan Duan, Jiusheng Chen, Ruofei Zhang and Ming Zhou.
1. **[XLM-RoBERTa](model_doc/xlm-roberta)** (from Facebook AI), released together with the paper [Unsupervised Cross-lingual Representation Learning at Scale](https://arxiv.org/abs/1911.02116) by Alexis Conneau*, Kartikay Khandelwal*, Naman Goyal, Vishrav Chaudhary, Guillaume Wenzek, Francisco Guzmรกn, Edouard Grave, Myle Ott, Luke Zettlemoyer and Veselin Stoyanov.
1. **[XLM-RoBERTa-XL](model_doc/xlm-roberta-xl)** (from Facebook AI), released together with the paper [Larger-Scale Transformers for Multilingual Masked Language Modeling](https://arxiv.org/abs/2105.00572) by Naman Goyal, Jingfei Du, Myle Ott, Giri Anantharaman, Alexis Conneau.
1. **[XLNet](model_doc/xlnet)** (from Google/CMU) released with the paper [โXLNet: Generalized Autoregressive Pretraining for Language Understanding](https://arxiv.org/abs/1906.08237) by Zhilin Yang*, Zihang Dai*, Yiming Yang, Jaime Carbonell, Ruslan Salakhutdinov, Quoc V. Le.
1. **[XLS-R](model_doc/xls_r)** (from Facebook AI) released with the paper [XLS-R: Self-supervised Cross-lingual Speech Representation Learning at Scale](https://arxiv.org/abs/2111.09296) by Arun Babu, Changhan Wang, Andros Tjandra, Kushal Lakhotia, Qiantong Xu, Naman Goyal, Kritika Singh, Patrick von Platen, Yatharth Saraf, Juan Pino, Alexei Baevski, Alexis Conneau, Michael Auli.
1. **[XLSR-Wav2Vec2](model_doc/xlsr_wav2vec2)** (from Facebook AI) released with the paper [Unsupervised Cross-Lingual Representation Learning For Speech Recognition](https://arxiv.org/abs/2006.13979) by Alexis Conneau, Alexei Baevski, Ronan Collobert, Abdelrahman Mohamed, Michael Auli.
1. **[YOLOS](model_doc/yolos)** (from Huazhong University of Science & Technology) released with the paper [You Only Look at One Sequence: Rethinking Transformer in Vision through Object Detection](https://arxiv.org/abs/2106.00666) by Yuxin Fang, Bencheng Liao, Xinggang Wang, Jiemin Fang, Jiyang Qi, Rui Wu, Jianwei Niu, Wenyu Liu.
1. **[YOSO](model_doc/yoso)** (from the University of Wisconsin - Madison) released with the paper [You Only Sample (Almost) Once: Linear Cost Self-Attention Via Bernoulli Sampling](https://arxiv.org/abs/2111.09714) by Zhanpeng Zeng, Yunyang Xiong, Sathya N. Ravi, Shailesh Acharya, Glenn Fung, Vikas Singh.
### ์ง์ ํ๋ ์์ํฌ[[supported-framework]]
์๋ ํ๋ ๋ผ์ด๋ธ๋ฌ๋ฆฌ ์ ๊ฐ ๋ชจ๋ธ์ ์ง์ ํํฉ์ ๋ํ๋
๋๋ค. ํ ํฐํ๋ฅผ ํ์ด์ฌ (๋ณ์นญ "slow") ๋๋ ๐ค Tokenizers (๋ณ์นญ "fast") ๋ผ์ด๋ธ๋ฌ๋ฆฌ๋ก ํ๋์ง; (Flax๋ฅผ ํตํ) Jax, PyTorch, TensorFlow ์ค ์ด๋ค ํ๋ ์์ํฌ๋ฅผ ์ง์ํ๋์ง ํ์๋์ด ์์ต๋๋ค.
<!--This table is updated automatically from the auto modules with _make fix-copies_. Do not update manually!-->
| Model | Tokenizer slow | Tokenizer fast | PyTorch support | TensorFlow support | Flax Support |
|:---------------------------:|:--------------:|:--------------:|:---------------:|:------------------:|:------------:|
| ALBERT | โ
| โ
| โ
| โ
| โ
|
| BART | โ
| โ
| โ
| โ
| โ
|
| BEiT | โ | โ | โ
| โ | โ
|
| BERT | โ
| โ
| โ
| โ
| โ
|
| Bert Generation | โ
| โ | โ
| โ | โ |
| BigBird | โ
| โ
| โ
| โ | โ
|
| BigBird-Pegasus | โ | โ | โ
| โ | โ |
| Blenderbot | โ
| โ
| โ
| โ
| โ
|
| BlenderbotSmall | โ
| โ
| โ
| โ
| โ
|
| BLOOM | โ | โ
| โ
| โ | โ |
| CamemBERT | โ
| โ
| โ
| โ
| โ |
| CANINE | โ
| โ | โ
| โ | โ |
| CLIP | โ
| โ
| โ
| โ
| โ
|
| CLIPSeg | โ | โ | โ
| โ | โ |
| CodeGen | โ
| โ
| โ
| โ | โ |
| Conditional DETR | โ | โ | โ
| โ | โ |
| ConvBERT | โ
| โ
| โ
| โ
| โ |
| ConvNeXT | โ | โ | โ
| โ
| โ |
| CTRL | โ
| โ | โ
| โ
| โ |
| CvT | โ | โ | โ
| โ
| โ |
| Data2VecAudio | โ | โ | โ
| โ | โ |
| Data2VecText | โ | โ | โ
| โ | โ |
| Data2VecVision | โ | โ | โ
| โ
| โ |
| DeBERTa | โ
| โ
| โ
| โ
| โ |
| DeBERTa-v2 | โ
| โ
| โ
| โ
| โ |
| Decision Transformer | โ | โ | โ
| โ | โ |
| Deformable DETR | โ | โ | โ
| โ | โ |
| DeiT | โ | โ | โ
| โ
| โ |
| DETR | โ | โ | โ
| โ | โ |
| DistilBERT | โ
| โ
| โ
| โ
| โ
|
| DonutSwin | โ | โ | โ
| โ | โ |
| DPR | โ
| โ
| โ
| โ
| โ |
| DPT | โ | โ | โ
| โ | โ |
| ELECTRA | โ
| โ
| โ
| โ
| โ
|
| Encoder decoder | โ | โ | โ
| โ
| โ
|
| ERNIE | โ | โ | โ
| โ | โ |
| ESM | โ
| โ | โ
| โ
| โ |
| FairSeq Machine-Translation | โ
| โ | โ
| โ | โ |
| FlauBERT | โ
| โ | โ
| โ
| โ |
| FLAVA | โ | โ | โ
| โ | โ |
| FNet | โ
| โ
| โ
| โ | โ |
| Funnel Transformer | โ
| โ
| โ
| โ
| โ |
| GLPN | โ | โ | โ
| โ | โ |
| GPT Neo | โ | โ | โ
| โ | โ
|
| GPT NeoX | โ | โ
| โ
| โ | โ |
| GPT NeoX Japanese | โ
| โ | โ
| โ | โ |
| GPT-J | โ | โ | โ
| โ
| โ
|
| GroupViT | โ | โ | โ
| โ
| โ |
| Hubert | โ | โ | โ
| โ
| โ |
| I-BERT | โ | โ | โ
| โ | โ |
| ImageGPT | โ | โ | โ
| โ | โ |
| Jukebox | โ
| โ | โ
| โ | โ |
| LayoutLM | โ
| โ
| โ
| โ
| โ |
| LayoutLMv2 | โ
| โ
| โ
| โ | โ |
| LayoutLMv3 | โ
| โ
| โ
| โ
| โ |
| LED | โ
| โ
| โ
| โ
| โ |
| LeViT | โ | โ | โ
| โ | โ |
| LiLT | โ | โ | โ
| โ | โ |
| Longformer | โ
| โ
| โ
| โ
| โ |
| LongT5 | โ | โ | โ
| โ | โ
|
| LUKE | โ
| โ | โ
| โ | โ |
| LXMERT | โ
| โ
| โ
| โ
| โ |
| M-CTC-T | โ | โ | โ
| โ | โ |
| M2M100 | โ
| โ | โ
| โ | โ |
| Marian | โ
| โ | โ
| โ
| โ
|
| MarkupLM | โ
| โ
| โ
| โ | โ |
| MaskFormer | โ | โ | โ
| โ | โ |
| mBART | โ
| โ
| โ
| โ
| โ
|
| Megatron-BERT | โ | โ | โ
| โ | โ |
| MobileBERT | โ
| โ
| โ
| โ
| โ |
| MobileViT | โ | โ | โ
| โ
| โ |
| MPNet | โ
| โ
| โ
| โ
| โ |
| MT5 | โ
| โ
| โ
| โ
| โ
|
| MVP | โ
| โ
| โ
| โ | โ |
| Nezha | โ | โ | โ
| โ | โ |
| Nystrรถmformer | โ | โ | โ
| โ | โ |
| OpenAI GPT | โ
| โ
| โ
| โ
| โ |
| OpenAI GPT-2 | โ
| โ
| โ
| โ
| โ
|
| OPT | โ | โ | โ
| โ
| โ
|
| OWL-ViT | โ | โ | โ
| โ | โ |
| Pegasus | โ
| โ
| โ
| โ
| โ
|
| PEGASUS-X | โ | โ | โ
| โ | โ |
| Perceiver | โ
| โ | โ
| โ | โ |
| PLBart | โ
| โ | โ
| โ | โ |
| PoolFormer | โ | โ | โ
| โ | โ |
| ProphetNet | โ
| โ | โ
| โ | โ |
| QDQBert | โ | โ | โ
| โ | โ |
| RAG | โ
| โ | โ
| โ
| โ |
| REALM | โ
| โ
| โ
| โ | โ |
| Reformer | โ
| โ
| โ
| โ | โ |
| RegNet | โ | โ | โ
| โ
| โ
|
| RemBERT | โ
| โ
| โ
| โ
| โ |
| ResNet | โ | โ | โ
| โ
| โ
|
| RetriBERT | โ
| โ
| โ
| โ | โ |
| RoBERTa | โ
| โ
| โ
| โ
| โ
|
| RoCBert | โ
| โ | โ
| โ | โ |
| RoFormer | โ
| โ
| โ
| โ
| โ
|
| SegFormer | โ | โ | โ
| โ
| โ |
| SEW | โ | โ | โ
| โ | โ |
| SEW-D | โ | โ | โ
| โ | โ |
| Speech Encoder decoder | โ | โ | โ
| โ | โ
|
| Speech2Text | โ
| โ | โ
| โ
| โ |
| Speech2Text2 | โ
| โ | โ | โ | โ |
| Splinter | โ
| โ
| โ
| โ | โ |
| SqueezeBERT | โ
| โ
| โ
| โ | โ |
| Swin Transformer | โ | โ | โ
| โ
| โ |
| Swin Transformer V2 | โ | โ | โ
| โ | โ |
| T5 | โ
| โ
| โ
| โ
| โ
|
| Table Transformer | โ | โ | โ
| โ | โ |
| TAPAS | โ
| โ | โ
| โ
| โ |
| Time Series Transformer | โ | โ | โ
| โ | โ |
| Trajectory Transformer | โ | โ | โ
| โ | โ |
| Transformer-XL | โ
| โ | โ
| โ
| โ |
| TrOCR | โ | โ | โ
| โ | โ |
| UniSpeech | โ | โ | โ
| โ | โ |
| UniSpeechSat | โ | โ | โ
| โ | โ |
| VAN | โ | โ | โ
| โ | โ |
| VideoMAE | โ | โ | โ
| โ | โ |
| ViLT | โ | โ | โ
| โ | โ |
| Vision Encoder decoder | โ | โ | โ
| โ
| โ
|
| VisionTextDualEncoder | โ | โ | โ
| โ | โ
|
| VisualBERT | โ | โ | โ
| โ | โ |
| ViT | โ | โ | โ
| โ
| โ
|
| ViTMAE | โ | โ | โ
| โ
| โ |
| ViTMSN | โ | โ | โ
| โ | โ |
| Wav2Vec2 | โ
| โ | โ
| โ
| โ
|
| Wav2Vec2-Conformer | โ | โ | โ
| โ | โ |
| WavLM | โ | โ | โ
| โ | โ |
| Whisper | โ
| โ | โ
| โ
| โ |
| X-CLIP | โ | โ | โ
| โ | โ |
| XGLM | โ
| โ
| โ
| โ
| โ
|
| XLM | โ
| โ | โ
| โ
| โ |
| XLM-ProphetNet | โ
| โ | โ
| โ | โ |
| XLM-RoBERTa | โ
| โ
| โ
| โ
| โ
|
| XLM-RoBERTa-XL | โ | โ | โ
| โ | โ |
| XLNet | โ
| โ
| โ
| โ
| โ |
| YOLOS | โ | โ | โ
| โ | โ |
| YOSO | โ | โ | โ
| โ | โ |
<!-- End table-->
| 0 |
hf_public_repos/transformers/docs/source | hf_public_repos/transformers/docs/source/ko/tflite.md | <!--Copyright 2023 The HuggingFace Team. All rights reserved.
Licensed under the Apache License, Version 2.0 (the "License"); you may not use this file except in compliance with
the License. You may obtain a copy of the License at
http://www.apache.org/licenses/LICENSE-2.0
Unless required by applicable law or agreed to in writing, software distributed under the License is distributed on
an "AS IS" BASIS, WITHOUT WARRANTIES OR CONDITIONS OF ANY KIND, either express or implied. See the License for the
specific language governing permissions and limitations under the License.
โ ๏ธ Note that this file is in Markdown but contain specific syntax for our doc-builder (similar to MDX) that may not be
rendered properly in your Markdown viewer.
-->
# TFLite๋ก ๋ด๋ณด๋ด๊ธฐ[[export-to-tflite]]
[TensorFlow Lite](https://www.tensorflow.org/lite/guide)๋ ์์์ด ์ ํ๋ ํด๋ํฐ, ์๋ฒ ๋๋ ์์คํ
, ์ฌ๋ฌผ์ธํฐ๋ท(IoT) ๊ธฐ๊ธฐ์์
๊ธฐ๊ณํ์ต ๋ชจ๋ธ์ ๋ฐฐํฌํ๊ธฐ ์ํ ๊ฒฝ๋ ํ๋ ์์ํฌ์
๋๋ค.
TFLite๋ ์ฐ์ฐ ๋ฅ๋ ฅ, ๋ฉ๋ชจ๋ฆฌ, ์ ๋ ฅ ์๋น๊ฐ ์ ํ๋ ๊ธฐ๊ธฐ์์ ๋ชจ๋ธ์ ํจ์จ์ ์ผ๋ก ์ต์ ํํ๊ณ ์คํํ๊ธฐ ์ํด
์ค๊ณ๋์์ต๋๋ค.
TensorFlow Lite ๋ชจ๋ธ์ `.tflite` ํ์ผ ํ์ฅ์๋ก ์๋ณ๋๋ ํน์ํ๊ณ ํจ์จ์ ์ธ ํด๋์ฉ ํฌ๋งท์ผ๋ก ํํ๋ฉ๋๋ค.
๐ค Optimum์ `exporters.tflite` ๋ชจ๋๋ก ๐ค Transformers ๋ชจ๋ธ์ TFLite๋ก ๋ด๋ณด๋ด๋ ๊ธฐ๋ฅ์ ์ ๊ณตํฉ๋๋ค.
์ง์๋๋ ๋ชจ๋ธ ์ํคํ
์ฒ ๋ชฉ๋ก์ [๐ค Optimum ๋ฌธ์](https://huggingface.co/docs/optimum/exporters/tflite/overview)๋ฅผ ์ฐธ๊ณ ํ์ธ์.
๋ชจ๋ธ์ TFLite๋ก ๋ด๋ณด๋ด๋ ค๋ฉด, ํ์ํ ์ข
์์ฑ์ ์ค์นํ์ธ์:
```bash
pip install optimum[exporters-tf]
```
๋ชจ๋ ์ฌ์ฉ ๊ฐ๋ฅํ ์ธ์๋ฅผ ํ์ธํ๋ ค๋ฉด, [๐ค Optimum ๋ฌธ์](https://huggingface.co/docs/optimum/main/en/exporters/tflite/usage_guides/export_a_model)๋ฅผ ์ฐธ๊ณ ํ๊ฑฐ๋
ํฐ๋ฏธ๋์์ ๋์๋ง์ ์ดํด๋ณด์ธ์:
```bash
optimum-cli export tflite --help
```
์๋ฅผ ๋ค์ด ๐ค Hub์์์ `bert-base-uncased` ๋ชจ๋ธ ์ฒดํฌํฌ์ธํธ๋ฅผ ๋ด๋ณด๋ด๋ ค๋ฉด, ๋ค์ ๋ช
๋ น์ ์คํํ์ธ์:
```bash
optimum-cli export tflite --model bert-base-uncased --sequence_length 128 bert_tflite/
```
๋ค์๊ณผ ๊ฐ์ด ์งํ ์ํฉ์ ๋ํ๋ด๋ ๋ก๊ทธ์ ๊ฒฐ๊ณผ๋ฌผ์ธ `model.tflite`๊ฐ ์ ์ฅ๋ ์์น๋ฅผ ๋ณด์ฌ์ฃผ๋ ๋ก๊ทธ๊ฐ ํ์๋ฉ๋๋ค:
```bash
Validating TFLite model...
-[โ] TFLite model output names match reference model (logits)
- Validating TFLite Model output "logits":
-[โ] (1, 128, 30522) matches (1, 128, 30522)
-[x] values not close enough, max diff: 5.817413330078125e-05 (atol: 1e-05)
The TensorFlow Lite export succeeded with the warning: The maximum absolute difference between the output of the reference model and the TFLite exported model is not within the set tolerance 1e-05:
- logits: max diff = 5.817413330078125e-05.
The exported model was saved at: bert_tflite
```
์ ์์ ๋ ๐ค Hub์์์ ์ฒดํฌํฌ์ธํธ๋ฅผ ๋ด๋ณด๋ด๋ ๋ฐฉ๋ฒ์ ๋ณด์ฌ์ค๋๋ค.
๋ก์ปฌ ๋ชจ๋ธ์ ๋ด๋ณด๋ธ๋ค๋ฉด, ๋จผ์ ๋ชจ๋ธ ๊ฐ์ค์น์ ํ ํฌ๋์ด์ ํ์ผ์ด ๋ชจ๋ ๊ฐ์ ๋๋ ํฐ๋ฆฌ( `local_path` )์ ์ ์ฅ๋๋์ง ํ์ธํ์ธ์.
CLI๋ฅผ ์ฌ์ฉํ ๋, ๐ค Hub์์์ ์ฒดํฌํฌ์ธํธ ์ด๋ฆ ๋์ `model` ์ธ์์ `local_path`๋ฅผ ์ ๋ฌํ๋ฉด ๋ฉ๋๋ค. | 0 |
hf_public_repos/transformers/docs/source | hf_public_repos/transformers/docs/source/ko/testing.md | <!--Copyright 2020 The HuggingFace Team. All rights reserved.
Licensed under the Apache License, Version 2.0 (the "License"); you may not use this file except in compliance with
the License. You may obtain a copy of the License at
http://www.apache.org/licenses/LICENSE-2.0
Unless required by applicable law or agreed to in writing, software distributed under the License is distributed on
an "AS IS" BASIS, WITHOUT WARRANTIES OR CONDITIONS OF ANY KIND, either express or implied. See the License for the
specific language governing permissions and limitations under the License.
โ ๏ธ Note that this file is in Markdown but contain specific syntax for our doc-builder (similar to MDX) that may not be
rendered properly in your Markdown viewer.
-->
# ํ
์คํธ[[testing]]
๋จผ์ ๐ค Transformers ๋ชจ๋ธ์ด ์ด๋ป๊ฒ ํ
์คํธ๋๋์ง ์ดํด๋ณด๊ณ , ์๋ก์ด ํ
์คํธ๋ฅผ ์์ฑ ๋ฐ ๊ธฐ์กด ํ
์คํธ๋ฅผ ๊ฐ์ ํ๋ ๋ฐฉ๋ฒ์ ์์๋ด
์๋ค.
์ด ์ ์ฅ์์๋ 2๊ฐ์ ํ
์คํธ ์ค์ํธ๊ฐ ์์ต๋๋ค:
1. `tests` - ์ผ๋ฐ API์ ๋ํ ํ
์คํธ
2. `examples` - API์ ์ผ๋ถ๊ฐ ์๋ ๋ค์ํ ์์ฉ ํ๋ก๊ทธ๋จ์ ๋ํ ํ
์คํธ
## Transformers ํ
์คํธ ๋ฐฉ๋ฒ[[how-transformers-are-tested]]
1. PR์ด ์ ์ถ๋๋ฉด 9๊ฐ์ CircleCi ์์
์ผ๋ก ํ
์คํธ๊ฐ ์งํ๋ฉ๋๋ค. ํด๋น PR์ ๋ํด ์๋ก์ด ์ปค๋ฐ์ด ์์ฑ๋ ๋๋ง๋ค ํ
์คํธ๋ ๋ค์ ์งํ๋ฉ๋๋ค. ์ด ์์
๋ค์
์ด [config ํ์ผ](https://github.com/huggingface/transformers/tree/main/.circleci/config.yml)์ ์ ์๋์ด ์์ผ๋ฏ๋ก ํ์ํ๋ค๋ฉด
์ฌ์ฉ์์ ๋ก์ปฌ ํ๊ฒฝ์์ ๋์ผํ๊ฒ ์ฌํํด ๋ณผ ์ ์์ต๋๋ค.
์ด CI ์์
์ `@slow` ํ
์คํธ๋ฅผ ์คํํ์ง ์์ต๋๋ค.
2. [github actions](https://github.com/huggingface/transformers/actions)์ ์ํด ์คํ๋๋ ์์
์ 3๊ฐ์
๋๋ค:
- [torch hub integration](https://github.com/huggingface/transformers/tree/main/.github/workflows/github-torch-hub.yml):
torch hub integration์ด ์๋ํ๋์ง ํ์ธํฉ๋๋ค.
- [self-hosted (push)](https://github.com/huggingface/transformers/tree/main/.github/workflows/self-push.yml): `main` ๋ธ๋์น์์ ์ปค๋ฐ์ด ์
๋ฐ์ดํธ๋ ๊ฒฝ์ฐ์๋ง GPU๋ฅผ ์ด์ฉํ ๋น ๋ฅธ ํ
์คํธ๋ฅผ ์คํํฉ๋๋ค.
์ด๋ `src`, `tests`, `.github` ํด๋ ์ค ํ๋์ ์ฝ๋๊ฐ ์
๋ฐ์ดํธ๋ ๊ฒฝ์ฐ์๋ง ์คํ๋ฉ๋๋ค.
(model card, notebook, ๊ธฐํ ๋ฑ๋ฑ์ ์ถ๊ฐํ ๊ฒฝ์ฐ ์คํ๋์ง ์๋๋ก ํ๊ธฐ ์ํด์์
๋๋ค)
- [self-hosted runner](https://github.com/huggingface/transformers/tree/main/.github/workflows/self-scheduled.yml): `tests` ๋ฐ `examples`์์
GPU๋ฅผ ์ด์ฉํ ์ผ๋ฐ ํ
์คํธ, ๋๋ฆฐ ํ
์คํธ๋ฅผ ์คํํฉ๋๋ค.
```bash
RUN_SLOW=1 pytest tests/
RUN_SLOW=1 pytest examples/
```
๊ฒฐ๊ณผ๋ [์ฌ๊ธฐ](https://github.com/huggingface/transformers/actions)์์ ํ์ธํ ์ ์์ต๋๋ค.
## ํ
์คํธ ์คํ[[running-tests]]
### ์คํํ ํ
์คํธ ์ ํ[[choosing-which-tests-to-run]]
์ด ๋ฌธ์๋ ํ
์คํธ๋ฅผ ์คํํ๋ ๋ค์ํ ๋ฐฉ๋ฒ์ ๋ํด ์์ธํ ์ค๋ช
ํฉ๋๋ค.
๋ชจ๋ ๋ด์ฉ์ ์ฝ์ ํ์๋, ๋ ์์ธํ ๋ด์ฉ์ด ํ์ํ๋ค๋ฉด [์ฌ๊ธฐ](https://docs.pytest.org/en/latest/usage.html)์์ ํ์ธํ ์ ์์ต๋๋ค.
๋ค์์ ๊ฐ์ฅ ์ ์ฉํ ํ
์คํธ ์คํ ๋ฐฉ๋ฒ ๋ช ๊ฐ์ง์
๋๋ค.
๋ชจ๋ ์คํ:
```console
pytest
```
๋๋:
```bash
make test
```
ํ์๋ ๋ค์๊ณผ ๊ฐ์ด ์ ์๋ฉ๋๋ค:
```bash
python -m pytest -n auto --dist=loadfile -s -v ./tests/
```
์์ ๋ช
๋ น์ด๋ pytest์๊ฒ ์๋์ ๋ด์ฉ์ ์ ๋ฌํฉ๋๋ค:
- ์ฌ์ฉ ๊ฐ๋ฅํ CPU ์ฝ์ด ์๋งํผ ํ
์คํธ ํ๋ก์ธ์ค๋ฅผ ์คํํฉ๋๋ค. (RAM์ด ์ถฉ๋ถํ์ง ์๋ค๋ฉด, ํ
์คํธ ํ๋ก์ธ์ค ์๊ฐ ๋๋ฌด ๋ง์ ์ ์์ต๋๋ค!)
- ๋์ผํ ํ์ผ์ ๋ชจ๋ ํ
์คํธ๋ ๋์ผํ ํ
์คํธ ํ๋ก์ธ์ค์์ ์คํ๋์ด์ผ ํฉ๋๋ค.
- ์ถ๋ ฅ์ ์บก์ฒํ์ง ์์ต๋๋ค.
- ์์ธํ ๋ชจ๋๋ก ์คํํฉ๋๋ค.
### ๋ชจ๋ ํ
์คํธ ๋ชฉ๋ก ๊ฐ์ ธ์ค๊ธฐ[[getting-the-list-of-all-tests]]
ํ
์คํธ ์ค์ํธ์ ๋ชจ๋ ํ
์คํธ:
```bash
pytest --collect-only -q
```
์ง์ ๋ ํ
์คํธ ํ์ผ์ ๋ชจ๋ ํ
์คํธ:
```bash
pytest tests/test_optimization.py --collect-only -q
```
### ํน์ ํ
์คํธ ๋ชจ๋ ์คํ[[run-a-specific-test-module]]
๊ฐ๋ณ ํ
์คํธ ๋ชจ๋ ์คํํ๊ธฐ:
```bash
pytest tests/test_logging.py
```
### ํน์ ํ
์คํธ ์คํ[[run-specific-tests]]
๋๋ถ๋ถ์ ํ
์คํธ ๋ด๋ถ์์๋ unittest๊ฐ ์ฌ์ฉ๋ฉ๋๋ค. ๋ฐ๋ผ์ ํน์ ํ์ ํ
์คํธ๋ฅผ ์คํํ๋ ค๋ฉด ํด๋น ํ
์คํธ๋ฅผ ํฌํจํ๋ unittest ํด๋์ค์ ์ด๋ฆ์ ์์์ผ ํฉ๋๋ค.
์๋ฅผ ๋ค์ด ๋ค์๊ณผ ๊ฐ์ ์ ์์ต๋๋ค:
```bash
pytest tests/test_optimization.py::OptimizationTest::test_adam_w
```
์์ ๋ช
๋ น์ด์ ์๋ฏธ๋ ๋ค์๊ณผ ๊ฐ์ต๋๋ค:
- `tests/test_optimization.py` - ํ
์คํธ๊ฐ ์๋ ํ์ผ
- `OptimizationTest` - ํด๋์ค์ ์ด๋ฆ
- `test_adam_w` - ํน์ ํ
์คํธ ํจ์์ ์ด๋ฆ
ํ์ผ์ ์ฌ๋ฌ ํด๋์ค๊ฐ ํฌํจ๋ ๊ฒฝ์ฐ, ํน์ ํด๋์ค์ ํ
์คํธ๋ง ์คํํ ์๋ ์์ต๋๋ค. ์๋ฅผ ๋ค์ด ๋ค์๊ณผ ๊ฐ์ต๋๋ค:
```bash
pytest tests/test_optimization.py::OptimizationTest
```
์ด ๋ช
๋ น์ด๋ ํด๋น ํด๋์ค ๋ด๋ถ์ ๋ชจ๋ ํ
์คํธ๋ฅผ ์คํํฉ๋๋ค.
์์์ ์ธ๊ธํ ๊ฒ์ฒ๋ผ `OptimizationTest` ํด๋์ค์ ํฌํจ๋ ํ
์คํธ๋ฅผ ํ์ธํ ์ ์์ต๋๋ค.
```bash
pytest tests/test_optimization.py::OptimizationTest --collect-only -q
```
ํค์๋ ํํ์์ ์ฌ์ฉํ์ฌ ํ
์คํธ๋ฅผ ์คํํ ์๋ ์์ต๋๋ค.
`adam`์ด๋ผ๋ ์ด๋ฆ์ ํฌํจํ๋ ํ
์คํธ๋ง ์คํํ๋ ค๋ฉด ๋ค์๊ณผ ๊ฐ์ต๋๋ค:
```bash
pytest -k adam tests/test_optimization.py
```
๋
ผ๋ฆฌ ์ฐ์ฐ์ `and`์ `or`๋ฅผ ์ฌ์ฉํ์ฌ ๋ชจ๋ ํค์๋๊ฐ ์ผ์นํด์ผ ํ๋์ง ๋๋ ์ด๋ ํ๋๊ฐ ์ผ์นํด์ผ ํ๋์ง๋ฅผ ๋ํ๋ผ ์ ์์ต๋๋ค.
`not`์ ๋ถ์ ํ ๋ ์ฌ์ฉํ ์ ์์ต๋๋ค.
`adam`์ด๋ผ๋ ์ด๋ฆ์ ํฌํจํ์ง ์๋ ๋ชจ๋ ํ
์คํธ๋ฅผ ์คํํ๋ ค๋ฉด ๋ค์๊ณผ ๊ฐ์ต๋๋ค:
```bash
pytest -k "not adam" tests/test_optimization.py
```
๋ ๊ฐ์ง ํจํด์ ํ๋๋ก ๊ฒฐํฉํ ์๋ ์์ต๋๋ค:
```bash
pytest -k "ada and not adam" tests/test_optimization.py
```
์๋ฅผ ๋ค์ด `test_adafactor`์ `test_adam_w`๋ฅผ ๋ชจ๋ ์คํํ๋ ค๋ฉด ๋ค์์ ์ฌ์ฉํ ์ ์์ต๋๋ค:
```bash
pytest -k "test_adam_w or test_adam_w" tests/test_optimization.py
```
์ฌ๊ธฐ์ `or`๋ฅผ ์ฌ์ฉํ๋ ๊ฒ์ ์ ์ํ์ธ์. ๋ ํค์๋ ์ค ํ๋๊ฐ ์ผ์นํ๋๋ก ํ๊ธฐ ์ํ ๋ชฉ์ ์ผ๋ก ์ฌ์ฉํ๊ธฐ ๋๋ฌธ์
๋๋ค.
๋ ํจํด์ด ๋ชจ๋ ํฌํจ๋์ด์ผ ํ๋ ํ
์คํธ๋ง ์คํํ๋ ค๋ฉด, `and`๋ฅผ ์ฌ์ฉํด์ผ ํฉ๋๋ค:
```bash
pytest -k "test and ada" tests/test_optimization.py
```
### `accelerate` ํ
์คํธ ์คํ[[run-`accelerate`-tests]]
๋ชจ๋ธ์์ `accelerate` ํ
์คํธ๋ฅผ ์คํํด์ผ ํ ๋๊ฐ ์์ต๋๋ค. ์ด๋ฅผ ์ํด์๋ ๋ช
๋ น์ด์ `-m accelerate_tests`๋ฅผ ์ถ๊ฐํ๋ฉด ๋ฉ๋๋ค.
์๋ฅผ ๋ค์ด, `OPT`์์ ์ด๋ฌํ ํ
์คํธ๋ฅผ ์คํํ๋ ค๋ฉด ๋ค์๊ณผ ๊ฐ์ต๋๋ค:
```bash
RUN_SLOW=1 pytest -m accelerate_tests tests/models/opt/test_modeling_opt.py
```
### ๋ฌธ์ ํ
์คํธ ์คํ[[run-documentation-tests]]
์์ ๋ฌธ์๊ฐ ์ฌ๋ฐ๋ฅธ์ง ํ
์คํธํ๋ ค๋ฉด `doctests`๊ฐ ํต๊ณผํ๋์ง ํ์ธํด์ผ ํฉ๋๋ค.
์๋ฅผ ๋ค์ด, [`WhisperModel.forward`'s docstring](https://github.com/huggingface/transformers/blob/main/src/transformers/models/whisper/modeling_whisper.py#L1017-L1035)๋ฅผ ์ฌ์ฉํด ๋ด
์๋ค:
```python
r"""
Returns:
Example:
```python
>>> import torch
>>> from transformers import WhisperModel, WhisperFeatureExtractor
>>> from datasets import load_dataset
>>> model = WhisperModel.from_pretrained("openai/whisper-base")
>>> feature_extractor = WhisperFeatureExtractor.from_pretrained("openai/whisper-base")
>>> ds = load_dataset("hf-internal-testing/librispeech_asr_dummy", "clean", split="validation")
>>> inputs = feature_extractor(ds[0]["audio"]["array"], return_tensors="pt")
>>> input_features = inputs.input_features
>>> decoder_input_ids = torch.tensor([[1, 1]]) * model.config.decoder_start_token_id
>>> last_hidden_state = model(input_features, decoder_input_ids=decoder_input_ids).last_hidden_state
>>> list(last_hidden_state.shape)
[1, 2, 512]
```"""
```
์ํ๋ ํ์ผ์ ๋ชจ๋ docstring ์์ ๋ฅผ ์๋์ผ๋ก ํ
์คํธํ๋ ค๋ฉด ๋ค์ ๋ช
๋ น์ ์คํํ๋ฉด ๋ฉ๋๋ค:
```bash
pytest --doctest-modules <path_to_file_or_dir>
```
ํ์ผ์ ํ์ฅ์๊ฐ markdown์ธ ๊ฒฝ์ฐ `--doctest-glob="*.md"` ์ธ์๋ฅผ ์ถ๊ฐํด์ผ ํฉ๋๋ค.
### ์์ ๋ ํ
์คํธ๋ง ์คํ[[run-only-modified-tests]]
์์ ๋ ํ์ผ ๋๋ ํ์ฌ ๋ธ๋์น (Git ๊ธฐ์ค)์ ๊ด๋ จ๋ ํ
์คํธ๋ฅผ ์คํํ๋ ค๋ฉด [pytest-picked](https://github.com/anapaulagomes/pytest-picked)์ ์ฌ์ฉํ ์ ์์ต๋๋ค.
์ด๋ ๋ณ๊ฒฝํ ๋ด์ฉ์ด ํ
์คํธ์ ์ํฅ์ ์ฃผ์ง ์์๋์ง ๋น ๋ฅด๊ฒ ํ์ธํ ์ ์๋ ์ข์ ๋ฐฉ๋ฒ์
๋๋ค.
```bash
pip install pytest-picked
```
```bash
pytest --picked
```
์์ ๋์์ง๋ง, ์์ง ์ปค๋ฐ๋์ง ์์ ๋ชจ๋ ํ์ผ ๋ฐ ํด๋์์ ํ
์คํธ๊ฐ ์คํ๋ฉ๋๋ค.
### ์์ค ์์ ์ ์คํจํ ํ
์คํธ ์๋ ์ฌ์คํ[[automatically-rerun-failed-tests-on-source-modification]]
[pytest-xdist](https://github.com/pytest-dev/pytest-xdist)๋ ๋ชจ๋ ์คํจํ ํ
์คํธ๋ฅผ ๊ฐ์งํ๊ณ ,
ํ์ผ์ ์์ ํ ํ์ ํ์ผ์ ๊ณ์ ์ฌ์คํํ์ฌ ํ
์คํธ๊ฐ ์ฑ๊ณตํ ๋๊น์ง ๊ธฐ๋ค๋ฆฌ๋ ๋งค์ฐ ์ ์ฉํ ๊ธฐ๋ฅ์ ์ ๊ณตํฉ๋๋ค.
๋ฐ๋ผ์ ์์ ํ ๋ด์ฉ์ ํ์ธํ ํ pytest๋ฅผ ๋ค์ ์์ํ ํ์๊ฐ ์์ต๋๋ค.
๋ชจ๋ ํ
์คํธ๊ฐ ํต๊ณผ๋ ๋๊น์ง ์ด ๊ณผ์ ์ ๋ฐ๋ณตํ ํ ๋ค์ ์ ์ฒด ์คํ์ด ์ด๋ฃจ์ด์ง๋๋ค.
```bash
pip install pytest-xdist
```
์ฌ๊ท์ ๋ชจ๋์ ์ฌ์ฉ: `pytest -f` ๋๋ `pytest --looponfail`
ํ์ผ์ ๋ณ๊ฒฝ ์ฌํญ์ `looponfailroots` ๋ฃจํธ ๋๋ ํฐ๋ฆฌ์ ํด๋น ๋ด์ฉ์ (์ฌ๊ท์ ์ผ๋ก) ํ์ธํ์ฌ ๊ฐ์ง๋ฉ๋๋ค.
์ด ๊ฐ์ ๊ธฐ๋ณธ๊ฐ์ด ์๋ํ์ง ์๋ ๊ฒฝ์ฐ,
`setup.cfg`์ ์ค์ ์ต์
์ ๋ณ๊ฒฝํ์ฌ ํ๋ก์ ํธ์์ ๋ณ๊ฒฝํ ์ ์์ต๋๋ค:
```ini
[tool:pytest]
looponfailroots = transformers tests
```
๋๋ `pytest.ini`/``tox.ini`` ํ์ผ:
```ini
[pytest]
looponfailroots = transformers tests
```
์ด๋ ๊ฒ ํ๋ฉด ini-file์ ๋๋ ํฐ๋ฆฌ๋ฅผ ๊ธฐ์ค์ผ๋ก ์๋์ ์ผ๋ก ์ง์ ๋ ๊ฐ ๋๋ ํฐ๋ฆฌ์์ ํ์ผ ๋ณ๊ฒฝ ์ฌํญ๋ง ์ฐพ๊ฒ ๋ฉ๋๋ค.
์ด ๊ธฐ๋ฅ์ ๋์ฒดํ ์ ์๋ ๊ตฌํ ๋ฐฉ๋ฒ์ธ [pytest-watch](https://github.com/joeyespo/pytest-watch)๋ ์์ต๋๋ค.
### ํน์ ํ
์คํธ ๋ชจ๋ ๊ฑด๋๋ฐ๊ธฐ[[skip-a-test-module]]
๋ชจ๋ ํ
์คํธ ๋ชจ๋์ ์คํํ๋ ํน์ ๋ชจ๋์ ์ ์ธํ๋ ค๋ฉด, ์คํํ ํ
์คํธ ๋ชฉ๋ก์ ๋ช
์์ ์ผ๋ก ์ง์ ํ ์ ์์ต๋๋ค.
์๋ฅผ ๋ค์ด, `test_modeling_*.py` ํ
์คํธ๋ฅผ ์ ์ธํ ๋ชจ๋ ํ
์คํธ๋ฅผ ์คํํ๋ ค๋ฉด ๋ค์์ ์ฌ์ฉํ ์ ์์ต๋๋ค:
```bash
pytest *ls -1 tests/*py | grep -v test_modeling*
```
### ์ํ ์ด๊ธฐํ[[clearing state]]
CI ๋น๋ ๋ฐ (์๋์ ๋ํ) ๊ฒฉ๋ฆฌ๊ฐ ์ค์ํ ๊ฒฝ์ฐ, ์บ์๋ฅผ ์ง์์ผ ํฉ๋๋ค:
```bash
pytest --cache-clear tests
```
### ํ
์คํธ๋ฅผ ๋ณ๋ ฌ๋ก ์คํ[[running-tests-in-parallel]]
์ด์ ์ ์ธ๊ธํ ๊ฒ์ฒ๋ผ `make test`๋ ํ
์คํธ๋ฅผ ๋ณ๋ ฌ๋ก ์คํํ๊ธฐ ์ํด
`pytest-xdist` ํ๋ฌ๊ทธ์ธ(`-n X` ์ธ์, ์๋ฅผ ๋ค์ด `-n 2`๋ฅผ ์ฌ์ฉํ์ฌ 2๊ฐ์ ๋ณ๋ ฌ ์์
์คํ)์ ํตํด ์คํ๋ฉ๋๋ค.
`pytest-xdist`์ `--dist=` ์ต์
์ ์ฌ์ฉํ์ฌ ํ
์คํธ๋ฅผ ์ด๋ป๊ฒ ๊ทธ๋ฃนํํ ์ง ์ ์ดํ ์ ์์ต๋๋ค.
`--dist=loadfile`์ ํ๋์ ํ์ผ์ ์๋ ํ
์คํธ๋ฅผ ๋์ผํ ํ๋ก์ธ์ค๋ก ๊ทธ๋ฃนํํฉ๋๋ค.
์คํ๋ ํ
์คํธ์ ์์๊ฐ ๋ค๋ฅด๊ณ ์์ธกํ ์ ์๊ธฐ ๋๋ฌธ์, `pytest-xdist`๋ก ํ
์คํธ ์ค์ํธ๋ฅผ ์คํํ๋ฉด ์คํจ๊ฐ ๋ฐ์ํ ์ ์์ต๋๋ค (๊ฒ์ถ๋์ง ์์ ๊ฒฐํฉ๋ ํ
์คํธ๊ฐ ์๋ ๊ฒฝ์ฐ).
์ด ๊ฒฝ์ฐ [pytest-replay](https://github.com/ESSS/pytest-replay)๋ฅผ ์ฌ์ฉํ๋ฉด ๋์ผํ ์์๋ก ํ
์คํธ๋ฅผ ๋ค์ ์คํํด์
์คํจํ๋ ์ํ์ค๋ฅผ ์ต์ํํ๋ ๋ฐ์ ๋์์ด ๋ฉ๋๋ค.
### ํ
์คํธ ์์์ ๋ฐ๋ณต[[test-order-and-repetition]]
์ ์ฌ์ ์ธ ์ข
์์ฑ ๋ฐ ์ํ ๊ด๋ จ ๋ฒ๊ทธ(tear down)๋ฅผ ๊ฐ์งํ๊ธฐ ์ํด
ํ
์คํธ๋ฅผ ์ฌ๋ฌ ๋ฒ, ์ฐ์์ผ๋ก, ๋ฌด์์๋ก ๋๋ ์ธํธ๋ก ๋ฐ๋ณตํ๋ ๊ฒ์ด ์ข์ต๋๋ค.
๊ทธ๋ฆฌ๊ณ ์ง์ ์ ์ธ ์ฌ๋ฌ ๋ฒ์ ๋ฐ๋ณต์ DL์ ๋ฌด์์์ฑ์ ์ํด ๋ฐ๊ฒฌ๋๋ ์ผ๋ถ ๋ฌธ์ ๋ฅผ ๊ฐ์งํ๋ ๋ฐ์๋ ์ ์ฉํฉ๋๋ค.
#### ํ
์คํธ๋ฅผ ๋ฐ๋ณต[[repeat-tests]]
- [pytest-flakefinder](https://github.com/dropbox/pytest-flakefinder):
```bash
pip install pytest-flakefinder
```
๋ชจ๋ ํ
์คํธ๋ฅผ ์ฌ๋ฌ ๋ฒ ์คํํฉ๋๋ค(๊ธฐ๋ณธ๊ฐ์ 50๋ฒ):
```bash
pytest --flake-finder --flake-runs=5 tests/test_failing_test.py
```
<Tip>
์ด ํ๋ฌ๊ทธ์ธ์ `pytest-xdist`์ `-n` ํ๋๊ทธ์ ํจ๊ป ์๋ํ์ง ์์ต๋๋ค.
</Tip>
<Tip>
`pytest-repeat`๋ผ๋ ๋ ๋ค๋ฅธ ํ๋ฌ๊ทธ์ธ๋ ์์ง๋ง `unittest`์ ํจ๊ป ์๋ํ์ง ์์ต๋๋ค.
</Tip>
#### ํ
์คํธ๋ฅผ ์์์ ์์๋ก ์คํ[[run-tests-in-a-random-order]]
```bash
pip install pytest-random-order
```
์ค์: `pytest-random-order`๊ฐ ์ค์น๋๋ฉด ํ
์คํธ๊ฐ ์๋์ผ๋ก ์์์ ์์๋ก ์์
๋๋ค.
๊ตฌ์ฑ ๋ณ๊ฒฝ์ด๋ ์ปค๋งจ๋ ๋ผ์ธ ์ต์
์ด ํ์ํ์ง ์์ต๋๋ค.
์์ ์ค๋ช
ํ ๊ฒ์ฒ๋ผ ์ด๋ฅผ ํตํด ํ ํ
์คํธ์ ์ํ๊ฐ ๋ค๋ฅธ ํ
์คํธ์ ์ํ์ ์ํฅ์ ๋ฏธ์น๋ ๊ฒฐํฉ๋ ํ
์คํธ๋ฅผ ๊ฐ์งํ ์ ์์ต๋๋ค.
`pytest-random-order`๊ฐ ์ค์น๋๋ฉด ํด๋น ์ธ์
์์ ์ฌ์ฉ๋ ๋๋ค ์๋๊ฐ ์ถ๋ ฅ๋๋ฉฐ ์๋ฅผ ๋ค์ด ๋ค์๊ณผ ๊ฐ์ต๋๋ค:
```bash
pytest tests
[...]
Using --random-order-bucket=module
Using --random-order-seed=573663
```
๋ฐ๋ผ์ ํน์ ์ํ์ค๊ฐ ์คํจํ๋ ๊ฒฝ์ฐ์๋ ์ ํํ ์๋๋ฅผ ์ถ๊ฐํ์ฌ ์ฌํํ ์ ์์ต๋๋ค. ์๋ฅผ ๋ค์ด ๋ค์๊ณผ ๊ฐ์ต๋๋ค:
```bash
pytest --random-order-seed=573663
[...]
Using --random-order-bucket=module
Using --random-order-seed=573663
```
์ ํํ ๋์ผํ ํ
์คํธ ๋ชฉ๋ก(๋๋ ๋ชฉ๋ก์ด ์์)์ ์ฌ์ฉํ๋ ๊ฒฝ์ฐ์๋ง ์ ํํ ์์๋ฅผ ์ฌํํฉ๋๋ค.
๋ชฉ๋ก์ ์๋์ผ๋ก ์ขํ๊ธฐ ์์ํ๋ฉด ๋ ์ด์ ์๋์ ์์กดํ ์ ์๊ณ ์คํจํ๋ ์ ํํ ์์๋ก ์๋์ผ๋ก ๋ชฉ๋ก์ ๋์ดํด์ผํฉ๋๋ค. ๊ทธ๋ฆฌ๊ณ `--random-order-bucket=none`์ ์ฌ์ฉํ์ฌ pytest์๊ฒ ์์๋ฅผ ์์๋ก ์ค์ ํ์ง ์๋๋ก ์๋ ค์ผ ํฉ๋๋ค.
์๋ฅผ ๋ค์ด ๋ค์๊ณผ ๊ฐ์ต๋๋ค:
```bash
pytest --random-order-bucket=none tests/test_a.py tests/test_c.py tests/test_b.py
```
๋ชจ๋ ํ
์คํธ์ ๋ํด ์๊ธฐ๋ฅผ ๋นํ์ฑํํ๋ ค๋ฉด ๋ค์๊ณผ ๊ฐ์ต๋๋ค:
```bash
pytest --random-order-bucket=none
```
๊ธฐ๋ณธ์ ์ผ๋ก `--random-order-bucket=module`์ด ๋ด์ฌ๋์ด ์์ผ๋ฏ๋ก, ๋ชจ๋ ์์ค์์ ํ์ผ์ ์์ต๋๋ค.
๋ํ `class`, `package`, `global` ๋ฐ `none` ์์ค์์๋ ์์ ์ ์์ต๋๋ค.
์์ธํ ๋ด์ฉ์ ํด๋น [๋ฌธ์](https://github.com/jbasko/pytest-random-order)๋ฅผ ์ฐธ์กฐํ์ธ์.
๋ ๋ค๋ฅธ ๋ฌด์์ํ์ ๋์์ [`pytest-randomly`](https://github.com/pytest-dev/pytest-randomly)์
๋๋ค.
์ด ๋ชจ๋์ ๋งค์ฐ ์ ์ฌํ ๊ธฐ๋ฅ/์ธํฐํ์ด์ค๋ฅผ ๊ฐ์ง๊ณ ์์ง๋ง, `pytest-random-order`์ ์๋ ๋ฒํท ๋ชจ๋๋ฅผ ์ฌ์ฉํ ์๋ ์์ต๋๋ค.
์ค์น ํ์๋ ์๋์ผ๋ก ์ ์ฉ๋๋ ๋ฌธ์ ๋ ๋์ผํ๊ฒ ๊ฐ์ง๋๋ค.
### ์ธ๊ด๊ณผ ๋๋์ ๋ณ๊ฒฝ[[look-and-feel-variations]
#### pytest-sugar ์ฌ์ฉ[[pytest-sugar]]
[pytest-sugar](https://github.com/Frozenball/pytest-sugar)๋ ํ
์คํธ๊ฐ ๋ณด์ฌ์ง๋ ํํ๋ฅผ ๊ฐ์ ํ๊ณ ,
์งํ ์ํฉ ๋ฐ๋ฅผ ์ถ๊ฐํ๋ฉฐ, ์คํจํ ํ
์คํธ์ ๊ฒ์ฆ์ ์ฆ์ ํ์ํ๋ ํ๋ฌ๊ทธ์ธ์
๋๋ค. ์ค์นํ๋ฉด ์๋์ผ๋ก ํ์ฑํ๋ฉ๋๋ค.
```bash
pip install pytest-sugar
```
pytest-sugar ์์ด ํ
์คํธ๋ฅผ ์คํํ๋ ค๋ฉด ๋ค์๊ณผ ๊ฐ์ต๋๋ค:
```bash
pytest -p no:sugar
```
๋๋ ์ ๊ฑฐํ์ธ์.
#### ๊ฐ ํ์ ํ
์คํธ ์ด๋ฆ๊ณผ ์งํ ์ํฉ ๋ณด๊ณ [[report-each-sub-test-name-and-its-progress]]
`pytest`๋ฅผ ํตํด ๋จ์ผ ๋๋ ๊ทธ๋ฃน์ ํ
์คํธ๋ฅผ ์คํํ๋ ๊ฒฝ์ฐ(`pip install pytest-pspec` ์ดํ):
```bash
pytest --pspec tests/test_optimization.py
```
#### ์คํจํ ํ
์คํธ ์ฆ์ ํ์[[instantly-shows-failed-tests]]
[pytest-instafail](https://github.com/pytest-dev/pytest-instafail)์ ํ
์คํธ ์ธ์
์ ๋๊น์ง ๊ธฐ๋ค๋ฆฌ์ง ์๊ณ
์คํจ ๋ฐ ์ค๋ฅ๋ฅผ ์ฆ์ ํ์ํฉ๋๋ค.
```bash
pip install pytest-instafail
```
```bash
pytest --instafail
```
### GPU ์ฌ์ฉ ์ฌ๋ถ[[to-GPU-or-not-to-GPU]]
GPU๊ฐ ํ์ฑํ๋ ํ๊ฒฝ์์, CPU ์ ์ฉ ๋ชจ๋๋ก ํ
์คํธํ๋ ค๋ฉด `CUDA_VISIBLE_DEVICES=""`๋ฅผ ์ถ๊ฐํฉ๋๋ค:
```bash
CUDA_VISIBLE_DEVICES="" pytest tests/test_logging.py
```
๋๋ ๋ค์ค GPU๊ฐ ์๋ ๊ฒฝ์ฐ `pytest`์์ ์ฌ์ฉํ GPU๋ฅผ ์ง์ ํ ์๋ ์์ต๋๋ค.
์๋ฅผ ๋ค์ด, GPU `0` ๋ฐ `1`์ด ์๋ ๊ฒฝ์ฐ ๋ค์์ ์คํํ ์ ์์ต๋๋ค:
```bash
CUDA_VISIBLE_DEVICES="1" pytest tests/test_logging.py
```
์ด๋ ๊ฒ ํ๋ฉด ๋ค๋ฅธ GPU์์ ๋ค๋ฅธ ์์
์ ์คํํ๋ ค๋ ๊ฒฝ์ฐ ์ ์ฉํฉ๋๋ค.
์ผ๋ถ ํ
์คํธ๋ ๋ฐ๋์ CPU ์ ์ฉ์ผ๋ก ์คํํด์ผ ํ๋ฉฐ, ์ผ๋ถ๋ CPU ๋๋ GPU ๋๋ TPU์์ ์คํํด์ผ ํ๊ณ , ์ผ๋ถ๋ ์ฌ๋ฌ GPU์์ ์คํํด์ผ ํฉ๋๋ค.
๋ค์ ์คํต ๋ฐ์ฝ๋ ์ดํฐ๋ ํ
์คํธ์ ์๊ตฌ ์ฌํญ์ CPU/GPU/TPU๋ณ๋ก ์ค์ ํ๋ ๋ฐ ์ฌ์ฉ๋ฉ๋๋ค:
- `require_torch` - ์ด ํ
์คํธ๋ torch์์๋ง ์คํ๋ฉ๋๋ค.
- `require_torch_gpu` - `require_torch`์ ์ถ๊ฐ๋ก ์ ์ด๋ 1๊ฐ์ GPU๊ฐ ํ์ํฉ๋๋ค.
- `require_torch_multi_gpu` - `require_torch`์ ์ถ๊ฐ๋ก ์ ์ด๋ 2๊ฐ์ GPU๊ฐ ํ์ํฉ๋๋ค.
- `require_torch_non_multi_gpu` - `require_torch`์ ์ถ๊ฐ๋ก 0๊ฐ ๋๋ 1๊ฐ์ GPU๊ฐ ํ์ํฉ๋๋ค.
- `require_torch_up_to_2_gpus` - `require_torch`์ ์ถ๊ฐ๋ก 0๊ฐ, 1๊ฐ ๋๋ 2๊ฐ์ GPU๊ฐ ํ์ํฉ๋๋ค.
- `require_torch_tpu` - `require_torch`์ ์ถ๊ฐ๋ก ์ ์ด๋ 1๊ฐ์ TPU๊ฐ ํ์ํฉ๋๋ค.
GPU ์๊ตฌ ์ฌํญ์ ํ๋ก ์ ๋ฆฌํ๋ฉด ์๋์ ๊ฐ์ต๋๋ใ
:
| n gpus | decorator |
|--------+--------------------------------|
| `>= 0` | `@require_torch` |
| `>= 1` | `@require_torch_gpu` |
| `>= 2` | `@require_torch_multi_gpu` |
| `< 2` | `@require_torch_non_multi_gpu` |
| `< 3` | `@require_torch_up_to_2_gpus` |
์๋ฅผ ๋ค์ด, 2๊ฐ ์ด์์ GPU๊ฐ ์๊ณ pytorch๊ฐ ์ค์น๋์ด ์์ ๋์๋ง ์คํ๋์ด์ผ ํ๋ ํ
์คํธ๋ ๋ค์๊ณผ ๊ฐ์ต๋๋ค:
```python no-style
@require_torch_multi_gpu
def test_example_with_multi_gpu():
```
`tensorflow`๊ฐ ํ์ํ ๊ฒฝ์ฐ `require_tf` ๋ฐ์ฝ๋ ์ดํฐ๋ฅผ ์ฌ์ฉํฉ๋๋ค. ์๋ฅผ ๋ค์ด ๋ค์๊ณผ ๊ฐ์ต๋๋ค:
```python no-style
@require_tf
def test_tf_thing_with_tensorflow():
```
์ด๋ฌํ ๋ฐ์ฝ๋ ์ดํฐ๋ ์ค์ฒฉ๋ ์ ์์ต๋๋ค.
์๋ฅผ ๋ค์ด, ๋๋ฆฐ ํ
์คํธ๋ก ์งํ๋๊ณ pytorch์์ ์ ์ด๋ ํ๋์ GPU๊ฐ ํ์ํ ๊ฒฝ์ฐ ๋ค์๊ณผ ๊ฐ์ด ์ค์ ํ ์ ์์ต๋๋ค:
```python no-style
@require_torch_gpu
@slow
def test_example_slow_on_gpu():
```
`@parametrized`์ ๊ฐ์ ์ผ๋ถ ๋ฐ์ฝ๋ ์ดํฐ๋ ํ
์คํธ ์ด๋ฆ์ ๋ค์ ์์ฑํ๊ธฐ ๋๋ฌธ์ `@require_*` ์คํต ๋ฐ์ฝ๋ ์ดํฐ๋ ์ฌ๋ฐ๋ฅด๊ฒ ์๋ํ๋ ค๋ฉด ํญ์ ๋งจ ๋ง์ง๋ง์ ๋์ด๋์ด์ผ ํฉ๋๋ค.
๋ค์์ ์ฌ๋ฐ๋ฅธ ์ฌ์ฉ ์์
๋๋ค:
```python no-style
@parameterized.expand(...)
@require_torch_multi_gpu
def test_integration_foo():
```
`@pytest.mark.parametrize`์๋ ์ด๋ฌํ ์์ ๋ฌธ์ ๋ ์์ผ๋ฏ๋ก ์ฒ์ ํน์ ๋ง์ง๋ง์ ์์น์ํฌ ์ ์๊ณ ์ด๋ฌํ ๊ฒฝ์ฐ์๋ ์ ์๋ํ ๊ฒ์
๋๋ค.
ํ์ง๋ง unittest๊ฐ ์๋ ๊ฒฝ์ฐ์๋ง ์๋ํฉ๋๋ค.
ํ
์คํธ ๋ด๋ถ์์ ๋ค์์ ์ฌ์ฉํ ์ ์์ต๋๋ค:
- ์ฌ์ฉ ๊ฐ๋ฅํ GPU ์:
```python
from transformers.testing_utils import get_gpu_count
n_gpu = get_gpu_count() #torch์ tf์ ํจ๊ป ์๋
```
### ๋ถ์ฐ ํ๋ จ[[distributed-training]]
`pytest`๋ ๋ถ์ฐ ํ๋ จ์ ์ง์ ์ ์ผ๋ก ๋ค๋ฃจ์ง ๋ชปํฉ๋๋ค.
์ด๋ฅผ ์๋ํ๋ฉด ํ์ ํ๋ก์ธ์ค๊ฐ ์ฌ๋ฐ๋ฅธ ์์
์ ์ํํ์ง ์๊ณ `pytest`๋ผ๊ณ ์๊ฐํ๊ธฐ์ ํ
์คํธ ์ค์ํธ๋ฅผ ๋ฐ๋ณตํด์ ์คํํ๊ฒ ๋ฉ๋๋ค.
๊ทธ๋ฌ๋ ์ผ๋ฐ ํ๋ก์ธ์ค๋ฅผ ์์ฑํ ๋ค์ ์ฌ๋ฌ ์์ปค๋ฅผ ์์ฑํ๊ณ IO ํ์ดํ๋ฅผ ๊ด๋ฆฌํ๋๋ก ํ๋ฉด ๋์ํฉ๋๋ค.
๋ค์์ ์ฌ์ฉ ๊ฐ๋ฅํ ํ
์คํธ์
๋๋ค:
- [test_trainer_distributed.py](https://github.com/huggingface/transformers/tree/main/tests/trainer/test_trainer_distributed.py)
- [test_deepspeed.py](https://github.com/huggingface/transformers/tree/main/tests/deepspeed/test_deepspeed.py)
์คํ ์ง์ ์ผ๋ก ๋ฐ๋ก ์ด๋ํ๋ ค๋ฉด, ํด๋น ํ
์คํธ์์ `execute_subprocess_async` ํธ์ถ์ ๊ฒ์ํ์ธ์.
์ด๋ฌํ ํ
์คํธ๋ฅผ ์คํํ๋ ค๋ฉด ์ ์ด๋ 2๊ฐ์ GPU๊ฐ ํ์ํฉ๋๋ค.
```bash
CUDA_VISIBLE_DEVICES=0,1 RUN_SLOW=1 pytest -sv tests/test_trainer_distributed.py
```
### ์ถ๋ ฅ ์บก์ฒ[[output-capture]]
ํ
์คํธ ์คํ ์ค `stdout` ๋ฐ `stderr`๋ก ์ ์ก๋ ๋ชจ๋ ์ถ๋ ฅ์ด ์บก์ฒ๋ฉ๋๋ค.
ํ
์คํธ๋ ์ค์ ๋ฉ์๋๊ฐ ์คํจํ๋ฉด ์บก์ฒ๋ ์ถ๋ ฅ์ ์ผ๋ฐ์ ์ผ๋ก ์คํจ ์ถ์ ์ ๋ณด์ ํจ๊ป ํ์๋ฉ๋๋ค.
์ถ๋ ฅ ์บก์ฒ๋ฅผ ๋นํ์ฑํํ๊ณ `stdout` ๋ฐ `stderr`๋ฅผ ์ ์์ ์ผ๋ก ๋ฐ์ผ๋ ค๋ฉด `-s` ๋๋ `--capture=no`๋ฅผ ์ฌ์ฉํ์ธ์:
```bash
pytest -s tests/test_logging.py
```
ํ
์คํธ ๊ฒฐ๊ณผ๋ฅผ JUnit ํ์์ ์ถ๋ ฅ์ผ๋ก ๋ณด๋ด๋ ค๋ฉด ๋ค์์ ์ฌ์ฉํ์ธ์:
```bash
py.test tests --junitxml=result.xml
```
### ์์ ์กฐ์ [[color-control]]
์์์ด ์๊ฒ ํ๋ ค๋ฉด ๋ค์๊ณผ ๊ฐ์ด ์ค์ ํ์ธ์(์๋ฅผ ๋ค์ด ํฐ์ ๋ฐฐ๊ฒฝ์ ๋
ธ๋์ ๊ธ์จ๋ ๊ฐ๋
์ฑ์ด ์ข์ง ์์ต๋๋ค):
```bash
pytest --color=no tests/test_logging.py
```
### online pastebin service์ ํ
์คํธ ๋ณด๊ณ ์ ์ ์ก[[sending test report to online pastebin service]]
๊ฐ ํ
์คํธ ์คํจ์ ๋ํ URL์ ๋ง๋ญ๋๋ค:
```bash
pytest --pastebin=failed tests/test_logging.py
```
์ด๋ ๊ฒ ํ๋ฉด ๊ฐ ์คํจ์ ๋ํ URL์ ์ ๊ณตํ๋ remote Paste service์ ํ
์คํธ ์คํ ์ ๋ณด๋ฅผ ์ ์ถํฉ๋๋ค.
์ผ๋ฐ์ ์ธ ํ
์คํธ๋ฅผ ์ ํํ ์๋ ์๊ณ ํน์ ํน์ ์คํจ๋ง ๋ณด๋ด๋ ค๋ฉด `-x`์ ๊ฐ์ด ์ถ๊ฐํ ์๋ ์์ต๋๋ค.
์ ์ฒด ํ
์คํธ ์ธ์
๋ก๊ทธ์ ๋ํ URL์ ์์ฑํฉ๋๋ค:
```bash
pytest --pastebin=all tests/test_logging.py
```
## ํ
์คํธ ์์ฑ[[writing-tests]]
๐ค transformers ํ
์คํธ๋ ๋๋ถ๋ถ `unittest`๋ฅผ ๊ธฐ๋ฐ์ผ๋ก ํ์ง๋ง,
`pytest`์์ ์คํ๋๋ฏ๋ก ๋๋ถ๋ถ์ ๊ฒฝ์ฐ ๋ ์์คํ
์ ๊ธฐ๋ฅ์ ์ฌ์ฉํ ์ ์์ต๋๋ค.
์ง์๋๋ ๊ธฐ๋ฅ์ ๋ํด [์ฌ๊ธฐ](https://docs.pytest.org/en/stable/unittest.html)์์ ํ์ธํ ์ ์์ง๋ง,
๊ธฐ์ตํด์ผ ํ ์ค์ํ ์ ์ ๋๋ถ๋ถ์ `pytest` fixture๊ฐ ์๋ํ์ง ์๋๋ค๋ ๊ฒ์
๋๋ค.
ํ๋ผ๋ฏธํฐํ๋ ์๋ํ์ง ์์ง๋ง, ์ฐ๋ฆฌ๋ ๋น์ทํ ๋ฐฉ์์ผ๋ก ์๋ํ๋ `parameterized` ๋ชจ๋์ ์ฌ์ฉํฉ๋๋ค.
### ๋งค๊ฐ๋ณ์ํ[[parametrization]]
๋์ผํ ํ
์คํธ๋ฅผ ๋ค๋ฅธ ์ธ์๋ก ์ฌ๋ฌ ๋ฒ ์คํํด์ผ ํ๋ ๊ฒฝ์ฐ๊ฐ ์ข
์ข
์์ต๋๋ค.
ํ
์คํธ ๋ด์์ ์ด ์์
์ ์ํํ ์ ์์ง๋ง, ๊ทธ๋ ๊ฒ ํ๋ฉด ํ๋์ ์ธ์ ์ธํธ์ ๋ํด ํ
์คํธ๋ฅผ ์คํํ ์ ์์ต๋๋ค.
```python
# test_this1.py
import unittest
from parameterized import parameterized
class TestMathUnitTest(unittest.TestCase):
@parameterized.expand(
[
("negative", -1.5, -2.0),
("integer", 1, 1.0),
("large fraction", 1.6, 1),
]
)
def test_floor(self, name, input, expected):
assert_equal(math.floor(input), expected)
```
์ด์ ๊ธฐ๋ณธ์ ์ผ๋ก ์ด ํ
์คํธ๋ `test_floor`์ ๋ง์ง๋ง 3๊ฐ ์ธ์๊ฐ
๋งค๊ฐ๋ณ์ ๋ชฉ๋ก์ ํด๋น ์ธ์์ ํ ๋น๋๋ ๊ฒ์ผ๋ก 3๋ฒ ์คํ๋ ๊ฒ์
๋๋ค.
๊ทธ๋ฆฌ๊ณ `negative` ๋ฐ `integer` ๋งค๊ฐ๋ณ์ ์งํฉ๋ง ์คํํ๋ ค๋ฉด ๋ค์๊ณผ ๊ฐ์ด ์คํํ ์ ์์ต๋๋ค:
```bash
pytest -k "negative and integer" tests/test_mytest.py
```
๋๋ `negative` ํ์ ํ
์คํธ๋ฅผ ์ ์ธํ ๋ชจ๋ ์๋ธ ํ
์คํธ๋ฅผ ๋ค์๊ณผ ๊ฐ์ด ์คํํ ์ ์์ต๋๋ค:
```bash
pytest -k "not negative" tests/test_mytest.py
```
์์์ ์ธ๊ธํ `-k` ํํฐ๋ฅผ ์ฌ์ฉํ๋ ๊ฒ ์ธ์๋,
๊ฐ ์๋ธ ํ
์คํธ์ ์ ํํ ์ด๋ฆ์ ํ์ธํ ํ์ ์ผ๋ถ ํน์ ์ ์ฒด ์๋ธ ํ
์คํธ๋ฅผ ์คํํ ์ ์์ต๋๋ค.
```bash
pytest test_this1.py --collect-only -q
```
๊ทธ๋ฆฌ๊ณ ๋ค์์ ๋ด์ฉ์ ํ์ธํ ์ ์์ ๊ฒ์
๋๋ค:
```bash
test_this1.py::TestMathUnitTest::test_floor_0_negative
test_this1.py::TestMathUnitTest::test_floor_1_integer
test_this1.py::TestMathUnitTest::test_floor_2_large_fraction
```
2๊ฐ์ ํน์ ํ ์๋ธ ํ
์คํธ๋ง ์คํํ ์๋ ์์ต๋๋ค:
```bash
pytest test_this1.py::TestMathUnitTest::test_floor_0_negative test_this1.py::TestMathUnitTest::test_floor_1_integer
```
`transformers`์ ๊ฐ๋ฐ์ ์ข
์์ฑ์ ์ด๋ฏธ ์๋ [parameterized](https://pypi.org/project/parameterized/) ๋ชจ๋์
`unittests`์ `pytest` ํ
์คํธ ๋ชจ๋์์ ์๋ํฉ๋๋ค.
๊ทธ๋ฌ๋ ํ
์คํธ๊ฐ `unittest`๊ฐ ์๋ ๊ฒฝ์ฐ `pytest.mark.parametrize`๋ฅผ ์ฌ์ฉํ ์ ์์ต๋๋ค(์ด๋ฏธ ์๋ ์ผ๋ถ ํ
์คํธ์์ ์ฌ์ฉ๋๋ ๊ฒฝ์ฐ๋ ์์ต๋๋ค.
์ฃผ๋ก `examples` ํ์์ ์์ต๋๋ค).
๋ค์์ `pytest`์ `parametrize` ๋ง์ปค๋ฅผ ์ฌ์ฉํ ๋์ผํ ์์
๋๋ค:
```python
# test_this2.py
import pytest
@pytest.mark.parametrize(
"name, input, expected",
[
("negative", -1.5, -2.0),
("integer", 1, 1.0),
("large fraction", 1.6, 1),
],
)
def test_floor(name, input, expected):
assert_equal(math.floor(input), expected)
```
`parameterized`์ ๋ง์ฐฌ๊ฐ์ง๋ก `pytest.mark.parametrize`๋ฅผ ์ฌ์ฉํ๋ฉด
`-k` ํํฐ๊ฐ ์๋ํ์ง ์๋ ๊ฒฝ์ฐ์๋ ์คํํ ์๋ธ ํ
์คํธ๋ฅผ ์ ํํ๊ฒ ์ง์ ํ ์ ์์ต๋๋ค.
๋จ, ์ด ๋งค๊ฐ๋ณ์ํ ํจ์๋ ์๋ธ ํ
์คํธ์ ์ด๋ฆ ์งํฉ์ ์ฝ๊ฐ ๋ค๋ฅด๊ฒ ์์ฑํฉ๋๋ค. ๋ค์๊ณผ ๊ฐ์ ๋ชจ์ต์
๋๋ค:
```bash
pytest test_this2.py --collect-only -q
```
๊ทธ๋ฆฌ๊ณ ๋ค์์ ๋ด์ฉ์ ํ์ธํ ์ ์์ ๊ฒ์
๋๋ค:
```bash
test_this2.py::test_floor[integer-1-1.0]
test_this2.py::test_floor[negative--1.5--2.0]
test_this2.py::test_floor[large fraction-1.6-1]
```
ํน์ ํ ํ
์คํธ์ ๋ํด์๋ง ์คํํ ์๋ ์์ต๋๋ค:
```bash
pytest test_this2.py::test_floor[negative--1.5--2.0] test_this2.py::test_floor[integer-1-1.0]
```
์ด์ ์ ์์์ ๊ฐ์ด ์คํํ ์ ์์ต๋๋ค.
### ํ์ผ ๋ฐ ๋๋ ํฐ๋ฆฌ[[files-and-directories]]
ํ
์คํธ์์ ์ข
์ข
ํ์ฌ ํ
์คํธ ํ์ผ๊ณผ ๊ด๋ จ๋ ์๋์ ์ธ ์์น๋ฅผ ์์์ผ ํ๋ ๊ฒฝ์ฐ๊ฐ ์์ต๋๋ค.
ํ
์คํธ๊ฐ ์ฌ๋ฌ ๋๋ ํฐ๋ฆฌ์์ ํธ์ถ๋๊ฑฐ๋ ๊น์ด๊ฐ ๋ค๋ฅธ ํ์ ๋๋ ํฐ๋ฆฌ์ ์์ ์ ์๊ธฐ ๋๋ฌธ์ ๊ทธ ์์น๋ฅผ ์๋ ๊ฒ์ ๊ฐ๋จํ์ง ์์ต๋๋ค.
`transformers.test_utils.TestCasePlus`๋ผ๋ ํฌํผ ํด๋์ค๋ ๋ชจ๋ ๊ธฐ๋ณธ ๊ฒฝ๋ก๋ฅผ ์ฒ๋ฆฌํ๊ณ ๊ฐ๋จํ ์ก์ธ์๋ฅผ ์ ๊ณตํ์ฌ ์ด ๋ฌธ์ ๋ฅผ ํด๊ฒฐํฉ๋๋ค:
- `pathlib` ๊ฐ์ฒด(์์ ํ ์ ํด์ง ๊ฒฝ๋ก)
- `test_file_path` - ํ์ฌ ํ
์คํธ ํ์ผ ๊ฒฝ๋ก (์: `__file__`)
- test_file_dir` - ํ์ฌ ํ
์คํธ ํ์ผ์ด ํฌํจ๋ ๋๋ ํฐ๋ฆฌ
- tests_dir` - `tests` ํ
์คํธ ์ค์ํธ์ ๋๋ ํฐ๋ฆฌ
- examples_dir` - `examples` ํ
์คํธ ์ค์ํธ์ ๋๋ ํฐ๋ฆฌ
- repo_root_dir` - ์ ์ฅ์ ๋๋ ํฐ๋ฆฌ
- src_dir` - `src`์ ๋๋ ํฐ๋ฆฌ(์: `transformers` ํ์ ๋๋ ํฐ๋ฆฌ๊ฐ ์๋ ๊ณณ)
- ๋ฌธ์์ด๋ก ๋ณํ๋ ๊ฒฝ๋ก---์์ ๋์ผํ์ง๋ง, `pathlib` ๊ฐ์ฒด๊ฐ ์๋ ๋ฌธ์์ด๋ก ๊ฒฝ๋ก๋ฅผ ๋ฐํํฉ๋๋ค:
- `test_file_path_str`
- `test_file_dir_str`
- `tests_dir_str`
- `examples_dir_str`
- `repo_root_dir_str`
- `src_dir_str`
์์ ๋ด์ฉ์ ์ฌ์ฉํ๋ ค๋ฉด ํ
์คํธ๊ฐ 'transformers.test_utils.TestCasePlus'์ ์๋ธํด๋์ค์ ์๋์ง ํ์ธํด์ผ ํฉ๋๋ค.
์๋ฅผ ๋ค์ด ๋ค์๊ณผ ๊ฐ์ต๋๋ค:
```python
from transformers.testing_utils import TestCasePlus
class PathExampleTest(TestCasePlus):
def test_something_involving_local_locations(self):
data_dir = self.tests_dir / "fixtures/tests_samples/wmt_en_ro"
```
๋ง์ฝ `pathlib`๋ฅผ ํตํด ๊ฒฝ๋ก๋ฅผ ์กฐ์ํ ํ์๊ฐ ์๊ฑฐ๋ ๊ฒฝ๋ก๋ฅผ ๋ฌธ์์ด๋ก๋ง ํ์๋ก ํ๋ ๊ฒฝ์ฐ์๋ `pathlib` ๊ฐ์ฒด์ `str()`์ ํธ์ถํ๊ฑฐ๋ `_str`๋ก ๋๋๋ ์ ๊ทผ์๋ฅผ ์ฌ์ฉํ ์ ์์ต๋๋ค.
์๋ฅผ ๋ค์ด ๋ค์๊ณผ ๊ฐ์ต๋๋ค:
```python
from transformers.testing_utils import TestCasePlus
class PathExampleTest(TestCasePlus):
def test_something_involving_stringified_locations(self):
examples_dir = self.examples_dir_str
```
### ์์ ํ์ผ ๋ฐ ๋๋ ํฐ๋ฆฌ[[temporary-files-and-directories]]
๊ณ ์ ํ ์์ ํ์ผ ๋ฐ ๋๋ ํฐ๋ฆฌ๋ฅผ ์ฌ์ฉํ๋ ๊ฒ์ ๋ณ๋ ฌ ํ
์คํธ ์คํ์ ์์ด ํ์์ ์
๋๋ค.
์ด๋ ๊ฒ ํจ์ผ๋ก์จ ํ
์คํธ๋ค์ด ์๋ก์ ๋ฐ์ดํฐ๋ฅผ ๋ฎ์ด์ฐ์ง ์๊ฒ ํ ์ ์์ต๋๋ค. ๋ํ ์ฐ๋ฆฌ๋ ์์ฑ๋ ํ
์คํธ์ ์ข
๋ฃ ๋จ๊ณ์์ ์ด๋ฌํ ์์ ํ์ผ ๋ฐ ๋๋ ํฐ๋ฆฌ๋ฅผ ์ ๊ฑฐํ๊ณ ์ถ์ต๋๋ค.
๋ฐ๋ผ์ ์ด๋ฌํ ์๊ตฌ ์ฌํญ์ ์ถฉ์กฑ์์ผ์ฃผ๋ `tempfile`๊ณผ ๊ฐ์ ํจํค์ง๋ฅผ ์ฌ์ฉํ๋ ๊ฒ์ด ์ค์ํฉ๋๋ค.
๊ทธ๋ฌ๋ ํ
์คํธ๋ฅผ ๋๋ฒ๊น
ํ ๋๋ ์์ ํ์ผ์ด๋ ๋๋ ํฐ๋ฆฌ์ ๋ค์ด๊ฐ๋ ๋ด์ฉ์ ํ์ธํ ์ ์์ด์ผ ํ๋ฉฐ,
์ฌ์คํ๋๋ ๊ฐ ํ
์คํธ๋ง๋ค ์์ ํ์ผ์ด๋ ๋๋ ํฐ๋ฆฌ์ ๊ฒฝ๋ก์ ๋ํด ๋ฌด์์ ๊ฐ์ด ์๋ ์ ํํ ๊ฐ์ ์๊ณ ์ถ์ ๊ฒ์
๋๋ค.
`transformers.test_utils.TestCasePlus`๋ผ๋ ๋์ฐ๋ฏธ ํด๋์ค๋ ์ด๋ฌํ ๋ชฉ์ ์ ๊ฐ์ฅ ์ ํฉํฉ๋๋ค.
์ด ํด๋์ค๋ `unittest.TestCase`์ ํ์ ํด๋์ค์ด๋ฏ๋ก, ์ฐ๋ฆฌ๋ ์ด๊ฒ์ ํ
์คํธ ๋ชจ๋์์ ์ฝ๊ฒ ์์ํ ์ ์์ต๋๋ค.
๋ค์์ ํด๋น ํด๋์ค๋ฅผ ์ฌ์ฉํ๋ ์์์
๋๋ค:
```python
from transformers.testing_utils import TestCasePlus
class ExamplesTests(TestCasePlus):
def test_whatever(self):
tmp_dir = self.get_auto_remove_tmp_dir()
```
์ด ์ฝ๋๋ ๊ณ ์ ํ ์์ ๋๋ ํฐ๋ฆฌ๋ฅผ ์์ฑํ๊ณ `tmp_dir`์ ํด๋น ์์น๋ก ์ค์ ํฉ๋๋ค.
- ๊ณ ์ ํ ์์ ๋๋ ํฐ๋ฆฌ๋ฅผ ์์ฑํฉ๋๋ค:
```python
def test_whatever(self):
tmp_dir = self.get_auto_remove_tmp_dir()
```
`tmp_dir`์๋ ์์ฑ๋ ์์ ๋๋ ํฐ๋ฆฌ์ ๊ฒฝ๋ก๊ฐ ํฌํจ๋ฉ๋๋ค.
์ด๋ ํ
์คํธ์ ์ข
๋ฃ ๋จ๊ณ์์ ์๋์ผ๋ก ์ ๊ฑฐ๋ฉ๋๋ค.
- ์ ํํ ๊ฒฝ๋ก๋ก ์์ ๋๋ ํฐ๋ฆฌ ์์ฑ ํ์ ํ
์คํธ ์์ ์ ์ ๋น์ด ์๋ ์ํ์ธ์ง ํ์ธํ๊ณ , ํ
์คํธ ํ์๋ ๋น์ฐ์ง ๋ง์ธ์.
```python
def test_whatever(self):
tmp_dir = self.get_auto_remove_tmp_dir("./xxx")
```
์ด๊ฒ์ ๋๋ฒ๊น
ํ ๋ ํน์ ๋๋ ํฐ๋ฆฌ๋ฅผ ๋ชจ๋ํฐ๋งํ๊ณ ,
๊ทธ ๋๋ ํฐ๋ฆฌ์ ์ด์ ์ ์คํ๋ ํ
์คํธ๊ฐ ๋ฐ์ดํฐ๋ฅผ ๋จ๊ธฐ์ง ์๋๋ก ํ๋ ๋ฐ์ ์ ์ฉํฉ๋๋ค.
- `before` ๋ฐ `after` ์ธ์๋ฅผ ์ง์ ์ค๋ฒ๋ผ์ด๋ฉํ์ฌ ๊ธฐ๋ณธ ๋์์ ๋ณ๊ฒฝํ ์ ์์ผ๋ฉฐ
๋ค์ ์ค ํ๋์ ๋์์ผ๋ก ์ด์ด์ง๋๋ค:
- `before=True`: ํ
์คํธ ์์ ์ ์์ ๋๋ ํฐ๋ฆฌ๊ฐ ํญ์ ์ง์์ง๋๋ค.
- `before=False`: ์์ ๋๋ ํฐ๋ฆฌ๊ฐ ์ด๋ฏธ ์กด์ฌํ๋ ๊ฒฝ์ฐ ๊ธฐ์กด ํ์ผ์ ๊ทธ๋๋ก ๋จ์ต๋๋ค.
- `after=True`: ํ
์คํธ ์ข
๋ฃ ์ ์์ ๋๋ ํฐ๋ฆฌ๊ฐ ํญ์ ์ญ์ ๋ฉ๋๋ค.
- `after=False`: ํ
์คํธ ์ข
๋ฃ ์ ์์ ๋๋ ํฐ๋ฆฌ๊ฐ ํญ์ ๊ทธ๋๋ก ์ ์ง๋ฉ๋๋ค.
<Tip>
`rm -r`์ ํด๋นํ๋ ๋ช
๋ น์ ์์ ํ๊ฒ ์คํํ๊ธฐ ์ํด,
๋ช
์์ ์ธ `tmp_dir`์ ์ฌ์ฉํ๋ ๊ฒฝ์ฐ ํ๋ก์ ํธ ์ ์ฅ์ ์ฒดํฌ ์์์ ํ์ ๋๋ ํฐ๋ฆฌ๋ง ํ์ฉ๋ฉ๋๋ค.
๋ฐ๋ผ์ ์ค์๋ก `/tmp`๊ฐ ์๋ ์ค์ํ ํ์ผ ์์คํ
์ ์ผ๋ถ๊ฐ ์ญ์ ๋์ง ์๋๋ก ํญ์ `./`๋ก ์์ํ๋ ๊ฒฝ๋ก๋ฅผ ์ ๋ฌํด์ผ ํฉ๋๋ค.
</Tip>
<Tip>
๊ฐ ํ
์คํธ๋ ์ฌ๋ฌ ๊ฐ์ ์์ ๋๋ ํฐ๋ฆฌ๋ฅผ ๋ฑ๋กํ ์ ์์ผ๋ฉฐ,
๋ณ๋๋ก ์์ฒญํ์ง ์๋ ํ ๋ชจ๋ ์๋์ผ๋ก ์ ๊ฑฐ๋ฉ๋๋ค.
</Tip>
### ์์ sys.path ์ค๋ฒ๋ผ์ด๋[[temporary-sys.path-override]]
`sys.path`๋ฅผ ๋ค๋ฅธ ํ
์คํธ๋ก ์์๋ก ์ค๋ฒ๋ผ์ด๋ํ๊ธฐ ์ํด ์๋ฅผ ๋ค์ด `ExtendSysPath` ์ปจํ
์คํธ ๊ด๋ฆฌ์๋ฅผ ์ฌ์ฉํ ์ ์์ต๋๋ค.
์๋ฅผ ๋ค์ด ๋ค์๊ณผ ๊ฐ์ต๋๋ค:
```python
import os
from transformers.testing_utils import ExtendSysPath
bindir = os.path.abspath(os.path.dirname(__file__))
with ExtendSysPath(f"{bindir}/.."):
from test_trainer import TrainerIntegrationCommon # noqa
```
### ํ
์คํธ ๊ฑด๋๋ฐ๊ธฐ[[skipping-tests]]
์ด๊ฒ์ ๋ฒ๊ทธ๊ฐ ๋ฐ๊ฒฌ๋์ด ์๋ก์ด ํ
์คํธ๊ฐ ์์ฑ๋์์ง๋ง ์์ง ๊ทธ ๋ฒ๊ทธ๊ฐ ์์ ๋์ง ์์ ๊ฒฝ์ฐ์ ์ ์ฉํฉ๋๋ค.
์ด ํ
์คํธ๋ฅผ ์ฃผ ์ ์ฅ์์ ์ปค๋ฐํ๋ ค๋ฉด `make test` ์ค์ ๊ฑด๋๋ฐ๋๋ก ํด์ผ ํฉ๋๋ค.
๋ฐฉ๋ฒ:
- **skip**์ ํ
์คํธ๊ฐ ์ผ๋ถ ์กฐ๊ฑด์ด ์ถฉ์กฑ๋ ๊ฒฝ์ฐ์๋ง ํต๊ณผ๋ ๊ฒ์ผ๋ก ์์๋๊ณ , ๊ทธ๋ ์ง ์์ผ๋ฉด pytest๊ฐ ์ ์ฒด ํ
์คํธ๋ฅผ ๊ฑด๋๋ฐ์ด์ผ ํจ์ ์๋ฏธํฉ๋๋ค.
์ผ๋ฐ์ ์ธ ์๋ก๋ Windows๊ฐ ์๋ ํ๋ซํผ์์ Windows ์ ์ฉ ํ
์คํธ๋ฅผ ๊ฑด๋๋ฐ๊ฑฐ๋
์ธ๋ถ ๋ฆฌ์์ค(์๋ฅผ ๋ค์ด ๋ฐ์ดํฐ๋ฒ ์ด์ค)์ ์์กดํ๋ ํ
์คํธ๋ฅผ ๊ฑด๋๋ฐ๋ ๊ฒ์ด ์์ต๋๋ค.
- **xfail**์ ํ
์คํธ๊ฐ ํน์ ํ ์ด์ ๋ก ์ธํด ์คํจํ ๊ฒ์ผ๋ก ์์ํ๋ ๊ฒ์ ์๋ฏธํฉ๋๋ค.
์ผ๋ฐ์ ์ธ ์๋ก๋ ์์ง ๊ตฌํ๋์ง ์์ ๊ธฐ๋ฅ์ด๋ ์์ง ์์ ๋์ง ์์ ๋ฒ๊ทธ์ ํ
์คํธ๊ฐ ์์ต๋๋ค.
`xfail`๋ก ํ์๋ ํ
์คํธ๊ฐ ์์๋๋ก ์คํจํ์ง ์๊ณ ํต๊ณผ๋ ๊ฒฝ์ฐ, ์ด๊ฒ์ xpass์ด๋ฉฐ ํ
์คํธ ๊ฒฐ๊ณผ ์์ฝ์ ๊ธฐ๋ก๋ฉ๋๋ค.
๋ ๊ฐ์ง ์ค์ํ ์ฐจ์ด์ ์ค ํ๋๋ `skip`์ ํ
์คํธ๋ฅผ ์คํํ์ง ์์ง๋ง `xfail`์ ์คํํ๋ค๋ ๊ฒ์
๋๋ค.
๋ฐ๋ผ์ ์ค๋ฅ๊ฐ ์๋ ์ฝ๋๊ฐ ์ผ๋ถ ํ
์คํธ์ ์ํฅ์ ๋ฏธ์น ์ ์๋ ๊ฒฝ์ฐ `xfail`์ ์ฌ์ฉํ์ง ๋ง์ธ์.
#### ๊ตฌํ[[implementation]]
- ์ ์ฒด ํ
์คํธ๋ฅผ ๋ฌด์กฐ๊ฑด ๊ฑด๋๋ฐ๋ ค๋ฉด ๋ค์๊ณผ ๊ฐ์ด ํ ์ ์์ต๋๋ค:
```python no-style
@unittest.skip("this bug needs to be fixed")
def test_feature_x():
```
๋๋ pytest๋ฅผ ํตํด:
```python no-style
@pytest.mark.skip(reason="this bug needs to be fixed")
```
๋๋ `xfail` ๋ฐฉ์์ผ๋ก:
```python no-style
@pytest.mark.xfail
def test_feature_x():
```
- ํ
์คํธ ๋ด๋ถ์์ ๋ด๋ถ ํ์ธ์ ๋ฐ๋ผ ํ
์คํธ๋ฅผ ๊ฑด๋๋ฐ๋ ๋ฐฉ๋ฒ์ ๋ค์๊ณผ ๊ฐ์ต๋๋ค:
```python
def test_feature_x():
if not has_something():
pytest.skip("unsupported configuration")
```
๋๋ ๋ชจ๋ ์ ์ฒด:
```python
import pytest
if not pytest.config.getoption("--custom-flag"):
pytest.skip("--custom-flag is missing, skipping tests", allow_module_level=True)
```
๋๋ `xfail` ๋ฐฉ์์ผ๋ก:
```python
def test_feature_x():
pytest.xfail("expected to fail until bug XYZ is fixed")
```
- import๊ฐ missing๋ ๋ชจ๋์ด ์์ ๋ ๊ทธ ๋ชจ๋์ ๋ชจ๋ ํ
์คํธ๋ฅผ ๊ฑด๋๋ฐ๋ ๋ฐฉ๋ฒ:
```python
docutils = pytest.importorskip("docutils", minversion="0.3")
```
- ์กฐ๊ฑด์ ๋ฐ๋ผ ํ
์คํธ๋ฅผ ๊ฑด๋๋ฐ๋ ๋ฐฉ๋ฒ:
```python no-style
@pytest.mark.skipif(sys.version_info < (3,6), reason="requires python3.6 or higher")
def test_feature_x():
```
๋๋:
```python no-style
@unittest.skipIf(torch_device == "cpu", "Can't do half precision")
def test_feature_x():
```
๋๋ ๋ชจ๋ ์ ์ฒด๋ฅผ ๊ฑด๋๋ฐ๋ ๋ฐฉ๋ฒ:
```python no-style
@pytest.mark.skipif(sys.platform == 'win32', reason="does not run on windows")
class TestClass():
def test_feature_x(self):
```
๋ณด๋ค ์์ธํ ์์ ๋ฐ ๋ฐฉ๋ฒ์ [์ฌ๊ธฐ](https://docs.pytest.org/en/latest/skipping.html)์์ ํ์ธํ ์ ์์ต๋๋ค.
### ๋๋ฆฐ ํ
์คํธ[[slow-tests]]
ํ
์คํธ ๋ผ์ด๋ธ๋ฌ๋ฆฌ๋ ์ง์์ ์ผ๋ก ํ์ฅ๋๊ณ ์์ผ๋ฉฐ, ์ผ๋ถ ํ
์คํธ๋ ์คํํ๋ ๋ฐ ๋ช ๋ถ์ด ๊ฑธ๋ฆฝ๋๋ค.
๊ทธ๋ฆฌ๊ณ ์ฐ๋ฆฌ์๊ฒ๋ ํ
์คํธ ์ค์ํธ๊ฐ CI๋ฅผ ํตํด ์๋ฃ๋๊ธฐ๊น์ง ํ ์๊ฐ์ ๊ธฐ๋ค๋ฆด ์ฌ์ ๊ฐ ์์ต๋๋ค.
๋ฐ๋ผ์ ํ์ ํ
์คํธ๋ฅผ ์ํ ์ผ๋ถ ์์ธ๋ฅผ ์ ์ธํ๊ณ ๋๋ฆฐ ํ
์คํธ๋ ๋ค์๊ณผ ๊ฐ์ด ํ์ํด์ผ ํฉ๋๋ค.
```python no-style
from transformers.testing_utils import slow
@slow
def test_integration_foo():
```
`@slow`๋ก ํ์๋ ํ
์คํธ๋ฅผ ์คํํ๋ ค๋ฉด `RUN_SLOW=1` ํ๊ฒฝ ๋ณ์๋ฅผ ์ค์ ํ์ธ์. ์๋ฅผ ๋ค์ด ๋ค์๊ณผ ๊ฐ์ต๋๋ค:
```bash
RUN_SLOW=1 pytest tests
```
`@parameterized`์ ๊ฐ์ ๋ช ๊ฐ์ง ๋ฐ์ฝ๋ ์ดํฐ๋ ํ
์คํธ ์ด๋ฆ์ ๋ค์ ์์ฑํฉ๋๋ค.
๊ทธ๋ฌ๋ฏ๋ก `@slow`์ ๋๋จธ์ง ๊ฑด๋๋ฐ๊ธฐ ๋ฐ์ฝ๋ ์ดํฐ `@require_*`๊ฐ ์ฌ๋ฐ๋ฅด๊ฒ ์๋๋๋ ค๋ฉด ๋ง์ง๋ง์ ๋์ด๋์ด์ผ ํฉ๋๋ค. ๋ค์์ ์ฌ๋ฐ๋ฅธ ์ฌ์ฉ ์์
๋๋ค.
```python no-style
@parameterized.expand(...)
@slow
def test_integration_foo():
```
์ด ๋ฌธ์์ ์ด๋ฐ๋ถ์ ์ค๋ช
๋ ๊ฒ์ฒ๋ผ ๋๋ฆฐ ํ
์คํธ๋ PR์ CI ํ์ธ์ด ์๋ ์์ฝ๋ ์ผ์ ๊ธฐ๋ฐ์ผ๋ก ์คํ๋ฉ๋๋ค.
๋ฐ๋ผ์ PR ์ ์ถ ์ค์ ์ผ๋ถ ๋ฌธ์ ๋ฅผ ๋์น ์ฑ๋ก ๋ณํฉ๋ ์ ์์ต๋๋ค.
์ด๋ฌํ ๋ฌธ์ ๋ค์ ๋ค์๋ฒ์ ์์ ๋ CI ์์
์ค์ ๊ฐ์ง๋ฉ๋๋ค.
ํ์ง๋ง PR์ ์ ์ถํ๊ธฐ ์ ์ ์์ ์ ์ปดํจํฐ์์ ๋๋ฆฐ ํ
์คํธ๋ฅผ ์คํํ๋ ๊ฒ ๋ํ ์ค์ํฉ๋๋ค.
๋๋ฆฐ ํ
์คํธ๋ก ํ์ํด์ผ ํ๋์ง ์ฌ๋ถ๋ฅผ ๊ฒฐ์ ํ๋ ๋๋ต์ ์ธ ๊ฒฐ์ ๊ธฐ์ค์ ๋ค์๊ณผ ๊ฐ์ต๋๋ค.
๋ง์ฝ ํ
์คํธ๊ฐ ๋ผ์ด๋ธ๋ฌ๋ฆฌ์ ๋ด๋ถ ๊ตฌ์ฑ ์์ ์ค ํ๋์ ์ง์ค๋์ด ์๋ค๋ฉด(์: ๋ชจ๋ธ๋ง ํ์ผ, ํ ํฐํ ํ์ผ, ํ์ดํ๋ผ์ธ),
ํด๋น ํ
์คํธ๋ฅผ ๋๋ฆฐ ํ
์คํธ ์ค์ํธ์์ ์คํํด์ผ ํฉ๋๋ค.
๋ง์ฝ ๋ผ์ด๋ธ๋ฌ๋ฆฌ์ ๋ค๋ฅธ ์ธก๋ฉด(์: ๋ฌธ์ ๋๋ ์์ )์ ์ง์ค๋์ด ์๋ค๋ฉด,
ํด๋น ํ
์คํธ๋ฅผ ๋๋ฆฐ ํ
์คํธ ์ค์ํธ์์ ์คํํด์ผ ํฉ๋๋ค. ๊ทธ๋ฆฌ๊ณ ์ด ์ ๊ทผ ๋ฐฉ์์ ๋ณด์ํ๊ธฐ ์ํด ์์ธ๋ฅผ ๋ง๋ค์ด์ผ ํฉ๋๋ค.
- ๋ฌด๊ฑฐ์ด ๊ฐ์ค์น ์ธํธ๋ 50MB๋ณด๋ค ํฐ ๋ฐ์ดํฐ์
์ ๋ค์ด๋ก๋ํด์ผ ํ๋ ๋ชจ๋ ํ
์คํธ(์: ๋ชจ๋ธ ํตํฉ ํ
์คํธ, ํ ํฌ๋์ด์ ํตํฉ ํ
์คํธ, ํ์ดํ๋ผ์ธ ํตํฉ ํ
์คํธ)๋ฅผ
๋๋ฆฐ ํ
์คํธ๋ก ์ค์ ํด์ผ ํฉ๋๋ค.
์๋ก์ด ๋ชจ๋ธ์ ์ถ๊ฐํ๋ ๊ฒฝ์ฐ ํตํฉ ํ
์คํธ์ฉ์ผ๋ก ๋ฌด์์ ๊ฐ์ค์น๋ก ์์ ๋ฒ์ ์ ๋ง๋ค์ด ํ๋ธ์ ์
๋ก๋ํด์ผ ํฉ๋๋ค.
์ด ๋ด์ฉ์ ์๋ ๋จ๋ฝ์์ ์ค๋ช
๋ฉ๋๋ค.
- ํน๋ณํ ๋น ๋ฅด๊ฒ ์คํ๋๋๋ก ์ต์ ํ๋์ง ์์ ํ์ต์ ์ํํด์ผ ํ๋ ํ
์คํธ๋ ๋๋ฆฐ ํ
์คํธ๋ก ์ค์ ํด์ผ ํฉ๋๋ค.
- ๋๋ฆฌ์ง ์์์ผ ํ ํ
์คํธ ์ค ์ผ๋ถ๊ฐ ๊ทน๋๋ก ๋๋ฆฐ ๊ฒฝ์ฐ
์์ธ๋ฅผ ๋์
ํ๊ณ ์ด๋ฅผ `@slow`๋ก ์ค์ ํ ์ ์์ต๋๋ค.
๋์ฉ๋ ํ์ผ์ ๋์คํฌ์ ์ ์ฅํ๊ณ ๋ถ๋ฌ์ค๋ ์๋ ๋ชจ๋ธ๋ง ํ
์คํธ๋ `@slow`์ผ๋ก ํ์๋ ํ
์คํธ์ ์ข์ ์์
๋๋ค.
- CI์์ 1์ด ์ด๋ด์ ํ
์คํธ๊ฐ ์๋ฃ๋๋ ๊ฒฝ์ฐ(๋ค์ด๋ก๋ ํฌํจ)์๋ ๋๋ฆฐ ํ
์คํธ๊ฐ ์๋์ด์ผ ํฉ๋๋ค.
๋๋ฆฐ ํ
์คํธ๊ฐ ์๋ ๊ฒฝ์ฐ์๋ ๋ค์ํ ๋ด๋ถ๋ฅผ ์์ ํ ์ปค๋ฒํ๋ฉด์ ๋น ๋ฅด๊ฒ ์ ์ง๋์ด์ผ ํฉ๋๋ค.
์๋ฅผ ๋ค์ด, ๋ฌด์์ ๊ฐ์ค์น๋ฅผ ์ฌ์ฉํ์ฌ ํน๋ณํ ์์ฑ๋ ์์ ๋ชจ๋ธ๋ก ํ
์คํธํ๋ฉด ์๋นํ ์ปค๋ฒ๋ฆฌ์ง๋ฅผ ์ป์ ์ ์์ต๋๋ค.
์ด๋ฌํ ๋ชจ๋ธ์ ์ต์ํ์ ๋ ์ด์ด ์(์: 2), ์ดํ ํฌ๊ธฐ(์: 1000) ๋ฑ์ ์์๋ง ๊ฐ์ง๋๋ค. ๊ทธ๋ฐ ๋ค์ `@slow` ํ
์คํธ๋ ๋ํ ๋๋ฆฐ ๋ชจ๋ธ์ ์ฌ์ฉํ์ฌ ์ ์ฑ์ ์ธ ํ
์คํธ๋ฅผ ์ํํ ์ ์์ต๋๋ค.
์ด๋ฌํ ์์ ๋ชจ๋ธ์ ์ฌ์ฉํ๋ ๋ฐฉ๋ฒ์ ํ์ธํ๋ ค๋ฉด ๋ค์๊ณผ ๊ฐ์ด *tiny* ๋ชจ๋ธ์ ์ฐพ์๋ณด์ธ์.
```bash
grep tiny tests examples
```
๋ค์์ ์์ ๋ชจ๋ธ[stas/tiny-wmt19-en-de](https://huggingface.co/stas/tiny-wmt19-en-de)์ ๋ง๋
[script](https://github.com/huggingface/transformers/tree/main/scripts/fsmt/fsmt-make-tiny-model.py) ์์์
๋๋ค.
ํน์ ๋ชจ๋ธ์ ์ํคํ
์ฒ์ ๋ง๊ฒ ์ฝ๊ฒ ์กฐ์ ํ ์ ์์ต๋๋ค.
์๋ฅผ ๋ค์ด ๋์ฉ๋ ๋ชจ๋ธ์ ๋ค์ด๋ก๋ํ๋ ๊ฒฝ์ฐ ๋ฐํ์์ ์๋ชป ์ธก์ ํ๊ธฐ ์ฝ์ง๋ง,
๋ก์ปฌ์์ ํ
์คํธํ๋ฉด ๋ค์ด๋ก๋ํ ํ์ผ์ด ์บ์๋์ด ๋ค์ด๋ก๋ ์๊ฐ์ด ์ธก์ ๋์ง ์์ต๋๋ค.
๋์ CI ๋ก๊ทธ์ ์คํ ์๋ ๋ณด๊ณ ์๋ฅผ ํ์ธํ์ธ์(`pytest --durations=0 tests`์ ์ถ๋ ฅ).
์ด ๋ณด๊ณ ์๋ ๋๋ฆฐ ์ด์๊ฐ์ผ๋ก ํ์๋์ง ์๊ฑฐ๋ ๋น ๋ฅด๊ฒ ๋ค์ ์์ฑํด์ผ ํ๋ ๋๋ฆฐ ์ด์๊ฐ์ ์ฐพ๋ ๋ฐ๋ ์ ์ฉํฉ๋๋ค.
CI์์ ํ
์คํธ ์ค์ํธ๊ฐ ๋๋ ค์ง๊ธฐ ์์ํ๋ฉด ์ด ๋ณด๊ณ ์์ ๋งจ ์ ๋ชฉ๋ก์ ๊ฐ์ฅ ๋๋ฆฐ ํ
์คํธ๊ฐ ํ์๋ฉ๋๋ค.
### stdout/stderr ์ถ๋ ฅ ํ
์คํธ[[testing-the-stdout/stderr-output]]
`stdout` ๋ฐ/๋๋ `stderr`๋ก ์ฐ๋ ํจ์๋ฅผ ํ
์คํธํ๋ ค๋ฉด `pytest`์ [capsys ์์คํ
](https://docs.pytest.org/en/latest/capture.html)์ ์ฌ์ฉํ์ฌ ํด๋น ์คํธ๋ฆผ์ ์ก์ธ์คํ ์ ์์ต๋๋ค.
๋ค์๊ณผ ๊ฐ์ด ์ํํ ์ ์์ต๋๋ค.
```python
import sys
def print_to_stdout(s):
print(s)
def print_to_stderr(s):
sys.stderr.write(s)
def test_result_and_stdout(capsys):
msg = "Hello"
print_to_stdout(msg)
print_to_stderr(msg)
out, err = capsys.readouterr() # ์บก์ฒ๋ ์ถ๋ ฅ ์คํธ๋ฆผ ์ฌ์ฉ
# ์ ํ ์ฌํญ: ์บก์ฒ๋ ์คํธ๋ฆผ ์ฌ์์ฑ
sys.stdout.write(out)
sys.stderr.write(err)
# ํ
์คํธ:
assert msg in out
assert msg in err
```
๊ทธ๋ฆฌ๊ณ , ๋ฌผ๋ก ๋๋ถ๋ถ์ ๊ฒฝ์ฐ์๋ `stderr`๋ ์์ธ์ ์ผ๋ถ๋ก ์ ๊ณต๋ฉ๋๋ค.
๊ทธ๋ฌ๋ฏ๋ก ํด๋น ๊ฒฝ์ฐ์๋ try/except๋ฅผ ์ฌ์ฉํด์ผ ํฉ๋๋ค.
```python
def raise_exception(msg):
raise ValueError(msg)
def test_something_exception():
msg = "Not a good value"
error = ""
try:
raise_exception(msg)
except Exception as e:
error = str(e)
assert msg in error, f"{msg} is in the exception:\n{error}"
```
`stdout`๋ฅผ ์บก์ฒํ๋ ๋ ๋ค๋ฅธ ๋ฐฉ๋ฒ์ `contextlib.redirect_stdout`๋ฅผ ์ฌ์ฉํ๋ ๊ฒ์
๋๋ค.
```python
from io import StringIO
from contextlib import redirect_stdout
def print_to_stdout(s):
print(s)
def test_result_and_stdout():
msg = "Hello"
buffer = StringIO()
with redirect_stdout(buffer):
print_to_stdout(msg)
out = buffer.getvalue()
# ์ ํ ์ฌํญ: ์บก์ฒ๋ ์คํธ๋ฆผ ์ฌ์์ฑ
sys.stdout.write(out)
# ํ
์คํธ:
assert msg in out
```
`stdout` ์บก์ฒ์ ๊ด๋ จ๋ ์ค์ํ ๋ฌธ์ ์ค ํ๋๋ ๋ณดํต `print`์์ ์ด์ ์ ์ธ์๋ ๋ด์ฉ์ ์ฌ์ค์ ํ๋ `\r` ๋ฌธ์๊ฐ ํฌํจ๋ ์ ์๋ค๋ ๊ฒ์
๋๋ค.
`pytest`์์๋ ๋ฌธ์ ๊ฐ ์์ง๋ง `pytest -s`์์๋ ์ด๋ฌํ ๋ฌธ์๊ฐ ๋ฒํผ์ ํฌํจ๋๋ฏ๋ก
`-s`๊ฐ ์๊ฑฐ๋ ์๋ ์ํ์์ ํ์คํธ๋ฅผ ์ํํ ์ ์์ผ๋ ค๋ฉด ์บก์ฒ๋ ์ถ๋ ฅ์ ๋ํด ์ถ๊ฐ์ ์ธ ์ ๋ฆฌ๊ฐ ํ์ํฉ๋๋ค.
์ด ๊ฒฝ์ฐ์๋ `re.sub(r'~.*\r', '', buf, 0, re.M)`์ ์ฌ์ฉํ ์ ์์ต๋๋ค.
ํ์ง๋ง ๋์ฐ๋ฏธ ์ปจํ
์คํธ ๊ด๋ฆฌ์ ๋ํผ๋ฅผ ์ฌ์ฉํ๋ฉด
์ถ๋ ฅ์ `\r`์ด ํฌํจ๋์ด ์๋์ง์ ์ฌ๋ถ์ ๊ด๊ณ์์ด ๋ชจ๋ ๊ฒ์ ์๋์ผ๋ก ์ฒ๋ฆฌํ๋ฏ๋ก ํธ๋ฆฌํฉ๋๋ค.
```python
from transformers.testing_utils import CaptureStdout
with CaptureStdout() as cs:
function_that_writes_to_stdout()
print(cs.out)
```
๋ค์์ ์ ์ฒด ํ
์คํธ ์์ ์
๋๋ค.
```python
from transformers.testing_utils import CaptureStdout
msg = "Secret message\r"
final = "Hello World"
with CaptureStdout() as cs:
print(msg + final)
assert cs.out == final + "\n", f"captured: {cs.out}, expecting {final}"
```
`stderr`๋ฅผ ์บก์ฒํ๊ณ ์ถ๋ค๋ฉด, ๋์ `CaptureStderr` ํด๋์ค๋ฅผ ์ฌ์ฉํ์ธ์.
```python
from transformers.testing_utils import CaptureStderr
with CaptureStderr() as cs:
function_that_writes_to_stderr()
print(cs.err)
```
๋ ์คํธ๋ฆผ์ ๋์์ ์บก์ฒํด์ผ ํ๋ค๋ฉด, ๋ถ๋ชจ `CaptureStd` ํด๋์ค๋ฅผ ์ฌ์ฉํ์ธ์.
```python
from transformers.testing_utils import CaptureStd
with CaptureStd() as cs:
function_that_writes_to_stdout_and_stderr()
print(cs.err, cs.out)
```
๋ํ, ํ
์คํธ์ ๋๋ฒ๊น
์ ์ง์ํ๊ธฐ ์ํด
์ด๋ฌํ ์ปจํ
์คํธ ๊ด๋ฆฌ์๋ ๊ธฐ๋ณธ์ ์ผ๋ก ์ปจํ
์คํธ์์ ์ข
๋ฃํ ๋ ์บก์ฒ๋ ์คํธ๋ฆผ์ ์๋์ผ๋ก ๋ค์ ์คํํฉ๋๋ค.
### ๋ก๊ฑฐ ์คํธ๋ฆผ ์บก์ฒ[[capturing-logger-stream]]
๋ก๊ฑฐ ์ถ๋ ฅ์ ๊ฒ์ฆํด์ผ ํ๋ ๊ฒฝ์ฐ `CaptureLogger`๋ฅผ ์ฌ์ฉํ ์ ์์ต๋๋ค.
```python
from transformers import logging
from transformers.testing_utils import CaptureLogger
msg = "Testing 1, 2, 3"
logging.set_verbosity_info()
logger = logging.get_logger("transformers.models.bart.tokenization_bart")
with CaptureLogger(logger) as cl:
logger.info(msg)
assert cl.out, msg + "\n"
```
### ํ๊ฒฝ ๋ณ์๋ฅผ ์ด์ฉํ์ฌ ํ
์คํธ[[testing-with-environment-variables]]
ํน์ ํ
์คํธ์ ํ๊ฒฝ ๋ณ์ ์ํฅ์ ๊ฒ์ฆํ๋ ค๋ฉด
`transformers.testing_utils.mockenv`๋ผ๋ ๋์ฐ๋ฏธ ๋ฐ์ฝ๋ ์ดํฐ๋ฅผ ์ฌ์ฉํ ์ ์์ต๋๋ค.
```python
from transformers.testing_utils import mockenv
class HfArgumentParserTest(unittest.TestCase):
@mockenv(TRANSFORMERS_VERBOSITY="error")
def test_env_override(self):
env_level_str = os.getenv("TRANSFORMERS_VERBOSITY", None)
```
์ผ๋ถ ๊ฒฝ์ฐ์๋ ์ธ๋ถ ํ๋ก๊ทธ๋จ์ ํธ์ถํด์ผํ ์๋ ์๋๋ฐ, ์ด ๋์๋ ์ฌ๋ฌ ๊ฐ์ ๋ก์ปฌ ๊ฒฝ๋ก๋ฅผ ํฌํจํ๋ `os.environ`์์ `PYTHONPATH`์ ์ค์ ์ด ํ์ํฉ๋๋ค.
ํฌํผ ํด๋์ค `transformers.test_utils.TestCasePlus`๊ฐ ๋์์ด ๋ฉ๋๋ค:
```python
from transformers.testing_utils import TestCasePlus
class EnvExampleTest(TestCasePlus):
def test_external_prog(self):
env = self.get_env()
# ์ด์ `env`๋ฅผ ์ฌ์ฉํ์ฌ ์ธ๋ถ ํ๋ก๊ทธ๋จ ํธ์ถ
```
ํ
์คํธ ํ์ผ์ด `tests` ํ
์คํธ ์ค์ํธ ๋๋ `examples`์ ์๋์ง์ ๋ฐ๋ผ
`env[PYTHONPATH]`๊ฐ ๋ ๋๋ ํฐ๋ฆฌ ์ค ํ๋๋ฅผ ํฌํจํ๋๋ก ์ค์ ๋๋ฉฐ,
ํ์ฌ ์ ์ฅ์์ ๋ํด ํ
์คํธ๊ฐ ์ํ๋๋๋ก `src` ๋๋ ํฐ๋ฆฌ๋ ํฌํจ๋ฉ๋๋ค.
ํ
์คํธ ํธ์ถ ์ด์ ์ ์ค์ ๋ ๊ฒฝ์ฐ์๋ `env[PYTHONPATH]`๋ฅผ ๊ทธ๋๋ก ์ฌ์ฉํฉ๋๋ค.
์ด ํฌํผ ๋ฉ์๋๋ `os.environ` ๊ฐ์ฒด์ ์ฌ๋ณธ์ ์์ฑํ๋ฏ๋ก ์๋ณธ์ ๊ทธ๋๋ก ์ ์ง๋ฉ๋๋ค.
### ์ฌํ ๊ฐ๋ฅํ ๊ฒฐ๊ณผ ์ป๊ธฐ[[getting-reproducible-results]]
์ผ๋ถ ์ํฉ์์ ํ
์คํธ์์ ์์์ฑ์ ์ ๊ฑฐํ์ฌ ๋์ผํ๊ฒ ์ฌํ ๊ฐ๋ฅํ ๊ฒฐ๊ณผ๋ฅผ ์ป๊ณ ์ถ์ ์ ์์ต๋๋ค.
์ด๋ฅผ ์ํด์๋ ๋ค์๊ณผ ๊ฐ์ด ์๋๋ฅผ ๊ณ ์ ํด์ผ ํฉ๋๋ค.
```python
seed = 42
# ํ์ด์ฌ RNG
import random
random.seed(seed)
# ํ์ดํ ์น RNG
import torch
torch.manual_seed(seed)
torch.backends.cudnn.deterministic = True
if torch.cuda.is_available():
torch.cuda.manual_seed_all(seed)
# ๋ํ์ด RNG
import numpy as np
np.random.seed(seed)
# ํ
์ํ๋ก RNG
tf.random.set_seed(seed)
```
### ํ
์คํธ ๋๋ฒ๊น
[[debugging tests]]
๊ฒฝ๊ณ ๊ฐ ์๋ ๊ณณ์์ ๋๋ฒ๊ฑฐ๋ฅผ ์์ํ๋ ค๋ฉด ๋ค์์ ์ํํ์ธ์.
```bash
pytest tests/test_logging.py -W error::UserWarning --pdb
```
## Github Actions ์ํฌํ๋ก์ฐ ์์
์ฒ๋ฆฌ[[working-with-github-actions-workflows]]
์
ํ ํธ์ ์ํฌํ๋ก์ฐ CI ์์
์ ํธ๋ฆฌ๊ฑฐํ๋ ค๋ฉด, ๋ค์์ ์ํํด์ผ ํฉ๋๋ค.
1. `transformers` ์๋ณธ์์ ์ ๋ธ๋์น๋ฅผ ๋ง๋ญ๋๋ค(ํฌํฌ๊ฐ ์๋๋๋ค!).
2. ๋ธ๋์น ์ด๋ฆ์ `ci_` ๋๋ `ci-`๋ก ์์ํด์ผ ํฉ๋๋ค(`main`๋ ํธ๋ฆฌ๊ฑฐํ์ง๋ง `main`์์๋ PR์ ํ ์ ์์ต๋๋ค).
๋ํ ํน์ ๊ฒฝ๋ก์ ๋ํด์๋ง ํธ๋ฆฌ๊ฑฐ๋๋ฏ๋ก ์ด ๋ฌธ์๊ฐ ์์ฑ๋ ํ์ ๋ณ๊ฒฝ๋ ๋ด์ฉ์
[์ฌ๊ธฐ](https://github.com/huggingface/transformers/blob/main/.github/workflows/self-push.yml)์ *push:*์์ ํ์ธํ ์ ์์ต๋๋ค.
3. ์ด ๋ธ๋์น์์ PR์ ์์ฑํฉ๋๋ค
4. ๊ทธ๋ฐ ๋ค์ [์ฌ๊ธฐ](https://github.com/huggingface/transformers/actions/workflows/self-push.yml)์์ ์์
์ด ๋ํ๋๋์ง ํ์ธํ ์ ์์ต๋๋ค.
๋ฐฑ๋ก๊ทธ๊ฐ ์๋ ๊ฒฝ์ฐ, ๋ฐ๋ก ์คํ๋์ง ์์ ์๋ ์์ต๋๋ค.
## ์คํ์ ์ธ CI ๊ธฐ๋ฅ ํ
์คํธ[[testing-Experimental-CI-Features]]
CI ๊ธฐ๋ฅ์ ํ
์คํธํ๋ ๊ฒ์ ์ผ๋ฐ CI ์๋์ ๋ฐฉํด๊ฐ ๋ ์ ์๊ธฐ ๋๋ฌธ์ ์ ์ฌ์ ์ผ๋ก ๋ฌธ์ ๊ฐ ๋ฐ์ํ ์ ์์ต๋๋ค.
๋ฐ๋ผ์ ์๋ก์ด CI ๊ธฐ๋ฅ์ ์ถ๊ฐํ๋ ๊ฒฝ์ฐ ๋ค์๊ณผ ๊ฐ์ด ์ํํด์ผ ํฉ๋๋ค.
1. ํ
์คํธํด์ผ ํ ๋ด์ฉ์ ํ
์คํธํ๋ ์๋ก์ด ์ ์ฉ ์์
์ ์์ฑํฉ๋๋ค.
2. ์๋ก์ด ์์
์ ํญ์ ์ฑ๊ณตํด์ผ๋ง ๋
น์ โ๋ฅผ ๋ฐ์ ์ ์์ต๋๋ค(์๋์ ์์ธํ ๋ด์ฉ์ด ์์ต๋๋ค).
3. ๋ค์ํ PR ์ ํ์ ๋ํ ํ์ธ์ ์ํด
(์ฌ์ฉ์ ํฌํฌ ๋ธ๋์น, ํฌํฌ๋์ง ์์ ๋ธ๋์น, github.com UI ์ง์ ํ์ผ ํธ์ง์์ ์์ฑ๋ ๋ธ๋์น, ๊ฐ์ ํธ์ ๋ฑ PR์ ์ ํ์ ์์ฃผ ๋ค์ํฉ๋๋ค.)
๋ฉฐ์น ๋์ ์คํ ์์
์ ๋ก๊ทธ๋ฅผ ๋ชจ๋ํฐ๋งํ๋ฉด์ ์คํํด๋ด
๋๋ค.
(์๋์ ์ผ๋ก ํญ์ ๋
น์์ ํ์ํ๋ฏ๋ก ์์
์ ์ฒด๊ฐ ๋
น์์ ์๋๋ผ๋ ์ ์ ์ ์ํฉ๋๋ค.)
4. ๋ชจ๋ ๊ฒ์ด ์์ ์ ์ธ์ง ํ์ธํ ํ, ์๋ก์ด ๋ณ๊ฒฝ ์ฌํญ์ ๊ธฐ์กด ์์
์ ๋ณํฉํฉ๋๋ค.
์ด๋ ๊ฒ ํ๋ฉด CI ๊ธฐ๋ฅ ์์ฒด์ ๋ํ ์คํ์ด ์ผ๋ฐ ์์
ํ๋ฆ์ ๋ฐฉํด๊ฐ ๋์ง ์์ต๋๋ค.
๊ทธ๋ฌ๋ ์๋ก์ด CI ๊ธฐ๋ฅ์ด ๊ฐ๋ฐ ์ค์ธ ๋์, ํญ์ ์ฑ๊ณตํ๋๋ก ํ ์ ์๋ ๋ฐฉ๋ฒ์ ๋ฌด์์ผ๊น์?
TravisCI์ ๊ฐ์ ์ผ๋ถ CI๋ `ignore-step-failure`๋ฅผ ์ง์ํ๋ฉฐ ์ ์ฒด ์์
์ ์ฑ๊ณตํ ๊ฒ์ผ๋ก ๋ณด๊ณ ํ์ง๋ง,
ํ์ฌ ์ฐ๋ฆฌ๊ฐ ์ฌ์ฉํ๋ CircleCI์ Github Actions๋ ์ด๋ฅผ ์ง์ํ์ง ์์ต๋๋ค.
๋ฐ๋ผ์ ๋ค์๊ณผ ๊ฐ์ ํด๊ฒฐ์ฑ
์ ์ฌ์ฉํ ์ ์์ต๋๋ค.
1. bash ์คํฌ๋ฆฝํธ์์ ๊ฐ๋ฅํ ๋ง์ ์ค๋ฅ๋ฅผ ์ต์ ํ๊ธฐ ์ํด ์คํ ๋ช
๋ น์ ์์ ๋ถ๋ถ์ `set +euo pipefail`์ ์ถ๊ฐํฉ๋๋ค.
2. ๋ง์ง๋ง ๋ช
๋ น์ ๋ฐ๋์ ์ฑ๊ณตํด์ผ ํฉ๋๋ค. `echo "done"` ๋๋ `true`๋ฅผ ์ฌ์ฉํ๋ฉด ๋ฉ๋๋ค.
์์๋ ๋ค์๊ณผ ๊ฐ์ต๋๋ค.
```yaml
- run:
name: run CI experiment
command: |
set +euo pipefail
echo "setting run-all-despite-any-errors-mode"
this_command_will_fail
echo "but bash continues to run"
# emulate another failure
false
# but the last command must be a success
echo "during experiment do not remove: reporting success to CI, even if there were failures"
```
๊ฐ๋จํ ๋ช
๋ น์ ๊ฒฝ์ฐ ๋ค์๊ณผ ๊ฐ์ด ์ํํ ์๋ ์์ต๋๋ค.
```bash
cmd_that_may_fail || true
```
๊ฒฐ๊ณผ์ ๋ง์กฑํ ํ์๋ ๋ฌผ๋ก , ์คํ์ ์ธ ๋จ๊ณ ๋๋ ์์
์ ์ผ๋ฐ ์์
์ ๋๋จธ์ง ๋ถ๋ถ๊ณผ ํตํฉํ๋ฉด์
`set +euo pipefail` ๋๋ ๊ธฐํ ์ถ๊ฐํ ์์๋ฅผ ์ ๊ฑฐํ์ฌ
์คํ ์์
์ด ์ผ๋ฐ CI ์๋์ ๋ฐฉํด๋์ง ์๋๋ก ํด์ผ ํฉ๋๋ค.
์ด ์ ๋ฐ์ ์ธ ๊ณผ์ ์ ์คํ ๋จ๊ณ๊ฐ PR์ ์ ๋ฐ์ ์ธ ์ํ์ ์ํฅ์ ์ฃผ์ง ์๊ณ ์คํจํ๋๋ก
`allow-failure`์ ๊ฐ์ ๊ธฐ๋ฅ์ ์ค์ ํ ์ ์๋ค๋ฉด ํจ์ฌ ๋ ์ฌ์ ์ ๊ฒ์
๋๋ค.
๊ทธ๋ฌ๋ ์์์ ์ธ๊ธํ ๋ฐ์ ๊ฐ์ด CircleCI์ Github Actions๋ ํ์ฌ ์ด๋ฌํ ๊ธฐ๋ฅ๋ค ์ง์ํ์ง ์์ต๋๋ค.
์ด ๊ธฐ๋ฅ์ ์ง์์ ์ํ ํฌํ์ ์ฐธ์ฌํ๊ณ CI ๊ด๋ จ ์ค๋ ๋๋ค์์ ์ด๋ฌํ ์ํฉ์ ํ์ธํ ์๋ ์์ต๋๋ค.
- [Github Actions:](https://github.com/actions/toolkit/issues/399)
- [CircleCI:](https://ideas.circleci.com/ideas/CCI-I-344)
| 0 |
hf_public_repos/transformers/docs/source | hf_public_repos/transformers/docs/source/ko/installation.md | <!---
Copyright 2022 The HuggingFace Team. All rights reserved.
Licensed under the Apache License, Version 2.0 (the "License");
you may not use this file except in compliance with the License.
You may obtain a copy of the License at
http://www.apache.org/licenses/LICENSE-2.0
Unless required by applicable law or agreed to in writing, software
distributed under the License is distributed on an "AS IS" BASIS,
WITHOUT WARRANTIES OR CONDITIONS OF ANY KIND, either express or implied.
See the License for the specific language governing permissions and
limitations under the License.
โ ๏ธ Note that this file is in Markdown but contain specific syntax for our doc-builder (similar to MDX) that may not be
rendered properly in your Markdown viewer.
-->
# ์ค์น๋ฐฉ๋ฒ[[installation]]
๐ค Transformers๋ฅผ ์ฌ์ฉ ์ค์ธ ๋ฅ๋ฌ๋ ๋ผ์ด๋ธ๋ฌ๋ฆฌ์ ๋ง์ถฐ ์ค์นํ๊ณ , ์บ์๋ฅผ ๊ตฌ์ฑํ๊ฑฐ๋ ์ ํ์ ์ผ๋ก ์คํ๋ผ์ธ์์๋ ์คํํ ์ ์๋๋ก ๐ค Transformers๋ฅผ ์ค์ ํ๋ ๋ฐฉ๋ฒ์ ๋ฐฐ์ฐ๊ฒ ์ต๋๋ค.
๐ค Transformers๋ Python 3.6+, PyTorch 1.1.0+, TensorFlow 2.0+ ๋ฐ Flax์์ ํ
์คํธ๋์์ต๋๋ค. ๋ฅ๋ฌ๋ ๋ผ์ด๋ธ๋ฌ๋ฆฌ๋ฅผ ์ค์นํ๋ ค๋ฉด ์๋ ๋งํฌ๋ ์ ๋ง๋ค์ ๊ณต์ ์ฌ์ดํธ๋ฅผ ์ฐธ๊ณ ํด์ฃผ์ธ์.
* [PyTorch](https://pytorch.org/get-started/locally/) ์ค์นํ๊ธฐ
* [TensorFlow 2.0](https://www.tensorflow.org/install/pip) ์ค์นํ๊ธฐ
* [Flax](https://flax.readthedocs.io/en/latest/) ์ค์นํ๊ธฐ
## pip์ผ๋ก ์ค์นํ๊ธฐ[[install-with-pip]]
๐ค Transformers๋ฅผ [๊ฐ์ ํ๊ฒฝ](https://docs.python.org/3/library/venv.html)์ ์ค์นํ๋ ๊ฒ์ ์ถ์ฒ๋๋ฆฝ๋๋ค. Python ๊ฐ์ ํ๊ฒฝ์ ์ต์ํ์ง ์๋ค๋ฉด, ์ด [๊ฐ์ด๋](https://packaging.python.org/guides/installing-using-pip-and-virtual-environments/)๋ฅผ ์ฐธ๊ณ ํ์ธ์. ๊ฐ์ ํ๊ฒฝ์ ์ฌ์ฉํ๋ฉด ์๋ก ๋ค๋ฅธ ํ๋ก์ ํธ๋ค์ ๋ณด๋ค ์ฝ๊ฒ ๊ด๋ฆฌํ ์ ์๊ณ , ์์กด์ฑ ๊ฐ์ ํธํ์ฑ ๋ฌธ์ ๋ฅผ ๋ฐฉ์งํ ์ ์์ต๋๋ค.
๋จผ์ ํ๋ก์ ํธ ๋๋ ํ ๋ฆฌ์์ ๊ฐ์ ํ๊ฒฝ์ ๋ง๋ค์ด ์ค๋๋ค.
```bash
python -m venv .env
```
๊ฐ์ ํ๊ฒฝ์ ํ์ฑํํด์ฃผ์ธ์. Linux๋ MacOS์ ๊ฒฝ์ฐ:
```bash
source .env/bin/activate
```
Windows์ ๊ฒฝ์ฐ:
```bash
.env/Scripts/activate
```
์ด์ ๐ค Transformers๋ฅผ ์ค์นํ ์ค๋น๊ฐ ๋์์ต๋๋ค. ๋ค์ ๋ช
๋ น์ ์
๋ ฅํด์ฃผ์ธ์.
```bash
pip install transformers
```
CPU๋ง ์จ๋ ๋๋ค๋ฉด, ๐ค Transformers์ ๋ฅ๋ฌ๋ ๋ผ์ด๋ธ๋ฌ๋ฆฌ๋ฅผ ๋จ 1์ค๋ก ์ค์นํ ์ ์์ต๋๋ค. ์๋ฅผ ๋ค์ด ๐ค Transformers์ PyTorch์ ๊ฒฝ์ฐ:
```bash
pip install transformers[torch]
```
๐ค Transformers์ TensorFlow 2.0์ ๊ฒฝ์ฐ:
```bash
pip install transformers[tf-cpu]
```
๐ค Transformers์ Flax์ ๊ฒฝ์ฐ:
```bash
pip install transformers[flax]
```
๋ง์ง๋ง์ผ๋ก ๐ค Transformers๊ฐ ์ ๋๋ก ์ค์น๋์๋์ง ํ์ธํ ์ฐจ๋ก์
๋๋ค. ์ฌ์ ํ๋ จ๋ ๋ชจ๋ธ์ ๋ค์ด๋ก๋ํ๋ ์ฝ๋์
๋๋ค.
```bash
python -c "from transformers import pipeline; print(pipeline('sentiment-analysis')('we love you'))"
```
๋ผ๋ฒจ๊ณผ ์ ์๊ฐ ์ถ๋ ฅ๋๋ฉด ์ ์ค์น๋ ๊ฒ์
๋๋ค.
```bash
[{'label': 'POSITIVE', 'score': 0.9998704791069031}]
```
## ์์ค์์ ์ค์นํ๊ธฐ[[install-from-source]]
๐ค Transformers๋ฅผ ์์ค์์ ์ค์นํ๋ ค๋ฉด ์๋ ๋ช
๋ น์ ์คํํ์ธ์.
```bash
pip install git+https://github.com/huggingface/transformers
```
์ ๋ช
๋ น์ ์ต์ ์ด์ง๋ง (์์ ์ ์ธ) `stable` ๋ฒ์ ์ด ์๋ ์คํ์ฑ์ด ์ง์ `main` ๋ฒ์ ์ ์ค์นํฉ๋๋ค. `main` ๋ฒ์ ์ ๊ฐ๋ฐ ํํฉ๊ณผ ๋ฐ๋ง์ถ๋๋ฐ ์ ์ฉํฉ๋๋ค. ์์๋ก ๋ง์ง๋ง ๊ณต์ ๋ฆด๋ฆฌ์ค ์ดํ ๋ฐ๊ฒฌ๋ ๋ฒ๊ทธ๊ฐ ํจ์น๋์์ง๋ง, ์ ๋ฆด๋ฆฌ์ค๋ก ์์ง ๋กค์์๋์ง๋ ์์ ๊ฒฝ์ฐ๋ฅผ ๋ค ์ ์์ต๋๋ค. ๋ฐ๊ฟ ๋งํ๋ฉด `main` ๋ฒ์ ์ด ์์ ์ฑ๊ณผ๋ ๊ฑฐ๋ฆฌ๊ฐ ์๋ค๋ ๋ป์ด๊ธฐ๋ ํฉ๋๋ค. ์ ํฌ๋ `main` ๋ฒ์ ์ ์ฌ์ฉํ๋๋ฐ ๋ฌธ์ ๊ฐ ์๋๋ก ๋
ธ๋ ฅํ๊ณ ์์ผ๋ฉฐ, ๋๋ถ๋ถ์ ๋ฌธ์ ๋ ๋๊ฐ ๋ช ์๊ฐ์ด๋ ํ๋ฃจ ์์ ํด๊ฒฐ๋ฉ๋๋ค. ๋ง์ฝ ๋ฌธ์ ๊ฐ ๋ฐ์ํ๋ฉด [์ด์](https://github.com/huggingface/transformers/issues)๋ฅผ ์ด์ด์ฃผ์๋ฉด ๋ ๋นจ๋ฆฌ ํด๊ฒฐํ ์ ์์ต๋๋ค!
์ ๊ณผ ๋ง์ฐฌ๊ฐ์ง๋ก ๐ค Transformers๊ฐ ์ ๋๋ก ์ค์น๋์๋์ง ํ์ธํ ์ฐจ๋ก์
๋๋ค.
```bash
python -c "from transformers import pipeline; print(pipeline('sentiment-analysis')('I love you'))"
```
## ์์ ๊ฐ๋ฅํ ์ค์น[[editable-install]]
์์ ๊ฐ๋ฅํ ์ค์น๊ฐ ํ์ํ ๊ฒฝ์ฐ๋ ๋ค์๊ณผ ๊ฐ์ต๋๋ค.
* `main` ๋ฒ์ ์ ์์ค ์ฝ๋๋ฅผ ์ฌ์ฉํ๊ธฐ ์ํด
* ๐ค Transformers์ ๊ธฐ์ฌํ๊ณ ์ถ์ด์ ์ฝ๋์ ๋ณ๊ฒฝ ์ฌํญ์ ํ
์คํธํ๊ธฐ ์ํด
๋ฆฌํฌ์งํฐ๋ฆฌ๋ฅผ ๋ณต์ ํ๊ณ ๐ค Transformers๋ฅผ ์ค์นํ๋ ค๋ฉด ๋ค์ ๋ช
๋ น์ ์
๋ ฅํด์ฃผ์ธ์.
```bash
git clone https://github.com/huggingface/transformers.git
cd transformers
pip install -e .
```
์ ๋ช
๋ น์ ๋ฆฌํฌ์งํฐ๋ฆฌ๋ฅผ ๋ณต์ ํ ์์น์ ํด๋์ Python ๋ผ์ด๋ธ๋ฌ๋ฆฌ์ ๊ฒฝ๋ก๋ฅผ ์ฐ๊ฒฐ์ํต๋๋ค. Python์ด ์ผ๋ฐ ๋ผ์ด๋ธ๋ฌ๋ฆฌ ๊ฒฝ๋ก ์ธ์ ๋ณต์ ํ ํด๋ ๋ด๋ถ๋ฅผ ํ์ธํ ๊ฒ์
๋๋ค. ์๋ฅผ ๋ค์ด Python ํจํค์ง๊ฐ ์ผ๋ฐ์ ์ผ๋ก `~/anaconda3/envs/main/lib/python3.7/site-packages/`์ ์ค์น๋์ด ์๋๋ฐ, ๋ช
๋ น์ ๋ฐ์ Python์ด ์ด์ ๋ณต์ ํ ํด๋์ธ `~/transformers/`๋ ๊ฒ์ํ๊ฒ ๋ฉ๋๋ค.
<Tip warning={true}>
๋ผ์ด๋ธ๋ฌ๋ฆฌ๋ฅผ ๊ณ์ ์ฌ์ฉํ๋ ค๋ฉด `transformers` ํด๋๋ฅผ ๊ผญ ์ ์งํด์ผ ํฉ๋๋ค.
</Tip>
๋ณต์ ๋ณธ์ ์ต์ ๋ฒ์ ์ ๐ค Transformers๋ก ์ฝ๊ฒ ์
๋ฐ์ดํธํ ์ ์์ต๋๋ค.
```bash
cd ~/transformers/
git pull
```
Python ํ๊ฒฝ์ ๋ค์ ์คํํ๋ฉด ์
๋ฐ์ดํธ๋ ๐ค Transformers์ `main` ๋ฒ์ ์ ์ฐพ์๋ผ ๊ฒ์
๋๋ค.
## conda๋ก ์ค์นํ๊ธฐ[[install-with-conda]]
`huggingface` conda ์ฑ๋์์ ์ค์นํ ์ ์์ต๋๋ค.
```bash
conda install -c huggingface transformers
```
## ์บ์ ๊ตฌ์ฑํ๊ธฐ[[cache-setup]]
์ฌ์ ํ๋ จ๋ ๋ชจ๋ธ์ ๋ค์ด๋ก๋๋ ํ ๋ก์ปฌ ๊ฒฝ๋ก `~/.cache/huggingface/hub`์ ์บ์๋ฉ๋๋ค. ์
ธ ํ๊ฒฝ ๋ณ์ `TRANSFORMERS_CACHE`์ ๊ธฐ๋ณธ ๋๋ ํฐ๋ฆฌ์
๋๋ค. Windows์ ๊ฒฝ์ฐ ๊ธฐ๋ณธ ๋๋ ํฐ๋ฆฌ๋ `C:\Users\username\.cache\huggingface\hub`์
๋๋ค. ์๋์ ์
ธ ํ๊ฒฝ ๋ณ์๋ฅผ (์ฐ์ ์์) ์์๋๋ก ๋ณ๊ฒฝํ์ฌ ๋ค๋ฅธ ์บ์ ๋๋ ํ ๋ฆฌ๋ฅผ ์ง์ ํ ์ ์์ต๋๋ค.
1. ์
ธ ํ๊ฒฝ ๋ณ์ (๊ธฐ๋ณธ): `HUGGINGFACE_HUB_CACHE` ๋๋ `TRANSFORMERS_CACHE`
2. ์
ธ ํ๊ฒฝ ๋ณ์: `HF_HOME`
3. ์
ธ ํ๊ฒฝ ๋ณ์: `XDG_CACHE_HOME` + `/huggingface`
<Tip>
๊ณผ๊ฑฐ ๐ค Transformers์์ ์ฐ์๋ ์
ธ ํ๊ฒฝ ๋ณ์ `PYTORCH_TRANSFORMERS_CACHE` ๋๋ `PYTORCH_PRETRAINED_BERT_CACHE`์ด ์ค์ ๋์๋ค๋ฉด, ์
ธ ํ๊ฒฝ ๋ณ์ `TRANSFORMERS_CACHE`์ ์ง์ ํ์ง ์๋ ํ ์ฐ์ ์ฌ์ฉ๋ฉ๋๋ค.
</Tip>
## ์คํ๋ผ์ธ ๋ชจ๋[[offline-mode]]
๐ค Transformers๋ฅผ ๋ก์ปฌ ํ์ผ๋ง ์ฌ์ฉํ๋๋ก ํด์ ๋ฐฉํ๋ฒฝ ๋๋ ์คํ๋ผ์ธ ํ๊ฒฝ์์ ์คํํ ์ ์์ต๋๋ค. ํ์ฑํํ๋ ค๋ฉด `TRANSFORMERS_OFFLINE=1` ํ๊ฒฝ ๋ณ์๋ฅผ ์ค์ ํ์ธ์.
<Tip>
`HF_DATASETS_OFFLINE=1` ํ๊ฒฝ ๋ณ์๋ฅผ ์ค์ ํ์ฌ ์คํ๋ผ์ธ ํ๋ จ ๊ณผ์ ์ [๐ค Datasets](https://huggingface.co/docs/datasets/)์ ์ถ๊ฐํ ์ ์์ต๋๋ค.
</Tip>
์๋ฅผ ๋ค์ด ์ธ๋ถ ๊ธฐ๊ธฐ ์ฌ์ด์ ๋ฐฉํ๋ฒฝ์ ๋ ์ผ๋ฐ ๋คํธ์ํฌ์์ ํ์์ฒ๋ผ ํ๋ก๊ทธ๋จ์ ๋ค์๊ณผ ๊ฐ์ด ์คํํ ์ ์์ต๋๋ค.
```bash
python examples/pytorch/translation/run_translation.py --model_name_or_path t5-small --dataset_name wmt16 --dataset_config ro-en ...
```
์คํ๋ผ์ธ ๊ธฐ๊ธฐ์์ ๋์ผํ ํ๋ก๊ทธ๋จ์ ๋ค์๊ณผ ๊ฐ์ด ์คํํ ์ ์์ต๋๋ค.
```bash
HF_DATASETS_OFFLINE=1 TRANSFORMERS_OFFLINE=1 \
python examples/pytorch/translation/run_translation.py --model_name_or_path t5-small --dataset_name wmt16 --dataset_config ro-en ...
```
์ด์ ์คํฌ๋ฆฝํธ๋ ๋ก์ปฌ ํ์ผ์ ํํด์๋ง ๊ฒ์ํ ๊ฒ์ด๋ฏ๋ก, ์คํฌ๋ฆฝํธ๊ฐ ์ค๋จ๋๊ฑฐ๋ ์๊ฐ์ด ์ด๊ณผ๋ ๋๊น์ง ๋ฉ์ถฐ์์ง ์๊ณ ์ ์คํ๋ ๊ฒ์
๋๋ค.
### ์คํ๋ผ์ธ์ฉ ๋ชจ๋ธ ๋ฐ ํ ํฌ๋์ด์ ๋ง๋ค์ด๋๊ธฐ[[fetch-models-and-tokenizers-to-use-offline]]
Another option for using ๐ค Transformers offline is to download the files ahead of time, and then point to their local path when you need to use them offline. There are three ways to do this:
๐ค Transformers๋ฅผ ์คํ๋ผ์ธ์ผ๋ก ์ฌ์ฉํ๋ ๋ ๋ค๋ฅธ ๋ฐฉ๋ฒ์ ํ์ผ์ ๋ฏธ๋ฆฌ ๋ค์ด๋ก๋ํ ๋ค์, ์คํ๋ผ์ธ์ผ ๋ ์ฌ์ฉํ ๋ก์ปฌ ๊ฒฝ๋ก๋ฅผ ์ง์ ํด๋๋ ๊ฒ์
๋๋ค. 3๊ฐ์ง ์ค ํธํ ๋ฐฉ๋ฒ์ ๊ณ ๋ฅด์ธ์.
* [Model Hub](https://huggingface.co/models)์ UI๋ฅผ ํตํด ํ์ผ์ ๋ค์ด๋ก๋ํ๋ ค๋ฉด โ ์์ด์ฝ์ ํด๋ฆญํ์ธ์.

* [`PreTrainedModel.from_pretrained`]์ [`PreTrainedModel.save_pretrained`] ์ํฌํ๋ก๋ฅผ ํ์ฉํ์ธ์.
1. ๋ฏธ๋ฆฌ [`PreTrainedModel.from_pretrained`]๋ก ํ์ผ์ ๋ค์ด๋ก๋ํด๋์ธ์.
```py
>>> from transformers import AutoTokenizer, AutoModelForSeq2SeqLM
>>> tokenizer = AutoTokenizer.from_pretrained("bigscience/T0_3B")
>>> model = AutoModelForSeq2SeqLM.from_pretrained("bigscience/T0_3B")
```
2. [`PreTrainedModel.save_pretrained`]๋ก ์ง์ ๋ ๊ฒฝ๋ก์ ํ์ผ์ ์ ์ฅํด๋์ธ์.
```py
>>> tokenizer.save_pretrained("./your/path/bigscience_t0")
>>> model.save_pretrained("./your/path/bigscience_t0")
```
3. ์ด์ ์คํ๋ผ์ธ์ผ ๋ [`PreTrainedModel.from_pretrained`]๋ก ์ ์ฅํด๋๋ ํ์ผ์ ์ง์ ๋ ๊ฒฝ๋ก์์ ๋ค์ ๋ถ๋ฌ์ค์ธ์.
```py
>>> tokenizer = AutoTokenizer.from_pretrained("./your/path/bigscience_t0")
>>> model = AutoModel.from_pretrained("./your/path/bigscience_t0")
```
* [huggingface_hub](https://github.com/huggingface/huggingface_hub/tree/main/src/huggingface_hub) ๋ผ์ด๋ธ๋ฌ๋ฆฌ๋ฅผ ํ์ฉํด์ ํ์ผ์ ๋ค์ด๋ก๋ํ์ธ์.
1. ๊ฐ์ํ๊ฒฝ์ `huggingface_hub` ๋ผ์ด๋ธ๋ฌ๋ฆฌ๋ฅผ ์ค์นํ์ธ์.
```bash
python -m pip install huggingface_hub
```
2. [`hf_hub_download`](https://huggingface.co/docs/hub/adding-a-library#download-files-from-the-hub) ํจ์๋ก ํ์ผ์ ํน์ ์์น์ ๋ค์ด๋ก๋ํ ์ ์์ต๋๋ค. ์๋ฅผ ๋ค์ด ์๋ ๋ช
๋ น์ [T0](https://huggingface.co/bigscience/T0_3B) ๋ชจ๋ธ์ `config.json` ํ์ผ์ ์ง์ ๋ ๊ฒฝ๋ก์ ๋ค์ด๋ก๋ํฉ๋๋ค.
```py
>>> from huggingface_hub import hf_hub_download
>>> hf_hub_download(repo_id="bigscience/T0_3B", filename="config.json", cache_dir="./your/path/bigscience_t0")
```
ํ์ผ์ ๋ค์ด๋ก๋ํ๊ณ ๋ก์ปฌ์ ์บ์ ํด๋๊ณ ๋๋ฉด, ๋์ค์ ๋ถ๋ฌ์ ์ฌ์ฉํ ์ ์๋๋ก ๋ก์ปฌ ๊ฒฝ๋ก๋ฅผ ์ง์ ํด๋์ธ์.
```py
>>> from transformers import AutoConfig
>>> config = AutoConfig.from_pretrained("./your/path/bigscience_t0/config.json")
```
<Tip>
Hub์ ์ ์ฅ๋ ํ์ผ์ ๋ค์ด๋ก๋ํ๋ ๋ฐฉ๋ฒ์ ๋ ์์ธํ ์์๋ณด๋ ค๋ฉด [Hub์์ ํ์ผ ๋ค์ด๋ก๋ํ๊ธฐ](https://huggingface.co/docs/hub/how-to-downstream) ์น์
์ ์ฐธ๊ณ ํด์ฃผ์ธ์.
</Tip> | 0 |
hf_public_repos/transformers/docs/source | hf_public_repos/transformers/docs/source/ko/pipeline_webserver.md | <!--โ ๏ธ Note that this file is in Markdown but contain specific syntax for our doc-builder (similar to MDX) that may not be
rendered properly in your Markdown viewer.
-->
# ์น ์๋ฒ๋ฅผ ์ํ ํ์ดํ๋ผ์ธ ์ฌ์ฉํ๊ธฐ[[using_pipelines_for_a_webserver]]
<Tip>
์ถ๋ก ์์ง์ ๋ง๋๋ ๊ฒ์ ๋ณต์กํ ์ฃผ์ ์ด๋ฉฐ, "์ต์ ์" ์๋ฃจ์
์ ๋ฌธ์ ๊ณต๊ฐ์ ๋ฐ๋ผ ๋ฌ๋ผ์ง ๊ฐ๋ฅ์ฑ์ด ๋์ต๋๋ค. CPU ๋๋ GPU๋ฅผ ์ฌ์ฉํ๋์ง์ ๋ฐ๋ผ ๋ค๋ฅด๊ณ ๋ฎ์ ์ง์ฐ ์๊ฐ์ ์ํ๋์ง, ๋์ ์ฒ๋ฆฌ๋์ ์ํ๋์ง, ๋ค์ํ ๋ชจ๋ธ์ ์ง์ํ ์ ์๊ธธ ์ํ๋์ง, ํ๋์ ํน์ ๋ชจ๋ธ์ ๊ณ ๋๋ก ์ต์ ํํ๊ธธ ์ํ๋์ง ๋ฑ์ ๋ฐ๋ผ ๋ฌ๋ผ์ง๋๋ค. ์ด ์ฃผ์ ๋ฅผ ํด๊ฒฐํ๋ ๋ฐฉ๋ฒ์๋ ์ฌ๋ฌ ๊ฐ์ง๊ฐ ์์ผ๋ฏ๋ก, ์ด ์ฅ์์ ์ ์ํ๋ ๊ฒ์ ์ฒ์ ์๋ํด ๋ณด๊ธฐ์ ์ข์ ์ถ๋ฐ์ ์ผ ์๋ ์์ง๋ง, ์ด ์ฅ์ ์ฝ๋ ์ฌ๋ฌ๋ถ์ด ํ์๋ก ํ๋ ์ต์ ์ ์๋ฃจ์
์ ์๋ ์ ์์ต๋๋ค.
</Tip>
ํต์ฌ์ ์ผ๋ก ์ดํดํด์ผ ํ ์ ์ [dataset](pipeline_tutorial#using-pipelines-on-a-dataset)๋ฅผ ๋ค๋ฃฐ ๋์ ๋ง์ฐฌ๊ฐ์ง๋ก ๋ฐ๋ณต์๋ฅผ ์ฌ์ฉ ๊ฐ๋ฅํ๋ค๋ ๊ฒ์
๋๋ค. ์๋ํ๋ฉด, ์น ์๋ฒ๋ ๊ธฐ๋ณธ์ ์ผ๋ก ์์ฒญ์ ๊ธฐ๋ค๋ฆฌ๊ณ ๋ค์ด์ค๋ ๋๋ก ์ฒ๋ฆฌํ๋ ์์คํ
์ด๊ธฐ ๋๋ฌธ์
๋๋ค.
๋ณดํต ์น ์๋ฒ๋ ๋ค์ํ ์์ฒญ์ ๋์์ ๋ค๋ฃจ๊ธฐ ์ํด ๋งค์ฐ ๋ค์คํ๋ ๊ตฌ์กฐ(๋ฉํฐ ์ค๋ ๋ฉ, ๋น๋๊ธฐ ๋ฑ)๋ฅผ ์ง๋๊ณ ์์ต๋๋ค. ๋ฐ๋ฉด์, ํ์ดํ๋ผ์ธ(๋๋ถ๋ถ ํ์ดํ๋ผ์ธ ์์ ์๋ ๋ชจ๋ธ)์ ๋ณ๋ ฌ์ฒ๋ฆฌ์ ๊ทธ๋ค์ง ์ข์ง ์์ต๋๋ค. ์๋ํ๋ฉด ํ์ดํ๋ผ์ธ์ ๋ง์ RAM์ ์ฐจ์งํ๊ธฐ ๋๋ฌธ์
๋๋ค. ๋ฐ๋ผ์, ํ์ดํ๋ผ์ธ์ด ์คํ ์ค์ด๊ฑฐ๋ ๊ณ์ฐ ์ง์ฝ์ ์ธ ์์
์ค์ผ ๋ ๋ชจ๋ ์ฌ์ฉ ๊ฐ๋ฅํ ๋ฆฌ์์ค๋ฅผ ์ ๊ณตํ๋ ๊ฒ์ด ๊ฐ์ฅ ์ข์ต๋๋ค.
์ด ๋ฌธ์ ๋ฅผ ์ฐ๋ฆฌ๋ ์น ์๋ฒ๊ฐ ์์ฒญ์ ๋ฐ๊ณ ๋ณด๋ด๋ ๊ฐ๋ฒผ์ด ๋ถํ๋ฅผ ์ฒ๋ฆฌํ๊ณ , ์ค์ ์์
์ ์ฒ๋ฆฌํ๋ ๋จ์ผ ์ค๋ ๋๋ฅผ ๊ฐ๋ ๋ฐฉ๋ฒ์ผ๋ก ํด๊ฒฐํ ๊ฒ์
๋๋ค. ์ด ์์ ๋ `starlette` ๋ผ์ด๋ธ๋ฌ๋ฆฌ๋ฅผ ์ฌ์ฉํฉ๋๋ค.
์ค์ ํ๋ ์์ํฌ๋ ์ค์ํ์ง ์์ง๋ง, ๋ค๋ฅธ ํ๋ ์์ํฌ๋ฅผ ์ฌ์ฉํ๋ ๊ฒฝ์ฐ ๋์ผํ ํจ๊ณผ๋ฅผ ๋ณด๊ธฐ ์ํด์ ์ฝ๋๋ฅผ ์กฐ์ ํ๊ฑฐ๋ ๋ณ๊ฒฝํด์ผ ํ ์ ์์ต๋๋ค.
`server.py`๋ฅผ ์์ฑํ์ธ์:
```py
from starlette.applications import Starlette
from starlette.responses import JSONResponse
from starlette.routing import Route
from transformers import pipeline
import asyncio
async def homepage(request):
payload = await request.body()
string = payload.decode("utf-8")
response_q = asyncio.Queue()
await request.app.model_queue.put((string, response_q))
output = await response_q.get()
return JSONResponse(output)
async def server_loop(q):
pipe = pipeline(model="bert-base-uncased")
while True:
(string, response_q) = await q.get()
out = pipe(string)
await response_q.put(out)
app = Starlette(
routes=[
Route("/", homepage, methods=["POST"]),
],
)
@app.on_event("startup")
async def startup_event():
q = asyncio.Queue()
app.model_queue = q
asyncio.create_task(server_loop(q))
```
์ด์ ๋ค์ ๋ช
๋ น์ด๋ก ์คํ์ํฌ ์ ์์ต๋๋ค:
```bash
uvicorn server:app
```
์ด์ ์ฟผ๋ฆฌ๋ฅผ ๋ ๋ ค๋ณผ ์ ์์ต๋๋ค:
```bash
curl -X POST -d "test [MASK]" http://localhost:8000/
#[{"score":0.7742936015129089,"token":1012,"token_str":".","sequence":"test."},...]
```
์, ์ด์ ์น ์๋ฒ๋ฅผ ๋ง๋๋ ๋ฐฉ๋ฒ์ ๋ํ ์ข์ ๊ฐ๋
์ ์๊ฒ ๋์์ต๋๋ค!
์ค์ํ ์ ์ ๋ชจ๋ธ์ **ํ ๋ฒ๋ง** ๊ฐ์ ธ์จ๋ค๋ ๊ฒ์
๋๋ค. ๋ฐ๋ผ์ ์น ์๋ฒ์๋ ๋ชจ๋ธ์ ์ฌ๋ณธ์ด ์์ต๋๋ค. ์ด๋ฐ ๋ฐฉ์์ ๋ถํ์ํ RAM์ด ์ฌ์ฉ๋์ง ์์ต๋๋ค. ๊ทธ๋ฐ ๋ค์ ํ ๋ฉ์ปค๋์ฆ์ ์ฌ์ฉํ๋ฉด, ๋ค์๊ณผ ๊ฐ์
๋์ ๋ฐฐ์น๋ฅผ ์ฌ์ฉํ๊ธฐ ์ํด ์ถ๋ก ์ ๋จ๊ณ์ ๋ช ๊ฐ์ ํญ๋ชฉ์ ์ถ์ ํ๋ ๊ฒ๊ณผ ๊ฐ์ ๋ฉ์ง ์์
์ ํ ์ ์์ต๋๋ค:
```py
(string, rq) = await q.get()
strings = []
queues = []
while True:
try:
(string, rq) = await asyncio.wait_for(q.get(), timeout=0.001) # 1ms
except asyncio.exceptions.TimeoutError:
break
strings.append(string)
queues.append(rq)
strings
outs = pipe(strings, batch_size=len(strings))
for rq, out in zip(queues, outs):
await rq.put(out)
```
<Tip warning={true}>
์์ ์ฝ๋๋ฅผ ์๋์ํค๊ธฐ ์ ์ ๋น์ ์ ์์คํ
์์์ด ์ถฉ๋ถํ์ง ํ์ธํ์ธ์!
</Tip>
์ ์๋ ์ฝ๋๋ ๊ฐ๋
์ฑ์ ์ํด ์ต์ ํ๋์์ผ๋ฉฐ, ์ต์์ ์ฝ๋๋ ์๋๋๋ค.
์ฒซ์งธ, ๋ฐฐ์น ํฌ๊ธฐ ์ ํ์ด ์์ผ๋ฉฐ ์ด๋ ์ผ๋ฐ์ ์ผ๋ก ์ข์ ๋ฐฉ์์ด ์๋๋๋ค.
๋์งธ, ๋ชจ๋ ํ ๊ฐ์ ธ์ค๊ธฐ์์ ํ์์์์ด ์ฌ์ค์ ๋๋ฏ๋ก ์ถ๋ก ์ ์คํํ๊ธฐ ์ ์ 1ms๋ณด๋ค ํจ์ฌ ์ค๋ ๊ธฐ๋ค๋ฆด ์ ์์ต๋๋ค(์ฒซ ๋ฒ์งธ ์์ฒญ์ ๊ทธ๋งํผ ์ง์ฐ์ํด).
๋จ์ผ 1ms ๊ธธ์ด์ ๋ฐ๋๋ผ์ธ์ ๋๋ ํธ์ด ๋ ์ข์ต๋๋ค.
์ด ๋ฐฉ์์ ์ฌ์ฉํ๋ฉด ํ๊ฐ ๋น์ด ์์ด๋ ํญ์ 1ms๋ฅผ ๊ธฐ๋ค๋ฆฌ๊ฒ ๋ ๊ฒ์
๋๋ค.
ํ์ ์๋ฌด๊ฒ๋ ์์ ๋ ์ถ๋ก ์ ์ํ๋ ๊ฒฝ์ฐ์๋ ์ต์ ์ ๋ฐฉ๋ฒ์ด ์๋ ์ ์์ต๋๋ค.
ํ์ง๋ง ๋ฐฐ์น ์์
์ด ์ฌ์ฉ๋ก์ ๋ฐ๋ผ ์ ๋ง๋ก ์ค์ํ๋ค๋ฉด ์๋ฏธ๊ฐ ์์ ์๋ ์์ต๋๋ค.
๋ค์ ๋งํ์ง๋ง, ์ต์์ ์๋ฃจ์
์ ์์ต๋๋ค.
## ๊ณ ๋ คํด์ผ ํ ๋ช ๊ฐ์ง ์ฌํญ[[few_things_you_might want_to_consider]]
### ์๋ฌ ํ์ธ[[error_checking]]
ํ๋ก๋์
ํ๊ฒฝ์์๋ ๋ฌธ์ ๊ฐ ๋ฐ์ํ ์ฌ์ง๊ฐ ๋ง์ต๋๋ค.
๋ฉ๋ชจ๋ฆฌ๊ฐ ๋ชจ์๋ผ๊ฑฐ๋, ๊ณต๊ฐ์ด ๋ถ์กฑํ๊ฑฐ๋, ๋ชจ๋ธ์ ๊ฐ์ ธ์ค๋ ๋ฐ์ ์คํจํ๊ฑฐ๋, ์ฟผ๋ฆฌ๊ฐ ์๋ชป๋์๊ฑฐ๋, ์ฟผ๋ฆฌ๋ ์ ํํด๋ ๋ชจ๋ธ ์ค์ ์ด ์๋ชป๋์ด ์คํ์ ์คํจํ๋ ๋ฑ๋ฑ ๋ง์ ๊ฒฝ์ฐ๊ฐ ์กด์ฌํฉ๋๋ค.
์ผ๋ฐ์ ์ผ๋ก ์๋ฒ๊ฐ ์ฌ์ฉ์์๊ฒ ์ค๋ฅ๋ฅผ ์ถ๋ ฅํ๋ ๊ฒ์ด ์ข์ผ๋ฏ๋ก
์ค๋ฅ๋ฅผ ํ์ํ๊ธฐ ์ํด `try...except` ๋ฌธ์ ๋ง์ด ์ถ๊ฐํ๋ ๊ฒ์ด ์ข์ต๋๋ค.
ํ์ง๋ง ๋ณด์ ์ํฉ์ ๋ฐ๋ผ ๋ชจ๋ ์ค๋ฅ๋ฅผ ํ์ํ๋ ๊ฒ์ ๋ณด์์ ์ํํ ์๋ ์๋ค๋ ์ ์ ๋ช
์ฌํด์ผํฉ๋๋ค.
### ์ํท ๋ธ๋ ์ดํน[[circuit_breaking]]
์น ์๋ฒ๋ ์ผ๋ฐ์ ์ผ๋ก ์ํท ๋ธ๋ ์ดํน์ ์ํํ ๋ ๋ ๋์ ์ํฉ์ ์ง๋ฉดํฉ๋๋ค.
์ฆ, ์ด๋ ์๋ฒ๊ฐ ์ฟผ๋ฆฌ๋ฅผ ๋ฌด๊ธฐํ ๊ธฐ๋ค๋ฆฌ๋ ๋์ ๊ณผ๋ถํ ์ํ์ผ ๋ ์ ์ ํ ์ค๋ฅ๋ฅผ ๋ฐํํ๋ ๊ฒ์ ์๋ฏธํฉ๋๋ค.
์๋ฒ๊ฐ ๋งค์ฐ ์ค๋ ์๊ฐ ๋์ ๋๊ธฐํ๊ฑฐ๋ ์ ๋นํ ์๊ฐ์ด ์ง๋ ํ์ 504 ์๋ฌ๋ฅผ ๋ฐํํ๋ ๋์ 503 ์๋ฌ๋ฅผ ๋น ๋ฅด๊ฒ ๋ฐํํ๊ฒ ํ๋ ๊ฒ์
๋๋ค.
์ ์๋ ์ฝ๋์๋ ๋จ์ผ ํ๊ฐ ์์ผ๋ฏ๋ก ๊ตฌํํ๊ธฐ๊ฐ ๋น๊ต์ ์ฝ์ต๋๋ค.
ํ ํฌ๊ธฐ๋ฅผ ํ์ธํ๋ ๊ฒ์ ์น ์๋ฒ๊ฐ ๊ณผ๋ถํ ์ํญ ํ์ ์์ ๋ ์๋ฌ๋ฅผ ๋ฐํํ๊ธฐ ์ํ ๊ฐ์ฅ ๊ธฐ์ด์ ์ธ ์์
์
๋๋ค.
### ๋ฉ์ธ ์ฐ๋ ๋ ์ฐจ๋จ[[blocking_the_main_thread]]
ํ์ฌ PyTorch๋ ๋น๋๊ธฐ ์ฒ๋ฆฌ๋ฅผ ์ง์ํ์ง ์์ผ๋ฉฐ, ์คํ ์ค์๋ ๋ฉ์ธ ์ค๋ ๋๊ฐ ์ฐจ๋จ๋ฉ๋๋ค.
๋ฐ๋ผ์ PyTorch๋ฅผ ๋ณ๋์ ์ค๋ ๋/ํ๋ก์ธ์ค์์ ์คํํ๋๋ก ๊ฐ์ ํ๋ ๊ฒ์ด ์ข์ต๋๋ค.
์ฌ๊ธฐ์๋ ์ด ์์
์ด ์ํ๋์ง ์์์ต๋๋ค. ์๋ํ๋ฉด ์ฝ๋๊ฐ ํจ์ฌ ๋ ๋ณต์กํ๊ธฐ ๋๋ฌธ์
๋๋ค(์ฃผ๋ก ์ค๋ ๋, ๋น๋๊ธฐ ์ฒ๋ฆฌ, ํ๊ฐ ์๋ก ์ ๋ง์ง ์๊ธฐ ๋๋ฌธ์
๋๋ค).
ํ์ง๋ง ๊ถ๊ทน์ ์ผ๋ก๋ ๊ฐ์ ์์
์ ์ํํ๋ ๊ฒ์
๋๋ค.
๋จ์ผ ํญ๋ชฉ์ ์ถ๋ก ์ด ์ค๋ ๊ฑธ๋ฆฐ๋ค๋ฉด (> 1์ด), ๋ฉ์ธ ์ฐ๋ ๋๋ฅผ ์ฐจ๋จํ๋ ๊ฒ์ ์ค์ํ ์ ์์ต๋๋ค. ์๋ํ๋ฉด ์ด ๊ฒฝ์ฐ ์ถ๋ก ์ค ๋ชจ๋ ์ฟผ๋ฆฌ๋ ์ค๋ฅ๋ฅผ ๋ฐ๊ธฐ ์ ์ 1์ด๋ฅผ ๊ธฐ๋ค๋ ค์ผ ํ๊ธฐ ๋๋ฌธ์
๋๋ค.
### ๋์ ๋ฐฐ์น[[dynamic_batching]]
์ผ๋ฐ์ ์ผ๋ก, ๋ฐฐ์น ์ฒ๋ฆฌ๊ฐ 1๊ฐ ํญ๋ชฉ์ ํ ๋ฒ์ ์ ๋ฌํ๋ ๊ฒ์ ๋นํด ๋ฐ๋์ ์ฑ๋ฅ ํฅ์์ด ์๋ ๊ฒ์ ์๋๋๋ค(์์ธํ ๋ด์ฉ์ [`batching details`](./main_classes/pipelines#pipeline-batching)์ ์ฐธ๊ณ ํ์ธ์).
ํ์ง๋ง ์ฌ๋ฐ๋ฅธ ์ค์ ์์ ์ฌ์ฉํ๋ฉด ๋งค์ฐ ํจ๊ณผ์ ์ผ ์ ์์ต๋๋ค.
API์๋ ๊ธฐ๋ณธ์ ์ผ๋ก ์๋ ์ ํ์ ๊ฐ๋ฅ์ฑ์ด ๋งค์ฐ ๋๊ธฐ ๋๋ฌธ์ ๋์ ๋ฐฐ์น ์ฒ๋ฆฌ๊ฐ ์์ต๋๋ค.
ํ์ง๋ง ๋งค์ฐ ํฐ ๋ชจ๋ธ์ธ BLOOM ์ถ๋ก ์ ๊ฒฝ์ฐ ๋์ ๋ฐฐ์น ์ฒ๋ฆฌ๋ ๋ชจ๋ ์ฌ๋์๊ฒ ์ ์ ํ ๊ฒฝํ์ ์ ๊ณตํ๋ ๋ฐ **ํ์**์
๋๋ค.
| 0 |
hf_public_repos/transformers/docs/source | hf_public_repos/transformers/docs/source/ko/_toctree.yml | - sections:
- local: index
title: ๐ค Transformers
- local: quicktour
title: ๋๋ฌ๋ณด๊ธฐ
- local: installation
title: ์ค์น๋ฐฉ๋ฒ
title: ์์ํ๊ธฐ
- sections:
- local: pipeline_tutorial
title: Pipeline์ผ๋ก ์ถ๋ก ํ๊ธฐ
- local: autoclass_tutorial
title: AutoClass๋ก ์ฌ์ ํ์ต๋ ์ธ์คํด์ค ๋ก๋ํ๊ธฐ
- local: preprocessing
title: ๋ฐ์ดํฐ ์ ์ฒ๋ฆฌํ๊ธฐ
- local: training
title: ์ฌ์ ํ์ต๋ ๋ชจ๋ธ ๋ฏธ์ธ ์กฐ์ ํ๊ธฐ
- local: run_scripts
title: ์คํฌ๋ฆฝํธ๋ก ํ์ตํ๊ธฐ
- local: accelerate
title: ๐ค Accelerate๋ก ๋ถ์ฐ ํ์ต ๊ตฌ์ฑํ๊ธฐ
- local: model_sharing
title: ๋ง๋ ๋ชจ๋ธ ๊ณต์ ํ๊ธฐ
title: ํํ ๋ฆฌ์ผ
- sections:
- sections:
- local: tasks/sequence_classification
title: ํ
์คํธ ๋ถ๋ฅ
- local: tasks/token_classification
title: ํ ํฐ ๋ถ๋ฅ
- local: tasks/question_answering
title: ์ง์ ์๋ต(Question Answering)
- local: tasks/language_modeling
title: ์ธ๊ณผ์ ์ธ์ด ๋ชจ๋ธ๋ง(Causal language modeling)
- local: tasks/masked_language_modeling
title: ๋ง์คํน๋ ์ธ์ด ๋ชจ๋ธ๋ง(Masked language modeling)
- local: tasks/translation
title: ๋ฒ์ญ
- local: tasks/summarization
title: ์์ฝ
- local: tasks/multiple_choice
title: ๊ฐ๊ด์ ๋ฌธ์ (Multiple Choice)
title: ์์ฐ์ด์ฒ๋ฆฌ
isExpanded: false
- sections:
- local: in_translation
title: (๋ฒ์ญ์ค) Audio classification
- local: tasks/asr
title: ์๋ ์์ฑ ์ธ์
title: (๋ฒ์ญ์ค) ์ค๋์ค
isExpanded: false
- sections:
- local: tasks/image_classification
title: ์ด๋ฏธ์ง ๋ถ๋ฅ
- local: in_translation
title: (๋ฒ์ญ์ค) Semantic segmentation
- local: tasks/video_classification
title: ์์ ๋ถ๋ฅ
- local: tasks/object_detection
title: ๊ฐ์ฒด ํ์ง
- local: tasks/zero_shot_object_detection
title: ์ ๋ก์ท(zero-shot) ๊ฐ์ฒด ํ์ง
- local: tasks/zero_shot_image_classification
title: ์ ๋ก์ท(zero-shot) ์ด๋ฏธ์ง ๋ถ๋ฅ
- local: tasks/monocular_depth_estimation
title: ๋จ์ผ ์์ ๊ธฐ๋ฐ ๊น์ด ์ถ์
title: (๋ฒ์ญ์ค) ์ปดํจํฐ ๋น์
isExpanded: false
- sections:
- local: tasks/image_captioning
title: ์ด๋ฏธ์ง ์บก์
๋
- local: tasks/document_question_answering
title: ๋ฌธ์ ์ง์ ์๋ต(Document Question Answering)
title: ๋ฉํฐ๋ชจ๋ฌ
isExpanded: false
title: ํ์คํฌ ๊ฐ์ด๋
- sections:
- local: fast_tokenizers
title: ๐ค Tokenizers ๋ผ์ด๋ธ๋ฌ๋ฆฌ์์ ํ ํฌ๋์ด์ ์ฌ์ฉํ๊ธฐ
- local: multilingual
title: ๋ค๊ตญ์ด ๋ชจ๋ธ ์ถ๋ก ํ๊ธฐ
- local: in_translation
title: (๋ฒ์ญ์ค) Customize text generation strategy
- local: create_a_model
title: ๋ชจ๋ธ๋ณ API ์ฌ์ฉํ๊ธฐ
- local: custom_models
title: ์ฌ์ฉ์ ์ ์ ๋ชจ๋ธ ๊ณต์ ํ๊ธฐ
- local: sagemaker
title: Amazon SageMaker์์ ํ์ต ์คํํ๊ธฐ
- local: serialization
title: ONNX๋ก ๋ด๋ณด๋ด๊ธฐ
- local: tflite
title: TFLite๋ก ๋ด๋ณด๋ด๊ธฐ
- local: torchscript
title: TorchScript๋ก ๋ด๋ณด๋ด๊ธฐ
- local: in_translation
title: (๋ฒ์ญ์ค) Benchmarks
- local: in_translation
title: (๋ฒ์ญ์ค) Notebooks with examples
- local: in_translation
title: (๋ฒ์ญ์ค) Community resources
- local: custom_tools
title: ์ฌ์ฉ์ ์ ์ ๋๊ตฌ์ ํ๋กฌํํธ
- local: troubleshooting
title: ๋ฌธ์ ํด๊ฒฐ
title: (๋ฒ์ญ์ค) ๊ฐ๋ฐ์ ๊ฐ์ด๋
- sections:
- local: performance
title: ์ฑ๋ฅ ๋ฐ ํ์ฅ์ฑ
- local: in_translation
title: (๋ฒ์ญ์ค) Training on one GPU
- local: in_translation
title: (๋ฒ์ญ์ค) Training on many GPUs
- local: perf_train_cpu
title: CPU์์ ํ๋ จ
- local: in_translation
title: (๋ฒ์ญ์ค) Training on many CPUs
- local: in_translation
title: (๋ฒ์ญ์ค) Training on TPUs
- local: in_translation
title: (๋ฒ์ญ์ค) Training on TPU with TensorFlow
- local: in_translation
title: (๋ฒ์ญ์ค) Training on Specialized Hardware
- local: perf_infer_cpu
title: CPU๋ก ์ถ๋ก ํ๊ธฐ
- local: in_translation
title: (๋ฒ์ญ์ค) Inference on one GPU
- local: in_translation
title: (๋ฒ์ญ์ค) Inference on many GPUs
- local: in_translation
title: (๋ฒ์ญ์ค) Inference on Specialized Hardware
- local: perf_hardware
title: ํ๋ จ์ฉ ์ฌ์ฉ์ ๋ง์ถคํ ํ๋์จ์ด
- local: in_translation
title: (๋ฒ์ญ์ค) Instantiating a big model
- local: in_translation
title: (๋ฒ์ญ์ค) Debugging
- local: hpo_train
title: Trainer API๋ฅผ ์ฌ์ฉํ ํ์ดํผํ๋ผ๋ฏธํฐ ํ์
- local: tf_xla
title: TensorFlow ๋ชจ๋ธ์ ์ํ XLA ํตํฉ
title: (๋ฒ์ญ์ค) ์ฑ๋ฅ ๋ฐ ํ์ฅ์ฑ
- sections:
- local: in_translation
title: (๋ฒ์ญ์ค) How to contribute to transformers?
- local: in_translation
title: (๋ฒ์ญ์ค) How to add a model to ๐ค Transformers?
- local: in_translation
title: (๋ฒ์ญ์ค) How to convert a ๐ค Transformers model to TensorFlow?
- local: in_translation
title: (๋ฒ์ญ์ค) How to add a pipeline to ๐ค Transformers?
- local: testing
title: ํ
์คํธ
- local: in_translation
title: (๋ฒ์ญ์ค) Checks on a Pull Request
title: (๋ฒ์ญ์ค) ๊ธฐ์ฌํ๊ธฐ
- sections:
- local: in_translation
title: (๋ฒ์ญ์ค) Philosophy
- local: in_translation
title: (๋ฒ์ญ์ค) Glossary
- local: task_summary
title: ๐ค Transformers๋ก ํ ์ ์๋ ์์
- local: tasks_explained
title: ๐ค Transformers๋ก ์์
์ ํด๊ฒฐํ๋ ๋ฐฉ๋ฒ
- local: in_translation
title: (๋ฒ์ญ์ค) The Transformer model family
- local: in_translation
title: (๋ฒ์ญ์ค) Summary of the tokenizers
- local: attention
title: ์ดํ
์
๋งค์ปค๋์ฆ
- local: pad_truncation
title: ํจ๋ฉ๊ณผ ์๋ผ๋ด๊ธฐ
- local: bertology
title: BERTology
- local: perplexity
title: ๊ณ ์ ๊ธธ์ด ๋ชจ๋ธ์ ํํ๋ ์ํฐ(Perplexity)
- local: pipeline_webserver
title: ์ถ๋ก ์น ์๋ฒ๋ฅผ ์ํ ํ์ดํ๋ผ์ธ
title: (๋ฒ์ญ์ค) ๊ฐ๋
๊ฐ์ด๋
- sections:
- sections:
- local: in_translation
title: (๋ฒ์ญ์ค) Auto Classes
- local: in_translation
title: (๋ฒ์ญ์ค) Callbacks
- local: in_translation
title: (๋ฒ์ญ์ค) Configuration
- local: in_translation
title: (๋ฒ์ญ์ค) Data Collator
- local: in_translation
title: (๋ฒ์ญ์ค) Keras callbacks
- local: in_translation
title: (๋ฒ์ญ์ค) Logging
- local: in_translation
title: (๋ฒ์ญ์ค) Models
- local: in_translation
title: (๋ฒ์ญ์ค) Text Generation
- local: in_translation
title: (๋ฒ์ญ์ค) ONNX
- local: in_translation
title: (๋ฒ์ญ์ค) Optimization
- local: in_translation
title: (๋ฒ์ญ์ค) Model outputs
- local: in_translation
title: (๋ฒ์ญ์ค) Pipelines
- local: in_translation
title: (๋ฒ์ญ์ค) Processors
- local: in_translation
title: (๋ฒ์ญ์ค) Quantization
- local: in_translation
title: (๋ฒ์ญ์ค) Tokenizer
- local: in_translation
title: (๋ฒ์ญ์ค) Trainer
- local: in_translation
title: (๋ฒ์ญ์ค) DeepSpeed Integration
- local: in_translation
title: (๋ฒ์ญ์ค) Feature Extractor
- local: in_translation
title: (๋ฒ์ญ์ค) Image Processor
title: (๋ฒ์ญ์ค) ๋ฉ์ธ ํด๋์ค
- sections:
- isExpanded: false
sections:
- local: in_translation
title: (๋ฒ์ญ์ค) ALBERT
- local: in_translation
title: (๋ฒ์ญ์ค) BART
- local: in_translation
title: (๋ฒ์ญ์ค) BARThez
- local: in_translation
title: (๋ฒ์ญ์ค) BARTpho
- local: in_translation
title: (๋ฒ์ญ์ค) BERT
- local: in_translation
title: (๋ฒ์ญ์ค) BertGeneration
- local: in_translation
title: (๋ฒ์ญ์ค) BertJapanese
- local: in_translation
title: (๋ฒ์ญ์ค) Bertweet
- local: in_translation
title: (๋ฒ์ญ์ค) BigBird
- local: in_translation
title: (๋ฒ์ญ์ค) BigBirdPegasus
- local: in_translation
title: (๋ฒ์ญ์ค) BioGpt
- local: in_translation
title: (๋ฒ์ญ์ค) Blenderbot
- local: in_translation
title: (๋ฒ์ญ์ค) Blenderbot Small
- local: in_translation
title: (๋ฒ์ญ์ค) BLOOM
- local: in_translation
title: (๋ฒ์ญ์ค) BORT
- local: in_translation
title: (๋ฒ์ญ์ค) ByT5
- local: in_translation
title: (๋ฒ์ญ์ค) CamemBERT
- local: in_translation
title: (๋ฒ์ญ์ค) CANINE
- local: in_translation
title: (๋ฒ์ญ์ค) CodeGen
- local: in_translation
title: (๋ฒ์ญ์ค) ConvBERT
- local: in_translation
title: (๋ฒ์ญ์ค) CPM
- local: in_translation
title: (๋ฒ์ญ์ค) CPMANT
- local: in_translation
title: (๋ฒ์ญ์ค) CTRL
- local: in_translation
title: (๋ฒ์ญ์ค) DeBERTa
- local: in_translation
title: (๋ฒ์ญ์ค) DeBERTa-v2
- local: in_translation
title: (๋ฒ์ญ์ค) DialoGPT
- local: in_translation
title: (๋ฒ์ญ์ค) DistilBERT
- local: in_translation
title: (๋ฒ์ญ์ค) DPR
- local: in_translation
title: (๋ฒ์ญ์ค) ELECTRA
- local: in_translation
title: (๋ฒ์ญ์ค) Encoder Decoder Models
- local: in_translation
title: (๋ฒ์ญ์ค) ERNIE
- local: in_translation
title: (๋ฒ์ญ์ค) ErnieM
- local: in_translation
title: (๋ฒ์ญ์ค) ESM
- local: in_translation
title: (๋ฒ์ญ์ค) FLAN-T5
- local: in_translation
title: (๋ฒ์ญ์ค) FLAN-UL2
- local: in_translation
title: (๋ฒ์ญ์ค) FlauBERT
- local: in_translation
title: (๋ฒ์ญ์ค) FNet
- local: in_translation
title: (๋ฒ์ญ์ค) FSMT
- local: in_translation
title: (๋ฒ์ญ์ค) Funnel Transformer
- local: in_translation
title: (๋ฒ์ญ์ค) GPT
- local: in_translation
title: (๋ฒ์ญ์ค) GPT Neo
- local: in_translation
title: (๋ฒ์ญ์ค) GPT NeoX
- local: in_translation
title: (๋ฒ์ญ์ค) GPT NeoX Japanese
- local: in_translation
title: (๋ฒ์ญ์ค) GPT-J
- local: in_translation
title: (๋ฒ์ญ์ค) GPT2
- local: in_translation
title: (๋ฒ์ญ์ค) GPTBigCode
- local: in_translation
title: (๋ฒ์ญ์ค) GPTSAN Japanese
- local: in_translation
title: (๋ฒ์ญ์ค) GPTSw3
- local: in_translation
title: (๋ฒ์ญ์ค) HerBERT
- local: in_translation
title: (๋ฒ์ญ์ค) I-BERT
- local: in_translation
title: (๋ฒ์ญ์ค) Jukebox
- local: in_translation
title: (๋ฒ์ญ์ค) LED
- local: in_translation
title: (๋ฒ์ญ์ค) LLaMA
- local: in_translation
title: (๋ฒ์ญ์ค) Longformer
- local: in_translation
title: (๋ฒ์ญ์ค) LongT5
- local: in_translation
title: (๋ฒ์ญ์ค) LUKE
- local: in_translation
title: (๋ฒ์ญ์ค) M2M100
- local: in_translation
title: (๋ฒ์ญ์ค) MarianMT
- local: in_translation
title: (๋ฒ์ญ์ค) MarkupLM
- local: in_translation
title: (๋ฒ์ญ์ค) MBart and MBart-50
- local: in_translation
title: (๋ฒ์ญ์ค) MEGA
- local: in_translation
title: (๋ฒ์ญ์ค) MegatronBERT
- local: in_translation
title: (๋ฒ์ญ์ค) MegatronGPT2
- local: in_translation
title: (๋ฒ์ญ์ค) mLUKE
- local: in_translation
title: (๋ฒ์ญ์ค) MobileBERT
- local: in_translation
title: (๋ฒ์ญ์ค) MPNet
- local: in_translation
title: (๋ฒ์ญ์ค) MT5
- local: in_translation
title: (๋ฒ์ญ์ค) MVP
- local: in_translation
title: (๋ฒ์ญ์ค) NEZHA
- local: in_translation
title: (๋ฒ์ญ์ค) NLLB
- local: in_translation
title: (๋ฒ์ญ์ค) NLLB-MoE
- local: in_translation
title: (๋ฒ์ญ์ค) Nystrรถmformer
- local: in_translation
title: (๋ฒ์ญ์ค) Open-Llama
- local: in_translation
title: (๋ฒ์ญ์ค) OPT
- local: in_translation
title: (๋ฒ์ญ์ค) Pegasus
- local: in_translation
title: (๋ฒ์ญ์ค) PEGASUS-X
- local: in_translation
title: (๋ฒ์ญ์ค) PhoBERT
- local: in_translation
title: (๋ฒ์ญ์ค) PLBart
- local: in_translation
title: (๋ฒ์ญ์ค) ProphetNet
- local: in_translation
title: (๋ฒ์ญ์ค) QDQBert
- local: in_translation
title: (๋ฒ์ญ์ค) RAG
- local: in_translation
title: (๋ฒ์ญ์ค) REALM
- local: in_translation
title: (๋ฒ์ญ์ค) Reformer
- local: in_translation
title: (๋ฒ์ญ์ค) RemBERT
- local: in_translation
title: (๋ฒ์ญ์ค) RetriBERT
- local: in_translation
title: (๋ฒ์ญ์ค) RoBERTa
- local: in_translation
title: (๋ฒ์ญ์ค) RoBERTa-PreLayerNorm
- local: in_translation
title: (๋ฒ์ญ์ค) RoCBert
- local: in_translation
title: (๋ฒ์ญ์ค) RoFormer
- local: in_translation
title: (๋ฒ์ญ์ค) Splinter
- local: in_translation
title: (๋ฒ์ญ์ค) SqueezeBERT
- local: in_translation
title: (๋ฒ์ญ์ค) SwitchTransformers
- local: in_translation
title: (๋ฒ์ญ์ค) T5
- local: in_translation
title: (๋ฒ์ญ์ค) T5v1.1
- local: in_translation
title: (๋ฒ์ญ์ค) TAPEX
- local: in_translation
title: (๋ฒ์ญ์ค) Transformer XL
- local: in_translation
title: (๋ฒ์ญ์ค) UL2
- local: in_translation
title: (๋ฒ์ญ์ค) X-MOD
- local: in_translation
title: (๋ฒ์ญ์ค) XGLM
- local: in_translation
title: (๋ฒ์ญ์ค) XLM
- local: in_translation
title: (๋ฒ์ญ์ค) XLM-ProphetNet
- local: in_translation
title: (๋ฒ์ญ์ค) XLM-RoBERTa
- local: in_translation
title: (๋ฒ์ญ์ค) XLM-RoBERTa-XL
- local: in_translation
title: (๋ฒ์ญ์ค) XLM-V
- local: in_translation
title: (๋ฒ์ญ์ค) XLNet
- local: in_translation
title: (๋ฒ์ญ์ค) YOSO
title: (๋ฒ์ญ์ค) ํ
์คํธ ๋ชจ๋ธ
- isExpanded: false
sections:
- local: in_translation
title: (๋ฒ์ญ์ค) BEiT
- local: in_translation
title: (๋ฒ์ญ์ค) BiT
- local: in_translation
title: (๋ฒ์ญ์ค) Conditional DETR
- local: in_translation
title: (๋ฒ์ญ์ค) ConvNeXT
- local: in_translation
title: (๋ฒ์ญ์ค) ConvNeXTV2
- local: in_translation
title: (๋ฒ์ญ์ค) CvT
- local: in_translation
title: (๋ฒ์ญ์ค) Deformable DETR
- local: in_translation
title: (๋ฒ์ญ์ค) DeiT
- local: in_translation
title: (๋ฒ์ญ์ค) DETA
- local: in_translation
title: (๋ฒ์ญ์ค) DETR
- local: in_translation
title: (๋ฒ์ญ์ค) DiNAT
- local: in_translation
title: (๋ฒ์ญ์ค) DiT
- local: in_translation
title: (๋ฒ์ญ์ค) DPT
- local: in_translation
title: (๋ฒ์ญ์ค) EfficientFormer
- local: in_translation
title: (๋ฒ์ญ์ค) EfficientNet
- local: in_translation
title: (๋ฒ์ญ์ค) FocalNet
- local: in_translation
title: (๋ฒ์ญ์ค) GLPN
- local: in_translation
title: (๋ฒ์ญ์ค) ImageGPT
- local: in_translation
title: (๋ฒ์ญ์ค) LeViT
- local: in_translation
title: (๋ฒ์ญ์ค) Mask2Former
- local: in_translation
title: (๋ฒ์ญ์ค) MaskFormer
- local: in_translation
title: (๋ฒ์ญ์ค) MobileNetV1
- local: in_translation
title: (๋ฒ์ญ์ค) MobileNetV2
- local: in_translation
title: (๋ฒ์ญ์ค) MobileViT
- local: in_translation
title: (๋ฒ์ญ์ค) NAT
- local: in_translation
title: (๋ฒ์ญ์ค) PoolFormer
- local: in_translation
title: (๋ฒ์ญ์ค) RegNet
- local: in_translation
title: (๋ฒ์ญ์ค) ResNet
- local: in_translation
title: (๋ฒ์ญ์ค) SegFormer
- local: in_translation
title: (๋ฒ์ญ์ค) Swin Transformer
- local: in_translation
title: (๋ฒ์ญ์ค) Swin Transformer V2
- local: in_translation
title: (๋ฒ์ญ์ค) Swin2SR
- local: in_translation
title: (๋ฒ์ญ์ค) Table Transformer
- local: in_translation
title: (๋ฒ์ญ์ค) TimeSformer
- local: in_translation
title: (๋ฒ์ญ์ค) UperNet
- local: in_translation
title: (๋ฒ์ญ์ค) VAN
- local: in_translation
title: (๋ฒ์ญ์ค) VideoMAE
- local: in_translation
title: (๋ฒ์ญ์ค) Vision Transformer (ViT)
- local: in_translation
title: (๋ฒ์ญ์ค) ViT Hybrid
- local: in_translation
title: (๋ฒ์ญ์ค) ViTMAE
- local: in_translation
title: (๋ฒ์ญ์ค) ViTMSN
- local: in_translation
title: (๋ฒ์ญ์ค) YOLOS
title: (๋ฒ์ญ์ค) ๋น์ ๋ชจ๋ธ
- isExpanded: false
sections:
- local: in_translation
title: (๋ฒ์ญ์ค) Audio Spectrogram Transformer
- local: in_translation
title: (๋ฒ์ญ์ค) CLAP
- local: in_translation
title: (๋ฒ์ญ์ค) Hubert
- local: in_translation
title: (๋ฒ์ญ์ค) MCTCT
- local: in_translation
title: (๋ฒ์ญ์ค) SEW
- local: in_translation
title: (๋ฒ์ญ์ค) SEW-D
- local: in_translation
title: (๋ฒ์ญ์ค) Speech2Text
- local: in_translation
title: (๋ฒ์ญ์ค) Speech2Text2
- local: in_translation
title: (๋ฒ์ญ์ค) SpeechT5
- local: in_translation
title: (๋ฒ์ญ์ค) UniSpeech
- local: in_translation
title: (๋ฒ์ญ์ค) UniSpeech-SAT
- local: in_translation
title: (๋ฒ์ญ์ค) Wav2Vec2
- local: in_translation
title: (๋ฒ์ญ์ค) Wav2Vec2-Conformer
- local: in_translation
title: (๋ฒ์ญ์ค) Wav2Vec2Phoneme
- local: in_translation
title: (๋ฒ์ญ์ค) WavLM
- local: in_translation
title: (๋ฒ์ญ์ค) Whisper
- local: in_translation
title: (๋ฒ์ญ์ค) XLS-R
- local: in_translation
title: (๋ฒ์ญ์ค) XLSR-Wav2Vec2
title: (๋ฒ์ญ์ค) ์ค๋์ค ๋ชจ๋ธ
- isExpanded: false
sections:
- local: in_translation
title: (๋ฒ์ญ์ค) ALIGN
- local: in_translation
title: (๋ฒ์ญ์ค) AltCLIP
- local: in_translation
title: (๋ฒ์ญ์ค) BLIP
- local: in_translation
title: (๋ฒ์ญ์ค) BLIP-2
- local: in_translation
title: (๋ฒ์ญ์ค) BridgeTower
- local: in_translation
title: (๋ฒ์ญ์ค) Chinese-CLIP
- local: in_translation
title: (๋ฒ์ญ์ค) CLIP
- local: in_translation
title: (๋ฒ์ญ์ค) CLIPSeg
- local: in_translation
title: (๋ฒ์ญ์ค) Data2Vec
- local: in_translation
title: (๋ฒ์ญ์ค) DePlot
- local: in_translation
title: (๋ฒ์ญ์ค) Donut
- local: in_translation
title: (๋ฒ์ญ์ค) FLAVA
- local: in_translation
title: (๋ฒ์ญ์ค) GIT
- local: in_translation
title: (๋ฒ์ญ์ค) GroupViT
- local: in_translation
title: (๋ฒ์ญ์ค) LayoutLM
- local: in_translation
title: (๋ฒ์ญ์ค) LayoutLMV2
- local: in_translation
title: (๋ฒ์ญ์ค) LayoutLMV3
- local: in_translation
title: (๋ฒ์ญ์ค) LayoutXLM
- local: in_translation
title: (๋ฒ์ญ์ค) LiLT
- local: in_translation
title: (๋ฒ์ญ์ค) LXMERT
- local: in_translation
title: (๋ฒ์ญ์ค) MatCha
- local: in_translation
title: (๋ฒ์ญ์ค) MGP-STR
- local: in_translation
title: (๋ฒ์ญ์ค) OneFormer
- local: in_translation
title: (๋ฒ์ญ์ค) OWL-ViT
- local: in_translation
title: (๋ฒ์ญ์ค) Perceiver
- local: in_translation
title: (๋ฒ์ญ์ค) Pix2Struct
- local: in_translation
title: (๋ฒ์ญ์ค) Segment Anything
- local: in_translation
title: (๋ฒ์ญ์ค) Speech Encoder Decoder Models
- local: in_translation
title: (๋ฒ์ญ์ค) TAPAS
- local: in_translation
title: (๋ฒ์ญ์ค) TrOCR
- local: in_translation
title: (๋ฒ์ญ์ค) TVLT
- local: in_translation
title: (๋ฒ์ญ์ค) ViLT
- local: in_translation
title: (๋ฒ์ญ์ค) Vision Encoder Decoder Models
- local: in_translation
title: (๋ฒ์ญ์ค) Vision Text Dual Encoder
- local: in_translation
title: (๋ฒ์ญ์ค) VisualBERT
- local: in_translation
title: (๋ฒ์ญ์ค) X-CLIP
title: (๋ฒ์ญ์ค) ๋ฉํฐ๋ชจ๋ฌ ๋ชจ๋ธ
- isExpanded: false
sections:
- local: in_translation
title: (๋ฒ์ญ์ค) Decision Transformer
- local: in_translation
title: (๋ฒ์ญ์ค) Trajectory Transformer
title: (๋ฒ์ญ์ค) ๊ฐํํ์ต ๋ชจ๋ธ
- isExpanded: false
sections:
- local: in_translation
title: (๋ฒ์ญ์ค) Informer
- local: in_translation
title: (๋ฒ์ญ์ค) Time Series Transformer
title: (๋ฒ์ญ์ค) ์๊ณ์ด ๋ชจ๋ธ
- isExpanded: false
sections:
- local: in_translation
title: (๋ฒ์ญ์ค) Graphormer
title: (๋ฒ์ญ์ค) Graph models
title: (๋ฒ์ญ์ค) ๋ชจ๋ธ
- sections:
- local: in_translation
title: (๋ฒ์ญ์ค) Custom Layers and Utilities
- local: in_translation
title: (๋ฒ์ญ์ค) Utilities for pipelines
- local: in_translation
title: (๋ฒ์ญ์ค) Utilities for Tokenizers
- local: in_translation
title: (๋ฒ์ญ์ค) Utilities for Trainer
- local: in_translation
title: (๋ฒ์ญ์ค) Utilities for Generation
- local: in_translation
title: (๋ฒ์ญ์ค) Utilities for Image Processors
- local: in_translation
title: (๋ฒ์ญ์ค) Utilities for Audio processing
- local: in_translation
title: (๋ฒ์ญ์ค) General Utilities
- local: in_translation
title: (๋ฒ์ญ์ค) Utilities for Time Series
title: (๋ฒ์ญ์ค) Internal Helpers
title: (๋ฒ์ญ์ค) API
| 0 |
hf_public_repos/transformers/docs/source | hf_public_repos/transformers/docs/source/ko/multilingual.md | <!--Copyright 2022 The HuggingFace Team. All rights reserved.
Licensed under the Apache License, Version 2.0 (the "License"); you may not use this file except in compliance with
the License. You may obtain a copy of the License at
http://www.apache.org/licenses/LICENSE-2.0
Unless required by applicable law or agreed to in writing, software distributed under the License is distributed on
an "AS IS" BASIS, WITHOUT WARRANTIES OR CONDITIONS OF ANY KIND, either express or implied. See the License for the
specific language governing permissions and limitations under the License.
โ ๏ธ Note that this file is in Markdown but contain specific syntax for our doc-builder (similar to MDX) that may not be
rendered properly in your Markdown viewer.
-->
# ๋ค๊ตญ์ด ๋ชจ๋ธ ์ถ๋ก ํ๊ธฐ[[multilingual-models-for-inference]]
[[open-in-colab]]
๐ค Transformers์๋ ์ฌ๋ฌ ์ข
๋ฅ์ ๋ค๊ตญ์ด(multilingual) ๋ชจ๋ธ์ด ์์ผ๋ฉฐ, ๋จ์ผ ์ธ์ด(monolingual) ๋ชจ๋ธ๊ณผ ์ถ๋ก ์ ์ฌ์ฉ๋ฒ์ด ๋ค๋ฆ
๋๋ค.
๊ทธ๋ ๋ค๊ณ ํด์ *๋ชจ๋ * ๋ค๊ตญ์ด ๋ชจ๋ธ์ ์ฌ์ฉ๋ฒ์ด ๋ค๋ฅธ ๊ฒ์ ์๋๋๋ค.
[bert-base-multilingual-uncased](https://huggingface.co/bert-base-multilingual-uncased)์ ๊ฐ์ ๋ช๋ช ๋ชจ๋ธ์ ๋จ์ผ ์ธ์ด ๋ชจ๋ธ์ฒ๋ผ ์ฌ์ฉํ ์ ์์ต๋๋ค.
์ด๋ฒ ๊ฐ์ด๋์์ ๋ค๊ตญ์ด ๋ชจ๋ธ์ ์ถ๋ก ์ ์ฌ์ฉ ๋ฐฉ๋ฒ์ ์์๋ณผ ๊ฒ์
๋๋ค.
## XLM[[xlm]]
XLM์๋ 10๊ฐ์ง ์ฒดํฌํฌ์ธํธ(checkpoint)๊ฐ ์๋๋ฐ, ์ด ์ค ํ๋๋ง ๋จ์ผ ์ธ์ด์
๋๋ค.
๋๋จธ์ง ์ฒดํฌํฌ์ธํธ 9๊ฐ๋ ์ธ์ด ์๋ฒ ๋ฉ์ ์ฌ์ฉํ๋ ์ฒดํฌํฌ์ธํธ์ ๊ทธ๋ ์ง ์์ ์ฒดํฌํฌ์ธํธ์ ๋ ๊ฐ์ง ๋ฒ์ฃผ๋ก ๋๋ ์ ์์ต๋๋ค.
### ์ธ์ด ์๋ฒ ๋ฉ์ ์ฌ์ฉํ๋ XLM[[xlm-with-language-embeddings]]
๋ค์ XLM ๋ชจ๋ธ์ ์ถ๋ก ์์ ์ธ์ด ์๋ฒ ๋ฉ์ ์ฌ์ฉํฉ๋๋ค:
- `xlm-mlm-ende-1024` (๋ง์คํน๋ ์ธ์ด ๋ชจ๋ธ๋ง, ์์ด-๋
์ผ์ด)
- `xlm-mlm-enfr-1024` (๋ง์คํน๋ ์ธ์ด ๋ชจ๋ธ๋ง, ์์ด-ํ๋์ค์ด)
- `xlm-mlm-enro-1024` (๋ง์คํน๋ ์ธ์ด ๋ชจ๋ธ๋ง, ์์ด-๋ฃจ๋ง๋์์ด)
- `xlm-mlm-xnli15-1024` (๋ง์คํน๋ ์ธ์ด ๋ชจ๋ธ๋ง, XNLI ๋ฐ์ดํฐ ์ธํธ์์ ์ ๊ณตํ๋ 15๊ฐ ๊ตญ์ด)
- `xlm-mlm-tlm-xnli15-1024` (๋ง์คํน๋ ์ธ์ด ๋ชจ๋ธ๋ง + ๋ฒ์ญ, XNLI ๋ฐ์ดํฐ ์ธํธ์์ ์ ๊ณตํ๋ 15๊ฐ ๊ตญ์ด)
- `xlm-clm-enfr-1024` (Causal language modeling, ์์ด-ํ๋์ค์ด)
- `xlm-clm-ende-1024` (Causal language modeling, ์์ด-๋
์ผ์ด)
์ธ์ด ์๋ฒ ๋ฉ์ ๋ชจ๋ธ์ ์ ๋ฌ๋ `input_ids`์ ๋์ผํ shape์ ํ
์๋ก ํํ๋ฉ๋๋ค.
์ด๋ฌํ ํ
์์ ๊ฐ์ ์ฌ์ฉ๋ ์ธ์ด์ ๋ฐ๋ผ ๋ค๋ฅด๋ฉฐ ํ ํฌ๋์ด์ ์ `lang2id` ๋ฐ `id2lang` ์์ฑ์ ์ํด ์๋ณ๋ฉ๋๋ค.
๋ค์ ์์ ์์๋ `xlm-clm-enfr-1024` ์ฒดํฌํฌ์ธํธ(์ฝ์ ์ธ์ด ๋ชจ๋ธ๋ง(causal language modeling), ์์ด-ํ๋์ค์ด)๋ฅผ ๊ฐ์ ธ์ต๋๋ค:
```py
>>> import torch
>>> from transformers import XLMTokenizer, XLMWithLMHeadModel
>>> tokenizer = XLMTokenizer.from_pretrained("xlm-clm-enfr-1024")
>>> model = XLMWithLMHeadModel.from_pretrained("xlm-clm-enfr-1024")
```
ํ ํฌ๋์ด์ ์ `lang2id` ์์ฑ์ ๋ชจ๋ธ์ ์ธ์ด์ ํด๋น ID๋ฅผ ํ์ํฉ๋๋ค:
```py
>>> print(tokenizer.lang2id)
{'en': 0, 'fr': 1}
```
๋ค์์ผ๋ก, ์์ ์
๋ ฅ์ ๋ง๋ญ๋๋ค:
```py
>>> input_ids = torch.tensor([tokenizer.encode("Wikipedia was used to")]) # ๋ฐฐ์น ํฌ๊ธฐ๋ 1์
๋๋ค
```
์ธ์ด ID๋ฅผ `"en"`์ผ๋ก ์ค์ ํด ์ธ์ด ์๋ฒ ๋ฉ์ ์ ์ํฉ๋๋ค.
์ธ์ด ์๋ฒ ๋ฉ์ ์์ด์ ์ธ์ด ID์ธ `0`์ผ๋ก ์ฑ์์ง ํ
์์
๋๋ค.
์ด ํ
์๋ `input_ids`์ ๊ฐ์ ํฌ๊ธฐ์ฌ์ผ ํฉ๋๋ค.
```py
>>> language_id = tokenizer.lang2id["en"] # 0
>>> langs = torch.tensor([language_id] * input_ids.shape[1]) # torch.tensor([0, 0, 0, ..., 0])
>>> # (batch_size, sequence_length) shape์ ํ
์๊ฐ ๋๋๋ก ๋ง๋ญ๋๋ค.
>>> langs = langs.view(1, -1) # ์ด์ [1, sequence_length] shape์ด ๋์์ต๋๋ค(๋ฐฐ์น ํฌ๊ธฐ๋ 1์
๋๋ค)
```
์ด์ `input_ids`์ ์ธ์ด ์๋ฒ ๋ฉ์ ๋ชจ๋ธ๋ก ์ ๋ฌํฉ๋๋ค:
```py
>>> outputs = model(input_ids, langs=langs)
```
[run_generation.py](https://github.com/huggingface/transformers/tree/main/examples/pytorch/text-generation/run_generation.py) ์คํฌ๋ฆฝํธ๋ก `xlm-clm` ์ฒดํฌํฌ์ธํธ๋ฅผ ์ฌ์ฉํด ํ
์คํธ์ ์ธ์ด ์๋ฒ ๋ฉ์ ์์ฑํ ์ ์์ต๋๋ค.
### ์ธ์ด ์๋ฒ ๋ฉ์ ์ฌ์ฉํ์ง ์๋ XLM[[xlm-without-language-embeddings]]
๋ค์ XLM ๋ชจ๋ธ์ ์ถ๋ก ์์ ์ธ์ด ์๋ฒ ๋ฉ์ด ํ์ํ์ง ์์ต๋๋ค:
- `xlm-mlm-17-1280` (๋ง์คํน๋ ์ธ์ด ๋ชจ๋ธ๋ง, 17๊ฐ ๊ตญ์ด)
- `xlm-mlm-100-1280` (๋ง์คํน๋ ์ธ์ด ๋ชจ๋ธ๋ง, 100๊ฐ ๊ตญ์ด)
์ด์ ์ XLM ์ฒดํฌํฌ์ธํธ์ ๋ฌ๋ฆฌ ์ด ๋ชจ๋ธ์ ์ผ๋ฐ ๋ฌธ์ฅ ํํ์ ์ฌ์ฉ๋ฉ๋๋ค.
## BERT[[bert]]
๋ค์ BERT ๋ชจ๋ธ์ ๋ค๊ตญ์ด ํ์คํฌ์ ์ฌ์ฉํ ์ ์์ต๋๋ค:
- `bert-base-multilingual-uncased` (๋ง์คํน๋ ์ธ์ด ๋ชจ๋ธ๋ง + ๋ค์ ๋ฌธ์ฅ ์์ธก, 102๊ฐ ๊ตญ์ด)
- `bert-base-multilingual-cased` (๋ง์คํน๋ ์ธ์ด ๋ชจ๋ธ๋ง + ๋ค์ ๋ฌธ์ฅ ์์ธก, 104๊ฐ ๊ตญ์ด)
์ด๋ฌํ ๋ชจ๋ธ์ ์ถ๋ก ์์ ์ธ์ด ์๋ฒ ๋ฉ์ด ํ์ํ์ง ์์ต๋๋ค.
๋ฌธ๋งฅ์์ ์ธ์ด๋ฅผ ์๋ณํ๊ณ , ์๋ณ๋ ์ธ์ด๋ก ์ถ๋ก ํฉ๋๋ค.
## XLM-RoBERTa[[xlmroberta]]
๋ค์ XLM-RoBERTa ๋ํ ๋ค๊ตญ์ด ๋ค๊ตญ์ด ํ์คํฌ์ ์ฌ์ฉํ ์ ์์ต๋๋ค:
- `xlm-roberta-base` (๋ง์คํน๋ ์ธ์ด ๋ชจ๋ธ๋ง, 100๊ฐ ๊ตญ์ด)
- `xlm-roberta-large` (๋ง์คํน๋ ์ธ์ด ๋ชจ๋ธ๋ง, 100๊ฐ ๊ตญ์ด)
XLM-RoBERTa๋ 100๊ฐ ๊ตญ์ด์ ๋ํด ์๋ก ์์ฑ๋๊ณ ์ ์ ๋ 2.5TB ๊ท๋ชจ์ CommonCrawl ๋ฐ์ดํฐ๋ก ํ์ต๋์์ต๋๋ค.
์ด์ ์ ๊ณต๊ฐ๋ mBERT๋ XLM๊ณผ ๊ฐ์ ๋ค๊ตญ์ด ๋ชจ๋ธ์ ๋นํด ๋ถ๋ฅ, ์ํ์ค ๋ผ๋ฒจ๋ง, ์ง์ ์๋ต๊ณผ ๊ฐ์ ๋ค์ด์คํธ๋ฆผ(downstream) ์์
์์ ์ด์ ์ด ์์ต๋๋ค.
## M2M100[[m2m100]]
๋ค์ M2M100 ๋ชจ๋ธ ๋ํ ๋ค๊ตญ์ด ๋ค๊ตญ์ด ํ์คํฌ์ ์ฌ์ฉํ ์ ์์ต๋๋ค:
- `facebook/m2m100_418M` (๋ฒ์ญ)
- `facebook/m2m100_1.2B` (๋ฒ์ญ)
์ด ์์ ์์๋ `facebook/m2m100_418M` ์ฒดํฌํฌ์ธํธ๋ฅผ ๊ฐ์ ธ์์ ์ค๊ตญ์ด๋ฅผ ์์ด๋ก ๋ฒ์ญํฉ๋๋ค.
ํ ํฌ๋์ด์ ์์ ๋ฒ์ญ ๋์ ์ธ์ด(source language)๋ฅผ ์ค์ ํ ์ ์์ต๋๋ค:
```py
>>> from transformers import M2M100ForConditionalGeneration, M2M100Tokenizer
>>> en_text = "Do not meddle in the affairs of wizards, for they are subtle and quick to anger."
>>> chinese_text = "ไธ่ฆๆๆๅทซๅธซ็ไบๅ, ๅ ็บไปๅๆฏๅพฎๅฆ็, ๅพๅฟซๅฐฑๆ็ผๆ."
>>> tokenizer = M2M100Tokenizer.from_pretrained("facebook/m2m100_418M", src_lang="zh")
>>> model = M2M100ForConditionalGeneration.from_pretrained("facebook/m2m100_418M")
```
๋ฌธ์ฅ์ ํ ํฐํํฉ๋๋ค:
```py
>>> encoded_zh = tokenizer(chinese_text, return_tensors="pt")
```
M2M100์ ๋ฒ์ญ์ ์งํํ๊ธฐ ์ํด ์ฒซ ๋ฒ์งธ๋ก ์์ฑ๋๋ ํ ํฐ์ ๋ฒ์ญํ ์ธ์ด(target language) ID๋ก ๊ฐ์ ์ง์ ํฉ๋๋ค.
์์ด๋ก ๋ฒ์ญํ๊ธฐ ์ํด `generate` ๋ฉ์๋์์ `forced_bos_token_id`๋ฅผ `en`์ผ๋ก ์ค์ ํฉ๋๋ค:
```py
>>> generated_tokens = model.generate(**encoded_zh, forced_bos_token_id=tokenizer.get_lang_id("en"))
>>> tokenizer.batch_decode(generated_tokens, skip_special_tokens=True)
'Do not interfere with the matters of the witches, because they are delicate and will soon be angry.'
```
## MBart[[mbart]]
๋ค์ MBart ๋ชจ๋ธ ๋ํ ๋ค๊ตญ์ด ํ์คํฌ์ ์ฌ์ฉํ ์ ์์ต๋๋ค:
- `facebook/mbart-large-50-one-to-many-mmt` (์ผ๋๋ค ๋ค๊ตญ์ด ๋ฒ์ญ, 50๊ฐ ๊ตญ์ด)
- `facebook/mbart-large-50-many-to-many-mmt` (๋ค๋๋ค ๋ค๊ตญ์ด ๋ฒ์ญ, 50๊ฐ ๊ตญ์ด)
- `facebook/mbart-large-50-many-to-one-mmt` (๋ค๋์ผ ๋ค๊ตญ์ด ๋ฒ์ญ, 50๊ฐ ๊ตญ์ด)
- `facebook/mbart-large-50` (๋ค๊ตญ์ด ๋ฒ์ญ, 50๊ฐ ๊ตญ์ด)
- `facebook/mbart-large-cc25`
์ด ์์ ์์๋ ํ๋๋์ด๋ฅผ ์์ด๋ก ๋ฒ์ญํ๊ธฐ ์ํด `facebook/mbart-large-50-many-to-many-mmt` ์ฒดํฌํฌ์ธํธ๋ฅผ ๊ฐ์ ธ์ต๋๋ค.
ํ ํฌ๋์ด์ ์์ ๋ฒ์ญ ๋์ ์ธ์ด(source language)๋ฅผ ์ค์ ํ ์ ์์ต๋๋ค:
```py
>>> from transformers import AutoTokenizer, AutoModelForSeq2SeqLM
>>> en_text = "Do not meddle in the affairs of wizards, for they are subtle and quick to anger."
>>> fi_text = "รlรค sekaannu velhojen asioihin, sillรค ne ovat hienovaraisia ja nopeasti vihaisia."
>>> tokenizer = AutoTokenizer.from_pretrained("facebook/mbart-large-50-many-to-many-mmt", src_lang="fi_FI")
>>> model = AutoModelForSeq2SeqLM.from_pretrained("facebook/mbart-large-50-many-to-many-mmt")
```
๋ฌธ์ฅ์ ํ ํฐํํฉ๋๋ค:
```py
>>> encoded_en = tokenizer(en_text, return_tensors="pt")
```
MBart๋ ๋ฒ์ญ์ ์งํํ๊ธฐ ์ํด ์ฒซ ๋ฒ์งธ๋ก ์์ฑ๋๋ ํ ํฐ์ ๋ฒ์ญํ ์ธ์ด(target language) ID๋ก ๊ฐ์ ์ง์ ํฉ๋๋ค.
์์ด๋ก ๋ฒ์ญํ๊ธฐ ์ํด `generate` ๋ฉ์๋์์ `forced_bos_token_id`๋ฅผ `en`์ผ๋ก ์ค์ ํฉ๋๋ค:
```py
>>> generated_tokens = model.generate(**encoded_en, forced_bos_token_id=tokenizer.lang_code_to_id("en_XX"))
>>> tokenizer.batch_decode(generated_tokens, skip_special_tokens=True)
"Don't interfere with the wizard's affairs, because they are subtle, will soon get angry."
```
`facebook/mbart-large-50-many-to-one-mmt` ์ฒดํฌํฌ์ธํธ๋ฅผ ์ฌ์ฉํ๊ณ ์๋ค๋ฉด, ์ฒซ ๋ฒ์งธ๋ก ์์ฑ๋๋ ํ ํฐ์ ๋ฒ์ญํ ์ธ์ด(target language) ID๋ก ๊ฐ์ ์ง์ ํ ํ์๋ ์์ต๋๋ค.
| 0 |
hf_public_repos/transformers/docs/source | hf_public_repos/transformers/docs/source/ko/in_translation.md | <!--โ ๏ธ Note that this file is in Markdown but contain specific syntax for our doc-builder (similar to MDX) that may not be
rendered properly in your Markdown viewer.
-->
# ์ด์ฌํ ๋ฒ์ญ ์ค์
๋๋ค. ์กฐ๊ธ ์ด๋ฐ ๋ง๋์! | 0 |
hf_public_repos/transformers/docs/source | hf_public_repos/transformers/docs/source/ko/tasks_explained.md | <!--Copyright 2023 The HuggingFace Team. All rights reserved.
Licensed under the Apache License, Version 2.0 (the "License"); you may not use this file except in compliance with
the License. You may obtain a copy of the License at
http://www.apache.org/licenses/LICENSE-2.0
Unless required by applicable law or agreed to in writing, software distributed under the License is distributed on
an "AS IS" BASIS, WITHOUT WARRANTIES OR CONDITIONS OF ANY KIND, either express or implied. See the License for the
specific language governing permissions and limitations under the License.
โ ๏ธ Note that this file is in Markdown but contain specific syntax for our doc-builder (similar to MDX) that may not be
rendered properly in your Markdown viewer.
-->
# ๐ค Transformers๋ก ์์
์ ํด๊ฒฐํ๋ ๋ฐฉ๋ฒ[[how-transformers-solve-tasks]]
[๐ค Transformers๋ก ํ ์ ์๋ ์์
](task_summary)์์ ์์ฐ์ด ์ฒ๋ฆฌ(NLP), ์์ฑ ๋ฐ ์ค๋์ค, ์ปดํจํฐ ๋น์ ์์
๋ฑ์ ์ค์ํ ์์ฉ์ ๋ฐฐ์ ์ต๋๋ค. ์ด ํ์ด์ง์์๋ ๋ชจ๋ธ์ด ์ด๋ฌํ ์์
์ ์ด๋ป๊ฒ ํด๊ฒฐํ๋์ง ์์ธํ ์ดํด๋ณด๊ณ ๋ด๋ถ์์ ์ด๋ค ์ผ์ด ์ผ์ด๋๋์ง ์ค๋ช
ํฉ๋๋ค. ์ฃผ์ด์ง ์์
์ ํด๊ฒฐํ๋ ๋ง์ ๋ฐฉ๋ฒ์ด ์์ผ๋ฉฐ, ์ผ๋ถ ๋ชจ๋ธ์ ํน์ ๊ธฐ์ ์ ๊ตฌํํ๊ฑฐ๋ ์ฌ์ง์ด ์๋ก์ด ๋ฐฉ์์ผ๋ก ์์
์ ์ ๊ทผํ ์๋ ์์ง๋ง, Transformer ๋ชจ๋ธ์ ๊ฒฝ์ฐ ์ผ๋ฐ์ ์ธ ์์ด๋์ด๋ ๋์ผํฉ๋๋ค. ์ ์ฐํ ์ํคํ
์ฒ ๋๋ถ์ ๋๋ถ๋ถ์ ๋ชจ๋ธ์ ์ธ์ฝ๋, ๋์ฝ๋ ๋๋ ์ธ์ฝ๋-๋์ฝ๋ ๊ตฌ์กฐ์ ๋ณํ์
๋๋ค. Transformer ๋ชจ๋ธ๋ฟ๋ง ์๋๋ผ ์ฐ๋ฆฌ์ ๋ผ์ด๋ธ๋ฌ๋ฆฌ์๋ ์ค๋๋ ์ปดํจํฐ ๋น์ ์์
์ ์ฌ์ฉ๋๋ ๋ช ๊ฐ์ง ํฉ์ฑ๊ณฑ ์ ๊ฒฝ๋ง(CNNs)๋ ์์ต๋๋ค. ๋ํ, ์ฐ๋ฆฌ๋ ํ๋ CNN์ ์๋ ๋ฐฉ์์ ๋ํด ์ค๋ช
ํ ๊ฒ์
๋๋ค.
์์
์ด ์ด๋ป๊ฒ ํด๊ฒฐ๋๋์ง ์ค๋ช
ํ๊ธฐ ์ํด, ์ ์ฉํ ์์ธก์ ์ถ๋ ฅํ๊ณ ์ ๋ชจ๋ธ ๋ด๋ถ์์ ์ด๋ค ์ผ์ด ์ผ์ด๋๋์ง ์ดํด๋ด
๋๋ค.
- ์ค๋์ค ๋ถ๋ฅ ๋ฐ ์๋ ์์ฑ ์ธ์(ASR)์ ์ํ [Wav2Vec2](model_doc/wav2vec2)
- ์ด๋ฏธ์ง ๋ถ๋ฅ๋ฅผ ์ํ [Vision Transformer (ViT)](model_doc/vit) ๋ฐ [ConvNeXT](model_doc/convnext)
- ๊ฐ์ฒด ํ์ง๋ฅผ ์ํ [DETR](model_doc/detr)
- ์ด๋ฏธ์ง ๋ถํ ์ ์ํ [Mask2Former](model_doc/mask2former)
- ๊น์ด ์ถ์ ์ ์ํ [GLPN](model_doc/glpn)
- ์ธ์ฝ๋๋ฅผ ์ฌ์ฉํ๋ ํ
์คํธ ๋ถ๋ฅ, ํ ํฐ ๋ถ๋ฅ ๋ฐ ์ง์์๋ต๊ณผ ๊ฐ์ NLP ์์
์ ์ํ [BERT](model_doc/bert)
- ๋์ฝ๋๋ฅผ ์ฌ์ฉํ๋ ํ
์คํธ ์์ฑ๊ณผ ๊ฐ์ NLP ์์
์ ์ํ [GPT2](model_doc/gpt2)
- ์ธ์ฝ๋-๋์ฝ๋๋ฅผ ์ฌ์ฉํ๋ ์์ฝ ๋ฐ ๋ฒ์ญ๊ณผ ๊ฐ์ NLP ์์
์ ์ํ [BART](model_doc/bart)
<Tip>
๋ ๋์๊ฐ๊ธฐ ์ ์, ๊ธฐ์กด Transformer ์ํคํ
์ฒ์ ๋ํ ๊ธฐ๋ณธ์ ์ธ ์ง์์ ์์งํ๋ ๊ฒ์ด ์ข์ต๋๋ค. ์ธ์ฝ๋, ๋์ฝ๋ ๋ฐ ์ดํ
์
์ ์๋ ๋ฐฉ์์ ์๋ฉด ๋ค์ํ Transformer ๋ชจ๋ธ์ด ์ด๋ป๊ฒ ์๋ํ๋์ง ์ดํดํ๋ ๋ฐ ๋์์ด ๋ฉ๋๋ค. ์์ ๋จ๊ณ๊ฑฐ๋ ๋ณต์ต์ด ํ์ํ ๊ฒฝ์ฐ, ๋ ๋ง์ ์ ๋ณด๋ฅผ ์ํด [์ฝ์ค](https://huggingface.co/course/chapter1/4?fw=pt)๋ฅผ ํ์ธํ์ธ์!
</Tip>
## ์์ฑ ๋ฐ ์ค๋์ค[[speech-and-audio]]
[Wav2Vec2](model_doc/wav2vec2)๋ ๋ ์ด๋ธ์ด ์ง์ ๋์ง ์์ ์์ฑ ๋ฐ์ดํฐ์ ๋ํด ์ฌ์ ํ๋ จ๋ ๋ชจ๋ธ๋ก, ์ค๋์ค ๋ถ๋ฅ ๋ฐ ์๋ ์์ฑ ์ธ์์ ์ํด ๋ ์ด๋ธ์ด ์ง์ ๋ ๋ฐ์ดํฐ๋ก ๋ฏธ์ธ ์กฐ์ ํฉ๋๋ค.
<div class="flex justify-center">
<img src="https://huggingface.co/datasets/huggingface/documentation-images/resolve/main/wav2vec2_architecture.png"/>
</div>
์ด ๋ชจ๋ธ์๋ 4๊ฐ์ง ์ฃผ์ ๊ตฌ์ฑ ์์๊ฐ ์์ต๋๋ค:
1. *ํน์ง ์ธ์ฝ๋(feature encoder)*๋ ์์ ์ค๋์ค ํํ(raw audio waveform)์ ๊ฐ์ ธ์์ ์ ๋ก ํ๊ท ๋ฐ ๋จ์ ๋ถ์ฐ์ผ๋ก ํ์คํํ๊ณ , ๊ฐ๊ฐ 20ms ๊ธธ์ด์ ํน์ง ๋ฒกํฐ์ ์ํ์ค๋ก ๋ณํํฉ๋๋ค.
2. ์ค๋์ค ํํ์ ๋ณธ์ง์ ์ผ๋ก ์ฐ์์ ์ด๊ธฐ ๋๋ฌธ์, ํ
์คํธ ์ํ์ค๋ฅผ ๋จ์ด๋ก ๋๋๋ ๊ฒ๊ณผ ๊ฐ์ด ๋ถํ ํ ์ ์์ต๋๋ค. ๊ทธ๋์ *์์ํ ๋ชจ๋(quantization module)*๋ก ์ ๋ฌ๋๋ ํน์ง ๋ฒกํฐ๋ ์ด์ฐํ ์์ฑ ๋จ์๋ฅผ ํ์ตํ๊ธฐ ์ํ ๊ฒ์
๋๋ค. ์์ฑ ๋จ์๋ *์ฝ๋๋ถ(codebook)*(์ดํ์ง์ด๋ผ๊ณ ์๊ฐํ ์ ์์ต๋๋ค)์ด๋ผ๋ ์ฝ๋๋จ์ด(codewords) ์ฝ๋ ์
์์ ์ ํ๋ฉ๋๋ค. ์ฝ๋๋ถ์์ ์ฐ์์ ์ธ ์ค๋์ค ์
๋ ฅ์ ๊ฐ์ฅ ์ ๋ํ๋ด๋ ๋ฒกํฐ ๋๋ ์์ฑ ๋จ์๊ฐ ์ ํ๋์ด ๋ชจ๋ธ์ ํต๊ณผํฉ๋๋ค.
3. ํน์ง ๋ฒกํฐ์ ์ ๋ฐ์ ๋ฌด์์๋ก ๋ง์คํฌ๊ฐ ์ ์ฉ๋๋ฉฐ, ๋ง์คํฌ๋ ํน์ง ๋ฒกํฐ๋ *์๋์ ์์น ์๋ฒ ๋ฉ*์ ์ถ๊ฐํ๋ Transformer ์ธ์ฝ๋์ธ *๋ฌธ๋งฅ ๋คํธ์ํฌ(context network)*๋ก ์ ๋ฌ๋ฉ๋๋ค.
4. ๋ฌธ๋งฅ ๋คํธ์ํฌ์ ์ฌ์ ํ๋ จ ๋ชฉํ๋ *๋์กฐ์ ์์
(contrastive task)*์
๋๋ค. ๋ชจ๋ธ์ ์๋ชป๋ ์์ธก ์ํ์ค์์ ๋ง์คํฌ๋ ์์ธก์ ์ค์ ์์ํ๋ ์์ฑ ํํ์ ์์ธกํ๋ฉฐ, ๋ชจ๋ธ์ด ๊ฐ์ฅ ์ ์ฌํ ์ปจํ
์คํธ ๋ฒกํฐ์ ์์ํ๋ ์์ฑ ๋จ์(ํ๊ฒ ๋ ์ด๋ธ)๋ฅผ ์ฐพ๋๋ก ๊ถ์ฅํฉ๋๋ค.
์ด์ wav2vec2๊ฐ ์ฌ์ ํ๋ จ๋์์ผ๋ฏ๋ก, ์ค๋์ค ๋ถ๋ฅ ๋๋ ์๋ ์์ฑ ์ธ์์ ์ํด ๋ฐ์ดํฐ์ ๋ง์ถฐ ๋ฏธ์ธ ์กฐ์ ํ ์ ์์ต๋๋ค!
### ์ค๋์ค ๋ถ๋ฅ[[audio-classification]]
์ฌ์ ํ๋ จ๋ ๋ชจ๋ธ์ ์ค๋์ค ๋ถ๋ฅ์ ์ฌ์ฉํ๋ ค๋ฉด, ๊ธฐ๋ณธ Wav2Vec2 ๋ชจ๋ธ ์๋จ์ ์ํ์ค ๋ถ๋ฅ ํค๋๋ฅผ ์ถ๊ฐํ๋ฉด ๋ฉ๋๋ค. ๋ถ๋ฅ ํค๋๋ ์ธ์ฝ๋์ ์๋ ์ํ(hidden states)๋ฅผ ๋ฐ๋ ์ ํ ๋ ์ด์ด์
๋๋ค. ์๋ ์ํ๋ ๊ฐ๊ฐ ๊ธธ์ด๊ฐ ๋ค๋ฅธ ์ค๋์ค ํ๋ ์์์ ํ์ต๋ ํน์ง์ ๋ํ๋
๋๋ค. ๊ณ ์ ๊ธธ์ด์ ๋ฒกํฐ ํ๋๋ฅผ ๋ง๋ค๊ธฐ ์ํด, ์๋ ์ํ๋ ๋จผ์ ํ๋ง๋๊ณ , ํด๋์ค ๋ ์ด๋ธ์ ๋ํ ๋ก์ง์ผ๋ก ๋ณํ๋ฉ๋๋ค. ๊ฐ์ฅ ๊ฐ๋ฅ์ฑ์ด ๋์ ํด๋์ค๋ฅผ ์ฐพ๊ธฐ ์ํด ๋ก์ง๊ณผ ํ๊ฒ ์ฌ์ด์ ๊ต์ฐจ ์ํธ๋กํผ ์์ค์ด ๊ณ์ฐ๋ฉ๋๋ค.
์ค๋์ค ๋ถ๋ฅ์ ์ง์ ๋์ ํ ์ค๋น๊ฐ ๋์
จ๋์? ์์ ํ [์ค๋์ค ๋ถ๋ฅ ๊ฐ์ด๋](tasks/audio_classification)๋ฅผ ํ์ธํ์ฌ Wav2Vec2๋ฅผ ๋ฏธ์ธ ์กฐ์ ํ๊ณ ์ถ๋ก ์ ์ฌ์ฉํ๋ ๋ฐฉ๋ฒ์ ํ์ตํ์ธ์!
### ์๋ ์์ฑ ์ธ์[[automatic-speech-recognition]]
์ฌ์ ํ๋ จ๋ ๋ชจ๋ธ์ ์๋ ์์ฑ ์ธ์์ ์ฌ์ฉํ๋ ค๋ฉด, [์ฐ๊ฒฐ์ฃผ์์ ์๊ฐ ๋ถ๋ฅ(CTC, Connectionist Temporal Classification)](glossary#connectionist-temporal-classification-ctc)๋ฅผ ์ํด ๊ธฐ๋ณธ Wav2Vec2 ๋ชจ๋ธ ์๋จ์ ์ธ์ด ๋ชจ๋ธ๋ง ํค๋๋ฅผ ์ถ๊ฐํฉ๋๋ค. ์ธ์ด ๋ชจ๋ธ๋ง ํค๋๋ ์ธ์ฝ๋์ ์๋ ์ํ๋ฅผ ๋ฐ์์ ๋ก์ง์ผ๋ก ๋ณํํฉ๋๋ค. ๊ฐ ๋ก์ง์ ํ ํฐ ํด๋์ค(ํ ํฐ ์๋ ์์
์ ์ดํ์์ ๋ํ๋ฉ๋๋ค)๋ฅผ ๋ํ๋
๋๋ค. CTC ์์ค์ ํ
์คํธ๋ก ๋์ฝ๋ฉ๋ ํ ํฐ์์ ๊ฐ์ฅ ๊ฐ๋ฅ์ฑ์ด ๋์ ํ ํฐ ์ํ์ค๋ฅผ ์ฐพ๊ธฐ ์ํด ๋ก์ง๊ณผ ํ๊ฒ ์ฌ์ด์์ ๊ณ์ฐ๋ฉ๋๋ค.
์๋ ์์ฑ ์ธ์์ ์ง์ ๋์ ํ ์ค๋น๊ฐ ๋์
จ๋์? ์์ ํ [์๋ ์์ฑ ์ธ์ ๊ฐ์ด๋](tasks/asr)๋ฅผ ํ์ธํ์ฌ Wav2Vec2๋ฅผ ๋ฏธ์ธ ์กฐ์ ํ๊ณ ์ถ๋ก ์ ์ฌ์ฉํ๋ ๋ฐฉ๋ฒ์ ํ์ตํ์ธ์!
## ์ปดํจํฐ ๋น์ [[computer-vision]]
์ปดํจํฐ ๋น์ ์์
์ ์ ๊ทผํ๋ 2๊ฐ์ง ๋ฐฉ๋ฒ์ด ์์ต๋๋ค:
1. ์ด๋ฏธ์ง๋ฅผ ํจ์น ์ํ์ค๋ก ๋ถ๋ฆฌํ๊ณ Transformer๋ก ๋ณ๋ ฌ ์ฒ๋ฆฌํฉ๋๋ค.
2. [ConvNeXT](model_doc/convnext)์ ๊ฐ์ ํ๋ CNN์ ์ฌ์ฉํฉ๋๋ค. ์ด๋ ํฉ์ฑ๊ณฑ ๋ ์ด์ด๋ฅผ ๊ธฐ๋ฐ์ผ๋ก ํ์ง๋ง ํ๋ ๋คํธ์ํฌ ์ค๊ณ๋ฅผ ์ ์ฉํฉ๋๋ค.
<Tip>
์ธ ๋ฒ์งธ ๋ฐฉ๋ฒ์ Transformer์ ํฉ์ฑ๊ณฑ(์๋ฅผ ๋ค์ด, [Convolutional Vision Transformer](model_doc/cvt) ๋๋ [LeViT](model_doc/levit))์ ๊ฒฐํฉํ๋ ๊ฒ์
๋๋ค. ์ฐ๋ฆฌ๋ ์ดํด๋ณผ ๋ ๊ฐ์ง ๋ฐฉ๋ฒ๋ง ๊ฒฐํฉํ๊ธฐ ๋๋ฌธ์ ์ฌ๊ธฐ์ ์ด ๋ฐฉ๋ฒ์ ๋ค๋ฃจ์ง ์์ต๋๋ค.
</Tip>
ViT์ ConvNeXT๋ ์ผ๋ฐ์ ์ผ๋ก ์ด๋ฏธ์ง ๋ถ๋ฅ์์ ์ฌ์ฉ๋์ง๋ง, ๋ฌผ์ฒด ๊ฐ์ง, ๋ถํ , ๊น์ด ์ถ์ ๊ณผ ๊ฐ์ ๋ค๋ฅธ ๋น์ ์์
์๋ ๊ฐ๊ฐ DETR, Mask2Former, GLPN์ด ๋ ์ ํฉํ๋ฏ๋ก ์ด๋ฌํ ๋ชจ๋ธ์ ์ดํด๋ณด๊ฒ ์ต๋๋ค.
### ์ด๋ฏธ์ง ๋ถ๋ฅ[[image-classification]]
ViT์ ConvNeXT ๋ชจ๋ ์ด๋ฏธ์ง ๋ถ๋ฅ์ ์ฌ์ฉ๋ ์ ์์ง๋ง, ViT๋ ์ดํ
์
๋ฉ์ปค๋์ฆ์, ConvNeXT๋ ํฉ์ฑ๊ณฑ์ ์ฌ์ฉํ๋ ๊ฒ์ด ์ฃผ๋ ์ฐจ์ด์
๋๋ค.
#### Transformer[[transformer]]
[ViT](model_doc/vit)์ ํฉ์ฑ๊ณฑ์ ์ ์ ์ผ๋ก ์์ Transformer ์ํคํ
์ฒ๋ก ๋์ฒดํฉ๋๋ค. ๊ธฐ์กด Transformer์ ์ต์ํ๋ค๋ฉด, ViT๋ฅผ ์ดํดํ๋ ๋ฐฉ๋ฒ์ ๋๋ถ๋ถ์ ์ด๋ฏธ ํ์
ํ๋ค๊ณ ๋ณผ ์ ์์ต๋๋ค.
<div class="flex justify-center">
<img src="https://huggingface.co/datasets/huggingface/documentation-images/resolve/main/transformers/model_doc/vit_architecture.jpg"/>
</div>
ViT๊ฐ ๋์
ํ ์ฃผ์ ๋ณ๊ฒฝ ์ฌํญ์ ์ด๋ฏธ์ง๊ฐ Transformer๋ก ์ด๋ป๊ฒ ์ ๋ฌ๋๋์ง์ ์์ต๋๋ค:
1. ์ด๋ฏธ์ง๋ ์๋ก ์ค์ฒฉ๋์ง ์๋ ์ ์ฌ๊ฐํ ํจ์น๋ก ๋ถํ ๋๊ณ , ๊ฐ ํจ์น๋ ๋ฒกํฐ ๋๋ *ํจ์น ์๋ฒ ๋ฉ(patch embedding)*์ผ๋ก ๋ณํ๋ฉ๋๋ค. ํจ์น ์๋ฒ ๋ฉ์ ์ ์ ํ ์
๋ ฅ ์ฐจ์์ ๋ง๋๋ 2D ํฉ์ฑ๊ณฑ ๊ณ์ธต์์ ์์ฑ๋ฉ๋๋ค(๊ธฐ๋ณธ Transformer์ ๊ฒฝ์ฐ ๊ฐ ํจ์น์ ์๋ฒ ๋ฉ๋ง๋ค 768๊ฐ์ ๊ฐ์ด ํ์ํฉ๋๋ค). 224x224 ํฝ์
์ด๋ฏธ์ง๊ฐ ์๋ค๋ฉด, 16x16 ์ด๋ฏธ์ง ํจ์น 196๊ฐ๋ก ๋ถํ ํ ์ ์์ต๋๋ค. ํ
์คํธ๊ฐ ๋จ์ด๋ก ํ ํฐํ๋๋ ๊ฒ์ฒ๋ผ, ์ด๋ฏธ์ง๋ ํจ์น ์ํ์ค๋ก "ํ ํฐํ"๋ฉ๋๋ค.
2. *ํ์ต ๊ฐ๋ฅํ ์๋ฒ ๋ฉ(learnable embedding)*(ํน์ํ `[CLS]` ํ ํฐ)์ด BERT์ ๊ฐ์ด ํจ์น ์๋ฒ ๋ฉ์ ์์ ๋ถ๋ถ์ ์ถ๊ฐ๋ฉ๋๋ค. `[CLS]` ํ ํฐ์ ๋ง์ง๋ง ์๋ ์ํ๋ ๋ถ์ฐฉ๋ ๋ถ๋ฅ ํค๋์ ์
๋ ฅ์ผ๋ก ์ฌ์ฉ๋๊ณ , ๋ค๋ฅธ ์ถ๋ ฅ์ ๋ฌด์๋ฉ๋๋ค. ์ด ํ ํฐ์ ๋ชจ๋ธ์ด ์ด๋ฏธ์ง์ ํํ์ ์ธ์ฝ๋ฉํ๋ ๋ฐฉ๋ฒ์ ํ์ตํ๋ ๋ฐ ๋์์ด ๋ฉ๋๋ค.
3. ํจ์น์ ํ์ต ๊ฐ๋ฅํ ์๋ฒ ๋ฉ์ ๋ง์ง๋ง์ผ๋ก ์ถ๊ฐํ ๊ฒ์ *์์น ์๋ฒ ๋ฉ*์
๋๋ค. ์๋ํ๋ฉด ๋ชจ๋ธ์ ์ด๋ฏธ์ง ํจ์น์ ์์๋ฅผ ๋ชจ๋ฅด๊ธฐ ๋๋ฌธ์
๋๋ค. ์์น ์๋ฒ ๋ฉ๋ ํ์ต ๊ฐ๋ฅํ๋ฉฐ, ํจ์น ์๋ฒ ๋ฉ๊ณผ ๋์ผํ ํฌ๊ธฐ๋ฅผ ๊ฐ์ง๋๋ค. ์ต์ข
์ ์ผ๋ก, ๋ชจ๋ ์๋ฒ ๋ฉ์ด Transformer ์ธ์ฝ๋์ ์ ๋ฌ๋ฉ๋๋ค.
4. `[CLS]` ํ ํฐ์ ํฌํจํ ์ถ๋ ฅ์ ๋ค์ธต ํผ์
ํธ๋ก ํค๋(MLP)์ ์ ๋ฌ๋ฉ๋๋ค. ViT์ ์ฌ์ ํ๋ จ ๋ชฉํ๋ ๋จ์ํ ๋ถ๋ฅ์
๋๋ค. ๋ค๋ฅธ ๋ถ๋ฅ ํค๋์ ๊ฐ์ด, MLP ํค๋๋ ์ถ๋ ฅ์ ํด๋์ค ๋ ์ด๋ธ์ ๋ํด ๋ก์ง์ผ๋ก ๋ณํํ๊ณ ๊ต์ฐจ ์ํธ๋กํผ ์์ค์ ๊ณ์ฐํ์ฌ ๊ฐ์ฅ ๊ฐ๋ฅ์ฑ์ด ๋์ ํด๋์ค๋ฅผ ์ฐพ์ต๋๋ค.
์ด๋ฏธ์ง ๋ถ๋ฅ์ ์ง์ ๋์ ํ ์ค๋น๊ฐ ๋์
จ๋์? ์์ ํ [์ด๋ฏธ์ง ๋ถ๋ฅ ๊ฐ์ด๋](tasks/image_classification)๋ฅผ ํ์ธํ์ฌ ViT๋ฅผ ๋ฏธ์ธ ์กฐ์ ํ๊ณ ์ถ๋ก ์ ์ฌ์ฉํ๋ ๋ฐฉ๋ฒ์ ํ์ตํ์ธ์!
#### CNN[[cnn]]
<Tip>
์ด ์น์
์์๋ ํฉ์ฑ๊ณฑ์ ๋ํด ๊ฐ๋ตํ๊ฒ ์ค๋ช
ํฉ๋๋ค. ๊ทธ๋ฌ๋ ์ด๋ฏธ์ง์ ๋ชจ์๊ณผ ํฌ๊ธฐ๊ฐ ์ด๋ป๊ฒ ๋ณํํ๋์ง์ ๋ํ ์ฌ์ ์ดํด๊ฐ ์๋ค๋ฉด ๋์์ด ๋ ๊ฒ์
๋๋ค. ํฉ์ฑ๊ณฑ์ ์ต์ํ์ง ์์ ๊ฒฝ์ฐ, fastai book์ [ํฉ์ฑ๊ณฑ ์ ๊ฒฝ๋ง ์ฑํฐ](https://github.com/fastai/fastbook/blob/master/13_convolutions.ipynb)๋ฅผ ํ์ธํ์ธ์!
</Tip>
[ConvNeXT](model_doc/convnext)๋ ์ฑ๋ฅ์ ๋์ด๊ธฐ ์ํด ์๋ก์ด ํ๋ ๋คํธ์ํฌ ์ค๊ณ๋ฅผ ์ ์ฉํ CNN ๊ตฌ์กฐ์
๋๋ค. ๊ทธ๋ฌ๋ ํฉ์ฑ๊ณฑ์ ์ฌ์ ํ ๋ชจ๋ธ์ ํต์ฌ์
๋๋ค. ๋์ ์์ค์ ๊ด์ ์์ ๋ณผ ๋, [ํฉ์ฑ๊ณฑ](glossary#convolution)์ ์์ ํ๋ ฌ(*์ปค๋*)์ ์ด๋ฏธ์ง ํฝ์
์ ์์ ์๋์ฐ๋ฅผ ๊ณฑํ๋ ์ฐ์ฐ์
๋๋ค. ์ด๋ ํน์ ํ
์ค์ณ(texture)์ด๋ ์ ์ ๊ณก๋ฅ ๊ณผ ๊ฐ์ ์ผ๋ถ ํน์ง์ ๊ณ์ฐํฉ๋๋ค. ๊ทธ๋ฌ๊ณ ๋ค์ ํฝ์
์๋์ฐ๋ก ๋์ด๊ฐ๋๋ฐ, ์ฌ๊ธฐ์ ํฉ์ฑ๊ณฑ์ด ์ด๋ํ๋ ๊ฑฐ๋ฆฌ๋ฅผ *๋ณดํญ(stride)*์ด๋ผ๊ณ ํฉ๋๋ค.
<div class="flex justify-center">
<img src="https://huggingface.co/datasets/huggingface/documentation-images/resolve/main/convolution.gif"/>
</div>
<small>ํจ๋ฉ์ด๋ ๋ณดํญ์ด ์๋ ๊ธฐ๋ณธ ํฉ์ฑ๊ณฑ, <a href="https://arxiv.org/abs/1603.07285">๋ฅ๋ฌ๋์ ์ํ ํฉ์ฑ๊ณฑ ์ฐ์ฐ ๊ฐ์ด๋</a></small>
์ด ์ถ๋ ฅ์ ๋ค๋ฅธ ํฉ์ฑ๊ณฑ ๋ ์ด์ด์ ์ ๋ฌํ ์ ์์ผ๋ฉฐ, ๊ฐ ์ฐ์์ ์ธ ๋ ์ด์ด๋ฅผ ํตํด ๋คํธ์ํฌ๋ ํซ๋๊ทธ๋ ๋ก์ผ๊ณผ ๊ฐ์ด ๋ ๋ณต์กํ๊ณ ์ถ์์ ์ธ ๊ฒ์ ํ์ตํฉ๋๋ค. ํฉ์ฑ๊ณฑ ๋ ์ด์ด ์ฌ์ด์ ํ๋ง ๋ ์ด์ด๋ฅผ ์ถ๊ฐํ์ฌ ์ฐจ์์ ์ค์ด๊ณ ํน์ง์ ์์น ๋ณํ์ ๋ํด ๋ชจ๋ธ์ ๋ ๊ฒฌ๊ณ ํ๊ฒ ๋ง๋๋ ๊ฒ์ด ์ผ๋ฐ์ ์
๋๋ค.
<div class="flex justify-center">
<img src="https://huggingface.co/datasets/huggingface/documentation-images/resolve/main/convnext_architecture.png"/>
</div>
ConvNeXT๋ CNN์ 5๊ฐ์ง ๋ฐฉ์์ผ๋ก ํ๋ํํฉ๋๋ค:
1. ๊ฐ ๋จ๊ณ์ ๋ธ๋ก ์๋ฅผ ๋ณ๊ฒฝํ๊ณ ๋ ํฐ ๋ณดํญ๊ณผ ๊ทธ์ ๋์ํ๋ ์ปค๋ ํฌ๊ธฐ๋ก ์ด๋ฏธ์ง๋ฅผ "ํจ์นํ(patchify)"ํฉ๋๋ค. ๊ฒน์น์ง ์๋ ์ฌ๋ผ์ด๋ฉ ์๋์ฐ๋ ViT๊ฐ ์ด๋ฏธ์ง๋ฅผ ํจ์น๋ก ๋ถํ ํ๋ ๋ฐฉ๋ฒ๊ณผ ์ ์ฌํ๊ฒ ์ด ํจ์นํ ์ ๋ต์ ๋ง๋ญ๋๋ค.
2. *๋ณ๋ชฉ(bottleneck)* ๋ ์ด์ด๋ ์ฑ๋ ์๋ฅผ ์ค์๋ค๊ฐ ๋ค์ ๋ณต์ํฉ๋๋ค. ์๋ํ๋ฉด 1x1 ํฉ์ฑ๊ณฑ์ ์ํํ๋ ๊ฒ์ด ๋ ๋น ๋ฅด๊ณ , ๊น์ด๋ฅผ ๋๋ฆด ์ ์๊ธฐ ๋๋ฌธ์
๋๋ค. ์ญ ๋ณ๋ชฉ(inverted bottlenect)์ ์ฑ๋ ์๋ฅผ ํ์ฅํ๊ณ ์ถ์ํจ์ผ๋ก์จ ๊ทธ ๋ฐ๋๋ก ์ํํ๋ฏ๋ก, ๋ฉ๋ชจ๋ฆฌ ํจ์จ์ด ๋ ๋์ต๋๋ค.
3. ๋ณ๋ชฉ ๋ ์ด์ด์ ์ผ๋ฐ์ ์ธ 3x3 ํฉ์ฑ๊ณฑ ๋ ์ด์ด๋ฅผ ๊ฐ ์
๋ ฅ ์ฑ๋์ ๊ฐ๋ณ์ ์ผ๋ก ํฉ์ฑ๊ณฑ์ ์ ์ฉํ ๋ค์ ๋ง์ง๋ง์ ์๋ *๊น์ด๋ณ ํฉ์ฑ๊ณฑ(depthwise convolution)*์ผ๋ก ๋์ฒดํฉ๋๋ค. ์ด๋ ๋คํธ์ํฌ ํญ์ด ๋ํ ์ฑ๋ฅ์ด ํฅ์๋ฉ๋๋ค.
4. ViT๋ ์ดํ
์
๋ฉ์ปค๋์ฆ ๋๋ถ์ ํ ๋ฒ์ ๋ ๋ง์ ์ด๋ฏธ์ง๋ฅผ ๋ณผ ์ ์๋ ์ ์ญ ์์ ํ๋๋ฅผ ๊ฐ์ง๊ณ ์์ต๋๋ค. ConvNeXT๋ ์ปค๋ ํฌ๊ธฐ๋ฅผ 7x7๋ก ๋๋ ค ์ด ํจ๊ณผ๋ฅผ ์ฌํํ๋ ค๊ณ ์๋ํฉ๋๋ค.
5. ๋ํ ConvNeXT๋ Transformer ๋ชจ๋ธ์ ๋ชจ๋ฐฉํ๋ ๋ช ๊ฐ์ง ๋ ์ด์ด ์ค๊ณ๋ฅผ ๋ณ๊ฒฝํฉ๋๋ค. ํ์ฑํ ๋ฐ ์ ๊ทํ ๋ ์ด์ด๊ฐ ๋ ์ ๊ณ , ํ์ฑํ ํจ์๊ฐ ReLU ๋์ GELU๋ก ์ ํ๋๊ณ , BatchNorm ๋์ LayerNorm์ ์ฌ์ฉํฉ๋๋ค.
ํฉ์ฑ๊ณฑ ๋ธ๋ก์ ์ถ๋ ฅ์ ๋ถ๋ฅ ํค๋๋ก ์ ๋ฌ๋๋ฉฐ, ๋ถ๋ฅ ํค๋๋ ์ถ๋ ฅ์ ๋ก์ง์ผ๋ก ๋ณํํ๊ณ ๊ต์ฐจ ์ํธ๋กํผ ์์ค์ ๊ณ์ฐํ์ฌ ๊ฐ์ฅ ๊ฐ๋ฅ์ฑ์ด ๋์ ๋ ์ด๋ธ์ ์ฐพ์ต๋๋ค.
### ๊ฐ์ฒด ํ์ง[[object-detection]]
[DETR](model_doc/detr), *DEtection TRansformer*๋ CNN๊ณผ Transformer ์ธ์ฝ๋-๋์ฝ๋๋ฅผ ๊ฒฐํฉํ ์ข
๋จ๊ฐ(end-to-end) ๊ฐ์ฒด ํ์ง ๋ชจ๋ธ์
๋๋ค.
<div class="flex justify-center">
<img src="https://huggingface.co/datasets/huggingface/documentation-images/resolve/main/detr_architecture.png"/>
</div>
1. ์ฌ์ ํ๋ จ๋ CNN *๋ฐฑ๋ณธ(backbone)*์ ํฝ์
๊ฐ์ผ๋ก ๋ํ๋ธ ์ด๋ฏธ์ง๋ฅผ ๊ฐ์ ธ์ ์ ํด์๋ ํน์ง ๋งต์ ๋ง๋ญ๋๋ค. ํน์ง ๋งต์ ๋ํด 1x1 ํฉ์ฑ๊ณฑ์ ์ ์ฉํ์ฌ ์ฐจ์์ ์ค์ด๊ณ , ๊ณ ์์ค ์ด๋ฏธ์ง ํํ์ ๊ฐ์ง ์๋ก์ด ํน์ง ๋งต์ ์์ฑํฉ๋๋ค. Transformer๋ ์ํ์ค ๋ชจ๋ธ์ด๊ธฐ ๋๋ฌธ์ ํน์ง ๋งต์ ์์น ์๋ฒ ๋ฉ๊ณผ ๊ฒฐํฉ๋ ํน์ง ๋ฒกํฐ์ ์ํ์ค๋ก ํํํํฉ๋๋ค.
2. ํน์ง ๋ฒกํฐ๋ ์ดํ
์
๋ ์ด์ด๋ฅผ ์ฌ์ฉํ์ฌ ์ด๋ฏธ์ง ํํ์ ํ์ตํ๋ ์ธ์ฝ๋์ ์ ๋ฌ๋ฉ๋๋ค. ๋ค์์ผ๋ก, ์ธ์ฝ๋์ ์๋ ์ํ๋ ๋์ฝ๋์์ *๊ฐ์ฒด ์ฟผ๋ฆฌ*์ ๊ฒฐํฉ๋ฉ๋๋ค. ๊ฐ์ฒด ์ฟผ๋ฆฌ๋ ์ด๋ฏธ์ง์ ๋ค๋ฅธ ์์ญ์ ์ด์ ์ ๋ง์ถ ํ์ต๋ ์๋ฒ ๋ฉ์ผ๋ก ํ์ต๋๊ณ , ๊ฐ ์ดํ
์
๋ ์ด์ด๋ฅผ ์งํํ๋ฉด์ ๊ฐฑ์ ๋ฉ๋๋ค. ๋์ฝ๋์ ์๋ ์ํ๋ ๊ฐ ๊ฐ์ฒด ์ฟผ๋ฆฌ์ ๋ํ ๋ฐ์ด๋ฉ ๋ฐ์ค ์ขํ์ ํด๋์ค ๋ ์ด๋ธ์ ์์ธกํ๋ ์๋ฐฉํฅ ๋คํธ์ํฌ์ ์ ๋ฌ๋๋ฉฐ, ๊ฐ์ฒด๊ฐ ์๋ ๊ฒฝ์ฐ `no object`๊ฐ ์ถ๋ ฅ๋ฉ๋๋ค.
DETR์ ๊ฐ ๊ฐ์ฒด ์ฟผ๋ฆฌ๋ฅผ ๋ณ๋ ฌ๋ก ๋์ฝ๋ฉํ์ฌ *N* ๊ฐ์ ์ต์ข
์์ธก์ ์ถ๋ ฅํฉ๋๋ค. ์ฌ๊ธฐ์ *N*์ ์ฟผ๋ฆฌ ์์
๋๋ค. ํ ๋ฒ์ ํ๋์ ์์๋ฅผ ์์ธกํ๋ ์ผ๋ฐ์ ์ธ ์๊ธฐํ๊ท ๋ชจ๋ธ๊ณผ ๋ฌ๋ฆฌ, ๊ฐ์ฒด ํ์ง๋ ํ ๋ฒ์ *N* ๊ฐ์ ์์ธก์ ์ํํ๋ ์งํฉ ์์ธก ์์
(`๋ฐ์ด๋ฉ ๋ฐ์ค`, `ํด๋์ค ๋ ์ด๋ธ`)์
๋๋ค.
3. DETR์ ํ๋ จ ์ค *์ด๋ถ ๋งค์นญ ์์ค(bipartite matching loss)*์ ์ฌ์ฉํ์ฌ ๊ณ ์ ๋ ์์ ์์ธก๊ณผ ๊ณ ์ ๋ ์ค์ ์ ๋ต ๋ ์ด๋ธ(ground truth labels) ์ธํธ๋ฅผ ๋น๊ตํฉ๋๋ค. *N*๊ฐ์ ๋ ์ด๋ธ ์ธํธ์ ์ค์ ์ ๋ต ๋ ์ด๋ธ๋ณด๋ค ์ ์ ๊ฒฝ์ฐ, `no object` ํด๋์ค๋ก ํจ๋ฉ๋ฉ๋๋ค. ์ด ์์ค ํจ์๋ DETR์ด ์์ธก๊ณผ ์ค์ ์ ๋ต ๋ ์ด๋ธ ๊ฐ 1:1 ๋์์ ์ฐพ๋๋ก ๊ถ์ฅํฉ๋๋ค. ๋ฐ์ด๋ฉ ๋ฐ์ค ๋๋ ํด๋์ค ๋ ์ด๋ธ ์ค ํ๋๋ผ๋ ์๋ชป๋ ๊ฒฝ์ฐ, ์์ค์ด ๋ฐ์ํฉ๋๋ค. ๋ง์ฐฌ๊ฐ์ง๋ก, ์กด์ฌํ์ง ์๋ ๊ฐ์ฒด๋ฅผ ์์ธกํ๋ ๊ฒฝ์ฐ, ํจ๋ํฐ๋ฅผ ๋ฐ์ต๋๋ค. ์ด๋ก ์ธํด DETR์ ์ด๋ฏธ์ง์์ ๋์ ์ ๋๋ ๋ฌผ์ฒด ํ๋์ ์ง์คํ๋ ๋์ , ๋ค๋ฅธ ๊ฐ์ฒด๋ฅผ ์ฐพ๋๋ก ๊ถ์ฅ๋ฉ๋๋ค.
๊ฐ์ฒด ํ์ง ํค๋๊ฐ DETR ์๋จ์ ์ถ๊ฐ๋์ด ํด๋์ค ๋ ์ด๋ธ๊ณผ ๋ฐ์ด๋ฉ ๋ฐ์ค์ ์ขํ๋ฅผ ์ฐพ์ต๋๋ค. ๊ฐ์ฒด ํ์ง ํค๋์๋ ๋ ๊ฐ์ง ๊ตฌ์ฑ ์์๊ฐ ์์ต๋๋ค: ๋์ฝ๋ ์๋ ์ํ๋ฅผ ํด๋์ค ๋ ์ด๋ธ์ ๋ก์ง์ผ๋ก ๋ณํํ๋ ์ ํ ๋ ์ด์ด ๋ฐ ๋ฐ์ด๋ฉ ๋ฐ์ค๋ฅผ ์์ธกํ๋ MLP
๊ฐ์ฒด ํ์ง์ ์ง์ ๋์ ํ ์ค๋น๊ฐ ๋์
จ๋์? ์์ ํ [๊ฐ์ฒด ํ์ง ๊ฐ์ด๋](tasks/object_detection)๋ฅผ ํ์ธํ์ฌ DETR์ ๋ฏธ์ธ ์กฐ์ ํ๊ณ ์ถ๋ก ์ ์ฌ์ฉํ๋ ๋ฐฉ๋ฒ์ ํ์ตํ์ธ์!
### ์ด๋ฏธ์ง ๋ถํ [[image-segmentation]]
[Mask2Former](model_doc/mask2former)๋ ๋ชจ๋ ์ ํ์ ์ด๋ฏธ์ง ๋ถํ ์์
์ ํด๊ฒฐํ๋ ๋ฒ์ฉ ์ํคํ
์ฒ์
๋๋ค. ์ ํต์ ์ธ ๋ถํ ๋ชจ๋ธ์ ์ผ๋ฐ์ ์ผ๋ก ์๋ฉํฑ(semantic) ๋๋ ํ๋ํฑ(panoptic) ๋ถํ ๊ณผ ๊ฐ์ ์ด๋ฏธ์ง ๋ถํ ์ ํน์ ํ์ ์์
์ ๋ง์ถฐ ์กฐ์ ๋ฉ๋๋ค. Mask2Former๋ ๋ชจ๋ ์์
์ *๋ง์คํฌ ๋ถ๋ฅ* ๋ฌธ์ ๋ก ๊ตฌ์ฑํฉ๋๋ค. ๋ง์คํฌ ๋ถ๋ฅ๋ ํฝ์
์ *N*๊ฐ ์ธ๊ทธ๋จผํธ๋ก ๊ทธ๋ฃนํํ๊ณ , ์ฃผ์ด์ง ์ด๋ฏธ์ง์ ๋ํด *N*๊ฐ์ ๋ง์คํฌ์ ๊ทธ์ ๋์ํ๋ ํด๋์ค ๋ ์ด๋ธ์ ์์ธกํฉ๋๋ค. ์ด ์น์
์์ Mask2Former์ ์๋ ๋ฐฉ๋ฒ์ ์ค๋ช
ํ ๋ค์, ๋ง์ง๋ง์ SegFormer๋ฅผ ๋ฏธ์ธ ์กฐ์ ํด๋ณผ ์ ์์ต๋๋ค.
<div class="flex justify-center">
<img src="https://huggingface.co/datasets/huggingface/documentation-images/resolve/main/mask2former_architecture.png"/>
</div>
Mask2Former์๋ 3๊ฐ์ง ์ฃผ์ ๊ตฌ์ฑ ์์๊ฐ ์์ต๋๋ค:
1. [Swin](model_doc/swin) ๋ฐฑ๋ณธ์ด ์ด๋ฏธ์ง๋ฅผ ๋ฐ์ 3๊ฐ์ ์ฐ์๋ 3x3 ํฉ์ฑ๊ณฑ์์ ์ ํด์๋ ์ด๋ฏธ์ง ํน์ง ๋งต์ ์์ฑํฉ๋๋ค.
2. ํน์ง ๋งต์ *ํฝ์
๋์ฝ๋*์ ์ ๋ฌ๋ฉ๋๋ค. ์ด ๋์ฝ๋๋ ์ ํด์๋ ํน์ง์ ๊ณ ํด์๋ ํฝ์
์๋ฒ ๋ฉ์ผ๋ก ์ ์ง์ ์ผ๋ก ์
์ํ๋งํฉ๋๋ค. ํฝ์
๋์ฝ๋๋ ์ค์ ๋ก ์๋ณธ ์ด๋ฏธ์ง์ 1/32, 1/16, 1/8 ํด์๋์ ๋ค์ค ์ค์ผ์ผ ํน์ง(์ ํด์๋ ๋ฐ ๊ณ ํด์๋ ํน์ง ๋ชจ๋ ํฌํจ)์ ์์ฑํฉ๋๋ค.
3. ์ด๋ฌํ ์๋ก ๋ค๋ฅธ ํฌ๊ธฐ์ ํน์ง ๋งต์ ๊ณ ํด์๋ ํน์ง์์ ์์ ๊ฐ์ฒด๋ฅผ ํฌ์ฐฉํ๊ธฐ ์ํด ํ ๋ฒ์ ํ๋์ Transformer ๋์ฝ๋ ๋ ์ด์ด์ ์ฐ์์ ์ผ๋ก ๊ณต๊ธ๋ฉ๋๋ค. Mask2Former์ ํต์ฌ์ ๋์ฝ๋์ *๋ง์คํฌ ์ดํ
์
* ๋ฉ์ปค๋์ฆ์
๋๋ค. ์ ์ฒด ์ด๋ฏธ์ง๋ฅผ ์ฐธ์กฐํ ์ ์๋ ํฌ๋ก์ค ์ดํ
์
(cross-attention)๊ณผ ๋ฌ๋ฆฌ, ๋ง์คํฌ ์ดํ
์
์ ์ด๋ฏธ์ง์ ํน์ ์์ญ์๋ง ์ง์คํฉ๋๋ค. ์ด๋ ์ด๋ฏธ์ง์ ์ง์ญ์ ํน์ง๋ง์ผ๋ก ๋ชจ๋ธ์ด ์ถฉ๋ถํ ํ์ตํ ์ ์๊ธฐ ๋๋ฌธ์ ๋ ๋น ๋ฅด๊ณ ์ฑ๋ฅ์ด ์ฐ์ํฉ๋๋ค.
4. [DETR](tasks_explained#object-detection)๊ณผ ๊ฐ์ด, Mask2Former๋ ํ์ต๋ ๊ฐ์ฒด ์ฟผ๋ฆฌ๋ฅผ ์ฌ์ฉํ๊ณ ์ด๋ฅผ ํฝ์
๋์ฝ๋์์์ ์ด๋ฏธ์ง ํน์ง๊ณผ ๊ฒฐํฉํ์ฌ ์์ธก ์งํฉ(`ํด๋์ค ๋ ์ด๋ธ`, `๋ง์คํฌ ์์ธก`)์ ์์ฑํฉ๋๋ค. ๋์ฝ๋์ ์๋ ์ํ๋ ์ ํ ๋ ์ด์ด๋ก ์ ๋ฌ๋์ด ํด๋์ค ๋ ์ด๋ธ์ ๋ํ ๋ก์ง์ผ๋ก ๋ณํ๋ฉ๋๋ค. ๋ก์ง๊ณผ ํด๋์ค ๋ ์ด๋ธ ์ฌ์ด์ ๊ต์ฐจ ์ํธ๋กํผ ์์ค์ ๊ณ์ฐํ์ฌ ๊ฐ์ฅ ๊ฐ๋ฅ์ฑ์ด ๋์ ๊ฒ์ ์ฐพ์ต๋๋ค.
๋ง์คํฌ ์์ธก์ ํฝ์
์๋ฒ ๋ฉ๊ณผ ์ต์ข
๋์ฝ๋ ์๋ ์ํ๋ฅผ ๊ฒฐํฉํ์ฌ ์์ฑ๋ฉ๋๋ค. ์๊ทธ๋ชจ์ด๋ ๊ต์ฐจ ์ํธ๋กํผ ๋ฐ Dice ์์ค์ ๋ก์ง๊ณผ ์ค์ ์ ๋ต ๋ง์คํฌ(ground truth mask) ์ฌ์ด์์ ๊ณ์ฐ๋์ด ๊ฐ์ฅ ๊ฐ๋ฅ์ฑ์ด ๋์ ๋ง์คํฌ๋ฅผ ์ฐพ์ต๋๋ค.
์ด๋ฏธ์ง ๋ถํ ์ ์ง์ ๋์ ํ ์ค๋น๊ฐ ๋์
จ๋์? ์์ ํ [์ด๋ฏธ์ง ๋ถํ ๊ฐ์ด๋](tasks/semantic_segmentation)๋ฅผ ํ์ธํ์ฌ SegFormer๋ฅผ ๋ฏธ์ธ ์กฐ์ ํ๊ณ ์ถ๋ก ์ ์ฌ์ฉํ๋ ๋ฐฉ๋ฒ์ ํ์ตํ์ธ์!
### ๊น์ด ์ถ์ [[depth-estimation]]
[GLPN](model_doc/glpn), *Global-Local Path Network*๋ [SegFormer](model_doc/segformer) ์ธ์ฝ๋์ ๊ฒฝ๋ ๋์ฝ๋๋ฅผ ๊ฒฐํฉํ ๊น์ด ์ถ์ ์ ์ํ Transformer์
๋๋ค.
<div class="flex justify-center">
<img src="https://huggingface.co/datasets/huggingface/documentation-images/resolve/main/glpn_architecture.jpg"/>
</div>
1. ViT์ ๊ฐ์ด, ์ด๋ฏธ์ง๋ ํจ์น ์ํ์ค๋ก ๋ถํ ๋์ง๋ง, ์ด๋ฏธ์ง ํจ์น๊ฐ ๋ ์๋ค๋ ์ ์ด ๋ค๋ฆ
๋๋ค. ์ด๋ ์ธ๊ทธ๋ฉํ
์ด์
์ด๋ ๊น์ด ์ถ์ ๊ณผ ๊ฐ์ ๋ฐ๋ ์์ธก ์์
์ ๋ ์ ํฉํฉ๋๋ค. ์ด๋ฏธ์ง ํจ์น๋ ํจ์น ์๋ฒ ๋ฉ์ผ๋ก ๋ณํ๋์ด(ํจ์น ์๋ฒ ๋ฉ์ด ์์ฑ๋๋ ๋ฐฉ๋ฒ์ [์ด๋ฏธ์ง ๋ถ๋ฅ](#image-classification) ์น์
์ ์ฐธ์กฐํ์ธ์), ์ธ์ฝ๋๋ก ์ ๋ฌ๋ฉ๋๋ค.
2. ์ธ์ฝ๋๋ ํจ์น ์๋ฒ ๋ฉ์ ๋ฐ์, ์ฌ๋ฌ ์ธ์ฝ๋ ๋ธ๋ก์ ์ ๋ฌํฉ๋๋ค. ๊ฐ ๋ธ๋ก์ ์ดํ
์
๋ฐ Mix-FFN ๋ ์ด์ด๋ก ๊ตฌ์ฑ๋ฉ๋๋ค. ํ์์ ๋ชฉ์ ์ ์์น ์ ๋ณด๋ฅผ ์ ๊ณตํ๋ ๊ฒ์
๋๋ค. ๊ฐ ์ธ์ฝ๋ ๋ธ๋ก์ ๋์๋ ๊ณ์ธต์ ํํ์ ์์ฑํ๊ธฐ ์ํ *ํจ์น ๋ณํฉ(patch merging)* ๋ ์ด์ด๊ฐ ์์ต๋๋ค. ๊ฐ ์ธ์ ํ ํจ์น ๊ทธ๋ฃน์ ํน์ง์ ์ฐ๊ฒฐ๋๊ณ , ์ฐ๊ฒฐ๋ ํน์ง์ ์ ํ ๋ ์ด์ด๊ฐ ์ ์ฉ๋์ด ํจ์น ์๋ฅผ 1/4์ ํด์๋๋ก ์ค์
๋๋ค. ์ด๋ ๋ค์ ์ธ์ฝ๋ ๋ธ๋ก์ ์
๋ ฅ์ด ๋๋ฉฐ, ์ด๋ฌํ ์ ์ฒด ํ๋ก์ธ์ค๋ 1/8, 1/16, 1/32 ํด์๋์ ์ด๋ฏธ์ง ํน์ง์ ๊ฐ์ง ๋๊น์ง ๋ฐ๋ณต๋ฉ๋๋ค.
3. ๊ฒฝ๋ ๋์ฝ๋๋ ์ธ์ฝ๋์์ ๋ง์ง๋ง ํน์ง ๋งต(1/32 ํฌ๊ธฐ)์ ๊ฐ์ ธ์ 1/16 ํฌ๊ธฐ๋ก ์
์ํ๋งํฉ๋๋ค. ์ฌ๊ธฐ์, ํน์ง์ *์ ํ์ ํน์ง ์ตํฉ(SFF, Selective Feature Fusion)* ๋ชจ๋๋ก ์ ๋ฌ๋ฉ๋๋ค. ์ด ๋ชจ๋์ ๊ฐ ํน์ง์ ๋ํด ์ดํ
์
๋งต์์ ๋ก์ปฌ ๋ฐ ์ ์ญ ํน์ง์ ์ ํํ๊ณ ๊ฒฐํฉํ ๋ค์, 1/8๋ก ์
์ํ๋งํฉ๋๋ค. ์ด ํ๋ก์ธ์ค๋ ๋์ฝ๋ฉ๋ ํน์ฑ์ด ์๋ณธ ์ด๋ฏธ์ง์ ๋์ผํ ํฌ๊ธฐ๊ฐ ๋ ๋๊น์ง ๋ฐ๋ณต๋ฉ๋๋ค. ์ถ๋ ฅ์ ๋ ๊ฐ์ ํฉ์ฑ๊ณฑ ๋ ์ด์ด๋ฅผ ๊ฑฐ์น ๋ค์, ์๊ทธ๋ชจ์ด๋ ํ์ฑํ๊ฐ ์ ์ฉ๋์ด ๊ฐ ํฝ์
์ ๊น์ด๋ฅผ ์์ธกํฉ๋๋ค.
## ์์ฐ์ด์ฒ๋ฆฌ[[natural-language-processing]]
Transformer๋ ์ด๊ธฐ์ ๊ธฐ๊ณ ๋ฒ์ญ์ ์ํด ์ค๊ณ๋์๊ณ , ๊ทธ ์ดํ๋ก๋ ์ฌ์ค์ ๋ชจ๋ NLP ์์
์ ํด๊ฒฐํ๊ธฐ ์ํ ๊ธฐ๋ณธ ์ํคํ
์ฒ๊ฐ ๋์์ต๋๋ค. ์ด๋ค ์์
์ Transformer์ ์ธ์ฝ๋ ๊ตฌ์กฐ์ ์ ํฉํ๋ฉฐ, ๋ค๋ฅธ ์์
์ ๋์ฝ๋์ ๋ ์ ํฉํฉ๋๋ค. ๋ ๋ค๋ฅธ ์์
์ Transformer์ ์ธ์ฝ๋-๋์ฝ๋ ๊ตฌ์กฐ๋ฅผ ๋ชจ๋ ํ์ฉํฉ๋๋ค.
### ํ
์คํธ ๋ถ๋ฅ[[text-classification]]
[BERT](model_doc/bert)๋ ์ธ์ฝ๋ ์ ์ฉ ๋ชจ๋ธ์ด๋ฉฐ, ํ
์คํธ์ ํ๋ถํ ํํ์ ํ์ตํ๊ธฐ ์ํด ์๋ฐฉํฅ์ ๋จ์ด์ ์ฃผ๋ชฉํจ์ผ๋ก์จ ์ฌ์ธต ์๋ฐฉํฅ์ฑ(deep bidirectionality)์ ํจ๊ณผ์ ์ผ๋ก ๊ตฌํํ ์ต์ด์ ๋ชจ๋ธ์
๋๋ค.
1. BERT๋ [WordPiece](tokenizer_summary#wordpiece) ํ ํฐํ๋ฅผ ์ฌ์ฉํ์ฌ ๋ฌธ์ฅ์ ํ ํฐ ์๋ฒ ๋ฉ์ ์์ฑํฉ๋๋ค. ๋จ์ผ ๋ฌธ์ฅ๊ณผ ํ ์์ ๋ฌธ์ฅ์ ๊ตฌ๋ถํ๊ธฐ ์ํด ํน์ํ `[SEP]` ํ ํฐ์ด ์ถ๊ฐ๋ฉ๋๋ค. ๋ชจ๋ ํ
์คํธ ์ํ์ค์ ์์ ๋ถ๋ถ์๋ ํน์ํ `[CLS]` ํ ํฐ์ด ์ถ๊ฐ๋ฉ๋๋ค. `[CLS]` ํ ํฐ์ด ์๋ ์ต์ข
์ถ๋ ฅ์ ๋ถ๋ฅ ์์
์ ์ํ ๋ถ๋ฅ ํค๋๋ก ์
๋ ฅ์ ์ฌ์ฉ๋ฉ๋๋ค. BERT๋ ๋ํ ํ ์์ ๋ฌธ์ฅ์์ ๊ฐ ํ ํฐ์ด ์ฒซ ๋ฒ์งธ ๋ฌธ์ฅ์ธ์ง ๋ ๋ฒ์งธ ๋ฌธ์ฅ์ ์ํ๋์ง ๋ํ๋ด๋ ์ธ๊ทธ๋จผํธ ์๋ฒ ๋ฉ(segment embedding)์ ์ถ๊ฐํฉ๋๋ค.
2. BERT๋ ๋ง์คํฌ๋ ์ธ์ด ๋ชจ๋ธ๋ง๊ณผ ๋ค์ ๋ฌธ์ฅ ์์ธก, ๋ ๊ฐ์ง ๋ชฉ์ ์ผ๋ก ์ฌ์ ํ๋ จ๋ฉ๋๋ค. ๋ง์คํฌ๋ ์ธ์ด ๋ชจ๋ธ๋ง์์๋ ์
๋ ฅ ํ ํฐ์ ์ผ๋ถ๊ฐ ๋ฌด์์๋ก ๋ง์คํน๋๊ณ , ๋ชจ๋ธ์ ์ด๋ฅผ ์์ธกํด์ผ ํฉ๋๋ค. ์ด๋ ๋ชจ๋ธ์ด ๋ชจ๋ ๋จ์ด๋ฅผ ๋ณด๊ณ ๋ค์ ๋จ์ด๋ฅผ "์์ธก"ํ ์ ์๋ ์๋ฐฉํฅ์ฑ ๋ฌธ์ ๋ฅผ ํด๊ฒฐํฉ๋๋ค. ์์ธก๋ ๋ง์คํฌ ํ ํฐ์ ์ต์ข
์๋ ์ํ๋ ์ดํ์ ๋ํ ์ํํธ๋งฅ์ค๊ฐ ์๋ ์๋ฐฉํฅ ๋คํธ์ํฌ๋ก ์ ๋ฌ๋์ด ๋ง์คํฌ๋ ๋จ์ด๋ฅผ ์์ธกํฉ๋๋ค.
๋ ๋ฒ์งธ ์ฌ์ ํ๋ จ ๋์์ ๋ค์ ๋ฌธ์ฅ ์์ธก์
๋๋ค. ๋ชจ๋ธ์ ๋ฌธ์ฅ B๊ฐ ๋ฌธ์ฅ A ๋ค์์ ์ค๋์ง ์์ธกํด์ผ ํฉ๋๋ค. ๋ฌธ์ฅ B๊ฐ ๋ค์ ๋ฌธ์ฅ์ธ ๊ฒฝ์ฐ์ ๋ฌด์์ ๋ฌธ์ฅ์ธ ๊ฒฝ์ฐ ๊ฐ๊ฐ 50%์ ํ๋ฅ ๋ก ๋ฐ์ํฉ๋๋ค. ๋ค์ ๋ฌธ์ฅ์ธ์ง ์๋์ง์ ๋ํ ์์ธก์ ๋ ๊ฐ์ ํด๋์ค(`IsNext` ๋ฐ `NotNext`)์ ๋ํ ์ํํธ๋งฅ์ค๊ฐ ์๋ ์๋ฐฉํฅ ๋คํธ์ํฌ๋ก ์ ๋ฌ๋ฉ๋๋ค.
3. ์
๋ ฅ ์๋ฒ ๋ฉ์ ์ฌ๋ฌ ์ธ์ฝ๋ ๋ ์ด์ด๋ฅผ ๊ฑฐ์ณ์ ์ต์ข
์๋ ์ํ๋ฅผ ์ถ๋ ฅํฉ๋๋ค.
์ฌ์ ํ๋ จ๋ ๋ชจ๋ธ์ ํ
์คํธ ๋ถ๋ฅ์ ์ฌ์ฉํ๋ ค๋ฉด, ๊ธฐ๋ณธ BERT ๋ชจ๋ธ ์๋จ์ ์ํ์ค ๋ถ๋ฅ ํค๋๋ฅผ ์ถ๊ฐํฉ๋๋ค. ์ํ์ค ๋ถ๋ฅ ํค๋๋ ์ต์ข
์๋ ์ํ๋ฅผ ๋ฐ๋ ์ ํ ๋ ์ด์ด์ด๋ฉฐ, ๋ก์ง์ผ๋ก ๋ณํํ๊ธฐ ์ํด ์ ํ ๋ณํ์ ์ํํฉ๋๋ค. ๊ต์ฐจ ์ํธ๋กํผ ์์ค์ ๋ก์ง๊ณผ ํ๊ฒ ๊ฐ์ ๊ณ์ฐ๋์ด ๊ฐ์ฅ ๊ฐ๋ฅ์ฑ์ด ๋์ ๋ ์ด๋ธ์ ์ฐพ์ต๋๋ค.
ํ
์คํธ ๋ถ๋ฅ์ ์ง์ ๋์ ํ ์ค๋น๊ฐ ๋์
จ๋์? ์์ ํ [ํ
์คํธ ๋ถ๋ฅ ๊ฐ์ด๋](tasks/sequence_classification)๋ฅผ ํ์ธํ์ฌ DistilBERT๋ฅผ ๋ฏธ์ธ ์กฐ์ ํ๊ณ ์ถ๋ก ์ ์ฌ์ฉํ๋ ๋ฐฉ๋ฒ์ ํ์ตํ์ธ์!
### ํ ํฐ ๋ถ๋ฅ[[token-classification]]
๊ฐ์ฒด๋ช
์ธ์(Named Entity Recognition, NER)๊ณผ ๊ฐ์ ํ ํฐ ๋ถ๋ฅ ์์
์ BERT๋ฅผ ์ฌ์ฉํ๋ ค๋ฉด, ๊ธฐ๋ณธ BERT ๋ชจ๋ธ ์๋จ์ ํ ํฐ ๋ถ๋ฅ ํค๋๋ฅผ ์ถ๊ฐํฉ๋๋ค. ํ ํฐ ๋ถ๋ฅ ํค๋๋ ์ต์ข
์๋ ์ํ๋ฅผ ๋ฐ๋ ์ ํ ๋ ์ด์ด์ด๋ฉฐ, ๋ก์ง์ผ๋ก ๋ณํํ๊ธฐ ์ํด ์ ํ ๋ณํ์ ์ํํฉ๋๋ค. ๊ต์ฐจ ์ํธ๋กํผ ์์ค์ ๋ก์ง๊ณผ ๊ฐ ํ ํฐ ๊ฐ์ ๊ณ์ฐ๋์ด ๊ฐ์ฅ ๊ฐ๋ฅ์ฑ์ด ๋์ ๋ ์ด๋ธ์ ์ฐพ์ต๋๋ค.
ํ ํฐ ๋ถ๋ฅ์ ์ง์ ๋์ ํ ์ค๋น๊ฐ ๋์
จ๋์? ์์ ํ [ํ ํฐ ๋ถ๋ฅ ๊ฐ์ด๋](tasks/token_classification)๋ฅผ ํ์ธํ์ฌ DistilBERT๋ฅผ ๋ฏธ์ธ ์กฐ์ ํ๊ณ ์ถ๋ก ์ ์ฌ์ฉํ๋ ๋ฐฉ๋ฒ์ ํ์ตํ์ธ์!
### ์ง์์๋ต[[question-answering]]
์ง์์๋ต์ BERT๋ฅผ ์ฌ์ฉํ๋ ค๋ฉด, ๊ธฐ๋ณธ BERT ๋ชจ๋ธ ์์ ์คํฌ(span) ๋ถ๋ฅ ํค๋๋ฅผ ์ถ๊ฐํฉ๋๋ค. ์ด ์ ํ ๋ ์ด์ด๋ ์ต์ข
์๋ ์ํ๋ฅผ ๋ฐ๊ณ , ๋ต๋ณ์ ๋์ํ๋ `์คํฌ`์ ์์๊ณผ ๋ ๋ก๊ทธ๋ฅผ ๊ณ์ฐํ๊ธฐ ์ํด ์ ํ ๋ณํ์ ์ํํฉ๋๋ค. ๊ต์ฐจ ์ํธ๋กํผ ์์ค์ ๋ก์ง๊ณผ ๊ฐ ๋ ์ด๋ธ ์์น ๊ฐ์ ๊ณ์ฐ๋์ด ๋ต๋ณ์ ๋์ํ๋ ๊ฐ์ฅ ๊ฐ๋ฅ์ฑ์ด ๋์ ํ
์คํธ์ ์คํฌ์ ์ฐพ์ต๋๋ค.
์ง์์๋ต์ ์ง์ ๋์ ํ ์ค๋น๊ฐ ๋์
จ๋์? ์์ ํ [์ง์์๋ต ๊ฐ์ด๋](tasks/question_answering)๋ฅผ ํ์ธํ์ฌ DistilBERT๋ฅผ ๋ฏธ์ธ ์กฐ์ ํ๊ณ ์ถ๋ก ์ ์ฌ์ฉํ๋ ๋ฐฉ๋ฒ์ ํ์ตํ์ธ์!
<Tip>
๐ก ์ฌ์ ํ๋ จ๋ BERT๋ฅผ ๋ค์ํ ์์
์ ์ฌ์ฉํ๋ ๊ฒ์ด ์ผ๋ง๋ ์ฌ์ด์ง ์ฃผ๋ชฉํ์ธ์. ์ฌ์ ํ๋ จ๋ ๋ชจ๋ธ์ ํน์ ํค๋๋ฅผ ์ถ๊ฐํ๊ธฐ๋ง ํ๋ฉด ์๋ ์ํ๋ฅผ ์ํ๋ ์ถ๋ ฅ์ผ๋ก ์กฐ์ํ ์ ์์ต๋๋ค!
</Tip>
### ํ
์คํธ ์์ฑ[[text-generation]]
[GPT-2](model_doc/gpt2)๋ ๋๋์ ํ
์คํธ์ ๋ํด ์ฌ์ ํ๋ จ๋ ๋์ฝ๋ฉ ์ ์ฉ ๋ชจ๋ธ์
๋๋ค. ํ๋กฌํํธ๋ฅผ ์ฃผ์ด์ง๋ฉด ์ค๋๋ ฅ ์๋ (ํญ์ ์ฌ์ค์ ์๋์ง๋ง!) ํ
์คํธ๋ฅผ ์์ฑํ๊ณ ๋ช
์์ ์ผ๋ก ํ๋ จ๋์ง ์์์์๋ ๋ถ๊ตฌํ๊ณ ์ง์์๋ต๊ณผ ๊ฐ์ ๋ค๋ฅธ NLP ์์
์ ์์ํ ์ ์์ต๋๋ค.
<div class="flex justify-center">
<img src="https://huggingface.co/datasets/huggingface/documentation-images/resolve/main/gpt2_architecture.png"/>
</div>
1. GPT-2๋ ๋จ์ด๋ฅผ ํ ํฐํํ๊ณ ํ ํฐ ์๋ฒ ๋ฉ์ ์์ฑํ๊ธฐ ์ํด [๋ฐ์ดํธ ํ์ด ์ธ์ฝ๋ฉ(BPE, byte pair encoding)](tokenizer_summary#bytepair-encoding-bpe)์ ์ฌ์ฉํฉ๋๋ค. ์์น ์ธ์ฝ๋ฉ์ ์ํ์ค์์ ๊ฐ ํ ํฐ์ ์์น๋ฅผ ๋ํ๋ด๊ธฐ ์ํด ํ ํฐ ์๋ฒ ๋ฉ์ ์ถ๊ฐ๋ฉ๋๋ค. ์
๋ ฅ ์๋ฒ ๋ฉ์ ์ฌ๋ฌ ๋์ฝ๋ ๋ธ๋ก์ ๊ฑฐ์ณ ์ผ๋ถ ์ต์ข
์๋ ์ํ๋ฅผ ์ถ๋ ฅํฉ๋๋ค. ๊ฐ ๋์ฝ๋ ๋ธ๋ก ๋ด์์ GPT-2๋ *๋ง์คํฌ๋ ์
ํ ์ดํ
์
(masked self-attention)* ๋ ์ด์ด๋ฅผ ์ฌ์ฉํฉ๋๋ค. ์ด๋ GPT-2๊ฐ ์ดํ ํ ํฐ(future tokens)์ ์ฃผ์๋ฅผ ๊ธฐ์ธ์ผ ์ ์๋๋ก ํฉ๋๋ค. ์ผ์ชฝ์ ์๋ ํ ํฐ์๋ง ์ฃผ์๋ฅผ ๊ธฐ์ธ์ผ ์ ์์ต๋๋ค. ๋ง์คํฌ๋ ์
ํ ์ดํ
์
์์๋ ์ดํ
์
๋ง์คํฌ๋ฅผ ์ฌ์ฉํ์ฌ ์ดํ ํ ํฐ์ ๋ํ ์ ์(score)๋ฅผ `0`์ผ๋ก ์ค์ ํ๊ธฐ ๋๋ฌธ์ BERT์ [`mask`] ํ ํฐ๊ณผ ๋ค๋ฆ
๋๋ค.
2. ๋์ฝ๋์ ์ถ๋ ฅ์ ์ธ์ด ๋ชจ๋ธ๋ง ํค๋์ ์ ๋ฌ๋๋ฉฐ, ์ธ์ด ๋ชจ๋ธ๋ง ํค๋๋ ์๋ ์ํ๋ฅผ ๋ก์ง์ผ๋ก ์ ํ ๋ณํ์ ์ํํฉ๋๋ค. ๋ ์ด๋ธ์ ์ํ์ค์ ๋ค์ ํ ํฐ์ผ๋ก, ๋ก์ง์ ์ค๋ฅธ์ชฝ์ผ๋ก ํ๋์ฉ ์ด๋ํ์ฌ ์์ฑ๋ฉ๋๋ค. ๊ต์ฐจ ์ํธ๋กํผ ์์ค์ ์ด๋๋ ๋ก์ง๊ณผ ๋ ์ด๋ธ ๊ฐ์ ๊ณ์ฐ๋์ด ๊ฐ์ฅ ๊ฐ๋ฅ์ฑ์ด ๋์ ๋ค์ ํ ํฐ์ ์ถ๋ ฅํฉ๋๋ค.
GPT-2์ ์ฌ์ ํ๋ จ ๋ชฉ์ ์ ์ ์ ์ผ๋ก [์ธ๊ณผ์ ์ธ์ด ๋ชจ๋ธ๋ง](glossary#causal-language-modeling)์ ๊ธฐ๋ฐํ์ฌ, ์ํ์ค์์ ๋ค์ ๋จ์ด๋ฅผ ์์ธกํ๋ ๊ฒ์
๋๋ค. ์ด๋ GPT-2๊ฐ ํ
์คํธ ์์ฑ์ ๊ด๋ จ๋ ์์
์ ํนํ ์ฐ์ํ๋๋ก ํฉ๋๋ค.
ํ
์คํธ ์์ฑ์ ์ง์ ๋์ ํ ์ค๋น๊ฐ ๋์
จ๋์? ์์ ํ [์ธ๊ณผ์ ์ธ์ด ๋ชจ๋ธ๋ง ๊ฐ์ด๋](tasks/language_modeling#causal-language-modeling)๋ฅผ ํ์ธํ์ฌ DistilGPT-2๋ฅผ ๋ฏธ์ธ ์กฐ์ ํ๊ณ ์ถ๋ก ์ ์ฌ์ฉํ๋ ๋ฐฉ๋ฒ์ ํ์ตํ์ธ์!
<Tip>
ํ
์คํธ ์์ฑ์ ๋ํ ์์ธํ ๋ด์ฉ์ [ํ
์คํธ ์์ฑ ์ ๋ต](generation_strategies) ๊ฐ์ด๋๋ฅผ ํ์ธํ์ธ์!
</Tip>
### ์์ฝ[[summarization]]
[BART](model_doc/bart) ๋ฐ [T5](model_doc/t5)์ ๊ฐ์ ์ธ์ฝ๋-๋์ฝ๋ ๋ชจ๋ธ์ ์์ฝ ์์
์ ์ํ์ค-ํฌ-์ํ์ค ํจํด์ ์ํด ์ค๊ณ๋์์ต๋๋ค. ์ด ์น์
์์ BART์ ์๋ ๋ฐฉ๋ฒ์ ์ค๋ช
ํ ๋ค์, ๋ง์ง๋ง์ T5๋ฅผ ๋ฏธ์ธ ์กฐ์ ํด๋ณผ ์ ์์ต๋๋ค.
<div class="flex justify-center">
<img src="https://huggingface.co/datasets/huggingface/documentation-images/resolve/main/bart_architecture.png"/>
</div>
1. BART์ ์ธ์ฝ๋ ์ํคํ
์ฒ๋ BERT์ ๋งค์ฐ ์ ์ฌํ๋ฉฐ ํ
์คํธ์ ํ ํฐ ๋ฐ ์์น ์๋ฒ ๋ฉ์ ๋ฐ์ต๋๋ค. BART๋ ์
๋ ฅ์ ๋ณํ์ํค๊ณ ๋์ฝ๋๋ก ์ฌ๊ตฌ์ฑํ์ฌ ์ฌ์ ํ๋ จ๋ฉ๋๋ค. ํน์ ๋ณํ ๊ธฐ๋ฒ์ด ์๋ ๋ค๋ฅธ ์ธ์ฝ๋์๋ ๋ฌ๋ฆฌ, BART๋ ๋ชจ๋ ์ ํ์ ๋ณํ์ ์ ์ฉํ ์ ์์ต๋๋ค. ๊ทธ๋ฌ๋ *text infilling* ๋ณํ ๊ธฐ๋ฒ์ด ๊ฐ์ฅ ์ ์๋ํฉ๋๋ค. Text Infiling์์๋ ์ฌ๋ฌ ํ
์คํธ ์คํฌ์ **๋จ์ผ** [`mask`] ํ ํฐ์ผ๋ก ๋์ฒดํฉ๋๋ค. ์ด๋ ๋ชจ๋ธ์ด ๋ง์คํฌ๋ ํ ํฐ์ ์์ธกํด์ผ ํ๊ณ , ๋ชจ๋ธ์ ๋๋ฝ๋ ํ ํฐ์ ์๋ฅผ ์์ธกํ๋๋ก ๊ฐ๋ฅด์น๊ธฐ ๋๋ฌธ์ ์ค์ํฉ๋๋ค. ์
๋ ฅ ์๋ฒ ๋ฉ๊ณผ ๋ง์คํฌ๋ ์คํฌ์ด ์ธ์ฝ๋๋ฅผ ๊ฑฐ์ณ ์ต์ข
์๋ ์ํ๋ฅผ ์ถ๋ ฅํ์ง๋ง, BERT์ ๋ฌ๋ฆฌ BART๋ ๋ง์ง๋ง์ ๋จ์ด๋ฅผ ์์ธกํ๋ ์๋ฐฉํฅ ๋คํธ์ํฌ๋ฅผ ์ถ๊ฐํ์ง ์์ต๋๋ค.
2. ์ธ์ฝ๋์ ์ถ๋ ฅ์ ๋์ฝ๋๋ก ์ ๋ฌ๋๋ฉฐ, ๋์ฝ๋๋ ์ธ์ฝ๋์ ์ถ๋ ฅ์์ ๋ง์คํฌ ํ ํฐ๊ณผ ๋ณํ๋์ง ์์ ํ ํฐ์ ์์ธกํด์ผ ํฉ๋๋ค. ์ด๋ ๋์ฝ๋๊ฐ ์๋ณธ ํ
์คํธ๋ฅผ ๋ณต์ํ๋ ๋ฐ ๋์์ด ๋๋ ์ถ๊ฐ์ ์ธ ๋ฌธ๋งฅ์ ์ป๋๋ก ํฉ๋๋ค. ๋์ฝ๋์ ์ถ๋ ฅ์ ์ธ์ด ๋ชจ๋ธ๋ง ํค๋์ ์ ๋ฌ๋๋ฉฐ, ์ธ์ด ๋ชจ๋ธ๋ง ํค๋๋ ์๋ ์ํ๋ฅผ ๋ก์ง์ผ๋ก ์ ํ ๋ณํ์ ์ํํฉ๋๋ค. ๊ต์ฐจ ์ํธ๋กํผ ์์ค์ ๋ก์ง๊ณผ ํ ํฐ์ด ์ค๋ฅธ์ชฝ์ผ๋ก ์ด๋๋ ๋ ์ด๋ธ ๊ฐ์ ๊ณ์ฐ๋ฉ๋๋ค.
์์ฝ์ ์ง์ ๋์ ํ ์ค๋น๊ฐ ๋์
จ๋์? ์์ ํ [์์ฝ ๊ฐ์ด๋](tasks/summarization)๋ฅผ ํ์ธํ์ฌ T5๋ฅผ ๋ฏธ์ธ ์กฐ์ ํ๊ณ ์ถ๋ก ์ ์ฌ์ฉํ๋ ๋ฐฉ๋ฒ์ ํ์ตํ์ธ์!
<Tip>
ํ
์คํธ ์์ฑ์ ๋ํ ์์ธํ ๋ด์ฉ์ [ํ
์คํธ ์์ฑ ์ ๋ต](generation_strategies) ๊ฐ์ด๋๋ฅผ ํ์ธํ์ธ์!
</Tip>
### ๋ฒ์ญ[[translation]]
๋ฒ์ญ์ ์ํ์ค-ํฌ-์ํ์ค ์์
์ ๋ ๋ค๋ฅธ ์๋ก, [BART](model_doc/bart) ๋๋ [T5](model_doc/t5)์ ๊ฐ์ ์ธ์ฝ๋-๋์ฝ๋ ๋ชจ๋ธ์ ์ฌ์ฉํ ์ ์์ต๋๋ค. ์ด ์น์
์์ BART์ ์๋ ๋ฐฉ๋ฒ์ ์ค๋ช
ํ ๋ค์, ๋ง์ง๋ง์ T5๋ฅผ ๋ฏธ์ธ ์กฐ์ ํด๋ณผ ์ ์์ต๋๋ค.
BART๋ ์์ฒ ์ธ์ด๋ฅผ ํ๊ฒ ์ธ์ด๋ก ๋์ฝ๋ฉํ ์ ์๋ ์
๋ ฅ์ ๋งคํํ๊ธฐ ์ํด ๋ฌด์์๋ก ์ด๊ธฐํ๋ ๋ณ๋์ ์ธ์ฝ๋๋ฅผ ์ถ๊ฐํ์ฌ ๋ฒ์ญ์ ์ ์ฉํฉ๋๋ค. ์ด ์๋ก์ด ์ธ์ฝ๋์ ์๋ฒ ๋ฉ์ ์๋ณธ ๋จ์ด ์๋ฒ ๋ฉ ๋์ ์ฌ์ ํ๋ จ๋ ์ธ์ฝ๋๋ก ์ ๋ฌ๋ฉ๋๋ค. ์์ฒ ์ธ์ฝ๋๋ ๋ชจ๋ธ ์ถ๋ ฅ์ ๊ต์ฐจ ์ํธ๋กํผ ์์ค๋ก๋ถํฐ ์์ฒ ์ธ์ฝ๋, ์์น ์๋ฒ ๋ฉ, ์
๋ ฅ ์๋ฒ ๋ฉ์ ๊ฐฑ์ ํ์ฌ ํ๋ จ๋ฉ๋๋ค. ์ฒซ ๋ฒ์งธ ๋จ๊ณ์์๋ ๋ชจ๋ธ ํ๋ผ๋ฏธํฐ๊ฐ ๊ณ ์ ๋๊ณ , ๋ ๋ฒ์งธ ๋จ๊ณ์์๋ ๋ชจ๋ ๋ชจ๋ธ ํ๋ผ๋ฏธํฐ๊ฐ ํจ๊ป ํ๋ จ๋ฉ๋๋ค.
BART๋ ์ดํ ๋ฒ์ญ์ ์ํด ๋ค์ํ ์ธ์ด๋ก ์ฌ์ ํ๋ จ๋ ๋ค๊ตญ์ด ๋ฒ์ ์ mBART๋ก ํ์ฅ๋์์ต๋๋ค.
๋ฒ์ญ์ ์ง์ ๋์ ํ ์ค๋น๊ฐ ๋์
จ๋์? ์์ ํ [๋ฒ์ญ ๊ฐ์ด๋](tasks/summarization)๋ฅผ ํ์ธํ์ฌ T5๋ฅผ ๋ฏธ์ธ ์กฐ์ ํ๊ณ ์ถ๋ก ์ ์ฌ์ฉํ๋ ๋ฐฉ๋ฒ์ ํ์ตํ์ธ์!
<Tip>
ํ
์คํธ ์์ฑ์ ๋ํ ์์ธํ ๋ด์ฉ์ [ํ
์คํธ ์์ฑ ์ ๋ต](generation_strategies) ๊ฐ์ด๋๋ฅผ ํ์ธํ์ธ์!
</Tip> | 0 |
hf_public_repos/transformers/docs/source | hf_public_repos/transformers/docs/source/ko/preprocessing.md | <!--Copyright 2023 The HuggingFace Team. All rights reserved.
Licensed under the Apache License, Version 2.0 (the "License"); you may not use this file except in compliance with
the License. You may obtain a copy of the License at
http://www.apache.org/licenses/LICENSE-2.0
Unless required by applicable law or agreed to in writing, software distributed under the License is distributed on
an "AS IS" BASIS, WITHOUT WARRANTIES OR CONDITIONS OF ANY KIND, either express or implied. See the License for the
specific language governing permissions and limitations under the License.
โ ๏ธ Note that this file is in Markdown but contain specific syntax for our doc-builder (similar to MDX) that may not be
rendered properly in your Markdown viewer.
-->
# ์ ์ฒ๋ฆฌ[[preprocess]]
[[open-in-colab]]
๋ชจ๋ธ์ ํ๋ จํ๋ ค๋ฉด ๋ฐ์ดํฐ ์ธํธ๋ฅผ ๋ชจ๋ธ์ ๋ง๋ ์
๋ ฅ ํ์์ผ๋ก ์ ์ฒ๋ฆฌํด์ผ ํฉ๋๋ค. ํ
์คํธ, ์ด๋ฏธ์ง ๋๋ ์ค๋์ค์ธ์ง ๊ด๊ณ์์ด ๋ฐ์ดํฐ๋ฅผ ํ
์ ๋ฐฐ์น๋ก ๋ณํํ๊ณ ์กฐ๋ฆฝํ ํ์๊ฐ ์์ต๋๋ค. ๐ค Transformers๋ ๋ชจ๋ธ์ ๋ํ ๋ฐ์ดํฐ๋ฅผ ์ค๋นํ๋ ๋ฐ ๋์์ด ๋๋ ์ผ๋ จ์ ์ ์ฒ๋ฆฌ ํด๋์ค๋ฅผ ์ ๊ณตํฉ๋๋ค. ์ด ํํ ๋ฆฌ์ผ์์๋ ๋ค์ ๋ด์ฉ์ ๋ฐฐ์ธ ์ ์์ต๋๋ค:
* ํ
์คํธ๋ [Tokenizer](./main_classes/tokenizer)๋ฅผ ์ฌ์ฉํ์ฌ ํ ํฐ ์ํ์ค๋ก ๋ณํํ๊ณ ํ ํฐ์ ์ซ์ ํํ์ ๋ง๋ ํ ํ
์๋ก ์กฐ๋ฆฝํฉ๋๋ค.
* ์์ฑ ๋ฐ ์ค๋์ค๋ [Feature extractor](./main_classes/feature_extractor)๋ฅผ ์ฌ์ฉํ์ฌ ์ค๋์ค ํํ์์ ์ํ์ค ํน์ฑ์ ํ์
ํ์ฌ ํ
์๋ก ๋ณํํฉ๋๋ค.
* ์ด๋ฏธ์ง ์
๋ ฅ์ [ImageProcessor](./main_classes/image)์ ์ฌ์ฉํ์ฌ ์ด๋ฏธ์ง๋ฅผ ํ
์๋ก ๋ณํํฉ๋๋ค.
* ๋ฉํฐ๋ชจ๋ฌ ์
๋ ฅ์ [Processor](./main_classes/processors)์ ์ฌ์ฉํ์ฌ ํ ํฌ๋์ด์ ์ ํน์ฑ ์ถ์ถ๊ธฐ ๋๋ ์ด๋ฏธ์ง ํ๋ก์ธ์๋ฅผ ๊ฒฐํฉํฉ๋๋ค.
<Tip>
`AutoProcessor`๋ **์ธ์ ๋** ์๋ํ์ฌ ํ ํฌ๋์ด์ , ์ด๋ฏธ์ง ํ๋ก์ธ์, ํน์ฑ ์ถ์ถ๊ธฐ ๋๋ ํ๋ก์ธ์ ๋ฑ ์ฌ์ฉ ์ค์ธ ๋ชจ๋ธ์ ๋ง๋ ํด๋์ค๋ฅผ ์๋์ผ๋ก ์ ํํฉ๋๋ค.
</Tip>
์์ํ๊ธฐ ์ ์ ๐ค Datasets๋ฅผ ์ค์นํ์ฌ ์คํ์ ์ฌ์ฉํ ๋ฐ์ดํฐ๋ฅผ ๋ถ๋ฌ์ฌ ์ ์์ต๋๋ค:
```bash
pip install datasets
```
## ์์ฐ์ด์ฒ๋ฆฌ[[natural-language-processing]]
<Youtube id="Yffk5aydLzg"/>
ํ
์คํธ ๋ฐ์ดํฐ๋ฅผ ์ ์ฒ๋ฆฌํ๊ธฐ ์ํ ๊ธฐ๋ณธ ๋๊ตฌ๋ [tokenizer](main_classes/tokenizer)์
๋๋ค. ํ ํฌ๋์ด์ ๋ ์ผ๋ จ์ ๊ท์น์ ๋ฐ๋ผ ํ
์คํธ๋ฅผ *ํ ํฐ*์ผ๋ก ๋๋๋๋ค. ํ ํฐ์ ์ซ์๋ก ๋ณํ๋๊ณ ํ
์๋ ๋ชจ๋ธ ์
๋ ฅ์ด ๋ฉ๋๋ค. ๋ชจ๋ธ์ ํ์ํ ์ถ๊ฐ ์
๋ ฅ์ ํ ํฌ๋์ด์ ์ ์ํด ์ถ๊ฐ๋ฉ๋๋ค.
<Tip>
์ฌ์ ํ๋ จ๋ ๋ชจ๋ธ์ ์ฌ์ฉํ ๊ณํ์ด๋ผ๋ฉด ๋ชจ๋ธ๊ณผ ํจ๊ป ์ฌ์ ํ๋ จ๋ ํ ํฌ๋์ด์ ๋ฅผ ์ฌ์ฉํ๋ ๊ฒ์ด ์ค์ํฉ๋๋ค. ์ด๋ ๊ฒ ํ๋ฉด ํ
์คํธ๊ฐ ์ฌ์ ํ๋ จ ๋ง๋ญ์น์ ๋์ผํ ๋ฐฉ์์ผ๋ก ๋ถํ ๋๊ณ ์ฌ์ ํ๋ จ ์ค์ ๋์ผํ ํด๋น ํ ํฐ-์ธ๋ฑ์ค ์(์ผ๋ฐ์ ์ผ๋ก *vocab*์ด๋ผ๊ณ ํจ)์ ์ฌ์ฉํฉ๋๋ค.
</Tip>
์์ํ๋ ค๋ฉด [`AutoTokenizer.from_pretrained`] ๋ฉ์๋๋ฅผ ์ฌ์ฉํ์ฌ ์ฌ์ ํ๋ จ๋ ํ ํฌ๋์ด์ ๋ฅผ ๋ถ๋ฌ์ค์ธ์. ๋ชจ๋ธ๊ณผ ํจ๊ป ์ฌ์ ํ๋ จ๋ *vocab*์ ๋ค์ด๋ก๋ํฉ๋๋ค:
```py
>>> from transformers import AutoTokenizer
>>> tokenizer = AutoTokenizer.from_pretrained("bert-base-cased")
```
๊ทธ ๋ค์์ผ๋ก ํ
์คํธ๋ฅผ ํ ํฌ๋์ด์ ์ ๋ฃ์ด์ฃผ์ธ์:
```py
>>> encoded_input = tokenizer("Do not meddle in the affairs of wizards, for they are subtle and quick to anger.")
>>> print(encoded_input)
{'input_ids': [101, 2079, 2025, 19960, 10362, 1999, 1996, 3821, 1997, 16657, 1010, 2005, 2027, 2024, 11259, 1998, 4248, 2000, 4963, 1012, 102],
'token_type_ids': [0, 0, 0, 0, 0, 0, 0, 0, 0, 0, 0, 0, 0, 0, 0, 0, 0, 0, 0, 0, 0],
'attention_mask': [1, 1, 1, 1, 1, 1, 1, 1, 1, 1, 1, 1, 1, 1, 1, 1, 1, 1, 1, 1, 1]}
```
ํ ํฌ๋์ด์ ๋ ์ธ ๊ฐ์ง ์ค์ํ ํญ๋ชฉ์ ํฌํจํ ๋์
๋๋ฆฌ๋ฅผ ๋ฐํํฉ๋๋ค:
* [input_ids](glossary#input-ids)๋ ๋ฌธ์ฅ์ ๊ฐ ํ ํฐ์ ํด๋นํ๋ ์ธ๋ฑ์ค์
๋๋ค.
* [attention_mask](glossary#attention-mask)๋ ํ ํฐ์ ์ฒ๋ฆฌํด์ผ ํ๋์ง ์ฌ๋ถ๋ฅผ ๋ํ๋
๋๋ค.
* [token_type_ids](glossary#token-type-ids)๋ ๋ ๊ฐ ์ด์์ ์ํ์ค๊ฐ ์์ ๋ ํ ํฐ์ด ์ํ ์ํ์ค๋ฅผ ์๋ณํฉ๋๋ค.
`input_ids`๋ฅผ ๋์ฝ๋ฉํ์ฌ ์
๋ ฅ์ ๋ฐํํฉ๋๋ค:
```py
>>> tokenizer.decode(encoded_input["input_ids"])
'[CLS] Do not meddle in the affairs of wizards, for they are subtle and quick to anger. [SEP]'
```
ํ ํฌ๋์ด์ ๊ฐ ๋ ๊ฐ์ ํน์ํ ํ ํฐ(๋ถ๋ฅ ํ ํฐ `CLS`์ ๋ถํ ํ ํฐ `SEP`)์ ๋ฌธ์ฅ์ ์ถ๊ฐํ์ต๋๋ค.
๋ชจ๋ ๋ชจ๋ธ์ ํน์ํ ํ ํฐ์ด ํ์ํ ๊ฒ์ ์๋์ง๋ง, ํ์ํ๋ค๋ฉด ํ ํฌ๋์ด์ ๊ฐ ์๋์ผ๋ก ์ถ๊ฐํฉ๋๋ค.
์ ์ฒ๋ฆฌํ ๋ฌธ์ฅ์ด ์ฌ๋ฌ ๊ฐ ์๋ ๊ฒฝ์ฐ์๋ ๋ฆฌ์คํธ๋ก ํ ํฌ๋์ด์ ์ ์ ๋ฌํฉ๋๋ค:
```py
>>> batch_sentences = [
... "But what about second breakfast?",
... "Don't think he knows about second breakfast, Pip.",
... "What about elevensies?",
... ]
>>> encoded_inputs = tokenizer(batch_sentences)
>>> print(encoded_inputs)
{'input_ids': [[101, 1252, 1184, 1164, 1248, 6462, 136, 102],
[101, 1790, 112, 189, 1341, 1119, 3520, 1164, 1248, 6462, 117, 21902, 1643, 119, 102],
[101, 1327, 1164, 5450, 23434, 136, 102]],
'token_type_ids': [[0, 0, 0, 0, 0, 0, 0, 0],
[0, 0, 0, 0, 0, 0, 0, 0, 0, 0, 0, 0, 0, 0, 0],
[0, 0, 0, 0, 0, 0, 0]],
'attention_mask': [[1, 1, 1, 1, 1, 1, 1, 1],
[1, 1, 1, 1, 1, 1, 1, 1, 1, 1, 1, 1, 1, 1, 1],
[1, 1, 1, 1, 1, 1, 1]]}
```
### ํจ๋ฉ[[pad]]
๋ชจ๋ธ ์
๋ ฅ์ธ ํ
์๋ ๋ชจ์์ด ๊ท ์ผํด์ผ ํ์ง๋ง, ๋ฌธ์ฅ์ ๊ธธ์ด๊ฐ ํญ์ ๊ฐ์ง๋ ์๊ธฐ ๋๋ฌธ์ ๋ฌธ์ ๊ฐ ๋ ์ ์์ต๋๋ค. ํจ๋ฉ์ ์งง์ ๋ฌธ์ฅ์ ํน์ํ *ํจ๋ฉ ํ ํฐ*์ ์ถ๊ฐํ์ฌ ํ
์๋ฅผ ์ง์ฌ๊ฐํ ๋ชจ์์ด ๋๋๋ก ํ๋ ์ ๋ต์
๋๋ค.
`padding` ๋งค๊ฐ๋ณ์๋ฅผ `True`๋ก ์ค์ ํ์ฌ ๋ฐฐ์น ๋ด์ ์งง์ ์ํ์ค๋ฅผ ๊ฐ์ฅ ๊ธด ์ํ์ค์ ๋ง์ถฐ ํจ๋ฉํฉ๋๋ค.
```py
>>> batch_sentences = [
... "But what about second breakfast?",
... "Don't think he knows about second breakfast, Pip.",
... "What about elevensies?",
... ]
>>> encoded_input = tokenizer(batch_sentences, padding=True)
>>> print(encoded_input)
{'input_ids': [[101, 1252, 1184, 1164, 1248, 6462, 136, 102, 0, 0, 0, 0, 0, 0, 0],
[101, 1790, 112, 189, 1341, 1119, 3520, 1164, 1248, 6462, 117, 21902, 1643, 119, 102],
[101, 1327, 1164, 5450, 23434, 136, 102, 0, 0, 0, 0, 0, 0, 0, 0]],
'token_type_ids': [[0, 0, 0, 0, 0, 0, 0, 0, 0, 0, 0, 0, 0, 0, 0],
[0, 0, 0, 0, 0, 0, 0, 0, 0, 0, 0, 0, 0, 0, 0],
[0, 0, 0, 0, 0, 0, 0, 0, 0, 0, 0, 0, 0, 0, 0]],
'attention_mask': [[1, 1, 1, 1, 1, 1, 1, 1, 0, 0, 0, 0, 0, 0, 0],
[1, 1, 1, 1, 1, 1, 1, 1, 1, 1, 1, 1, 1, 1, 1],
[1, 1, 1, 1, 1, 1, 1, 0, 0, 0, 0, 0, 0, 0, 0]]}
```
๊ธธ์ด๊ฐ ์งง์ ์ฒซ ๋ฌธ์ฅ๊ณผ ์ธ ๋ฒ์งธ ๋ฌธ์ฅ์ด ์ด์ `0`์ผ๋ก ์ฑ์์ก์ต๋๋ค.
### ์๋ผ๋ด๊ธฐ[[truncation]]
ํํธ, ๋๋ก๋ ์ํ์ค๊ฐ ๋ชจ๋ธ์์ ์ฒ๋ฆฌํ๊ธฐ์ ๋๋ฌด ๊ธธ ์๋ ์์ต๋๋ค. ์ด ๊ฒฝ์ฐ, ์ํ์ค๋ฅผ ๋ ์งง๊ฒ ์ค์ผ ํ์๊ฐ ์์ต๋๋ค.
๋ชจ๋ธ์์ ํ์ฉํ๋ ์ต๋ ๊ธธ์ด๋ก ์ํ์ค๋ฅผ ์๋ฅด๋ ค๋ฉด `truncation` ๋งค๊ฐ๋ณ์๋ฅผ `True`๋ก ์ค์ ํ์ธ์:
```py
>>> batch_sentences = [
... "But what about second breakfast?",
... "Don't think he knows about second breakfast, Pip.",
... "What about elevensies?",
... ]
>>> encoded_input = tokenizer(batch_sentences, padding=True, truncation=True)
>>> print(encoded_input)
{'input_ids': [[101, 1252, 1184, 1164, 1248, 6462, 136, 102, 0, 0, 0, 0, 0, 0, 0],
[101, 1790, 112, 189, 1341, 1119, 3520, 1164, 1248, 6462, 117, 21902, 1643, 119, 102],
[101, 1327, 1164, 5450, 23434, 136, 102, 0, 0, 0, 0, 0, 0, 0, 0]],
'token_type_ids': [[0, 0, 0, 0, 0, 0, 0, 0, 0, 0, 0, 0, 0, 0, 0],
[0, 0, 0, 0, 0, 0, 0, 0, 0, 0, 0, 0, 0, 0, 0],
[0, 0, 0, 0, 0, 0, 0, 0, 0, 0, 0, 0, 0, 0, 0]],
'attention_mask': [[1, 1, 1, 1, 1, 1, 1, 1, 0, 0, 0, 0, 0, 0, 0],
[1, 1, 1, 1, 1, 1, 1, 1, 1, 1, 1, 1, 1, 1, 1],
[1, 1, 1, 1, 1, 1, 1, 0, 0, 0, 0, 0, 0, 0, 0]]}
```
<Tip>
๋ค์ํ ํจ๋ฉ๊ณผ ์๋ผ๋ด๊ธฐ ์ธ์์ ๋ํด ๋ ์์๋ณด๋ ค๋ฉด [ํจ๋ฉ๊ณผ ์๋ผ๋ด๊ธฐ](./pad_truncation) ๊ฐ๋
๊ฐ์ด๋๋ฅผ ํ์ธํด๋ณด์ธ์.
</Tip>
### ํ
์ ๋ง๋ค๊ธฐ[[build-tensors]]
๋ง์ง๋ง์ผ๋ก, ํ ํฌ๋์ด์ ๊ฐ ๋ชจ๋ธ์ ๊ณต๊ธ๋๋ ์ค์ ํ
์๋ฅผ ๋ฐํํ๋๋ก ํฉ๋๋ค.
`return_tensors` ๋งค๊ฐ๋ณ์๋ฅผ PyTorch์ ๊ฒฝ์ฐ `pt`, TensorFlow์ ๊ฒฝ์ฐ `tf`๋ก ์ค์ ํ์ธ์:
<frameworkcontent>
<pt>
```py
>>> batch_sentences = [
... "But what about second breakfast?",
... "Don't think he knows about second breakfast, Pip.",
... "What about elevensies?",
... ]
>>> encoded_input = tokenizer(batch_sentences, padding=True, truncation=True, return_tensors="pt")
>>> print(encoded_input)
{'input_ids': tensor([[101, 1252, 1184, 1164, 1248, 6462, 136, 102, 0, 0, 0, 0, 0, 0, 0],
[101, 1790, 112, 189, 1341, 1119, 3520, 1164, 1248, 6462, 117, 21902, 1643, 119, 102],
[101, 1327, 1164, 5450, 23434, 136, 102, 0, 0, 0, 0, 0, 0, 0, 0]]),
'token_type_ids': tensor([[0, 0, 0, 0, 0, 0, 0, 0, 0, 0, 0, 0, 0, 0, 0],
[0, 0, 0, 0, 0, 0, 0, 0, 0, 0, 0, 0, 0, 0, 0],
[0, 0, 0, 0, 0, 0, 0, 0, 0, 0, 0, 0, 0, 0, 0]]),
'attention_mask': tensor([[1, 1, 1, 1, 1, 1, 1, 1, 0, 0, 0, 0, 0, 0, 0],
[1, 1, 1, 1, 1, 1, 1, 1, 1, 1, 1, 1, 1, 1, 1],
[1, 1, 1, 1, 1, 1, 1, 0, 0, 0, 0, 0, 0, 0, 0]])}
```
</pt>
<tf>
```py
>>> batch_sentences = [
... "But what about second breakfast?",
... "Don't think he knows about second breakfast, Pip.",
... "What about elevensies?",
... ]
>>> encoded_input = tokenizer(batch_sentences, padding=True, truncation=True, return_tensors="tf")
>>> print(encoded_input)
{'input_ids': <tf.Tensor: shape=(2, 9), dtype=int32, numpy=
array([[101, 1252, 1184, 1164, 1248, 6462, 136, 102, 0, 0, 0, 0, 0, 0, 0],
[101, 1790, 112, 189, 1341, 1119, 3520, 1164, 1248, 6462, 117, 21902, 1643, 119, 102],
[101, 1327, 1164, 5450, 23434, 136, 102, 0, 0, 0, 0, 0, 0, 0, 0]],
dtype=int32)>,
'token_type_ids': <tf.Tensor: shape=(2, 9), dtype=int32, numpy=
array([[0, 0, 0, 0, 0, 0, 0, 0, 0, 0, 0, 0, 0, 0, 0],
[0, 0, 0, 0, 0, 0, 0, 0, 0, 0, 0, 0, 0, 0, 0],
[0, 0, 0, 0, 0, 0, 0, 0, 0, 0, 0, 0, 0, 0, 0]], dtype=int32)>,
'attention_mask': <tf.Tensor: shape=(2, 9), dtype=int32, numpy=
array([[1, 1, 1, 1, 1, 1, 1, 1, 0, 0, 0, 0, 0, 0, 0],
[1, 1, 1, 1, 1, 1, 1, 1, 1, 1, 1, 1, 1, 1, 1],
[1, 1, 1, 1, 1, 1, 1, 0, 0, 0, 0, 0, 0, 0, 0]], dtype=int32)>}
```
</tf>
</frameworkcontent>
## ์ค๋์ค[[audio]]
์ค๋์ค ์์
์ ๋ชจ๋ธ์ ๋ง๋ ๋ฐ์ดํฐ ์ธํธ๋ฅผ ์ค๋นํ๊ธฐ ์ํด [ํน์ฑ ์ถ์ถ๊ธฐ](main_classes/feature_extractor)๊ฐ ํ์ํฉ๋๋ค. ํน์ฑ ์ถ์ถ๊ธฐ๋ ์์ ์ค๋์ค ๋ฐ์ดํฐ์์ ํน์ฑ๋ฅผ ์ถ์ถํ๊ณ ์ด๋ฅผ ํ
์๋ก ๋ณํํ๋ ๊ฒ์ด ๋ชฉ์ ์
๋๋ค.
์ค๋์ค ๋ฐ์ดํฐ ์ธํธ์ ํน์ฑ ์ถ์ถ๊ธฐ๋ฅผ ์ฌ์ฉํ๋ ๋ฐฉ๋ฒ์ ๋ณด๊ธฐ ์ํด [MInDS-14](https://huggingface.co/datasets/PolyAI/minds14) ๋ฐ์ดํฐ ์ธํธ๋ฅผ ๊ฐ์ ธ์ค์ธ์. (๋ฐ์ดํฐ ์ธํธ๋ฅผ ๊ฐ์ ธ์ค๋ ๋ฐฉ๋ฒ์ ๐ค [๋ฐ์ดํฐ ์ธํธ ํํ ๋ฆฌ์ผ](https://huggingface.co/docs/datasets/load_hub.html)์์ ์์ธํ ์ค๋ช
ํ๊ณ ์์ต๋๋ค.)
```py
>>> from datasets import load_dataset, Audio
>>> dataset = load_dataset("PolyAI/minds14", name="en-US", split="train")
```
`audio` ์ด์ ์ฒซ ๋ฒ์งธ ์์์ ์ ๊ทผํ์ฌ ์
๋ ฅ์ ์ดํด๋ณด์ธ์. `audio` ์ด์ ํธ์ถํ๋ฉด ์ค๋์ค ํ์ผ์ ์๋์ผ๋ก ๊ฐ์ ธ์ค๊ณ ๋ฆฌ์ํ๋งํฉ๋๋ค.
```py
>>> dataset[0]["audio"]
{'array': array([ 0. , 0.00024414, -0.00024414, ..., -0.00024414,
0. , 0. ], dtype=float32),
'path': '/root/.cache/huggingface/datasets/downloads/extracted/f14948e0e84be638dd7943ac36518a4cf3324e8b7aa331c5ab11541518e9368c/en-US~JOINT_ACCOUNT/602ba55abb1e6d0fbce92065.wav',
'sampling_rate': 8000}
```
์ด๋ ๊ฒ ํ๋ฉด ์ธ ๊ฐ์ง ํญ๋ชฉ์ด ๋ฐํ๋ฉ๋๋ค:
* `array`๋ 1D ๋ฐฐ์ด๋ก ๊ฐ์ ธ์์ (ํ์ํ ๊ฒฝ์ฐ) ๋ฆฌ์ํ๋ง๋ ์์ฑ ์ ํธ์
๋๋ค.
* `path`๋ ์ค๋์ค ํ์ผ์ ์์น๋ฅผ ๊ฐ๋ฆฌํต๋๋ค.
* `sampling_rate`๋ ์์ฑ ์ ํธ์์ ์ด๋น ์ธก์ ๋๋ ๋ฐ์ดํฐ ํฌ์ธํธ ์๋ฅผ ๋ํ๋
๋๋ค.
์ด ํํ ๋ฆฌ์ผ์์๋ [Wav2Vec2](https://huggingface.co/facebook/wav2vec2-base) ๋ชจ๋ธ์ ์ฌ์ฉํฉ๋๋ค. ๋ชจ๋ธ ์นด๋๋ฅผ ๋ณด๋ฉด Wav2Vec2๊ฐ 16kHz ์ํ๋ง๋ ์์ฑ ์ค๋์ค๋ฅผ ๊ธฐ๋ฐ์ผ๋ก ์ฌ์ ํ๋ จ๋ ๊ฒ์ ์ ์ ์์ต๋๋ค.
๋ชจ๋ธ์ ์ฌ์ ํ๋ จํ๋ ๋ฐ ์ฌ์ฉ๋ ๋ฐ์ดํฐ ์ธํธ์ ์ํ๋ง ๋ ์ดํธ์ ์ค๋์ค ๋ฐ์ดํฐ์ ์ํ๋ง ๋ ์ดํธ๊ฐ ์ผ์นํด์ผ ํฉ๋๋ค. ๋ฐ์ดํฐ์ ์ํ๋ง ๋ ์ดํธ๊ฐ ๋ค๋ฅด๋ฉด ๋ฐ์ดํฐ๋ฅผ ๋ฆฌ์ํ๋งํด์ผ ํฉ๋๋ค.
1. ๐ค Datasets์ [`~datasets.Dataset.cast_column`] ๋ฉ์๋๋ฅผ ์ฌ์ฉํ์ฌ ์ํ๋ง ๋ ์ดํธ๋ฅผ 16kHz๋ก ์
์ํ๋งํ์ธ์:
```py
>>> dataset = dataset.cast_column("audio", Audio(sampling_rate=16_000))
```
2. ์ค๋์ค ํ์ผ์ ๋ฆฌ์ํ๋งํ๊ธฐ ์ํด `audio` ์ด์ ๋ค์ ํธ์ถํฉ๋๋ค:
```py
>>> dataset[0]["audio"]
{'array': array([ 2.3443763e-05, 2.1729663e-04, 2.2145823e-04, ...,
3.8356509e-05, -7.3497440e-06, -2.1754686e-05], dtype=float32),
'path': '/root/.cache/huggingface/datasets/downloads/extracted/f14948e0e84be638dd7943ac36518a4cf3324e8b7aa331c5ab11541518e9368c/en-US~JOINT_ACCOUNT/602ba55abb1e6d0fbce92065.wav',
'sampling_rate': 16000}
```
๋ค์์ผ๋ก, ์
๋ ฅ์ ์ ๊ทํํ๊ณ ํจ๋ฉํ ํน์ฑ ์ถ์ถ๊ธฐ๋ฅผ ๊ฐ์ ธ์ค์ธ์. ํ
์คํธ ๋ฐ์ดํฐ์ ๊ฒฝ์ฐ, ๋ ์งง์ ์ํ์ค์ ๋ํด `0`์ด ์ถ๊ฐ๋ฉ๋๋ค. ์ค๋์ค ๋ฐ์ดํฐ์๋ ๊ฐ์ ๊ฐ๋
์ด ์ ์ฉ๋ฉ๋๋ค.
ํน์ฑ ์ถ์ถ๊ธฐ๋ ๋ฐฐ์ด์ `0`(๋ฌต์์ผ๋ก ํด์)์ ์ถ๊ฐํฉ๋๋ค.
[`AutoFeatureExtractor.from_pretrained`]๋ฅผ ์ฌ์ฉํ์ฌ ํน์ฑ ์ถ์ถ๊ธฐ๋ฅผ ๊ฐ์ ธ์ค์ธ์:
```py
>>> from transformers import AutoFeatureExtractor
>>> feature_extractor = AutoFeatureExtractor.from_pretrained("facebook/wav2vec2-base")
```
์ค๋์ค `array`๋ฅผ ํน์ฑ ์ถ์ถ๊ธฐ์ ์ ๋ฌํ์ธ์. ๋ํ, ๋ฐ์ํ ์ ์๋ ์กฐ์ฉํ ์ค๋ฅ(silent errors)๋ฅผ ๋ ์ ๋๋ฒ๊น
ํ ์ ์๋๋ก ํน์ฑ ์ถ์ถ๊ธฐ์ `sampling_rate` ์ธ์๋ฅผ ์ถ๊ฐํ๋ ๊ฒ์ ๊ถ์ฅํฉ๋๋ค.
```py
>>> audio_input = [dataset[0]["audio"]["array"]]
>>> feature_extractor(audio_input, sampling_rate=16000)
{'input_values': [array([ 3.8106556e-04, 2.7506407e-03, 2.8015103e-03, ...,
5.6335266e-04, 4.6588284e-06, -1.7142107e-04], dtype=float32)]}
```
ํ ํฌ๋์ด์ ์ ๋ง์ฐฌ๊ฐ์ง๋ก ๋ฐฐ์น ๋ด์์ ๊ฐ๋ณ์ ์ธ ์ํ์ค๋ฅผ ์ฒ๋ฆฌํ๊ธฐ ์ํด ํจ๋ฉ ๋๋ ์๋ผ๋ด๊ธฐ๋ฅผ ์ ์ฉํ ์ ์์ต๋๋ค. ์ด ๋ ๊ฐ์ ์ค๋์ค ์ํ์ ์ํ์ค ๊ธธ์ด๋ฅผ ํ์ธํด๋ณด์ธ์:
```py
>>> dataset[0]["audio"]["array"].shape
(173398,)
>>> dataset[1]["audio"]["array"].shape
(106496,)
```
์ค๋์ค ์ํ์ ๊ธธ์ด๊ฐ ๋์ผํ๋๋ก ๋ฐ์ดํฐ ์ธํธ๋ฅผ ์ ์ฒ๋ฆฌํ๋ ํจ์๋ฅผ ๋ง๋์ธ์. ์ต๋ ์ํ ๊ธธ์ด๋ฅผ ์ง์ ํ๋ฉด ํน์ฑ ์ถ์ถ๊ธฐ๊ฐ ํด๋น ๊ธธ์ด์ ๋ง์ถฐ ์ํ์ค๋ฅผ ํจ๋ฉํ๊ฑฐ๋ ์๋ผ๋
๋๋ค:
```py
>>> def preprocess_function(examples):
... audio_arrays = [x["array"] for x in examples["audio"]]
... inputs = feature_extractor(
... audio_arrays,
... sampling_rate=16000,
... padding=True,
... max_length=100000,
... truncation=True,
... )
... return inputs
```
`preprocess_function`์ ๋ฐ์ดํฐ ์ธํธ์ ์ฒ์ ์์ ๋ช ๊ฐ์ ์ ์ฉํด๋ณด์ธ์:
```py
>>> processed_dataset = preprocess_function(dataset[:5])
```
์ด์ ์ํ ๊ธธ์ด๊ฐ ๋ชจ๋ ๊ฐ๊ณ ์ง์ ๋ ์ต๋ ๊ธธ์ด์ ๋ง๊ฒ ๋์์ต๋๋ค. ๋๋์ด ์ ์ฒ๋ฆฌ๋ ๋ฐ์ดํฐ ์ธํธ๋ฅผ ๋ชจ๋ธ์ ์ ๋ฌํ ์ ์์ต๋๋ค!
```py
>>> processed_dataset["input_values"][0].shape
(100000,)
>>> processed_dataset["input_values"][1].shape
(100000,)
```
## ์ปดํจํฐ ๋น์ [[computer-vision]]
์ปดํจํฐ ๋น์ ์์
์ ๊ฒฝ์ฐ, ๋ชจ๋ธ์ ๋ํ ๋ฐ์ดํฐ ์ธํธ๋ฅผ ์ค๋นํ๊ธฐ ์ํด [์ด๋ฏธ์ง ํ๋ก์ธ์](main_classes/image_processor)๊ฐ ํ์ํฉ๋๋ค.
์ด๋ฏธ์ง ์ ์ฒ๋ฆฌ๋ ์ด๋ฏธ์ง๋ฅผ ๋ชจ๋ธ์ด ์์ํ๋ ์
๋ ฅ์ผ๋ก ๋ณํํ๋ ์ฌ๋ฌ ๋จ๊ณ๋ก ์ด๋ฃจ์ด์ง๋๋ค.
์ด๋ฌํ ๋จ๊ณ์๋ ํฌ๊ธฐ ์กฐ์ , ์ ๊ทํ, ์์ ์ฑ๋ ๋ณด์ , ์ด๋ฏธ์ง์ ํ
์ ๋ณํ ๋ฑ์ด ํฌํจ๋ฉ๋๋ค.
<Tip>
์ด๋ฏธ์ง ์ ์ฒ๋ฆฌ๋ ์ด๋ฏธ์ง ์ฆ๊ฐ ๊ธฐ๋ฒ์ ๋ช ๊ฐ์ง ์ ์ฉํ ๋ค์ ํ ์๋ ์์ต๋๋ค.
์ด๋ฏธ์ง ์ ์ฒ๋ฆฌ ๋ฐ ์ด๋ฏธ์ง ์ฆ๊ฐ์ ๋ชจ๋ ์ด๋ฏธ์ง ๋ฐ์ดํฐ๋ฅผ ๋ณํํ์ง๋ง, ์๋ก ๋ค๋ฅธ ๋ชฉ์ ์ ๊ฐ์ง๊ณ ์์ต๋๋ค:
* ์ด๋ฏธ์ง ์ฆ๊ฐ์ ๊ณผ์ ํฉ(over-fitting)์ ๋ฐฉ์งํ๊ณ ๋ชจ๋ธ์ ๊ฒฌ๊ณ ํจ(resiliency)์ ๋์ด๋ ๋ฐ ๋์์ด ๋๋ ๋ฐฉ์์ผ๋ก ์ด๋ฏธ์ง๋ฅผ ์์ ํฉ๋๋ค.
๋ฐ๊ธฐ์ ์์ ์กฐ์ , ์๋ฅด๊ธฐ, ํ์ , ํฌ๊ธฐ ์กฐ์ , ํ๋/์ถ์ ๋ฑ ๋ค์ํ ๋ฐฉ๋ฒ์ผ๋ก ๋ฐ์ดํฐ๋ฅผ ์ฆ๊ฐํ ์ ์์ต๋๋ค.
๊ทธ๋ฌ๋ ์ฆ๊ฐ์ผ๋ก ์ด๋ฏธ์ง์ ์๋ฏธ๊ฐ ๋ฐ๋์ง ์๋๋ก ์ฃผ์ํด์ผ ํฉ๋๋ค.
* ์ด๋ฏธ์ง ์ ์ฒ๋ฆฌ๋ ์ด๋ฏธ์ง๊ฐ ๋ชจ๋ธ์ด ์์ํ๋ ์
๋ ฅ ํ์๊ณผ ์ผ์นํ๋๋ก ๋ณด์ฅํฉ๋๋ค.
์ปดํจํฐ ๋น์ ๋ชจ๋ธ์ ๋ฏธ์ธ ์กฐ์ ํ ๋ ์ด๋ฏธ์ง๋ ๋ชจ๋ธ์ด ์ด๊ธฐ์ ํ๋ จ๋ ๋์ ์ ํํ ๊ฐ์ ๋ฐฉ์์ผ๋ก ์ ์ฒ๋ฆฌ๋์ด์ผ ํฉ๋๋ค.
์ด๋ฏธ์ง ์ฆ๊ฐ์๋ ์ํ๋ ๋ผ์ด๋ธ๋ฌ๋ฆฌ๋ฅผ ๋ฌด์์ด๋ ์ฌ์ฉํ ์ ์์ต๋๋ค. ์ด๋ฏธ์ง ์ ์ฒ๋ฆฌ์๋ ๋ชจ๋ธ๊ณผ ์ฐ๊ฒฐ๋ `ImageProcessor`๋ฅผ ์ฌ์ฉํฉ๋๋ค.
</Tip>
[food101](https://huggingface.co/datasets/food101) ๋ฐ์ดํฐ ์ธํธ๋ฅผ ๊ฐ์ ธ์์ ์ปดํจํฐ ๋น์ ๋ฐ์ดํฐ ์ธํธ์์ ์ด๋ฏธ์ง ํ๋ก์ธ์๋ฅผ ์ด๋ป๊ฒ ์ฌ์ฉํ๋์ง ์์๋ณด์ธ์.
๋ฐ์ดํฐ ์ธํธ๋ฅผ ๋ถ๋ฌ์ค๋ ๋ฐฉ๋ฒ์ ๐ค [๋ฐ์ดํฐ ์ธํธ ํํ ๋ฆฌ์ผ](https://huggingface.co/docs/datasets/load_hub.html)์ ์ฐธ๊ณ ํ์ธ์.
<Tip>
๋ฐ์ดํฐ ์ธํธ๊ฐ ์๋นํ ํฌ๊ธฐ ๋๋ฌธ์ ๐ค Datasets์ `split` ๋งค๊ฐ๋ณ์๋ฅผ ์ฌ์ฉํ์ฌ ํ๋ จ ์ธํธ์์ ์์ ์ํ๋ง ๊ฐ์ ธ์ค์ธ์!
</Tip>
```py
>>> from datasets import load_dataset
>>> dataset = load_dataset("food101", split="train[:100]")
```
๋ค์์ผ๋ก, ๐ค Datasets์ [`image`](https://huggingface.co/docs/datasets/package_reference/main_classes.html?highlight=image#datasets.Image)๋ก ์ด๋ฏธ์ง๋ฅผ ํ์ธํด๋ณด์ธ์:
```py
>>> dataset[0]["image"]
```
<div class="flex justify-center">
<img src="https://huggingface.co/datasets/huggingface/documentation-images/resolve/main/vision-preprocess-tutorial.png"/>
</div>
[`AutoImageProcessor.from_pretrained`]๋ก ์ด๋ฏธ์ง ํ๋ก์ธ์๋ฅผ ๊ฐ์ ธ์ค์ธ์:
```py
>>> from transformers import AutoImageProcessor
>>> image_processor = AutoImageProcessor.from_pretrained("google/vit-base-patch16-224")
```
๋จผ์ ์ด๋ฏธ์ง ์ฆ๊ฐ ๋จ๊ณ๋ฅผ ์ถ๊ฐํด ๋ด
์๋ค. ์๋ฌด ๋ผ์ด๋ธ๋ฌ๋ฆฌ๋ ์ฌ์ฉํด๋ ๊ด์ฐฎ์ง๋ง, ์ด๋ฒ ํํ ๋ฆฌ์ผ์์๋ torchvision์ [`transforms`](https://pytorch.org/vision/stable/transforms.html) ๋ชจ๋์ ์ฌ์ฉํ๊ฒ ์ต๋๋ค.
๋ค๋ฅธ ๋ฐ์ดํฐ ์ฆ๊ฐ ๋ผ์ด๋ธ๋ฌ๋ฆฌ๋ฅผ ์ฌ์ฉํด๋ณด๊ณ ์ถ๋ค๋ฉด, [Albumentations](https://colab.research.google.com/github/huggingface/notebooks/blob/main/examples/image_classification_albumentations.ipynb) ๋๋ [Kornia notebooks](https://colab.research.google.com/github/huggingface/notebooks/blob/main/examples/image_classification_kornia.ipynb)์์ ์ด๋ป๊ฒ ์ฌ์ฉํ๋์ง ๋ฐฐ์ธ ์ ์์ต๋๋ค.
1. [`Compose`](https://pytorch.org/vision/master/generated/torchvision.transforms.Compose.html)๋ก [`RandomResizedCrop`](https://pytorch.org/vision/main/generated/torchvision.transforms.RandomResizedCrop.html)์ [`ColorJitter`](https://pytorch.org/vision/main/generated/torchvision.transforms.ColorJitter.html) ๋ฑ ๋ณํ์ ๋ช ๊ฐ์ง ์ฐ๊ฒฐํ์ธ์.
์ฐธ๊ณ ๋ก ํฌ๊ธฐ ์กฐ์ ์ ํ์ํ ์ด๋ฏธ์ง์ ํฌ๊ธฐ ์๊ตฌ์ฌํญ์ `image_processor`์์ ๊ฐ์ ธ์ฌ ์ ์์ต๋๋ค.
์ผ๋ถ ๋ชจ๋ธ์ ์ ํํ ๋์ด์ ๋๋น๋ฅผ ์๊ตฌํ์ง๋ง, ์ ์ผ ์งง์ ๋ณ์ ๊ธธ์ด(`shortest_edge`)๋ง ์ ์๋ ๋ชจ๋ธ๋ ์์ต๋๋ค.
```py
>>> from torchvision.transforms import RandomResizedCrop, ColorJitter, Compose
>>> size = (
... image_processor.size["shortest_edge"]
... if "shortest_edge" in image_processor.size
... else (image_processor.size["height"], image_processor.size["width"])
... )
>>> _transforms = Compose([RandomResizedCrop(size), ColorJitter(brightness=0.5, hue=0.5)])
```
2. ๋ชจ๋ธ์ ์
๋ ฅ์ผ๋ก [`pixel_values`](model_doc/visionencoderdecoder#transformers.VisionEncoderDecoderModel.forward.pixel_values)๋ฅผ ๋ฐ์ต๋๋ค.
`ImageProcessor`๋ ์ด๋ฏธ์ง ์ ๊ทํ ๋ฐ ์ ์ ํ ํ
์ ์์ฑ์ ์ฒ๋ฆฌํ ์ ์์ต๋๋ค.
๋ฐฐ์น ์ด๋ฏธ์ง์ ๋ํ ์ด๋ฏธ์ง ์ฆ๊ฐ ๋ฐ ์ด๋ฏธ์ง ์ ์ฒ๋ฆฌ๋ฅผ ๊ฒฐํฉํ๊ณ `pixel_values`๋ฅผ ์์ฑํ๋ ํจ์๋ฅผ ๋ง๋ญ๋๋ค:
```py
>>> def transforms(examples):
... images = [_transforms(img.convert("RGB")) for img in examples["image"]]
... examples["pixel_values"] = image_processor(images, do_resize=False, return_tensors="pt")["pixel_values"]
... return examples
```
<Tip>
์์ ์์์๋ ์ด๋ฏธ์ง ์ฆ๊ฐ ์ค์ ์ด๋ฏธ์ง ํฌ๊ธฐ๋ฅผ ์กฐ์ ํ๊ธฐ ๋๋ฌธ์ `do_resize=False`๋ก ์ค์ ํ๊ณ , ํด๋น `image_processor`์์ `size` ์์ฑ์ ํ์ฉํ์ต๋๋ค.
์ด๋ฏธ์ง ์ฆ๊ฐ ์ค์ ์ด๋ฏธ์ง ํฌ๊ธฐ๋ฅผ ์กฐ์ ํ์ง ์์ ๊ฒฝ์ฐ ์ด ๋งค๊ฐ๋ณ์๋ฅผ ์๋ตํ์ธ์.
๊ธฐ๋ณธ์ ์ผ๋ก๋ `ImageProcessor`๊ฐ ํฌ๊ธฐ ์กฐ์ ์ ์ฒ๋ฆฌํฉ๋๋ค.
์ฆ๊ฐ ๋ณํ ๊ณผ์ ์์ ์ด๋ฏธ์ง๋ฅผ ์ ๊ทํํ๋ ค๋ฉด `image_processor.image_mean` ๋ฐ `image_processor.image_std` ๊ฐ์ ์ฌ์ฉํ์ธ์.
</Tip>
3. ๐ค Datasets์ [`set_transform`](https://huggingface.co/docs/datasets/process.html#format-transform)๋ฅผ ์ฌ์ฉํ์ฌ ์ค์๊ฐ์ผ๋ก ๋ณํ์ ์ ์ฉํฉ๋๋ค:
```py
>>> dataset.set_transform(transforms)
```
4. ์ด์ ์ด๋ฏธ์ง์ ์ ๊ทผํ๋ฉด ์ด๋ฏธ์ง ํ๋ก์ธ์๊ฐ `pixel_values`๋ฅผ ์ถ๊ฐํ ๊ฒ์ ์ ์ ์์ต๋๋ค.
๋๋์ด ์ฒ๋ฆฌ๋ ๋ฐ์ดํฐ ์ธํธ๋ฅผ ๋ชจ๋ธ์ ์ ๋ฌํ ์ ์์ต๋๋ค!
```py
>>> dataset[0].keys()
```
๋ค์์ ๋ณํ์ด ์ ์ฉ๋ ํ์ ์ด๋ฏธ์ง์
๋๋ค. ์ด๋ฏธ์ง๊ฐ ๋ฌด์์๋ก ์๋ ค๋๊ฐ๊ณ ์์ ์์ฑ์ด ๋ค๋ฆ
๋๋ค.
```py
>>> import numpy as np
>>> import matplotlib.pyplot as plt
>>> img = dataset[0]["pixel_values"]
>>> plt.imshow(img.permute(1, 2, 0))
```
<div class="flex justify-center">
<img src="https://huggingface.co/datasets/huggingface/documentation-images/resolve/main/preprocessed_image.png"/>
</div>
<Tip>
`ImageProcessor`๋ ๊ฐ์ฒด ๊ฐ์ง, ์๋งจํฑ ์ธ๊ทธ๋ฉํ
์ด์
(semantic segmentation), ์ธ์คํด์ค ์ธ๊ทธ๋ฉํ
์ด์
(instance segmentation), ํ๋ํฑ ์ธ๊ทธ๋ฉํ
์ด์
(panoptic segmentation)๊ณผ ๊ฐ์ ์์
์ ๋ํ ํ์ฒ๋ฆฌ ๋ฐฉ๋ฒ์ ์ ๊ณตํฉ๋๋ค.
์ด๋ฌํ ๋ฐฉ๋ฒ์ ๋ชจ๋ธ์ ์์ ์ถ๋ ฅ์ ๊ฒฝ๊ณ ์์๋ ์ธ๊ทธ๋ฉํ
์ด์
๋งต๊ณผ ๊ฐ์ ์๋ฏธ ์๋ ์์ธก์ผ๋ก ๋ณํํด์ค๋๋ค.
</Tip>
### ํจ๋ฉ[[pad]]
์๋ฅผ ๋ค์ด, [DETR](./model_doc/detr)์ ๊ฐ์ ๊ฒฝ์ฐ์๋ ๋ชจ๋ธ์ด ํ๋ จํ ๋ ํฌ๊ธฐ ์กฐ์ ์ฆ๊ฐ์ ์ ์ฉํฉ๋๋ค.
์ด๋ก ์ธํด ๋ฐฐ์น ๋ด ์ด๋ฏธ์ง ํฌ๊ธฐ๊ฐ ๋ฌ๋ผ์ง ์ ์์ต๋๋ค.
[`DetrImageProcessor`]์ [`DetrImageProcessor.pad`]๋ฅผ ์ฌ์ฉํ๊ณ ์ฌ์ฉ์ ์ ์ `collate_fn`์ ์ ์ํด์ ๋ฐฐ์น ์ด๋ฏธ์ง๋ฅผ ์ฒ๋ฆฌํ ์ ์์ต๋๋ค.
```py
>>> def collate_fn(batch):
... pixel_values = [item["pixel_values"] for item in batch]
... encoding = image_processor.pad(pixel_values, return_tensors="pt")
... labels = [item["labels"] for item in batch]
... batch = {}
... batch["pixel_values"] = encoding["pixel_values"]
... batch["pixel_mask"] = encoding["pixel_mask"]
... batch["labels"] = labels
... return batch
```
## ๋ฉํฐ๋ชจ๋ฌ[[multimodal]]
๋ฉํฐ๋ชจ๋ฌ ์
๋ ฅ์ด ํ์ํ ์์
์ ๊ฒฝ์ฐ, ๋ชจ๋ธ์ ๋ฐ์ดํฐ ์ธํธ๋ฅผ ์ค๋นํ๊ธฐ ์ํ [ํ๋ก์ธ์](main_classes/processors)๊ฐ ํ์ํฉ๋๋ค.
ํ๋ก์ธ์๋ ํ ํฌ๋์ด์ ์ ํน์ฑ ์ถ์ถ๊ธฐ์ ๊ฐ์ ๋ ๊ฐ์ง ์ฒ๋ฆฌ ๊ฐ์ฒด๋ฅผ ๊ฒฐํฉํฉ๋๋ค.
[LJ Speech](https://huggingface.co/datasets/lj_speech) ๋ฐ์ดํฐ ์ธํธ๋ฅผ ๊ฐ์ ธ์์ ์๋ ์์ฑ ์ธ์(ASR)์ ์ํ ํ๋ก์ธ์๋ฅผ ์ฌ์ฉํ๋ ๋ฐฉ๋ฒ์ ํ์ธํ์ธ์.
(๋ฐ์ดํฐ ์ธํธ๋ฅผ ๊ฐ์ ธ์ค๋ ๋ฐฉ๋ฒ์ ๋ํ ์์ธํ ๋ด์ฉ์ ๐ค [๋ฐ์ดํฐ ์ธํธ ํํ ๋ฆฌ์ผ](https://huggingface.co/docs/datasets/load_hub.html)์์ ๋ณผ ์ ์์ต๋๋ค.)
```py
>>> from datasets import load_dataset
>>> lj_speech = load_dataset("lj_speech", split="train")
```
์๋ ์์ฑ ์ธ์(ASR)์์๋ `audio`์ `text`์๋ง ์ง์คํ๋ฉด ๋๋ฏ๋ก, ๋ค๋ฅธ ์ด๋ค์ ์ ๊ฑฐํ ์ ์์ต๋๋ค:
```py
>>> lj_speech = lj_speech.map(remove_columns=["file", "id", "normalized_text"])
```
์ด์ `audio`์ `text`์ด์ ์ดํด๋ณด์ธ์:
```py
>>> lj_speech[0]["audio"]
{'array': array([-7.3242188e-04, -7.6293945e-04, -6.4086914e-04, ...,
7.3242188e-04, 2.1362305e-04, 6.1035156e-05], dtype=float32),
'path': '/root/.cache/huggingface/datasets/downloads/extracted/917ece08c95cf0c4115e45294e3cd0dee724a1165b7fc11798369308a465bd26/LJSpeech-1.1/wavs/LJ001-0001.wav',
'sampling_rate': 22050}
>>> lj_speech[0]["text"]
'Printing, in the only sense with which we are at present concerned, differs from most if not from all the arts and crafts represented in the Exhibition'
```
๊ธฐ์กด์ ์ฌ์ ํ๋ จ๋ ๋ชจ๋ธ์์ ์ฌ์ฉ๋ ๋ฐ์ดํฐ ์ธํธ์ ์๋ก์ด ์ค๋์ค ๋ฐ์ดํฐ ์ธํธ์ ์ํ๋ง ๋ ์ดํธ๋ฅผ ์ผ์น์ํค๊ธฐ ์ํด ์ค๋์ค ๋ฐ์ดํฐ ์ธํธ์ ์ํ๋ง ๋ ์ดํธ๋ฅผ [๋ฆฌ์ํ๋ง](preprocessing#audio)ํด์ผ ํฉ๋๋ค!
```py
>>> lj_speech = lj_speech.cast_column("audio", Audio(sampling_rate=16_000))
```
[`AutoProcessor.from_pretrained`]๋ก ํ๋ก์ธ์๋ฅผ ๊ฐ์ ธ์ค์ธ์:
```py
>>> from transformers import AutoProcessor
>>> processor = AutoProcessor.from_pretrained("facebook/wav2vec2-base-960h")
```
1. `array`์ ๋ค์ด ์๋ ์ค๋์ค ๋ฐ์ดํฐ๋ฅผ `input_values`๋ก ๋ณํํ๊ณ `text`๋ฅผ ํ ํฐํํ์ฌ `labels`๋ก ๋ณํํ๋ ํจ์๋ฅผ ๋ง๋ญ๋๋ค.
๋ชจ๋ธ์ ์
๋ ฅ์ ๋ค์๊ณผ ๊ฐ์ต๋๋ค:
```py
>>> def prepare_dataset(example):
... audio = example["audio"]
... example.update(processor(audio=audio["array"], text=example["text"], sampling_rate=16000))
... return example
```
2. ์ํ์ `prepare_dataset` ํจ์์ ์ ์ฉํ์ธ์:
```py
>>> prepare_dataset(lj_speech[0])
```
์ด์ ํ๋ก์ธ์๊ฐ `input_values`์ `labels`๋ฅผ ์ถ๊ฐํ๊ณ , ์ํ๋ง ๋ ์ดํธ๋ ์ฌ๋ฐ๋ฅด๊ฒ 16kHz๋ก ๋ค์ด์ํ๋งํ์ต๋๋ค.
๋๋์ด ์ฒ๋ฆฌ๋ ๋ฐ์ดํฐ ์ธํธ๋ฅผ ๋ชจ๋ธ์ ์ ๋ฌํ ์ ์์ต๋๋ค!
| 0 |
hf_public_repos/transformers/docs/source | hf_public_repos/transformers/docs/source/ko/performance.md | <!---
Copyright 2021 The HuggingFace Team. All rights reserved.
Licensed under the Apache License, Version 2.0 (the "License");
you may not use this file except in compliance with the License.
You may obtain a copy of the License at
http://www.apache.org/licenses/LICENSE-2.0
Unless required by applicable law or agreed to in writing, software
distributed under the License is distributed on an "AS IS" BASIS,
WITHOUT WARRANTIES OR CONDITIONS OF ANY KIND, either express or implied.
See the License for the specific language governing permissions and
limitations under the License.
โ ๏ธ Note that this file is in Markdown but contain specific syntax for our doc-builder (similar to MDX) that may not be
rendered properly in your Markdown viewer.
-->
# ์ฑ๋ฅ ๋ฐ ํ์ฅ์ฑ [[performance-and-scalability]]
์ ์ ๋ ํฐ ๊ท๋ชจ์ ํธ๋์คํฌ๋จธ ๋ชจ๋ธ์ ํ๋ จํ๊ณ ํ๋ก๋์
์ ๋ฐฐํฌํ๋ ๋ฐ์๋ ๋ค์ํ ์ด๋ ค์์ด ๋ฐ๋ฆ
๋๋ค. ํ๋ จ ์ค์๋ ๋ชจ๋ธ์ด ์ฌ์ฉ ๊ฐ๋ฅํ GPU ๋ฉ๋ชจ๋ฆฌ๋ณด๋ค ๋ ๋ง์ ๋ฉ๋ชจ๋ฆฌ๋ฅผ ํ์๋ก ํ๊ฑฐ๋ ํ๋ จ ์๋๊ฐ ๋งค์ฐ ๋๋ฆด ์ ์์ผ๋ฉฐ, ์ถ๋ก ์ ์ํด ๋ฐฐํฌํ ๋๋ ์ ํ ํ๊ฒฝ์์ ์๊ตฌ๋๋ ์ฒ๋ฆฌ๋์ผ๋ก ์ธํด ๊ณผ๋ถํ๊ฐ ๋ฐ์ํ ์ ์์ต๋๋ค. ์ด ๋ฌธ์๋ ์ด๋ฌํ ๋ฌธ์ ๋ฅผ ๊ทน๋ณตํ๊ณ ์ฌ์ฉ ์ฌ๋ก์ ๊ฐ์ฅ ์ ํฉํ ์ค์ ์ ์ฐพ๋๋ก ๋์์ ์ฃผ๊ธฐ ์ํด ์ค๊ณ๋์์ต๋๋ค. ํ๋ จ๊ณผ ์ถ๋ก ์ผ๋ก ๊ฐ์ด๋๋ฅผ ๋ถํ ํ๋๋ฐ, ์ด๋ ๊ฐ๊ฐ ๋ค๋ฅธ ๋ฌธ์ ์ ํด๊ฒฐ ๋ฐฉ๋ฒ์ด ์๊ธฐ ๋๋ฌธ์
๋๋ค. ๊ทธ๋ฆฌ๊ณ ๊ฐ ๊ฐ์ด๋์๋ ๋ค์ํ ์ข
๋ฅ์ ํ๋์จ์ด ์ค์ ์ ๋ํ ๋ณ๋์ ๊ฐ์ด๋๊ฐ ์์ต๋๋ค(์: ํ๋ จ์ ์ํ ๋จ์ผ GPU vs ๋ค์ค GPU ๋๋ ์ถ๋ก ์ ์ํ CPU vs GPU).
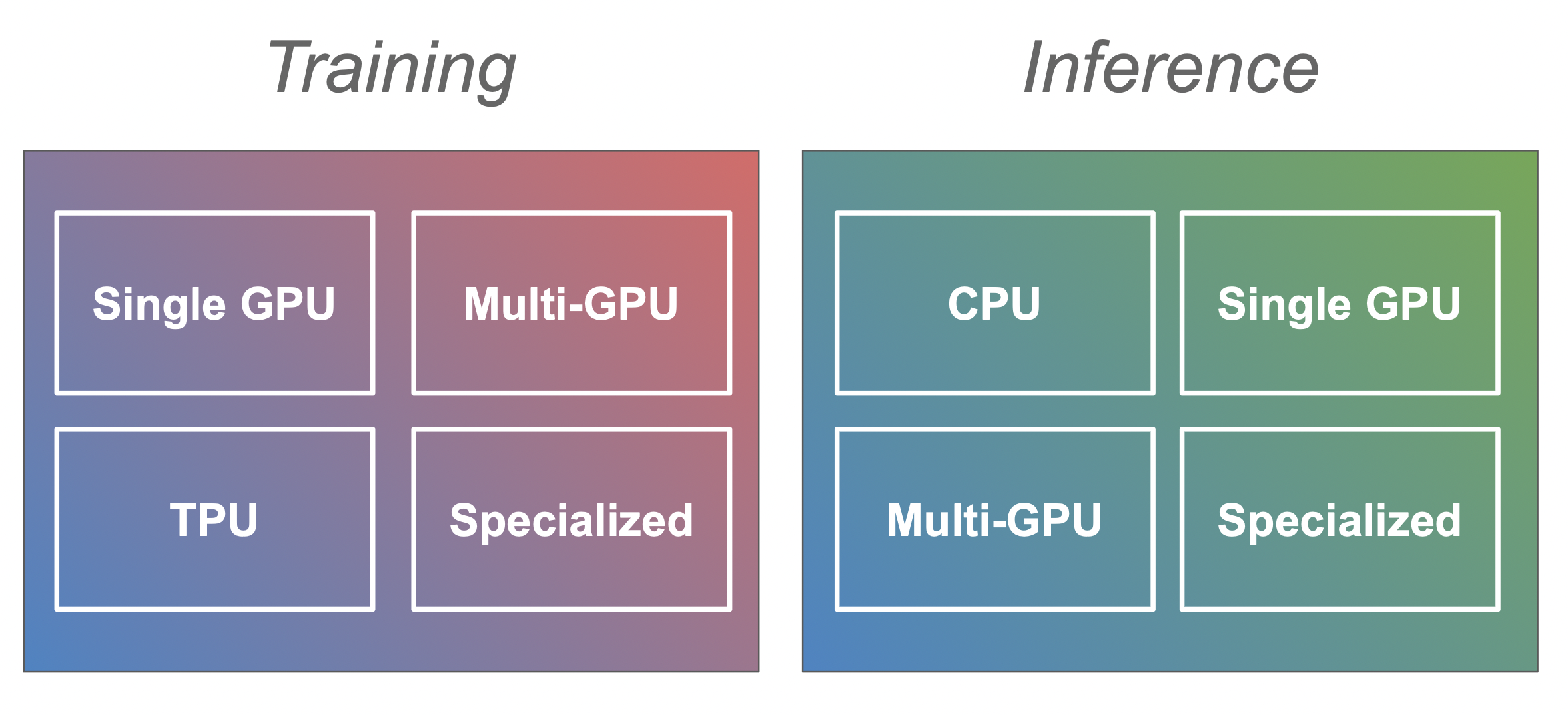
์ด ๋ฌธ์๋ ์ฌ์ฉ์์ ์ํฉ์ ์ ์ฉํ ์ ์๋ ๋ฐฉ๋ฒ๋ค์ ๋ํ ๊ฐ์ ๋ฐ ์์์ ์ญํ ์ ํฉ๋๋ค.
## ํ๋ จ [[training]]
ํจ์จ์ ์ธ ํธ๋์คํฌ๋จธ ๋ชจ๋ธ ํ๋ จ์๋ GPU๋ TPU์ ๊ฐ์ ๊ฐ์๊ธฐ๊ฐ ํ์ํฉ๋๋ค. ๊ฐ์ฅ ์ผ๋ฐ์ ์ธ ๊ฒฝ์ฐ๋ ๋จ์ผ GPU๋ง ์ฌ์ฉํ๋ ๊ฒฝ์ฐ์ง๋ง, ๋ค์ค GPU ๋ฐ CPU ํ๋ จ์ ๋ํ ์น์
๋ ์์ต๋๋ค(๊ณง ๋ ๋ง์ ๋ด์ฉ์ด ์ถ๊ฐ๋ ์์ ).
<Tip>
์ฐธ๊ณ : ๋จ์ผ GPU ์น์
์์ ์๊ฐ๋ ๋๋ถ๋ถ์ ์ ๋ต(์: ํผํฉ ์ ๋ฐ๋ ํ๋ จ ๋๋ ๊ทธ๋ผ๋์ธํธ ๋์ )์ ์ผ๋ฐ์ ์ธ ๋ชจ๋ธ ํ๋ จ์๋ ์ ์ฉ๋๋ฏ๋ก, ๋ค์ค GPU๋ CPU ํ๋ จ๊ณผ ๊ฐ์ ์น์
์ ์ดํด๋ณด๊ธฐ ์ ์ ๊ผญ ์ฐธ๊ณ ํ์๊ธธ ๋ฐ๋๋๋ค.
</Tip>
### ๋จ์ผ GPU [[single-gpu]]
๋จ์ผ GPU์์ ๋๊ท๋ชจ ๋ชจ๋ธ์ ํ๋ จํ๋ ๊ฒ์ ์ด๋ ค์ธ ์ ์์ง๋ง, ์ด๋ฅผ ๊ฐ๋ฅํ๊ฒ ํ๋ ์ฌ๋ฌ ๊ฐ์ง ๋๊ตฌ์ ๋ฐฉ๋ฒ์ด ์์ต๋๋ค. ์ด ์น์
์์๋ ํผํฉ ์ ๋ฐ๋ ํ๋ จ, ๊ทธ๋ผ๋์ธํธ ๋์ ๋ฐ ์ฒดํฌํฌ์ธํ
, ํจ์จ์ ์ธ ์ตํฐ๋ง์ด์ , ์ต์ ์ ๋ฐฐ์น ํฌ๊ธฐ๋ฅผ ๊ฒฐ์ ํ๊ธฐ ์ํ ์ ๋ต ๋ฑ์ ๋ํด ๋
ผ์ํฉ๋๋ค.
[๋จ์ผ GPU ํ๋ จ ์น์
์ผ๋ก ์ด๋](perf_train_gpu_one)
### ๋ค์ค GPU [[multigpu]]
๋จ์ผ GPU์์ ํ๋ จํ๋ ๊ฒ์ด ๋๋ฌด ๋๋ฆฌ๊ฑฐ๋ ๋๊ท๋ชจ ๋ชจ๋ธ์ ์ ํฉํ์ง ์์ ๊ฒฝ์ฐ๋ ์์ต๋๋ค. ๋ค์ค GPU ์ค์ ์ผ๋ก ์ ํํ๋ ๊ฒ์ ๋
ผ๋ฆฌ์ ์ธ ๋จ๊ณ์ด์ง๋ง, ์ฌ๋ฌ GPU์์ ํ ๋ฒ์ ํ๋ จํ๋ ค๋ฉด ๊ฐ GPU๋ง๋ค ๋ชจ๋ธ์ ์ ์ฒด ์ฌ๋ณธ์ ๋์ง, ํน์ ๋ชจ๋ธ ์์ฒด๋ ์ฌ๋ฌ GPU์ ๋ถ์ฐํ์ฌ ๋์ง ๋ฑ ์๋ก์ด ๊ฒฐ์ ์ ๋ด๋ ค์ผ ํฉ๋๋ค. ์ด ์น์
์์๋ ๋ฐ์ดํฐ, ํ
์ ๋ฐ ํ์ดํ๋ผ์ธ ๋ณ๋ ฌํ์ ๋ํด ์ดํด๋ด
๋๋ค.
[๋ค์ค GPU ํ๋ จ ์น์
์ผ๋ก ์ด๋](perf_train_gpu_many)
### CPU [[cpu]]
[CPU ํ๋ จ ์น์
์ผ๋ก ์ด๋](perf_train_cpu)
### TPU [[tpu]]
[_๊ณง ์ ๊ณต๋ ์์ _](perf_train_tpu)
### ํน์ํ ํ๋์จ์ด [[specialized-hardware]]
[_๊ณง ์ ๊ณต๋ ์์ _](perf_train_special)
## ์ถ๋ก [[inference]]
์ ํ ๋ฐ ์๋น์ค ํ๊ฒฝ์์ ๋๊ท๋ชจ ๋ชจ๋ธ์ ํจ์จ์ ์ผ๋ก ์ถ๋ก ํ๋ ๊ฒ์ ๋ชจ๋ธ์ ํ๋ จํ๋ ๊ฒ๋งํผ ์ด๋ ค์ธ ์ ์์ต๋๋ค. ์ด์ด์ง๋ ์น์
์์๋ CPU ๋ฐ ๋จ์ผ/๋ค์ค GPU ์ค์ ์์ ์ถ๋ก ์ ์งํํ๋ ๋จ๊ณ๋ฅผ ์ดํด๋ด
๋๋ค.
### CPU [[cpu]]
[CPU ์ถ๋ก ์น์
์ผ๋ก ์ด๋](perf_infer_cpu)
### ๋จ์ผ GPU [[single-gpu]]
[๋จ์ผ GPU ์ถ๋ก ์น์
์ผ๋ก ์ด๋](perf_infer_gpu_one)
### ๋ค์ค GPU [[multigpu]]
[๋ค์ค GPU ์ถ๋ก ์น์
์ผ๋ก ์ด๋](perf_infer_gpu_many)
### ํน์ํ ํ๋์จ์ด [[specialized-hardware]]
[_๊ณง ์ ๊ณต๋ ์์ _](perf_infer_special)
## ํ๋์จ์ด [[hardware]]
ํ๋์จ์ด ์น์
์์๋ ์์ ๋ง์ ๋ฅ๋ฌ๋ ์ฅ๋น๋ฅผ ๊ตฌ์ถํ ๋ ์ ์ฉํ ํ๊ณผ ์๋ น์ ์ดํด๋ณผ ์ ์์ต๋๋ค.
[ํ๋์จ์ด ์น์
์ผ๋ก ์ด๋](perf_hardware)
## ๊ธฐ์ฌํ๊ธฐ [[contribute]]
์ด ๋ฌธ์๋ ์์ฑ๋์ง ์์ ์ํ์ด๋ฉฐ, ์ถ๊ฐํด์ผ ํ ๋ด์ฉ์ด๋ ์์ ์ฌํญ์ด ๋ง์ด ์์ต๋๋ค. ๋ฐ๋ผ์ ์ถ๊ฐํ๊ฑฐ๋ ์์ ํ ๋ด์ฉ์ด ์์ผ๋ฉด ์ฃผ์ ํ์ง ๋ง๊ณ PR์ ์ด์ด ์ฃผ์๊ฑฐ๋, ์์ธํ ๋ด์ฉ์ ๋
ผ์ํ๊ธฐ ์ํด Issue๋ฅผ ์์ํด ์ฃผ์๊ธฐ ๋ฐ๋๋๋ค.
A๊ฐ B๋ณด๋ค ์ข๋ค๊ณ ํ๋ ๊ธฐ์ฌ๋ฅผ ํ ๋๋, ์ฌํ ๊ฐ๋ฅํ ๋ฒค์น๋งํฌ์/๋๋ ํด๋น ์ ๋ณด์ ์ถ์ฒ ๋งํฌ๋ฅผ ํฌํจํด์ฃผ์ธ์(๋น์ ์ผ๋ก๋ถํฐ์ ์ง์ ์ ์ธ ์ ๋ณด๊ฐ ์๋ ๊ฒฝ์ฐ). | 0 |
hf_public_repos/transformers/docs/source | hf_public_repos/transformers/docs/source/ko/hpo_train.md | <!--Copyright 2022 The HuggingFace Team. All rights reserved.
Licensed under the Apache License, Version 2.0 (the "License"); you may not use this file except in compliance with
the License. You may obtain a copy of the License at
http://www.apache.org/licenses/LICENSE-2.0
Unless required by applicable law or agreed to in writing, software distributed under the License is distributed on
an "AS IS" BASIS, WITHOUT WARRANTIES OR CONDITIONS OF ANY KIND, either express or implied. See the License for the
โ ๏ธ Note that this file is in Markdown but contain specific syntax for our doc-builder (similar to MDX) that may not be
rendered properly in your Markdown viewer.
-->
# Trainer API๋ฅผ ์ฌ์ฉํ ํ์ดํผํ๋ผ๋ฏธํฐ ํ์ [[hyperparameter-search-using-trainer-api]]
๐ค Transformers์์๋ ๐ค Transformers ๋ชจ๋ธ์ ํ์ต์ํค๋๋ฐ ์ต์ ํ๋ [`Trainer`] ํด๋์ค๋ฅผ ์ ๊ณตํ๊ธฐ ๋๋ฌธ์, ์ฌ์ฉ์๋ ์ง์ ํ๋ จ ๋ฃจํ๋ฅผ ์์ฑํ ํ์ ์์ด ๋์ฑ ๊ฐํธํ๊ฒ ํ์ต์ ์ํฌ ์ ์์ต๋๋ค. ๋ํ, [`Trainer`]๋ ํ์ดํผํ๋ผ๋ฏธํฐ ํ์์ ์ํ API๋ฅผ ์ ๊ณตํฉ๋๋ค. ์ด ๋ฌธ์์์ ์ด API๋ฅผ ํ์ฉํ๋ ๋ฐฉ๋ฒ์ ์์์ ํจ๊ป ๋ณด์ฌ๋๋ฆฌ๊ฒ ์ต๋๋ค.
## ํ์ดํผํ๋ผ๋ฏธํฐ ํ์ ๋ฐฑ์๋ [[hyperparameter-search-backend]]
[`Trainer`]๋ ํ์ฌ ์๋ 4๊ฐ์ง ํ์ดํผํ๋ผ๋ฏธํฐ ํ์ ๋ฐฑ์๋๋ฅผ ์ง์ํฉ๋๋ค:
[optuna](https://optuna.org/)์ [sigopt](https://sigopt.com/), [raytune](https://docs.ray.io/en/latest/tune/index.html), [wandb](https://wandb.ai/site/sweeps) ์
๋๋ค.
ํ์ดํผํ๋ผ๋ฏธํฐ ํ์ ๋ฐฑ์๋๋ก ์ฌ์ฉํ๊ธฐ ์ ์ ์๋์ ๋ช
๋ น์ด๋ฅผ ์ฌ์ฉํ์ฌ ๋ผ์ด๋ธ๋ฌ๋ฆฌ๋ค์ ์ค์นํ์ธ์.
```bash
pip install optuna/sigopt/wandb/ray[tune]
```
## ์์ ์์ ํ์ดํผํ๋ผ๋ฏธํฐ ํ์์ ํ์ฑํํ๋ ๋ฐฉ๋ฒ [[how-to-enable-hyperparameter-search-in-example]]
ํ์ดํผํ๋ผ๋ฏธํฐ ํ์ ๊ณต๊ฐ์ ์ ์ํ์ธ์. ํ์ดํผํ๋ผ๋ฏธํฐ ํ์ ๋ฐฑ์๋๋ง๋ค ์๋ก ๋ค๋ฅธ ํ์์ด ํ์ํฉ๋๋ค.
sigopt์ ๊ฒฝ์ฐ, ํด๋น [object_parameter](https://docs.sigopt.com/ai-module-api-references/api_reference/objects/object_parameter) ๋ฌธ์๋ฅผ ์ฐธ์กฐํ์ฌ ์๋์ ๊ฐ์ด ์์ฑํ์ธ์:
```py
>>> def sigopt_hp_space(trial):
... return [
... {"bounds": {"min": 1e-6, "max": 1e-4}, "name": "learning_rate", "type": "double"},
... {
... "categorical_values": ["16", "32", "64", "128"],
... "name": "per_device_train_batch_size",
... "type": "categorical",
... },
... ]
```
optuna์ ๊ฒฝ์ฐ, ํด๋น [object_parameter](https://optuna.readthedocs.io/en/stable/tutorial/10_key_features/002_configurations.html#sphx-glr-tutorial-10-key-features-002-configurations-py) ๋ฌธ์๋ฅผ ์ฐธ์กฐํ์ฌ ์๋์ ๊ฐ์ด ์์ฑํ์ธ์:
```py
>>> def optuna_hp_space(trial):
... return {
... "learning_rate": trial.suggest_float("learning_rate", 1e-6, 1e-4, log=True),
... "per_device_train_batch_size": trial.suggest_categorical("per_device_train_batch_size", [16, 32, 64, 128]),
... }
```
raytune์ ๊ฒฝ์ฐ, ํด๋น [object_parameter](https://docs.ray.io/en/latest/tune/api/search_space.html) ๋ฌธ์๋ฅผ ์ฐธ์กฐํ์ฌ ์๋์ ๊ฐ์ด ์์ฑํ์ธ์:
```py
>>> def ray_hp_space(trial):
... return {
... "learning_rate": tune.loguniform(1e-6, 1e-4),
... "per_device_train_batch_size": tune.choice([16, 32, 64, 128]),
... }
```
wandb์ ๊ฒฝ์ฐ, ํด๋น [object_parameter](https://docs.wandb.ai/guides/sweeps/configuration) ๋ฌธ์๋ฅผ ์ฐธ์กฐํ์ฌ ์๋์ ๊ฐ์ด ์์ฑํ์ธ์:
```py
>>> def wandb_hp_space(trial):
... return {
... "method": "random",
... "metric": {"name": "objective", "goal": "minimize"},
... "parameters": {
... "learning_rate": {"distribution": "uniform", "min": 1e-6, "max": 1e-4},
... "per_device_train_batch_size": {"values": [16, 32, 64, 128]},
... },
... }
```
`model_init` ํจ์๋ฅผ ์ ์ํ๊ณ ์ด๋ฅผ [`Trainer`]์ ์ ๋ฌํ์ธ์. ์๋๋ ๊ทธ ์์์
๋๋ค.
```py
>>> def model_init(trial):
... return AutoModelForSequenceClassification.from_pretrained(
... model_args.model_name_or_path,
... from_tf=bool(".ckpt" in model_args.model_name_or_path),
... config=config,
... cache_dir=model_args.cache_dir,
... revision=model_args.model_revision,
... use_auth_token=True if model_args.use_auth_token else None,
... )
```
์๋์ ๊ฐ์ด `model_init` ํจ์, ํ๋ จ ์ธ์, ํ๋ จ ๋ฐ ํ
์คํธ ๋ฐ์ดํฐ์
, ๊ทธ๋ฆฌ๊ณ ํ๊ฐ ํจ์๋ฅผ ์ฌ์ฉํ์ฌ [`Trainer`]๋ฅผ ์์ฑํ์ธ์:
```py
>>> trainer = Trainer(
... model=None,
... args=training_args,
... train_dataset=small_train_dataset,
... eval_dataset=small_eval_dataset,
... compute_metrics=compute_metrics,
... tokenizer=tokenizer,
... model_init=model_init,
... data_collator=data_collator,
... )
```
ํ์ดํผํ๋ผ๋ฏธํฐ ํ์์ ํธ์ถํ๊ณ , ์ต์ ์ ์ํ ๋งค๊ฐ๋ณ์๋ฅผ ๊ฐ์ ธ์ค์ธ์. ๋ฐฑ์๋๋ `"optuna"`/`"sigopt"`/`"wandb"`/`"ray"` ์ค์์ ์ ํํ ์ ์์ต๋๋ค. ๋ฐฉํฅ์ `"minimize"` ๋๋ `"maximize"` ์ค ์ ํํ๋ฉฐ, ๋ชฉํ๋ฅผ ์ต์ํํ ๊ฒ์ธ์ง ์ต๋ํํ ๊ฒ์ธ์ง๋ฅผ ๊ฒฐ์ ํฉ๋๋ค.
์์ ๋ง์ compute_objective ํจ์๋ฅผ ์ ์ํ ์ ์์ต๋๋ค. ๋ง์ฝ ์ด ํจ์๋ฅผ ์ ์ํ์ง ์์ผ๋ฉด, ๊ธฐ๋ณธ compute_objective๊ฐ ํธ์ถ๋๊ณ , f1๊ณผ ๊ฐ์ ํ๊ฐ ์งํ์ ํฉ์ด ๋ชฉํฏ๊ฐ์ผ๋ก ๋ฐํ๋ฉ๋๋ค.
```py
>>> best_trial = trainer.hyperparameter_search(
... direction="maximize",
... backend="optuna",
... hp_space=optuna_hp_space,
... n_trials=20,
... compute_objective=compute_objective,
... )
```
## DDP ๋ฏธ์ธ ์กฐ์ ์ ์ํ ํ์ดํผํ๋ผ๋ฏธํฐ ํ์ [[hyperparameter-search-for-ddp-finetune]]
ํ์ฌ, DDP(Distributed Data Parallelism; ๋ถ์ฐ ๋ฐ์ดํฐ ๋ณ๋ ฌ์ฒ๋ฆฌ)๋ฅผ ์ํ ํ์ดํผํ๋ผ๋ฏธํฐ ํ์์ optuna์ sigopt์์ ๊ฐ๋ฅํฉ๋๋ค. ์ต์์ ํ๋ก์ธ์ค๊ฐ ํ์ดํผํ๋ผ๋ฏธํฐ ํ์ ๊ณผ์ ์ ์์ํ๊ณ ๊ทธ ๊ฒฐ๊ณผ๋ฅผ ๋ค๋ฅธ ํ๋ก์ธ์ค์ ์ ๋ฌํฉ๋๋ค.
| 0 |
hf_public_repos/transformers/docs/source | hf_public_repos/transformers/docs/source/ko/perf_infer_cpu.md | <!--Copyright 2022 The HuggingFace Team. All rights reserved.
Licensed under the Apache License, Version 2.0 (the "License"); you may not use this file except in compliance with
the License. You may obtain a copy of the License at
http://www.apache.org/licenses/LICENSE-2.0
Unless required by applicable law or agreed to in writing, software distributed under the License is distributed on
an "AS IS" BASIS, WITHOUT WARRANTIES OR CONDITIONS OF ANY KIND, either express or implied. See the License for the
โ ๏ธ Note that this file is in Markdown but contain specific syntax for our doc-builder (similar to MDX) that may not be
rendered properly in your Markdown viewer.
-->
# CPU์์ ํจ์จ์ ์ธ ์ถ๋ก ํ๊ธฐ [[efficient-inference-on-cpu]]
์ด ๊ฐ์ด๋๋ CPU์์ ๋๊ท๋ชจ ๋ชจ๋ธ์ ํจ์จ์ ์ผ๋ก ์ถ๋ก ํ๋ ๋ฐฉ๋ฒ์ ์ค์ ์ ๋๊ณ ์์ต๋๋ค.
## ๋ ๋น ๋ฅธ ์ถ๋ก ์ ์ํ `BetterTransformer` [[bettertransformer-for-faster-inference]]
์ฐ๋ฆฌ๋ ์ต๊ทผ CPU์์ ํ
์คํธ, ์ด๋ฏธ์ง ๋ฐ ์ค๋์ค ๋ชจ๋ธ์ ๋น ๋ฅธ ์ถ๋ก ์ ์ํด `BetterTransformer`๋ฅผ ํตํฉํ์ต๋๋ค. ์ด ํตํฉ์ ๋ํ ๋ ์์ธํ ๋ด์ฉ์ [์ด ๋ฌธ์](https://huggingface.co/docs/optimum/bettertransformer/overview)๋ฅผ ์ฐธ์กฐํ์ธ์.
## PyTorch JIT ๋ชจ๋ (TorchScript) [[pytorch-jitmode-torchscript]]
TorchScript๋ PyTorch ์ฝ๋์์ ์ง๋ ฌํ์ ์ต์ ํ๊ฐ ๊ฐ๋ฅํ ๋ชจ๋ธ์ ์์ฑํ ๋ ์ฐ์
๋๋ค. TorchScript๋ก ๋ง๋ค์ด์ง ํ๋ก๊ทธ๋จ์ ๊ธฐ์กด Python ํ๋ก์ธ์ค์์ ์ ์ฅํ ๋ค, ์ข
์์ฑ์ด ์๋ ์๋ก์ด ํ๋ก์ธ์ค๋ก ๊ฐ์ ธ์ฌ ์ ์์ต๋๋ค. PyTorch์ ๊ธฐ๋ณธ ์ค์ ์ธ `eager` ๋ชจ๋์ ๋น๊ตํ์๋, `jit` ๋ชจ๋๋ ์ฐ์ฐ์ ๊ฒฐํฉ๊ณผ ๊ฐ์ ์ต์ ํ ๋ฐฉ๋ฒ๋ก ์ ํตํด ๋ชจ๋ธ ์ถ๋ก ์์ ๋๋ถ๋ถ ๋ ๋์ ์ฑ๋ฅ์ ์ ๊ณตํฉ๋๋ค.
TorchScript์ ๋ํ ์น์ ํ ์๊ฐ๋ [PyTorch TorchScript ํํ ๋ฆฌ์ผ](https://pytorch.org/tutorials/beginner/Intro_to_TorchScript_tutorial.html#tracing-modules)์ ์ฐธ์กฐํ์ธ์.
### JIT ๋ชจ๋์ ํจ๊ปํ๋ IPEX ๊ทธ๋ํ ์ต์ ํ [[ipex-graph-optimization-with-jitmode]]
Intelยฎ Extension for PyTorch(IPEX)๋ Transformers ๊ณ์ด ๋ชจ๋ธ์ jit ๋ชจ๋์์ ์ถ๊ฐ์ ์ธ ์ต์ ํ๋ฅผ ์ ๊ณตํฉ๋๋ค. jit ๋ชจ๋์ ๋๋ถ์ด Intelยฎ Extension for PyTorch(IPEX)๋ฅผ ํ์ฉํ์๊ธธ ๊ฐ๋ ฅํ ๊ถ์ฅ๋๋ฆฝ๋๋ค. Transformers ๋ชจ๋ธ์์ ์์ฃผ ์ฌ์ฉ๋๋ ์ผ๋ถ ์ฐ์ฐ์ ํจํด์ ์ด๋ฏธ jit ๋ชจ๋ ์ฐ์ฐ์ ๊ฒฐํฉ(operator fusion)์ ํํ๋ก Intelยฎ Extension for PyTorch(IPEX)์์ ์ง์๋๊ณ ์์ต๋๋ค. Multi-head-attention, Concat Linear, Linear+Add, Linear+Gelu, Add+LayerNorm ๊ฒฐํฉ ํจํด ๋ฑ์ด ์ด์ฉ ๊ฐ๋ฅํ๋ฉฐ ํ์ฉํ์ ๋ ์ฑ๋ฅ์ด ์ฐ์ํฉ๋๋ค. ์ฐ์ฐ์ ๊ฒฐํฉ์ ์ด์ ์ ์ฌ์ฉ์์๊ฒ ๊ณ ์ค๋ํ ์ ๋ฌ๋ฉ๋๋ค. ๋ถ์์ ๋ฐ๋ฅด๋ฉด, ์ง์ ์๋ต, ํ
์คํธ ๋ถ๋ฅ ๋ฐ ํ ํฐ ๋ถ๋ฅ์ ๊ฐ์ ๊ฐ์ฅ ์ธ๊ธฐ ์๋ NLP ํ์คํฌ ์ค ์ฝ 70%๊ฐ ์ด๋ฌํ ๊ฒฐํฉ ํจํด์ ์ฌ์ฉํ์ฌ Float32 ์ ๋ฐ๋์ BFloat16 ํผํฉ ์ ๋ฐ๋ ๋ชจ๋์์ ์ฑ๋ฅ์์ ์ด์ ์ ์ป์ ์ ์์ต๋๋ค.
[IPEX ๊ทธ๋ํ ์ต์ ํ](https://intel.github.io/intel-extension-for-pytorch/cpu/latest/tutorials/features/graph_optimization.html)์ ๋ํ ์์ธํ ์ ๋ณด๋ฅผ ํ์ธํ์ธ์.
#### IPEX ์ค์น: [[ipex-installation]]
IPEX ๋ฐฐํฌ ์ฃผ๊ธฐ๋ PyTorch๋ฅผ ๋ฐ๋ผ์ ์ด๋ฃจ์ด์ง๋๋ค. ์์ธํ ์ ๋ณด๋ [IPEX ์ค์น ๋ฐฉ๋ฒ](https://intel.github.io/intel-extension-for-pytorch/)์ ํ์ธํ์ธ์.
### JIT ๋ชจ๋ ์ฌ์ฉ๋ฒ [[usage-of-jitmode]]
ํ๊ฐ ๋๋ ์์ธก์ ์ํด Trainer์์ JIT ๋ชจ๋๋ฅผ ์ฌ์ฉํ๋ ค๋ฉด Trainer์ ๋ช
๋ น ์ธ์์ `jit_mode_eval`์ ์ถ๊ฐํด์ผ ํฉ๋๋ค.
<Tip warning={true}>
PyTorch์ ๋ฒ์ ์ด 1.14.0 ์ด์์ด๋ผ๋ฉด, jit ๋ชจ๋๋ jit.trace์์ dict ์
๋ ฅ์ด ์ง์๋๋ฏ๋ก, ๋ชจ๋ ๋ชจ๋ธ์ ์์ธก๊ณผ ํ๊ฐ๊ฐ ๊ฐ์ ๋ ์ ์์ต๋๋ค.
PyTorch์ ๋ฒ์ ์ด 1.14.0 ๋ฏธ๋ง์ด๋ผ๋ฉด, ์ง์ ์๋ต ๋ชจ๋ธ๊ณผ ๊ฐ์ด forward ๋งค๊ฐ๋ณ์์ ์์๊ฐ jit.trace์ ํํ ์
๋ ฅ ์์์ ์ผ์นํ๋ ๋ชจ๋ธ์ ๋์ด ๋ ์ ์์ต๋๋ค. ํ
์คํธ ๋ถ๋ฅ ๋ชจ๋ธ๊ณผ ๊ฐ์ด forward ๋งค๊ฐ๋ณ์ ์์๊ฐ jit.trace์ ํํ ์
๋ ฅ ์์์ ๋ค๋ฅธ ๊ฒฝ์ฐ, jit.trace๊ฐ ์คํจํ๋ฉฐ ์์ธ๊ฐ ๋ฐ์ํฉ๋๋ค. ์ด๋ ์์ธ์ํฉ์ ์ฌ์ฉ์์๊ฒ ์๋ฆฌ๊ธฐ ์ํด Logging์ด ์ฌ์ฉ๋ฉ๋๋ค.
</Tip>
[Transformers ์ง์ ์๋ต](https://github.com/huggingface/transformers/tree/main/examples/pytorch/question-answering)์ ์ฌ์ฉ ์ฌ๋ก ์์๋ฅผ ์ฐธ์กฐํ์ธ์.
- CPU์์ jit ๋ชจ๋๋ฅผ ์ฌ์ฉํ ์ถ๋ก :
<pre>python run_qa.py \
--model_name_or_path csarron/bert-base-uncased-squad-v1 \
--dataset_name squad \
--do_eval \
--max_seq_length 384 \
--doc_stride 128 \
--output_dir /tmp/ \
--no_cuda \
<b>--jit_mode_eval </b></pre>
- CPU์์ IPEX์ ํจ๊ป jit ๋ชจ๋๋ฅผ ์ฌ์ฉํ ์ถ๋ก :
<pre>python run_qa.py \
--model_name_or_path csarron/bert-base-uncased-squad-v1 \
--dataset_name squad \
--do_eval \
--max_seq_length 384 \
--doc_stride 128 \
--output_dir /tmp/ \
--no_cuda \
<b>--use_ipex \</b>
<b>--jit_mode_eval</b></pre>
| 0 |
hf_public_repos/transformers/docs/source | hf_public_repos/transformers/docs/source/ko/model_sharing.md | <!--Copyright 2022 The HuggingFace Team. All rights reserved.
Licensed under the Apache License, Version 2.0 (the "License"); you may not use this file except in compliance with
the License. You may obtain a copy of the License at
http://www.apache.org/licenses/LICENSE-2.0
Unless required by applicable law or agreed to in writing, software distributed under the License is distributed on
an "AS IS" BASIS, WITHOUT WARRANTIES OR CONDITIONS OF ANY KIND, either express or implied. See the License for the
specific language governing permissions and limitations under the License.
โ ๏ธ Note that this file is in Markdown but contain specific syntax for our doc-builder (similar to MDX) that may not be
rendered properly in your Markdown viewer.
-->
# ๋ชจ๋ธ ๊ณต์ ํ๊ธฐ[[share-a-model]]
์ง๋ ๋ ํํ ๋ฆฌ์ผ์์ ๋ถ์ฐ ์ค์ ์ ์ํด PyTorch, Keras ๋ฐ ๐ค Accelerate๋ฅผ ์ฌ์ฉํ์ฌ ๋ชจ๋ธ์ ๋ฏธ์ธ ์กฐ์ ํ๋ ๋ฐฉ๋ฒ์ ๋ณด์์ต๋๋ค. ๋ค์ ๋จ๊ณ๋ ๋ชจ๋ธ์ ์ปค๋ฎค๋ํฐ์ ๊ณต์ ํ๋ ๊ฒ์
๋๋ค! Hugging Face๋ ์ธ๊ณต์ง๋ฅ์ ๋ฏผ์ฃผํ๋ฅผ ์ํด ๋ชจ๋์๊ฒ ์ง์๊ณผ ์์์ ๊ณต๊ฐ์ ์ผ๋ก ๊ณต์ ํด์ผ ํ๋ค๊ณ ๋ฏฟ์ต๋๋ค. ๋ค๋ฅธ ์ฌ๋๋ค์ด ์๊ฐ๊ณผ ์์์ ์ ์ฝํ ์ ์๋๋ก ์ปค๋ฎค๋ํฐ์ ๋ชจ๋ธ์ ๊ณต์ ํ๋ ๊ฒ์ ๊ณ ๋ คํด ๋ณด์ธ์.
์ด ํํ ๋ฆฌ์ผ์์ [Model Hub](https://huggingface.co/models)์์ ํ๋ จ๋๊ฑฐ๋ ๋ฏธ์ธ ์กฐ์ ๋ชจ๋ธ์ ๊ณต์ ํ๋ ๋ ๊ฐ์ง ๋ฐฉ๋ฒ์ ๋ํด ์์๋ด
์๋ค:
- API๋ฅผ ํตํด ํ์ผ์ Hub์ ํธ์ํฉ๋๋ค.
- ์น์ฌ์ดํธ๋ฅผ ํตํด ํ์ผ์ Hub๋ก ๋์ด๋ค ๋์ต๋๋ค.
<iframe width="560" height="315" src="https://www.youtube.com/embed/XvSGPZFEjDY" title="YouTube video player"
frameborder="0" allow="accelerometer; autoplay; clipboard-write; encrypted-media; gyroscope;
picture-in-picture" allowfullscreen></iframe>
<Tip>
์ปค๋ฎค๋ํฐ์ ๋ชจ๋ธ์ ๊ณต์ ํ๋ ค๋ฉด, [huggingface.co](https://huggingface.co/join)์ ๊ณ์ ์ด ํ์ํฉ๋๋ค. ๊ธฐ์กด ์กฐ์ง์ ๊ฐ์
ํ๊ฑฐ๋ ์๋ก ๋ง๋ค ์๋ ์์ต๋๋ค.
</Tip>
## ์ ์ฅ์ ํน์ง[[repository-features]]
๋ชจ๋ธ ํ๋ธ์ ๊ฐ ์ ์ฅ์๋ ์ผ๋ฐ์ ์ธ GitHub ์ ์ฅ์์ฒ๋ผ ์๋ํฉ๋๋ค. ์ ์ฅ์๋ ๋ฒ์ ๊ด๋ฆฌ, ์ปค๋ฐ ๊ธฐ๋ก, ์ฐจ์ด์ ์๊ฐํ ๊ธฐ๋ฅ์ ์ ๊ณตํฉ๋๋ค.
๋ชจ๋ธ ํ๋ธ์ ๋ด์ฅ๋ ๋ฒ์ ๊ด๋ฆฌ๋ git ๋ฐ [git-lfs](https://git-lfs.github.com/)๋ฅผ ๊ธฐ๋ฐ์ผ๋ก ํฉ๋๋ค. ์ฆ, ํ๋์ ๋ชจ๋ธ์ ํ๋์ ์ ์ฅ์๋ก ์ทจ๊ธํ์ฌ ์ ๊ทผ ์ ์ด ๋ฐ ํ์ฅ์ฑ์ด ํฅ์๋ฉ๋๋ค. ๋ฒ์ ์ ์ด๋ ์ปค๋ฐ ํด์, ํ๊ทธ ๋๋ ๋ธ๋์น๋ก ๋ชจ๋ธ์ ํน์ ๋ฒ์ ์ ๊ณ ์ ํ๋ ๋ฐฉ๋ฒ์ธ *revision*์ ํ์ฉํฉ๋๋ค.
๋ฐ๋ผ์ `revision` ๋งค๊ฐ๋ณ์๋ฅผ ์ฌ์ฉํ์ฌ ํน์ ๋ชจ๋ธ ๋ฒ์ ์ ๊ฐ์ ธ์ฌ ์ ์์ต๋๋ค:
```py
>>> model = AutoModel.from_pretrained(
... "julien-c/EsperBERTo-small", revision="v2.0.1" # tag name, or branch name, or commit hash
... )
```
๋ํ ์ ์ฅ์์์ ํ์ผ์ ์ฝ๊ฒ ํธ์งํ ์ ์์ผ๋ฉฐ, ์ปค๋ฐ ๊ธฐ๋ก๊ณผ ์ฐจ์ด๋ฅผ ๋ณผ ์ ์์ต๋๋ค:

## ์ค์ [[setup]]
๋ชจ๋ธ์ ํ๋ธ์ ๊ณต์ ํ๊ธฐ ์ ์ Hugging Face ์๊ฒฉ ์ฆ๋ช
์ด ํ์ํฉ๋๋ค. ํฐ๋ฏธ๋์ ์ก์ธ์คํ ์ ์๋ ๊ฒฝ์ฐ, ๐ค Transformers๊ฐ ์ค์น๋ ๊ฐ์ ํ๊ฒฝ์์ ๋ค์ ๋ช
๋ น์ ์คํํฉ๋๋ค. ๊ทธ๋ฌ๋ฉด Hugging Face ์บ์ ํด๋(๊ธฐ๋ณธ์ ์ผ๋ก `~/.cache/`)์ ์ก์ธ์ค ํ ํฐ์ ์ ์ฅํฉ๋๋ค:
```bash
huggingface-cli login
```
Jupyter ๋๋ Colaboratory์ ๊ฐ์ ๋
ธํธ๋ถ์ ์ฌ์ฉ ์ค์ธ ๊ฒฝ์ฐ, [`huggingface_hub`](https://huggingface.co/docs/hub/adding-a-library) ๋ผ์ด๋ธ๋ฌ๋ฆฌ๊ฐ ์ค์น๋์๋์ง ํ์ธํ์ธ์. ์ด ๋ผ์ด๋ธ๋ฌ๋ฆฌ๋ฅผ ์ฌ์ฉํ๋ฉด API๋ก ํ๋ธ์ ์ํธ ์์ฉํ ์ ์์ต๋๋ค.
```bash
pip install huggingface_hub
```
๊ทธ๋ฐ ๋ค์ `notebook_login`๋ก ํ๋ธ์ ๋ก๊ทธ์ธํ๊ณ , [์ฌ๊ธฐ](https://huggingface.co/settings/token) ๋งํฌ์์ ๋ก๊ทธ์ธํ ํ ํฐ์ ์์ฑํฉ๋๋ค:
```py
>>> from huggingface_hub import notebook_login
>>> notebook_login()
```
## ํ๋ ์์ํฌ ๊ฐ ๋ชจ๋ธ ๋ณํํ๊ธฐ[[convert-a-model-for-all-frameworks]]
๋ค๋ฅธ ํ๋ ์์ํฌ๋ก ์์
ํ๋ ์ฌ์ฉ์๊ฐ ๋ชจ๋ธ์ ์ฌ์ฉํ ์ ์๋๋ก ํ๋ ค๋ฉด, PyTorch ๋ฐ TensorFlow ์ฒดํฌํฌ์ธํธ๋ฅผ ๋ชจ๋ ์ฌ์ฉํ์ฌ ๋ชจ๋ธ์ ๋ณํํ๊ณ ์
๋ก๋ํ๋ ๊ฒ์ด ์ข์ต๋๋ค. ์ด ๋จ๊ณ๋ฅผ ๊ฑด๋๋ฐ์ด๋ ์ฌ์ฉ์๋ ๋ค๋ฅธ ํ๋ ์์ํฌ์์ ๋ชจ๋ธ์ ๊ฐ์ ธ์ฌ ์ ์์ง๋ง, ๐ค Transformers๊ฐ ์ฒดํฌํฌ์ธํธ๋ฅผ ์ฆ์์์ ๋ณํํด์ผ ํ๋ฏ๋ก ์๋๊ฐ ๋๋ ค์ง ์ ์์ต๋๋ค.
์ฒดํฌํฌ์ธํธ๋ฅผ ๋ค๋ฅธ ํ๋ ์์ํฌ๋ก ๋ณํํ๋ ๊ฒ์ ์ฝ์ต๋๋ค. PyTorch ๋ฐ TensorFlow๊ฐ ์ค์น๋์ด ์๋์ง ํ์ธํ ๋ค์(์ค์น ์ง์นจ์ [์ฌ๊ธฐ](installation) ์ฐธ์กฐ) ๋ค๋ฅธ ํ๋ ์์ํฌ์์ ์์
์ ๋ํ ํน์ ๋ชจ๋ธ์ ์ฐพ์ต๋๋ค.
<frameworkcontent>
<pt>
์ฒดํฌํฌ์ธํธ๋ฅผ TensorFlow์์ PyTorch๋ก ๋ณํํ๋ ค๋ฉด `from_tf=True`๋ฅผ ์ง์ ํ์ธ์:
```py
>>> pt_model = DistilBertForSequenceClassification.from_pretrained("path/to/awesome-name-you-picked", from_tf=True)
>>> pt_model.save_pretrained("path/to/awesome-name-you-picked")
```
</pt>
<tf>
์ฒดํฌํฌ์ธํธ๋ฅผ PyTorch์์ TensorFlow๋ก ๋ณํํ๋ ค๋ฉด `from_pt=True`๋ฅผ ์ง์ ํ์ธ์:
```py
>>> tf_model = TFDistilBertForSequenceClassification.from_pretrained("path/to/awesome-name-you-picked", from_pt=True)
```
๊ทธ๋ฐ ๋ค์ ์๋ก์ด ์ฒดํฌํฌ์ธํธ์ ํจ๊ป ์๋ก์ด TensorFlow ๋ชจ๋ธ์ ์ ์ฅํ ์ ์์ต๋๋ค:
```py
>>> tf_model.save_pretrained("path/to/awesome-name-you-picked")
```
</tf>
<jax>
Flax์์ ๋ชจ๋ธ์ ์ฌ์ฉํ๋ ๊ฒฝ์ฐ, PyTorch์์ Flax๋ก ์ฒดํฌํฌ์ธํธ๋ฅผ ๋ณํํ ์๋ ์์ต๋๋ค:
```py
>>> flax_model = FlaxDistilBertForSequenceClassification.from_pretrained(
... "path/to/awesome-name-you-picked", from_pt=True
... )
```
</jax>
</frameworkcontent>
## ํ๋ จ ์ค ๋ชจ๋ธ ํธ์ํ๊ธฐ[[push-a-model-during-training]]
<frameworkcontent>
<pt>
<Youtube id="Z1-XMy-GNLQ"/>
๋ชจ๋ธ์ ํ๋ธ์ ๊ณต์ ํ๋ ๊ฒ์ ์ถ๊ฐ ๋งค๊ฐ๋ณ์๋ ์ฝ๋ฐฑ์ ์ถ๊ฐํ๋ ๊ฒ๋งํผ ๊ฐ๋จํฉ๋๋ค. [๋ฏธ์ธ ์กฐ์ ํํ ๋ฆฌ์ผ](training)์์ [`TrainingArguments`] ํด๋์ค๋ ํ์ดํผํ๋ผ๋ฏธํฐ์ ์ถ๊ฐ ํ๋ จ ์ต์
์ ์ง์ ํ๋ ๊ณณ์ด๋ผ๋ ๊ฒ์ ๊ธฐ์ตํ์ธ์. ์ด๋ฌํ ํ๋ จ ์ต์
์ค ํ๋๋ ๋ชจ๋ธ์ ํ๋ธ๋ก ์ง์ ํธ์ํ๋ ๊ธฐ๋ฅ์ ํฌํจํฉ๋๋ค. [`TrainingArguments`]์์ `push_to_hub=True`๋ฅผ ์ค์ ํ์ธ์:
```py
>>> training_args = TrainingArguments(output_dir="my-awesome-model", push_to_hub=True)
```
ํ์์ ๊ฐ์ด ํ๋ จ ์ธ์๋ฅผ [`Trainer`]์ ์ ๋ฌํฉ๋๋ค:
```py
>>> trainer = Trainer(
... model=model,
... args=training_args,
... train_dataset=small_train_dataset,
... eval_dataset=small_eval_dataset,
... compute_metrics=compute_metrics,
... )
```
๋ชจ๋ธ์ ๋ฏธ์ธ ์กฐ์ ํ ํ, [`Trainer`]์์ [`~transformers.Trainer.push_to_hub`]๋ฅผ ํธ์ถํ์ฌ ํ๋ จ๋ ๋ชจ๋ธ์ ํ๋ธ๋ก ํธ์ํ์ธ์. ๐ค Transformers๋ ํ๋ จ ํ์ดํผํ๋ผ๋ฏธํฐ, ํ๋ จ ๊ฒฐ๊ณผ ๋ฐ ํ๋ ์์ํฌ ๋ฒ์ ์ ๋ชจ๋ธ ์นด๋์ ์๋์ผ๋ก ์ถ๊ฐํฉ๋๋ค!
```py
>>> trainer.push_to_hub()
```
</pt>
<tf>
[`PushToHubCallback`]์ ์ฌ์ฉํ์ฌ ๋ชจ๋ธ์ ํ๋ธ์ ๊ณต์ ํ๋ ค๋ฉด, [`PushToHubCallback`]์ ๋ค์ ์ธ์๋ฅผ ์ ์ํ์ธ์:
- ์ถ๋ ฅ๋ ๋ชจ๋ธ์ ํ์ผ ๊ฒฝ๋ก
- ํ ํฌ๋์ด์
- `{Hub ์ฌ์ฉ์ ์ด๋ฆ}/{๋ชจ๋ธ ์ด๋ฆ}` ํ์์ `hub_model_id`
```py
>>> from transformers import PushToHubCallback
>>> push_to_hub_callback = PushToHubCallback(
... output_dir="./your_model_save_path", tokenizer=tokenizer, hub_model_id="your-username/my-awesome-model"
... )
```
[`fit`](https://keras.io/api/models/model_training_apis/)์ ์ฝ๋ฐฑ์ ์ถ๊ฐํ๋ฉด, ๐ค Transformers๊ฐ ํ๋ จ๋ ๋ชจ๋ธ์ ํ๋ธ๋ก ํธ์ํฉ๋๋ค:
```py
>>> model.fit(tf_train_dataset, validation_data=tf_validation_dataset, epochs=3, callbacks=push_to_hub_callback)
```
</tf>
</frameworkcontent>
## `push_to_hub` ํจ์ ์ฌ์ฉํ๊ธฐ[[use-the-pushtohub-function]]
๋ชจ๋ธ์์ ์ง์ `push_to_hub`๋ฅผ ํธ์ถํ์ฌ ํ๋ธ์ ์
๋ก๋ํ ์๋ ์์ต๋๋ค.
`push_to_hub`์ ๋ชจ๋ธ ์ด๋ฆ์ ์ง์ ํ์ธ์:
```py
>>> pt_model.push_to_hub("my-awesome-model")
```
์ด๋ ๊ฒ ํ๋ฉด ์ฌ์ฉ์ ์ด๋ฆ ์๋์ ๋ชจ๋ธ ์ด๋ฆ `my-awesome-model`๋ก ์ ์ฅ์๊ฐ ์์ฑ๋ฉ๋๋ค. ์ด์ ์ฌ์ฉ์๋ `from_pretrained` ํจ์๋ฅผ ์ฌ์ฉํ์ฌ ๋ชจ๋ธ์ ๊ฐ์ ธ์ฌ ์ ์์ต๋๋ค:
```py
>>> from transformers import AutoModel
>>> model = AutoModel.from_pretrained("your_username/my-awesome-model")
```
์กฐ์ง์ ์ํ๊ณ ๋ชจ๋ธ์ ์กฐ์ง ์ด๋ฆ์ผ๋ก ๋์ ํธ์ํ๋ ค๋ฉด `repo_id`์ ์ถ๊ฐํ์ธ์:
```py
>>> pt_model.push_to_hub("my-awesome-org/my-awesome-model")
```
`push_to_hub` ํจ์๋ ๋ชจ๋ธ ์ ์ฅ์์ ๋ค๋ฅธ ํ์ผ์ ์ถ๊ฐํ๋ ๋ฐ์๋ ์ฌ์ฉํ ์ ์์ต๋๋ค. ์๋ฅผ ๋ค์ด ๋ชจ๋ธ ์ ์ฅ์์ ํ ํฌ๋์ด์ ๋ฅผ ์ถ๊ฐํ ์ ์์ต๋๋ค:
```py
>>> tokenizer.push_to_hub("my-awesome-model")
```
๋๋ ๋ฏธ์ธ ์กฐ์ ๋ PyTorch ๋ชจ๋ธ์ TensorFlow ๋ฒ์ ์ ์ถ๊ฐํ ์๋ ์์ต๋๋ค:
```py
>>> tf_model.push_to_hub("my-awesome-model")
```
์ด์ Hugging Face ํ๋กํ๋ก ์ด๋ํ๋ฉด, ์๋ก ์์ฑํ ๋ชจ๋ธ ์ ์ฅ์๊ฐ ํ์๋ฉ๋๋ค. **Files** ํญ์ ํด๋ฆญํ๋ฉด ์ ์ฅ์์ ์
๋ก๋ํ ๋ชจ๋ ํ์ผ์ด ํ์๋ฉ๋๋ค.
์ ์ฅ์์ ํ์ผ์ ๋ง๋ค๊ณ ์
๋ก๋ํ๋ ๋ฐฉ๋ฒ์ ๋ํ ์์ธํ ๋ด์ฉ์ ํ๋ธ ์ค๋ช
์ [์ฌ๊ธฐ](https://huggingface.co/docs/hub/how-to-upstream)๋ฅผ ์ฐธ์กฐํ์ธ์.
## ์น ์ธํฐํ์ด์ค๋ก ์
๋ก๋ํ๊ธฐ[[upload-with-the-web-interface]]
์ฝ๋ ์๋ ์ ๊ทผ ๋ฐฉ์์ ์ ํธํ๋ ์ฌ์ฉ์๋ ํ๋ธ์ ์น ์ธํฐํ์ด์ค๋ฅผ ํตํด ๋ชจ๋ธ์ ์
๋ก๋ํ ์ ์์ต๋๋ค. [huggingface.co/new](https://huggingface.co/new)๋ฅผ ๋ฐฉ๋ฌธํ์ฌ ์๋ก์ด ์ ์ฅ์๋ฅผ ์์ฑํ์ธ์:
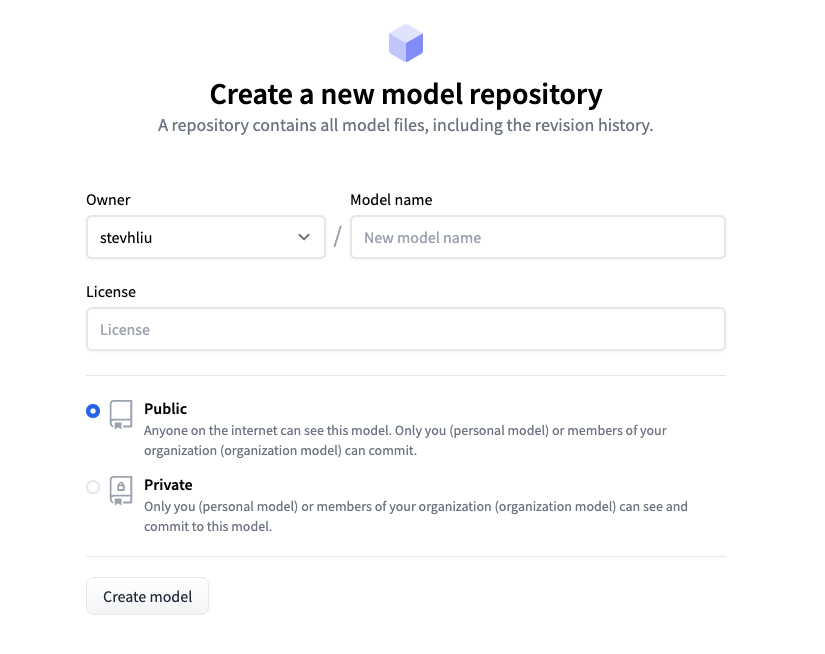
์ฌ๊ธฐ์ ๋ชจ๋ธ์ ๋ํ ๋ช ๊ฐ์ง ์ ๋ณด๋ฅผ ์ถ๊ฐํ์ธ์:
- ์ ์ฅ์์ **์์ ์**๋ฅผ ์ ํํฉ๋๋ค. ์ด๋ ์ฌ์ฉ์ ๋๋ ์ฌ์ฉ์๊ฐ ์ํ ์กฐ์ง์ผ ์ ์์ต๋๋ค.
- ์ ์ฅ์ ์ด๋ฆ์ด ๋ ๋ชจ๋ธ์ ์ด๋ฆ์ ์ ํํฉ๋๋ค.
- ๋ชจ๋ธ์ด ๊ณต๊ฐ์ธ์ง ๋น๊ณต๊ฐ์ธ์ง ์ ํํฉ๋๋ค.
- ๋ชจ๋ธ์ ๋ผ์ด์ผ์ค ์ฌ์ฉ์ ์ง์ ํฉ๋๋ค.
์ด์ **Files** ํญ์ ํด๋ฆญํ๊ณ **Add file** ๋ฒํผ์ ํด๋ฆญํ์ฌ ์๋ก์ด ํ์ผ์ ์ ์ฅ์์ ์
๋ก๋ํฉ๋๋ค. ๊ทธ๋ฐ ๋ค์ ์
๋ก๋ํ ํ์ผ์ ๋์ด๋ค ๋๊ณ ์ปค๋ฐ ๋ฉ์์ง๋ฅผ ์ถ๊ฐํ์ธ์.
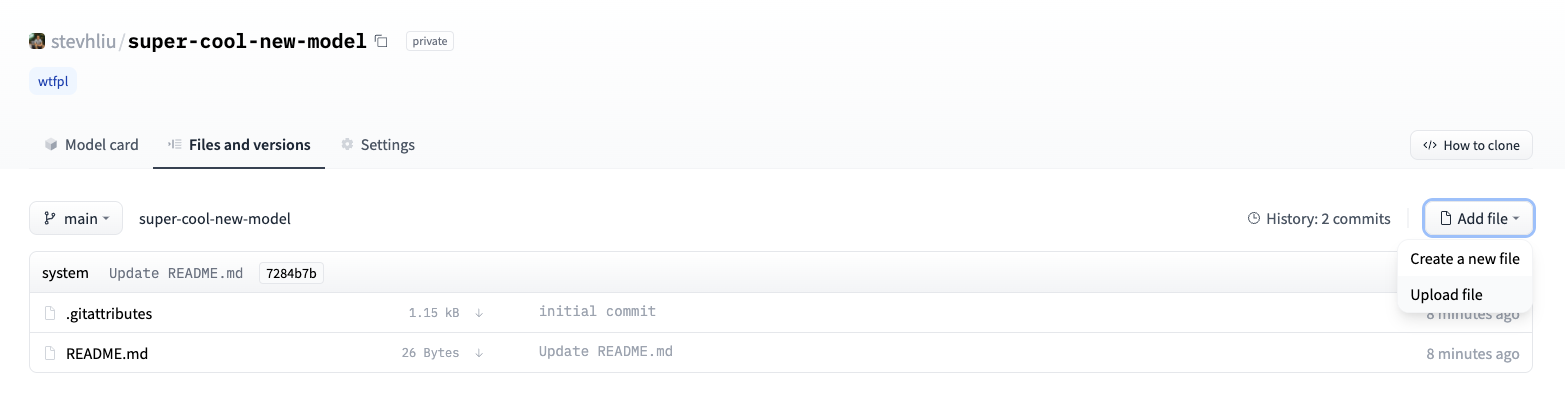
## ๋ชจ๋ธ ์นด๋ ์ถ๊ฐํ๊ธฐ[[add-a-model-card]]
์ฌ์ฉ์๊ฐ ๋ชจ๋ธ์ ๊ธฐ๋ฅ, ์ ํ, ์ ์ฌ์ ํธํฅ ๋ฐ ์ค๋ฆฌ์ ๊ณ ๋ ค ์ฌํญ์ ์ดํดํ ์ ์๋๋ก ์ ์ฅ์์ ๋ชจ๋ธ ์นด๋๋ฅผ ์ถ๊ฐํ์ธ์. ๋ชจ๋ธ ์นด๋๋ `README.md` ํ์ผ์ ์ ์๋์ด ์์ต๋๋ค. ๋ค์ ๋ฐฉ๋ฒ์ผ๋ก ๋ชจ๋ธ ์นด๋๋ฅผ ์ถ๊ฐํ ์ ์์ต๋๋ค:
* `README.md` ํ์ผ์ ์๋์ผ๋ก ์์ฑํ์ฌ ์
๋ก๋ํฉ๋๋ค.
* ๋ชจ๋ธ ์ ์ฅ์์์ **Edit model card** ๋ฒํผ์ ํด๋ฆญํฉ๋๋ค.
๋ชจ๋ธ ์นด๋์ ํฌํจํ ์ ๋ณด ์ ํ์ ๋ํ ์ข์ ์๋ DistilBert [๋ชจ๋ธ ์นด๋](https://huggingface.co/distilbert-base-uncased)๋ฅผ ์ฐธ์กฐํ์ธ์. ๋ชจ๋ธ์ ํ์ ๋ฐ์๊ตญ์ด๋ ์์ ฏ ์์ ๋ฑ `README.md` ํ์ผ์์ ์ ์ดํ ์ ์๋ ๋ค๋ฅธ ์ต์
์ ๋ํ ์์ธํ ๋ด์ฉ์ [์ฌ๊ธฐ](https://huggingface.co/docs/hub/models-cards) ๋ฌธ์๋ฅผ ์ฐธ์กฐํ์ธ์.
| 0 |
hf_public_repos/transformers/docs/source | hf_public_repos/transformers/docs/source/ko/pipeline_tutorial.md | <!--Copyright 2022 The HuggingFace Team. All rights reserved.
Licensed under the Apache License, Version 2.0 (the "License"); you may not use this file except in compliance with
the License. You may obtain a copy of the License at
http://www.apache.org/licenses/LICENSE-2.0
Unless required by applicable law or agreed to in writing, software distributed under the License is distributed on
an "AS IS" BASIS, WITHOUT WARRANTIES OR CONDITIONS OF ANY KIND, either express or implied. See the License for the
specific language governing permissions and limitations under the License.
โ ๏ธ Note that this file is in Markdown but contain specific syntax for our doc-builder (similar to MDX) that may not be
rendered properly in your Markdown viewer.
-->
# ์ถ๋ก ์ ์ํ Pipeline[[pipelines-for-inference]]
[`pipeline`]์ ์ฌ์ฉํ๋ฉด ์ธ์ด, ์ปดํจํฐ ๋น์ , ์ค๋์ค ๋ฐ ๋ฉํฐ๋ชจ๋ฌ ํ์คํฌ์ ๋ํ ์ถ๋ก ์ ์ํด [Hub](https://huggingface.co/models)์ ์ด๋ค ๋ชจ๋ธ์ด๋ ์ฝ๊ฒ ์ฌ์ฉํ ์ ์์ต๋๋ค. ํน์ ๋ถ์ผ์ ๋ํ ๊ฒฝํ์ด ์๊ฑฐ๋, ๋ชจ๋ธ์ ์ด๋ฃจ๋ ์ฝ๋๊ฐ ์ต์ํ์ง ์์ ๊ฒฝ์ฐ์๋ [`pipeline`]์ ์ฌ์ฉํด์ ์ถ๋ก ํ ์ ์์ด์! ์ด ํํ ๋ฆฌ์ผ์์๋ ๋ค์์ ๋ฐฐ์๋ณด๊ฒ ์ต๋๋ค.
* ์ถ๋ก ์ ์ํด [`pipeline`]์ ์ฌ์ฉํ๋ ๋ฐฉ๋ฒ
* ํน์ ํ ํฌ๋์ด์ ๋๋ ๋ชจ๋ธ์ ์ฌ์ฉํ๋ ๋ฐฉ๋ฒ
* ์ธ์ด, ์ปดํจํฐ ๋น์ , ์ค๋์ค ๋ฐ ๋ฉํฐ๋ชจ๋ฌ ํ์คํฌ์์ [`pipeline`]์ ์ฌ์ฉํ๋ ๋ฐฉ๋ฒ
<Tip>
์ง์ํ๋ ๋ชจ๋ ํ์คํฌ์ ์ธ ์ ์๋ ๋งค๊ฐ๋ณ์๋ฅผ ๋ด์ ๋ชฉ๋ก์ [`pipeline`] ์ค๋ช
์๋ฅผ ์ฐธ๊ณ ํด์ฃผ์ธ์.
</Tip>
## Pipeline ์ฌ์ฉํ๊ธฐ[[pipeline-usage]]
๊ฐ ํ์คํฌ๋ง๋ค ๊ณ ์ ์ [`pipeline`]์ด ์์ง๋ง, ๊ฐ๋ณ ํ์ดํ๋ผ์ธ์ ๋ด๊ณ ์๋ ์ถ์ํ๋ [`pipeline`]๋ฅผ ์ฌ์ฉํ๋ ๊ฒ์ด ์ผ๋ฐ์ ์ผ๋ก ๋ ๊ฐ๋จํฉ๋๋ค. [`pipeline`]์ ํ์คํฌ์ ์๋ง๊ฒ ์ถ๋ก ์ด ๊ฐ๋ฅํ ๊ธฐ๋ณธ ๋ชจ๋ธ๊ณผ ์ ์ฒ๋ฆฌ ํด๋์ค๋ฅผ ์๋์ผ๋ก ๋ก๋ํฉ๋๋ค.
1. ๋จผ์ [`pipeline`]์ ์์ฑํ๊ณ ํ์คํฌ๋ฅผ ์ง์ ํ์ธ์.
```py
>>> from transformers import pipeline
>>> generator = pipeline(task="automatic-speech-recognition")
```
2. ๊ทธ๋ฆฌ๊ณ [`pipeline`]์ ์
๋ ฅ์ ๋ฃ์ด์ฃผ์ธ์.
```py
>>> generator("https://huggingface.co/datasets/Narsil/asr_dummy/resolve/main/mlk.flac")
{'text': 'I HAVE A DREAM BUT ONE DAY THIS NATION WILL RISE UP LIVE UP THE TRUE MEANING OF ITS TREES'}
```
๊ธฐ๋ํ๋ ๊ฒฐ๊ณผ๊ฐ ์๋๊ฐ์? Hub์์ [๊ฐ์ฅ ๋ง์ด ๋ค์ด๋ก๋๋ ์๋ ์์ฑ ์ธ์ ๋ชจ๋ธ](https://huggingface.co/models?pipeline_tag=automatic-speech-recognition&sort=downloads)๋ก ๋ ๋์ ๊ฒฐ๊ณผ๋ฅผ ์ป์ ์ ์๋์ง ํ์ธํด๋ณด์ธ์.
๋ค์์ [openai/whisper-large](https://huggingface.co/openai/whisper-large)๋ก ์๋ํด๋ณด๊ฒ ์ต๋๋ค.
```py
>>> generator = pipeline(model="openai/whisper-large")
>>> generator("https://huggingface.co/datasets/Narsil/asr_dummy/resolve/main/mlk.flac")
{'text': ' I have a dream that one day this nation will rise up and live out the true meaning of its creed.'}
```
ํจ์ฌ ๋ ๋์์ก๊ตฐ์!
Hub์ ๋ชจ๋ธ๋ค์ ์ฌ๋ฌ ๋ค์ํ ์ธ์ด์ ์ ๋ฌธ๋ถ์ผ๋ฅผ ์์ฐ๋ฅด๊ธฐ ๋๋ฌธ์ ๊ผญ ์์ ์ ์ธ์ด๋ ๋ถ์ผ์ ํนํ๋ ๋ชจ๋ธ์ ์ฐพ์๋ณด์๊ธฐ ๋ฐ๋๋๋ค.
๋ธ๋ผ์ฐ์ ๋ฅผ ๋ฒ์ด๋ ํ์์์ด Hub์์ ์ง์ ๋ชจ๋ธ์ ์ถ๋ ฅ์ ํ์ธํ๊ณ ๋ค๋ฅธ ๋ชจ๋ธ๊ณผ ๋น๊ตํด์ ์์ ์ ์ํฉ์ ๋ ์ ํฉํ์ง, ์ ๋งคํ ์
๋ ฅ์ ๋ ์ ์ฒ๋ฆฌํ๋์ง๋ ํ์ธํ ์ ์์ต๋๋ค.
๋ง์ฝ ์ํฉ์ ์๋ง๋ ๋ชจ๋ธ์ ์๋ค๋ฉด ์ธ์ ๋ ์ง์ [ํ๋ จ](training)์ํฌ ์ ์์ต๋๋ค!
์
๋ ฅ์ด ์ฌ๋ฌ ๊ฐ ์๋ ๊ฒฝ์ฐ, ๋ฆฌ์คํธ ํํ๋ก ์ ๋ฌํ ์ ์์ต๋๋ค.
```py
generator(
[
"https://huggingface.co/datasets/Narsil/asr_dummy/resolve/main/mlk.flac",
"https://huggingface.co/datasets/Narsil/asr_dummy/resolve/main/1.flac",
]
)
```
์ ์ฒด ๋ฐ์ดํฐ์ธํธ์ ์ํํ๊ฑฐ๋ ์น์๋ฒ์ ์ฌ๋ ค๋์ด ์ถ๋ก ์ ์ฌ์ฉํ๊ณ ์ถ๋ค๋ฉด, ๊ฐ ์์ธ ํ์ด์ง๋ฅผ ์ฐธ์กฐํ์ธ์.
[๋ฐ์ดํฐ์ธํธ์์ Pipeline ์ฌ์ฉํ๊ธฐ](#using-pipelines-on-a-dataset)
[์น์๋ฒ์์ Pipeline ์ฌ์ฉํ๊ธฐ](./pipeline_webserver)
## ๋งค๊ฐ๋ณ์[[parameters]]
[`pipeline`]์ ๋ง์ ๋งค๊ฐ๋ณ์๋ฅผ ์ง์ํฉ๋๋ค. ํน์ ํ์คํฌ์ฉ์ธ ๊ฒ๋ ์๊ณ , ๋ฒ์ฉ์ธ ๊ฒ๋ ์์ต๋๋ค.
์ผ๋ฐ์ ์ผ๋ก ์ํ๋ ์์น์ ์ด๋๋ ๋งค๊ฐ๋ณ์๋ฅผ ๋ฃ์ ์ ์์ต๋๋ค.
```py
generator(model="openai/whisper-large", my_parameter=1)
out = generate(...) # This will use `my_parameter=1`.
out = generate(..., my_parameter=2) # This will override and use `my_parameter=2`.
out = generate(...) # This will go back to using `my_parameter=1`.
```
์ค์ํ 3๊ฐ์ง ๋งค๊ฐ๋ณ์๋ฅผ ์ดํด๋ณด๊ฒ ์ต๋๋ค.
### ๊ธฐ๊ธฐ(device)[[device]]
`device=n`์ฒ๋ผ ๊ธฐ๊ธฐ๋ฅผ ์ง์ ํ๋ฉด ํ์ดํ๋ผ์ธ์ด ์๋์ผ๋ก ํด๋น ๊ธฐ๊ธฐ์ ๋ชจ๋ธ์ ๋ฐฐ์นํฉ๋๋ค.
ํ์ดํ ์น์์๋ ํ
์ํ๋ก์ฐ์์๋ ๋ชจ๋ ์๋ํฉ๋๋ค.
```py
generator(model="openai/whisper-large", device=0)
```
๋ชจ๋ธ์ด GPU ํ๋์ ๋์๊ฐ๊ธฐ ๋ฒ๊ฒ๋ค๋ฉด, `device_map="auto"`๋ฅผ ์ง์ ํด์ ๐ค [Accelerate](https://huggingface.co/docs/accelerate)๊ฐ ๋ชจ๋ธ ๊ฐ์ค์น๋ฅผ ์ด๋ป๊ฒ ๋ก๋ํ๊ณ ์ ์ฅํ ์ง ์๋์ผ๋ก ๊ฒฐ์ ํ๋๋ก ํ ์ ์์ต๋๋ค.
```py
#!pip install accelerate
generator(model="openai/whisper-large", device_map="auto")
```
### ๋ฐฐ์น ์ฌ์ด์ฆ[[batch-size]]
๊ธฐ๋ณธ์ ์ผ๋ก ํ์ดํ๋ผ์ธ์ [์ฌ๊ธฐ](https://huggingface.co/docs/transformers/main_classes/pipelines#pipeline-batching)์ ๋์จ ์ด์ ๋ก ์ถ๋ก ์ ์ผ๊ด ์ฒ๋ฆฌํ์ง ์์ต๋๋ค. ๊ฐ๋จํ ์ค๋ช
ํ์๋ฉด ์ผ๊ด ์ฒ๋ฆฌ๊ฐ ๋ฐ๋์ ๋ ๋น ๋ฅด์ง ์๊ณ ์คํ๋ ค ๋ ๋๋ ค์ง ์๋ ์๊ธฐ ๋๋ฌธ์
๋๋ค.
ํ์ง๋ง ์์ ์ ์ํฉ์ ์ ํฉํ๋ค๋ฉด, ์ด๋ ๊ฒ ์ฌ์ฉํ์ธ์.
```py
generator(model="openai/whisper-large", device=0, batch_size=2)
audio_filenames = [f"audio_{i}.flac" for i in range(10)]
texts = generator(audio_filenames)
```
ํ์ดํ๋ผ์ธ ์ ์ ๊ณต๋ 10๊ฐ์ ์ค๋์ค ํ์ผ์ ์ถ๊ฐ๋ก ์ฒ๋ฆฌํ๋ ์ฝ๋ ์์ด (์ผ๊ด ์ฒ๋ฆฌ์ ๋ณด๋ค ํจ๊ณผ์ ์ธ GPU ์) ๋ชจ๋ธ์ 2๊ฐ์ฉ ์ ๋ฌํฉ๋๋ค.
์ถ๋ ฅ์ ์ผ๊ด ์ฒ๋ฆฌํ์ง ์์์ ๋์ ๋๊ฐ์์ผ ํฉ๋๋ค. ํ์ดํ๋ผ์ธ์์ ์๋๋ฅผ ๋ ๋ผ ์๋ ์๋ ๋ฐฉ๋ฒ ์ค ํ๋์ผ ๋ฟ์
๋๋ค.
ํ์ดํ๋ผ์ธ์ ์ผ๊ด ์ฒ๋ฆฌ์ ๋ณต์กํ ๋ถ๋ถ์ ์ค์ฌ์ฃผ๊ธฐ๋ ํฉ๋๋ค. (์๋ฅผ ๋ค์ด ๊ธด ์ค๋์ค ํ์ผ์ฒ๋ผ) ์ฌ๋ฌ ๋ถ๋ถ์ผ๋ก ๋๋ ์ผ ๋ชจ๋ธ์ด ์ฒ๋ฆฌํ ์ ์๋ ๊ฒ์ [*chunk batching*](./main_classes/pipelines#pipeline-chunk-batching)์ด๋ผ๊ณ ํ๋๋ฐ, ํ์ดํ๋ผ์ธ์ ์ฌ์ฉํ๋ฉด ์๋์ผ๋ก ๋๋ ์ค๋๋ค.
### ํน์ ํ์คํฌ์ฉ ๋งค๊ฐ๋ณ์[[task-specific-parameters]]
๊ฐ ํ์คํฌ๋ง๋ค ๊ตฌํํ ๋ ์ ์ฐ์ฑ๊ณผ ์ต์
์ ์ ๊ณตํ๊ธฐ ์ํด ํ์คํฌ์ฉ ๋งค๊ฐ๋ณ์๊ฐ ์์ต๋๋ค.
์๋ฅผ ๋ค์ด [`transformers.AutomaticSpeechRecognitionPipeline.__call__`] ๋ฉ์๋์๋ ๋์์์ ์๋ง์ ๋ฃ์ ๋ ์ ์ฉํ ๊ฒ ๊ฐ์ `return_timestamps` ๋งค๊ฐ๋ณ์๊ฐ ์์ต๋๋ค.
```py
>>> # Not using whisper, as it cannot provide timestamps.
>>> generator = pipeline(model="facebook/wav2vec2-large-960h-lv60-self", return_timestamps="word")
>>> generator("https://huggingface.co/datasets/Narsil/asr_dummy/resolve/main/mlk.flac")
{'text': 'I HAVE A DREAM BUT ONE DAY THIS NATION WILL RISE UP AND LIVE OUT THE TRUE MEANING OF ITS CREED', 'chunks': [{'text': 'I', 'timestamp': (1.22, 1.24)}, {'text': 'HAVE', 'timestamp': (1.42, 1.58)}, {'text': 'A', 'timestamp': (1.66, 1.68)}, {'text': 'DREAM', 'timestamp': (1.76, 2.14)}, {'text': 'BUT', 'timestamp': (3.68, 3.8)}, {'text': 'ONE', 'timestamp': (3.94, 4.06)}, {'text': 'DAY', 'timestamp': (4.16, 4.3)}, {'text': 'THIS', 'timestamp': (6.36, 6.54)}, {'text': 'NATION', 'timestamp': (6.68, 7.1)}, {'text': 'WILL', 'timestamp': (7.32, 7.56)}, {'text': 'RISE', 'timestamp': (7.8, 8.26)}, {'text': 'UP', 'timestamp': (8.38, 8.48)}, {'text': 'AND', 'timestamp': (10.08, 10.18)}, {'text': 'LIVE', 'timestamp': (10.26, 10.48)}, {'text': 'OUT', 'timestamp': (10.58, 10.7)}, {'text': 'THE', 'timestamp': (10.82, 10.9)}, {'text': 'TRUE', 'timestamp': (10.98, 11.18)}, {'text': 'MEANING', 'timestamp': (11.26, 11.58)}, {'text': 'OF', 'timestamp': (11.66, 11.7)}, {'text': 'ITS', 'timestamp': (11.76, 11.88)}, {'text': 'CREED', 'timestamp': (12.0, 12.38)}]}
```
๋ณด์๋ค์ํผ ๋ชจ๋ธ์ด ํ
์คํธ๋ฅผ ์ถ๋ก ํ ๋ฟ๋ง ์๋๋ผ ๊ฐ ๋จ์ด๋ฅผ ๋งํ ์์ ๊น์ง๋ ์ถ๋ ฅํ์ต๋๋ค.
ํ์คํฌ๋ง๋ค ๋ค์ํ ๋งค๊ฐ๋ณ์๋ฅผ ๊ฐ์ง๊ณ ์๋๋ฐ์. ์ํ๋ ํ์คํฌ์ API๋ฅผ ์ฐธ์กฐํด์ ๋ฐ๊ฟ๋ณผ ์ ์๋ ์ฌ๋ฌ ๋งค๊ฐ๋ณ์๋ฅผ ์ดํด๋ณด์ธ์!
์ง๊ธ๊น์ง ๋ค๋ค๋ณธ [`~transformers.AutomaticSpeechRecognitionPipeline`]์๋ `chunk_length_s` ๋งค๊ฐ๋ณ์๊ฐ ์์ต๋๋ค. ์ํ๋ 1์๊ฐ ๋ถ๋์ ๋์์์ ์๋ง ์์
์ ํ ๋์ฒ๋ผ, ์ผ๋ฐ์ ์ผ๋ก ๋ชจ๋ธ์ด ์์ฒด์ ์ผ๋ก ์ฒ๋ฆฌํ ์ ์๋ ๋งค์ฐ ๊ธด ์ค๋์ค ํ์ผ์ ์ฒ๋ฆฌํ ๋ ์ ์ฉํ์ฃ .
๋์์ด ๋ ๋งํ ๋งค๊ฐ๋ณ์๋ฅผ ์ฐพ์ง ๋ชปํ๋ค๋ฉด ์ธ์ ๋ ์ง [์์ฒญ](https://github.com/huggingface/transformers/issues/new?assignees=&labels=feature&template=feature-request.yml)ํด์ฃผ์ธ์!
## ๋ฐ์ดํฐ์ธํธ์์ Pipeline ์ฌ์ฉํ๊ธฐ[[using-pipelines-on-a-dataset]]
ํ์ดํ๋ผ์ธ์ ๋๊ท๋ชจ ๋ฐ์ดํฐ์ธํธ์์๋ ์ถ๋ก ์์
์ ํ ์ ์์ต๋๋ค. ์ด๋ ์ดํฐ๋ ์ดํฐ๋ฅผ ์ฌ์ฉํ๋ ๊ฑธ ์ถ์ฒ๋๋ฆฝ๋๋ค.
```py
def data():
for i in range(1000):
yield f"My example {i}"
pipe = pipe(model="gpt2", device=0)
generated_characters = 0
for out in pipe(data()):
generated_characters += len(out["generated_text"])
```
์ดํฐ๋ ์ดํฐ `data()`๋ ๊ฐ ๊ฒฐ๊ณผ๋ฅผ ํธ์ถ๋ง๋ค ์์ฑํ๊ณ , ํ์ดํ๋ผ์ธ์ ์
๋ ฅ์ด ์ํํ ์ ์๋ ์๋ฃ๊ตฌ์กฐ์์ ์๋์ผ๋ก ์ธ์ํ์ฌ GPU์์ ๊ธฐ์กด ๋ฐ์ดํฐ๊ฐ ์ฒ๋ฆฌ๋๋ ๋์ ์๋ก์ด ๋ฐ์ดํฐ๋ฅผ ๊ฐ์ ธ์ค๊ธฐ ์์ํฉ๋๋ค.(์ด๋ ๋ด๋ถ์ ์ผ๋ก [DataLoader](https://pytorch.org/docs/stable/data.html#torch.utils.data.DataLoader)๋ฅผ ์ฌ์ฉํด์.) ์ด ๊ณผ์ ์ ์ ์ฒด ๋ฐ์ดํฐ์ธํธ๋ฅผ ๋ฉ๋ชจ๋ฆฌ์ ์ ์ฌํ์ง ์๊ณ ๋ GPU์ ์ต๋ํ ๋น ๋ฅด๊ฒ ์๋ก์ด ์์
์ ๊ณต๊ธํ ์ ์๊ธฐ ๋๋ฌธ์ ์ค์ํฉ๋๋ค.
๊ทธ๋ฆฌ๊ณ ์ผ๊ด ์ฒ๋ฆฌ๊ฐ ๋ ๋น ๋ฅผ ์ ์๊ธฐ ๋๋ฌธ์, `batch_size` ๋งค๊ฐ๋ณ์๋ฅผ ์กฐ์ ํด๋ด๋ ์ข์์.
๋ฐ์ดํฐ์ธํธ๋ฅผ ์ํํ๋ ๊ฐ์ฅ ๊ฐ๋จํ ๋ฐฉ๋ฒ์ ๐ค [Datasets](https://github.com/huggingface/datasets/)๋ฅผ ํ์ฉํ๋ ๊ฒ์ธ๋ฐ์.
```py
# KeyDataset is a util that will just output the item we're interested in.
from transformers.pipelines.pt_utils import KeyDataset
pipe = pipeline(model="hf-internal-testing/tiny-random-wav2vec2", device=0)
dataset = load_dataset("hf-internal-testing/librispeech_asr_dummy", "clean", split="validation[:10]")
for out in pipe(KeyDataset(dataset["audio"])):
print(out)
```
## ์น์๋ฒ์์ Pipeline ์ฌ์ฉํ๊ธฐ[[using-pipelines-for-a-webserver]]
<Tip>
์ถ๋ก ์์ง์ ๋ง๋๋ ๊ณผ์ ์ ๋ฐ๋ก ํ์ด์ง๋ฅผ ์์ฑํ ๋งํ ๋ณต์กํ ์ฃผ์ ์
๋๋ค.
</Tip>
[Link](./pipeline_webserver)
## ๋น์ Pipeline[[vision-pipeline]]
๋น์ ํ์คํฌ๋ฅผ ์ํด [`pipeline`]์ ์ฌ์ฉํ๋ ์ผ์ ๊ฑฐ์ ๋์ผํฉ๋๋ค.
ํ์คํฌ๋ฅผ ์ง์ ํ๊ณ ์ด๋ฏธ์ง๋ฅผ ๋ถ๋ฅ๊ธฐ์ ์ ๋ฌํ๋ฉด ๋ฉ๋๋ค. ์ด๋ฏธ์ง๋ ์ธํฐ๋ท ๋งํฌ ๋๋ ๋ก์ปฌ ๊ฒฝ๋ก์ ํํ๋ก ์ ๋ฌํด์ฃผ์ธ์. ์๋ฅผ ๋ค์ด ์๋์ ํ์๋ ๊ณ ์์ด๋ ์ด๋ค ์ข
์ธ๊ฐ์?

```py
>>> from transformers import pipeline
>>> vision_classifier = pipeline(model="google/vit-base-patch16-224")
>>> preds = vision_classifier(
... images="https://huggingface.co/datasets/huggingface/documentation-images/resolve/main/pipeline-cat-chonk.jpeg"
... )
>>> preds = [{"score": round(pred["score"], 4), "label": pred["label"]} for pred in preds]
>>> preds
[{'score': 0.4335, 'label': 'lynx, catamount'}, {'score': 0.0348, 'label': 'cougar, puma, catamount, mountain lion, painter, panther, Felis concolor'}, {'score': 0.0324, 'label': 'snow leopard, ounce, Panthera uncia'}, {'score': 0.0239, 'label': 'Egyptian cat'}, {'score': 0.0229, 'label': 'tiger cat'}]
```
### ํ
์คํธ Pipeline[[text-pipeline]]
NLP ํ์คํฌ๋ฅผ ์ํด [`pipeline`]์ ์ฌ์ฉํ๋ ์ผ๋ ๊ฑฐ์ ๋์ผํฉ๋๋ค.
```py
>>> from transformers import pipeline
>>> # This model is a `zero-shot-classification` model.
>>> # It will classify text, except you are free to choose any label you might imagine
>>> classifier = pipeline(model="facebook/bart-large-mnli")
>>> classifier(
... "I have a problem with my iphone that needs to be resolved asap!!",
... candidate_labels=["urgent", "not urgent", "phone", "tablet", "computer"],
... )
{'sequence': 'I have a problem with my iphone that needs to be resolved asap!!', 'labels': ['urgent', 'phone', 'computer', 'not urgent', 'tablet'], 'scores': [0.504, 0.479, 0.013, 0.003, 0.002]}
```
### ๋ฉํฐ๋ชจ๋ฌ Pipeline[[multimodal-pipeline]]
[`pipeline`]์ ์ฌ๋ฌ ๋ชจ๋ฌ๋ฆฌํฐ(์ญ์ฃผ: ์ค๋์ค, ๋น๋์ค, ํ
์คํธ์ ๊ฐ์ ๋ฐ์ดํฐ ํํ)๋ฅผ ์ง์ํฉ๋๋ค. ์์๋ก ์๊ฐ์ ์ง์์๋ต(VQA; Visual Question Answering) ํ์คํฌ๋ ํ
์คํธ์ ์ด๋ฏธ์ง๋ฅผ ๋ชจ๋ ์ฌ์ฉํฉ๋๋ค. ๊ทธ ์ด๋ค ์ด๋ฏธ์ง ๋งํฌ๋ ๋ฌป๊ณ ์ถ์ ์ง๋ฌธ๋ ์์ ๋กญ๊ฒ ์ ๋ฌํ ์ ์์ต๋๋ค. ์ด๋ฏธ์ง๋ URL ๋๋ ๋ก์ปฌ ๊ฒฝ๋ก์ ํํ๋ก ์ ๋ฌํด์ฃผ์ธ์.
์๋ฅผ ๋ค์ด ์ด [๊ฑฐ๋๋ช
์ธ์ ์ฌ์ง](https://huggingface.co/spaces/impira/docquery/resolve/2359223c1837a7587402bda0f2643382a6eefeab/invoice.png)์์ ๊ฑฐ๋๋ช
์ธ์ ๋ฒํธ๋ฅผ ๋ฌป๊ณ ์ถ๋ค๋ฉด,
```py
>>> from transformers import pipeline
>>> vqa = pipeline(model="impira/layoutlm-document-qa")
>>> vqa(
... image="https://huggingface.co/spaces/impira/docquery/resolve/2359223c1837a7587402bda0f2643382a6eefeab/invoice.png",
... question="What is the invoice number?",
... )
[{'score': 0.42514941096305847, 'answer': 'us-001', 'start': 16, 'end': 16}]
```
| 0 |
hf_public_repos/transformers/docs/source | hf_public_repos/transformers/docs/source/ko/fast_tokenizers.md | <!--Copyright 2020 The HuggingFace Team. All rights reserved.
Licensed under the Apache License, Version 2.0 (the "License"); you may not use this file except in compliance with
the License. You may obtain a copy of the License at
http://www.apache.org/licenses/LICENSE-2.0
Unless required by applicable law or agreed to in writing, software distributed under the License is distributed on
an "AS IS" BASIS, WITHOUT WARRANTIES OR CONDITIONS OF ANY KIND, either express or implied. See the License for the
specific language governing permissions and limitations under the License.
โ ๏ธ Note that this file is in Markdown but contain specific syntax for our doc-builder (similar to MDX) that may not be
rendered properly in your Markdown viewer.
-->
# ๐ค Tokenizers ๋ผ์ด๋ธ๋ฌ๋ฆฌ์ ํ ํฌ๋์ด์ ์ฌ์ฉํ๊ธฐ[[use-tokenizers-from-tokenizers]]
[`PreTrainedTokenizerFast`]๋ [๐ค Tokenizers](https://huggingface.co/docs/tokenizers) ๋ผ์ด๋ธ๋ฌ๋ฆฌ์ ๊ธฐ๋ฐํฉ๋๋ค. ๐ค Tokenizers ๋ผ์ด๋ธ๋ฌ๋ฆฌ์ ํ ํฌ๋์ด์ ๋
๐ค Transformers๋ก ๋งค์ฐ ๊ฐ๋จํ๊ฒ ๋ถ๋ฌ์ฌ ์ ์์ต๋๋ค.
๊ตฌ์ฒด์ ์ธ ๋ด์ฉ์ ๋ค์ด๊ฐ๊ธฐ ์ ์, ๋ช ์ค์ ์ฝ๋๋ก ๋๋ฏธ ํ ํฌ๋์ด์ ๋ฅผ ๋ง๋ค์ด ๋ณด๊ฒ ์ต๋๋ค:
```python
>>> from tokenizers import Tokenizer
>>> from tokenizers.models import BPE
>>> from tokenizers.trainers import BpeTrainer
>>> from tokenizers.pre_tokenizers import Whitespace
>>> tokenizer = Tokenizer(BPE(unk_token="[UNK]"))
>>> trainer = BpeTrainer(special_tokens=["[UNK]", "[CLS]", "[SEP]", "[PAD]", "[MASK]"])
>>> tokenizer.pre_tokenizer = Whitespace()
>>> files = [...]
>>> tokenizer.train(files, trainer)
```
์ฐ๋ฆฌ๊ฐ ์ ์ํ ํ์ผ์ ํตํด ์ด์ ํ์ต๋ ํ ํฌ๋์ด์ ๋ฅผ ๊ฐ๊ฒ ๋์์ต๋๋ค. ์ด ๋ฐํ์์์ ๊ณ์ ์ฌ์ฉํ๊ฑฐ๋ JSON ํ์ผ๋ก ์ ์ฅํ์ฌ ๋์ค์ ์ฌ์ฉํ ์ ์์ต๋๋ค.
## ํ ํฌ๋์ด์ ๊ฐ์ฒด๋ก๋ถํฐ ์ง์ ๋ถ๋ฌ์ค๊ธฐ[[loading-directly-from-the-tokenizer-object]]
๐ค Transformers ๋ผ์ด๋ธ๋ฌ๋ฆฌ์์ ์ด ํ ํฌ๋์ด์ ๊ฐ์ฒด๋ฅผ ํ์ฉํ๋ ๋ฐฉ๋ฒ์ ์ดํด๋ณด๊ฒ ์ต๋๋ค.
[`PreTrainedTokenizerFast`] ํด๋์ค๋ ์ธ์คํด์คํ๋ *ํ ํฌ๋์ด์ * ๊ฐ์ฒด๋ฅผ ์ธ์๋ก ๋ฐ์ ์ฝ๊ฒ ์ธ์คํด์คํํ ์ ์์ต๋๋ค:
```python
>>> from transformers import PreTrainedTokenizerFast
>>> fast_tokenizer = PreTrainedTokenizerFast(tokenizer_object=tokenizer)
```
์ด์ `fast_tokenizer` ๊ฐ์ฒด๋ ๐ค Transformers ํ ํฌ๋์ด์ ์์ ๊ณต์ ํ๋ ๋ชจ๋ ๋ฉ์๋์ ํจ๊ป ์ฌ์ฉํ ์ ์์ต๋๋ค! ์์ธํ ๋ด์ฉ์ [ํ ํฌ๋์ด์ ํ์ด์ง](main_classes/tokenizer)๋ฅผ ์ฐธ์กฐํ์ธ์.
## JSON ํ์ผ์์ ๋ถ๋ฌ์ค๊ธฐ[[loading-from-a-JSON-file]]
<!--In order to load a tokenizer from a JSON file, let's first start by saving our tokenizer:-->
JSON ํ์ผ์์ ํ ํฌ๋์ด์ ๋ฅผ ๋ถ๋ฌ์ค๊ธฐ ์ํด, ๋จผ์ ํ ํฌ๋์ด์ ๋ฅผ ์ ์ฅํด ๋ณด๊ฒ ์ต๋๋ค:
```python
>>> tokenizer.save("tokenizer.json")
```
JSON ํ์ผ์ ์ ์ฅํ ๊ฒฝ๋ก๋ `tokenizer_file` ๋งค๊ฐ๋ณ์๋ฅผ ์ฌ์ฉํ์ฌ [`PreTrainedTokenizerFast`] ์ด๊ธฐํ ๋ฉ์๋์ ์ ๋ฌํ ์ ์์ต๋๋ค:
```python
>>> from transformers import PreTrainedTokenizerFast
>>> fast_tokenizer = PreTrainedTokenizerFast(tokenizer_file="tokenizer.json")
```
์ด์ `fast_tokenizer` ๊ฐ์ฒด๋ ๐ค Transformers ํ ํฌ๋์ด์ ์์ ๊ณต์ ํ๋ ๋ชจ๋ ๋ฉ์๋์ ํจ๊ป ์ฌ์ฉํ ์ ์์ต๋๋ค! ์์ธํ ๋ด์ฉ์ [ํ ํฌ๋์ด์ ํ์ด์ง](main_classes/tokenizer)๋ฅผ ์ฐธ์กฐํ์ธ์.
| 0 |
hf_public_repos/transformers/docs/source | hf_public_repos/transformers/docs/source/ko/tf_xla.md | <!--Copyright 2023 The HuggingFace Team. All rights reserved.
Licensed under the Apache License, Version 2.0 (the "License"); you may not use this file except in compliance with
the License. You may obtain a copy of the License at
http://www.apache.org/licenses/LICENSE-2.0
Unless required by applicable law or agreed to in writing, software distributed under the License is distributed on
an "AS IS" BASIS, WITHOUT WARRANTIES OR CONDITIONS OF ANY KIND, either express or implied. See the License for the
specific language governing permissions and limitations under the License.
โ ๏ธ Note that this file is in Markdown but contain specific syntax for our doc-builder (similar to MDX) that may not be
rendered properly in your Markdown viewer.
-->
# TensorFlow ๋ชจ๋ธ์ ์ํ XLA ํตํฉ [[xla-integration-for-tensorflow-models]]
[[open-in-colab]]
XLA(Accelerated Linear Algebra)๋ TensorFlow ๋ชจ๋ธ์ ์คํ ์๊ฐ์ ๊ฐ์ํํ๊ธฐ ์ํ ์ปดํ์ผ๋ฌ์
๋๋ค. [๊ณต์ ๋ฌธ์](https://www.tensorflow.org/xla)์ ๋ฐ๋ฅด๋ฉด ๋ค์๊ณผ ๊ฐ์ต๋๋ค:
XLA(Accelerated Linear Algebra)๋ ์ ํ ๋์๋ฅผ ์ํ ๋๋ฉ์ธ ํนํ ์ปดํ์ผ๋ฌ๋ก, TensorFlow ๋ชจ๋ธ์ ์์ค ์ฝ๋ ๋ณ๊ฒฝ ์์ด ๊ฐ์ํํ ์ ์์ต๋๋ค.
TensorFlow์์ XLA๋ฅผ ์ฌ์ฉํ๋ ๊ฒ์ ๊ฐ๋จํฉ๋๋ค. XLA๋ `tensorflow` ๋ผ์ด๋ธ๋ฌ๋ฆฌ ๋ด์ ํจํค์ง๋ก ์ ๊ณต๋๋ฉฐ, [`tf.function`](https://www.tensorflow.org/guide/intro_to_graphs)๊ณผ ๊ฐ์ ๊ทธ๋ํ ์์ฑ ํจ์์์ `jit_compile` ์ธ์๋ฅผ ์ฌ์ฉํ์ฌ ํ์ฑํํ ์ ์์ต๋๋ค. `fit()` ๋ฐ `predict()`์ ๊ฐ์ Keras ๋ฉ์๋๋ฅผ ์ฌ์ฉํ๋ ๊ฒฝ์ฐ, `jit_compile` ์ธ์๋ฅผ `model.compile()`์ ์ ๋ฌํ์ฌ XLA๋ฅผ ๊ฐ๋จํ๊ฒ ํ์ฑํํ ์ ์์ต๋๋ค. ๊ทธ๋ฌ๋ XLA๋ ์ด๋ฌํ ๋ฉ์๋์ ๊ตญํ๋์ง ์๊ณ ์์์ `tf.function`์ ๊ฐ์ํํ๋ ๋ฐ์๋ ์ฌ์ฉํ ์ ์์ต๋๋ค.
๐ค Transformers์์๋ [GPT2](https://huggingface.co/docs/transformers/model_doc/gpt2), [T5](https://huggingface.co/docs/transformers/model_doc/t5), [OPT](https://huggingface.co/docs/transformers/model_doc/opt)์ ๊ฐ์ ๋ชจ๋ธ์ ํ
์คํธ ์์ฑ, ๊ทธ๋ฆฌ๊ณ [Whisper](https://huggingface.co/docs/transformers/model_doc/whisper)์ ๊ฐ์ ๋ชจ๋ธ์ ์์ฑ ์ฒ๋ฆฌ๋ฅผ ํฌํจํ์ฌ ์ฌ๋ฌ TensorFlow ๋ฉ์๋๊ฐ XLA์ ํธํ๋๋๋ก ๋ค์ ์์ฑ๋์์ต๋๋ค.
์ ํํ ์๋ ํฅ์์ ๋ชจ๋ธ์ ๋ฐ๋ผ ๋ค๋ฅด์ง๋ง, ๐ค Transformers ๋ด์ TensorFlow ํ
์คํธ ์์ฑ ๋ชจ๋ธ์ ๊ฒฝ์ฐ ์ต๋ 100๋ฐฐ์ ์๋ ํฅ์์ ํ์ธํ์ต๋๋ค. ์ด ๋ฌธ์์์๋ ์ด๋ฌํ ๋ชจ๋ธ์ ๋ํด XLA๋ฅผ ์ฌ์ฉํ์ฌ ์ต๋ ์ฑ๋ฅ์ ์ป๋ ๋ฐฉ๋ฒ์ ์ค๋ช
ํฉ๋๋ค. ๋ํ XLA ํตํฉ์ ๋ฒค์น๋งํฌ ๋ฐ ๋์์ธ ์ฒ ํ์ ๋ํ ์ถ๊ฐ ์๋ฃ ๋งํฌ๋ ์ ๊ณตํ ๊ฒ์
๋๋ค.
## XLA๋ฅผ ์ฌ์ฉํ์ฌ TF ํจ์ ์คํํ๊ธฐ [[running-tf-functions-with-xla]]
TensorFlow์์ ๋ค์๊ณผ ๊ฐ์ ๋ชจ๋ธ์ ๊ณ ๋ คํด ๋ด
์๋ค:
```py
import tensorflow as tf
model = tf.keras.Sequential(
[tf.keras.layers.Dense(10, input_shape=(10,), activation="relu"), tf.keras.layers.Dense(5, activation="softmax")]
)
```
์ ๋ชจ๋ธ์ ์ฐจ์์ด `(10, )`์ธ ์
๋ ฅ์ ๋ฐ์ต๋๋ค. ๋ค์๊ณผ ๊ฐ์ด ๋ชจ๋ธ์ ์ฌ์ฉํ์ฌ ์์ ํ๋ฅผ ์คํํ ์ ์์ต๋๋ค:
```py
# ๋ชจ๋ธ์ ๋ํ ์์์ ์
๋ ฅ์ ์์ฑํฉ๋๋ค.
batch_size = 16
input_vector_dim = 10
random_inputs = tf.random.normal((batch_size, input_vector_dim))
# ์์ ํ๋ฅผ ์คํํฉ๋๋ค.
_ = model(random_inputs)
```
XLA๋ก ์ปดํ์ผ๋ ํจ์๋ก ์์ ํ๋ฅผ ์คํํ๋ ค๋ฉด ๋ค์๊ณผ ๊ฐ์ด ํด์ผ ํฉ๋๋ค:
```py
xla_fn = tf.function(model, jit_compile=True)
_ = xla_fn(random_inputs)
```
`model`์ ๊ธฐ๋ณธ `call()` ํจ์๋ XLA ๊ทธ๋ํ๋ฅผ ์ปดํ์ผํ๋ ๋ฐ ์ฌ์ฉ๋ฉ๋๋ค. ๊ทธ๋ฌ๋ ๋ค๋ฅธ ๋ชจ๋ธ ํจ์๋ฅผ XLA๋ก ์ปดํ์ผํ๋ ค๋ฉด ๋ค์๊ณผ ๊ฐ์ด ํ ์๋ ์์ต๋๋ค:
```py
my_xla_fn = tf.function(model.my_xla_fn, jit_compile=True)
```
## ๐ค Transformers์์ XLA๋ฅผ ์ฌ์ฉํ์ฌ TF ํ
์คํธ ์์ฑ ๋ชจ๋ธ ์คํํ๊ธฐ [[running-a-tf-text-generation-model-with-xla-from-transformers]]
๐ค Transformers์์ XLA๋ก ๊ฐ์ํ๋ ์์ฑ์ ํ์ฑํํ๋ ค๋ฉด ์ต์ ๋ฒ์ ์ `transformers`๊ฐ ์ค์น๋์ด ์์ด์ผ ํฉ๋๋ค. ๋ค์๊ณผ ๊ฐ์ด ์ค์นํ ์ ์์ต๋๋ค:
```bash
pip install transformers --upgrade
```
๊ทธ๋ฆฌ๊ณ ๋ค์ ์ฝ๋๋ฅผ ์คํํ ์ ์์ต๋๋ค:
```py
import tensorflow as tf
from transformers import AutoTokenizer, TFAutoModelForCausalLM
# ์ต์ ๋ฒ์ ์ Transformers๊ฐ ์ค์น๋์ด ์์ง ์๋ค๋ฉด ์ค๋ฅ๊ฐ ๋ฐ์ํฉ๋๋ค.
from transformers.utils import check_min_version
check_min_version("4.21.0")
tokenizer = AutoTokenizer.from_pretrained("gpt2", padding_side="left", pad_token="</s>")
model = TFAutoModelForCausalLM.from_pretrained("gpt2")
input_string = ["TensorFlow is"]
# XLA ์์ฑ ํจ์๋ฅผ ๋ง๋ค๊ธฐ ์ํ ํ ์ค
xla_generate = tf.function(model.generate, jit_compile=True)
tokenized_input = tokenizer(input_string, return_tensors="tf")
generated_tokens = xla_generate(**tokenized_input, num_beams=2)
decoded_text = tokenizer.decode(generated_tokens[0], skip_special_tokens=True)
print(f"Generated -- {decoded_text}")
# Generated -- TensorFlow is an open-source, open-source, distributed-source application # framework for the
```
์ ์ ์๋ฏ์ด, `generate()`์์ XLA๋ฅผ ํ์ฑํํ๋ ๊ฒ์ ๋จ ํ ์ค์ ์ฝ๋์
๋๋ค. ์ฝ๋์ ๋๋จธ์ง ๋ถ๋ถ์ ๋ณ๊ฒฝ๋์ง ์์ต๋๋ค. ๊ทธ๋ฌ๋ ์ ์ฝ๋ ์ค๋ํซ์์๋ XLA์ ํน์ ํ ๋ช ๊ฐ์ง ์ฃผ์ํ ์ ์ด ์์ต๋๋ค. XLA๊ฐ ๊ฐ์ ธ๋ค์ค ์๋ ํฅ์์ ์คํํ๊ธฐ ์ํด์๋ ์ด๋ฅผ ์๊ณ ์์ด์ผ ํฉ๋๋ค. ๋ค์ ์น์
์์ ์ด์ ๋ํด ๋
ผ์ํฉ๋๋ค.
## ์ฃผ์ํ ์ [[gotchas-to-be-aware-of]]
XLA ํ์ฑํ ํจ์(`xla_generate()`์ ๊ฐ์)๋ฅผ ์ฒ์ ์คํํ ๋ ๋ด๋ถ์ ์ผ๋ก ๊ณ์ฐ ๊ทธ๋ํ๋ฅผ ์ถ๋ก ํ๋ ค๊ณ ํ๋ฉฐ, ์ด๋ ์๊ฐ์ด ์์๋ฉ๋๋ค. ์ด ๊ณผ์ ์ [โ์ถ์ (tracing)โ](https://www.tensorflow.org/guide/intro_to_graphs#when_is_a_function_tracing)์ด๋ผ๊ณ ์๋ ค์ ธ ์์ต๋๋ค.
์์ฑ ์๊ฐ์ด ๋น ๋ฅด์ง ์๋ค๋ ๊ฒ์ ์ ์ ์์ ๊ฒ์
๋๋ค. `xla_generate()`(๋๋ ๋ค๋ฅธ XLA ํ์ฑํ ํจ์)์ ์ฐ์ ํธ์ถ์ ํจ์์ ์ ๋ฌ๋ ์
๋ ฅ์ด ์ด๊ธฐ์ ๊ตฌ์ถ๋ ๊ณ์ฐ ๊ทธ๋ํ์ ๋์ผํ ํํ๋ฅผ ๋ฐ๋ฅธ๋ค๋ฉด, ๊ณ์ฐ ๊ทธ๋ํ๋ฅผ ์ถ๋ก ํ ํ์๊ฐ ์์ต๋๋ค. ์ด๋ ์
๋ ฅ ํํ๊ฐ ๊ณ ์ ๋ ๋ชจ๋ฌ๋ฆฌํฐ(์: ์ด๋ฏธ์ง)์๋ ๋ฌธ์ ๊ฐ ๋์ง ์์ง๋ง, ๊ฐ๋ณ ์
๋ ฅ ํํ ๋ชจ๋ฌ๋ฆฌํฐ(์: ํ
์คํธ)๋ฅผ ์ฌ์ฉํ ๋ ์ฃผ์ํด์ผ ํฉ๋๋ค.
`xla_generate()`๊ฐ ํญ์ ๋์ผํ ์
๋ ฅ ํํ๋ก ๋์ํ๋๋ก ํ๋ ค๋ฉด, ํ ํฌ๋์ด์ ๋ฅผ ํธ์ถํ ๋ `padding` ์ธ์๋ฅผ ์ง์ ํ ์ ์์ต๋๋ค.
```py
import tensorflow as tf
from transformers import AutoTokenizer, TFAutoModelForCausalLM
tokenizer = AutoTokenizer.from_pretrained("gpt2", padding_side="left", pad_token="</s>")
model = TFAutoModelForCausalLM.from_pretrained("gpt2")
input_string = ["TensorFlow is"]
xla_generate = tf.function(model.generate, jit_compile=True)
# ์ฌ๊ธฐ์, padding ์ต์
์ด ์๋ ํ ํฌ๋์ด์ ๋ฅผ ํธ์ถํฉ๋๋ค.
tokenized_input = tokenizer(input_string, pad_to_multiple_of=8, padding=True, return_tensors="tf")
generated_tokens = xla_generate(**tokenized_input, num_beams=2)
decoded_text = tokenizer.decode(generated_tokens[0], skip_special_tokens=True)
print(f"Generated -- {decoded_text}")
```
์ด๋ ๊ฒ ํ๋ฉด `xla_generate()`์ ๋ํ ์
๋ ฅ์ด ํญ์ ์ถ์ ๋ ํํ๋ก ์ ๋ฌ๋์ด ์์ฑ ์๊ฐ์ด ๊ฐ์ํ๋ฉ๋๋ค. ๋ค์ ์ฝ๋๋ก ์ด๋ฅผ ํ์ธํ ์ ์์ต๋๋ค:
```py
import time
import tensorflow as tf
from transformers import AutoTokenizer, TFAutoModelForCausalLM
tokenizer = AutoTokenizer.from_pretrained("gpt2", padding_side="left", pad_token="</s>")
model = TFAutoModelForCausalLM.from_pretrained("gpt2")
xla_generate = tf.function(model.generate, jit_compile=True)
for input_string in ["TensorFlow is", "TensorFlow is a", "TFLite is a"]:
tokenized_input = tokenizer(input_string, pad_to_multiple_of=8, padding=True, return_tensors="tf")
start = time.time_ns()
generated_tokens = xla_generate(**tokenized_input, num_beams=2)
end = time.time_ns()
print(f"Execution time -- {(end - start) / 1e6:.1f} ms\n")
```
Tesla T4 GPU์์๋ ๋ค์๊ณผ ๊ฐ์ ์ถ๋ ฅ์ ์์ํ ์ ์์ต๋๋ค:
```bash
Execution time -- 30819.6 ms
Execution time -- 79.0 ms
Execution time -- 78.9 ms
```
`xla_generate()`์ ์ฒซ ๋ฒ์งธ ํธ์ถ์ ์ถ์ ๋๋ฌธ์ ์๊ฐ์ด ์ค๋ ๊ฑธ๋ฆฌ์ง๋ง, ์ฐ์ ํธ์ถ์ ๋ช ๋ฐฐ๋ ๋น ๋ฆ
๋๋ค. ์์ฑ ์ต์
์ ๋ํ ์ด๋ค ๋ณ๊ฒฝ์ด๋ ๋ค์ ์ถ์ ์ ์ ๋ฐํ๋ฏ๋ก ์์ฑ ์๊ฐ์ด ๋๋ ค์ง ์ ์์์ ๋ช
์ฌํ์ธ์.
์ด ๋ฌธ์์์๋ ๐ค Transformers์์ ์ ๊ณตํ๋ ๋ชจ๋ ํ
์คํธ ์์ฑ ์ต์
์ ๋ค๋ฃจ์ง ์์์ต๋๋ค. ๊ณ ๊ธ ์ฌ์ฉ ์ฌ๋ก์ ๋ํด ๋ฌธ์๋ฅผ ์ฐธ์กฐํ์๊ธฐ ๋ฐ๋๋๋ค.
## ์ถ๊ฐ ์๋ฃ [[additional-resources]]
์ฌ๊ธฐ์ ๐ค Transformers์ XLA์ ๋ํด ๋ ์์ธํ ์๊ณ ์ถ์ ๊ฒฝ์ฐ ๋์์ด ๋ ์ ์๋ ๋ช ๊ฐ์ง ์ถ๊ฐ ์๋ฃ๋ฅผ ์ ๊ณตํฉ๋๋ค.
* [์ด Colab ๋
ธํธ๋ถ](https://colab.research.google.com/github/huggingface/blog/blob/main/notebooks/91_tf_xla_generate.ipynb)์ XLA์ ํธํ๋๋ ์ธ์ฝ๋-๋์ฝ๋([T5](https://huggingface.co/docs/transformers/model_doc/t5)์ ๊ฐ์) ๋ฐ ๋์ฝ๋ ์ ์ฉ([GPT2](https://huggingface.co/docs/transformers/model_doc/gpt2)์ ๊ฐ์) ํ
์คํธ ์์ฑ ๋ชจ๋ธ์ ์คํํด ๋ณผ ์ ์๋ ๋ํํ ๋ฐ๋ชจ๋ฅผ ์ ๊ณตํฉ๋๋ค.
* [์ด ๋ธ๋ก๊ทธ ๊ธ](https://huggingface.co/blog/tf-xla-generate)์ TensorFlow์์ XLA์ ๋ํ ์น์ ํ ์๊ฐ์ ํจ๊ป XLA์ ํธํ๋๋ ๋ชจ๋ธ์ ๋น๊ต ๋ฒค์น๋งํฌ์ ๋ํ ๊ฐ์๋ฅผ ์ ๊ณตํฉ๋๋ค.
* [์ด ๋ธ๋ก๊ทธ ๊ธ](https://blog.tensorflow.org/2022/11/how-hugging-face-improved-text-generation-performance-with-xla.html)์ ๐ค Transformers์ TensorFlow ๋ชจ๋ธ์ XLA ์ง์์ ์ถ๊ฐํ๋ ๊ฒ์ ๋ํ ๋์์ธ ์ฒ ํ์ ๋
ผ์ํฉ๋๋ค.
* XLA์ TensorFlow ๊ทธ๋ํ์ ๋ํด ๋ ์์ธํ ์๊ณ ์ถ์ ๊ฒฝ์ฐ ์ถ์ฒํ๋ ๊ธ:
* [XLA: ๊ธฐ๊ณ ํ์ต์ ์ํ ์ต์ ํ ์ปดํ์ผ๋ฌ](https://www.tensorflow.org/xla)
* [๊ทธ๋ํ ๋ฐ tf.function ์๊ฐ](https://www.tensorflow.org/guide/intro_to_graphs)
* [tf.function์ผ๋ก ์ฑ๋ฅ ํฅ์ํ๊ธฐ](https://www.tensorflow.org/guide/function) | 0 |
hf_public_repos/transformers/docs/source | hf_public_repos/transformers/docs/source/ko/_config.py | # docstyle-ignore
INSTALL_CONTENT = """
# Transformers ์ค์น ๋ฐฉ๋ฒ
! pip install transformers datasets
# ๋ง์ง๋ง ๋ฆด๋ฆฌ์ค ๋์ ์์ค์์ ์ค์นํ๋ ค๋ฉด, ์ ๋ช
๋ น์ ์ฃผ์์ผ๋ก ๋ฐ๊พธ๊ณ ์๋ ๋ช
๋ น์ ํด์ ํ์ธ์.
# ! pip install git+https://github.com/huggingface/transformers.git
"""
notebook_first_cells = [{"type": "code", "content": INSTALL_CONTENT}]
black_avoid_patterns = {
"{processor_class}": "FakeProcessorClass",
"{model_class}": "FakeModelClass",
"{object_class}": "FakeObjectClass",
}
| 0 |
hf_public_repos/transformers/docs/source | hf_public_repos/transformers/docs/source/ko/serialization.md | <!--Copyright 2020 The HuggingFace Team. All rights reserved.
Licensed under the Apache License, Version 2.0 (the "License"); you may not use this file except in compliance with
the License. You may obtain a copy of the License at
http://www.apache.org/licenses/LICENSE-2.0
Unless required by applicable law or agreed to in writing, software distributed under the License is distributed on
an "AS IS" BASIS, WITHOUT WARRANTIES OR CONDITIONS OF ANY KIND, either express or implied. See the License for the
specific language governing permissions and limitations under the License.
โ ๏ธ Note that this file is in Markdown but contain specific syntax for our doc-builder (similar to MDX) that may not be
rendered properly in your Markdown viewer.
-->
# ONNX๋ก ๋ด๋ณด๋ด๊ธฐ [[export-to-onnx]]
๐ค Transformers ๋ชจ๋ธ์ ์ ํ ํ๊ฒฝ์์ ๋ฐฐํฌํ๊ธฐ ์ํด์๋ ๋ชจ๋ธ์ ์ง๋ ฌํ๋ ํ์์ผ๋ก ๋ด๋ณด๋ด๊ณ ํน์ ๋ฐํ์๊ณผ ํ๋์จ์ด์์ ๋ก๋ํ๊ณ ์คํํ ์ ์์ผ๋ฉด ์ ์ฉํฉ๋๋ค.
๐ค Optimum์ Transformers์ ํ์ฅ์ผ๋ก, PyTorch ๋๋ TensorFlow์์ ๋ชจ๋ธ์ ONNX์ TFLite์ ๊ฐ์ ์ง๋ ฌํ๋ ํ์์ผ๋ก ๋ด๋ณด๋ผ ์ ์๋๋ก ํ๋ `exporters` ๋ชจ๋์ ํตํด ์ ๊ณต๋ฉ๋๋ค. ๐ค Optimum์ ๋ํ ์ฑ๋ฅ ์ต์ ํ ๋๊ตฌ ์ธํธ๋ฅผ ์ ๊ณตํ์ฌ ํน์ ํ๋์จ์ด์์ ๋ชจ๋ธ์ ํ๋ จํ๊ณ ์คํํ ๋ ์ต๋ ํจ์จ์ฑ์ ๋ฌ์ฑํ ์ ์์ต๋๋ค.
์ด ์๋ด์๋ ๐ค Optimum์ ์ฌ์ฉํ์ฌ ๐ค Transformers ๋ชจ๋ธ์ ONNX๋ก ๋ด๋ณด๋ด๋ ๋ฐฉ๋ฒ์ ๋ณด์ฌ์ค๋๋ค. TFLite๋ก ๋ชจ๋ธ์ ๋ด๋ณด๋ด๋ ์๋ด์๋ [TFLite๋ก ๋ด๋ณด๋ด๊ธฐ ํ์ด์ง](tflite)๋ฅผ ์ฐธ์กฐํ์ธ์.
## ONNX๋ก ๋ด๋ณด๋ด๊ธฐ [[export-to-onnx]]
[ONNX (Open Neural Network eXchange)](http://onnx.ai)๋ PyTorch์ TensorFlow๋ฅผ ํฌํจํ ๋ค์ํ ํ๋ ์์ํฌ์์ ์ฌ์ธต ํ์ต ๋ชจ๋ธ์ ๋ํ๋ด๋ ๋ฐ ์ฌ์ฉ๋๋ ๊ณตํต ์ฐ์ฐ์ ์ธํธ์ ๊ณตํต ํ์ผ ํ์์ ์ ์ํ๋ ์คํ ํ์ค์
๋๋ค. ๋ชจ๋ธ์ด ONNX ํ์์ผ๋ก ๋ด๋ณด๋ด์ง๋ฉด ์ด๋ฌํ ์ฐ์ฐ์๋ฅผ ์ฌ์ฉํ์ฌ ์ ๊ฒฝ๋ง์ ํตํด ๋ฐ์ดํฐ๊ฐ ํ๋ฅด๋ ํ๋ฆ์ ๋ํ๋ด๋ ๊ณ์ฐ ๊ทธ๋ํ(์ผ๋ฐ์ ์ผ๋ก _์ค๊ฐ ํํ_์ด๋ผ๊ณ ํจ)๊ฐ ๊ตฌ์ฑ๋ฉ๋๋ค.
ํ์คํ๋ ์ฐ์ฐ์์ ๋ฐ์ดํฐ ์ ํ์ ๊ฐ์ง ๊ทธ๋ํ๋ฅผ ๋
ธ์ถํจ์ผ๋ก์จ, ONNX๋ ํ๋ ์์ํฌ ๊ฐ์ ์ฝ๊ฒ ์ ํํ ์ ์์ต๋๋ค. ์๋ฅผ ๋ค์ด, PyTorch์์ ํ๋ จ๋ ๋ชจ๋ธ์ ONNX ํ์์ผ๋ก ๋ด๋ณด๋ด๊ณ TensorFlow์์ ๊ฐ์ ธ์ฌ ์ ์์ต๋๋ค(๊ทธ ๋ฐ๋๋ ๊ฐ๋ฅํฉ๋๋ค).
ONNX ํ์์ผ๋ก ๋ด๋ณด๋ธ ๋ชจ๋ธ์ ๋ค์๊ณผ ๊ฐ์ด ์ฌ์ฉํ ์ ์์ต๋๋ค:
- [๊ทธ๋ํ ์ต์ ํ](https://huggingface.co/docs/optimum/onnxruntime/usage_guides/optimization) ๋ฐ [์์ํ](https://huggingface.co/docs/optimum/onnxruntime/usage_guides/quantization)์ ๊ฐ์ ๊ธฐ๋ฒ์ ์ฌ์ฉํ์ฌ ์ถ๋ก ์ ์ํด ์ต์ ํ๋ฉ๋๋ค.
- ONNX Runtime์ ํตํด ์คํํ ์ ์์ต๋๋ค. [`ORTModelForXXX` ํด๋์ค๋ค](https://huggingface.co/docs/optimum/onnxruntime/package_reference/modeling_ort)์ ํตํด ๋์ผํ `AutoModel` API๋ฅผ ๋ฐ๋ฆ
๋๋ค. ์ด API๋ ๐ค Transformers์์ ์ฌ์ฉํ๋ ๊ฒ๊ณผ ๋์ผํฉ๋๋ค.
- [์ต์ ํ๋ ์ถ๋ก ํ์ดํ๋ผ์ธ](https://huggingface.co/docs/optimum/main/en/onnxruntime/usage_guides/pipelines)์ ์ฌ์ฉํ ์ ์์ต๋๋ค. ์ด๋ ๐ค Transformers์ [`pipeline`] ํจ์์ ๋์ผํ API๋ฅผ ๊ฐ์ง๊ณ ์์ต๋๋ค.
๐ค Optimum์ ๊ตฌ์ฑ ๊ฐ์ฒด๋ฅผ ํ์ฉํ์ฌ ONNX ๋ด๋ณด๋ด๊ธฐ๋ฅผ ์ง์ํฉ๋๋ค. ์ด๋ฌํ ๊ตฌ์ฑ ๊ฐ์ฒด๋ ์ฌ๋ฌ ๋ชจ๋ธ ์ํคํ
์ฒ์ ๋ํด ๋ฏธ๋ฆฌ ์ค๋น๋์ด ์์ผ๋ฉฐ ๋ค๋ฅธ ์ํคํ
์ฒ์ ์ฝ๊ฒ ํ์ฅํ ์ ์๋๋ก ์ค๊ณ๋์์ต๋๋ค.
๋ฏธ๋ฆฌ ์ค๋น๋ ๊ตฌ์ฑ ๋ชฉ๋ก์ [๐ค Optimum ๋ฌธ์](https://huggingface.co/docs/optimum/exporters/onnx/overview)๋ฅผ ์ฐธ์กฐํ์ธ์.
๐ค Transformers ๋ชจ๋ธ์ ONNX๋ก ๋ด๋ณด๋ด๋ ๋ ๊ฐ์ง ๋ฐฉ๋ฒ์ด ์์ต๋๋ค. ์ฌ๊ธฐ์์ ๋ ๊ฐ์ง ๋ฐฉ๋ฒ์ ๋ชจ๋ ๋ณด์ฌ์ค๋๋ค:
- ๐ค Optimum์ ์ฌ์ฉํ์ฌ CLI๋ก ๋ด๋ณด๋ด๊ธฐ
- `optimum.onnxruntime`์ ์ฌ์ฉํ์ฌ ๐ค Optimum์ผ๋ก ONNX๋ก ๋ด๋ณด๋ด๊ธฐ
### CLI๋ฅผ ์ฌ์ฉํ์ฌ ๐ค Transformers ๋ชจ๋ธ์ ONNX๋ก ๋ด๋ณด๋ด๊ธฐ [[exporting-a-transformers-model-to-onnx-with-cli]]
๐ค Transformers ๋ชจ๋ธ์ ONNX๋ก ๋ด๋ณด๋ด๋ ค๋ฉด ๋จผ์ ์ถ๊ฐ ์ข
์์ฑ์ ์ค์นํ์ธ์:
```bash
pip install optimum[exporters]
```
์ฌ์ฉ ๊ฐ๋ฅํ ๋ชจ๋ ์ธ์๋ฅผ ํ์ธํ๋ ค๋ฉด [๐ค Optimum ๋ฌธ์](https://huggingface.co/docs/optimum/exporters/onnx/usage_guides/export_a_model#exporting-a-model-to-onnx-using-the-cli)๋ฅผ ์ฐธ์กฐํ๊ฑฐ๋ ๋ช
๋ น์ค์์ ๋์๋ง์ ๋ณด์ธ์.
```bash
optimum-cli export onnx --help
```
์๋ฅผ ๋ค์ด, ๐ค Hub์์ `distilbert-base-uncased-distilled-squad`์ ๊ฐ์ ๋ชจ๋ธ์ ์ฒดํฌํฌ์ธํธ๋ฅผ ๋ด๋ณด๋ด๋ ค๋ฉด ๋ค์ ๋ช
๋ น์ ์คํํ์ธ์:
```bash
optimum-cli export onnx --model distilbert-base-uncased-distilled-squad distilbert_base_uncased_squad_onnx/
```
์์ ๊ฐ์ด ์งํ ์ํฉ์ ๋ํ๋ด๋ ๋ก๊ทธ๊ฐ ํ์๋๊ณ ๊ฒฐ๊ณผ์ธ `model.onnx`๊ฐ ์ ์ฅ๋ ์์น๊ฐ ํ์๋ฉ๋๋ค.
```bash
Validating ONNX model distilbert_base_uncased_squad_onnx/model.onnx...
-[โ] ONNX model output names match reference model (start_logits, end_logits)
- Validating ONNX Model output "start_logits":
-[โ] (2, 16) matches (2, 16)
-[โ] all values close (atol: 0.0001)
- Validating ONNX Model output "end_logits":
-[โ] (2, 16) matches (2, 16)
-[โ] all values close (atol: 0.0001)
The ONNX export succeeded and the exported model was saved at: distilbert_base_uncased_squad_onnx
```
์์ ์์ ๋ ๐ค Hub์์ ์ฒดํฌํฌ์ธํธ๋ฅผ ๋ด๋ณด๋ด๋ ๊ฒ์ ์ค๋ช
ํฉ๋๋ค. ๋ก์ปฌ ๋ชจ๋ธ์ ๋ด๋ณด๋ผ ๋์๋ ๋ชจ๋ธ์ ๊ฐ์ค์น์ ํ ํฌ๋์ด์ ํ์ผ์ ๋์ผํ ๋๋ ํ ๋ฆฌ(`local_path`)์ ์ ์ฅํ๋์ง ํ์ธํ์ธ์. CLI๋ฅผ ์ฌ์ฉํ ๋์๋ ๐ค Hub์ ์ฒดํฌํฌ์ธํธ ์ด๋ฆ ๋์ `model` ์ธ์์ `local_path`๋ฅผ ์ ๋ฌํ๊ณ `--task` ์ธ์๋ฅผ ์ ๊ณตํ์ธ์. ์ง์๋๋ ์์
์ ๋ชฉ๋ก์ [๐ค Optimum ๋ฌธ์](https://huggingface.co/docs/optimum/exporters/task_manager)๋ฅผ ์ฐธ์กฐํ์ธ์. `task` ์ธ์๊ฐ ์ ๊ณต๋์ง ์์ผ๋ฉด ์์
์ ํนํ๋ ํค๋ ์์ด ๋ชจ๋ธ ์ํคํ
์ฒ๋ก ๊ธฐ๋ณธ ์ค์ ๋ฉ๋๋ค.
```bash
optimum-cli export onnx --model local_path --task question-answering distilbert_base_uncased_squad_onnx/
```
๊ทธ ๊ฒฐ๊ณผ๋ก ์์ฑ๋ `model.onnx` ํ์ผ์ ONNX ํ์ค์ ์ง์ํ๋ ๋ง์ [๊ฐ์๊ธฐ](https://onnx.ai/supported-tools.html#deployModel) ์ค ํ๋์์ ์คํํ ์ ์์ต๋๋ค. ์๋ฅผ ๋ค์ด, [ONNX Runtime](https://onnxruntime.ai/)์ ์ฌ์ฉํ์ฌ ๋ชจ๋ธ์ ๋ก๋ํ๊ณ ์คํํ ์ ์์ต๋๋ค:
```python
>>> from transformers import AutoTokenizer
>>> from optimum.onnxruntime import ORTModelForQuestionAnswering
>>> tokenizer = AutoTokenizer.from_pretrained("distilbert_base_uncased_squad_onnx")
>>> model = ORTModelForQuestionAnswering.from_pretrained("distilbert_base_uncased_squad_onnx")
>>> inputs = tokenizer("What am I using?", "Using DistilBERT with ONNX Runtime!", return_tensors="pt")
>>> outputs = model(**inputs)
```
Hub์ TensorFlow ์ฒดํฌํฌ์ธํธ์ ๋ํด์๋ ๋์ผํ ํ๋ก์ธ์ค๊ฐ ์ ์ฉ๋ฉ๋๋ค. ์๋ฅผ ๋ค์ด, [Keras organization](https://huggingface.co/keras-io)์์ ์์ํ TensorFlow ์ฒดํฌํฌ์ธํธ๋ฅผ ๋ด๋ณด๋ด๋ ๋ฐฉ๋ฒ์ ๋ค์๊ณผ ๊ฐ์ต๋๋ค:
```bash
optimum-cli export onnx --model keras-io/transformers-qa distilbert_base_cased_squad_onnx/
```
### `optimum.onnxruntime`์ ์ฌ์ฉํ์ฌ ๐ค Transformers ๋ชจ๋ธ์ ONNX๋ก ๋ด๋ณด๋ด๊ธฐ [[exporting-a-transformers-model-to-onnx-with-optimumonnxruntime]]
CLI ๋์ ์ `optimum.onnxruntime`์ ์ฌ์ฉํ์ฌ ํ๋ก๊ทธ๋๋ฐ ๋ฐฉ์์ผ๋ก ๐ค Transformers ๋ชจ๋ธ์ ONNX๋ก ๋ด๋ณด๋ผ ์๋ ์์ต๋๋ค. ๋ค์๊ณผ ๊ฐ์ด ์งํํ์ธ์:
```python
>>> from optimum.onnxruntime import ORTModelForSequenceClassification
>>> from transformers import AutoTokenizer
>>> model_checkpoint = "distilbert_base_uncased_squad"
>>> save_directory = "onnx/"
>>> # Load a model from transformers and export it to ONNX
>>> ort_model = ORTModelForSequenceClassification.from_pretrained(model_checkpoint, export=True)
>>> tokenizer = AutoTokenizer.from_pretrained(model_checkpoint)
>>> # Save the onnx model and tokenizer
>>> ort_model.save_pretrained(save_directory)
>>> tokenizer.save_pretrained(save_directory)
```
### ์ง์๋์ง ์๋ ์ํคํ
์ฒ์ ๋ชจ๋ธ ๋ด๋ณด๋ด๊ธฐ [[exporting-a-model-for-an-unsupported-architecture]]
ํ์ฌ ๋ด๋ณด๋ผ ์ ์๋ ๋ชจ๋ธ์ ์ง์ํ๊ธฐ ์ํด ๊ธฐ์ฌํ๋ ค๋ฉด, ๋จผ์ [`optimum.exporters.onnx`](https://huggingface.co/docs/optimum/exporters/onnx/overview)์์ ์ง์๋๋์ง ํ์ธํ ํ ์ง์๋์ง ์๋ ๊ฒฝ์ฐ์๋ [๐ค Optimum์ ๊ธฐ์ฌ](https://huggingface.co/docs/optimum/exporters/onnx/usage_guides/contribute)ํ์ธ์.
### `transformers.onnx`๋ฅผ ์ฌ์ฉํ์ฌ ๋ชจ๋ธ ๋ด๋ณด๋ด๊ธฐ [[exporting-a-model-with-transformersonnx]]
<Tip warning={true}>
`tranformers.onnx`๋ ๋ ์ด์ ์ ์ง๋์ง ์์ต๋๋ค. ์์์ ์ค๋ช
ํ ๋๋ก ๐ค Optimum์ ์ฌ์ฉํ์ฌ ๋ชจ๋ธ์ ๋ด๋ณด๋ด์ธ์. ์ด ์น์
์ ํฅํ ๋ฒ์ ์์ ์ ๊ฑฐ๋ ์์ ์
๋๋ค.
</Tip>
๐ค Transformers ๋ชจ๋ธ์ ONNX๋ก ๋ด๋ณด๋ด๋ ค๋ฉด ์ถ๊ฐ ์ข
์์ฑ์ ์ค์นํ์ธ์:
```bash
pip install transformers[onnx]
```
`transformers.onnx` ํจํค์ง๋ฅผ Python ๋ชจ๋๋ก ์ฌ์ฉํ์ฌ ์ค๋น๋ ๊ตฌ์ฑ์ ์ฌ์ฉํ์ฌ ์ฒดํฌํฌ์ธํธ๋ฅผ ๋ด๋ณด๋
๋๋ค:
```bash
python -m transformers.onnx --model=distilbert-base-uncased onnx/
```
์ด๋ ๊ฒ ํ๋ฉด `--model` ์ธ์์ ์ ์๋ ์ฒดํฌํฌ์ธํธ์ ONNX ๊ทธ๋ํ๊ฐ ๋ด๋ณด๋ด์ง๋๋ค. ๐ค Hub์์ ์ ๊ณตํ๋ ์ฒดํฌํฌ์ธํธ๋ ๋ก์ปฌ์ ์ ์ฅ๋ ์ฒดํฌํฌ์ธํธ๋ฅผ ์ ๋ฌํ ์ ์์ต๋๋ค. ๊ฒฐ๊ณผ๋ก ์์ฑ๋ `model.onnx` ํ์ผ์ ONNX ํ์ค์ ์ง์ํ๋ ๋ง์ ๊ฐ์๊ธฐ ์ค ํ๋์์ ์คํํ ์ ์์ต๋๋ค. ์๋ฅผ ๋ค์ด, ๋ค์๊ณผ ๊ฐ์ด ONNX Runtime์ ์ฌ์ฉํ์ฌ ๋ชจ๋ธ์ ๋ก๋ํ๊ณ ์คํํ ์ ์์ต๋๋ค:
```python
>>> from transformers import AutoTokenizer
>>> from onnxruntime import InferenceSession
>>> tokenizer = AutoTokenizer.from_pretrained("distilbert-base-uncased")
>>> session = InferenceSession("onnx/model.onnx")
>>> # ONNX Runtime expects NumPy arrays as input
>>> inputs = tokenizer("Using DistilBERT with ONNX Runtime!", return_tensors="np")
>>> outputs = session.run(output_names=["last_hidden_state"], input_feed=dict(inputs))
```
ํ์ํ ์ถ๋ ฅ ์ด๋ฆ(์: `["last_hidden_state"]`)์ ๊ฐ ๋ชจ๋ธ์ ONNX ๊ตฌ์ฑ์ ํ์ธํ์ฌ ์ป์ ์ ์์ต๋๋ค. ์๋ฅผ ๋ค์ด, DistilBERT์ ๊ฒฝ์ฐ ๋ค์๊ณผ ๊ฐ์ต๋๋ค:
```python
>>> from transformers.models.distilbert import DistilBertConfig, DistilBertOnnxConfig
>>> config = DistilBertConfig()
>>> onnx_config = DistilBertOnnxConfig(config)
>>> print(list(onnx_config.outputs.keys()))
["last_hidden_state"]
```
Hub์ TensorFlow ์ฒดํฌํฌ์ธํธ์ ๋ํด์๋ ๋์ผํ ํ๋ก์ธ์ค๊ฐ ์ ์ฉ๋ฉ๋๋ค. ์๋ฅผ ๋ค์ด, ๋ค์๊ณผ ๊ฐ์ด ์์ํ TensorFlow ์ฒดํฌํฌ์ธํธ๋ฅผ ๋ด๋ณด๋
๋๋ค:
```bash
python -m transformers.onnx --model=keras-io/transformers-qa onnx/
```
๋ก์ปฌ์ ์ ์ฅ๋ ๋ชจ๋ธ์ ๋ด๋ณด๋ด๋ ค๋ฉด ๋ชจ๋ธ์ ๊ฐ์ค์น ํ์ผ๊ณผ ํ ํฌ๋์ด์ ํ์ผ์ ๋์ผํ ๋๋ ํ ๋ฆฌ์ ์ ์ฅํ ๋ค์, transformers.onnx ํจํค์ง์ --model ์ธ์๋ฅผ ์ํ๋ ๋๋ ํ ๋ฆฌ๋ก ์ง์ ํ์ฌ ONNX๋ก ๋ด๋ณด๋
๋๋ค:
```bash
python -m transformers.onnx --model=local-pt-checkpoint onnx/
``` | 0 |
hf_public_repos/transformers/docs/source | hf_public_repos/transformers/docs/source/ko/perf_train_cpu.md | <!--Copyright 2022 The HuggingFace Team. All rights reserved.
Licensed under the Apache License, Version 2.0 (the "License"); you may not use this file except in compliance with
the License. You may obtain a copy of the License at
http://www.apache.org/licenses/LICENSE-2.0
Unless required by applicable law or agreed to in writing, software distributed under the License is distributed on
an "AS IS" BASIS, WITHOUT WARRANTIES OR CONDITIONS OF ANY KIND, either express or implied. See the License for the
โ ๏ธ Note that this file is in Markdown but contain specific syntax for our doc-builder (similar to MDX) that may not be
rendered properly in your Markdown viewer.
-->
# CPU์์ ํจ์จ์ ์ธ ํ๋ จ [[efficient-training-on-cpu]]
์ด ๊ฐ์ด๋๋ CPU์์ ๋๊ท๋ชจ ๋ชจ๋ธ์ ํจ์จ์ ์ผ๋ก ํ๋ จํ๋ ๋ฐ ์ด์ ์ ๋ง์ถฅ๋๋ค.
## IPEX์ ํผํฉ ์ ๋ฐ๋ [[mixed-precision-with-ipex]]
IPEX๋ AVX-512 ์ด์์ ์ง์ํ๋ CPU์ ์ต์ ํ๋์ด ์์ผ๋ฉฐ, AVX2๋ง ์ง์ํ๋ CPU์๋ ๊ธฐ๋ฅ์ ์ผ๋ก ์๋ํฉ๋๋ค. ๋ฐ๋ผ์ AVX-512 ์ด์์ Intel CPU ์ธ๋์์๋ ์ฑ๋ฅ์ ์ด์ ์ด ์์ ๊ฒ์ผ๋ก ์์๋์ง๋ง, AVX2๋ง ์ง์ํ๋ CPU (์: AMD CPU ๋๋ ์ค๋๋ Intel CPU)์ ๊ฒฝ์ฐ์๋ IPEX ์๋์์ ๋ ๋์ ์ฑ๋ฅ์ ๋ณด์ผ ์ ์์ง๋ง ์ด๋ ๋ณด์ฅ๋์ง ์์ต๋๋ค. IPEX๋ Float32์ BFloat16๋ฅผ ๋ชจ๋ ์ฌ์ฉํ์ฌ CPU ํ๋ จ์ ์ํ ์ฑ๋ฅ ์ต์ ํ๋ฅผ ์ ๊ณตํฉ๋๋ค. BFloat16์ ์ฌ์ฉ์ ๋ค์ ์น์
์ ์ฃผ์ ์ด์ ์
๋๋ค.
์ ์ ๋ฐ๋ ๋ฐ์ดํฐ ํ์
์ธ BFloat16์ 3์ธ๋ Xeonยฎ Scalable ํ๋ก์ธ์ (์ฝ๋๋ช
: Cooper Lake)์์ AVX512 ๋ช
๋ น์ด ์งํฉ์ ๋ค์ดํฐ๋ธ๋ก ์ง์ํด ์์ผ๋ฉฐ, ๋ค์ ์ธ๋์ Intelยฎ Xeonยฎ Scalable ํ๋ก์ธ์์์ Intelยฎ Advanced Matrix Extensions (Intelยฎ AMX) ๋ช
๋ น์ด ์งํฉ์ ์ง์ํ์ฌ ์ฑ๋ฅ์ ํฌ๊ฒ ํฅ์์ํฌ ์์ ์
๋๋ค. CPU ๋ฐฑ์๋์ ์๋ ํผํฉ ์ ๋ฐ๋ ๊ธฐ๋ฅ์ PyTorch-1.10๋ถํฐ ํ์ฑํ๋์์ต๋๋ค. ๋์์, Intelยฎ Extension for PyTorch์์ BFloat16์ ๋ํ CPU์ ์๋ ํผํฉ ์ ๋ฐ๋ ๋ฐ ์ฐ์ฐ์์ BFloat16 ์ต์ ํ๋ฅผ ๋๊ท๋ชจ๋ก ํ์ฑํํ๊ณ , PyTorch ๋ง์คํฐ ๋ธ๋์น๋ก ๋ถ๋ถ์ ์ผ๋ก ์
์คํธ๋ฆผ์ ๋ฐ์ํ์ต๋๋ค. ์ฌ์ฉ์๋ค์ IPEX ์๋ ํผํฉ ์ ๋ฐ๋๋ฅผ ์ฌ์ฉํ์ฌ ๋ ๋์ ์ฑ๋ฅ๊ณผ ์ฌ์ฉ์ ๊ฒฝํ์ ์ป์ ์ ์์ต๋๋ค.
[์๋ ํผํฉ ์ ๋ฐ๋](https://intel.github.io/intel-extension-for-pytorch/cpu/latest/tutorials/features/amp.html)์ ๋ํ ์์ธํ ์ ๋ณด๋ฅผ ํ์ธํ์ญ์์ค.
### IPEX ์ค์น: [[ipex-installation]]
IPEX ๋ฆด๋ฆฌ์ค๋ PyTorch๋ฅผ ๋ฐ๋ผ๊ฐ๋๋ค. pip๋ฅผ ํตํด ์ค์นํ๋ ค๋ฉด:
| PyTorch Version | IPEX version |
| :---------------: | :----------: |
| 1.13 | 1.13.0+cpu |
| 1.12 | 1.12.300+cpu |
| 1.11 | 1.11.200+cpu |
| 1.10 | 1.10.100+cpu |
```
pip install intel_extension_for_pytorch==<version_name> -f https://developer.intel.com/ipex-whl-stable-cpu
```
[IPEX ์ค์น](https://intel.github.io/intel-extension-for-pytorch/cpu/latest/tutorials/installation.html)์ ๋ํ ๋ ๋ง์ ์ ๊ทผ ๋ฐฉ๋ฒ์ ํ์ธํ์ญ์์ค.
### Trainer์์์ ์ฌ์ฉ๋ฒ [[usage-in-trainer]]
Trainer์์ IPEX์ ์๋ ํผํฉ ์ ๋ฐ๋๋ฅผ ํ์ฑํํ๋ ค๋ฉด ์ฌ์ฉ์๋ ํ๋ จ ๋ช
๋ น ์ธ์์ `use_ipex`, `bf16`, `no_cuda`๋ฅผ ์ถ๊ฐํด์ผ ํฉ๋๋ค.
[Transformers ์ง๋ฌธ-์๋ต](https://github.com/huggingface/transformers/tree/main/examples/pytorch/question-answering)์ ์ฌ์ฉ ์ฌ๋ก๋ฅผ ์ดํด๋ณด๊ฒ ์ต๋๋ค.
- CPU์์ BF16 ์๋ ํผํฉ ์ ๋ฐ๋๋ฅผ ์ฌ์ฉํ์ฌ IPEX๋ก ํ๋ จํ๊ธฐ:
<pre> python run_qa.py \
--model_name_or_path bert-base-uncased \
--dataset_name squad \
--do_train \
--do_eval \
--per_device_train_batch_size 12 \
--learning_rate 3e-5 \
--num_train_epochs 2 \
--max_seq_length 384 \
--doc_stride 128 \
--output_dir /tmp/debug_squad/ \
<b>--use_ipex \</b>
<b>--bf16 --no_cuda</b></pre>
### ์ค์ต ์์ [[practice-example]]
๋ธ๋ก๊ทธ: [Intel Sapphire Rapids๋ก PyTorch Transformers ๊ฐ์ํ](https://huggingface.co/blog/intel-sapphire-rapids) | 0 |
hf_public_repos/transformers/docs/source | hf_public_repos/transformers/docs/source/ko/bertology.md | <!--Copyright 2020 The HuggingFace Team. All rights reserved.
Licensed under the Apache License, Version 2.0 (the "License"); you may not use this file except in compliance with
the License. You may obtain a copy of the License at
http://www.apache.org/licenses/LICENSE-2.0
Unless required by applicable law or agreed to in writing, software distributed under the License is distributed on
an "AS IS" BASIS, WITHOUT WARRANTIES OR CONDITIONS OF ANY KIND, either express or implied. See the License for the
specific language governing permissions and limitations under the License.
โ ๏ธ Note that this file is in Markdown but contain specific syntax for our doc-builder (similar to MDX) that may not be
rendered properly in your Markdown viewer.
-->
# BERTology
BERT์ ๊ฐ์ ๋๊ท๋ชจ ํธ๋์คํฌ๋จธ์ ๋ด๋ถ ๋์์ ์กฐ์ฌํ๋ ์ฐ๊ตฌ ๋ถ์ผ๊ฐ ์ ์ ๋ ์ค์ํด์ง๊ณ ์์ต๋๋ค.
ํน์๋ "BERTology"๋ผ ์นญํ๊ธฐ๋ ํฉ๋๋ค. ์ด ๋ถ์ผ์ ์ข์ ์์๋ ๋ค์๊ณผ ๊ฐ์ต๋๋ค:
- BERT๋ ๊ณ ์ ์ ์ธ NLP ํ์ดํ๋ผ์ธ์ ์ฌ๋ฐ๊ฒฌ - Ian Tenney, Dipanjan Das, Ellie Pavlick:
https://arxiv.org/abs/1905.05950
- 16๊ฐ์ ํค๋๊ฐ ์ ๋ง๋ก 1๊ฐ๋ณด๋ค ๋์๊ฐ? - Paul Michel, Omer Levy, Graham Neubig:
https://arxiv.org/abs/1905.10650
- BERT๋ ๋ฌด์์ ๋ณด๋๊ฐ? BERT์ ์ดํ
์
๋ถ์ - Kevin Clark, Urvashi Khandelwal, Omer Levy, Christopher D. Manning:
https://arxiv.org/abs/1906.04341
- CAT-probing: ํ๋ก๊ทธ๋๋ฐ ์ธ์ด์ ๋ํด ์ฌ์ ํ๋ จ๋ ๋ชจ๋ธ์ด ์ด๋ป๊ฒ ์ฝ๋ ๊ตฌ์กฐ๋ฅผ ๋ณด๋์ง ์์๋ณด๊ธฐ ์ํ ๋ฉํธ๋ฆญ ๊ธฐ๋ฐ ์ ๊ทผ ๋ฐฉ๋ฒ:
https://arxiv.org/abs/2210.04633
์ฐ๋ฆฌ๋ ์ด ์๋ก์ด ์ฐ๊ตฌ ๋ถ์ผ์ ๋ฐ์ ์ ๋๊ธฐ ์ํด, BERT/GPT/GPT-2 ๋ชจ๋ธ์ ๋ด๋ถ ํํ์ ์ดํด๋ณผ ์ ์๋ ๋ช ๊ฐ์ง ๊ธฐ๋ฅ์ ์ถ๊ฐํ์ต๋๋ค.
์ด ๊ธฐ๋ฅ๋ค์ ์ฃผ๋ก Paul Michel์ ํ๋ฅญํ ์์
์ ์ฐธ๊ณ ํ์ฌ ๊ฐ๋ฐ๋์์ต๋๋ค
(https://arxiv.org/abs/1905.10650):
- BERT/GPT/GPT-2์ ๋ชจ๋ ์๋ ์ํ์ ์ ๊ทผํ๊ธฐ,
- BERT/GPT/GPT-2์ ๊ฐ ํค๋์ ๋ชจ๋ ์ดํ
์
๊ฐ์ค์น์ ์ ๊ทผํ๊ธฐ,
- ํค๋์ ์ถ๋ ฅ ๊ฐ๊ณผ ๊ทธ๋๋์ธํธ๋ฅผ ๊ฒ์ํ์ฌ ํค๋ ์ค์๋ ์ ์๋ฅผ ๊ณ์ฐํ๊ณ https://arxiv.org/abs/1905.10650์์ ์ค๋ช
๋ ๋๋ก ํค๋๋ฅผ ์ ๊ฑฐํ๋ ๊ธฐ๋ฅ์ ์ ๊ณตํฉ๋๋ค.
์ด๋ฌํ ๊ธฐ๋ฅ๋ค์ ์ดํดํ๊ณ ์ง์ ์ฌ์ฉํด๋ณผ ์ ์๋๋ก [bertology.py](https://github.com/huggingface/transformers/tree/main/examples/research_projects/bertology/run_bertology.py) ์์ ์คํฌ๋ฆฝํธ๋ฅผ ์ถ๊ฐํ์ต๋๋ค. ์ด ์์ ์คํฌ๋ฆฝํธ์์๋ GLUE์ ๋ํด ์ฌ์ ํ๋ จ๋ ๋ชจ๋ธ์์ ์ ๋ณด๋ฅผ ์ถ์ถํ๊ณ ๋ชจ๋ธ์ ๊ฐ์ง์น๊ธฐ(prune)ํด๋ด
๋๋ค.
| 0 |
hf_public_repos/transformers/docs/source | hf_public_repos/transformers/docs/source/ko/create_a_model.md | <!--Copyright 2022 The HuggingFace Team. All rights reserved.
Licensed under the Apache License, Version 2.0 (the "License"); you may not use this file except in compliance with
the License. You may obtain a copy of the License at
http://www.apache.org/licenses/LICENSE-2.0
Unless required by applicable law or agreed to in writing, software distributed under the License is distributed on
an "AS IS" BASIS, WITHOUT WARRANTIES OR CONDITIONS OF ANY KIND, either express or implied. See the License for the
specific language governing permissions and limitations under the License.
โ ๏ธ Note that this file is in Markdown but contain specific syntax for our doc-builder (similar to MDX) that may not be
rendered properly in your Markdown viewer.
-->
# ๋ง์ถคํ ์ํคํ
์ฒ ๋ง๋ค๊ธฐ[[create-a-custom-architecture]]
[`AutoClass`](model_doc/auto)๋ ๋ชจ๋ธ ์ํคํ
์ฒ๋ฅผ ์๋์ผ๋ก ์ถ๋ก ํ๊ณ ๋ฏธ๋ฆฌ ํ์ต๋ configuration๊ณผ ๊ฐ์ค์น๋ฅผ ๋ค์ด๋ก๋ํฉ๋๋ค. ์ผ๋ฐ์ ์ผ๋ก ์ฒดํฌํฌ์ธํธ์ ๊ตฌ์ ๋ฐ์ง ์๋ ์ฝ๋๋ฅผ ์์ฑํ๋ ค๋ฉด `AutoClass`๋ฅผ ์ฌ์ฉํ๋ ๊ฒ์ด ์ข์ต๋๋ค. ํ์ง๋ง ํน์ ๋ชจ๋ธ ํ๋ผ๋ฏธํฐ๋ฅผ ๋ณด๋ค ์ธ๋ฐํ๊ฒ ์ ์ดํ๊ณ ์ ํ๋ ์ฌ์ฉ์๋ ๋ช ๊ฐ์ง ๊ธฐ๋ณธ ํด๋์ค๋ง์ผ๋ก ์ปค์คํ
๐ค Transformers ๋ชจ๋ธ์ ์์ฑํ ์ ์์ต๋๋ค. ์ด๋ ๐ค Transformers ๋ชจ๋ธ์ ์ฐ๊ตฌ, ๊ต์ก ๋๋ ์คํํ๋ ๋ฐ ๊ด์ฌ์ด ์๋ ๋ชจ๋ ์ฌ์ฉ์์๊ฒ ํนํ ์ ์ฉํ ์ ์์ต๋๋ค. ์ด ๊ฐ์ด๋์์๋ 'AutoClass'๋ฅผ ์ฌ์ฉํ์ง ์๊ณ ์ปค์คํ
๋ชจ๋ธ์ ๋ง๋๋ ๋ฐฉ๋ฒ์ ๋ํด ์์๋ณด๊ฒ ์ต๋๋ค:
- ๋ชจ๋ธ configuration์ ๊ฐ์ ธ์ค๊ณ ์ฌ์ฉ์ ์ง์ ํฉ๋๋ค.
- ๋ชจ๋ธ ์ํคํ
์ฒ๋ฅผ ์์ฑํฉ๋๋ค.
- ํ
์คํธ์ ์ฌ์ฉํ ๋๋ฆฌ๊ฑฐ๋ ๋น ๋ฅธ ํ ํฐํ๊ธฐ๋ฅผ ๋ง๋ญ๋๋ค.
- ๋น์ ์์
์ ์ํ ์ด๋ฏธ์ง ํ๋ก์ธ์๋ฅผ ์์ฑํฉ๋๋ค.
- ์ค๋์ค ์์
์ ์ํ ํน์ฑ ์ถ์ถ๊ธฐ๋ฅผ ์์ฑํฉ๋๋ค.
- ๋ฉํฐ๋ชจ๋ฌ ์์
์ฉ ํ๋ก์ธ์๋ฅผ ์์ฑํฉ๋๋ค.
## Configuration[[configuration]]
[configuration](main_classes/configuration)์ ๋ชจ๋ธ์ ํน์ ์์ฑ์ ๋ํ๋
๋๋ค. ๊ฐ ๋ชจ๋ธ ๊ตฌ์ฑ์๋ ์๋ก ๋ค๋ฅธ ์์ฑ์ด ์์ต๋๋ค. ์๋ฅผ ๋ค์ด, ๋ชจ๋ NLP ๋ชจ๋ธ์๋ `hidden_size`, `num_attention_heads`, `num_hidden_layers` ๋ฐ `vocab_size` ์์ฑ์ด ๊ณตํต์ผ๋ก ์์ต๋๋ค. ์ด๋ฌํ ์์ฑ์ ๋ชจ๋ธ์ ๊ตฌ์ฑํ attention heads ๋๋ hidden layers์ ์๋ฅผ ์ง์ ํฉ๋๋ค.
[DistilBERT](model_doc/distilbert) ์์ฑ์ ๊ฒ์ฌํ๊ธฐ ์ํด [`DistilBertConfig`]์ ์ ๊ทผํ์ฌ ์์ธํ ์ดํด๋ด
๋๋ค:
```py
>>> from transformers import DistilBertConfig
>>> config = DistilBertConfig()
>>> print(config)
DistilBertConfig {
"activation": "gelu",
"attention_dropout": 0.1,
"dim": 768,
"dropout": 0.1,
"hidden_dim": 3072,
"initializer_range": 0.02,
"max_position_embeddings": 512,
"model_type": "distilbert",
"n_heads": 12,
"n_layers": 6,
"pad_token_id": 0,
"qa_dropout": 0.1,
"seq_classif_dropout": 0.2,
"sinusoidal_pos_embds": false,
"transformers_version": "4.16.2",
"vocab_size": 30522
}
```
[`DistilBertConfig`]๋ ๊ธฐ๋ณธ [`DistilBertModel`]์ ๋น๋ํ๋ ๋ฐ ์ฌ์ฉ๋๋ ๋ชจ๋ ๊ธฐ๋ณธ ์์ฑ์ ํ์ํฉ๋๋ค. ๋ชจ๋ ์์ฑ์ ์ปค์คํฐ๋ง์ด์ง์ด ๊ฐ๋ฅํ๋ฏ๋ก ์คํ์ ์ํ ๊ณต๊ฐ์ ๋ง๋ค ์ ์์ต๋๋ค. ์๋ฅผ ๋ค์ด ๊ธฐ๋ณธ ๋ชจ๋ธ์ ๋ค์๊ณผ ๊ฐ์ด ์ปค์คํฐ๋ง์ด์ฆํ ์ ์์ต๋๋ค:
- `activation` ํ๋ผ๋ฏธํฐ๋ก ๋ค๋ฅธ ํ์ฑํ ํจ์๋ฅผ ์ฌ์ฉํด ๋ณด์ธ์.
- `attention_dropout` ํ๋ผ๋ฏธํฐ๋ฅผ ์ฌ์ฉํ์ฌ ์ดํ
์
ํ๋ฅ ์ ๋ ๋์ ๋๋กญ์์ ๋น์จ์ ์ฌ์ฉํ์ธ์.
```py
>>> my_config = DistilBertConfig(activation="relu", attention_dropout=0.4)
>>> print(my_config)
DistilBertConfig {
"activation": "relu",
"attention_dropout": 0.4,
"dim": 768,
"dropout": 0.1,
"hidden_dim": 3072,
"initializer_range": 0.02,
"max_position_embeddings": 512,
"model_type": "distilbert",
"n_heads": 12,
"n_layers": 6,
"pad_token_id": 0,
"qa_dropout": 0.1,
"seq_classif_dropout": 0.2,
"sinusoidal_pos_embds": false,
"transformers_version": "4.16.2",
"vocab_size": 30522
}
```
์ฌ์ ํ์ต๋ ๋ชจ๋ธ ์์ฑ์ [`~PretrainedConfig.from_pretrained`] ํจ์์์ ์์ ํ ์ ์์ต๋๋ค:
```py
>>> my_config = DistilBertConfig.from_pretrained("distilbert-base-uncased", activation="relu", attention_dropout=0.4)
```
๋ชจ๋ธ ๊ตฌ์ฑ์ด ๋ง์กฑ์ค๋ฌ์ฐ๋ฉด [`~PretrainedConfig.save_pretrained`]๋ก ์ ์ฅํ ์ ์์ต๋๋ค. ์ค์ ํ์ผ์ ์ง์ ๋ ์์
๊ฒฝ๋ก์ JSON ํ์ผ๋ก ์ ์ฅ๋ฉ๋๋ค:
```py
>>> my_config.save_pretrained(save_directory="./your_model_save_path")
```
configuration ํ์ผ์ ์ฌ์ฌ์ฉํ๋ ค๋ฉด [`~PretrainedConfig.from_pretrained`]๋ฅผ ์ฌ์ฉํ์ฌ ๊ฐ์ ธ์ค์ธ์:
```py
>>> my_config = DistilBertConfig.from_pretrained("./your_model_save_path/config.json")
```
<Tip>
configuration ํ์ผ์ ๋์
๋๋ฆฌ๋ก ์ ์ฅํ๊ฑฐ๋ ์ฌ์ฉ์ ์ ์ configuration ์์ฑ๊ณผ ๊ธฐ๋ณธ configuration ์์ฑ์ ์ฐจ์ด์ ๋ง ์ ์ฅํ ์๋ ์์ต๋๋ค! ์์ธํ ๋ด์ฉ์ [configuration](main_classes/configuration) ๋ฌธ์๋ฅผ ์ฐธ์กฐํ์ธ์.
</Tip>
## ๋ชจ๋ธ[[model]]
๋ค์ ๋จ๊ณ๋ [๋ชจ๋ธ(model)](main_classes/models)์ ๋ง๋๋ ๊ฒ์
๋๋ค. ๋์จํ๊ฒ ์ํคํ
์ฒ๋ผ๊ณ ๋ ๋ถ๋ฆฌ๋ ๋ชจ๋ธ์ ๊ฐ ๊ณ์ธต์ด ์ํํ๋ ๋์๊ณผ ๋ฐ์ํ๋ ์์
์ ์ ์ํฉ๋๋ค. configuration์ `num_hidden_layers`์ ๊ฐ์ ์์ฑ์ ์ํคํ
์ฒ๋ฅผ ์ ์ํ๋ ๋ฐ ์ฌ์ฉ๋ฉ๋๋ค. ๋ชจ๋ ๋ชจ๋ธ์ ๊ธฐ๋ณธ ํด๋์ค [`PreTrainedModel`]๊ณผ ์
๋ ฅ ์๋ฒ ๋ฉ ํฌ๊ธฐ ์กฐ์ ๋ฐ ์
ํ ์ดํ
์
ํค๋ ๊ฐ์ง ์น๊ธฐ์ ๊ฐ์ ๋ช ๊ฐ์ง ์ผ๋ฐ์ ์ธ ๋ฉ์๋๋ฅผ ๊ณต์ ํฉ๋๋ค. ๋ํ ๋ชจ๋ ๋ชจ๋ธ์ [`torch.nn.Module`](https://pytorch.org/docs/stable/generated/torch.nn.Module.html), [`tf.keras.Model`](https://www.tensorflow.org/api_docs/python/tf/keras/Model) ๋๋ [`flax.linen.Module`](https://flax.readthedocs.io/en/latest/flax.linen.html#module)์ ์๋ธํด๋์ค์ด๊ธฐ๋ ํฉ๋๋ค. ์ฆ, ๋ชจ๋ธ์ ๊ฐ ํ๋ ์์ํฌ์ ์ฌ์ฉ๋ฒ๊ณผ ํธํ๋ฉ๋๋ค.
<frameworkcontent>
<pt>
์ฌ์ฉ์ ์ง์ configuration ์์ฑ์ ๋ชจ๋ธ์ ๊ฐ์ ธ์ต๋๋ค:
```py
>>> from transformers import DistilBertModel
>>> my_config = DistilBertConfig.from_pretrained("./your_model_save_path/config.json")
>>> model = DistilBertModel(my_config)
```
์ด์ ์ฌ์ ํ์ต๋ ๊ฐ์ค์น ๋์ ์์์ ๊ฐ์ ๊ฐ์ง ๋ชจ๋ธ์ด ์์ฑ๋ฉ๋๋ค. ์ด ๋ชจ๋ธ์ ํ๋ จํ๊ธฐ ์ ๊น์ง๋ ์ ์ฉํ๊ฒ ์ฌ์ฉํ ์ ์์ต๋๋ค. ํ๋ จ์ ๋น์ฉ๊ณผ ์๊ฐ์ด ๋ง์ด ์์๋๋ ํ๋ก์ธ์ค์
๋๋ค. ์ผ๋ฐ์ ์ผ๋ก ํ๋ จ์ ํ์ํ ๋ฆฌ์์ค์ ์ผ๋ถ๋ง ์ฌ์ฉํ๋ฉด์ ๋ ๋์ ๊ฒฐ๊ณผ๋ฅผ ๋ ๋นจ๋ฆฌ ์ป์ผ๋ ค๋ฉด ์ฌ์ ํ๋ จ๋ ๋ชจ๋ธ์ ์ฌ์ฉํ๋ ๊ฒ์ด ์ข์ต๋๋ค.
์ฌ์ ํ์ต๋ ๋ชจ๋ธ์ [`~PreTrainedModel.from_pretrained`]๋ก ์์ฑํฉ๋๋ค:
```py
>>> model = DistilBertModel.from_pretrained("distilbert-base-uncased")
```
๐ค Transformers์์ ์ ๊ณตํ ๋ชจ๋ธ์ ์ฌ์ ํ์ต๋ ๊ฐ์ค์น๋ฅผ ์ฌ์ฉํ๋ ๊ฒฝ์ฐ ๊ธฐ๋ณธ ๋ชจ๋ธ configuration์ ์๋์ผ๋ก ๋ถ๋ฌ์ต๋๋ค. ๊ทธ๋ฌ๋ ์ํ๋ ๊ฒฝ์ฐ ๊ธฐ๋ณธ ๋ชจ๋ธ configuration ์์ฑ์ ์ผ๋ถ ๋๋ ์ ๋ถ๋ฅผ ์ฌ์ฉ์ ์ง์ ์ผ๋ก ๋ฐ๊ฟ ์ ์์ต๋๋ค:
```py
>>> model = DistilBertModel.from_pretrained("distilbert-base-uncased", config=my_config)
```
</pt>
<tf>
์ฌ์ฉ์ ์ง์ configuration ์์ฑ์ ๋ชจ๋ธ์ ๋ถ๋ฌ์ต๋๋ค:
```py
>>> from transformers import TFDistilBertModel
>>> my_config = DistilBertConfig.from_pretrained("./your_model_save_path/my_config.json")
>>> tf_model = TFDistilBertModel(my_config)
```
์ด์ ์ฌ์ ํ์ต๋ ๊ฐ์ค์น ๋์ ์์์ ๊ฐ์ ๊ฐ์ง ๋ชจ๋ธ์ด ์์ฑ๋ฉ๋๋ค. ์ด ๋ชจ๋ธ์ ํ๋ จํ๊ธฐ ์ ๊น์ง๋ ์ ์ฉํ๊ฒ ์ฌ์ฉํ ์ ์์ต๋๋ค. ํ๋ จ์ ๋น์ฉ๊ณผ ์๊ฐ์ด ๋ง์ด ์์๋๋ ํ๋ก์ธ์ค์
๋๋ค. ์ผ๋ฐ์ ์ผ๋ก ํ๋ จ์ ํ์ํ ๋ฆฌ์์ค์ ์ผ๋ถ๋ง ์ฌ์ฉํ๋ฉด์ ๋ ๋์ ๊ฒฐ๊ณผ๋ฅผ ๋ ๋นจ๋ฆฌ ์ป์ผ๋ ค๋ฉด ์ฌ์ ํ๋ จ๋ ๋ชจ๋ธ์ ์ฌ์ฉํ๋ ๊ฒ์ด ์ข์ต๋๋ค.
์ฌ์ ํ์ต๋ ๋ชจ๋ธ์ [`~TFPreTrainedModel.from_pretrained`]๋ก ์์ฑํฉ๋๋ค:
```py
>>> tf_model = TFDistilBertModel.from_pretrained("distilbert-base-uncased")
```
๐ค Transformers์์ ์ ๊ณตํ ๋ชจ๋ธ์ ์ฌ์ ํ์ต๋ ๊ฐ์ค์น๋ฅผ ์ฌ์ฉํ๋ ๊ฒฝ์ฐ ๊ธฐ๋ณธ ๋ชจ๋ธ configuration์ ์๋์ผ๋ก ๋ถ๋ฌ์ต๋๋ค. ๊ทธ๋ฌ๋ ์ํ๋ ๊ฒฝ์ฐ ๊ธฐ๋ณธ ๋ชจ๋ธ configuration ์์ฑ์ ์ผ๋ถ ๋๋ ์ ๋ถ๋ฅผ ์ฌ์ฉ์ ์ง์ ์ผ๋ก ๋ฐ๊ฟ ์ ์์ต๋๋ค:
```py
>>> tf_model = TFDistilBertModel.from_pretrained("distilbert-base-uncased", config=my_config)
```
</tf>
</frameworkcontent>
### ๋ชจ๋ธ ํค๋[[model-heads]]
์ด ์์ ์์ *์๋ ์ํ(hidden state)*๋ฅผ ์ถ๋ ฅํ๋ ๊ธฐ๋ณธ DistilBERT ๋ชจ๋ธ์ ๊ฐ๊ฒ ๋ฉ๋๋ค. ์๋ ์ํ๋ ์ต์ข
์ถ๋ ฅ์ ์์ฑํ๊ธฐ ์ํด ๋ชจ๋ธ ํค๋์ ์
๋ ฅ์ผ๋ก ์ ๋ฌ๋ฉ๋๋ค. ๐ค Transformers๋ ๋ชจ๋ธ์ด ํด๋น ์์
์ ์ง์ํ๋ ํ ๊ฐ ์์
๋ง๋ค ๋ค๋ฅธ ๋ชจ๋ธ ํค๋๋ฅผ ์ ๊ณตํฉ๋๋ค(์ฆ, ๋ฒ์ญ๊ณผ ๊ฐ์ ์ํ์ค ๊ฐ ์์
์๋ DistilBERT๋ฅผ ์ฌ์ฉํ ์ ์์).
<frameworkcontent>
<pt>
์๋ฅผ ๋ค์ด, [`DistilBertForSequenceClassification`]์ ์ํ์ค ๋ถ๋ฅ ํค๋๊ฐ ์๋ ๊ธฐ๋ณธ DistilBERT ๋ชจ๋ธ์
๋๋ค. ์ํ์ค ๋ถ๋ฅ ํค๋๋ ํ๋ง๋ ์ถ๋ ฅ ์์ ์๋ ์ ํ ๋ ์ด์ด์
๋๋ค.
```py
>>> from transformers import DistilBertForSequenceClassification
>>> model = DistilBertForSequenceClassification.from_pretrained("distilbert-base-uncased")
```
๋ค๋ฅธ ๋ชจ๋ธ ํค๋๋ก ์ ํํ์ฌ ์ด ์ฒดํฌํฌ์ธํธ๋ฅผ ๋ค๋ฅธ ์์
์ ์ฝ๊ฒ ์ฌ์ฌ์ฉํ ์ ์์ต๋๋ค. ์ง์์๋ต ์์
์ ๊ฒฝ์ฐ, [`DistilBertForQuestionAnswering`] ๋ชจ๋ธ ํค๋๋ฅผ ์ฌ์ฉํ ์ ์์ต๋๋ค. ์ง์์๋ต ํค๋๋ ์จ๊ฒจ์ง ์ํ ์ถ๋ ฅ ์์ ์ ํ ๋ ์ด์ด๊ฐ ์๋ค๋ ์ ์ ์ ์ธํ๋ฉด ์ํ์ค ๋ถ๋ฅ ํค๋์ ์ ์ฌํฉ๋๋ค.
```py
>>> from transformers import DistilBertForQuestionAnswering
>>> model = DistilBertForQuestionAnswering.from_pretrained("distilbert-base-uncased")
```
</pt>
<tf>
์๋ฅผ ๋ค์ด, [`TFDistilBertForSequenceClassification`]์ ์ํ์ค ๋ถ๋ฅ ํค๋๊ฐ ์๋ ๊ธฐ๋ณธ DistilBERT ๋ชจ๋ธ์
๋๋ค. ์ํ์ค ๋ถ๋ฅ ํค๋๋ ํ๋ง๋ ์ถ๋ ฅ ์์ ์๋ ์ ํ ๋ ์ด์ด์
๋๋ค.
```py
>>> from transformers import TFDistilBertForSequenceClassification
>>> tf_model = TFDistilBertForSequenceClassification.from_pretrained("distilbert-base-uncased")
```
๋ค๋ฅธ ๋ชจ๋ธ ํค๋๋ก ์ ํํ์ฌ ์ด ์ฒดํฌํฌ์ธํธ๋ฅผ ๋ค๋ฅธ ์์
์ ์ฝ๊ฒ ์ฌ์ฌ์ฉํ ์ ์์ต๋๋ค. ์ง์์๋ต ์์
์ ๊ฒฝ์ฐ, [`TFDistilBertForQuestionAnswering`] ๋ชจ๋ธ ํค๋๋ฅผ ์ฌ์ฉํ ์ ์์ต๋๋ค. ์ง์์๋ต ํค๋๋ ์จ๊ฒจ์ง ์ํ ์ถ๋ ฅ ์์ ์ ํ ๋ ์ด์ด๊ฐ ์๋ค๋ ์ ์ ์ ์ธํ๋ฉด ์ํ์ค ๋ถ๋ฅ ํค๋์ ์ ์ฌํฉ๋๋ค.
```py
>>> from transformers import TFDistilBertForQuestionAnswering
>>> tf_model = TFDistilBertForQuestionAnswering.from_pretrained("distilbert-base-uncased")
```
</tf>
</frameworkcontent>
## ํ ํฌ๋์ด์ [[tokenizer]]
ํ
์คํธ ๋ฐ์ดํฐ์ ๋ชจ๋ธ์ ์ฌ์ฉํ๊ธฐ ์ ์ ๋ง์ง๋ง์ผ๋ก ํ์ํ ๊ธฐ๋ณธ ํด๋์ค๋ ์์ ํ
์คํธ๋ฅผ ํ
์๋ก ๋ณํํ๋ [ํ ํฌ๋์ด์ ](main_classes/tokenizer)์
๋๋ค. ๐ค Transformers์ ์ฌ์ฉํ ์ ์๋ ํ ํฌ๋์ด์ ๋ ๋ ๊ฐ์ง ์ ํ์ด ์์ต๋๋ค:
- [`PreTrainedTokenizer`]: ํ์ด์ฌ์ผ๋ก ๊ตฌํ๋ ํ ํฌ๋์ด์ ์
๋๋ค.
- [`PreTrainedTokenizerFast`]: Rust ๊ธฐ๋ฐ [๐ค Tokenizer](https://huggingface.co/docs/tokenizers/python/latest/) ๋ผ์ด๋ธ๋ฌ๋ฆฌ๋ก ๋ง๋ค์ด์ง ํ ํฌ๋์ด์ ์
๋๋ค. ์ด ํ ํฌ๋์ด์ ๋ Rust๋ก ๊ตฌํ๋์ด ๋ฐฐ์น ํ ํฐํ์์ ํนํ ๋น ๋ฆ
๋๋ค. ๋น ๋ฅธ ํ ํฌ๋์ด์ ๋ ํ ํฐ์ ์๋ ๋จ์ด๋ ๋ฌธ์์ ๋งคํํ๋ *์คํ์
๋งคํ*๊ณผ ๊ฐ์ ์ถ๊ฐ ๋ฉ์๋๋ ์ ๊ณตํฉ๋๋ค.
๋ ํ ํฌ๋์ด์ ๋ชจ๋ ์ธ์ฝ๋ฉ ๋ฐ ๋์ฝ๋ฉ, ์ ํ ํฐ ์ถ๊ฐ, ํน์ ํ ํฐ ๊ด๋ฆฌ์ ๊ฐ์ ์ผ๋ฐ์ ์ธ ๋ฐฉ๋ฒ์ ์ง์ํฉ๋๋ค.
<Tip warning={true}>
๋ชจ๋ ๋ชจ๋ธ์ด ๋น ๋ฅธ ํ ํฌ๋์ด์ ๋ฅผ ์ง์ํ๋ ๊ฒ์ ์๋๋๋ค. ์ด [ํ](index#supported-frameworks)์์ ๋ชจ๋ธ์ ๋น ๋ฅธ ํ ํฌ๋์ด์ ์ง์ ์ฌ๋ถ๋ฅผ ํ์ธํ์ธ์.
</Tip>
ํ ํฌ๋์ด์ ๋ฅผ ์ง์ ํ์ตํ ๊ฒฝ์ฐ, *์ดํ(vocabulary)* ํ์ผ์์ ํ ํฌ๋์ด์ ๋ฅผ ๋ง๋ค ์ ์์ต๋๋ค:
```py
>>> from transformers import DistilBertTokenizer
>>> my_tokenizer = DistilBertTokenizer(vocab_file="my_vocab_file.txt", do_lower_case=False, padding_side="left")
```
์ฌ์ฉ์ ์ง์ ํ ํฌ๋์ด์ ์ ์ดํ๋ ์ฌ์ ํ์ต๋ ๋ชจ๋ธ์ ํ ํฌ๋์ด์ ์์ ์์ฑ๋ ์ดํ์ ๋ค๋ฅผ ์ ์๋ค๋ ์ ์ ๊ธฐ์ตํ๋ ๊ฒ์ด ์ค์ํฉ๋๋ค. ์ฌ์ ํ์ต๋ ๋ชจ๋ธ์ ์ฌ์ฉํ๋ ๊ฒฝ์ฐ ์ฌ์ ํ์ต๋ ๋ชจ๋ธ์ ์ดํ๋ฅผ ์ฌ์ฉํด์ผ ํ๋ฉฐ, ๊ทธ๋ ์ง ์์ผ๋ฉด ์
๋ ฅ์ด ์๋ฏธ๋ฅผ ๊ฐ์ง ๋ชปํฉ๋๋ค. [`DistilBertTokenizer`] ํด๋์ค๋ฅผ ์ฌ์ฉํ์ฌ ์ฌ์ ํ์ต๋ ๋ชจ๋ธ์ ์ดํ๋ก ํ ํฌ๋์ด์ ๋ฅผ ์์ฑํฉ๋๋ค:
```py
>>> from transformers import DistilBertTokenizer
>>> slow_tokenizer = DistilBertTokenizer.from_pretrained("distilbert-base-uncased")
```
[`DistilBertTokenizerFast`] ํด๋์ค๋ก ๋น ๋ฅธ ํ ํฌ๋์ด์ ๋ฅผ ์์ฑํฉ๋๋ค:
```py
>>> from transformers import DistilBertTokenizerFast
>>> fast_tokenizer = DistilBertTokenizerFast.from_pretrained("distilbert-base-uncased")
```
<Tip>
[`AutoTokenizer`]๋ ๊ธฐ๋ณธ์ ์ผ๋ก ๋น ๋ฅธ ํ ํฌ๋์ด์ ๋ฅผ ๊ฐ์ ธ์ค๋ ค๊ณ ํฉ๋๋ค. ์ด ๋์์ ๋นํ์ฑํํ๋ ค๋ฉด `from_pretrained`์์ `use_fast=False`๋ฅผ ์ค์ ํ๋ฉด ๋ฉ๋๋ค.
</Tip>
## ์ด๋ฏธ์ง ํ๋ก์ธ์[[image-processor]]
์ด๋ฏธ์ง ํ๋ก์ธ์(image processor)๋ ๋น์ ์
๋ ฅ์ ์ฒ๋ฆฌํฉ๋๋ค. ๊ธฐ๋ณธ [`~image_processing_utils.ImageProcessingMixin`] ํด๋์ค์์ ์์ํฉ๋๋ค.
์ฌ์ฉํ๋ ค๋ฉด ์ฌ์ฉ ์ค์ธ ๋ชจ๋ธ๊ณผ ์ฐ๊ฒฐ๋ ์ด๋ฏธ์ง ํ๋ก์ธ์๋ฅผ ์์ฑํฉ๋๋ค. ์๋ฅผ ๋ค์ด, ์ด๋ฏธ์ง ๋ถ๋ฅ์ [ViT](model_doc/vit)๋ฅผ ์ฌ์ฉํ๋ ๊ฒฝ์ฐ ๊ธฐ๋ณธ [`ViTImageProcessor`]๋ฅผ ์์ฑํฉ๋๋ค:
```py
>>> from transformers import ViTImageProcessor
>>> vit_extractor = ViTImageProcessor()
>>> print(vit_extractor)
ViTImageProcessor {
"do_normalize": true,
"do_resize": true,
"feature_extractor_type": "ViTImageProcessor",
"image_mean": [
0.5,
0.5,
0.5
],
"image_std": [
0.5,
0.5,
0.5
],
"resample": 2,
"size": 224
}
```
<Tip>
์ฌ์ฉ์ ์ง์ ์ ์ํ์ง ์๋ ๊ฒฝ์ฐ `from_pretrained` ๋ฉ์๋๋ฅผ ์ฌ์ฉํ์ฌ ๋ชจ๋ธ์ ๊ธฐ๋ณธ ์ด๋ฏธ์ง ํ๋ก์ธ์ ๋งค๊ฐ๋ณ์๋ฅผ ๋ถ๋ฌ์ค๋ฉด ๋ฉ๋๋ค.
</Tip>
์ฌ์ฉ์ ์ง์ ์ด๋ฏธ์ง ํ๋ก์ธ์๋ฅผ ์์ฑํ๋ ค๋ฉด [`ViTImageProcessor`] ํ๋ผ๋ฏธํฐ๋ฅผ ์์ ํฉ๋๋ค:
```py
>>> from transformers import ViTImageProcessor
>>> my_vit_extractor = ViTImageProcessor(resample="PIL.Image.BOX", do_normalize=False, image_mean=[0.3, 0.3, 0.3])
>>> print(my_vit_extractor)
ViTImageProcessor {
"do_normalize": false,
"do_resize": true,
"feature_extractor_type": "ViTImageProcessor",
"image_mean": [
0.3,
0.3,
0.3
],
"image_std": [
0.5,
0.5,
0.5
],
"resample": "PIL.Image.BOX",
"size": 224
}
```
## ํน์ฑ ์ถ์ถ๊ธฐ[[feature-extractor]]
ํน์ฑ ์ถ์ถ๊ธฐ(feature extractor)๋ ์ค๋์ค ์
๋ ฅ์ ์ฒ๋ฆฌํฉ๋๋ค. ๊ธฐ๋ณธ [`~feature_extraction_utils.FeatureExtractionMixin`] ํด๋์ค์์ ์์๋๋ฉฐ, ์ค๋์ค ์
๋ ฅ์ ์ฒ๋ฆฌํ๊ธฐ ์ํด [`SequenceFeatureExtractor`] ํด๋์ค์์ ์์ํ ์๋ ์์ต๋๋ค.
์ฌ์ฉํ๋ ค๋ฉด ์ฌ์ฉ ์ค์ธ ๋ชจ๋ธ๊ณผ ์ฐ๊ฒฐ๋ ํน์ฑ ์ถ์ถ๊ธฐ๋ฅผ ์์ฑํฉ๋๋ค. ์๋ฅผ ๋ค์ด, ์ค๋์ค ๋ถ๋ฅ์ [Wav2Vec2](model_doc/wav2vec2)๋ฅผ ์ฌ์ฉํ๋ ๊ฒฝ์ฐ ๊ธฐ๋ณธ [`Wav2Vec2FeatureExtractor`]๋ฅผ ์์ฑํฉ๋๋ค:
```py
>>> from transformers import Wav2Vec2FeatureExtractor
>>> w2v2_extractor = Wav2Vec2FeatureExtractor()
>>> print(w2v2_extractor)
Wav2Vec2FeatureExtractor {
"do_normalize": true,
"feature_extractor_type": "Wav2Vec2FeatureExtractor",
"feature_size": 1,
"padding_side": "right",
"padding_value": 0.0,
"return_attention_mask": false,
"sampling_rate": 16000
}
```
<Tip>
์ฌ์ฉ์ ์ง์ ์ด ํ์ํ์ง ์์ ๊ฒฝ์ฐ `from_pretrained` ๋ฉ์๋๋ฅผ ์ฌ์ฉํ์ฌ ๋ชจ๋ธ์ ๊ธฐ๋ณธ ํน์ฑ ์ถ์ถ๊ธฐ ใ
๊ฐ๋ณ์๋ฅผ ๋ถ๋ฌ ์ค๋ฉด ๋ฉ๋๋ค.
</Tip>
์ฌ์ฉ์ ์ง์ ํน์ฑ ์ถ์ถ๊ธฐ๋ฅผ ๋ง๋ค๋ ค๋ฉด [`Wav2Vec2FeatureExtractor`] ๋งค๊ฐ๋ณ์๋ฅผ ์์ ํฉ๋๋ค:
```py
>>> from transformers import Wav2Vec2FeatureExtractor
>>> w2v2_extractor = Wav2Vec2FeatureExtractor(sampling_rate=8000, do_normalize=False)
>>> print(w2v2_extractor)
Wav2Vec2FeatureExtractor {
"do_normalize": false,
"feature_extractor_type": "Wav2Vec2FeatureExtractor",
"feature_size": 1,
"padding_side": "right",
"padding_value": 0.0,
"return_attention_mask": false,
"sampling_rate": 8000
}
```
## ํ๋ก์ธ์[[processor]]
๋ฉํฐ๋ชจ๋ฌ ์์
์ ์ง์ํ๋ ๋ชจ๋ธ์ ๊ฒฝ์ฐ, ๐ค Transformers๋ ํน์ฑ ์ถ์ถ๊ธฐ ๋ฐ ํ ํฌ๋์ด์ ์ ๊ฐ์ ์ฒ๋ฆฌ ํด๋์ค๋ฅผ ๋จ์ผ ๊ฐ์ฒด๋ก ํธ๋ฆฌํ๊ฒ ๋ํํ๋ ํ๋ก์ธ์ ํด๋์ค๋ฅผ ์ ๊ณตํฉ๋๋ค. ์๋ฅผ ๋ค์ด, ์๋ ์์ฑ ์ธ์ ์์
(Automatic Speech Recognition task (ASR))์ [`Wav2Vec2Processor`]๋ฅผ ์ฌ์ฉํ๋ค๊ณ ๊ฐ์ ํด ๋ณด๊ฒ ์ต๋๋ค. ์๋ ์์ฑ ์ธ์ ์์
์ ์ค๋์ค๋ฅผ ํ
์คํธ๋ก ๋ณํํ๋ฏ๋ก ํน์ฑ ์ถ์ถ๊ธฐ์ ํ ํฌ๋์ด์ ๊ฐ ํ์ํฉ๋๋ค.
์ค๋์ค ์
๋ ฅ์ ์ฒ๋ฆฌํ ํน์ฑ ์ถ์ถ๊ธฐ๋ฅผ ๋ง๋ญ๋๋ค:
```py
>>> from transformers import Wav2Vec2FeatureExtractor
>>> feature_extractor = Wav2Vec2FeatureExtractor(padding_value=1.0, do_normalize=True)
```
ํ
์คํธ ์
๋ ฅ์ ์ฒ๋ฆฌํ ํ ํฌ๋์ด์ ๋ฅผ ๋ง๋ญ๋๋ค:
```py
>>> from transformers import Wav2Vec2CTCTokenizer
>>> tokenizer = Wav2Vec2CTCTokenizer(vocab_file="my_vocab_file.txt")
```
[`Wav2Vec2Processor`]์์ ํน์ฑ ์ถ์ถ๊ธฐ์ ํ ํฌ๋์ด์ ๋ฅผ ๊ฒฐํฉํฉ๋๋ค:
```py
>>> from transformers import Wav2Vec2Processor
>>> processor = Wav2Vec2Processor(feature_extractor=feature_extractor, tokenizer=tokenizer)
```
configuration๊ณผ ๋ชจ๋ธ์ด๋ผ๋ ๋ ๊ฐ์ง ๊ธฐ๋ณธ ํด๋์ค์ ์ถ๊ฐ ์ ์ฒ๋ฆฌ ํด๋์ค(ํ ํฌ๋์ด์ , ์ด๋ฏธ์ง ํ๋ก์ธ์, ํน์ฑ ์ถ์ถ๊ธฐ ๋๋ ํ๋ก์ธ์)๋ฅผ ์ฌ์ฉํ๋ฉด ๐ค Transformers์์ ์ง์ํ๋ ๋ชจ๋ ๋ชจ๋ธ์ ๋ง๋ค ์ ์์ต๋๋ค. ์ด๋ฌํ ๊ฐ ๊ธฐ๋ณธ ํด๋์ค๋ ๊ตฌ์ฑ์ด ๊ฐ๋ฅํ๋ฏ๋ก ์ํ๋ ํน์ ์์ฑ์ ์ฌ์ฉํ ์ ์์ต๋๋ค. ํ์ต์ ์ํด ๋ชจ๋ธ์ ์ฝ๊ฒ ์ค์ ํ๊ฑฐ๋ ๊ธฐ์กด์ ์ฌ์ ํ์ต๋ ๋ชจ๋ธ์ ์์ ํ์ฌ ๋ฏธ์ธ ์กฐ์ ํ ์ ์์ต๋๋ค.
| 0 |
hf_public_repos/transformers/docs/source | hf_public_repos/transformers/docs/source/ko/custom_tools.md | <!--Copyright 2023 The HuggingFace Team. All rights reserved.
Licensed under the Apache License, Version 2.0 (the "License"); you may not use this file except in compliance with
the License. You may obtain a copy of the License at
http://www.apache.org/licenses/LICENSE-2.0
Unless required by applicable law or agreed to in writing, software distributed under the License is distributed on
an "AS IS" BASIS, WITHOUT WARRANTIES OR CONDITIONS OF ANY KIND, either express or implied. See the License for the
specific language governing permissions and limitations under the License.
-->
# ์ฌ์ฉ์ ์ ์ ๋๊ตฌ์ ํ๋กฌํํธ[[custom-tools-and-prompts]]
<Tip>
Transformers์ ๊ด๋ จํ์ฌ ์ด๋ค ๋๊ตฌ์ ์์ด์ ํธ๊ฐ ์๋์ง ์ ๋ชจ๋ฅด์ ๋ค๋ฉด [Transformers Agents](transformers_agents) ํ์ด์ง๋ฅผ ๋จผ์ ์ฝ์ด๋ณด์๊ธฐ ๋ฐ๋๋๋ค.
</Tip>
<Tip warning={true}>
Transformers Agent๋ ์คํ ์ค์ธ API๋ก ์ธ์ ๋ ์ง ๋ณ๊ฒฝ๋ ์ ์์ต๋๋ค.
API ๋๋ ๊ธฐ๋ฐ ๋ชจ๋ธ์ด ๋ณ๊ฒฝ๋๊ธฐ ์ฝ๊ธฐ ๋๋ฌธ์ ์์ด์ ํธ๊ฐ ๋ฐํํ๋ ๊ฒฐ๊ณผ๋ ๋ฌ๋ผ์ง ์ ์์ต๋๋ค.
</Tip>
์์ด์ ํธ์๊ฒ ๊ถํ์ ๋ถ์ฌํ๊ณ ์๋ก์ด ์์
์ ์ํํ๊ฒ ํ๋ ค๋ฉด ์ฌ์ฉ์ ์ ์ ๋๊ตฌ์ ํ๋กฌํํธ๋ฅผ ๋ง๋ค๊ณ ์ฌ์ฉํ๋ ๊ฒ์ด ๋ฌด์๋ณด๋ค ์ค์ํฉ๋๋ค.
์ด ๊ฐ์ด๋์์๋ ๋ค์๊ณผ ๊ฐ์ ๋ด์ฉ์ ์ดํด๋ณด๊ฒ ์ต๋๋ค:
- ํ๋กฌํํธ๋ฅผ ์ฌ์ฉ์ ์ ์ํ๋ ๋ฐฉ๋ฒ
- ์ฌ์ฉ์ ์ ์ ๋๊ตฌ๋ฅผ ์ฌ์ฉํ๋ ๋ฐฉ๋ฒ
- ์ฌ์ฉ์ ์ ์ ๋๊ตฌ๋ฅผ ๋ง๋๋ ๋ฐฉ๋ฒ
## ํ๋กฌํํธ๋ฅผ ์ฌ์ฉ์ ์ ์ํ๊ธฐ[[customizing-the-prompt]]
[Transformers Agents](transformers_agents)์์ ์ค๋ช
ํ ๊ฒ์ฒ๋ผ ์์ด์ ํธ๋ [`~Agent.run`] ๋ฐ [`~Agent.chat`] ๋ชจ๋์์ ์คํํ ์ ์์ต๋๋ค.
`run`(์คํ) ๋ชจ๋์ `chat`(์ฑํ
) ๋ชจ๋ ๋ชจ๋ ๋์ผํ ๋ก์ง์ ๊ธฐ๋ฐ์ผ๋ก ํฉ๋๋ค.
์์ด์ ํธ๋ฅผ ๊ตฌ๋ํ๋ ์ธ์ด ๋ชจ๋ธ์ ๊ธด ํ๋กฌํํธ์ ๋ฐ๋ผ ์กฐ๊ฑด์ด ์ง์ ๋๊ณ , ์ค์ง ํ ํฐ์ ๋๋ฌํ ๋๊น์ง ๋ค์ ํ ํฐ์ ์์ฑํ์ฌ ํ๋กฌํํธ๋ฅผ ์์ํฉ๋๋ค.
`chat` ๋ชจ๋์์๋ ํ๋กฌํํธ๊ฐ ์ด์ ์ฌ์ฉ์ ์
๋ ฅ ๋ฐ ๋ชจ๋ธ ์์ฑ์ผ๋ก ์ฐ์ฅ๋๋ค๋ ์ ์ด ๋ ๋ชจ๋์ ์ ์ผํ ์ฐจ์ด์ ์
๋๋ค.
์ด๋ฅผ ํตํด ์์ด์ ํธ๊ฐ ๊ณผ๊ฑฐ ์ํธ์์ฉ์ ์ ๊ทผํ ์ ์๊ฒ ๋๋ฏ๋ก ์์ด์ ํธ์๊ฒ ์ผ์ข
์ ๋ฉ๋ชจ๋ฆฌ๋ฅผ ์ ๊ณตํ๋ ์
์
๋๋ค.
### ํ๋กฌํํธ์ ๊ตฌ์กฐ[[structure-of-the-prompt]]
์ด๋ป๊ฒ ํ๋กฌํํธ ์ฌ์ฉ์ ์ ์๋ฅผ ์ ํ ์ ์๋์ง ์ดํดํ๊ธฐ ์ํด ํ๋กฌํํธ์ ๊ตฌ์กฐ๋ฅผ ์์ธํ ์ดํด๋ด
์๋ค.
ํ๋กฌํํธ๋ ํฌ๊ฒ ๋ค ๋ถ๋ถ์ผ๋ก ๊ตฌ์ฑ๋์ด ์์ต๋๋ค.
- 1. ๋์
: ์์ด์ ํธ๊ฐ ์ด๋ป๊ฒ ํ๋ํด์ผ ํ๋์ง, ๋๊ตฌ์ ๊ฐ๋
์ ๋ํ ์ค๋ช
.
- 2. ๋ชจ๋ ๋๊ตฌ์ ๋ํ ์ค๋ช
. ์ด๋ ๋ฐํ์์ ์ฌ์ฉ์๊ฐ ์ ์/์ ํํ ๋๊ตฌ๋ก ๋์ ์ผ๋ก ๋์ฒด๋๋ `<<all_tools>>` ํ ํฐ์ผ๋ก ์ ์๋ฉ๋๋ค.
- 3. ์์
์์ ๋ฐ ํด๋น ์๋ฃจ์
์ธํธ.
- 4. ํ์ฌ ์์ ๋ฐ ํด๊ฒฐ ์์ฒญ.
๊ฐ ๋ถ๋ถ์ ๋ ์ ์ดํดํ ์ ์๋๋ก ์งง์ ๋ฒ์ ์ ํตํด `run` ํ๋กฌํํธ๊ฐ ์ด๋ป๊ฒ ๋ณด์ด๋์ง ์ดํด๋ณด๊ฒ ์ต๋๋ค:
````text
I will ask you to perform a task, your job is to come up with a series of simple commands in Python that will perform the task.
[...]
You can print intermediate results if it makes sense to do so.
Tools:
- document_qa: This is a tool that answers a question about a document (pdf). It takes an input named `document` which should be the document containing the information, as well as a `question` that is the question about the document. It returns a text that contains the answer to the question.
- image_captioner: This is a tool that generates a description of an image. It takes an input named `image` which should be the image to the caption and returns a text that contains the description in English.
[...]
Task: "Answer the question in the variable `question` about the image stored in the variable `image`. The question is in French."
I will use the following tools: `translator` to translate the question into English and then `image_qa` to answer the question on the input image.
Answer:
```py
translated_question = translator(question=question, src_lang="French", tgt_lang="English")
print(f"The translated question is {translated_question}.")
answer = image_qa(image=image, question=translated_question)
print(f"The answer is {answer}")
```
Task: "Identify the oldest person in the `document` and create an image showcasing the result as a banner."
I will use the following tools: `document_qa` to find the oldest person in the document, then `image_generator` to generate an image according to the answer.
Answer:
```py
answer = document_qa(document, question="What is the oldest person?")
print(f"The answer is {answer}.")
image = image_generator("A banner showing " + answer)
```
[...]
Task: "Draw me a picture of rivers and lakes"
I will use the following
````
๋์
(*"๋๊ตฌ:"* ์์ ํ
์คํธ)์์๋ ๋ชจ๋ธ์ด ์ด๋ป๊ฒ ์๋ํ๊ณ ๋ฌด์์ ํด์ผ ํ๋์ง ์ ํํ๊ฒ ์ค๋ช
ํฉ๋๋ค.
์์ด์ ํธ๋ ํญ์ ๊ฐ์ ๋ฐฉ์์ผ๋ก ์๋ํด์ผ ํ๋ฏ๋ก ์ด ๋ถ๋ถ์ ์ฌ์ฉ์ ์ ์ํ ํ์๊ฐ ์์ ๊ฐ๋ฅ์ฑ์ด ๋์ต๋๋ค.
๋ ๋ฒ์งธ ๋ถ๋ถ(*"๋๊ตฌ"* ์๋์ ๊ธ๋จธ๋ฆฌ ๊ธฐํธ)์ `run` ๋๋ `chat`์ ํธ์ถํ ๋ ๋์ ์ผ๋ก ์ถ๊ฐ๋ฉ๋๋ค.
์ ํํ `agent.toolbox`์ ์๋ ๋๊ตฌ ์๋งํผ ๊ธ๋จธ๋ฆฌ ๊ธฐํธ๊ฐ ์๊ณ , ๊ฐ ๊ธ๋จธ๋ฆฌ ๊ธฐํธ๋ ๋๊ตฌ์ ์ด๋ฆ๊ณผ ์ค๋ช
์ผ๋ก ๊ตฌ์ฑ๋ฉ๋๋ค:
```text
- <tool.name>: <tool.description>
```
๋ฌธ์ ์ง์์๋ต ๋๊ตฌ๋ฅผ ๊ฐ์ ธ์ค๊ณ ์ด๋ฆ๊ณผ ์ค๋ช
์ ์ถ๋ ฅํด์ ๋น ๋ฅด๊ฒ ํ์ธํด ๋ณด๊ฒ ์ต๋๋ค.
```py
from transformers import load_tool
document_qa = load_tool("document-question-answering")
print(f"- {document_qa.name}: {document_qa.description}")
```
๊ทธ๋ฌ๋ฉด ๋ค์ ๊ฒฐ๊ณผ๊ฐ ์ถ๋ ฅ๋ฉ๋๋ค:
```text
- document_qa: This is a tool that answers a question about a document (pdf). It takes an input named `document` which should be the document containing the information, as well as a `question` that is the question about the document. It returns a text that contains the answer to the question.
```
์ฌ๊ธฐ์ ๋๊ตฌ ์ด๋ฆ์ด ์งง๊ณ ์ ํํ๋ค๋ ๊ฒ์ ์ ์ ์์ต๋๋ค.
์ค๋ช
์ ๋ ๋ถ๋ถ์ผ๋ก ๊ตฌ์ฑ๋์ด ์๋๋ฐ, ์ฒซ ๋ฒ์งธ ๋ถ๋ถ์์๋ ๋๊ตฌ์ ๊ธฐ๋ฅ์ ์ค๋ช
ํ๊ณ ๋ ๋ฒ์งธ ๋ถ๋ถ์์๋ ์์๋๋ ์
๋ ฅ ์ธ์์ ๋ฐํ ๊ฐ์ ๋ช
์ํฉ๋๋ค.
์์ด์ ํธ๊ฐ ๋๊ตฌ๋ฅผ ์ฌ๋ฐ๋ฅด๊ฒ ์ฌ์ฉํ๋ ค๋ฉด ์ข์ ๋๊ตฌ ์ด๋ฆ๊ณผ ๋๊ตฌ ์ค๋ช
์ด ๋งค์ฐ ์ค์ํฉ๋๋ค.
์์ด์ ํธ๊ฐ ๋๊ตฌ์ ๋ํด ์ ์ ์๋ ์ ์ผํ ์ ๋ณด๋ ์ด๋ฆ๊ณผ ์ค๋ช
๋ฟ์ด๋ฏ๋ก, ์ด ๋ ๊ฐ์ง๋ฅผ ์ ํํ๊ฒ ์์ฑํ๊ณ ๋๊ตฌ ์์์ ์๋ ๊ธฐ์กด ๋๊ตฌ์ ์คํ์ผ๊ณผ ์ผ์นํ๋์ง ํ์ธํด์ผ ํฉ๋๋ค.
ํนํ ์ด๋ฆ์ ๋ฐ๋ผ ์์๋๋ ๋ชจ๋ ์ธ์๊ฐ ์ค๋ช
์ ์ฝ๋ ์คํ์ผ๋ก ์ธ๊ธ๋์ด ์๋์ง, ์์๋๋ ์ ํ๊ณผ ๊ทธ ์ ํ์ด ๋ฌด์์ธ์ง์ ๋ํ ์ค๋ช
์ด ํฌํจ๋์ด ์๋์ง ํ์ธํ์ธ์.
<Tip>
๋๊ตฌ์ ์ด๋ค ์ด๋ฆ๊ณผ ์ค๋ช
์ด ์์ด์ผ ํ๋์ง ์ดํดํ๋ ค๋ฉด ์์ ๋ Transformers ๋๊ตฌ์ ์ด๋ฆ๊ณผ ์ค๋ช
์ ํ์ธํ์ธ์.
[`Agent.toolbox`] ์์ฑ์ ๊ฐ์ง ๋ชจ๋ ๋๊ตฌ๋ฅผ ๋ณผ ์ ์์ต๋๋ค.
</Tip>
์ธ ๋ฒ์งธ ๋ถ๋ถ์๋ ์์ด์ ํธ๊ฐ ์ด๋ค ์ข
๋ฅ์ ์ฌ์ฉ์ ์์ฒญ์ ๋ํด ์ด๋ค ์ฝ๋๋ฅผ ์์ฑํด์ผ ํ๋์ง ์ ํํ๊ฒ ๋ณด์ฌ์ฃผ๋ ์์ ๋ ์์ ์ธํธ๊ฐ ํฌํจ๋์ด ์์ต๋๋ค.
์์ด์ ํธ๋ฅผ ์ง์ํ๋ ๋๊ท๋ชจ ์ธ์ด ๋ชจ๋ธ์ ํ๋กฌํํธ์์ ํจํด์ ์ธ์ํ๊ณ ์๋ก์ด ๋ฐ์ดํฐ๋ก ํจํด์ ๋ฐ๋ณตํ๋ ๋ฐ ๋งค์ฐ ๋ฅ์ํฉ๋๋ค.
๋ฐ๋ผ์ ์์ด์ ํธ๊ฐ ์ค์ ๋ก ์ฌ๋ฐ๋ฅธ ์คํ ๊ฐ๋ฅํ ์ฝ๋๋ฅผ ์์ฑํ ๊ฐ๋ฅ์ฑ์ ๊ทน๋ํํ๋ ๋ฐฉ์์ผ๋ก ์์ ๋ฅผ ์์ฑํ๋ ๊ฒ์ด ๋งค์ฐ ์ค์ํฉ๋๋ค.
ํ ๊ฐ์ง ์๋ฅผ ์ดํด๋ณด๊ฒ ์ต๋๋ค:
````text
Task: "Identify the oldest person in the `document` and create an image showcasing the result as a banner."
I will use the following tools: `document_qa` to find the oldest person in the document, then `image_generator` to generate an image according to the answer.
Answer:
```py
answer = document_qa(document, question="What is the oldest person?")
print(f"The answer is {answer}.")
image = image_generator("A banner showing " + answer)
```
````
์์
์ค๋ช
, ์์ด์ ํธ๊ฐ ์ํํ๋ ค๋ ์์
์ ๋ํ ์ค๋ช
, ๋ง์ง๋ง์ผ๋ก ์์ฑ๋ ์ฝ๋, ์ด ์ธ ๋ถ๋ถ์ผ๋ก ๊ตฌ์ฑ๋ ํ๋กฌํํธ๋ ๋ชจ๋ธ์ ๋ฐ๋ณตํ์ฌ ์ ๊ณต๋ฉ๋๋ค.
ํ๋กฌํํธ์ ์ผ๋ถ์ธ ๋ชจ๋ ์์ ๋ ์ด๋ฌํ ์ ํํ ํจํด์ผ๋ก ๋์ด ์์ผ๋ฏ๋ก, ์์ด์ ํธ๊ฐ ์ ํ ํฐ์ ์์ฑํ ๋ ์ ํํ ๋์ผํ ํจํด์ ์ฌํํ ์ ์์ต๋๋ค.
ํ๋กฌํํธ ์์ ๋ Transformers ํ์ด ์ ๋ณํ๊ณ ์ผ๋ จ์ [problem statements](https://github.com/huggingface/transformers/blob/main/src/transformers/tools/evaluate_agent.py)์ ๋ฐ๋ผ ์๊ฒฉํ๊ฒ ํ๊ฐํ์ฌ
์์ด์ ํธ์ ํ๋กฌํํธ๊ฐ ์์ด์ ํธ์ ์ค์ ์ฌ์ฉ ์ฌ๋ก๋ฅผ ์ต๋ํ ์ ํด๊ฒฐํ ์ ์๋๋ก ๋ณด์ฅํฉ๋๋ค.
ํ๋กฌํํธ์ ๋ง์ง๋ง ๋ถ๋ถ์ ๋ค์์ ํด๋นํฉ๋๋ค:
```text
Task: "Draw me a picture of rivers and lakes"
I will use the following
```
์ด๋ ์์ด์ ํธ๊ฐ ์๋ฃํด์ผ ํ ์ต์ข
์ ์ธ ๋ฏธ์์ฑ ์์ ์
๋๋ค. ๋ฏธ์์ฑ ์์ ๋ ์ค์ ์ฌ์ฉ์ ์
๋ ฅ์ ๋ฐ๋ผ ๋์ ์ผ๋ก ๋ง๋ค์ด์ง๋๋ค.
์ ์์์ ๊ฒฝ์ฐ ์ฌ์ฉ์๊ฐ ๋ค์๊ณผ ๊ฐ์ด ์คํํ์ต๋๋ค:
```py
agent.run("Draw me a picture of rivers and lakes")
```
์ฌ์ฉ์ ์
๋ ฅ - *์ฆ* Task: *"Draw me a picture of rivers and lakes"*๊ฐ ํ๋กฌํํธ ํ
ํ๋ฆฟ์ ๋ง์ถฐ "Task: <task> \n\n I will use the following"๋ก ์บ์คํ
๋ฉ๋๋ค.
์ด ๋ฌธ์ฅ์ ์์ด์ ํธ์๊ฒ ์กฐ๊ฑด์ด ์ ์ฉ๋๋ ํ๋กฌํํธ์ ๋ง์ง๋ง ์ค์ ๊ตฌ์ฑํ๋ฏ๋ก ์์ด์ ํธ๊ฐ ์ด์ ์์ ์์ ์ํํ ๊ฒ๊ณผ ์ ํํ ๋์ผํ ๋ฐฉ์์ผ๋ก ์์ ๋ฅผ ์๋ฃํ๋๋ก ๊ฐ๋ ฅํ๊ฒ ์ํฅ์ ๋ฏธ์นฉ๋๋ค.
๋๋ฌด ์์ธํ ์ค๋ช
ํ์ง ์๋๋ผ๋ ์ฑํ
ํ
ํ๋ฆฟ์ ํ๋กฌํํธ ๊ตฌ์กฐ๋ ๋์ผํ์ง๋ง ์์ ์ ์คํ์ผ์ด ์ฝ๊ฐ ๋ค๋ฆ
๋๋ค. *์๋ฅผ ๋ค๋ฉด*:
````text
[...]
=====
Human: Answer the question in the variable `question` about the image stored in the variable `image`.
Assistant: I will use the tool `image_qa` to answer the question on the input image.
```py
answer = image_qa(text=question, image=image)
print(f"The answer is {answer}")
```
Human: I tried this code, it worked but didn't give me a good result. The question is in French
Assistant: In this case, the question needs to be translated first. I will use the tool `translator` to do this.
```py
translated_question = translator(question=question, src_lang="French", tgt_lang="English")
print(f"The translated question is {translated_question}.")
answer = image_qa(text=translated_question, image=image)
print(f"The answer is {answer}")
```
=====
[...]
````
`run` ํ๋กฌํํธ์ ์์๋ ๋ฐ๋๋ก, ๊ฐ `chat` ํ๋กฌํํธ์ ์์๋ *Human(์ฌ๋)*๊ณผ *Assistant(์ด์์คํดํธ)* ๊ฐ์ ํ๋ ์ด์์ ๊ตํ์ด ์์ต๋๋ค. ๋ชจ๋ ๊ตํ์ `run` ํ๋กฌํํธ์ ์์ ์ ์ฌํ ๊ตฌ์กฐ๋ก ๋์ด ์์ต๋๋ค.
์ฌ์ฉ์์ ์
๋ ฅ์ด *Human:* ๋ค์ ์ถ๊ฐ๋๋ฉฐ, ์์ด์ ํธ์๊ฒ ์ฝ๋๋ฅผ ์์ฑํ๊ธฐ ์ ์ ์ํํด์ผ ํ ์์
์ ๋จผ์ ์์ฑํ๋ผ๋ ๋ฉ์์ง๊ฐ ํ์๋ฉ๋๋ค.
๊ตํ์ ์ด์ ๊ตํ์ ๊ธฐ๋ฐ์ผ๋ก ํ ์ ์์ผ๋ฏ๋ก ์์ ๊ฐ์ด ์ฌ์ฉ์๊ฐ "**์ด** ์ฝ๋๋ฅผ ์๋ํ์ต๋๋ค"๋ผ๊ณ ์
๋ ฅํ๋ฉด ์ด์ ์ ์์ฑ๋ ์์ด์ ํธ์ ์ฝ๋๋ฅผ ์ฐธ์กฐํ์ฌ ๊ณผ๊ฑฐ ๊ตํ์ ์ฐธ์กฐํ ์ ์์ต๋๋ค.
`.chat`์ ์คํํ๋ฉด ์ฌ์ฉ์์ ์
๋ ฅ ๋๋ *์์
*์ด ๋ฏธ์์ฑ๋ ์์์ ์์๋ก ์บ์คํ
๋ฉ๋๋ค:
```text
Human: <user-input>\n\nAssistant:
```
๊ทธ๋ฌ๋ฉด ์์ด์ ํธ๊ฐ ์ด๋ฅผ ์์ฑํฉ๋๋ค. `run` ๋ช
๋ น๊ณผ ๋ฌ๋ฆฌ `chat` ๋ช
๋ น์ ์๋ฃ๋ ์์ ๋ฅผ ํ๋กฌํํธ์ ์ถ๊ฐํ์ฌ ์์ด์ ํธ์๊ฒ ๋ค์ `chat` ์ฐจ๋ก์ ๋ํ ๋ ๋ง์ ๋ฌธ๋งฅ์ ์ ๊ณตํฉ๋๋ค.
์ด์ ํ๋กฌํํธ๊ฐ ์ด๋ป๊ฒ ๊ตฌ์ฑ๋์ด ์๋์ง ์์์ผ๋ ์ด๋ป๊ฒ ์ฌ์ฉ์ ์ ์ํ ์ ์๋์ง ์ดํด๋ด
์๋ค!
### ์ข์ ์ฌ์ฉ์ ์
๋ ฅ ์์ฑํ๊ธฐ[[writing-good-user-inputs]]
๋๊ท๋ชจ ์ธ์ด ๋ชจ๋ธ์ด ์ฌ์ฉ์์ ์๋๋ฅผ ์ดํดํ๋ ๋ฅ๋ ฅ์ด ์ ์ ๋ ํฅ์๋๊ณ ์์ง๋ง, ์์ด์ ํธ๊ฐ ์ฌ๋ฐ๋ฅธ ์์
์ ์ ํํ ์ ์๋๋ก ์ต๋ํ ์ ํ์ฑ์ ์ ์งํ๋ ๊ฒ์ ํฐ ๋์์ด ๋ฉ๋๋ค.
์ต๋ํ ์ ํํ๋ค๋ ๊ฒ์ ๋ฌด์์ ์๋ฏธํ ๊น์?
์์ด์ ํธ๋ ํ๋กฌํํธ์์ ๋๊ตฌ ์ด๋ฆ ๋ชฉ๋ก๊ณผ ํด๋น ์ค๋ช
์ ๋ณผ ์ ์์ต๋๋ค.
๋ ๋ง์ ๋๊ตฌ๊ฐ ์ถ๊ฐ๋ ์๋ก ์์ด์ ํธ๊ฐ ์ฌ๋ฐ๋ฅธ ๋๊ตฌ๋ฅผ ์ ํํ๊ธฐ๊ฐ ๋ ์ด๋ ค์์ง๊ณ ์คํํ ๋๊ตฌ์ ์ฌ๋ฐ๋ฅธ ์์๋ฅผ ์ ํํ๋ ๊ฒ์ ๋์ฑ ์ด๋ ค์์ง๋๋ค.
์ผ๋ฐ์ ์ธ ์คํจ ์ฌ๋ก๋ฅผ ์ดํด๋ณด๊ฒ ์ต๋๋ค. ์ฌ๊ธฐ์๋ ๋ถ์ํ ์ฝ๋๋ง ๋ฐํํ๊ฒ ์ต๋๋ค.
```py
from transformers import HfAgent
agent = HfAgent("https://api-inference.huggingface.co/models/bigcode/starcoder")
agent.run("Show me a tree", return_code=True)
```
๊ทธ๋ฌ๋ฉด ๋ค์ ๊ฒฐ๊ณผ๊ฐ ์ถ๋ ฅ๋ฉ๋๋ค:
```text
==Explanation from the agent==
I will use the following tool: `image_segmenter` to create a segmentation mask for the image.
==Code generated by the agent==
mask = image_segmenter(image, prompt="tree")
```
์ฐ๋ฆฌ๊ฐ ์ํ๋ ๊ฒฐ๊ณผ๊ฐ ์๋ ์๋ ์์ต๋๋ค. ๋์ ๋๋ฌด ์ด๋ฏธ์ง๊ฐ ์์ฑ๋๊ธฐ๋ฅผ ์ํ ๊ฐ๋ฅ์ฑ์ด ๋ ๋์ต๋๋ค.
๋ฐ๋ผ์ ์์ด์ ํธ๊ฐ ํน์ ๋๊ตฌ๋ฅผ ์ฌ์ฉํ๋๋ก ์ ๋ํ๋ ค๋ฉด ๋๊ตฌ์ ์ด๋ฆ๊ณผ ์ค๋ช
์ ์๋ ์ค์ํ ํค์๋๋ฅผ ์ฌ์ฉํ๋ ๊ฒ์ด ๋งค์ฐ ์ ์ฉํ ์ ์์ต๋๋ค. ํ๋ฒ ์ดํด๋ณด๊ฒ ์ต๋๋ค.
```py
agent.toolbox["image_generator"].description
```
```text
'This is a tool that creates an image according to a prompt, which is a text description. It takes an input named `prompt` which contains the image description and outputs an image.
```
์ด๋ฆ๊ณผ ์ค๋ช
์ "image", "prompt", "create" ๋ฐ "generate" ํค์๋๋ฅผ ์ฌ์ฉํฉ๋๋ค. ์ด ๋จ์ด๋ค์ ์ฌ์ฉํ๋ฉด ๋ ์ ์๋ํ ๊ฐ๋ฅ์ฑ์ด ๋์ต๋๋ค. ํ๋กฌํํธ๋ฅผ ์กฐ๊ธ ๋ ๊ตฌ์ฒดํํด ๋ณด๊ฒ ์ต๋๋ค.
```py
agent.run("Create an image of a tree", return_code=True)
```
์ด ์ฝ๋๋ ๋ค์ ํ๋กฌํํธ๋ฅผ ๋ง๋ค์ด๋
๋๋ค:
```text
==Explanation from the agent==
I will use the following tool `image_generator` to generate an image of a tree.
==Code generated by the agent==
image = image_generator(prompt="tree")
```
ํจ์ฌ ๋ซ๋ค์! ์ ํฌ๊ฐ ์ํ๋ ๊ฒ๊ณผ ๋น์ทํด ๋ณด์
๋๋ค.
์ฆ, ์์ด์ ํธ๊ฐ ์์
์ ์ฌ๋ฐ๋ฅธ ๋๊ตฌ์ ์ฌ๋ฐ๋ฅด๊ฒ ๋งคํํ๋ ๋ฐ ์ด๋ ค์์ ๊ฒช๊ณ ์๋ค๋ฉด ๋๊ตฌ ์ด๋ฆ๊ณผ ์ค๋ช
์์ ๊ฐ์ฅ ๊ด๋ จ์ฑ์ด ๋์ ํค์๋๋ฅผ ์ฐพ์๋ณด๊ณ ์ด๋ฅผ ํตํด ์์
์์ฒญ์ ๊ตฌ์ฒดํํด ๋ณด์ธ์.
### ๋๊ตฌ ์ค๋ช
์ฌ์ฉ์ ์ ์ํ๊ธฐ[[customizing-the-tool-descriptions]]
์์ ์ดํด๋ณธ ๊ฒ์ฒ๋ผ ์์ด์ ํธ๋ ๊ฐ ๋๊ตฌ์ ์ด๋ฆ๊ณผ ์ค๋ช
์ ์ก์ธ์คํ ์ ์์ต๋๋ค.
๊ธฐ๋ณธ ๋๊ตฌ์๋ ๋งค์ฐ ์ ํํ ์ด๋ฆ๊ณผ ์ค๋ช
์ด ์์ด์ผ ํ์ง๋ง ํน์ ์ฌ์ฉ ์ฌ๋ก์ ๋ง๊ฒ ๋๊ตฌ์ ์ค๋ช
์ด๋ ์ด๋ฆ์ ๋ณ๊ฒฝํ๋ ๊ฒ์ด ๋์์ด ๋ ์๋ ์์ต๋๋ค.
์ด๋ ๋งค์ฐ ์ ์ฌํ ์ฌ๋ฌ ๋๊ตฌ๋ฅผ ์ถ๊ฐํ๊ฑฐ๋ ํน์ ๋๋ฉ์ธ(*์*: ์ด๋ฏธ์ง ์์ฑ ๋ฐ ๋ณํ)์๋ง ์์ด์ ํธ๋ฅผ ์ฌ์ฉํ๋ ค๋ ๊ฒฝ์ฐ์ ํนํ ์ค์ํด์ง ์ ์์ต๋๋ค.
์ผ๋ฐ์ ์ธ ๋ฌธ์ ๋ ์ด๋ฏธ์ง ์์ฑ ์์
์ ๋ง์ด ์ฌ์ฉ๋๋ ๊ฒฝ์ฐ ์์ด์ ํธ๊ฐ ์ด๋ฏธ์ง ์์ฑ๊ณผ ์ด๋ฏธ์ง ๋ณํ/์์ ์ ํผ๋ํ๋ ๊ฒ์
๋๋ค. *์๋ฅผ ๋ค์ด,*
```py
agent.run("Make an image of a house and a car", return_code=True)
```
๊ทธ๋ฌ๋ฉด ๋ค์ ๊ฒฐ๊ณผ๊ฐ ์ถ๋ ฅ๋ฉ๋๋ค:
```text
==Explanation from the agent==
I will use the following tools `image_generator` to generate an image of a house and `image_transformer` to transform the image of a car into the image of a house.
==Code generated by the agent==
house_image = image_generator(prompt="A house")
car_image = image_generator(prompt="A car")
house_car_image = image_transformer(image=car_image, prompt="A house")
```
๊ฒฐ๊ณผ๋ฌผ์ด ์ฐ๋ฆฌ๊ฐ ์ฌ๊ธฐ์ ์ํ๋ ๊ฒ๊ณผ ์ ํํ ์ผ์นํ์ง ์์ ์ ์์ต๋๋ค. ์์ด์ ํธ๊ฐ `image_generator`์ `image_transformer`์ ์ฐจ์ด์ ์ ์ดํดํ๊ธฐ ์ด๋ ค์์ ๋ ๊ฐ์ง๋ฅผ ํจ๊ป ์ฌ์ฉํ๋ ๊ฒฝ์ฐ๊ฐ ๋ง์ ๊ฒ ๊ฐ์ต๋๋ค.
์ฌ๊ธฐ์ `image_transformer`์ ๋๊ตฌ ์ด๋ฆ๊ณผ ์ค๋ช
์ ๋ณ๊ฒฝํ์ฌ ์์ด์ ํธ๊ฐ ๋์ธ ์ ์์ต๋๋ค.
"image" ๋ฐ "prompt"์ ์ฝ๊ฐ ๋ถ๋ฆฌํ๊ธฐ ์ํด `modifier`๋ผ๊ณ ๋์ ๋ถ๋ฅด๊ฒ ์ต๋๋ค:
```py
agent.toolbox["modifier"] = agent.toolbox.pop("image_transformer")
agent.toolbox["modifier"].description = agent.toolbox["modifier"].description.replace(
"transforms an image according to a prompt", "modifies an image"
)
```
์ด์ "modify"์ ์ ์ด๋ฏธ์ง ํ๋ก์ธ์๋ฅผ ์ฌ์ฉํ๋ผ๋ ๊ฐ๋ ฅํ ์ ํธ์ด๋ฏ๋ก ์์ ํ๋กฌํํธ์ ๋์์ด ๋ ๊ฒ์
๋๋ค. ๋ค์ ์คํํด ๋ด
์๋ค.
```py
agent.run("Make an image of a house and a car", return_code=True)
```
์ฌ๊ธฐ์ ๋ค์๊ณผ ๊ฐ์ ๊ฒฐ๊ณผ๋ฅผ ์ป๊ฒ ๋ฉ๋๋ค:
```text
==Explanation from the agent==
I will use the following tools: `image_generator` to generate an image of a house, then `image_generator` to generate an image of a car.
==Code generated by the agent==
house_image = image_generator(prompt="A house")
car_image = image_generator(prompt="A car")
```
์ฐ๋ฆฌ๊ฐ ์ผ๋์ ๋์๋ ๊ฒ๊ณผ ํ์คํ ๋ ๊ฐ๊น์์ก์ต๋๋ค! ํ์ง๋ง ์ง๊ณผ ์๋์ฐจ๊ฐ ๋ชจ๋ ๊ฐ์ ์ด๋ฏธ์ง์ ํฌํจ๋๋ฉด ์ข๊ฒ ์ต๋๋ค. ์์
์ ๋จ์ผ ์ด๋ฏธ์ง ์์ฑ์ ๋ ์ง์คํ๋ฉด ๋์์ด ๋ ๊ฒ์
๋๋ค:
```py
agent.run("Create image: 'A house and car'", return_code=True)
```
```text
==Explanation from the agent==
I will use the following tool: `image_generator` to generate an image.
==Code generated by the agent==
image = image_generator(prompt="A house and car")
```
<Tip warning={true}>
์์ด์ ํธ๋ ์ฌ์ ํ ํนํ ์ฌ๋ฌ ๊ฐ์ฒด์ ์ด๋ฏธ์ง๋ฅผ ์์ฑํ๋ ๊ฒ๊ณผ ๊ฐ์ด ์ฝ๊ฐ ๋ ๋ณต์กํ ์ฌ์ฉ ์ฌ๋ก์์ ์ทจ์ฝํ ๊ฒฝ์ฐ๊ฐ ๋ง์ต๋๋ค.
์์ผ๋ก ๋ช ๋ฌ ์์ ์์ด์ ํธ ์์ฒด์ ๊ธฐ๋ณธ ํ๋กฌํํธ๊ฐ ๋์ฑ ๊ฐ์ ๋์ด ์์ด์ ํธ๊ฐ ๋ค์ํ ์ฌ์ฉ์ ์
๋ ฅ์ ๋์ฑ ๊ฐ๋ ฅํ๊ฒ ๋์ํ ์ ์๋๋ก ํ ์์ ์
๋๋ค.
</Tip>
### ์ ์ฒด ํ๋กฌํํธ ์ฌ์ฉ์ ์ ์ํ๊ธฐ[[customizing-the-whole-prompt]]
์ฌ์ฉ์์๊ฒ ์ต๋ํ์ ์ ์ฐ์ฑ์ ์ ๊ณตํ๊ธฐ ์ํด [์](#structure-of-the-prompt)์ ์ค๋ช
๋ ์ ์ฒด ํ๋กฌํํธ ํ
ํ๋ฆฟ์ ์ฌ์ฉ์๊ฐ ๋ฎ์ด์ธ ์ ์์ต๋๋ค.
์ด ๊ฒฝ์ฐ ์ฌ์ฉ์ ์ ์ ํ๋กฌํํธ์ ์๊ฐ ์น์
, ๋๊ตฌ ์น์
, ์์ ์น์
๋ฐ ๋ฏธ์์ฑ ์์ ์น์
์ด ํฌํจ๋์ด ์๋์ง ํ์ธํ์ธ์.
`run` ํ๋กฌํํธ ํ
ํ๋ฆฟ์ ๋ฎ์ด์ฐ๋ ค๋ฉด ๋ค์๊ณผ ๊ฐ์ด ํ๋ฉด ๋ฉ๋๋ค:
```py
template = """ [...] """
agent = HfAgent(your_endpoint, run_prompt_template=template)
```
<Tip warning={true}>
์์ด์ ํธ๊ฐ ์ฌ์ฉ ๊ฐ๋ฅํ ๋๊ตฌ๋ฅผ ์ธ์ํ๊ณ ์ฌ์ฉ์์ ํ๋กฌํํธ๋ฅผ ์ฌ๋ฐ๋ฅด๊ฒ ์ฝ์
ํ ์ ์๋๋ก `<<all_tools>>` ๋ฌธ์์ด๊ณผ `<<prompt>>`๋ฅผ `template` ์ด๋๊ฐ์ ์ ์ํด์ผ ํฉ๋๋ค.
</Tip>
๋ง์ฐฌ๊ฐ์ง๋ก `chat` ํ๋กฌํํธ ํ
ํ๋ฆฟ์ ๋ฎ์ด์ธ ์ ์์ต๋๋ค. `chat` ๋ชจ๋์์๋ ํญ์ ๋ค์๊ณผ ๊ฐ์ ๊ตํ ํ์์ ์ฌ์ฉํ๋ค๋ ์ ์ ์ ์ํ์ธ์:
```text
Human: <<task>>
Assistant:
```
๋ฐ๋ผ์ ์ฌ์ฉ์ ์ ์ `chat` ํ๋กฌํํธ ํ
ํ๋ฆฟ์ ์์ ์์๋ ์ด ํ์์ ์ฌ์ฉํ๋ ๊ฒ์ด ์ค์ํฉ๋๋ค.
๋ค์๊ณผ ๊ฐ์ด ์ธ์คํด์คํ ํ ๋ `chat` ํ
ํ๋ฆฟ์ ๋ฎ์ด์ธ ์ ์์ต๋๋ค.
```
template = """ [...] """
agent = HfAgent(url_endpoint=your_endpoint, chat_prompt_template=template)
```
<Tip warning={true}>
์์ด์ ํธ๊ฐ ์ฌ์ฉ ๊ฐ๋ฅํ ๋๊ตฌ๋ฅผ ์ธ์ํ ์ ์๋๋ก `<<all_tools>>` ๋ฌธ์์ด์ `template` ์ด๋๊ฐ์ ์ ์ํด์ผ ํฉ๋๋ค.
</Tip>
๋ ๊ฒฝ์ฐ ๋ชจ๋ ์ปค๋ฎค๋ํฐ์ ๋๊ตฐ๊ฐ๊ฐ ํธ์คํ
ํ๋ ํ
ํ๋ฆฟ์ ์ฌ์ฉํ๋ ค๋ ๊ฒฝ์ฐ ํ๋กฌํํธ ํ
ํ๋ฆฟ ๋์ ์ ์ฅ์ ID๋ฅผ ์ ๋ฌํ ์ ์์ต๋๋ค.
๊ธฐ๋ณธ ํ๋กฌํํธ๋ [์ด ์ ์ฅ์](https://huggingface.co/datasets/huggingface-tools/default-prompts)๋ฅผ ์๋ก ๋ค ์ ์์ต๋๋ค.
Hub์ ์ ์ฅ์์ ์ฌ์ฉ์ ์ ์ ํ๋กฌํํธ๋ฅผ ์
๋ก๋ํ์ฌ ์ปค๋ฎค๋ํฐ์ ๊ณต์ ํ๋ ค๋ฉด ๋ค์์ ํ์ธํ์ธ์:
- ๋ฐ์ดํฐ ์ธํธ ์ ์ฅ์๋ฅผ ์ฌ์ฉํ์ธ์.
- `run` ๋ช
๋ น์ ๋ํ ํ๋กฌํํธ ํ
ํ๋ฆฟ์ `run_prompt_template.txt`๋ผ๋ ํ์ผ์ ๋ฃ์ผ์ธ์.
- `chat` ๋ช
๋ น์ ๋ํ ํ๋กฌํํธ ํ
ํ๋ฆฟ์ `chat_prompt_template.txt`๋ผ๋ ํ์ผ์ ๋ฃ์ผ์ธ์.
## ์ฌ์ฉ์ ์ ์ ๋๊ตฌ ์ฌ์ฉํ๊ธฐ[[using-custom-tools]]
์ด ์น์
์์๋ ์ด๋ฏธ์ง ์์ฑ์ ํนํ๋ ๋ ๊ฐ์ง ๊ธฐ์กด ์ฌ์ฉ์ ์ ์ ๋๊ตฌ๋ฅผ ํ์ฉํ๊ฒ ์ต๋๋ค:
- ๋ ๋ง์ ์ด๋ฏธ์ง ์์ ์ ํ์ฉํ๊ธฐ ์ํด [huggingface-tools/image-transformation](https://huggingface.co/spaces/huggingface-tools/image-transformation)์
[diffusers/controlnet-canny-tool](https://huggingface.co/spaces/diffusers/controlnet-canny-tool)๋ก ๋์ฒดํฉ๋๋ค.
- ๊ธฐ๋ณธ ๋๊ตฌ ์์์ ์ด๋ฏธ์ง ์
์ค์ผ์ผ๋ง์ ์ํ ์๋ก์ด ๋๊ตฌ๊ฐ ์ถ๊ฐ๋์์ต๋๋ค:
[diffusers/latent-upscaler-tool](https://huggingface.co/spaces/diffusers/latent-upscaler-tool)๊ฐ ๊ธฐ์กด ์ด๋ฏธ์ง ๋ณํ ๋๊ตฌ๋ฅผ ๋์ฒดํฉ๋๋ค.
ํธ๋ฆฌํ [`load_tool`] ํจ์๋ฅผ ์ฌ์ฉํ์ฌ ์ฌ์ฉ์ ์ ์ ๋๊ตฌ๋ฅผ ๊ฐ์ ธ์ค๋ ๊ฒ์ผ๋ก ์์ํ๊ฒ ์ต๋๋ค:
```py
from transformers import load_tool
controlnet_transformer = load_tool("diffusers/controlnet-canny-tool")
upscaler = load_tool("diffusers/latent-upscaler-tool")
```
์์ด์ ํธ์๊ฒ ์ฌ์ฉ์ ์ ์ ๋๊ตฌ๋ฅผ ์ถ๊ฐํ๋ฉด ๋๊ตฌ์ ์ค๋ช
๊ณผ ์ด๋ฆ์ด ์์ด์ ํธ์ ํ๋กฌํํธ์ ์๋์ผ๋ก ํฌํจ๋ฉ๋๋ค.
๋ฐ๋ผ์ ์์ด์ ํธ๊ฐ ์ฌ์ฉ ๋ฐฉ๋ฒ์ ์ดํดํ ์ ์๋๋ก ์ฌ์ฉ์ ์ ์ ๋๊ตฌ์ ์ค๋ช
๊ณผ ์ด๋ฆ์ ์ ์์ฑํด์ผ ํฉ๋๋ค.
`controlnet_transformer`์ ์ค๋ช
๊ณผ ์ด๋ฆ์ ์ดํด๋ณด๊ฒ ์ต๋๋ค:
```py
print(f"Description: '{controlnet_transformer.description}'")
print(f"Name: '{controlnet_transformer.name}'")
```
๊ทธ๋ฌ๋ฉด ๋ค์ ๊ฒฐ๊ณผ๊ฐ ์ถ๋ ฅ๋ฉ๋๋ค:
```text
Description: 'This is a tool that transforms an image with ControlNet according to a prompt.
It takes two inputs: `image`, which should be the image to transform, and `prompt`, which should be the prompt to use to change it. It returns the modified image.'
Name: 'image_transformer'
```
์ด๋ฆ๊ณผ ์ค๋ช
์ด ์ ํํ๊ณ [ํ๋ ์ดํ
๋ ๋๊ตฌ ์ธํธ(curated set of tools)](./transformers_agents#a-curated-set-of-tools)์ ์คํ์ผ์ ๋ง์ต๋๋ค.
๋ค์์ผ๋ก, `controlnet_transformer`์ `upscaler`๋ก ์์ด์ ํธ๋ฅผ ์ธ์คํด์คํํด ๋ด
์๋ค:
```py
tools = [controlnet_transformer, upscaler]
agent = HfAgent("https://api-inference.huggingface.co/models/bigcode/starcoder", additional_tools=tools)
```
์ด ๋ช
๋ น์ ์คํํ๋ฉด ๋ค์ ์ ๋ณด๊ฐ ํ์๋ฉ๋๋ค:
```text
image_transformer has been replaced by <transformers_modules.diffusers.controlnet-canny-tool.bd76182c7777eba9612fc03c0
8718a60c0aa6312.image_transformation.ControlNetTransformationTool object at 0x7f1d3bfa3a00> as provided in `additional_tools`
```
ํ๋ ์ดํ
๋ ๋๊ตฌ ์ธํธ์๋ ์ด๋ฏธ 'image_transformer' ๋๊ตฌ๊ฐ ์์ผ๋ฉฐ, ์ด ๋๊ตฌ๋ ์ฌ์ฉ์ ์ ์ ๋๊ตฌ๋ก ๋์ฒด๋ฉ๋๋ค.
<Tip>
๊ธฐ์กด ๋๊ตฌ์ ๋๊ฐ์ ์์
์ ์ฌ์ฉ์ ์ ์ ๋๊ตฌ๋ฅผ ์ฌ์ฉํ๋ ค๋ ๊ฒฝ์ฐ ๊ธฐ์กด ๋๊ตฌ๋ฅผ ๋ฎ์ด์ฐ๋ ๊ฒ์ด ์ ์ฉํ ์ ์์ต๋๋ค.
์์ด์ ํธ๊ฐ ํด๋น ์์
์ ๋ฅ์ํ๊ธฐ ๋๋ฌธ์
๋๋ค.
์ด ๊ฒฝ์ฐ ์ฌ์ฉ์ ์ ์ ๋๊ตฌ๊ฐ ๋ฎ์ด์ด ๋๊ตฌ์ ์ ํํ ๋์ผํ API๋ฅผ ๋ฐ๋ผ์ผ ํ๋ฉฐ, ๊ทธ๋ ์ง ์์ผ๋ฉด ํด๋น ๋๊ตฌ๋ฅผ ์ฌ์ฉํ๋ ๋ชจ๋ ์์ ๊ฐ ์
๋ฐ์ดํธ๋๋๋ก ํ๋กฌํํธ ํ
ํ๋ฆฟ์ ์กฐ์ ํด์ผ ํ๋ค๋ ์ ์ ์ ์ํ์ธ์.
</Tip>
์
์ค์ผ์ผ๋ฌ ๋๊ตฌ์ ์ง์ ๋ 'image_upscaler'๋ผ๋ ์ด๋ฆ ์์ง ๊ธฐ๋ณธ ๋๊ตฌ ์์์๋ ์กด์ฌํ์ง ์๊ธฐ ๋๋ฌธ์, ๋๊ตฌ ๋ชฉ๋ก์ ํด๋น ์ด๋ฆ์ด ๊ฐ๋จํ ์ถ๊ฐ๋์์ต๋๋ค.
์์ด์ ํธ๊ฐ ํ์ฌ ์ฌ์ฉํ ์ ์๋ ๋๊ตฌ ์์๋ ์ธ์ ๋ ์ง `agent.toolbox` ์์ฑ์ ํตํด ํ์ธํ ์ ์์ต๋๋ค:
```py
print("\n".join([f"- {a}" for a in agent.toolbox.keys()]))
```
```text
- document_qa
- image_captioner
- image_qa
- image_segmenter
- transcriber
- summarizer
- text_classifier
- text_qa
- text_reader
- translator
- image_transformer
- text_downloader
- image_generator
- video_generator
- image_upscaler
```
์์ด์ ํธ์ ๋๊ตฌ ์์์ `image_upscaler`๊ฐ ์ถ๊ฐ๋ ์ ์ ์ฃผ๋ชฉํ์ธ์.
์ด์ ์๋ก์ด ๋๊ตฌ๋ฅผ ์ฌ์ฉํด๋ด
์๋ค! [Transformers Agents Quickstart](./transformers_agents#single-execution-run)์์ ์์ฑํ ์ด๋ฏธ์ง๋ฅผ ๋ค์ ์ฌ์ฉํ๊ฒ ์ต๋๋ค.
```py
from diffusers.utils import load_image
image = load_image(
"https://huggingface.co/datasets/huggingface/documentation-images/resolve/main/transformers/rivers_and_lakes.png"
)
```
<img src="https://huggingface.co/datasets/huggingface/documentation-images/resolve/main/transformers/rivers_and_lakes.png" width=200>
์ด๋ฏธ์ง๋ฅผ ์๋ฆ๋ค์ด ๊ฒจ์ธ ํ๊ฒฝ์ผ๋ก ๋ฐ๊ฟ ๋ด
์๋ค:
```py
image = agent.run("Transform the image: 'A frozen lake and snowy forest'", image=image)
```
```text
==Explanation from the agent==
I will use the following tool: `image_transformer` to transform the image.
==Code generated by the agent==
image = image_transformer(image, prompt="A frozen lake and snowy forest")
```
<img src="https://huggingface.co/datasets/huggingface/documentation-images/resolve/main/transformers/rivers_and_lakes_winter.png" width=200>
์๋ก์ด ์ด๋ฏธ์ง ์ฒ๋ฆฌ ๋๊ตฌ๋ ์ด๋ฏธ์ง๋ฅผ ๋งค์ฐ ๊ฐ๋ ฅํ๊ฒ ์์ ํ ์ ์๋ ControlNet์ ๊ธฐ๋ฐ์ผ๋ก ํฉ๋๋ค.
๊ธฐ๋ณธ์ ์ผ๋ก ์ด๋ฏธ์ง ์ฒ๋ฆฌ ๋๊ตฌ๋ 512x512 ํฝ์
ํฌ๊ธฐ์ ์ด๋ฏธ์ง๋ฅผ ๋ฐํํฉ๋๋ค. ์ด๋ฅผ ์
์ค์ผ์ผ๋งํ ์ ์๋์ง ์ดํด๋ด
์๋ค.
```py
image = agent.run("Upscale the image", image)
```
```text
==Explanation from the agent==
I will use the following tool: `image_upscaler` to upscale the image.
==Code generated by the agent==
upscaled_image = image_upscaler(image)
```
<img src="https://huggingface.co/datasets/huggingface/documentation-images/resolve/main/transformers/rivers_and_lakes_winter_upscale.png" width=400>
์์ด์ ํธ๋ ์
์ค์ผ์ผ๋ฌ ๋๊ตฌ์ ์ค๋ช
๊ณผ ์ด๋ฆ๋ง ๋ณด๊ณ ๋ฐฉ๊ธ ์ถ๊ฐํ ์
์ค์ผ์ผ๋ฌ ๋๊ตฌ์ "์ด๋ฏธ์ง ์
์ค์ผ์ผ๋ง"์ด๋ผ๋ ํ๋กฌํํธ๋ฅผ ์๋์ผ๋ก ๋งคํํ์ฌ ์ฌ๋ฐ๋ฅด๊ฒ ์คํํ์ต๋๋ค.
๋ค์์ผ๋ก ์ ์ฌ์ฉ์ ์ ์ ๋๊ตฌ๋ฅผ ๋ง๋๋ ๋ฐฉ๋ฒ์ ์ดํด๋ณด๊ฒ ์ต๋๋ค.
### ์ ๋๊ตฌ ์ถ๊ฐํ๊ธฐ[[adding-new-tools]]
์ด ์น์
์์๋ ์์ด์ ํธ์๊ฒ ์ถ๊ฐํ ์ ์๋ ์ ๋๊ตฌ๋ฅผ ๋ง๋๋ ๋ฐฉ๋ฒ์ ๋ณด์ฌ ๋๋ฆฝ๋๋ค.
#### ์ ๋๊ตฌ ๋ง๋ค๊ธฐ[[creating-a-new-tool]]
๋จผ์ ๋๊ตฌ๋ฅผ ๋ง๋๋ ๊ฒ๋ถํฐ ์์ํ๊ฒ ์ต๋๋ค.
ํน์ ์์
์ ๋ํด ๊ฐ์ฅ ๋ง์ ๋ค์ด๋ก๋๋ฅผ ๋ฐ์ Hugging Face Hub์ ๋ชจ๋ธ์ ๊ฐ์ ธ์ค๋, ๊ทธ๋ค์ง ์ ์ฉํ์ง๋ ์์ง๋ง ์ฌ๋ฏธ์๋ ์์
์ ์ถ๊ฐํ๊ฒ ์ต๋๋ค.
๋ค์ ์ฝ๋๋ฅผ ์ฌ์ฉํ๋ฉด ๋ฉ๋๋ค:
```python
from huggingface_hub import list_models
task = "text-classification"
model = next(iter(list_models(filter=task, sort="downloads", direction=-1)))
print(model.id)
```
`text-classification`(ํ
์คํธ ๋ถ๋ฅ) ์์
์ ๊ฒฝ์ฐ `'facebook/bart-large-mnli'`๋ฅผ ๋ฐํํ๊ณ , `translation`(๋ฒ์ญ) ์์
์ ๊ฒฝ์ฐ `'t5-base'`๋ฅผ ๋ฐํํฉ๋๋ค.
์ด๋ฅผ ์์ด์ ํธ๊ฐ ํ์ฉํ ์ ์๋ ๋๊ตฌ๋ก ๋ณํํ๋ ค๋ฉด ์ด๋ป๊ฒ ํด์ผ ํ ๊น์?
๋ชจ๋ ๋๊ตฌ๋ ํ์ํ ์ฃผ์ ์์ฑ์ ๋ณด์ ํ๋ ์ํผํด๋์ค `Tool`์ ์์กดํฉ๋๋ค. ์ด๋ฅผ ์์ํ๋ ํด๋์ค๋ฅผ ๋ง๋ค์ด ๋ณด๊ฒ ์ต๋๋ค:
```python
from transformers import Tool
class HFModelDownloadsTool(Tool):
pass
```
์ด ํด๋์ค์๋ ๋ช ๊ฐ์ง ์๊ตฌ์ฌํญ์ด ์์ต๋๋ค:
- ๋๊ตฌ ์์ฒด์ ์ด๋ฆ์ ํด๋นํ๋ `name` ์์ฑ. ์ํ๋ช
์ด ์๋ ๋ค๋ฅธ ๋๊ตฌ์ ํธํ๋๋๋ก `model_download_counter`๋ก ์ด๋ฆ์ ์ง์ ํ๊ฒ ์ต๋๋ค.
- ์์ด์ ํธ์ ํ๋กฌํํธ๋ฅผ ์ฑ์ฐ๋ ๋ฐ ์ฌ์ฉ๋๋ ์์ฑ `description`.
- `inputs` ๋ฐ `outputs` ์์ฑ. ์ด๋ฅผ ์ ์ํ๋ฉด Python ์ธํฐํ๋ฆฌํฐ๊ฐ ์ ํ์ ๋ํ ์ ๋ณด์ ์
๊ฐํ ์ ํ์ ํ๋ ๋ฐ ๋์์ด ๋๋ฉฐ,
๋๊ตฌ๋ฅผ ํ๋ธ์ ํธ์ํ ๋ gradio ๋ฐ๋ชจ๋ฅผ ์์ฑํ ์ ์์ต๋๋ค.
๋ ์์ฑ ๋ชจ๋ ๊ฐ์ 'ํ
์คํธ', '์ด๋ฏธ์ง' ๋๋ '์ค๋์ค'๊ฐ ๋ ์ ์๋ ์์ ๊ฐ์ ๋ฆฌ์คํธ์
๋๋ค.
- ์ถ๋ก ์ฝ๋๊ฐ ํฌํจ๋ `__call__` ๋ฉ์๋. ์ด๊ฒ์ด ์ฐ๋ฆฌ๊ฐ ์์์ ๋ค๋ฃจ์๋ ์ฝ๋์
๋๋ค!
์ด์ ํด๋์ค์ ๋ชจ์ต์ ๋ค์๊ณผ ๊ฐ์ต๋๋ค:
```python
from transformers import Tool
from huggingface_hub import list_models
class HFModelDownloadsTool(Tool):
name = "model_download_counter"
description = (
"This is a tool that returns the most downloaded model of a given task on the Hugging Face Hub. "
"It takes the name of the category (such as text-classification, depth-estimation, etc), and "
"returns the name of the checkpoint."
)
inputs = ["text"]
outputs = ["text"]
def __call__(self, task: str):
model = next(iter(list_models(filter=task, sort="downloads", direction=-1)))
return model.id
```
์ด์ ๋๊ตฌ๋ฅผ ์์ฝ๊ฒ ์ฌ์ฉํ ์ ์๊ฒ ๋์์ต๋๋ค.
๋๊ตฌ๋ฅผ ํ์ผ์ ์ ์ฅํ๊ณ ๋ฉ์ธ ์คํฌ๋ฆฝํธ์์ ๊ฐ์ ธ์ต๋๋ค. ์ด ํ์ผ์ ์ด๋ฆ์ `model_downloads.py`๋ก ์ง์ ํ๋ฉด ๊ฒฐ๊ณผ์ ์ผ๋ก ๊ฐ์ ธ์ค๊ธฐ ์ฝ๋๋ ๋ค์๊ณผ ๊ฐ์ต๋๋ค:
```python
from model_downloads import HFModelDownloadsTool
tool = HFModelDownloadsTool()
```
๋ค๋ฅธ ์ฌ๋๋ค์ด ์ด ๊ธฐ๋ฅ์ ํ์ฉํ ์ ์๋๋ก ํ๊ณ ์ด๊ธฐํ๋ฅผ ๋ ๊ฐ๋จํ๊ฒ ํ๋ ค๋ฉด ๋ค์์คํ์ด์ค ์๋์ Hub๋ก ํธ์ํ๋ ๊ฒ์ด ์ข์ต๋๋ค.
๊ทธ๋ ๊ฒ ํ๋ ค๋ฉด `tool` ๋ณ์์์ `push_to_hub`๋ฅผ ํธ์ถํ๋ฉด ๋ฉ๋๋ค:
```python
tool.push_to_hub("hf-model-downloads")
```
์ด์ ํ๋ธ์ ์ฝ๋๊ฐ ์๊ฒผ์ต๋๋ค! ๋ง์ง๋ง ๋จ๊ณ์ธ ์์ด์ ํธ๊ฐ ์ฝ๋๋ฅผ ์ฌ์ฉํ๋๋ก ํ๋ ๋จ๊ณ๋ฅผ ์ดํด๋ณด๊ฒ ์ต๋๋ค.
#### ์์ด์ ํธ๊ฐ ๋๊ตฌ๋ฅผ ์ฌ์ฉํ๊ฒ ํ๊ธฐ[[Having-the-agent-use-the-tool]]
์ด์ ์ด๋ฐ ์์ผ๋ก ํ๋ธ์ ์กด์ฌํ๋ ๋๊ตฌ๋ฅผ ์ธ์คํด์คํํ ์ ์์ต๋๋ค(๋๊ตฌ์ ์ฌ์ฉ์ ์ด๋ฆ์ ๋ณ๊ฒฝํ์ธ์):
We now have our tool that lives on the Hub which can be instantiated as such (change the user name for your tool):
```python
from transformers import load_tool
tool = load_tool("lysandre/hf-model-downloads")
```
์ด ๋๊ตฌ๋ฅผ ์์ด์ ํธ์์ ์ฌ์ฉํ๋ ค๋ฉด ์์ด์ ํธ ์ด๊ธฐํ ๋ฉ์๋์ `additional_tools` ๋งค๊ฐ๋ณ์์ ์ ๋ฌํ๊ธฐ๋ง ํ๋ฉด ๋ฉ๋๋ค:
```python
from transformers import HfAgent
agent = HfAgent("https://api-inference.huggingface.co/models/bigcode/starcoder", additional_tools=[tool])
agent.run(
"Can you read out loud the name of the model that has the most downloads in the 'text-to-video' task on the Hugging Face Hub?"
)
```
๊ทธ๋ฌ๋ฉด ๋ค์๊ณผ ๊ฐ์ ๊ฒฐ๊ณผ๊ฐ ์ถ๋ ฅ๋ฉ๋๋ค:
```text
==Code generated by the agent==
model = model_download_counter(task="text-to-video")
print(f"The model with the most downloads is {model}.")
audio_model = text_reader(model)
==Result==
The model with the most downloads is damo-vilab/text-to-video-ms-1.7b.
```
and generates the following audio.
| **Audio** |
|------------------------------------------------------------------------------------------------------------------------------------------------------|
| <audio controls><source src="https://huggingface.co/datasets/huggingface/documentation-images/resolve/main/transformers/damo.wav" type="audio/wav"/> |
<Tip>
LLM์ ๋ฐ๋ผ ์ผ๋ถ๋ ๋งค์ฐ ์ทจ์ฝํ๊ธฐ ๋๋ฌธ์ ์ ๋๋ก ์๋ํ๋ ค๋ฉด ๋งค์ฐ ์ ํํ ํ๋กฌํํธ๊ฐ ํ์ํฉ๋๋ค.
์์ด์ ํธ๊ฐ ๋๊ตฌ๋ฅผ ์ ํ์ฉํ๊ธฐ ์ํด์๋ ๋๊ตฌ์ ์ด๋ฆ๊ณผ ์ค๋ช
์ ์ ์ ์ํ๋ ๊ฒ์ด ๋ฌด์๋ณด๋ค ์ค์ํฉ๋๋ค.
</Tip>
### ๊ธฐ์กด ๋๊ตฌ ๋์ฒดํ๊ธฐ[[replacing-existing-tools]]
์์ด์ ํธ์ ๋๊ตฌ ์์์ ์ ํญ๋ชฉ์ ๋ฐฐ์ ํ๊ธฐ๋ง ํ๋ฉด ๊ธฐ์กด ๋๊ตฌ๋ฅผ ๋์ฒดํ ์ ์์ต๋๋ค. ๋ฐฉ๋ฒ์ ๋ค์๊ณผ ๊ฐ์ต๋๋ค:
```python
from transformers import HfAgent, load_tool
agent = HfAgent("https://api-inference.huggingface.co/models/bigcode/starcoder")
agent.toolbox["image-transformation"] = load_tool("diffusers/controlnet-canny-tool")
```
<Tip>
๋ค๋ฅธ ๋๊ตฌ๋ก ๊ต์ฒดํ ๋๋ ์ฃผ์ํ์ธ์! ์ด ์์
์ผ๋ก ์์ด์ ํธ์ ํ๋กฌํํธ๋ ์กฐ์ ๋ฉ๋๋ค.
์์
์ ๋ ์ ํฉํ ํ๋กฌํํธ๊ฐ ์์ผ๋ฉด ์ข์ ์ ์์ง๋ง,
๋ค๋ฅธ ๋๊ตฌ๋ณด๋ค ๋ ๋ง์ด ์ ํ๋๊ฑฐ๋ ์ ์ํ ๋๊ตฌ ๋์ ๋ค๋ฅธ ๋๊ตฌ๊ฐ ์ ํ๋ ์๋ ์์ต๋๋ค.
</Tip>
## gradio-tools ์ฌ์ฉํ๊ธฐ[[leveraging-gradio-tools]]
[gradio-tools](https://github.com/freddyaboulton/gradio-tools)๋ Hugging Face Spaces๋ฅผ ๋๊ตฌ๋ก ์ฌ์ฉํ ์ ์๋ ๊ฐ๋ ฅํ ๋ผ์ด๋ธ๋ฌ๋ฆฌ์
๋๋ค.
๊ธฐ์กด์ ๋ง์ Spaces๋ฟ๋ง ์๋๋ผ ์ฌ์ฉ์ ์ ์ Spaces๋ฅผ ์ฌ์ฉํ์ฌ ๋์์ธํ ์ ์๋๋ก ์ง์ํฉ๋๋ค.
์ฐ๋ฆฌ๋ `Tool.from_gradio` ๋ฉ์๋๋ฅผ ์ฌ์ฉํ์ฌ `gradio_tools`์ ๋ํ ์ง์์ ์ ๊ณตํฉ๋๋ค.
์๋ฅผ ๋ค์ด, ํ๋กฌํํธ๋ฅผ ๊ฐ์ ํ๊ณ ๋ ๋์ ์ด๋ฏธ์ง๋ฅผ ์์ฑํ๊ธฐ ์ํด `gradio-tools` ํดํท์์ ์ ๊ณต๋๋ `StableDiffusionPromptGeneratorTool` ๋๊ตฌ๋ฅผ ํ์ฉํ๊ณ ์ ํฉ๋๋ค.
๋จผ์ `gradio_tools`์์ ๋๊ตฌ๋ฅผ ๊ฐ์ ธ์์ ์ธ์คํด์คํํฉ๋๋ค:
```python
from gradio_tools import StableDiffusionPromptGeneratorTool
gradio_tool = StableDiffusionPromptGeneratorTool()
```
ํด๋น ์ธ์คํด์ค๋ฅผ `Tool.from_gradio` ๋ฉ์๋์ ์ ๋ฌํฉ๋๋ค:
```python
from transformers import Tool
tool = Tool.from_gradio(gradio_tool)
```
์ด์ ์ผ๋ฐ์ ์ธ ์ฌ์ฉ์ ์ ์ ๋๊ตฌ์ ๋๊ฐ์ด ๊ด๋ฆฌํ ์ ์์ต๋๋ค.
์ด๋ฅผ ํ์ฉํ์ฌ `a rabbit wearing a space suit'(์ฐ์ฃผ๋ณต์ ์
์ ํ ๋ผ)๋ผ๋ ํ๋กฌํํธ๋ฅผ ๊ฐ์ ํ์ต๋๋ค:
```python
from transformers import HfAgent
agent = HfAgent("https://api-inference.huggingface.co/models/bigcode/starcoder", additional_tools=[tool])
agent.run("Generate an image of the `prompt` after improving it.", prompt="A rabbit wearing a space suit")
```
๋ชจ๋ธ์ด ๋๊ตฌ๋ฅผ ์ ์ ํ ํ์ฉํฉ๋๋ค:
```text
==Explanation from the agent==
I will use the following tools: `StableDiffusionPromptGenerator` to improve the prompt, then `image_generator` to generate an image according to the improved prompt.
==Code generated by the agent==
improved_prompt = StableDiffusionPromptGenerator(prompt)
print(f"The improved prompt is {improved_prompt}.")
image = image_generator(improved_prompt)
```
๋ง์ง๋ง์ผ๋ก ์ด๋ฏธ์ง๋ฅผ ์์ฑํ๊ธฐ ์ ์:
<img src="https://huggingface.co/datasets/huggingface/documentation-images/resolve/main/transformers/rabbit.png">
<Tip warning={true}>
gradio-tools๋ ๋ค๋ฅธ ๋ชจ๋ฌ๋ฆฌํฐ๋ก ์์
ํ ๋์๋ *ํ
์คํธ* ์
๋ ฅ ๋ฐ ์ถ๋ ฅ์ ํ์๋ก ํฉ๋๋ค.
์ด ๊ตฌํ์ ์ด๋ฏธ์ง ๋ฐ ์ค๋์ค ๊ฐ์ฒด์์ ์๋ํฉ๋๋ค.
ํ์ฌ๋ ์ด ๋ ๊ฐ์ง๊ฐ ํธํ๋์ง ์์ง๋ง ์ง์ ๊ฐ์ ์ ์ํด ๋
ธ๋ ฅํ๋ฉด์ ๋น ๋ฅด๊ฒ ํธํ๋ ๊ฒ์
๋๋ค.
</Tip>
## ํฅํ Langchain๊ณผ์ ํธํ์ฑ[[future-compatibility-with-langchain]]
์ ํฌ๋ Langchain์ ์ข์ํ๋ฉฐ ๋งค์ฐ ๋งค๋ ฅ์ ์ธ ๋๊ตฌ ๋ชจ์์ ๊ฐ์ง๊ณ ์๋ค๊ณ ์๊ฐํฉ๋๋ค.
์ด๋ฌํ ๋๊ตฌ๋ฅผ ์ฒ๋ฆฌํ๊ธฐ ์ํด Langchain์ ๋ค๋ฅธ ๋ชจ๋ฌ๋ฆฌํฐ์ ์์
ํ ๋์๋ *ํ
์คํธ* ์
๋ ฅ๊ณผ ์ถ๋ ฅ์ ํ์๋ก ํฉ๋๋ค.
์ด๋ ์ข
์ข
๊ฐ์ฒด์ ์ง๋ ฌํ๋(์ฆ, ๋์คํฌ์ ์ ์ฅ๋) ๋ฒ์ ์
๋๋ค.
์ด ์ฐจ์ด๋ก ์ธํด transformers-agents์ Langchain ๊ฐ์๋ ๋ฉํฐ ๋ชจ๋ฌ๋ฆฌํฐ๊ฐ ์ฒ๋ฆฌ๋์ง ์์ต๋๋ค.
ํฅํ ๋ฒ์ ์์ ์ด ์ ํ์ด ํด๊ฒฐ๋๊ธฐ๋ฅผ ๋ฐ๋ผ๋ฉฐ, ์ด ํธํ์ฑ์ ๋ฌ์ฑํ ์ ์๋๋ก ์ด๋ ฌํ Langchain ์ฌ์ฉ์์ ๋์์ ํ์ํฉ๋๋ค.
์ ํฌ๋ ๋ ๋์ ์ง์์ ์ ๊ณตํ๊ณ ์ ํฉ๋๋ค. ๋์์ ์ฃผ๊ณ ์ถ์ผ์๋ค๋ฉด, [์ด์๋ฅผ ์ด์ด](https://github.com/huggingface/transformers/issues/new) ์๊ฒฌ์ ๊ณต์ ํด ์ฃผ์ธ์.
| 0 |
hf_public_repos/transformers/docs/source | hf_public_repos/transformers/docs/source/ko/accelerate.md | <!--Copyright 2022 The HuggingFace Team. All rights reserved.
Licensed under the Apache License, Version 2.0 (the "License"); you may not use this file except in compliance with
the License. You may obtain a copy of the License at
http://www.apache.org/licenses/LICENSE-2.0
Unless required by applicable law or agreed to in writing, software distributed under the License is distributed on
an "AS IS" BASIS, WITHOUT WARRANTIES OR CONDITIONS OF ANY KIND, either express or implied. See the License for the
specific language governing permissions and limitations under the License.
โ ๏ธ Note that this file is in Markdown but contain specific syntax for our doc-builder (similar to MDX) that may not be
rendered properly in your Markdown viewer.
-->
# ๐ค Accelerate๋ฅผ ํ์ฉํ ๋ถ์ฐ ํ์ต[[distributed-training-with-accelerate]]
๋ชจ๋ธ์ด ์ปค์ง๋ฉด์ ๋ณ๋ ฌ ์ฒ๋ฆฌ๋ ์ ํ๋ ํ๋์จ์ด์์ ๋ ํฐ ๋ชจ๋ธ์ ํ๋ จํ๊ณ ํ๋ จ ์๋๋ฅผ ๋ช ๋ฐฐ๋ก ๊ฐ์ํํ๊ธฐ ์ํ ์ ๋ต์ผ๋ก ๋ฑ์ฅํ์ต๋๋ค. Hugging Face์์๋ ์ฌ์ฉ์๊ฐ ํ๋์ ๋จธ์ ์ ์ฌ๋ฌ ๊ฐ์ GPU๋ฅผ ์ฌ์ฉํ๋ ์ฌ๋ฌ ๋จธ์ ์ ์ฌ๋ฌ ๊ฐ์ GPU๋ฅผ ์ฌ์ฉํ๋ ๋ชจ๋ ์ ํ์ ๋ถ์ฐ ์ค์ ์์ ๐ค Transformers ๋ชจ๋ธ์ ์ฝ๊ฒ ํ๋ จํ ์ ์๋๋ก ๋๊ธฐ ์ํด [๐ค Accelerate](https://huggingface.co/docs/accelerate) ๋ผ์ด๋ธ๋ฌ๋ฆฌ๋ฅผ ๋ง๋ค์์ต๋๋ค. ์ด ํํ ๋ฆฌ์ผ์์๋ ๋ถ์ฐ ํ๊ฒฝ์์ ํ๋ จํ ์ ์๋๋ก ๊ธฐ๋ณธ PyTorch ํ๋ จ ๋ฃจํ๋ฅผ ์ปค์คํฐ๋ง์ด์ฆํ๋ ๋ฐฉ๋ฒ์ ์์๋ด
์๋ค.
## ์ค์ [[setup]]
๐ค Accelerate ์ค์น ์์ํ๊ธฐ:
```bash
pip install accelerate
```
๊ทธ ๋ค์, [`~accelerate.Accelerator`] ๊ฐ์ฒด๋ฅผ ๋ถ๋ฌ์ค๊ณ ์์ฑํฉ๋๋ค. [`~accelerate.Accelerator`]๋ ์๋์ผ๋ก ๋ถ์ฐ ์ค์ ์ ํ์ ๊ฐ์งํ๊ณ ํ๋ จ์ ํ์ํ ๋ชจ๋ ๊ตฌ์ฑ ์์๋ฅผ ์ด๊ธฐํํฉ๋๋ค. ์ฅ์น์ ๋ชจ๋ธ์ ๋ช
์์ ์ผ๋ก ๋ฐฐ์นํ ํ์๋ ์์ต๋๋ค.
```py
>>> from accelerate import Accelerator
>>> accelerator = Accelerator()
```
## ๊ฐ์ํ๋ฅผ ์ํ ์ค๋น[[prepare-to-accelerate]]
๋ค์ ๋จ๊ณ๋ ๊ด๋ จ๋ ๋ชจ๋ ํ๋ จ ๊ฐ์ฒด๋ฅผ [`~accelerate.Accelerator.prepare`] ๋ฉ์๋์ ์ ๋ฌํ๋ ๊ฒ์
๋๋ค. ์ฌ๊ธฐ์๋ ํ๋ จ ๋ฐ ํ๊ฐ ๋ฐ์ดํฐ๋ก๋, ๋ชจ๋ธ ๋ฐ ์ตํฐ๋ง์ด์ ๊ฐ ํฌํจ๋ฉ๋๋ค:
```py
>>> train_dataloader, eval_dataloader, model, optimizer = accelerator.prepare(
... train_dataloader, eval_dataloader, model, optimizer
... )
```
## ๋ฐฑ์๋(Backward)[[backward]]
๋ง์ง๋ง์ผ๋ก ํ๋ จ ๋ฃจํ์ ์ผ๋ฐ์ ์ธ `loss.backward()`๋ฅผ ๐ค Accelerate์ [`~accelerate.Accelerator.backward`] ๋ฉ์๋๋ก ๋์ฒดํ๊ธฐ๋ง ํ๋ฉด ๋ฉ๋๋ค:
```py
>>> for epoch in range(num_epochs):
... for batch in train_dataloader:
... outputs = model(**batch)
... loss = outputs.loss
... accelerator.backward(loss)
... optimizer.step()
... lr_scheduler.step()
... optimizer.zero_grad()
... progress_bar.update(1)
```
๋ค์ ์ฝ๋์์ ๋ณผ ์ ์๋ฏ์ด, ํ๋ จ ๋ฃจํ์ ์ฝ๋ ๋ค ์ค๋ง ์ถ๊ฐํ๋ฉด ๋ถ์ฐ ํ์ต์ ํ์ฑํํ ์ ์์ต๋๋ค!
```diff
+ from accelerate import Accelerator
from transformers import AdamW, AutoModelForSequenceClassification, get_scheduler
+ accelerator = Accelerator()
model = AutoModelForSequenceClassification.from_pretrained(checkpoint, num_labels=2)
optimizer = AdamW(model.parameters(), lr=3e-5)
- device = torch.device("cuda") if torch.cuda.is_available() else torch.device("cpu")
- model.to(device)
+ train_dataloader, eval_dataloader, model, optimizer = accelerator.prepare(
+ train_dataloader, eval_dataloader, model, optimizer
+ )
num_epochs = 3
num_training_steps = num_epochs * len(train_dataloader)
lr_scheduler = get_scheduler(
"linear",
optimizer=optimizer,
num_warmup_steps=0,
num_training_steps=num_training_steps
)
progress_bar = tqdm(range(num_training_steps))
model.train()
for epoch in range(num_epochs):
for batch in train_dataloader:
- batch = {k: v.to(device) for k, v in batch.items()}
outputs = model(**batch)
loss = outputs.loss
- loss.backward()
+ accelerator.backward(loss)
optimizer.step()
lr_scheduler.step()
optimizer.zero_grad()
progress_bar.update(1)
```
## ํ์ต[[train]]
๊ด๋ จ ์ฝ๋๋ฅผ ์ถ๊ฐํ ํ์๋ ์คํฌ๋ฆฝํธ๋ Colaboratory์ ๊ฐ์ ๋
ธํธ๋ถ์์ ํ๋ จ์ ์์ํ์ธ์.
### ์คํฌ๋ฆฝํธ๋ก ํ์ตํ๊ธฐ[[train-with-a-script]]
์คํฌ๋ฆฝํธ์์ ํ๋ จ์ ์คํํ๋ ๊ฒฝ์ฐ, ๋ค์ ๋ช
๋ น์ ์คํํ์ฌ ๊ตฌ์ฑ ํ์ผ์ ์์ฑํ๊ณ ์ ์ฅํฉ๋๋ค:
```bash
accelerate config
```
Then launch your training with:
```bash
accelerate launch train.py
```
### ๋
ธํธ๋ถ์ผ๋ก ํ์ตํ๊ธฐ[[train-with-a-notebook]]
Collaboratory์ TPU๋ฅผ ์ฌ์ฉํ๋ ค๋ ๊ฒฝ์ฐ, ๋
ธํธ๋ถ์์๋ ๐ค Accelerate๋ฅผ ์คํํ ์ ์์ต๋๋ค. ํ๋ จ์ ๋ด๋นํ๋ ๋ชจ๋ ์ฝ๋๋ฅผ ํจ์๋ก ๊ฐ์ธ์ [`~accelerate.notebook_launcher`]์ ์ ๋ฌํ์ธ์:
```py
>>> from accelerate import notebook_launcher
>>> notebook_launcher(training_function)
```
๐ค Accelerate ๋ฐ ๋ค์ํ ๊ธฐ๋ฅ์ ๋ํ ์์ธํ ๋ด์ฉ์ [documentation](https://huggingface.co/docs/accelerate)๋ฅผ ์ฐธ์กฐํ์ธ์. | 0 |
hf_public_repos/transformers/docs/source | hf_public_repos/transformers/docs/source/ko/perplexity.md | <!--Copyright 2020 The HuggingFace Team. All rights reserved.
Licensed under the Apache License, Version 2.0 (the "License"); you may not use this file except in compliance with
the License. You may obtain a copy of the License at
http://www.apache.org/licenses/LICENSE-2.0
Unless required by applicable law or agreed to in writing, software distributed under the License is distributed on
an "AS IS" BASIS, WITHOUT WARRANTIES OR CONDITIONS OF ANY KIND, either express or implied. See the License for the
specific language governing permissions and limitations under the License.
-->
# ๊ณ ์ ๊ธธ์ด ๋ชจ๋ธ์ ํํ๋ ์ํฐ(Perplexity)[[perplexity-of-fixedlength-models]]
[[open-in-colab]]
ํํ๋ ์ํฐ(Perplexity, PPL)๋ ๊ฐ์ฅ ์ผ๋ฐ์ ์ธ ์ธ์ด ๋ชจ๋ธ ํ๊ฐ์งํ ์ค ํ๋์
๋๋ค.
์์ธํ ์์๋ณด๊ธฐ ์ ์ ์ด ํ๊ฐ์งํ๋ ๊ณ ์ ์ ์ธ ์ธ์ด ๋ชจ๋ธ(์๊ธฐํ๊ท ๋๋ ์ธ๊ณผ์ ์ธ์ด ๋ชจ๋ธ์ด๋ผ๊ณ ๋ ํจ)์๋ง ์ ์ฉ๋๋ฉฐ BERT์ ๊ฐ์ ๋ง์คํน๋ ์ธ์ด ๋ชจ๋ธ์๋ ์ ์ ์ฉํ์ง ์์ต๋๋ค (BERT๋ [summary of the models](../en/model_summary) ๋ฌธ์๋ฅผ ์ฐธ๊ณ ํ์ธ์).
ํํ๋ ์ํฐ๋ ์ํ์ค์ ์์ ๋ก๊ทธ ์ฐ๋(negative log-likelihood, NLL) ๊ฐ์ ํ๊ท ์ ์ง์(exponentiate)๋ฅผ ์ทจํ ๊ฐ์ผ๋ก ์ ์๋ฉ๋๋ค.
ํ ํฐํ๋ ์ํ์ค \\(X = (x_0, x_1, \dots, x_t)\\) ๊ฐ ์์ ๋, \\(X\\) ์ ํํ๋ ์ํฐ๋ ์๋ ์์๊ณผ ๊ฐ์ด ๊ตฌํ ์ ์์ต๋๋ค.
$$\text{PPL}(X) = \exp \left\{ {-\frac{1}{t}\sum_i^t \log p_\theta (x_i|x_{<i}) } \right\}$$
\\(\log p_\theta (x_i|x_{<i})\\) ๋ ๋ชจ๋ธ์ i๋ฒ์งธ ์ด์ ๊น์ง ํ ํฐ์ด ์ฃผ์ด์ก์ ๋ i๋ฒ์งธ ํ ํฐ์ ๋ก๊ทธ ์ฐ๋๊ฐ์
๋๋ค.
์ง๊ด์ ์ผ๋ก ๋ง๋ญ์น์์ ์ง์ ๋ ํ ํฐ ์งํฉ์ ๊ท ์ผํ๊ฒ ์์ธกํ๋ ๋ชจ๋ธ์ ๋ฅ๋ ฅ์ ๋ํ ํ๊ฐ๋ก ์๊ฐํ ์ ์์ต๋๋ค.
์ค์ํ ์ ์ ํ ํฐํ ๊ณผ์ ์ด ๋ชจ๋ธ์ ํํ๋ ์ํฐ์ ์ง์ ์ ์ธ ์ํฅ์ ๋ฏธ์น๋ฏ๋ก ์๋ก ๋ค๋ฅธ ๋ชจ๋ธ์ ๋น๊ตํ ๋ ํญ์ ์ด๋ฅผ ๊ณ ๋ คํด์ผ ํฉ๋๋ค.
์ด๋ ๋ฐ์ดํฐ์ ๋ชจ๋ธ ์์ธก ๊ฐ์ cross-entropy ๊ฐ์ ์ง์๋ฅผ ์ทจํ ๊ฒ๊ณผ ๋์ผํฉ๋๋ค.
ํํ๋ ์ํฐ์ ๋ฌธ์๋น ๋นํธ ์(BPC) ๋ฐ ๋ฐ์ดํฐ ์์ถ๊ณผ์ ๊ด๊ณ์ ๋ํด ๋ ์ง๊ด์ ์ธ ์ดํด๋ฅผ ์ํ์ ๋ค๋ฉด ๋ค์ ๊ธ
[fantastic blog post on The Gradient](https://thegradient.pub/understanding-evaluation-metrics-for-language-models/)์ ํ์ธํ์ธ์.
## ๊ณ ์ ๊ธธ์ด ๋ชจ๋ธ์ ํํ๋ ์ํฐ(PPL) ๊ณ์ฐํ๊ธฐ[[calculating-ppl-with-fixedlength-models]]
๋ชจ๋ธ์ ์ปจํ
์คํธ ํฌ๊ธฐ๊ฐ ์ ํด์ ธ์์ง ์๋ค๋ฉด,
์๋์ ๊ฐ์ด ์ํ์ค๋ฅผ ์๋ ํ๊ท์ ์ผ๋ก ๋ถํดํ๊ณ ๊ฐ ๋จ๊ณ์์ ์ ํ ํ๋ ์ ์ฒด ์ํ์ค๋ฅผ ์กฐ๊ฑด๋ถ ํ๋ฅ ์ ๋ฃ์ด ๋ชจ๋ธ์ ํํ๋ ์ํฐ๋ฅผ ๊ณ์ฐํ ๊ฒ์
๋๋ค.
<img width="600" alt="Full decomposition of a sequence with unlimited context length" src="https://huggingface.co/datasets/huggingface/documentation-images/resolve/main/ppl_full.gif"/>
๊ทธ๋ฌ๋ ๋ชจ๋ธ์ ๊ทผ์ฌ์น๋ฅผ ๊ตฌํ ๋๋ ์ผ๋ฐ์ ์ผ๋ก ๋ชจ๋ธ์ด ์ฒ๋ฆฌํ ์ ์๋ ํ ํฐ ์์ ์ ํ์ด ์์ต๋๋ค.
์๋ฅผ ๋ค์ด, ๊ฐ์ฅ ํฐ ๋ฒ์ ์ [GPT-2](model_doc/gpt2)๋ ํ ํฐ์ ๊ธธ์ด๊ฐ 1024๋ก ๊ณ ์ ๋์ด ์์ต๋๋ค.
๋ฐ๋ผ์ \\(t\\) ๊ฐ 1024๋ณด๋ค ํฐ ๊ฒฝ์ฐ์ \\(p_\theta(x_t|x_{<t})\\) ์ ๊ณ์ฐํ ์ ์์ต๋๋ค.
๋์ ์ํ์ค๋ ์ผ๋ฐ์ ์ผ๋ก ๋ชจ๋ธ์ ์ต๋ ์
๋ ฅ ํฌ๊ธฐ์ ๋์ผํ ๊ธธ์ด๋ ๊ฐ์ง๋ ๋ถ๋ถ ์ํ์ค๋ก ์ชผ๊ฐญ๋๋ค.
๋ง์ฝ ๋ชจ๋ธ์ ์ต๋ ์
๋ ฅ ๊ธธ์ด๊ฐ \\(k\\) ๋ผ๋ฉด,
ํ ํฐ \\(x_t\\) ์ ์ฐ๋ ๊ฐ์ ๊ณ์ฐํ ๋ ์ด์ ํ ํฐ์ ๋ชจ๋ ์ฌ์ฉํ์ง ์๊ณ , \\(k-1\\) ํ ํฐ๊น์ง ์ฌ์ฉํด ๋๋ต์ ์ธ ์ฐ๋ ๊ฐ์ ์ถ์ ํฉ๋๋ค.
๋ชจ๋ธ์ ์ํ์ค์ ๋ํ ํํ๋ ์ํฐ๋ฅผ ๊ณ์ฐํ ๋,
์์ํ์ง๋ง ์ฐจ์ ์ฑ
์ ์ํ์ค๋ฅผ ์ฒญํฌ๋ก ์ชผ๊ฐ๊ณ ๋ถํด๋ ๊ฐ ๋ถ๋ถ์ ๋ก๊ทธ ์ฐ๋ ๊ฐ์ ๋
๋ฆฝ์ ์ผ๋ก ํฉ์ฐํ๋ ๊ฒ์
๋๋ค.
<img width="600" alt="Suboptimal PPL not taking advantage of full available context" src="https://huggingface.co/datasets/huggingface/documentation-images/resolve/main/ppl_chunked.gif"/>
์ด ๋ฐฉ๋ฒ์ ๊ฐ ๋ถ๋ถ์ ํํ๋ ์ํฐ๋ฅผ ํ ๋ฒ์ ํฌ์๋ ํจ์ค๋ก ๊ณ์ฐํ ์ ์์ด ๋น ๋ฅด์ง๋ง ์ผ๋ฐ์ ์ผ๋ก ๋ ๋์(๋ ๋์) PPL์ ์ฐ์ถํฉ๋๋ค.
์๋ํ๋ฉด ๋๋ถ๋ถ์ ์์ธก ๋จ๊ณ์์ ๋ชจ๋ธ์ ์ปจํ
์คํธ๊ฐ ์ ๊ธฐ ๋๋ฌธ์
๋๋ค.
๋์ , ๊ณ ์ ๊ธธ์ด ๋ชจ๋ธ์ PPL์ ์ฌ๋ผ์ด๋ฉ ์๋์ฐ ์ ๋ต์ผ๋ก ํ๊ฐํด์ผ ํฉ๋๋ค.
์ด ์ ๋ต์๋ ์ปจํ
์คํธ ์๋์ฐ์ ๋ฐ๋ณต์ ์ผ๋ก ์ฌ๋ผ์ด๋ฉํด ๋ชจ๋ธ์ด ๊ฐ ์์ธก์ ์ํํ ๋ ๋ ๋ง์ ์ปจํ
์คํธ๋ฅผ ๊ฐ๋๋ก ํ๋ ์์
์ด ํฌํจ๋ฉ๋๋ค.
<img width="600" alt="Sliding window PPL taking advantage of all available context" src="https://huggingface.co/datasets/huggingface/documentation-images/resolve/main/ppl_sliding.gif"/>
์ด๋ ์ํ์ค ํ๋ฅ ์ ์ค์ ๋ถํด์ ๋ ๊ฐ๊น์ด ๊ทผ์ฌ์น์ด๋ฉฐ ์ผ๋ฐ์ ์ผ๋ก ๋ ์ ๋ฆฌํ ์ ์๋ฅผ ์ฐ์ถํฉ๋๋ค.
๋จ์ ์ ๋ง๋ญ์น์ ๊ฐ ํ ํฐ์ ๋ํด ๋ณ๋์ ํฌ์๋ ํจ์ค๊ฐ ํ์ํ๋ค๋ ๊ฒ์
๋๋ค.
ํ์ค์ ์ผ๋ก ์ข์ ์ ์ถฉ์์ ํ ๋ฒ์ ํ ํ ํฐ์ฉ ์ฌ๋ผ์ด๋ฉํ๋ ๊ฒ์ด ์๋๋ผ ๋ ํฐ ๊ฐ๊ฒฉ์ผ๋ก ์ปจํ
์คํธ๋ฅผ ์ด๋ํ๋ ์คํธ๋ผ์ด๋๊ฐ ์ ์ฉ๋ ์ฌ๋ผ์ด๋ฉ ์๋์ฐ์ ์ฌ์ฉํ๋ ๊ฒ์
๋๋ค.
์ด๋ ๊ฒ ํ๋ฉด ๊ณ์ฐ์ ํจ์ฌ ๋ ๋น ๋ฅด๊ฒ ์งํํ๋ฉด์๋ ๋ชจ๋ธ์ ๊ฐ ๋จ๊ณ์์ ์์ธก์ ์ํํ ์ ์๋ ๊ธด ์ปจํ
์คํธ๋ฅผ ์ ๊ณตํ ์ ์์ต๋๋ค.
## ์์ : ๐ค Transformers์์ GPT-2๋ก ํํ๋ ์ํฐ(perplexity) ๊ณ์ฐํ๊ธฐ[[example-calculating-perplexity-with-gpt2-in-transformers]]
์ด์ GPT-2๋ก ์์ ๊ณผ์ ์ ์์ฐํด ๋ณด๊ฒ ์ต๋๋ค.
```python
from transformers import GPT2LMHeadModel, GPT2TokenizerFast
device = "cuda"
model_id = "gpt2-large"
model = GPT2LMHeadModel.from_pretrained(model_id).to(device)
tokenizer = GPT2TokenizerFast.from_pretrained(model_id)
```
WikiText-2 ๋ฐ์ดํฐ ์ธํธ๋ฅผ ๊ฐ์ ธ์ค๊ณ ๋ช ๊ฐ์ง ์ฌ๋ผ์ด๋ฉ ์๋์ฐ ์ ๋ต์ ์ฌ์ฉํด ํํ๋ ์ํฐ๋ฅผ ๊ณ์ฐํด๋ณด๊ฒ ์ต๋๋ค.
์ด ๋ฐ์ดํฐ ์ธํธ๋ ํฌ๊ธฐ๊ฐ ์๊ณ ํฌ์๋ ํจ์ค ํ ๋ฒ๋ง ์ํํ๊ธฐ ๋๋ฌธ์ ์ ์ฒด ๋ฐ์ดํฐ ์ธํธ๋ฅผ ๋ฉ๋ชจ๋ฆฌ์ ๊ฐ์ ธ์ค๊ณ ์ธ์ฝ๋ฉํ ์ ์์ต๋๋ค.
```python
from datasets import load_dataset
test = load_dataset("wikitext", "wikitext-2-raw-v1", split="test")
encodings = tokenizer("\n\n".join(test["text"]), return_tensors="pt")
```
๐ค Transformers๋ฅผ ์ฌ์ฉํ๋ฉด ๋ชจ๋ธ์ `labels`๋ก `input_ids`๋ฅผ ์ ๋ฌํด ๊ฐ ํ ํฐ์ ๋ํ ํ๊ท ์์ ์ฐ๋ ๊ฐ์ ์์ค๋ก ๋ฐํํ ์ ์์ต๋๋ค.
ํ์ง๋ง ์ฌ๋ผ์ด๋ฉ ์๋์ฐ ๋ฐฉ์์ ์ฌ์ฉํ๋ฉด ๊ฐ ๋ฐ๋ณต๋ง๋ค ๋ชจ๋ธ์ ์ ๋ฌํ๋ ํ ํฐ์ด ๊ฒน์นฉ๋๋ค.
์ปจํ
์คํธ๋ก ์ฒ๋ฆฌํ๋ ํ ํฐ์ ๋ํ ๋ก๊ทธ ์ฐ๋ ๊ฐ์ด ์์ค์ ํฌํจ๋๋ ๊ฒ์ ์ํ์ง ์๊ธฐ ๋๋ฌธ์ ์ด๋ฌํ ํ ํฐ์ `input_ids`๋ฅผ `-100`์ผ๋ก ์ค์ ํ์ฌ ๋ฌด์ํ ์ ์์ต๋๋ค.
๋ค์์ ์คํธ๋ผ์ด๋(stride)๋ฅผ `512`๋ก ์ฌ์ฉํ ์์์
๋๋ค.
์ฆ, ๋ชจ๋ธ์ด ํ ํ ํฐ์ ์กฐ๊ฑด๋ถ ์ฐ๋ ๊ฐ์ ๊ณ์ฐํ ๋ ์ปจํ
์คํธ์ ์ต์ํ 512๊ฐ์ ํ ํฐ์ด ํฌํจ๋์ด์๋ค๋ ์๋ฏธ์
๋๋ค (ํด๋น ํ ํฐ ์์ 512๊ฐ์ ํ ํฐ์ด ์๋ ๊ฒฝ์ฐ).
```python
import torch
from tqdm import tqdm
max_length = model.config.n_positions
stride = 512
seq_len = encodings.input_ids.size(1)
nlls = []
prev_end_loc = 0
for begin_loc in tqdm(range(0, seq_len, stride)):
end_loc = min(begin_loc + max_length, seq_len)
trg_len = end_loc - prev_end_loc # ๋ง์ง๋ง ๋ฃจํ์ ์คํธ๋ผ์ด๋ ๊ฐ๊ณผ ๋ค๋ฅผ ์ ์์
input_ids = encodings.input_ids[:, begin_loc:end_loc].to(device)
target_ids = input_ids.clone()
target_ids[:, :-trg_len] = -100
with torch.no_grad():
outputs = model(input_ids, labels=target_ids)
# ์์ค์ ๋ชจ๋ ์ ํจํ ๋ ์ด๋ธ์ ๋ํ ํ๊ท ๊ฐ์ ๊ตฌํ๋ ๊ต์ฐจ ์ํธ๋กํผ(cross entropy)๋ก ๊ณ์ฐ๋ฉ๋๋ค.
# ๋์ด๋ธ ๋ฒ ์ด์ง์ ๋ชจ๋ธ์ ๋ด๋ถ์ ์ผ๋ก ๋ ์ด๋ธ์ ์ผ์ชฝ์ผ๋ก 1๊ฐ์ฉ ๋ฐ๊ธฐ ๋๋ฌธ์, (ํ์ผ - 1)๊ฐ ๋งํผ์ ๋ ์ด๋ธ์ ๋ํด ์์ค์ ๊ณ์ฐํฉ๋๋ค.
neg_log_likelihood = outputs.loss
nlls.append(neg_log_likelihood)
prev_end_loc = end_loc
if end_loc == seq_len:
break
ppl = torch.exp(torch.stack(nlls).mean())
```
์คํธ๋ผ์ด๋๋ฅผ ์ต๋ ์
๋ ฅ ๊ธธ์ด์ ๋์ผํ๊ฒ ์ค์ ํ๋ฉด ์์์ ์ค๋ช
ํ ์ฐจ์ ์ฑ
์ธ ๋น์ฌ๋ผ์ด๋ฉ ์๋์ฐ ์ ๋ต๊ณผ ๋์ผํฉ๋๋ค.
์ผ๋ฐ์ ์ผ๋ก ์คํธ๋ผ์ด๋๊ฐ ์์์๋ก ๋ชจ๋ธ์ด ๊ฐ ์์ธก์ ํ ๋ ๋ ๋ง์ ์ปจํ
์คํธ๋ฅผ ๋ณผ ์ ์๊ฒ ๋์ด ํํ๋ ์ํฐ ๊ฐ์ด ์ข์์ง๋๋ค.
์์ ๊ณ์ฐ์ ํ ํฐ์ด ๊ฒน์น์ง ์๋๋ก `stride = 1024`๋ก ์ค์ ํ๋ฉด PPL์ `19.44`๋ก GPT-2 ๋
ผ๋ฌธ์์ ๋ณด๊ณ ๋ `19.93`๊ณผ ๊ฑฐ์ ๋์ผํฉ๋๋ค.
`stride = 512`๋ก ์ฌ๋ผ์ด๋ฉ ์๋์ฐ ์ ๋ต์ ์ฌ์ฉํ๋ฉด PPL์ `16.45`๋ก ๋จ์ด์ง๋๋ค.
์ด๋ ๋ ์ข์ ์ ์์ผ ๋ฟ๋ง ์๋๋ผ ์ํ์ค ํ๋ฅ ์ ์ค์ ์๋ ํ๊ท ๋ถํด์ ๋ ๊ฐ๊น์ด ๋ฐฉ์์ผ๋ก ๊ณ์ฐ๋ฉ๋๋ค. | 0 |
hf_public_repos/transformers/docs/source | hf_public_repos/transformers/docs/source/ko/training.md | <!--Copyright 2022 The HuggingFace Team. All rights reserved.
Licensed under the Apache License, Version 2.0 (the "License"); you may not use this file except in compliance with
the License. You may obtain a copy of the License at
http://www.apache.org/licenses/LICENSE-2.0
Unless required by applicable law or agreed to in writing, software distributed under the License is distributed on
an "AS IS" BASIS, WITHOUT WARRANTIES OR CONDITIONS OF ANY KIND, either express or implied. See the License for the
specific language governing permissions and limitations under the License.
โ ๏ธ Note that this file is in Markdown but contain specific syntax for our doc-builder (similar to MDX) that may not be
rendered properly in your Markdown viewer.
-->
# ์ฌ์ ํ์ต๋ ๋ชจ๋ธ ๋ฏธ์ธ ํ๋ํ๊ธฐ[[finetune-a-pretrained-model]]
[[open-in-colab]]
์ฌ์ ํ์ต๋ ๋ชจ๋ธ์ ์ฌ์ฉํ๋ฉด ์๋นํ ์ด์ ์ด ์์ต๋๋ค. ๊ณ์ฐ ๋น์ฉ๊ณผ ํ์๋ฐ์๊ตญ์ ์ค์ด๊ณ , ์ฒ์๋ถํฐ ๋ชจ๋ธ์ ํ์ต์ํฌ ํ์ ์์ด ์ต์ ๋ชจ๋ธ์ ์ฌ์ฉํ ์ ์์ต๋๋ค. ๐ค Transformers๋ ๋ค์ํ ์์
์ ์ํด ์ฌ์ ํ์ต๋ ์์ฒ ๊ฐ์ ๋ชจ๋ธ์ ์ก์ธ์คํ ์ ์์ต๋๋ค. ์ฌ์ ํ์ต๋ ๋ชจ๋ธ์ ์ฌ์ฉํ๋ ๊ฒฝ์ฐ, ์์ ์ ์์
๊ณผ ๊ด๋ จ๋ ๋ฐ์ดํฐ์
์ ์ฌ์ฉํด ํ์ตํฉ๋๋ค. ์ด๊ฒ์ ๋ฏธ์ธ ํ๋์ด๋ผ๊ณ ํ๋ ๋งค์ฐ ๊ฐ๋ ฅํ ํ๋ จ ๊ธฐ๋ฒ์
๋๋ค. ์ด ํํ ๋ฆฌ์ผ์์๋ ๋น์ ์ด ์ ํํ ๋ฅ๋ฌ๋ ํ๋ ์์ํฌ๋ก ์ฌ์ ํ์ต๋ ๋ชจ๋ธ์ ๋ฏธ์ธ ํ๋ํฉ๋๋ค:
* ๐ค Transformers๋ก ์ฌ์ ํ์ต๋ ๋ชจ๋ธ ๋ฏธ์ธ ํ๋ํ๊ธฐ [`Trainer`].
* Keras๋ฅผ ์ฌ์ฉํ์ฌ TensorFlow์์ ์ฌ์ ํ์ต๋ ๋ชจ๋ธ์ ๋ฏธ์ธ ํ๋ํ๊ธฐ.
* ๊ธฐ๋ณธ PyTorch์์ ์ฌ์ ํ์ต๋ ๋ชจ๋ธ์ ๋ฏธ์ธ ํ๋ํ๊ธฐ.
<a id='data-processing'></a>
## ๋ฐ์ดํฐ์
์ค๋น[[prepare-a-dataset]]
<Youtube id="_BZearw7f0w"/>
์ฌ์ ํ์ต๋ ๋ชจ๋ธ์ ๋ฏธ์ธ ํ๋ํ๊ธฐ ์ํด์ ๋ฐ์ดํฐ์
์ ๋ค์ด๋ก๋ํ๊ณ ํ๋ จํ ์ ์๋๋ก ์ค๋นํ์ธ์. ์ด์ ํํ ๋ฆฌ์ผ์์ ํ๋ จ์ ์ํด ๋ฐ์ดํฐ๋ฅผ ์ฒ๋ฆฌํ๋ ๋ฐฉ๋ฒ์ ๋ณด์ฌ๋๋ ธ๋๋ฐ, ์ง๊ธ์ด ๋ฐฐ์ธ ๊ฑธ ๋์ง์ ๊ธฐํ์
๋๋ค!
๋จผ์ [Yelp ๋ฆฌ๋ทฐ](https://huggingface.co/datasets/yelp_review_full) ๋ฐ์ดํฐ ์ธํธ๋ฅผ ๋ก๋ํฉ๋๋ค:
```py
>>> from datasets import load_dataset
>>> dataset = load_dataset("yelp_review_full")
>>> dataset["train"][100]
{'label': 0,
'text': 'My expectations for McDonalds are t rarely high. But for one to still fail so spectacularly...that takes something special!\\nThe cashier took my friends\'s order, then promptly ignored me. I had to force myself in front of a cashier who opened his register to wait on the person BEHIND me. I waited over five minutes for a gigantic order that included precisely one kid\'s meal. After watching two people who ordered after me be handed their food, I asked where mine was. The manager started yelling at the cashiers for \\"serving off their orders\\" when they didn\'t have their food. But neither cashier was anywhere near those controls, and the manager was the one serving food to customers and clearing the boards.\\nThe manager was rude when giving me my order. She didn\'t make sure that I had everything ON MY RECEIPT, and never even had the decency to apologize that I felt I was getting poor service.\\nI\'ve eaten at various McDonalds restaurants for over 30 years. I\'ve worked at more than one location. I expect bad days, bad moods, and the occasional mistake. But I have yet to have a decent experience at this store. It will remain a place I avoid unless someone in my party needs to avoid illness from low blood sugar. Perhaps I should go back to the racially biased service of Steak n Shake instead!'}
```
ํ
์คํธ๋ฅผ ์ฒ๋ฆฌํ๊ณ ์๋ก ๋ค๋ฅธ ๊ธธ์ด์ ์ํ์ค ํจ๋ฉ ๋ฐ ์๋ผ๋ด๊ธฐ ์ ๋ต์ ํฌํจํ๋ ค๋ฉด ํ ํฌ๋์ด์ ๊ฐ ํ์ํฉ๋๋ค. ๋ฐ์ดํฐ์
์ ํ ๋ฒ์ ์ฒ๋ฆฌํ๋ ค๋ฉด ๐ค Dataset [`map`](https://huggingface.co/docs/datasets/process.html#map) ๋ฉ์๋๋ฅผ ์ฌ์ฉํ์ฌ ์ ์ฒด ๋ฐ์ดํฐ์
์ ์ ์ฒ๋ฆฌ ํจ์๋ฅผ ์ ์ฉํ์ธ์:
```py
>>> from transformers import AutoTokenizer
>>> tokenizer = AutoTokenizer.from_pretrained("bert-base-cased")
>>> def tokenize_function(examples):
... return tokenizer(examples["text"], padding="max_length", truncation=True)
>>> tokenized_datasets = dataset.map(tokenize_function, batched=True)
```
ํ์ํ ๊ฒฝ์ฐ ๋ฏธ์ธ ํ๋์ ์ํด ๋ฐ์ดํฐ์
์ ์์ ๋ถ๋ถ ์งํฉ์ ๋ง๋ค์ด ๋ฏธ์ธ ํ๋ ์์
์๊ฐ์ ์ค์ผ ์ ์์ต๋๋ค:
```py
>>> small_train_dataset = tokenized_datasets["train"].shuffle(seed=42).select(range(1000))
>>> small_eval_dataset = tokenized_datasets["test"].shuffle(seed=42).select(range(1000))
```
<a id='trainer'></a>
## Train
์ฌ๊ธฐ์๋ถํฐ๋ ์ฌ์ฉํ๋ ค๋ ํ๋ ์์ํฌ์ ํด๋นํ๋ ์น์
์ ๋ฐ๋ผ์ผ ํฉ๋๋ค. ์ค๋ฅธ์ชฝ ์ฌ์ด๋๋ฐ์ ๋งํฌ๋ฅผ ์ฌ์ฉํ์ฌ ์ํ๋ ํ๋ ์์ํฌ๋ก ์ด๋ํ ์ ์์ผ๋ฉฐ, ํน์ ํ๋ ์์ํฌ์ ๋ชจ๋ ์ฝํ
์ธ ๋ฅผ ์จ๊ธฐ๋ ค๋ฉด ํด๋น ํ๋ ์์ํฌ ๋ธ๋ก์ ์ค๋ฅธ์ชฝ ์๋จ์ ์๋ ๋ฒํผ์ ์ฌ์ฉํ๋ฉด ๋ฉ๋๋ค!
<frameworkcontent>
<pt>
<Youtube id="nvBXf7s7vTI"/>
## ํ์ดํ ์น Trainer๋ก ํ๋ จํ๊ธฐ[[train-with-pytorch-trainer]]
๐ค Transformers๋ ๐ค Transformers ๋ชจ๋ธ ํ๋ จ์ ์ต์ ํ๋ [`Trainer`] ํด๋์ค๋ฅผ ์ ๊ณตํ์ฌ ํ๋ จ ๋ฃจํ๋ฅผ ์ง์ ์์ฑํ์ง ์๊ณ ๋ ์ฝ๊ฒ ํ๋ จ์ ์์ํ ์ ์์ต๋๋ค. [`Trainer`] API๋ ๋ก๊น
(logging), ๊ฒฝ์ฌ ๋์ (gradient accumulation), ํผํฉ ์ ๋ฐ๋(mixed precision) ๋ฑ ๋ค์ํ ํ๋ จ ์ต์
๊ณผ ๊ธฐ๋ฅ์ ์ง์ํฉ๋๋ค.
๋จผ์ ๋ชจ๋ธ์ ๊ฐ์ ธ์ค๊ณ ์์๋๋ ๋ ์ด๋ธ ์๋ฅผ ์ง์ ํฉ๋๋ค. Yelp ๋ฆฌ๋ทฐ [๋ฐ์ดํฐ์
์นด๋](https://huggingface.co/datasets/yelp_review_full#data-fields)์์ 5๊ฐ์ ๋ ์ด๋ธ์ด ์์์ ์ ์ ์์ต๋๋ค:
```py
>>> from transformers import AutoModelForSequenceClassification
>>> model = AutoModelForSequenceClassification.from_pretrained("bert-base-cased", num_labels=5)
```
<Tip>
์ฌ์ ํ๋ จ๋ ๊ฐ์ค์น ์ค ์ผ๋ถ๊ฐ ์ฌ์ฉ๋์ง ์๊ณ ์ผ๋ถ ๊ฐ์ค์น๊ฐ ๋ฌด์์๋ก ํ์๋๋ค๋ ๊ฒฝ๊ณ ๊ฐ ํ์๋ฉ๋๋ค.
๊ฑฑ์ ๋ง์ธ์. ์ด๊ฒ์ ์ฌ๋ฐ๋ฅธ ๋์์
๋๋ค! ์ฌ์ ํ์ต๋ BERT ๋ชจ๋ธ์ ํค๋๋ ํ๊ธฐ๋๊ณ ๋ฌด์์๋ก ์ด๊ธฐํ๋ ๋ถ๋ฅ ํค๋๋ก ๋์ฒด๋ฉ๋๋ค. ์ด์ ์ฌ์ ํ์ต๋ ๋ชจ๋ธ์ ์ง์์ผ๋ก ์ํ์ค ๋ถ๋ฅ ์์
์ ์ํ ์๋ก์ด ๋ชจ๋ธ ํค๋๋ฅผ ๋ฏธ์ธ ํ๋ ํฉ๋๋ค.
</Tip>
### ํ์ดํผํ๋ผ๋ฏธํฐ ํ๋ จ[[training-hyperparameters]]
๋ค์์ผ๋ก ์ ํ ์ ์๋ ๋ชจ๋ ํ์ดํผํ๋ผ๋ฏธํฐ์ ๋ค์ํ ํ๋ จ ์ต์
์ ํ์ฑํํ๊ธฐ ์ํ ํ๋๊ทธ๋ฅผ ํฌํจํ๋ [`TrainingArguments`] ํด๋์ค๋ฅผ ์์ฑํฉ๋๋ค.
์ด ํํ ๋ฆฌ์ผ์์๋ ๊ธฐ๋ณธ ํ๋ จ [ํ์ดํผํ๋ผ๋ฏธํฐ](https://huggingface.co/docs/transformers/main_classes/trainer#transformers.TrainingArguments)๋ก ์์ํ์ง๋ง, ์์ ๋กญ๊ฒ ์คํํ์ฌ ์ฌ๋ฌ๋ถ๋ค์๊ฒ ๋ง๋ ์ต์ ์ ์ค์ ์ ์ฐพ์ ์ ์์ต๋๋ค.
ํ๋ จ์์ ์ฒดํฌํฌ์ธํธ(checkpoints)๋ฅผ ์ ์ฅํ ์์น๋ฅผ ์ง์ ํฉ๋๋ค:
```py
>>> from transformers import TrainingArguments
>>> training_args = TrainingArguments(output_dir="test_trainer")
```
### ํ๊ฐ ํ๊ธฐ[[evaluate]]
[`Trainer`]๋ ํ๋ จ ์ค์ ๋ชจ๋ธ ์ฑ๋ฅ์ ์๋์ผ๋ก ํ๊ฐํ์ง ์์ต๋๋ค. ํ๊ฐ ์งํ๋ฅผ ๊ณ์ฐํ๊ณ ๋ณด๊ณ ํ ํจ์๋ฅผ [`Trainer`]์ ์ ๋ฌํด์ผ ํฉ๋๋ค.
[๐ค Evaluate](https://huggingface.co/docs/evaluate/index) ๋ผ์ด๋ธ๋ฌ๋ฆฌ๋ [`evaluate.load`](https://huggingface.co/spaces/evaluate-metric/accuracy) ํจ์๋ก ๋ก๋ํ ์ ์๋ ๊ฐ๋จํ [`accuracy`]ํจ์๋ฅผ ์ ๊ณตํฉ๋๋ค (์์ธํ ๋ด์ฉ์ [๋๋ฌ๋ณด๊ธฐ](https://huggingface.co/docs/evaluate/a_quick_tour)๋ฅผ ์ฐธ์กฐํ์ธ์):
```py
>>> import numpy as np
>>> import evaluate
>>> metric = evaluate.load("accuracy")
```
`metric`์์ [`~evaluate.compute`]๋ฅผ ํธ์ถํ์ฌ ์์ธก์ ์ ํ๋๋ฅผ ๊ณ์ฐํฉ๋๋ค. ์์ธก์ `compute`์ ์ ๋ฌํ๊ธฐ ์ ์ ์์ธก์ ๋ก์ง์ผ๋ก ๋ณํํด์ผ ํฉ๋๋ค(๋ชจ๋ ๐ค Transformers ๋ชจ๋ธ์ ๋ก์ง์ผ๋ก ๋ฐํํ๋ค๋ ์ ์ ๊ธฐ์ตํ์ธ์):
```py
>>> def compute_metrics(eval_pred):
... logits, labels = eval_pred
... predictions = np.argmax(logits, axis=-1)
... return metric.compute(predictions=predictions, references=labels)
```
๋ฏธ์ธ ํ๋ ์ค์ ํ๊ฐ ์งํ๋ฅผ ๋ชจ๋ํฐ๋งํ๋ ค๋ฉด ํ๋ จ ์ธ์์ `evaluation_strategy` ํ๋ผ๋ฏธํฐ๋ฅผ ์ง์ ํ์ฌ ๊ฐ ์ํญ์ด ๋๋ ๋ ํ๊ฐ ์งํ๋ฅผ ํ์ธํ ์ ์์ต๋๋ค:
```py
>>> from transformers import TrainingArguments, Trainer
>>> training_args = TrainingArguments(output_dir="test_trainer", evaluation_strategy="epoch")
```
### ํ๋ จ ํ๊ธฐ[[trainer]]
๋ชจ๋ธ, ํ๋ จ ์ธ์, ํ๋ จ ๋ฐ ํ
์คํธ ๋ฐ์ดํฐ์
, ํ๊ฐ ํจ์๊ฐ ํฌํจ๋ [`Trainer`] ๊ฐ์ฒด๋ฅผ ๋ง๋ญ๋๋ค:
```py
>>> trainer = Trainer(
... model=model,
... args=training_args,
... train_dataset=small_train_dataset,
... eval_dataset=small_eval_dataset,
... compute_metrics=compute_metrics,
... )
```
๊ทธ๋ฆฌ๊ณ [`~transformers.Trainer.train`]์ ํธ์ถํ์ฌ ๋ชจ๋ธ์ ๋ฏธ์ธ ํ๋ํฉ๋๋ค:
```py
>>> trainer.train()
```
</pt>
<tf>
<a id='keras'></a>
<Youtube id="rnTGBy2ax1c"/>
## Keras๋ก ํ
์ํ๋ก์ฐ ๋ชจ๋ธ ํ๋ จํ๊ธฐ[[train-a-tensorflow-model-with-keras]]
Keras API๋ฅผ ์ฌ์ฉํ์ฌ ํ
์ํ๋ก์ฐ์์ ๐ค Transformers ๋ชจ๋ธ์ ํ๋ จํ ์๋ ์์ต๋๋ค!
### Keras์ฉ ๋ฐ์ดํฐ ๋ก๋[[loading-data-for-keras]]
Keras API๋ก ๐ค Transformers ๋ชจ๋ธ์ ํ์ต์ํค๋ ค๋ฉด ๋ฐ์ดํฐ์
์ Keras๊ฐ ์ดํดํ ์ ์๋ ํ์์ผ๋ก ๋ณํํด์ผ ํฉ๋๋ค.
๋ฐ์ดํฐ ์ธํธ๊ฐ ์์ ๊ฒฝ์ฐ, ์ ์ฒด๋ฅผ NumPy ๋ฐฐ์ด๋ก ๋ณํํ์ฌ Keras๋ก ์ ๋ฌํ๋ฉด ๋ฉ๋๋ค.
๋ ๋ณต์กํ ์์
์ ์ํํ๊ธฐ ์ ์ ๋จผ์ ์ด ์์
์ ์๋ํด ๋ณด๊ฒ ์ต๋๋ค.
๋จผ์ ๋ฐ์ดํฐ ์ธํธ๋ฅผ ๋ก๋ํฉ๋๋ค. [GLUE ๋ฒค์น๋งํฌ](https://huggingface.co/datasets/glue)์ CoLA ๋ฐ์ดํฐ ์ธํธ๋ฅผ ์ฌ์ฉํ๊ฒ ์ต๋๋ค.
๊ฐ๋จํ ๋ฐ์ด๋๋ฆฌ ํ
์คํธ ๋ถ๋ฅ ์์
์ด๋ฏ๋ก ์ง๊ธ์ ํ๋ จ ๋ฐ์ดํฐ ๋ถํ ๋ง ์ฌ์ฉํฉ๋๋ค.
```py
from datasets import load_dataset
dataset = load_dataset("glue", "cola")
dataset = dataset["train"] # Just take the training split for now
```
๋ค์์ผ๋ก ํ ํฌ๋์ด์ ๋ฅผ ๋ก๋ํ๊ณ ๋ฐ์ดํฐ๋ฅผ NumPy ๋ฐฐ์ด๋ก ํ ํฐํํฉ๋๋ค. ๋ ์ด๋ธ์ ์ด๋ฏธ 0๊ณผ 1๋ก ๋ ๋ฆฌ์คํธ์ด๊ธฐ ๋๋ฌธ์ ํ ํฐํํ์ง ์๊ณ ๋ฐ๋ก NumPy ๋ฐฐ์ด๋ก ๋ณํํ ์ ์์ต๋๋ค!
```py
from transformers import AutoTokenizer
tokenizer = AutoTokenizer.from_pretrained("bert-base-cased")
tokenized_data = tokenizer(dataset["sentence"], return_tensors="np", padding=True)
# Tokenizer returns a BatchEncoding, but we convert that to a dict for Keras
tokenized_data = dict(tokenized_data)
labels = np.array(dataset["label"]) # Label is already an array of 0 and 1
```
๋ง์ง๋ง์ผ๋ก ๋ชจ๋ธ์ ๋ก๋, [`compile`](https://keras.io/api/models/model_training_apis/#compile-method), [`fit`](https://keras.io/api/models/model_training_apis/#fit-method)ํฉ๋๋ค:
```py
from transformers import TFAutoModelForSequenceClassification
from tensorflow.keras.optimizers import Adam
# Load and compile our model
model = TFAutoModelForSequenceClassification.from_pretrained("bert-base-cased")
# Lower learning rates are often better for fine-tuning transformers
model.compile(optimizer=Adam(3e-5))
model.fit(tokenized_data, labels)
```
<Tip>
๋ชจ๋ธ์ `compile()`ํ ๋ ์์ค ์ธ์๋ฅผ ๋ชจ๋ธ์ ์ ๋ฌํ ํ์๊ฐ ์์ต๋๋ค!
์ด ์ธ์๋ฅผ ๋น์๋๋ฉด ํ๊น
ํ์ด์ค ๋ชจ๋ธ์ ์์
๊ณผ ๋ชจ๋ธ ์ํคํ
์ฒ์ ์ ํฉํ ์์ค์ ์๋์ผ๋ก ์ ํํฉ๋๋ค.
์ํ๋ค๋ฉด ์ธ์ ๋ ์ง ์ง์ ์์ค์ ์ง์ ํ์ฌ ์ด๋ฅผ ์ฌ์ ์ํ ์ ์์ต๋๋ค!
</Tip>
์ด ์ ๊ทผ ๋ฐฉ์์ ์๊ท๋ชจ ๋ฐ์ดํฐ ์งํฉ์์๋ ์ ์๋ํ์ง๋ง, ๋๊ท๋ชจ ๋ฐ์ดํฐ ์งํฉ์์๋ ๋ฌธ์ ๊ฐ ๋ ์ ์์ต๋๋ค. ์ ๊ทธ๋ด๊น์?
ํ ํฐํ๋ ๋ฐฐ์ด๊ณผ ๋ ์ด๋ธ์ ๋ฉ๋ชจ๋ฆฌ์ ์์ ํ ๋ก๋ํ๊ณ NumPy๋ "๋ค์ญ๋ ์ญํ" ๋ฐฐ์ด์ ์ฒ๋ฆฌํ์ง ์๊ธฐ ๋๋ฌธ์,
๋ชจ๋ ํ ํฐํ๋ ์ํ์ ์ ์ฒด ๋ฐ์ดํฐ์
์์ ๊ฐ์ฅ ๊ธด ์ํ์ ๊ธธ์ด๋งํผ ํจ๋ฉํด์ผ ํฉ๋๋ค. ์ด๋ ๊ฒ ํ๋ฉด ๋ฐฐ์ด์ด ํจ์ฌ ๋ ์ปค์ง๊ณ ์ด ํจ๋ฉ ํ ํฐ์ผ๋ก ์ธํด ํ์ต ์๋๋ ๋๋ ค์ง๋๋ค!
### ๋ฐ์ดํฐ๋ฅผ tf.data.Dataset์ผ๋ก ๋ก๋ํ๊ธฐ[[loading-data-as-a-tfdatadataset]]
ํ์ต ์๋๊ฐ ๋๋ ค์ง๋ ๊ฒ์ ํผํ๋ ค๋ฉด ๋ฐ์ดํฐ๋ฅผ `tf.data.Dataset`์ผ๋ก ๋ก๋ํ ์ ์์ต๋๋ค. ์ํ๋ค๋ฉด ์ง์
`tf.data` ํ์ดํ๋ผ์ธ์ ์ง์ ์์ฑํ ์๋ ์์ง๋ง, ์ด ์์
์ ๊ฐํธํ๊ฒ ์ํํ๋ ์ ์๋ ๋ ๊ฐ์ง ๋ฐฉ๋ฒ์ด ์์ต๋๋ค:
- [`~TFPreTrainedModel.prepare_tf_dataset`]: ๋๋ถ๋ถ์ ๊ฒฝ์ฐ ์ด ๋ฐฉ๋ฒ์ ๊ถ์ฅํฉ๋๋ค. ๋ชจ๋ธ์ ๋ฉ์๋์ด๊ธฐ ๋๋ฌธ์ ๋ชจ๋ธ์ ๊ฒ์ฌํ์ฌ ๋ชจ๋ธ ์
๋ ฅ์ผ๋ก ์ฌ์ฉํ ์ ์๋ ์ด์ ์๋์ผ๋ก ํ์
ํ๊ณ
๋๋จธ์ง๋ ๋ฒ๋ ค์ ๋ ๋จ์ํ๊ณ ์ฑ๋ฅ์ด ์ข์ ๋ฐ์ดํฐ ์งํฉ์ ๋ง๋ค ์ ์์ต๋๋ค.
- [`~datasets.Dataset.to_tf_dataset`]: ์ด ๋ฐฉ๋ฒ์ ์ข ๋ ๋ฎ์ ์์ค์ด๋ฉฐ, ํฌํจํ '์ด'๊ณผ '๋ ์ด๋ธ'์ ์ ํํ ์ง์ ํ์ฌ
๋ฐ์ดํฐ์
์ ์์ฑํ๋ ๋ฐฉ๋ฒ์ ์ ํํ ์ ์ดํ๊ณ ์ถ์ ๋ ์ ์ฉํ๋ฉฐ, ํฌํจํ 'columns'๊ณผ 'label_cols'์ ์ ํํ ์ง์ ํ ์ ์์ต๋๋ค.
[`~TFPreTrainedModel.prepare_tf_dataset`]์ ์ฌ์ฉํ๋ ค๋ฉด ๋จผ์ ๋ค์ ์ฝ๋ ์ํ๊ณผ ๊ฐ์ด ํ ํฌ๋์ด์ ์ถ๋ ฅ์ ๋ฐ์ดํฐ ์ธํธ์ ์ด๋ก ์ถ๊ฐํด์ผ ํฉ๋๋ค:
```py
def tokenize_dataset(data):
# Keys of the returned dictionary will be added to the dataset as columns
return tokenizer(data["text"])
dataset = dataset.map(tokenize_dataset)
```
ํ๊น
ํ์ด์ค ๋ฐ์ดํฐ์
์ ๊ธฐ๋ณธ์ ์ผ๋ก ๋์คํฌ์ ์ ์ฅ๋๋ฏ๋ก ๋ฉ๋ชจ๋ฆฌ ์ฌ์ฉ๋์ ๋๋ฆฌ์ง ์๋๋ค๋ ์ ์ ๊ธฐ์ตํ์ธ์!
์ด์ด ์ถ๊ฐ๋๋ฉด ๋ฐ์ดํฐ์
์์ ๋ฐฐ์น๋ฅผ ์คํธ๋ฆฌ๋ฐํ๊ณ ๊ฐ ๋ฐฐ์น์ ํจ๋ฉ์ ์ถ๊ฐํ ์ ์์ผ๋ฏ๋ก ์ ์ฒด ๋ฐ์ดํฐ์
์ ํจ๋ฉ์ ์ถ๊ฐํ๋ ๊ฒ๋ณด๋ค ํจ๋ฉ ํ ํฐ์ ์๋ฅผ ํฌ๊ฒ ์ค์ผ ์ ์์ต๋๋ค.
```py
>>> tf_dataset = model.prepare_tf_dataset(dataset, batch_size=16, shuffle=True, tokenizer=tokenizer)
```
์์ ์ฝ๋ ์ํ์์๋ ๋ฐฐ์น๊ฐ ๋ก๋๋ ๋ ์ฌ๋ฐ๋ฅด๊ฒ ํจ๋ฉํ ์ ์๋๋ก `prepare_tf_dataset`์ ํ ํฌ๋์ด์ ๋ฅผ ์ ๋ฌํด์ผ ํฉ๋๋ค.
๋ฐ์ดํฐ์
์ ๋ชจ๋ ์ํ ๊ธธ์ด๊ฐ ๊ฐ๊ณ ํจ๋ฉ์ด ํ์ํ์ง ์์ ๊ฒฝ์ฐ ์ด ์ธ์๋ฅผ ๊ฑด๋๋ธ ์ ์์ต๋๋ค.
์ํ์ ์ฑ์ฐ๋ ๊ฒ๋ณด๋ค ๋ ๋ณต์กํ ์์
(์: ๋ง์คํน๋ ์ธ์ด์ ํ ํฐ ์์ ๋ชจ๋ธ๋ง)์ ์ํํ๊ธฐ ์ํด ํ ํฐ์ ์์์์ผ์ผ ํ๋ ๊ฒฝ์ฐ,
`collate_fn` ์ธ์๋ฅผ ์ฌ์ฉํ์ฌ ์ํ ๋ชฉ๋ก์ ๋ฐฐ์น๋ก ๋ณํํ๊ณ ์ํ๋ ์ ์ฒ๋ฆฌ๋ฅผ ์ ์ฉํ ํจ์๋ฅผ ์ ๋ฌํ ์ ์์ต๋๋ค.
[์์](https://github.com/huggingface/transformers/tree/main/examples) ๋๋
[๋
ธํธ๋ถ](https://huggingface.co/docs/transformers/notebooks)์ ์ฐธ์กฐํ์ฌ ์ด ์ ๊ทผ ๋ฐฉ์์ด ์ค์ ๋ก ์๋ํ๋ ๋ชจ์ต์ ํ์ธํ์ธ์.
`tf.data.Dataset`์ ์์ฑํ ํ์๋ ์ด์ ๊ณผ ๋ง์ฐฌ๊ฐ์ง๋ก ๋ชจ๋ธ์ ์ปดํ์ผํ๊ณ ํ๋ จ(fit)ํ ์ ์์ต๋๋ค:
```py
model.compile(optimizer=Adam(3e-5))
model.fit(tf_dataset)
```
</tf>
</frameworkcontent>
<a id='pytorch_native'></a>
## ๊ธฐ๋ณธ ํ์ดํ ์น๋ก ํ๋ จํ๊ธฐ[[train-in-native-pytorch]]
<frameworkcontent>
<pt>
<Youtube id="Dh9CL8fyG80"/>
[`Trainer`]๋ ํ๋ จ ๋ฃจํ๋ฅผ ์ฒ๋ฆฌํ๋ฉฐ ํ ์ค์ ์ฝ๋๋ก ๋ชจ๋ธ์ ๋ฏธ์ธ ์กฐ์ ํ ์ ์์ต๋๋ค. ์ง์ ํ๋ จ ๋ฃจํ๋ฅผ ์์ฑํ๋ ๊ฒ์ ์ ํธํ๋ ์ฌ์ฉ์์ ๊ฒฝ์ฐ, ๊ธฐ๋ณธ PyTorch์์ ๐ค Transformers ๋ชจ๋ธ์ ๋ฏธ์ธ ์กฐ์ ํ ์๋ ์์ต๋๋ค.
์ด ์์ ์์ ๋
ธํธ๋ถ์ ๋ค์ ์์ํ๊ฑฐ๋ ๋ค์ ์ฝ๋๋ฅผ ์คํํด ๋ฉ๋ชจ๋ฆฌ๋ฅผ ํ๋ณดํด์ผ ํ ์ ์์ต๋๋ค:
```py
del model
del trainer
torch.cuda.empty_cache()
```
๋ค์์ผ๋ก, 'ํ ํฐํ๋ ๋ฐ์ดํฐ์
'์ ์๋์ผ๋ก ํ์ฒ๋ฆฌํ์ฌ ํ๋ จ๋ จ์ ์ฌ์ฉํ ์ ์๋๋ก ์ค๋นํฉ๋๋ค.
1. ๋ชจ๋ธ์ด ์์ ํ
์คํธ๋ฅผ ์
๋ ฅ์ผ๋ก ํ์ฉํ์ง ์์ผ๋ฏ๋ก `text` ์ด์ ์ ๊ฑฐํฉ๋๋ค:
```py
>>> tokenized_datasets = tokenized_datasets.remove_columns(["text"])
```
2. ๋ชจ๋ธ์์ ์ธ์์ ์ด๋ฆ์ด `labels`๋ก ์ง์ ๋ ๊ฒ์ผ๋ก ์์ํ๋ฏ๋ก `label` ์ด์ ์ด๋ฆ์ `labels`๋ก ๋ณ๊ฒฝํฉ๋๋ค:
```py
>>> tokenized_datasets = tokenized_datasets.rename_column("label", "labels")
```
3. ๋ฐ์ดํฐ์
์ ํ์์ List ๋์ PyTorch ํ
์๋ฅผ ๋ฐํํ๋๋ก ์ค์ ํฉ๋๋ค:
```py
>>> tokenized_datasets.set_format("torch")
```
๊ทธ๋ฆฌ๊ณ ์์ ํ์๋ ๋๋ก ๋ฐ์ดํฐ์
์ ๋ ์์ ํ์ ์งํฉ์ ์์ฑํ์ฌ ๋ฏธ์ธ ์กฐ์ ์๋๋ฅผ ๋์
๋๋ค:
```py
>>> small_train_dataset = tokenized_datasets["train"].shuffle(seed=42).select(range(1000))
>>> small_eval_dataset = tokenized_datasets["test"].shuffle(seed=42).select(range(1000))
```
### DataLoader[[dataloader]]
ํ๋ จ ๋ฐ ํ
์คํธ ๋ฐ์ดํฐ์
์ ๋ํ 'DataLoader'๋ฅผ ์์ฑํ์ฌ ๋ฐ์ดํฐ ๋ฐฐ์น๋ฅผ ๋ฐ๋ณตํ ์ ์์ต๋๋ค:
```py
>>> from torch.utils.data import DataLoader
>>> train_dataloader = DataLoader(small_train_dataset, shuffle=True, batch_size=8)
>>> eval_dataloader = DataLoader(small_eval_dataset, batch_size=8)
```
์์ธก์ ์ํ ๋ ์ด๋ธ ๊ฐ์๋ฅผ ์ฌ์ฉํ์ฌ ๋ชจ๋ธ์ ๋ก๋ํฉ๋๋ค:
```py
>>> from transformers import AutoModelForSequenceClassification
>>> model = AutoModelForSequenceClassification.from_pretrained("bert-base-cased", num_labels=5)
```
### ์ตํฐ๋ง์ด์ ๋ฐ ํ์ต ์๋ ์ค์ผ์ค๋ฌ[[optimizer-and-learning-rate-scheduler]]
์ตํฐ๋ง์ด์ ์ ํ์ต ์๋ ์ค์ผ์ค๋ฌ๋ฅผ ์์ฑํ์ฌ ๋ชจ๋ธ์ ๋ฏธ์ธ ์กฐ์ ํฉ๋๋ค. ํ์ดํ ์น์์ ์ ๊ณตํ๋ [`AdamW`](https://pytorch.org/docs/stable/generated/torch.optim.AdamW.html) ์ตํฐ๋ง์ด์ ๋ฅผ ์ฌ์ฉํด ๋ณด๊ฒ ์ต๋๋ค:
```py
>>> from torch.optim import AdamW
>>> optimizer = AdamW(model.parameters(), lr=5e-5)
```
[`Trainer`]์์ ๊ธฐ๋ณธ ํ์ต ์๋ ์ค์ผ์ค๋ฌ๋ฅผ ์์ฑํฉ๋๋ค:
```py
>>> from transformers import get_scheduler
>>> num_epochs = 3
>>> num_training_steps = num_epochs * len(train_dataloader)
>>> lr_scheduler = get_scheduler(
... name="linear", optimizer=optimizer, num_warmup_steps=0, num_training_steps=num_training_steps
... )
```
๋ง์ง๋ง์ผ๋ก, GPU์ ์ก์ธ์คํ ์ ์๋ ๊ฒฝ์ฐ 'device'๋ฅผ ์ง์ ํ์ฌ GPU๋ฅผ ์ฌ์ฉํ๋๋ก ํฉ๋๋ค. ๊ทธ๋ ์ง ์์ผ๋ฉด CPU์์ ํ๋ จํ๋ฉฐ ๋ช ๋ถ์ด ์๋ ๋ช ์๊ฐ์ด ๊ฑธ๋ฆด ์ ์์ต๋๋ค.
```py
>>> import torch
>>> device = torch.device("cuda") if torch.cuda.is_available() else torch.device("cpu")
>>> model.to(device)
```
<Tip>
[Colaboratory](https://colab.research.google.com/) ๋๋ [SageMaker StudioLab](https://studiolab.sagemaker.aws/)๊ณผ ๊ฐ์ ํธ์คํ
๋
ธํธ๋ถ์ด ์๋ ๊ฒฝ์ฐ ํด๋ผ์ฐ๋ GPU์ ๋ฌด๋ฃ๋ก ์ก์ธ์คํ ์ ์์ต๋๋ค.
</Tip>
์ด์ ํ๋ จํ ์ค๋น๊ฐ ๋์์ต๋๋ค! ๐ฅณ
### ํ๋ จ ๋ฃจํ[[training-loop]]
ํ๋ จ ์งํ ์ํฉ์ ์ถ์ ํ๋ ค๋ฉด [tqdm](https://tqdm.github.io/) ๋ผ์ด๋ธ๋ฌ๋ฆฌ๋ฅผ ์ฌ์ฉํ์ฌ ํธ๋ ์ด๋ ๋จ๊ณ ์์ ์งํ๋ฅ ํ์์ค์ ์ถ๊ฐํ์ธ์:
```py
>>> from tqdm.auto import tqdm
>>> progress_bar = tqdm(range(num_training_steps))
>>> model.train()
>>> for epoch in range(num_epochs):
... for batch in train_dataloader:
... batch = {k: v.to(device) for k, v in batch.items()}
... outputs = model(**batch)
... loss = outputs.loss
... loss.backward()
... optimizer.step()
... lr_scheduler.step()
... optimizer.zero_grad()
... progress_bar.update(1)
```
### ํ๊ฐ ํ๊ธฐ[[evaluate]]
[`Trainer`]์ ํ๊ฐ ํจ์๋ฅผ ์ถ๊ฐํ ๋ฐฉ๋ฒ๊ณผ ๋ง์ฐฌ๊ฐ์ง๋ก, ํ๋ จ ๋ฃจํ๋ฅผ ์ง์ ์์ฑํ ๋๋ ๋์ผํ ์์
์ ์ํํด์ผ ํฉ๋๋ค. ํ์ง๋ง ์ด๋ฒ์๋ ๊ฐ ์ํฌํฌ๊ฐ ๋๋ ๋๋ง๋ค ํ๊ฐ์งํ๋ฅผ ๊ณ์ฐํ์ฌ ๋ณด๊ณ ํ๋ ๋์ , [`~evaluate.add_batch`]๋ฅผ ์ฌ์ฉํ์ฌ ๋ชจ๋ ๋ฐฐ์น๋ฅผ ๋์ ํ๊ณ ๋งจ ๋ง์ง๋ง์ ํ๊ฐ์งํ๋ฅผ ๊ณ์ฐํฉ๋๋ค.
```py
>>> import evaluate
>>> metric = evaluate.load("accuracy")
>>> model.eval()
>>> for batch in eval_dataloader:
... batch = {k: v.to(device) for k, v in batch.items()}
... with torch.no_grad():
... outputs = model(**batch)
... logits = outputs.logits
... predictions = torch.argmax(logits, dim=-1)
... metric.add_batch(predictions=predictions, references=batch["labels"])
>>> metric.compute()
```
</pt>
</frameworkcontent>
<a id='additional-resources'></a>
## ์ถ๊ฐ ์๋ฃ[[additional-resources]]
๋ ๋ง์ ๋ฏธ์ธ ํ๋ ์์ ๋ ๋ค์์ ์ฐธ์กฐํ์ธ์:
- [๐ค Trnasformers ์์ ](https://github.com/huggingface/transformers/tree/main/examples)์๋ PyTorch ๋ฐ ํ
์ํ๋ก์ฐ์์ ์ผ๋ฐ์ ์ธ NLP ์์
์ ํ๋ จํ ์ ์๋ ์คํฌ๋ฆฝํธ๊ฐ ํฌํจ๋์ด ์์ต๋๋ค.
- [๐ค Transformers ๋
ธํธ๋ถ](notebooks)์๋ PyTorch ๋ฐ ํ
์ํ๋ก์ฐ์์ ํน์ ์์
์ ์ํด ๋ชจ๋ธ์ ๋ฏธ์ธ ํ๋ํ๋ ๋ฐฉ๋ฒ์ ๋ํ ๋ค์ํ ๋
ธํธ๋ถ์ด ํฌํจ๋์ด ์์ต๋๋ค.
| 0 |
hf_public_repos/transformers/docs/source | hf_public_repos/transformers/docs/source/ko/sagemaker.md | <!---
Copyright 2020 The HuggingFace Team. All rights reserved.
Licensed under the Apache License, Version 2.0 (the "License");
you may not use this file except in compliance with the License.
You may obtain a copy of the License at
http://www.apache.org/licenses/LICENSE-2.0
Unless required by applicable law or agreed to in writing, software
distributed under the License is distributed on an "AS IS" BASIS,
WITHOUT WARRANTIES OR CONDITIONS OF ANY KIND, either express or implied.
See the License for the specific language governing permissions and
limitations under the License.
โ ๏ธ Note that this file is in Markdown but contain specific syntax for our doc-builder (similar to MDX) that may not be
rendered properly in your Markdown viewer.
-->
# Amazon SageMaker์์ ํ์ต ์คํํ๊ธฐ[[run-training-on-amazon-sagemaker]]
๋ฌธ์๊ฐ [hf.co/docs/sagemaker](https://huggingface.co/docs/sagemaker)๋ก ์ด๋๋์์ต๋๋ค. ์ด ํ์ด์ง๋ `transformers` 5.0 ์์ ์ญ์ ๋ ์์ ์
๋๋ค.
### ๋ชฉ์ฐจ[[table-of-content]]
- [Train Hugging Face models on Amazon SageMaker with the SageMaker Python SDK](https://huggingface.co/docs/sagemaker/train)
- [Deploy Hugging Face models to Amazon SageMaker with the SageMaker Python SDK](https://huggingface.co/docs/sagemaker/inference)
- [Frequently Asked Questions](https://huggingface.co/docs/sagemaker/faq)
| 0 |
hf_public_repos/transformers/docs/source/ko | hf_public_repos/transformers/docs/source/ko/tasks/question_answering.md | <!--Copyright 2022 The HuggingFace Team. All rights reserved.
Licensed under the Apache License, Version 2.0 (the "License"); you may not use this file except in compliance with
the License. You may obtain a copy of the License at
http://www.apache.org/licenses/LICENSE-2.0
Unless required by applicable law or agreed to in writing, software distributed under the License is distributed on
an "AS IS" BASIS, WITHOUT WARRANTIES OR CONDITIONS OF ANY KIND, either express or implied. See the License for the
specific language governing permissions and limitations under the License.
โ ๏ธ Note that this file is in Markdown but contain specific syntax for our doc-builder (similar to MDX) that may not be
rendered properly in your Markdown viewer.
-->
# ์ง์ ์๋ต(Question Answering)[[question-answering]]
[[open-in-colab]]
<Youtube id="ajPx5LwJD-I"/>
์ง์ ์๋ต ํ์คํฌ๋ ์ฃผ์ด์ง ์ง๋ฌธ์ ๋ํ ๋ต๋ณ์ ์ ๊ณตํฉ๋๋ค. Alexa, Siri ๋๋ Google๊ณผ ๊ฐ์ ๊ฐ์ ๋น์์๊ฒ ๋ ์จ๊ฐ ์ด๋ค์ง ๋ฌผ์ด๋ณธ ์ ์ด ์๋ค๋ฉด ์ง์ ์๋ต ๋ชจ๋ธ์ ์ฌ์ฉํด๋ณธ ์ ์ด ์์ ๊ฒ์
๋๋ค. ์ง์ ์๋ต ํ์คํฌ์๋ ์ผ๋ฐ์ ์ผ๋ก ๋ ๊ฐ์ง ์ ํ์ด ์์ต๋๋ค.
- ์ถ์ถ์ (Extractive) ์ง์ ์๋ต: ์ฃผ์ด์ง ๋ฌธ๋งฅ์์ ๋ต๋ณ์ ์ถ์ถํฉ๋๋ค.
- ์์ฑ์ (Abstractive) ์ง์ ์๋ต: ๋ฌธ๋งฅ์์ ์ง๋ฌธ์ ์ฌ๋ฐ๋ฅด๊ฒ ๋ตํ๋ ๋ต๋ณ์ ์์ฑํฉ๋๋ค.
์ด ๊ฐ์ด๋๋ ๋ค์๊ณผ ๊ฐ์ ๋ฐฉ๋ฒ๋ค์ ๋ณด์ฌ์ค๋๋ค.
1. ์ถ์ถ์ ์ง์ ์๋ต์ ํ๊ธฐ ์ํด [SQuAD](https://huggingface.co/datasets/squad) ๋ฐ์ดํฐ ์ธํธ์์ [DistilBERT](https://huggingface.co/distilbert-base-uncased) ๋ฏธ์ธ ์กฐ์ ํ๊ธฐ
2. ์ถ๋ก ์ ๋ฏธ์ธ ์กฐ์ ๋ ๋ชจ๋ธ ์ฌ์ฉํ๊ธฐ
<Tip>
์ด ํํ ๋ฆฌ์ผ์์ ์ค๋ช
ํ๋ ํ์คํฌ๋ ๋ค์๊ณผ ๊ฐ์ ๋ชจ๋ธ ์ํคํ
์ฒ์์ ์ง์๋ฉ๋๋ค.
<!--This tip is automatically generated by `make fix-copies`, do not fill manually!-->
[ALBERT](../model_doc/albert), [BART](../model_doc/bart), [BERT](../model_doc/bert), [BigBird](../model_doc/big_bird), [BigBird-Pegasus](../model_doc/bigbird_pegasus), [BLOOM](../model_doc/bloom), [CamemBERT](../model_doc/camembert), [CANINE](../model_doc/canine), [ConvBERT](../model_doc/convbert), [Data2VecText](../model_doc/data2vec-text), [DeBERTa](../model_doc/deberta), [DeBERTa-v2](../model_doc/deberta-v2), [DistilBERT](../model_doc/distilbert), [ELECTRA](../model_doc/electra), [ERNIE](../model_doc/ernie), [ErnieM](../model_doc/ernie_m), [FlauBERT](../model_doc/flaubert), [FNet](../model_doc/fnet), [Funnel Transformer](../model_doc/funnel), [GPT-J](../model_doc/gptj), [I-BERT](../model_doc/ibert), [LayoutLMv2](../model_doc/layoutlmv2), [LayoutLMv3](../model_doc/layoutlmv3), [LED](../model_doc/led), [LiLT](../model_doc/lilt), [Longformer](../model_doc/longformer), [LUKE](../model_doc/luke), [LXMERT](../model_doc/lxmert), [MarkupLM](../model_doc/markuplm), [mBART](../model_doc/mbart), [MEGA](../model_doc/mega), [Megatron-BERT](../model_doc/megatron-bert), [MobileBERT](../model_doc/mobilebert), [MPNet](../model_doc/mpnet), [MVP](../model_doc/mvp), [Nezha](../model_doc/nezha), [Nystrรถmformer](../model_doc/nystromformer), [OPT](../model_doc/opt), [QDQBert](../model_doc/qdqbert), [Reformer](../model_doc/reformer), [RemBERT](../model_doc/rembert), [RoBERTa](../model_doc/roberta), [RoBERTa-PreLayerNorm](../model_doc/roberta-prelayernorm), [RoCBert](../model_doc/roc_bert), [RoFormer](../model_doc/roformer), [Splinter](../model_doc/splinter), [SqueezeBERT](../model_doc/squeezebert), [XLM](../model_doc/xlm), [XLM-RoBERTa](../model_doc/xlm-roberta), [XLM-RoBERTa-XL](../model_doc/xlm-roberta-xl), [XLNet](../model_doc/xlnet), [X-MOD](../model_doc/xmod), [YOSO](../model_doc/yoso)
<!--End of the generated tip-->
</Tip>
์์ํ๊ธฐ ์ ์, ํ์ํ ๋ผ์ด๋ธ๋ฌ๋ฆฌ๊ฐ ๋ชจ๋ ์ค์น๋์ด ์๋์ง ํ์ธํ์ธ์:
```bash
pip install transformers datasets evaluate
```
์ฌ๋ฌ๋ถ์ ๋ชจ๋ธ์ ์
๋ก๋ํ๊ณ ์ปค๋ฎค๋ํฐ์ ๊ณต์ ํ ์ ์๋๋ก Hugging Face ๊ณ์ ์ ๋ก๊ทธ์ธํ๋ ๊ฒ์ด ์ข์ต๋๋ค. ๋ฉ์์ง๊ฐ ํ์๋๋ฉด ํ ํฐ์ ์
๋ ฅํด์ ๋ก๊ทธ์ธํฉ๋๋ค:
```py
>>> from huggingface_hub import notebook_login
>>> notebook_login()
```
## SQuAD ๋ฐ์ดํฐ ์ธํธ ๊ฐ์ ธ์ค๊ธฐ[[load-squad-dataset]]
๋จผ์ ๐ค Datasets ๋ผ์ด๋ธ๋ฌ๋ฆฌ์์ SQuAD ๋ฐ์ดํฐ ์ธํธ์ ์ผ๋ถ๋ฅผ ๊ฐ์ ธ์ต๋๋ค. ์ด๋ ๊ฒ ํ๋ฉด ์ ์ฒด ๋ฐ์ดํฐ ์ธํธ๋ก ํ๋ จํ๋ฉฐ ๋ ๋ง์ ์๊ฐ์ ํ ์ ํ๊ธฐ ์ ์ ๋ชจ๋ ๊ฒ์ด ์ ์๋ํ๋์ง ์คํํ๊ณ ํ์ธํ ์ ์์ต๋๋ค.
```py
>>> from datasets import load_dataset
>>> squad = load_dataset("squad", split="train[:5000]")
```
๋ฐ์ดํฐ ์ธํธ์ ๋ถํ ๋ `train`์ [`~datasets.Dataset.train_test_split`] ๋ฉ์๋๋ฅผ ์ฌ์ฉํด ํ๋ จ ๋ฐ์ดํฐ ์ธํธ์ ํ
์คํธ ๋ฐ์ดํฐ ์ธํธ๋ก ๋๋์ด์ค๋๋ค:
```py
>>> squad = squad.train_test_split(test_size=0.2)
```
๊ทธ๋ฆฌ๊ณ ๋์ ์์๋ก ๋ฐ์ดํฐ๋ฅผ ํ๋ ์ดํด๋ด
๋๋ค:
```py
>>> squad["train"][0]
{'answers': {'answer_start': [515], 'text': ['Saint Bernadette Soubirous']},
'context': 'Architecturally, the school has a Catholic character. Atop the Main Building\'s gold dome is a golden statue of the Virgin Mary. Immediately in front of the Main Building and facing it, is a copper statue of Christ with arms upraised with the legend "Venite Ad Me Omnes". Next to the Main Building is the Basilica of the Sacred Heart. Immediately behind the basilica is the Grotto, a Marian place of prayer and reflection. It is a replica of the grotto at Lourdes, France where the Virgin Mary reputedly appeared to Saint Bernadette Soubirous in 1858. At the end of the main drive (and in a direct line that connects through 3 statues and the Gold Dome), is a simple, modern stone statue of Mary.',
'id': '5733be284776f41900661182',
'question': 'To whom did the Virgin Mary allegedly appear in 1858 in Lourdes France?',
'title': 'University_of_Notre_Dame'
}
```
์ด ์ค์์ ๋ช ๊ฐ์ง ์ค์ํ ํญ๋ชฉ์ด ์์ต๋๋ค:
- `answers`: ๋ต์ ํ ํฐ์ ์์ ์์น์ ๋ต์ ํ
์คํธ
- `context`: ๋ชจ๋ธ์ด ๋ต์ ์ถ์ถํ๋๋ฐ ํ์ํ ๋ฐฐ๊ฒฝ ์ง์
- `question`: ๋ชจ๋ธ์ด ๋ตํด์ผ ํ๋ ์ง๋ฌธ
## ์ ์ฒ๋ฆฌ[[preprocess]]
<Youtube id="qgaM0weJHpA"/>
๋ค์ ๋จ๊ณ์์๋ `question` ๋ฐ `context` ํญ๋ชฉ์ ์ฒ๋ฆฌํ๊ธฐ ์ํด DistilBERT ํ ํฌ๋์ด์ ๋ฅผ ๊ฐ์ ธ์ต๋๋ค:
```py
>>> from transformers import AutoTokenizer
>>> tokenizer = AutoTokenizer.from_pretrained("distilbert-base-uncased")
```
์ง์ ์๋ต ํ์คํฌ์ ๊ด๋ จํด์ ํนํ ์ ์ํด์ผํ ๋ช ๊ฐ์ง ์ ์ฒ๋ฆฌ ๋จ๊ณ๊ฐ ์์ต๋๋ค:
1. ๋ฐ์ดํฐ ์ธํธ์ ์ผ๋ถ ์์ ์๋ ๋ชจ๋ธ์ ์ต๋ ์
๋ ฅ ๊ธธ์ด๋ฅผ ์ด๊ณผํ๋ ๋งค์ฐ ๊ธด `context`๊ฐ ์์ ์ ์์ต๋๋ค. ๊ธด ์ํ์ค๋ฅผ ๋ค๋ฃจ๊ธฐ ์ํด์๋, `truncation="only_second"`๋ก ์ค์ ํด `context`๋ง ์๋ผ๋ด๋ฉด ๋ฉ๋๋ค.
2. ๊ทธ ๋ค์, `return_offset_mapping=True`๋ก ์ค์ ํด ๋ต๋ณ์ ์์๊ณผ ์ข
๋ฃ ์์น๋ฅผ ์๋์ `context`์ ๋งคํํฉ๋๋ค.
3. ๋งคํ์ ์๋ฃํ๋ฉด, ์ด์ ๋ต๋ณ์์ ์์ ํ ํฐ๊ณผ ์ข
๋ฃ ํ ํฐ์ ์ฐพ์ ์ ์์ต๋๋ค. ์คํ์
์ ์ด๋ ๋ถ๋ถ์ด `question`๊ณผ `context`์ ํด๋นํ๋์ง ์ฐพ์ ์ ์๋๋ก [`~tokenizers.Encoding.sequence_ids`] ๋ฉ์๋๋ฅผ ์ฌ์ฉํ์ธ์.
๋ค์์ `answer`์ ์์ ํ ํฐ๊ณผ ์ข
๋ฃ ํ ํฐ์ ์๋ผ๋ด์ `context`์ ๋งคํํ๋ ํจ์๋ฅผ ๋ง๋๋ ๋ฐฉ๋ฒ์
๋๋ค:
```py
>>> def preprocess_function(examples):
... questions = [q.strip() for q in examples["question"]]
... inputs = tokenizer(
... questions,
... examples["context"],
... max_length=384,
... truncation="only_second",
... return_offsets_mapping=True,
... padding="max_length",
... )
... offset_mapping = inputs.pop("offset_mapping")
... answers = examples["answers"]
... start_positions = []
... end_positions = []
... for i, offset in enumerate(offset_mapping):
... answer = answers[i]
... start_char = answer["answer_start"][0]
... end_char = answer["answer_start"][0] + len(answer["text"][0])
... sequence_ids = inputs.sequence_ids(i)
... # Find the start and end of the context
... idx = 0
... while sequence_ids[idx] != 1:
... idx += 1
... context_start = idx
... while sequence_ids[idx] == 1:
... idx += 1
... context_end = idx - 1
... # If the answer is not fully inside the context, label it (0, 0)
... if offset[context_start][0] > end_char or offset[context_end][1] < start_char:
... start_positions.append(0)
... end_positions.append(0)
... else:
... # Otherwise it's the start and end token positions
... idx = context_start
... while idx <= context_end and offset[idx][0] <= start_char:
... idx += 1
... start_positions.append(idx - 1)
... idx = context_end
... while idx >= context_start and offset[idx][1] >= end_char:
... idx -= 1
... end_positions.append(idx + 1)
... inputs["start_positions"] = start_positions
... inputs["end_positions"] = end_positions
... return inputs
```
๋ชจ๋ ๋ฐ์ดํฐ ์ธํธ์ ์ ์ฒ๋ฆฌ๋ฅผ ์ ์ฉํ๋ ค๋ฉด, ๐ค Datasets [`~datasets.Dataset.map`] ํจ์๋ฅผ ์ฌ์ฉํ์ธ์. `batched=True`๋ก ์ค์ ํด ๋ฐ์ดํฐ ์ธํธ์ ์ฌ๋ฌ ์์๋ค์ ํ ๋ฒ์ ์ฒ๋ฆฌํ๋ฉด `map` ํจ์์ ์๋๋ฅผ ๋น ๋ฅด๊ฒ ํ ์ ์์ต๋๋ค. ํ์ํ์ง ์์ ์ด์ ๋ชจ๋ ์ ๊ฑฐํฉ๋๋ค:
```py
>>> tokenized_squad = squad.map(preprocess_function, batched=True, remove_columns=squad["train"].column_names)
```
์ด์ [`DefaultDataCollator`]๋ฅผ ์ด์ฉํด ์์ ๋ฐฐ์น๋ฅผ ์์ฑํฉ๋๋ค. ๐ค Transformers์ ๋ค๋ฅธ ๋ฐ์ดํฐ ์ฝ๋ ์ดํฐ(data collator)์ ๋ฌ๋ฆฌ, [`DefaultDataCollator`]๋ ํจ๋ฉ๊ณผ ๊ฐ์ ์ถ๊ฐ ์ ์ฒ๋ฆฌ๋ฅผ ์ ์ฉํ์ง ์์ต๋๋ค:
<frameworkcontent>
<pt>
```py
>>> from transformers import DefaultDataCollator
>>> data_collator = DefaultDataCollator()
```
</pt>
<tf>
```py
>>> from transformers import DefaultDataCollator
>>> data_collator = DefaultDataCollator(return_tensors="tf")
```
</tf>
</frameworkcontent>
## ํ๋ จ[[train]]
<frameworkcontent>
<pt>
<Tip>
[`Trainer`]๋ฅผ ์ด์ฉํด ๋ชจ๋ธ์ ๋ฏธ์ธ ์กฐ์ ํ๋ ๊ฒ์ ์ต์ํ์ง ์๋ค๋ฉด, [์ฌ๊ธฐ](../training#train-with-pytorch-trainer)์์ ๊ธฐ์ด ํํ ๋ฆฌ์ผ์ ์ดํด๋ณด์ธ์!
</Tip>
์ด์ ๋ชจ๋ธ ํ๋ จ์ ์์ํ ์ค๋น๊ฐ ๋์์ต๋๋ค! [`AutoModelForQuestionAnswering`]์ผ๋ก DistilBERT๋ฅผ ๊ฐ์ ธ์ต๋๋ค:
```py
>>> from transformers import AutoModelForQuestionAnswering, TrainingArguments, Trainer
>>> model = AutoModelForQuestionAnswering.from_pretrained("distilbert-base-uncased")
```
์ด์ ์ธ ๋จ๊ณ๋ง ๋จ์์ต๋๋ค:
1. [`TrainingArguments`]์์ ํ๋ จ ํ์ดํผํ๋ผ๋ฏธํฐ๋ฅผ ์ ํฉ๋๋ค. ๊ผญ ํ์ํ ๋งค๊ฐ๋ณ์๋ ๋ชจ๋ธ์ ์ ์ฅํ ์์น๋ฅผ ์ง์ ํ๋ `output_dir` ์
๋๋ค. `push_to_hub=True`๋ก ์ค์ ํด์ ์ด ๋ชจ๋ธ์ Hub๋ก ํธ์ํฉ๋๋ค (๋ชจ๋ธ์ ์
๋ก๋ํ๋ ค๋ฉด Hugging Face์ ๋ก๊ทธ์ธํด์ผ ํฉ๋๋ค).
2. ๋ชจ๋ธ, ๋ฐ์ดํฐ ์ธํธ, ํ ํฌ๋์ด์ , ๋ฐ์ดํฐ ์ฝ๋ ์ดํฐ์ ํจ๊ป [`Trainer`]์ ํ๋ จ ์ธ์๋ค์ ์ ๋ฌํฉ๋๋ค.
3. [`~Trainer.train`]์ ํธ์ถํด์ ๋ชจ๋ธ์ ๋ฏธ์ธ ์กฐ์ ํฉ๋๋ค.
```py
>>> training_args = TrainingArguments(
... output_dir="my_awesome_qa_model",
... evaluation_strategy="epoch",
... learning_rate=2e-5,
... per_device_train_batch_size=16,
... per_device_eval_batch_size=16,
... num_train_epochs=3,
... weight_decay=0.01,
... push_to_hub=True,
... )
>>> trainer = Trainer(
... model=model,
... args=training_args,
... train_dataset=tokenized_squad["train"],
... eval_dataset=tokenized_squad["test"],
... tokenizer=tokenizer,
... data_collator=data_collator,
... )
>>> trainer.train()
```
ํ๋ จ์ด ์๋ฃ๋๋ฉด, [`~transformers.Trainer.push_to_hub`] ๋งค์๋๋ฅผ ์ฌ์ฉํด ๋ชจ๋ธ์ Hub์ ๊ณต์ ํด์ ๋ชจ๋ ์ฌ๋๋ค์ด ์ฌ์ฉํ ์ ์๊ฒ ๊ณต์ ํด์ฃผ์ธ์:
```py
>>> trainer.push_to_hub()
```
</pt>
<tf>
<Tip>
Keras๋ก ๋ชจ๋ธ์ ๋ฏธ์ธ ์กฐ์ ํ๋ ๊ฒ์ ์ต์ํ์ง ์๋ค๋ฉด, [์ฌ๊ธฐ](../training#train-a-tensorflow-model-with-keras)์์ ๊ธฐ์ด ํํ ๋ฆฌ์ผ์ ์ดํด๋ณด์ธ์!
</Tip>
TensorFlow๋ฅผ ์ด์ฉํ ๋ชจ๋ธ์ ๋ฏธ์ธ ์กฐ์ ํ๋ ค๋ฉด ์ตํฐ๋ง์ด์ ํจ์, ํ์ต๋ฅ ์ค์ผ์ฅด ๋ฐ ๋ช ๊ฐ์ง ํ๋ จ ํ์ดํผํ๋ผ๋ฏธํฐ๋ฅผ ์ค์ ํ๋ ๊ฒ๋ถํฐ ์์ํด์ผํฉ๋๋ค:
```py
>>> from transformers import create_optimizer
>>> batch_size = 16
>>> num_epochs = 2
>>> total_train_steps = (len(tokenized_squad["train"]) // batch_size) * num_epochs
>>> optimizer, schedule = create_optimizer(
... init_lr=2e-5,
... num_warmup_steps=0,
... num_train_steps=total_train_steps,
... )
```
๊ทธ ๋ค์ [`TFAutoModelForQuestionAnswering`]์ผ๋ก DistilBERT๋ฅผ ๊ฐ์ ธ์ต๋๋ค:
```py
>>> from transformers import TFAutoModelForQuestionAnswering
>>> model = TFAutoModelForQuestionAnswering("distilbert-base-uncased")
```
[`~transformers.TFPreTrainedModel.prepare_tf_dataset`]์ ์ฌ์ฉํด์ ๋ฐ์ดํฐ ์ธํธ๋ฅผ `tf.data.Dataset` ํ์์ผ๋ก ๋ณํํฉ๋๋ค:
```py
>>> tf_train_set = model.prepare_tf_dataset(
... tokenized_squad["train"],
... shuffle=True,
... batch_size=16,
... collate_fn=data_collator,
... )
>>> tf_validation_set = model.prepare_tf_dataset(
... tokenized_squad["test"],
... shuffle=False,
... batch_size=16,
... collate_fn=data_collator,
... )
```
[`compile`](https://keras.io/api/models/model_training_apis/#compile-method)๋ก ํ๋ จํ ๋ชจ๋ธ์ ์ค์ ํฉ๋๋ค:
```py
>>> import tensorflow as tf
>>> model.compile(optimizer=optimizer)
```
๋ง์ง๋ง์ผ๋ก ๋ชจ๋ธ์ Hub๋ก ํธ์ํ ๋ฐฉ๋ฒ์ ์ค์ ํฉ๋๋ค. [`~transformers.PushToHubCallback`]์์ ๋ชจ๋ธ๊ณผ ํ ํฌ๋์ด์ ๋ฅผ ํธ์ํ ๊ฒฝ๋ก๋ฅผ ์ค์ ํฉ๋๋ค:
```py
>>> from transformers.keras_callbacks import PushToHubCallback
>>> callback = PushToHubCallback(
... output_dir="my_awesome_qa_model",
... tokenizer=tokenizer,
... )
```
๋๋์ด ๋ชจ๋ธ ํ๋ จ์ ์์ํ ์ค๋น๊ฐ ๋์์ต๋๋ค! ํ๋ จ ๋ฐ์ดํฐ ์ธํธ์ ํ๊ฐ ๋ฐ์ดํฐ ์ธํธ, ์ํญ ์, ์ฝ๋ฐฑ์ ์ค์ ํ ํ [`fit`](https://keras.io/api/models/model_training_apis/#fit-method)์ ์ด์ฉํด ๋ชจ๋ธ์ ๋ฏธ์ธ ์กฐ์ ํฉ๋๋ค:
```py
>>> model.fit(x=tf_train_set, validation_data=tf_validation_set, epochs=3, callbacks=[callback])
```
ํ๋ จ์ด ์๋ฃ๋๋ฉด ๋ชจ๋ธ์ด ์๋์ผ๋ก Hub์ ์
๋ก๋๋์ด ๋๊ตฌ๋ ์ฌ์ฉํ ์ ์์ต๋๋ค!
</tf>
</frameworkcontent>
<Tip>
์ง์ ์๋ต์ ์ํด ๋ชจ๋ธ์ ๋ฏธ์ธ ์กฐ์ ํ๋ ๋ฐฉ๋ฒ์ ๋ํ ๋ ์์ธํ ์์๋ [PyTorch notebook](https://colab.research.google.com/github/huggingface/notebooks/blob/main/examples/question_answering.ipynb) ๋๋ [TensorFlow notebook](https://colab.research.google.com/github/huggingface/notebooks/blob/main/examples/question_answering-tf.ipynb)์ ์ฐธ์กฐํ์ธ์.
</Tip>
## ํ๊ฐ[[evaluate]]
์ง์ ์๋ต์ ํ๊ฐํ๋ ค๋ฉด ์๋นํ ์์ ํ์ฒ๋ฆฌ๊ฐ ํ์ํฉ๋๋ค. ์๊ฐ์ด ๋๋ฌด ๋ง์ด ๊ฑธ๋ฆฌ์ง ์๋๋ก ์ด ๊ฐ์ด๋์์๋ ํ๊ฐ ๋จ๊ณ๋ฅผ ์๋ตํฉ๋๋ค. [`Trainer`]๋ ํ๋ จ ๊ณผ์ ์์ ํ๊ฐ ์์ค(evaluation loss)์ ๊ณ์ ๊ณ์ฐํ๊ธฐ ๋๋ฌธ์ ๋ชจ๋ธ์ ์ฑ๋ฅ์ ๋๋ต์ ์ผ๋ก ์ ์ ์์ต๋๋ค.
์๊ฐ์ ์ฌ์ ๊ฐ ์๊ณ ์ง์ ์๋ต ๋ชจ๋ธ์ ํ๊ฐํ๋ ๋ฐฉ๋ฒ์ ๊ด์ฌ์ด ์๋ค๋ฉด ๐ค Hugging Face Course์ [Question answering](https://huggingface.co/course/chapter7/7?fw=pt#postprocessing) ์ฑํฐ๋ฅผ ์ดํด๋ณด์ธ์!
## ์ถ๋ก [[inference]]
์ด์ ๋ชจ๋ธ์ ๋ฏธ์ธ ์กฐ์ ํ์ผ๋ ์ถ๋ก ์ ์ฌ์ฉํ ์ ์์ต๋๋ค!
์ง๋ฌธ๊ณผ ๋ชจ๋ธ์ด ์์ธกํ๊ธฐ ์ํ๋ ๋ฌธ๋งฅ(context)๋ฅผ ์๊ฐํด๋ณด์ธ์:
```py
>>> question = "How many programming languages does BLOOM support?"
>>> context = "BLOOM has 176 billion parameters and can generate text in 46 languages natural languages and 13 programming languages."
```
์ถ๋ก ์ ์ํด ๋ฏธ์ธ ์กฐ์ ํ ๋ชจ๋ธ์ ํ
์คํธํ๋ ๊ฐ์ฅ ์ฌ์ด ๋ฐฉ๋ฒ์ [`pipeline`]์ ์ฌ์ฉํ๋ ๊ฒ ์
๋๋ค. ๋ชจ๋ธ์ ์ฌ์ฉํด ์ง์ ์๋ต์ ํ๊ธฐ ์ํด์ `pipeline`์ ์ธ์คํด์คํํ๊ณ ํ
์คํธ๋ฅผ ์
๋ ฅํฉ๋๋ค:
```py
>>> from transformers import pipeline
>>> question_answerer = pipeline("question-answering", model="my_awesome_qa_model")
>>> question_answerer(question=question, context=context)
{'score': 0.2058267742395401,
'start': 10,
'end': 95,
'answer': '176 billion parameters and can generate text in 46 languages natural languages and 13'}
```
์ํ๋ค๋ฉด `pipeline`์ ๊ฒฐ๊ณผ๋ฅผ ์ง์ ๋ณต์ ํ ์๋ ์์ต๋๋ค:
<frameworkcontent>
<pt>
ํ
์คํธ๋ฅผ ํ ํฐํํด์ PyTorch ํ
์๋ฅผ ๋ฐํํฉ๋๋ค:
```py
>>> from transformers import AutoTokenizer
>>> tokenizer = AutoTokenizer.from_pretrained("my_awesome_qa_model")
>>> inputs = tokenizer(question, context, return_tensors="pt")
```
๋ชจ๋ธ์ ์
๋ ฅ์ ์ ๋ฌํ๊ณ `logits`์ ๋ฐํํฉ๋๋ค:
```py
>>> from transformers import AutoModelForQuestionAnswering
>>> model = AutoModelForQuestionAnswering.from_pretrained("my_awesome_qa_model")
>>> with torch.no_grad():
... outputs = model(**inputs)
```
๋ชจ๋ธ์ ์ถ๋ ฅ์์ ์์ ๋ฐ ์ข
๋ฃ ์์น๊ฐ ์ด๋์ง ๊ฐ์ฅ ๋์ ํ๋ฅ ์ ์ป์ต๋๋ค:
```py
>>> answer_start_index = outputs.start_logits.argmax()
>>> answer_end_index = outputs.end_logits.argmax()
```
์์ธก๋ ํ ํฐ์ ํด๋
ํด์ ๋ต์ ์ป์ต๋๋ค:
```py
>>> predict_answer_tokens = inputs.input_ids[0, answer_start_index : answer_end_index + 1]
>>> tokenizer.decode(predict_answer_tokens)
'176 billion parameters and can generate text in 46 languages natural languages and 13'
```
</pt>
<tf>
ํ
์คํธ๋ฅผ ํ ํฐํํด์ TensorFlow ํ
์๋ฅผ ๋ฐํํฉ๋๋ค:
```py
>>> from transformers import AutoTokenizer
>>> tokenizer = AutoTokenizer.from_pretrained("my_awesome_qa_model")
>>> inputs = tokenizer(question, text, return_tensors="tf")
```
๋ชจ๋ธ์ ์
๋ ฅ์ ์ ๋ฌํ๊ณ `logits`์ ๋ฐํํฉ๋๋ค:
```py
>>> from transformers import TFAutoModelForQuestionAnswering
>>> model = TFAutoModelForQuestionAnswering.from_pretrained("my_awesome_qa_model")
>>> outputs = model(**inputs)
```
๋ชจ๋ธ์ ์ถ๋ ฅ์์ ์์ ๋ฐ ์ข
๋ฃ ์์น๊ฐ ์ด๋์ง ๊ฐ์ฅ ๋์ ํ๋ฅ ์ ์ป์ต๋๋ค:
```py
>>> answer_start_index = int(tf.math.argmax(outputs.start_logits, axis=-1)[0])
>>> answer_end_index = int(tf.math.argmax(outputs.end_logits, axis=-1)[0])
```
์์ธก๋ ํ ํฐ์ ํด๋
ํด์ ๋ต์ ์ป์ต๋๋ค:
```py
>>> predict_answer_tokens = inputs.input_ids[0, answer_start_index : answer_end_index + 1]
>>> tokenizer.decode(predict_answer_tokens)
'176 billion parameters and can generate text in 46 languages natural languages and 13'
```
</tf>
</frameworkcontent>
| 0 |
hf_public_repos/transformers/docs/source/ko | hf_public_repos/transformers/docs/source/ko/tasks/language_modeling.md | <!--Copyright 2023 The HuggingFace Team. All rights reserved.
Licensed under the Apache License, Version 2.0 (the "License"); you may not use this file except in compliance with
the License. You may obtain a copy of the License at
http://www.apache.org/licenses/LICENSE-2.0
Unless required by applicable law or agreed to in writing, software distributed under the License is distributed on
an "AS IS" BASIS, WITHOUT WARRANTIES OR CONDITIONS OF ANY KIND, either express or implied. See the License for the
specific language governing permissions and limitations under the License.
โ ๏ธ Note that this file is in Markdown but contain specific syntax for our doc-builder (similar to MDX) that may not be
rendered properly in your Markdown viewer.
-->
# ์ธ๊ณผ ์ธ์ด ๋ชจ๋ธ๋ง[[causal-language-modeling]]
[[open-in-colab]]
์ธ์ด ๋ชจ๋ธ๋ง์ ์ธ๊ณผ์ ์ธ์ด ๋ชจ๋ธ๋ง๊ณผ ๋ง์คํฌ๋ ์ธ์ด ๋ชจ๋ธ๋ง, ๋ ๊ฐ์ง ์ ํ์ผ๋ก ๋๋ฉ๋๋ค. ์ด ๊ฐ์ด๋์์๋ ์ธ๊ณผ์ ์ธ์ด ๋ชจ๋ธ๋ง์ ์ค๋ช
ํฉ๋๋ค.
์ธ๊ณผ ์ธ์ด ๋ชจ๋ธ์ ํ
์คํธ ์์ฑ์ ์์ฃผ ์ฌ์ฉ๋ฉ๋๋ค. ๋ ์ฐฝ์์ ์ธ ๋ฐฉํฅ์ผ๋ก ์์ฉํ ์ ์์ต๋๋ค.
์ง์ ์ฌ์ฉํ๋ฉฐ ์ฌ๋ฏธ์๋ ํ๊ตฌ๋ฅผ ํด๋ณด๊ฑฐ๋, Copilot ๋๋ CodeParrot์ ๊ฐ์ ์ง๋ฅํ ์ฝ๋ฉ ์ด์์คํดํธ์ ๊ธฐ๋ฐ์ด ๋๊ธฐ๋ ํฉ๋๋ค.
<Youtube id="Vpjb1lu0MDk"/>
์ธ๊ณผ ์ธ์ด ๋ชจ๋ธ๋ง์ ํ ํฐ ์ํ์ค์์ ๋ค์ ํ ํฐ์ ์์ธกํ๋ฉฐ, ๋ชจ๋ธ์ ์ผ์ชฝ์ ํ ํฐ์๋ง ์ ๊ทผํ ์ ์์ต๋๋ค.
์ด๋ ๋ชจ๋ธ์ด ๋ฏธ๋์ ํ ํฐ์ ๋ณผ ์ ์๋ค๋ ๊ฒ์ ์๋ฏธํฉ๋๋ค. ์ธ๊ณผ ์ธ์ด ๋ชจ๋ธ์ ์๋ก GPT-2๊ฐ ์์ฃ .
์ด ๊ฐ์ด๋์์๋ ๋ค์ ์์
์ ์ํํ๋ ๋ฐฉ๋ฒ์ ์๋ดํฉ๋๋ค:
1. [DistilGPT2](https://huggingface.co/distilgpt2) ๋ชจ๋ธ์ [ELI5](https://huggingface.co/datasets/eli5) ๋ฐ์ดํฐ ์ธํธ์ [r/askscience](https://www.reddit.com/r/askscience/) ํ์ ์งํฉ์ผ๋ก ๋ฏธ์ธ ์กฐ์
2. ๋ฏธ์ธ ์กฐ์ ๋ ๋ชจ๋ธ์ ์ถ๋ก ์ ์ฌ์ฉ
<Tip>
์ด ์๋ด์์ ๋จ๊ณ์ ๋์ผํ ๋ฐฉ๋ฒ์ผ๋ก ์ธ๊ณผ ์ธ์ด ๋ชจ๋ธ๋ง์ ์ํด ๋ค๋ฅธ ์ํคํ
์ฒ๋ฅผ ๋ฏธ์ธ ์กฐ์ ํ ์ ์์ต๋๋ค.
๋ค์ ์ํคํ
์ฒ ์ค ํ๋๋ฅผ ์ ํํ์ธ์:
<!--This tip is automatically generated by `make fix-copies`, do not fill manually!-->
[BART](../model_doc/bart), [BERT](../model_doc/bert), [Bert Generation](../model_doc/bert-generation), [BigBird](../model_doc/big_bird), [BigBird-Pegasus](../model_doc/bigbird_pegasus), [BioGpt](../model_doc/biogpt), [Blenderbot](../model_doc/blenderbot), [BlenderbotSmall](../model_doc/blenderbot-small), [BLOOM](../model_doc/bloom), [CamemBERT](../model_doc/camembert), [CodeGen](../model_doc/codegen), [CPM-Ant](../model_doc/cpmant), [CTRL](../model_doc/ctrl), [Data2VecText](../model_doc/data2vec-text), [ELECTRA](../model_doc/electra), [ERNIE](../model_doc/ernie), [GIT](../model_doc/git), [GPT-Sw3](../model_doc/gpt-sw3), [OpenAI GPT-2](../model_doc/gpt2), [GPTBigCode](../model_doc/gpt_bigcode), [GPT Neo](../model_doc/gpt_neo), [GPT NeoX](../model_doc/gpt_neox), [GPT NeoX Japanese](../model_doc/gpt_neox_japanese), [GPT-J](../model_doc/gptj), [LLaMA](../model_doc/llama), [Marian](../model_doc/marian), [mBART](../model_doc/mbart), [MEGA](../model_doc/mega), [Megatron-BERT](../model_doc/megatron-bert), [MVP](../model_doc/mvp), [OpenLlama](../model_doc/open-llama), [OpenAI GPT](../model_doc/openai-gpt), [OPT](../model_doc/opt), [Pegasus](../model_doc/pegasus), [PLBart](../model_doc/plbart), [ProphetNet](../model_doc/prophetnet), [QDQBert](../model_doc/qdqbert), [Reformer](../model_doc/reformer), [RemBERT](../model_doc/rembert), [RoBERTa](../model_doc/roberta), [RoBERTa-PreLayerNorm](../model_doc/roberta-prelayernorm), [RoCBert](../model_doc/roc_bert), [RoFormer](../model_doc/roformer), [RWKV](../model_doc/rwkv), [Speech2Text2](../model_doc/speech_to_text_2), [Transformer-XL](../model_doc/transfo-xl), [TrOCR](../model_doc/trocr), [XGLM](../model_doc/xglm), [XLM](../model_doc/xlm), [XLM-ProphetNet](../model_doc/xlm-prophetnet), [XLM-RoBERTa](../model_doc/xlm-roberta), [XLM-RoBERTa-XL](../model_doc/xlm-roberta-xl), [XLNet](../model_doc/xlnet), [X-MOD](../model_doc/xmod)
<!--End of the generated tip-->
</Tip>
์์ํ๊ธฐ ์ ์ ํ์ํ ๋ผ์ด๋ธ๋ฌ๋ฆฌ๊ฐ ๋ชจ๋ ์ค์น๋์ด ์๋์ง ํ์ธํ์ธ์:
```bash
pip install transformers datasets evaluate
```
์ปค๋ฎค๋ํฐ์ ๋ชจ๋ธ์ ์
๋ก๋ํ๊ณ ๊ณต์ ํ๊ธฐ ์ํด Hugging Face ๊ณ์ ์ ๋ก๊ทธ์ธํ๋ ๊ฒ์ ๊ถ์ฅํฉ๋๋ค. ์๋ฆผ์ด ํ์๋๋ฉด ํ ํฐ์ ์
๋ ฅํ์ฌ ๋ก๊ทธ์ธํ์ธ์:
```py
>>> from huggingface_hub import notebook_login
>>> notebook_login()
```
## ELI5 ๋ฐ์ดํฐ ์ธํธ ๋ถ๋ฌ์ค๊ธฐ[[load-eli5-dataset]]
๋จผ์ , ๐ค Datasets ๋ผ์ด๋ธ๋ฌ๋ฆฌ์์ r/askscience์ ์์ ํ์ ์งํฉ์ธ ELI5 ๋ฐ์ดํฐ ์ธํธ๋ฅผ ๋ถ๋ฌ์ต๋๋ค.
์ด๋ฅผ ํตํด ์ ์ฒด ๋ฐ์ดํฐ ์ธํธ์์ ํ์ตํ๋ ๋ฐ ๋ ๋ง์ ์๊ฐ์ ํฌ์ํ๊ธฐ ์ ์, ์คํํด๋ด์ผ๋ก์จ ๋ชจ๋ ๊ฒ์ด ์๋ํ๋์ง ํ์ธํ ์ ์์ต๋๋ค.
```py
>>> from datasets import load_dataset
>>> eli5 = load_dataset("eli5", split="train_asks[:5000]")
```
๋ฐ์ดํฐ ์ธํธ์ `train_asks` ๋ถํ ์ [`~datasets.Dataset.train_test_split`] ๋ฉ์๋๋ฅผ ์ฌ์ฉํ์ฌ ํ์ต ๋ฐ ํ
์คํธ ์ธํธ๋ก ๋ถํ ํฉ๋๋ค:
```py
>>> eli5 = eli5.train_test_split(test_size=0.2)
```
๊ทธ๋ฐ ๋ค์ ์์ ๋ฅผ ์ดํด๋ณด์ธ์:
```py
>>> eli5["train"][0]
{'answers': {'a_id': ['c3d1aib', 'c3d4lya'],
'score': [6, 3],
'text': ["The velocity needed to remain in orbit is equal to the square root of Newton's constant times the mass of earth divided by the distance from the center of the earth. I don't know the altitude of that specific mission, but they're usually around 300 km. That means he's going 7-8 km/s.\n\nIn space there are no other forces acting on either the shuttle or the guy, so they stay in the same position relative to each other. If he were to become unable to return to the ship, he would presumably run out of oxygen, or slowly fall into the atmosphere and burn up.",
"Hope you don't mind me asking another question, but why aren't there any stars visible in this photo?"]},
'answers_urls': {'url': []},
'document': '',
'q_id': 'nyxfp',
'selftext': '_URL_0_\n\nThis was on the front page earlier and I have a few questions about it. Is it possible to calculate how fast the astronaut would be orbiting the earth? Also how does he stay close to the shuttle so that he can return safely, i.e is he orbiting at the same speed and can therefore stay next to it? And finally if his propulsion system failed, would he eventually re-enter the atmosphere and presumably die?',
'selftext_urls': {'url': ['http://apod.nasa.gov/apod/image/1201/freeflyer_nasa_3000.jpg']},
'subreddit': 'askscience',
'title': 'Few questions about this space walk photograph.',
'title_urls': {'url': []}}
```
๋ง์ ๋ณด์ผ ์ ์์ง๋ง, ์ค์ ๋ก๋ `text` ํ๋๋ง ์ค์ํฉ๋๋ค. ์ธ์ด ๋ชจ๋ธ๋ง ์์
์ ์ฅ์ ์ ๋ ์ด๋ธ์ด ํ์ํ์ง ์๋ค๋ ๊ฒ์
๋๋ค. ๋ค์ ๋จ์ด *์์ฒด๊ฐ* ๋ ์ด๋ธ์
๋๋ค. (์ด๋ ๊ฒ ๋ ์ด๋ธ์ ์ ๊ณตํ์ง ์์๋ ๋๋ ํ์ต์ ๋น์ง๋ ํ์ต์ด๋ผ๊ณ ์ผ์ปซ์ต๋๋ค)
## ์ ์ฒ๋ฆฌ[[preprocess]]
<Youtube id="ma1TrR7gE7I"/>
๋ค์ ๋จ๊ณ๋ `text` ํ๋๋ฅผ ์ ์ฒ๋ฆฌํ๊ธฐ ์ํด DistilGPT2 ํ ํฌ๋์ด์ ๋ฅผ ๋ถ๋ฌ์ค๋ ๊ฒ์
๋๋ค.
```py
>>> from transformers import AutoTokenizer
>>> tokenizer = AutoTokenizer.from_pretrained("distilgpt2")
```
์์ ์์ ์์ ์ ์ ์๋ฏ์ด, `text` ํ๋๋ `answers` ์๋์ ์ค์ฒฉ๋์ด ์์ต๋๋ค. ๋ฐ๋ผ์ [`flatten`](https://huggingface.co/docs/datasets/process.html#flatten) ๋ฉ์๋๋ฅผ ์ฌ์ฉํ์ฌ ์ค์ฒฉ ๊ตฌ์กฐ์์ `text` ํ์ ํ๋๋ฅผ ์ถ์ถํด์ผ ํฉ๋๋ค.
```py
>>> eli5 = eli5.flatten()
>>> eli5["train"][0]
{'answers.a_id': ['c3d1aib', 'c3d4lya'],
'answers.score': [6, 3],
'answers.text': ["The velocity needed to remain in orbit is equal to the square root of Newton's constant times the mass of earth divided by the distance from the center of the earth. I don't know the altitude of that specific mission, but they're usually around 300 km. That means he's going 7-8 km/s.\n\nIn space there are no other forces acting on either the shuttle or the guy, so they stay in the same position relative to each other. If he were to become unable to return to the ship, he would presumably run out of oxygen, or slowly fall into the atmosphere and burn up.",
"Hope you don't mind me asking another question, but why aren't there any stars visible in this photo?"],
'answers_urls.url': [],
'document': '',
'q_id': 'nyxfp',
'selftext': '_URL_0_\n\nThis was on the front page earlier and I have a few questions about it. Is it possible to calculate how fast the astronaut would be orbiting the earth? Also how does he stay close to the shuttle so that he can return safely, i.e is he orbiting at the same speed and can therefore stay next to it? And finally if his propulsion system failed, would he eventually re-enter the atmosphere and presumably die?',
'selftext_urls.url': ['http://apod.nasa.gov/apod/image/1201/freeflyer_nasa_3000.jpg'],
'subreddit': 'askscience',
'title': 'Few questions about this space walk photograph.',
'title_urls.url': []}
```
๊ฐ ํ์ ํ๋๋ ์ด์ `answers` ์ ๋์ฌ๋ฅผ ๊ฐ์ง ๋ณ๋์ ์ด๋ก ๋๋์์ผ๋ฉฐ, `text` ํ๋๋ ์ด์ ๋ฆฌ์คํธ์
๋๋ค. ๊ฐ ๋ฌธ์ฅ์ ๊ฐ๋ณ์ ์ผ๋ก ํ ํฐํํ๋ ๋์ , ๋จผ์ ๋ฆฌ์คํธ๋ฅผ ๋ฌธ์์ด๋ก ๋ณํํ์ฌ ํ๊บผ๋ฒ์ ํ ํฐํํ ์ ์์ต๋๋ค.
๋ค์์ ๋ฌธ์์ด ๋ฆฌ์คํธ๋ฅผ ๊ฒฐํฉํ๊ณ ๊ฒฐ๊ณผ๋ฅผ ํ ํฐํํ๋ ์ฒซ ๋ฒ์งธ ์ ์ฒ๋ฆฌ ํจ์์
๋๋ค:
```py
>>> def preprocess_function(examples):
... return tokenizer([" ".join(x) for x in examples["answers.text"]])
```
์ด ์ ์ฒ๋ฆฌ ํจ์๋ฅผ ์ ์ฒด ๋ฐ์ดํฐ ์ธํธ์ ์ ์ฉํ๋ ค๋ฉด ๐ค Datasets [`~datasets.Dataset.map`] ๋ฉ์๋๋ฅผ ์ฌ์ฉํ์ธ์. `batched=True`๋ก ์ค์ ํ์ฌ ๋ฐ์ดํฐ์
์ ์ฌ๋ฌ ์์๋ฅผ ํ ๋ฒ์ ์ฒ๋ฆฌํ๊ณ , `num_proc`๋ฅผ ์ฆ๊ฐ์์ผ ํ๋ก์ธ์ค ์๋ฅผ ๋๋ฆด ์ ์์ต๋๋ค. ํ์ ์๋ ์ด์ ์ ๊ฑฐํ์ธ์:
```py
>>> tokenized_eli5 = eli5.map(
... preprocess_function,
... batched=True,
... num_proc=4,
... remove_columns=eli5["train"].column_names,
... )
```
์ด์ ๋ฐ์ดํฐ ์ธํธ๋ ์ํ์ค๊ฐ ํ ํฐํ๋์ง๋ง, ์ผ๋ถ ์ํ์ค๋ ๋ชจ๋ธ์ ์ต๋ ์
๋ ฅ ๊ธธ์ด๋ณด๋ค ๊ธธ ์ ์์ต๋๋ค.
์ด์ ๋ ๋ฒ์งธ ์ ์ฒ๋ฆฌ ํจ์๋ฅผ ์ฌ์ฉํ์ฌ
- ๋ชจ๋ ์ํ์ค๋ฅผ ์ฐ๊ฒฐํ๊ณ ,
- `block_size`๋ก ์ ์๋ ๊ธธ์ด๋ก ์ฐ๊ฒฐ๋ ์ํ์ค๋ฅผ ์ฌ๋ฌ ๊ฐ์ ์งง์ ๋ฌถ์์ผ๋ก ๋๋๋๋ค. ์ด ๊ฐ์ ์ต๋ ์
๋ ฅ ๊ธธ์ด์ GPU RAM์ ๊ณ ๋ คํด ์ถฉ๋ถํ ์งง์์ผ ํฉ๋๋ค.
```py
>>> block_size = 128
>>> def group_texts(examples):
... # Concatenate all texts.
... concatenated_examples = {k: sum(examples[k], []) for k in examples.keys()}
... total_length = len(concatenated_examples[list(examples.keys())[0]])
... # We drop the small remainder, we could add padding if the model supported it instead of this drop, you can
... # customize this part to your needs.
... if total_length >= block_size:
... total_length = (total_length // block_size) * block_size
... # Split by chunks of block_size.
... result = {
... k: [t[i : i + block_size] for i in range(0, total_length, block_size)]
... for k, t in concatenated_examples.items()
... }
... result["labels"] = result["input_ids"].copy()
... return result
```
์ ์ฒด ๋ฐ์ดํฐ ์ธํธ์ `group_texts` ํจ์๋ฅผ ์ ์ฉํ์ธ์:
```py
>>> lm_dataset = tokenized_eli5.map(group_texts, batched=True, num_proc=4)
```
๊ทธ๋ฐ ๋ค์ [`DataCollatorForLanguageModeling`]์ ์ฌ์ฉํ์ฌ ์์ ์ ๋ฐฐ์น๋ฅผ ๋ง๋ญ๋๋ค. ๋ฐ์ดํฐ ์ธํธ ์ ์ฒด๋ฅผ ์ต๋ ๊ธธ์ด๋ก ํจ๋ฉํ๋ ๊ฒ๋ณด๋ค, ์ทจํฉ ๋จ๊ณ์์ ๊ฐ ๋ฐฐ์น์ ์ต๋ ๊ธธ์ด๋ก ๋ฌธ์ฅ์ *๋์ ์ผ๋ก ํจ๋ฉ*ํ๋ ๊ฒ์ด ๋ ํจ์จ์ ์
๋๋ค.
<frameworkcontent>
<pt>
ํจ๋ฉ ํ ํฐ์ผ๋ก ์ข
๊ฒฐ ํ ํฐ์ ์ฌ์ฉํ๊ณ `mlm=False`๋ก ์ค์ ํ์ธ์. ์ด๋ ๊ฒ ํ๋ฉด ์
๋ ฅ์ ์ค๋ฅธ์ชฝ์ผ๋ก ํ ์นธ์ฉ ์ํํธํ ๊ฐ์ ๋ ์ด๋ธ๋ก ์ฌ์ฉํฉ๋๋ค:
```py
>>> from transformers import DataCollatorForLanguageModeling
>>> tokenizer.pad_token = tokenizer.eos_token
>>> data_collator = DataCollatorForLanguageModeling(tokenizer=tokenizer, mlm=False)
```
</pt>
<tf>
ํจ๋ฉ ํ ํฐ์ผ๋ก ์ข
๊ฒฐ ํ ํฐ์ ์ฌ์ฉํ๊ณ `mlm=False`๋ก ์ค์ ํ์ธ์. ์ด๋ ๊ฒ ํ๋ฉด ์
๋ ฅ์ ์ค๋ฅธ์ชฝ์ผ๋ก ํ ์นธ์ฉ ์ํํธํ ๊ฐ์ ๋ ์ด๋ธ๋ก ์ฌ์ฉํฉ๋๋ค:
```py
>>> from transformers import DataCollatorForLanguageModeling
>>> data_collator = DataCollatorForLanguageModeling(tokenizer=tokenizer, mlm=False, return_tensors="tf")
```
</tf>
</frameworkcontent>
## ํ๋ จ[[train]]
<frameworkcontent>
<pt>
<Tip>
[`Trainer`]๋ฅผ ์ฌ์ฉํ์ฌ ๋ชจ๋ธ์ ๋ฏธ์ธ ์กฐ์ ํ๋ ๋ฐฉ๋ฒ์ ์ ๋ชจ๋ฅด์ ๋ค๋ฉด [๊ธฐ๋ณธ ํํ ๋ฆฌ์ผ](../training#train-with-pytorch-trainer)์ ํ์ธํด๋ณด์ธ์!
</Tip>
์ด์ ๋ชจ๋ธ์ ํ๋ จํ๊ธฐ ์ค๋น๊ฐ ๋์์ต๋๋ค! [`AutoModelForCausalLM`]๋ฅผ ์ฌ์ฉํ์ฌ DistilGPT2๋ฅผ ๋ถ๋ฌ์ต๋๋ค:
```py
>>> from transformers import AutoModelForCausalLM, TrainingArguments, Trainer
>>> model = AutoModelForCausalLM.from_pretrained("distilgpt2")
```
์ฌ๊ธฐ๊น์ง ์งํํ๋ฉด ์ธ ๋จ๊ณ๋ง ๋จ์์ต๋๋ค:
1. [`TrainingArguments`]์์ ํ๋ จ ํ์ดํผํ๋ผ๋ฏธํฐ๋ฅผ ์ ์ํ์ธ์. `output_dir`์ ์ ์ผํ ํ์ ๋งค๊ฐ๋ณ์๋ก, ๋ชจ๋ธ์ ์ ์ฅํ ์์น๋ฅผ ์ง์ ํฉ๋๋ค. (๋จผ์ Hugging Face์ ๋ก๊ทธ์ธ ํ์) `push_to_hub=True`๋ก ์ค์ ํ์ฌ ์ด ๋ชจ๋ธ์ ํ๋ธ์ ์
๋ก๋ํ ์ ์์ต๋๋ค.
2. ํ๋ จ ์ธ์๋ฅผ [`Trainer`]์ ๋ชจ๋ธ, ๋ฐ์ดํฐ ์ธํธ ๋ฐ ๋ฐ์ดํฐ ์ฝ๋ ์ดํฐ์ ํจ๊ป ์ ๋ฌํ์ธ์.
3. [`~Trainer.train`]์ ํธ์ถํ์ฌ ๋ชจ๋ธ์ ๋ฏธ์ธ ์กฐ์ ํ์ธ์.
```py
>>> training_args = TrainingArguments(
... output_dir="my_awesome_eli5_clm-model",
... evaluation_strategy="epoch",
... learning_rate=2e-5,
... weight_decay=0.01,
... push_to_hub=True,
... )
>>> trainer = Trainer(
... model=model,
... args=training_args,
... train_dataset=lm_dataset["train"],
... eval_dataset=lm_dataset["test"],
... data_collator=data_collator,
... )
>>> trainer.train()
```
ํ๋ จ์ด ์๋ฃ๋๋ฉด [`~transformers.Trainer.evaluate`] ๋ฉ์๋๋ฅผ ์ฌ์ฉํ์ฌ ๋ชจ๋ธ์ ํ๊ฐํ๊ณ ํผํ๋ ์ํฐ๋ฅผ ์ป์ ์ ์์ต๋๋ค:
```py
>>> import math
>>> eval_results = trainer.evaluate()
>>> print(f"Perplexity: {math.exp(eval_results['eval_loss']):.2f}")
Perplexity: 49.61
```
๊ทธ๋ฐ ๋ค์ [`~transformers.Trainer.push_to_hub`] ๋ฉ์๋๋ฅผ ์ฌ์ฉํ์ฌ ๋ชจ๋ธ์ ํ๋ธ์ ๊ณต์ ํ์ธ์. ์ด๋ ๊ฒ ํ๋ฉด ๋๊ตฌ๋ ๋ชจ๋ธ์ ์ฌ์ฉํ ์ ์์ต๋๋ค:
```py
>>> trainer.push_to_hub()
```
</pt>
<tf>
<Tip>
Keras๋ฅผ ์ฌ์ฉํ์ฌ ๋ชจ๋ธ์ ๋ฏธ์ธ ์กฐ์ ํ๋ ๋ฐฉ๋ฒ์ ์ต์ํ์ง ์๋ค๋ฉด [๊ธฐ๋ณธ ํํ ๋ฆฌ์ผ](../training#train-a-tensorflow-model-with-keras)์ ํ์ธํด๋ณด์ธ์!
</Tip>
TensorFlow์์ ๋ชจ๋ธ์ ๋ฏธ์ธ ์กฐ์ ํ๋ ค๋ฉด, ๋จผ์ ์ตํฐ๋ง์ด์ ํจ์, ํ์ต๋ฅ ์ค์ผ์ค ๋ฐ ์ผ๋ถ ํ๋ จ ํ์ดํผํ๋ผ๋ฏธํฐ๋ฅผ ์ค์ ํ์ธ์:
```py
>>> from transformers import create_optimizer, AdamWeightDecay
>>> optimizer = AdamWeightDecay(learning_rate=2e-5, weight_decay_rate=0.01)
```
๊ทธ๋ฐ ๋ค์ [`TFAutoModelForCausalLM`]๋ฅผ ์ฌ์ฉํ์ฌ DistilGPT2๋ฅผ ๋ถ๋ฌ์ต๋๋ค:
```py
>>> from transformers import TFAutoModelForCausalLM
>>> model = TFAutoModelForCausalLM.from_pretrained("distilgpt2")
```
[`~transformers.TFPreTrainedModel.prepare_tf_dataset`]์ ์ฌ์ฉํ์ฌ ๋ฐ์ดํฐ ์ธํธ๋ฅผ `tf.data.Dataset` ํ์์ผ๋ก ๋ณํํ์ธ์:
```py
>>> tf_train_set = model.prepare_tf_dataset(
... lm_dataset["train"],
... shuffle=True,
... batch_size=16,
... collate_fn=data_collator,
... )
>>> tf_test_set = model.prepare_tf_dataset(
... lm_dataset["test"],
... shuffle=False,
... batch_size=16,
... collate_fn=data_collator,
... )
```
[`compile`](https://keras.io/api/models/model_training_apis/#compile-method)์ ์ฌ์ฉํ์ฌ ๋ชจ๋ธ์ ํ๋ จํ๊ธฐ ์ํด ๊ตฌ์ฑํ์ธ์. Transformers ๋ชจ๋ธ์ ๋ชจ๋ ๊ธฐ๋ณธ์ ์ธ ์์
๊ด๋ จ ์์ค ํจ์๋ฅผ ๊ฐ์ง๊ณ ์์ผ๋ฏ๋ก, ์ํ๋ค๋ฉด ๋ณ๋๋ก ์ง์ ํ์ง ์์๋ ๋ฉ๋๋ค:
```py
>>> import tensorflow as tf
>>> model.compile(optimizer=optimizer) # ๋ณ๋๋ก loss ์ธ์๋ฅผ ๋ฃ์ง ์์์ด์!
```
[`~transformers.PushToHubCallback`]์์ ๋ชจ๋ธ๊ณผ ํ ํฌ๋์ด์ ๋ฅผ ์
๋ก๋ํ ์์น๋ฅผ ์ง์ ํ ์ ์์ต๋๋ค:
```py
>>> from transformers.keras_callbacks import PushToHubCallback
>>> callback = PushToHubCallback(
... output_dir="my_awesome_eli5_clm-model",
... tokenizer=tokenizer,
... )
```
๋ง์ง๋ง์ผ๋ก, ๋ชจ๋ธ์ ํ๋ จํ๊ธฐ ์ํด [`fit`](https://keras.io/api/models/model_training_apis/#fit-method)์ ํธ์ถํ์ธ์. ํ๋ จ ๋ฐ์ดํฐ ์ธํธ, ๊ฒ์ฆ ๋ฐ์ดํฐ ์ธํธ, ์ํญ ์ ๋ฐ ์ฝ๋ฐฑ์ ์ ๋ฌํ์ธ์:
```py
>>> model.fit(x=tf_train_set, validation_data=tf_test_set, epochs=3, callbacks=[callback])
```
ํ๋ จ์ด ์๋ฃ๋๋ฉด ๋ชจ๋ธ์ด ์๋์ผ๋ก ํ๋ธ์ ์
๋ก๋๋์ด ๋ชจ๋๊ฐ ์ฌ์ฉํ ์ ์์ต๋๋ค!
</tf>
</frameworkcontent>
<Tip>
์ธ๊ณผ ์ธ์ด ๋ชจ๋ธ๋ง์ ์ํด ๋ชจ๋ธ์ ๋ฏธ์ธ ์กฐ์ ํ๋ ๋ ์์ธํ ์์ ๋ ํด๋นํ๋ [PyTorch ๋
ธํธ๋ถ](https://colab.research.google.com/github/huggingface/notebooks/blob/main/examples/language_modeling.ipynb) ๋๋ [TensorFlow ๋
ธํธ๋ถ](https://colab.research.google.com/github/huggingface/notebooks/blob/main/examples/language_modeling-tf.ipynb)์ ์ฐธ์กฐํ์ธ์.
</Tip>
## ์ถ๋ก [[inference]]
์ข์์, ์ด์ ๋ชจ๋ธ์ ๋ฏธ์ธ ์กฐ์ ํ์ผ๋ฏ๋ก ์ถ๋ก ์ ์ฌ์ฉํ ์ ์์ต๋๋ค!
์์ฑํ ํ
์คํธ๋ฅผ ์ํ ํ๋กฌํํธ๋ฅผ ๋ง๋ค์ด๋ณด์ธ์:
```py
>>> prompt = "Somatic hypermutation allows the immune system to"
```
์ถ๋ก ์ ์ํด ๋ฏธ์ธ ์กฐ์ ๋ ๋ชจ๋ธ์ ๊ฐ๋จํ ์ฌ์ฉํ๋ ๊ฐ์ฅ ๊ฐ๋จํ ๋ฐฉ๋ฒ์ [`pipeline`]์์ ์ฌ์ฉํ๋ ๊ฒ์
๋๋ค. ๋ชจ๋ธ๊ณผ ํจ๊ป ํ
์คํธ ์์ฑ์ ์ํ `pipeline`์ ์ธ์คํด์คํํ๊ณ ํ
์คํธ๋ฅผ ์ ๋ฌํ์ธ์:
```py
>>> from transformers import pipeline
>>> generator = pipeline("text-generation", model="my_awesome_eli5_clm-model")
>>> generator(prompt)
[{'generated_text': "Somatic hypermutation allows the immune system to be able to effectively reverse the damage caused by an infection.\n\n\nThe damage caused by an infection is caused by the immune system's ability to perform its own self-correcting tasks."}]
```
<frameworkcontent>
<pt>
ํ
์คํธ๋ฅผ ํ ํฐํํ๊ณ `input_ids`๋ฅผ PyTorch ํ
์๋ก ๋ฐํํ์ธ์:
```py
>>> from transformers import AutoTokenizer
>>> tokenizer = AutoTokenizer.from_pretrained("my_awesome_eli5_clm-model")
>>> inputs = tokenizer(prompt, return_tensors="pt").input_ids
```
[`~transformers.generation_utils.GenerationMixin.generate`] ๋ฉ์๋๋ฅผ ์ฌ์ฉํ์ฌ ํ
์คํธ๋ฅผ ์์ฑํ์ธ์. ์์ฑ์ ์ ์ดํ๋ ๋ค์ํ ํ
์คํธ ์์ฑ ์ ๋ต๊ณผ ๋งค๊ฐ๋ณ์์ ๋ํ ์์ธํ ๋ด์ฉ์ [ํ
์คํธ ์์ฑ ์ ๋ต](../generation_strategies) ํ์ด์ง๋ฅผ ํ์ธํ์ธ์.
```py
>>> from transformers import AutoModelForCausalLM
>>> model = AutoModelForCausalLM.from_pretrained("my_awesome_eli5_clm-model")
>>> outputs = model.generate(inputs, max_new_tokens=100, do_sample=True, top_k=50, top_p=0.95)
```
์์ฑ๋ ํ ํฐ ID๋ฅผ ๋ค์ ํ
์คํธ๋ก ๋์ฝ๋ฉํ์ธ์:
```py
>>> tokenizer.batch_decode(outputs, skip_special_tokens=True)
["Somatic hypermutation allows the immune system to react to drugs with the ability to adapt to a different environmental situation. In other words, a system of 'hypermutation' can help the immune system to adapt to a different environmental situation or in some cases even a single life. In contrast, researchers at the University of Massachusetts-Boston have found that 'hypermutation' is much stronger in mice than in humans but can be found in humans, and that it's not completely unknown to the immune system. A study on how the immune system"]
```
</pt>
<tf>
ํ
์คํธ๋ฅผ ํ ํฐํํ๊ณ `input_ids`๋ฅผ TensorFlow ํ
์๋ก ๋ฐํํ์ธ์:
```py
>>> from transformers import AutoTokenizer
>>> tokenizer = AutoTokenizer.from_pretrained("my_awesome_eli5_clm-model")
>>> inputs = tokenizer(prompt, return_tensors="tf").input_ids
```
[`~transformers.generation_tf_utils.TFGenerationMixin.generate`] ๋ฉ์๋๋ฅผ ์ฌ์ฉํ์ฌ ์์ฝ์ ์์ฑํ์ธ์. ์์ฑ์ ์ ์ดํ๋ ๋ค์ํ ํ
์คํธ ์์ฑ ์ ๋ต๊ณผ ๋งค๊ฐ๋ณ์์ ๋ํ ์์ธํ ๋ด์ฉ์ [ํ
์คํธ ์์ฑ ์ ๋ต](../generation_strategies) ํ์ด์ง๋ฅผ ํ์ธํ์ธ์.
```py
>>> from transformers import TFAutoModelForCausalLM
>>> model = TFAutoModelForCausalLM.from_pretrained("my_awesome_eli5_clm-model")
>>> outputs = model.generate(input_ids=inputs, max_new_tokens=100, do_sample=True, top_k=50, top_p=0.95)
```
์์ฑ๋ ํ ํฐ ID๋ฅผ ๋ค์ ํ
์คํธ๋ก ๋์ฝ๋ฉํ์ธ์:
```py
>>> tokenizer.batch_decode(outputs, skip_special_tokens=True)
['Somatic hypermutation allows the immune system to detect the presence of other viruses as they become more prevalent. Therefore, researchers have identified a high proportion of human viruses. The proportion of virus-associated viruses in our study increases with age. Therefore, we propose a simple algorithm to detect the presence of these new viruses in our samples as a sign of improved immunity. A first study based on this algorithm, which will be published in Science on Friday, aims to show that this finding could translate into the development of a better vaccine that is more effective for']
```
</tf>
</frameworkcontent>
| 0 |
hf_public_repos/transformers/docs/source/ko | hf_public_repos/transformers/docs/source/ko/tasks/document_question_answering.md | <!--Copyright 2023 The HuggingFace Team. All rights reserved.
Licensed under the Apache License, Version 2.0 (the "License"); you may not use this file except in compliance with
the License. You may obtain a copy of the License at
http://www.apache.org/licenses/LICENSE-2.0
Unless required by applicable law or agreed to in writing, software distributed under the License is distributed on
an "AS IS" BASIS, WITHOUT WARRANTIES OR CONDITIONS OF ANY KIND, either express or implied. See the License for the
specific language governing permissions and limitations under the License.
โ ๏ธ Note that this file is in Markdown but contain specific syntax for our doc-builder (similar to MDX) that may not be
rendered properly in your Markdown viewer.
-->
# ๋ฌธ์ ์ง์ ์๋ต(Document Question Answering) [[document_question_answering]]
[[open-in-colab]]
๋ฌธ์ ์๊ฐ์ ์ง์ ์๋ต(Document Visual Question Answering)์ด๋ผ๊ณ ๋ ํ๋
๋ฌธ์ ์ง์ ์๋ต(Document Question Answering)์ ๋ฌธ์ ์ด๋ฏธ์ง์ ๋ํ ์ง๋ฌธ์ ๋ต๋ณ์ ์ฃผ๋ ํ์คํฌ์
๋๋ค.
์ด ํ์คํฌ๋ฅผ ์ง์ํ๋ ๋ชจ๋ธ์ ์
๋ ฅ์ ์ผ๋ฐ์ ์ผ๋ก ์ด๋ฏธ์ง์ ์ง๋ฌธ์ ์กฐํฉ์ด๊ณ , ์ถ๋ ฅ์ ์์ฐ์ด๋ก ๋ ๋ต๋ณ์
๋๋ค. ์ด๋ฌํ ๋ชจ๋ธ์ ํ
์คํธ, ๋จ์ด์ ์์น(๋ฐ์ด๋ฉ ๋ฐ์ค), ์ด๋ฏธ์ง ๋ฑ ๋ค์ํ ๋ชจ๋ฌ๋ฆฌํฐ๋ฅผ ํ์ฉํฉ๋๋ค.
์ด ๊ฐ์ด๋๋ ๋ค์ ๋ด์ฉ์ ์ค๋ช
ํฉ๋๋ค:
- [DocVQA dataset](https://huggingface.co/datasets/nielsr/docvqa_1200_examples_donut)์ ์ฌ์ฉํด [LayoutLMv2](../model_doc/layoutlmv2) ๋ฏธ์ธ ์กฐ์ ํ๊ธฐ
- ์ถ๋ก ์ ์ํด ๋ฏธ์ธ ์กฐ์ ๋ ๋ชจ๋ธ์ ์ฌ์ฉํ๊ธฐ
<Tip>
์ด ํํ ๋ฆฌ์ผ์์ ์ค๋ช
ํ๋ ํ์คํฌ๋ ๋ค์๊ณผ ๊ฐ์ ๋ชจ๋ธ ์ํคํ
์ฒ์์ ์ง์๋ฉ๋๋ค:
<!--This tip is automatically generated by `make fix-copies`, do not fill manually!-->
[LayoutLM](../model_doc/layoutlm), [LayoutLMv2](../model_doc/layoutlmv2), [LayoutLMv3](../model_doc/layoutlmv3)
<!--End of the generated tip-->
</Tip>
LayoutLMv2๋ ํ ํฐ์ ๋ง์ง๋ง ์๋์ธต ์์ ์ง์ ์๋ต ํค๋๋ฅผ ์ถ๊ฐํด ๋ต๋ณ์ ์์ ํ ํฐ๊ณผ ๋ ํ ํฐ์ ์์น๋ฅผ ์์ธกํจ์ผ๋ก์จ ๋ฌธ์ ์ง์ ์๋ต ํ์คํฌ๋ฅผ ํด๊ฒฐํฉ๋๋ค. ์ฆ, ๋ฌธ๋งฅ์ด ์ฃผ์ด์ก์ ๋ ์ง๋ฌธ์ ๋ตํ๋ ์ ๋ณด๋ฅผ ์ถ์ถํ๋ ์ถ์ถํ ์ง์ ์๋ต(Extractive question answering)์ผ๋ก ๋ฌธ์ ๋ฅผ ์ฒ๋ฆฌํฉ๋๋ค.
๋ฌธ๋งฅ์ OCR ์์ง์ ์ถ๋ ฅ์์ ๊ฐ์ ธ์ค๋ฉฐ, ์ฌ๊ธฐ์๋ Google์ Tesseract๋ฅผ ์ฌ์ฉํฉ๋๋ค.
์์ํ๊ธฐ ์ ์ ํ์ํ ๋ผ์ด๋ธ๋ฌ๋ฆฌ๊ฐ ๋ชจ๋ ์ค์น๋์ด ์๋์ง ํ์ธํ์ธ์. LayoutLMv2๋ detectron2, torchvision ๋ฐ ํ
์๋ํธ๋ฅผ ํ์๋ก ํฉ๋๋ค.
```bash
pip install -q transformers datasets
```
```bash
pip install 'git+https://github.com/facebookresearch/detectron2.git'
pip install torchvision
```
```bash
sudo apt install tesseract-ocr
pip install -q pytesseract
```
ํ์ํ ๋ผ์ด๋ธ๋ฌ๋ฆฌ๋ค์ ๋ชจ๋ ์ค์นํ ํ ๋ฐํ์์ ๋ค์ ์์ํฉ๋๋ค.
์ปค๋ฎค๋ํฐ์ ๋น์ ์ ๋ชจ๋ธ์ ๊ณต์ ํ๋ ๊ฒ์ ๊ถ์ฅํฉ๋๋ค. Hugging Face ๊ณ์ ์ ๋ก๊ทธ์ธํด์ ๋ชจ๋ธ์ ๐ค Hub์ ์
๋ก๋ํ์ธ์.
ํ๋กฌํํธ๊ฐ ์คํ๋๋ฉด, ๋ก๊ทธ์ธ์ ์ํด ํ ํฐ์ ์
๋ ฅํ์ธ์:
```py
>>> from huggingface_hub import notebook_login
>>> notebook_login()
```
๋ช ๊ฐ์ง ์ ์ญ ๋ณ์๋ฅผ ์ ์ํด ๋ณด๊ฒ ์ต๋๋ค.
```py
>>> model_checkpoint = "microsoft/layoutlmv2-base-uncased"
>>> batch_size = 4
```
## ๋ฐ์ดํฐ ๋ถ๋ฌ์ค๊ธฐ [[load-the-data]]
์ด ๊ฐ์ด๋์์๋ ๐ค Hub์์ ์ฐพ์ ์ ์๋ ์ ์ฒ๋ฆฌ๋ DocVQA์ ์์ ์ํ์ ์ฌ์ฉํฉ๋๋ค.
DocVQA์ ์ ์ฒด ๋ฐ์ดํฐ ์ธํธ๋ฅผ ์ฌ์ฉํ๊ณ ์ถ๋ค๋ฉด, [DocVQA homepage](https://rrc.cvc.uab.es/?ch=17)์ ๊ฐ์
ํ ๋ค์ด๋ก๋ ํ ์ ์์ต๋๋ค. ์ ์ฒด ๋ฐ์ดํฐ ์ธํธ๋ฅผ ๋ค์ด๋ก๋ ํ๋ค๋ฉด, ์ด ๊ฐ์ด๋๋ฅผ ๊ณ์ ์งํํ๊ธฐ ์ํด [๐ค dataset์ ํ์ผ์ ๊ฐ์ ธ์ค๋ ๋ฐฉ๋ฒ](https://huggingface.co/docs/datasets/loading#local-and-remote-files)์ ํ์ธํ์ธ์.
```py
>>> from datasets import load_dataset
>>> dataset = load_dataset("nielsr/docvqa_1200_examples")
>>> dataset
DatasetDict({
train: Dataset({
features: ['id', 'image', 'query', 'answers', 'words', 'bounding_boxes', 'answer'],
num_rows: 1000
})
test: Dataset({
features: ['id', 'image', 'query', 'answers', 'words', 'bounding_boxes', 'answer'],
num_rows: 200
})
})
```
๋ณด์๋ค์ํผ, ๋ฐ์ดํฐ ์ธํธ๋ ์ด๋ฏธ ํ๋ จ ์ธํธ์ ํ
์คํธ ์ธํธ๋ก ๋๋์ด์ ธ ์์ต๋๋ค. ๋ฌด์์๋ก ์์ ๋ฅผ ์ดํด๋ณด๋ฉด์ ํน์ฑ์ ํ์ธํด๋ณด์ธ์.
```py
>>> dataset["train"].features
```
๊ฐ ํ๋๊ฐ ๋ํ๋ด๋ ๋ด์ฉ์ ๋ค์๊ณผ ๊ฐ์ต๋๋ค:
* `id`: ์์ ์ id
* `image`: ๋ฌธ์ ์ด๋ฏธ์ง๋ฅผ ํฌํจํ๋ PIL.Image.Image ๊ฐ์ฒด
* `query`: ์ง๋ฌธ ๋ฌธ์์ด - ์ฌ๋ฌ ์ธ์ด์ ์์ฐ์ด๋ก ๋ ์ง๋ฌธ
* `answers`: ์ฌ๋์ด ์ฃผ์์ ๋จ ์ ๋ต ๋ฆฌ์คํธ
* `words` and `bounding_boxes`: OCR์ ๊ฒฐ๊ณผ๊ฐ๋ค์ด๋ฉฐ ์ด ๊ฐ์ด๋์์๋ ์ฌ์ฉํ์ง ์์ ์์
* `answer`: ๋ค๋ฅธ ๋ชจ๋ธ๊ณผ ์ผ์นํ๋ ๋ต๋ณ์ด๋ฉฐ ์ด ๊ฐ์ด๋์์๋ ์ฌ์ฉํ์ง ์์ ์์
์์ด๋ก ๋ ์ง๋ฌธ๋ง ๋จ๊ธฐ๊ณ ๋ค๋ฅธ ๋ชจ๋ธ์ ๋ํ ์์ธก์ ํฌํจํ๋ `answer` ํน์ฑ์ ์ญ์ ํ๊ฒ ์ต๋๋ค.
๊ทธ๋ฆฌ๊ณ ์ฃผ์ ์์ฑ์๊ฐ ์ ๊ณตํ ๋ฐ์ดํฐ ์ธํธ์์ ์ฒซ ๋ฒ์งธ ๋ต๋ณ์ ๊ฐ์ ธ์ต๋๋ค. ๋๋ ๋ฌด์์๋ก ์ํ์ ์ถ์ถํ ์๋ ์์ต๋๋ค.
```py
>>> updated_dataset = dataset.map(lambda example: {"question": example["query"]["en"]}, remove_columns=["query"])
>>> updated_dataset = updated_dataset.map(
... lambda example: {"answer": example["answers"][0]}, remove_columns=["answer", "answers"]
... )
```
์ด ๊ฐ์ด๋์์ ์ฌ์ฉํ๋ LayoutLMv2 ์ฒดํฌํฌ์ธํธ๋ `max_position_embeddings = 512`๋ก ํ๋ จ๋์์ต๋๋ค(์ด ์ ๋ณด๋ [์ฒดํฌํฌ์ธํธ์ `config.json` ํ์ผ](https://huggingface.co/microsoft/layoutlmv2-base-uncased/blob/main/config.json#L18)์์ ํ์ธํ ์ ์์ต๋๋ค).
๋ฐ๋ก ์์ ๋ฅผ ์๋ผ๋ผ ์๋ ์์ง๋ง, ๊ธด ๋ฌธ์์ ๋์ ๋ต๋ณ์ด ์์ด ์๋ฆฌ๋ ์ํฉ์ ํผํ๊ธฐ ์ํด ์ฌ๊ธฐ์๋ ์๋ฒ ๋ฉ์ด 512๋ณด๋ค ๊ธธ์ด์ง ๊ฐ๋ฅ์ฑ์ด ์๋ ๋ช ๊ฐ์ง ์์ ๋ฅผ ์ ๊ฑฐํ๊ฒ ์ต๋๋ค.
๋ฐ์ดํฐ ์ธํธ์ ์๋ ๋๋ถ๋ถ์ ๋ฌธ์๊ฐ ๊ธด ๊ฒฝ์ฐ ์ฌ๋ผ์ด๋ฉ ์๋์ฐ ๋ฐฉ๋ฒ์ ์ฌ์ฉํ ์ ์์ต๋๋ค - ์์ธํ ๋ด์ฉ์ ํ์ธํ๊ณ ์ถ์ผ๋ฉด ์ด [๋
ธํธ๋ถ](https://github.com/huggingface/notebooks/blob/main/examples/question_answering.ipynb)์ ํ์ธํ์ธ์.
```py
>>> updated_dataset = updated_dataset.filter(lambda x: len(x["words"]) + len(x["question"].split()) < 512)
```
์ด ์์ ์์ ์ด ๋ฐ์ดํฐ ์ธํธ์ OCR ํน์ฑ๋ ์ ๊ฑฐํด ๋ณด๊ฒ ์ต๋๋ค. OCR ํน์ฑ์ ๋ค๋ฅธ ๋ชจ๋ธ์ ๋ฏธ์ธ ์กฐ์ ํ๊ธฐ ์ํ ๊ฒ์ผ๋ก, ์ด ๊ฐ์ด๋์์ ์ฌ์ฉํ๋ ๋ชจ๋ธ์ ์
๋ ฅ ์๊ตฌ ์ฌํญ๊ณผ ์ผ์นํ์ง ์๊ธฐ ๋๋ฌธ์ ์ด ํน์ฑ์ ์ฌ์ฉํ๊ธฐ ์ํด์๋ ์ผ๋ถ ์ฒ๋ฆฌ๊ฐ ํ์ํฉ๋๋ค.
๋์ , ์๋ณธ ๋ฐ์ดํฐ์ [`LayoutLMv2Processor`]๋ฅผ ์ฌ์ฉํ์ฌ OCR ๋ฐ ํ ํฐํ๋ฅผ ๋ชจ๋ ์ํํ ์ ์์ต๋๋ค.
์ด๋ ๊ฒ ํ๋ฉด ๋ชจ๋ธ์ด ์๊ตฌํ๋ ์
๋ ฅ์ ์ป์ ์ ์์ต๋๋ค.
์ด๋ฏธ์ง๋ฅผ ์๋์ผ๋ก ์ฒ๋ฆฌํ๋ ค๋ฉด, [`LayoutLMv2` model documentation](../model_doc/layoutlmv2)์์ ๋ชจ๋ธ์ด ์๊ตฌํ๋ ์
๋ ฅ ํฌ๋งท์ ํ์ธํด๋ณด์ธ์.
```py
>>> updated_dataset = updated_dataset.remove_columns("words")
>>> updated_dataset = updated_dataset.remove_columns("bounding_boxes")
```
๋ง์ง๋ง์ผ๋ก, ๋ฐ์ดํฐ ํ์์ ์๋ฃํ๊ธฐ ์ํด ์ด๋ฏธ์ง ์์๋ฅผ ์ดํด๋ด
์๋ค.
```py
>>> updated_dataset["train"][11]["image"]
```
<div class="flex justify-center">
<img src="https://huggingface.co/datasets/huggingface/documentation-images/resolve/main/transformers/tasks/docvqa_example.jpg" alt="DocVQA Image Example"/>
</div>
## ๋ฐ์ดํฐ ์ ์ฒ๋ฆฌ [[preprocess-the-data]]
๋ฌธ์ ์ง์ ์๋ต ํ์คํฌ๋ ๋ฉํฐ๋ชจ๋ฌ ํ์คํฌ์ด๋ฉฐ, ๊ฐ ๋ชจ๋ฌ๋ฆฌํฐ์ ์
๋ ฅ์ด ๋ชจ๋ธ์ ์๊ตฌ์ ๋ง๊ฒ ์ ์ฒ๋ฆฌ ๋์๋์ง ํ์ธํด์ผ ํฉ๋๋ค.
์ด๋ฏธ์ง ๋ฐ์ดํฐ๋ฅผ ์ฒ๋ฆฌํ ์ ์๋ ์ด๋ฏธ์ง ํ๋ก์ธ์์ ํ
์คํธ ๋ฐ์ดํฐ๋ฅผ ์ธ์ฝ๋ฉํ ์ ์๋ ํ ํฌ๋์ด์ ๋ฅผ ๊ฒฐํฉํ [`LayoutLMv2Processor`]๋ฅผ ๊ฐ์ ธ์ค๋ ๊ฒ๋ถํฐ ์์ํด ๋ณด๊ฒ ์ต๋๋ค.
```py
>>> from transformers import AutoProcessor
>>> processor = AutoProcessor.from_pretrained(model_checkpoint)
```
### ๋ฌธ์ ์ด๋ฏธ์ง ์ ์ฒ๋ฆฌ [[preprocessing-document-images]]
๋จผ์ , ํ๋ก์ธ์์ `image_processor`๋ฅผ ์ฌ์ฉํด ๋ชจ๋ธ์ ๋ํ ๋ฌธ์ ์ด๋ฏธ์ง๋ฅผ ์ค๋นํด ๋ณด๊ฒ ์ต๋๋ค.
๊ธฐ๋ณธ๊ฐ์ผ๋ก, ์ด๋ฏธ์ง ํ๋ก์ธ์๋ ์ด๋ฏธ์ง ํฌ๊ธฐ๋ฅผ 224x224๋ก ์กฐ์ ํ๊ณ ์์ ์ฑ๋์ ์์๊ฐ ์ฌ๋ฐ๋ฅธ์ง ํ์ธํ ํ ๋จ์ด์ ์ ๊ทํ๋ ๋ฐ์ด๋ฉ ๋ฐ์ค๋ฅผ ์ป๊ธฐ ์ํด ํ
์๋ํธ๋ฅผ ์ฌ์ฉํด OCR๋ฅผ ์ ์ฉํฉ๋๋ค.
์ด ํํ ๋ฆฌ์ผ์์ ์ฐ๋ฆฌ๊ฐ ํ์ํ ๊ฒ๊ณผ ๊ธฐ๋ณธ๊ฐ์ ์์ ํ ๋์ผํฉ๋๋ค. ์ด๋ฏธ์ง ๋ฐฐ์น์ ๊ธฐ๋ณธ ์ด๋ฏธ์ง ์ฒ๋ฆฌ๋ฅผ ์ ์ฉํ๊ณ OCR์ ๊ฒฐ๊ณผ๋ฅผ ๋ณํํ๋ ํจ์๋ฅผ ์์ฑํฉ๋๋ค.
```py
>>> image_processor = processor.image_processor
>>> def get_ocr_words_and_boxes(examples):
... images = [image.convert("RGB") for image in examples["image"]]
... encoded_inputs = image_processor(images)
... examples["image"] = encoded_inputs.pixel_values
... examples["words"] = encoded_inputs.words
... examples["boxes"] = encoded_inputs.boxes
... return examples
```
์ด ์ ์ฒ๋ฆฌ๋ฅผ ๋ฐ์ดํฐ ์ธํธ ์ ์ฒด์ ๋น ๋ฅด๊ฒ ์ ์ฉํ๋ ค๋ฉด [`~datasets.Dataset.map`]๋ฅผ ์ฌ์ฉํ์ธ์.
```py
>>> dataset_with_ocr = updated_dataset.map(get_ocr_words_and_boxes, batched=True, batch_size=2)
```
### ํ
์คํธ ๋ฐ์ดํฐ ์ ์ฒ๋ฆฌ [[preprocessing-text-data]]
์ด๋ฏธ์ง์ OCR์ ์ ์ฉํ์ผ๋ฉด ๋ฐ์ดํฐ ์ธํธ์ ํ
์คํธ ๋ถ๋ถ์ ๋ชจ๋ธ์ ๋ง๊ฒ ์ธ์ฝ๋ฉํด์ผ ํฉ๋๋ค.
์ด ์ธ์ฝ๋ฉ์๋ ์ด์ ๋จ๊ณ์์ ๊ฐ์ ธ์จ ๋จ์ด์ ๋ฐ์ค๋ฅผ ํ ํฐ ์์ค์ `input_ids`, `attention_mask`, `token_type_ids` ๋ฐ `bbox`๋ก ๋ณํํ๋ ์์
์ด ํฌํจ๋ฉ๋๋ค.
ํ
์คํธ๋ฅผ ์ ์ฒ๋ฆฌํ๋ ค๋ฉด ํ๋ก์ธ์์ `tokenizer`๊ฐ ํ์ํฉ๋๋ค.
```py
>>> tokenizer = processor.tokenizer
```
์์์ ์ธ๊ธํ ์ ์ฒ๋ฆฌ ์ธ์๋ ๋ชจ๋ธ์ ์ํด ๋ ์ด๋ธ์ ์ถ๊ฐํด์ผ ํฉ๋๋ค. ๐ค Transformers์ `xxxForQuestionAnswering` ๋ชจ๋ธ์ ๊ฒฝ์ฐ, ๋ ์ด๋ธ์ `start_positions`์ `end_positions`๋ก ๊ตฌ์ฑ๋๋ฉฐ ์ด๋ค ํ ํฐ์ด ๋ต๋ณ์ ์์๊ณผ ๋์ ์๋์ง๋ฅผ ๋ํ๋
๋๋ค.
๋ ์ด๋ธ ์ถ๊ฐ๋ฅผ ์ํด์, ๋จผ์ ๋ ํฐ ๋ฆฌ์คํธ(๋จ์ด ๋ฆฌ์คํธ)์์ ํ์ ๋ฆฌ์คํธ(๋จ์ด๋ก ๋ถํ ๋ ๋ต๋ณ)์ ์ฐพ์ ์ ์๋ ํฌํผ ํจ์๋ฅผ ์ ์ํฉ๋๋ค.
์ด ํจ์๋ `words_list`์ `answer_list`, ์ด๋ ๊ฒ ๋ ๋ฆฌ์คํธ๋ฅผ ์
๋ ฅ์ผ๋ก ๋ฐ์ต๋๋ค.
๊ทธ๋ฐ ๋ค์ `words_list`๋ฅผ ๋ฐ๋ณตํ์ฌ `words_list`์ ํ์ฌ ๋จ์ด(words_list[i])๊ฐ `answer_list`์ ์ฒซ ๋ฒ์งธ ๋จ์ด(answer_list[0])์ ๊ฐ์์ง,
ํ์ฌ ๋จ์ด์์ ์์ํด `answer_list`์ ๊ฐ์ ๊ธธ์ด๋งํผ์ `words_list`์ ํ์ ๋ฆฌ์คํธ๊ฐ `answer_list`์ ์ผ์นํ๋์ง ํ์ธํฉ๋๋ค.
์ด ์กฐ๊ฑด์ด ์ฐธ์ด๋ผ๋ฉด ์ผ์นํ๋ ํญ๋ชฉ์ ๋ฐ๊ฒฌํ์์ ์๋ฏธํ๋ฉฐ, ํจ์๋ ์ผ์น ํญ๋ชฉ, ์์ ์ธ๋ฑ์ค(idx) ๋ฐ ์ข
๋ฃ ์ธ๋ฑ์ค(idx + len(answer_list) - 1)๋ฅผ ๊ธฐ๋กํฉ๋๋ค. ์ผ์นํ๋ ํญ๋ชฉ์ด ๋ ๊ฐ ์ด์ ๋ฐ๊ฒฌ๋๋ฉด ํจ์๋ ์ฒซ ๋ฒ์งธ ํญ๋ชฉ๋ง ๋ฐํํฉ๋๋ค. ์ผ์นํ๋ ํญ๋ชฉ์ด ์๋ค๋ฉด ํจ์๋ (`None`, 0, 0)์ ๋ฐํํฉ๋๋ค.
```py
>>> def subfinder(words_list, answer_list):
... matches = []
... start_indices = []
... end_indices = []
... for idx, i in enumerate(range(len(words_list))):
... if words_list[i] == answer_list[0] and words_list[i : i + len(answer_list)] == answer_list:
... matches.append(answer_list)
... start_indices.append(idx)
... end_indices.append(idx + len(answer_list) - 1)
... if matches:
... return matches[0], start_indices[0], end_indices[0]
... else:
... return None, 0, 0
```
์ด ํจ์๊ฐ ์ด๋ป๊ฒ ์ ๋ต์ ์์น๋ฅผ ์ฐพ๋์ง ์ค๋ช
ํ๊ธฐ ์ํด ๋ค์ ์์ ์์ ํจ์๋ฅผ ์ฌ์ฉํด ๋ณด๊ฒ ์ต๋๋ค:
```py
>>> example = dataset_with_ocr["train"][1]
>>> words = [word.lower() for word in example["words"]]
>>> match, word_idx_start, word_idx_end = subfinder(words, example["answer"].lower().split())
>>> print("Question: ", example["question"])
>>> print("Words:", words)
>>> print("Answer: ", example["answer"])
>>> print("start_index", word_idx_start)
>>> print("end_index", word_idx_end)
Question: Who is in cc in this letter?
Words: ['wie', 'baw', 'brown', '&', 'williamson', 'tobacco', 'corporation', 'research', '&', 'development', 'internal', 'correspondence', 'to:', 'r.', 'h.', 'honeycutt', 'ce:', 't.f.', 'riehl', 'from:', '.', 'c.j.', 'cook', 'date:', 'may', '8,', '1995', 'subject:', 'review', 'of', 'existing', 'brainstorming', 'ideas/483', 'the', 'major', 'function', 'of', 'the', 'product', 'innovation', 'graup', 'is', 'to', 'develop', 'marketable', 'nove!', 'products', 'that', 'would', 'be', 'profitable', 'to', 'manufacture', 'and', 'sell.', 'novel', 'is', 'defined', 'as:', 'of', 'a', 'new', 'kind,', 'or', 'different', 'from', 'anything', 'seen', 'or', 'known', 'before.', 'innovation', 'is', 'defined', 'as:', 'something', 'new', 'or', 'different', 'introduced;', 'act', 'of', 'innovating;', 'introduction', 'of', 'new', 'things', 'or', 'methods.', 'the', 'products', 'may', 'incorporate', 'the', 'latest', 'technologies,', 'materials', 'and', 'know-how', 'available', 'to', 'give', 'then', 'a', 'unique', 'taste', 'or', 'look.', 'the', 'first', 'task', 'of', 'the', 'product', 'innovation', 'group', 'was', 'to', 'assemble,', 'review', 'and', 'categorize', 'a', 'list', 'of', 'existing', 'brainstorming', 'ideas.', 'ideas', 'were', 'grouped', 'into', 'two', 'major', 'categories', 'labeled', 'appearance', 'and', 'taste/aroma.', 'these', 'categories', 'are', 'used', 'for', 'novel', 'products', 'that', 'may', 'differ', 'from', 'a', 'visual', 'and/or', 'taste/aroma', 'point', 'of', 'view', 'compared', 'to', 'canventional', 'cigarettes.', 'other', 'categories', 'include', 'a', 'combination', 'of', 'the', 'above,', 'filters,', 'packaging', 'and', 'brand', 'extensions.', 'appearance', 'this', 'category', 'is', 'used', 'for', 'novel', 'cigarette', 'constructions', 'that', 'yield', 'visually', 'different', 'products', 'with', 'minimal', 'changes', 'in', 'smoke', 'chemistry', 'two', 'cigarettes', 'in', 'cne.', 'emulti-plug', 'te', 'build', 'yaur', 'awn', 'cigarette.', 'eswitchable', 'menthol', 'or', 'non', 'menthol', 'cigarette.', '*cigarettes', 'with', 'interspaced', 'perforations', 'to', 'enable', 'smoker', 'to', 'separate', 'unburned', 'section', 'for', 'future', 'smoking.', 'ยซshort', 'cigarette,', 'tobacco', 'section', '30', 'mm.', 'ยซextremely', 'fast', 'buming', 'cigarette.', 'ยซnovel', 'cigarette', 'constructions', 'that', 'permit', 'a', 'significant', 'reduction', 'iretobacco', 'weight', 'while', 'maintaining', 'smoking', 'mechanics', 'and', 'visual', 'characteristics.', 'higher', 'basis', 'weight', 'paper:', 'potential', 'reduction', 'in', 'tobacco', 'weight.', 'ยซmore', 'rigid', 'tobacco', 'column;', 'stiffing', 'agent', 'for', 'tobacco;', 'e.g.', 'starch', '*colored', 'tow', 'and', 'cigarette', 'papers;', 'seasonal', 'promotions,', 'e.g.', 'pastel', 'colored', 'cigarettes', 'for', 'easter', 'or', 'in', 'an', 'ebony', 'and', 'ivory', 'brand', 'containing', 'a', 'mixture', 'of', 'all', 'black', '(black', 'paper', 'and', 'tow)', 'and', 'ail', 'white', 'cigarettes.', '499150498']
Answer: T.F. Riehl
start_index 17
end_index 18
```
ํํธ, ์ ์์ ๊ฐ ์ธ์ฝ๋ฉ๋๋ฉด ๋ค์๊ณผ ๊ฐ์ด ํ์๋ฉ๋๋ค:
```py
>>> encoding = tokenizer(example["question"], example["words"], example["boxes"])
>>> tokenizer.decode(encoding["input_ids"])
[CLS] who is in cc in this letter? [SEP] wie baw brown & williamson tobacco corporation research & development ...
```
์ด์ ์ธ์ฝ๋ฉ๋ ์
๋ ฅ์์ ์ ๋ต์ ์์น๋ฅผ ์ฐพ์์ผ ํฉ๋๋ค.
* `token_type_ids`๋ ์ด๋ค ํ ํฐ์ด ์ง๋ฌธ์ ์ํ๋์ง, ๊ทธ๋ฆฌ๊ณ ์ด๋ค ํ ํฐ์ด ๋ฌธ์์ ๋จ์ด์ ํฌํจ๋๋์ง๋ฅผ ์๋ ค์ค๋๋ค.
* `tokenizer.cls_token_id` ์
๋ ฅ์ ์์ ๋ถ๋ถ์ ์๋ ํน์ ํ ํฐ์ ์ฐพ๋ ๋ฐ ๋์์ ์ค๋๋ค.
* `word_ids`๋ ์๋ณธ `words`์์ ์ฐพ์ ๋ต๋ณ์ ์ ์ฒด ์ธ์ฝ๋ฉ๋ ์
๋ ฅ์ ๋์ผํ ๋ต๊ณผ ์ผ์น์ํค๊ณ ์ธ์ฝ๋ฉ๋ ์
๋ ฅ์์ ๋ต๋ณ์ ์์/๋ ์์น๋ฅผ ๊ฒฐ์ ํฉ๋๋ค.
์ ๋ด์ฉ๋ค์ ์ผ๋์ ๋๊ณ ๋ฐ์ดํฐ ์ธํธ ์์ ์ ๋ฐฐ์น๋ฅผ ์ธ์ฝ๋ฉํ๋ ํจ์๋ฅผ ๋ง๋ค์ด ๋ณด๊ฒ ์ต๋๋ค:
```py
>>> def encode_dataset(examples, max_length=512):
... questions = examples["question"]
... words = examples["words"]
... boxes = examples["boxes"]
... answers = examples["answer"]
... # ์์ ๋ฐฐ์น๋ฅผ ์ธ์ฝ๋ฉํ๊ณ start_positions์ end_positions๋ฅผ ์ด๊ธฐํํฉ๋๋ค
... encoding = tokenizer(questions, words, boxes, max_length=max_length, padding="max_length", truncation=True)
... start_positions = []
... end_positions = []
... # ๋ฐฐ์น์ ์์ ๋ฅผ ๋ฐ๋ณตํฉ๋๋ค
... for i in range(len(questions)):
... cls_index = encoding["input_ids"][i].index(tokenizer.cls_token_id)
... # ์์ ์ words์์ ๋ต๋ณ์ ์์น๋ฅผ ์ฐพ์ต๋๋ค
... words_example = [word.lower() for word in words[i]]
... answer = answers[i]
... match, word_idx_start, word_idx_end = subfinder(words_example, answer.lower().split())
... if match:
... # ์ผ์นํ๋ ํญ๋ชฉ์ ๋ฐ๊ฒฌํ๋ฉด, `token_type_ids`๋ฅผ ์ฌ์ฉํด ์ธ์ฝ๋ฉ์์ ๋จ์ด๊ฐ ์์ํ๋ ์์น๋ฅผ ์ฐพ์ต๋๋ค
... token_type_ids = encoding["token_type_ids"][i]
... token_start_index = 0
... while token_type_ids[token_start_index] != 1:
... token_start_index += 1
... token_end_index = len(encoding["input_ids"][i]) - 1
... while token_type_ids[token_end_index] != 1:
... token_end_index -= 1
... word_ids = encoding.word_ids(i)[token_start_index : token_end_index + 1]
... start_position = cls_index
... end_position = cls_index
... # words์ ๋ต๋ณ ์์น์ ์ผ์นํ ๋๊น์ง word_ids๋ฅผ ๋ฐ๋ณตํ๊ณ `token_start_index`๋ฅผ ๋๋ฆฝ๋๋ค
... # ์ผ์นํ๋ฉด `token_start_index`๋ฅผ ์ธ์ฝ๋ฉ์์ ๋ต๋ณ์ `start_position`์ผ๋ก ์ ์ฅํฉ๋๋ค
... for id in word_ids:
... if id == word_idx_start:
... start_position = token_start_index
... else:
... token_start_index += 1
... # ๋น์ทํ๊ฒ, ๋์์ ์์ํด `word_ids`๋ฅผ ๋ฐ๋ณตํ๋ฉฐ ๋ต๋ณ์ `end_position`์ ์ฐพ์ต๋๋ค
... for id in word_ids[::-1]:
... if id == word_idx_end:
... end_position = token_end_index
... else:
... token_end_index -= 1
... start_positions.append(start_position)
... end_positions.append(end_position)
... else:
... start_positions.append(cls_index)
... end_positions.append(cls_index)
... encoding["image"] = examples["image"]
... encoding["start_positions"] = start_positions
... encoding["end_positions"] = end_positions
... return encoding
```
์ด์ ์ด ์ ์ฒ๋ฆฌ ํจ์๊ฐ ์์ผ๋ ์ ์ฒด ๋ฐ์ดํฐ ์ธํธ๋ฅผ ์ธ์ฝ๋ฉํ ์ ์์ต๋๋ค:
```py
>>> encoded_train_dataset = dataset_with_ocr["train"].map(
... encode_dataset, batched=True, batch_size=2, remove_columns=dataset_with_ocr["train"].column_names
... )
>>> encoded_test_dataset = dataset_with_ocr["test"].map(
... encode_dataset, batched=True, batch_size=2, remove_columns=dataset_with_ocr["test"].column_names
... )
```
์ธ์ฝ๋ฉ๋ ๋ฐ์ดํฐ ์ธํธ์ ํน์ฑ์ด ์ด๋ป๊ฒ ์๊ฒผ๋์ง ํ์ธํด ๋ณด๊ฒ ์ต๋๋ค:
```py
>>> encoded_train_dataset.features
{'image': Sequence(feature=Sequence(feature=Sequence(feature=Value(dtype='uint8', id=None), length=-1, id=None), length=-1, id=None), length=-1, id=None),
'input_ids': Sequence(feature=Value(dtype='int32', id=None), length=-1, id=None),
'token_type_ids': Sequence(feature=Value(dtype='int8', id=None), length=-1, id=None),
'attention_mask': Sequence(feature=Value(dtype='int8', id=None), length=-1, id=None),
'bbox': Sequence(feature=Sequence(feature=Value(dtype='int64', id=None), length=-1, id=None), length=-1, id=None),
'start_positions': Value(dtype='int64', id=None),
'end_positions': Value(dtype='int64', id=None)}
```
## ํ๊ฐ [[evaluation]]
๋ฌธ์ ์ง์ ์๋ต์ ํ๊ฐํ๋ ค๋ฉด ์๋นํ ์์ ํ์ฒ๋ฆฌ๊ฐ ํ์ํฉ๋๋ค. ์๊ฐ์ด ๋๋ฌด ๋ง์ด ๊ฑธ๋ฆฌ์ง ์๋๋ก ์ด ๊ฐ์ด๋์์๋ ํ๊ฐ ๋จ๊ณ๋ฅผ ์๋ตํฉ๋๋ค.
[`Trainer`]๊ฐ ํ๋ จ ๊ณผ์ ์์ ํ๊ฐ ์์ค(evaluation loss)์ ๊ณ์ ๊ณ์ฐํ๊ธฐ ๋๋ฌธ์ ๋ชจ๋ธ์ ์ฑ๋ฅ์ ๋๋ต์ ์ผ๋ก ์ ์ ์์ต๋๋ค.
์ถ์ถ์ (Extractive) ์ง์ ์๋ต์ ๋ณดํต F1/exact match ๋ฐฉ๋ฒ์ ์ฌ์ฉํด ํ๊ฐ๋ฉ๋๋ค.
์ง์ ๊ตฌํํด๋ณด๊ณ ์ถ์ผ์๋ค๋ฉด, Hugging Face course์ [Question Answering chapter](https://huggingface.co/course/chapter7/7?fw=pt#postprocessing)์ ์ฐธ๊ณ ํ์ธ์.
## ํ๋ จ [[train]]
์ถํํฉ๋๋ค! ์ด ๊ฐ์ด๋์ ๊ฐ์ฅ ์ด๋ ค์ด ๋ถ๋ถ์ ์ฑ๊ณต์ ์ผ๋ก ์ฒ๋ฆฌํ์ผ๋ ์ด์ ๋๋ง์ ๋ชจ๋ธ์ ํ๋ จํ ์ค๋น๊ฐ ๋์์ต๋๋ค.
ํ๋ จ์ ๋ค์๊ณผ ๊ฐ์ ๋จ๊ณ๋ก ์ด๋ฃจ์ด์ ธ ์์ต๋๋ค:
* ์ ์ฒ๋ฆฌ์์์ ๋์ผํ ์ฒดํฌํฌ์ธํธ๋ฅผ ์ฌ์ฉํ๊ธฐ ์ํด [`AutoModelForDocumentQuestionAnswering`]์ผ๋ก ๋ชจ๋ธ์ ๊ฐ์ ธ์ต๋๋ค.
* [`TrainingArguments`]๋ก ํ๋ จ ํ์ดํผํ๋ผ๋ฏธํฐ๋ฅผ ์ ํฉ๋๋ค.
* ์์ ๋ฅผ ๋ฐฐ์น ์ฒ๋ฆฌํ๋ ํจ์๋ฅผ ์ ์ํฉ๋๋ค. ์ฌ๊ธฐ์๋ [`DefaultDataCollator`]๊ฐ ์ ๋นํฉ๋๋ค.
* ๋ชจ๋ธ, ๋ฐ์ดํฐ ์ธํธ, ๋ฐ์ดํฐ ์ฝ๋ ์ดํฐ(Data collator)์ ํจ๊ป [`Trainer`]์ ํ๋ จ ์ธ์๋ค์ ์ ๋ฌํฉ๋๋ค.
* [`~Trainer.train`]์ ํธ์ถํด์ ๋ชจ๋ธ์ ๋ฏธ์ธ ์กฐ์ ํฉ๋๋ค.
```py
>>> from transformers import AutoModelForDocumentQuestionAnswering
>>> model = AutoModelForDocumentQuestionAnswering.from_pretrained(model_checkpoint)
```
[`TrainingArguments`]์์ `output_dir`์ ์ฌ์ฉํ์ฌ ๋ชจ๋ธ์ ์ ์ฅํ ์์น๋ฅผ ์ง์ ํ๊ณ , ์ ์ ํ ํ์ดํผํ๋ผ๋ฏธํฐ๋ฅผ ์ค์ ํฉ๋๋ค.
๋ชจ๋ธ์ ์ปค๋ฎค๋ํฐ์ ๊ณต์ ํ๋ ค๋ฉด `push_to_hub`๋ฅผ `True`๋ก ์ค์ ํ์ธ์ (๋ชจ๋ธ์ ์
๋ก๋ํ๋ ค๋ฉด Hugging Face์ ๋ก๊ทธ์ธํด์ผ ํฉ๋๋ค).
์ด ๊ฒฝ์ฐ `output_dir`์ ๋ชจ๋ธ์ ์ฒดํฌํฌ์ธํธ๋ฅผ ํธ์ํ ๋ ํฌ์งํ ๋ฆฌ์ ์ด๋ฆ์ด ๋ฉ๋๋ค.
```py
>>> from transformers import TrainingArguments
>>> # ๋ณธ์ธ์ ๋ ํฌ์งํ ๋ฆฌ ID๋ก ๋ฐ๊พธ์ธ์
>>> repo_id = "MariaK/layoutlmv2-base-uncased_finetuned_docvqa"
>>> training_args = TrainingArguments(
... output_dir=repo_id,
... per_device_train_batch_size=4,
... num_train_epochs=20,
... save_steps=200,
... logging_steps=50,
... evaluation_strategy="steps",
... learning_rate=5e-5,
... save_total_limit=2,
... remove_unused_columns=False,
... push_to_hub=True,
... )
```
๊ฐ๋จํ ๋ฐ์ดํฐ ์ฝ๋ ์ดํฐ๋ฅผ ์ ์ํ์ฌ ์์ ๋ฅผ ํจ๊ป ๋ฐฐ์นํฉ๋๋ค.
```py
>>> from transformers import DefaultDataCollator
>>> data_collator = DefaultDataCollator()
```
๋ง์ง๋ง์ผ๋ก, ๋ชจ๋ ๊ฒ์ ํ ๊ณณ์ ๋ชจ์ [`~Trainer.train`]์ ํธ์ถํฉ๋๋ค:
```py
>>> from transformers import Trainer
>>> trainer = Trainer(
... model=model,
... args=training_args,
... data_collator=data_collator,
... train_dataset=encoded_train_dataset,
... eval_dataset=encoded_test_dataset,
... tokenizer=processor,
... )
>>> trainer.train()
```
์ต์ข
๋ชจ๋ธ์ ๐ค Hub์ ์ถ๊ฐํ๋ ค๋ฉด, ๋ชจ๋ธ ์นด๋๋ฅผ ์์ฑํ๊ณ `push_to_hub`๋ฅผ ํธ์ถํฉ๋๋ค:
```py
>>> trainer.create_model_card()
>>> trainer.push_to_hub()
```
## ์ถ๋ก [[inference]]
์ด์ LayoutLMv2 ๋ชจ๋ธ์ ๋ฏธ์ธ ์กฐ์ ํ๊ณ ๐ค Hub์ ์
๋ก๋ํ์ผ๋ ์ถ๋ก ์๋ ์ฌ์ฉํ ์ ์์ต๋๋ค.
์ถ๋ก ์ ์ํด ๋ฏธ์ธ ์กฐ์ ๋ ๋ชจ๋ธ์ ์ฌ์ฉํด ๋ณด๋ ๊ฐ์ฅ ๊ฐ๋จํ ๋ฐฉ๋ฒ์ [`Pipeline`]์ ์ฌ์ฉํ๋ ๊ฒ ์
๋๋ค.
์๋ฅผ ๋ค์ด ๋ณด๊ฒ ์ต๋๋ค:
```py
>>> example = dataset["test"][2]
>>> question = example["query"]["en"]
>>> image = example["image"]
>>> print(question)
>>> print(example["answers"])
'Who is โpresidingโ TRRF GENERAL SESSION (PART 1)?'
['TRRF Vice President', 'lee a. waller']
```
๊ทธ ๋ค์, ๋ชจ๋ธ๋ก ๋ฌธ์ ์ง์ ์๋ต์ ํ๊ธฐ ์ํด ํ์ดํ๋ผ์ธ์ ์ธ์คํด์คํํ๊ณ ์ด๋ฏธ์ง + ์ง๋ฌธ ์กฐํฉ์ ์ ๋ฌํฉ๋๋ค.
```py
>>> from transformers import pipeline
>>> qa_pipeline = pipeline("document-question-answering", model="MariaK/layoutlmv2-base-uncased_finetuned_docvqa")
>>> qa_pipeline(image, question)
[{'score': 0.9949808120727539,
'answer': 'Lee A. Waller',
'start': 55,
'end': 57}]
```
์ํ๋ค๋ฉด ํ์ดํ๋ผ์ธ์ ๊ฒฐ๊ณผ๋ฅผ ์๋์ผ๋ก ๋ณต์ ํ ์๋ ์์ต๋๋ค:
1. ์ด๋ฏธ์ง์ ์ง๋ฌธ์ ๊ฐ์ ธ์ ๋ชจ๋ธ์ ํ๋ก์ธ์๋ฅผ ์ฌ์ฉํด ๋ชจ๋ธ์ ๋ง๊ฒ ์ค๋นํฉ๋๋ค.
2. ๋ชจ๋ธ์ ํตํด ๊ฒฐ๊ณผ ๋๋ ์ ์ฒ๋ฆฌ๋ฅผ ์ ๋ฌํฉ๋๋ค.
3. ๋ชจ๋ธ์ ์ด๋ค ํ ํฐ์ด ๋ต๋ณ์ ์์์ ์๋์ง, ์ด๋ค ํ ํฐ์ด ๋ต๋ณ์ด ๋์ ์๋์ง๋ฅผ ๋ํ๋ด๋ `start_logits`์ `end_logits`๋ฅผ ๋ฐํํฉ๋๋ค. ๋ ๋ค (batch_size, sequence_length) ํํ๋ฅผ ๊ฐ์ต๋๋ค.
4. `start_logits`์ `end_logits`์ ๋ง์ง๋ง ์ฐจ์์ ์ต๋๋ก ๋ง๋๋ ๊ฐ์ ์ฐพ์ ์์ `start_idx`์ `end_idx`๋ฅผ ์ป์ต๋๋ค.
5. ํ ํฌ๋์ด์ ๋ก ๋ต๋ณ์ ๋์ฝ๋ฉํฉ๋๋ค.
```py
>>> import torch
>>> from transformers import AutoProcessor
>>> from transformers import AutoModelForDocumentQuestionAnswering
>>> processor = AutoProcessor.from_pretrained("MariaK/layoutlmv2-base-uncased_finetuned_docvqa")
>>> model = AutoModelForDocumentQuestionAnswering.from_pretrained("MariaK/layoutlmv2-base-uncased_finetuned_docvqa")
>>> with torch.no_grad():
... encoding = processor(image.convert("RGB"), question, return_tensors="pt")
... outputs = model(**encoding)
... start_logits = outputs.start_logits
... end_logits = outputs.end_logits
... predicted_start_idx = start_logits.argmax(-1).item()
... predicted_end_idx = end_logits.argmax(-1).item()
>>> processor.tokenizer.decode(encoding.input_ids.squeeze()[predicted_start_idx : predicted_end_idx + 1])
'lee a. waller'
``` | 0 |
hf_public_repos/transformers/docs/source/ko | hf_public_repos/transformers/docs/source/ko/tasks/asr.md | <!--Copyright 2023 The HuggingFace Team. All rights reserved.
Licensed under the Apache License, Version 2.0 (the "License"); you may not use this file except in compliance with
the License. You may obtain a copy of the License at
http://www.apache.org/licenses/LICENSE-2.0
Unless required by applicable law or agreed to in writing, software distributed under the License is distributed on
an "AS IS" BASIS, WITHOUT WARRANTIES OR CONDITIONS OF ANY KIND, either express or implied. See the License for the
specific language governing permissions and limitations under the License.
โ ๏ธ Note that this file is in Markdown but contain specific syntax for our doc-builder (similar to MDX) that may not be
rendered properly in your Markdown viewer.
-->
# ์๋ ์์ฑ ์ธ์[[automatic-speech-recognition]]
[[open-in-colab]]
<Youtube id="TksaY_FDgnk"/>
์๋ ์์ฑ ์ธ์(Automatic Speech Recognition, ASR)์ ์์ฑ ์ ํธ๋ฅผ ํ
์คํธ๋ก ๋ณํํ์ฌ ์์ฑ ์
๋ ฅ ์ํ์ค๋ฅผ ํ
์คํธ ์ถ๋ ฅ์ ๋งคํํฉ๋๋ค.
Siri์ Alexa์ ๊ฐ์ ๊ฐ์ ์ด์์คํดํธ๋ ASR ๋ชจ๋ธ์ ์ฌ์ฉํ์ฌ ์ผ์์ ์ผ๋ก ์ฌ์ฉ์๋ฅผ ๋๊ณ ์์ผ๋ฉฐ, ํ์ ์ค ๋ผ์ด๋ธ ์บก์
๋ฐ ๋ฉ๋ชจ ์์ฑ๊ณผ ๊ฐ์ ์ ์ฉํ ์ฌ์ฉ์ ์นํ์ ์์ฉ ํ๋ก๊ทธ๋จ๋ ๋ง์ด ์์ต๋๋ค.
์ด ๊ฐ์ด๋์์ ์๊ฐํ ๋ด์ฉ์ ์๋์ ๊ฐ์ต๋๋ค:
1. [MInDS-14](https://huggingface.co/datasets/PolyAI/minds14) ๋ฐ์ดํฐ ์ธํธ์์ [Wav2Vec2](https://huggingface.co/facebook/wav2vec2-base)๋ฅผ ๋ฏธ์ธ ์กฐ์ ํ์ฌ ์ค๋์ค๋ฅผ ํ
์คํธ๋ก ๋ณํํฉ๋๋ค.
2. ๋ฏธ์ธ ์กฐ์ ํ ๋ชจ๋ธ์ ์ถ๋ก ์ ์ฌ์ฉํฉ๋๋ค.
<Tip>
์ด ํํ ๋ฆฌ์ผ์์ ์ค๋ช
ํ๋ ์์
์ ๋ค์ ๋ชจ๋ธ ์ํคํ
์ฒ์ ์ํด ์ง์๋ฉ๋๋ค:
<!--This tip is automatically generated by `make fix-copies`, do not fill manually!-->
[Data2VecAudio](../model_doc/data2vec-audio), [Hubert](../model_doc/hubert), [M-CTC-T](../model_doc/mctct), [SEW](../model_doc/sew), [SEW-D](../model_doc/sew-d), [UniSpeech](../model_doc/unispeech), [UniSpeechSat](../model_doc/unispeech-sat), [Wav2Vec2](../model_doc/wav2vec2), [Wav2Vec2-Conformer](../model_doc/wav2vec2-conformer), [WavLM](../model_doc/wavlm)
<!--End of the generated tip-->
</Tip>
์์ํ๊ธฐ ์ ์ ํ์ํ ๋ชจ๋ ๋ผ์ด๋ธ๋ฌ๋ฆฌ๊ฐ ์ค์น๋์ด ์๋์ง ํ์ธํ์ธ์:
```bash
pip install transformers datasets evaluate jiwer
```
Hugging Face ๊ณ์ ์ ๋ก๊ทธ์ธํ๋ฉด ๋ชจ๋ธ์ ์
๋ก๋ํ๊ณ ์ปค๋ฎค๋ํฐ์ ๊ณต์ ํ ์ ์์ต๋๋ค. ํ ํฐ์ ์
๋ ฅํ์ฌ ๋ก๊ทธ์ธํ์ธ์.
```py
>>> from huggingface_hub import notebook_login
>>> notebook_login()
```
## MInDS-14 ๋ฐ์ดํฐ ์ธํธ ๊ฐ์ ธ์ค๊ธฐ[[load-minds-14-dataset]]
๋จผ์ , ๐ค Datasets ๋ผ์ด๋ธ๋ฌ๋ฆฌ์์ [MInDS-14](https://huggingface.co/datasets/PolyAI/minds14) ๋ฐ์ดํฐ ์ธํธ์ ์ผ๋ถ๋ถ์ ๊ฐ์ ธ์ค์ธ์.
์ด๋ ๊ฒ ํ๋ฉด ์ ์ฒด ๋ฐ์ดํฐ ์ธํธ์ ๋ํ ํ๋ จ์ ์๊ฐ์ ๋ค์ด๊ธฐ ์ ์ ๋ชจ๋ ๊ฒ์ด ์๋ํ๋์ง ์คํํ๊ณ ๊ฒ์ฆํ ์ ์์ต๋๋ค.
```py
>>> from datasets import load_dataset, Audio
>>> minds = load_dataset("PolyAI/minds14", name="en-US", split="train[:100]")
```
[`~Dataset.train_test_split`] ๋ฉ์๋๋ฅผ ์ฌ์ฉํ์ฌ ๋ฐ์ดํฐ ์ธํธ์ `train`์ ํ๋ จ ์ธํธ์ ํ
์คํธ ์ธํธ๋ก ๋๋์ธ์:
```py
>>> minds = minds.train_test_split(test_size=0.2)
```
๊ทธ๋ฆฌ๊ณ ๋ฐ์ดํฐ ์ธํธ๋ฅผ ํ์ธํ์ธ์:
```py
>>> minds
DatasetDict({
train: Dataset({
features: ['path', 'audio', 'transcription', 'english_transcription', 'intent_class', 'lang_id'],
num_rows: 16
})
test: Dataset({
features: ['path', 'audio', 'transcription', 'english_transcription', 'intent_class', 'lang_id'],
num_rows: 4
})
})
```
๋ฐ์ดํฐ ์ธํธ์๋ `lang_id`์ `english_transcription`๊ณผ ๊ฐ์ ์ ์ฉํ ์ ๋ณด๊ฐ ๋ง์ด ํฌํจ๋์ด ์์ง๋ง, ์ด ๊ฐ์ด๋์์๋ `audio`์ `transcription`์ ์ด์ ์ ๋ง์ถ ๊ฒ์
๋๋ค. ๋ค๋ฅธ ์ด์ [`~datasets.Dataset.remove_columns`] ๋ฉ์๋๋ฅผ ์ฌ์ฉํ์ฌ ์ ๊ฑฐํ์ธ์:
```py
>>> minds = minds.remove_columns(["english_transcription", "intent_class", "lang_id"])
```
์์๋ฅผ ๋ค์ ํ๋ฒ ํ์ธํด๋ณด์ธ์:
```py
>>> minds["train"][0]
{'audio': {'array': array([-0.00024414, 0. , 0. , ..., 0.00024414,
0.00024414, 0.00024414], dtype=float32),
'path': '/root/.cache/huggingface/datasets/downloads/extracted/f14948e0e84be638dd7943ac36518a4cf3324e8b7aa331c5ab11541518e9368c/en-US~APP_ERROR/602ba9e2963e11ccd901cd4f.wav',
'sampling_rate': 8000},
'path': '/root/.cache/huggingface/datasets/downloads/extracted/f14948e0e84be638dd7943ac36518a4cf3324e8b7aa331c5ab11541518e9368c/en-US~APP_ERROR/602ba9e2963e11ccd901cd4f.wav',
'transcription': "hi I'm trying to use the banking app on my phone and currently my checking and savings account balance is not refreshing"}
```
๋ ๊ฐ์ ํ๋๊ฐ ์์ต๋๋ค:
- `audio`: ์ค๋์ค ํ์ผ์ ๊ฐ์ ธ์ค๊ณ ๋ฆฌ์ํ๋งํ๊ธฐ ์ํด ํธ์ถํด์ผ ํ๋ ์์ฑ ์ ํธ์ 1์ฐจ์ `array(๋ฐฐ์ด)`
- `transcription`: ๋ชฉํ ํ
์คํธ
## ์ ์ฒ๋ฆฌ[[preprocess]]
๋ค์์ผ๋ก ์ค๋์ค ์ ํธ๋ฅผ ์ฒ๋ฆฌํ๊ธฐ ์ํ Wav2Vec2 ํ๋ก์ธ์๋ฅผ ๊ฐ์ ธ์ต๋๋ค:
```py
>>> from transformers import AutoProcessor
>>> processor = AutoProcessor.from_pretrained("facebook/wav2vec2-base")
```
MInDS-14 ๋ฐ์ดํฐ ์ธํธ์ ์ํ๋ง ๋ ์ดํธ๋ 8000kHz์ด๋ฏ๋ก([๋ฐ์ดํฐ ์ธํธ ์นด๋](https://huggingface.co/datasets/PolyAI/minds14)์์ ํ์ธ), ์ฌ์ ํ๋ จ๋ Wav2Vec2 ๋ชจ๋ธ์ ์ฌ์ฉํ๋ ค๋ฉด ๋ฐ์ดํฐ ์ธํธ๋ฅผ 16000kHz๋ก ๋ฆฌ์ํ๋งํด์ผ ํฉ๋๋ค:
```py
>>> minds = minds.cast_column("audio", Audio(sampling_rate=16_000))
>>> minds["train"][0]
{'audio': {'array': array([-2.38064706e-04, -1.58618059e-04, -5.43987835e-06, ...,
2.78103951e-04, 2.38446111e-04, 1.18740834e-04], dtype=float32),
'path': '/root/.cache/huggingface/datasets/downloads/extracted/f14948e0e84be638dd7943ac36518a4cf3324e8b7aa331c5ab11541518e9368c/en-US~APP_ERROR/602ba9e2963e11ccd901cd4f.wav',
'sampling_rate': 16000},
'path': '/root/.cache/huggingface/datasets/downloads/extracted/f14948e0e84be638dd7943ac36518a4cf3324e8b7aa331c5ab11541518e9368c/en-US~APP_ERROR/602ba9e2963e11ccd901cd4f.wav',
'transcription': "hi I'm trying to use the banking app on my phone and currently my checking and savings account balance is not refreshing"}
```
์์ 'transcription'์์ ๋ณผ ์ ์๋ฏ์ด ํ
์คํธ๋ ๋๋ฌธ์์ ์๋ฌธ์๊ฐ ์์ฌ ์์ต๋๋ค. Wav2Vec2 ํ ํฌ๋์ด์ ๋ ๋๋ฌธ์ ๋ฌธ์์ ๋ํด์๋ง ํ๋ จ๋์ด ์์ผ๋ฏ๋ก ํ
์คํธ๊ฐ ํ ํฌ๋์ด์ ์ ์ดํ์ ์ผ์นํ๋์ง ํ์ธํด์ผ ํฉ๋๋ค:
```py
>>> def uppercase(example):
... return {"transcription": example["transcription"].upper()}
>>> minds = minds.map(uppercase)
```
์ด์ ๋ค์ ์์
์ ์ํํ ์ ์ฒ๋ฆฌ ํจ์๋ฅผ ๋ง๋ค์ด๋ณด๊ฒ ์ต๋๋ค:
1. `audio` ์ด์ ํธ์ถํ์ฌ ์ค๋์ค ํ์ผ์ ๊ฐ์ ธ์ค๊ณ ๋ฆฌ์ํ๋งํฉ๋๋ค.
2. ์ค๋์ค ํ์ผ์์ `input_values`๋ฅผ ์ถ์ถํ๊ณ ํ๋ก์ธ์๋ก `transcription` ์ด์ ํ ํฐํํฉ๋๋ค.
```py
>>> def prepare_dataset(batch):
... audio = batch["audio"]
... batch = processor(audio["array"], sampling_rate=audio["sampling_rate"], text=batch["transcription"])
... batch["input_length"] = len(batch["input_values"][0])
... return batch
```
์ ์ฒด ๋ฐ์ดํฐ ์ธํธ์ ์ ์ฒ๋ฆฌ ํจ์๋ฅผ ์ ์ฉํ๋ ค๋ฉด ๐ค Datasets [`~datasets.Dataset.map`] ํจ์๋ฅผ ์ฌ์ฉํ์ธ์. `num_proc` ๋งค๊ฐ๋ณ์๋ฅผ ์ฌ์ฉํ์ฌ ํ๋ก์ธ์ค ์๋ฅผ ๋๋ฆฌ๋ฉด `map`์ ์๋๋ฅผ ๋์ผ ์ ์์ต๋๋ค. [`~datasets.Dataset.remove_columns`] ๋ฉ์๋๋ฅผ ์ฌ์ฉํ์ฌ ํ์ํ์ง ์์ ์ด์ ์ ๊ฑฐํ์ธ์:
```py
>>> encoded_minds = minds.map(prepare_dataset, remove_columns=minds.column_names["train"], num_proc=4)
```
๐ค Transformers์๋ ์๋ ์์ฑ ์ธ์์ฉ ๋ฐ์ดํฐ ์ฝ๋ ์ดํฐ๊ฐ ์์ผ๋ฏ๋ก ์์ ๋ฐฐ์น๋ฅผ ์์ฑํ๋ ค๋ฉด [`DataCollatorWithPadding`]์ ์กฐ์ ํด์ผ ํฉ๋๋ค. ์ด๋ ๊ฒ ํ๋ฉด ๋ฐ์ดํฐ ์ฝ๋ ์ดํฐ๋ ํ
์คํธ์ ๋ ์ด๋ธ์ ๋ฐฐ์น์์ ๊ฐ์ฅ ๊ธด ์์์ ๊ธธ์ด์ ๋์ ์ผ๋ก ํจ๋ฉํ์ฌ ๊ธธ์ด๋ฅผ ๊ท ์ผํ๊ฒ ํฉ๋๋ค. `tokenizer` ํจ์์์ `padding=True`๋ฅผ ์ค์ ํ์ฌ ํ
์คํธ๋ฅผ ํจ๋ฉํ ์ ์์ง๋ง, ๋์ ํจ๋ฉ์ด ๋ ํจ์จ์ ์
๋๋ค.
๋ค๋ฅธ ๋ฐ์ดํฐ ์ฝ๋ ์ดํฐ์ ๋ฌ๋ฆฌ ์ด ํน์ ๋ฐ์ดํฐ ์ฝ๋ ์ดํฐ๋ `input_values`์ `labels`์ ๋ํด ๋ค๋ฅธ ํจ๋ฉ ๋ฐฉ๋ฒ์ ์ ์ฉํด์ผ ํฉ๋๋ค.
```py
>>> import torch
>>> from dataclasses import dataclass, field
>>> from typing import Any, Dict, List, Optional, Union
>>> @dataclass
... class DataCollatorCTCWithPadding:
... processor: AutoProcessor
... padding: Union[bool, str] = "longest"
... def __call__(self, features: List[Dict[str, Union[List[int], torch.Tensor]]]) -> Dict[str, torch.Tensor]:
... # ์
๋ ฅ๊ณผ ๋ ์ด๋ธ์ ๋ถํ ํฉ๋๋ค
... # ๊ธธ์ด๊ฐ ๋ค๋ฅด๊ณ , ๊ฐ๊ฐ ๋ค๋ฅธ ํจ๋ฉ ๋ฐฉ๋ฒ์ ์ฌ์ฉํด์ผ ํ๊ธฐ ๋๋ฌธ์
๋๋ค
... input_features = [{"input_values": feature["input_values"][0]} for feature in features]
... label_features = [{"input_ids": feature["labels"]} for feature in features]
... batch = self.processor.pad(input_features, padding=self.padding, return_tensors="pt")
... labels_batch = self.processor.pad(labels=label_features, padding=self.padding, return_tensors="pt")
... # ํจ๋ฉ์ ๋ํด ์์ค์ ์ ์ฉํ์ง ์๋๋ก -100์ผ๋ก ๋์ฒดํฉ๋๋ค
... labels = labels_batch["input_ids"].masked_fill(labels_batch.attention_mask.ne(1), -100)
... batch["labels"] = labels
... return batch
```
์ด์ `DataCollatorForCTCWithPadding`์ ์ธ์คํด์คํํฉ๋๋ค:
```py
>>> data_collator = DataCollatorCTCWithPadding(processor=processor, padding="longest")
```
## ํ๊ฐํ๊ธฐ[[evaluate]]
ํ๋ จ ์ค์ ํ๊ฐ ์งํ๋ฅผ ํฌํจํ๋ฉด ๋ชจ๋ธ์ ์ฑ๋ฅ์ ํ๊ฐํ๋ ๋ฐ ๋์์ด ๋๋ ๊ฒฝ์ฐ๊ฐ ๋ง์ต๋๋ค. ๐ค [Evaluate](https://huggingface.co/docs/evaluate/index) ๋ผ์ด๋ธ๋ฌ๋ฆฌ๋ฅผ ์ฌ์ฉํ๋ฉด ํ๊ฐ ๋ฐฉ๋ฒ์ ๋น ๋ฅด๊ฒ ๋ถ๋ฌ์ฌ ์ ์์ต๋๋ค.
์ด ์์
์์๋ [๋จ์ด ์ค๋ฅ์จ(Word Error Rate, WER)](https://huggingface.co/spaces/evaluate-metric/wer) ํ๊ฐ ์งํ๋ฅผ ๊ฐ์ ธ์ต๋๋ค.
(ํ๊ฐ ์งํ๋ฅผ ๋ถ๋ฌ์ค๊ณ ๊ณ์ฐํ๋ ๋ฐฉ๋ฒ์ ๐ค Evaluate [๋๋ฌ๋ณด๊ธฐ](https://huggingface.co/docs/evaluate/a_quick_tour)๋ฅผ ์ฐธ์กฐํ์ธ์):
```py
>>> import evaluate
>>> wer = evaluate.load("wer")
```
๊ทธ๋ฐ ๋ค์ ์์ธก๊ฐ๊ณผ ๋ ์ด๋ธ์ [`~evaluate.EvaluationModule.compute`]์ ์ ๋ฌํ์ฌ WER์ ๊ณ์ฐํ๋ ํจ์๋ฅผ ๋ง๋ญ๋๋ค:
```py
>>> import numpy as np
>>> def compute_metrics(pred):
... pred_logits = pred.predictions
... pred_ids = np.argmax(pred_logits, axis=-1)
... pred.label_ids[pred.label_ids == -100] = processor.tokenizer.pad_token_id
... pred_str = processor.batch_decode(pred_ids)
... label_str = processor.batch_decode(pred.label_ids, group_tokens=False)
... wer = wer.compute(predictions=pred_str, references=label_str)
... return {"wer": wer}
```
์ด์ `compute_metrics` ํจ์๋ฅผ ์ฌ์ฉํ ์ค๋น๊ฐ ๋์์ผ๋ฉฐ, ํ๋ จ์ ์ค์ ํ ๋ ์ด ํจ์๋ก ๋๋์์ฌ ๊ฒ์
๋๋ค.
## ํ๋ จํ๊ธฐ[[train]]
<frameworkcontent>
<pt>
<Tip>
[`Trainer`]๋ก ๋ชจ๋ธ์ ๋ฏธ์ธ ์กฐ์ ํ๋ ๊ฒ์ด ์ต์ํ์ง ์๋ค๋ฉด, [์ฌ๊ธฐ](../training#train-with-pytorch-trainer)์์ ๊ธฐ๋ณธ ํํ ๋ฆฌ์ผ์ ํ์ธํด๋ณด์ธ์!
</Tip>
์ด์ ๋ชจ๋ธ ํ๋ จ์ ์์ํ ์ค๋น๊ฐ ๋์์ต๋๋ค! [`AutoModelForCTC`]๋ก Wav2Vec2๋ฅผ ๊ฐ์ ธ์ค์ธ์. `ctc_loss_reduction` ๋งค๊ฐ๋ณ์๋ก CTC ์์ค์ ์ ์ฉํ ์ถ์(reduction) ๋ฐฉ๋ฒ์ ์ง์ ํ์ธ์. ๊ธฐ๋ณธ๊ฐ์ธ ํฉ๊ณ ๋์ ํ๊ท ์ ์ฌ์ฉํ๋ ๊ฒ์ด ๋ ์ข์ ๊ฒฝ์ฐ๊ฐ ๋ง์ต๋๋ค:
```py
>>> from transformers import AutoModelForCTC, TrainingArguments, Trainer
>>> model = AutoModelForCTC.from_pretrained(
... "facebook/wav2vec2-base",
... ctc_loss_reduction="mean",
... pad_token_id=processor.tokenizer.pad_token_id,
... )
```
์ด์ ์ธ ๋จ๊ณ๋ง ๋จ์์ต๋๋ค:
1. [`TrainingArguments`]์์ ํ๋ จ ํ์ดํผํ๋ผ๋ฏธํฐ๋ฅผ ์ ์ํ์ธ์. `output_dir`์ ๋ชจ๋ธ์ ์ ์ฅํ ๊ฒฝ๋ก๋ฅผ ์ง์ ํ๋ ์ ์ผํ ํ์ ๋งค๊ฐ๋ณ์์
๋๋ค. `push_to_hub=True`๋ฅผ ์ค์ ํ์ฌ ๋ชจ๋ธ์ Hub์ ์
๋ก๋ ํ ์ ์์ต๋๋ค(๋ชจ๋ธ์ ์
๋ก๋ํ๋ ค๋ฉด Hugging Face์ ๋ก๊ทธ์ธํด์ผ ํฉ๋๋ค). [`Trainer`]๋ ๊ฐ ์ํญ๋ง๋ค WER์ ํ๊ฐํ๊ณ ํ๋ จ ์ฒดํฌํฌ์ธํธ๋ฅผ ์ ์ฅํฉ๋๋ค.
2. ๋ชจ๋ธ, ๋ฐ์ดํฐ ์ธํธ, ํ ํฌ๋์ด์ , ๋ฐ์ดํฐ ์ฝ๋ ์ดํฐ, `compute_metrics` ํจ์์ ํจ๊ป [`Trainer`]์ ํ๋ จ ์ธ์๋ฅผ ์ ๋ฌํ์ธ์.
3. [`~Trainer.train`]์ ํธ์ถํ์ฌ ๋ชจ๋ธ์ ๋ฏธ์ธ ์กฐ์ ํ์ธ์.
```py
>>> training_args = TrainingArguments(
... output_dir="my_awesome_asr_mind_model",
... per_device_train_batch_size=8,
... gradient_accumulation_steps=2,
... learning_rate=1e-5,
... warmup_steps=500,
... max_steps=2000,
... gradient_checkpointing=True,
... fp16=True,
... group_by_length=True,
... evaluation_strategy="steps",
... per_device_eval_batch_size=8,
... save_steps=1000,
... eval_steps=1000,
... logging_steps=25,
... load_best_model_at_end=True,
... metric_for_best_model="wer",
... greater_is_better=False,
... push_to_hub=True,
... )
>>> trainer = Trainer(
... model=model,
... args=training_args,
... train_dataset=encoded_minds["train"],
... eval_dataset=encoded_minds["test"],
... tokenizer=processor.feature_extractor,
... data_collator=data_collator,
... compute_metrics=compute_metrics,
... )
>>> trainer.train()
```
ํ๋ จ์ด ์๋ฃ๋๋ฉด ๋ชจ๋๊ฐ ๋ชจ๋ธ์ ์ฌ์ฉํ ์ ์๋๋ก [`~transformers.Trainer.push_to_hub`] ๋ฉ์๋๋ฅผ ์ฌ์ฉํ์ฌ ๋ชจ๋ธ์ Hub์ ๊ณต์ ํ์ธ์:
```py
>>> trainer.push_to_hub()
```
</pt>
</frameworkcontent>
<Tip>
์๋ ์์ฑ ์ธ์์ ์ํด ๋ชจ๋ธ์ ๋ฏธ์ธ ์กฐ์ ํ๋ ๋ ์์ธํ ์์ ๋ ์์ด ์๋ ์์ฑ ์ธ์์ ์ํ [๋ธ๋ก๊ทธ ํฌ์คํธ](https://huggingface.co/blog/fine-tune-wav2vec2-english)์ ๋ค๊ตญ์ด ์๋ ์์ฑ ์ธ์์ ์ํ [ํฌ์คํธ](https://huggingface.co/blog/fine-tune-xlsr-wav2vec2)๋ฅผ ์ฐธ์กฐํ์ธ์.
</Tip>
## ์ถ๋ก ํ๊ธฐ[[inference]]
์ข์์, ์ด์ ๋ชจ๋ธ์ ๋ฏธ์ธ ์กฐ์ ํ์ผ๋ ์ถ๋ก ์ ์ฌ์ฉํ ์ ์์ต๋๋ค!
์ถ๋ก ์ ์ฌ์ฉํ ์ค๋์ค ํ์ผ์ ๊ฐ์ ธ์ค์ธ์. ํ์ํ ๊ฒฝ์ฐ ์ค๋์ค ํ์ผ์ ์ํ๋ง ๋น์จ์ ๋ชจ๋ธ์ ์ํ๋ง ๋ ์ดํธ์ ๋ง๊ฒ ๋ฆฌ์ํ๋งํ๋ ๊ฒ์ ์์ง ๋ง์ธ์!
```py
>>> from datasets import load_dataset, Audio
>>> dataset = load_dataset("PolyAI/minds14", "en-US", split="train")
>>> dataset = dataset.cast_column("audio", Audio(sampling_rate=16000))
>>> sampling_rate = dataset.features["audio"].sampling_rate
>>> audio_file = dataset[0]["audio"]["path"]
```
์ถ๋ก ์ ์ํด ๋ฏธ์ธ ์กฐ์ ๋ ๋ชจ๋ธ์ ์ํํด๋ณด๋ ๊ฐ์ฅ ๊ฐ๋จํ ๋ฐฉ๋ฒ์ [`pipeline`]์ ์ฌ์ฉํ๋ ๊ฒ์
๋๋ค. ๋ชจ๋ธ์ ์ฌ์ฉํ์ฌ ์๋ ์์ฑ ์ธ์์ ์ํ `pipeline`์ ์ธ์คํด์คํํ๊ณ ์ค๋์ค ํ์ผ์ ์ ๋ฌํ์ธ์:
```py
>>> from transformers import pipeline
>>> transcriber = pipeline("automatic-speech-recognition", model="stevhliu/my_awesome_asr_minds_model")
>>> transcriber(audio_file)
{'text': 'I WOUD LIKE O SET UP JOINT ACOUNT WTH Y PARTNER'}
```
<Tip>
ํ
์คํธ๋ก ๋ณํ๋ ๊ฒฐ๊ณผ๊ฐ ๊ฝค ๊ด์ฐฎ์ง๋ง ๋ ์ข์ ์๋ ์์ต๋๋ค! ๋ ๋์ ๊ฒฐ๊ณผ๋ฅผ ์ป์ผ๋ ค๋ฉด ๋ ๋ง์ ์์ ๋ก ๋ชจ๋ธ์ ๋ฏธ์ธ ์กฐ์ ํ์ธ์!
</Tip>
`pipeline`์ ๊ฒฐ๊ณผ๋ฅผ ์๋์ผ๋ก ์ฌํํ ์๋ ์์ต๋๋ค:
<frameworkcontent>
<pt>
์ค๋์ค ํ์ผ๊ณผ ํ
์คํธ๋ฅผ ์ ์ฒ๋ฆฌํ๊ณ PyTorch ํ
์๋ก `input`์ ๋ฐํํ ํ๋ก์ธ์๋ฅผ ๊ฐ์ ธ์ค์ธ์:
```py
>>> from transformers import AutoProcessor
>>> processor = AutoProcessor.from_pretrained("stevhliu/my_awesome_asr_mind_model")
>>> inputs = processor(dataset[0]["audio"]["array"], sampling_rate=sampling_rate, return_tensors="pt")
```
์
๋ ฅ์ ๋ชจ๋ธ์ ์ ๋ฌํ๊ณ ๋ก์ง์ ๋ฐํํ์ธ์:
```py
>>> from transformers import AutoModelForCTC
>>> model = AutoModelForCTC.from_pretrained("stevhliu/my_awesome_asr_mind_model")
>>> with torch.no_grad():
... logits = model(**inputs).logits
```
๊ฐ์ฅ ๋์ ํ๋ฅ ์ `input_ids`๋ฅผ ์์ธกํ๊ณ , ํ๋ก์ธ์๋ฅผ ์ฌ์ฉํ์ฌ ์์ธก๋ `input_ids`๋ฅผ ๋ค์ ํ
์คํธ๋ก ๋์ฝ๋ฉํ์ธ์:
```py
>>> import torch
>>> predicted_ids = torch.argmax(logits, dim=-1)
>>> transcription = processor.batch_decode(predicted_ids)
>>> transcription
['I WOUL LIKE O SET UP JOINT ACOUNT WTH Y PARTNER']
```
</pt>
</frameworkcontent> | 0 |
hf_public_repos/transformers/docs/source/ko | hf_public_repos/transformers/docs/source/ko/tasks/zero_shot_object_detection.md | <!--Copyright 2023 The HuggingFace Team. All rights reserved.
Licensed under the Apache License, Version 2.0 (the "License"); you may not use this file except in compliance with
the License. You may obtain a copy of the License at
http://www.apache.org/licenses/LICENSE-2.0
Unless required by applicable law or agreed to in writing, software distributed under the License is distributed on
an "AS IS" BASIS, WITHOUT WARRANTIES OR CONDITIONS OF ANY KIND, either express or implied. See the License for the
specific language governing permissions and limitations under the License.
โ ๏ธ Note that this file is in Markdown but contain specific syntax for our doc-builder (similar to MDX) that may not be
rendered properly in your Markdown viewer.
-->
# ์ ๋ก์ท(zero-shot) ๊ฐ์ฒด ํ์ง[[zeroshot-object-detection]]
[[open-in-colab]]
์ผ๋ฐ์ ์ผ๋ก [๊ฐ์ฒด ํ์ง](object_detection)์ ์ฌ์ฉ๋๋ ๋ชจ๋ธ์ ํ์ตํ๊ธฐ ์ํด์๋ ๋ ์ด๋ธ์ด ์ง์ ๋ ์ด๋ฏธ์ง ๋ฐ์ดํฐ ์ธํธ๊ฐ ํ์ํฉ๋๋ค.
๊ทธ๋ฆฌ๊ณ ํ์ต ๋ฐ์ดํฐ์ ์กด์ฌํ๋ ํด๋์ค(๋ ์ด๋ธ)๋ง ํ์งํ ์ ์๋ค๋ ํ๊ณ์ ์ด ์์ต๋๋ค.
๋ค๋ฅธ ๋ฐฉ์์ ์ฌ์ฉํ๋ [OWL-ViT](../model_doc/owlvit) ๋ชจ๋ธ๋ก ์ ๋ก์ท ๊ฐ์ฒด ํ์ง๊ฐ ๊ฐ๋ฅํฉ๋๋ค.
OWL-ViT๋ ๊ฐ๋ฐฉํ ์ดํ(open-vocabulary) ๊ฐ์ฒด ํ์ง๊ธฐ์
๋๋ค.
์ฆ, ๋ ์ด๋ธ์ด ์ง์ ๋ ๋ฐ์ดํฐ ์ธํธ์ ๋ฏธ์ธ ์กฐ์ ํ์ง ์๊ณ ์์ ํ
์คํธ ์ฟผ๋ฆฌ๋ฅผ ๊ธฐ๋ฐ์ผ๋ก ์ด๋ฏธ์ง์์ ๊ฐ์ฒด๋ฅผ ํ์งํ ์ ์์ต๋๋ค.
OWL-ViT ๋ชจ๋ธ์ ๋ฉํฐ ๋ชจ๋ฌ ํํ์ ํ์ฉํด ๊ฐ๋ฐฉํ ์ดํ ํ์ง(open-vocabulary detection)๋ฅผ ์ํํฉ๋๋ค.
[CLIP](../model_doc/clip) ๋ชจ๋ธ์ ๊ฒฝ๋ํ(lightweight)๋ ๊ฐ์ฒด ๋ถ๋ฅ์ ์ง์ญํ(localization) ํค๋๋ฅผ ๊ฒฐํฉํฉ๋๋ค.
๊ฐ๋ฐฉํ ์ดํ ํ์ง๋ CLIP์ ํ
์คํธ ์ธ์ฝ๋๋ก free-text ์ฟผ๋ฆฌ๋ฅผ ์๋ฒ ๋ฉํ๊ณ , ๊ฐ์ฒด ๋ถ๋ฅ์ ์ง์ญํ ํค๋์ ์
๋ ฅ์ผ๋ก ์ฌ์ฉํฉ๋๋ค.
์ด๋ฏธ์ง์ ํด๋น ํ
์คํธ ์ค๋ช
์ ์ฐ๊ฒฐํ๋ฉด ViT๊ฐ ์ด๋ฏธ์ง ํจ์น(image patches)๋ฅผ ์
๋ ฅ์ผ๋ก ์ฒ๋ฆฌํฉ๋๋ค.
OWL-ViT ๋ชจ๋ธ์ ์ ์๋ค์ CLIP ๋ชจ๋ธ์ ์ฒ์๋ถํฐ ํ์ต(scratch learning)ํ ํ์, bipartite matching loss๋ฅผ ์ฌ์ฉํ์ฌ ํ์ค ๊ฐ์ฒด ์ธ์ ๋ฐ์ดํฐ์
์ผ๋ก OWL-ViT ๋ชจ๋ธ์ ๋ฏธ์ธ ์กฐ์ ํ์ต๋๋ค.
์ด ์ ๊ทผ ๋ฐฉ์์ ์ฌ์ฉํ๋ฉด ๋ชจ๋ธ์ ๋ ์ด๋ธ์ด ์ง์ ๋ ๋ฐ์ดํฐ ์ธํธ์ ๋ํ ์ฌ์ ํ์ต ์์ด๋ ํ
์คํธ ์ค๋ช
์ ๊ธฐ๋ฐ์ผ๋ก ๊ฐ์ฒด๋ฅผ ํ์งํ ์ ์์ต๋๋ค.
์ด๋ฒ ๊ฐ์ด๋์์๋ OWL-ViT ๋ชจ๋ธ์ ์ฌ์ฉ๋ฒ์ ๋ค๋ฃฐ ๊ฒ์
๋๋ค:
- ํ
์คํธ ํ๋กฌํํธ ๊ธฐ๋ฐ ๊ฐ์ฒด ํ์ง
- ์ผ๊ด ๊ฐ์ฒด ํ์ง
- ์ด๋ฏธ์ง ๊ฐ์ด๋ ๊ฐ์ฒด ํ์ง
์์ํ๊ธฐ ์ ์ ํ์ํ ๋ผ์ด๋ธ๋ฌ๋ฆฌ๊ฐ ๋ชจ๋ ์ค์น๋์ด ์๋์ง ํ์ธํ์ธ์:
```bash
pip install -q transformers
```
## ์ ๋ก์ท(zero-shot) ๊ฐ์ฒด ํ์ง ํ์ดํ๋ผ์ธ[[zeroshot-object-detection-pipeline]]
[`pipeline`]์ ํ์ฉํ๋ฉด ๊ฐ์ฅ ๊ฐ๋จํ๊ฒ OWL-ViT ๋ชจ๋ธ์ ์ถ๋ก ํด๋ณผ ์ ์์ต๋๋ค.
[Hugging Face Hub์ ์
๋ก๋๋ ์ฒดํฌํฌ์ธํธ](https://huggingface.co/models?pipeline_tag=zero-shot-image-classification&sort=downloads)์์ ์ ๋ก์ท(zero-shot) ๊ฐ์ฒด ํ์ง์ฉ ํ์ดํ๋ผ์ธ์ ์ธ์คํด์คํํฉ๋๋ค:
```python
>>> from transformers import pipeline
>>> checkpoint = "google/owlvit-base-patch32"
>>> detector = pipeline(model=checkpoint, task="zero-shot-object-detection")
```
๋ค์์ผ๋ก, ๊ฐ์ฒด๋ฅผ ํ์งํ๊ณ ์ถ์ ์ด๋ฏธ์ง๋ฅผ ์ ํํ์ธ์.
์ฌ๊ธฐ์๋ [NASA](https://www.nasa.gov/multimedia/imagegallery/index.html) Great Images ๋ฐ์ดํฐ ์ธํธ์ ์ผ๋ถ์ธ ์ฐ์ฃผ๋นํ์ฌ ์์ผ๋ฆฐ ์ฝ๋ฆฐ์ค(Eileen Collins) ์ฌ์ง์ ์ฌ์ฉํ๊ฒ ์ต๋๋ค.
```py
>>> import skimage
>>> import numpy as np
>>> from PIL import Image
>>> image = skimage.data.astronaut()
>>> image = Image.fromarray(np.uint8(image)).convert("RGB")
>>> image
```
<div class="flex justify-center">
<img src="https://huggingface.co/datasets/huggingface/documentation-images/resolve/main/transformers/tasks/zero-sh-obj-detection_1.png" alt="Astronaut Eileen Collins"/>
</div>
์ด๋ฏธ์ง์ ํด๋น ์ด๋ฏธ์ง์ ํ๋ณด ๋ ์ด๋ธ์ ํ์ดํ๋ผ์ธ์ผ๋ก ์ ๋ฌํฉ๋๋ค.
์ฌ๊ธฐ์๋ ์ด๋ฏธ์ง๋ฅผ ์ง์ ์ ๋ฌํ์ง๋ง, ์ปดํจํฐ์ ์ ์ฅ๋ ์ด๋ฏธ์ง์ ๊ฒฝ๋ก๋ url๋ก ์ ๋ฌํ ์๋ ์์ต๋๋ค.
candidate_labels๋ ์ด ์์์ฒ๋ผ ๊ฐ๋จํ ๋จ์ด์ผ ์๋ ์๊ณ ์ข ๋ ์ค๋ช
์ ์ธ ๋จ์ด์ผ ์๋ ์์ต๋๋ค.
๋ํ, ์ด๋ฏธ์ง๋ฅผ ๊ฒ์(query)ํ๋ ค๋ ๋ชจ๋ ํญ๋ชฉ์ ๋ํ ํ
์คํธ ์ค๋ช
๋ ์ ๋ฌํฉ๋๋ค.
```py
>>> predictions = detector(
... image,
... candidate_labels=["human face", "rocket", "nasa badge", "star-spangled banner"],
... )
>>> predictions
[{'score': 0.3571370542049408,
'label': 'human face',
'box': {'xmin': 180, 'ymin': 71, 'xmax': 271, 'ymax': 178}},
{'score': 0.28099656105041504,
'label': 'nasa badge',
'box': {'xmin': 129, 'ymin': 348, 'xmax': 206, 'ymax': 427}},
{'score': 0.2110239565372467,
'label': 'rocket',
'box': {'xmin': 350, 'ymin': -1, 'xmax': 468, 'ymax': 288}},
{'score': 0.13790413737297058,
'label': 'star-spangled banner',
'box': {'xmin': 1, 'ymin': 1, 'xmax': 105, 'ymax': 509}},
{'score': 0.11950037628412247,
'label': 'nasa badge',
'box': {'xmin': 277, 'ymin': 338, 'xmax': 327, 'ymax': 380}},
{'score': 0.10649408400058746,
'label': 'rocket',
'box': {'xmin': 358, 'ymin': 64, 'xmax': 424, 'ymax': 280}}]
```
์ด์ ์์ธก๊ฐ์ ์๊ฐํํด๋ด
์๋ค:
```py
>>> from PIL import ImageDraw
>>> draw = ImageDraw.Draw(image)
>>> for prediction in predictions:
... box = prediction["box"]
... label = prediction["label"]
... score = prediction["score"]
... xmin, ymin, xmax, ymax = box.values()
... draw.rectangle((xmin, ymin, xmax, ymax), outline="red", width=1)
... draw.text((xmin, ymin), f"{label}: {round(score,2)}", fill="white")
>>> image
```
<div class="flex justify-center">
<img src="https://huggingface.co/datasets/huggingface/documentation-images/resolve/main/transformers/tasks/zero-sh-obj-detection_2.png" alt="Visualized predictions on NASA image"/>
</div>
## ํ
์คํธ ํ๋กฌํํธ ๊ธฐ๋ฐ ๊ฐ์ฒด ํ์ง[[textprompted-zeroshot-object-detection-by-hand]]
์ ๋ก์ท ๊ฐ์ฒด ํ์ง ํ์ดํ๋ผ์ธ ์ฌ์ฉ๋ฒ์ ๋ํด ์ดํด๋ณด์์ผ๋, ์ด์ ๋์ผํ ๊ฒฐ๊ณผ๋ฅผ ๋ณต์ ํด๋ณด๊ฒ ์ต๋๋ค.
[Hugging Face Hub์ ์
๋ก๋๋ ์ฒดํฌํฌ์ธํธ](https://huggingface.co/models?other=owlvit)์์ ๊ด๋ จ ๋ชจ๋ธ๊ณผ ํ๋ก์ธ์๋ฅผ ๊ฐ์ ธ์ค๋ ๊ฒ์ผ๋ก ์์ํฉ๋๋ค.
์ฌ๊ธฐ์๋ ์ด์ ๊ณผ ๋์ผํ ์ฒดํฌํฌ์ธํธ๋ฅผ ์ฌ์ฉํ๊ฒ ์ต๋๋ค:
```py
>>> from transformers import AutoProcessor, AutoModelForZeroShotObjectDetection
>>> model = AutoModelForZeroShotObjectDetection.from_pretrained(checkpoint)
>>> processor = AutoProcessor.from_pretrained(checkpoint)
```
๋ค๋ฅธ ์ด๋ฏธ์ง๋ฅผ ์ฌ์ฉํด ๋ณด๊ฒ ์ต๋๋ค:
```py
>>> import requests
>>> url = "https://unsplash.com/photos/oj0zeY2Ltk4/download?ixid=MnwxMjA3fDB8MXxzZWFyY2h8MTR8fHBpY25pY3xlbnwwfHx8fDE2Nzc0OTE1NDk&force=true&w=640"
>>> im = Image.open(requests.get(url, stream=True).raw)
>>> im
```
<div class="flex justify-center">
<img src="https://huggingface.co/datasets/huggingface/documentation-images/resolve/main/transformers/tasks/zero-sh-obj-detection_3.png" alt="Beach photo"/>
</div>
ํ๋ก์ธ์๋ฅผ ์ฌ์ฉํด ๋ชจ๋ธ์ ์
๋ ฅ์ ์ค๋นํฉ๋๋ค.
ํ๋ก์ธ์๋ ๋ชจ๋ธ์ ์
๋ ฅ์ผ๋ก ์ฌ์ฉํ๊ธฐ ์ํด ์ด๋ฏธ์ง ํฌ๊ธฐ๋ฅผ ๋ณํํ๊ณ ์ ๊ทํํ๋ ์ด๋ฏธ์ง ํ๋ก์ธ์์ ํ
์คํธ ์
๋ ฅ์ ์ฒ๋ฆฌํ๋ [`CLIPTokenizer`]๋ก ๊ตฌ์ฑ๋ฉ๋๋ค.
```py
>>> text_queries = ["hat", "book", "sunglasses", "camera"]
>>> inputs = processor(text=text_queries, images=im, return_tensors="pt")
```
๋ชจ๋ธ์ ์
๋ ฅ์ ์ ๋ฌํ๊ณ ๊ฒฐ๊ณผ๋ฅผ ํ์ฒ๋ฆฌ ๋ฐ ์๊ฐํํฉ๋๋ค.
์ด๋ฏธ์ง ํ๋ก์ธ์๊ฐ ๋ชจ๋ธ์ ์ด๋ฏธ์ง๋ฅผ ์
๋ ฅํ๊ธฐ ์ ์ ์ด๋ฏธ์ง ํฌ๊ธฐ๋ฅผ ์กฐ์ ํ๊ธฐ ๋๋ฌธ์, [`~OwlViTImageProcessor.post_process_object_detection`] ๋ฉ์๋๋ฅผ ์ฌ์ฉํด
์์ธก๊ฐ์ ๋ฐ์ด๋ฉ ๋ฐ์ค(bounding box)๊ฐ ์๋ณธ ์ด๋ฏธ์ง์ ์ขํ์ ์๋์ ์ผ๋ก ๋์ผํ์ง ํ์ธํด์ผ ํฉ๋๋ค.
```py
>>> import torch
>>> with torch.no_grad():
... outputs = model(**inputs)
... target_sizes = torch.tensor([im.size[::-1]])
... results = processor.post_process_object_detection(outputs, threshold=0.1, target_sizes=target_sizes)[0]
>>> draw = ImageDraw.Draw(im)
>>> scores = results["scores"].tolist()
>>> labels = results["labels"].tolist()
>>> boxes = results["boxes"].tolist()
>>> for box, score, label in zip(boxes, scores, labels):
... xmin, ymin, xmax, ymax = box
... draw.rectangle((xmin, ymin, xmax, ymax), outline="red", width=1)
... draw.text((xmin, ymin), f"{text_queries[label]}: {round(score,2)}", fill="white")
>>> im
```
<div class="flex justify-center">
<img src="https://huggingface.co/datasets/huggingface/documentation-images/resolve/main/transformers/tasks/zero-sh-obj-detection_4.png" alt="Beach photo with detected objects"/>
</div>
## ์ผ๊ด ์ฒ๋ฆฌ[[batch-processing]]
์ฌ๋ฌ ์ด๋ฏธ์ง์ ํ
์คํธ ์ฟผ๋ฆฌ๋ฅผ ์ ๋ฌํ์ฌ ์ฌ๋ฌ ์ด๋ฏธ์ง์์ ์๋ก ๋ค๋ฅธ(๋๋ ๋์ผํ) ๊ฐ์ฒด๋ฅผ ๊ฒ์ํ ์ ์์ต๋๋ค.
์ผ๊ด ์ฒ๋ฆฌ๋ฅผ ์ํด์ ํ
์คํธ ์ฟผ๋ฆฌ๋ ์ด์ค ๋ฆฌ์คํธ๋ก, ์ด๋ฏธ์ง๋ PIL ์ด๋ฏธ์ง, PyTorch ํ
์, ๋๋ NumPy ๋ฐฐ์ด๋ก ์ด๋ฃจ์ด์ง ๋ฆฌ์คํธ๋ก ํ๋ก์ธ์์ ์ ๋ฌํด์ผ ํฉ๋๋ค.
```py
>>> images = [image, im]
>>> text_queries = [
... ["human face", "rocket", "nasa badge", "star-spangled banner"],
... ["hat", "book", "sunglasses", "camera"],
... ]
>>> inputs = processor(text=text_queries, images=images, return_tensors="pt")
```
์ด์ ์๋ ํ์ฒ๋ฆฌ๋ฅผ ์ํด ๋จ์ผ ์ด๋ฏธ์ง์ ํฌ๊ธฐ๋ฅผ ํ
์๋ก ์ ๋ฌํ์ง๋ง, ํํ์ ์ ๋ฌํ ์ ์๊ณ , ์ฌ๋ฌ ์ด๋ฏธ์ง๋ฅผ ์ฒ๋ฆฌํ๋ ๊ฒฝ์ฐ์๋ ํํ๋ก ์ด๋ฃจ์ด์ง ๋ฆฌ์คํธ๋ฅผ ์ ๋ฌํ ์๋ ์์ต๋๋ค.
์๋ ๋ ์์ ์ ๋ํ ์์ธก์ ์์ฑํ๊ณ , ๋ ๋ฒ์งธ ์ด๋ฏธ์ง(`image_idx = 1`)๋ฅผ ์๊ฐํํด ๋ณด๊ฒ ์ต๋๋ค.
```py
>>> with torch.no_grad():
... outputs = model(**inputs)
... target_sizes = [x.size[::-1] for x in images]
... results = processor.post_process_object_detection(outputs, threshold=0.1, target_sizes=target_sizes)
>>> image_idx = 1
>>> draw = ImageDraw.Draw(images[image_idx])
>>> scores = results[image_idx]["scores"].tolist()
>>> labels = results[image_idx]["labels"].tolist()
>>> boxes = results[image_idx]["boxes"].tolist()
>>> for box, score, label in zip(boxes, scores, labels):
... xmin, ymin, xmax, ymax = box
... draw.rectangle((xmin, ymin, xmax, ymax), outline="red", width=1)
... draw.text((xmin, ymin), f"{text_queries[image_idx][label]}: {round(score,2)}", fill="white")
>>> images[image_idx]
```
<div class="flex justify-center">
<img src="https://huggingface.co/datasets/huggingface/documentation-images/resolve/main/transformers/tasks/zero-sh-obj-detection_4.png" alt="Beach photo with detected objects"/>
</div>
## ์ด๋ฏธ์ง ๊ฐ์ด๋ ๊ฐ์ฒด ํ์ง[[imageguided-object-detection]]
ํ
์คํธ ์ฟผ๋ฆฌ๋ฅผ ์ด์ฉํ ์ ๋ก์ท ๊ฐ์ฒด ํ์ง ์ธ์๋ OWL-ViT ๋ชจ๋ธ์ ์ด๋ฏธ์ง ๊ฐ์ด๋ ๊ฐ์ฒด ํ์ง ๊ธฐ๋ฅ์ ์ ๊ณตํฉ๋๋ค.
์ด๋ฏธ์ง๋ฅผ ์ฟผ๋ฆฌ๋ก ์ฌ์ฉํด ๋์ ์ด๋ฏธ์ง์์ ์ ์ฌํ ๊ฐ์ฒด๋ฅผ ์ฐพ์ ์ ์๋ค๋ ์๋ฏธ์
๋๋ค.
ํ
์คํธ ์ฟผ๋ฆฌ์ ๋ฌ๋ฆฌ ํ๋์ ์์ ์ด๋ฏธ์ง์์๋ง ๊ฐ๋ฅํฉ๋๋ค.
์ํ์ ๊ณ ์์ด ๋ ๋ง๋ฆฌ๊ฐ ์๋ ์ด๋ฏธ์ง๋ฅผ ๋์ ์ด๋ฏธ์ง(target image)๋ก, ๊ณ ์์ด ํ ๋ง๋ฆฌ๊ฐ ์๋ ์ด๋ฏธ์ง๋ฅผ ์ฟผ๋ฆฌ๋ก ์ฌ์ฉํด๋ณด๊ฒ ์ต๋๋ค:
```py
>>> url = "http://images.cocodataset.org/val2017/000000039769.jpg"
>>> image_target = Image.open(requests.get(url, stream=True).raw)
>>> query_url = "http://images.cocodataset.org/val2017/000000524280.jpg"
>>> query_image = Image.open(requests.get(query_url, stream=True).raw)
```
๋ค์ ์ด๋ฏธ์ง๋ฅผ ์ดํด๋ณด๊ฒ ์ต๋๋ค:
```py
>>> import matplotlib.pyplot as plt
>>> fig, ax = plt.subplots(1, 2)
>>> ax[0].imshow(image_target)
>>> ax[1].imshow(query_image)
```
<div class="flex justify-center">
<img src="https://huggingface.co/datasets/huggingface/documentation-images/resolve/main/transformers/tasks/zero-sh-obj-detection_5.png" alt="Cats"/>
</div>
์ ์ฒ๋ฆฌ ๋จ๊ณ์์ ํ
์คํธ ์ฟผ๋ฆฌ ๋์ ์ `query_images`๋ฅผ ์ฌ์ฉํฉ๋๋ค:
```py
>>> inputs = processor(images=image_target, query_images=query_image, return_tensors="pt")
```
์์ธก์ ๊ฒฝ์ฐ, ๋ชจ๋ธ์ ์
๋ ฅ์ ์ ๋ฌํ๋ ๋์ [`~OwlViTForObjectDetection.image_guided_detection`]์ ์ ๋ฌํฉ๋๋ค.
๋ ์ด๋ธ์ด ์๋ค๋ ์ ์ ์ ์ธํ๋ฉด ์ด์ ๊ณผ ๋์ผํฉ๋๋ค.
์ด์ ๊ณผ ๋์ผํ๊ฒ ์ด๋ฏธ์ง๋ฅผ ์๊ฐํํฉ๋๋ค.
```py
>>> with torch.no_grad():
... outputs = model.image_guided_detection(**inputs)
... target_sizes = torch.tensor([image_target.size[::-1]])
... results = processor.post_process_image_guided_detection(outputs=outputs, target_sizes=target_sizes)[0]
>>> draw = ImageDraw.Draw(image_target)
>>> scores = results["scores"].tolist()
>>> boxes = results["boxes"].tolist()
>>> for box, score, label in zip(boxes, scores, labels):
... xmin, ymin, xmax, ymax = box
... draw.rectangle((xmin, ymin, xmax, ymax), outline="white", width=4)
>>> image_target
```
<div class="flex justify-center">
<img src="https://huggingface.co/datasets/huggingface/documentation-images/resolve/main/transformers/tasks/zero-sh-obj-detection_6.png" alt="Cats with bounding boxes"/>
</div>
OWL-ViT ๋ชจ๋ธ์ ์ถ๋ก ํ๊ณ ์ถ๋ค๋ฉด ์๋ ๋ฐ๋ชจ๋ฅผ ํ์ธํ์ธ์:
<iframe
src="https://adirik-owl-vit.hf.space"
frameborder="0"
width="850"
height="450"
></iframe>
| 0 |
hf_public_repos/transformers/docs/source/ko | hf_public_repos/transformers/docs/source/ko/tasks/token_classification.md | <!--Copyright 2022 The HuggingFace Team. All rights reserved.
Licensed under the Apache License, Version 2.0 (the "License"); you may not use this file except in compliance with
the License. You may obtain a copy of the License at
http://www.apache.org/licenses/LICENSE-2.0
Unless required by applicable law or agreed to in writing, software distributed under the License is distributed on
an "AS IS" BASIS, WITHOUT WARRANTIES OR CONDITIONS OF ANY KIND, either express or implied. See the License for the
specific language governing permissions and limitations under the License.
โ ๏ธ Note that this file is in Markdown but contain specific syntax for our doc-builder (similar to MDX) that may not be
rendered properly in your Markdown viewer.
-->
# ํ ํฐ ๋ถ๋ฅ[[token-classification]]
[[open-in-colab]]
<Youtube id="wVHdVlPScxA"/>
ํ ํฐ ๋ถ๋ฅ๋ ๋ฌธ์ฅ์ ๊ฐ๋ณ ํ ํฐ์ ๋ ์ด๋ธ์ ํ ๋นํฉ๋๋ค. ๊ฐ์ฅ ์ผ๋ฐ์ ์ธ ํ ํฐ ๋ถ๋ฅ ์์
์ค ํ๋๋ ๊ฐ์ฒด๋ช
์ธ์(Named Entity Recognition, NER)์
๋๋ค. ๊ฐ์ฒด๋ช
์ธ์์ ๋ฌธ์ฅ์์ ์ฌ๋, ์์น ๋๋ ์กฐ์ง๊ณผ ๊ฐ์ ๊ฐ ๊ฐ์ฒด์ ๋ ์ด๋ธ์ ์ฐพ์ผ๋ ค๊ณ ์๋ํฉ๋๋ค.
์ด ๊ฐ์ด๋์์ ํ์ตํ ๋ด์ฉ์:
1. [WNUT 17](https://huggingface.co/datasets/wnut_17) ๋ฐ์ดํฐ ์ธํธ์์ [DistilBERT](https://huggingface.co/distilbert-base-uncased)๋ฅผ ํ์ธ ํ๋ํ์ฌ ์๋ก์ด ๊ฐ์ฒด๋ฅผ ํ์งํฉ๋๋ค.
2. ์ถ๋ก ์ ์ํด ํ์ธ ํ๋ ๋ชจ๋ธ์ ์ฌ์ฉํฉ๋๋ค.
<Tip>
์ด ํํ ๋ฆฌ์ผ์์ ์ค๋ช
ํ๋ ์์
์ ๋ค์ ๋ชจ๋ธ ์ํคํ
์ฒ์ ์ํด ์ง์๋ฉ๋๋ค:
<!--This tip is automatically generated by `make fix-copies`, do not fill manually!-->
[ALBERT](../model_doc/albert), [BERT](../model_doc/bert), [BigBird](../model_doc/big_bird), [BioGpt](../model_doc/biogpt), [BLOOM](../model_doc/bloom), [CamemBERT](../model_doc/camembert), [CANINE](../model_doc/canine), [ConvBERT](../model_doc/convbert), [Data2VecText](../model_doc/data2vec-text), [DeBERTa](../model_doc/deberta), [DeBERTa-v2](../model_doc/deberta-v2), [DistilBERT](../model_doc/distilbert), [ELECTRA](../model_doc/electra), [ERNIE](../model_doc/ernie), [ErnieM](../model_doc/ernie_m), [ESM](../model_doc/esm), [FlauBERT](../model_doc/flaubert), [FNet](../model_doc/fnet), [Funnel Transformer](../model_doc/funnel), [GPT-Sw3](../model_doc/gpt-sw3), [OpenAI GPT-2](../model_doc/gpt2), [GPTBigCode](../model_doc/gpt_bigcode), [I-BERT](../model_doc/ibert), [LayoutLM](../model_doc/layoutlm), [LayoutLMv2](../model_doc/layoutlmv2), [LayoutLMv3](../model_doc/layoutlmv3), [LiLT](../model_doc/lilt), [Longformer](../model_doc/longformer), [LUKE](../model_doc/luke), [MarkupLM](../model_doc/markuplm), [MEGA](../model_doc/mega), [Megatron-BERT](../model_doc/megatron-bert), [MobileBERT](../model_doc/mobilebert), [MPNet](../model_doc/mpnet), [Nezha](../model_doc/nezha), [Nystrรถmformer](../model_doc/nystromformer), [QDQBert](../model_doc/qdqbert), [RemBERT](../model_doc/rembert), [RoBERTa](../model_doc/roberta), [RoBERTa-PreLayerNorm](../model_doc/roberta-prelayernorm), [RoCBert](../model_doc/roc_bert), [RoFormer](../model_doc/roformer), [SqueezeBERT](../model_doc/squeezebert), [XLM](../model_doc/xlm), [XLM-RoBERTa](../model_doc/xlm-roberta), [XLM-RoBERTa-XL](../model_doc/xlm-roberta-xl), [XLNet](../model_doc/xlnet), [X-MOD](../model_doc/xmod), [YOSO](../model_doc/yoso)
<!--End of the generated tip-->
</Tip>
์์ํ๊ธฐ ์ ์, ํ์ํ ๋ชจ๋ ๋ผ์ด๋ธ๋ฌ๋ฆฌ๊ฐ ์ค์น๋์ด ์๋์ง ํ์ธํ์ธ์:
```bash
pip install transformers datasets evaluate seqeval
```
Hugging Face ๊ณ์ ์ ๋ก๊ทธ์ธํ์ฌ ๋ชจ๋ธ์ ์
๋ก๋ํ๊ณ ์ปค๋ฎค๋ํฐ์ ๊ณต์ ํ๋ ๊ฒ์ ๊ถ์ฅํฉ๋๋ค. ๋ฉ์์ง๊ฐ ํ์๋๋ฉด, ํ ํฐ์ ์
๋ ฅํ์ฌ ๋ก๊ทธ์ธํ์ธ์:
```py
>>> from huggingface_hub import notebook_login
>>> notebook_login()
```
## WNUT 17 ๋ฐ์ดํฐ ์ธํธ ๊ฐ์ ธ์ค๊ธฐ[[load-wnut-17-dataset]]
๋จผ์ ๐ค Datasets ๋ผ์ด๋ธ๋ฌ๋ฆฌ์์ WNUT 17 ๋ฐ์ดํฐ ์ธํธ๋ฅผ ๊ฐ์ ธ์ต๋๋ค:
```py
>>> from datasets import load_dataset
>>> wnut = load_dataset("wnut_17")
```
๋ค์ ์์ ๋ฅผ ์ดํด๋ณด์ธ์:
```py
>>> wnut["train"][0]
{'id': '0',
'ner_tags': [0, 0, 0, 0, 0, 0, 0, 0, 0, 0, 0, 0, 0, 0, 7, 8, 8, 0, 7, 0, 0, 0, 0, 0, 0, 0, 0],
'tokens': ['@paulwalk', 'It', "'s", 'the', 'view', 'from', 'where', 'I', "'m", 'living', 'for', 'two', 'weeks', '.', 'Empire', 'State', 'Building', '=', 'ESB', '.', 'Pretty', 'bad', 'storm', 'here', 'last', 'evening', '.']
}
```
`ner_tags`์ ๊ฐ ์ซ์๋ ๊ฐ์ฒด๋ฅผ ๋ํ๋
๋๋ค. ์ซ์๋ฅผ ๋ ์ด๋ธ ์ด๋ฆ์ผ๋ก ๋ณํํ์ฌ ๊ฐ์ฒด๊ฐ ๋ฌด์์ธ์ง ํ์ธํฉ๋๋ค:
```py
>>> label_list = wnut["train"].features[f"ner_tags"].feature.names
>>> label_list
[
"O",
"B-corporation",
"I-corporation",
"B-creative-work",
"I-creative-work",
"B-group",
"I-group",
"B-location",
"I-location",
"B-person",
"I-person",
"B-product",
"I-product",
]
```
๊ฐ `ner_tag`์ ์์ ๋ถ์ ๋ฌธ์๋ ๊ฐ์ฒด์ ํ ํฐ ์์น๋ฅผ ๋ํ๋
๋๋ค:
- `B-`๋ ๊ฐ์ฒด์ ์์์ ๋ํ๋
๋๋ค.
- `I-`๋ ํ ํฐ์ด ๋์ผํ ๊ฐ์ฒด ๋ด๋ถ์ ํฌํจ๋์ด ์์์ ๋ํ๋
๋๋ค(์๋ฅผ ๋ค์ด `State` ํ ํฐ์ `Empire State Building`์ ๊ฐ์ ๊ฐ์ฒด์ ์ผ๋ถ์
๋๋ค).
- `0`๋ ํ ํฐ์ด ์ด๋ค ๊ฐ์ฒด์๋ ํด๋นํ์ง ์์์ ๋ํ๋
๋๋ค.
## ์ ์ฒ๋ฆฌ[[preprocess]]
<Youtube id="iY2AZYdZAr0"/>
๋ค์์ผ๋ก `tokens` ํ๋๋ฅผ ์ ์ฒ๋ฆฌํ๊ธฐ ์ํด DistilBERT ํ ํฌ๋์ด์ ๋ฅผ ๊ฐ์ ธ์ต๋๋ค:
```py
>>> from transformers import AutoTokenizer
>>> tokenizer = AutoTokenizer.from_pretrained("distilbert-base-uncased")
```
์์ ์์ `tokens` ํ๋๋ฅผ ๋ณด๋ฉด ์
๋ ฅ์ด ์ด๋ฏธ ํ ํฐํ๋ ๊ฒ์ฒ๋ผ ๋ณด์
๋๋ค. ๊ทธ๋ฌ๋ ์ค์ ๋ก ์
๋ ฅ์ ์์ง ํ ํฐํ๋์ง ์์์ผ๋ฏ๋ก ๋จ์ด๋ฅผ ํ์ ๋จ์ด๋ก ํ ํฐํํ๊ธฐ ์ํด `is_split_into_words=True`๋ฅผ ์ค์ ํด์ผ ํฉ๋๋ค. ์์ ๋ก ํ์ธํฉ๋๋ค:
```py
>>> example = wnut["train"][0]
>>> tokenized_input = tokenizer(example["tokens"], is_split_into_words=True)
>>> tokens = tokenizer.convert_ids_to_tokens(tokenized_input["input_ids"])
>>> tokens
['[CLS]', '@', 'paul', '##walk', 'it', "'", 's', 'the', 'view', 'from', 'where', 'i', "'", 'm', 'living', 'for', 'two', 'weeks', '.', 'empire', 'state', 'building', '=', 'es', '##b', '.', 'pretty', 'bad', 'storm', 'here', 'last', 'evening', '.', '[SEP]']
```
๊ทธ๋ฌ๋ ์ด๋ก ์ธํด `[CLS]`๊ณผ `[SEP]`๋ผ๋ ํน์ ํ ํฐ์ด ์ถ๊ฐ๋๊ณ , ํ์ ๋จ์ด ํ ํฐํ๋ก ์ธํด ์
๋ ฅ๊ณผ ๋ ์ด๋ธ ๊ฐ์ ๋ถ์ผ์น๊ฐ ๋ฐ์ํฉ๋๋ค. ํ๋์ ๋ ์ด๋ธ์ ํด๋นํ๋ ๋จ์ผ ๋จ์ด๋ ์ด์ ๋ ๊ฐ์ ํ์ ๋จ์ด๋ก ๋ถํ ๋ ์ ์์ต๋๋ค. ํ ํฐ๊ณผ ๋ ์ด๋ธ์ ๋ค์๊ณผ ๊ฐ์ด ์ฌ์ ๋ ฌํด์ผ ํฉ๋๋ค:
1. [`word_ids`](https://huggingface.co/docs/transformers/main_classes/tokenizer#transformers.BatchEncoding.word_ids) ๋ฉ์๋๋ก ๋ชจ๋ ํ ํฐ์ ํด๋น ๋จ์ด์ ๋งคํํฉ๋๋ค.
2. ํน์ ํ ํฐ `[CLS]`์ `[SEP]`์ `-100` ๋ ์ด๋ธ์ ํ ๋นํ์ฌ, PyTorch ์์ค ํจ์๊ฐ ํด๋น ํ ํฐ์ ๋ฌด์ํ๋๋ก ํฉ๋๋ค.
3. ์ฃผ์ด์ง ๋จ์ด์ ์ฒซ ๋ฒ์งธ ํ ํฐ์๋ง ๋ ์ด๋ธ์ ์ง์ ํฉ๋๋ค. ๊ฐ์ ๋จ์ด์ ๋ค๋ฅธ ํ์ ํ ํฐ์ `-100`์ ํ ๋นํฉ๋๋ค.
๋ค์์ ํ ํฐ๊ณผ ๋ ์ด๋ธ์ ์ฌ์ ๋ ฌํ๊ณ DistilBERT์ ์ต๋ ์
๋ ฅ ๊ธธ์ด๋ณด๋ค ๊ธธ์ง ์๋๋ก ์ํ์ค๋ฅผ ์๋ผ๋ด๋ ํจ์๋ฅผ ๋ง๋๋ ๋ฐฉ๋ฒ์
๋๋ค:
```py
>>> def tokenize_and_align_labels(examples):
... tokenized_inputs = tokenizer(examples["tokens"], truncation=True, is_split_into_words=True)
... labels = []
... for i, label in enumerate(examples[f"ner_tags"]):
... word_ids = tokenized_inputs.word_ids(batch_index=i) # Map tokens to their respective word.
... previous_word_idx = None
... label_ids = []
... for word_idx in word_ids: # Set the special tokens to -100.
... if word_idx is None:
... label_ids.append(-100)
... elif word_idx != previous_word_idx: # Only label the first token of a given word.
... label_ids.append(label[word_idx])
... else:
... label_ids.append(-100)
... previous_word_idx = word_idx
... labels.append(label_ids)
... tokenized_inputs["labels"] = labels
... return tokenized_inputs
```
์ ์ฒด ๋ฐ์ดํฐ ์ธํธ์ ์ ์ฒ๋ฆฌ ํจ์๋ฅผ ์ ์ฉํ๋ ค๋ฉด, ๐ค Datasets [`~datasets.Dataset.map`] ํจ์๋ฅผ ์ฌ์ฉํ์ธ์. `batched=True`๋ก ์ค์ ํ์ฌ ๋ฐ์ดํฐ ์ธํธ์ ์ฌ๋ฌ ์์๋ฅผ ํ ๋ฒ์ ์ฒ๋ฆฌํ๋ฉด `map` ํจ์์ ์๋๋ฅผ ๋์ผ ์ ์์ต๋๋ค:
```py
>>> tokenized_wnut = wnut.map(tokenize_and_align_labels, batched=True)
```
์ด์ [`DataCollatorWithPadding`]๋ฅผ ์ฌ์ฉํ์ฌ ์์ ๋ฐฐ์น๋ฅผ ๋ง๋ค์ด๋ด
์๋ค. ๋ฐ์ดํฐ ์ธํธ ์ ์ฒด๋ฅผ ์ต๋ ๊ธธ์ด๋ก ํจ๋ฉํ๋ ๋์ , *๋์ ํจ๋ฉ*์ ์ฌ์ฉํ์ฌ ๋ฐฐ์น์์ ๊ฐ์ฅ ๊ธด ๊ธธ์ด์ ๋ง๊ฒ ๋ฌธ์ฅ์ ํจ๋ฉํ๋ ๊ฒ์ด ํจ์จ์ ์
๋๋ค.
<frameworkcontent>
<pt>
```py
>>> from transformers import DataCollatorForTokenClassification
>>> data_collator = DataCollatorForTokenClassification(tokenizer=tokenizer)
```
</pt>
<tf>
```py
>>> from transformers import DataCollatorForTokenClassification
>>> data_collator = DataCollatorForTokenClassification(tokenizer=tokenizer, return_tensors="tf")
```
</tf>
</frameworkcontent>
## ํ๊ฐ[[evaluation]]
ํ๋ จ ์ค ๋ชจ๋ธ์ ์ฑ๋ฅ์ ํ๊ฐํ๊ธฐ ์ํด ํ๊ฐ ์งํ๋ฅผ ํฌํจํ๋ ๊ฒ์ด ์ ์ฉํฉ๋๋ค. ๐ค [Evaluate](https://huggingface.co/docs/evaluate/index) ๋ผ์ด๋ธ๋ฌ๋ฆฌ๋ฅผ ์ฌ์ฉํ์ฌ ๋น ๋ฅด๊ฒ ํ๊ฐ ๋ฐฉ๋ฒ์ ๊ฐ์ ธ์ฌ ์ ์์ต๋๋ค. ์ด ์์
์์๋ [seqeval](https://huggingface.co/spaces/evaluate-metric/seqeval) ํ๊ฐ ์งํ๋ฅผ ๊ฐ์ ธ์ต๋๋ค. (ํ๊ฐ ์งํ๋ฅผ ๊ฐ์ ธ์ค๊ณ ๊ณ์ฐํ๋ ๋ฐฉ๋ฒ์ ๋ํด์๋ ๐ค Evaluate [๋น ๋ฅธ ๋๋ฌ๋ณด๊ธฐ](https://huggingface.co/docs/evaluate/a_quick_tour)๋ฅผ ์ฐธ์กฐํ์ธ์). Seqeval์ ์ค์ ๋ก ์ ๋ฐ๋, ์ฌํ๋ฅ , F1 ๋ฐ ์ ํ๋์ ๊ฐ์ ์ฌ๋ฌ ์ ์๋ฅผ ์ฐ์ถํฉ๋๋ค.
```py
>>> import evaluate
>>> seqeval = evaluate.load("seqeval")
```
๋จผ์ NER ๋ ์ด๋ธ์ ๊ฐ์ ธ์จ ๋ค์, [`~evaluate.EvaluationModule.compute`]์ ์ค์ ์์ธก๊ณผ ์ค์ ๋ ์ด๋ธ์ ์ ๋ฌํ์ฌ ์ ์๋ฅผ ๊ณ์ฐํ๋ ํจ์๋ฅผ ๋ง๋ญ๋๋ค:
```py
>>> import numpy as np
>>> labels = [label_list[i] for i in example[f"ner_tags"]]
>>> def compute_metrics(p):
... predictions, labels = p
... predictions = np.argmax(predictions, axis=2)
... true_predictions = [
... [label_list[p] for (p, l) in zip(prediction, label) if l != -100]
... for prediction, label in zip(predictions, labels)
... ]
... true_labels = [
... [label_list[l] for (p, l) in zip(prediction, label) if l != -100]
... for prediction, label in zip(predictions, labels)
... ]
... results = seqeval.compute(predictions=true_predictions, references=true_labels)
... return {
... "precision": results["overall_precision"],
... "recall": results["overall_recall"],
... "f1": results["overall_f1"],
... "accuracy": results["overall_accuracy"],
... }
```
์ด์ `compute_metrics` ํจ์๋ฅผ ์ฌ์ฉํ ์ค๋น๊ฐ ๋์์ผ๋ฉฐ, ํ๋ จ์ ์ค์ ํ๋ฉด ์ด ํจ์๋ก ๋๋์์ฌ ๊ฒ์
๋๋ค.
## ํ๋ จ[[train]]
๋ชจ๋ธ์ ํ๋ จํ๊ธฐ ์ ์, `id2label`์ `label2id`๋ฅผ ์ฌ์ฉํ์ฌ ์์๋๋ id์ ๋ ์ด๋ธ์ ๋งต์ ์์ฑํ์ธ์:
```py
>>> id2label = {
... 0: "O",
... 1: "B-corporation",
... 2: "I-corporation",
... 3: "B-creative-work",
... 4: "I-creative-work",
... 5: "B-group",
... 6: "I-group",
... 7: "B-location",
... 8: "I-location",
... 9: "B-person",
... 10: "I-person",
... 11: "B-product",
... 12: "I-product",
... }
>>> label2id = {
... "O": 0,
... "B-corporation": 1,
... "I-corporation": 2,
... "B-creative-work": 3,
... "I-creative-work": 4,
... "B-group": 5,
... "I-group": 6,
... "B-location": 7,
... "I-location": 8,
... "B-person": 9,
... "I-person": 10,
... "B-product": 11,
... "I-product": 12,
... }
```
<frameworkcontent>
<pt>
<Tip>
[`Trainer`]๋ฅผ ์ฌ์ฉํ์ฌ ๋ชจ๋ธ์ ํ์ธ ํ๋ํ๋ ๋ฐฉ๋ฒ์ ์ต์ํ์ง ์์ ๊ฒฝ์ฐ, [์ฌ๊ธฐ](../training#train-with-pytorch-trainer)์์ ๊ธฐ๋ณธ ํํ ๋ฆฌ์ผ์ ํ์ธํ์ธ์!
</Tip>
์ด์ ๋ชจ๋ธ์ ํ๋ จ์ํฌ ์ค๋น๊ฐ ๋์์ต๋๋ค! [`AutoModelForSequenceClassification`]๋ก DistilBERT๋ฅผ ๊ฐ์ ธ์ค๊ณ ์์๋๋ ๋ ์ด๋ธ ์์ ๋ ์ด๋ธ ๋งคํ์ ์ง์ ํ์ธ์:
```py
>>> from transformers import AutoModelForTokenClassification, TrainingArguments, Trainer
>>> model = AutoModelForTokenClassification.from_pretrained(
... "distilbert-base-uncased", num_labels=13, id2label=id2label, label2id=label2id
... )
```
์ด์ ์ธ ๋จ๊ณ๋ง ๊ฑฐ์น๋ฉด ๋์
๋๋ค:
1. [`TrainingArguments`]์์ ํ์ดํผํ๋ผ๋ฏธํฐ๋ฅผ ์ ์ํ์ธ์. `output_dir`๋ ๋ชจ๋ธ์ ์ ์ฅํ ์์น๋ฅผ ์ง์ ํ๋ ์ ์ผํ ๋งค๊ฐ๋ณ์์
๋๋ค. ์ด ๋ชจ๋ธ์ ํ๋ธ์ ์
๋ก๋ํ๊ธฐ ์ํด `push_to_hub=True`๋ฅผ ์ค์ ํฉ๋๋ค(๋ชจ๋ธ์ ์
๋ก๋ํ๊ธฐ ์ํด Hugging Face์ ๋ก๊ทธ์ธํด์ผํฉ๋๋ค.) ๊ฐ ์ํญ์ด ๋๋ ๋๋ง๋ค, [`Trainer`]๋ seqeval ์ ์๋ฅผ ํ๊ฐํ๊ณ ํ๋ จ ์ฒดํฌํฌ์ธํธ๋ฅผ ์ ์ฅํฉ๋๋ค.
2. [`Trainer`]์ ํ๋ จ ์ธ์์ ๋ชจ๋ธ, ๋ฐ์ดํฐ ์ธํธ, ํ ํฌ๋์ด์ , ๋ฐ์ดํฐ ์ฝ๋ ์ดํฐ ๋ฐ `compute_metrics` ํจ์๋ฅผ ์ ๋ฌํ์ธ์.
3. [`~Trainer.train`]๋ฅผ ํธ์ถํ์ฌ ๋ชจ๋ธ์ ํ์ธ ํ๋ํ์ธ์.
```py
>>> training_args = TrainingArguments(
... output_dir="my_awesome_wnut_model",
... learning_rate=2e-5,
... per_device_train_batch_size=16,
... per_device_eval_batch_size=16,
... num_train_epochs=2,
... weight_decay=0.01,
... evaluation_strategy="epoch",
... save_strategy="epoch",
... load_best_model_at_end=True,
... push_to_hub=True,
... )
>>> trainer = Trainer(
... model=model,
... args=training_args,
... train_dataset=tokenized_wnut["train"],
... eval_dataset=tokenized_wnut["test"],
... tokenizer=tokenizer,
... data_collator=data_collator,
... compute_metrics=compute_metrics,
... )
>>> trainer.train()
```
ํ๋ จ์ด ์๋ฃ๋๋ฉด, [`~transformers.Trainer.push_to_hub`] ๋ฉ์๋๋ฅผ ์ฌ์ฉํ์ฌ ๋ชจ๋ธ์ ํ๋ธ์ ๊ณต์ ํ ์ ์์ต๋๋ค.
```py
>>> trainer.push_to_hub()
```
</pt>
<tf>
<Tip>
Keras๋ฅผ ์ฌ์ฉํ์ฌ ๋ชจ๋ธ์ ํ์ธ ํ๋ํ๋ ๋ฐฉ๋ฒ์ ์ต์ํ์ง ์์ ๊ฒฝ์ฐ, [์ฌ๊ธฐ](../training#train-a-tensorflow-model-with-keras)์ ๊ธฐ๋ณธ ํํ ๋ฆฌ์ผ์ ํ์ธํ์ธ์!
</Tip>
TensorFlow์์ ๋ชจ๋ธ์ ํ์ธ ํ๋ํ๋ ค๋ฉด, ๋จผ์ ์ตํฐ๋ง์ด์ ํจ์์ ํ์ต๋ฅ ์ค์ผ์ฅด, ๊ทธ๋ฆฌ๊ณ ์ผ๋ถ ํ๋ จ ํ์ดํผํ๋ผ๋ฏธํฐ๋ฅผ ์ค์ ํด์ผ ํฉ๋๋ค:
```py
>>> from transformers import create_optimizer
>>> batch_size = 16
>>> num_train_epochs = 3
>>> num_train_steps = (len(tokenized_wnut["train"]) // batch_size) * num_train_epochs
>>> optimizer, lr_schedule = create_optimizer(
... init_lr=2e-5,
... num_train_steps=num_train_steps,
... weight_decay_rate=0.01,
... num_warmup_steps=0,
... )
```
๊ทธ๋ฐ ๋ค์ [`TFAutoModelForSequenceClassification`]์ ์ฌ์ฉํ์ฌ DistilBERT๋ฅผ ๊ฐ์ ธ์ค๊ณ , ์์๋๋ ๋ ์ด๋ธ ์์ ๋ ์ด๋ธ ๋งคํ์ ์ง์ ํฉ๋๋ค:
```py
>>> from transformers import TFAutoModelForTokenClassification
>>> model = TFAutoModelForTokenClassification.from_pretrained(
... "distilbert-base-uncased", num_labels=13, id2label=id2label, label2id=label2id
... )
```
[`~transformers.TFPreTrainedModel.prepare_tf_dataset`]์ ์ฌ์ฉํ์ฌ ๋ฐ์ดํฐ ์ธํธ๋ฅผ `tf.data.Dataset` ํ์์ผ๋ก ๋ณํํฉ๋๋ค:
```py
>>> tf_train_set = model.prepare_tf_dataset(
... tokenized_wnut["train"],
... shuffle=True,
... batch_size=16,
... collate_fn=data_collator,
... )
>>> tf_validation_set = model.prepare_tf_dataset(
... tokenized_wnut["validation"],
... shuffle=False,
... batch_size=16,
... collate_fn=data_collator,
... )
```
[`compile`](https://keras.io/api/models/model_training_apis/#compile-method)๋ฅผ ์ฌ์ฉํ์ฌ ํ๋ จํ ๋ชจ๋ธ์ ๊ตฌ์ฑํฉ๋๋ค:
```py
>>> import tensorflow as tf
>>> model.compile(optimizer=optimizer)
```
ํ๋ จ์ ์์ํ๊ธฐ ์ ์ ์ค์ ํด์ผํ ๋ง์ง๋ง ๋ ๊ฐ์ง๋ ์์ธก์์ seqeval ์ ์๋ฅผ ๊ณ์ฐํ๊ณ , ๋ชจ๋ธ์ ํ๋ธ์ ์
๋ก๋ํ ๋ฐฉ๋ฒ์ ์ ๊ณตํ๋ ๊ฒ์
๋๋ค. ๋ชจ๋ [Keras callbacks](../main_classes/keras_callbacks)๋ฅผ ์ฌ์ฉํ์ฌ ์ํ๋ฉ๋๋ค.
[`~transformers.KerasMetricCallback`]์ `compute_metrics` ํจ์๋ฅผ ์ ๋ฌํ์ธ์:
```py
>>> from transformers.keras_callbacks import KerasMetricCallback
>>> metric_callback = KerasMetricCallback(metric_fn=compute_metrics, eval_dataset=tf_validation_set)
```
[`~transformers.PushToHubCallback`]์์ ๋ชจ๋ธ๊ณผ ํ ํฌ๋์ด์ ๋ฅผ ์
๋ก๋ํ ์์น๋ฅผ ์ง์ ํฉ๋๋ค:
```py
>>> from transformers.keras_callbacks import PushToHubCallback
>>> push_to_hub_callback = PushToHubCallback(
... output_dir="my_awesome_wnut_model",
... tokenizer=tokenizer,
... )
```
๊ทธ๋ฐ ๋ค์ ์ฝ๋ฐฑ์ ํจ๊ป ๋ฌถ์ต๋๋ค:
```py
>>> callbacks = [metric_callback, push_to_hub_callback]
```
๋๋์ด, ๋ชจ๋ธ ํ๋ จ์ ์์ํ ์ค๋น๊ฐ ๋์์ต๋๋ค! [`fit`](https://keras.io/api/models/model_training_apis/#fit-method)์ ํ๋ จ ๋ฐ์ดํฐ ์ธํธ, ๊ฒ์ฆ ๋ฐ์ดํฐ ์ธํธ, ์ํญ์ ์ ๋ฐ ์ฝ๋ฐฑ์ ์ ๋ฌํ์ฌ ํ์ธ ํ๋ํฉ๋๋ค:
```py
>>> model.fit(x=tf_train_set, validation_data=tf_validation_set, epochs=3, callbacks=callbacks)
```
ํ๋ จ์ด ์๋ฃ๋๋ฉด, ๋ชจ๋ธ์ด ์๋์ผ๋ก ํ๋ธ์ ์
๋ก๋๋์ด ๋๊ตฌ๋ ์ฌ์ฉํ ์ ์์ต๋๋ค!
</tf>
</frameworkcontent>
<Tip>
ํ ํฐ ๋ถ๋ฅ๋ฅผ ์ํ ๋ชจ๋ธ์ ํ์ธ ํ๋ํ๋ ์์ธํ ์์ ๋ ๋ค์
[PyTorch notebook](https://colab.research.google.com/github/huggingface/notebooks/blob/main/examples/token_classification.ipynb)
๋๋ [TensorFlow notebook](https://colab.research.google.com/github/huggingface/notebooks/blob/main/examples/token_classification-tf.ipynb)๋ฅผ ์ฐธ์กฐํ์ธ์.
</Tip>
## ์ถ๋ก [[inference]]
์ข์์, ์ด์ ๋ชจ๋ธ์ ํ์ธ ํ๋ํ์ผ๋ ์ถ๋ก ์ ์ฌ์ฉํ ์ ์์ต๋๋ค!
์ถ๋ก ์ ์ํํ๊ณ ์ ํ๋ ํ
์คํธ๋ฅผ ๊ฐ์ ธ์๋ด
์๋ค:
```py
>>> text = "The Golden State Warriors are an American professional basketball team based in San Francisco."
```
ํ์ธ ํ๋๋ ๋ชจ๋ธ๋ก ์ถ๋ก ์ ์๋ํ๋ ๊ฐ์ฅ ๊ฐ๋จํ ๋ฐฉ๋ฒ์ [`pipeline`]๋ฅผ ์ฌ์ฉํ๋ ๊ฒ์
๋๋ค. ๋ชจ๋ธ๋ก NER์ `pipeline`์ ์ธ์คํด์คํํ๊ณ , ํ
์คํธ๋ฅผ ์ ๋ฌํด๋ณด์ธ์:
```py
>>> from transformers import pipeline
>>> classifier = pipeline("ner", model="stevhliu/my_awesome_wnut_model")
>>> classifier(text)
[{'entity': 'B-location',
'score': 0.42658573,
'index': 2,
'word': 'golden',
'start': 4,
'end': 10},
{'entity': 'I-location',
'score': 0.35856336,
'index': 3,
'word': 'state',
'start': 11,
'end': 16},
{'entity': 'B-group',
'score': 0.3064001,
'index': 4,
'word': 'warriors',
'start': 17,
'end': 25},
{'entity': 'B-location',
'score': 0.65523505,
'index': 13,
'word': 'san',
'start': 80,
'end': 83},
{'entity': 'B-location',
'score': 0.4668663,
'index': 14,
'word': 'francisco',
'start': 84,
'end': 93}]
```
์ํ๋ค๋ฉด, `pipeline`์ ๊ฒฐ๊ณผ๋ฅผ ์๋์ผ๋ก ๋ณต์ ํ ์๋ ์์ต๋๋ค:
<frameworkcontent>
<pt>
ํ
์คํธ๋ฅผ ํ ํฐํํ๊ณ PyTorch ํ
์๋ฅผ ๋ฐํํฉ๋๋ค:
```py
>>> from transformers import AutoTokenizer
>>> tokenizer = AutoTokenizer.from_pretrained("stevhliu/my_awesome_wnut_model")
>>> inputs = tokenizer(text, return_tensors="pt")
```
์
๋ ฅ์ ๋ชจ๋ธ์ ์ ๋ฌํ๊ณ `logits`์ ๋ฐํํฉ๋๋ค:
```py
>>> from transformers import AutoModelForTokenClassification
>>> model = AutoModelForTokenClassification.from_pretrained("stevhliu/my_awesome_wnut_model")
>>> with torch.no_grad():
... logits = model(**inputs).logits
```
๊ฐ์ฅ ๋์ ํ๋ฅ ์ ๊ฐ์ง ํด๋์ค๋ฅผ ๋ชจ๋ธ์ `id2label` ๋งคํ์ ์ฌ์ฉํ์ฌ ํ
์คํธ ๋ ์ด๋ธ๋ก ๋ณํํฉ๋๋ค:
```py
>>> predictions = torch.argmax(logits, dim=2)
>>> predicted_token_class = [model.config.id2label[t.item()] for t in predictions[0]]
>>> predicted_token_class
['O',
'O',
'B-location',
'I-location',
'B-group',
'O',
'O',
'O',
'O',
'O',
'O',
'O',
'O',
'B-location',
'B-location',
'O',
'O']
```
</pt>
<tf>
ํ
์คํธ๋ฅผ ํ ํฐํํ๊ณ TensorFlow ํ
์๋ฅผ ๋ฐํํฉ๋๋ค:
```py
>>> from transformers import AutoTokenizer
>>> tokenizer = AutoTokenizer.from_pretrained("stevhliu/my_awesome_wnut_model")
>>> inputs = tokenizer(text, return_tensors="tf")
```
์
๋ ฅ๊ฐ์ ๋ชจ๋ธ์ ์ ๋ฌํ๊ณ `logits`์ ๋ฐํํฉ๋๋ค:
```py
>>> from transformers import TFAutoModelForTokenClassification
>>> model = TFAutoModelForTokenClassification.from_pretrained("stevhliu/my_awesome_wnut_model")
>>> logits = model(**inputs).logits
```
๊ฐ์ฅ ๋์ ํ๋ฅ ์ ๊ฐ์ง ํด๋์ค๋ฅผ ๋ชจ๋ธ์ `id2label` ๋งคํ์ ์ฌ์ฉํ์ฌ ํ
์คํธ ๋ ์ด๋ธ๋ก ๋ณํํฉ๋๋ค:
```py
>>> predicted_token_class_ids = tf.math.argmax(logits, axis=-1)
>>> predicted_token_class = [model.config.id2label[t] for t in predicted_token_class_ids[0].numpy().tolist()]
>>> predicted_token_class
['O',
'O',
'B-location',
'I-location',
'B-group',
'O',
'O',
'O',
'O',
'O',
'O',
'O',
'O',
'B-location',
'B-location',
'O',
'O']
```
</tf>
</frameworkcontent>
| 0 |
hf_public_repos/transformers/docs/source/ko | hf_public_repos/transformers/docs/source/ko/tasks/zero_shot_image_classification.md | <!--Copyright 2023 The HuggingFace Team. All rights reserved.
Licensed under the Apache License, Version 2.0 (the "License"); you may not use this file except in compliance with
the License. You may obtain a copy of the License at
http://www.apache.org/licenses/LICENSE-2.0
Unless required by applicable law or agreed to in writing, software distributed under the License is distributed on
an "AS IS" BASIS, WITHOUT WARRANTIES OR CONDITIONS OF ANY KIND, either express or implied. See the License for the
specific language governing permissions and limitations under the License.
โ ๏ธ Note that this file is in Markdown but contain specific syntax for our doc-builder (similar to MDX) that may not be
rendered properly in your Markdown viewer.
-->
# ์ ๋ก์ท(zero-shot) ์ด๋ฏธ์ง ๋ถ๋ฅ[[zeroshot-image-classification]]
[[open-in-colab]]
์ ๋ก์ท(zero-shot) ์ด๋ฏธ์ง ๋ถ๋ฅ๋ ํน์ ์นดํ
๊ณ ๋ฆฌ์ ์์๊ฐ ํฌํจ๋ ๋ฐ์ดํฐ๋ฅผ ํ์ต๋์ง ์์ ๋ชจ๋ธ์ ์ฌ์ฉํด ์ด๋ฏธ์ง ๋ถ๋ฅ๋ฅผ ์ํํ๋ ์์
์
๋๋ค.
์ผ๋ฐ์ ์ผ๋ก ์ด๋ฏธ์ง ๋ถ๋ฅ๋ฅผ ์ํด์๋ ๋ ์ด๋ธ์ด ๋ฌ๋ฆฐ ํน์ ์ด๋ฏธ์ง ๋ฐ์ดํฐ๋ก ๋ชจ๋ธ ํ์ต์ด ํ์ํ๋ฉฐ, ์ด ๋ชจ๋ธ์ ํน์ ์ด๋ฏธ์ง์ ํน์ง์ ๋ ์ด๋ธ์ "๋งคํ"ํ๋ ๋ฐฉ๋ฒ์ ํ์ตํฉ๋๋ค.
์๋ก์ด ๋ ์ด๋ธ์ด ์๋ ๋ถ๋ฅ ์์
์ ์ด๋ฌํ ๋ชจ๋ธ์ ์ฌ์ฉํด์ผ ํ๋ ๊ฒฝ์ฐ์๋, ๋ชจ๋ธ์ "์ฌ๋ณด์ "ํ๊ธฐ ์ํด ๋ฏธ์ธ ์กฐ์ ์ด ํ์ํฉ๋๋ค.
์ด์ ๋์กฐ์ ์ผ๋ก, ์ ๋ก์ท ๋๋ ๊ฐ๋ฐฉํ ์ดํ(open vocabulary) ์ด๋ฏธ์ง ๋ถ๋ฅ ๋ชจ๋ธ์ ์ผ๋ฐ์ ์ผ๋ก ๋๊ท๋ชจ ์ด๋ฏธ์ง ๋ฐ์ดํฐ์ ํด๋น ์ค๋ช
์ ๋ํด ํ์ต๋ ๋ฉํฐ๋ชจ๋ฌ(multimodal) ๋ชจ๋ธ์
๋๋ค.
์ด๋ฌํ ๋ชจ๋ธ์ ์ ๋ก์ท ์ด๋ฏธ์ง ๋ถ๋ฅ๋ฅผ ํฌํจํ ๋ง์ ๋ค์ด์คํธ๋ฆผ ์์
์ ์ฌ์ฉํ ์ ์๋ ์ ๋ ฌ๋(aligned) ๋น์ ์ธ์ด ํํ์ ํ์ตํฉ๋๋ค.
์ด๋ ์ด๋ฏธ์ง ๋ถ๋ฅ์ ๋ํ ๋ณด๋ค ์ ์ฐํ ์ ๊ทผ ๋ฐฉ์์ผ๋ก, ์ถ๊ฐ ํ์ต ๋ฐ์ดํฐ ์์ด ์๋ก์ด ๋ ์ด๋ธ์ด๋ ํ์ตํ์ง ๋ชปํ ์นดํ
๊ณ ๋ฆฌ์ ๋ํด ๋ชจ๋ธ์ ์ผ๋ฐํํ ์ ์์ต๋๋ค.
๋ํ, ์ฌ์ฉ์๊ฐ ๋์ ๊ฐ์ฒด์ ๋ํ ์์ ํ์์ ํ
์คํธ ์ค๋ช
์ผ๋ก ์ด๋ฏธ์ง๋ฅผ ๊ฒ์ํ ์ ์์ต๋๋ค.
์ด๋ฒ ๊ฐ์ด๋์์ ๋ฐฐ์ธ ๋ด์ฉ์ ๋ค์๊ณผ ๊ฐ์ต๋๋ค:
* ์ ๋ก์ท ์ด๋ฏธ์ง ๋ถ๋ฅ ํ์ดํ๋ผ์ธ ๋ง๋ค๊ธฐ
* ์ง์ ์ ๋ก์ท ์ด๋ฏธ์ง ๋ถ๋ฅ ๋ชจ๋ธ ์ถ๋ก ์คํํ๊ธฐ
์์ํ๊ธฐ ์ ์ ํ์ํ ๋ผ์ด๋ธ๋ฌ๋ฆฌ๊ฐ ๋ชจ๋ ์ค์น๋์ด ์๋์ง ํ์ธํ์ธ์:
```bash
pip install -q transformers
```
## ์ ๋ก์ท(zero-shot) ์ด๋ฏธ์ง ๋ถ๋ฅ ํ์ดํ๋ผ์ธ[[zeroshot-image-classification-pipeline]]
[`pipeline`]์ ํ์ฉํ๋ฉด ๊ฐ์ฅ ๊ฐ๋จํ๊ฒ ์ ๋ก์ท ์ด๋ฏธ์ง ๋ถ๋ฅ๋ฅผ ์ง์ํ๋ ๋ชจ๋ธ๋ก ์ถ๋ก ํด๋ณผ ์ ์์ต๋๋ค.
[Hugging Face Hub์ ์
๋ก๋๋ ์ฒดํฌํฌ์ธํธ](https://huggingface.co/models?pipeline_tag=zero-shot-image-classification&sort=downloads)์์ ํ์ดํ๋ผ์ธ์ ์ธ์คํด์คํํฉ๋๋ค.
```python
>>> from transformers import pipeline
>>> checkpoint = "openai/clip-vit-large-patch14"
>>> detector = pipeline(model=checkpoint, task="zero-shot-image-classification")
```
๋ค์์ผ๋ก, ๋ถ๋ฅํ๊ณ ์ถ์ ์ด๋ฏธ์ง๋ฅผ ์ ํํ์ธ์.
```py
>>> from PIL import Image
>>> import requests
>>> url = "https://unsplash.com/photos/g8oS8-82DxI/download?ixid=MnwxMjA3fDB8MXx0b3BpY3x8SnBnNktpZGwtSGt8fHx8fDJ8fDE2NzgxMDYwODc&force=true&w=640"
>>> image = Image.open(requests.get(url, stream=True).raw)
>>> image
```
<div class="flex justify-center">
<img src="https://huggingface.co/datasets/huggingface/documentation-images/resolve/main/transformers/tasks/owl.jpg" alt="Photo of an owl"/>
</div>
์ด๋ฏธ์ง์ ํด๋น ์ด๋ฏธ์ง์ ํ๋ณด ๋ ์ด๋ธ์ธ `candidate_labels`๋ฅผ ํ์ดํ๋ผ์ธ์ผ๋ก ์ ๋ฌํฉ๋๋ค.
์ฌ๊ธฐ์๋ ์ด๋ฏธ์ง๋ฅผ ์ง์ ์ ๋ฌํ์ง๋ง, ์ปดํจํฐ์ ์ ์ฅ๋ ์ด๋ฏธ์ง์ ๊ฒฝ๋ก๋ url๋ก ์ ๋ฌํ ์๋ ์์ต๋๋ค.
`candidate_labels`๋ ์ด ์์์ฒ๋ผ ๊ฐ๋จํ ๋จ์ด์ผ ์๋ ์๊ณ ์ข ๋ ์ค๋ช
์ ์ธ ๋จ์ด์ผ ์๋ ์์ต๋๋ค.
```py
>>> predictions = classifier(image, candidate_labels=["fox", "bear", "seagull", "owl"])
>>> predictions
[{'score': 0.9996670484542847, 'label': 'owl'},
{'score': 0.000199399160919711, 'label': 'seagull'},
{'score': 7.392891711788252e-05, 'label': 'fox'},
{'score': 5.96074532950297e-05, 'label': 'bear'}]
```
## ์ง์ ์ ๋ก์ท(zero-shot) ์ด๋ฏธ์ง ๋ถ๋ฅํ๊ธฐ[[zeroshot-image-classification-by-hand]]
์ด์ ์ ๋ก์ท ์ด๋ฏธ์ง ๋ถ๋ฅ ํ์ดํ๋ผ์ธ ์ฌ์ฉ ๋ฐฉ๋ฒ์ ์ดํด๋ณด์์ผ๋, ์คํํ๋ ๋ฐฉ๋ฒ์ ์ดํด๋ณด๊ฒ ์ต๋๋ค.
[Hugging Face Hub์ ์
๋ก๋๋ ์ฒดํฌํฌ์ธํธ](https://huggingface.co/models?pipeline_tag=zero-shot-image-classification&sort=downloads)์์ ๋ชจ๋ธ๊ณผ ํ๋ก์ธ์๋ฅผ ๊ฐ์ ธ์ค๋ ๊ฒ์ผ๋ก ์์ํฉ๋๋ค.
์ฌ๊ธฐ์๋ ์ด์ ๊ณผ ๋์ผํ ์ฒดํฌํฌ์ธํธ๋ฅผ ์ฌ์ฉํ๊ฒ ์ต๋๋ค:
```py
>>> from transformers import AutoProcessor, AutoModelForZeroShotImageClassification
>>> model = AutoModelForZeroShotImageClassification.from_pretrained(checkpoint)
>>> processor = AutoProcessor.from_pretrained(checkpoint)
```
๋ค๋ฅธ ์ด๋ฏธ์ง๋ฅผ ์ฌ์ฉํด ๋ณด๊ฒ ์ต๋๋ค.
```py
>>> from PIL import Image
>>> import requests
>>> url = "https://unsplash.com/photos/xBRQfR2bqNI/download?ixid=MnwxMjA3fDB8MXxhbGx8fHx8fHx8fHwxNjc4Mzg4ODEx&force=true&w=640"
>>> image = Image.open(requests.get(url, stream=True).raw)
>>> image
```
<div class="flex justify-center">
<img src="https://huggingface.co/datasets/huggingface/documentation-images/resolve/main/transformers/tasks/car.jpg" alt="Photo of a car"/>
</div>
ํ๋ก์ธ์๋ฅผ ์ฌ์ฉํด ๋ชจ๋ธ์ ์
๋ ฅ์ ์ค๋นํฉ๋๋ค.
ํ๋ก์ธ์๋ ๋ชจ๋ธ์ ์
๋ ฅ์ผ๋ก ์ฌ์ฉํ๊ธฐ ์ํด ์ด๋ฏธ์ง ํฌ๊ธฐ๋ฅผ ๋ณํํ๊ณ ์ ๊ทํํ๋ ์ด๋ฏธ์ง ํ๋ก์ธ์์ ํ
์คํธ ์
๋ ฅ์ ์ฒ๋ฆฌํ๋ ํ ํฌ๋์ด์ ๋ก ๊ตฌ์ฑ๋ฉ๋๋ค.
```py
>>> candidate_labels = ["tree", "car", "bike", "cat"]
>>> inputs = processor(images=image, text=candidate_labels, return_tensors="pt", padding=True)
```
๋ชจ๋ธ์ ์
๋ ฅ์ ์ ๋ฌํ๊ณ , ๊ฒฐ๊ณผ๋ฅผ ํ์ฒ๋ฆฌํฉ๋๋ค:
```py
>>> import torch
>>> with torch.no_grad():
... outputs = model(**inputs)
>>> logits = outputs.logits_per_image[0]
>>> probs = logits.softmax(dim=-1).numpy()
>>> scores = probs.tolist()
>>> result = [
... {"score": score, "label": candidate_label}
... for score, candidate_label in sorted(zip(probs, candidate_labels), key=lambda x: -x[0])
... ]
>>> result
[{'score': 0.998572, 'label': 'car'},
{'score': 0.0010570387, 'label': 'bike'},
{'score': 0.0003393686, 'label': 'tree'},
{'score': 3.1572064e-05, 'label': 'cat'}]
``` | 0 |
hf_public_repos/transformers/docs/source/ko | hf_public_repos/transformers/docs/source/ko/tasks/video_classification.md | <!--Copyright 2023 The HuggingFace Team. All rights reserved.
Licensed under the Apache License, Version 2.0 (the "License"); you may not use this file except in compliance with
the License. You may obtain a copy of the License at
http://www.apache.org/licenses/LICENSE-2.0
Unless required by applicable law or agreed to in writing, software distributed under the License is distributed on
an "AS IS" BASIS, WITHOUT WARRANTIES OR CONDITIONS OF ANY KIND, either express or implied. See the License for the
specific language governing permissions and limitations under the License.
โ ๏ธ Note that this file is in Markdown but contain specific syntax for our doc-builder (similar to MDX) that may not be
rendered properly in your Markdown viewer.
-->
# ์์ ๋ถ๋ฅ [[video-classification]]
[[open-in-colab]]
์์ ๋ถ๋ฅ๋ ์์ ์ ์ฒด์ ๋ ์ด๋ธ ๋๋ ํด๋์ค๋ฅผ ์ง์ ํ๋ ์์
์
๋๋ค. ๊ฐ ์์์๋ ํ๋์ ํด๋์ค๊ฐ ์์ ๊ฒ์ผ๋ก ์์๋ฉ๋๋ค. ์์ ๋ถ๋ฅ ๋ชจ๋ธ์ ์์์ ์
๋ ฅ์ผ๋ก ๋ฐ์ ์ด๋ ํด๋์ค์ ์ํ๋์ง์ ๋ํ ์์ธก์ ๋ฐํํฉ๋๋ค. ์ด๋ฌํ ๋ชจ๋ธ์ ์์์ด ์ด๋ค ๋ด์ฉ์ธ์ง ๋ถ๋ฅํ๋ ๋ฐ ์ฌ์ฉ๋ ์ ์์ต๋๋ค. ์์ ๋ถ๋ฅ์ ์ค์ ์์ฉ ์๋ ํผํธ๋์ค ์ฑ์์ ์ ์ฉํ ๋์ / ์ด๋ ์ธ์ ์๋น์ค๊ฐ ์์ต๋๋ค. ์ด๋ ๋ํ ์๊ฐ ์ฅ์ ์ธ์ด ์ด๋ํ ๋ ๋ณด์กฐํ๋๋ฐ ์ฌ์ฉ๋ ์ ์์ต๋๋ค
์ด ๊ฐ์ด๋์์๋ ๋ค์์ ์ํํ๋ ๋ฐฉ๋ฒ์ ๋ณด์ฌ์ค๋๋ค:
1. [UCF101](https://www.crcv.ucf.edu/data/UCF101.php) ๋ฐ์ดํฐ ์ธํธ์ ํ์ ์งํฉ์ ํตํด [VideoMAE](https://huggingface.co/docs/transformers/main/en/model_doc/videomae) ๋ชจ๋ธ์ ๋ฏธ์ธ ์กฐ์ ํ๊ธฐ.
2. ๋ฏธ์ธ ์กฐ์ ํ ๋ชจ๋ธ์ ์ถ๋ก ์ ์ฌ์ฉํ๊ธฐ.
<Tip>
์ด ํํ ๋ฆฌ์ผ์์ ์ค๋ช
ํ๋ ์์
์ ๋ค์ ๋ชจ๋ธ ์ํคํ
์ฒ์์ ์ง์๋ฉ๋๋ค:
<!--This tip is automatically generated by `make fix-copies`, do not fill manually!-->
[TimeSformer](../model_doc/timesformer), [VideoMAE](../model_doc/videomae)
<!--End of the generated tip-->
</Tip>
์์ํ๊ธฐ ์ ์ ํ์ํ ๋ชจ๋ ๋ผ์ด๋ธ๋ฌ๋ฆฌ๊ฐ ์ค์น๋์๋์ง ํ์ธํ์ธ์:
```bash
pip install -q pytorchvideo transformers evaluate
```
์์์ ์ฒ๋ฆฌํ๊ณ ์ค๋นํ๊ธฐ ์ํด [PyTorchVideo](https://pytorchvideo.org/)(์ดํ `pytorchvideo`)๋ฅผ ์ฌ์ฉํฉ๋๋ค.
์ปค๋ฎค๋ํฐ์ ๋ชจ๋ธ์ ์
๋ก๋ํ๊ณ ๊ณต์ ํ ์ ์๋๋ก Hugging Face ๊ณ์ ์ ๋ก๊ทธ์ธํ๋ ๊ฒ์ ๊ถ์ฅํฉ๋๋ค. ํ๋กฌํํธ๊ฐ ๋ํ๋๋ฉด ํ ํฐ์ ์
๋ ฅํ์ฌ ๋ก๊ทธ์ธํ์ธ์:
```py
>>> from huggingface_hub import notebook_login
>>> notebook_login()
```
## UCF101 ๋ฐ์ดํฐ์
๋ถ๋ฌ์ค๊ธฐ [[load-ufc101-dataset]]
[UCF-101](https://www.crcv.ucf.edu/data/UCF101.php) ๋ฐ์ดํฐ ์ธํธ์ ํ์ ์งํฉ(subset)์ ๋ถ๋ฌ์ค๋ ๊ฒ์ผ๋ก ์์ํ ์ ์์ต๋๋ค. ์ ์ฒด ๋ฐ์ดํฐ ์ธํธ๋ฅผ ํ์ตํ๋๋ฐ ๋ ๋ง์ ์๊ฐ์ ํ ์ ํ๊ธฐ ์ ์ ๋ฐ์ดํฐ์ ํ์ ์งํฉ์ ๋ถ๋ฌ์ ๋ชจ๋ ๊ฒ์ด ์ ์๋ํ๋์ง ์คํํ๊ณ ํ์ธํ ์ ์์ต๋๋ค.
```py
>>> from huggingface_hub import hf_hub_download
>>> hf_dataset_identifier = "sayakpaul/ucf101-subset"
>>> filename = "UCF101_subset.tar.gz"
>>> file_path = hf_hub_download(repo_id=hf_dataset_identifier, filename=filename, repo_type="dataset")
```
๋ฐ์ดํฐ ์ธํธ์ ํ์ ์งํฉ์ด ๋ค์ด๋ก๋ ๋๋ฉด, ์์ถ๋ ํ์ผ์ ์์ถ์ ํด์ ํด์ผ ํฉ๋๋ค:
```py
>>> import tarfile
>>> with tarfile.open(file_path) as t:
... t.extractall(".")
```
์ ์ฒด ๋ฐ์ดํฐ ์ธํธ๋ ๋ค์๊ณผ ๊ฐ์ด ๊ตฌ์ฑ๋์ด ์์ต๋๋ค.
```bash
UCF101_subset/
train/
BandMarching/
video_1.mp4
video_2.mp4
...
Archery
video_1.mp4
video_2.mp4
...
...
val/
BandMarching/
video_1.mp4
video_2.mp4
...
Archery
video_1.mp4
video_2.mp4
...
...
test/
BandMarching/
video_1.mp4
video_2.mp4
...
Archery
video_1.mp4
video_2.mp4
...
...
```
์ ๋ ฌ๋ ์์์ ๊ฒฝ๋ก๋ ๋ค์๊ณผ ๊ฐ์ต๋๋ค:
```bash
...
'UCF101_subset/train/ApplyEyeMakeup/v_ApplyEyeMakeup_g07_c04.avi',
'UCF101_subset/train/ApplyEyeMakeup/v_ApplyEyeMakeup_g07_c06.avi',
'UCF101_subset/train/ApplyEyeMakeup/v_ApplyEyeMakeup_g08_c01.avi',
'UCF101_subset/train/ApplyEyeMakeup/v_ApplyEyeMakeup_g09_c02.avi',
'UCF101_subset/train/ApplyEyeMakeup/v_ApplyEyeMakeup_g09_c06.avi'
...
```
๋์ผํ ๊ทธ๋ฃน/์ฅ๋ฉด์ ์ํ๋ ์์ ํด๋ฆฝ์ ํ์ผ ๊ฒฝ๋ก์์ `g`๋ก ํ์๋์ด ์์ต๋๋ค. ์๋ฅผ ๋ค๋ฉด, `v_ApplyEyeMakeup_g07_c04.avi`์ `v_ApplyEyeMakeup_g07_c06.avi` ์ด ์์ต๋๋ค. ์ด ๋์ ๊ฐ์ ๊ทธ๋ฃน์
๋๋ค.
๊ฒ์ฆ ๋ฐ ํ๊ฐ ๋ฐ์ดํฐ ๋ถํ ์ ํ ๋, [๋ฐ์ดํฐ ๋์ถ(data leakage)](https://www.kaggle.com/code/alexisbcook/data-leakage)์ ๋ฐฉ์งํ๊ธฐ ์ํด ๋์ผํ ๊ทธ๋ฃน / ์ฅ๋ฉด์ ์์ ํด๋ฆฝ์ ์ฌ์ฉํ์ง ์์์ผ ํฉ๋๋ค. ์ด ํํ ๋ฆฌ์ผ์์ ์ฌ์ฉํ๋ ํ์ ์งํฉ์ ์ด๋ฌํ ์ ๋ณด๋ฅผ ๊ณ ๋ คํ๊ณ ์์ต๋๋ค.
๊ทธ ๋ค์์ผ๋ก, ๋ฐ์ดํฐ ์ธํธ์ ์กด์ฌํ๋ ๋ผ๋ฒจ์ ์ถ์ถํฉ๋๋ค. ๋ํ, ๋ชจ๋ธ์ ์ด๊ธฐํํ ๋ ๋์์ด ๋ ๋์
๋๋ฆฌ(dictionary data type)๋ฅผ ์์ฑํฉ๋๋ค.
* `label2id`: ํด๋์ค ์ด๋ฆ์ ์ ์์ ๋งคํํฉ๋๋ค.
* `id2label`: ์ ์๋ฅผ ํด๋์ค ์ด๋ฆ์ ๋งคํํฉ๋๋ค.
```py
>>> class_labels = sorted({str(path).split("/")[2] for path in all_video_file_paths})
>>> label2id = {label: i for i, label in enumerate(class_labels)}
>>> id2label = {i: label for label, i in label2id.items()}
>>> print(f"Unique classes: {list(label2id.keys())}.")
# Unique classes: ['ApplyEyeMakeup', 'ApplyLipstick', 'Archery', 'BabyCrawling', 'BalanceBeam', 'BandMarching', 'BaseballPitch', 'Basketball', 'BasketballDunk', 'BenchPress'].
```
์ด ๋ฐ์ดํฐ ์ธํธ์๋ ์ด 10๊ฐ์ ๊ณ ์ ํ ํด๋์ค๊ฐ ์์ต๋๋ค. ๊ฐ ํด๋์ค๋ง๋ค 30๊ฐ์ ์์์ด ํ๋ จ ์ธํธ์ ์์ต๋๋ค
## ๋ฏธ์ธ ์กฐ์ ํ๊ธฐ ์ํด ๋ชจ๋ธ ๊ฐ์ ธ์ค๊ธฐ [[load-a-model-to-fine-tune]]
์ฌ์ ํ๋ จ๋ ์ฒดํฌํฌ์ธํธ์ ์ฒดํฌํฌ์ธํธ์ ์ฐ๊ด๋ ์ด๋ฏธ์ง ํ๋ก์ธ์๋ฅผ ์ฌ์ฉํ์ฌ ์์ ๋ถ๋ฅ ๋ชจ๋ธ์ ์ธ์คํด์คํํฉ๋๋ค. ๋ชจ๋ธ์ ์ธ์ฝ๋์๋ ๋ฏธ๋ฆฌ ํ์ต๋ ๋งค๊ฐ๋ณ์๊ฐ ์ ๊ณต๋๋ฉฐ, ๋ถ๋ฅ ํค๋(๋ฐ์ดํฐ๋ฅผ ๋ถ๋ฅํ๋ ๋ง์ง๋ง ๋ ์ด์ด)๋ ๋ฌด์์๋ก ์ด๊ธฐํ๋ฉ๋๋ค. ๋ฐ์ดํฐ ์ธํธ์ ์ ์ฒ๋ฆฌ ํ์ดํ๋ผ์ธ์ ์์ฑํ ๋๋ ์ด๋ฏธ์ง ํ๋ก์ธ์๊ฐ ์ ์ฉํฉ๋๋ค.
```py
>>> from transformers import VideoMAEImageProcessor, VideoMAEForVideoClassification
>>> model_ckpt = "MCG-NJU/videomae-base"
>>> image_processor = VideoMAEImageProcessor.from_pretrained(model_ckpt)
>>> model = VideoMAEForVideoClassification.from_pretrained(
... model_ckpt,
... label2id=label2id,
... id2label=id2label,
... ignore_mismatched_sizes=True, # provide this in case you're planning to fine-tune an already fine-tuned checkpoint
... )
```
๋ชจ๋ธ์ ๊ฐ์ ธ์ค๋ ๋์, ๋ค์๊ณผ ๊ฐ์ ๊ฒฝ๊ณ ๋ฅผ ๋ง์ฃผ์น ์ ์์ต๋๋ค:
```bash
Some weights of the model checkpoint at MCG-NJU/videomae-base were not used when initializing VideoMAEForVideoClassification: [..., 'decoder.decoder_layers.1.attention.output.dense.bias', 'decoder.decoder_layers.2.attention.attention.key.weight']
- This IS expected if you are initializing VideoMAEForVideoClassification from the checkpoint of a model trained on another task or with another architecture (e.g. initializing a BertForSequenceClassification model from a BertForPreTraining model).
- This IS NOT expected if you are initializing VideoMAEForVideoClassification from the checkpoint of a model that you expect to be exactly identical (initializing a BertForSequenceClassification model from a BertForSequenceClassification model).
Some weights of VideoMAEForVideoClassification were not initialized from the model checkpoint at MCG-NJU/videomae-base and are newly initialized: ['classifier.bias', 'classifier.weight']
You should probably TRAIN this model on a down-stream task to be able to use it for predictions and inference.
```
์ ๊ฒฝ๊ณ ๋ ์ฐ๋ฆฌ๊ฐ ์ผ๋ถ ๊ฐ์ค์น(์: `classifier` ์ธต์ ๊ฐ์ค์น์ ํธํฅ)๋ฅผ ๋ฒ๋ฆฌ๊ณ ์๋ก์ด `classifier` ์ธต์ ๊ฐ์ค์น์ ํธํฅ์ ๋ฌด์์๋ก ์ด๊ธฐํํ๊ณ ์๋ค๋ ๊ฒ์ ์๋ ค์ค๋๋ค. ์ด ๊ฒฝ์ฐ์๋ ๋ฏธ๋ฆฌ ํ์ต๋ ๊ฐ์ค์น๊ฐ ์๋ ์๋ก์ด ํค๋๋ฅผ ์ถ๊ฐํ๊ณ ์์ผ๋ฏ๋ก, ๋ผ์ด๋ธ๋ฌ๋ฆฌ๊ฐ ๋ชจ๋ธ์ ์ถ๋ก ์ ์ฌ์ฉํ๊ธฐ ์ ์ ๋ฏธ์ธ ์กฐ์ ํ๋ผ๊ณ ๊ฒฝ๊ณ ๋ฅผ ๋ณด๋ด๋ ๊ฒ์ ๋น์ฐํฉ๋๋ค. ๊ทธ๋ฆฌ๊ณ ์ด์ ์ฐ๋ฆฌ๋ ์ด ๋ชจ๋ธ์ ๋ฏธ์ธ ์กฐ์ ํ ์์ ์
๋๋ค.
**์ฐธ๊ณ ** ์ด [์ฒดํฌํฌ์ธํธ](https://huggingface.co/MCG-NJU/videomae-base-finetuned-kinetics)๋ ๋๋ฉ์ธ์ด ๋ง์ด ์ค์ฒฉ๋ ์ ์ฌํ ๋ค์ด์คํธ๋ฆผ ์์
์ ๋ํด ๋ฏธ์ธ ์กฐ์ ํ์ฌ ์ป์ ์ฒดํฌํฌ์ธํธ์ด๋ฏ๋ก ์ด ์์
์์ ๋ ๋์ ์ฑ๋ฅ์ ๋ณด์ผ ์ ์์ต๋๋ค. `MCG-NJU/videomae-base-finetuned-kinetics` ๋ฐ์ดํฐ ์ธํธ๋ฅผ ๋ฏธ์ธ ์กฐ์ ํ์ฌ ์ป์ [์ฒดํฌํฌ์ธํธ](https://huggingface.co/sayakpaul/videomae-base-finetuned-kinetics-finetuned-ucf101-subset)๋ ์์ต๋๋ค.
## ํ๋ จ์ ์ํ ๋ฐ์ดํฐ ์ธํธ ์ค๋นํ๊ธฐ[[prepare-the-datasets-for-training]]
์์ ์ ์ฒ๋ฆฌ๋ฅผ ์ํด [PyTorchVideo ๋ผ์ด๋ธ๋ฌ๋ฆฌ](https://pytorchvideo.org/)๋ฅผ ํ์ฉํ ๊ฒ์
๋๋ค. ํ์ํ ์ข
์์ฑ์ ๊ฐ์ ธ์ค๋ ๊ฒ์ผ๋ก ์์ํ์ธ์.
```py
>>> import pytorchvideo.data
>>> from pytorchvideo.transforms import (
... ApplyTransformToKey,
... Normalize,
... RandomShortSideScale,
... RemoveKey,
... ShortSideScale,
... UniformTemporalSubsample,
... )
>>> from torchvision.transforms import (
... Compose,
... Lambda,
... RandomCrop,
... RandomHorizontalFlip,
... Resize,
... )
```
ํ์ต ๋ฐ์ดํฐ ์ธํธ ๋ณํ์๋ '๊ท ์ผํ ์๊ฐ ์ํ๋ง(uniform temporal subsampling)', 'ํฝ์
์ ๊ทํ(pixel normalization)', '๋๋ค ์๋ผ๋ด๊ธฐ(random cropping)' ๋ฐ '๋๋ค ์ํ ๋ค์ง๊ธฐ(random horizontal flipping)'์ ์กฐํฉ์ ์ฌ์ฉํฉ๋๋ค. ๊ฒ์ฆ ๋ฐ ํ๊ฐ ๋ฐ์ดํฐ ์ธํธ ๋ณํ์๋ '๋๋ค ์๋ผ๋ด๊ธฐ'์ '๋๋ค ๋ค์ง๊ธฐ'๋ฅผ ์ ์ธํ ๋์ผํ ๋ณํ ์ฒด์ธ์ ์ ์งํฉ๋๋ค. ์ด๋ฌํ ๋ณํ์ ๋ํด ์์ธํ ์์๋ณด๋ ค๋ฉด [PyTorchVideo ๊ณต์ ๋ฌธ์](https://pytorchvideo.org)๋ฅผ ํ์ธํ์ธ์.
์ฌ์ ํ๋ จ๋ ๋ชจ๋ธ๊ณผ ๊ด๋ จ๋ ์ด๋ฏธ์ง ํ๋ก์ธ์๋ฅผ ์ฌ์ฉํ์ฌ ๋ค์ ์ ๋ณด๋ฅผ ์ป์ ์ ์์ต๋๋ค:
* ์์ ํ๋ ์ ํฝ์
์ ์ ๊ทํํ๋ ๋ฐ ์ฌ์ฉ๋๋ ์ด๋ฏธ์ง ํ๊ท ๊ณผ ํ์ค ํธ์ฐจ
* ์์ ํ๋ ์์ด ์กฐ์ ๋ ๊ณต๊ฐ ํด์๋
๋จผ์ , ๋ช ๊ฐ์ง ์์๋ฅผ ์ ์ํฉ๋๋ค.
```py
>>> mean = image_processor.image_mean
>>> std = image_processor.image_std
>>> if "shortest_edge" in image_processor.size:
... height = width = image_processor.size["shortest_edge"]
>>> else:
... height = image_processor.size["height"]
... width = image_processor.size["width"]
>>> resize_to = (height, width)
>>> num_frames_to_sample = model.config.num_frames
>>> sample_rate = 4
>>> fps = 30
>>> clip_duration = num_frames_to_sample * sample_rate / fps
```
์ด์ ๋ฐ์ดํฐ ์ธํธ์ ํนํ๋ ์ ์ฒ๋ฆฌ(transform)๊ณผ ๋ฐ์ดํฐ ์ธํธ ์์ฒด๋ฅผ ์ ์ํฉ๋๋ค. ๋จผ์ ํ๋ จ ๋ฐ์ดํฐ ์ธํธ๋ก ์์ํฉ๋๋ค:
```py
>>> train_transform = Compose(
... [
... ApplyTransformToKey(
... key="video",
... transform=Compose(
... [
... UniformTemporalSubsample(num_frames_to_sample),
... Lambda(lambda x: x / 255.0),
... Normalize(mean, std),
... RandomShortSideScale(min_size=256, max_size=320),
... RandomCrop(resize_to),
... RandomHorizontalFlip(p=0.5),
... ]
... ),
... ),
... ]
... )
>>> train_dataset = pytorchvideo.data.Ucf101(
... data_path=os.path.join(dataset_root_path, "train"),
... clip_sampler=pytorchvideo.data.make_clip_sampler("random", clip_duration),
... decode_audio=False,
... transform=train_transform,
... )
```
๊ฐ์ ๋ฐฉ์์ ์์
ํ๋ฆ์ ๊ฒ์ฆ๊ณผ ํ๊ฐ ์ธํธ์๋ ์ ์ฉํ ์ ์์ต๋๋ค.
```py
>>> val_transform = Compose(
... [
... ApplyTransformToKey(
... key="video",
... transform=Compose(
... [
... UniformTemporalSubsample(num_frames_to_sample),
... Lambda(lambda x: x / 255.0),
... Normalize(mean, std),
... Resize(resize_to),
... ]
... ),
... ),
... ]
... )
>>> val_dataset = pytorchvideo.data.Ucf101(
... data_path=os.path.join(dataset_root_path, "val"),
... clip_sampler=pytorchvideo.data.make_clip_sampler("uniform", clip_duration),
... decode_audio=False,
... transform=val_transform,
... )
>>> test_dataset = pytorchvideo.data.Ucf101(
... data_path=os.path.join(dataset_root_path, "test"),
... clip_sampler=pytorchvideo.data.make_clip_sampler("uniform", clip_duration),
... decode_audio=False,
... transform=val_transform,
... )
```
**์ฐธ๊ณ **: ์์ ๋ฐ์ดํฐ ์ธํธ์ ํ์ดํ๋ผ์ธ์ [๊ณต์ ํ์ดํ ์น ์์ ](https://pytorchvideo.org/docs/tutorial_classification#dataset)์์ ๊ฐ์ ธ์จ ๊ฒ์
๋๋ค. ์ฐ๋ฆฌ๋ UCF-101 ๋ฐ์ดํฐ์
์ ๋ง๊ฒ [`pytorchvideo.data.Ucf101()`](https://pytorchvideo.readthedocs.io/en/latest/api/data/data.html#pytorchvideo.data.Ucf101) ํจ์๋ฅผ ์ฌ์ฉํ๊ณ ์์ต๋๋ค. ๋ด๋ถ์ ์ผ๋ก ์ด ํจ์๋ [`pytorchvideo.data.labeled_video_dataset.LabeledVideoDataset`](https://pytorchvideo.readthedocs.io/en/latest/api/data/data.html#pytorchvideo.data.LabeledVideoDataset) ๊ฐ์ฒด๋ฅผ ๋ฐํํฉ๋๋ค. `LabeledVideoDataset` ํด๋์ค๋ PyTorchVideo ๋ฐ์ดํฐ์
์์ ๋ชจ๋ ์์ ๊ด๋ จ ์์
์ ๊ธฐ๋ณธ ํด๋์ค์
๋๋ค. ๋ฐ๋ผ์ PyTorchVideo์์ ๋ฏธ๋ฆฌ ์ ๊ณตํ์ง ์๋ ์ฌ์ฉ์ ์ง์ ๋ฐ์ดํฐ ์ธํธ๋ฅผ ์ฌ์ฉํ๋ ค๋ฉด, ์ด ํด๋์ค๋ฅผ ์ ์ ํ๊ฒ ํ์ฅํ๋ฉด ๋ฉ๋๋ค. ๋ ์์ธํ ์ฌํญ์ด ์๊ณ ์ถ๋ค๋ฉด `data` API [๋ฌธ์](https://pytorchvideo.readthedocs.io/en/latest/api/data/data.html) ๋ฅผ ์ฐธ๊ณ ํ์ธ์. ๋ํ ์์ ์์์ ์ ์ฌํ ๊ตฌ์กฐ๋ฅผ ๊ฐ๋ ๋ฐ์ดํฐ ์ธํธ๋ฅผ ์ฌ์ฉํ๊ณ ์๋ค๋ฉด, `pytorchvideo.data.Ucf101()` ํจ์๋ฅผ ์ฌ์ฉํ๋ ๋ฐ ๋ฌธ์ ๊ฐ ์์ ๊ฒ์
๋๋ค.
๋ฐ์ดํฐ ์ธํธ์ ์์์ ๊ฐ์๋ฅผ ์๊ธฐ ์ํด `num_videos` ์ธ์์ ์ ๊ทผํ ์ ์์ต๋๋ค.
```py
>>> print(train_dataset.num_videos, val_dataset.num_videos, test_dataset.num_videos)
# (300, 30, 75)
```
## ๋ ๋์ ๋๋ฒ๊น
์ ์ํด ์ ์ฒ๋ฆฌ ์์ ์๊ฐํํ๊ธฐ[[visualize-the-preprocessed-video-for-better-debugging]]
```py
>>> import imageio
>>> import numpy as np
>>> from IPython.display import Image
>>> def unnormalize_img(img):
... """Un-normalizes the image pixels."""
... img = (img * std) + mean
... img = (img * 255).astype("uint8")
... return img.clip(0, 255)
>>> def create_gif(video_tensor, filename="sample.gif"):
... """Prepares a GIF from a video tensor.
...
... The video tensor is expected to have the following shape:
... (num_frames, num_channels, height, width).
... """
... frames = []
... for video_frame in video_tensor:
... frame_unnormalized = unnormalize_img(video_frame.permute(1, 2, 0).numpy())
... frames.append(frame_unnormalized)
... kargs = {"duration": 0.25}
... imageio.mimsave(filename, frames, "GIF", **kargs)
... return filename
>>> def display_gif(video_tensor, gif_name="sample.gif"):
... """Prepares and displays a GIF from a video tensor."""
... video_tensor = video_tensor.permute(1, 0, 2, 3)
... gif_filename = create_gif(video_tensor, gif_name)
... return Image(filename=gif_filename)
>>> sample_video = next(iter(train_dataset))
>>> video_tensor = sample_video["video"]
>>> display_gif(video_tensor)
```
<div class="flex justify-center">
<img src="https://huggingface.co/datasets/huggingface/documentation-images/resolve/main/transformers/tasks/sample_gif.gif" alt="Person playing basketball"/>
</div>
## ๋ชจ๋ธ ํ๋ จํ๊ธฐ[[train-the-model]]
๐ค Transformers์ [`Trainer`](https://huggingface.co/docs/transformers/main_classes/trainer)๋ฅผ ์ฌ์ฉํ์ฌ ๋ชจ๋ธ์ ํ๋ จ์์ผ๋ณด์ธ์. `Trainer`๋ฅผ ์ธ์คํด์คํํ๋ ค๋ฉด ํ๋ จ ์ค์ ๊ณผ ํ๊ฐ ์งํ๋ฅผ ์ ์ํด์ผ ํฉ๋๋ค. ๊ฐ์ฅ ์ค์ํ ๊ฒ์ [`TrainingArguments`](https://huggingface.co/transformers/main_classes/trainer.html#transformers.TrainingArguments)์
๋๋ค. ์ด ํด๋์ค๋ ํ๋ จ์ ๊ตฌ์ฑํ๋ ๋ชจ๋ ์์ฑ์ ํฌํจํ๋ฉฐ, ํ๋ จ ์ค ์ฒดํฌํฌ์ธํธ๋ฅผ ์ ์ฅํ ์ถ๋ ฅ ํด๋ ์ด๋ฆ์ ํ์๋ก ํฉ๋๋ค. ๋ํ ๐ค Hub์ ๋ชจ๋ธ ์ ์ฅ์์ ๋ชจ๋ ์ ๋ณด๋ฅผ ๋๊ธฐํํ๋ ๋ฐ ๋์์ด ๋ฉ๋๋ค.
๋๋ถ๋ถ์ ํ๋ จ ์ธ์๋ ๋ฐ๋ก ์ค๋ช
ํ ํ์๋ ์์ต๋๋ค. ํ์ง๋ง ์ฌ๊ธฐ์์ ์ค์ํ ์ธ์๋ `remove_unused_columns=False` ์
๋๋ค. ์ด ์ธ์๋ ๋ชจ๋ธ์ ํธ์ถ ํจ์์์ ์ฌ์ฉ๋์ง ์๋ ๋ชจ๋ ์์ฑ ์ด(columns)์ ์ญ์ ํฉ๋๋ค. ๊ธฐ๋ณธ๊ฐ์ ์ผ๋ฐ์ ์ผ๋ก True์
๋๋ค. ์ด๋ ์ฌ์ฉ๋์ง ์๋ ๊ธฐ๋ฅ ์ด์ ์ญ์ ํ๋ ๊ฒ์ด ์ด์์ ์ด๋ฉฐ, ์
๋ ฅ์ ๋ชจ๋ธ์ ํธ์ถ ํจ์๋ก ํ๊ธฐ(unpack)๊ฐ ์ฌ์์ง๊ธฐ ๋๋ฌธ์
๋๋ค. ํ์ง๋ง ์ด ๊ฒฝ์ฐ์๋ `pixel_values`(๋ชจ๋ธ์ ์
๋ ฅ์ผ๋ก ํ์์ ์ธ ํค)๋ฅผ ์์ฑํ๊ธฐ ์ํด ์ฌ์ฉ๋์ง ์๋ ๊ธฐ๋ฅ('video'๊ฐ ํนํ ๊ทธ๋ ์ต๋๋ค)์ด ํ์ํฉ๋๋ค. ๋ฐ๋ผ์ remove_unused_columns์ False๋ก ์ค์ ํด์ผ ํฉ๋๋ค.
```py
>>> from transformers import TrainingArguments, Trainer
>>> model_name = model_ckpt.split("/")[-1]
>>> new_model_name = f"{model_name}-finetuned-ucf101-subset"
>>> num_epochs = 4
>>> args = TrainingArguments(
... new_model_name,
... remove_unused_columns=False,
... evaluation_strategy="epoch",
... save_strategy="epoch",
... learning_rate=5e-5,
... per_device_train_batch_size=batch_size,
... per_device_eval_batch_size=batch_size,
... warmup_ratio=0.1,
... logging_steps=10,
... load_best_model_at_end=True,
... metric_for_best_model="accuracy",
... push_to_hub=True,
... max_steps=(train_dataset.num_videos // batch_size) * num_epochs,
... )
```
`pytorchvideo.data.Ucf101()` ํจ์๋ก ๋ฐํ๋๋ ๋ฐ์ดํฐ ์ธํธ๋ `__len__` ๋ฉ์๋๊ฐ ์ด์๋์ด ์์ง ์์ต๋๋ค. ๋ฐ๋ผ์, `TrainingArguments`๋ฅผ ์ธ์คํด์คํํ ๋ `max_steps`๋ฅผ ์ ์ํด์ผ ํฉ๋๋ค.
๋ค์์ผ๋ก, ํ๊ฐ์งํ๋ฅผ ๋ถ๋ฌ์ค๊ณ , ์์ธก๊ฐ์์ ํ๊ฐ์งํ๋ฅผ ๊ณ์ฐํ ํจ์๋ฅผ ์ ์ํฉ๋๋ค. ํ์ํ ์ ์ฒ๋ฆฌ ์์
์ ์์ธก๋ ๋ก์ง(logits)์ argmax ๊ฐ์ ์ทจํ๋ ๊ฒ๋ฟ์
๋๋ค:
```py
import evaluate
metric = evaluate.load("accuracy")
def compute_metrics(eval_pred):
predictions = np.argmax(eval_pred.predictions, axis=1)
return metric.compute(predictions=predictions, references=eval_pred.label_ids)
```
**ํ๊ฐ์ ๋ํ ์ฐธ๊ณ ์ฌํญ**:
[VideoMAE ๋
ผ๋ฌธ](https://arxiv.org/abs/2203.12602)์์ ์ ์๋ ๋ค์๊ณผ ๊ฐ์ ํ๊ฐ ์ ๋ต์ ์ฌ์ฉํฉ๋๋ค. ํ
์คํธ ์์์์ ์ฌ๋ฌ ํด๋ฆฝ์ ์ ํํ๊ณ ๊ทธ ํด๋ฆฝ์ ๋ค์ํ ํฌ๋กญ์ ์ ์ฉํ์ฌ ์ง๊ณ ์ ์๋ฅผ ๋ณด๊ณ ํฉ๋๋ค. ๊ทธ๋ฌ๋ ์ด๋ฒ ํํ ๋ฆฌ์ผ์์๋ ๊ฐ๋จํจ๊ณผ ๊ฐ๊ฒฐํจ์ ์ํด ํด๋น ์ ๋ต์ ๊ณ ๋ คํ์ง ์์ต๋๋ค.
๋ํ, ์์ ๋ฅผ ๋ฌถ์ด์ ๋ฐฐ์น๋ฅผ ํ์ฑํ๋ `collate_fn`์ ์ ์ํด์ผํฉ๋๋ค. ๊ฐ ๋ฐฐ์น๋ `pixel_values`์ `labels`๋ผ๋ 2๊ฐ์ ํค๋ก ๊ตฌ์ฑ๋ฉ๋๋ค.
```py
>>> def collate_fn(examples):
... # permute to (num_frames, num_channels, height, width)
... pixel_values = torch.stack(
... [example["video"].permute(1, 0, 2, 3) for example in examples]
... )
... labels = torch.tensor([example["label"] for example in examples])
... return {"pixel_values": pixel_values, "labels": labels}
```
๊ทธ๋ฐ ๋ค์ ์ด ๋ชจ๋ ๊ฒ์ ๋ฐ์ดํฐ ์ธํธ์ ํจ๊ป `Trainer`์ ์ ๋ฌํ๊ธฐ๋ง ํ๋ฉด ๋ฉ๋๋ค:
```py
>>> trainer = Trainer(
... model,
... args,
... train_dataset=train_dataset,
... eval_dataset=val_dataset,
... tokenizer=image_processor,
... compute_metrics=compute_metrics,
... data_collator=collate_fn,
... )
```
๋ฐ์ดํฐ๋ฅผ ์ด๋ฏธ ์ฒ๋ฆฌํ๋๋ฐ๋ ๋ถ๊ตฌํ๊ณ `image_processor`๋ฅผ ํ ํฌ๋์ด์ ์ธ์๋ก ๋ฃ์ ์ด์ ๋ JSON์ผ๋ก ์ ์ฅ๋๋ ์ด๋ฏธ์ง ํ๋ก์ธ์ ๊ตฌ์ฑ ํ์ผ์ด Hub์ ์ ์ฅ์์ ์
๋ก๋๋๋๋ก ํ๊ธฐ ์ํจ์
๋๋ค.
`train` ๋ฉ์๋๋ฅผ ํธ์ถํ์ฌ ๋ชจ๋ธ์ ๋ฏธ์ธ ์กฐ์ ํ์ธ์:
```py
>>> train_results = trainer.train()
```
ํ์ต์ด ์๋ฃ๋๋ฉด, ๋ชจ๋ธ์ [`~transformers.Trainer.push_to_hub`] ๋ฉ์๋๋ฅผ ์ฌ์ฉํ์ฌ ํ๋ธ์ ๊ณต์ ํ์ฌ ๋๊ตฌ๋ ๋ชจ๋ธ์ ์ฌ์ฉํ ์ ์๋๋ก ํฉ๋๋ค:
```py
>>> trainer.push_to_hub()
```
## ์ถ๋ก ํ๊ธฐ[[inference]]
์ข์ต๋๋ค. ์ด์ ๋ฏธ์ธ ์กฐ์ ๋ ๋ชจ๋ธ์ ์ถ๋ก ํ๋ ๋ฐ ์ฌ์ฉํ ์ ์์ต๋๋ค.
์ถ๋ก ์ ์ฌ์ฉํ ์์์ ๋ถ๋ฌ์ค์ธ์:
```py
>>> sample_test_video = next(iter(test_dataset))
```
<div class="flex justify-center">
<img src="https://huggingface.co/datasets/huggingface/documentation-images/resolve/main/transformers/tasks/sample_gif_two.gif" alt="Teams playing basketball"/>
</div>
๋ฏธ์ธ ์กฐ์ ๋ ๋ชจ๋ธ์ ์ถ๋ก ์ ์ฌ์ฉํ๋ ๊ฐ์ฅ ๊ฐ๋จํ ๋ฐฉ๋ฒ์ [`pipeline`](https://huggingface.co/docs/transformers/main/en/main_classes/pipelines#transformers.VideoClassificationPipeline)์์ ๋ชจ๋ธ์ ์ฌ์ฉํ๋ ๊ฒ์
๋๋ค. ๋ชจ๋ธ๋ก ์์ ๋ถ๋ฅ๋ฅผ ํ๊ธฐ ์ํด `pipeline`์ ์ธ์คํด์คํํ๊ณ ์์์ ์ ๋ฌํ์ธ์:
```py
>>> from transformers import pipeline
>>> video_cls = pipeline(model="my_awesome_video_cls_model")
>>> video_cls("https://huggingface.co/datasets/sayakpaul/ucf101-subset/resolve/main/v_BasketballDunk_g14_c06.avi")
[{'score': 0.9272987842559814, 'label': 'BasketballDunk'},
{'score': 0.017777055501937866, 'label': 'BabyCrawling'},
{'score': 0.01663011871278286, 'label': 'BalanceBeam'},
{'score': 0.009560945443809032, 'label': 'BandMarching'},
{'score': 0.0068979403004050255, 'label': 'BaseballPitch'}]
```
๋ง์ฝ ์ํ๋ค๋ฉด ์๋์ผ๋ก `pipeline`์ ๊ฒฐ๊ณผ๋ฅผ ์ฌํํ ์ ์์ต๋๋ค:
```py
>>> def run_inference(model, video):
... # (num_frames, num_channels, height, width)
... perumuted_sample_test_video = video.permute(1, 0, 2, 3)
... inputs = {
... "pixel_values": perumuted_sample_test_video.unsqueeze(0),
... "labels": torch.tensor(
... [sample_test_video["label"]]
... ), # this can be skipped if you don't have labels available.
... }
... device = torch.device("cuda" if torch.cuda.is_available() else "cpu")
... inputs = {k: v.to(device) for k, v in inputs.items()}
... model = model.to(device)
... # forward pass
... with torch.no_grad():
... outputs = model(**inputs)
... logits = outputs.logits
... return logits
```
๋ชจ๋ธ์ ์
๋ ฅ๊ฐ์ ๋ฃ๊ณ `logits`์ ๋ฐํ๋ฐ์ผ์ธ์:
```
>>> logits = run_inference(trained_model, sample_test_video["video"])
```
`logits`์ ๋์ฝ๋ฉํ๋ฉด, ์ฐ๋ฆฌ๋ ๋ค์ ๊ฒฐ๊ณผ๋ฅผ ์ป์ ์ ์์ต๋๋ค:
```py
>>> predicted_class_idx = logits.argmax(-1).item()
>>> print("Predicted class:", model.config.id2label[predicted_class_idx])
# Predicted class: BasketballDunk
```
| 0 |
hf_public_repos/transformers/docs/source/ko | hf_public_repos/transformers/docs/source/ko/tasks/monocular_depth_estimation.md | <!--Copyright 2023 The HuggingFace Team. All rights reserved.
Licensed under the Apache License, Version 2.0 (the "License"); you may not use this file except in compliance with
the License. You may obtain a copy of the License at
http://www.apache.org/licenses/LICENSE-2.0
Unless required by applicable law or agreed to in writing, software distributed under the License is distributed on
an "AS IS" BASIS, WITHOUT WARRANTIES OR CONDITIONS OF ANY KIND, either express or implied. See the License for the
specific language governing permissions and limitations under the License.
โ ๏ธ Note that this file is in Markdown but contain specific syntax for our doc-builder (similar to MDX) that may not be
rendered properly in your Markdown viewer.
-->
# ๋จ์ผ ์์ ๊ธฐ๋ฐ ๊น์ด ์ถ์ [[depth-estimation-pipeline]]
๋จ์ผ ์์ ๊ธฐ๋ฐ ๊น์ด ์ถ์ ์ ํ ์ฅ๋ฉด์ ๋จ์ผ ์ด๋ฏธ์ง์์ ์ฅ๋ฉด์ ๊น์ด ์ ๋ณด๋ฅผ ์์ธกํ๋ ์ปดํจํฐ ๋น์ ์์
์
๋๋ค.
์ฆ, ๋จ์ผ ์นด๋ฉ๋ผ ์์ ์ ์ฅ๋ฉด์ ์๋ ๋ฌผ์ฒด์ ๊ฑฐ๋ฆฌ๋ฅผ ์์ธกํ๋ ๊ณผ์ ์
๋๋ค.
๋จ์ผ ์์ ๊ธฐ๋ฐ ๊น์ด ์ถ์ ์ 3D ์ฌ๊ตฌ์ฑ, ์ฆ๊ฐ ํ์ค, ์์จ ์ฃผํ, ๋ก๋ด ๊ณตํ ๋ฑ ๋ค์ํ ๋ถ์ผ์์ ์์ฉ๋ฉ๋๋ค.
์กฐ๋ช
์กฐ๊ฑด, ๊ฐ๋ ค์ง, ํ
์ค์ฒ์ ๊ฐ์ ์์์ ์ํฅ์ ๋ฐ์ ์ ์๋ ์ฅ๋ฉด ๋ด ๋ฌผ์ฒด์ ํด๋น ๊น์ด ์ ๋ณด ๊ฐ์ ๋ณต์กํ ๊ด๊ณ๋ฅผ ๋ชจ๋ธ์ด ์ดํดํด์ผ ํ๋ฏ๋ก ๊น๋ค๋ก์ด ์์
์
๋๋ค.
<Tip>
์ด ํํ ๋ฆฌ์ผ์์ ๋ค๋ฃจ๋ ์์
์ ๋ค์ ๋ชจ๋ธ ์ํคํ
์ฒ์์ ์ง์๋ฉ๋๋ค:
<!--This tip is automatically generated by `make fix-copies`, do not fill manually!-->
[DPT](../model_doc/dpt), [GLPN](../model_doc/glpn)
<!--End of the generated tip-->
</Tip>
์ด๋ฒ ๊ฐ์ด๋์์ ๋ฐฐ์ธ ๋ด์ฉ์ ๋ค์๊ณผ ๊ฐ์ต๋๋ค:
* ๊น์ด ์ถ์ ํ์ดํ๋ผ์ธ ๋ง๋ค๊ธฐ
* ์ง์ ๊น์ด ์ถ์ ์ถ๋ก ํ๊ธฐ
์์ํ๊ธฐ ์ ์, ํ์ํ ๋ชจ๋ ๋ผ์ด๋ธ๋ฌ๋ฆฌ๊ฐ ์ค์น๋์ด ์๋์ง ํ์ธํ์ธ์:
```bash
pip install -q transformers
```
## ๊น์ด ์ถ์ ํ์ดํ๋ผ์ธ[[depth-estimation-inference-by-hand]]
๊น์ด ์ถ์ ์ ์ถ๋ก ํ๋ ๊ฐ์ฅ ๊ฐ๋จํ ๋ฐฉ๋ฒ์ ํด๋น ๊ธฐ๋ฅ์ ์ ๊ณตํ๋ [`pipeline`]์ ์ฌ์ฉํ๋ ๊ฒ์
๋๋ค.
[Hugging Face Hub ์ฒดํฌํฌ์ธํธ](https://huggingface.co/models?pipeline_tag=depth-estimation&sort=downloads)์์ ํ์ดํ๋ผ์ธ์ ์ด๊ธฐํํฉ๋๋ค:
```py
>>> from transformers import pipeline
>>> checkpoint = "vinvino02/glpn-nyu"
>>> depth_estimator = pipeline("depth-estimation", model=checkpoint)
```
๋ค์์ผ๋ก, ๋ถ์ํ ์ด๋ฏธ์ง๋ฅผ ํ ์ฅ ์ ํํ์ธ์:
```py
>>> from PIL import Image
>>> import requests
>>> url = "https://unsplash.com/photos/HwBAsSbPBDU/download?ixid=MnwxMjA3fDB8MXxzZWFyY2h8MzR8fGNhciUyMGluJTIwdGhlJTIwc3RyZWV0fGVufDB8MHx8fDE2Nzg5MDEwODg&force=true&w=640"
>>> image = Image.open(requests.get(url, stream=True).raw)
>>> image
```
<div class="flex justify-center">
<img src="https://huggingface.co/datasets/huggingface/documentation-images/resolve/main/transformers/tasks/depth-estimation-example.jpg" alt="Photo of a busy street"/>
</div>
์ด๋ฏธ์ง๋ฅผ ํ์ดํ๋ผ์ธ์ผ๋ก ์ ๋ฌํฉ๋๋ค.
```py
>>> predictions = depth_estimator(image)
```
ํ์ดํ๋ผ์ธ์ ๋ ๊ฐ์ ํญ๋ชฉ์ ๊ฐ์ง๋ ๋์
๋๋ฆฌ๋ฅผ ๋ฐํํฉ๋๋ค.
์ฒซ ๋ฒ์งธ๋ `predicted_depth`๋ก ๊ฐ ํฝ์
์ ๊น์ด๋ฅผ ๋ฏธํฐ๋ก ํํํ ๊ฐ์ ๊ฐ์ง๋ ํ
์์
๋๋ค.
๋ ๋ฒ์งธ๋ `depth`๋ก ๊น์ด ์ถ์ ๊ฒฐ๊ณผ๋ฅผ ์๊ฐํํ๋ PIL ์ด๋ฏธ์ง์
๋๋ค.
์ด์ ์๊ฐํํ ๊ฒฐ๊ณผ๋ฅผ ์ดํด๋ณด๊ฒ ์ต๋๋ค:
```py
>>> predictions["depth"]
```
<div class="flex justify-center">
<img src="https://huggingface.co/datasets/huggingface/documentation-images/resolve/main/transformers/tasks/depth-visualization.png" alt="Depth estimation visualization"/>
</div>
## ์ง์ ๊น์ด ์ถ์ ์ถ๋ก ํ๊ธฐ[[depth-estimation-inference-by-hand]]
์ด์ ๊น์ด ์ถ์ ํ์ดํ๋ผ์ธ ์ฌ์ฉ๋ฒ์ ์ดํด๋ณด์์ผ๋ ๋์ผํ ๊ฒฐ๊ณผ๋ฅผ ๋ณต์ ํ๋ ๋ฐฉ๋ฒ์ ์ดํด๋ณด๊ฒ ์ต๋๋ค.
[Hugging Face Hub ์ฒดํฌํฌ์ธํธ](https://huggingface.co/models?pipeline_tag=depth-estimation&sort=downloads)์์ ๋ชจ๋ธ๊ณผ ๊ด๋ จ ํ๋ก์ธ์๋ฅผ ๊ฐ์ ธ์ค๋ ๊ฒ๋ถํฐ ์์ํฉ๋๋ค.
์ฌ๊ธฐ์ ์ด์ ์ ์ฌ์ฉํ ์ฒดํฌํฌ์ธํธ์ ๋์ผํ ๊ฒ์ ์ฌ์ฉํฉ๋๋ค:
```py
>>> from transformers import AutoImageProcessor, AutoModelForDepthEstimation
>>> checkpoint = "vinvino02/glpn-nyu"
>>> image_processor = AutoImageProcessor.from_pretrained(checkpoint)
>>> model = AutoModelForDepthEstimation.from_pretrained(checkpoint)
```
ํ์ํ ์ด๋ฏธ์ง ๋ณํ์ ์ฒ๋ฆฌํ๋ `image_processor`๋ฅผ ์ฌ์ฉํ์ฌ ๋ชจ๋ธ์ ๋ํ ์ด๋ฏธ์ง ์
๋ ฅ์ ์ค๋นํฉ๋๋ค.
`image_processor`๋ ํฌ๊ธฐ ์กฐ์ ๋ฐ ์ ๊ทํ ๋ฑ ํ์ํ ์ด๋ฏธ์ง ๋ณํ์ ์ฒ๋ฆฌํฉ๋๋ค:
```py
>>> pixel_values = image_processor(image, return_tensors="pt").pixel_values
```
์ค๋นํ ์
๋ ฅ์ ๋ชจ๋ธ๋ก ์ ๋ฌํฉ๋๋ค:
```py
>>> import torch
>>> with torch.no_grad():
... outputs = model(pixel_values)
... predicted_depth = outputs.predicted_depth
```
๊ฒฐ๊ณผ๋ฅผ ์๊ฐํํฉ๋๋ค:
```py
>>> import numpy as np
>>> # ์๋ณธ ์ฌ์ด์ฆ๋ก ๋ณต์
>>> prediction = torch.nn.functional.interpolate(
... predicted_depth.unsqueeze(1),
... size=image.size[::-1],
... mode="bicubic",
... align_corners=False,
... ).squeeze()
>>> output = prediction.numpy()
>>> formatted = (output * 255 / np.max(output)).astype("uint8")
>>> depth = Image.fromarray(formatted)
>>> depth
```
<div class="flex justify-center">
<img src="https://huggingface.co/datasets/huggingface/documentation-images/resolve/main/transformers/tasks/depth-visualization.png" alt="Depth estimation visualization"/>
</div>
| 0 |
hf_public_repos/transformers/docs/source/ko | hf_public_repos/transformers/docs/source/ko/tasks/multiple_choice.md | <!--Copyright 2022 The HuggingFace Team. All rights reserved.
Licensed under the Apache License, Version 2.0 (the "License"); you may not use this file except in compliance with
the License. You may obtain a copy of the License at
http://www.apache.org/licenses/LICENSE-2.0
Unless required by applicable law or agreed to in writing, software distributed under the License is distributed on
an "AS IS" BASIS, WITHOUT WARRANTIES OR CONDITIONS OF ANY KIND, either express or implied. See the License for the
specific language governing permissions and limitations under the License.
โ ๏ธ Note that this file is in Markdown but contain specific syntax for our doc-builder (similar to MDX) that may not be
rendered properly in your Markdown viewer.
-->
# ๊ฐ๊ด์ ๋ฌธ์ [[multiple-choice]]
[[open-in-colab]]
๊ฐ๊ด์ ๊ณผ์ ๋ ๋ฌธ๋งฅ๊ณผ ํจ๊ป ์ฌ๋ฌ ๊ฐ์ ํ๋ณด ๋ต๋ณ์ด ์ ๊ณต๋๊ณ ๋ชจ๋ธ์ด ์ ๋ต์ ์ ํํ๋๋ก ํ์ต๋๋ค๋ ์ ์ ์ ์ธํ๋ฉด ์ง์์๋ต๊ณผ ์ ์ฌํฉ๋๋ค.
์งํํ๋ ๋ฐฉ๋ฒ์ ์๋์ ๊ฐ์ต๋๋ค:
1. [SWAG](https://huggingface.co/datasets/swag) ๋ฐ์ดํฐ ์ธํธ์ 'regular' ๊ตฌ์ฑ์ผ๋ก [BERT](https://huggingface.co/bert-base-uncased)๋ฅผ ๋ฏธ์ธ ์กฐ์ ํ์ฌ ์ฌ๋ฌ ์ต์
๊ณผ ์ผ๋ถ ์ปจํ
์คํธ๊ฐ ์ฃผ์ด์ก์ ๋ ๊ฐ์ฅ ์ ํฉํ ๋ต์ ์ ํํฉ๋๋ค.
2. ์ถ๋ก ์ ๋ฏธ์ธ ์กฐ์ ๋ ๋ชจ๋ธ์ ์ฌ์ฉํฉ๋๋ค.
<Tip>
์ด ํํ ๋ฆฌ์ผ์์ ์ค๋ช
ํ๋ ์์
์ ๋ค์ ๋ชจ๋ธ ์ํคํ
์ฒ์์ ์ง์๋ฉ๋๋ค:
<!--This tip is automatically generated by `make fix-copies`, do not fill manually!-->
[ALBERT](../model_doc/albert), [BERT](../model_doc/bert), [BigBird](../model_doc/big_bird), [CamemBERT](../model_doc/camembert), [CANINE](../model_doc/canine), [ConvBERT](../model_doc/convbert), [Data2VecText](../model_doc/data2vec-text), [DeBERTa-v2](../model_doc/deberta-v2), [DistilBERT](../model_doc/distilbert), [ELECTRA](../model_doc/electra), [ERNIE](../model_doc/ernie), [ErnieM](../model_doc/ernie_m), [FlauBERT](../model_doc/flaubert), [FNet](../model_doc/fnet), [Funnel Transformer](../model_doc/funnel), [I-BERT](../model_doc/ibert), [Longformer](../model_doc/longformer), [LUKE](../model_doc/luke), [MEGA](../model_doc/mega), [Megatron-BERT](../model_doc/megatron-bert), [MobileBERT](../model_doc/mobilebert), [MPNet](../model_doc/mpnet), [Nezha](../model_doc/nezha), [Nystrรถmformer](../model_doc/nystromformer), [QDQBert](../model_doc/qdqbert), [RemBERT](../model_doc/rembert), [RoBERTa](../model_doc/roberta), [RoBERTa-PreLayerNorm](../model_doc/roberta-prelayernorm), [RoCBert](../model_doc/roc_bert), [RoFormer](../model_doc/roformer), [SqueezeBERT](../model_doc/squeezebert), [XLM](../model_doc/xlm), [XLM-RoBERTa](../model_doc/xlm-roberta), [XLM-RoBERTa-XL](../model_doc/xlm-roberta-xl), [XLNet](../model_doc/xlnet), [X-MOD](../model_doc/xmod), [YOSO](../model_doc/yoso)
<!--End of the generated tip-->
</Tip>
์์ํ๊ธฐ ์ ์ ํ์ํ ๋ผ์ด๋ธ๋ฌ๋ฆฌ๊ฐ ๋ชจ๋ ์ค์น๋์ด ์๋์ง ํ์ธํ์ธ์:
```bash
pip install transformers datasets evaluate
```
๋ชจ๋ธ์ ์
๋ก๋ํ๊ณ ์ปค๋ฎค๋ํฐ์ ๊ณต์ ํ ์ ์๋๋ก ํ๊น
ํ์ด์ค ๊ณ์ ์ ๋ก๊ทธ์ธํ๋ ๊ฒ์ด ์ข์ต๋๋ค. ๋ฉ์์ง๊ฐ ํ์๋๋ฉด ํ ํฐ์ ์
๋ ฅํ์ฌ ๋ก๊ทธ์ธํฉ๋๋ค:
```py
>>> from huggingface_hub import notebook_login
>>> notebook_login()
```
## SWAG ๋ฐ์ดํฐ ์ธํธ ๊ฐ์ ธ์ค๊ธฐ[[load-swag-dataset]]
๋จผ์ ๐ค Datasets ๋ผ์ด๋ธ๋ฌ๋ฆฌ์์ SWAG ๋ฐ์ดํฐ์
์ '์ผ๋ฐ' ๊ตฌ์ฑ์ ๊ฐ์ ธ์ต๋๋ค:
```py
>>> from datasets import load_dataset
>>> swag = load_dataset("swag", "regular")
```
์ด์ ๋ฐ์ดํฐ๋ฅผ ์ดํด๋ด
๋๋ค:
```py
>>> swag["train"][0]
{'ending0': 'passes by walking down the street playing their instruments.',
'ending1': 'has heard approaching them.',
'ending2': "arrives and they're outside dancing and asleep.",
'ending3': 'turns the lead singer watches the performance.',
'fold-ind': '3416',
'gold-source': 'gold',
'label': 0,
'sent1': 'Members of the procession walk down the street holding small horn brass instruments.',
'sent2': 'A drum line',
'startphrase': 'Members of the procession walk down the street holding small horn brass instruments. A drum line',
'video-id': 'anetv_jkn6uvmqwh4'}
```
์ฌ๊ธฐ์๋ ๋ง์ ํ๋๊ฐ ์๋ ๊ฒ์ฒ๋ผ ๋ณด์ด์ง๋ง ์ค์ ๋ก๋ ๋งค์ฐ ๊ฐ๋จํฉ๋๋ค:
- `sent1` ๋ฐ `sent2`: ์ด ํ๋๋ ๋ฌธ์ฅ์ด ์ด๋ป๊ฒ ์์๋๋์ง ๋ณด์ฌ์ฃผ๋ฉฐ, ์ด ๋ ํ๋๋ฅผ ํฉ์น๋ฉด `์์ ๊ตฌ์ (startphrase)` ํ๋๊ฐ ๋ฉ๋๋ค.
- `์ข
๋ฃ ๊ตฌ์ (ending)`: ๋ฌธ์ฅ์ด ์ด๋ป๊ฒ ๋๋ ์ ์๋์ง์ ๋ํ ๊ฐ๋ฅํ ์ข
๋ฃ ๊ตฌ์ ๋ฅผ ์ ์ํ์ง๋ง ๊ทธ ์ค ํ๋๋ง ์ ๋ต์
๋๋ค.
- `๋ ์ด๋ธ(label)`: ์ฌ๋ฐ๋ฅธ ๋ฌธ์ฅ ์ข
๋ฃ ๊ตฌ์ ์ ์๋ณํฉ๋๋ค.
## ์ ์ฒ๋ฆฌ[[preprocess]]
๋ค์ ๋จ๊ณ๋ ๋ฌธ์ฅ์ ์์๊ณผ ๋ค ๊ฐ์ง ๊ฐ๋ฅํ ๊ตฌ์ ์ ์ฒ๋ฆฌํ๊ธฐ ์ํด BERT ํ ํฌ๋์ด์ ๋ฅผ ๋ถ๋ฌ์ต๋๋ค:
```py
>>> from transformers import AutoTokenizer
>>> tokenizer = AutoTokenizer.from_pretrained("bert-base-uncased")
```
์์ฑํ๋ ค๋ ์ ์ฒ๋ฆฌ ํจ์๋ ๋ค์๊ณผ ๊ฐ์์ผ ํฉ๋๋ค:
1. `sent1` ํ๋๋ฅผ ๋ค ๊ฐ ๋ณต์ฌํ ๋ค์ ๊ฐ๊ฐ์ `sent2`์ ๊ฒฐํฉํ์ฌ ๋ฌธ์ฅ์ด ์์๋๋ ๋ฐฉ์์ ์ฌํํฉ๋๋ค.
2. `sent2`๋ฅผ ๋ค ๊ฐ์ง ๊ฐ๋ฅํ ๋ฌธ์ฅ ๊ตฌ์ ๊ฐ๊ฐ๊ณผ ๊ฒฐํฉํฉ๋๋ค.
3. ์ด ๋ ๋ชฉ๋ก์ ํ ํฐํํ ์ ์๋๋ก ํํํ(flatten)ํ๊ณ , ๊ฐ ์์ ์ ํด๋นํ๋ `input_ids`, `attention_mask` ๋ฐ `labels` ํ๋๋ฅผ ๊ฐ๋๋ก ๋ค์ฐจ์ํ(unflatten) ํฉ๋๋ค.
```py
>>> ending_names = ["ending0", "ending1", "ending2", "ending3"]
>>> def preprocess_function(examples):
... first_sentences = [[context] * 4 for context in examples["sent1"]]
... question_headers = examples["sent2"]
... second_sentences = [
... [f"{header} {examples[end][i]}" for end in ending_names] for i, header in enumerate(question_headers)
... ]
... first_sentences = sum(first_sentences, [])
... second_sentences = sum(second_sentences, [])
... tokenized_examples = tokenizer(first_sentences, second_sentences, truncation=True)
... return {k: [v[i : i + 4] for i in range(0, len(v), 4)] for k, v in tokenized_examples.items()}
```
์ ์ฒด ๋ฐ์ดํฐ ์งํฉ์ ์ ์ฒ๋ฆฌ ๊ธฐ๋ฅ์ ์ ์ฉํ๋ ค๋ฉด ๐ค Datasets [`~datasets.Dataset.map`] ๋ฉ์๋๋ฅผ ์ฌ์ฉํฉ๋๋ค. `batched=True`๋ฅผ ์ค์ ํ์ฌ ๋ฐ์ดํฐ ์งํฉ์ ์ฌ๋ฌ ์์๋ฅผ ํ ๋ฒ์ ์ฒ๋ฆฌํ๋ฉด `map` ํจ์์ ์๋๋ฅผ ๋์ผ ์ ์์ต๋๋ค:
```py
tokenized_swag = swag.map(preprocess_function, batched=True)
```
๐ค Transformers์๋ ๊ฐ๊ด์์ฉ ๋ฐ์ดํฐ ์ฝ๋ ์ดํฐ๊ฐ ์์ผ๋ฏ๋ก ์์ ๋ฐฐ์น๋ฅผ ๋ง๋ค๋ ค๋ฉด [`DataCollatorWithPadding`]์ ์กฐ์ ํด์ผ ํฉ๋๋ค. ๋ฐ์ดํฐ ์ ๋ ฌ ์ค์ ์ ์ฒด ๋ฐ์ดํฐ ์งํฉ์ ์ต๋ ๊ธธ์ด๋ก ํจ๋ฉํ๋ ๋์ ๋ฐฐ์น ์ค ๊ฐ์ฅ ๊ธด ๊ธธ์ด๋ก ๋ฌธ์ฅ์ *๋์ ํจ๋ฉ*ํ๋ ๊ฒ์ด ๋ ํจ์จ์ ์
๋๋ค.
`DataCollatorForMultipleChoice`๋ ๋ชจ๋ ๋ชจ๋ธ ์
๋ ฅ์ ํํํํ๊ณ ํจ๋ฉ์ ์ ์ฉํ๋ฉฐ ๊ทธ ๊ฒฐ๊ณผ๋ฅผ ๊ฒฐ๊ณผ๋ฅผ ๋ค์ฐจ์ํํฉ๋๋ค:
<frameworkcontent>
<pt>
```py
>>> from dataclasses import dataclass
>>> from transformers.tokenization_utils_base import PreTrainedTokenizerBase, PaddingStrategy
>>> from typing import Optional, Union
>>> import torch
>>> @dataclass
... class DataCollatorForMultipleChoice:
... """
... Data collator that will dynamically pad the inputs for multiple choice received.
... """
... tokenizer: PreTrainedTokenizerBase
... padding: Union[bool, str, PaddingStrategy] = True
... max_length: Optional[int] = None
... pad_to_multiple_of: Optional[int] = None
... def __call__(self, features):
... label_name = "label" if "label" in features[0].keys() else "labels"
... labels = [feature.pop(label_name) for feature in features]
... batch_size = len(features)
... num_choices = len(features[0]["input_ids"])
... flattened_features = [
... [{k: v[i] for k, v in feature.items()} for i in range(num_choices)] for feature in features
... ]
... flattened_features = sum(flattened_features, [])
... batch = self.tokenizer.pad(
... flattened_features,
... padding=self.padding,
... max_length=self.max_length,
... pad_to_multiple_of=self.pad_to_multiple_of,
... return_tensors="pt",
... )
... batch = {k: v.view(batch_size, num_choices, -1) for k, v in batch.items()}
... batch["labels"] = torch.tensor(labels, dtype=torch.int64)
... return batch
```
</pt>
<tf>
```py
>>> from dataclasses import dataclass
>>> from transformers.tokenization_utils_base import PreTrainedTokenizerBase, PaddingStrategy
>>> from typing import Optional, Union
>>> import tensorflow as tf
>>> @dataclass
... class DataCollatorForMultipleChoice:
... """
... Data collator that will dynamically pad the inputs for multiple choice received.
... """
... tokenizer: PreTrainedTokenizerBase
... padding: Union[bool, str, PaddingStrategy] = True
... max_length: Optional[int] = None
... pad_to_multiple_of: Optional[int] = None
... def __call__(self, features):
... label_name = "label" if "label" in features[0].keys() else "labels"
... labels = [feature.pop(label_name) for feature in features]
... batch_size = len(features)
... num_choices = len(features[0]["input_ids"])
... flattened_features = [
... [{k: v[i] for k, v in feature.items()} for i in range(num_choices)] for feature in features
... ]
... flattened_features = sum(flattened_features, [])
... batch = self.tokenizer.pad(
... flattened_features,
... padding=self.padding,
... max_length=self.max_length,
... pad_to_multiple_of=self.pad_to_multiple_of,
... return_tensors="tf",
... )
... batch = {k: tf.reshape(v, (batch_size, num_choices, -1)) for k, v in batch.items()}
... batch["labels"] = tf.convert_to_tensor(labels, dtype=tf.int64)
... return batch
```
</tf>
</frameworkcontent>
## ํ๊ฐ ํ๊ธฐ[[evaluate]]
ํ๋ จ ์ค์ ๋ฉํธ๋ฆญ์ ํฌํจํ๋ฉด ๋ชจ๋ธ์ ์ฑ๋ฅ์ ํ๊ฐํ๋ ๋ฐ ๋์์ด ๋๋ ๊ฒฝ์ฐ๊ฐ ๋ง์ต๋๋ค. ๐ค[Evaluate](https://huggingface.co/docs/evaluate/index) ๋ผ์ด๋ธ๋ฌ๋ฆฌ๋ฅผ ์ฌ์ฉํ์ฌ ํ๊ฐ ๋ฐฉ๋ฒ์ ๋น ๋ฅด๊ฒ ๊ฐ์ ธ์ฌ ์ ์์ต๋๋ค. ์ด ์์
์์๋ [accuracy](https://huggingface.co/spaces/evaluate-metric/accuracy) ์งํ๋ฅผ ๊ฐ์ ธ์ต๋๋ค(๐ค Evaluate [๋๋ฌ๋ณด๊ธฐ](https://huggingface.co/docs/evaluate/a_quick_tour)๋ฅผ ์ฐธ์กฐํ์ฌ ์งํ๋ฅผ ๊ฐ์ ธ์ค๊ณ ๊ณ์ฐํ๋ ๋ฐฉ๋ฒ์ ๋ํด ์์ธํ ์์๋ณด์ธ์):
```py
>>> import evaluate
>>> accuracy = evaluate.load("accuracy")
```
๊ทธ๋ฆฌ๊ณ ์์ธก๊ณผ ๋ ์ด๋ธ์ [`~evaluate.EvaluationModule.compute`]์ ์ ๋ฌํ์ฌ ์ ํ๋๋ฅผ ๊ณ์ฐํ๋ ํจ์๋ฅผ ๋ง๋ญ๋๋ค:
```py
>>> import numpy as np
>>> def compute_metrics(eval_pred):
... predictions, labels = eval_pred
... predictions = np.argmax(predictions, axis=1)
... return accuracy.compute(predictions=predictions, references=labels)
```
์ด์ `compute_metrics` ํจ์๋ฅผ ์ฌ์ฉํ ์ค๋น๊ฐ ๋์์ผ๋ฉฐ, ํ๋ จ์ ์ค์ ํ ๋ ์ด ํจ์๋ก ๋์๊ฐ๊ฒ ๋ฉ๋๋ค.
## ํ๋ จ ํ๊ธฐ[[train]]
<frameworkcontent>
<pt>
<Tip>
[`Trainer`]๋ก ๋ชจ๋ธ์ ๋ฏธ์ธ ์กฐ์ ํ๋ ๋ฐ ์ต์ํ์ง ์๋ค๋ฉด ๊ธฐ๋ณธ ํํ ๋ฆฌ์ผ [์ฌ๊ธฐ](../training#train-with-pytorch-trainer)๋ฅผ ์ดํด๋ณด์ธ์!
</Tip>
์ด์ ๋ชจ๋ธ ํ๋ จ์ ์์ํ ์ค๋น๊ฐ ๋์์ต๋๋ค! [`AutoModelForMultipleChoice`]๋ก BERT๋ฅผ ๋ก๋ํฉ๋๋ค:
```py
>>> from transformers import AutoModelForMultipleChoice, TrainingArguments, Trainer
>>> model = AutoModelForMultipleChoice.from_pretrained("bert-base-uncased")
```
์ด์ ์ธ ๋จ๊ณ๋ง ๋จ์์ต๋๋ค:
1. ํ๋ จ ํ์ดํผํ๋ผ๋ฏธํฐ๋ฅผ [`TrainingArguments`]์ ์ ์ํฉ๋๋ค. ์ ์ผํ ํ์ ๋งค๊ฐ๋ณ์๋ ๋ชจ๋ธ์ ์ ์ฅํ ์์น๋ฅผ ์ง์ ํ๋ `output_dir`์
๋๋ค. `push_to_hub=True`๋ฅผ ์ค์ ํ์ฌ ์ด ๋ชจ๋ธ์ ํ๋ธ์ ํธ์ํฉ๋๋ค(๋ชจ๋ธ์ ์
๋ก๋ํ๋ ค๋ฉด ํ๊น
ํ์ด์ค์ ๋ก๊ทธ์ธํด์ผ ํฉ๋๋ค). ๊ฐ ์ํญ์ด ๋๋ ๋๋ง๋ค [`Trainer`]๊ฐ ์ ํ๋๋ฅผ ํ๊ฐํ๊ณ ํ๋ จ ์ฒดํฌํฌ์ธํธ๋ฅผ ์ ์ฅํฉ๋๋ค.
2. ๋ชจ๋ธ, ๋ฐ์ดํฐ ์ธํธ, ํ ํฌ๋์ด์ , ๋ฐ์ดํฐ ์ฝ๋ ์ดํฐ, `compute_metrics` ํจ์์ ํจ๊ป ํ๋ จ ์ธ์๋ฅผ [`Trainer`]์ ์ ๋ฌํฉ๋๋ค.
3. [`~Trainer.train`]์ ์ฌ์ฉํ์ฌ ๋ชจ๋ธ์ ๋ฏธ์ธ ์กฐ์ ํฉ๋๋ค.
```py
>>> training_args = TrainingArguments(
... output_dir="my_awesome_swag_model",
... evaluation_strategy="epoch",
... save_strategy="epoch",
... load_best_model_at_end=True,
... learning_rate=5e-5,
... per_device_train_batch_size=16,
... per_device_eval_batch_size=16,
... num_train_epochs=3,
... weight_decay=0.01,
... push_to_hub=True,
... )
>>> trainer = Trainer(
... model=model,
... args=training_args,
... train_dataset=tokenized_swag["train"],
... eval_dataset=tokenized_swag["validation"],
... tokenizer=tokenizer,
... data_collator=DataCollatorForMultipleChoice(tokenizer=tokenizer),
... compute_metrics=compute_metrics,
... )
>>> trainer.train()
```
ํ๋ จ์ด ์๋ฃ๋๋ฉด ๋ชจ๋ ์ฌ๋์ด ๋ชจ๋ธ์ ์ฌ์ฉํ ์ ์๋๋ก [`~transformers.Trainer.push_to_hub`] ๋ฉ์๋๋ฅผ ์ฌ์ฉํ์ฌ ๋ชจ๋ธ์ ํ๋ธ์ ๊ณต์ ํ์ธ์:
```py
>>> trainer.push_to_hub()
```
</pt>
<tf>
<Tip>
Keras๋ก ๋ชจ๋ธ์ ๋ฏธ์ธ ์กฐ์ ํ๋ ๋ฐ ์ต์ํ์ง ์๋ค๋ฉด ๊ธฐ๋ณธ ํํ ๋ฆฌ์ผ [์ฌ๊ธฐ](../training#train-a-tensorflow-model-with-keras)๋ฅผ ์ดํด๋ณด์๊ธฐ ๋ฐ๋๋๋ค!
</Tip>
TensorFlow์์ ๋ชจ๋ธ์ ๋ฏธ์ธ ์กฐ์ ํ๋ ค๋ฉด ์ต์ ํ ํจ์, ํ์ต๋ฅ ์ค์ผ์ฅด ๋ฐ ๋ช ๊ฐ์ง ํ์ต ํ์ดํผํ๋ผ๋ฏธํฐ๋ฅผ ์ค์ ํ๋ ๊ฒ๋ถํฐ ์์ํ์ธ์:
```py
>>> from transformers import create_optimizer
>>> batch_size = 16
>>> num_train_epochs = 2
>>> total_train_steps = (len(tokenized_swag["train"]) // batch_size) * num_train_epochs
>>> optimizer, schedule = create_optimizer(init_lr=5e-5, num_warmup_steps=0, num_train_steps=total_train_steps)
```
๊ทธ๋ฆฌ๊ณ [`TFAutoModelForMultipleChoice`]๋ก BERT๋ฅผ ๊ฐ์ ธ์ฌ ์ ์์ต๋๋ค:
```py
>>> from transformers import TFAutoModelForMultipleChoice
>>> model = TFAutoModelForMultipleChoice.from_pretrained("bert-base-uncased")
```
[`~transformers.TFPreTrainedModel.prepare_tf_dataset`]์ ์ฌ์ฉํ์ฌ ๋ฐ์ดํฐ ์ธํธ๋ฅผ `tf.data.Dataset` ํ์์ผ๋ก ๋ณํํฉ๋๋ค:
```py
>>> data_collator = DataCollatorForMultipleChoice(tokenizer=tokenizer)
>>> tf_train_set = model.prepare_tf_dataset(
... tokenized_swag["train"],
... shuffle=True,
... batch_size=batch_size,
... collate_fn=data_collator,
... )
>>> tf_validation_set = model.prepare_tf_dataset(
... tokenized_swag["validation"],
... shuffle=False,
... batch_size=batch_size,
... collate_fn=data_collator,
... )
```
[`compile`](https://keras.io/api/models/model_training_apis/#compile-method)์ ์ฌ์ฉํ์ฌ ํ๋ จ ๋ชจ๋ธ์ ๊ตฌ์ฑํฉ๋๋ค:
```py
>>> model.compile(optimizer=optimizer)
```
ํ๋ จ์ ์์ํ๊ธฐ ์ ์ ์ค์ ํด์ผ ํ ๋ง์ง๋ง ๋ ๊ฐ์ง๋ ์์ธก์ ์ ํ๋๋ฅผ ๊ณ์ฐํ๊ณ ๋ชจ๋ธ์ ํ๋ธ๋ก ํธ์ํ๋ ๋ฐฉ๋ฒ์ ์ ๊ณตํ๋ ๊ฒ์
๋๋ค. ์ด ๋ ๊ฐ์ง ์์
์ ๋ชจ๋ [Keras ์ฝ๋ฐฑ](../main_classes/keras_callbacks)์ ์ฌ์ฉํ์ฌ ์ํํ ์ ์์ต๋๋ค.
`compute_metrics`ํจ์๋ฅผ [`~transformers.KerasMetricCallback`]์ ์ ๋ฌํ์ธ์:
```py
>>> from transformers.keras_callbacks import KerasMetricCallback
>>> metric_callback = KerasMetricCallback(metric_fn=compute_metrics, eval_dataset=tf_validation_set)
```
๋ชจ๋ธ๊ณผ ํ ํฌ๋์ด์ ๋ฅผ ์
๋ก๋ํ ์์น๋ฅผ [`~transformers.PushToHubCallback`]์์ ์ง์ ํ์ธ์:
```py
>>> from transformers.keras_callbacks import PushToHubCallback
>>> push_to_hub_callback = PushToHubCallback(
... output_dir="my_awesome_model",
... tokenizer=tokenizer,
... )
```
๊ทธ๋ฆฌ๊ณ ์ฝ๋ฐฑ์ ํจ๊ป ๋ฌถ์ต๋๋ค:
```py
>>> callbacks = [metric_callback, push_to_hub_callback]
```
์ด์ ๋ชจ๋ธ ํ๋ จ์ ์์ํฉ๋๋ค! ํ๋ จ ๋ฐ ๊ฒ์ฆ ๋ฐ์ดํฐ ์ธํธ, ์ํญ ์, ์ฝ๋ฐฑ์ ์ฌ์ฉํ์ฌ [`fit`](https://keras.io/api/models/model_training_apis/#fit-method)์ ํธ์ถํ๊ณ ๋ชจ๋ธ์ ๋ฏธ์ธ ์กฐ์ ํฉ๋๋ค:
```py
>>> model.fit(x=tf_train_set, validation_data=tf_validation_set, epochs=2, callbacks=callbacks)
```
ํ๋ จ์ด ์๋ฃ๋๋ฉด ๋ชจ๋ธ์ด ์๋์ผ๋ก ํ๋ธ์ ์
๋ก๋๋์ด ๋๊ตฌ๋ ์ฌ์ฉํ ์ ์์ต๋๋ค!
</tf>
</frameworkcontent>
<Tip>
๊ฐ๊ด์ ๋ชจ๋ธ์ ๋ฏธ์ธ ์กฐ์ ํ๋ ๋ฐฉ๋ฒ์ ๋ํ ๋ณด๋ค ์ฌ์ธต์ ์ธ ์๋ ์๋ ๋ฌธ์๋ฅผ ์ฐธ์กฐํ์ธ์.
[PyTorch notebook](https://colab.research.google.com/github/huggingface/notebooks/blob/main/examples/multiple_choice.ipynb)
๋๋ [TensorFlow notebook](https://colab.research.google.com/github/huggingface/notebooks/blob/main/examples/multiple_choice-tf.ipynb).
</Tip>
## ์ถ๋ก ํ๊ธฐ[[inference]]
์ด์ ๋ชจ๋ธ์ ๋ฏธ์ธ ์กฐ์ ํ์ผ๋ ์ถ๋ก ์ ์ฌ์ฉํ ์ ์์ต๋๋ค!
ํ
์คํธ์ ๋ ๊ฐ์ ํ๋ณด ๋ต์์ ์์ฑํฉ๋๋ค:
```py
>>> prompt = "France has a bread law, Le Dรฉcret Pain, with strict rules on what is allowed in a traditional baguette."
>>> candidate1 = "The law does not apply to croissants and brioche."
>>> candidate2 = "The law applies to baguettes."
```
<frameworkcontent>
<pt>
๊ฐ ํ๋กฌํํธ์ ํ๋ณด ๋ต๋ณ ์์ ํ ํฐํํ์ฌ PyTorch ํ
์๋ฅผ ๋ฐํํฉ๋๋ค. ๋ํ `labels`์ ์์ฑํด์ผ ํฉ๋๋ค:
```py
>>> from transformers import AutoTokenizer
>>> tokenizer = AutoTokenizer.from_pretrained("my_awesome_swag_model")
>>> inputs = tokenizer([[prompt, candidate1], [prompt, candidate2]], return_tensors="pt", padding=True)
>>> labels = torch.tensor(0).unsqueeze(0)
```
์
๋ ฅ๊ณผ ๋ ์ด๋ธ์ ๋ชจ๋ธ์ ์ ๋ฌํ๊ณ `logits`์ ๋ฐํํฉ๋๋ค:
```py
>>> from transformers import AutoModelForMultipleChoice
>>> model = AutoModelForMultipleChoice.from_pretrained("my_awesome_swag_model")
>>> outputs = model(**{k: v.unsqueeze(0) for k, v in inputs.items()}, labels=labels)
>>> logits = outputs.logits
```
๊ฐ์ฅ ๋์ ํ๋ฅ ์ ๊ฐ์ง ํด๋์ค๋ฅผ ๊ฐ์ ธ์ต๋๋ค:
```py
>>> predicted_class = logits.argmax().item()
>>> predicted_class
'0'
```
</pt>
<tf>
๊ฐ ํ๋กฌํํธ์ ํ๋ณด ๋ต์ ์์ ํ ํฐํํ์ฌ ํ
์ํ๋ก ํ
์๋ฅผ ๋ฐํํฉ๋๋ค:
```py
>>> from transformers import AutoTokenizer
>>> tokenizer = AutoTokenizer.from_pretrained("my_awesome_swag_model")
>>> inputs = tokenizer([[prompt, candidate1], [prompt, candidate2]], return_tensors="tf", padding=True)
```
๋ชจ๋ธ์ ์
๋ ฅ์ ์ ๋ฌํ๊ณ `logits`๋ฅผ ๋ฐํํฉ๋๋ค:
```py
>>> from transformers import TFAutoModelForMultipleChoice
>>> model = TFAutoModelForMultipleChoice.from_pretrained("my_awesome_swag_model")
>>> inputs = {k: tf.expand_dims(v, 0) for k, v in inputs.items()}
>>> outputs = model(inputs)
>>> logits = outputs.logits
```
๊ฐ์ฅ ๋์ ํ๋ฅ ์ ๊ฐ์ง ํด๋์ค๋ฅผ ๊ฐ์ ธ์ต๋๋ค:
```py
>>> predicted_class = int(tf.math.argmax(logits, axis=-1)[0])
>>> predicted_class
'0'
```
</tf>
</frameworkcontent>
| 0 |
hf_public_repos/transformers/docs/source/ko | hf_public_repos/transformers/docs/source/ko/tasks/image_captioning.md | <!--Copyright 2023 The HuggingFace Team. All rights reserved.
Licensed under the Apache License, Version 2.0 (the "License"); you may not use this file except in compliance with
the License. You may obtain a copy of the License at
http://www.apache.org/licenses/LICENSE-2.0
Unless required by applicable law or agreed to in writing, software distributed under the License is distributed on
an "AS IS" BASIS, WITHOUT WARRANTIES OR CONDITIONS OF ANY KIND, either express or implied. See the License for the
specific language governing permissions and limitations under the License.
โ ๏ธ Note that this file is in Markdown but contain specific syntax for our doc-builder (similar to MDX) that may not be
rendered properly in your Markdown viewer.
-->
# ์ด๋ฏธ์ง ์บก์
๋[[image-captioning]]
[[open-in-colab]]
์ด๋ฏธ์ง ์บก์
๋(Image captioning)์ ์ฃผ์ด์ง ์ด๋ฏธ์ง์ ๋ํ ์บก์
์ ์์ธกํ๋ ์์
์
๋๋ค.
์ด๋ฏธ์ง ์บก์
๋์ ์๊ฐ ์ฅ์ ์ธ์ด ๋ค์ํ ์ํฉ์ ํ์ํ๋ ๋ฐ ๋์์ ์ค ์ ์๋๋ก ์๊ฐ ์ฅ์ ์ธ์ ๋ณด์กฐํ๋ ๋ฑ ์ค์ํ์์ ํํ ํ์ฉ๋ฉ๋๋ค.
๋ฐ๋ผ์ ์ด๋ฏธ์ง ์บก์
๋์ ์ด๋ฏธ์ง๋ฅผ ์ค๋ช
ํจ์ผ๋ก์จ ์ฌ๋๋ค์ ์ฝํ
์ธ ์ ๊ทผ์ฑ์ ๊ฐ์ ํ๋ ๋ฐ ๋์์ด ๋ฉ๋๋ค.
์ด ๊ฐ์ด๋์์๋ ์๊ฐํ ๋ด์ฉ์ ์๋์ ๊ฐ์ต๋๋ค:
* ์ด๋ฏธ์ง ์บก์
๋ ๋ชจ๋ธ์ ํ์ธํ๋ํฉ๋๋ค.
* ํ์ธํ๋๋ ๋ชจ๋ธ์ ์ถ๋ก ์ ์ฌ์ฉํฉ๋๋ค.
์์ํ๊ธฐ ์ ์ ํ์ํ ๋ชจ๋ ๋ผ์ด๋ธ๋ฌ๋ฆฌ๊ฐ ์ค์น๋์ด ์๋์ง ํ์ธํ์ธ์:
```bash
pip install transformers datasets evaluate -q
pip install jiwer -q
```
Hugging Face ๊ณ์ ์ ๋ก๊ทธ์ธํ๋ฉด ๋ชจ๋ธ์ ์
๋ก๋ํ๊ณ ์ปค๋ฎค๋ํฐ์ ๊ณต์ ํ ์ ์์ต๋๋ค.
ํ ํฐ์ ์
๋ ฅํ์ฌ ๋ก๊ทธ์ธํ์ธ์.
```python
from huggingface_hub import notebook_login
notebook_login()
```
## ํฌ์ผ๋ชฌ BLIP ์บก์
๋ฐ์ดํฐ์ธํธ ๊ฐ์ ธ์ค๊ธฐ[[load-the-pokmon-blip-captions-dataset]]
{์ด๋ฏธ์ง-์บก์
} ์์ผ๋ก ๊ตฌ์ฑ๋ ๋ฐ์ดํฐ์ธํธ๋ฅผ ๊ฐ์ ธ์ค๋ ค๋ฉด ๐ค Dataset ๋ผ์ด๋ธ๋ฌ๋ฆฌ๋ฅผ ์ฌ์ฉํฉ๋๋ค.
PyTorch์์ ์์ ๋ง์ ์ด๋ฏธ์ง ์บก์
๋ฐ์ดํฐ์ธํธ๋ฅผ ๋ง๋ค๋ ค๋ฉด [์ด ๋
ธํธ๋ถ](https://github.com/NielsRogge/Transformers-Tutorials/blob/master/GIT/Fine_tune_GIT_on_an_image_captioning_dataset.ipynb)์ ์ฐธ์กฐํ์ธ์.
```python
from datasets import load_dataset
ds = load_dataset("lambdalabs/pokemon-blip-captions")
ds
```
```bash
DatasetDict({
train: Dataset({
features: ['image', 'text'],
num_rows: 833
})
})
```
์ด ๋ฐ์ดํฐ์ธํธ๋ `image`์ `text`๋ผ๋ ๋ ํน์ฑ์ ๊ฐ์ง๊ณ ์์ต๋๋ค.
<Tip>
๋ง์ ์ด๋ฏธ์ง ์บก์
๋ฐ์ดํฐ์ธํธ์๋ ์ด๋ฏธ์ง๋น ์ฌ๋ฌ ๊ฐ์ ์บก์
์ด ํฌํจ๋์ด ์์ต๋๋ค.
์ด๋ฌํ ๊ฒฝ์ฐ, ์ผ๋ฐ์ ์ผ๋ก ํ์ต ์ค์ ์ฌ์ฉ ๊ฐ๋ฅํ ์บก์
์ค์์ ๋ฌด์์๋ก ์ํ์ ์ถ์ถํฉ๋๋ค.
</Tip>
[~datasets.Dataset.train_test_split] ๋ฉ์๋๋ฅผ ์ฌ์ฉํ์ฌ ๋ฐ์ดํฐ์ธํธ์ ํ์ต ๋ถํ ์ ํ์ต ๋ฐ ํ
์คํธ ์ธํธ๋ก ๋๋๋๋ค:
```python
ds = ds["train"].train_test_split(test_size=0.1)
train_ds = ds["train"]
test_ds = ds["test"]
```
ํ์ต ์ธํธ์ ์ํ ๋ช ๊ฐ๋ฅผ ์๊ฐํํด ๋ด
์๋ค.
Let's visualize a couple of samples from the training set.
```python
from textwrap import wrap
import matplotlib.pyplot as plt
import numpy as np
def plot_images(images, captions):
plt.figure(figsize=(20, 20))
for i in range(len(images)):
ax = plt.subplot(1, len(images), i + 1)
caption = captions[i]
caption = "\n".join(wrap(caption, 12))
plt.title(caption)
plt.imshow(images[i])
plt.axis("off")
sample_images_to_visualize = [np.array(train_ds[i]["image"]) for i in range(5)]
sample_captions = [train_ds[i]["text"] for i in range(5)]
plot_images(sample_images_to_visualize, sample_captions)
```
<div class="flex justify-center">
<img src="https://huggingface.co/datasets/huggingface/documentation-images/resolve/main/transformers/tasks/sample_training_images_image_cap.png" alt="Sample training images"/>
</div>
## ๋ฐ์ดํฐ์ธํธ ์ ์ฒ๋ฆฌ[[preprocess-the-dataset]]
๋ฐ์ดํฐ์ธํธ์๋ ์ด๋ฏธ์ง์ ํ
์คํธ๋ผ๋ ๋ ๊ฐ์ง ์์์ด ์๊ธฐ ๋๋ฌธ์, ์ ์ฒ๋ฆฌ ํ์ดํ๋ผ์ธ์์ ์ด๋ฏธ์ง์ ์บก์
์ ๋ชจ๋ ์ ์ฒ๋ฆฌํฉ๋๋ค.
์ ์ฒ๋ฆฌ ์์
์ ์ํด, ํ์ธํ๋ํ๋ ค๋ ๋ชจ๋ธ์ ์ฐ๊ฒฐ๋ ํ๋ก์ธ์ ํด๋์ค๋ฅผ ๊ฐ์ ธ์ต๋๋ค.
```python
from transformers import AutoProcessor
checkpoint = "microsoft/git-base"
processor = AutoProcessor.from_pretrained(checkpoint)
```
ํ๋ก์ธ์๋ ๋ด๋ถ์ ์ผ๋ก ํฌ๊ธฐ ์กฐ์ ๋ฐ ํฝ์
ํฌ๊ธฐ ์กฐ์ ์ ํฌํจํ ์ด๋ฏธ์ง ์ ์ฒ๋ฆฌ๋ฅผ ์ํํ๊ณ ์บก์
์ ํ ํฐํํฉ๋๋ค.
```python
def transforms(example_batch):
images = [x for x in example_batch["image"]]
captions = [x for x in example_batch["text"]]
inputs = processor(images=images, text=captions, padding="max_length")
inputs.update({"labels": inputs["input_ids"]})
return inputs
train_ds.set_transform(transforms)
test_ds.set_transform(transforms)
```
๋ฐ์ดํฐ์ธํธ๊ฐ ์ค๋น๋์์ผ๋ ์ด์ ํ์ธํ๋์ ์ํด ๋ชจ๋ธ์ ์ค์ ํ ์ ์์ต๋๋ค.
## ๊ธฐ๋ณธ ๋ชจ๋ธ ๊ฐ์ ธ์ค๊ธฐ[[load-a-base-model]]
["microsoft/git-base"](https://huggingface.co/microsoft/git-base)๋ฅผ [`AutoModelForCausalLM`](https://huggingface.co/docs/transformers/model_doc/auto#transformers.AutoModelForCausalLM) ๊ฐ์ฒด๋ก ๊ฐ์ ธ์ต๋๋ค.
```python
from transformers import AutoModelForCausalLM
model = AutoModelForCausalLM.from_pretrained(checkpoint)
```
## ํ๊ฐ[[evaluate]]
์ด๋ฏธ์ง ์บก์
๋ชจ๋ธ์ ์ผ๋ฐ์ ์ผ๋ก [Rouge ์ ์](https://huggingface.co/spaces/evaluate-metric/rouge) ๋๋ [๋จ์ด ์ค๋ฅ์จ(Word Error Rate)](https://huggingface.co/spaces/evaluate-metric/wer)๋ก ํ๊ฐํฉ๋๋ค.
์ด ๊ฐ์ด๋์์๋ ๋จ์ด ์ค๋ฅ์จ(WER)์ ์ฌ์ฉํฉ๋๋ค.
์ด๋ฅผ ์ํด ๐ค Evaluate ๋ผ์ด๋ธ๋ฌ๋ฆฌ๋ฅผ ์ฌ์ฉํฉ๋๋ค.
WER์ ์ ์ฌ์ ์ ํ ์ฌํญ ๋ฐ ๊ธฐํ ๋ฌธ์ ์ ์ [์ด ๊ฐ์ด๋](https://huggingface.co/spaces/evaluate-metric/wer)๋ฅผ ์ฐธ์กฐํ์ธ์.
```python
from evaluate import load
import torch
wer = load("wer")
def compute_metrics(eval_pred):
logits, labels = eval_pred
predicted = logits.argmax(-1)
decoded_labels = processor.batch_decode(labels, skip_special_tokens=True)
decoded_predictions = processor.batch_decode(predicted, skip_special_tokens=True)
wer_score = wer.compute(predictions=decoded_predictions, references=decoded_labels)
return {"wer_score": wer_score}
```
## ํ์ต![[train!]]
์ด์ ๋ชจ๋ธ ํ์ธํ๋์ ์์ํ ์ค๋น๊ฐ ๋์์ต๋๋ค. ์ด๋ฅผ ์ํด ๐ค [`Trainer`]๋ฅผ ์ฌ์ฉํฉ๋๋ค.
๋จผ์ , [`TrainingArguments`]๋ฅผ ์ฌ์ฉํ์ฌ ํ์ต ์ธ์๋ฅผ ์ ์ํฉ๋๋ค.
```python
from transformers import TrainingArguments, Trainer
model_name = checkpoint.split("/")[1]
training_args = TrainingArguments(
output_dir=f"{model_name}-pokemon",
learning_rate=5e-5,
num_train_epochs=50,
fp16=True,
per_device_train_batch_size=32,
per_device_eval_batch_size=32,
gradient_accumulation_steps=2,
save_total_limit=3,
evaluation_strategy="steps",
eval_steps=50,
save_strategy="steps",
save_steps=50,
logging_steps=50,
remove_unused_columns=False,
push_to_hub=True,
label_names=["labels"],
load_best_model_at_end=True,
)
```
ํ์ต ์ธ์๋ฅผ ๋ฐ์ดํฐ์ธํธ, ๋ชจ๋ธ๊ณผ ํจ๊ป ๐ค Trainer์ ์ ๋ฌํฉ๋๋ค.
```python
trainer = Trainer(
model=model,
args=training_args,
train_dataset=train_ds,
eval_dataset=test_ds,
compute_metrics=compute_metrics,
)
```
ํ์ต์ ์์ํ๋ ค๋ฉด [`Trainer`] ๊ฐ์ฒด์์ [`~Trainer.train`]์ ํธ์ถํ๊ธฐ๋ง ํ๋ฉด ๋ฉ๋๋ค.
```python
trainer.train()
```
ํ์ต์ด ์งํ๋๋ฉด์ ํ์ต ์์ค์ด ์ํํ๊ฒ ๊ฐ์ํ๋ ๊ฒ์ ๋ณผ ์ ์์ต๋๋ค.
ํ์ต์ด ์๋ฃ๋๋ฉด ๋ชจ๋ ์ฌ๋์ด ๋ชจ๋ธ์ ์ฌ์ฉํ ์ ์๋๋ก [`~Trainer.push_to_hub`] ๋ฉ์๋๋ฅผ ์ฌ์ฉํ์ฌ ๋ชจ๋ธ์ ํ๋ธ์ ๊ณต์ ํ์ธ์:
```python
trainer.push_to_hub()
```
## ์ถ๋ก [[inference]]
`test_ds`์์ ์ํ ์ด๋ฏธ์ง๋ฅผ ๊ฐ์ ธ์ ๋ชจ๋ธ์ ํ
์คํธํฉ๋๋ค.
```python
from PIL import Image
import requests
url = "https://huggingface.co/datasets/sayakpaul/sample-datasets/resolve/main/pokemon.png"
image = Image.open(requests.get(url, stream=True).raw)
image
```
<div class="flex justify-center">
<img src="https://huggingface.co/datasets/huggingface/documentation-images/resolve/main/transformers/tasks/test_image_image_cap.png" alt="Test image"/>
</div>
๋ชจ๋ธ์ ์ฌ์ฉํ ์ด๋ฏธ์ง๋ฅผ ์ค๋นํฉ๋๋ค.
```python
device = "cuda" if torch.cuda.is_available() else "cpu"
inputs = processor(images=image, return_tensors="pt").to(device)
pixel_values = inputs.pixel_values
```
[`generate`]๋ฅผ ํธ์ถํ๊ณ ์์ธก์ ๋์ฝ๋ฉํฉ๋๋ค.
```python
generated_ids = model.generate(pixel_values=pixel_values, max_length=50)
generated_caption = processor.batch_decode(generated_ids, skip_special_tokens=True)[0]
print(generated_caption)
```
```bash
a drawing of a pink and blue pokemon
```
ํ์ธํ๋๋ ๋ชจ๋ธ์ด ๊ฝค ๊ด์ฐฎ์ ์บก์
์ ์์ฑํ ๊ฒ ๊ฐ์ต๋๋ค!
| 0 |
hf_public_repos/transformers/docs/source/ko | hf_public_repos/transformers/docs/source/ko/tasks/sequence_classification.md | <!--Copyright 2022 The HuggingFace Team. All rights reserved.
Licensed under the Apache License, Version 2.0 (the "License"); you may not use this file except in compliance with
the License. You may obtain a copy of the License at
http://www.apache.org/licenses/LICENSE-2.0
Unless required by applicable law or agreed to in writing, software distributed under the License is distributed on
an "AS IS" BASIS, WITHOUT WARRANTIES OR CONDITIONS OF ANY KIND, either express or implied. See the License for the
specific language governing permissions and limitations under the License.
โ ๏ธ Note that this file is in Markdown but contain specific syntax for our doc-builder (similar to MDX) that may not be
rendered properly in your Markdown viewer.
-->
# ํ
์คํธ ๋ถ๋ฅ[[text-classification]]
[[open-in-colab]]
<Youtube id="leNG9fN9FQU"/>
ํ
์คํธ ๋ถ๋ฅ๋ ์์ฐ์ด ์ฒ๋ฆฌ์ ์ผ์ข
์ผ๋ก, ํ
์คํธ์ ๋ ์ด๋ธ ๋๋ ํด๋์ค๋ฅผ ์ง์ ํ๋ ์์
์
๋๋ค. ๋ง์ ๋๊ธฐ์
์ด ๋ค์ํ ์ค์ฉ์ ์ธ ์์ฉ ๋ถ์ผ์์ ํ
์คํธ ๋ถ๋ฅ๋ฅผ ์ด์ํ๊ณ ์์ต๋๋ค. ๊ฐ์ฅ ์ธ๊ธฐ ์๋ ํ
์คํธ ๋ถ๋ฅ ํํ ์ค ํ๋๋ ๊ฐ์ฑ ๋ถ์์ผ๋ก, ํ
์คํธ ์ํ์ค์ ๐ ๊ธ์ , ๐ ๋ถ์ ๋๋ ๐ ์ค๋ฆฝ๊ณผ ๊ฐ์ ๋ ์ด๋ธ์ ์ง์ ํฉ๋๋ค.
์ด ๊ฐ์ด๋์์ ํ์ตํ ๋ด์ฉ์:
1. [IMDb](https://huggingface.co/datasets/imdb) ๋ฐ์ดํฐ์
์์ [DistilBERT](https://huggingface.co/distilbert-base-uncased)๋ฅผ ํ์ธ ํ๋ํ์ฌ ์ํ ๋ฆฌ๋ทฐ๊ฐ ๊ธ์ ์ ์ธ์ง ๋ถ์ ์ ์ธ์ง ํ๋จํฉ๋๋ค.
2. ์ถ๋ก ์ ์ํด ํ์ธ ํ๋ ๋ชจ๋ธ์ ์ฌ์ฉํฉ๋๋ค.
<Tip>
์ด ํํ ๋ฆฌ์ผ์์ ์ค๋ช
ํ๋ ์์
์ ๋ค์ ๋ชจ๋ธ ์ํคํ
์ฒ์ ์ํด ์ง์๋ฉ๋๋ค:
<!--This tip is automatically generated by `make fix-copies`, do not fill manually!-->
[ALBERT](../model_doc/albert), [BART](../model_doc/bart), [BERT](../model_doc/bert), [BigBird](../model_doc/big_bird), [BigBird-Pegasus](../model_doc/bigbird_pegasus), [BLOOM](../model_doc/bloom), [CamemBERT](../model_doc/camembert), [CANINE](../model_doc/canine), [ConvBERT](../model_doc/convbert), [CTRL](../model_doc/ctrl), [Data2VecText](../model_doc/data2vec-text), [DeBERTa](../model_doc/deberta), [DeBERTa-v2](../model_doc/deberta-v2), [DistilBERT](../model_doc/distilbert), [ELECTRA](../model_doc/electra), [ERNIE](../model_doc/ernie), [ErnieM](../model_doc/ernie_m), [ESM](../model_doc/esm), [FlauBERT](../model_doc/flaubert), [FNet](../model_doc/fnet), [Funnel Transformer](../model_doc/funnel), [GPT-Sw3](../model_doc/gpt-sw3), [OpenAI GPT-2](../model_doc/gpt2), [GPT Neo](../model_doc/gpt_neo), [GPT-J](../model_doc/gptj), [I-BERT](../model_doc/ibert), [LayoutLM](../model_doc/layoutlm), [LayoutLMv2](../model_doc/layoutlmv2), [LayoutLMv3](../model_doc/layoutlmv3), [LED](../model_doc/led), [LiLT](../model_doc/lilt), [LLaMA](../model_doc/llama), [Longformer](../model_doc/longformer), [LUKE](../model_doc/luke), [MarkupLM](../model_doc/markuplm), [mBART](../model_doc/mbart), [MEGA](../model_doc/mega), [Megatron-BERT](../model_doc/megatron-bert), [MobileBERT](../model_doc/mobilebert), [MPNet](../model_doc/mpnet), [MVP](../model_doc/mvp), [Nezha](../model_doc/nezha), [Nystrรถmformer](../model_doc/nystromformer), [OpenAI GPT](../model_doc/openai-gpt), [OPT](../model_doc/opt), [Perceiver](../model_doc/perceiver), [PLBart](../model_doc/plbart), [QDQBert](../model_doc/qdqbert), [Reformer](../model_doc/reformer), [RemBERT](../model_doc/rembert), [RoBERTa](../model_doc/roberta), [RoBERTa-PreLayerNorm](../model_doc/roberta-prelayernorm), [RoCBert](../model_doc/roc_bert), [RoFormer](../model_doc/roformer), [SqueezeBERT](../model_doc/squeezebert), [TAPAS](../model_doc/tapas), [Transformer-XL](../model_doc/transfo-xl), [XLM](../model_doc/xlm), [XLM-RoBERTa](../model_doc/xlm-roberta), [XLM-RoBERTa-XL](../model_doc/xlm-roberta-xl), [XLNet](../model_doc/xlnet), [X-MOD](../model_doc/xmod), [YOSO](../model_doc/yoso)
<!--End of the generated tip-->
</Tip>
์์ํ๊ธฐ ์ ์, ํ์ํ ๋ชจ๋ ๋ผ์ด๋ธ๋ฌ๋ฆฌ๊ฐ ์ค์น๋์ด ์๋์ง ํ์ธํ์ธ์:
```bash
pip install transformers datasets evaluate
```
Hugging Face ๊ณ์ ์ ๋ก๊ทธ์ธํ์ฌ ๋ชจ๋ธ์ ์
๋ก๋ํ๊ณ ์ปค๋ฎค๋ํฐ์ ๊ณต์ ํ๋ ๊ฒ์ ๊ถ์ฅํฉ๋๋ค. ๋ฉ์์ง๊ฐ ํ์๋๋ฉด, ํ ํฐ์ ์
๋ ฅํ์ฌ ๋ก๊ทธ์ธํ์ธ์:
```py
>>> from huggingface_hub import notebook_login
>>> notebook_login()
```
## IMDb ๋ฐ์ดํฐ์
๊ฐ์ ธ์ค๊ธฐ[[load-imdb-dataset]]
๋จผ์ ๐ค Datasets ๋ผ์ด๋ธ๋ฌ๋ฆฌ์์ IMDb ๋ฐ์ดํฐ์
์ ๊ฐ์ ธ์ต๋๋ค:
```py
>>> from datasets import load_dataset
>>> imdb = load_dataset("imdb")
```
๊ทธ๋ฐ ๋ค์ ์์๋ฅผ ์ดํด๋ด
์๋ค:
```py
>>> imdb["test"][0]
{
"label": 0,
"text": "I love sci-fi and am willing to put up with a lot. Sci-fi movies/TV are usually underfunded, under-appreciated and misunderstood. I tried to like this, I really did, but it is to good TV sci-fi as Babylon 5 is to Star Trek (the original). Silly prosthetics, cheap cardboard sets, stilted dialogues, CG that doesn't match the background, and painfully one-dimensional characters cannot be overcome with a 'sci-fi' setting. (I'm sure there are those of you out there who think Babylon 5 is good sci-fi TV. It's not. It's clichรฉd and uninspiring.) While US viewers might like emotion and character development, sci-fi is a genre that does not take itself seriously (cf. Star Trek). It may treat important issues, yet not as a serious philosophy. It's really difficult to care about the characters here as they are not simply foolish, just missing a spark of life. Their actions and reactions are wooden and predictable, often painful to watch. The makers of Earth KNOW it's rubbish as they have to always say \"Gene Roddenberry's Earth...\" otherwise people would not continue watching. Roddenberry's ashes must be turning in their orbit as this dull, cheap, poorly edited (watching it without advert breaks really brings this home) trudging Trabant of a show lumbers into space. Spoiler. So, kill off a main character. And then bring him back as another actor. Jeeez! Dallas all over again.",
}
```
์ด ๋ฐ์ดํฐ์
์๋ ๋ ๊ฐ์ง ํ๋๊ฐ ์์ต๋๋ค:
- `text`: ์ํ ๋ฆฌ๋ทฐ ํ
์คํธ
- `label`: `0`์ ๋ถ์ ์ ์ธ ๋ฆฌ๋ทฐ, `1`์ ๊ธ์ ์ ์ธ ๋ฆฌ๋ทฐ๋ฅผ ๋ํ๋
๋๋ค.
## ์ ์ฒ๋ฆฌ[[preprocess]]
๋ค์ ๋จ๊ณ๋ DistilBERT ํ ํฌ๋์ด์ ๋ฅผ ๊ฐ์ ธ์์ `text` ํ๋๋ฅผ ์ ์ฒ๋ฆฌํ๋ ๊ฒ์
๋๋ค:
```py
>>> from transformers import AutoTokenizer
>>> tokenizer = AutoTokenizer.from_pretrained("distilbert-base-uncased")
```
`text`๋ฅผ ํ ํฐํํ๊ณ ์ํ์ค๊ฐ DistilBERT์ ์ต๋ ์
๋ ฅ ๊ธธ์ด๋ณด๋ค ๊ธธ์ง ์๋๋ก ์๋ฅด๊ธฐ ์ํ ์ ์ฒ๋ฆฌ ํจ์๋ฅผ ์์ฑํ์ธ์:
```py
>>> def preprocess_function(examples):
... return tokenizer(examples["text"], truncation=True)
```
์ ์ฒด ๋ฐ์ดํฐ์
์ ์ ์ฒ๋ฆฌ ํจ์๋ฅผ ์ ์ฉํ๋ ค๋ฉด, ๐ค Datasets [`~datasets.Dataset.map`] ํจ์๋ฅผ ์ฌ์ฉํ์ธ์. ๋ฐ์ดํฐ์
์ ์ฌ๋ฌ ์์๋ฅผ ํ ๋ฒ์ ์ฒ๋ฆฌํ๊ธฐ ์ํด `batched=True`๋ก ์ค์ ํจ์ผ๋ก์จ ๋ฐ์ดํฐ์
`map`๋ฅผ ๋ ๋น ๋ฅด๊ฒ ์ฒ๋ฆฌํ ์ ์์ต๋๋ค:
```py
tokenized_imdb = imdb.map(preprocess_function, batched=True)
```
์ด์ [`DataCollatorWithPadding`]๋ฅผ ์ฌ์ฉํ์ฌ ์์ ๋ฐฐ์น๋ฅผ ๋ง๋ค์ด๋ด
์๋ค. ๋ฐ์ดํฐ์
์ ์ฒด๋ฅผ ์ต๋ ๊ธธ์ด๋ก ํจ๋ฉํ๋ ๋์ , *๋์ ํจ๋ฉ*์ ์ฌ์ฉํ์ฌ ๋ฐฐ์น์์ ๊ฐ์ฅ ๊ธด ๊ธธ์ด์ ๋ง๊ฒ ๋ฌธ์ฅ์ ํจ๋ฉํ๋ ๊ฒ์ด ํจ์จ์ ์
๋๋ค.
<frameworkcontent>
<pt>
```py
>>> from transformers import DataCollatorWithPadding
>>> data_collator = DataCollatorWithPadding(tokenizer=tokenizer)
```
</pt>
<tf>
```py
>>> from transformers import DataCollatorWithPadding
>>> data_collator = DataCollatorWithPadding(tokenizer=tokenizer, return_tensors="tf")
```
</tf>
</frameworkcontent>
## ํ๊ฐํ๊ธฐ[[evaluate]]
ํ๋ จ ์ค ๋ชจ๋ธ์ ์ฑ๋ฅ์ ํ๊ฐํ๊ธฐ ์ํด ๋ฉํธ๋ฆญ์ ํฌํจํ๋ ๊ฒ์ด ์ ์ฉํฉ๋๋ค. ๐ค [Evaluate](https://huggingface.co/docs/evaluate/index) ๋ผ์ด๋ธ๋ฌ๋ฆฌ๋ฅผ ์ฌ์ฉํ์ฌ ๋น ๋ฅด๊ฒ ํ๊ฐ ๋ฐฉ๋ฒ์ ๋ก๋ํ ์ ์์ต๋๋ค. ์ด ์์
์์๋ [accuracy](https://huggingface.co/spaces/evaluate-metric/accuracy) ๋ฉํธ๋ฆญ์ ๊ฐ์ ธ์ต๋๋ค. (๋ฉํธ๋ฆญ์ ๊ฐ์ ธ์ค๊ณ ๊ณ์ฐํ๋ ๋ฐฉ๋ฒ์ ๋ํด์๋ ๐ค Evaluate [quick tour](https://huggingface.co/docs/evaluate/a_quick_tour)๋ฅผ ์ฐธ์กฐํ์ธ์):
```py
>>> import evaluate
>>> accuracy = evaluate.load("accuracy")
```
๊ทธ๋ฐ ๋ค์ `compute_metrics` ํจ์๋ฅผ ๋ง๋ค์ด์ ์์ธก๊ณผ ๋ ์ด๋ธ์ ๊ณ์ฐํ์ฌ ์ ํ๋๋ฅผ ๊ณ์ฐํ๋๋ก [`~evaluate.EvaluationModule.compute`]๋ฅผ ํธ์ถํฉ๋๋ค:
```py
>>> import numpy as np
>>> def compute_metrics(eval_pred):
... predictions, labels = eval_pred
... predictions = np.argmax(predictions, axis=1)
... return accuracy.compute(predictions=predictions, references=labels)
```
์ด์ `compute_metrics` ํจ์๋ ์ค๋น๋์๊ณ , ํ๋ จ ๊ณผ์ ์ ์ค์ ํ ๋ ๋ค์ ์ดํด๋ณผ ์์ ์
๋๋ค.
## ํ๋ จ[[train]]
๋ชจ๋ธ์ ํ๋ จํ๊ธฐ ์ ์, `id2label`์ `label2id`๋ฅผ ์ฌ์ฉํ์ฌ ์์๋๋ id์ ๋ ์ด๋ธ์ ๋งต์ ์์ฑํ์ธ์:
```py
>>> id2label = {0: "NEGATIVE", 1: "POSITIVE"}
>>> label2id = {"NEGATIVE": 0, "POSITIVE": 1}
```
<frameworkcontent>
<pt>
<Tip>
[`Trainer`]๋ฅผ ์ฌ์ฉํ์ฌ ๋ชจ๋ธ์ ํ์ธ ํ๋ํ๋ ๋ฐฉ๋ฒ์ ์ต์ํ์ง ์์ ๊ฒฝ์ฐ, [์ฌ๊ธฐ](../training#train-with-pytorch-trainer)์ ๊ธฐ๋ณธ ํํ ๋ฆฌ์ผ์ ํ์ธํ์ธ์!
</Tip>
์ด์ ๋ชจ๋ธ์ ํ๋ จ์ํฌ ์ค๋น๊ฐ ๋์์ต๋๋ค! [`AutoModelForSequenceClassification`]๋ก DistilBERT๋ฅผ ๊ฐ์ณ์ค๊ณ ์์๋๋ ๋ ์ด๋ธ ์์ ๋ ์ด๋ธ ๋งคํ์ ์ง์ ํ์ธ์:
```py
>>> from transformers import AutoModelForSequenceClassification, TrainingArguments, Trainer
>>> model = AutoModelForSequenceClassification.from_pretrained(
... "distilbert-base-uncased", num_labels=2, id2label=id2label, label2id=label2id
... )
```
์ด์ ์ธ ๋จ๊ณ๋ง ๊ฑฐ์น๋ฉด ๋์
๋๋ค:
1. [`TrainingArguments`]์์ ํ์ดํผํ๋ผ๋ฏธํฐ๋ฅผ ์ ์ํ์ธ์. `output_dir`๋ ๋ชจ๋ธ์ ์ ์ฅํ ์์น๋ฅผ ์ง์ ํ๋ ์ ์ผํ ํ๋ผ๋ฏธํฐ์
๋๋ค. ์ด ๋ชจ๋ธ์ Hub์ ์
๋ก๋ํ๊ธฐ ์ํด `push_to_hub=True`๋ฅผ ์ค์ ํฉ๋๋ค. (๋ชจ๋ธ์ ์
๋ก๋ํ๊ธฐ ์ํด Hugging Face์ ๋ก๊ทธ์ธํด์ผํฉ๋๋ค.) ๊ฐ ์ํญ์ด ๋๋ ๋๋ง๋ค, [`Trainer`]๋ ์ ํ๋๋ฅผ ํ๊ฐํ๊ณ ํ๋ จ ์ฒดํฌํฌ์ธํธ๋ฅผ ์ ์ฅํฉ๋๋ค.
2. [`Trainer`]์ ํ๋ จ ์ธ์์ ๋ชจ๋ธ, ๋ฐ์ดํฐ์
, ํ ํฌ๋์ด์ , ๋ฐ์ดํฐ ์์ง๊ธฐ ๋ฐ `compute_metrics` ํจ์๋ฅผ ์ ๋ฌํ์ธ์.
3. [`~Trainer.train`]๋ฅผ ํธ์ถํ์ฌ ๋ชจ๋ธ์ ํ์ธ ํ๋ํ์ธ์.
```py
>>> training_args = TrainingArguments(
... output_dir="my_awesome_model",
... learning_rate=2e-5,
... per_device_train_batch_size=16,
... per_device_eval_batch_size=16,
... num_train_epochs=2,
... weight_decay=0.01,
... evaluation_strategy="epoch",
... save_strategy="epoch",
... load_best_model_at_end=True,
... push_to_hub=True,
... )
>>> trainer = Trainer(
... model=model,
... args=training_args,
... train_dataset=tokenized_imdb["train"],
... eval_dataset=tokenized_imdb["test"],
... tokenizer=tokenizer,
... data_collator=data_collator,
... compute_metrics=compute_metrics,
... )
>>> trainer.train()
```
<Tip>
[`Trainer`]๋ `tokenizer`๋ฅผ ์ ๋ฌํ๋ฉด ๊ธฐ๋ณธ์ ์ผ๋ก ๋์ ๋งคํ์ ์ ์ฉํฉ๋๋ค. ์ด ๊ฒฝ์ฐ, ๋ช
์์ ์ผ๋ก ๋ฐ์ดํฐ ์์ง๊ธฐ๋ฅผ ์ง์ ํ ํ์๊ฐ ์์ต๋๋ค.
</Tip>
ํ๋ จ์ด ์๋ฃ๋๋ฉด, [`~transformers.Trainer.push_to_hub`] ๋ฉ์๋๋ฅผ ์ฌ์ฉํ์ฌ ๋ชจ๋ธ์ Hub์ ๊ณต์ ํ ์ ์์ต๋๋ค.
```py
>>> trainer.push_to_hub()
```
</pt>
<tf>
<Tip>
Keras๋ฅผ ์ฌ์ฉํ์ฌ ๋ชจ๋ธ์ ํ์ธ ํ๋ํ๋ ๋ฐฉ๋ฒ์ ์ต์ํ์ง ์์ ๊ฒฝ์ฐ, [์ฌ๊ธฐ](../training#train-a-tensorflow-model-with-keras)์ ๊ธฐ๋ณธ ํํ ๋ฆฌ์ผ์ ํ์ธํ์ธ์!
</Tip>
TensorFlow์์ ๋ชจ๋ธ์ ํ์ธ ํ๋ํ๋ ค๋ฉด, ๋จผ์ ์ตํฐ๋ง์ด์ ํจ์์ ํ์ต๋ฅ ์ค์ผ์ฅด, ๊ทธ๋ฆฌ๊ณ ์ผ๋ถ ํ๋ จ ํ์ดํผํ๋ผ๋ฏธํฐ๋ฅผ ์ค์ ํด์ผ ํฉ๋๋ค:
```py
>>> from transformers import create_optimizer
>>> import tensorflow as tf
>>> batch_size = 16
>>> num_epochs = 5
>>> batches_per_epoch = len(tokenized_imdb["train"]) // batch_size
>>> total_train_steps = int(batches_per_epoch * num_epochs)
>>> optimizer, schedule = create_optimizer(init_lr=2e-5, num_warmup_steps=0, num_train_steps=total_train_steps)
```
๊ทธ๋ฐ ๋ค์ [`TFAutoModelForSequenceClassification`]์ ์ฌ์ฉํ์ฌ DistilBERT๋ฅผ ๋ก๋ํ๊ณ , ์์๋๋ ๋ ์ด๋ธ ์์ ๋ ์ด๋ธ ๋งคํ์ ๋ก๋ํ ์ ์์ต๋๋ค:
```py
>>> from transformers import TFAutoModelForSequenceClassification
>>> model = TFAutoModelForSequenceClassification.from_pretrained(
... "distilbert-base-uncased", num_labels=2, id2label=id2label, label2id=label2id
... )
```
[`~transformers.TFPreTrainedModel.prepare_tf_dataset`]์ ์ฌ์ฉํ์ฌ ๋ฐ์ดํฐ์
์ `tf.data.Dataset` ํ์์ผ๋ก ๋ณํํฉ๋๋ค:
```py
>>> tf_train_set = model.prepare_tf_dataset(
... tokenized_imdb["train"],
... shuffle=True,
... batch_size=16,
... collate_fn=data_collator,
... )
>>> tf_validation_set = model.prepare_tf_dataset(
... tokenized_imdb["test"],
... shuffle=False,
... batch_size=16,
... collate_fn=data_collator,
... )
```
[`compile`](https://keras.io/api/models/model_training_apis/#compile-method)๋ฅผ ์ฌ์ฉํ์ฌ ํ๋ จํ ๋ชจ๋ธ์ ๊ตฌ์ฑํฉ๋๋ค:
```py
>>> import tensorflow as tf
>>> model.compile(optimizer=optimizer)
```
ํ๋ จ์ ์์ํ๊ธฐ ์ ์ ์ค์ ํด์ผํ ๋ง์ง๋ง ๋ ๊ฐ์ง๋ ์์ธก์์ ์ ํ๋๋ฅผ ๊ณ์ฐํ๊ณ , ๋ชจ๋ธ์ Hub์ ์
๋ก๋ํ ๋ฐฉ๋ฒ์ ์ ๊ณตํ๋ ๊ฒ์
๋๋ค. ๋ชจ๋ [Keras callbacks](../main_classes/keras_callbacks)๋ฅผ ์ฌ์ฉํ์ฌ ์ํ๋ฉ๋๋ค.
[`~transformers.KerasMetricCallback`]์ `compute_metrics`๋ฅผ ์ ๋ฌํ์ฌ ์ ํ๋๋ฅผ ๋์
๋๋ค.
```py
>>> from transformers.keras_callbacks import KerasMetricCallback
>>> metric_callback = KerasMetricCallback(metric_fn=compute_metrics, eval_dataset=tf_validation_set)
```
[`~transformers.PushToHubCallback`]์์ ๋ชจ๋ธ๊ณผ ํ ํฌ๋์ด์ ๋ฅผ ์
๋ก๋ํ ์์น๋ฅผ ์ง์ ํฉ๋๋ค:
```py
>>> from transformers.keras_callbacks import PushToHubCallback
>>> push_to_hub_callback = PushToHubCallback(
... output_dir="my_awesome_model",
... tokenizer=tokenizer,
... )
```
๊ทธ๋ฐ ๋ค์ ์ฝ๋ฐฑ์ ํจ๊ป ๋ฌถ์ต๋๋ค:
```py
>>> callbacks = [metric_callback, push_to_hub_callback]
```
๋๋์ด, ๋ชจ๋ธ ํ๋ จ์ ์์ํ ์ค๋น๊ฐ ๋์์ต๋๋ค! [`fit`](https://keras.io/api/models/model_training_apis/#fit-method)์ ํ๋ จ ๋ฐ์ดํฐ์
, ๊ฒ์ฆ ๋ฐ์ดํฐ์
, ์ํญ์ ์ ๋ฐ ์ฝ๋ฐฑ์ ์ ๋ฌํ์ฌ ํ์ธ ํ๋ํฉ๋๋ค:
```py
>>> model.fit(x=tf_train_set, validation_data=tf_validation_set, epochs=3, callbacks=callbacks)
```
ํ๋ จ์ด ์๋ฃ๋๋ฉด, ๋ชจ๋ธ์ด ์๋์ผ๋ก Hub์ ์
๋ก๋๋์ด ๋ชจ๋ ์ฌ๋์ด ์ฌ์ฉํ ์ ์์ต๋๋ค!
</tf>
</frameworkcontent>
<Tip>
ํ
์คํธ ๋ถ๋ฅ๋ฅผ ์ํ ๋ชจ๋ธ์ ํ์ธ ํ๋ํ๋ ์์ธํ ์์ ๋ ๋ค์ [PyTorch notebook](https://colab.research.google.com/github/huggingface/notebooks/blob/main/examples/text_classification.ipynb) ๋๋ [TensorFlow notebook](https://colab.research.google.com/github/huggingface/notebooks/blob/main/examples/text_classification-tf.ipynb)๋ฅผ ์ฐธ์กฐํ์ธ์.
</Tip>
## ์ถ๋ก [[inference]]
์ข์์, ์ด์ ๋ชจ๋ธ์ ํ์ธ ํ๋ํ์ผ๋ ์ถ๋ก ์ ์ฌ์ฉํ ์ ์์ต๋๋ค!
์ถ๋ก ์ ์ํํ๊ณ ์ ํ๋ ํ
์คํธ๋ฅผ ๊ฐ์ ธ์๋ด
์๋ค:
```py
>>> text = "This was a masterpiece. Not completely faithful to the books, but enthralling from beginning to end. Might be my favorite of the three."
```
ํ์ธ ํ๋๋ ๋ชจ๋ธ๋ก ์ถ๋ก ์ ์๋ํ๋ ๊ฐ์ฅ ๊ฐ๋จํ ๋ฐฉ๋ฒ์ [`pipeline`]๋ฅผ ์ฌ์ฉํ๋ ๊ฒ์
๋๋ค. ๋ชจ๋ธ๋ก ๊ฐ์ ๋ถ์์ ์ํ `pipeline`์ ์ธ์คํด์คํํ๊ณ , ํ
์คํธ๋ฅผ ์ ๋ฌํด๋ณด์ธ์:
```py
>>> from transformers import pipeline
>>> classifier = pipeline("sentiment-analysis", model="stevhliu/my_awesome_model")
>>> classifier(text)
[{'label': 'POSITIVE', 'score': 0.9994940757751465}]
```
์ํ๋ค๋ฉด, `pipeline`์ ๊ฒฐ๊ณผ๋ฅผ ์๋์ผ๋ก ๋ณต์ ํ ์๋ ์์ต๋๋ค.
<frameworkcontent>
<pt>
ํ
์คํธ๋ฅผ ํ ํฐํํ๊ณ PyTorch ํ
์๋ฅผ ๋ฐํํฉ๋๋ค.
```py
>>> from transformers import AutoTokenizer
>>> tokenizer = AutoTokenizer.from_pretrained("stevhliu/my_awesome_model")
>>> inputs = tokenizer(text, return_tensors="pt")
```
์
๋ ฅ์ ๋ชจ๋ธ์ ์ ๋ฌํ๊ณ `logits`์ ๋ฐํํฉ๋๋ค:
```py
>>> from transformers import AutoModelForSequenceClassification
>>> model = AutoModelForSequenceClassification.from_pretrained("stevhliu/my_awesome_model")
>>> with torch.no_grad():
... logits = model(**inputs).logits
```
๊ฐ์ฅ ๋์ ํ๋ฅ ์ ๊ฐ์ง ํด๋์ค๋ฅผ ๋ชจ๋ธ์ `id2label` ๋งคํ์ ์ฌ์ฉํ์ฌ ํ
์คํธ ๋ ์ด๋ธ๋ก ๋ณํํฉ๋๋ค:
```py
>>> predicted_class_id = logits.argmax().item()
>>> model.config.id2label[predicted_class_id]
'POSITIVE'
```
</pt>
<tf>
ํ
์คํธ๋ฅผ ํ ํฐํํ๊ณ TensorFlow ํ
์๋ฅผ ๋ฐํํฉ๋๋ค:
```py
>>> from transformers import AutoTokenizer
>>> tokenizer = AutoTokenizer.from_pretrained("stevhliu/my_awesome_model")
>>> inputs = tokenizer(text, return_tensors="tf")
```
์
๋ ฅ๊ฐ์ ๋ชจ๋ธ์ ์ ๋ฌํ๊ณ `logits`์ ๋ฐํํฉ๋๋ค:
```py
>>> from transformers import TFAutoModelForSequenceClassification
>>> model = TFAutoModelForSequenceClassification.from_pretrained("stevhliu/my_awesome_model")
>>> logits = model(**inputs).logits
```
๊ฐ์ฅ ๋์ ํ๋ฅ ์ ๊ฐ์ง ํด๋์ค๋ฅผ ๋ชจ๋ธ์ `id2label` ๋งคํ์ ์ฌ์ฉํ์ฌ ํ
์คํธ ๋ ์ด๋ธ๋ก ๋ณํํฉ๋๋ค:
```py
>>> predicted_class_id = int(tf.math.argmax(logits, axis=-1)[0])
>>> model.config.id2label[predicted_class_id]
'POSITIVE'
```
</tf>
</frameworkcontent>
| 0 |
hf_public_repos/transformers/docs/source/ko | hf_public_repos/transformers/docs/source/ko/tasks/masked_language_modeling.md | <!--Copyright 2023 The HuggingFace Team. All rights reserved.
Licensed under the Apache License, Version 2.0 (the "License"); you may not use this file except in compliance with
the License. You may obtain a copy of the License at
http://www.apache.org/licenses/LICENSE-2.0
Unless required by applicable law or agreed to in writing, software distributed under the License is distributed on
an "AS IS" BASIS, WITHOUT WARRANTIES OR CONDITIONS OF ANY KIND, either express or implied. See the License for the
specific language governing permissions and limitations under the License.
โ ๏ธ Note that this file is in Markdown but contain specific syntax for our doc-builder (similar to MDX) that may not be
rendered properly in your Markdown viewer.
-->
# ๋ง์คํน๋ ์ธ์ด ๋ชจ๋ธ๋ง(Masked language modeling)[[masked-language-modeling]]
[[open-in-colab]]
<Youtube id="mqElG5QJWUg"/>
๋ง์คํน๋ ์ธ์ด ๋ชจ๋ธ๋ง์ ์ํ์ค์์ ๋ง์คํน๋ ํ ํฐ์ ์์ธกํ๋ฉฐ, ๋ชจ๋ธ์ ์๋ฐฉํฅ์ผ๋ก ํ ํฐ์ ์ก์ธ์คํ ์ ์์ต๋๋ค.
์ฆ, ๋ชจ๋ธ์ ํ ํฐ์ ์ผ์ชฝ๊ณผ ์ค๋ฅธ์ชฝ ์์ชฝ์์ ์ ๊ทผํ ์ ์์ต๋๋ค.
๋ง์คํน๋ ์ธ์ด ๋ชจ๋ธ๋ง์ ์ ์ฒด ์ํ์ค์ ๋ํ ๋ฌธ๋งฅ์ ์ดํด๊ฐ ํ์ํ ์์
์ ์ ํฉํ๋ฉฐ, BERT๊ฐ ๊ทธ ์์ ํด๋นํฉ๋๋ค.
์ด๋ฒ ๊ฐ์ด๋์์ ๋ค๋ฃฐ ๋ด์ฉ์ ๋ค์๊ณผ ๊ฐ์ต๋๋ค:
1. [ELI5](https://huggingface.co/datasets/eli5) ๋ฐ์ดํฐ ์ธํธ์์ [r/askscience](https://www.reddit.com/r/askscience/) ๋ถ๋ถ์ ์ฌ์ฉํด [DistilRoBERTa](https://huggingface.co/distilroberta-base) ๋ชจ๋ธ์ ๋ฏธ์ธ ์กฐ์ ํฉ๋๋ค.
2. ์ถ๋ก ์์ ์ง์ ๋ฏธ์ธ ์กฐ์ ํ ๋ชจ๋ธ์ ์ฌ์ฉํฉ๋๋ค.
<Tip>
์ด๋ฒ ๊ฐ์ด๋์์์ฒ๋ผ ๋ค๋ฅธ ์ํคํ
์ฒ๋ฅผ ๋ฏธ์ธ ์กฐ์ ํด ๋ง์คํน๋ ์ธ์ด ๋ชจ๋ธ๋ง์ ํ ์ ์์ต๋๋ค.
๋ค์ ์ํคํ
์ณ ์ค ํ๋๋ฅผ ์ ํํ์ธ์:
<!--This tip is automatically generated by `make fix-copies`, do not fill manually!-->
[ALBERT](../model_doc/albert), [BART](../model_doc/bart), [BERT](../model_doc/bert), [BigBird](../model_doc/big_bird), [CamemBERT](../model_doc/camembert), [ConvBERT](../model_doc/convbert), [Data2VecText](../model_doc/data2vec-text), [DeBERTa](../model_doc/deberta), [DeBERTa-v2](../model_doc/deberta-v2), [DistilBERT](../model_doc/distilbert), [ELECTRA](../model_doc/electra), [ERNIE](../model_doc/ernie), [ESM](../model_doc/esm), [FlauBERT](../model_doc/flaubert), [FNet](../model_doc/fnet), [Funnel Transformer](../model_doc/funnel), [I-BERT](../model_doc/ibert), [LayoutLM](../model_doc/layoutlm), [Longformer](../model_doc/longformer), [LUKE](../model_doc/luke), [mBART](../model_doc/mbart), [MEGA](../model_doc/mega), [Megatron-BERT](../model_doc/megatron-bert), [MobileBERT](../model_doc/mobilebert), [MPNet](../model_doc/mpnet), [MVP](../model_doc/mvp), [Nezha](../model_doc/nezha), [Nystrรถmformer](../model_doc/nystromformer), [Perceiver](../model_doc/perceiver), [QDQBert](../model_doc/qdqbert), [Reformer](../model_doc/reformer), [RemBERT](../model_doc/rembert), [RoBERTa](../model_doc/roberta), [RoBERTa-PreLayerNorm](../model_doc/roberta-prelayernorm), [RoCBert](../model_doc/roc_bert), [RoFormer](../model_doc/roformer), [SqueezeBERT](../model_doc/squeezebert), [TAPAS](../model_doc/tapas), [Wav2Vec2](../model_doc/wav2vec2), [XLM](../model_doc/xlm), [XLM-RoBERTa](../model_doc/xlm-roberta), [XLM-RoBERTa-XL](../model_doc/xlm-roberta-xl), [X-MOD](../model_doc/xmod), [YOSO](../model_doc/yoso)
<!--End of the generated tip-->
</Tip>
์์ํ๊ธฐ ์ ์ ํ์ํ ๋ผ์ด๋ธ๋ฌ๋ฆฌ๊ฐ ๋ชจ๋ ์ค์น๋์ด ์๋์ง ํ์ธํ์ธ์:
```bash
pip install transformers datasets evaluate
```
Hugging Face ๊ณ์ ์ ๋ก๊ทธ์ธํ์ฌ ๋ชจ๋ธ์ ์
๋ก๋ํ๊ณ ์ปค๋ฎค๋ํฐ์์ ๊ณต์ ๋ฅผ ๊ถ์ฅํฉ๋๋ค. ๋ฉ์์ง๊ฐ ํ์๋๋ฉด(When prompted) ํ ํฐ์ ์
๋ ฅํ์ฌ ๋ก๊ทธ์ธํฉ๋๋ค:
```py
>>> from huggingface_hub import notebook_login
>>> notebook_login()
```
## ELI5 ๋ฐ์ดํฐ ์ธํธ ๊ฐ์ ธ์ค๊ธฐ[[load-eli5-dataset]]
๋จผ์ ๐ค Datasets ๋ผ์ด๋ธ๋ฌ๋ฆฌ์์ ELI5 ๋ฐ์ดํฐ ์ธํธ์ r/askscience ์ค ์ผ๋ถ๋ง ๊ฐ์ ธ์ต๋๋ค.
์ด๋ ๊ฒ ํ๋ฉด ์ ์ฒด ๋ฐ์ดํฐ ์ธํธ ํ์ต์ ๋ ๋ง์ ์๊ฐ์ ํ ์ ํ๊ธฐ ์ ์ ๋ชจ๋ ๊ฒ์ด ์๋ํ๋์ง ์คํํ๊ณ ํ์ธํ ์ ์์ต๋๋ค.
```py
>>> from datasets import load_dataset
>>> eli5 = load_dataset("eli5", split="train_asks[:5000]")
```
๋ฐ์ดํฐ ์ธํธ์ `train_asks`๋ฅผ [`~datasets.Dataset.train_test_split`] ๋ฉ์๋๋ฅผ ์ฌ์ฉํด ํ๋ จ ๋ฐ์ดํฐ์ ํ
์คํธ ๋ฐ์ดํฐ๋ก ๋ถํ ํฉ๋๋ค:
```py
>>> eli5 = eli5.train_test_split(test_size=0.2)
```
๊ทธ๋ฆฌ๊ณ ์๋ ์์๋ฅผ ์ดํด๋ณด์ธ์:
```py
>>> eli5["train"][0]
{'answers': {'a_id': ['c3d1aib', 'c3d4lya'],
'score': [6, 3],
'text': ["The velocity needed to remain in orbit is equal to the square root of Newton's constant times the mass of earth divided by the distance from the center of the earth. I don't know the altitude of that specific mission, but they're usually around 300 km. That means he's going 7-8 km/s.\n\nIn space there are no other forces acting on either the shuttle or the guy, so they stay in the same position relative to each other. If he were to become unable to return to the ship, he would presumably run out of oxygen, or slowly fall into the atmosphere and burn up.",
"Hope you don't mind me asking another question, but why aren't there any stars visible in this photo?"]},
'answers_urls': {'url': []},
'document': '',
'q_id': 'nyxfp',
'selftext': '_URL_0_\n\nThis was on the front page earlier and I have a few questions about it. Is it possible to calculate how fast the astronaut would be orbiting the earth? Also how does he stay close to the shuttle so that he can return safely, i.e is he orbiting at the same speed and can therefore stay next to it? And finally if his propulsion system failed, would he eventually re-enter the atmosphere and presumably die?',
'selftext_urls': {'url': ['http://apod.nasa.gov/apod/image/1201/freeflyer_nasa_3000.jpg']},
'subreddit': 'askscience',
'title': 'Few questions about this space walk photograph.',
'title_urls': {'url': []}}
```
๋ง์ ๋ณด์ผ ์ ์์ง๋ง ์ค์ ๋ก๋ `text` ํ๋์๋ง ์ง์คํ๋ฉด ๋ฉ๋๋ค.
์ธ์ด ๋ชจ๋ธ๋ง ์์
์ ๋ฉ์ง ์ ์ (๋น์ง๋ ํ์ต์ผ๋ก) *๋ค์ ๋จ์ด๊ฐ ๋ ์ด๋ธ*์ด๊ธฐ ๋๋ฌธ์ ๋ ์ด๋ธ์ด ๋ฐ๋ก ํ์ํ์ง ์์ต๋๋ค.
## ์ ์ฒ๋ฆฌ[[preprocess]]
<Youtube id="8PmhEIXhBvI"/>
๋ง์คํน๋ ์ธ์ด ๋ชจ๋ธ๋ง์ ์ํด, ๋ค์ ๋จ๊ณ๋ก DistilRoBERTa ํ ํฌ๋์ด์ ๋ฅผ ๊ฐ์ ธ์์ `text` ํ์ ํ๋๋ฅผ ์ฒ๋ฆฌํฉ๋๋ค:
```py
>>> from transformers import AutoTokenizer
>>> tokenizer = AutoTokenizer.from_pretrained("distilroberta-base")
```
์์ ์์ ์์์ ๋ง์ฐฌ๊ฐ์ง๋ก, `text` ํ๋๋ `answers` ์์ ์ค์ฒฉ๋์ด ์์ต๋๋ค.
๋ฐ๋ผ์ ์ค์ฒฉ๋ ๊ตฌ์กฐ์์ [`flatten`](https://huggingface.co/docs/datasets/process.html#flatten) ๋ฉ์๋๋ฅผ ์ฌ์ฉํ์ฌ `text` ํ์ ํ๋๋ฅผ ์ถ์ถํฉ๋๋ค:
```py
>>> eli5 = eli5.flatten()
>>> eli5["train"][0]
{'answers.a_id': ['c3d1aib', 'c3d4lya'],
'answers.score': [6, 3],
'answers.text': ["The velocity needed to remain in orbit is equal to the square root of Newton's constant times the mass of earth divided by the distance from the center of the earth. I don't know the altitude of that specific mission, but they're usually around 300 km. That means he's going 7-8 km/s.\n\nIn space there are no other forces acting on either the shuttle or the guy, so they stay in the same position relative to each other. If he were to become unable to return to the ship, he would presumably run out of oxygen, or slowly fall into the atmosphere and burn up.",
"Hope you don't mind me asking another question, but why aren't there any stars visible in this photo?"],
'answers_urls.url': [],
'document': '',
'q_id': 'nyxfp',
'selftext': '_URL_0_\n\nThis was on the front page earlier and I have a few questions about it. Is it possible to calculate how fast the astronaut would be orbiting the earth? Also how does he stay close to the shuttle so that he can return safely, i.e is he orbiting at the same speed and can therefore stay next to it? And finally if his propulsion system failed, would he eventually re-enter the atmosphere and presumably die?',
'selftext_urls.url': ['http://apod.nasa.gov/apod/image/1201/freeflyer_nasa_3000.jpg'],
'subreddit': 'askscience',
'title': 'Few questions about this space walk photograph.',
'title_urls.url': []}
```
์ด์ ๊ฐ ํ์ ํ๋๋ `answers` ์ ๋์ฌ(prefix)๋ก ํ์๋ ๋๋ก ๋ณ๋์ ์ด์ด ๋๊ณ , `text` ํ๋๋ ์ด์ ๋ฆฌ์คํธ๊ฐ ๋์์ต๋๋ค.
๊ฐ ๋ฌธ์ฅ์ ๊ฐ๋ณ์ ์ผ๋ก ํ ํฐํํ๋ ๋์ ๋ฆฌ์คํธ๋ฅผ ๋ฌธ์์ด๋ก ๋ณํํ์ฌ ํ๋ฒ์ ํ ํฐํํ ์ ์์ต๋๋ค.
๋ค์์ ๊ฐ ์์ ์ ๋ํด ๋ฌธ์์ด๋ก ์ด๋ฃจ์ด์ง ๋ฆฌ์คํธ๋ฅผ `join`ํ๊ณ ๊ฒฐ๊ณผ๋ฅผ ํ ํฐํํ๋ ์ฒซ ๋ฒ์งธ ์ ์ฒ๋ฆฌ ํจ์์
๋๋ค:
```py
>>> def preprocess_function(examples):
... return tokenizer([" ".join(x) for x in examples["answers.text"]])
```
์ด ์ ์ฒ๋ฆฌ ํจ์๋ฅผ ์ ์ฒด ๋ฐ์ดํฐ ์ธํธ์ ์ ์ฉํ๊ธฐ ์ํด ๐ค Datasets [`~datasets.Dataset.map`] ๋ฉ์๋๋ฅผ ์ฌ์ฉํฉ๋๋ค.
๋ฐ์ดํฐ ์ธํธ์ ์ฌ๋ฌ ์์๋ฅผ ํ ๋ฒ์ ์ฒ๋ฆฌํ๋๋ก `batched=True`๋ฅผ ์ค์ ํ๊ณ `num_proc`๋ก ์ฒ๋ฆฌ ํ์๋ฅผ ๋๋ฆฌ๋ฉด `map` ํจ์์ ์๋๋ฅผ ๋์ผ ์ ์์ต๋๋ค.
ํ์ํ์ง ์์ ์ด์ ์ ๊ฑฐํฉ๋๋ค:
```py
>>> tokenized_eli5 = eli5.map(
... preprocess_function,
... batched=True,
... num_proc=4,
... remove_columns=eli5["train"].column_names,
... )
```
์ด ๋ฐ์ดํฐ ์ธํธ์๋ ํ ํฐ ์ํ์ค๊ฐ ํฌํจ๋์ด ์์ง๋ง ์ด ์ค ์ผ๋ถ๋ ๋ชจ๋ธ์ ์ต๋ ์
๋ ฅ ๊ธธ์ด๋ณด๋ค ๊น๋๋ค.
์ด์ ๋ ๋ฒ์งธ ์ ์ฒ๋ฆฌ ํจ์๋ฅผ ์ฌ์ฉํด
- ๋ชจ๋ ์ํ์ค๋ฅผ ์ฐ๊ฒฐํ๊ณ
- ์ฐ๊ฒฐ๋ ์ํ์ค๋ฅผ ์ ์ํ `block_size` ๋ณด๋ค ๋ ์งง์ ๋ฉ์ด๋ฆฌ๋ก ๋ถํ ํ๋๋ฐ, ์ด ๋ฉ์ด๋ฆฌ๋ ๋ชจ๋ธ์ ์ต๋ ์
๋ ฅ ๊ธธ์ด๋ณด๋ค ์งง๊ณ GPU RAM์ด ์์ฉํ ์ ์๋ ๊ธธ์ด์ฌ์ผ ํฉ๋๋ค.
```py
>>> block_size = 128
>>> def group_texts(examples):
... # Concatenate all texts.
... concatenated_examples = {k: sum(examples[k], []) for k in examples.keys()}
... total_length = len(concatenated_examples[list(examples.keys())[0]])
... # We drop the small remainder, we could add padding if the model supported it instead of this drop, you can
... # customize this part to your needs.
... if total_length >= block_size:
... total_length = (total_length // block_size) * block_size
... # Split by chunks of block_size.
... result = {
... k: [t[i : i + block_size] for i in range(0, total_length, block_size)]
... for k, t in concatenated_examples.items()
... }
... result["labels"] = result["input_ids"].copy()
... return result
```
์ ์ฒด ๋ฐ์ดํฐ ์ธํธ์ `group_texts` ํจ์๋ฅผ ์ ์ฉํฉ๋๋ค:
```py
>>> lm_dataset = tokenized_eli5.map(group_texts, batched=True, num_proc=4)
```
์ด์ [`DataCollatorForLanguageModeling`]์ ์ฌ์ฉํ์ฌ ๋ฐ์ดํฐ ์์ ์ ๋ฐฐ์น๋ฅผ ์์ฑํฉ๋๋ค.
๋ฐ์ดํฐ ์ธํธ ์ ์ฒด๋ฅผ ์ต๋ ๊ธธ์ด๋ก ํจ๋ฉํ๋ ๊ฒ๋ณด๋ค collation ๋จ๊ณ์์ ๋งค ๋ฐฐ์น์์์์ ์ต๋ ๊ธธ์ด๋ก ๋ฌธ์ฅ์ *๋์ ์ผ๋ก ํจ๋ฉ*ํ๋ ๊ฒ์ด ๋ ํจ์จ์ ์
๋๋ค.
<frameworkcontent>
<pt>
์ํ์ค ๋ ํ ํฐ์ ํจ๋ฉ ํ ํฐ์ผ๋ก ์ฌ์ฉํ๊ณ ๋ฐ์ดํฐ๋ฅผ ๋ฐ๋ณตํ ๋๋ง๋ค ํ ํฐ์ ๋ฌด์์๋ก ๋ง์คํนํ๋๋ก `mlm_-probability`๋ฅผ ์ง์ ํฉ๋๋ค:
```py
>>> from transformers import DataCollatorForLanguageModeling
>>> tokenizer.pad_token = tokenizer.eos_token
>>> data_collator = DataCollatorForLanguageModeling(tokenizer=tokenizer, mlm_probability=0.15)
```
</pt>
<tf>
์ํ์ค ๋ ํ ํฐ์ ํจ๋ฉ ํ ํฐ์ผ๋ก ์ฌ์ฉํ๊ณ ๋ฐ์ดํฐ๋ฅผ ๋ฐ๋ณตํ ๋๋ง๋ค ํ ํฐ์ ๋ฌด์์๋ก ๋ง์คํนํ๋๋ก `mlm_-probability`๋ฅผ ์ง์ ํฉ๋๋ค:
```py
>>> from transformers import DataCollatorForLanguageModeling
>>> data_collator = DataCollatorForLanguageModeling(tokenizer=tokenizer, mlm_probability=0.15, return_tensors="tf")
```
</tf>
</frameworkcontent>
## ํ๋ จ[[train]]
<frameworkcontent>
<pt>
<Tip>
[`Trainer`]๋ก ๋ชจ๋ธ์ ๋ฏธ์ธ ์กฐ์ ํ๋ ๋ฐ ์ต์ํ์ง ์๋ค๋ฉด ๊ธฐ๋ณธ ํํ ๋ฆฌ์ผ [์ฌ๊ธฐ](../training#train-with-pytorch-trainer)๋ฅผ ์ดํด๋ณด์ธ์!
</Tip>
์ด์ ๋ชจ๋ธ ํ๋ จ์ ์์ํ ์ค๋น๊ฐ ๋์์ต๋๋ค! [`AutoModelForMaskedLM`]๋ฅผ ์ฌ์ฉํด DistilRoBERTa ๋ชจ๋ธ์ ๊ฐ์ ธ์ต๋๋ค:
```py
>>> from transformers import AutoModelForMaskedLM
>>> model = AutoModelForMaskedLM.from_pretrained("distilroberta-base")
```
์ด์ ์ธ ๋จ๊ณ๊ฐ ๋จ์์ต๋๋ค:
1. [`TrainingArguments`]์ ํ๋ จ ํ์ดํผํ๋ผ๋ฏธํฐ๋ฅผ ์ ์ํฉ๋๋ค. ๋ชจ๋ธ ์ ์ฅ ์์น๋ฅผ ์ง์ ํ๋ `output_dir`์ ์ ์ผํ ํ์ ํ๋ผ๋ฏธํฐ์
๋๋ค. `push_to_hub=True`๋ฅผ ์ค์ ํ์ฌ ์ด ๋ชจ๋ธ์ Hub์ ์
๋ก๋ํฉ๋๋ค (๋ชจ๋ธ์ ์
๋ก๋ํ๋ ค๋ฉด Hugging Face์ ๋ก๊ทธ์ธํด์ผ ํฉ๋๋ค).
2. ๋ชจ๋ธ, ๋ฐ์ดํฐ ์ธํธ ๋ฐ ๋ฐ์ดํฐ ์ฝ๋ ์ดํฐ(collator)์ ํจ๊ป ํ๋ จ ์ธ์๋ฅผ [`Trainer`]์ ์ ๋ฌํฉ๋๋ค.
3. [`~Trainer.train`]์ ํธ์ถํ์ฌ ๋ชจ๋ธ์ ๋ฏธ์ธ ์กฐ์ ํฉ๋๋ค.
```py
>>> training_args = TrainingArguments(
... output_dir="my_awesome_eli5_mlm_model",
... evaluation_strategy="epoch",
... learning_rate=2e-5,
... num_train_epochs=3,
... weight_decay=0.01,
... push_to_hub=True,
... )
>>> trainer = Trainer(
... model=model,
... args=training_args,
... train_dataset=lm_dataset["train"],
... eval_dataset=lm_dataset["test"],
... data_collator=data_collator,
... )
>>> trainer.train()
```
ํ๋ จ์ด ์๋ฃ๋๋ฉด [`~transformers.Trainer.evaluate`] ๋ฉ์๋๋ฅผ ์ฌ์ฉํ์ฌ ํํ๋ ์ํฐ(perplexity)๋ฅผ ๊ณ์ฐํ๊ณ ๋ชจ๋ธ์ ํ๊ฐํฉ๋๋ค:
```py
>>> import math
>>> eval_results = trainer.evaluate()
>>> print(f"Perplexity: {math.exp(eval_results['eval_loss']):.2f}")
Perplexity: 8.76
```
๊ทธ๋ฆฌ๊ณ [`~transformers.Trainer.push_to_hub`] ๋ฉ์๋๋ฅผ ์ฌ์ฉํด ๋ค๋ฅธ ์ฌ๋๋ค์ด ์ฌ์ฉํ ์ ์๋๋ก, Hub๋ก ๋ชจ๋ธ์ ์
๋ก๋ํฉ๋๋ค.
```py
>>> trainer.push_to_hub()
```
</pt>
<tf>
<Tip>
Keras๋ก ๋ชจ๋ธ์ ๋ฏธ์ธ ์กฐ์ ํ๋ ๋ฐ ์ต์ํ์ง ์๋ค๋ฉด ๊ธฐ๋ณธ ํํ ๋ฆฌ์ผ [์ฌ๊ธฐ](../training#train-a-tensorflow-model-with-keras)๋ฅผ ์ดํด๋ณด์ธ์!
</Tip>
TensorFlow๋ก ๋ชจ๋ธ์ ๋ฏธ์ธ ์กฐ์ ํ๊ธฐ ์ํด์๋ ์ตํฐ๋ง์ด์ (optimizer) ํจ์ ์ค์ , ํ์ต๋ฅ (learning rate) ์ค์ผ์ฅด๋ง, ํ๋ จ ํ์ดํผํ๋ผ๋ฏธํฐ ์ค์ ๋ถํฐ ์์ํ์ธ์:
```py
>>> from transformers import create_optimizer, AdamWeightDecay
>>> optimizer = AdamWeightDecay(learning_rate=2e-5, weight_decay_rate=0.01)
```
๋ค์์ผ๋ก [`TFAutoModelForMaskedLM`]๋ฅผ ์ฌ์ฉํด DistilRoBERTa ๋ชจ๋ธ์ ๊ฐ์ ธ์ต๋๋ค:
```py
>>> from transformers import TFAutoModelForMaskedLM
>>> model = TFAutoModelForMaskedLM.from_pretrained("distilroberta-base")
```
[`~transformers.TFPreTrainedModel.prepare_tf_dataset`] ๋ฉ์๋๋ฅผ ์ฌ์ฉํด ๋ฐ์ดํฐ ์ธํธ๋ฅผ `tf.data.Dataset` ํ์์ผ๋ก ๋ณํํ์ธ์:
```py
>>> tf_train_set = model.prepare_tf_dataset(
... lm_dataset["train"],
... shuffle=True,
... batch_size=16,
... collate_fn=data_collator,
... )
>>> tf_test_set = model.prepare_tf_dataset(
... lm_dataset["test"],
... shuffle=False,
... batch_size=16,
... collate_fn=data_collator,
... )
```
[`compile`](https://keras.io/api/models/model_training_apis/#compile-method) ๋ฉ์๋๋ฅผ ํตํด ๋ชจ๋ธ ํ๋ จ์ ๊ตฌ์ฑํฉ๋๋ค:
```py
>>> import tensorflow as tf
>>> model.compile(optimizer=optimizer)
```
์ด๋ ์
๋ก๋ํ ๋ชจ๋ธ๊ณผ ํ ํฌ๋์ด์ ์ ์์น๋ฅผ [`~transformers.PushToHubCallback`]์ ์ง์ ํ์ฌ ์ํํ ์ ์์ต๋๋ค:
```py
>>> from transformers.keras_callbacks import PushToHubCallback
>>> callback = PushToHubCallback(
... output_dir="my_awesome_eli5_mlm_model",
... tokenizer=tokenizer,
... )
```
๋๋์ด ๋ชจ๋ธ์ ํ๋ จํ ์ค๋น๊ฐ ๋์์ต๋๋ค!
๋ชจ๋ธ์ ๋ฏธ์ธ ์กฐ์ ํ ๋ ํ๋ จ ๋ฐ ๊ฒ์ฆ ๋ฐ์ดํฐ ์ธํธ, ์ํฌํฌ ์, ์ฝ๋ฐฑ์ด ํฌํจ๋ [`fit`](https://keras.io/api/models/model_training_apis/#fit-method)์ ํธ์ถํฉ๋๋ค:
```py
>>> model.fit(x=tf_train_set, validation_data=tf_test_set, epochs=3, callbacks=[callback])
```
ํ๋ จ์ด ์๋ฃ๋๋ฉด, ์๋์ผ๋ก Hub๋ก ์
๋ก๋๋์ด ๋๊ตฌ๋ ์ฌ์ฉํ ์ ์์ต๋๋ค!
</tf>
</frameworkcontent>
<Tip>
๋ง์คํน๋ ์ธ์ด ๋ชจ๋ธ๋ง์ ์ํด ๋ชจ๋ธ์ ๋ฏธ์ธ ์กฐ์ ํ๋ ๋ฐฉ๋ฒ์ ๋ํ ๋ณด๋ค ์ฌ์ธต์ ์ธ ์์ ๋
[PyTorch notebook](https://colab.research.google.com/github/huggingface/notebooks/blob/main/examples/language_modeling.ipynb)
๋๋ [TensorFlow notebook](https://colab.research.google.com/github/huggingface/notebooks/blob/main/examples/language_modeling-tf.ipynb)์ ์ฐธ์กฐํ์ธ์.
</Tip>
## ์ถ๋ก [[inference]]
์ง๊ธ๊น์ง ๋ชจ๋ธ ๋ฏธ์ธ ์กฐ์ ์ ์ ํ์ผ๋, ์ถ๋ก ์ ์ฌ์ฉํ ์ ์์ต๋๋ค!
๋ชจ๋ธ์ด ๋น์นธ์ ์ฑ์ธ ํ
์คํธ๋ฅผ ์คํ์
ํ ํฐ(special token)์ธ `<mask>` ํ ํฐ์ผ๋ก ํ์ํฉ๋๋ค:
```py
>>> text = "The Milky Way is a <mask> galaxy."
```
์ถ๋ก ์ ์ํด ๋ฏธ์ธ ์กฐ์ ํ ๋ชจ๋ธ์ ํ
์คํธํ๋ ๊ฐ์ฅ ๊ฐ๋จํ ๋ฐฉ๋ฒ์ [`pipeline`]์์ ์ฌ์ฉํ๋ ๊ฒ์
๋๋ค.
`fill-mask`ํ์คํฌ๋ก `pipeline`์ ์ธ์คํด์คํํ๊ณ ํ
์คํธ๋ฅผ ์ ๋ฌํฉ๋๋ค.
`top_k` ๋งค๊ฐ๋ณ์๋ฅผ ์ฌ์ฉํ์ฌ ๋ฐํํ๋ ์์ธก์ ์๋ฅผ ์ง์ ํ ์ ์์ต๋๋ค:
```py
>>> from transformers import pipeline
>>> mask_filler = pipeline("fill-mask", "stevhliu/my_awesome_eli5_mlm_model")
>>> mask_filler(text, top_k=3)
[{'score': 0.5150994658470154,
'token': 21300,
'token_str': ' spiral',
'sequence': 'The Milky Way is a spiral galaxy.'},
{'score': 0.07087188959121704,
'token': 2232,
'token_str': ' massive',
'sequence': 'The Milky Way is a massive galaxy.'},
{'score': 0.06434620916843414,
'token': 650,
'token_str': ' small',
'sequence': 'The Milky Way is a small galaxy.'}]
```
<frameworkcontent>
<pt>
ํ
์คํธ๋ฅผ ํ ํฐํํ๊ณ `input_ids`๋ฅผ PyTorch ํ
์ ํํ๋ก ๋ฐํํฉ๋๋ค.
๋ํ, `<mask>` ํ ํฐ์ ์์น๋ฅผ ์ง์ ํด์ผ ํฉ๋๋ค:
```py
>>> from transformers import AutoTokenizer
>>> tokenizer = AutoTokenizer.from_pretrained("my_awesome_eli5_mlm_model")
>>> inputs = tokenizer(text, return_tensors="pt")
>>> mask_token_index = torch.where(inputs["input_ids"] == tokenizer.mask_token_id)[1]
```
๋ชจ๋ธ์ `inputs`๋ฅผ ์
๋ ฅํ๊ณ , ๋ง์คํน๋ ํ ํฐ์ `logits`๋ฅผ ๋ฐํํฉ๋๋ค:
```py
>>> from transformers import AutoModelForMaskedLM
>>> model = AutoModelForMaskedLM.from_pretrained("stevhliu/my_awesome_eli5_mlm_model")
>>> logits = model(**inputs).logits
>>> mask_token_logits = logits[0, mask_token_index, :]
```
๊ทธ๋ฐ ๋ค์ ๊ฐ์ฅ ๋์ ํ๋ฅ ์ ๊ฐ์ง ๋ง์คํฌ ํ ํฐ 3๊ฐ๋ฅผ ๋ฐํํ๊ณ , ์ถ๋ ฅํฉ๋๋ค:
```py
>>> top_3_tokens = torch.topk(mask_token_logits, 3, dim=1).indices[0].tolist()
>>> for token in top_3_tokens:
... print(text.replace(tokenizer.mask_token, tokenizer.decode([token])))
The Milky Way is a spiral galaxy.
The Milky Way is a massive galaxy.
The Milky Way is a small galaxy.
```
</pt>
<tf>
ํ
์คํธ๋ฅผ ํ ํฐํํ๊ณ `input_ids`๋ฅผ TensorFlow ํ
์ ํํ๋ก ๋ฐํํฉ๋๋ค.
๋ํ, `<mask>` ํ ํฐ์ ์์น๋ฅผ ์ง์ ํด์ผ ํฉ๋๋ค:
```py
>>> from transformers import AutoTokenizer
>>> tokenizer = AutoTokenizer.from_pretrained("my_awesome_eli5_mlm_model")
>>> inputs = tokenizer(text, return_tensors="tf")
>>> mask_token_index = tf.where(inputs["input_ids"] == tokenizer.mask_token_id)[0, 1]
```
๋ชจ๋ธ์ `inputs`๋ฅผ ์
๋ ฅํ๊ณ , ๋ง์คํน๋ ํ ํฐ์ `logits`๋ฅผ ๋ฐํํฉ๋๋ค:
```py
>>> from transformers import TFAutoModelForMaskedLM
>>> model = TFAutoModelForMaskedLM.from_pretrained("stevhliu/my_awesome_eli5_mlm_model")
>>> logits = model(**inputs).logits
>>> mask_token_logits = logits[0, mask_token_index, :]
```
๊ทธ๋ฐ ๋ค์ ๊ฐ์ฅ ๋์ ํ๋ฅ ์ ๊ฐ์ง ๋ง์คํฌ ํ ํฐ 3๊ฐ๋ฅผ ๋ฐํํ๊ณ , ์ถ๋ ฅํฉ๋๋ค:
```py
>>> top_3_tokens = tf.math.top_k(mask_token_logits, 3).indices.numpy()
>>> for token in top_3_tokens:
... print(text.replace(tokenizer.mask_token, tokenizer.decode([token])))
The Milky Way is a spiral galaxy.
The Milky Way is a massive galaxy.
The Milky Way is a small galaxy.
```
</tf>
</frameworkcontent>
| 0 |
hf_public_repos/transformers/docs/source/ko | hf_public_repos/transformers/docs/source/ko/tasks/object_detection.md | <!--Copyright 2022 The HuggingFace Team. All rights reserved.
Licensed under the Apache License, Version 2.0 (the "License"); you may not use this file except in compliance with
the License. You may obtain a copy of the License at
http://www.apache.org/licenses/LICENSE-2.0
Unless required by applicable law or agreed to in writing, software distributed under the License is distributed on
an "AS IS" BASIS, WITHOUT WARRANTIES OR CONDITIONS OF ANY KIND, either express or implied. See the License for the
specific language governing permissions and limitations under the License.
โ ๏ธ Note that this file is in Markdown but contain specific syntax for our doc-builder (similar to MDX) that may not be
rendered properly in your Markdown viewer.
-->
# ๊ฐ์ฒด ํ์ง [[object-detection]]
[[open-in-colab]]
๊ฐ์ฒด ํ์ง๋ ์ด๋ฏธ์ง์์ ์ธ์คํด์ค(์: ์ฌ๋, ๊ฑด๋ฌผ ๋๋ ์๋์ฐจ)๋ฅผ ๊ฐ์งํ๋ ์ปดํจํฐ ๋น์ ์์
์
๋๋ค. ๊ฐ์ฒด ํ์ง ๋ชจ๋ธ์ ์ด๋ฏธ์ง๋ฅผ ์
๋ ฅ์ผ๋ก ๋ฐ๊ณ ํ์ง๋ ๋ฐ์ด๋ฉ ๋ฐ์ค์ ์ขํ์ ๊ด๋ จ๋ ๋ ์ด๋ธ์ ์ถ๋ ฅํฉ๋๋ค.
ํ๋์ ์ด๋ฏธ์ง์๋ ์ฌ๋ฌ ๊ฐ์ฒด๊ฐ ์์ ์ ์์ผ๋ฉฐ ๊ฐ๊ฐ์ ์์ฒด์ ์ธ ๋ฐ์ด๋ฉ ๋ฐ์ค์ ๋ ์ด๋ธ์ ๊ฐ์ง ์ ์์ต๋๋ค(์: ์ฐจ์ ๊ฑด๋ฌผ์ด ์๋ ์ด๋ฏธ์ง).
๋ํ ๊ฐ ๊ฐ์ฒด๋ ์ด๋ฏธ์ง์ ๋ค๋ฅธ ๋ถ๋ถ์ ์กด์ฌํ ์ ์์ต๋๋ค(์: ์ด๋ฏธ์ง์ ์ฌ๋ฌ ๋์ ์ฐจ๊ฐ ์์ ์ ์์).
์ด ์์
์ ๋ณดํ์, ๋๋ก ํ์งํ, ์ ํธ๋ฑ๊ณผ ๊ฐ์ ๊ฒ๋ค์ ๊ฐ์งํ๋ ์์จ ์ฃผํ์ ์ผ๋ฐ์ ์ผ๋ก ์ฌ์ฉ๋ฉ๋๋ค.
๋ค๋ฅธ ์์ฉ ๋ถ์ผ๋ก๋ ์ด๋ฏธ์ง ๋ด ๊ฐ์ฒด ์ ๊ณ์ฐ ๋ฐ ์ด๋ฏธ์ง ๊ฒ์ ๋ฑ์ด ์์ต๋๋ค.
์ด ๊ฐ์ด๋์์ ๋ค์์ ๋ฐฐ์ธ ๊ฒ์
๋๋ค:
1. ํฉ์ฑ๊ณฑ ๋ฐฑ๋ณธ(์ธํ ๋ฐ์ดํฐ์ ํน์ฑ์ ์ถ์ถํ๋ ํฉ์ฑ๊ณฑ ๋คํธ์ํฌ)๊ณผ ์ธ์ฝ๋-๋์ฝ๋ ํธ๋์คํฌ๋จธ ๋ชจ๋ธ์ ๊ฒฐํฉํ [DETR](https://huggingface.co/docs/transformers/model_doc/detr) ๋ชจ๋ธ์ [CPPE-5](https://huggingface.co/datasets/cppe-5) ๋ฐ์ดํฐ ์ธํธ์ ๋ํด ๋ฏธ์ธ์กฐ์ ํ๊ธฐ
2. ๋ฏธ์ธ์กฐ์ ํ ๋ชจ๋ธ์ ์ถ๋ก ์ ์ฌ์ฉํ๊ธฐ.
<Tip>
์ด ํํ ๋ฆฌ์ผ์ ํ์คํฌ๋ ๋ค์ ๋ชจ๋ธ ์ํคํ
์ฒ์์ ์ง์๋ฉ๋๋ค:
<!--This tip is automatically generated by `make fix-copies`, do not fill manually!-->
[Conditional DETR](../model_doc/conditional_detr), [Deformable DETR](../model_doc/deformable_detr), [DETA](../model_doc/deta), [DETR](../model_doc/detr), [Table Transformer](../model_doc/table-transformer), [YOLOS](../model_doc/yolos)
<!--End of the generated tip-->
</Tip>
์์ํ๊ธฐ ์ ์ ํ์ํ ๋ชจ๋ ๋ผ์ด๋ธ๋ฌ๋ฆฌ๊ฐ ์ค์น๋์ด ์๋์ง ํ์ธํ์ธ์:
```bash
pip install -q datasets transformers evaluate timm albumentations
```
ํ๊น
ํ์ด์ค ํ๋ธ์์ ๋ฐ์ดํฐ ์ธํธ๋ฅผ ๊ฐ์ ธ์ค๊ธฐ ์ํ ๐ค Datasets๊ณผ ๋ชจ๋ธ์ ํ์ตํ๊ธฐ ์ํ ๐ค Transformers, ๋ฐ์ดํฐ๋ฅผ ์ฆ๊ฐํ๊ธฐ ์ํ `albumentations`๋ฅผ ์ฌ์ฉํฉ๋๋ค.
DETR ๋ชจ๋ธ์ ํฉ์ฑ๊ณฑ ๋ฐฑ๋ณธ์ ๊ฐ์ ธ์ค๊ธฐ ์ํด์๋ ํ์ฌ `timm`์ด ํ์ํฉ๋๋ค.
์ปค๋ฎค๋ํฐ์ ๋ชจ๋ธ์ ์
๋ก๋ํ๊ณ ๊ณต์ ํ ์ ์๋๋ก Hugging Face ๊ณ์ ์ ๋ก๊ทธ์ธํ๋ ๊ฒ์ ๊ถ์ฅํฉ๋๋ค. ํ๋กฌํํธ๊ฐ ๋ํ๋๋ฉด ํ ํฐ์ ์
๋ ฅํ์ฌ ๋ก๊ทธ์ธํ์ธ์:
```py
>>> from huggingface_hub import notebook_login
>>> notebook_login()
```
## CPPE-5 ๋ฐ์ดํฐ ์ธํธ ๊ฐ์ ธ์ค๊ธฐ [[load-the-CPPE-5-dataset]]
[CPPE-5](https://huggingface.co/datasets/cppe-5) ๋ฐ์ดํฐ ์ธํธ๋ COVID-19 ๋์ ํ ์ํฉ์์ ์๋ฃ ์ ๋ฌธ์ธ๋ ฅ ๋ณดํธ ์ฅ๋น(PPE)๋ฅผ ์๋ณํ๋ ์ด๋
ธํ
์ด์
์ด ํฌํจ๋ ์ด๋ฏธ์ง๋ฅผ ๋ด๊ณ ์์ต๋๋ค.
๋ฐ์ดํฐ ์ธํธ๋ฅผ ๊ฐ์ ธ์ค์ธ์:
```py
>>> from datasets import load_dataset
>>> cppe5 = load_dataset("cppe-5")
>>> cppe5
DatasetDict({
train: Dataset({
features: ['image_id', 'image', 'width', 'height', 'objects'],
num_rows: 1000
})
test: Dataset({
features: ['image_id', 'image', 'width', 'height', 'objects'],
num_rows: 29
})
})
```
์ด ๋ฐ์ดํฐ ์ธํธ๋ ํ์ต ์ธํธ ์ด๋ฏธ์ง 1,000๊ฐ์ ํ
์คํธ ์ธํธ ์ด๋ฏธ์ง 29๊ฐ๋ฅผ ๊ฐ๊ณ ์์ต๋๋ค.
๋ฐ์ดํฐ์ ์ต์ํด์ง๊ธฐ ์ํด, ์์๊ฐ ์ด๋ป๊ฒ ๊ตฌ์ฑ๋์ด ์๋์ง ์ดํด๋ณด์ธ์.
```py
>>> cppe5["train"][0]
{'image_id': 15,
'image': <PIL.JpegImagePlugin.JpegImageFile image mode=RGB size=943x663 at 0x7F9EC9E77C10>,
'width': 943,
'height': 663,
'objects': {'id': [114, 115, 116, 117],
'area': [3796, 1596, 152768, 81002],
'bbox': [[302.0, 109.0, 73.0, 52.0],
[810.0, 100.0, 57.0, 28.0],
[160.0, 31.0, 248.0, 616.0],
[741.0, 68.0, 202.0, 401.0]],
'category': [4, 4, 0, 0]}}
```
๋ฐ์ดํฐ ์ธํธ์ ์๋ ์์๋ ๋ค์์ ์์ญ์ ๊ฐ์ง๊ณ ์์ต๋๋ค:
- `image_id`: ์์ ์ด๋ฏธ์ง id
- `image`: ์ด๋ฏธ์ง๋ฅผ ํฌํจํ๋ `PIL.Image.Image` ๊ฐ์ฒด
- `width`: ์ด๋ฏธ์ง์ ๋๋น
- `height`: ์ด๋ฏธ์ง์ ๋์ด
- `objects`: ์ด๋ฏธ์ง ์์ ๊ฐ์ฒด๋ค์ ๋ฐ์ด๋ฉ ๋ฐ์ค ๋ฉํ๋ฐ์ดํฐ๋ฅผ ํฌํจํ๋ ๋์
๋๋ฆฌ:
- `id`: ์ด๋
ธํ
์ด์
id
- `area`: ๋ฐ์ด๋ฉ ๋ฐ์ค์ ๋ฉด์
- `bbox`: ๊ฐ์ฒด์ ๋ฐ์ด๋ฉ ๋ฐ์ค ([COCO ํฌ๋งท](https://albumentations.ai/docs/getting_started/bounding_boxes_augmentation/#coco)์ผ๋ก)
- `category`: ๊ฐ์ฒด์ ์นดํ
๊ณ ๋ฆฌ, ๊ฐ๋ฅํ ๊ฐ์ผ๋ก๋ `Coverall (0)`, `Face_Shield (1)`, `Gloves (2)`, `Goggles (3)` ๋ฐ `Mask (4)` ๊ฐ ํฌํจ๋ฉ๋๋ค.
`bbox` ํ๋๊ฐ DETR ๋ชจ๋ธ์ด ์๊ตฌํ๋ COCO ํ์์ ๋ฐ๋ฅธ๋ค๋ ๊ฒ์ ์ ์ ์์ต๋๋ค.
๊ทธ๋ฌ๋ `objects` ๋ด๋ถ์ ํ๋ ๊ทธ๋ฃน์ DETR์ด ์๊ตฌํ๋ ์ด๋
ธํ
์ด์
ํ์๊ณผ ๋ค๋ฆ
๋๋ค. ๋ฐ๋ผ์ ์ด ๋ฐ์ดํฐ๋ฅผ ํ์ต์ ์ฌ์ฉํ๊ธฐ ์ ์ ์ ์ฒ๋ฆฌ๋ฅผ ์ ์ฉํด์ผ ํฉ๋๋ค.
๋ฐ์ดํฐ๋ฅผ ๋ ์ ์ดํดํ๊ธฐ ์ํด์ ๋ฐ์ดํฐ ์ธํธ์์ ํ ๊ฐ์ง ์์๋ฅผ ์๊ฐํํ์ธ์.
```py
>>> import numpy as np
>>> import os
>>> from PIL import Image, ImageDraw
>>> image = cppe5["train"][0]["image"]
>>> annotations = cppe5["train"][0]["objects"]
>>> draw = ImageDraw.Draw(image)
>>> categories = cppe5["train"].features["objects"].feature["category"].names
>>> id2label = {index: x for index, x in enumerate(categories, start=0)}
>>> label2id = {v: k for k, v in id2label.items()}
>>> for i in range(len(annotations["id"])):
... box = annotations["bbox"][i - 1]
... class_idx = annotations["category"][i - 1]
... x, y, w, h = tuple(box)
... draw.rectangle((x, y, x + w, y + h), outline="red", width=1)
... draw.text((x, y), id2label[class_idx], fill="white")
>>> image
```
<div class="flex justify-center">
<img src="https://i.imgur.com/TdaqPJO.png" alt="CPPE-5 Image Example"/>
</div>
๋ฐ์ด๋ฉ ๋ฐ์ค์ ์ฐ๊ฒฐ๋ ๋ ์ด๋ธ์ ์๊ฐํํ๋ ค๋ฉด ๋ฐ์ดํฐ ์ธํธ์ ๋ฉํ ๋ฐ์ดํฐ, ํนํ `category` ํ๋์์ ๋ ์ด๋ธ์ ๊ฐ์ ธ์์ผ ํฉ๋๋ค.
๋ํ ๋ ์ด๋ธ ID๋ฅผ ๋ ์ด๋ธ ํด๋์ค์ ๋งคํํ๋ `id2label`๊ณผ ๋ฐ๋๋ก ๋งคํํ๋ `label2id` ๋์
๋๋ฆฌ๋ฅผ ๋ง๋ค์ด์ผ ํฉ๋๋ค.
๋ชจ๋ธ์ ์ค์ ํ ๋ ์ด๋ฌํ ๋งคํ์ ์ฌ์ฉํ ์ ์์ต๋๋ค. ์ด๋ฌํ ๋งคํ์ ํ๊น
ํ์ด์ค ํ๋ธ์์ ๋ชจ๋ธ์ ๊ณต์ ํ์ ๋ ๋ค๋ฅธ ์ฌ๋๋ค์ด ์ฌ์ฌ์ฉํ ์ ์์ต๋๋ค.
๋ฐ์ดํฐ๋ฅผ ๋ ์ ์ดํดํ๊ธฐ ์ํ ์ต์ข
๋จ๊ณ๋ก, ์ ์ฌ์ ์ธ ๋ฌธ์ ๋ฅผ ์ฐพ์๋ณด์ธ์.
๊ฐ์ฒด ๊ฐ์ง๋ฅผ ์ํ ๋ฐ์ดํฐ ์ธํธ์์ ์์ฃผ ๋ฐ์ํ๋ ๋ฌธ์ ์ค ํ๋๋ ๋ฐ์ด๋ฉ ๋ฐ์ค๊ฐ ์ด๋ฏธ์ง์ ๊ฐ์ฅ์๋ฆฌ๋ฅผ ๋์ด๊ฐ๋ ๊ฒ์
๋๋ค.
์ด๋ฌํ ๋ฐ์ด๋ฉ ๋ฐ์ค๋ฅผ "๋์ด๊ฐ๋ ๊ฒ(run away)"์ ํ๋ จ ์ค์ ์ค๋ฅ๋ฅผ ๋ฐ์์ํฌ ์ ์๊ธฐ์ ์ด ๋จ๊ณ์์ ์ฒ๋ฆฌํด์ผ ํฉ๋๋ค.
์ด ๋ฐ์ดํฐ ์ธํธ์๋ ๊ฐ์ ๋ฌธ์ ๊ฐ ์๋ ๋ช ๊ฐ์ง ์๊ฐ ์์ต๋๋ค. ์ด ๊ฐ์ด๋์์๋ ๊ฐ๋จํ๊ฒํ๊ธฐ ์ํด ๋ฐ์ดํฐ์์ ์ด๋ฌํ ์ด๋ฏธ์ง๋ฅผ ์ ๊ฑฐํฉ๋๋ค.
```py
>>> remove_idx = [590, 821, 822, 875, 876, 878, 879]
>>> keep = [i for i in range(len(cppe5["train"])) if i not in remove_idx]
>>> cppe5["train"] = cppe5["train"].select(keep)
```
## ๋ฐ์ดํฐ ์ ์ฒ๋ฆฌํ๊ธฐ [[preprocess-the-data]]
๋ชจ๋ธ์ ๋ฏธ์ธ ์กฐ์ ํ๋ ค๋ฉด, ๋ฏธ๋ฆฌ ํ์ต๋ ๋ชจ๋ธ์์ ์ฌ์ฉํ ์ ์ฒ๋ฆฌ ๋ฐฉ์๊ณผ ์ ํํ๊ฒ ์ผ์นํ๋๋ก ์ฌ์ฉํ ๋ฐ์ดํฐ๋ฅผ ์ ์ฒ๋ฆฌํด์ผ ํฉ๋๋ค.
[`AutoImageProcessor`]๋ ์ด๋ฏธ์ง ๋ฐ์ดํฐ๋ฅผ ์ฒ๋ฆฌํ์ฌ DETR ๋ชจ๋ธ์ด ํ์ต์ ์ฌ์ฉํ ์ ์๋ `pixel_values`, `pixel_mask`, ๊ทธ๋ฆฌ๊ณ `labels`๋ฅผ ์์ฑํ๋ ์์
์ ๋ด๋นํฉ๋๋ค.
์ด ์ด๋ฏธ์ง ํ๋ก์ธ์์๋ ๊ฑฑ์ ํ์ง ์์๋ ๋๋ ๋ช ๊ฐ์ง ์์ฑ์ด ์์ต๋๋ค:
- `image_mean = [0.485, 0.456, 0.406 ]`
- `image_std = [0.229, 0.224, 0.225]`
์ด ๊ฐ๋ค์ ๋ชจ๋ธ ์ฌ์ ํ๋ จ ์ค ์ด๋ฏธ์ง๋ฅผ ์ ๊ทํํ๋ ๋ฐ ์ฌ์ฉ๋๋ ํ๊ท ๊ณผ ํ์ค ํธ์ฐจ์
๋๋ค.
์ด ๊ฐ๋ค์ ์ถ๋ก ๋๋ ์ฌ์ ํ๋ จ๋ ์ด๋ฏธ์ง ๋ชจ๋ธ์ ์ธ๋ฐํ๊ฒ ์กฐ์ ํ ๋ ๋ณต์ ํด์ผ ํ๋ ์ค์ํ ๊ฐ์
๋๋ค.
์ฌ์ ํ๋ จ๋ ๋ชจ๋ธ๊ณผ ๋์ผํ ์ฒดํฌํฌ์ธํธ์์ ์ด๋ฏธ์ง ํ๋ก์ธ์๋ฅผ ์ธ์คํด์คํํฉ๋๋ค.
```py
>>> from transformers import AutoImageProcessor
>>> checkpoint = "facebook/detr-resnet-50"
>>> image_processor = AutoImageProcessor.from_pretrained(checkpoint)
```
`image_processor`์ ์ด๋ฏธ์ง๋ฅผ ์ ๋ฌํ๊ธฐ ์ ์, ๋ฐ์ดํฐ ์ธํธ์ ๋ ๊ฐ์ง ์ ์ฒ๋ฆฌ๋ฅผ ์ ์ฉํด์ผ ํฉ๋๋ค:
- ์ด๋ฏธ์ง ์ฆ๊ฐ
- DETR ๋ชจ๋ธ์ ์๊ตฌ์ ๋ง๊ฒ ์ด๋
ธํ
์ด์
์ ๋ค์ ํฌ๋งทํ
์ฒซ์งธ๋ก, ๋ชจ๋ธ์ด ํ์ต ๋ฐ์ดํฐ์ ๊ณผ์ ํฉ ๋์ง ์๋๋ก ๋ฐ์ดํฐ ์ฆ๊ฐ ๋ผ์ด๋ธ๋ฌ๋ฆฌ ์ค ์๋ฌด๊ฑฐ๋ ์ฌ์ฉํ์ฌ ๋ณํ์ ์ ์ฉํ ์ ์์ต๋๋ค. ์ฌ๊ธฐ์์๋ [Albumentations](https://albumentations.ai/docs/) ๋ผ์ด๋ธ๋ฌ๋ฆฌ๋ฅผ ์ฌ์ฉํฉ๋๋ค...
์ด ๋ผ์ด๋ธ๋ฌ๋ฆฌ๋ ๋ณํ์ ์ด๋ฏธ์ง์ ์ ์ฉํ๊ณ ๋ฐ์ด๋ฉ ๋ฐ์ค๋ฅผ ์ ์ ํ๊ฒ ์
๋ฐ์ดํธํ๋๋ก ๋ณด์ฅํฉ๋๋ค.
๐ค Datasets ๋ผ์ด๋ธ๋ฌ๋ฆฌ ๋ฌธ์์๋ [๊ฐ์ฒด ํ์ง๋ฅผ ์ํด ์ด๋ฏธ์ง๋ฅผ ๋ณด๊ฐํ๋ ๋ฐฉ๋ฒ์ ๋ํ ์์ธํ ๊ฐ์ด๋](https://huggingface.co/docs/datasets/object_detection)๊ฐ ์์ผ๋ฉฐ,
์ด ์์ ์ ์ ํํ ๋์ผํ ๋ฐ์ดํฐ ์ธํธ๋ฅผ ์ฌ์ฉํฉ๋๋ค. ์ฌ๊ธฐ์๋ ๊ฐ ์ด๋ฏธ์ง๋ฅผ (480, 480) ํฌ๊ธฐ๋ก ์กฐ์ ํ๊ณ , ์ข์ฐ๋ก ๋ค์ง๊ณ , ๋ฐ๊ธฐ๋ฅผ ๋์ด๋ ๋์ผํ ์ ๊ทผ๋ฒ์ ์ ์ฉํฉ๋๋ค:
```py
>>> import albumentations
>>> import numpy as np
>>> import torch
>>> transform = albumentations.Compose(
... [
... albumentations.Resize(480, 480),
... albumentations.HorizontalFlip(p=1.0),
... albumentations.RandomBrightnessContrast(p=1.0),
... ],
... bbox_params=albumentations.BboxParams(format="coco", label_fields=["category"]),
... )
```
์ด๋ฏธ์ง ํ๋ก์ธ์๋ ์ด๋
ธํ
์ด์
์ด ๋ค์๊ณผ ๊ฐ์ ํ์์ผ ๊ฒ์ผ๋ก ์์ํฉ๋๋ค: `{'image_id': int, 'annotations': List[Dict]}`, ์ฌ๊ธฐ์ ๊ฐ ๋์
๋๋ฆฌ๋ COCO ๊ฐ์ฒด ์ด๋
ธํ
์ด์
์
๋๋ค. ๋จ์ผ ์์ ์ ๋ํด ์ด๋
ธํ
์ด์
์ ํ์์ ๋ค์ ์ง์ ํ๋ ํจ์๋ฅผ ์ถ๊ฐํด ๋ณด๊ฒ ์ต๋๋ค:
```py
>>> def formatted_anns(image_id, category, area, bbox):
... annotations = []
... for i in range(0, len(category)):
... new_ann = {
... "image_id": image_id,
... "category_id": category[i],
... "isCrowd": 0,
... "area": area[i],
... "bbox": list(bbox[i]),
... }
... annotations.append(new_ann)
... return annotations
```
์ด์ ์ด๋ฏธ์ง์ ์ด๋
ธํ
์ด์
์ ์ฒ๋ฆฌ ๋ณํ์ ๊ฒฐํฉํ์ฌ ์์ ๋ฐฐ์น์ ์ฌ์ฉํ ์ ์์ต๋๋ค:
```py
>>> # transforming a batch
>>> def transform_aug_ann(examples):
... image_ids = examples["image_id"]
... images, bboxes, area, categories = [], [], [], []
... for image, objects in zip(examples["image"], examples["objects"]):
... image = np.array(image.convert("RGB"))[:, :, ::-1]
... out = transform(image=image, bboxes=objects["bbox"], category=objects["category"])
... area.append(objects["area"])
... images.append(out["image"])
... bboxes.append(out["bboxes"])
... categories.append(out["category"])
... targets = [
... {"image_id": id_, "annotations": formatted_anns(id_, cat_, ar_, box_)}
... for id_, cat_, ar_, box_ in zip(image_ids, categories, area, bboxes)
... ]
... return image_processor(images=images, annotations=targets, return_tensors="pt")
```
์ด์ ๋จ๊ณ์์ ๋ง๋ ์ ์ฒ๋ฆฌ ํจ์๋ฅผ ๐ค Datasets์ [`~datasets.Dataset.with_transform`] ๋ฉ์๋๋ฅผ ์ฌ์ฉํ์ฌ ๋ฐ์ดํฐ ์ธํธ ์ ์ฒด์ ์ ์ฉํฉ๋๋ค.
์ด ๋ฉ์๋๋ ๋ฐ์ดํฐ ์ธํธ์ ์์๋ฅผ ๊ฐ์ ธ์ฌ ๋๋ง๋ค ์ ์ฒ๋ฆฌ ํจ์๋ฅผ ์ ์ฉํฉ๋๋ค.
์ด ์์ ์์๋ ์ ์ฒ๋ฆฌ ํ ๋ฐ์ดํฐ ์ธํธ์์ ์์ ํ๋๋ฅผ ๊ฐ์ ธ์์ ๋ณํ ํ ๋ชจ์์ด ์ด๋ป๊ฒ ๋๋์ง ํ์ธํด ๋ณผ ์ ์์ต๋๋ค.
์ด๋, `pixel_values` ํ
์, `pixel_mask` ํ
์, ๊ทธ๋ฆฌ๊ณ `labels`๋ก ๊ตฌ์ฑ๋ ํ
์๊ฐ ์์ด์ผ ํฉ๋๋ค.
```py
>>> cppe5["train"] = cppe5["train"].with_transform(transform_aug_ann)
>>> cppe5["train"][15]
{'pixel_values': tensor([[[ 0.9132, 0.9132, 0.9132, ..., -1.9809, -1.9809, -1.9809],
[ 0.9132, 0.9132, 0.9132, ..., -1.9809, -1.9809, -1.9809],
[ 0.9132, 0.9132, 0.9132, ..., -1.9638, -1.9638, -1.9638],
...,
[-1.5699, -1.5699, -1.5699, ..., -1.9980, -1.9980, -1.9980],
[-1.5528, -1.5528, -1.5528, ..., -1.9980, -1.9809, -1.9809],
[-1.5528, -1.5528, -1.5528, ..., -1.9980, -1.9809, -1.9809]],
[[ 1.3081, 1.3081, 1.3081, ..., -1.8431, -1.8431, -1.8431],
[ 1.3081, 1.3081, 1.3081, ..., -1.8431, -1.8431, -1.8431],
[ 1.3081, 1.3081, 1.3081, ..., -1.8256, -1.8256, -1.8256],
...,
[-1.3179, -1.3179, -1.3179, ..., -1.8606, -1.8606, -1.8606],
[-1.3004, -1.3004, -1.3004, ..., -1.8606, -1.8431, -1.8431],
[-1.3004, -1.3004, -1.3004, ..., -1.8606, -1.8431, -1.8431]],
[[ 1.4200, 1.4200, 1.4200, ..., -1.6476, -1.6476, -1.6476],
[ 1.4200, 1.4200, 1.4200, ..., -1.6476, -1.6476, -1.6476],
[ 1.4200, 1.4200, 1.4200, ..., -1.6302, -1.6302, -1.6302],
...,
[-1.0201, -1.0201, -1.0201, ..., -1.5604, -1.5604, -1.5604],
[-1.0027, -1.0027, -1.0027, ..., -1.5604, -1.5430, -1.5430],
[-1.0027, -1.0027, -1.0027, ..., -1.5604, -1.5430, -1.5430]]]),
'pixel_mask': tensor([[1, 1, 1, ..., 1, 1, 1],
[1, 1, 1, ..., 1, 1, 1],
[1, 1, 1, ..., 1, 1, 1],
...,
[1, 1, 1, ..., 1, 1, 1],
[1, 1, 1, ..., 1, 1, 1],
[1, 1, 1, ..., 1, 1, 1]]),
'labels': {'size': tensor([800, 800]), 'image_id': tensor([756]), 'class_labels': tensor([4]), 'boxes': tensor([[0.7340, 0.6986, 0.3414, 0.5944]]), 'area': tensor([519544.4375]), 'iscrowd': tensor([0]), 'orig_size': tensor([480, 480])}}
```
๊ฐ๊ฐ์ ์ด๋ฏธ์ง๋ฅผ ์ฑ๊ณต์ ์ผ๋ก ์ฆ๊ฐํ๊ณ ์ด๋ฏธ์ง์ ์ด๋
ธํ
์ด์
์ ์ค๋นํ์ต๋๋ค.
๊ทธ๋ฌ๋ ์ ์ฒ๋ฆฌ๋ ์์ง ๋๋์ง ์์์ต๋๋ค. ๋ง์ง๋ง ๋จ๊ณ๋ก, ์ด๋ฏธ์ง๋ฅผ ๋ฐฐ์น๋ก ๋ง๋ค ์ฌ์ฉ์ ์ ์ `collate_fn`์ ์์ฑํฉ๋๋ค.
ํด๋น ๋ฐฐ์น์์ ๊ฐ์ฅ ํฐ ์ด๋ฏธ์ง์ ์ด๋ฏธ์ง(ํ์ฌ `pixel_values` ์ธ)๋ฅผ ํจ๋ํ๊ณ , ์ค์ ํฝ์
(1)๊ณผ ํจ๋ฉ(0)์ ๋ํ๋ด๊ธฐ ์ํด ๊ทธ์ ํด๋นํ๋ ์๋ก์ด `pixel_mask`๋ฅผ ์์ฑํด์ผ ํฉ๋๋ค.
```py
>>> def collate_fn(batch):
... pixel_values = [item["pixel_values"] for item in batch]
... encoding = image_processor.pad(pixel_values, return_tensors="pt")
... labels = [item["labels"] for item in batch]
... batch = {}
... batch["pixel_values"] = encoding["pixel_values"]
... batch["pixel_mask"] = encoding["pixel_mask"]
... batch["labels"] = labels
... return batch
```
## DETR ๋ชจ๋ธ ํ์ต์ํค๊ธฐ [[training-the-DETR-model]]
์ด์ ์น์
์์ ๋๋ถ๋ถ์ ์์
์ ์ํํ์ฌ ์ด์ ๋ชจ๋ธ์ ํ์ตํ ์ค๋น๊ฐ ๋์์ต๋๋ค!
์ด ๋ฐ์ดํฐ ์ธํธ์ ์ด๋ฏธ์ง๋ ๋ฆฌ์ฌ์ด์ฆ ํ์๋ ์ฌ์ ํ ์ฉ๋์ด ํฌ๊ธฐ ๋๋ฌธ์, ์ด ๋ชจ๋ธ์ ๋ฏธ์ธ ์กฐ์ ํ๋ ค๋ฉด ์ ์ด๋ ํ๋์ GPU๊ฐ ํ์ํฉ๋๋ค.
ํ์ต์ ๋ค์์ ๋จ๊ณ๋ฅผ ์ํํฉ๋๋ค:
1. [`AutoModelForObjectDetection`]์ ์ฌ์ฉํ์ฌ ์ ์ฒ๋ฆฌ์ ๋์ผํ ์ฒดํฌํฌ์ธํธ๋ฅผ ์ฌ์ฉํ์ฌ ๋ชจ๋ธ์ ๊ฐ์ ธ์ต๋๋ค.
2. [`TrainingArguments`]์์ ํ์ต ํ์ดํผํ๋ผ๋ฏธํฐ๋ฅผ ์ ์ํฉ๋๋ค.
3. ๋ชจ๋ธ, ๋ฐ์ดํฐ ์ธํธ, ์ด๋ฏธ์ง ํ๋ก์ธ์ ๋ฐ ๋ฐ์ดํฐ ์ฝ๋ ์ดํฐ์ ํจ๊ป [`Trainer`]์ ํ๋ จ ์ธ์๋ฅผ ์ ๋ฌํฉ๋๋ค.
4. [`~Trainer.train`]๋ฅผ ํธ์ถํ์ฌ ๋ชจ๋ธ์ ๋ฏธ์ธ ์กฐ์ ํฉ๋๋ค.
์ ์ฒ๋ฆฌ์ ์ฌ์ฉํ ์ฒดํฌํฌ์ธํธ์ ๋์ผํ ์ฒดํฌํฌ์ธํธ์์ ๋ชจ๋ธ์ ๊ฐ์ ธ์ฌ ๋, ๋ฐ์ดํฐ ์ธํธ์ ๋ฉํ๋ฐ์ดํฐ์์ ๋ง๋ `label2id`์ `id2label` ๋งคํ์ ์ ๋ฌํด์ผ ํฉ๋๋ค.
๋ํ, `ignore_mismatched_sizes=True`๋ฅผ ์ง์ ํ์ฌ ๊ธฐ์กด ๋ถ๋ฅ ํค๋(๋ชจ๋ธ์์ ๋ถ๋ฅ์ ์ฌ์ฉ๋๋ ๋ง์ง๋ง ๋ ์ด์ด)๋ฅผ ์ ๋ถ๋ฅ ํค๋๋ก ๋์ฒดํฉ๋๋ค.
```py
>>> from transformers import AutoModelForObjectDetection
>>> model = AutoModelForObjectDetection.from_pretrained(
... checkpoint,
... id2label=id2label,
... label2id=label2id,
... ignore_mismatched_sizes=True,
... )
```
[`TrainingArguments`]์์ `output_dir`์ ์ฌ์ฉํ์ฌ ๋ชจ๋ธ์ ์ ์ฅํ ์์น๋ฅผ ์ง์ ํ ๋ค์, ํ์์ ๋ฐ๋ผ ํ์ดํผํ๋ผ๋ฏธํฐ๋ฅผ ๊ตฌ์ฑํ์ธ์.
์ฌ์ฉํ์ง ์๋ ์ด์ ์ ๊ฑฐํ์ง ์๋๋ก ์ฃผ์ํด์ผ ํฉ๋๋ค. ๋ง์ฝ `remove_unused_columns`๊ฐ `True`์ผ ๊ฒฝ์ฐ ์ด๋ฏธ์ง ์ด์ด ์ญ์ ๋ฉ๋๋ค.
์ด๋ฏธ์ง ์ด์ด ์๋ ๊ฒฝ์ฐ `pixel_values`๋ฅผ ์์ฑํ ์ ์๊ธฐ ๋๋ฌธ์ `remove_unused_columns`๋ฅผ `False`๋ก ์ค์ ํด์ผ ํฉ๋๋ค.
๋ชจ๋ธ์ Hub์ ์
๋ก๋ํ์ฌ ๊ณต์ ํ๋ ค๋ฉด `push_to_hub`๋ฅผ `True`๋ก ์ค์ ํ์ญ์์ค(ํ๊น
ํ์ด์ค์ ๋ก๊ทธ์ธํ์ฌ ๋ชจ๋ธ์ ์
๋ก๋ํด์ผ ํฉ๋๋ค).
```py
>>> from transformers import TrainingArguments
>>> training_args = TrainingArguments(
... output_dir="detr-resnet-50_finetuned_cppe5",
... per_device_train_batch_size=8,
... num_train_epochs=10,
... fp16=True,
... save_steps=200,
... logging_steps=50,
... learning_rate=1e-5,
... weight_decay=1e-4,
... save_total_limit=2,
... remove_unused_columns=False,
... push_to_hub=True,
... )
```
๋ง์ง๋ง์ผ๋ก `model`, `training_args`, `collate_fn`, `image_processor`์ ๋ฐ์ดํฐ ์ธํธ(`cppe5`)๋ฅผ ๋ชจ๋ ๊ฐ์ ธ์จ ํ, [`~transformers.Trainer.train`]๋ฅผ ํธ์ถํฉ๋๋ค.
```py
>>> from transformers import Trainer
>>> trainer = Trainer(
... model=model,
... args=training_args,
... data_collator=collate_fn,
... train_dataset=cppe5["train"],
... tokenizer=image_processor,
... )
>>> trainer.train()
```
`training_args`์์ `push_to_hub`๋ฅผ `True`๋ก ์ค์ ํ ๊ฒฝ์ฐ, ํ์ต ์ฒดํฌํฌ์ธํธ๋ ํ๊น
ํ์ด์ค ํ๋ธ์ ์
๋ก๋๋ฉ๋๋ค.
ํ์ต ์๋ฃ ํ, [`~transformers.Trainer.push_to_hub`] ๋ฉ์๋๋ฅผ ํธ์ถํ์ฌ ์ต์ข
๋ชจ๋ธ์ ํ๊น
ํ์ด์ค ํ๋ธ์ ์
๋ก๋ํฉ๋๋ค.
```py
>>> trainer.push_to_hub()
```
## ํ๊ฐํ๊ธฐ [[evaluate]]
๊ฐ์ฒด ํ์ง ๋ชจ๋ธ์ ์ผ๋ฐ์ ์ผ๋ก ์ผ๋ จ์ <a href="https://cocodataset.org/#detection-eval">COCO-์คํ์ผ ์งํ</a>๋ก ํ๊ฐ๋ฉ๋๋ค.
๊ธฐ์กด์ ๊ตฌํ๋ ํ๊ฐ ์งํ ์ค ํ๋๋ฅผ ์ฌ์ฉํ ์๋ ์์ง๋ง, ์ฌ๊ธฐ์์๋ ํ๊น
ํ์ด์ค ํ๋ธ์ ํธ์ํ ์ต์ข
๋ชจ๋ธ์ ํ๊ฐํ๋ ๋ฐ `torchvision`์์ ์ ๊ณตํ๋ ํ๊ฐ ์งํ๋ฅผ ์ฌ์ฉํฉ๋๋ค.
`torchvision` ํ๊ฐ์(evaluator)๋ฅผ ์ฌ์ฉํ๋ ค๋ฉด ์ค์ธก๊ฐ์ธ COCO ๋ฐ์ดํฐ ์ธํธ๋ฅผ ์ค๋นํด์ผ ํฉ๋๋ค.
COCO ๋ฐ์ดํฐ ์ธํธ๋ฅผ ๋น๋ํ๋ API๋ ๋ฐ์ดํฐ๋ฅผ ํน์ ํ์์ผ๋ก ์ ์ฅํด์ผ ํ๋ฏ๋ก, ๋จผ์ ์ด๋ฏธ์ง์ ์ด๋
ธํ
์ด์
์ ๋์คํฌ์ ์ ์ฅํด์ผ ํฉ๋๋ค.
ํ์ต์ ์ํด ๋ฐ์ดํฐ๋ฅผ ์ค๋นํ ๋์ ๋ง์ฐฌ๊ฐ์ง๋ก, cppe5["test"]์์์ ์ด๋
ธํ
์ด์
์ ํฌ๋งท์ ๋ง์ถฐ์ผ ํฉ๋๋ค. ๊ทธ๋ฌ๋ ์ด๋ฏธ์ง๋ ๊ทธ๋๋ก ์ ์งํด์ผ ํฉ๋๋ค.
ํ๊ฐ ๋จ๊ณ๋ ์ฝ๊ฐ์ ์์
์ด ํ์ํ์ง๋ง, ํฌ๊ฒ ์ธ ๊ฐ์ง ์ฃผ์ ๋จ๊ณ๋ก ๋๋ ์ ์์ต๋๋ค.
๋จผ์ , `cppe5["test"]` ์ธํธ๋ฅผ ์ค๋นํฉ๋๋ค: ์ด๋
ธํ
์ด์
์ ํฌ๋งท์ ๋ง๊ฒ ๋ง๋ค๊ณ ๋ฐ์ดํฐ๋ฅผ ๋์คํฌ์ ์ ์ฅํฉ๋๋ค.
```py
>>> import json
>>> # format annotations the same as for training, no need for data augmentation
>>> def val_formatted_anns(image_id, objects):
... annotations = []
... for i in range(0, len(objects["id"])):
... new_ann = {
... "id": objects["id"][i],
... "category_id": objects["category"][i],
... "iscrowd": 0,
... "image_id": image_id,
... "area": objects["area"][i],
... "bbox": objects["bbox"][i],
... }
... annotations.append(new_ann)
... return annotations
>>> # Save images and annotations into the files torchvision.datasets.CocoDetection expects
>>> def save_cppe5_annotation_file_images(cppe5):
... output_json = {}
... path_output_cppe5 = f"{os.getcwd()}/cppe5/"
... if not os.path.exists(path_output_cppe5):
... os.makedirs(path_output_cppe5)
... path_anno = os.path.join(path_output_cppe5, "cppe5_ann.json")
... categories_json = [{"supercategory": "none", "id": id, "name": id2label[id]} for id in id2label]
... output_json["images"] = []
... output_json["annotations"] = []
... for example in cppe5:
... ann = val_formatted_anns(example["image_id"], example["objects"])
... output_json["images"].append(
... {
... "id": example["image_id"],
... "width": example["image"].width,
... "height": example["image"].height,
... "file_name": f"{example['image_id']}.png",
... }
... )
... output_json["annotations"].extend(ann)
... output_json["categories"] = categories_json
... with open(path_anno, "w") as file:
... json.dump(output_json, file, ensure_ascii=False, indent=4)
... for im, img_id in zip(cppe5["image"], cppe5["image_id"]):
... path_img = os.path.join(path_output_cppe5, f"{img_id}.png")
... im.save(path_img)
... return path_output_cppe5, path_anno
```
๋ค์์ผ๋ก, `cocoevaluator`์ ํจ๊ป ์ฌ์ฉํ ์ ์๋ `CocoDetection` ํด๋์ค์ ์ธ์คํด์ค๋ฅผ ์ค๋นํฉ๋๋ค.
```py
>>> import torchvision
>>> class CocoDetection(torchvision.datasets.CocoDetection):
... def __init__(self, img_folder, image_processor, ann_file):
... super().__init__(img_folder, ann_file)
... self.image_processor = image_processor
... def __getitem__(self, idx):
... # read in PIL image and target in COCO format
... img, target = super(CocoDetection, self).__getitem__(idx)
... # preprocess image and target: converting target to DETR format,
... # resizing + normalization of both image and target)
... image_id = self.ids[idx]
... target = {"image_id": image_id, "annotations": target}
... encoding = self.image_processor(images=img, annotations=target, return_tensors="pt")
... pixel_values = encoding["pixel_values"].squeeze() # remove batch dimension
... target = encoding["labels"][0] # remove batch dimension
... return {"pixel_values": pixel_values, "labels": target}
>>> im_processor = AutoImageProcessor.from_pretrained("devonho/detr-resnet-50_finetuned_cppe5")
>>> path_output_cppe5, path_anno = save_cppe5_annotation_file_images(cppe5["test"])
>>> test_ds_coco_format = CocoDetection(path_output_cppe5, im_processor, path_anno)
```
๋ง์ง๋ง์ผ๋ก, ํ๊ฐ ์งํ๋ฅผ ๊ฐ์ ธ์์ ํ๊ฐ๋ฅผ ์คํํฉ๋๋ค.
```py
>>> import evaluate
>>> from tqdm import tqdm
>>> model = AutoModelForObjectDetection.from_pretrained("devonho/detr-resnet-50_finetuned_cppe5")
>>> module = evaluate.load("ybelkada/cocoevaluate", coco=test_ds_coco_format.coco)
>>> val_dataloader = torch.utils.data.DataLoader(
... test_ds_coco_format, batch_size=8, shuffle=False, num_workers=4, collate_fn=collate_fn
... )
>>> with torch.no_grad():
... for idx, batch in enumerate(tqdm(val_dataloader)):
... pixel_values = batch["pixel_values"]
... pixel_mask = batch["pixel_mask"]
... labels = [
... {k: v for k, v in t.items()} for t in batch["labels"]
... ] # these are in DETR format, resized + normalized
... # forward pass
... outputs = model(pixel_values=pixel_values, pixel_mask=pixel_mask)
... orig_target_sizes = torch.stack([target["orig_size"] for target in labels], dim=0)
... results = im_processor.post_process(outputs, orig_target_sizes) # convert outputs of model to COCO api
... module.add(prediction=results, reference=labels)
... del batch
>>> results = module.compute()
>>> print(results)
Accumulating evaluation results...
DONE (t=0.08s).
IoU metric: bbox
Average Precision (AP) @[ IoU=0.50:0.95 | area= all | maxDets=100 ] = 0.352
Average Precision (AP) @[ IoU=0.50 | area= all | maxDets=100 ] = 0.681
Average Precision (AP) @[ IoU=0.75 | area= all | maxDets=100 ] = 0.292
Average Precision (AP) @[ IoU=0.50:0.95 | area= small | maxDets=100 ] = 0.168
Average Precision (AP) @[ IoU=0.50:0.95 | area=medium | maxDets=100 ] = 0.208
Average Precision (AP) @[ IoU=0.50:0.95 | area= large | maxDets=100 ] = 0.429
Average Recall (AR) @[ IoU=0.50:0.95 | area= all | maxDets= 1 ] = 0.274
Average Recall (AR) @[ IoU=0.50:0.95 | area= all | maxDets= 10 ] = 0.484
Average Recall (AR) @[ IoU=0.50:0.95 | area= all | maxDets=100 ] = 0.501
Average Recall (AR) @[ IoU=0.50:0.95 | area= small | maxDets=100 ] = 0.191
Average Recall (AR) @[ IoU=0.50:0.95 | area=medium | maxDets=100 ] = 0.323
Average Recall (AR) @[ IoU=0.50:0.95 | area= large | maxDets=100 ] = 0.590
```
์ด๋ฌํ ๊ฒฐ๊ณผ๋ [`~transformers.TrainingArguments`]์ ํ์ดํผํ๋ผ๋ฏธํฐ๋ฅผ ์กฐ์ ํ์ฌ ๋์ฑ ๊ฐ์ ๋ ์ ์์ต๋๋ค. ํ๋ฒ ์๋ํด ๋ณด์ธ์!
## ์ถ๋ก ํ๊ธฐ [[inference]]
DETR ๋ชจ๋ธ์ ๋ฏธ์ธ ์กฐ์ ๋ฐ ํ๊ฐํ๊ณ , ํ๊น
ํ์ด์ค ํ๋ธ์ ์
๋ก๋ ํ์ผ๋ฏ๋ก ์ถ๋ก ์ ์ฌ์ฉํ ์ ์์ต๋๋ค.
๋ฏธ์ธ ์กฐ์ ๋ ๋ชจ๋ธ์ ์ถ๋ก ์ ์ฌ์ฉํ๋ ๊ฐ์ฅ ๊ฐ๋จํ ๋ฐฉ๋ฒ์ [`pipeline`]์์ ๋ชจ๋ธ์ ์ฌ์ฉํ๋ ๊ฒ์
๋๋ค.
๋ชจ๋ธ๊ณผ ํจ๊ป ๊ฐ์ฒด ํ์ง๋ฅผ ์ํ ํ์ดํ๋ผ์ธ์ ์ธ์คํด์คํํ๊ณ , ์ด๋ฏธ์ง๋ฅผ ์ ๋ฌํ์ธ์:
```py
>>> from transformers import pipeline
>>> import requests
>>> url = "https://i.imgur.com/2lnWoly.jpg"
>>> image = Image.open(requests.get(url, stream=True).raw)
>>> obj_detector = pipeline("object-detection", model="devonho/detr-resnet-50_finetuned_cppe5")
>>> obj_detector(image)
```
๋ง์ฝ ์ํ๋ค๋ฉด ์๋์ผ๋ก `pipeline`์ ๊ฒฐ๊ณผ๋ฅผ ์ฌํํ ์ ์์ต๋๋ค:
```py
>>> image_processor = AutoImageProcessor.from_pretrained("devonho/detr-resnet-50_finetuned_cppe5")
>>> model = AutoModelForObjectDetection.from_pretrained("devonho/detr-resnet-50_finetuned_cppe5")
>>> with torch.no_grad():
... inputs = image_processor(images=image, return_tensors="pt")
... outputs = model(**inputs)
... target_sizes = torch.tensor([image.size[::-1]])
... results = image_processor.post_process_object_detection(outputs, threshold=0.5, target_sizes=target_sizes)[0]
>>> for score, label, box in zip(results["scores"], results["labels"], results["boxes"]):
... box = [round(i, 2) for i in box.tolist()]
... print(
... f"Detected {model.config.id2label[label.item()]} with confidence "
... f"{round(score.item(), 3)} at location {box}"
... )
Detected Coverall with confidence 0.566 at location [1215.32, 147.38, 4401.81, 3227.08]
Detected Mask with confidence 0.584 at location [2449.06, 823.19, 3256.43, 1413.9]
```
๊ฒฐ๊ณผ๋ฅผ ์๊ฐํํ๊ฒ ์ต๋๋ค:
```py
>>> draw = ImageDraw.Draw(image)
>>> for score, label, box in zip(results["scores"], results["labels"], results["boxes"]):
... box = [round(i, 2) for i in box.tolist()]
... x, y, x2, y2 = tuple(box)
... draw.rectangle((x, y, x2, y2), outline="red", width=1)
... draw.text((x, y), model.config.id2label[label.item()], fill="white")
>>> image
```
<div class="flex justify-center">
<img src="https://i.imgur.com/4QZnf9A.png" alt="Object detection result on a new image"/>
</div>
| 0 |
hf_public_repos/transformers/docs/source/ko | hf_public_repos/transformers/docs/source/ko/tasks/image_classification.md | <!--Copyright 2022 The HuggingFace Team. All rights reserved.
Licensed under the Apache License, Version 2.0 (the "License"); you may not use this file except in compliance with
the License. You may obtain a copy of the License at
http://www.apache.org/licenses/LICENSE-2.0
Unless required by applicable law or agreed to in writing, software distributed under the License is distributed on
an "AS IS" BASIS, WITHOUT WARRANTIES OR CONDITIONS OF ANY KIND, either express or implied. See the License for the
specific language governing permissions and limitations under the License.
โ ๏ธ Note that this file is in Markdown but contain specific syntax for our doc-builder (similar to MDX) that may not be
rendered properly in your Markdown viewer.
-->
# ์ด๋ฏธ์ง ๋ถ๋ฅ[[image-classification]]
[[open-in-colab]]
<Youtube id="tjAIM7BOYhw"/>
์ด๋ฏธ์ง ๋ถ๋ฅ๋ ์ด๋ฏธ์ง์ ๋ ์ด๋ธ ๋๋ ํด๋์ค๋ฅผ ํ ๋นํฉ๋๋ค. ํ
์คํธ ๋๋ ์ค๋์ค ๋ถ๋ฅ์ ๋ฌ๋ฆฌ ์
๋ ฅ์
์ด๋ฏธ์ง๋ฅผ ๊ตฌ์ฑํ๋ ํฝ์
๊ฐ์
๋๋ค. ์ด๋ฏธ์ง ๋ถ๋ฅ์๋ ์์ฐ์ฌํด ํ ํผํด ๊ฐ์ง, ๋์๋ฌผ ๊ฑด๊ฐ ๋ชจ๋ํฐ๋ง, ์๋ฃ ์ด๋ฏธ์ง์์ ์ง๋ณ์ ์งํ ๊ฒ์ฌ ์ง์ ๋ฑ
๋ค์ํ ์์ฉ ์ฌ๋ก๊ฐ ์์ต๋๋ค.
์ด ๊ฐ์ด๋์์๋ ๋ค์์ ์ค๋ช
ํฉ๋๋ค:
1. [Food-101](https://huggingface.co/datasets/food101) ๋ฐ์ดํฐ ์ธํธ์์ [ViT](model_doc/vit)๋ฅผ ๋ฏธ์ธ ์กฐ์ ํ์ฌ ์ด๋ฏธ์ง์์ ์ํ ํญ๋ชฉ์ ๋ถ๋ฅํฉ๋๋ค.
2. ์ถ๋ก ์ ์ํด ๋ฏธ์ธ ์กฐ์ ๋ชจ๋ธ์ ์ฌ์ฉํฉ๋๋ค.
<Tip>
์ด ํํ ๋ฆฌ์ผ์์ ์ค๋ช
ํ๋ ์์
์ ๋ค์ ๋ชจ๋ธ ์ํคํ
์ฒ์ ์ํด ์ง์๋ฉ๋๋ค:
<!--This tip is automatically generated by `make fix-copies`, do not fill manually!-->
[BEiT](../model_doc/beit), [BiT](../model_doc/bit), [ConvNeXT](../model_doc/convnext), [ConvNeXTV2](../model_doc/convnextv2), [CvT](../model_doc/cvt), [Data2VecVision](../model_doc/data2vec-vision), [DeiT](../model_doc/deit), [DiNAT](../model_doc/dinat), [EfficientFormer](../model_doc/efficientformer), [EfficientNet](../model_doc/efficientnet), [FocalNet](../model_doc/focalnet), [ImageGPT](../model_doc/imagegpt), [LeViT](../model_doc/levit), [MobileNetV1](../model_doc/mobilenet_v1), [MobileNetV2](../model_doc/mobilenet_v2), [MobileViT](../model_doc/mobilevit), [NAT](../model_doc/nat), [Perceiver](../model_doc/perceiver), [PoolFormer](../model_doc/poolformer), [RegNet](../model_doc/regnet), [ResNet](../model_doc/resnet), [SegFormer](../model_doc/segformer), [Swin Transformer](../model_doc/swin), [Swin Transformer V2](../model_doc/swinv2), [VAN](../model_doc/van), [ViT](../model_doc/vit), [ViT Hybrid](../model_doc/vit_hybrid), [ViTMSN](../model_doc/vit_msn)
<!--End of the generated tip-->
</Tip>
์์ํ๊ธฐ ์ ์, ํ์ํ ๋ชจ๋ ๋ผ์ด๋ธ๋ฌ๋ฆฌ๊ฐ ์ค์น๋์ด ์๋์ง ํ์ธํ์ธ์:
```bash
pip install transformers datasets evaluate
```
Hugging Face ๊ณ์ ์ ๋ก๊ทธ์ธํ์ฌ ๋ชจ๋ธ์ ์
๋ก๋ํ๊ณ ์ปค๋ฎค๋ํฐ์ ๊ณต์ ํ๋ ๊ฒ์ ๊ถ์ฅํฉ๋๋ค. ๋ฉ์์ง๊ฐ ํ์๋๋ฉด, ํ ํฐ์ ์
๋ ฅํ์ฌ ๋ก๊ทธ์ธํ์ธ์:
```py
>>> from huggingface_hub import notebook_login
>>> notebook_login()
```
## Food-101 ๋ฐ์ดํฐ ์ธํธ ๊ฐ์ ธ์ค๊ธฐ[[load-food101-dataset]]
๐ค Datasets ๋ผ์ด๋ธ๋ฌ๋ฆฌ์์ Food-101 ๋ฐ์ดํฐ ์ธํธ์ ๋ ์์ ๋ถ๋ถ ์งํฉ์ ๊ฐ์ ธ์ค๋ ๊ฒ์ผ๋ก ์์ํฉ๋๋ค. ์ด๋ ๊ฒ ํ๋ฉด ์ ์ฒด ๋ฐ์ดํฐ ์ธํธ์ ๋ํ
ํ๋ จ์ ๋ง์ ์๊ฐ์ ํ ์ ํ๊ธฐ ์ ์ ์คํ์ ํตํด ๋ชจ๋ ๊ฒ์ด ์ ๋๋ก ์๋ํ๋์ง ํ์ธํ ์ ์์ต๋๋ค.
```py
>>> from datasets import load_dataset
>>> food = load_dataset("food101", split="train[:5000]")
```
๋ฐ์ดํฐ ์ธํธ์ `train`์ [`~datasets.Dataset.train_test_split`] ๋ฉ์๋๋ฅผ ์ฌ์ฉํ์ฌ ํ๋ จ ๋ฐ ํ
์คํธ ์ธํธ๋ก ๋ถํ ํ์ธ์:
```py
>>> food = food.train_test_split(test_size=0.2)
```
๊ทธ๋ฆฌ๊ณ ์์๋ฅผ ์ดํด๋ณด์ธ์:
```py
>>> food["train"][0]
{'image': <PIL.JpegImagePlugin.JpegImageFile image mode=RGB size=512x512 at 0x7F52AFC8AC50>,
'label': 79}
```
๋ฐ์ดํฐ ์ธํธ์ ๊ฐ ์์ ์๋ ๋ ๊ฐ์ ํ๋๊ฐ ์์ต๋๋ค:
- `image`: ์ํ ํญ๋ชฉ์ PIL ์ด๋ฏธ์ง
- `label`: ์ํ ํญ๋ชฉ์ ๋ ์ด๋ธ ํด๋์ค
๋ชจ๋ธ์ด ๋ ์ด๋ธ ID์์ ๋ ์ด๋ธ ์ด๋ฆ์ ์ฝ๊ฒ ๊ฐ์ ธ์ฌ ์ ์๋๋ก
๋ ์ด๋ธ ์ด๋ฆ์ ์ ์๋ก ๋งคํํ๊ณ , ์ ์๋ฅผ ๋ ์ด๋ธ ์ด๋ฆ์ผ๋ก ๋งคํํ๋ ์ฌ์ ์ ๋ง๋์ธ์:
```py
>>> labels = food["train"].features["label"].names
>>> label2id, id2label = dict(), dict()
>>> for i, label in enumerate(labels):
... label2id[label] = str(i)
... id2label[str(i)] = label
```
์ด์ ๋ ์ด๋ธ ID๋ฅผ ๋ ์ด๋ธ ์ด๋ฆ์ผ๋ก ๋ณํํ ์ ์์ต๋๋ค:
```py
>>> id2label[str(79)]
'prime_rib'
```
## ์ ์ฒ๋ฆฌ[[preprocess]]
๋ค์ ๋จ๊ณ๋ ์ด๋ฏธ์ง๋ฅผ ํ
์๋ก ์ฒ๋ฆฌํ๊ธฐ ์ํด ViT ์ด๋ฏธ์ง ํ๋ก์ธ์๋ฅผ ๊ฐ์ ธ์ค๋ ๊ฒ์
๋๋ค:
```py
>>> from transformers import AutoImageProcessor
>>> checkpoint = "google/vit-base-patch16-224-in21k"
>>> image_processor = AutoImageProcessor.from_pretrained(checkpoint)
```
<frameworkcontent>
<pt>
์ด๋ฏธ์ง์ ๋ช ๊ฐ์ง ์ด๋ฏธ์ง ๋ณํ์ ์ ์ฉํ์ฌ ๊ณผ์ ํฉ์ ๋ํด ๋ชจ๋ธ์ ๋ ๊ฒฌ๊ณ ํ๊ฒ ๋ง๋ญ๋๋ค. ์ฌ๊ธฐ์ Torchvision์ [`transforms`](https://pytorch.org/vision/stable/transforms.html) ๋ชจ๋์ ์ฌ์ฉํ์ง๋ง, ์ํ๋ ์ด๋ฏธ์ง ๋ผ์ด๋ธ๋ฌ๋ฆฌ๋ฅผ ์ฌ์ฉํ ์๋ ์์ต๋๋ค.
์ด๋ฏธ์ง์ ์์ ๋ถ๋ถ์ ํฌ๋กญํ๊ณ ํฌ๊ธฐ๋ฅผ ์กฐ์ ํ ๋ค์, ์ด๋ฏธ์ง ํ๊ท ๊ณผ ํ์ค ํธ์ฐจ๋ก ์ ๊ทํํ์ธ์:
```py
>>> from torchvision.transforms import RandomResizedCrop, Compose, Normalize, ToTensor
>>> normalize = Normalize(mean=image_processor.image_mean, std=image_processor.image_std)
>>> size = (
... image_processor.size["shortest_edge"]
... if "shortest_edge" in image_processor.size
... else (image_processor.size["height"], image_processor.size["width"])
... )
>>> _transforms = Compose([RandomResizedCrop(size), ToTensor(), normalize])
```
๊ทธ๋ฐ ๋ค์ ์ ์ฒ๋ฆฌ ํจ์๋ฅผ ๋ง๋ค์ด ๋ณํ์ ์ ์ฉํ๊ณ ์ด๋ฏธ์ง์ `pixel_values`(๋ชจ๋ธ์ ๋ํ ์
๋ ฅ)๋ฅผ ๋ฐํํ์ธ์:
```py
>>> def transforms(examples):
... examples["pixel_values"] = [_transforms(img.convert("RGB")) for img in examples["image"]]
... del examples["image"]
... return examples
```
์ ์ฒด ๋ฐ์ดํฐ ์ธํธ์ ์ ์ฒ๋ฆฌ ๊ธฐ๋ฅ์ ์ ์ฉํ๋ ค๋ฉด ๐ค Datasets [`~datasets.Dataset.with_transform`]์ ์ฌ์ฉํฉ๋๋ค. ๋ฐ์ดํฐ ์ธํธ์ ์์๋ฅผ ๊ฐ์ ธ์ฌ ๋ ๋ณํ์ด ์ฆ์ ์ ์ฉ๋ฉ๋๋ค:
```py
>>> food = food.with_transform(transforms)
```
์ด์ [`DefaultDataCollator`]๋ฅผ ์ฌ์ฉํ์ฌ ์์ ๋ฐฐ์น๋ฅผ ๋ง๋ญ๋๋ค. ๐ค Transformers์ ๋ค๋ฅธ ๋ฐ์ดํฐ ์ฝ๋ ์ดํฐ์ ๋ฌ๋ฆฌ, `DefaultDataCollator`๋ ํจ๋ฉ๊ณผ ๊ฐ์ ์ถ๊ฐ์ ์ธ ์ ์ฒ๋ฆฌ๋ฅผ ์ ์ฉํ์ง ์์ต๋๋ค.
```py
>>> from transformers import DefaultDataCollator
>>> data_collator = DefaultDataCollator()
```
</pt>
</frameworkcontent>
<frameworkcontent>
<tf>
๊ณผ์ ํฉ์ ๋ฐฉ์งํ๊ณ ๋ชจ๋ธ์ ๋ณด๋ค ๊ฒฌ๊ณ ํ๊ฒ ๋ง๋ค๊ธฐ ์ํด ๋ฐ์ดํฐ ์ธํธ์ ํ๋ จ ๋ถ๋ถ์ ๋ฐ์ดํฐ ์ฆ๊ฐ์ ์ถ๊ฐํฉ๋๋ค.
์ฌ๊ธฐ์ Keras ์ ์ฒ๋ฆฌ ๋ ์ด์ด๋ก ํ๋ จ ๋ฐ์ดํฐ์ ๋ํ ๋ณํ(๋ฐ์ดํฐ ์ฆ๊ฐ ํฌํจ)๊ณผ
๊ฒ์ฆ ๋ฐ์ดํฐ์ ๋ํ ๋ณํ(์ค์ ํฌ๋กํ, ํฌ๊ธฐ ์กฐ์ , ์ ๊ทํ๋ง)์ ์ ์ํฉ๋๋ค.
`tf.image` ๋๋ ๋ค๋ฅธ ์ํ๋ ๋ผ์ด๋ธ๋ฌ๋ฆฌ๋ฅผ ์ฌ์ฉํ ์ ์์ต๋๋ค.
```py
>>> from tensorflow import keras
>>> from tensorflow.keras import layers
>>> size = (image_processor.size["height"], image_processor.size["width"])
>>> train_data_augmentation = keras.Sequential(
... [
... layers.RandomCrop(size[0], size[1]),
... layers.Rescaling(scale=1.0 / 127.5, offset=-1),
... layers.RandomFlip("horizontal"),
... layers.RandomRotation(factor=0.02),
... layers.RandomZoom(height_factor=0.2, width_factor=0.2),
... ],
... name="train_data_augmentation",
... )
>>> val_data_augmentation = keras.Sequential(
... [
... layers.CenterCrop(size[0], size[1]),
... layers.Rescaling(scale=1.0 / 127.5, offset=-1),
... ],
... name="val_data_augmentation",
... )
```
๋ค์์ผ๋ก ํ ๋ฒ์ ํ๋์ ์ด๋ฏธ์ง๊ฐ ์๋๋ผ ์ด๋ฏธ์ง ๋ฐฐ์น์ ์ ์ ํ ๋ณํ์ ์ ์ฉํ๋ ํจ์๋ฅผ ๋ง๋ญ๋๋ค.
```py
>>> import numpy as np
>>> import tensorflow as tf
>>> from PIL import Image
>>> def convert_to_tf_tensor(image: Image):
... np_image = np.array(image)
... tf_image = tf.convert_to_tensor(np_image)
... # `expand_dims()` is used to add a batch dimension since
... # the TF augmentation layers operates on batched inputs.
... return tf.expand_dims(tf_image, 0)
>>> def preprocess_train(example_batch):
... """Apply train_transforms across a batch."""
... images = [
... train_data_augmentation(convert_to_tf_tensor(image.convert("RGB"))) for image in example_batch["image"]
... ]
... example_batch["pixel_values"] = [tf.transpose(tf.squeeze(image)) for image in images]
... return example_batch
... def preprocess_val(example_batch):
... """Apply val_transforms across a batch."""
... images = [
... val_data_augmentation(convert_to_tf_tensor(image.convert("RGB"))) for image in example_batch["image"]
... ]
... example_batch["pixel_values"] = [tf.transpose(tf.squeeze(image)) for image in images]
... return example_batch
```
๐ค Datasets [`~datasets.Dataset.set_transform`]๋ฅผ ์ฌ์ฉํ์ฌ ์ฆ์ ๋ณํ์ ์ ์ฉํ์ธ์:
```py
food["train"].set_transform(preprocess_train)
food["test"].set_transform(preprocess_val)
```
์ต์ข
์ ์ฒ๋ฆฌ ๋จ๊ณ๋ก `DefaultDataCollator`๋ฅผ ์ฌ์ฉํ์ฌ ์์ ๋ฐฐ์น๋ฅผ ๋ง๋ญ๋๋ค. ๐ค Transformers์ ๋ค๋ฅธ ๋ฐ์ดํฐ ์ฝ๋ ์ดํฐ์ ๋ฌ๋ฆฌ
`DefaultDataCollator`๋ ํจ๋ฉ๊ณผ ๊ฐ์ ์ถ๊ฐ ์ ์ฒ๋ฆฌ๋ฅผ ์ ์ฉํ์ง ์์ต๋๋ค.
```py
>>> from transformers import DefaultDataCollator
>>> data_collator = DefaultDataCollator(return_tensors="tf")
```
</tf>
</frameworkcontent>
## ํ๊ฐ[[evaluate]]
ํ๋ จ ์ค์ ํ๊ฐ ์งํ๋ฅผ ํฌํจํ๋ฉด ๋ชจ๋ธ์ ์ฑ๋ฅ์ ํ๊ฐํ๋ ๋ฐ ๋์์ด ๋๋ ๊ฒฝ์ฐ๊ฐ ๋ง์ต๋๋ค.
๐ค [Evaluate](https://huggingface.co/docs/evaluate/index) ๋ผ์ด๋ธ๋ฌ๋ฆฌ๋ก ํ๊ฐ ๋ฐฉ๋ฒ์ ๋น ๋ฅด๊ฒ ๊ฐ์ ธ์ฌ ์ ์์ต๋๋ค. ์ด ์์
์์๋
[accuracy](https://huggingface.co/spaces/evaluate-metric/accuracy) ํ๊ฐ ์งํ๋ฅผ ๊ฐ์ ธ์ต๋๋ค. (๐ค Evaluate [๋น ๋ฅธ ๋๋ฌ๋ณด๊ธฐ](https://huggingface.co/docs/evaluate/a_quick_tour)๋ฅผ ์ฐธ์กฐํ์ฌ ํ๊ฐ ์งํ๋ฅผ ๊ฐ์ ธ์ค๊ณ ๊ณ์ฐํ๋ ๋ฐฉ๋ฒ์ ๋ํด ์์ธํ ์์๋ณด์ธ์):
```py
>>> import evaluate
>>> accuracy = evaluate.load("accuracy")
```
๊ทธ๋ฐ ๋ค์ ์์ธก๊ณผ ๋ ์ด๋ธ์ [`~evaluate.EvaluationModule.compute`]์ ์ ๋ฌํ์ฌ ์ ํ๋๋ฅผ ๊ณ์ฐํ๋ ํจ์๋ฅผ ๋ง๋ญ๋๋ค:
```py
>>> import numpy as np
>>> def compute_metrics(eval_pred):
... predictions, labels = eval_pred
... predictions = np.argmax(predictions, axis=1)
... return accuracy.compute(predictions=predictions, references=labels)
```
์ด์ `compute_metrics` ํจ์๋ฅผ ์ฌ์ฉํ ์ค๋น๊ฐ ๋์์ผ๋ฉฐ, ํ๋ จ์ ์ค์ ํ๋ฉด ์ด ํจ์๋ก ๋๋์์ฌ ๊ฒ์
๋๋ค.
## ํ๋ จ[[train]]
<frameworkcontent>
<pt>
<Tip>
[`Trainer`]๋ฅผ ์ฌ์ฉํ์ฌ ๋ชจ๋ธ์ ๋ฏธ์ธ ์กฐ์ ํ๋ ๋ฐฉ๋ฒ์ ์ต์ํ์ง ์์ ๊ฒฝ์ฐ, [์ฌ๊ธฐ](../training#train-with-pytorch-trainer)์์ ๊ธฐ๋ณธ ํํ ๋ฆฌ์ผ์ ํ์ธํ์ธ์!
</Tip>
์ด์ ๋ชจ๋ธ์ ํ๋ จ์ํฌ ์ค๋น๊ฐ ๋์์ต๋๋ค! [`AutoModelForImageClassification`]๋ก ViT๋ฅผ ๊ฐ์ ธ์ต๋๋ค. ์์๋๋ ๋ ์ด๋ธ ์, ๋ ์ด๋ธ ๋งคํ ๋ฐ ๋ ์ด๋ธ ์๋ฅผ ์ง์ ํ์ธ์:
```py
>>> from transformers import AutoModelForImageClassification, TrainingArguments, Trainer
>>> model = AutoModelForImageClassification.from_pretrained(
... checkpoint,
... num_labels=len(labels),
... id2label=id2label,
... label2id=label2id,
... )
```
์ด์ ์ธ ๋จ๊ณ๋ง ๊ฑฐ์น๋ฉด ๋์
๋๋ค:
1. [`TrainingArguments`]์์ ํ๋ จ ํ์ดํผํ๋ผ๋ฏธํฐ๋ฅผ ์ ์ํ์ธ์. `image` ์ด์ด ์ญ์ ๋๊ธฐ ๋๋ฌธ์ ๋ฏธ์ฌ์ฉ ์ด์ ์ ๊ฑฐํ์ง ์๋ ๊ฒ์ด ์ค์ํฉ๋๋ค. `image` ์ด์ด ์์ผ๋ฉด `pixel_values`์ ์์ฑํ ์ ์์ต๋๋ค. ์ด ๋์์ ๋ฐฉ์งํ๋ ค๋ฉด `remove_unused_columns=False`๋ก ์ค์ ํ์ธ์! ๋ค๋ฅธ ์ ์ผํ ํ์ ๋งค๊ฐ๋ณ์๋ ๋ชจ๋ธ ์ ์ฅ ์์น๋ฅผ ์ง์ ํ๋ `output_dir`์
๋๋ค. `push_to_hub=True`๋ก ์ค์ ํ๋ฉด ์ด ๋ชจ๋ธ์ ํ๋ธ์ ํธ์ํฉ๋๋ค(๋ชจ๋ธ์ ์
๋ก๋ํ๋ ค๋ฉด Hugging Face์ ๋ก๊ทธ์ธํด์ผ ํฉ๋๋ค). ๊ฐ ์ํญ์ด ๋๋ ๋๋ง๋ค, [`Trainer`]๊ฐ ์ ํ๋๋ฅผ ํ๊ฐํ๊ณ ํ๋ จ ์ฒดํฌํฌ์ธํธ๋ฅผ ์ ์ฅํฉ๋๋ค.
2. [`Trainer`]์ ๋ชจ๋ธ, ๋ฐ์ดํฐ ์ธํธ, ํ ํฌ๋์ด์ , ๋ฐ์ดํฐ ์ฝ๋ ์ดํฐ ๋ฐ `compute_metrics` ํจ์์ ํจ๊ป ํ๋ จ ์ธ์๋ฅผ ์ ๋ฌํ์ธ์.
3. [`~Trainer.train`]์ ํธ์ถํ์ฌ ๋ชจ๋ธ์ ๋ฏธ์ธ ์กฐ์ ํ์ธ์.
```py
>>> training_args = TrainingArguments(
... output_dir="my_awesome_food_model",
... remove_unused_columns=False,
... evaluation_strategy="epoch",
... save_strategy="epoch",
... learning_rate=5e-5,
... per_device_train_batch_size=16,
... gradient_accumulation_steps=4,
... per_device_eval_batch_size=16,
... num_train_epochs=3,
... warmup_ratio=0.1,
... logging_steps=10,
... load_best_model_at_end=True,
... metric_for_best_model="accuracy",
... push_to_hub=True,
... )
>>> trainer = Trainer(
... model=model,
... args=training_args,
... data_collator=data_collator,
... train_dataset=food["train"],
... eval_dataset=food["test"],
... tokenizer=image_processor,
... compute_metrics=compute_metrics,
... )
>>> trainer.train()
```
ํ๋ จ์ด ์๋ฃ๋๋ฉด, ๋ชจ๋ ์ฌ๋์ด ๋ชจ๋ธ์ ์ฌ์ฉํ ์ ์๋๋ก [`~transformers.Trainer.push_to_hub`] ๋ฉ์๋๋ก ๋ชจ๋ธ์ ํ๋ธ์ ๊ณต์ ํ์ธ์:
```py
>>> trainer.push_to_hub()
```
</pt>
</frameworkcontent>
<frameworkcontent>
<tf>
<Tip>
Keras๋ฅผ ์ฌ์ฉํ์ฌ ๋ชจ๋ธ์ ๋ฏธ์ธ ์กฐ์ ํ๋ ๋ฐฉ๋ฒ์ ์ต์ํ์ง ์์ ๊ฒฝ์ฐ, ๋จผ์ [๊ธฐ๋ณธ ํํ ๋ฆฌ์ผ](./training#train-a-tensorflow-model-with-keras)์ ํ์ธํ์ธ์!
</Tip>
TensorFlow์์ ๋ชจ๋ธ์ ๋ฏธ์ธ ์กฐ์ ํ๋ ค๋ฉด ๋ค์ ๋จ๊ณ๋ฅผ ๋ฐ๋ฅด์ธ์:
1. ํ๋ จ ํ์ดํผํ๋ผ๋ฏธํฐ๋ฅผ ์ ์ํ๊ณ ์ตํฐ๋ง์ด์ ์ ํ์ต๋ฅ ์ค์ผ์ฅด์ ์ค์ ํฉ๋๋ค.
2. ์ฌ์ ํ๋ จ๋ ๋ชจ๋ธ์ ์ธ์คํด์คํํฉ๋๋ค.
3. ๐ค Dataset์ `tf.data.Dataset`์ผ๋ก ๋ณํํฉ๋๋ค.
4. ๋ชจ๋ธ์ ์ปดํ์ผํฉ๋๋ค.
5. ์ฝ๋ฐฑ์ ์ถ๊ฐํ๊ณ ํ๋ จ์ ์ํํ๊ธฐ ์ํด `fit()` ๋ฉ์๋๋ฅผ ์ฌ์ฉํฉ๋๋ค.
6. ์ปค๋ฎค๋ํฐ์ ๊ณต์ ํ๊ธฐ ์ํด ๋ชจ๋ธ์ ๐ค Hub์ ์
๋ก๋ํฉ๋๋ค.
ํ์ดํผํ๋ผ๋ฏธํฐ, ์ตํฐ๋ง์ด์ ๋ฐ ํ์ต๋ฅ ์ค์ผ์ฅด์ ์ ์ํ๋ ๊ฒ์ผ๋ก ์์ํฉ๋๋ค:
```py
>>> from transformers import create_optimizer
>>> batch_size = 16
>>> num_epochs = 5
>>> num_train_steps = len(food["train"]) * num_epochs
>>> learning_rate = 3e-5
>>> weight_decay_rate = 0.01
>>> optimizer, lr_schedule = create_optimizer(
... init_lr=learning_rate,
... num_train_steps=num_train_steps,
... weight_decay_rate=weight_decay_rate,
... num_warmup_steps=0,
... )
```
๊ทธ๋ฐ ๋ค์ ๋ ์ด๋ธ ๋งคํ๊ณผ ํจ๊ป [`TFAuto ModelForImageClassification`]์ผ๋ก ViT๋ฅผ ๊ฐ์ ธ์ต๋๋ค:
```py
>>> from transformers import TFAutoModelForImageClassification
>>> model = TFAutoModelForImageClassification.from_pretrained(
... checkpoint,
... id2label=id2label,
... label2id=label2id,
... )
```
๋ฐ์ดํฐ ์ธํธ๋ฅผ [`~datasets.Dataset.to_tf_dataset`]์ `data_collator`๋ฅผ ์ฌ์ฉํ์ฌ `tf.data.Dataset` ํ์์ผ๋ก ๋ณํํ์ธ์:
```py
>>> # converting our train dataset to tf.data.Dataset
>>> tf_train_dataset = food["train"].to_tf_dataset(
... columns="pixel_values", label_cols="label", shuffle=True, batch_size=batch_size, collate_fn=data_collator
... )
>>> # converting our test dataset to tf.data.Dataset
>>> tf_eval_dataset = food["test"].to_tf_dataset(
... columns="pixel_values", label_cols="label", shuffle=True, batch_size=batch_size, collate_fn=data_collator
... )
```
`compile()`๋ฅผ ์ฌ์ฉํ์ฌ ํ๋ จ ๋ชจ๋ธ์ ๊ตฌ์ฑํ์ธ์:
```py
>>> from tensorflow.keras.losses import SparseCategoricalCrossentropy
>>> loss = tf.keras.losses.SparseCategoricalCrossentropy(from_logits=True)
>>> model.compile(optimizer=optimizer, loss=loss)
```
์์ธก์์ ์ ํ๋๋ฅผ ๊ณ์ฐํ๊ณ ๋ชจ๋ธ์ ๐ค Hub๋ก ํธ์ํ๋ ค๋ฉด [Keras callbacks](../main_classes/keras_callbacks)๋ฅผ ์ฌ์ฉํ์ธ์.
`compute_metrics` ํจ์๋ฅผ [KerasMetricCallback](../main_classes/keras_callbacks#transformers.KerasMetricCallback)์ ์ ๋ฌํ๊ณ ,
[PushToHubCallback](../main_classes/keras_callbacks#transformers.PushToHubCallback)์ ์ฌ์ฉํ์ฌ ๋ชจ๋ธ์ ์
๋ก๋ํฉ๋๋ค:
```py
>>> from transformers.keras_callbacks import KerasMetricCallback, PushToHubCallback
>>> metric_callback = KerasMetricCallback(metric_fn=compute_metrics, eval_dataset=tf_eval_dataset)
>>> push_to_hub_callback = PushToHubCallback(
... output_dir="food_classifier",
... tokenizer=image_processor,
... save_strategy="no",
... )
>>> callbacks = [metric_callback, push_to_hub_callback]
```
์ด์ ๋ชจ๋ธ์ ํ๋ จํ ์ค๋น๊ฐ ๋์์ต๋๋ค! ํ๋ จ ๋ฐ ๊ฒ์ฆ ๋ฐ์ดํฐ ์ธํธ, ์ํญ ์์ ํจ๊ป `fit()`์ ํธ์ถํ๊ณ ,
์ฝ๋ฐฑ์ ์ฌ์ฉํ์ฌ ๋ชจ๋ธ์ ๋ฏธ์ธ ์กฐ์ ํฉ๋๋ค:
```py
>>> model.fit(tf_train_dataset, validation_data=tf_eval_dataset, epochs=num_epochs, callbacks=callbacks)
Epoch 1/5
250/250 [==============================] - 313s 1s/step - loss: 2.5623 - val_loss: 1.4161 - accuracy: 0.9290
Epoch 2/5
250/250 [==============================] - 265s 1s/step - loss: 0.9181 - val_loss: 0.6808 - accuracy: 0.9690
Epoch 3/5
250/250 [==============================] - 252s 1s/step - loss: 0.3910 - val_loss: 0.4303 - accuracy: 0.9820
Epoch 4/5
250/250 [==============================] - 251s 1s/step - loss: 0.2028 - val_loss: 0.3191 - accuracy: 0.9900
Epoch 5/5
250/250 [==============================] - 238s 949ms/step - loss: 0.1232 - val_loss: 0.3259 - accuracy: 0.9890
```
์ถํํฉ๋๋ค! ๋ชจ๋ธ์ ๋ฏธ์ธ ์กฐ์ ํ๊ณ ๐ค Hub์ ๊ณต์ ํ์ต๋๋ค. ์ด์ ์ถ๋ก ์ ์ฌ์ฉํ ์ ์์ต๋๋ค!
</tf>
</frameworkcontent>
<Tip>
์ด๋ฏธ์ง ๋ถ๋ฅ๋ฅผ ์ํ ๋ชจ๋ธ์ ๋ฏธ์ธ ์กฐ์ ํ๋ ์์ธํ ์์ ๋ ๋ค์ [PyTorch notebook](https://colab.research.google.com/github/huggingface/notebooks/blob/main/examples/image_classification.ipynb)์ ์ฐธ์กฐํ์ธ์.
</Tip>
## ์ถ๋ก [[inference]]
์ข์์, ์ด์ ๋ชจ๋ธ์ ๋ฏธ์ธ ์กฐ์ ํ์ผ๋ ์ถ๋ก ์ ์ฌ์ฉํ ์ ์์ต๋๋ค!
์ถ๋ก ์ ์ํํ๊ณ ์ ํ๋ ์ด๋ฏธ์ง๋ฅผ ๊ฐ์ ธ์๋ด
์๋ค:
```py
>>> ds = load_dataset("food101", split="validation[:10]")
>>> image = ds["image"][0]
```
<div class="flex justify-center">
<img src="https://huggingface.co/datasets/huggingface/documentation-images/resolve/main/beignets-task-guide.png" alt="image of beignets"/>
</div>
๋ฏธ์ธ ์กฐ์ ๋ชจ๋ธ๋ก ์ถ๋ก ์ ์๋ํ๋ ๊ฐ์ฅ ๊ฐ๋จํ ๋ฐฉ๋ฒ์ [`pipeline`]์ ์ฌ์ฉํ๋ ๊ฒ์
๋๋ค. ๋ชจ๋ธ๋ก ์ด๋ฏธ์ง ๋ถ๋ฅ๋ฅผ ์ํ `pipeline`์ ์ธ์คํด์คํํ๊ณ ์ด๋ฏธ์ง๋ฅผ ์ ๋ฌํฉ๋๋ค:
```py
>>> from transformers import pipeline
>>> classifier = pipeline("image-classification", model="my_awesome_food_model")
>>> classifier(image)
[{'score': 0.31856709718704224, 'label': 'beignets'},
{'score': 0.015232225880026817, 'label': 'bruschetta'},
{'score': 0.01519392803311348, 'label': 'chicken_wings'},
{'score': 0.013022331520915031, 'label': 'pork_chop'},
{'score': 0.012728818692266941, 'label': 'prime_rib'}]
```
์ํ๋ค๋ฉด, `pipeline`์ ๊ฒฐ๊ณผ๋ฅผ ์๋์ผ๋ก ๋ณต์ ํ ์๋ ์์ต๋๋ค:
<frameworkcontent>
<pt>
์ด๋ฏธ์ง๋ฅผ ์ ์ฒ๋ฆฌํ๊ธฐ ์ํด ์ด๋ฏธ์ง ํ๋ก์ธ์๋ฅผ ๊ฐ์ ธ์ค๊ณ `input`์ PyTorch ํ
์๋ก ๋ฐํํฉ๋๋ค:
```py
>>> from transformers import AutoImageProcessor
>>> import torch
>>> image_processor = AutoImageProcessor.from_pretrained("my_awesome_food_model")
>>> inputs = image_processor(image, return_tensors="pt")
```
์
๋ ฅ์ ๋ชจ๋ธ์ ์ ๋ฌํ๊ณ logits์ ๋ฐํํฉ๋๋ค:
```py
>>> from transformers import AutoModelForImageClassification
>>> model = AutoModelForImageClassification.from_pretrained("my_awesome_food_model")
>>> with torch.no_grad():
... logits = model(**inputs).logits
```
ํ๋ฅ ์ด ๊ฐ์ฅ ๋์ ์์ธก ๋ ์ด๋ธ์ ๊ฐ์ ธ์ค๊ณ , ๋ชจ๋ธ์ `id2label` ๋งคํ์ ์ฌ์ฉํ์ฌ ๋ ์ด๋ธ๋ก ๋ณํํฉ๋๋ค:
```py
>>> predicted_label = logits.argmax(-1).item()
>>> model.config.id2label[predicted_label]
'beignets'
```
</pt>
</frameworkcontent>
<frameworkcontent>
<tf>
์ด๋ฏธ์ง๋ฅผ ์ ์ฒ๋ฆฌํ๊ธฐ ์ํด ์ด๋ฏธ์ง ํ๋ก์ธ์๋ฅผ ๊ฐ์ ธ์ค๊ณ `input`์ TensorFlow ํ
์๋ก ๋ฐํํฉ๋๋ค:
```py
>>> from transformers import AutoImageProcessor
>>> image_processor = AutoImageProcessor.from_pretrained("MariaK/food_classifier")
>>> inputs = image_processor(image, return_tensors="tf")
```
์
๋ ฅ์ ๋ชจ๋ธ์ ์ ๋ฌํ๊ณ logits์ ๋ฐํํฉ๋๋ค:
```py
>>> from transformers import TFAutoModelForImageClassification
>>> model = TFAutoModelForImageClassification.from_pretrained("MariaK/food_classifier")
>>> logits = model(**inputs).logits
```
ํ๋ฅ ์ด ๊ฐ์ฅ ๋์ ์์ธก ๋ ์ด๋ธ์ ๊ฐ์ ธ์ค๊ณ , ๋ชจ๋ธ์ `id2label` ๋งคํ์ ์ฌ์ฉํ์ฌ ๋ ์ด๋ธ๋ก ๋ณํํฉ๋๋ค:
```py
>>> predicted_class_id = int(tf.math.argmax(logits, axis=-1)[0])
>>> model.config.id2label[predicted_class_id]
'beignets'
```
</tf>
</frameworkcontent>
| 0 |
hf_public_repos/transformers/docs/source/ko | hf_public_repos/transformers/docs/source/ko/tasks/summarization.md | <!--Copyright 2022 The HuggingFace Team. All rights reserved.
Licensed under the Apache License, Version 2.0 (the "License"); you may not use this file except in compliance with
the License. You may obtain a copy of the License at
http://www.apache.org/licenses/LICENSE-2.0
Unless required by applicable law or agreed to in writing, software distributed under the License is distributed on
an "AS IS" BASIS, WITHOUT WARRANTIES OR CONDITIONS OF ANY KIND, either express or implied. See the License for the
specific language governing permissions and limitations under the License.
โ ๏ธ Note that this file is in Markdown but contain specific syntax for our doc-builder (similar to MDX) that may not be
rendered properly in your Markdown viewer.
-->
# ์์ฝ[[summarization]]
[[open-in-colab]]
<Youtube id="yHnr5Dk2zCI"/>
์์ฝ์ ๋ฌธ์๋ ๊ธฐ์ฌ์์ ์ค์ํ ์ ๋ณด๋ฅผ ๋ชจ๋ ํฌํจํ๋ ์งง๊ฒ ๋ง๋๋ ์ผ์
๋๋ค.
๋ฒ์ญ๊ณผ ๋ง์ฐฌ๊ฐ์ง๋ก, ์ํ์ค-ํฌ-์ํ์ค ๋ฌธ์ ๋ก ๊ตฌ์ฑํ ์ ์๋ ๋ํ์ ์ธ ์์
์ค ํ๋์
๋๋ค.
์์ฝ์๋ ์๋์ ๊ฐ์ด ์ ํ์ด ์์ต๋๋ค:
- ์ถ์ถ(Extractive) ์์ฝ: ๋ฌธ์์์ ๊ฐ์ฅ ๊ด๋ จ์ฑ ๋์ ์ ๋ณด๋ฅผ ์ถ์ถํฉ๋๋ค.
- ์์ฑ(Abstractive) ์์ฝ: ๊ฐ์ฅ ๊ด๋ จ์ฑ ๋์ ์ ๋ณด๋ฅผ ํฌ์ฐฉํด๋ด๋ ์๋ก์ด ํ
์คํธ๋ฅผ ์์ฑํฉ๋๋ค.
์ด ๊ฐ์ด๋์์ ์๊ฐํ ๋ด์ฉ์ ์๋์ ๊ฐ์ต๋๋ค:
1. ์์ฑ ์์ฝ์ ์ํ [BillSum](https://huggingface.co/datasets/billsum) ๋ฐ์ดํฐ์
์ค ์บ๋ฆฌํฌ๋์ ์ฃผ ๋ฒ์ ํ์ ์งํฉ์ผ๋ก [T5](https://huggingface.co/t5-small)๋ฅผ ํ์ธํ๋ํฉ๋๋ค.
2. ํ์ธํ๋๋ ๋ชจ๋ธ์ ์ฌ์ฉํ์ฌ ์ถ๋ก ํฉ๋๋ค.
<Tip>
์ด ํํ ๋ฆฌ์ผ์์ ์ค๋ช
ํ๋ ์์
์ ๋ค์ ๋ชจ๋ธ ์ํคํ
์ฒ์์ ์ง์๋ฉ๋๋ค:
<!--This tip is automatically generated by `make fix-copies`, do not fill manually!-->
[BART](../model_doc/bart), [BigBird-Pegasus](../model_doc/bigbird_pegasus), [Blenderbot](../model_doc/blenderbot), [BlenderbotSmall](../model_doc/blenderbot-small), [Encoder decoder](../model_doc/encoder-decoder), [FairSeq Machine-Translation](../model_doc/fsmt), [GPTSAN-japanese](../model_doc/gptsan-japanese), [LED](../model_doc/led), [LongT5](../model_doc/longt5), [M2M100](../model_doc/m2m_100), [Marian](../model_doc/marian), [mBART](../model_doc/mbart), [MT5](../model_doc/mt5), [MVP](../model_doc/mvp), [NLLB](../model_doc/nllb), [NLLB-MOE](../model_doc/nllb-moe), [Pegasus](../model_doc/pegasus), [PEGASUS-X](../model_doc/pegasus_x), [PLBart](../model_doc/plbart), [ProphetNet](../model_doc/prophetnet), [SwitchTransformers](../model_doc/switch_transformers), [T5](../model_doc/t5), [XLM-ProphetNet](../model_doc/xlm-prophetnet)
<!--End of the generated tip-->
</Tip>
์์ํ๊ธฐ ์ ์ ํ์ํ ๋ผ์ด๋ธ๋ฌ๋ฆฌ๊ฐ ๋ชจ๋ ์ค์น๋์ด ์๋์ง ํ์ธํ์ธ์:
```bash
pip install transformers datasets evaluate rouge_score
```
Hugging Face ๊ณ์ ์ ๋ก๊ทธ์ธํ๋ฉด ๋ชจ๋ธ์ ์
๋ก๋ํ๊ณ ์ปค๋ฎค๋ํฐ์ ๊ณต์ ํ ์ ์์ต๋๋ค.
ํ ํฐ์ ์
๋ ฅํ์ฌ ๋ก๊ทธ์ธํ์ธ์.
```py
>>> from huggingface_hub import notebook_login
>>> notebook_login()
```
## BillSum ๋ฐ์ดํฐ์
๊ฐ์ ธ์ค๊ธฐ[[load-billsum-dataset]]
๐ค Datasets ๋ผ์ด๋ธ๋ฌ๋ฆฌ์์ BillSum ๋ฐ์ดํฐ์
์ ์์ ๋ฒ์ ์ธ ์บ๋ฆฌํฌ๋์ ์ฃผ ๋ฒ์ ํ์ ์งํฉ์ ๊ฐ์ ธ์ค์ธ์:
```py
>>> from datasets import load_dataset
>>> billsum = load_dataset("billsum", split="ca_test")
```
[`~datasets.Dataset.train_test_split`] ๋ฉ์๋๋ก ๋ฐ์ดํฐ์
์ ํ์ต์ฉ์ ํ
์คํธ์ฉ์ผ๋ก ๋๋์ธ์:
```py
>>> billsum = billsum.train_test_split(test_size=0.2)
```
๊ทธ๋ฐ ๋ค์ ์์๋ฅผ ํ๋ ์ดํด๋ณด์ธ์:
```py
>>> billsum["train"][0]
{'summary': 'Existing law authorizes state agencies to enter into contracts for the acquisition of goods or services upon approval by the Department of General Services. Existing law sets forth various requirements and prohibitions for those contracts, including, but not limited to, a prohibition on entering into contracts for the acquisition of goods or services of $100,000 or more with a contractor that discriminates between spouses and domestic partners or same-sex and different-sex couples in the provision of benefits. Existing law provides that a contract entered into in violation of those requirements and prohibitions is void and authorizes the state or any person acting on behalf of the state to bring a civil action seeking a determination that a contract is in violation and therefore void. Under existing law, a willful violation of those requirements and prohibitions is a misdemeanor.\nThis bill would also prohibit a state agency from entering into contracts for the acquisition of goods or services of $100,000 or more with a contractor that discriminates between employees on the basis of gender identity in the provision of benefits, as specified. By expanding the scope of a crime, this bill would impose a state-mandated local program.\nThe California Constitution requires the state to reimburse local agencies and school districts for certain costs mandated by the state. Statutory provisions establish procedures for making that reimbursement.\nThis bill would provide that no reimbursement is required by this act for a specified reason.',
'text': 'The people of the State of California do enact as follows:\n\n\nSECTION 1.\nSection 10295.35 is added to the Public Contract Code, to read:\n10295.35.\n(a) (1) Notwithstanding any other law, a state agency shall not enter into any contract for the acquisition of goods or services in the amount of one hundred thousand dollars ($100,000) or more with a contractor that, in the provision of benefits, discriminates between employees on the basis of an employeeโs or dependentโs actual or perceived gender identity, including, but not limited to, the employeeโs or dependentโs identification as transgender.\n(2) For purposes of this section, โcontractโ includes contracts with a cumulative amount of one hundred thousand dollars ($100,000) or more per contractor in each fiscal year.\n(3) For purposes of this section, an employee health plan is discriminatory if the plan is not consistent with Section 1365.5 of the Health and Safety Code and Section 10140 of the Insurance Code.\n(4) The requirements of this section shall apply only to those portions of a contractorโs operations that occur under any of the following conditions:\n(A) Within the state.\n(B) On real property outside the state if the property is owned by the state or if the state has a right to occupy the property, and if the contractorโs presence at that location is connected to a contract with the state.\n(C) Elsewhere in the United States where work related to a state contract is being performed.\n(b) Contractors shall treat as confidential, to the maximum extent allowed by law or by the requirement of the contractorโs insurance provider, any request by an employee or applicant for employment benefits or any documentation of eligibility for benefits submitted by an employee or applicant for employment.\n(c) After taking all reasonable measures to find a contractor that complies with this section, as determined by the state agency, the requirements of this section may be waived under any of the following circumstances:\n(1) There is only one prospective contractor willing to enter into a specific contract with the state agency.\n(2) The contract is necessary to respond to an emergency, as determined by the state agency, that endangers the public health, welfare, or safety, or the contract is necessary for the provision of essential services, and no entity that complies with the requirements of this section capable of responding to the emergency is immediately available.\n(3) The requirements of this section violate, or are inconsistent with, the terms or conditions of a grant, subvention, or agreement, if the agency has made a good faith attempt to change the terms or conditions of any grant, subvention, or agreement to authorize application of this section.\n(4) The contractor is providing wholesale or bulk water, power, or natural gas, the conveyance or transmission of the same, or ancillary services, as required for ensuring reliable services in accordance with good utility practice, if the purchase of the same cannot practically be accomplished through the standard competitive bidding procedures and the contractor is not providing direct retail services to end users.\n(d) (1) A contractor shall not be deemed to discriminate in the provision of benefits if the contractor, in providing the benefits, pays the actual costs incurred in obtaining the benefit.\n(2) If a contractor is unable to provide a certain benefit, despite taking reasonable measures to do so, the contractor shall not be deemed to discriminate in the provision of benefits.\n(e) (1) Every contract subject to this chapter shall contain a statement by which the contractor certifies that the contractor is in compliance with this section.\n(2) The department or other contracting agency shall enforce this section pursuant to its existing enforcement powers.\n(3) (A) If a contractor falsely certifies that it is in compliance with this section, the contract with that contractor shall be subject to Article 9 (commencing with Section 10420), unless, within a time period specified by the department or other contracting agency, the contractor provides to the department or agency proof that it has complied, or is in the process of complying, with this section.\n(B) The application of the remedies or penalties contained in Article 9 (commencing with Section 10420) to a contract subject to this chapter shall not preclude the application of any existing remedies otherwise available to the department or other contracting agency under its existing enforcement powers.\n(f) Nothing in this section is intended to regulate the contracting practices of any local jurisdiction.\n(g) This section shall be construed so as not to conflict with applicable federal laws, rules, or regulations. In the event that a court or agency of competent jurisdiction holds that federal law, rule, or regulation invalidates any clause, sentence, paragraph, or section of this code or the application thereof to any person or circumstances, it is the intent of the state that the court or agency sever that clause, sentence, paragraph, or section so that the remainder of this section shall remain in effect.\nSEC. 2.\nSection 10295.35 of the Public Contract Code shall not be construed to create any new enforcement authority or responsibility in the Department of General Services or any other contracting agency.\nSEC. 3.\nNo reimbursement is required by this act pursuant to Section 6 of Article XIII\u2009B of the California Constitution because the only costs that may be incurred by a local agency or school district will be incurred because this act creates a new crime or infraction, eliminates a crime or infraction, or changes the penalty for a crime or infraction, within the meaning of Section 17556 of the Government Code, or changes the definition of a crime within the meaning of Section 6 of Article XIII\u2009B of the California Constitution.',
'title': 'An act to add Section 10295.35 to the Public Contract Code, relating to public contracts.'}
```
์ฌ๊ธฐ์ ๋ค์ ๋ ๊ฐ์ ํ๋๋ฅผ ์ฌ์ฉํ๊ฒ ๋ฉ๋๋ค:
- `text`: ๋ชจ๋ธ์ ์
๋ ฅ์ด ๋ ๋ฒ์ ํ
์คํธ์
๋๋ค.
- `summary`: `text`์ ๊ฐ๋ตํ ๋ฒ์ ์ผ๋ก ๋ชจ๋ธ์ ํ๊ฒ์ด ๋ฉ๋๋ค.
## ์ ์ฒ๋ฆฌ[[preprocess]]
๋ค์์ผ๋ก `text`์ `summary`๋ฅผ ์ฒ๋ฆฌํ๊ธฐ ์ํ T5 ํ ํฌ๋์ด์ ๋ฅผ ๊ฐ์ ธ์ต๋๋ค:
```py
>>> from transformers import AutoTokenizer
>>> checkpoint = "t5-small"
>>> tokenizer = AutoTokenizer.from_pretrained(checkpoint)
```
์์ฑํ๋ ค๋ ์ ์ฒ๋ฆฌ ํจ์๋ ์๋ ์กฐ๊ฑด์ ๋ง์กฑํด์ผ ํฉ๋๋ค:
1. ์
๋ ฅ ์์ ํ๋กฌํํธ๋ฅผ ๋ถ์ฌ T5๊ฐ ์์ฝ ์์
์์ ์ธ์ํ ์ ์๋๋ก ํฉ๋๋ค. ์ฌ๋ฌ NLP ์์
์ ์ํํ ์ ์๋ ์ผ๋ถ ๋ชจ๋ธ์ ํน์ ์์
์ ๋ํ ํ๋กฌํํธ๊ฐ ํ์ํฉ๋๋ค.
2. ๋ ์ด๋ธ์ ํ ํฐํํ ๋ `text_target` ์ธ์๋ฅผ ์ฌ์ฉํฉ๋๋ค.
3. `max_length` ๋งค๊ฐ๋ณ์๋ก ์ค์ ๋ ์ต๋ ๊ธธ์ด๋ฅผ ๋์ง ์๋๋ก ๊ธด ์ํ์ค๋ฅผ ์๋ผ๋
๋๋ค.
```py
>>> prefix = "summarize: "
>>> def preprocess_function(examples):
... inputs = [prefix + doc for doc in examples["text"]]
... model_inputs = tokenizer(inputs, max_length=1024, truncation=True)
... labels = tokenizer(text_target=examples["summary"], max_length=128, truncation=True)
... model_inputs["labels"] = labels["input_ids"]
... return model_inputs
```
์ ์ฒด ๋ฐ์ดํฐ์
์ ์ ์ฒ๋ฆฌ ํจ์๋ฅผ ์ ์ฉํ๋ ค๋ฉด ๐ค Datasets์ [`~datasets.Dataset.map`] ๋ฉ์๋๋ฅผ ์ฌ์ฉํ์ธ์.
`batched=True`๋ก ์ค์ ํ์ฌ ๋ฐ์ดํฐ์
์ ์ฌ๋ฌ ์์๋ฅผ ํ ๋ฒ์ ์ฒ๋ฆฌํ๋ฉด `map` ํจ์์ ์๋๋ฅผ ๋์ผ ์ ์์ต๋๋ค.
```py
>>> tokenized_billsum = billsum.map(preprocess_function, batched=True)
```
์ด์ [`DataCollatorForSeq2Seq`]๋ฅผ ์ฌ์ฉํ์ฌ ์์ ๋ฐฐ์น๋ฅผ ๋ง๋์ธ์.
์ ์ฒด ๋ฐ์ดํฐ์
์ ์ต๋ ๊ธธ์ด๋ก ํจ๋ฉํ๋ ๊ฒ๋ณด๋ค ๋ฐฐ์น๋ง๋ค ๊ฐ์ฅ ๊ธด ๋ฌธ์ฅ ๊ธธ์ด์ ๋ง์ถฐ *๋์ ํจ๋ฉ*ํ๋ ๊ฒ์ด ๋ ํจ์จ์ ์
๋๋ค.
<frameworkcontent>
<pt>
```py
>>> from transformers import DataCollatorForSeq2Seq
>>> data_collator = DataCollatorForSeq2Seq(tokenizer=tokenizer, model=checkpoint)
```
</pt>
<tf>
```py
>>> from transformers import DataCollatorForSeq2Seq
>>> data_collator = DataCollatorForSeq2Seq(tokenizer=tokenizer, model=checkpoint, return_tensors="tf")
```
</tf>
</frameworkcontent>
## ํ๊ฐ[[evaluate]]
ํ์ต ์ค์ ํ๊ฐ ์งํ๋ฅผ ํฌํจํ๋ฉด ๋ชจ๋ธ์ ์ฑ๋ฅ์ ํ๊ฐํ๋ ๋ฐ ๋์์ด ๋๋ ๊ฒฝ์ฐ๊ฐ ๋ง์ต๋๋ค.
๐ค [Evaluate](https://huggingface.co/docs/evaluate/index) ๋ผ์ด๋ธ๋ฌ๋ฆฌ๋ฅผ ์ฌ์ฉํ๋ฉด ํ๊ฐ ๋ฐฉ๋ฒ์ ๋น ๋ฅด๊ฒ ๋ถ๋ฌ์ฌ ์ ์์ต๋๋ค.
์ด ์์
์์๋ [ROUGE](https://huggingface.co/spaces/evaluate-metric/rouge) ํ๊ฐ ์งํ๋ฅผ ๊ฐ์ ธ์ต๋๋ค.
(ํ๊ฐ ์งํ๋ฅผ ๋ถ๋ฌ์ค๊ณ ๊ณ์ฐํ๋ ๋ฐฉ๋ฒ์ ๐ค Evaluate [๋๋ฌ๋ณด๊ธฐ](https://huggingface.co/docs/evaluate/a_quick_tour)๋ฅผ ์ฐธ์กฐํ์ธ์.)
```py
>>> import evaluate
>>> rouge = evaluate.load("rouge")
```
๊ทธ๋ฐ ๋ค์ ์์ธก๊ฐ๊ณผ ๋ ์ด๋ธ์ [`~evaluate.EvaluationModule.compute`]์ ์ ๋ฌํ์ฌ ROUGE ์งํ๋ฅผ ๊ณ์ฐํ๋ ํจ์๋ฅผ ๋ง๋ญ๋๋ค:
```py
>>> import numpy as np
>>> def compute_metrics(eval_pred):
... predictions, labels = eval_pred
... decoded_preds = tokenizer.batch_decode(predictions, skip_special_tokens=True)
... labels = np.where(labels != -100, labels, tokenizer.pad_token_id)
... decoded_labels = tokenizer.batch_decode(labels, skip_special_tokens=True)
... result = rouge.compute(predictions=decoded_preds, references=decoded_labels, use_stemmer=True)
... prediction_lens = [np.count_nonzero(pred != tokenizer.pad_token_id) for pred in predictions]
... result["gen_len"] = np.mean(prediction_lens)
... return {k: round(v, 4) for k, v in result.items()}
```
์ด์ `compute_metrics` ํจ์๋ฅผ ์ฌ์ฉํ ์ค๋น๊ฐ ๋์์ผ๋ฉฐ, ํ์ต์ ์ค์ ํ ๋ ์ด ํจ์๋ก ๋๋์์ฌ ๊ฒ์
๋๋ค.
## ํ์ต[[train]]
<frameworkcontent>
<pt>
<Tip>
๋ชจ๋ธ์ [`Trainer`]๋ก ํ์ธํ๋ ํ๋ ๊ฒ์ด ์ต์ํ์ง ์๋ค๋ฉด, [์ฌ๊ธฐ](../training#train-with-pytorch-trainer)์์ ๊ธฐ๋ณธ ํํ ๋ฆฌ์ผ์ ํ์ธํด๋ณด์ธ์!
</Tip>
์ด์ ๋ชจ๋ธ ํ์ต์ ์์ํ ์ค๋น๊ฐ ๋์์ต๋๋ค! [`AutoModelForSeq2SeqLM`]๋ก T5๋ฅผ ๊ฐ์ ธ์ค์ธ์:
```py
>>> from transformers import AutoModelForSeq2SeqLM, Seq2SeqTrainingArguments, Seq2SeqTrainer
>>> model = AutoModelForSeq2SeqLM.from_pretrained(checkpoint)
```
์ด์ ์ธ ๋จ๊ณ๋ง ๋จ์์ต๋๋ค:
1. [`Seq2SeqTrainingArguments`]์์ ํ์ต ํ์ดํผํ๋ผ๋ฏธํฐ๋ฅผ ์ ์ํ์ธ์.
์ ์ผํ ํ์ ๋งค๊ฐ๋ณ์๋ ๋ชจ๋ธ์ ์ ์ฅํ ์์น๋ฅผ ์ง์ ํ๋ `output_dir`์
๋๋ค.
`push_to_hub=True`๋ฅผ ์ค์ ํ์ฌ ์ด ๋ชจ๋ธ์ Hub์ ํธ์ํ ์ ์์ต๋๋ค(๋ชจ๋ธ์ ์
๋ก๋ํ๋ ค๋ฉด Hugging Face์ ๋ก๊ทธ์ธํด์ผ ํฉ๋๋ค.)
[`Trainer`]๋ ๊ฐ ์ํญ์ด ๋๋ ๋๋ง๋ค ROUGE ์งํ๋ฅผ ํ๊ฐํ๊ณ ํ์ต ์ฒดํฌํฌ์ธํธ๋ฅผ ์ ์ฅํฉ๋๋ค.
2. ๋ชจ๋ธ, ๋ฐ์ดํฐ์
, ํ ํฌ๋์ด์ , ๋ฐ์ดํฐ ์ฝ๋ ์ดํฐ ๋ฐ `compute_metrics` ํจ์์ ํจ๊ป ํ์ต ์ธ์๋ฅผ [`Seq2SeqTrainer`]์ ์ ๋ฌํ์ธ์.
3. [`~Trainer.train`]์ ํธ์ถํ์ฌ ๋ชจ๋ธ์ ํ์ธํ๋ํ์ธ์.
```py
>>> training_args = Seq2SeqTrainingArguments(
... output_dir="my_awesome_billsum_model",
... evaluation_strategy="epoch",
... learning_rate=2e-5,
... per_device_train_batch_size=16,
... per_device_eval_batch_size=16,
... weight_decay=0.01,
... save_total_limit=3,
... num_train_epochs=4,
... predict_with_generate=True,
... fp16=True,
... push_to_hub=True,
... )
>>> trainer = Seq2SeqTrainer(
... model=model,
... args=training_args,
... train_dataset=tokenized_billsum["train"],
... eval_dataset=tokenized_billsum["test"],
... tokenizer=tokenizer,
... data_collator=data_collator,
... compute_metrics=compute_metrics,
... )
>>> trainer.train()
```
ํ์ต์ด ์๋ฃ๋๋ฉด, ๋๊ตฌ๋ ๋ชจ๋ธ์ ์ฌ์ฉํ ์ ์๋๋ก [`~transformers.Trainer.push_to_hub`] ๋ฉ์๋๋ก Hub์ ๊ณต์ ํฉ๋๋ค:
```py
>>> trainer.push_to_hub()
```
</pt>
<tf>
<Tip>
Keras๋ก ๋ชจ๋ธ ํ์ธํ๋์ ํ๋ ๊ฒ์ด ์ต์ํ์ง ์๋ค๋ฉด, [์ฌ๊ธฐ](../training#train-a-tensorflow-model-with-keras)์์ ๊ธฐ๋ณธ์ ์ธ ํํ ๋ฆฌ์ผ์ ํ์ธํ์ธ์!
</Tip>
TensorFlow์์ ๋ชจ๋ธ์ ํ์ธํ๋ํ๋ ค๋ฉด, ๋จผ์ ์ตํฐ๋ง์ด์ , ํ์ต๋ฅ ์ค์ผ์ค ๊ทธ๋ฆฌ๊ณ ๋ช ๊ฐ์ง ํ์ต ํ์ดํผํ๋ผ๋ฏธํฐ๋ฅผ ์ค์ ํ์ธ์:
```py
>>> from transformers import create_optimizer, AdamWeightDecay
>>> optimizer = AdamWeightDecay(learning_rate=2e-5, weight_decay_rate=0.01)
```
๊ทธ๋ฐ ๋ค์ [`TFAutoModelForSeq2SeqLM`]์ ์ฌ์ฉํ์ฌ T5๋ฅผ ๊ฐ์ ธ์ค์ธ์:
```py
>>> from transformers import TFAutoModelForSeq2SeqLM
>>> model = TFAutoModelForSeq2SeqLM.from_pretrained(checkpoint)
```
[`~transformers.TFPreTrainedModel.prepare_tf_dataset`]์ ์ฌ์ฉํ์ฌ ๋ฐ์ดํฐ์
์ `tf.data.Dataset` ํ์์ผ๋ก ๋ณํํ์ธ์:
```py
>>> tf_train_set = model.prepare_tf_dataset(
... tokenized_billsum["train"],
... shuffle=True,
... batch_size=16,
... collate_fn=data_collator,
... )
>>> tf_test_set = model.prepare_tf_dataset(
... tokenized_billsum["test"],
... shuffle=False,
... batch_size=16,
... collate_fn=data_collator,
... )
```
[`compile`](https://keras.io/api/models/model_training_apis/#compile-method)์ ์ฌ์ฉํ์ฌ ๋ชจ๋ธ์ ํ์ตํ ์ ์๋๋ก ๊ตฌ์ฑํ์ธ์:
```py
>>> import tensorflow as tf
>>> model.compile(optimizer=optimizer)
```
ํ์ต์ ์์ํ๊ธฐ ์ ์ ์ค์ ํด์ผ ํ ๋ง์ง๋ง ๋ ๊ฐ์ง๋ ์์ธก์์ ROUGE ์ ์๋ฅผ ๊ณ์ฐํ๊ณ ๋ชจ๋ธ์ Hub์ ํธ์ํ๋ ๋ฐฉ๋ฒ์ ์ ๊ณตํ๋ ๊ฒ์
๋๋ค.
๋ ์์
๋ชจ๋ [Keras callbacks](../main_classes/keras_callbacks)์ผ๋ก ์ํํ ์ ์์ต๋๋ค.
[`~transformers.KerasMetricCallback`]์ `compute_metrics` ํจ์๋ฅผ ์ ๋ฌํ์ธ์:
```py
>>> from transformers.keras_callbacks import KerasMetricCallback
>>> metric_callback = KerasMetricCallback(metric_fn=compute_metrics, eval_dataset=tf_validation_set)
```
[`~transformers.PushToHubCallback`]์์ ๋ชจ๋ธ๊ณผ ํ ํฌ๋์ด์ ๋ฅผ ํธ์ํ ์์น๋ฅผ ์ง์ ํ์ธ์:
```py
>>> from transformers.keras_callbacks import PushToHubCallback
>>> push_to_hub_callback = PushToHubCallback(
... output_dir="my_awesome_billsum_model",
... tokenizer=tokenizer,
... )
```
๊ทธ๋ฐ ๋ค์ ์ฝ๋ฐฑ์ ๋ฒ๋ค๋ก ๋ฌถ์ด์ค๋๋ค:
```py
>>> callbacks = [metric_callback, push_to_hub_callback]
```
๋๋์ด ๋ชจ๋ธ ํ์ต์ ์์ํ ์ค๋น๊ฐ ๋์์ต๋๋ค!
ํ์ต ๋ฐ ๊ฒ์ฆ ๋ฐ์ดํฐ์
, ์ํญ ์ ๋ฐ ์ฝ๋ฐฑ๊ณผ ํจ๊ป [`fit`](https://keras.io/api/models/model_training_apis/#fit-method)์ ํธ์ถํ์ฌ ๋ชจ๋ธ์ ํ์ธํ๋ํ์ธ์.
```py
>>> model.fit(x=tf_train_set, validation_data=tf_test_set, epochs=3, callbacks=callbacks)
```
ํ์ต์ด ์๋ฃ๋๋ฉด ๋ชจ๋ธ์ด ์๋์ผ๋ก Hub์ ์
๋ก๋๋์ด ๋๊ตฌ๋ ์ฌ์ฉํ ์ ์๊ฒ ๋ฉ๋๋ค!
</tf>
</frameworkcontent>
<Tip>
์์ฝ์ ์ํด ๋ชจ๋ธ์ ํ์ธํ๋ํ๋ ๋ฐฉ๋ฒ์ ๋ํ ๋ ์์ธํ ์์ ๋ฅผ ๋ณด๋ ค๋ฉด [PyTorch notebook](https://colab.research.google.com/github/huggingface/notebooks/blob/main/examples/summarization.ipynb)
๋๋ [TensorFlow notebook](https://colab.research.google.com/github/huggingface/notebooks/blob/main/examples/summarization-tf.ipynb)์ ์ฐธ๊ณ ํ์ธ์.
</Tip>
## ์ถ๋ก [[inference]]
์ข์์, ์ด์ ๋ชจ๋ธ์ ํ์ธํ๋ํ์ผ๋ ์ถ๋ก ์ ์ฌ์ฉํ ์ ์์ต๋๋ค!
์์ฝํ ํ
์คํธ๋ฅผ ์์ฑํด๋ณด์ธ์. T5์ ๊ฒฝ์ฐ ์์
์ ๋ฐ๋ผ ์
๋ ฅ ์์ ์ ๋์ฌ๋ฅผ ๋ถ์ฌ์ผ ํฉ๋๋ค. ์์ฝ์ ๊ฒฝ์ฐ, ์๋์ ๊ฐ์ ์ ๋์ฌ๋ฅผ ์
๋ ฅ ์์ ๋ถ์ฌ์ผ ํฉ๋๋ค:
```py
>>> text = "summarize: The Inflation Reduction Act lowers prescription drug costs, health care costs, and energy costs. It's the most aggressive action on tackling the climate crisis in American history, which will lift up American workers and create good-paying, union jobs across the country. It'll lower the deficit and ask the ultra-wealthy and corporations to pay their fair share. And no one making under $400,000 per year will pay a penny more in taxes."
```
์ถ๋ก ์ ์ํด ํ์ธํ๋ํ ๋ชจ๋ธ์ ์ํํด ๋ณด๋ ๊ฐ์ฅ ๊ฐ๋จํ ๋ฐฉ๋ฒ์ [`pipeline`]์์ ์ฌ์ฉํ๋ ๊ฒ์
๋๋ค.
๋ชจ๋ธ์ ์ฌ์ฉํ์ฌ ์์ฝ์ ์ํํ [`pipeline`]์ ์ธ์คํด์คํํ๊ณ ํ
์คํธ๋ฅผ ์ ๋ฌํ์ธ์:
```py
>>> from transformers import pipeline
>>> summarizer = pipeline("summarization", model="stevhliu/my_awesome_billsum_model")
>>> summarizer(text)
[{"summary_text": "The Inflation Reduction Act lowers prescription drug costs, health care costs, and energy costs. It's the most aggressive action on tackling the climate crisis in American history, which will lift up American workers and create good-paying, union jobs across the country."}]
```
์ํ๋ค๋ฉด ์๋์ผ๋ก ๋ค์๊ณผ ๊ฐ์ ์์
์ ์ํํ์ฌ [`pipeline`]์ ๊ฒฐ๊ณผ์ ๋์ผํ ๊ฒฐ๊ณผ๋ฅผ ์ป์ ์ ์์ต๋๋ค:
<frameworkcontent>
<pt>
ํ
์คํธ๋ฅผ ํ ํฌ๋์ด์ฆํ๊ณ `input_ids`๋ฅผ PyTorch ํ
์๋ก ๋ฐํํฉ๋๋ค:
```py
>>> from transformers import AutoTokenizer
>>> tokenizer = AutoTokenizer.from_pretrained("stevhliu/my_awesome_billsum_model")
>>> inputs = tokenizer(text, return_tensors="pt").input_ids
```
์์ฝ๋ฌธ์ ์์ฑํ๋ ค๋ฉด [`~transformers.generation_utils.GenerationMixin.generate`] ๋ฉ์๋๋ฅผ ์ฌ์ฉํ์ธ์.
ํ
์คํธ ์์ฑ์ ๋ํ ๋ค์ํ ์ ๋ต๊ณผ ์์ฑ์ ์ ์ดํ๊ธฐ ์ํ ๋งค๊ฐ๋ณ์์ ๋ํ ์์ธํ ๋ด์ฉ์ [ํ
์คํธ ์์ฑ](../main_classes/text_generation) API๋ฅผ ์ฐธ์กฐํ์ธ์.
```py
>>> from transformers import AutoModelForSeq2SeqLM
>>> model = AutoModelForSeq2SeqLM.from_pretrained("stevhliu/my_awesome_billsum_model")
>>> outputs = model.generate(inputs, max_new_tokens=100, do_sample=False)
```
์์ฑ๋ ํ ํฐ ID๋ฅผ ํ
์คํธ๋ก ๋์ฝ๋ฉํฉ๋๋ค:
```py
>>> tokenizer.decode(outputs[0], skip_special_tokens=True)
'the inflation reduction act lowers prescription drug costs, health care costs, and energy costs. it's the most aggressive action on tackling the climate crisis in american history. it will ask the ultra-wealthy and corporations to pay their fair share.'
```
</pt>
<tf>
ํ
์คํธ๋ฅผ ํ ํฌ๋์ด์ฆํ๊ณ `input_ids`๋ฅผ TensorFlow ํ
์๋ก ๋ฐํํฉ๋๋ค:
```py
>>> from transformers import AutoTokenizer
>>> tokenizer = AutoTokenizer.from_pretrained("stevhliu/my_awesome_billsum_model")
>>> inputs = tokenizer(text, return_tensors="tf").input_ids
```
์์ฝ๋ฌธ์ ์์ฑํ๋ ค๋ฉด [`~transformers.generation_tf_utils.TFGenerationMixin.generate`] ๋ฉ์๋๋ฅผ ์ฌ์ฉํ์ธ์.
ํ
์คํธ ์์ฑ์ ๋ํ ๋ค์ํ ์ ๋ต๊ณผ ์์ฑ์ ์ ์ดํ๊ธฐ ์ํ ๋งค๊ฐ๋ณ์์ ๋ํ ์์ธํ ๋ด์ฉ์ [ํ
์คํธ ์์ฑ](../main_classes/text_generation) API๋ฅผ ์ฐธ์กฐํ์ธ์.
```py
>>> from transformers import TFAutoModelForSeq2SeqLM
>>> model = TFAutoModelForSeq2SeqLM.from_pretrained("stevhliu/my_awesome_billsum_model")
>>> outputs = model.generate(inputs, max_new_tokens=100, do_sample=False)
```
์์ฑ๋ ํ ํฐ ID๋ฅผ ํ
์คํธ๋ก ๋์ฝ๋ฉํฉ๋๋ค:
```py
>>> tokenizer.decode(outputs[0], skip_special_tokens=True)
'the inflation reduction act lowers prescription drug costs, health care costs, and energy costs. it's the most aggressive action on tackling the climate crisis in american history. it will ask the ultra-wealthy and corporations to pay their fair share.'
```
</tf>
</frameworkcontent>
| 0 |
hf_public_repos/transformers/docs/source/ko | hf_public_repos/transformers/docs/source/ko/tasks/translation.md | <!--Copyright 2022 The HuggingFace Team. All rights reserved.
Licensed under the Apache License, Version 2.0 (the "License"); you may not use this file except in compliance with
the License. You may obtain a copy of the License at
http://www.apache.org/licenses/LICENSE-2.0
Unless required by applicable law or agreed to in writing, software distributed under the License is distributed on
an "AS IS" BASIS, WITHOUT WARRANTIES OR CONDITIONS OF ANY KIND, either express or implied. See the License for the
specific language governing permissions and limitations under the License.
โ ๏ธ Note that this file is in Markdown but contain specific syntax for our doc-builder (similar to MDX) that may not be
rendered properly in your Markdown viewer.
-->
# ๋ฒ์ญ[[translation]]
[[open-in-colab]]
<Youtube id="1JvfrvZgi6c"/>
๋ฒ์ญ์ ํ ์ธ์ด๋ก ๋ ์ํ์ค๋ฅผ ๋ค๋ฅธ ์ธ์ด๋ก ๋ณํํฉ๋๋ค. ๋ฒ์ญ์ด๋ ์์ฝ์ ์
๋ ฅ์ ๋ฐ์ ์ผ๋ จ์ ์ถ๋ ฅ์ ๋ฐํํ๋ ๊ฐ๋ ฅํ ํ๋ ์์ํฌ์ธ ์ํ์ค-ํฌ-์ํ์ค ๋ฌธ์ ๋ก ๊ตฌ์ฑํ ์ ์๋ ๋ํ์ ์ธ ํ์คํฌ์
๋๋ค. ๋ฒ์ญ ์์คํ
์ ์ผ๋ฐ์ ์ผ๋ก ๋ค๋ฅธ ์ธ์ด๋ก ๋ ํ
์คํธ ๊ฐ์ ๋ฒ์ญ์ ์ฌ์ฉ๋์ง๋ง, ์์ฑ ๊ฐ์ ํต์ญ์ด๋ ํ
์คํธ-์์ฑ ๋๋ ์์ฑ-ํ
์คํธ์ ๊ฐ์ ์กฐํฉ์๋ ์ฌ์ฉ๋ ์ ์์ต๋๋ค.
์ด ๊ฐ์ด๋์์ ํ์ตํ ๋ด์ฉ์:
1. ์์ด ํ
์คํธ๋ฅผ ํ๋์ค์ด๋ก ๋ฒ์ญํ๊ธฐ ์ํด [T5](https://huggingface.co/t5-small) ๋ชจ๋ธ์ OPUS Books ๋ฐ์ดํฐ์ธํธ์ ์์ด-ํ๋์ค์ด ํ์ ์งํฉ์ผ๋ก ํ์ธํ๋ํ๋ ๋ฐฉ๋ฒ๊ณผ
2. ํ์ธํ๋๋ ๋ชจ๋ธ์ ์ถ๋ก ์ ์ฌ์ฉํ๋ ๋ฐฉ๋ฒ์
๋๋ค.
<Tip>
์ด ํ์คํฌ ๊ฐ์ด๋๋ ์๋ ๋ชจ๋ธ ์ํคํ
์ฒ์๋ ์์ฉํ ์ ์์ต๋๋ค.
<!--This tip is automatically generated by `make fix-copies`, do not fill manually!-->
[BART](../model_doc/bart), [BigBird-Pegasus](../model_doc/bigbird_pegasus), [Blenderbot](../model_doc/blenderbot), [BlenderbotSmall](../model_doc/blenderbot-small), [Encoder decoder](../model_doc/encoder-decoder), [FairSeq Machine-Translation](../model_doc/fsmt), [GPTSAN-japanese](../model_doc/gptsan-japanese), [LED](../model_doc/led), [LongT5](../model_doc/longt5), [M2M100](../model_doc/m2m_100), [Marian](../model_doc/marian), [mBART](../model_doc/mbart), [MT5](../model_doc/mt5), [MVP](../model_doc/mvp), [NLLB](../model_doc/nllb), [NLLB-MOE](../model_doc/nllb-moe), [Pegasus](../model_doc/pegasus), [PEGASUS-X](../model_doc/pegasus_x), [PLBart](../model_doc/plbart), [ProphetNet](../model_doc/prophetnet), [SwitchTransformers](../model_doc/switch_transformers), [T5](../model_doc/t5), [XLM-ProphetNet](../model_doc/xlm-prophetnet)
<!--End of the generated tip-->
</Tip>
์์ํ๊ธฐ ์ ์ ํ์ํ ๋ผ์ด๋ธ๋ฌ๋ฆฌ๊ฐ ๋ชจ๋ ์ค์น๋์ด ์๋์ง ํ์ธํ์ธ์:
```bash
pip install transformers datasets evaluate sacrebleu
```
๋ชจ๋ธ์ ์
๋ก๋ํ๊ณ ์ปค๋ฎค๋ํฐ์ ๊ณต์ ํ ์ ์๋๋ก Hugging Face ๊ณ์ ์ ๋ก๊ทธ์ธํ๋ ๊ฒ์ด ์ข์ต๋๋ค. ์๋ก์ด ์ฐฝ์ด ํ์๋๋ฉด ํ ํฐ์ ์
๋ ฅํ์ฌ ๋ก๊ทธ์ธํ์ธ์.
```py
>>> from huggingface_hub import notebook_login
>>> notebook_login()
```
## OPUS Books ๋ฐ์ดํฐ์ธํธ ๊ฐ์ ธ์ค๊ธฐ[[load-opus-books-dataset]]
๋จผ์ ๐ค Datasets ๋ผ์ด๋ธ๋ฌ๋ฆฌ์์ [OPUS Books](https://huggingface.co/datasets/opus_books) ๋ฐ์ดํฐ์ธํธ์ ์์ด-ํ๋์ค์ด ํ์ ์งํฉ์ ๊ฐ์ ธ์ค์ธ์.
```py
>>> from datasets import load_dataset
>>> books = load_dataset("opus_books", "en-fr")
```
๋ฐ์ดํฐ์ธํธ๋ฅผ [`~datasets.Dataset.train_test_split`] ๋ฉ์๋๋ฅผ ์ฌ์ฉํ์ฌ ํ๋ จ ๋ฐ ํ
์คํธ ๋ฐ์ดํฐ๋ก ๋ถํ ํ์ธ์.
```py
>>> books = books["train"].train_test_split(test_size=0.2)
```
ํ๋ จ ๋ฐ์ดํฐ์์ ์์๋ฅผ ์ดํด๋ณผ๊น์?
```py
>>> books["train"][0]
{'id': '90560',
'translation': {'en': 'But this lofty plateau measured only a few fathoms, and soon we reentered Our Element.',
'fr': 'Mais ce plateau รฉlevรฉ ne mesurait que quelques toises, et bientรดt nous fรปmes rentrรฉs dans notre รฉlรฉment.'}}
```
๋ฐํ๋ ๋์
๋๋ฆฌ์ `translation` ํค๊ฐ ํ
์คํธ์ ์์ด, ํ๋์ค์ด ๋ฒ์ ์ ํฌํจํ๊ณ ์๋ ๊ฒ์ ๋ณผ ์ ์์ต๋๋ค.
## ์ ์ฒ๋ฆฌ[[preprocess]]
<Youtube id="XAR8jnZZuUs"/>
๋ค์ ๋จ๊ณ๋ก ์์ด-ํ๋์ค์ด ์์ ์ฒ๋ฆฌํ๊ธฐ ์ํด T5 ํ ํฌ๋์ด์ ๋ฅผ ๊ฐ์ ธ์ค์ธ์.
```py
>>> from transformers import AutoTokenizer
>>> checkpoint = "t5-small"
>>> tokenizer = AutoTokenizer.from_pretrained(checkpoint)
```
๋ง๋ค ์ ์ฒ๋ฆฌ ํจ์๋ ์๋ ์๊ตฌ์ฌํญ์ ์ถฉ์กฑํด์ผ ํฉ๋๋ค:
1. T5๊ฐ ๋ฒ์ญ ํ์คํฌ์์ ์ธ์งํ ์ ์๋๋ก ์
๋ ฅ ์์ ํ๋กฌํํธ๋ฅผ ์ถ๊ฐํ์ธ์. ์ฌ๋ฌ NLP ํ์คํฌ๋ฅผ ํ ์ ์๋ ๋ชจ๋ธ ์ค ์ผ๋ถ๋ ์ด๋ ๊ฒ ํ์คํฌ ํ๋กฌํํธ๋ฅผ ๋ฏธ๋ฆฌ ์ค์ผํฉ๋๋ค.
2. ์์ด(์์ด)๊ณผ ๋ฒ์ญ์ด(ํ๋์ค์ด)๋ฅผ ๋ณ๋๋ก ํ ํฐํํ์ธ์. ์์ด ์ดํ๋ก ์ฌ์ ํ์ต๋ ํ ํฌ๋์ด์ ๋ก ํ๋์ค์ด ํ
์คํธ๋ฅผ ํ ํฐํํ ์๋ ์๊ธฐ ๋๋ฌธ์
๋๋ค.
3. `max_length` ๋งค๊ฐ๋ณ์๋ก ์ค์ ํ ์ต๋ ๊ธธ์ด๋ณด๋ค ๊ธธ์ง ์๋๋ก ์ํ์ค๋ฅผ truncateํ์ธ์.
```py
>>> source_lang = "en"
>>> target_lang = "fr"
>>> prefix = "translate English to French: "
>>> def preprocess_function(examples):
... inputs = [prefix + example[source_lang] for example in examples["translation"]]
... targets = [example[target_lang] for example in examples["translation"]]
... model_inputs = tokenizer(inputs, text_target=targets, max_length=128, truncation=True)
... return model_inputs
```
์ ์ฒด ๋ฐ์ดํฐ์ธํธ์ ์ ์ฒ๋ฆฌ ํจ์๋ฅผ ์ ์ฉํ๋ ค๋ฉด ๐ค Datasets์ [`~datasets.Dataset.map`] ๋ฉ์๋๋ฅผ ์ฌ์ฉํ์ธ์. `map` ํจ์์ ์๋๋ฅผ ๋์ด๋ ค๋ฉด `batched=True`๋ฅผ ์ค์ ํ์ฌ ๋ฐ์ดํฐ์ธํธ์ ์ฌ๋ฌ ์์๋ฅผ ํ ๋ฒ์ ์ฒ๋ฆฌํ๋ ๋ฐฉ๋ฒ์ด ์์ต๋๋ค.
```py
>>> tokenized_books = books.map(preprocess_function, batched=True)
```
์ด์ [`DataCollatorForSeq2Seq`]๋ฅผ ์ฌ์ฉํ์ฌ ์์ ๋ฐฐ์น๋ฅผ ์์ฑํฉ๋๋ค. ๋ฐ์ดํฐ์ธํธ์ ์ต๋ ๊ธธ์ด๋ก ์ ๋ถ๋ฅผ paddingํ๋ ๋์ , ๋ฐ์ดํฐ ์ ๋ ฌ ์ค ๊ฐ ๋ฐฐ์น์ ์ต๋ ๊ธธ์ด๋ก ๋ฌธ์ฅ์ *๋์ ์ผ๋ก padding*ํ๋ ๊ฒ์ด ๋ ํจ์จ์ ์
๋๋ค.
<frameworkcontent>
<pt>
```py
>>> from transformers import DataCollatorForSeq2Seq
>>> data_collator = DataCollatorForSeq2Seq(tokenizer=tokenizer, model=checkpoint)
```
</pt>
<tf>
```py
>>> from transformers import DataCollatorForSeq2Seq
>>> data_collator = DataCollatorForSeq2Seq(tokenizer=tokenizer, model=checkpoint, return_tensors="tf")
```
</tf>
</frameworkcontent>
## ํ๊ฐ[[evalulate]]
ํ๋ จ ์ค์ ๋ฉํธ๋ฆญ์ ํฌํจํ๋ฉด ๋ชจ๋ธ์ ์ฑ๋ฅ์ ํ๊ฐํ๋ ๋ฐ ๋์์ด ๋ฉ๋๋ค. ๐ค [Evaluate](https://huggingface.co/docs/evaluate/index) ๋ผ์ด๋ธ๋ฌ๋ฆฌ๋ก ํ๊ฐ ๋ฐฉ๋ฒ(evaluation method)์ ๋น ๋ฅด๊ฒ ๊ฐ์ ธ์ฌ ์ ์์ต๋๋ค. ํ์ฌ ํ์คํฌ์ ์ ํฉํ SacreBLEU ๋ฉํธ๋ฆญ์ ๊ฐ์ ธ์ค์ธ์. (๋ฉํธ๋ฆญ์ ๊ฐ์ ธ์ค๊ณ ๊ณ์ฐํ๋ ๋ฐฉ๋ฒ์ ๋ํด ์์ธํ ์์๋ณด๋ ค๋ฉด ๐ค Evaluate [๋๋ฌ๋ณด๊ธฐ](https://huggingface.co/docs/evaluate/a_quick_tour)๋ฅผ ์ฐธ์กฐํ์ธ์):
```py
>>> import evaluate
>>> metric = evaluate.load("sacrebleu")
```
๊ทธ๋ฐ ๋ค์ [`~evaluate.EvaluationModule.compute`]์ ์์ธก๊ฐ๊ณผ ๋ ์ด๋ธ์ ์ ๋ฌํ์ฌ SacreBLEU ์ ์๋ฅผ ๊ณ์ฐํ๋ ํจ์๋ฅผ ์์ฑํ์ธ์:
```py
>>> import numpy as np
>>> def postprocess_text(preds, labels):
... preds = [pred.strip() for pred in preds]
... labels = [[label.strip()] for label in labels]
... return preds, labels
>>> def compute_metrics(eval_preds):
... preds, labels = eval_preds
... if isinstance(preds, tuple):
... preds = preds[0]
... decoded_preds = tokenizer.batch_decode(preds, skip_special_tokens=True)
... labels = np.where(labels != -100, labels, tokenizer.pad_token_id)
... decoded_labels = tokenizer.batch_decode(labels, skip_special_tokens=True)
... decoded_preds, decoded_labels = postprocess_text(decoded_preds, decoded_labels)
... result = metric.compute(predictions=decoded_preds, references=decoded_labels)
... result = {"bleu": result["score"]}
... prediction_lens = [np.count_nonzero(pred != tokenizer.pad_token_id) for pred in preds]
... result["gen_len"] = np.mean(prediction_lens)
... result = {k: round(v, 4) for k, v in result.items()}
... return result
```
์ด์ `compute_metrics` ํจ์๋ ์ค๋น๋์๊ณ , ํ๋ จ ๊ณผ์ ์ ์ค์ ํ ๋ ๋ค์ ์ดํด๋ณผ ์์ ์
๋๋ค.
## ํ๋ จ[[train]]
<frameworkcontent>
<pt>
<Tip>
[`Trainer`]๋ก ๋ชจ๋ธ์ ํ์ธํ๋ํ๋ ๋ฐฉ๋ฒ์ ์ต์ํ์ง ์๋ค๋ฉด [์ฌ๊ธฐ](../training#train-with-pytorch-trainer)์์ ๊ธฐ๋ณธ ํํ ๋ฆฌ์ผ์ ์ดํด๋ณด์๊ธฐ ๋ฐ๋๋๋ค!
</Tip>
๋ชจ๋ธ์ ํ๋ จ์ํฌ ์ค๋น๊ฐ ๋์๊ตฐ์! [`AutoModelForSeq2SeqLM`]์ผ๋ก T5๋ฅผ ๋ก๋ํ์ธ์:
```py
>>> from transformers import AutoModelForSeq2SeqLM, Seq2SeqTrainingArguments, Seq2SeqTrainer
>>> model = AutoModelForSeq2SeqLM.from_pretrained(checkpoint)
```
์ด์ ์ธ ๋จ๊ณ๋ง ๊ฑฐ์น๋ฉด ๋์
๋๋ค:
1. [`Seq2SeqTrainingArguments`]์์ ํ๋ จ ํ์ดํผํ๋ผ๋ฏธํฐ๋ฅผ ์ ์ํ์ธ์. ์ ์ผํ ํ์ ๋งค๊ฐ๋ณ์๋ ๋ชจ๋ธ์ ์ ์ฅํ ์์น์ธ `output_dir`์
๋๋ค. ๋ชจ๋ธ์ Hub์ ํธ์ํ๊ธฐ ์ํด `push_to_hub=True`๋ก ์ค์ ํ์ธ์. (๋ชจ๋ธ์ ์
๋ก๋ํ๋ ค๋ฉด Hugging Face์ ๋ก๊ทธ์ธํด์ผ ํฉ๋๋ค.) [`Trainer`]๋ ์ํญ์ด ๋๋ ๋๋ง๋ค SacreBLEU ๋ฉํธ๋ฆญ์ ํ๊ฐํ๊ณ ํ๋ จ ์ฒดํฌํฌ์ธํธ๋ฅผ ์ ์ฅํฉ๋๋ค.
2. [`Seq2SeqTrainer`]์ ํ๋ จ ์ธ์๋ฅผ ์ ๋ฌํ์ธ์. ๋ชจ๋ธ, ๋ฐ์ดํฐ ์ธํธ, ํ ํฌ๋์ด์ , data collator ๋ฐ `compute_metrics` ํจ์๋ ๋ฉ๋ฌ์ ์ ๋ฌํด์ผ ํฉ๋๋ค.
3. [`~Trainer.train`]์ ํธ์ถํ์ฌ ๋ชจ๋ธ์ ํ์ธํ๋ํ์ธ์.
```py
>>> training_args = Seq2SeqTrainingArguments(
... output_dir="my_awesome_opus_books_model",
... evaluation_strategy="epoch",
... learning_rate=2e-5,
... per_device_train_batch_size=16,
... per_device_eval_batch_size=16,
... weight_decay=0.01,
... save_total_limit=3,
... num_train_epochs=2,
... predict_with_generate=True,
... fp16=True,
... push_to_hub=True,
... )
>>> trainer = Seq2SeqTrainer(
... model=model,
... args=training_args,
... train_dataset=tokenized_books["train"],
... eval_dataset=tokenized_books["test"],
... tokenizer=tokenizer,
... data_collator=data_collator,
... compute_metrics=compute_metrics,
... )
>>> trainer.train()
````
ํ์ต์ด ์๋ฃ๋๋ฉด [`~transformers.Trainer.push_to_hub`] ๋ฉ์๋๋ก ๋ชจ๋ธ์ Hub์ ๊ณต์ ํ์ธ์. ์ด๋ฌ๋ฉด ๋๊ตฌ๋ ๋ชจ๋ธ์ ์ฌ์ฉํ ์ ์๊ฒ ๋ฉ๋๋ค:
```py
>>> trainer.push_to_hub()
```
</pt>
<tf>
<Tip>
Keras๋ก ๋ชจ๋ธ์ ํ์ธํ๋ํ๋ ๋ฐฉ๋ฒ์ด ์ต์ํ์ง ์๋ค๋ฉด, [์ฌ๊ธฐ](../training#train-a-tensorflow-model-with-keras)์์ ๊ธฐ๋ณธ ํํ ๋ฆฌ์ผ์ ์ดํด๋ณด์๊ธฐ ๋ฐ๋๋๋ค!
</Tip>
TensorFlow์์ ๋ชจ๋ธ์ ํ์ธํ๋ํ๋ ค๋ฉด ์ฐ์ optimizer ํจ์, ํ์ต๋ฅ ์ค์ผ์ค ๋ฑ์ ํ๋ จ ํ์ดํผํ๋ผ๋ฏธํฐ๋ฅผ ์ค์ ํ์ธ์:
```py
>>> from transformers import AdamWeightDecay
>>> optimizer = AdamWeightDecay(learning_rate=2e-5, weight_decay_rate=0.01)
```
์ด์ [`TFAutoModelForSeq2SeqLM`]๋ก T5๋ฅผ ๊ฐ์ ธ์ค์ธ์:
```py
>>> from transformers import TFAutoModelForSeq2SeqLM
>>> model = TFAutoModelForSeq2SeqLM.from_pretrained(checkpoint)
```
[`~transformers.TFPreTrainedModel.prepare_tf_dataset`]๋ก ๋ฐ์ดํฐ ์ธํธ๋ฅผ `tf.data.Dataset` ํ์์ผ๋ก ๋ณํํ์ธ์:
```py
>>> tf_train_set = model.prepare_tf_dataset(
... tokenized_books["train"],
... shuffle=True,
... batch_size=16,
... collate_fn=data_collator,
... )
>>> tf_test_set = model.prepare_tf_dataset(
... tokenized_books["test"],
... shuffle=False,
... batch_size=16,
... collate_fn=data_collator,
... )
```
ํ๋ จํ๊ธฐ ์ํด [`compile`](https://keras.io/api/models/model_training_apis/#compile-method) ๋ฉ์๋๋ก ๋ชจ๋ธ์ ๊ตฌ์ฑํ์ธ์:
```py
>>> import tensorflow as tf
>>> model.compile(optimizer=optimizer)
```
ํ๋ จ์ ์์ํ๊ธฐ ์ ์ ์์ธก๊ฐ์ผ๋ก๋ถํฐ SacreBLEU ๋ฉํธ๋ฆญ์ ๊ณ์ฐํ๋ ๋ฐฉ๋ฒ๊ณผ ๋ชจ๋ธ์ Hub์ ์
๋ก๋ํ๋ ๋ฐฉ๋ฒ ๋ ๊ฐ์ง๋ฅผ ๋ฏธ๋ฆฌ ์ค์ ํด๋ฌ์ผ ํฉ๋๋ค. ๋ ๋ค [Keras callbacks](../main_classes/keras_callbacks)๋ก ๊ตฌํํ์ธ์.
[`~transformers.KerasMetricCallback`]์ `compute_metrics` ํจ์๋ฅผ ์ ๋ฌํ์ธ์.
```py
>>> from transformers.keras_callbacks import KerasMetricCallback
>>> metric_callback = KerasMetricCallback(metric_fn=compute_metrics, eval_dataset=tf_validation_set)
```
๋ชจ๋ธ๊ณผ ํ ํฌ๋์ด์ ๋ฅผ ์
๋ก๋ํ ์์น๋ฅผ [`~transformers.PushToHubCallback`]์์ ์ง์ ํ์ธ์:
```py
>>> from transformers.keras_callbacks import PushToHubCallback
>>> push_to_hub_callback = PushToHubCallback(
... output_dir="my_awesome_opus_books_model",
... tokenizer=tokenizer,
... )
```
์ด์ ์ฝ๋ฐฑ๋ค์ ํ๋ฐ๋ก ๋ฌถ์ด์ฃผ์ธ์:
```py
>>> callbacks = [metric_callback, push_to_hub_callback]
```
๋๋์ด ๋ชจ๋ธ์ ํ๋ จ์ํฌ ๋ชจ๋ ์ค๋น๋ฅผ ๋ง์ณค๊ตฐ์! ์ด์ ํ๋ จ ๋ฐ ๊ฒ์ฆ ๋ฐ์ดํฐ ์ธํธ์ [`fit`](https://keras.io/api/models/model_training_apis/#fit-method) ๋ฉ์๋๋ฅผ ์ํญ ์์ ๋ง๋ค์ด๋ ์ฝ๋ฐฑ๊ณผ ํจ๊ป ํธ์ถํ์ฌ ๋ชจ๋ธ์ ํ์ธํ๋ํ์ธ์:
```py
>>> model.fit(x=tf_train_set, validation_data=tf_test_set, epochs=3, callbacks=callbacks)
```
ํ์ต์ด ์๋ฃ๋๋ฉด ๋ชจ๋ธ์ด ์๋์ผ๋ก Hub์ ์
๋ก๋๋๊ณ , ๋๊ตฌ๋ ์ฌ์ฉํ ์ ์๊ฒ ๋ฉ๋๋ค!
</tf>
</frameworkcontent>
<Tip>
๋ฒ์ญ์ ์ํด ๋ชจ๋ธ์ ํ์ธํ๋ํ๋ ๋ฐฉ๋ฒ์ ๋ํ ๋ณด๋ค ์์ธํ ์์ ๋ ํด๋น [PyTorch ๋
ธํธ๋ถ](https://colab.research.google.com/github/huggingface/notebooks/blob/main/examples/translation.ipynb) ๋๋ [TensorFlow ๋
ธํธ๋ถ](https://colab.research.google.com/github/huggingface/notebooks/blob/main/examples/translation-tf.ipynb)์ ์ฐธ์กฐํ์ธ์.
</Tip>
## ์ถ๋ก [[inference]]
์ข์์, ์ด์ ๋ชจ๋ธ์ ํ์ธํ๋ํ์ผ๋ ์ถ๋ก ์ ์ฌ์ฉํ ์ ์์ต๋๋ค!
๋ค๋ฅธ ์ธ์ด๋ก ๋ฒ์ญํ๊ณ ์ถ์ ํ
์คํธ๋ฅผ ์จ๋ณด์ธ์. T5์ ๊ฒฝ์ฐ ์ํ๋ ํ์คํฌ๋ฅผ ์
๋ ฅ์ ์ ๋์ฌ๋ก ์ถ๊ฐํด์ผ ํฉ๋๋ค. ์๋ฅผ ๋ค์ด ์์ด์์ ํ๋์ค์ด๋ก ๋ฒ์ญํ๋ ๊ฒฝ์ฐ, ์๋์ ๊ฐ์ ์ ๋์ฌ๊ฐ ์ถ๊ฐ๋ฉ๋๋ค:
```py
>>> text = "translate English to French: Legumes share resources with nitrogen-fixing bacteria."
```
ํ์ธํ๋๋ ๋ชจ๋ธ๋ก ์ถ๋ก ํ๊ธฐ์ ์ ์ผ ๊ฐ๋จํ ๋ฐฉ๋ฒ์ [`pipeline`]์ ์ฌ์ฉํ๋ ๊ฒ์
๋๋ค. ํด๋น ๋ชจ๋ธ๋ก ๋ฒ์ญ `pipeline`์ ๋ง๋ ๋ค, ํ
์คํธ๋ฅผ ์ ๋ฌํ์ธ์:
```py
>>> from transformers import pipeline
>>> translator = pipeline("translation", model="my_awesome_opus_books_model")
>>> translator(text)
[{'translation_text': 'Legumes partagent des ressources avec des bactรฉries azotantes.'}]
```
์ํ๋ค๋ฉด `pipeline`์ ๊ฒฐ๊ณผ๋ฅผ ์ง์ ๋ณต์ ํ ์๋ ์์ต๋๋ค:
<frameworkcontent>
<pt>
ํ
์คํธ๋ฅผ ํ ํฐํํ๊ณ `input_ids`๋ฅผ PyTorch ํ
์๋ก ๋ฐํํ์ธ์:
```py
>>> from transformers import AutoTokenizer
>>> tokenizer = AutoTokenizer.from_pretrained("my_awesome_opus_books_model")
>>> inputs = tokenizer(text, return_tensors="pt").input_ids
```
[`~transformers.generation_utils.GenerationMixin.generate`] ๋ฉ์๋๋ก ๋ฒ์ญ์ ์์ฑํ์ธ์. ๋ค์ํ ํ
์คํธ ์์ฑ ์ ๋ต ๋ฐ ์์ฑ์ ์ ์ดํ๊ธฐ ์ํ ๋งค๊ฐ๋ณ์์ ๋ํ ์์ธํ ๋ด์ฉ์ [Text Generation](../main_classes/text_generation) API๋ฅผ ์ดํด๋ณด์๊ธฐ ๋ฐ๋๋๋ค.
```py
>>> from transformers import AutoModelForSeq2SeqLM
>>> model = AutoModelForSeq2SeqLM.from_pretrained("my_awesome_opus_books_model")
>>> outputs = model.generate(inputs, max_new_tokens=40, do_sample=True, top_k=30, top_p=0.95)
```
์์ฑ๋ ํ ํฐ ID๋ค์ ๋ค์ ํ
์คํธ๋ก ๋์ฝ๋ฉํ์ธ์:
```py
>>> tokenizer.decode(outputs[0], skip_special_tokens=True)
'Les lignรฉes partagent des ressources avec des bactรฉries enfixant l'azote.'
```
</pt>
<tf>
ํ
์คํธ๋ฅผ ํ ํฐํํ๊ณ `input_ids`๋ฅผ TensorFlow ํ
์๋ก ๋ฐํํ์ธ์:
```py
>>> from transformers import AutoTokenizer
>>> tokenizer = AutoTokenizer.from_pretrained("my_awesome_opus_books_model")
>>> inputs = tokenizer(text, return_tensors="tf").input_ids
```
[`~transformers.generation_tf_utils.TFGenerationMixin.generate`] ๋ฉ์๋๋ก ๋ฒ์ญ์ ์์ฑํ์ธ์. ๋ค์ํ ํ
์คํธ ์์ฑ ์ ๋ต ๋ฐ ์์ฑ์ ์ ์ดํ๊ธฐ ์ํ ๋งค๊ฐ๋ณ์์ ๋ํ ์์ธํ ๋ด์ฉ์ [Text Generation](../main_classes/text_generation) API๋ฅผ ์ดํด๋ณด์๊ธฐ ๋ฐ๋๋๋ค.
```py
>>> from transformers import TFAutoModelForSeq2SeqLM
>>> model = TFAutoModelForSeq2SeqLM.from_pretrained("my_awesome_opus_books_model")
>>> outputs = model.generate(inputs, max_new_tokens=40, do_sample=True, top_k=30, top_p=0.95)
```
์์ฑ๋ ํ ํฐ ID๋ค์ ๋ค์ ํ
์คํธ๋ก ๋์ฝ๋ฉํ์ธ์:
```py
>>> tokenizer.decode(outputs[0], skip_special_tokens=True)
'Les lugumes partagent les ressources avec des bactรฉries fixatrices d'azote.'
```
</tf>
</frameworkcontent>
| 0 |
hf_public_repos/transformers/docs/source | hf_public_repos/transformers/docs/source/ms/index.md | <!--Copyright 2020 The HuggingFace Team. All rights reserved.
Dilesenkan di bawah Lesen Apache, Versi 2.0 ("Lesen"); anda tidak boleh menggunakan fail ini kecuali dengan mematuhi
Lesen. Anda boleh mendapatkan salinan Lesen di
http://www.apache.org/licenses/LICENSE-2.0
Melainkan diperlukan oleh undang-undang yang terpakai atau dipersetujui secara bertulis, perisian yang diedarkan di bawah Lesen diedarkan pada
ASAS ""SEBAGAIMANA ADANYA"", TANPA WARANTI ATAU SEBARANG JENIS SYARAT, sama ada nyata atau tersirat. Lihat Lesen untuk
bahasa tertentu yang mengawal kebenaran dan pengehadan di bawah Lesen.
โ ๏ธ Note that this file is in Markdown but contain specific syntax for our doc-builder (similar to MDX) that may not be
rendered properly in your Markdown viewer.
-->
# ๐ค Transformers
Pembelajaran Mesin terkini untuk [PyTorch](https://pytorch.org/), [TensorFlow](https://www.tensorflow.org/), dan [JAX](https://jax.readthedocs.io/en/latest/).
๐ค Transformers menyediakan API dan alatan untuk memuat turun dan melatih model pra-latihan terkini dengan mudah. Menggunakan model terlatih boleh mengurangkan kos pengiraan anda, jejak karbon dan menjimatkan masa serta sumber yang diperlukan untuk melatih model dari awal. Model ini menyokong tugas biasa dalam modaliti yang berbeza, seperti:
๐ **Natural Language Processing**: klasifikasi teks, pengecaman entiti bernama, menjawab soalan, pemodelan bahasa, ringkasan, terjemahan, pilihan berganda dan penjanaan teks.<br>
๐ผ๏ธ **Computer Vision**: pengelasan imej, pengesanan objek dan pembahagian.<br>
๐ฃ๏ธ **Audio**: pengecaman pertuturan automatik dan klasifikasi audio.<br>
๐ **Multimodal**: jawapan soalan jadual, pengecaman aksara optik, pengekstrakan maklumat daripada dokumen yang diimbas, klasifikasi video dan jawapan soalan visual.
๐ค Transformer menyokong kebolehoperasian rangka kerja antara PyTorch, TensorFlow, and JAX. Ini memberikan fleksibiliti untuk menggunakan rangka kerja yang berbeza pada setiap peringkat kehidupan model; latih model dalam tiga baris kod dalam satu rangka kerja, dan muatkannya untuk inferens dalam rangka kerja yang lain. Model juga boleh dieksport ke format seperti ONNX.
Sertai komuniti yang semakin berkembang di [Hub](https://huggingface.co/models), [forum](https://discuss.huggingface.co/), atau [Discord](https://discord.com/invite/JfAtkvEtRb) hari ini!
## Jika anda sedang mencari sokongan tersuai daripada pasukan Hugging Face
<a target="_blank" href="https://huggingface.co/support">
<img alt="HuggingFace Expert Acceleration Program" src="https://cdn-media.huggingface.co/marketing/transformers/new-support-improved.png" style="width: 100%; max-width: 600px; border: 1px solid #eee; border-radius: 4px; box-shadow: 0 1px 2px 0 rgba(0, 0, 0, 0.05);">
</a>
## Kandungan
Dokumentasi disusun kepada lima bahagian:
- **MULAKAN** menyediakan lawatan pantas ke perpustakaan dan arahan pemasangan untuk bangun dan berjalan.
- **TUTORIAL** ialah tempat yang bagus untuk bermula jika anda seorang pemula. Bahagian ini akan membantu anda memperoleh kemahiran asas yang anda perlukan untuk mula menggunakan perpustakaan.
- **PANDUAN CARA-CARA** menunjukkan kepada anda cara untuk mencapai matlamat tertentu, seperti memperhalusi model terlatih untuk pemodelan bahasa atau cara menulis dan berkongsi model tersuai.
- **PANDUAN KONSEP** menawarkan lebih banyak perbincangan dan penjelasan tentang konsep dan idea asas di sebalik model, tugasan dan falsafah reka bentuk ๐ค Transformers.
- **API** menerangkan semua kelas dan fungsi:
- **KELAS UTAMA** memperincikan kelas yang paling penting seperti konfigurasi, model, tokenizer dan saluran paip.
- **MODEL** memperincikan kelas dan fungsi yang berkaitan dengan setiap model yang dilaksanakan dalam perpustakaan.
- **PEMBANTU DALAMAN** memperincikan kelas utiliti dan fungsi yang digunakan secara dalaman.
### Model yang disokong
<!--Senarai ini dikemas kini secara automatik daripada README dengan _make fix-copies_. Jangan kemas kini secara manual! -->
1. **[ALBERT](model_doc/albert)** (from Google Research and the Toyota Technological Institute at Chicago) released with the paper [ALBERT: A Lite BERT for Self-supervised Learning of Language Representations](https://arxiv.org/abs/1909.11942), by Zhenzhong Lan, Mingda Chen, Sebastian Goodman, Kevin Gimpel, Piyush Sharma, Radu Soricut.
1. **[ALIGN](model_doc/align)** (from Google Research) released with the paper [Scaling Up Visual and Vision-Language Representation Learning With Noisy Text Supervision](https://arxiv.org/abs/2102.05918) by Chao Jia, Yinfei Yang, Ye Xia, Yi-Ting Chen, Zarana Parekh, Hieu Pham, Quoc V. Le, Yunhsuan Sung, Zhen Li, Tom Duerig.
1. **[AltCLIP](model_doc/altclip)** (from BAAI) released with the paper [AltCLIP: Altering the Language Encoder in CLIP for Extended Language Capabilities](https://arxiv.org/abs/2211.06679) by Chen, Zhongzhi and Liu, Guang and Zhang, Bo-Wen and Ye, Fulong and Yang, Qinghong and Wu, Ledell.
1. **[Audio Spectrogram Transformer](model_doc/audio-spectrogram-transformer)** (from MIT) released with the paper [AST: Audio Spectrogram Transformer](https://arxiv.org/abs/2104.01778) by Yuan Gong, Yu-An Chung, James Glass.
1. **[Autoformer](model_doc/autoformer)** (from Tsinghua University) released with the paper [Autoformer: Decomposition Transformers with Auto-Correlation for Long-Term Series Forecasting](https://arxiv.org/abs/2106.13008) by Haixu Wu, Jiehui Xu, Jianmin Wang, Mingsheng Long.
1. **[BART](model_doc/bart)** (from Facebook) released with the paper [BART: Denoising Sequence-to-Sequence Pre-training for Natural Language Generation, Translation, and Comprehension](https://arxiv.org/abs/1910.13461) by Mike Lewis, Yinhan Liu, Naman Goyal, Marjan Ghazvininejad, Abdelrahman Mohamed, Omer Levy, Ves Stoyanov and Luke Zettlemoyer.
1. **[BARThez](model_doc/barthez)** (from รcole polytechnique) released with the paper [BARThez: a Skilled Pretrained French Sequence-to-Sequence Model](https://arxiv.org/abs/2010.12321) by Moussa Kamal Eddine, Antoine J.-P. Tixier, Michalis Vazirgiannis.
1. **[BARTpho](model_doc/bartpho)** (from VinAI Research) released with the paper [BARTpho: Pre-trained Sequence-to-Sequence Models for Vietnamese](https://arxiv.org/abs/2109.09701) by Nguyen Luong Tran, Duong Minh Le and Dat Quoc Nguyen.
1. **[BEiT](model_doc/beit)** (from Microsoft) released with the paper [BEiT: BERT Pre-Training of Image Transformers](https://arxiv.org/abs/2106.08254) by Hangbo Bao, Li Dong, Furu Wei.
1. **[BERT](model_doc/bert)** (from Google) released with the paper [BERT: Pre-training of Deep Bidirectional Transformers for Language Understanding](https://arxiv.org/abs/1810.04805) by Jacob Devlin, Ming-Wei Chang, Kenton Lee and Kristina Toutanova.
1. **[BERT For Sequence Generation](model_doc/bert-generation)** (from Google) released with the paper [Leveraging Pre-trained Checkpoints for Sequence Generation Tasks](https://arxiv.org/abs/1907.12461) by Sascha Rothe, Shashi Narayan, Aliaksei Severyn.
1. **[BERTweet](model_doc/bertweet)** (from VinAI Research) released with the paper [BERTweet: A pre-trained language model for English Tweets](https://aclanthology.org/2020.emnlp-demos.2/) by Dat Quoc Nguyen, Thanh Vu and Anh Tuan Nguyen.
1. **[BigBird-Pegasus](model_doc/bigbird_pegasus)** (from Google Research) released with the paper [Big Bird: Transformers for Longer Sequences](https://arxiv.org/abs/2007.14062) by Manzil Zaheer, Guru Guruganesh, Avinava Dubey, Joshua Ainslie, Chris Alberti, Santiago Ontanon, Philip Pham, Anirudh Ravula, Qifan Wang, Li Yang, Amr Ahmed.
1. **[BigBird-RoBERTa](model_doc/big_bird)** (from Google Research) released with the paper [Big Bird: Transformers for Longer Sequences](https://arxiv.org/abs/2007.14062) by Manzil Zaheer, Guru Guruganesh, Avinava Dubey, Joshua Ainslie, Chris Alberti, Santiago Ontanon, Philip Pham, Anirudh Ravula, Qifan Wang, Li Yang, Amr Ahmed.
1. **[BioGpt](model_doc/biogpt)** (from Microsoft Research AI4Science) released with the paper [BioGPT: generative pre-trained transformer for biomedical text generation and mining](https://academic.oup.com/bib/advance-article/doi/10.1093/bib/bbac409/6713511?guestAccessKey=a66d9b5d-4f83-4017-bb52-405815c907b9) by Renqian Luo, Liai Sun, Yingce Xia, Tao Qin, Sheng Zhang, Hoifung Poon and Tie-Yan Liu.
1. **[BiT](model_doc/bit)** (from Google AI) released with the paper [Big Transfer (BiT): General Visual Representation Learning](https://arxiv.org/abs/1912.11370) by Alexander Kolesnikov, Lucas Beyer, Xiaohua Zhai, Joan Puigcerver, Jessica Yung, Sylvain Gelly, Neil Houlsby.
1. **[Blenderbot](model_doc/blenderbot)** (from Facebook) released with the paper [Recipes for building an open-domain chatbot](https://arxiv.org/abs/2004.13637) by Stephen Roller, Emily Dinan, Naman Goyal, Da Ju, Mary Williamson, Yinhan Liu, Jing Xu, Myle Ott, Kurt Shuster, Eric M. Smith, Y-Lan Boureau, Jason Weston.
1. **[BlenderbotSmall](model_doc/blenderbot-small)** (from Facebook) released with the paper [Recipes for building an open-domain chatbot](https://arxiv.org/abs/2004.13637) by Stephen Roller, Emily Dinan, Naman Goyal, Da Ju, Mary Williamson, Yinhan Liu, Jing Xu, Myle Ott, Kurt Shuster, Eric M. Smith, Y-Lan Boureau, Jason Weston.
1. **[BLIP](model_doc/blip)** (from Salesforce) released with the paper [BLIP: Bootstrapping Language-Image Pre-training for Unified Vision-Language Understanding and Generation](https://arxiv.org/abs/2201.12086) by Junnan Li, Dongxu Li, Caiming Xiong, Steven Hoi.
1. **[BLIP-2](model_doc/blip-2)** (from Salesforce) released with the paper [BLIP-2: Bootstrapping Language-Image Pre-training with Frozen Image Encoders and Large Language Models](https://arxiv.org/abs/2301.12597) by Junnan Li, Dongxu Li, Silvio Savarese, Steven Hoi.
1. **[BLOOM](model_doc/bloom)** (from BigScience workshop) released by the [BigScience Workshop](https://bigscience.huggingface.co/).
1. **[BORT](model_doc/bort)** (from Alexa) released with the paper [Optimal Subarchitecture Extraction For BERT](https://arxiv.org/abs/2010.10499) by Adrian de Wynter and Daniel J. Perry.
1. **[BridgeTower](model_doc/bridgetower)** (from Harbin Institute of Technology/Microsoft Research Asia/Intel Labs) released with the paper [BridgeTower: Building Bridges Between Encoders in Vision-Language Representation Learning](https://arxiv.org/abs/2206.08657) by Xiao Xu, Chenfei Wu, Shachar Rosenman, Vasudev Lal, Wanxiang Che, Nan Duan.
1. **[ByT5](model_doc/byt5)** (from Google Research) released with the paper [ByT5: Towards a token-free future with pre-trained byte-to-byte models](https://arxiv.org/abs/2105.13626) by Linting Xue, Aditya Barua, Noah Constant, Rami Al-Rfou, Sharan Narang, Mihir Kale, Adam Roberts, Colin Raffel.
1. **[CamemBERT](model_doc/camembert)** (from Inria/Facebook/Sorbonne) released with the paper [CamemBERT: a Tasty French Language Model](https://arxiv.org/abs/1911.03894) by Louis Martin*, Benjamin Muller*, Pedro Javier Ortiz Suรกrez*, Yoann Dupont, Laurent Romary, รric Villemonte de la Clergerie, Djamรฉ Seddah and Benoรฎt Sagot.
1. **[CANINE](model_doc/canine)** (from Google Research) released with the paper [CANINE: Pre-training an Efficient Tokenization-Free Encoder for Language Representation](https://arxiv.org/abs/2103.06874) by Jonathan H. Clark, Dan Garrette, Iulia Turc, John Wieting.
1. **[Chinese-CLIP](model_doc/chinese_clip)** (from OFA-Sys) released with the paper [Chinese CLIP: Contrastive Vision-Language Pretraining in Chinese](https://arxiv.org/abs/2211.01335) by An Yang, Junshu Pan, Junyang Lin, Rui Men, Yichang Zhang, Jingren Zhou, Chang Zhou.
1. **[CLAP](model_doc/clap)** (from LAION-AI) released with the paper [Large-scale Contrastive Language-Audio Pretraining with Feature Fusion and Keyword-to-Caption Augmentation](https://arxiv.org/abs/2211.06687) by Yusong Wu, Ke Chen, Tianyu Zhang, Yuchen Hui, Taylor Berg-Kirkpatrick, Shlomo Dubnov.
1. **[CLIP](model_doc/clip)** (from OpenAI) released with the paper [Learning Transferable Visual Models From Natural Language Supervision](https://arxiv.org/abs/2103.00020) by Alec Radford, Jong Wook Kim, Chris Hallacy, Aditya Ramesh, Gabriel Goh, Sandhini Agarwal, Girish Sastry, Amanda Askell, Pamela Mishkin, Jack Clark, Gretchen Krueger, Ilya Sutskever.
1. **[CLIPSeg](model_doc/clipseg)** (from University of Gรถttingen) released with the paper [Image Segmentation Using Text and Image Prompts](https://arxiv.org/abs/2112.10003) by Timo Lรผddecke and Alexander Ecker.
1. **[CodeGen](model_doc/codegen)** (from Salesforce) released with the paper [A Conversational Paradigm for Program Synthesis](https://arxiv.org/abs/2203.13474) by Erik Nijkamp, Bo Pang, Hiroaki Hayashi, Lifu Tu, Huan Wang, Yingbo Zhou, Silvio Savarese, Caiming Xiong.
1. **[Conditional DETR](model_doc/conditional_detr)** (from Microsoft Research Asia) released with the paper [Conditional DETR for Fast Training Convergence](https://arxiv.org/abs/2108.06152) by Depu Meng, Xiaokang Chen, Zejia Fan, Gang Zeng, Houqiang Li, Yuhui Yuan, Lei Sun, Jingdong Wang.
1. **[ConvBERT](model_doc/convbert)** (from YituTech) released with the paper [ConvBERT: Improving BERT with Span-based Dynamic Convolution](https://arxiv.org/abs/2008.02496) by Zihang Jiang, Weihao Yu, Daquan Zhou, Yunpeng Chen, Jiashi Feng, Shuicheng Yan.
1. **[ConvNeXT](model_doc/convnext)** (from Facebook AI) released with the paper [A ConvNet for the 2020s](https://arxiv.org/abs/2201.03545) by Zhuang Liu, Hanzi Mao, Chao-Yuan Wu, Christoph Feichtenhofer, Trevor Darrell, Saining Xie.
1. **[ConvNeXTV2](model_doc/convnextv2)** (from Facebook AI) released with the paper [ConvNeXt V2: Co-designing and Scaling ConvNets with Masked Autoencoders](https://arxiv.org/abs/2301.00808) by Sanghyun Woo, Shoubhik Debnath, Ronghang Hu, Xinlei Chen, Zhuang Liu, In So Kweon, Saining Xie.
1. **[CPM](model_doc/cpm)** (from Tsinghua University) released with the paper [CPM: A Large-scale Generative Chinese Pre-trained Language Model](https://arxiv.org/abs/2012.00413) by Zhengyan Zhang, Xu Han, Hao Zhou, Pei Ke, Yuxian Gu, Deming Ye, Yujia Qin, Yusheng Su, Haozhe Ji, Jian Guan, Fanchao Qi, Xiaozhi Wang, Yanan Zheng, Guoyang Zeng, Huanqi Cao, Shengqi Chen, Daixuan Li, Zhenbo Sun, Zhiyuan Liu, Minlie Huang, Wentao Han, Jie Tang, Juanzi Li, Xiaoyan Zhu, Maosong Sun.
1. **[CPM-Ant](model_doc/cpmant)** (from OpenBMB) released by the [OpenBMB](https://www.openbmb.org/).
1. **[CTRL](model_doc/ctrl)** (from Salesforce) released with the paper [CTRL: A Conditional Transformer Language Model for Controllable Generation](https://arxiv.org/abs/1909.05858) by Nitish Shirish Keskar*, Bryan McCann*, Lav R. Varshney, Caiming Xiong and Richard Socher.
1. **[CvT](model_doc/cvt)** (from Microsoft) released with the paper [CvT: Introducing Convolutions to Vision Transformers](https://arxiv.org/abs/2103.15808) by Haiping Wu, Bin Xiao, Noel Codella, Mengchen Liu, Xiyang Dai, Lu Yuan, Lei Zhang.
1. **[Data2Vec](model_doc/data2vec)** (from Facebook) released with the paper [Data2Vec: A General Framework for Self-supervised Learning in Speech, Vision and Language](https://arxiv.org/abs/2202.03555) by Alexei Baevski, Wei-Ning Hsu, Qiantong Xu, Arun Babu, Jiatao Gu, Michael Auli.
1. **[DeBERTa](model_doc/deberta)** (from Microsoft) released with the paper [DeBERTa: Decoding-enhanced BERT with Disentangled Attention](https://arxiv.org/abs/2006.03654) by Pengcheng He, Xiaodong Liu, Jianfeng Gao, Weizhu Chen.
1. **[DeBERTa-v2](model_doc/deberta-v2)** (from Microsoft) released with the paper [DeBERTa: Decoding-enhanced BERT with Disentangled Attention](https://arxiv.org/abs/2006.03654) by Pengcheng He, Xiaodong Liu, Jianfeng Gao, Weizhu Chen.
1. **[Decision Transformer](model_doc/decision_transformer)** (from Berkeley/Facebook/Google) released with the paper [Decision Transformer: Reinforcement Learning via Sequence Modeling](https://arxiv.org/abs/2106.01345) by Lili Chen, Kevin Lu, Aravind Rajeswaran, Kimin Lee, Aditya Grover, Michael Laskin, Pieter Abbeel, Aravind Srinivas, Igor Mordatch.
1. **[Deformable DETR](model_doc/deformable_detr)** (from SenseTime Research) released with the paper [Deformable DETR: Deformable Transformers for End-to-End Object Detection](https://arxiv.org/abs/2010.04159) by Xizhou Zhu, Weijie Su, Lewei Lu, Bin Li, Xiaogang Wang, Jifeng Dai.
1. **[DeiT](model_doc/deit)** (from Facebook) released with the paper [Training data-efficient image transformers & distillation through attention](https://arxiv.org/abs/2012.12877) by Hugo Touvron, Matthieu Cord, Matthijs Douze, Francisco Massa, Alexandre Sablayrolles, Hervรฉ Jรฉgou.
1. **[DePlot](model_doc/deplot)** (from Google AI) released with the paper [DePlot: One-shot visual language reasoning by plot-to-table translation](https://arxiv.org/abs/2212.10505) by Fangyu Liu, Julian Martin Eisenschlos, Francesco Piccinno, Syrine Krichene, Chenxi Pang, Kenton Lee, Mandar Joshi, Wenhu Chen, Nigel Collier, Yasemin Altun.
1. **[DETA](model_doc/deta)** (from The University of Texas at Austin) released with the paper [NMS Strikes Back](https://arxiv.org/abs/2212.06137) by Jeffrey Ouyang-Zhang, Jang Hyun Cho, Xingyi Zhou, Philipp Krรคhenbรผhl.
1. **[DETR](model_doc/detr)** (from Facebook) released with the paper [End-to-End Object Detection with Transformers](https://arxiv.org/abs/2005.12872) by Nicolas Carion, Francisco Massa, Gabriel Synnaeve, Nicolas Usunier, Alexander Kirillov, Sergey Zagoruyko.
1. **[DialoGPT](model_doc/dialogpt)** (from Microsoft Research) released with the paper [DialoGPT: Large-Scale Generative Pre-training for Conversational Response Generation](https://arxiv.org/abs/1911.00536) by Yizhe Zhang, Siqi Sun, Michel Galley, Yen-Chun Chen, Chris Brockett, Xiang Gao, Jianfeng Gao, Jingjing Liu, Bill Dolan.
1. **[DiNAT](model_doc/dinat)** (from SHI Labs) released with the paper [Dilated Neighborhood Attention Transformer](https://arxiv.org/abs/2209.15001) by Ali Hassani and Humphrey Shi.
1. **[DistilBERT](model_doc/distilbert)** (from HuggingFace), released together with the paper [DistilBERT, a distilled version of BERT: smaller, faster, cheaper and lighter](https://arxiv.org/abs/1910.01108) by Victor Sanh, Lysandre Debut and Thomas Wolf. The same method has been applied to compress GPT2 into [DistilGPT2](https://github.com/huggingface/transformers/tree/main/examples/research_projects/distillation), RoBERTa into [DistilRoBERTa](https://github.com/huggingface/transformers/tree/main/examples/research_projects/distillation), Multilingual BERT into [DistilmBERT](https://github.com/huggingface/transformers/tree/main/examples/research_projects/distillation) and a German version of DistilBERT.
1. **[DiT](model_doc/dit)** (from Microsoft Research) released with the paper [DiT: Self-supervised Pre-training for Document Image Transformer](https://arxiv.org/abs/2203.02378) by Junlong Li, Yiheng Xu, Tengchao Lv, Lei Cui, Cha Zhang, Furu Wei.
1. **[Donut](model_doc/donut)** (from NAVER), released together with the paper [OCR-free Document Understanding Transformer](https://arxiv.org/abs/2111.15664) by Geewook Kim, Teakgyu Hong, Moonbin Yim, Jeongyeon Nam, Jinyoung Park, Jinyeong Yim, Wonseok Hwang, Sangdoo Yun, Dongyoon Han, Seunghyun Park.
1. **[DPR](model_doc/dpr)** (from Facebook) released with the paper [Dense Passage Retrieval for Open-Domain Question Answering](https://arxiv.org/abs/2004.04906) by Vladimir Karpukhin, Barlas Oฤuz, Sewon Min, Patrick Lewis, Ledell Wu, Sergey Edunov, Danqi Chen, and Wen-tau Yih.
1. **[DPT](master/model_doc/dpt)** (from Intel Labs) released with the paper [Vision Transformers for Dense Prediction](https://arxiv.org/abs/2103.13413) by Renรฉ Ranftl, Alexey Bochkovskiy, Vladlen Koltun.
1. **[EfficientFormer](model_doc/efficientformer)** (from Snap Research) released with the paper [EfficientFormer: Vision Transformers at MobileNetSpeed](https://arxiv.org/abs/2206.01191) by Yanyu Li, Geng Yuan, Yang Wen, Ju Hu, Georgios Evangelidis, Sergey Tulyakov, Yanzhi Wang, Jian Ren.
1. **[EfficientNet](model_doc/efficientnet)** (from Google Brain) released with the paper [EfficientNet: Rethinking Model Scaling for Convolutional Neural Networks](https://arxiv.org/abs/1905.11946) by Mingxing Tan, Quoc V. Le.
1. **[ELECTRA](model_doc/electra)** (from Google Research/Stanford University) released with the paper [ELECTRA: Pre-training text encoders as discriminators rather than generators](https://arxiv.org/abs/2003.10555) by Kevin Clark, Minh-Thang Luong, Quoc V. Le, Christopher D. Manning.
1. **[EncoderDecoder](model_doc/encoder-decoder)** (from Google Research) released with the paper [Leveraging Pre-trained Checkpoints for Sequence Generation Tasks](https://arxiv.org/abs/1907.12461) by Sascha Rothe, Shashi Narayan, Aliaksei Severyn.
1. **[ERNIE](model_doc/ernie)** (from Baidu) released with the paper [ERNIE: Enhanced Representation through Knowledge Integration](https://arxiv.org/abs/1904.09223) by Yu Sun, Shuohuan Wang, Yukun Li, Shikun Feng, Xuyi Chen, Han Zhang, Xin Tian, Danxiang Zhu, Hao Tian, Hua Wu.
1. **[ErnieM](model_doc/ernie_m)** (from Baidu) released with the paper [ERNIE-M: Enhanced Multilingual Representation by Aligning Cross-lingual Semantics with Monolingual Corpora](https://arxiv.org/abs/2012.15674) by Xuan Ouyang, Shuohuan Wang, Chao Pang, Yu Sun, Hao Tian, Hua Wu, Haifeng Wang.
1. **[ESM](model_doc/esm)** (from Meta AI) are transformer protein language models. **ESM-1b** was released with the paper [Biological structure and function emerge from scaling unsupervised learning to 250 million protein sequences](https://www.pnas.org/content/118/15/e2016239118) by Alexander Rives, Joshua Meier, Tom Sercu, Siddharth Goyal, Zeming Lin, Jason Liu, Demi Guo, Myle Ott, C. Lawrence Zitnick, Jerry Ma, and Rob Fergus. **ESM-1v** was released with the paper [Language models enable zero-shot prediction of the effects of mutations on protein function](https://doi.org/10.1101/2021.07.09.450648) by Joshua Meier, Roshan Rao, Robert Verkuil, Jason Liu, Tom Sercu and Alexander Rives. **ESM-2 and ESMFold** were released with the paper [Language models of protein sequences at the scale of evolution enable accurate structure prediction](https://doi.org/10.1101/2022.07.20.500902) by Zeming Lin, Halil Akin, Roshan Rao, Brian Hie, Zhongkai Zhu, Wenting Lu, Allan dos Santos Costa, Maryam Fazel-Zarandi, Tom Sercu, Sal Candido, Alexander Rives.
1. **[FLAN-T5](model_doc/flan-t5)** (from Google AI) released in the repository [google-research/t5x](https://github.com/google-research/t5x/blob/main/docs/models.md#flan-t5-checkpoints) by Hyung Won Chung, Le Hou, Shayne Longpre, Barret Zoph, Yi Tay, William Fedus, Eric Li, Xuezhi Wang, Mostafa Dehghani, Siddhartha Brahma, Albert Webson, Shixiang Shane Gu, Zhuyun Dai, Mirac Suzgun, Xinyun Chen, Aakanksha Chowdhery, Sharan Narang, Gaurav Mishra, Adams Yu, Vincent Zhao, Yanping Huang, Andrew Dai, Hongkun Yu, Slav Petrov, Ed H. Chi, Jeff Dean, Jacob Devlin, Adam Roberts, Denny Zhou, Quoc V. Le, and Jason Wei
1. **[FLAN-UL2](model_doc/flan-ul2)** (from Google AI) released in the repository [google-research/t5x](https://github.com/google-research/t5x/blob/main/docs/models.md#flan-ul2-checkpoints) by Hyung Won Chung, Le Hou, Shayne Longpre, Barret Zoph, Yi Tay, William Fedus, Eric Li, Xuezhi Wang, Mostafa Dehghani, Siddhartha Brahma, Albert Webson, Shixiang Shane Gu, Zhuyun Dai, Mirac Suzgun, Xinyun Chen, Aakanksha Chowdhery, Sharan Narang, Gaurav Mishra, Adams Yu, Vincent Zhao, Yanping Huang, Andrew Dai, Hongkun Yu, Slav Petrov, Ed H. Chi, Jeff Dean, Jacob Devlin, Adam Roberts, Denny Zhou, Quoc V. Le, and Jason Wei
1. **[FlauBERT](model_doc/flaubert)** (from CNRS) released with the paper [FlauBERT: Unsupervised Language Model Pre-training for French](https://arxiv.org/abs/1912.05372) by Hang Le, Loรฏc Vial, Jibril Frej, Vincent Segonne, Maximin Coavoux, Benjamin Lecouteux, Alexandre Allauzen, Benoรฎt Crabbรฉ, Laurent Besacier, Didier Schwab.
1. **[FLAVA](model_doc/flava)** (from Facebook AI) released with the paper [FLAVA: A Foundational Language And Vision Alignment Model](https://arxiv.org/abs/2112.04482) by Amanpreet Singh, Ronghang Hu, Vedanuj Goswami, Guillaume Couairon, Wojciech Galuba, Marcus Rohrbach, and Douwe Kiela.
1. **[FNet](model_doc/fnet)** (from Google Research) released with the paper [FNet: Mixing Tokens with Fourier Transforms](https://arxiv.org/abs/2105.03824) by James Lee-Thorp, Joshua Ainslie, Ilya Eckstein, Santiago Ontanon.
1. **[FocalNet](model_doc/focalnet)** (from Microsoft Research) released with the paper [Focal Modulation Networks](https://arxiv.org/abs/2203.11926) by Jianwei Yang, Chunyuan Li, Xiyang Dai, Lu Yuan, Jianfeng Gao.
1. **[Funnel Transformer](model_doc/funnel)** (from CMU/Google Brain) released with the paper [Funnel-Transformer: Filtering out Sequential Redundancy for Efficient Language Processing](https://arxiv.org/abs/2006.03236) by Zihang Dai, Guokun Lai, Yiming Yang, Quoc V. Le.
1. **[GIT](model_doc/git)** (from Microsoft Research) released with the paper [GIT: A Generative Image-to-text Transformer for Vision and Language](https://arxiv.org/abs/2205.14100) by Jianfeng Wang, Zhengyuan Yang, Xiaowei Hu, Linjie Li, Kevin Lin, Zhe Gan, Zicheng Liu, Ce Liu, Lijuan Wang.
1. **[GLPN](model_doc/glpn)** (from KAIST) released with the paper [Global-Local Path Networks for Monocular Depth Estimation with Vertical CutDepth](https://arxiv.org/abs/2201.07436) by Doyeon Kim, Woonghyun Ga, Pyungwhan Ahn, Donggyu Joo, Sehwan Chun, Junmo Kim.
1. **[GPT](model_doc/openai-gpt)** (from OpenAI) released with the paper [Improving Language Understanding by Generative Pre-Training](https://blog.openai.com/language-unsupervised/) by Alec Radford, Karthik Narasimhan, Tim Salimans and Ilya Sutskever.
1. **[GPT Neo](model_doc/gpt_neo)** (from EleutherAI) released in the repository [EleutherAI/gpt-neo](https://github.com/EleutherAI/gpt-neo) by Sid Black, Stella Biderman, Leo Gao, Phil Wang and Connor Leahy.
1. **[GPT NeoX](model_doc/gpt_neox)** (from EleutherAI) released with the paper [GPT-NeoX-20B: An Open-Source Autoregressive Language Model](https://arxiv.org/abs/2204.06745) by Sid Black, Stella Biderman, Eric Hallahan, Quentin Anthony, Leo Gao, Laurence Golding, Horace He, Connor Leahy, Kyle McDonell, Jason Phang, Michael Pieler, USVSN Sai Prashanth, Shivanshu Purohit, Laria Reynolds, Jonathan Tow, Ben Wang, Samuel Weinbach
1. **[GPT NeoX Japanese](model_doc/gpt_neox_japanese)** (from ABEJA) released by Shinya Otani, Takayoshi Makabe, Anuj Arora, and Kyo Hattori.
1. **[GPT-2](model_doc/gpt2)** (from OpenAI) released with the paper [Language Models are Unsupervised Multitask Learners](https://blog.openai.com/better-language-models/) by Alec Radford*, Jeffrey Wu*, Rewon Child, David Luan, Dario Amodei** and Ilya Sutskever**.
1. **[GPT-J](model_doc/gptj)** (from EleutherAI) released in the repository [kingoflolz/mesh-transformer-jax](https://github.com/kingoflolz/mesh-transformer-jax/) by Ben Wang and Aran Komatsuzaki.
1. **[GPT-Sw3](model_doc/gpt-sw3)** (from AI-Sweden) released with the paper [Lessons Learned from GPT-SW3: Building the First Large-Scale Generative Language Model for Swedish](http://www.lrec-conf.org/proceedings/lrec2022/pdf/2022.lrec-1.376.pdf) by Ariel Ekgren, Amaru Cuba Gyllensten, Evangelia Gogoulou, Alice Heiman, Severine Verlinden, Joey รhman, Fredrik Carlsson, Magnus Sahlgren.
1. **[GPTBigCode](model_doc/gpt_bigcode)** (from BigCode) released with the paper [SantaCoder: don't reach for the stars!](https://arxiv.org/abs/2301.03988) by Loubna Ben Allal, Raymond Li, Denis Kocetkov, Chenghao Mou, Christopher Akiki, Carlos Munoz Ferrandis, Niklas Muennighoff, Mayank Mishra, Alex Gu, Manan Dey, Logesh Kumar Umapathi, Carolyn Jane Anderson, Yangtian Zi, Joel Lamy Poirier, Hailey Schoelkopf, Sergey Troshin, Dmitry Abulkhanov, Manuel Romero, Michael Lappert, Francesco De Toni, Bernardo Garcรญa del Rรญo, Qian Liu, Shamik Bose, Urvashi Bhattacharyya, Terry Yue Zhuo, Ian Yu, Paulo Villegas, Marco Zocca, Sourab Mangrulkar, David Lansky, Huu Nguyen, Danish Contractor, Luis Villa, Jia Li, Dzmitry Bahdanau, Yacine Jernite, Sean Hughes, Daniel Fried, Arjun Guha, Harm de Vries, Leandro von Werra.
1. **[GPTSAN-japanese](model_doc/gptsan-japanese)** released in the repository [tanreinama/GPTSAN](https://github.com/tanreinama/GPTSAN/blob/main/report/model.md) by Toshiyuki Sakamoto(tanreinama).
1. **[Graphormer](model_doc/graphormer)** (from Microsoft) released with the paper [Do Transformers Really Perform Bad for Graph Representation?](https://arxiv.org/abs/2106.05234) by Chengxuan Ying, Tianle Cai, Shengjie Luo, Shuxin Zheng, Guolin Ke, Di He, Yanming Shen, Tie-Yan Liu.
1. **[GroupViT](model_doc/groupvit)** (from UCSD, NVIDIA) released with the paper [GroupViT: Semantic Segmentation Emerges from Text Supervision](https://arxiv.org/abs/2202.11094) by Jiarui Xu, Shalini De Mello, Sifei Liu, Wonmin Byeon, Thomas Breuel, Jan Kautz, Xiaolong Wang.
1. **[Hubert](model_doc/hubert)** (from Facebook) released with the paper [HuBERT: Self-Supervised Speech Representation Learning by Masked Prediction of Hidden Units](https://arxiv.org/abs/2106.07447) by Wei-Ning Hsu, Benjamin Bolte, Yao-Hung Hubert Tsai, Kushal Lakhotia, Ruslan Salakhutdinov, Abdelrahman Mohamed.
1. **[I-BERT](model_doc/ibert)** (from Berkeley) released with the paper [I-BERT: Integer-only BERT Quantization](https://arxiv.org/abs/2101.01321) by Sehoon Kim, Amir Gholami, Zhewei Yao, Michael W. Mahoney, Kurt Keutzer.
1. **[ImageGPT](model_doc/imagegpt)** (from OpenAI) released with the paper [Generative Pretraining from Pixels](https://openai.com/blog/image-gpt/) by Mark Chen, Alec Radford, Rewon Child, Jeffrey Wu, Heewoo Jun, David Luan, Ilya Sutskever.
1. **[Informer](model_doc/informer)** (from Beihang University, UC Berkeley, Rutgers University, SEDD Company) released with the paper [Informer: Beyond Efficient Transformer for Long Sequence Time-Series Forecasting](https://arxiv.org/abs/2012.07436) by Haoyi Zhou, Shanghang Zhang, Jieqi Peng, Shuai Zhang, Jianxin Li, Hui Xiong, and Wancai Zhang.
1. **[Jukebox](model_doc/jukebox)** (from OpenAI) released with the paper [Jukebox: A Generative Model for Music](https://arxiv.org/pdf/2005.00341.pdf) by Prafulla Dhariwal, Heewoo Jun, Christine Payne, Jong Wook Kim, Alec Radford, Ilya Sutskever.
1. **[LayoutLM](model_doc/layoutlm)** (from Microsoft Research Asia) released with the paper [LayoutLM: Pre-training of Text and Layout for Document Image Understanding](https://arxiv.org/abs/1912.13318) by Yiheng Xu, Minghao Li, Lei Cui, Shaohan Huang, Furu Wei, Ming Zhou.
1. **[LayoutLMv2](model_doc/layoutlmv2)** (from Microsoft Research Asia) released with the paper [LayoutLMv2: Multi-modal Pre-training for Visually-Rich Document Understanding](https://arxiv.org/abs/2012.14740) by Yang Xu, Yiheng Xu, Tengchao Lv, Lei Cui, Furu Wei, Guoxin Wang, Yijuan Lu, Dinei Florencio, Cha Zhang, Wanxiang Che, Min Zhang, Lidong Zhou.
1. **[LayoutLMv3](model_doc/layoutlmv3)** (from Microsoft Research Asia) released with the paper [LayoutLMv3: Pre-training for Document AI with Unified Text and Image Masking](https://arxiv.org/abs/2204.08387) by Yupan Huang, Tengchao Lv, Lei Cui, Yutong Lu, Furu Wei.
1. **[LayoutXLM](model_doc/layoutxlm)** (from Microsoft Research Asia) released with the paper [LayoutXLM: Multimodal Pre-training for Multilingual Visually-rich Document Understanding](https://arxiv.org/abs/2104.08836) by Yiheng Xu, Tengchao Lv, Lei Cui, Guoxin Wang, Yijuan Lu, Dinei Florencio, Cha Zhang, Furu Wei.
1. **[LED](model_doc/led)** (from AllenAI) released with the paper [Longformer: The Long-Document Transformer](https://arxiv.org/abs/2004.05150) by Iz Beltagy, Matthew E. Peters, Arman Cohan.
1. **[LeViT](model_doc/levit)** (from Meta AI) released with the paper [LeViT: A Vision Transformer in ConvNet's Clothing for Faster Inference](https://arxiv.org/abs/2104.01136) by Ben Graham, Alaaeldin El-Nouby, Hugo Touvron, Pierre Stock, Armand Joulin, Hervรฉ Jรฉgou, Matthijs Douze.
1. **[LiLT](model_doc/lilt)** (from South China University of Technology) released with the paper [LiLT: A Simple yet Effective Language-Independent Layout Transformer for Structured Document Understanding](https://arxiv.org/abs/2202.13669) by Jiapeng Wang, Lianwen Jin, Kai Ding.
1. **[LLaMA](model_doc/llama)** (from The FAIR team of Meta AI) released with the paper [LLaMA: Open and Efficient Foundation Language Models](https://arxiv.org/abs/2302.13971) by Hugo Touvron, Thibaut Lavril, Gautier Izacard, Xavier Martinet, Marie-Anne Lachaux, Timothรฉe Lacroix, Baptiste Roziรจre, Naman Goyal, Eric Hambro, Faisal Azhar, Aurelien Rodriguez, Armand Joulin, Edouard Grave, Guillaume Lample.
1. **[Longformer](model_doc/longformer)** (from AllenAI) released with the paper [Longformer: The Long-Document Transformer](https://arxiv.org/abs/2004.05150) by Iz Beltagy, Matthew E. Peters, Arman Cohan.
1. **[LongT5](model_doc/longt5)** (from Google AI) released with the paper [LongT5: Efficient Text-To-Text Transformer for Long Sequences](https://arxiv.org/abs/2112.07916) by Mandy Guo, Joshua Ainslie, David Uthus, Santiago Ontanon, Jianmo Ni, Yun-Hsuan Sung, Yinfei Yang.
1. **[LUKE](model_doc/luke)** (from Studio Ousia) released with the paper [LUKE: Deep Contextualized Entity Representations with Entity-aware Self-attention](https://arxiv.org/abs/2010.01057) by Ikuya Yamada, Akari Asai, Hiroyuki Shindo, Hideaki Takeda, Yuji Matsumoto.
1. **[LXMERT](model_doc/lxmert)** (from UNC Chapel Hill) released with the paper [LXMERT: Learning Cross-Modality Encoder Representations from Transformers for Open-Domain Question Answering](https://arxiv.org/abs/1908.07490) by Hao Tan and Mohit Bansal.
1. **[M-CTC-T](model_doc/mctct)** (from Facebook) released with the paper [Pseudo-Labeling For Massively Multilingual Speech Recognition](https://arxiv.org/abs/2111.00161) by Loren Lugosch, Tatiana Likhomanenko, Gabriel Synnaeve, and Ronan Collobert.
1. **[M2M100](model_doc/m2m_100)** (from Facebook) released with the paper [Beyond English-Centric Multilingual Machine Translation](https://arxiv.org/abs/2010.11125) by Angela Fan, Shruti Bhosale, Holger Schwenk, Zhiyi Ma, Ahmed El-Kishky, Siddharth Goyal, Mandeep Baines, Onur Celebi, Guillaume Wenzek, Vishrav Chaudhary, Naman Goyal, Tom Birch, Vitaliy Liptchinsky, Sergey Edunov, Edouard Grave, Michael Auli, Armand Joulin.
1. **[MarianMT](model_doc/marian)** Machine translation models trained using [OPUS](http://opus.nlpl.eu/) data by Jรถrg Tiedemann. The [Marian Framework](https://marian-nmt.github.io/) is being developed by the Microsoft Translator Team.
1. **[MarkupLM](model_doc/markuplm)** (from Microsoft Research Asia) released with the paper [MarkupLM: Pre-training of Text and Markup Language for Visually-rich Document Understanding](https://arxiv.org/abs/2110.08518) by Junlong Li, Yiheng Xu, Lei Cui, Furu Wei.
1. **[Mask2Former](model_doc/mask2former)** (from FAIR and UIUC) released with the paper [Masked-attention Mask Transformer for Universal Image Segmentation](https://arxiv.org/abs/2112.01527) by Bowen Cheng, Ishan Misra, Alexander G. Schwing, Alexander Kirillov, Rohit Girdhar.
1. **[MaskFormer](model_doc/maskformer)** (from Meta and UIUC) released with the paper [Per-Pixel Classification is Not All You Need for Semantic Segmentation](https://arxiv.org/abs/2107.06278) by Bowen Cheng, Alexander G. Schwing, Alexander Kirillov.
1. **[MatCha](model_doc/matcha)** (from Google AI) released with the paper [MatCha: Enhancing Visual Language Pretraining with Math Reasoning and Chart Derendering](https://arxiv.org/abs/2212.09662) by Fangyu Liu, Francesco Piccinno, Syrine Krichene, Chenxi Pang, Kenton Lee, Mandar Joshi, Yasemin Altun, Nigel Collier, Julian Martin Eisenschlos.
1. **[mBART](model_doc/mbart)** (from Facebook) released with the paper [Multilingual Denoising Pre-training for Neural Machine Translation](https://arxiv.org/abs/2001.08210) by Yinhan Liu, Jiatao Gu, Naman Goyal, Xian Li, Sergey Edunov, Marjan Ghazvininejad, Mike Lewis, Luke Zettlemoyer.
1. **[mBART-50](model_doc/mbart)** (from Facebook) released with the paper [Multilingual Translation with Extensible Multilingual Pretraining and Finetuning](https://arxiv.org/abs/2008.00401) by Yuqing Tang, Chau Tran, Xian Li, Peng-Jen Chen, Naman Goyal, Vishrav Chaudhary, Jiatao Gu, Angela Fan.
1. **[MEGA](model_doc/mega)** (from Meta/USC/CMU/SJTU) released with the paper [Mega: Moving Average Equipped Gated Attention](https://arxiv.org/abs/2209.10655) by Xuezhe Ma, Chunting Zhou, Xiang Kong, Junxian He, Liangke Gui, Graham Neubig, Jonathan May, and Luke Zettlemoyer.
1. **[Megatron-BERT](model_doc/megatron-bert)** (from NVIDIA) released with the paper [Megatron-LM: Training Multi-Billion Parameter Language Models Using Model Parallelism](https://arxiv.org/abs/1909.08053) by Mohammad Shoeybi, Mostofa Patwary, Raul Puri, Patrick LeGresley, Jared Casper and Bryan Catanzaro.
1. **[Megatron-GPT2](model_doc/megatron_gpt2)** (from NVIDIA) released with the paper [Megatron-LM: Training Multi-Billion Parameter Language Models Using Model Parallelism](https://arxiv.org/abs/1909.08053) by Mohammad Shoeybi, Mostofa Patwary, Raul Puri, Patrick LeGresley, Jared Casper and Bryan Catanzaro.
1. **[MGP-STR](model_doc/mgp-str)** (from Alibaba Research) released with the paper [Multi-Granularity Prediction for Scene Text Recognition](https://arxiv.org/abs/2209.03592) by Peng Wang, Cheng Da, and Cong Yao.
1. **[mLUKE](model_doc/mluke)** (from Studio Ousia) released with the paper [mLUKE: The Power of Entity Representations in Multilingual Pretrained Language Models](https://arxiv.org/abs/2110.08151) by Ryokan Ri, Ikuya Yamada, and Yoshimasa Tsuruoka.
1. **[MobileBERT](model_doc/mobilebert)** (from CMU/Google Brain) released with the paper [MobileBERT: a Compact Task-Agnostic BERT for Resource-Limited Devices](https://arxiv.org/abs/2004.02984) by Zhiqing Sun, Hongkun Yu, Xiaodan Song, Renjie Liu, Yiming Yang, and Denny Zhou.
1. **[MobileNetV1](model_doc/mobilenet_v1)** (from Google Inc.) released with the paper [MobileNets: Efficient Convolutional Neural Networks for Mobile Vision Applications](https://arxiv.org/abs/1704.04861) by Andrew G. Howard, Menglong Zhu, Bo Chen, Dmitry Kalenichenko, Weijun Wang, Tobias Weyand, Marco Andreetto, Hartwig Adam.
1. **[MobileNetV2](model_doc/mobilenet_v2)** (from Google Inc.) released with the paper [MobileNetV2: Inverted Residuals and Linear Bottlenecks](https://arxiv.org/abs/1801.04381) by Mark Sandler, Andrew Howard, Menglong Zhu, Andrey Zhmoginov, Liang-Chieh Chen.
1. **[MobileViT](model_doc/mobilevit)** (from Apple) released with the paper [MobileViT: Light-weight, General-purpose, and Mobile-friendly Vision Transformer](https://arxiv.org/abs/2110.02178) by Sachin Mehta and Mohammad Rastegari.
1. **[MPNet](model_doc/mpnet)** (from Microsoft Research) released with the paper [MPNet: Masked and Permuted Pre-training for Language Understanding](https://arxiv.org/abs/2004.09297) by Kaitao Song, Xu Tan, Tao Qin, Jianfeng Lu, Tie-Yan Liu.
1. **[MT5](model_doc/mt5)** (from Google AI) released with the paper [mT5: A massively multilingual pre-trained text-to-text transformer](https://arxiv.org/abs/2010.11934) by Linting Xue, Noah Constant, Adam Roberts, Mihir Kale, Rami Al-Rfou, Aditya Siddhant, Aditya Barua, Colin Raffel.
1. **[MVP](model_doc/mvp)** (from RUC AI Box) released with the paper [MVP: Multi-task Supervised Pre-training for Natural Language Generation](https://arxiv.org/abs/2206.12131) by Tianyi Tang, Junyi Li, Wayne Xin Zhao and Ji-Rong Wen.
1. **[NAT](model_doc/nat)** (from SHI Labs) released with the paper [Neighborhood Attention Transformer](https://arxiv.org/abs/2204.07143) by Ali Hassani, Steven Walton, Jiachen Li, Shen Li, and Humphrey Shi.
1. **[Nezha](model_doc/nezha)** (from Huawei Noahโs Ark Lab) released with the paper [NEZHA: Neural Contextualized Representation for Chinese Language Understanding](https://arxiv.org/abs/1909.00204) by Junqiu Wei, Xiaozhe Ren, Xiaoguang Li, Wenyong Huang, Yi Liao, Yasheng Wang, Jiashu Lin, Xin Jiang, Xiao Chen and Qun Liu.
1. **[NLLB](model_doc/nllb)** (from Meta) released with the paper [No Language Left Behind: Scaling Human-Centered Machine Translation](https://arxiv.org/abs/2207.04672) by the NLLB team.
1. **[NLLB-MOE](model_doc/nllb-moe)** (from Meta) released with the paper [No Language Left Behind: Scaling Human-Centered Machine Translation](https://arxiv.org/abs/2207.04672) by the NLLB team.
1. **[Nystrรถmformer](model_doc/nystromformer)** (from the University of Wisconsin - Madison) released with the paper [Nystrรถmformer: A Nystrรถm-Based Algorithm for Approximating Self-Attention](https://arxiv.org/abs/2102.03902) by Yunyang Xiong, Zhanpeng Zeng, Rudrasis Chakraborty, Mingxing Tan, Glenn Fung, Yin Li, Vikas Singh.
1. **[OneFormer](model_doc/oneformer)** (from SHI Labs) released with the paper [OneFormer: One Transformer to Rule Universal Image Segmentation](https://arxiv.org/abs/2211.06220) by Jitesh Jain, Jiachen Li, MangTik Chiu, Ali Hassani, Nikita Orlov, Humphrey Shi.
1. **[OpenLlama](model_doc/open-llama)** (from [s-JoL](https://huggingface.co/s-JoL)) released in [Open-Llama](https://github.com/s-JoL/Open-Llama).
1. **[OPT](master/model_doc/opt)** (from Meta AI) released with the paper [OPT: Open Pre-trained Transformer Language Models](https://arxiv.org/abs/2205.01068) by Susan Zhang, Stephen Roller, Naman Goyal, Mikel Artetxe, Moya Chen, Shuohui Chen et al.
1. **[OWL-ViT](model_doc/owlvit)** (from Google AI) released with the paper [Simple Open-Vocabulary Object Detection with Vision Transformers](https://arxiv.org/abs/2205.06230) by Matthias Minderer, Alexey Gritsenko, Austin Stone, Maxim Neumann, Dirk Weissenborn, Alexey Dosovitskiy, Aravindh Mahendran, Anurag Arnab, Mostafa Dehghani, Zhuoran Shen, Xiao Wang, Xiaohua Zhai, Thomas Kipf, and Neil Houlsby.
1. **[Pegasus](model_doc/pegasus)** (from Google) released with the paper [PEGASUS: Pre-training with Extracted Gap-sentences for Abstractive Summarization](https://arxiv.org/abs/1912.08777) by Jingqing Zhang, Yao Zhao, Mohammad Saleh and Peter J. Liu.
1. **[PEGASUS-X](model_doc/pegasus_x)** (from Google) released with the paper [Investigating Efficiently Extending Transformers for Long Input Summarization](https://arxiv.org/abs/2208.04347) by Jason Phang, Yao Zhao, and Peter J. Liu.
1. **[Perceiver IO](model_doc/perceiver)** (from Deepmind) released with the paper [Perceiver IO: A General Architecture for Structured Inputs & Outputs](https://arxiv.org/abs/2107.14795) by Andrew Jaegle, Sebastian Borgeaud, Jean-Baptiste Alayrac, Carl Doersch, Catalin Ionescu, David Ding, Skanda Koppula, Daniel Zoran, Andrew Brock, Evan Shelhamer, Olivier Hรฉnaff, Matthew M. Botvinick, Andrew Zisserman, Oriol Vinyals, Joรฃo Carreira.
1. **[PhoBERT](model_doc/phobert)** (from VinAI Research) released with the paper [PhoBERT: Pre-trained language models for Vietnamese](https://www.aclweb.org/anthology/2020.findings-emnlp.92/) by Dat Quoc Nguyen and Anh Tuan Nguyen.
1. **[Pix2Struct](model_doc/pix2struct)** (from Google) released with the paper [Pix2Struct: Screenshot Parsing as Pretraining for Visual Language Understanding](https://arxiv.org/abs/2210.03347) by Kenton Lee, Mandar Joshi, Iulia Turc, Hexiang Hu, Fangyu Liu, Julian Eisenschlos, Urvashi Khandelwal, Peter Shaw, Ming-Wei Chang, Kristina Toutanova.
1. **[PLBart](model_doc/plbart)** (from UCLA NLP) released with the paper [Unified Pre-training for Program Understanding and Generation](https://arxiv.org/abs/2103.06333) by Wasi Uddin Ahmad, Saikat Chakraborty, Baishakhi Ray, Kai-Wei Chang.
1. **[PoolFormer](model_doc/poolformer)** (from Sea AI Labs) released with the paper [MetaFormer is Actually What You Need for Vision](https://arxiv.org/abs/2111.11418) by Yu, Weihao and Luo, Mi and Zhou, Pan and Si, Chenyang and Zhou, Yichen and Wang, Xinchao and Feng, Jiashi and Yan, Shuicheng.
1. **[ProphetNet](model_doc/prophetnet)** (from Microsoft Research) released with the paper [ProphetNet: Predicting Future N-gram for Sequence-to-Sequence Pre-training](https://arxiv.org/abs/2001.04063) by Yu Yan, Weizhen Qi, Yeyun Gong, Dayiheng Liu, Nan Duan, Jiusheng Chen, Ruofei Zhang and Ming Zhou.
1. **[QDQBert](model_doc/qdqbert)** (from NVIDIA) released with the paper [Integer Quantization for Deep Learning Inference: Principles and Empirical Evaluation](https://arxiv.org/abs/2004.09602) by Hao Wu, Patrick Judd, Xiaojie Zhang, Mikhail Isaev and Paulius Micikevicius.
1. **[RAG](model_doc/rag)** (from Facebook) released with the paper [Retrieval-Augmented Generation for Knowledge-Intensive NLP Tasks](https://arxiv.org/abs/2005.11401) by Patrick Lewis, Ethan Perez, Aleksandara Piktus, Fabio Petroni, Vladimir Karpukhin, Naman Goyal, Heinrich Kรผttler, Mike Lewis, Wen-tau Yih, Tim Rocktรคschel, Sebastian Riedel, Douwe Kiela.
1. **[REALM](model_doc/realm.html)** (from Google Research) released with the paper [REALM: Retrieval-Augmented Language Model Pre-Training](https://arxiv.org/abs/2002.08909) by Kelvin Guu, Kenton Lee, Zora Tung, Panupong Pasupat and Ming-Wei Chang.
1. **[Reformer](model_doc/reformer)** (from Google Research) released with the paper [Reformer: The Efficient Transformer](https://arxiv.org/abs/2001.04451) by Nikita Kitaev, ลukasz Kaiser, Anselm Levskaya.
1. **[RegNet](model_doc/regnet)** (from META Platforms) released with the paper [Designing Network Design Space](https://arxiv.org/abs/2003.13678) by Ilija Radosavovic, Raj Prateek Kosaraju, Ross Girshick, Kaiming He, Piotr Dollรกr.
1. **[RemBERT](model_doc/rembert)** (from Google Research) released with the paper [Rethinking embedding coupling in pre-trained language models](https://arxiv.org/abs/2010.12821) by Hyung Won Chung, Thibault Fรฉvry, Henry Tsai, M. Johnson, Sebastian Ruder.
1. **[ResNet](model_doc/resnet)** (from Microsoft Research) released with the paper [Deep Residual Learning for Image Recognition](https://arxiv.org/abs/1512.03385) by Kaiming He, Xiangyu Zhang, Shaoqing Ren, Jian Sun.
1. **[RoBERTa](model_doc/roberta)** (from Facebook), released together with the paper [RoBERTa: A Robustly Optimized BERT Pretraining Approach](https://arxiv.org/abs/1907.11692) by Yinhan Liu, Myle Ott, Naman Goyal, Jingfei Du, Mandar Joshi, Danqi Chen, Omer Levy, Mike Lewis, Luke Zettlemoyer, Veselin Stoyanov.
1. **[RoBERTa-PreLayerNorm](model_doc/roberta-prelayernorm)** (from Facebook) released with the paper [fairseq: A Fast, Extensible Toolkit for Sequence Modeling](https://arxiv.org/abs/1904.01038) by Myle Ott, Sergey Edunov, Alexei Baevski, Angela Fan, Sam Gross, Nathan Ng, David Grangier, Michael Auli.
1. **[RoCBert](model_doc/roc_bert)** (from WeChatAI) released with the paper [RoCBert: Robust Chinese Bert with Multimodal Contrastive Pretraining](https://aclanthology.org/2022.acl-long.65.pdf) by HuiSu, WeiweiShi, XiaoyuShen, XiaoZhou, TuoJi, JiaruiFang, JieZhou.
1. **[RoFormer](model_doc/roformer)** (from ZhuiyiTechnology), released together with the paper [RoFormer: Enhanced Transformer with Rotary Position Embedding](https://arxiv.org/abs/2104.09864) by Jianlin Su and Yu Lu and Shengfeng Pan and Bo Wen and Yunfeng Liu.
1. **[RWKV](model_doc/rwkv)** (from Bo Peng), released on [this repo](https://github.com/BlinkDL/RWKV-LM) by Bo Peng.
1. **[SegFormer](model_doc/segformer)** (from NVIDIA) released with the paper [SegFormer: Simple and Efficient Design for Semantic Segmentation with Transformers](https://arxiv.org/abs/2105.15203) by Enze Xie, Wenhai Wang, Zhiding Yu, Anima Anandkumar, Jose M. Alvarez, Ping Luo.
1. **[Segment Anything](model_doc/sam)** (from Meta AI) released with the paper [Segment Anything](https://arxiv.org/pdf/2304.02643v1.pdf) by Alexander Kirillov, Eric Mintun, Nikhila Ravi, Hanzi Mao, Chloe Rolland, Laura Gustafson, Tete Xiao, Spencer Whitehead, Alex Berg, Wan-Yen Lo, Piotr Dollar, Ross Girshick.
1. **[SEW](model_doc/sew)** (from ASAPP) released with the paper [Performance-Efficiency Trade-offs in Unsupervised Pre-training for Speech Recognition](https://arxiv.org/abs/2109.06870) by Felix Wu, Kwangyoun Kim, Jing Pan, Kyu Han, Kilian Q. Weinberger, Yoav Artzi.
1. **[SEW-D](model_doc/sew_d)** (from ASAPP) released with the paper [Performance-Efficiency Trade-offs in Unsupervised Pre-training for Speech Recognition](https://arxiv.org/abs/2109.06870) by Felix Wu, Kwangyoun Kim, Jing Pan, Kyu Han, Kilian Q. Weinberger, Yoav Artzi.
1. **[SpeechT5](model_doc/speecht5)** (from Microsoft Research) released with the paper [SpeechT5: Unified-Modal Encoder-Decoder Pre-Training for Spoken Language Processing](https://arxiv.org/abs/2110.07205) by Junyi Ao, Rui Wang, Long Zhou, Chengyi Wang, Shuo Ren, Yu Wu, Shujie Liu, Tom Ko, Qing Li, Yu Zhang, Zhihua Wei, Yao Qian, Jinyu Li, Furu Wei.
1. **[SpeechToTextTransformer](model_doc/speech_to_text)** (from Facebook), released together with the paper [fairseq S2T: Fast Speech-to-Text Modeling with fairseq](https://arxiv.org/abs/2010.05171) by Changhan Wang, Yun Tang, Xutai Ma, Anne Wu, Dmytro Okhonko, Juan Pino.
1. **[SpeechToTextTransformer2](model_doc/speech_to_text_2)** (from Facebook), released together with the paper [Large-Scale Self- and Semi-Supervised Learning for Speech Translation](https://arxiv.org/abs/2104.06678) by Changhan Wang, Anne Wu, Juan Pino, Alexei Baevski, Michael Auli, Alexis Conneau.
1. **[Splinter](model_doc/splinter)** (from Tel Aviv University), released together with the paper [Few-Shot Question Answering by Pretraining Span Selection](https://arxiv.org/abs/2101.00438) by Ori Ram, Yuval Kirstain, Jonathan Berant, Amir Globerson, Omer Levy.
1. **[SqueezeBERT](model_doc/squeezebert)** (from Berkeley) released with the paper [SqueezeBERT: What can computer vision teach NLP about efficient neural networks?](https://arxiv.org/abs/2006.11316) by Forrest N. Iandola, Albert E. Shaw, Ravi Krishna, and Kurt W. Keutzer.
1. **[SwiftFormer](model_doc/swiftformer)** (from MBZUAI) released with the paper [SwiftFormer: Efficient Additive Attention for Transformer-based Real-time Mobile Vision Applications](https://arxiv.org/abs/2303.15446) by Abdelrahman Shaker, Muhammad Maaz, Hanoona Rasheed, Salman Khan, Ming-Hsuan Yang, Fahad Shahbaz Khan.
1. **[Swin Transformer](model_doc/swin)** (from Microsoft) released with the paper [Swin Transformer: Hierarchical Vision Transformer using Shifted Windows](https://arxiv.org/abs/2103.14030) by Ze Liu, Yutong Lin, Yue Cao, Han Hu, Yixuan Wei, Zheng Zhang, Stephen Lin, Baining Guo.
1. **[Swin Transformer V2](model_doc/swinv2)** (from Microsoft) released with the paper [Swin Transformer V2: Scaling Up Capacity and Resolution](https://arxiv.org/abs/2111.09883) by Ze Liu, Han Hu, Yutong Lin, Zhuliang Yao, Zhenda Xie, Yixuan Wei, Jia Ning, Yue Cao, Zheng Zhang, Li Dong, Furu Wei, Baining Guo.
1. **[Swin2SR](model_doc/swin2sr)** (from University of Wรผrzburg) released with the paper [Swin2SR: SwinV2 Transformer for Compressed Image Super-Resolution and Restoration](https://arxiv.org/abs/2209.11345) by Marcos V. Conde, Ui-Jin Choi, Maxime Burchi, Radu Timofte.
1. **[SwitchTransformers](model_doc/switch_transformers)** (from Google) released with the paper [Switch Transformers: Scaling to Trillion Parameter Models with Simple and Efficient Sparsity](https://arxiv.org/abs/2101.03961) by William Fedus, Barret Zoph, Noam Shazeer.
1. **[T5](model_doc/t5)** (from Google AI) released with the paper [Exploring the Limits of Transfer Learning with a Unified Text-to-Text Transformer](https://arxiv.org/abs/1910.10683) by Colin Raffel and Noam Shazeer and Adam Roberts and Katherine Lee and Sharan Narang and Michael Matena and Yanqi Zhou and Wei Li and Peter J. Liu.
1. **[T5v1.1](model_doc/t5v1.1)** (from Google AI) released in the repository [google-research/text-to-text-transfer-transformer](https://github.com/google-research/text-to-text-transfer-transformer/blob/main/released_checkpoints.md#t511) by Colin Raffel and Noam Shazeer and Adam Roberts and Katherine Lee and Sharan Narang and Michael Matena and Yanqi Zhou and Wei Li and Peter J. Liu.
1. **[Table Transformer](model_doc/table-transformer)** (from Microsoft Research) released with the paper [PubTables-1M: Towards Comprehensive Table Extraction From Unstructured Documents](https://arxiv.org/abs/2110.00061) by Brandon Smock, Rohith Pesala, Robin Abraham.
1. **[TAPAS](model_doc/tapas)** (from Google AI) released with the paper [TAPAS: Weakly Supervised Table Parsing via Pre-training](https://arxiv.org/abs/2004.02349) by Jonathan Herzig, Paweล Krzysztof Nowak, Thomas Mรผller, Francesco Piccinno and Julian Martin Eisenschlos.
1. **[TAPEX](model_doc/tapex)** (from Microsoft Research) released with the paper [TAPEX: Table Pre-training via Learning a Neural SQL Executor](https://arxiv.org/abs/2107.07653) by Qian Liu, Bei Chen, Jiaqi Guo, Morteza Ziyadi, Zeqi Lin, Weizhu Chen, Jian-Guang Lou.
1. **[Time Series Transformer](model_doc/time_series_transformer)** (from HuggingFace).
1. **[TimeSformer](model_doc/timesformer)** (from Facebook) released with the paper [Is Space-Time Attention All You Need for Video Understanding?](https://arxiv.org/abs/2102.05095) by Gedas Bertasius, Heng Wang, Lorenzo Torresani.
1. **[Trajectory Transformer](model_doc/trajectory_transformers)** (from the University of California at Berkeley) released with the paper [Offline Reinforcement Learning as One Big Sequence Modeling Problem](https://arxiv.org/abs/2106.02039) by Michael Janner, Qiyang Li, Sergey Levine
1. **[Transformer-XL](model_doc/transfo-xl)** (from Google/CMU) released with the paper [Transformer-XL: Attentive Language Models Beyond a Fixed-Length Context](https://arxiv.org/abs/1901.02860) by Zihang Dai*, Zhilin Yang*, Yiming Yang, Jaime Carbonell, Quoc V. Le, Ruslan Salakhutdinov.
1. **[TrOCR](model_doc/trocr)** (from Microsoft), released together with the paper [TrOCR: Transformer-based Optical Character Recognition with Pre-trained Models](https://arxiv.org/abs/2109.10282) by Minghao Li, Tengchao Lv, Lei Cui, Yijuan Lu, Dinei Florencio, Cha Zhang, Zhoujun Li, Furu Wei.
1. **[TVLT](model_doc/tvlt)** (from UNC Chapel Hill) released with the paper [TVLT: Textless Vision-Language Transformer](https://arxiv.org/abs/2209.14156) by Zineng Tang, Jaemin Cho, Yixin Nie, Mohit Bansal.
1. **[UL2](model_doc/ul2)** (from Google Research) released with the paper [Unifying Language Learning Paradigms](https://arxiv.org/abs/2205.05131v1) by Yi Tay, Mostafa Dehghani, Vinh Q. Tran, Xavier Garcia, Dara Bahri, Tal Schuster, Huaixiu Steven Zheng, Neil Houlsby, Donald Metzler
1. **[UniSpeech](model_doc/unispeech)** (from Microsoft Research) released with the paper [UniSpeech: Unified Speech Representation Learning with Labeled and Unlabeled Data](https://arxiv.org/abs/2101.07597) by Chengyi Wang, Yu Wu, Yao Qian, Kenichi Kumatani, Shujie Liu, Furu Wei, Michael Zeng, Xuedong Huang.
1. **[UniSpeechSat](model_doc/unispeech-sat)** (from Microsoft Research) released with the paper [UNISPEECH-SAT: UNIVERSAL SPEECH REPRESENTATION LEARNING WITH SPEAKER AWARE PRE-TRAINING](https://arxiv.org/abs/2110.05752) by Sanyuan Chen, Yu Wu, Chengyi Wang, Zhengyang Chen, Zhuo Chen, Shujie Liu, Jian Wu, Yao Qian, Furu Wei, Jinyu Li, Xiangzhan Yu.
1. **[UPerNet](model_doc/upernet)** (from Peking University) released with the paper [Unified Perceptual Parsing for Scene Understanding](https://arxiv.org/abs/1807.10221) by Tete Xiao, Yingcheng Liu, Bolei Zhou, Yuning Jiang, Jian Sun.
1. **[VAN](model_doc/van)** (from Tsinghua University and Nankai University) released with the paper [Visual Attention Network](https://arxiv.org/abs/2202.09741) by Meng-Hao Guo, Cheng-Ze Lu, Zheng-Ning Liu, Ming-Ming Cheng, Shi-Min Hu.
1. **[VideoMAE](model_doc/videomae)** (from Multimedia Computing Group, Nanjing University) released with the paper [VideoMAE: Masked Autoencoders are Data-Efficient Learners for Self-Supervised Video Pre-Training](https://arxiv.org/abs/2203.12602) by Zhan Tong, Yibing Song, Jue Wang, Limin Wang.
1. **[ViLT](model_doc/vilt)** (from NAVER AI Lab/Kakao Enterprise/Kakao Brain) released with the paper [ViLT: Vision-and-Language Transformer Without Convolution or Region Supervision](https://arxiv.org/abs/2102.03334) by Wonjae Kim, Bokyung Son, Ildoo Kim.
1. **[Vision Transformer (ViT)](model_doc/vit)** (from Google AI) released with the paper [An Image is Worth 16x16 Words: Transformers for Image Recognition at Scale](https://arxiv.org/abs/2010.11929) by Alexey Dosovitskiy, Lucas Beyer, Alexander Kolesnikov, Dirk Weissenborn, Xiaohua Zhai, Thomas Unterthiner, Mostafa Dehghani, Matthias Minderer, Georg Heigold, Sylvain Gelly, Jakob Uszkoreit, Neil Houlsby.
1. **[VisualBERT](model_doc/visual_bert)** (from UCLA NLP) released with the paper [VisualBERT: A Simple and Performant Baseline for Vision and Language](https://arxiv.org/pdf/1908.03557) by Liunian Harold Li, Mark Yatskar, Da Yin, Cho-Jui Hsieh, Kai-Wei Chang.
1. **[ViT Hybrid](model_doc/vit_hybrid)** (from Google AI) released with the paper [An Image is Worth 16x16 Words: Transformers for Image Recognition at Scale](https://arxiv.org/abs/2010.11929) by Alexey Dosovitskiy, Lucas Beyer, Alexander Kolesnikov, Dirk Weissenborn, Xiaohua Zhai, Thomas Unterthiner, Mostafa Dehghani, Matthias Minderer, Georg Heigold, Sylvain Gelly, Jakob Uszkoreit, Neil Houlsby.
1. **[ViTMAE](model_doc/vit_mae)** (from Meta AI) released with the paper [Masked Autoencoders Are Scalable Vision Learners](https://arxiv.org/abs/2111.06377) by Kaiming He, Xinlei Chen, Saining Xie, Yanghao Li, Piotr Dollรกr, Ross Girshick.
1. **[ViTMSN](model_doc/vit_msn)** (from Meta AI) released with the paper [Masked Siamese Networks for Label-Efficient Learning](https://arxiv.org/abs/2204.07141) by Mahmoud Assran, Mathilde Caron, Ishan Misra, Piotr Bojanowski, Florian Bordes, Pascal Vincent, Armand Joulin, Michael Rabbat, Nicolas Ballas.
1. **[Wav2Vec2](model_doc/wav2vec2)** (from Facebook AI) released with the paper [wav2vec 2.0: A Framework for Self-Supervised Learning of Speech Representations](https://arxiv.org/abs/2006.11477) by Alexei Baevski, Henry Zhou, Abdelrahman Mohamed, Michael Auli.
1. **[Wav2Vec2-Conformer](model_doc/wav2vec2-conformer)** (from Facebook AI) released with the paper [FAIRSEQ S2T: Fast Speech-to-Text Modeling with FAIRSEQ](https://arxiv.org/abs/2010.05171) by Changhan Wang, Yun Tang, Xutai Ma, Anne Wu, Sravya Popuri, Dmytro Okhonko, Juan Pino.
1. **[Wav2Vec2Phoneme](model_doc/wav2vec2_phoneme)** (from Facebook AI) released with the paper [Simple and Effective Zero-shot Cross-lingual Phoneme Recognition](https://arxiv.org/abs/2109.11680) by Qiantong Xu, Alexei Baevski, Michael Auli.
1. **[WavLM](model_doc/wavlm)** (from Microsoft Research) released with the paper [WavLM: Large-Scale Self-Supervised Pre-Training for Full Stack Speech Processing](https://arxiv.org/abs/2110.13900) by Sanyuan Chen, Chengyi Wang, Zhengyang Chen, Yu Wu, Shujie Liu, Zhuo Chen, Jinyu Li, Naoyuki Kanda, Takuya Yoshioka, Xiong Xiao, Jian Wu, Long Zhou, Shuo Ren, Yanmin Qian, Yao Qian, Jian Wu, Michael Zeng, Furu Wei.
1. **[Whisper](model_doc/whisper)** (from OpenAI) released with the paper [Robust Speech Recognition via Large-Scale Weak Supervision](https://cdn.openai.com/papers/whisper.pdf) by Alec Radford, Jong Wook Kim, Tao Xu, Greg Brockman, Christine McLeavey, Ilya Sutskever.
1. **[X-CLIP](model_doc/xclip)** (from Microsoft Research) released with the paper [Expanding Language-Image Pretrained Models for General Video Recognition](https://arxiv.org/abs/2208.02816) by Bolin Ni, Houwen Peng, Minghao Chen, Songyang Zhang, Gaofeng Meng, Jianlong Fu, Shiming Xiang, Haibin Ling.
1. **[X-MOD](model_doc/xmod)** (from Meta AI) released with the paper [Lifting the Curse of Multilinguality by Pre-training Modular Transformers](http://dx.doi.org/10.18653/v1/2022.naacl-main.255) by Jonas Pfeiffer, Naman Goyal, Xi Lin, Xian Li, James Cross, Sebastian Riedel, Mikel Artetxe.
1. **[XGLM](model_doc/xglm)** (From Facebook AI) released with the paper [Few-shot Learning with Multilingual Language Models](https://arxiv.org/abs/2112.10668) by Xi Victoria Lin, Todor Mihaylov, Mikel Artetxe, Tianlu Wang, Shuohui Chen, Daniel Simig, Myle Ott, Naman Goyal, Shruti Bhosale, Jingfei Du, Ramakanth Pasunuru, Sam Shleifer, Punit Singh Koura, Vishrav Chaudhary, Brian O'Horo, Jeff Wang, Luke Zettlemoyer, Zornitsa Kozareva, Mona Diab, Veselin Stoyanov, Xian Li.
1. **[XLM](model_doc/xlm)** (from Facebook) released together with the paper [Cross-lingual Language Model Pretraining](https://arxiv.org/abs/1901.07291) by Guillaume Lample and Alexis Conneau.
1. **[XLM-ProphetNet](model_doc/xlm-prophetnet)** (from Microsoft Research) released with the paper [ProphetNet: Predicting Future N-gram for Sequence-to-Sequence Pre-training](https://arxiv.org/abs/2001.04063) by Yu Yan, Weizhen Qi, Yeyun Gong, Dayiheng Liu, Nan Duan, Jiusheng Chen, Ruofei Zhang and Ming Zhou.
1. **[XLM-RoBERTa](model_doc/xlm-roberta)** (from Facebook AI), released together with the paper [Unsupervised Cross-lingual Representation Learning at Scale](https://arxiv.org/abs/1911.02116) by Alexis Conneau*, Kartikay Khandelwal*, Naman Goyal, Vishrav Chaudhary, Guillaume Wenzek, Francisco Guzmรกn, Edouard Grave, Myle Ott, Luke Zettlemoyer and Veselin Stoyanov.
1. **[XLM-RoBERTa-XL](model_doc/xlm-roberta-xl)** (from Facebook AI), released together with the paper [Larger-Scale Transformers for Multilingual Masked Language Modeling](https://arxiv.org/abs/2105.00572) by Naman Goyal, Jingfei Du, Myle Ott, Giri Anantharaman, Alexis Conneau.
1. **[XLM-V](model_doc/xlm-v)** (from Meta AI) released with the paper [XLM-V: Overcoming the Vocabulary Bottleneck in Multilingual Masked Language Models](https://arxiv.org/abs/2301.10472) by Davis Liang, Hila Gonen, Yuning Mao, Rui Hou, Naman Goyal, Marjan Ghazvininejad, Luke Zettlemoyer, Madian Khabsa.
1. **[XLNet](model_doc/xlnet)** (from Google/CMU) released with the paper [โXLNet: Generalized Autoregressive Pretraining for Language Understanding](https://arxiv.org/abs/1906.08237) by Zhilin Yang*, Zihang Dai*, Yiming Yang, Jaime Carbonell, Ruslan Salakhutdinov, Quoc V. Le.
1. **[XLS-R](model_doc/xls_r)** (from Facebook AI) released with the paper [XLS-R: Self-supervised Cross-lingual Speech Representation Learning at Scale](https://arxiv.org/abs/2111.09296) by Arun Babu, Changhan Wang, Andros Tjandra, Kushal Lakhotia, Qiantong Xu, Naman Goyal, Kritika Singh, Patrick von Platen, Yatharth Saraf, Juan Pino, Alexei Baevski, Alexis Conneau, Michael Auli.
1. **[XLSR-Wav2Vec2](model_doc/xlsr_wav2vec2)** (from Facebook AI) released with the paper [Unsupervised Cross-Lingual Representation Learning For Speech Recognition](https://arxiv.org/abs/2006.13979) by Alexis Conneau, Alexei Baevski, Ronan Collobert, Abdelrahman Mohamed, Michael Auli.
1. **[YOLOS](model_doc/yolos)** (from Huazhong University of Science & Technology) released with the paper [You Only Look at One Sequence: Rethinking Transformer in Vision through Object Detection](https://arxiv.org/abs/2106.00666) by Yuxin Fang, Bencheng Liao, Xinggang Wang, Jiemin Fang, Jiyang Qi, Rui Wu, Jianwei Niu, Wenyu Liu.
1. **[YOSO](model_doc/yoso)** (from the University of Wisconsin - Madison) released with the paper [You Only Sample (Almost) Once: Linear Cost Self-Attention Via Bernoulli Sampling](https://arxiv.org/abs/2111.09714) by Zhanpeng Zeng, Yunyang Xiong, Sathya N. Ravi, Shailesh Acharya, Glenn Fung, Vikas Singh.
### Rangka kerja yang disokong
Jadual di bawah mewakili sokongan semasa dalam perpustakaan untuk setiap model tersebut, sama ada model tersebut mempunyai Python
tokenizer (dipanggil ""lambat""). Tokenizer ""pantas"" yang disokong oleh perpustakaan Tokenizers ๐ค, sama ada mereka mempunyai sokongan dalam Jax (melalui
Flax), PyTorch, dan/atau TensorFlow.
<!--Jadual ini dikemas kini secara automatik daripada modul auto dengan _make fix-copies_. Jangan kemas kini secara manual!-->
| Model | Tokenizer slow | Tokenizer fast | PyTorch support | TensorFlow support | Flax Support |
|:-----------------------------:|:--------------:|:--------------:|:---------------:|:------------------:|:------------:|
| ALBERT | โ
| โ
| โ
| โ
| โ
|
| ALIGN | โ | โ | โ
| โ | โ |
| AltCLIP | โ | โ | โ
| โ | โ |
| Audio Spectrogram Transformer | โ | โ | โ
| โ | โ |
| Autoformer | โ | โ | โ
| โ | โ |
| BART | โ
| โ
| โ
| โ
| โ
|
| BEiT | โ | โ | โ
| โ | โ
|
| BERT | โ
| โ
| โ
| โ
| โ
|
| Bert Generation | โ
| โ | โ
| โ | โ |
| BigBird | โ
| โ
| โ
| โ | โ
|
| BigBird-Pegasus | โ | โ | โ
| โ | โ |
| BioGpt | โ
| โ | โ
| โ | โ |
| BiT | โ | โ | โ
| โ | โ |
| Blenderbot | โ
| โ
| โ
| โ
| โ
|
| BlenderbotSmall | โ
| โ
| โ
| โ
| โ
|
| BLIP | โ | โ | โ
| โ
| โ |
| BLIP-2 | โ | โ | โ
| โ | โ |
| BLOOM | โ | โ
| โ
| โ | โ |
| BridgeTower | โ | โ | โ
| โ | โ |
| CamemBERT | โ
| โ
| โ
| โ
| โ |
| CANINE | โ
| โ | โ
| โ | โ |
| Chinese-CLIP | โ | โ | โ
| โ | โ |
| CLAP | โ | โ | โ
| โ | โ |
| CLIP | โ
| โ
| โ
| โ
| โ
|
| CLIPSeg | โ | โ | โ
| โ | โ |
| CodeGen | โ
| โ
| โ
| โ | โ |
| Conditional DETR | โ | โ | โ
| โ | โ |
| ConvBERT | โ
| โ
| โ
| โ
| โ |
| ConvNeXT | โ | โ | โ
| โ
| โ |
| ConvNeXTV2 | โ | โ | โ
| โ | โ |
| CPM-Ant | โ
| โ | โ
| โ | โ |
| CTRL | โ
| โ | โ
| โ
| โ |
| CvT | โ | โ | โ
| โ
| โ |
| Data2VecAudio | โ | โ | โ
| โ | โ |
| Data2VecText | โ | โ | โ
| โ | โ |
| Data2VecVision | โ | โ | โ
| โ
| โ |
| DeBERTa | โ
| โ
| โ
| โ
| โ |
| DeBERTa-v2 | โ
| โ
| โ
| โ
| โ |
| Decision Transformer | โ | โ | โ
| โ | โ |
| Deformable DETR | โ | โ | โ
| โ | โ |
| DeiT | โ | โ | โ
| โ
| โ |
| DETA | โ | โ | โ
| โ | โ |
| DETR | โ | โ | โ
| โ | โ |
| DiNAT | โ | โ | โ
| โ | โ |
| DistilBERT | โ
| โ
| โ
| โ
| โ
|
| DonutSwin | โ | โ | โ
| โ | โ |
| DPR | โ
| โ
| โ
| โ
| โ |
| DPT | โ | โ | โ
| โ | โ |
| EfficientFormer | โ | โ | โ
| โ
| โ |
| EfficientNet | โ | โ | โ
| โ | โ |
| ELECTRA | โ
| โ
| โ
| โ
| โ
|
| Encoder decoder | โ | โ | โ
| โ
| โ
|
| ERNIE | โ | โ | โ
| โ | โ |
| ErnieM | โ
| โ | โ
| โ | โ |
| ESM | โ
| โ | โ
| โ
| โ |
| FairSeq Machine-Translation | โ
| โ | โ
| โ | โ |
| FlauBERT | โ
| โ | โ
| โ
| โ |
| FLAVA | โ | โ | โ
| โ | โ |
| FNet | โ
| โ
| โ
| โ | โ |
| FocalNet | โ | โ | โ
| โ | โ |
| Funnel Transformer | โ
| โ
| โ
| โ
| โ |
| GIT | โ | โ | โ
| โ | โ |
| GLPN | โ | โ | โ
| โ | โ |
| GPT Neo | โ | โ | โ
| โ | โ
|
| GPT NeoX | โ | โ
| โ
| โ | โ |
| GPT NeoX Japanese | โ
| โ | โ
| โ | โ |
| GPT-J | โ | โ | โ
| โ
| โ
|
| GPT-Sw3 | โ
| โ
| โ
| โ
| โ
|
| GPTBigCode | โ | โ | โ
| โ | โ |
| GPTSAN-japanese | โ
| โ | โ
| โ | โ |
| Graphormer | โ | โ | โ
| โ | โ |
| GroupViT | โ | โ | โ
| โ
| โ |
| Hubert | โ | โ | โ
| โ
| โ |
| I-BERT | โ | โ | โ
| โ | โ |
| ImageGPT | โ | โ | โ
| โ | โ |
| Informer | โ | โ | โ
| โ | โ |
| Jukebox | โ
| โ | โ
| โ | โ |
| LayoutLM | โ
| โ
| โ
| โ
| โ |
| LayoutLMv2 | โ
| โ
| โ
| โ | โ |
| LayoutLMv3 | โ
| โ
| โ
| โ
| โ |
| LED | โ
| โ
| โ
| โ
| โ |
| LeViT | โ | โ | โ
| โ | โ |
| LiLT | โ | โ | โ
| โ | โ |
| LLaMA | โ
| โ
| โ
| โ | โ |
| Longformer | โ
| โ
| โ
| โ
| โ |
| LongT5 | โ | โ | โ
| โ | โ
|
| LUKE | โ
| โ | โ
| โ | โ |
| LXMERT | โ
| โ
| โ
| โ
| โ |
| M-CTC-T | โ | โ | โ
| โ | โ |
| M2M100 | โ
| โ | โ
| โ | โ |
| Marian | โ
| โ | โ
| โ
| โ
|
| MarkupLM | โ
| โ
| โ
| โ | โ |
| Mask2Former | โ | โ | โ
| โ | โ |
| MaskFormer | โ | โ | โ
| โ | โ |
| MaskFormerSwin | โ | โ | โ | โ | โ |
| mBART | โ
| โ
| โ
| โ
| โ
|
| MEGA | โ | โ | โ
| โ | โ |
| Megatron-BERT | โ | โ | โ
| โ | โ |
| MGP-STR | โ
| โ | โ
| โ | โ |
| MobileBERT | โ
| โ
| โ
| โ
| โ |
| MobileNetV1 | โ | โ | โ
| โ | โ |
| MobileNetV2 | โ | โ | โ
| โ | โ |
| MobileViT | โ | โ | โ
| โ
| โ |
| MPNet | โ
| โ
| โ
| โ
| โ |
| MT5 | โ
| โ
| โ
| โ
| โ
|
| MVP | โ
| โ
| โ
| โ | โ |
| NAT | โ | โ | โ
| โ | โ |
| Nezha | โ | โ | โ
| โ | โ |
| NLLB-MOE | โ | โ | โ
| โ | โ |
| Nystrรถmformer | โ | โ | โ
| โ | โ |
| OneFormer | โ | โ | โ
| โ | โ |
| OpenAI GPT | โ
| โ
| โ
| โ
| โ |
| OpenAI GPT-2 | โ
| โ
| โ
| โ
| โ
|
| OpenLlama | โ | โ | โ
| โ | โ |
| OPT | โ | โ | โ
| โ
| โ
|
| OWL-ViT | โ | โ | โ
| โ | โ |
| Pegasus | โ
| โ
| โ
| โ
| โ
|
| PEGASUS-X | โ | โ | โ
| โ | โ |
| Perceiver | โ
| โ | โ
| โ | โ |
| Pix2Struct | โ | โ | โ
| โ | โ |
| PLBart | โ
| โ | โ
| โ | โ |
| PoolFormer | โ | โ | โ
| โ | โ |
| ProphetNet | โ
| โ | โ
| โ | โ |
| QDQBert | โ | โ | โ
| โ | โ |
| RAG | โ
| โ | โ
| โ
| โ |
| REALM | โ
| โ
| โ
| โ | โ |
| Reformer | โ
| โ
| โ
| โ | โ |
| RegNet | โ | โ | โ
| โ
| โ
|
| RemBERT | โ
| โ
| โ
| โ
| โ |
| ResNet | โ | โ | โ
| โ
| โ
|
| RetriBERT | โ
| โ
| โ
| โ | โ |
| RoBERTa | โ
| โ
| โ
| โ
| โ
|
| RoBERTa-PreLayerNorm | โ | โ | โ
| โ
| โ
|
| RoCBert | โ
| โ | โ
| โ | โ |
| RoFormer | โ
| โ
| โ
| โ
| โ
|
| RWKV | โ | โ | โ
| โ | โ |
| SAM | โ | โ | โ
| โ
| โ |
| SegFormer | โ | โ | โ
| โ
| โ |
| SEW | โ | โ | โ
| โ | โ |
| SEW-D | โ | โ | โ
| โ | โ |
| Speech Encoder decoder | โ | โ | โ
| โ | โ
|
| Speech2Text | โ
| โ | โ
| โ
| โ |
| Speech2Text2 | โ
| โ | โ | โ | โ |
| SpeechT5 | โ
| โ | โ
| โ | โ |
| Splinter | โ
| โ
| โ
| โ | โ |
| SqueezeBERT | โ
| โ
| โ
| โ | โ |
| SwiftFormer | โ | โ | โ
| โ | โ |
| Swin Transformer | โ | โ | โ
| โ
| โ |
| Swin Transformer V2 | โ | โ | โ
| โ | โ |
| Swin2SR | โ | โ | โ
| โ | โ |
| SwitchTransformers | โ | โ | โ
| โ | โ |
| T5 | โ
| โ
| โ
| โ
| โ
|
| Table Transformer | โ | โ | โ
| โ | โ |
| TAPAS | โ
| โ | โ
| โ
| โ |
| Time Series Transformer | โ | โ | โ
| โ | โ |
| TimeSformer | โ | โ | โ
| โ | โ |
| Trajectory Transformer | โ | โ | โ
| โ | โ |
| Transformer-XL | โ
| โ | โ
| โ
| โ |
| TrOCR | โ | โ | โ
| โ | โ |
| TVLT | โ | โ | โ
| โ | โ |
| UniSpeech | โ | โ | โ
| โ | โ |
| UniSpeechSat | โ | โ | โ
| โ | โ |
| UPerNet | โ | โ | โ
| โ | โ |
| VAN | โ | โ | โ
| โ | โ |
| VideoMAE | โ | โ | โ
| โ | โ |
| ViLT | โ | โ | โ
| โ | โ |
| Vision Encoder decoder | โ | โ | โ
| โ
| โ
|
| VisionTextDualEncoder | โ | โ | โ
| โ
| โ
|
| VisualBERT | โ | โ | โ
| โ | โ |
| ViT | โ | โ | โ
| โ
| โ
|
| ViT Hybrid | โ | โ | โ
| โ | โ |
| ViTMAE | โ | โ | โ
| โ
| โ |
| ViTMSN | โ | โ | โ
| โ | โ |
| Wav2Vec2 | โ
| โ | โ
| โ
| โ
|
| Wav2Vec2-Conformer | โ | โ | โ
| โ | โ |
| WavLM | โ | โ | โ
| โ | โ |
| Whisper | โ
| โ
| โ
| โ
| โ
|
| X-CLIP | โ | โ | โ
| โ | โ |
| X-MOD | โ | โ | โ
| โ | โ |
| XGLM | โ
| โ
| โ
| โ
| โ
|
| XLM | โ
| โ | โ
| โ
| โ |
| XLM-ProphetNet | โ
| โ | โ
| โ | โ |
| XLM-RoBERTa | โ
| โ
| โ
| โ
| โ
|
| XLM-RoBERTa-XL | โ | โ | โ
| โ | โ |
| XLNet | โ
| โ
| โ
| โ
| โ |
| YOLOS | โ | โ | โ
| โ | โ |
| YOSO | โ | โ | โ
| โ | โ |
<!-- Tamat -->
| 0 |
hf_public_repos/transformers/docs/source | hf_public_repos/transformers/docs/source/ms/_toctree.yml | - sections:
- local: index
title: ๐ค Transformers
- local: quicktour
title: Lawatan cepat
- local: installation
title: Pemasangan
title: Mulakan
- sections:
- local: pipeline_tutorial
title: Jalankan inferens dengan saluran paip
- local: autoclass_tutorial
title: Tulis kod mudah alih dengan AutoClass
- local: preprocessing
title: Praproses data
- local: training
title: Perhalusi model yang telah dilatih
- local: run_scripts
title: Latih dengan skrip
- local: accelerate
title: Sediakan latihan yang diedarkan dengan ๐ค Accelerate
- local: model_sharing
title: Kongsi model anda
- local: transformers_agents
title: Ejen
title: Tutorials
- sections:
- sections:
- local: tasks/sequence_classification
title: Klasifikasi teks
- local: tasks/token_classification
title: Klasifikasi token
- local: tasks/question_answering
title: Soalan menjawab
- local: tasks/language_modeling
title: Pemodelan bahasa sebab-akibat
- local: tasks/masked_language_modeling
title: Pemodelan bahasa Masked
- local: tasks/translation
title: Terjemahan
- local: tasks/summarization
title: Rumusan
- local: tasks/multiple_choice
title: Pilihan
title: Natural Language Processing
isExpanded: false
- sections:
- local: tasks/audio_classification
title: Klasifikasi audio
- local: tasks/asr
title: Pengecaman pertuturan automatik
title: Audio
isExpanded: false
- sections:
- local: tasks/image_classification
title: Klasifikasi imej
- local: tasks/semantic_segmentation
title: Segmentasi semantik
- local: tasks/video_classification
title: Klasifikasi video
- local: tasks/object_detection
title: Pengesanan objek
- local: tasks/zero_shot_object_detection
title: Pengesanan objek Zero-Shot
- local: tasks/zero_shot_image_classification
title: Klasifikasi imej tangkapan Zero-Shot
- local: tasks/monocular_depth_estimation
title: Anggaran kedalaman
title: Visi komputer
isExpanded: false
- sections:
- local: tasks/image_captioning
title: Kapsyen imej
- local: tasks/document_question_answering
title: Menjawab Soalan Dokumen
- local: tasks/text-to-speech
title: Teks kepada ucapan
title: Multimodal
isExpanded: false
title: Panduan Tugasan
- sections:
- local: fast_tokenizers
title: Gunakan tokenizer cepat dari ๐ค Tokenizers
- local: multilingual
title: Jalankan inferens dengan model berbilang bahasa
- local: generation_strategies
title: Sesuaikan strategi penjanaan teks
- local: create_a_model
title: Gunakan API khusus model
- local: custom_models
title: Kongsi model tersuai
- local: sagemaker
title: Jalankan latihan di Amazon SageMaker
- local: serialization
title: Eksport ke ONNX
- local: torchscript
title: Eksport ke TorchScript
- local: benchmarks
title: Penanda aras
- local: Buku nota dengan contoh
title: Notebooks with examples
- local: Sumber komuniti
title: Community resources
- local: Sumber komuniti
title: Custom Tools and Prompts
- local: Alat dan Gesaan Tersuai
title: Selesaikan masalah
title: Panduan Developer
- sections:
- local: performance
title: Gambaran keseluruhan
- local: perf_train_gpu_one
title: Latihan pada satu GPU
- local: perf_train_gpu_many
title: Latihan pada banyak GPU
- local: perf_train_cpu
title: Latihan mengenai CPU
- local: perf_train_cpu_many
title: Latihan pada banyak CPU
- local: perf_train_tpu
title: Latihan mengenai TPU
- local: perf_train_tpu_tf
title: Latihan tentang TPU dengan TensorFlow
- local: perf_train_special
title: Latihan mengenai Perkakasan Khusus
- local: perf_infer_cpu
title: Inferens pada CPU
- local: perf_infer_gpu_one
title: Inferens pada satu GPU
- local: perf_infer_gpu_many
title: Inferens pada banyak GPUs
- local: perf_infer_special
title: Inferens pada Perkakasan Khusus
- local: perf_hardware
title: Perkakasan tersuai untuk latihan
- local: big_models
title: Menghidupkan model besar
- local: debugging
title: Penyahpepijatan
- local: hpo_train
title: Carian Hiperparameter menggunakan API Pelatih
- local: tf_xla
title: Penyepaduan XLA untuk Model TensorFlow
title: Prestasi dan kebolehskalaan
- sections:
- local: contributing
title: Bagaimana untuk menyumbang kepada transformer?
- local: add_new_model
title: Bagaimana untuk menambah model pada ๐ค Transformers?
- local: add_tensorflow_model
title: Bagaimana untuk menukar model Transformers kepada TensorFlow?
- local: add_new_pipeline
title: Bagaimana untuk menambah saluran paip ke ๐ค Transformers?
- local: testing
title: Ujian
- local: pr_checks
title: Menyemak Permintaan Tarik
title: Sumbangkan
- sections:
- local: philosophy
title: Falsafah
- local: glossary
title: Glosari
- local: task_summary
title: Apa ๐ค Transformers boleh buat
- local: tasks_explained
title: Bagaimana ๐ค Transformers menyelesaikan tugasan
- local: model_summary
title: Keluarga model Transformer
- local: tokenizer_summary
title: Ringkasan tokenizer
- local: attention
title: Mekanisme perhatian
- local: pad_truncation
title: Padding dan pemotongan
- local: bertology
title: BERTology
- local: perplexity
title: Kekeliruan model panjang tetap
- local: pipeline_webserver
title: Saluran paip untuk inferens pelayan web
title: Panduan konsep
- sections:
- sections:
- local: main_classes/agent
title: Ejen dan Alat
- local: model_doc/auto
title: Kelas Auto
- local: main_classes/callback
title: Panggilan balik
- local: main_classes/configuration
title: Configuration
- local: main_classes/data_collator
title: Data Collator
- local: main_classes/keras_callbacks
title: Keras callbacks
- local: main_classes/logging
title: Logging
- local: main_classes/model
title: Models
- local: main_classes/text_generation
title: Text Generation
- local: main_classes/onnx
title: ONNX
- local: main_classes/optimizer_schedules
title: Optimization
- local: main_classes/output
title: Model outputs
- local: main_classes/pipelines
title: Pipelines
- local: main_classes/processors
title: Processors
- local: main_classes/quantization
title: Quantization
- local: main_classes/tokenizer
title: Tokenizer
- local: main_classes/trainer
title: Trainer
- local: main_classes/deepspeed
title: DeepSpeed Integration
- local: main_classes/feature_extractor
title: Feature Extractor
- local: main_classes/image_processor
title: Image Processor
title: Main Classes
- sections:
- isExpanded: false
sections:
- local: model_doc/albert
title: ALBERT
- local: model_doc/bart
title: BART
- local: model_doc/barthez
title: BARThez
- local: model_doc/bartpho
title: BARTpho
- local: model_doc/bert
title: BERT
- local: model_doc/bert-generation
title: BertGeneration
- local: model_doc/bert-japanese
title: BertJapanese
- local: model_doc/bertweet
title: Bertweet
- local: model_doc/big_bird
title: BigBird
- local: model_doc/bigbird_pegasus
title: BigBirdPegasus
- local: model_doc/biogpt
title: BioGpt
- local: model_doc/blenderbot
title: Blenderbot
- local: model_doc/blenderbot-small
title: Blenderbot Small
- local: model_doc/bloom
title: BLOOM
- local: model_doc/bort
title: BORT
- local: model_doc/byt5
title: ByT5
- local: model_doc/camembert
title: CamemBERT
- local: model_doc/canine
title: CANINE
- local: model_doc/codegen
title: CodeGen
- local: model_doc/convbert
title: ConvBERT
- local: model_doc/cpm
title: CPM
- local: model_doc/cpmant
title: CPMANT
- local: model_doc/ctrl
title: CTRL
- local: model_doc/deberta
title: DeBERTa
- local: model_doc/deberta-v2
title: DeBERTa-v2
- local: model_doc/dialogpt
title: DialoGPT
- local: model_doc/distilbert
title: DistilBERT
- local: model_doc/dpr
title: DPR
- local: model_doc/electra
title: ELECTRA
- local: model_doc/encoder-decoder
title: Encoder Decoder Models
- local: model_doc/ernie
title: ERNIE
- local: model_doc/ernie_m
title: ErnieM
- local: model_doc/esm
title: ESM
- local: model_doc/flan-t5
title: FLAN-T5
- local: model_doc/flan-ul2
title: FLAN-UL2
- local: model_doc/flaubert
title: FlauBERT
- local: model_doc/fnet
title: FNet
- local: model_doc/fsmt
title: FSMT
- local: model_doc/funnel
title: Funnel Transformer
- local: model_doc/openai-gpt
title: GPT
- local: model_doc/gpt_neo
title: GPT Neo
- local: model_doc/gpt_neox
title: GPT NeoX
- local: model_doc/gpt_neox_japanese
title: GPT NeoX Japanese
- local: model_doc/gptj
title: GPT-J
- local: model_doc/gpt2
title: GPT2
- local: model_doc/gpt_bigcode
title: GPTBigCode
- local: model_doc/gptsan-japanese
title: GPTSAN Japanese
- local: model_doc/gpt-sw3
title: GPTSw3
- local: model_doc/herbert
title: HerBERT
- local: model_doc/ibert
title: I-BERT
- local: model_doc/jukebox
title: Jukebox
- local: model_doc/led
title: LED
- local: model_doc/llama
title: LLaMA
- local: model_doc/longformer
title: Longformer
- local: model_doc/longt5
title: LongT5
- local: model_doc/luke
title: LUKE
- local: model_doc/m2m_100
title: M2M100
- local: model_doc/marian
title: MarianMT
- local: model_doc/markuplm
title: MarkupLM
- local: model_doc/mbart
title: MBart and MBart-50
- local: model_doc/mega
title: MEGA
- local: model_doc/megatron-bert
title: MegatronBERT
- local: model_doc/megatron_gpt2
title: MegatronGPT2
- local: model_doc/mluke
title: mLUKE
- local: model_doc/mobilebert
title: MobileBERT
- local: model_doc/mpnet
title: MPNet
- local: model_doc/mt5
title: MT5
- local: model_doc/mvp
title: MVP
- local: model_doc/nezha
title: NEZHA
- local: model_doc/nllb
title: NLLB
- local: model_doc/nllb-moe
title: NLLB-MoE
- local: model_doc/nystromformer
title: Nystrรถmformer
- local: model_doc/open-llama
title: Open-Llama
- local: model_doc/opt
title: OPT
- local: model_doc/pegasus
title: Pegasus
- local: model_doc/pegasus_x
title: PEGASUS-X
- local: model_doc/phobert
title: PhoBERT
- local: model_doc/plbart
title: PLBart
- local: model_doc/prophetnet
title: ProphetNet
- local: model_doc/qdqbert
title: QDQBert
- local: model_doc/rag
title: RAG
- local: model_doc/realm
title: REALM
- local: model_doc/reformer
title: Reformer
- local: model_doc/rembert
title: RemBERT
- local: model_doc/retribert
title: RetriBERT
- local: model_doc/roberta
title: RoBERTa
- local: model_doc/roberta-prelayernorm
title: RoBERTa-PreLayerNorm
- local: model_doc/roc_bert
title: RoCBert
- local: model_doc/roformer
title: RoFormer
- local: model_doc/rwkv
title: RWKV
- local: model_doc/splinter
title: Splinter
- local: model_doc/squeezebert
title: SqueezeBERT
- local: model_doc/switch_transformers
title: SwitchTransformers
- local: model_doc/t5
title: T5
- local: model_doc/t5v1.1
title: T5v1.1
- local: model_doc/tapex
title: TAPEX
- local: model_doc/transfo-xl
title: Transformer XL
- local: model_doc/ul2
title: UL2
- local: model_doc/xmod
title: X-MOD
- local: model_doc/xglm
title: XGLM
- local: model_doc/xlm
title: XLM
- local: model_doc/xlm-prophetnet
title: XLM-ProphetNet
- local: model_doc/xlm-roberta
title: XLM-RoBERTa
- local: model_doc/xlm-roberta-xl
title: XLM-RoBERTa-XL
- local: model_doc/xlm-v
title: XLM-V
- local: model_doc/xlnet
title: XLNet
- local: model_doc/yoso
title: YOSO
title: Text models
- isExpanded: false
sections:
- local: model_doc/beit
title: BEiT
- local: model_doc/bit
title: BiT
- local: model_doc/conditional_detr
title: Conditional DETR
- local: model_doc/convnext
title: ConvNeXT
- local: model_doc/convnextv2
title: ConvNeXTV2
- local: model_doc/cvt
title: CvT
- local: model_doc/deformable_detr
title: Deformable DETR
- local: model_doc/deit
title: DeiT
- local: model_doc/deta
title: DETA
- local: model_doc/detr
title: DETR
- local: model_doc/dinat
title: DiNAT
- local: model_doc/dit
title: DiT
- local: model_doc/dpt
title: DPT
- local: model_doc/efficientformer
title: EfficientFormer
- local: model_doc/efficientnet
title: EfficientNet
- local: model_doc/focalnet
title: FocalNet
- local: model_doc/glpn
title: GLPN
- local: model_doc/imagegpt
title: ImageGPT
- local: model_doc/levit
title: LeViT
- local: model_doc/mask2former
title: Mask2Former
- local: model_doc/maskformer
title: MaskFormer
- local: model_doc/mobilenet_v1
title: MobileNetV1
- local: model_doc/mobilenet_v2
title: MobileNetV2
- local: model_doc/mobilevit
title: MobileViT
- local: model_doc/nat
title: NAT
- local: model_doc/poolformer
title: PoolFormer
- local: model_doc/regnet
title: RegNet
- local: model_doc/resnet
title: ResNet
- local: model_doc/segformer
title: SegFormer
- local: model_doc/swiftformer
title: SwiftFormer
- local: model_doc/swin
title: Swin Transformer
- local: model_doc/swinv2
title: Swin Transformer V2
- local: model_doc/swin2sr
title: Swin2SR
- local: model_doc/table-transformer
title: Table Transformer
- local: model_doc/timesformer
title: TimeSformer
- local: model_doc/upernet
title: UperNet
- local: model_doc/van
title: VAN
- local: model_doc/videomae
title: VideoMAE
- local: model_doc/vit
title: Vision Transformer (ViT)
- local: model_doc/vit_hybrid
title: ViT Hybrid
- local: model_doc/vit_mae
title: ViTMAE
- local: model_doc/vit_msn
title: ViTMSN
- local: model_doc/yolos
title: YOLOS
title: Vision models
- isExpanded: false
sections:
- local: model_doc/audio-spectrogram-transformer
title: Audio Spectrogram Transformer
- local: model_doc/clap
title: CLAP
- local: model_doc/hubert
title: Hubert
- local: model_doc/mctct
title: MCTCT
- local: model_doc/sew
title: SEW
- local: model_doc/sew-d
title: SEW-D
- local: model_doc/speech_to_text
title: Speech2Text
- local: model_doc/speech_to_text_2
title: Speech2Text2
- local: model_doc/speecht5
title: SpeechT5
- local: model_doc/unispeech
title: UniSpeech
- local: model_doc/unispeech-sat
title: UniSpeech-SAT
- local: model_doc/wav2vec2
title: Wav2Vec2
- local: model_doc/wav2vec2-conformer
title: Wav2Vec2-Conformer
- local: model_doc/wav2vec2_phoneme
title: Wav2Vec2Phoneme
- local: model_doc/wavlm
title: WavLM
- local: model_doc/whisper
title: Whisper
- local: model_doc/xls_r
title: XLS-R
- local: model_doc/xlsr_wav2vec2
title: XLSR-Wav2Vec2
title: Audio models
- isExpanded: false
sections:
- local: model_doc/align
title: ALIGN
- local: model_doc/altclip
title: AltCLIP
- local: model_doc/blip
title: BLIP
- local: model_doc/blip-2
title: BLIP-2
- local: model_doc/bridgetower
title: BridgeTower
- local: model_doc/chinese_clip
title: Chinese-CLIP
- local: model_doc/clip
title: CLIP
- local: model_doc/clipseg
title: CLIPSeg
- local: model_doc/data2vec
title: Data2Vec
- local: model_doc/deplot
title: DePlot
- local: model_doc/donut
title: Donut
- local: model_doc/flava
title: FLAVA
- local: model_doc/git
title: GIT
- local: model_doc/groupvit
title: GroupViT
- local: model_doc/layoutlm
title: LayoutLM
- local: model_doc/layoutlmv2
title: LayoutLMV2
- local: model_doc/layoutlmv3
title: LayoutLMV3
- local: model_doc/layoutxlm
title: LayoutXLM
- local: model_doc/lilt
title: LiLT
- local: model_doc/lxmert
title: LXMERT
- local: model_doc/matcha
title: MatCha
- local: model_doc/mgp-str
title: MGP-STR
- local: model_doc/oneformer
title: OneFormer
- local: model_doc/owlvit
title: OWL-ViT
- local: model_doc/perceiver
title: Perceiver
- local: model_doc/pix2struct
title: Pix2Struct
- local: model_doc/sam
title: Segment Anything
- local: model_doc/speech-encoder-decoder
title: Speech Encoder Decoder Models
- local: model_doc/tapas
title: TAPAS
- local: model_doc/trocr
title: TrOCR
- local: model_doc/tvlt
title: TVLT
- local: model_doc/vilt
title: ViLT
- local: model_doc/vision-encoder-decoder
title: Vision Encoder Decoder Models
- local: model_doc/vision-text-dual-encoder
title: Vision Text Dual Encoder
- local: model_doc/visual_bert
title: VisualBERT
- local: model_doc/xclip
title: X-CLIP
title: Multimodal models
- isExpanded: false
sections:
- local: model_doc/decision_transformer
title: Decision Transformer
- local: model_doc/trajectory_transformer
title: Trajectory Transformer
title: Reinforcement learning models
- isExpanded: false
sections:
- local: model_doc/informer
title: Informer
- local: model_doc/time_series_transformer
title: Time Series Transformer
title: Time series models
- isExpanded: false
sections:
- local: model_doc/graphormer
title: Graphormer
title: Graph models
title: Models
- sections:
- local: internal/modeling_utils
title: Custom Layers and Utilities
- local: internal/pipelines_utils
title: Utilities for pipelines
- local: internal/tokenization_utils
title: Utilities for Tokenizers
- local: internal/trainer_utils
title: Utilities for Trainer
- local: internal/generation_utils
title: Utilities for Generation
- local: internal/image_processing_utils
title: Utilities for Image Processors
- local: internal/audio_utils
title: Utilities for Audio processing
- local: internal/file_utils
title: General Utilities
- local: internal/time_series_utils
title: Utilities for Time Series
title: Internal Helpers
title: API
| 0 |
hf_public_repos/transformers/docs/source | hf_public_repos/transformers/docs/source/fr/quicktour.md | <!--Copyright 2022 The HuggingFace Team. All rights reserved.
Licensed under the Apache License, Version 2.0 (the "License"); you may not use this file except in compliance with
the License. You may obtain a copy of the License at
http://www.apache.org/licenses/LICENSE-2.0
Unless required by applicable law or agreed to in writing, software distributed under the License is distributed on
an "AS IS" BASIS, WITHOUT WARRANTIES OR CONDITIONS OF ANY KIND, either express or implied. See the License for the
specific language governing permissions and limitations under the License.
โ ๏ธ Note that this file is in Markdown but contain specific syntax for our doc-builder (similar to MDX) that may not be
rendered properly in your Markdown viewer.
-->
# Visite rapide
[[open-in-colab]]
Soyez opรฉrationnel avec ๐ค Transformers ! Que vous soyez un dรฉveloppeur ou un utilisateur lambda, cette visite rapide vous aidera ร dรฉmarrer et vous montrera comment utiliser le [`pipeline`] pour l'infรฉrence, charger un modรจle prรฉ-entraรฎnรฉ et un prรฉprocesseur avec une [AutoClass](./model_doc/auto), et entraรฎner rapidement un modรจle avec PyTorch ou TensorFlow. Si vous รชtes un dรฉbutant, nous vous recommandons de consulter nos tutoriels ou notre [cours](https://huggingface.co/course/chapter1/1) suivant pour des explications plus approfondies des concepts prรฉsentรฉs ici.
Avant de commencer, assurez-vous que vous avez installรฉ toutes les bibliothรจques nรฉcessaires :
```bash
!pip install transformers datasets
```
Vous aurez aussi besoin d'installer votre bibliothรจque d'apprentissage profond favorite :
<frameworkcontent>
<pt>
```bash
pip install torch
```
</pt>
<tf>
```bash
pip install tensorflow
```
</tf>
</frameworkcontent>
## Pipeline
<Youtube id="tiZFewofSLM"/>
Le [`pipeline`] est le moyen le plus simple d'utiliser un modรจle prรฉ-entraรฎnรฉ pour l'infรฉrence. Vous pouvez utiliser le [`pipeline`] prรชt ร l'emploi pour de nombreuses tรขches dans diffรฉrentes modalitรฉs. Consultez le tableau ci-dessous pour connaรฎtre les tรขches prises en charge :
| **Tรขche** | **Description** | **Modalitรฉ** | **Identifiant du pipeline** |
|------------------------------|--------------------------------------------------------------------------------------------------------------|----------------------|-----------------------------------------------|
| Classification de texte | Attribue une catรฉgorie ร une sรฉquence de texte donnรฉe | Texte | pipeline(task="sentiment-analysis") |
| Gรฉnรฉration de texte | Gรฉnรจre du texte ร partir d'une consigne donnรฉe | Texte | pipeline(task="text-generation") |
| Reconnaissance de token nommรฉ | Attribue une catรฉgorie ร chaque token dans une sรฉquence (personnes, organisation, localisation, etc.) | Texte | pipeline(task="ner") |
| Question rรฉponse | Extrait une rรฉponse du texte en fonction du contexte et d'une question | Texte | pipeline(task="question-answering") |
| Prรฉdiction de token masquรฉ | Prรฉdit correctement le token masquรฉ dans une sรฉquence | Texte | pipeline(task="fill-mask") |
| Gรฉnรฉration de rรฉsumรฉ | Gรฉnรจre un rรฉsumรฉ d'une sรฉquence de texte donnรฉe ou d'un document | Texte | pipeline(task="summarization") |
| Traduction | Traduit du texte d'un langage ร un autre | Texte | pipeline(task="translation") |
| Classification d'image | Attribue une catรฉgorie ร une image | Image | pipeline(task="image-classification") |
| Segmentation d'image | Attribue une catรฉgorie ร chaque pixel d'une image (supporte la segmentation sรฉmantique, panoptique et d'instance) | Image | pipeline(task="image-segmentation") |
| Dรฉtection d'objects | Prรฉdit les dรฉlimitations et catรฉgories d'objects dans une image | Image | pipeline(task="object-detection") |
| Classification d'audio | Attribue une catรฉgorie ร un fichier audio | Audio | pipeline(task="audio-classification") |
| Reconnaissance automatique de la parole | Extrait le discours d'un fichier audio en texte | Audio | pipeline(task="automatic-speech-recognition") |
| Question rรฉponse visuels | Etant donnรฉes une image et une question, rรฉpond correctement ร une question sur l'image | Modalitรฉs multiples | pipeline(task="vqa") |
Commencez par crรฉer une instance de [`pipeline`] et spรฉcifiez la tรขche pour laquelle vous souhaitez l'utiliser. Vous pouvez utiliser le [`pipeline`] pour n'importe laquelle des tรขches mentionnรฉes dans le tableau prรฉcรฉdent. Pour obtenir une liste complรจte des tรขches prises en charge, consultez la documentation de l'[API pipeline](./main_classes/pipelines). Dans ce guide, nous utiliserons le [`pipeline`] pour l'analyse des sentiments ร titre d'exemple :
```py
>>> from transformers import pipeline
>>> classifier = pipeline("sentiment-analysis")
```
Le [`pipeline`] tรฉlรฉcharge et stocke en cache un [modรจle prรฉ-entraรฎnรฉ](https://huggingface.co/distilbert-base-uncased-finetuned-sst-2-english) et un tokenizer par dรฉfaut pour l'analyse des sentiments. Vous pouvez maintenant utiliser le `classifier` sur le texte de votre choix :
```py
>>> classifier("We are very happy to show you the ๐ค Transformers library.")
[{'label': 'POSITIVE', 'score': 0.9998}]
```
Si vous voulez classifier plus qu'un texte, donnez une liste de textes au [`pipeline`] pour obtenir une liste de dictionnaires en retour :
```py
>>> results = classifier(["We are very happy to show you the ๐ค Transformers library.", "We hope you don't hate it."])
>>> for result in results:
... print(f"label: {result['label']}, avec le score de: {round(result['score'], 4)}")
label: POSITIVE, avec le score de: 0.9998
label: NEGATIVE, avec le score de: 0.5309
```
Le [`pipeline`] peut aussi itรฉrer sur un jeu de donnรฉes entier pour n'importe quelle tรขche. Prenons par exemple la reconnaissance automatique de la parole :
```py
>>> import torch
>>> from transformers import pipeline
>>> speech_recognizer = pipeline("automatic-speech-recognition", model="facebook/wav2vec2-base-960h")
```
Chargez un jeu de donnรฉes audio (voir le ๐ค Datasets [Quick Start](https://huggingface.co/docs/datasets/quickstart#audio) pour plus de dรฉtails) sur lequel vous souhaitez itรฉrer. Pour cet example, nous chargons le jeu de donnรฉes [MInDS-14](https://huggingface.co/datasets/PolyAI/minds14) :
```py
>>> from datasets import load_dataset, Audio
>>> dataset = load_dataset("PolyAI/minds14", name="en-US", split="train") # doctest: +IGNORE_RESULT
```
Vous devez vous assurer que le taux d'รฉchantillonnage de l'ensemble de donnรฉes correspond au taux d'รฉchantillonnage sur lequel [`facebook/wav2vec2-base-960h`](https://huggingface.co/facebook/wav2vec2-base-960h) a รฉtรฉ entraรฎnรฉ :
```py
>>> dataset = dataset.cast_column("audio", Audio(sampling_rate=speech_recognizer.feature_extractor.sampling_rate))
```
Les fichiers audio sont automatiquement chargรฉs et rรฉรฉchantillonnรฉs lors de l'appel de la colonne `"audio"`.
Extrayez les tableaux de formes d'ondes brutes des quatre premiers รฉchantillons et passez-les comme une liste au pipeline :
```py
>>> result = speech_recognizer(dataset[:4]["audio"])
>>> print([d["text"] for d in result])
['I WOULD LIKE TO SET UP A JOINT ACCOUNT WITH MY PARTNER HOW DO I PROCEED WITH DOING THAT', "FODING HOW I'D SET UP A JOIN TO HET WITH MY WIFE AND WHERE THE AP MIGHT BE", "I I'D LIKE TOY SET UP A JOINT ACCOUNT WITH MY PARTNER I'M NOT SEEING THE OPTION TO DO IT ON THE AP SO I CALLED IN TO GET SOME HELP CAN I JUST DO IT OVER THE PHONE WITH YOU AND GIVE YOU THE INFORMATION OR SHOULD I DO IT IN THE AP AND I'M MISSING SOMETHING UQUETTE HAD PREFERRED TO JUST DO IT OVER THE PHONE OF POSSIBLE THINGS", 'HOW DO I THURN A JOIN A COUNT']
```
Pour les ensembles de donnรฉes plus importants oรน les entrรฉes sont volumineuses (comme dans les domaines de la parole ou de la vision), utilisez plutรดt un gรฉnรฉrateur au lieu d'une liste pour charger toutes les entrรฉes en mรฉmoire. Pour plus d'informations, consultez la documentation de l'[API pipeline](./main_classes/pipelines).
### Utiliser une autre modรจle et tokenizer dans le pipeline
Le [`pipeline`] peut รชtre utilisรฉ avec n'importe quel modรจle du [Hub](https://huggingface.co/models), ce qui permet d'adapter facilement le [`pipeline`] ร d'autres cas d'utilisation. Par exemple, si vous souhaitez un modรจle capable de traiter du texte franรงais, utilisez les filtres du Hub pour trouver un modรจle appropriรฉ. Le premier rรฉsultat renvoie un [modรจle BERT](https://huggingface.co/nlptown/bert-base-multilingual-uncased-sentiment) multilingue finetunรฉ pour l'analyse des sentiments que vous pouvez utiliser pour le texte franรงais :
```py
>>> model_name = "nlptown/bert-base-multilingual-uncased-sentiment"
```
<frameworkcontent>
<pt>
Utilisez [`AutoModelForSequenceClassification`] et [`AutoTokenizer`] pour charger le modรจle prรฉ-entraรฎnรฉ et le tokenizer adaptรฉ (plus de dรฉtails sur une `AutoClass` dans la section suivante) :
```py
>>> from transformers import AutoTokenizer, AutoModelForSequenceClassification
>>> model = AutoModelForSequenceClassification.from_pretrained(model_name)
>>> tokenizer = AutoTokenizer.from_pretrained(model_name)
```
</pt>
<tf>
Utilisez [`TFAutoModelForSequenceClassification`] et [`AutoTokenizer`] pour charger le modรจle prรฉ-entraรฎnรฉ et le tokenizer adaptรฉ (plus de dรฉtails sur une `TFAutoClass` dans la section suivante) :
```py
>>> from transformers import AutoTokenizer, TFAutoModelForSequenceClassification
>>> model = TFAutoModelForSequenceClassification.from_pretrained(model_name)
>>> tokenizer = AutoTokenizer.from_pretrained(model_name)
```
</tf>
</frameworkcontent>
Specifiez le modรจle et le tokenizer dans le [`pipeline`], et utilisez le `classifier` sur le texte en franรงais :
```py
>>> classifier = pipeline("sentiment-analysis", model=model, tokenizer=tokenizer)
>>> classifier("Nous sommes trรจs heureux de vous prรฉsenter la bibliothรจque ๐ค Transformers.")
[{'label': '5 stars', 'score': 0.7273}]
```
Si vous ne parvenez pas ร trouver un modรจle adaptรฉ ร votre cas d'utilisation, vous devrez finetuner un modรจle prรฉ-entraรฎnรฉ sur vos donnรฉes. Jetez un coup d'ลil ร notre [tutoriel sur le finetuning](./training) pour apprendre comment faire. Enfin, aprรจs avoir finetunรฉ votre modรจle prรฉ-entraรฎnรฉ, pensez ร [partager](./model_sharing) le modรจle avec la communautรฉ sur le Hub afin de dรฉmocratiser l'apprentissage automatique pour tous ! ๐ค
## AutoClass
<Youtube id="AhChOFRegn4"/>
Les classes [`AutoModelForSequenceClassification`] et [`AutoTokenizer`] fonctionnent ensemble pour crรฉer un [`pipeline`] comme celui que vous avez utilisรฉ ci-dessus. Une [AutoClass](./model_doc/auto) est un raccourci qui rรฉcupรจre automatiquement l'architecture d'un modรจle prรฉ-entraรฎnรฉ ร partir de son nom ou de son emplacement. Il vous suffit de sรฉlectionner l'`AutoClass` appropriรฉe ร votre tรขche et la classe de prรฉtraitement qui lui est associรฉe.
Reprenons l'exemple de la section prรฉcรฉdente et voyons comment vous pouvez utiliser l'`AutoClass` pour reproduire les rรฉsultats du [`pipeline`].
### AutoTokenizer
Un tokenizer est chargรฉ de prรฉtraiter le texte pour en faire un tableau de chiffres qui servira d'entrรฉe ร un modรจle. De nombreuses rรจgles rรฉgissent le processus de tokenisation, notamment la maniรจre de diviser un mot et le niveau auquel les mots doivent รชtre divisรฉs (pour en savoir plus sur la tokenisation, consultez le [rรฉsumรฉ](./tokenizer_summary)). La chose la plus importante ร retenir est que vous devez instancier un tokenizer avec le mรชme nom de modรจle pour vous assurer que vous utilisez les mรชmes rรจgles de tokenisation que celles avec lesquelles un modรจle a รฉtรฉ prรฉ-entraรฎnรฉ.
Chargez un tokenizer avec [`AutoTokenizer`] :
```py
>>> from transformers import AutoTokenizer
>>> model_name = "nlptown/bert-base-multilingual-uncased-sentiment"
>>> tokenizer = AutoTokenizer.from_pretrained(model_name)
```
Passez votre texte au tokenizer :
```py
>>> encoding = tokenizer("We are very happy to show you the ๐ค Transformers library.")
>>> print(encoding)
{'input_ids': [101, 11312, 10320, 12495, 19308, 10114, 11391, 10855, 10103, 100, 58263, 13299, 119, 102],
'token_type_ids': [0, 0, 0, 0, 0, 0, 0, 0, 0, 0, 0, 0, 0, 0],
'attention_mask': [1, 1, 1, 1, 1, 1, 1, 1, 1, 1, 1, 1, 1, 1]}
```
Le tokenizer retourne un dictionnaire contenant :
* [input_ids](./glossary#input-ids): la reprรฉsentation numรฉrique des tokens.
* [attention_mask](.glossary#attention-mask): indique quels tokens doivent faire l'objet d'une attention particuliรจre (plus particuliรจrement les tokens de remplissage).
Un tokenizer peut รฉgalement accepter une liste de textes, et remplir et tronquer le texte pour retourner un รฉchantillon de longueur uniforme :
<frameworkcontent>
<pt>
```py
>>> pt_batch = tokenizer(
... ["We are very happy to show you the ๐ค Transformers library.", "We hope you don't hate it."],
... padding=True,
... truncation=True,
... max_length=512,
... return_tensors="pt",
... )
```
</pt>
<tf>
```py
>>> tf_batch = tokenizer(
... ["We are very happy to show you the ๐ค Transformers library.", "We hope you don't hate it."],
... padding=True,
... truncation=True,
... max_length=512,
... return_tensors="tf",
... )
```
</tf>
</frameworkcontent>
<Tip>
Consultez le tutoriel [prรฉtraitement](./preprocessing) pour plus de dรฉtails sur la tokenisation, et sur la maniรจre d'utiliser un [`AutoImageProcessor`], un [`AutoFeatureExtractor`] et un [`AutoProcessor`] pour prรฉtraiter les images, l'audio et les contenus multimodaux.
</Tip>
### AutoModel
<frameworkcontent>
<pt>
๐ค Transformers fournit un moyen simple et unifiรฉ de charger des instances prรฉ-entraรฎnรฉes. Cela signifie que vous pouvez charger un [`AutoModel`] comme vous chargeriez un [`AutoTokenizer`]. La seule diffรฉrence est de sรฉlectionner l'[`AutoModel`] appropriรฉ pour la tรขche. Pour une classification de texte (ou de sรฉquence de textes), vous devez charger [`AutoModelForSequenceClassification`] :
```py
>>> from transformers import AutoModelForSequenceClassification
>>> model_name = "nlptown/bert-base-multilingual-uncased-sentiment"
>>> pt_model = AutoModelForSequenceClassification.from_pretrained(model_name)
```
<Tip>
Voir le [rรฉsumรฉ de la tรขche](./task_summary) pour vรฉrifier si elle est prise en charge par une classe [`AutoModel`].
</Tip>
Maintenant, passez votre รฉchantillon d'entrรฉes prรฉtraitรฉes directement au modรจle. Il vous suffit de dรฉcompresser le dictionnaire en ajoutant `**` :
```py
>>> pt_outputs = pt_model(**pt_batch)
```
Le modรจle produit les activations finales dans l'attribut `logits`. Appliquez la fonction softmax aux `logits` pour rรฉcupรฉrer les probabilitรฉs :
```py
>>> from torch import nn
>>> pt_predictions = nn.functional.softmax(pt_outputs.logits, dim=-1)
>>> print(pt_predictions)
tensor([[0.0021, 0.0018, 0.0115, 0.2121, 0.7725],
[0.2084, 0.1826, 0.1969, 0.1755, 0.2365]], grad_fn=<SoftmaxBackward0>)
```
</pt>
<tf>
๐ค Transformers fournit un moyen simple et unifiรฉ de charger des instances prรฉ-entraรฎnรฉs. Cela signifie que vous pouvez charger un [`TFAutoModel`] comme vous chargeriez un [`AutoTokenizer`]. La seule diffรฉrence est de sรฉlectionner le [`TFAutoModel`] appropriรฉ pour la tรขche. Pour une classification de texte (ou de sรฉquence de textes), vous devez charger [`TFAutoModelForSequenceClassification`] :
```py
>>> from transformers import TFAutoModelForSequenceClassification
>>> model_name = "nlptown/bert-base-multilingual-uncased-sentiment"
>>> tf_model = TFAutoModelForSequenceClassification.from_pretrained(model_name)
```
<Tip>
Voir le [rรฉsumรฉ de la tรขche](./task_summary) pour vรฉrifier si elle est prise en charge par une classe [`AutoModel`].
</Tip>
Passez maintenant votre รฉchantillon d'entrรฉes prรฉtraitรฉes directement au modรจle en passant les clรฉs du dictionnaire directement aux tensors :
```py
>>> tf_outputs = tf_model(tf_batch)
```
Le modรจle produit les activations finales dans l'attribut `logits`. Appliquez la fonction softmax aux `logits` pour rรฉcupรฉrer les probabilitรฉs :
```py
>>> import tensorflow as tf
>>> tf_predictions = tf.nn.softmax(tf_outputs.logits, axis=-1)
>>> tf_predictions # doctest: +IGNORE_RESULT
```
</tf>
</frameworkcontent>
<Tip>
Tous les modรจles ๐ค Transformers (PyTorch ou TensorFlow) produisent les tensors *avant* la fonction d'activation finale (comme softmax) car la fonction d'activation finale est souvent fusionnรฉe avec le calcul de la perte. Les structures produites par le modรจle sont des classes de donnรฉes spรฉciales, de sorte que leurs attributs sont autocomplรฉtรฉs dans un environnement de dรฉveloppement. Les structures produites par le modรจle se comportent comme un tuple ou un dictionnaire (vous pouvez les indexer avec un entier, une tranche ou une chaรฎne), auquel cas les attributs qui sont None sont ignorรฉs.
</Tip>
### Sauvegarder un modรจle
<frameworkcontent>
<pt>
Une fois que votre modรจle est finetunรฉ, vous pouvez le sauvegarder avec son tokenizer en utilisant [`PreTrainedModel.save_pretrained`] :
```py
>>> pt_save_directory = "./pt_save_pretrained"
>>> tokenizer.save_pretrained(pt_save_directory) # doctest: +IGNORE_RESULT
>>> pt_model.save_pretrained(pt_save_directory)
```
Lorsque vous voulez rรฉutiliser le modรจle, rechargez-le avec [`PreTrainedModel.from_pretrained`] :
```py
>>> pt_model = AutoModelForSequenceClassification.from_pretrained("./pt_save_pretrained")
```
</pt>
<tf>
Une fois que votre modรจle est finetunรฉ, vous pouvez le sauvegarder avec son tokenizer en utilisant [`TFPreTrainedModel.save_pretrained`] :
```py
>>> tf_save_directory = "./tf_save_pretrained"
>>> tokenizer.save_pretrained(tf_save_directory) # doctest: +IGNORE_RESULT
>>> tf_model.save_pretrained(tf_save_directory)
```
Lorsque vous voulez rรฉutiliser le modรจle, rechargez-le avec [`TFPreTrainedModel.from_pretrained`] :
```py
>>> tf_model = TFAutoModelForSequenceClassification.from_pretrained("./tf_save_pretrained")
```
</tf>
</frameworkcontent>
Une fonctionnalitรฉ particuliรจrement cool ๐ค Transformers est la possibilitรฉ d'enregistrer un modรจle et de le recharger en tant que modรจle PyTorch ou TensorFlow. Le paramรจtre `from_pt` ou `from_tf` permet de convertir le modรจle d'un framework ร l'autre :
<frameworkcontent>
<pt>
```py
>>> from transformers import AutoModel
>>> tokenizer = AutoTokenizer.from_pretrained(tf_save_directory)
>>> pt_model = AutoModelForSequenceClassification.from_pretrained(tf_save_directory, from_tf=True)
```
</pt>
<tf>
```py
>>> from transformers import TFAutoModel
>>> tokenizer = AutoTokenizer.from_pretrained(pt_save_directory)
>>> tf_model = TFAutoModelForSequenceClassification.from_pretrained(pt_save_directory, from_pt=True)
```
</tf>
</frameworkcontent>
## Constructions de modรจles personnalisรฉs
Vous pouvez modifier la configuration du modรจle pour changer la faรงon dont un modรจle est construit. La configuration spรฉcifie les attributs d'un modรจle, tels que le nombre de couches ou de tรชtes d'attention. Vous partez de zรฉro lorsque vous initialisez un modรจle ร partir d'une configuration personnalisรฉe. Les attributs du modรจle sont initialisรฉs de maniรจre alรฉatoire et vous devrez entraรฎner le modรจle avant de pouvoir l'utiliser pour obtenir des rรฉsultats significatifs.
Commencez par importer [`AutoConfig`], puis chargez le modรจle prรฉ-entraรฎnรฉ que vous voulez modifier. Dans [`AutoConfig.from_pretrained`], vous pouvez spรฉcifier l'attribut que vous souhaitez modifier, tel que le nombre de tรชtes d'attention :
```py
>>> from transformers import AutoConfig
>>> my_config = AutoConfig.from_pretrained("distilbert-base-uncased", n_heads=12)
```
<frameworkcontent>
<pt>
Crรฉez un modรจle personnalisรฉ ร partir de votre configuration avec [`AutoModel.from_config`] :
```py
>>> from transformers import AutoModel
>>> my_model = AutoModel.from_config(my_config)
```
</pt>
<tf>
Crรฉez un modรจle personnalisรฉ ร partir de votre configuration avec [`TFAutoModel.from_config`] :
```py
>>> from transformers import TFAutoModel
>>> my_model = TFAutoModel.from_config(my_config)
```
</tf>
</frameworkcontent>
Consultez le guide [Crรฉer une architecture personnalisรฉe](./create_a_model) pour plus d'informations sur la crรฉation de configurations personnalisรฉes.
## Trainer - une boucle d'entraรฎnement optimisรฉe par PyTorch
Tous les modรจles sont des [`torch.nn.Module`](https://pytorch.org/docs/stable/nn.html#torch.nn.Module) standard, vous pouvez donc les utiliser dans n'importe quelle boucle d'entraรฎnement typique. Bien que vous puissiez รฉcrire votre propre boucle d'entraรฎnement, ๐ค Transformers fournit une classe [`Trainer`] pour PyTorch, qui contient la boucle d'entraรฎnement de base et ajoute des fonctionnalitรฉs supplรฉmentaires comme l'entraรฎnement distribuรฉ, la prรฉcision mixte, et plus encore.
En fonction de votre tรขche, vous passerez gรฉnรฉralement les paramรจtres suivants ร [`Trainer`] :
1. Un [`PreTrainedModel`] ou un [`torch.nn.Module`](https://pytorch.org/docs/stable/nn.html#torch.nn.Module):
```py
>>> from transformers import AutoModelForSequenceClassification
>>> model = AutoModelForSequenceClassification.from_pretrained("distilbert-base-uncased")
```
2. [`TrainingArguments`] contient les hyperparamรจtres du modรจle que vous pouvez changer comme le taux d'apprentissage, la taille due l'รฉchantillon, et le nombre d'รฉpoques pour s'entraรฎner. Les valeurs par dรฉfaut sont utilisรฉes si vous ne spรฉcifiez pas d'hyperparamรจtres d'apprentissage :
```py
>>> from transformers import TrainingArguments
>>> training_args = TrainingArguments(
... output_dir="path/to/save/folder/",
... learning_rate=2e-5,
... per_device_train_batch_size=8,
... per_device_eval_batch_size=8,
... num_train_epochs=2,
... )
```
3. Une classe de prรฉtraitement comme un tokenizer, un processeur d'images ou un extracteur de caractรฉristiques :
```py
>>> from transformers import AutoTokenizer
>>> tokenizer = AutoTokenizer.from_pretrained("distilbert-base-uncased")
```
4. Chargez un jeu de donnรฉes :
```py
>>> from datasets import load_dataset
>>> dataset = load_dataset("rotten_tomatoes") # doctest: +IGNORE_RESULT
```
5. Crรฉez une fonction qui transforme le texte du jeu de donnรฉes en token :
```py
>>> def tokenize_dataset(dataset):
... return tokenizer(dataset["text"])
```
Puis appliquez-la ร l'intรฉgralitรฉ du jeu de donnรฉes avec [`~datasets.Dataset.map`]:
```py
>>> dataset = dataset.map(tokenize_dataset, batched=True)
```
6. Un [`DataCollatorWithPadding`] pour crรฉer un รฉchantillon d'exemples ร partir de votre jeu de donnรฉes :
```py
>>> from transformers import DataCollatorWithPadding
>>> data_collator = DataCollatorWithPadding(tokenizer=tokenizer)
```
Maintenant, rassemblez tous ces รฉlรฉments dans un [`Trainer`] :
```py
>>> from transformers import Trainer
>>> trainer = Trainer(
... model=model,
... args=training_args,
... train_dataset=dataset["train"],
... eval_dataset=dataset["test"],
... tokenizer=tokenizer,
... data_collator=data_collator,
... ) # doctest: +SKIP
```
Une fois que vous รชtes prรชt, appelez la fonction [`~Trainer.train`] pour commencer l'entraรฎnement :
```py
>>> trainer.train() # doctest: +SKIP
```
<Tip>
Pour les tรขches - comme la traduction ou la gรฉnรฉration de rรฉsumรฉ - qui utilisent un modรจle sรฉquence ร sรฉquence, utilisez plutรดt les classes [`Seq2SeqTrainer`] et [`Seq2SeqTrainingArguments`].
</Tip>
Vous pouvez personnaliser le comportement de la boucle d'apprentissage en redรฉfinissant les mรฉthodes ร l'intรฉrieur de [`Trainer`]. Cela vous permet de personnaliser des caractรฉristiques telles que la fonction de perte, l'optimiseur et le planificateur. Consultez la documentation de [`Trainer`] pour savoir quelles mรฉthodes peuvent รชtre redรฉfinies.
L'autre moyen de personnaliser la boucle d'apprentissage est d'utiliser les [Callbacks](./main_classes/callbacks). Vous pouvez utiliser les callbacks pour intรฉgrer d'autres bibliothรจques et inspecter la boucle d'apprentissage afin de suivre la progression ou d'arrรชter l'apprentissage plus tรดt. Les callbacks ne modifient rien dans la boucle d'apprentissage elle-mรชme. Pour personnaliser quelque chose comme la fonction de perte, vous devez redรฉfinir le [`Trainer`] ร la place.
## Entraรฎnement avec TensorFlow
Tous les modรจles sont des modรจles standard [`tf.keras.Model`](https://www.tensorflow.org/api_docs/python/tf/keras/Model) afin qu'ils puissent รชtre entraรฎnรฉs avec TensorFlow avec l'API [Keras](https://keras.io/). ๐ค Transformers fournit la fonction [`~TFPreTrainedModel.prepare_tf_dataset`] pour charger facilement votre jeu de donnรฉes comme un `tf.data.Dataset` afin que vous puissiez commencer l'entraรฎnement immรฉdiatement avec les fonctions [`compile`](https://keras.io/api/models/model_training_apis/#compile-method) et [`fit`](https://keras.io/api/models/model_training_apis/#fit-method) de Keras.
1. Vous commencez avec un modรจle [`TFPreTrainedModel`] ou [`tf.keras.Model`](https://www.tensorflow.org/api_docs/python/tf/keras/Model) :
```py
>>> from transformers import TFAutoModelForSequenceClassification
>>> model = TFAutoModelForSequenceClassification.from_pretrained("distilbert-base-uncased")
```
2. Une classe de prรฉtraitement comme un tokenizer, un processeur d'images ou un extracteur de caractรฉristiques :
```py
>>> from transformers import AutoTokenizer
>>> tokenizer = AutoTokenizer.from_pretrained("distilbert-base-uncased")
```
3. Crรฉez une fonction qui transforme le texte du jeu de donnรฉes en token :
```py
>>> def tokenize_dataset(dataset):
... return tokenizer(dataset["text"]) # doctest: +SKIP
```
4. Appliquez le tokenizer ร l'ensemble du jeu de donnรฉes avec [`~datasets.Dataset.map`] et passez ensuite le jeu de donnรฉes et le tokenizer ร [`~TFPreTrainedModel.prepare_tf_dataset`]. Vous pouvez รฉgalement modifier la taille de l'รฉchantillon et mรฉlanger le jeu de donnรฉes ici si vous le souhaitez :
```py
>>> dataset = dataset.map(tokenize_dataset) # doctest: +SKIP
>>> tf_dataset = model.prepare_tf_dataset(
... dataset, batch_size=16, shuffle=True, tokenizer=tokenizer
... ) # doctest: +SKIP
```
5. Une fois que vous รชtes prรชt, appelez les fonctions `compile` et `fit` pour commencer l'entraรฎnement :
```py
>>> from tensorflow.keras.optimizers import Adam
>>> model.compile(optimizer=Adam(3e-5))
>>> model.fit(dataset) # doctest: +SKIP
```
## Et aprรจs ?
Maintenant que vous avez terminรฉ la visite rapide de ๐ค Transformers, consultez nos guides et apprenez ร faire des choses plus spรฉcifiques comme crรฉer un modรจle personnalisรฉ, finetuner un modรจle pour une tรขche, et comment entraรฎner un modรจle avec un script. Si vous souhaitez en savoir plus sur les concepts fondamentaux de ๐ค Transformers, jetez un ลil ร nos guides conceptuels ! | 0 |
hf_public_repos/transformers/docs/source | hf_public_repos/transformers/docs/source/fr/index.md | <!--Copyright 2020 The HuggingFace Team. All rights reserved.
Licensed under the Apache License, Version 2.0 (the "License"); you may not use this file except in compliance with
the License. You may obtain a copy of the License at
http://www.apache.org/licenses/LICENSE-2.0
Unless required by applicable law or agreed to in writing, software distributed under the License is distributed on
an "AS IS" BASIS, WITHOUT WARRANTIES OR CONDITIONS OF ANY KIND, either express or implied. See the License for the
specific language governing permissions and limitations under the License.
โ ๏ธ Note that this file is in Markdown but contain specific syntax for our doc-builder (similar to MDX) that may not be
rendered properly in your Markdown viewer.
-->
# ๐ค Transformers
Apprentissage automatique de pointe pour [PyTorch](https://pytorch.org/), [TensorFlow](https://www.tensorflow.org/), et [JAX](https://jax.readthedocs.io/en/latest/).
๐ค Transformers fournit des API et des outils pour tรฉlรฉcharger et entraรฎner facilement des modรจles prรฉ-entraรฎnรฉs de pointe. L'utilisation de modรจles prรฉ-entraรฎnรฉs peut rรฉduire vos coรปts de calcul, votre empreinte carbone, et vous faire รฉconomiser le temps et les ressources nรฉcessaires pour entraรฎner un modรจle ร partir de zรฉro. Ces modรจles prennent en charge des tรขches courantes dans diffรฉrentes modalitรฉs, telles que :
๐ **Traitement automatique des langues**: classification de texte, reconnaissance d'entitรฉs, systรจme de question-rรฉponse, modรจle de langage, gรฉnรฉration de rรฉsumรฉ, traduction, question ร choix multiples et gรฉnรฉration de texte.<br>
๐ผ๏ธ **Vision par ordinateur**: classification d'image, dรฉtection d'objet et segmentation.<br>
๐ฃ๏ธ **Audio**: reconnaissance automatique de la parole et classification audio.<br>
๐ **Multimodalitรฉ**: systรจme de question-rรฉponse avec des tableaux ou images, reconnaissance optique de caractรจres, extraction d'information depuis des documents scannรฉs et classification de vidรฉo.
๐ค Transformers prend en charge l'interopรฉrabilitรฉ entre PyTorch, TensorFlow et JAX. Cela permet d'utiliser un framework diffรฉrent ร chaque รฉtape de la vie d'un modรจle, par example entraรฎner un modรจle en trois lignes de code avec un framework, et le charger pour l'infรฉrence avec un autre. Les modรจles peuvent รฉgalement รชtre exportรฉs dans un format comme ONNX et TorchScript pour รชtre dรฉployรฉs dans des environnements de production.
Rejoignez la communautรฉ grandissante sur le [Hub](https://huggingface.co/models), le [forum](https://discuss.huggingface.co/) ou [Discord](https://discord.com/invite/JfAtkvEtRb) dรจs aujourd'hui !
## Si vous cherchez un support personnalisรฉ de l'รฉquipe Hugging Face
<a target="_blank" href="https://huggingface.co/support">
<img alt="HuggingFace Expert Acceleration Program" src="https://cdn-media.huggingface.co/marketing/transformers/new-support-improved.png" style="width: 100%; max-width: 600px; border: 1px solid #eee; border-radius: 4px; box-shadow: 0 1px 2px 0 rgba(0, 0, 0, 0.05);">
</a>
## Contents
La documentation est organisรฉe en 5 parties:
- **DEMARRER** propose une visite rapide de la bibliothรจque et des instructions d'installation pour รชtre opรฉrationnel.
- **TUTORIELS** excellent point de dรฉpart pour les dรฉbutants. Cette section vous aidera ร acquรฉrir les compรฉtences de base dont vous avez besoin pour commencer ร utiliser la bibliothรจque.
- **GUIDES D'UTILISATION** pour diffรฉrentes tรขches comme par exemple le finetuning d'un modรจle prรฉ-entraรฎnรฉ pour la classification de texte ou comment crรฉer et partager votre propre modรจle.
- **GUIDES CONCEPTUELS** pour plus de discussions et d'explications sur les concepts et les idรฉes sous-jacentes aux modรจles, aux tรขches et ร la philosophie de conception de ๐ค Transformers.
- **API** dรฉcrit toutes les classes et fonctions :
- **CLASSES PRINCIPALES** dรฉtaille les classes les plus importantes comme la configuration, le modรจle, le tokenizer et le pipeline..
- **MODELES** dรฉtaille les classes et les fonctions propres ร chaque modรจle de la bibliothรจque.
- **UTILITAIRES INTERNES** dรฉtaille les classes et fonctions utilitaires utilisรฉes en interne.
### Modรจles supportรฉs
<!--This list is updated automatically from the README with _make fix-copies_. Do not update manually! -->
1. **[ALBERT](model_doc/albert)** (from Google Research and the Toyota Technological Institute at Chicago) released with the paper [ALBERT: A Lite BERT for Self-supervised Learning of Language Representations](https://arxiv.org/abs/1909.11942), by Zhenzhong Lan, Mingda Chen, Sebastian Goodman, Kevin Gimpel, Piyush Sharma, Radu Soricut.
1. **[ALIGN](model_doc/align)** (from Google Research) released with the paper [Scaling Up Visual and Vision-Language Representation Learning With Noisy Text Supervision](https://arxiv.org/abs/2102.05918) by Chao Jia, Yinfei Yang, Ye Xia, Yi-Ting Chen, Zarana Parekh, Hieu Pham, Quoc V. Le, Yunhsuan Sung, Zhen Li, Tom Duerig.
1. **[AltCLIP](model_doc/altclip)** (from BAAI) released with the paper [AltCLIP: Altering the Language Encoder in CLIP for Extended Language Capabilities](https://arxiv.org/abs/2211.06679) by Chen, Zhongzhi and Liu, Guang and Zhang, Bo-Wen and Ye, Fulong and Yang, Qinghong and Wu, Ledell.
1. **[Audio Spectrogram Transformer](model_doc/audio-spectrogram-transformer)** (from MIT) released with the paper [AST: Audio Spectrogram Transformer](https://arxiv.org/abs/2104.01778) by Yuan Gong, Yu-An Chung, James Glass.
1. **[BART](model_doc/bart)** (from Facebook) released with the paper [BART: Denoising Sequence-to-Sequence Pre-training for Natural Language Generation, Translation, and Comprehension](https://arxiv.org/abs/1910.13461) by Mike Lewis, Yinhan Liu, Naman Goyal, Marjan Ghazvininejad, Abdelrahman Mohamed, Omer Levy, Ves Stoyanov and Luke Zettlemoyer.
1. **[BARThez](model_doc/barthez)** (from รcole polytechnique) released with the paper [BARThez: a Skilled Pretrained French Sequence-to-Sequence Model](https://arxiv.org/abs/2010.12321) by Moussa Kamal Eddine, Antoine J.-P. Tixier, Michalis Vazirgiannis.
1. **[BARTpho](model_doc/bartpho)** (from VinAI Research) released with the paper [BARTpho: Pre-trained Sequence-to-Sequence Models for Vietnamese](https://arxiv.org/abs/2109.09701) by Nguyen Luong Tran, Duong Minh Le and Dat Quoc Nguyen.
1. **[BEiT](model_doc/beit)** (from Microsoft) released with the paper [BEiT: BERT Pre-Training of Image Transformers](https://arxiv.org/abs/2106.08254) by Hangbo Bao, Li Dong, Furu Wei.
1. **[BERT](model_doc/bert)** (from Google) released with the paper [BERT: Pre-training of Deep Bidirectional Transformers for Language Understanding](https://arxiv.org/abs/1810.04805) by Jacob Devlin, Ming-Wei Chang, Kenton Lee and Kristina Toutanova.
1. **[BERT For Sequence Generation](model_doc/bert-generation)** (from Google) released with the paper [Leveraging Pre-trained Checkpoints for Sequence Generation Tasks](https://arxiv.org/abs/1907.12461) by Sascha Rothe, Shashi Narayan, Aliaksei Severyn.
1. **[BERTweet](model_doc/bertweet)** (from VinAI Research) released with the paper [BERTweet: A pre-trained language model for English Tweets](https://aclanthology.org/2020.emnlp-demos.2/) by Dat Quoc Nguyen, Thanh Vu and Anh Tuan Nguyen.
1. **[BigBird-Pegasus](model_doc/bigbird_pegasus)** (from Google Research) released with the paper [Big Bird: Transformers for Longer Sequences](https://arxiv.org/abs/2007.14062) by Manzil Zaheer, Guru Guruganesh, Avinava Dubey, Joshua Ainslie, Chris Alberti, Santiago Ontanon, Philip Pham, Anirudh Ravula, Qifan Wang, Li Yang, Amr Ahmed.
1. **[BigBird-RoBERTa](model_doc/big_bird)** (from Google Research) released with the paper [Big Bird: Transformers for Longer Sequences](https://arxiv.org/abs/2007.14062) by Manzil Zaheer, Guru Guruganesh, Avinava Dubey, Joshua Ainslie, Chris Alberti, Santiago Ontanon, Philip Pham, Anirudh Ravula, Qifan Wang, Li Yang, Amr Ahmed.
1. **[BioGpt](model_doc/biogpt)** (from Microsoft Research AI4Science) released with the paper [BioGPT: generative pre-trained transformer for biomedical text generation and mining](https://academic.oup.com/bib/advance-article/doi/10.1093/bib/bbac409/6713511?guestAccessKey=a66d9b5d-4f83-4017-bb52-405815c907b9) by Renqian Luo, Liai Sun, Yingce Xia, Tao Qin, Sheng Zhang, Hoifung Poon and Tie-Yan Liu.
1. **[BiT](model_doc/bit)** (from Google AI) released with the paper [Big Transfer (BiT): General Visual Representation Learning](https://arxiv.org/abs/1912.11370) by Alexander Kolesnikov, Lucas Beyer, Xiaohua Zhai, Joan Puigcerver, Jessica Yung, Sylvain Gelly, Neil Houlsby.
1. **[Blenderbot](model_doc/blenderbot)** (from Facebook) released with the paper [Recipes for building an open-domain chatbot](https://arxiv.org/abs/2004.13637) by Stephen Roller, Emily Dinan, Naman Goyal, Da Ju, Mary Williamson, Yinhan Liu, Jing Xu, Myle Ott, Kurt Shuster, Eric M. Smith, Y-Lan Boureau, Jason Weston.
1. **[BlenderbotSmall](model_doc/blenderbot-small)** (from Facebook) released with the paper [Recipes for building an open-domain chatbot](https://arxiv.org/abs/2004.13637) by Stephen Roller, Emily Dinan, Naman Goyal, Da Ju, Mary Williamson, Yinhan Liu, Jing Xu, Myle Ott, Kurt Shuster, Eric M. Smith, Y-Lan Boureau, Jason Weston.
1. **[BLIP](model_doc/blip)** (from Salesforce) released with the paper [BLIP: Bootstrapping Language-Image Pre-training for Unified Vision-Language Understanding and Generation](https://arxiv.org/abs/2201.12086) by Junnan Li, Dongxu Li, Caiming Xiong, Steven Hoi.
1. **[BLOOM](model_doc/bloom)** (from BigScience workshop) released by the [BigScience Workshop](https://bigscience.huggingface.co/).
1. **[BORT](model_doc/bort)** (from Alexa) released with the paper [Optimal Subarchitecture Extraction For BERT](https://arxiv.org/abs/2010.10499) by Adrian de Wynter and Daniel J. Perry.
1. **[BridgeTower](model_doc/bridgetower)** (from Harbin Institute of Technology/Microsoft Research Asia/Intel Labs) released with the paper [BridgeTower: Building Bridges Between Encoders in Vision-Language Representation Learning](https://arxiv.org/abs/2206.08657) by Xiao Xu, Chenfei Wu, Shachar Rosenman, Vasudev Lal, Wanxiang Che, Nan Duan.
1. **[ByT5](model_doc/byt5)** (from Google Research) released with the paper [ByT5: Towards a token-free future with pre-trained byte-to-byte models](https://arxiv.org/abs/2105.13626) by Linting Xue, Aditya Barua, Noah Constant, Rami Al-Rfou, Sharan Narang, Mihir Kale, Adam Roberts, Colin Raffel.
1. **[CamemBERT](model_doc/camembert)** (from Inria/Facebook/Sorbonne) released with the paper [CamemBERT: a Tasty French Language Model](https://arxiv.org/abs/1911.03894) by Louis Martin*, Benjamin Muller*, Pedro Javier Ortiz Suรกrez*, Yoann Dupont, Laurent Romary, รric Villemonte de la Clergerie, Djamรฉ Seddah and Benoรฎt Sagot.
1. **[CANINE](model_doc/canine)** (from Google Research) released with the paper [CANINE: Pre-training an Efficient Tokenization-Free Encoder for Language Representation](https://arxiv.org/abs/2103.06874) by Jonathan H. Clark, Dan Garrette, Iulia Turc, John Wieting.
1. **[Chinese-CLIP](model_doc/chinese_clip)** (from OFA-Sys) released with the paper [Chinese CLIP: Contrastive Vision-Language Pretraining in Chinese](https://arxiv.org/abs/2211.01335) by An Yang, Junshu Pan, Junyang Lin, Rui Men, Yichang Zhang, Jingren Zhou, Chang Zhou.
1. **[CLIP](model_doc/clip)** (from OpenAI) released with the paper [Learning Transferable Visual Models From Natural Language Supervision](https://arxiv.org/abs/2103.00020) by Alec Radford, Jong Wook Kim, Chris Hallacy, Aditya Ramesh, Gabriel Goh, Sandhini Agarwal, Girish Sastry, Amanda Askell, Pamela Mishkin, Jack Clark, Gretchen Krueger, Ilya Sutskever.
1. **[CLIPSeg](model_doc/clipseg)** (from University of Gรถttingen) released with the paper [Image Segmentation Using Text and Image Prompts](https://arxiv.org/abs/2112.10003) by Timo Lรผddecke and Alexander Ecker.
1. **[CodeGen](model_doc/codegen)** (from Salesforce) released with the paper [A Conversational Paradigm for Program Synthesis](https://arxiv.org/abs/2203.13474) by Erik Nijkamp, Bo Pang, Hiroaki Hayashi, Lifu Tu, Huan Wang, Yingbo Zhou, Silvio Savarese, Caiming Xiong.
1. **[Conditional DETR](model_doc/conditional_detr)** (from Microsoft Research Asia) released with the paper [Conditional DETR for Fast Training Convergence](https://arxiv.org/abs/2108.06152) by Depu Meng, Xiaokang Chen, Zejia Fan, Gang Zeng, Houqiang Li, Yuhui Yuan, Lei Sun, Jingdong Wang.
1. **[ConvBERT](model_doc/convbert)** (from YituTech) released with the paper [ConvBERT: Improving BERT with Span-based Dynamic Convolution](https://arxiv.org/abs/2008.02496) by Zihang Jiang, Weihao Yu, Daquan Zhou, Yunpeng Chen, Jiashi Feng, Shuicheng Yan.
1. **[ConvNeXT](model_doc/convnext)** (from Facebook AI) released with the paper [A ConvNet for the 2020s](https://arxiv.org/abs/2201.03545) by Zhuang Liu, Hanzi Mao, Chao-Yuan Wu, Christoph Feichtenhofer, Trevor Darrell, Saining Xie.
1. **[ConvNeXTV2](model_doc/convnextv2)** (from Facebook AI) released with the paper [ConvNeXt V2: Co-designing and Scaling ConvNets with Masked Autoencoders](https://arxiv.org/abs/2301.00808) by Sanghyun Woo, Shoubhik Debnath, Ronghang Hu, Xinlei Chen, Zhuang Liu, In So Kweon, Saining Xie.
1. **[CPM](model_doc/cpm)** (from Tsinghua University) released with the paper [CPM: A Large-scale Generative Chinese Pre-trained Language Model](https://arxiv.org/abs/2012.00413) by Zhengyan Zhang, Xu Han, Hao Zhou, Pei Ke, Yuxian Gu, Deming Ye, Yujia Qin, Yusheng Su, Haozhe Ji, Jian Guan, Fanchao Qi, Xiaozhi Wang, Yanan Zheng, Guoyang Zeng, Huanqi Cao, Shengqi Chen, Daixuan Li, Zhenbo Sun, Zhiyuan Liu, Minlie Huang, Wentao Han, Jie Tang, Juanzi Li, Xiaoyan Zhu, Maosong Sun.
1. **[CTRL](model_doc/ctrl)** (from Salesforce) released with the paper [CTRL: A Conditional Transformer Language Model for Controllable Generation](https://arxiv.org/abs/1909.05858) by Nitish Shirish Keskar*, Bryan McCann*, Lav R. Varshney, Caiming Xiong and Richard Socher.
1. **[CvT](model_doc/cvt)** (from Microsoft) released with the paper [CvT: Introducing Convolutions to Vision Transformers](https://arxiv.org/abs/2103.15808) by Haiping Wu, Bin Xiao, Noel Codella, Mengchen Liu, Xiyang Dai, Lu Yuan, Lei Zhang.
1. **[Data2Vec](model_doc/data2vec)** (from Facebook) released with the paper [Data2Vec: A General Framework for Self-supervised Learning in Speech, Vision and Language](https://arxiv.org/abs/2202.03555) by Alexei Baevski, Wei-Ning Hsu, Qiantong Xu, Arun Babu, Jiatao Gu, Michael Auli.
1. **[DeBERTa](model_doc/deberta)** (from Microsoft) released with the paper [DeBERTa: Decoding-enhanced BERT with Disentangled Attention](https://arxiv.org/abs/2006.03654) by Pengcheng He, Xiaodong Liu, Jianfeng Gao, Weizhu Chen.
1. **[DeBERTa-v2](model_doc/deberta-v2)** (from Microsoft) released with the paper [DeBERTa: Decoding-enhanced BERT with Disentangled Attention](https://arxiv.org/abs/2006.03654) by Pengcheng He, Xiaodong Liu, Jianfeng Gao, Weizhu Chen.
1. **[Decision Transformer](model_doc/decision_transformer)** (from Berkeley/Facebook/Google) released with the paper [Decision Transformer: Reinforcement Learning via Sequence Modeling](https://arxiv.org/abs/2106.01345) by Lili Chen, Kevin Lu, Aravind Rajeswaran, Kimin Lee, Aditya Grover, Michael Laskin, Pieter Abbeel, Aravind Srinivas, Igor Mordatch.
1. **[Deformable DETR](model_doc/deformable_detr)** (from SenseTime Research) released with the paper [Deformable DETR: Deformable Transformers for End-to-End Object Detection](https://arxiv.org/abs/2010.04159) by Xizhou Zhu, Weijie Su, Lewei Lu, Bin Li, Xiaogang Wang, Jifeng Dai.
1. **[DeiT](model_doc/deit)** (from Facebook) released with the paper [Training data-efficient image transformers & distillation through attention](https://arxiv.org/abs/2012.12877) by Hugo Touvron, Matthieu Cord, Matthijs Douze, Francisco Massa, Alexandre Sablayrolles, Hervรฉ Jรฉgou.
1. **[DETA](model_doc/deta)** (from The University of Texas at Austin) released with the paper [NMS Strikes Back](https://arxiv.org/abs/2212.06137) by Jeffrey Ouyang-Zhang, Jang Hyun Cho, Xingyi Zhou, Philipp Krรคhenbรผhl.
1. **[DETR](model_doc/detr)** (from Facebook) released with the paper [End-to-End Object Detection with Transformers](https://arxiv.org/abs/2005.12872) by Nicolas Carion, Francisco Massa, Gabriel Synnaeve, Nicolas Usunier, Alexander Kirillov, Sergey Zagoruyko.
1. **[DialoGPT](model_doc/dialogpt)** (from Microsoft Research) released with the paper [DialoGPT: Large-Scale Generative Pre-training for Conversational Response Generation](https://arxiv.org/abs/1911.00536) by Yizhe Zhang, Siqi Sun, Michel Galley, Yen-Chun Chen, Chris Brockett, Xiang Gao, Jianfeng Gao, Jingjing Liu, Bill Dolan.
1. **[DiNAT](model_doc/dinat)** (from SHI Labs) released with the paper [Dilated Neighborhood Attention Transformer](https://arxiv.org/abs/2209.15001) by Ali Hassani and Humphrey Shi.
1. **[DistilBERT](model_doc/distilbert)** (from HuggingFace), released together with the paper [DistilBERT, a distilled version of BERT: smaller, faster, cheaper and lighter](https://arxiv.org/abs/1910.01108) by Victor Sanh, Lysandre Debut and Thomas Wolf. The same method has been applied to compress GPT2 into [DistilGPT2](https://github.com/huggingface/transformers/tree/main/examples/research_projects/distillation), RoBERTa into [DistilRoBERTa](https://github.com/huggingface/transformers/tree/main/examples/research_projects/distillation), Multilingual BERT into [DistilmBERT](https://github.com/huggingface/transformers/tree/main/examples/research_projects/distillation) and a German version of DistilBERT.
1. **[DiT](model_doc/dit)** (from Microsoft Research) released with the paper [DiT: Self-supervised Pre-training for Document Image Transformer](https://arxiv.org/abs/2203.02378) by Junlong Li, Yiheng Xu, Tengchao Lv, Lei Cui, Cha Zhang, Furu Wei.
1. **[Donut](model_doc/donut)** (from NAVER), released together with the paper [OCR-free Document Understanding Transformer](https://arxiv.org/abs/2111.15664) by Geewook Kim, Teakgyu Hong, Moonbin Yim, Jeongyeon Nam, Jinyoung Park, Jinyeong Yim, Wonseok Hwang, Sangdoo Yun, Dongyoon Han, Seunghyun Park.
1. **[DPR](model_doc/dpr)** (from Facebook) released with the paper [Dense Passage Retrieval for Open-Domain Question Answering](https://arxiv.org/abs/2004.04906) by Vladimir Karpukhin, Barlas Oฤuz, Sewon Min, Patrick Lewis, Ledell Wu, Sergey Edunov, Danqi Chen, and Wen-tau Yih.
1. **[DPT](master/model_doc/dpt)** (from Intel Labs) released with the paper [Vision Transformers for Dense Prediction](https://arxiv.org/abs/2103.13413) by Renรฉ Ranftl, Alexey Bochkovskiy, Vladlen Koltun.
1. **[EfficientFormer](model_doc/efficientformer)** (from Snap Research) released with the paper [EfficientFormer: Vision Transformers at MobileNetSpeed](https://arxiv.org/abs/2206.01191) by Yanyu Li, Geng Yuan, Yang Wen, Ju Hu, Georgios Evangelidis, Sergey Tulyakov, Yanzhi Wang, Jian Ren.
1. **[ELECTRA](model_doc/electra)** (from Google Research/Stanford University) released with the paper [ELECTRA: Pre-training text encoders as discriminators rather than generators](https://arxiv.org/abs/2003.10555) by Kevin Clark, Minh-Thang Luong, Quoc V. Le, Christopher D. Manning.
1. **[EncoderDecoder](model_doc/encoder-decoder)** (from Google Research) released with the paper [Leveraging Pre-trained Checkpoints for Sequence Generation Tasks](https://arxiv.org/abs/1907.12461) by Sascha Rothe, Shashi Narayan, Aliaksei Severyn.
1. **[ERNIE](model_doc/ernie)** (from Baidu) released with the paper [ERNIE: Enhanced Representation through Knowledge Integration](https://arxiv.org/abs/1904.09223) by Yu Sun, Shuohuan Wang, Yukun Li, Shikun Feng, Xuyi Chen, Han Zhang, Xin Tian, Danxiang Zhu, Hao Tian, Hua Wu.
1. **[ESM](model_doc/esm)** (from Meta AI) are transformer protein language models. **ESM-1b** was released with the paper [Biological structure and function emerge from scaling unsupervised learning to 250 million protein sequences](https://www.pnas.org/content/118/15/e2016239118) by Alexander Rives, Joshua Meier, Tom Sercu, Siddharth Goyal, Zeming Lin, Jason Liu, Demi Guo, Myle Ott, C. Lawrence Zitnick, Jerry Ma, and Rob Fergus. **ESM-1v** was released with the paper [Language models enable zero-shot prediction of the effects of mutations on protein function](https://doi.org/10.1101/2021.07.09.450648) by Joshua Meier, Roshan Rao, Robert Verkuil, Jason Liu, Tom Sercu and Alexander Rives. **ESM-2 and ESMFold** were released with the paper [Language models of protein sequences at the scale of evolution enable accurate structure prediction](https://doi.org/10.1101/2022.07.20.500902) by Zeming Lin, Halil Akin, Roshan Rao, Brian Hie, Zhongkai Zhu, Wenting Lu, Allan dos Santos Costa, Maryam Fazel-Zarandi, Tom Sercu, Sal Candido, Alexander Rives.
1. **[FLAN-T5](model_doc/flan-t5)** (from Google AI) released in the repository [google-research/t5x](https://github.com/google-research/t5x/blob/main/docs/models.md#flan-t5-checkpoints) by Hyung Won Chung, Le Hou, Shayne Longpre, Barret Zoph, Yi Tay, William Fedus, Eric Li, Xuezhi Wang, Mostafa Dehghani, Siddhartha Brahma, Albert Webson, Shixiang Shane Gu, Zhuyun Dai, Mirac Suzgun, Xinyun Chen, Aakanksha Chowdhery, Sharan Narang, Gaurav Mishra, Adams Yu, Vincent Zhao, Yanping Huang, Andrew Dai, Hongkun Yu, Slav Petrov, Ed H. Chi, Jeff Dean, Jacob Devlin, Adam Roberts, Denny Zhou, Quoc V. Le, and Jason Wei
1. **[FlauBERT](model_doc/flaubert)** (from CNRS) released with the paper [FlauBERT: Unsupervised Language Model Pre-training for French](https://arxiv.org/abs/1912.05372) by Hang Le, Loรฏc Vial, Jibril Frej, Vincent Segonne, Maximin Coavoux, Benjamin Lecouteux, Alexandre Allauzen, Benoรฎt Crabbรฉ, Laurent Besacier, Didier Schwab.
1. **[FLAVA](model_doc/flava)** (from Facebook AI) released with the paper [FLAVA: A Foundational Language And Vision Alignment Model](https://arxiv.org/abs/2112.04482) by Amanpreet Singh, Ronghang Hu, Vedanuj Goswami, Guillaume Couairon, Wojciech Galuba, Marcus Rohrbach, and Douwe Kiela.
1. **[FNet](model_doc/fnet)** (from Google Research) released with the paper [FNet: Mixing Tokens with Fourier Transforms](https://arxiv.org/abs/2105.03824) by James Lee-Thorp, Joshua Ainslie, Ilya Eckstein, Santiago Ontanon.
1. **[Funnel Transformer](model_doc/funnel)** (from CMU/Google Brain) released with the paper [Funnel-Transformer: Filtering out Sequential Redundancy for Efficient Language Processing](https://arxiv.org/abs/2006.03236) by Zihang Dai, Guokun Lai, Yiming Yang, Quoc V. Le.
1. **[GIT](model_doc/git)** (from Microsoft Research) released with the paper [GIT: A Generative Image-to-text Transformer for Vision and Language](https://arxiv.org/abs/2205.14100) by Jianfeng Wang, Zhengyuan Yang, Xiaowei Hu, Linjie Li, Kevin Lin, Zhe Gan, Zicheng Liu, Ce Liu, Lijuan Wang.
1. **[GLPN](model_doc/glpn)** (from KAIST) released with the paper [Global-Local Path Networks for Monocular Depth Estimation with Vertical CutDepth](https://arxiv.org/abs/2201.07436) by Doyeon Kim, Woonghyun Ga, Pyungwhan Ahn, Donggyu Joo, Sehwan Chun, Junmo Kim.
1. **[GPT](model_doc/openai-gpt)** (from OpenAI) released with the paper [Improving Language Understanding by Generative Pre-Training](https://blog.openai.com/language-unsupervised/) by Alec Radford, Karthik Narasimhan, Tim Salimans and Ilya Sutskever.
1. **[GPT Neo](model_doc/gpt_neo)** (from EleutherAI) released in the repository [EleutherAI/gpt-neo](https://github.com/EleutherAI/gpt-neo) by Sid Black, Stella Biderman, Leo Gao, Phil Wang and Connor Leahy.
1. **[GPT NeoX](model_doc/gpt_neox)** (from EleutherAI) released with the paper [GPT-NeoX-20B: An Open-Source Autoregressive Language Model](https://arxiv.org/abs/2204.06745) by Sid Black, Stella Biderman, Eric Hallahan, Quentin Anthony, Leo Gao, Laurence Golding, Horace He, Connor Leahy, Kyle McDonell, Jason Phang, Michael Pieler, USVSN Sai Prashanth, Shivanshu Purohit, Laria Reynolds, Jonathan Tow, Ben Wang, Samuel Weinbach
1. **[GPT NeoX Japanese](model_doc/gpt_neox_japanese)** (from ABEJA) released by Shinya Otani, Takayoshi Makabe, Anuj Arora, and Kyo Hattori.
1. **[GPT-2](model_doc/gpt2)** (from OpenAI) released with the paper [Language Models are Unsupervised Multitask Learners](https://blog.openai.com/better-language-models/) by Alec Radford*, Jeffrey Wu*, Rewon Child, David Luan, Dario Amodei** and Ilya Sutskever**.
1. **[GPT-J](model_doc/gptj)** (from EleutherAI) released in the repository [kingoflolz/mesh-transformer-jax](https://github.com/kingoflolz/mesh-transformer-jax/) by Ben Wang and Aran Komatsuzaki.
1. **[GPT-Sw3](model_doc/gpt-sw3)** (from AI-Sweden) released with the paper [Lessons Learned from GPT-SW3: Building the First Large-Scale Generative Language Model for Swedish](http://www.lrec-conf.org/proceedings/lrec2022/pdf/2022.lrec-1.376.pdf) by Ariel Ekgren, Amaru Cuba Gyllensten, Evangelia Gogoulou, Alice Heiman, Severine Verlinden, Joey รhman, Fredrik Carlsson, Magnus Sahlgren.
1. **[Graphormer](model_doc/graphormer)** (from Microsoft) released with the paper [Do Transformers Really Perform Bad for Graph Representation?](https://arxiv.org/abs/2106.05234) by Chengxuan Ying, Tianle Cai, Shengjie Luo, Shuxin Zheng, Guolin Ke, Di He, Yanming Shen, Tie-Yan Liu.
1. **[GroupViT](model_doc/groupvit)** (from UCSD, NVIDIA) released with the paper [GroupViT: Semantic Segmentation Emerges from Text Supervision](https://arxiv.org/abs/2202.11094) by Jiarui Xu, Shalini De Mello, Sifei Liu, Wonmin Byeon, Thomas Breuel, Jan Kautz, Xiaolong Wang.
1. **[Hubert](model_doc/hubert)** (from Facebook) released with the paper [HuBERT: Self-Supervised Speech Representation Learning by Masked Prediction of Hidden Units](https://arxiv.org/abs/2106.07447) by Wei-Ning Hsu, Benjamin Bolte, Yao-Hung Hubert Tsai, Kushal Lakhotia, Ruslan Salakhutdinov, Abdelrahman Mohamed.
1. **[I-BERT](model_doc/ibert)** (from Berkeley) released with the paper [I-BERT: Integer-only BERT Quantization](https://arxiv.org/abs/2101.01321) by Sehoon Kim, Amir Gholami, Zhewei Yao, Michael W. Mahoney, Kurt Keutzer.
1. **[ImageGPT](model_doc/imagegpt)** (from OpenAI) released with the paper [Generative Pretraining from Pixels](https://openai.com/blog/image-gpt/) by Mark Chen, Alec Radford, Rewon Child, Jeffrey Wu, Heewoo Jun, David Luan, Ilya Sutskever.
1. **[Jukebox](model_doc/jukebox)** (from OpenAI) released with the paper [Jukebox: A Generative Model for Music](https://arxiv.org/pdf/2005.00341.pdf) by Prafulla Dhariwal, Heewoo Jun, Christine Payne, Jong Wook Kim, Alec Radford, Ilya Sutskever.
1. **[LayoutLM](model_doc/layoutlm)** (from Microsoft Research Asia) released with the paper [LayoutLM: Pre-training of Text and Layout for Document Image Understanding](https://arxiv.org/abs/1912.13318) by Yiheng Xu, Minghao Li, Lei Cui, Shaohan Huang, Furu Wei, Ming Zhou.
1. **[LayoutLMv2](model_doc/layoutlmv2)** (from Microsoft Research Asia) released with the paper [LayoutLMv2: Multi-modal Pre-training for Visually-Rich Document Understanding](https://arxiv.org/abs/2012.14740) by Yang Xu, Yiheng Xu, Tengchao Lv, Lei Cui, Furu Wei, Guoxin Wang, Yijuan Lu, Dinei Florencio, Cha Zhang, Wanxiang Che, Min Zhang, Lidong Zhou.
1. **[LayoutLMv3](model_doc/layoutlmv3)** (from Microsoft Research Asia) released with the paper [LayoutLMv3: Pre-training for Document AI with Unified Text and Image Masking](https://arxiv.org/abs/2204.08387) by Yupan Huang, Tengchao Lv, Lei Cui, Yutong Lu, Furu Wei.
1. **[LayoutXLM](model_doc/layoutxlm)** (from Microsoft Research Asia) released with the paper [LayoutXLM: Multimodal Pre-training for Multilingual Visually-rich Document Understanding](https://arxiv.org/abs/2104.08836) by Yiheng Xu, Tengchao Lv, Lei Cui, Guoxin Wang, Yijuan Lu, Dinei Florencio, Cha Zhang, Furu Wei.
1. **[LED](model_doc/led)** (from AllenAI) released with the paper [Longformer: The Long-Document Transformer](https://arxiv.org/abs/2004.05150) by Iz Beltagy, Matthew E. Peters, Arman Cohan.
1. **[LeViT](model_doc/levit)** (from Meta AI) released with the paper [LeViT: A Vision Transformer in ConvNet's Clothing for Faster Inference](https://arxiv.org/abs/2104.01136) by Ben Graham, Alaaeldin El-Nouby, Hugo Touvron, Pierre Stock, Armand Joulin, Hervรฉ Jรฉgou, Matthijs Douze.
1. **[LiLT](model_doc/lilt)** (from South China University of Technology) released with the paper [LiLT: A Simple yet Effective Language-Independent Layout Transformer for Structured Document Understanding](https://arxiv.org/abs/2202.13669) by Jiapeng Wang, Lianwen Jin, Kai Ding.
1. **[Longformer](model_doc/longformer)** (from AllenAI) released with the paper [Longformer: The Long-Document Transformer](https://arxiv.org/abs/2004.05150) by Iz Beltagy, Matthew E. Peters, Arman Cohan.
1. **[LongT5](model_doc/longt5)** (from Google AI) released with the paper [LongT5: Efficient Text-To-Text Transformer for Long Sequences](https://arxiv.org/abs/2112.07916) by Mandy Guo, Joshua Ainslie, David Uthus, Santiago Ontanon, Jianmo Ni, Yun-Hsuan Sung, Yinfei Yang.
1. **[LUKE](model_doc/luke)** (from Studio Ousia) released with the paper [LUKE: Deep Contextualized Entity Representations with Entity-aware Self-attention](https://arxiv.org/abs/2010.01057) by Ikuya Yamada, Akari Asai, Hiroyuki Shindo, Hideaki Takeda, Yuji Matsumoto.
1. **[LXMERT](model_doc/lxmert)** (from UNC Chapel Hill) released with the paper [LXMERT: Learning Cross-Modality Encoder Representations from Transformers for Open-Domain Question Answering](https://arxiv.org/abs/1908.07490) by Hao Tan and Mohit Bansal.
1. **[M-CTC-T](model_doc/mctct)** (from Facebook) released with the paper [Pseudo-Labeling For Massively Multilingual Speech Recognition](https://arxiv.org/abs/2111.00161) by Loren Lugosch, Tatiana Likhomanenko, Gabriel Synnaeve, and Ronan Collobert.
1. **[M2M100](model_doc/m2m_100)** (from Facebook) released with the paper [Beyond English-Centric Multilingual Machine Translation](https://arxiv.org/abs/2010.11125) by Angela Fan, Shruti Bhosale, Holger Schwenk, Zhiyi Ma, Ahmed El-Kishky, Siddharth Goyal, Mandeep Baines, Onur Celebi, Guillaume Wenzek, Vishrav Chaudhary, Naman Goyal, Tom Birch, Vitaliy Liptchinsky, Sergey Edunov, Edouard Grave, Michael Auli, Armand Joulin.
1. **[MarianMT](model_doc/marian)** Machine translation models trained using [OPUS](http://opus.nlpl.eu/) data by Jรถrg Tiedemann. The [Marian Framework](https://marian-nmt.github.io/) is being developed by the Microsoft Translator Team.
1. **[MarkupLM](model_doc/markuplm)** (from Microsoft Research Asia) released with the paper [MarkupLM: Pre-training of Text and Markup Language for Visually-rich Document Understanding](https://arxiv.org/abs/2110.08518) by Junlong Li, Yiheng Xu, Lei Cui, Furu Wei.
1. **[Mask2Former](model_doc/mask2former)** (from FAIR and UIUC) released with the paper [Masked-attention Mask Transformer for Universal Image Segmentation](https://arxiv.org/abs/2112.01527) by Bowen Cheng, Ishan Misra, Alexander G. Schwing, Alexander Kirillov, Rohit Girdhar.
1. **[MaskFormer](model_doc/maskformer)** (from Meta and UIUC) released with the paper [Per-Pixel Classification is Not All You Need for Semantic Segmentation](https://arxiv.org/abs/2107.06278) by Bowen Cheng, Alexander G. Schwing, Alexander Kirillov.
1. **[mBART](model_doc/mbart)** (from Facebook) released with the paper [Multilingual Denoising Pre-training for Neural Machine Translation](https://arxiv.org/abs/2001.08210) by Yinhan Liu, Jiatao Gu, Naman Goyal, Xian Li, Sergey Edunov, Marjan Ghazvininejad, Mike Lewis, Luke Zettlemoyer.
1. **[mBART-50](model_doc/mbart)** (from Facebook) released with the paper [Multilingual Translation with Extensible Multilingual Pretraining and Finetuning](https://arxiv.org/abs/2008.00401) by Yuqing Tang, Chau Tran, Xian Li, Peng-Jen Chen, Naman Goyal, Vishrav Chaudhary, Jiatao Gu, Angela Fan.
1. **[Megatron-BERT](model_doc/megatron-bert)** (from NVIDIA) released with the paper [Megatron-LM: Training Multi-Billion Parameter Language Models Using Model Parallelism](https://arxiv.org/abs/1909.08053) by Mohammad Shoeybi, Mostofa Patwary, Raul Puri, Patrick LeGresley, Jared Casper and Bryan Catanzaro.
1. **[Megatron-GPT2](model_doc/megatron_gpt2)** (from NVIDIA) released with the paper [Megatron-LM: Training Multi-Billion Parameter Language Models Using Model Parallelism](https://arxiv.org/abs/1909.08053) by Mohammad Shoeybi, Mostofa Patwary, Raul Puri, Patrick LeGresley, Jared Casper and Bryan Catanzaro.
1. **[mLUKE](model_doc/mluke)** (from Studio Ousia) released with the paper [mLUKE: The Power of Entity Representations in Multilingual Pretrained Language Models](https://arxiv.org/abs/2110.08151) by Ryokan Ri, Ikuya Yamada, and Yoshimasa Tsuruoka.
1. **[MobileBERT](model_doc/mobilebert)** (from CMU/Google Brain) released with the paper [MobileBERT: a Compact Task-Agnostic BERT for Resource-Limited Devices](https://arxiv.org/abs/2004.02984) by Zhiqing Sun, Hongkun Yu, Xiaodan Song, Renjie Liu, Yiming Yang, and Denny Zhou.
1. **[MobileNetV1](model_doc/mobilenet_v1)** (from Google Inc.) released with the paper [MobileNets: Efficient Convolutional Neural Networks for Mobile Vision Applications](https://arxiv.org/abs/1704.04861) by Andrew G. Howard, Menglong Zhu, Bo Chen, Dmitry Kalenichenko, Weijun Wang, Tobias Weyand, Marco Andreetto, Hartwig Adam.
1. **[MobileNetV2](model_doc/mobilenet_v2)** (from Google Inc.) released with the paper [MobileNetV2: Inverted Residuals and Linear Bottlenecks](https://arxiv.org/abs/1801.04381) by Mark Sandler, Andrew Howard, Menglong Zhu, Andrey Zhmoginov, Liang-Chieh Chen.
1. **[MobileViT](model_doc/mobilevit)** (from Apple) released with the paper [MobileViT: Light-weight, General-purpose, and Mobile-friendly Vision Transformer](https://arxiv.org/abs/2110.02178) by Sachin Mehta and Mohammad Rastegari.
1. **[MPNet](model_doc/mpnet)** (from Microsoft Research) released with the paper [MPNet: Masked and Permuted Pre-training for Language Understanding](https://arxiv.org/abs/2004.09297) by Kaitao Song, Xu Tan, Tao Qin, Jianfeng Lu, Tie-Yan Liu.
1. **[MT5](model_doc/mt5)** (from Google AI) released with the paper [mT5: A massively multilingual pre-trained text-to-text transformer](https://arxiv.org/abs/2010.11934) by Linting Xue, Noah Constant, Adam Roberts, Mihir Kale, Rami Al-Rfou, Aditya Siddhant, Aditya Barua, Colin Raffel.
1. **[MVP](model_doc/mvp)** (from RUC AI Box) released with the paper [MVP: Multi-task Supervised Pre-training for Natural Language Generation](https://arxiv.org/abs/2206.12131) by Tianyi Tang, Junyi Li, Wayne Xin Zhao and Ji-Rong Wen.
1. **[NAT](model_doc/nat)** (from SHI Labs) released with the paper [Neighborhood Attention Transformer](https://arxiv.org/abs/2204.07143) by Ali Hassani, Steven Walton, Jiachen Li, Shen Li, and Humphrey Shi.
1. **[Nezha](model_doc/nezha)** (from Huawei Noahโs Ark Lab) released with the paper [NEZHA: Neural Contextualized Representation for Chinese Language Understanding](https://arxiv.org/abs/1909.00204) by Junqiu Wei, Xiaozhe Ren, Xiaoguang Li, Wenyong Huang, Yi Liao, Yasheng Wang, Jiashu Lin, Xin Jiang, Xiao Chen and Qun Liu.
1. **[NLLB](model_doc/nllb)** (from Meta) released with the paper [No Language Left Behind: Scaling Human-Centered Machine Translation](https://arxiv.org/abs/2207.04672) by the NLLB team.
1. **[Nystrรถmformer](model_doc/nystromformer)** (from the University of Wisconsin - Madison) released with the paper [Nystrรถmformer: A Nystrรถm-Based Algorithm for Approximating Self-Attention](https://arxiv.org/abs/2102.03902) by Yunyang Xiong, Zhanpeng Zeng, Rudrasis Chakraborty, Mingxing Tan, Glenn Fung, Yin Li, Vikas Singh.
1. **[OneFormer](model_doc/oneformer)** (from SHI Labs) released with the paper [OneFormer: One Transformer to Rule Universal Image Segmentation](https://arxiv.org/abs/2211.06220) by Jitesh Jain, Jiachen Li, MangTik Chiu, Ali Hassani, Nikita Orlov, Humphrey Shi.
1. **[OPT](master/model_doc/opt)** (from Meta AI) released with the paper [OPT: Open Pre-trained Transformer Language Models](https://arxiv.org/abs/2205.01068) by Susan Zhang, Stephen Roller, Naman Goyal, Mikel Artetxe, Moya Chen, Shuohui Chen et al.
1. **[OWL-ViT](model_doc/owlvit)** (from Google AI) released with the paper [Simple Open-Vocabulary Object Detection with Vision Transformers](https://arxiv.org/abs/2205.06230) by Matthias Minderer, Alexey Gritsenko, Austin Stone, Maxim Neumann, Dirk Weissenborn, Alexey Dosovitskiy, Aravindh Mahendran, Anurag Arnab, Mostafa Dehghani, Zhuoran Shen, Xiao Wang, Xiaohua Zhai, Thomas Kipf, and Neil Houlsby.
1. **[Pegasus](model_doc/pegasus)** (from Google) released with the paper [PEGASUS: Pre-training with Extracted Gap-sentences for Abstractive Summarization](https://arxiv.org/abs/1912.08777) by Jingqing Zhang, Yao Zhao, Mohammad Saleh and Peter J. Liu.
1. **[PEGASUS-X](model_doc/pegasus_x)** (from Google) released with the paper [Investigating Efficiently Extending Transformers for Long Input Summarization](https://arxiv.org/abs/2208.04347) by Jason Phang, Yao Zhao, and Peter J. Liu.
1. **[Perceiver IO](model_doc/perceiver)** (from Deepmind) released with the paper [Perceiver IO: A General Architecture for Structured Inputs & Outputs](https://arxiv.org/abs/2107.14795) by Andrew Jaegle, Sebastian Borgeaud, Jean-Baptiste Alayrac, Carl Doersch, Catalin Ionescu, David Ding, Skanda Koppula, Daniel Zoran, Andrew Brock, Evan Shelhamer, Olivier Hรฉnaff, Matthew M. Botvinick, Andrew Zisserman, Oriol Vinyals, Joรฃo Carreira.
1. **[PhoBERT](model_doc/phobert)** (from VinAI Research) released with the paper [PhoBERT: Pre-trained language models for Vietnamese](https://www.aclweb.org/anthology/2020.findings-emnlp.92/) by Dat Quoc Nguyen and Anh Tuan Nguyen.
1. **[PLBart](model_doc/plbart)** (from UCLA NLP) released with the paper [Unified Pre-training for Program Understanding and Generation](https://arxiv.org/abs/2103.06333) by Wasi Uddin Ahmad, Saikat Chakraborty, Baishakhi Ray, Kai-Wei Chang.
1. **[PoolFormer](model_doc/poolformer)** (from Sea AI Labs) released with the paper [MetaFormer is Actually What You Need for Vision](https://arxiv.org/abs/2111.11418) by Yu, Weihao and Luo, Mi and Zhou, Pan and Si, Chenyang and Zhou, Yichen and Wang, Xinchao and Feng, Jiashi and Yan, Shuicheng.
1. **[ProphetNet](model_doc/prophetnet)** (from Microsoft Research) released with the paper [ProphetNet: Predicting Future N-gram for Sequence-to-Sequence Pre-training](https://arxiv.org/abs/2001.04063) by Yu Yan, Weizhen Qi, Yeyun Gong, Dayiheng Liu, Nan Duan, Jiusheng Chen, Ruofei Zhang and Ming Zhou.
1. **[QDQBert](model_doc/qdqbert)** (from NVIDIA) released with the paper [Integer Quantization for Deep Learning Inference: Principles and Empirical Evaluation](https://arxiv.org/abs/2004.09602) by Hao Wu, Patrick Judd, Xiaojie Zhang, Mikhail Isaev and Paulius Micikevicius.
1. **[RAG](model_doc/rag)** (from Facebook) released with the paper [Retrieval-Augmented Generation for Knowledge-Intensive NLP Tasks](https://arxiv.org/abs/2005.11401) by Patrick Lewis, Ethan Perez, Aleksandara Piktus, Fabio Petroni, Vladimir Karpukhin, Naman Goyal, Heinrich Kรผttler, Mike Lewis, Wen-tau Yih, Tim Rocktรคschel, Sebastian Riedel, Douwe Kiela.
1. **[REALM](model_doc/realm.html)** (from Google Research) released with the paper [REALM: Retrieval-Augmented Language Model Pre-Training](https://arxiv.org/abs/2002.08909) by Kelvin Guu, Kenton Lee, Zora Tung, Panupong Pasupat and Ming-Wei Chang.
1. **[Reformer](model_doc/reformer)** (from Google Research) released with the paper [Reformer: The Efficient Transformer](https://arxiv.org/abs/2001.04451) by Nikita Kitaev, ลukasz Kaiser, Anselm Levskaya.
1. **[RegNet](model_doc/regnet)** (from META Platforms) released with the paper [Designing Network Design Space](https://arxiv.org/abs/2003.13678) by Ilija Radosavovic, Raj Prateek Kosaraju, Ross Girshick, Kaiming He, Piotr Dollรกr.
1. **[RemBERT](model_doc/rembert)** (from Google Research) released with the paper [Rethinking embedding coupling in pre-trained language models](https://arxiv.org/abs/2010.12821) by Hyung Won Chung, Thibault Fรฉvry, Henry Tsai, M. Johnson, Sebastian Ruder.
1. **[ResNet](model_doc/resnet)** (from Microsoft Research) released with the paper [Deep Residual Learning for Image Recognition](https://arxiv.org/abs/1512.03385) by Kaiming He, Xiangyu Zhang, Shaoqing Ren, Jian Sun.
1. **[RoBERTa](model_doc/roberta)** (from Facebook), released together with the paper [RoBERTa: A Robustly Optimized BERT Pretraining Approach](https://arxiv.org/abs/1907.11692) by Yinhan Liu, Myle Ott, Naman Goyal, Jingfei Du, Mandar Joshi, Danqi Chen, Omer Levy, Mike Lewis, Luke Zettlemoyer, Veselin Stoyanov.
1. **[RoBERTa-PreLayerNorm](model_doc/roberta-prelayernorm)** (from Facebook) released with the paper [fairseq: A Fast, Extensible Toolkit for Sequence Modeling](https://arxiv.org/abs/1904.01038) by Myle Ott, Sergey Edunov, Alexei Baevski, Angela Fan, Sam Gross, Nathan Ng, David Grangier, Michael Auli.
1. **[RoCBert](model_doc/roc_bert)** (from WeChatAI) released with the paper [RoCBert: Robust Chinese Bert with Multimodal Contrastive Pretraining](https://aclanthology.org/2022.acl-long.65.pdf) by HuiSu, WeiweiShi, XiaoyuShen, XiaoZhou, TuoJi, JiaruiFang, JieZhou.
1. **[RoFormer](model_doc/roformer)** (from ZhuiyiTechnology), released together with the paper [RoFormer: Enhanced Transformer with Rotary Position Embedding](https://arxiv.org/abs/2104.09864) by Jianlin Su and Yu Lu and Shengfeng Pan and Bo Wen and Yunfeng Liu.
1. **[SegFormer](model_doc/segformer)** (from NVIDIA) released with the paper [SegFormer: Simple and Efficient Design for Semantic Segmentation with Transformers](https://arxiv.org/abs/2105.15203) by Enze Xie, Wenhai Wang, Zhiding Yu, Anima Anandkumar, Jose M. Alvarez, Ping Luo.
1. **[SEW](model_doc/sew)** (from ASAPP) released with the paper [Performance-Efficiency Trade-offs in Unsupervised Pre-training for Speech Recognition](https://arxiv.org/abs/2109.06870) by Felix Wu, Kwangyoun Kim, Jing Pan, Kyu Han, Kilian Q. Weinberger, Yoav Artzi.
1. **[SEW-D](model_doc/sew_d)** (from ASAPP) released with the paper [Performance-Efficiency Trade-offs in Unsupervised Pre-training for Speech Recognition](https://arxiv.org/abs/2109.06870) by Felix Wu, Kwangyoun Kim, Jing Pan, Kyu Han, Kilian Q. Weinberger, Yoav Artzi.
1. **[SpeechT5](model_doc/speecht5)** (from Microsoft Research) released with the paper [SpeechT5: Unified-Modal Encoder-Decoder Pre-Training for Spoken Language Processing](https://arxiv.org/abs/2110.07205) by Junyi Ao, Rui Wang, Long Zhou, Chengyi Wang, Shuo Ren, Yu Wu, Shujie Liu, Tom Ko, Qing Li, Yu Zhang, Zhihua Wei, Yao Qian, Jinyu Li, Furu Wei.
1. **[SpeechToTextTransformer](model_doc/speech_to_text)** (from Facebook), released together with the paper [fairseq S2T: Fast Speech-to-Text Modeling with fairseq](https://arxiv.org/abs/2010.05171) by Changhan Wang, Yun Tang, Xutai Ma, Anne Wu, Dmytro Okhonko, Juan Pino.
1. **[SpeechToTextTransformer2](model_doc/speech_to_text_2)** (from Facebook), released together with the paper [Large-Scale Self- and Semi-Supervised Learning for Speech Translation](https://arxiv.org/abs/2104.06678) by Changhan Wang, Anne Wu, Juan Pino, Alexei Baevski, Michael Auli, Alexis Conneau.
1. **[Splinter](model_doc/splinter)** (from Tel Aviv University), released together with the paper [Few-Shot Question Answering by Pretraining Span Selection](https://arxiv.org/abs/2101.00438) by Ori Ram, Yuval Kirstain, Jonathan Berant, Amir Globerson, Omer Levy.
1. **[SqueezeBERT](model_doc/squeezebert)** (from Berkeley) released with the paper [SqueezeBERT: What can computer vision teach NLP about efficient neural networks?](https://arxiv.org/abs/2006.11316) by Forrest N. Iandola, Albert E. Shaw, Ravi Krishna, and Kurt W. Keutzer.
1. **[Swin Transformer](model_doc/swin)** (from Microsoft) released with the paper [Swin Transformer: Hierarchical Vision Transformer using Shifted Windows](https://arxiv.org/abs/2103.14030) by Ze Liu, Yutong Lin, Yue Cao, Han Hu, Yixuan Wei, Zheng Zhang, Stephen Lin, Baining Guo.
1. **[Swin Transformer V2](model_doc/swinv2)** (from Microsoft) released with the paper [Swin Transformer V2: Scaling Up Capacity and Resolution](https://arxiv.org/abs/2111.09883) by Ze Liu, Han Hu, Yutong Lin, Zhuliang Yao, Zhenda Xie, Yixuan Wei, Jia Ning, Yue Cao, Zheng Zhang, Li Dong, Furu Wei, Baining Guo.
1. **[Swin2SR](model_doc/swin2sr)** (from University of Wรผrzburg) released with the paper [Swin2SR: SwinV2 Transformer for Compressed Image Super-Resolution and Restoration](https://arxiv.org/abs/2209.11345) by Marcos V. Conde, Ui-Jin Choi, Maxime Burchi, Radu Timofte.
1. **[SwitchTransformers](model_doc/switch_transformers)** (from Google) released with the paper [Switch Transformers: Scaling to Trillion Parameter Models with Simple and Efficient Sparsity](https://arxiv.org/abs/2101.03961) by William Fedus, Barret Zoph, Noam Shazeer.
1. **[T5](model_doc/t5)** (from Google AI) released with the paper [Exploring the Limits of Transfer Learning with a Unified Text-to-Text Transformer](https://arxiv.org/abs/1910.10683) by Colin Raffel and Noam Shazeer and Adam Roberts and Katherine Lee and Sharan Narang and Michael Matena and Yanqi Zhou and Wei Li and Peter J. Liu.
1. **[T5v1.1](model_doc/t5v1.1)** (from Google AI) released in the repository [google-research/text-to-text-transfer-transformer](https://github.com/google-research/text-to-text-transfer-transformer/blob/main/released_checkpoints.md#t511) by Colin Raffel and Noam Shazeer and Adam Roberts and Katherine Lee and Sharan Narang and Michael Matena and Yanqi Zhou and Wei Li and Peter J. Liu.
1. **[Table Transformer](model_doc/table-transformer)** (from Microsoft Research) released with the paper [PubTables-1M: Towards Comprehensive Table Extraction From Unstructured Documents](https://arxiv.org/abs/2110.00061) by Brandon Smock, Rohith Pesala, Robin Abraham.
1. **[TAPAS](model_doc/tapas)** (from Google AI) released with the paper [TAPAS: Weakly Supervised Table Parsing via Pre-training](https://arxiv.org/abs/2004.02349) by Jonathan Herzig, Paweล Krzysztof Nowak, Thomas Mรผller, Francesco Piccinno and Julian Martin Eisenschlos.
1. **[TAPEX](model_doc/tapex)** (from Microsoft Research) released with the paper [TAPEX: Table Pre-training via Learning a Neural SQL Executor](https://arxiv.org/abs/2107.07653) by Qian Liu, Bei Chen, Jiaqi Guo, Morteza Ziyadi, Zeqi Lin, Weizhu Chen, Jian-Guang Lou.
1. **[Time Series Transformer](model_doc/time_series_transformer)** (from HuggingFace).
1. **[TimeSformer](model_doc/timesformer)** (from Facebook) released with the paper [Is Space-Time Attention All You Need for Video Understanding?](https://arxiv.org/abs/2102.05095) by Gedas Bertasius, Heng Wang, Lorenzo Torresani.
1. **[Trajectory Transformer](model_doc/trajectory_transformers)** (from the University of California at Berkeley) released with the paper [Offline Reinforcement Learning as One Big Sequence Modeling Problem](https://arxiv.org/abs/2106.02039) by Michael Janner, Qiyang Li, Sergey Levine
1. **[Transformer-XL](model_doc/transfo-xl)** (from Google/CMU) released with the paper [Transformer-XL: Attentive Language Models Beyond a Fixed-Length Context](https://arxiv.org/abs/1901.02860) by Zihang Dai*, Zhilin Yang*, Yiming Yang, Jaime Carbonell, Quoc V. Le, Ruslan Salakhutdinov.
1. **[TrOCR](model_doc/trocr)** (from Microsoft), released together with the paper [TrOCR: Transformer-based Optical Character Recognition with Pre-trained Models](https://arxiv.org/abs/2109.10282) by Minghao Li, Tengchao Lv, Lei Cui, Yijuan Lu, Dinei Florencio, Cha Zhang, Zhoujun Li, Furu Wei.
1. **[UL2](model_doc/ul2)** (from Google Research) released with the paper [Unifying Language Learning Paradigms](https://arxiv.org/abs/2205.05131v1) by Yi Tay, Mostafa Dehghani, Vinh Q. Tran, Xavier Garcia, Dara Bahri, Tal Schuster, Huaixiu Steven Zheng, Neil Houlsby, Donald Metzler
1. **[UniSpeech](model_doc/unispeech)** (from Microsoft Research) released with the paper [UniSpeech: Unified Speech Representation Learning with Labeled and Unlabeled Data](https://arxiv.org/abs/2101.07597) by Chengyi Wang, Yu Wu, Yao Qian, Kenichi Kumatani, Shujie Liu, Furu Wei, Michael Zeng, Xuedong Huang.
1. **[UniSpeechSat](model_doc/unispeech-sat)** (from Microsoft Research) released with the paper [UNISPEECH-SAT: UNIVERSAL SPEECH REPRESENTATION LEARNING WITH SPEAKER AWARE PRE-TRAINING](https://arxiv.org/abs/2110.05752) by Sanyuan Chen, Yu Wu, Chengyi Wang, Zhengyang Chen, Zhuo Chen, Shujie Liu, Jian Wu, Yao Qian, Furu Wei, Jinyu Li, Xiangzhan Yu.
1. **[UPerNet](model_doc/upernet)** (from Peking University) released with the paper [Unified Perceptual Parsing for Scene Understanding](https://arxiv.org/abs/1807.10221) by Tete Xiao, Yingcheng Liu, Bolei Zhou, Yuning Jiang, Jian Sun.
1. **[VAN](model_doc/van)** (from Tsinghua University and Nankai University) released with the paper [Visual Attention Network](https://arxiv.org/abs/2202.09741) by Meng-Hao Guo, Cheng-Ze Lu, Zheng-Ning Liu, Ming-Ming Cheng, Shi-Min Hu.
1. **[VideoMAE](model_doc/videomae)** (from Multimedia Computing Group, Nanjing University) released with the paper [VideoMAE: Masked Autoencoders are Data-Efficient Learners for Self-Supervised Video Pre-Training](https://arxiv.org/abs/2203.12602) by Zhan Tong, Yibing Song, Jue Wang, Limin Wang.
1. **[ViLT](model_doc/vilt)** (from NAVER AI Lab/Kakao Enterprise/Kakao Brain) released with the paper [ViLT: Vision-and-Language Transformer Without Convolution or Region Supervision](https://arxiv.org/abs/2102.03334) by Wonjae Kim, Bokyung Son, Ildoo Kim.
1. **[Vision Transformer (ViT)](model_doc/vit)** (from Google AI) released with the paper [An Image is Worth 16x16 Words: Transformers for Image Recognition at Scale](https://arxiv.org/abs/2010.11929) by Alexey Dosovitskiy, Lucas Beyer, Alexander Kolesnikov, Dirk Weissenborn, Xiaohua Zhai, Thomas Unterthiner, Mostafa Dehghani, Matthias Minderer, Georg Heigold, Sylvain Gelly, Jakob Uszkoreit, Neil Houlsby.
1. **[VisualBERT](model_doc/visual_bert)** (from UCLA NLP) released with the paper [VisualBERT: A Simple and Performant Baseline for Vision and Language](https://arxiv.org/pdf/1908.03557) by Liunian Harold Li, Mark Yatskar, Da Yin, Cho-Jui Hsieh, Kai-Wei Chang.
1. **[ViT Hybrid](model_doc/vit_hybrid)** (from Google AI) released with the paper [An Image is Worth 16x16 Words: Transformers for Image Recognition at Scale](https://arxiv.org/abs/2010.11929) by Alexey Dosovitskiy, Lucas Beyer, Alexander Kolesnikov, Dirk Weissenborn, Xiaohua Zhai, Thomas Unterthiner, Mostafa Dehghani, Matthias Minderer, Georg Heigold, Sylvain Gelly, Jakob Uszkoreit, Neil Houlsby.
1. **[ViTMAE](model_doc/vit_mae)** (from Meta AI) released with the paper [Masked Autoencoders Are Scalable Vision Learners](https://arxiv.org/abs/2111.06377) by Kaiming He, Xinlei Chen, Saining Xie, Yanghao Li, Piotr Dollรกr, Ross Girshick.
1. **[ViTMSN](model_doc/vit_msn)** (from Meta AI) released with the paper [Masked Siamese Networks for Label-Efficient Learning](https://arxiv.org/abs/2204.07141) by Mahmoud Assran, Mathilde Caron, Ishan Misra, Piotr Bojanowski, Florian Bordes, Pascal Vincent, Armand Joulin, Michael Rabbat, Nicolas Ballas.
1. **[Wav2Vec2](model_doc/wav2vec2)** (from Facebook AI) released with the paper [wav2vec 2.0: A Framework for Self-Supervised Learning of Speech Representations](https://arxiv.org/abs/2006.11477) by Alexei Baevski, Henry Zhou, Abdelrahman Mohamed, Michael Auli.
1. **[Wav2Vec2-Conformer](model_doc/wav2vec2-conformer)** (from Facebook AI) released with the paper [FAIRSEQ S2T: Fast Speech-to-Text Modeling with FAIRSEQ](https://arxiv.org/abs/2010.05171) by Changhan Wang, Yun Tang, Xutai Ma, Anne Wu, Sravya Popuri, Dmytro Okhonko, Juan Pino.
1. **[Wav2Vec2Phoneme](model_doc/wav2vec2_phoneme)** (from Facebook AI) released with the paper [Simple and Effective Zero-shot Cross-lingual Phoneme Recognition](https://arxiv.org/abs/2109.11680) by Qiantong Xu, Alexei Baevski, Michael Auli.
1. **[WavLM](model_doc/wavlm)** (from Microsoft Research) released with the paper [WavLM: Large-Scale Self-Supervised Pre-Training for Full Stack Speech Processing](https://arxiv.org/abs/2110.13900) by Sanyuan Chen, Chengyi Wang, Zhengyang Chen, Yu Wu, Shujie Liu, Zhuo Chen, Jinyu Li, Naoyuki Kanda, Takuya Yoshioka, Xiong Xiao, Jian Wu, Long Zhou, Shuo Ren, Yanmin Qian, Yao Qian, Jian Wu, Michael Zeng, Furu Wei.
1. **[Whisper](model_doc/whisper)** (from OpenAI) released with the paper [Robust Speech Recognition via Large-Scale Weak Supervision](https://cdn.openai.com/papers/whisper.pdf) by Alec Radford, Jong Wook Kim, Tao Xu, Greg Brockman, Christine McLeavey, Ilya Sutskever.
1. **[X-CLIP](model_doc/xclip)** (from Microsoft Research) released with the paper [Expanding Language-Image Pretrained Models for General Video Recognition](https://arxiv.org/abs/2208.02816) by Bolin Ni, Houwen Peng, Minghao Chen, Songyang Zhang, Gaofeng Meng, Jianlong Fu, Shiming Xiang, Haibin Ling.
1. **[XGLM](model_doc/xglm)** (From Facebook AI) released with the paper [Few-shot Learning with Multilingual Language Models](https://arxiv.org/abs/2112.10668) by Xi Victoria Lin, Todor Mihaylov, Mikel Artetxe, Tianlu Wang, Shuohui Chen, Daniel Simig, Myle Ott, Naman Goyal, Shruti Bhosale, Jingfei Du, Ramakanth Pasunuru, Sam Shleifer, Punit Singh Koura, Vishrav Chaudhary, Brian O'Horo, Jeff Wang, Luke Zettlemoyer, Zornitsa Kozareva, Mona Diab, Veselin Stoyanov, Xian Li.
1. **[XLM](model_doc/xlm)** (from Facebook) released together with the paper [Cross-lingual Language Model Pretraining](https://arxiv.org/abs/1901.07291) by Guillaume Lample and Alexis Conneau.
1. **[XLM-ProphetNet](model_doc/xlm-prophetnet)** (from Microsoft Research) released with the paper [ProphetNet: Predicting Future N-gram for Sequence-to-Sequence Pre-training](https://arxiv.org/abs/2001.04063) by Yu Yan, Weizhen Qi, Yeyun Gong, Dayiheng Liu, Nan Duan, Jiusheng Chen, Ruofei Zhang and Ming Zhou.
1. **[XLM-RoBERTa](model_doc/xlm-roberta)** (from Facebook AI), released together with the paper [Unsupervised Cross-lingual Representation Learning at Scale](https://arxiv.org/abs/1911.02116) by Alexis Conneau*, Kartikay Khandelwal*, Naman Goyal, Vishrav Chaudhary, Guillaume Wenzek, Francisco Guzmรกn, Edouard Grave, Myle Ott, Luke Zettlemoyer and Veselin Stoyanov.
1. **[XLM-RoBERTa-XL](model_doc/xlm-roberta-xl)** (from Facebook AI), released together with the paper [Larger-Scale Transformers for Multilingual Masked Language Modeling](https://arxiv.org/abs/2105.00572) by Naman Goyal, Jingfei Du, Myle Ott, Giri Anantharaman, Alexis Conneau.
1. **[XLNet](model_doc/xlnet)** (from Google/CMU) released with the paper [โXLNet: Generalized Autoregressive Pretraining for Language Understanding](https://arxiv.org/abs/1906.08237) by Zhilin Yang*, Zihang Dai*, Yiming Yang, Jaime Carbonell, Ruslan Salakhutdinov, Quoc V. Le.
1. **[XLS-R](model_doc/xls_r)** (from Facebook AI) released with the paper [XLS-R: Self-supervised Cross-lingual Speech Representation Learning at Scale](https://arxiv.org/abs/2111.09296) by Arun Babu, Changhan Wang, Andros Tjandra, Kushal Lakhotia, Qiantong Xu, Naman Goyal, Kritika Singh, Patrick von Platen, Yatharth Saraf, Juan Pino, Alexei Baevski, Alexis Conneau, Michael Auli.
1. **[XLSR-Wav2Vec2](model_doc/xlsr_wav2vec2)** (from Facebook AI) released with the paper [Unsupervised Cross-Lingual Representation Learning For Speech Recognition](https://arxiv.org/abs/2006.13979) by Alexis Conneau, Alexei Baevski, Ronan Collobert, Abdelrahman Mohamed, Michael Auli.
1. **[YOLOS](model_doc/yolos)** (from Huazhong University of Science & Technology) released with the paper [You Only Look at One Sequence: Rethinking Transformer in Vision through Object Detection](https://arxiv.org/abs/2106.00666) by Yuxin Fang, Bencheng Liao, Xinggang Wang, Jiemin Fang, Jiyang Qi, Rui Wu, Jianwei Niu, Wenyu Liu.
1. **[YOSO](model_doc/yoso)** (from the University of Wisconsin - Madison) released with the paper [You Only Sample (Almost) Once: Linear Cost Self-Attention Via Bernoulli Sampling](https://arxiv.org/abs/2111.09714) by Zhanpeng Zeng, Yunyang Xiong, Sathya N. Ravi, Shailesh Acharya, Glenn Fung, Vikas Singh.
### Frameworks compatibles
Le tableau ci-dessous reprรฉsente la prise en charge actuelle dans la bibliothรจque pour chacun de ces modรจles, qu'ils aient ou non un tokenizer Python (appelรฉ "slow"). Un tokenizer rapide ("fast") soutenu par la bibliothรจque ๐ค Tokenizers, qu'ils aient un support en Jax (via Flax), PyTorch, et/ou TensorFlow.
<!--This table is updated automatically from the auto modules with _make fix-copies_. Do not update manually!-->
| Modรจle | Tokenizer slow | Tokenizer fast | PyTorch support | TensorFlow support | Flax Support |
|:-----------------------------:|:--------------:|:--------------:|:---------------:|:------------------:|:------------:|
| ALBERT | โ
| โ
| โ
| โ
| โ
|
| AltCLIP | โ | โ | โ
| โ | โ |
| Audio Spectrogram Transformer | โ | โ | โ
| โ | โ |
| BART | โ
| โ
| โ
| โ
| โ
|
| BEiT | โ | โ | โ
| โ | โ
|
| BERT | โ
| โ
| โ
| โ
| โ
|
| Bert Generation | โ
| โ | โ
| โ | โ |
| BigBird | โ
| โ
| โ
| โ | โ
|
| BigBird-Pegasus | โ | โ | โ
| โ | โ |
| BioGpt | โ
| โ | โ
| โ | โ |
| BiT | โ | โ | โ
| โ | โ |
| Blenderbot | โ
| โ
| โ
| โ
| โ
|
| BlenderbotSmall | โ
| โ
| โ
| โ
| โ
|
| BLIP | โ | โ | โ
| โ | โ |
| BLOOM | โ | โ
| โ
| โ | โ |
| BridgeTower | โ | โ | โ
| โ | โ |
| CamemBERT | โ
| โ
| โ
| โ
| โ |
| CANINE | โ
| โ | โ
| โ | โ |
| Chinese-CLIP | โ | โ | โ
| โ | โ |
| CLIP | โ
| โ
| โ
| โ
| โ
|
| CLIPSeg | โ | โ | โ
| โ | โ |
| CodeGen | โ
| โ
| โ
| โ | โ |
| Conditional DETR | โ | โ | โ
| โ | โ |
| ConvBERT | โ
| โ
| โ
| โ
| โ |
| ConvNeXT | โ | โ | โ
| โ
| โ |
| CTRL | โ
| โ | โ
| โ
| โ |
| CvT | โ | โ | โ
| โ
| โ |
| Data2VecAudio | โ | โ | โ
| โ | โ |
| Data2VecText | โ | โ | โ
| โ | โ |
| Data2VecVision | โ | โ | โ
| โ
| โ |
| DeBERTa | โ
| โ
| โ
| โ
| โ |
| DeBERTa-v2 | โ
| โ
| โ
| โ
| โ |
| Decision Transformer | โ | โ | โ
| โ | โ |
| Deformable DETR | โ | โ | โ
| โ | โ |
| DeiT | โ | โ | โ
| โ
| โ |
| DETA | โ | โ | โ
| โ | โ |
| DETR | โ | โ | โ
| โ | โ |
| DiNAT | โ | โ | โ
| โ | โ |
| DistilBERT | โ
| โ
| โ
| โ
| โ
|
| DonutSwin | โ | โ | โ
| โ | โ |
| DPR | โ
| โ
| โ
| โ
| โ |
| DPT | โ | โ | โ
| โ | โ |
| EfficientFormer | โ | โ | โ
| โ | โ |
| ELECTRA | โ
| โ
| โ
| โ
| โ
|
| Encoder decoder | โ | โ | โ
| โ
| โ
|
| ERNIE | โ | โ | โ
| โ | โ |
| ESM | โ
| โ | โ
| โ
| โ |
| FairSeq Machine-Translation | โ
| โ | โ
| โ | โ |
| FlauBERT | โ
| โ | โ
| โ
| โ |
| FLAVA | โ | โ | โ
| โ | โ |
| FNet | โ
| โ
| โ
| โ | โ |
| Funnel Transformer | โ
| โ
| โ
| โ
| โ |
| GIT | โ | โ | โ
| โ | โ |
| GLPN | โ | โ | โ
| โ | โ |
| GPT Neo | โ | โ | โ
| โ | โ
|
| GPT NeoX | โ | โ
| โ
| โ | โ |
| GPT NeoX Japanese | โ
| โ | โ
| โ | โ |
| GPT-J | โ | โ | โ
| โ
| โ
|
| GPT-Sw3 | โ
| โ
| โ
| โ
| โ
|
| Graphormer | โ | โ | โ
| โ | โ |
| GroupViT | โ | โ | โ
| โ
| โ |
| Hubert | โ | โ | โ
| โ
| โ |
| I-BERT | โ | โ | โ
| โ | โ |
| ImageGPT | โ | โ | โ
| โ | โ |
| Jukebox | โ
| โ | โ
| โ | โ |
| LayoutLM | โ
| โ
| โ
| โ
| โ |
| LayoutLMv2 | โ
| โ
| โ
| โ | โ |
| LayoutLMv3 | โ
| โ
| โ
| โ
| โ |
| LED | โ
| โ
| โ
| โ
| โ |
| LeViT | โ | โ | โ
| โ | โ |
| LiLT | โ | โ | โ
| โ | โ |
| Longformer | โ
| โ
| โ
| โ
| โ |
| LongT5 | โ | โ | โ
| โ | โ
|
| LUKE | โ
| โ | โ
| โ | โ |
| LXMERT | โ
| โ
| โ
| โ
| โ |
| M-CTC-T | โ | โ | โ
| โ | โ |
| M2M100 | โ
| โ | โ
| โ | โ |
| Marian | โ
| โ | โ
| โ
| โ
|
| MarkupLM | โ
| โ
| โ
| โ | โ |
| Mask2Former | โ | โ | โ
| โ | โ |
| MaskFormer | โ | โ | โ
| โ | โ |
| MaskFormerSwin | โ | โ | โ | โ | โ |
| mBART | โ
| โ
| โ
| โ
| โ
|
| Megatron-BERT | โ | โ | โ
| โ | โ |
| MobileBERT | โ
| โ
| โ
| โ
| โ |
| MobileNetV1 | โ | โ | โ
| โ | โ |
| MobileNetV2 | โ | โ | โ
| โ | โ |
| MobileViT | โ | โ | โ
| โ
| โ |
| MPNet | โ
| โ
| โ
| โ
| โ |
| MT5 | โ
| โ
| โ
| โ
| โ
|
| MVP | โ
| โ
| โ
| โ | โ |
| NAT | โ | โ | โ
| โ | โ |
| Nezha | โ | โ | โ
| โ | โ |
| Nystrรถmformer | โ | โ | โ
| โ | โ |
| OneFormer | โ | โ | โ
| โ | โ |
| OpenAI GPT | โ
| โ
| โ
| โ
| โ |
| OpenAI GPT-2 | โ
| โ
| โ
| โ
| โ
|
| OPT | โ | โ | โ
| โ
| โ
|
| OWL-ViT | โ | โ | โ
| โ | โ |
| Pegasus | โ
| โ
| โ
| โ
| โ
|
| PEGASUS-X | โ | โ | โ
| โ | โ |
| Perceiver | โ
| โ | โ
| โ | โ |
| PLBart | โ
| โ | โ
| โ | โ |
| PoolFormer | โ | โ | โ
| โ | โ |
| ProphetNet | โ
| โ | โ
| โ | โ |
| QDQBert | โ | โ | โ
| โ | โ |
| RAG | โ
| โ | โ
| โ
| โ |
| REALM | โ
| โ
| โ
| โ | โ |
| Reformer | โ
| โ
| โ
| โ | โ |
| RegNet | โ | โ | โ
| โ
| โ
|
| RemBERT | โ
| โ
| โ
| โ
| โ |
| ResNet | โ | โ | โ
| โ
| โ |
| RetriBERT | โ
| โ
| โ
| โ | โ |
| RoBERTa | โ
| โ
| โ
| โ
| โ
|
| RoBERTa-PreLayerNorm | โ | โ | โ
| โ
| โ
|
| RoCBert | โ
| โ | โ
| โ | โ |
| RoFormer | โ
| โ
| โ
| โ
| โ
|
| SegFormer | โ | โ | โ
| โ
| โ |
| SEW | โ | โ | โ
| โ | โ |
| SEW-D | โ | โ | โ
| โ | โ |
| Speech Encoder decoder | โ | โ | โ
| โ | โ
|
| Speech2Text | โ
| โ | โ
| โ
| โ |
| Speech2Text2 | โ
| โ | โ | โ | โ |
| SpeechT5 | โ
| โ | โ
| โ | โ |
| Splinter | โ
| โ
| โ
| โ | โ |
| SqueezeBERT | โ
| โ
| โ
| โ | โ |
| Swin Transformer | โ | โ | โ
| โ
| โ |
| Swin Transformer V2 | โ | โ | โ
| โ | โ |
| Swin2SR | โ | โ | โ
| โ | โ |
| SwitchTransformers | โ | โ | โ
| โ | โ |
| T5 | โ
| โ
| โ
| โ
| โ
|
| Table Transformer | โ | โ | โ
| โ | โ |
| TAPAS | โ
| โ | โ
| โ
| โ |
| Time Series Transformer | โ | โ | โ
| โ | โ |
| TimeSformer | โ | โ | โ
| โ | โ |
| Trajectory Transformer | โ | โ | โ
| โ | โ |
| Transformer-XL | โ
| โ | โ
| โ
| โ |
| TrOCR | โ | โ | โ
| โ | โ |
| UniSpeech | โ | โ | โ
| โ | โ |
| UniSpeechSat | โ | โ | โ
| โ | โ |
| UPerNet | โ | โ | โ
| โ | โ |
| VAN | โ | โ | โ
| โ | โ |
| VideoMAE | โ | โ | โ
| โ | โ |
| ViLT | โ | โ | โ
| โ | โ |
| Vision Encoder decoder | โ | โ | โ
| โ
| โ
|
| VisionTextDualEncoder | โ | โ | โ
| โ | โ
|
| VisualBERT | โ | โ | โ
| โ | โ |
| ViT | โ | โ | โ
| โ
| โ
|
| ViT Hybrid | โ | โ | โ
| โ | โ |
| ViTMAE | โ | โ | โ
| โ
| โ |
| ViTMSN | โ | โ | โ
| โ | โ |
| Wav2Vec2 | โ
| โ | โ
| โ
| โ
|
| Wav2Vec2-Conformer | โ | โ | โ
| โ | โ |
| WavLM | โ | โ | โ
| โ | โ |
| Whisper | โ
| โ | โ
| โ
| โ |
| X-CLIP | โ | โ | โ
| โ | โ |
| XGLM | โ
| โ
| โ
| โ
| โ
|
| XLM | โ
| โ | โ
| โ
| โ |
| XLM-ProphetNet | โ
| โ | โ
| โ | โ |
| XLM-RoBERTa | โ
| โ
| โ
| โ
| โ
|
| XLM-RoBERTa-XL | โ | โ | โ
| โ | โ |
| XLNet | โ
| โ
| โ
| โ
| โ |
| YOLOS | โ | โ | โ
| โ | โ |
| YOSO | โ | โ | โ
| โ | โ |
<!-- End table--> | 0 |
hf_public_repos/transformers/docs/source | hf_public_repos/transformers/docs/source/fr/_toctree.yml | - sections:
- local: index
title: ๐ค Transformers
- local: quicktour
title: Visite rapide
- local: in_translation
title: Installation
title: Dรฉmarrer
- sections:
- local: in_translation
title: Pipelines pour l'infรฉrence
- local: in_translation
title: Chargement d'instances prรฉ-entraรฎnรฉes avec une AutoClass
- local: in_translation
title: Prรฉparation des donnรฉes
- local: in_translation
title: Fine-tune un modรจle prรฉ-entraรฎnรฉ
- local: in_translation
title: Entraรฎnement distribuรฉ avec ๐ค Accelerate
- local: in_translation
title: Partager un modรจle
title: Tutoriels
- sections:
- sections:
- local: in_translation
title: Crรฉer votre architecture
- local: in_translation
title: Partager vos modรจles
- local: in_translation
title: Entraรฎnement avec un script
- local: in_translation
title: Entraรฎnement avec Amazon SageMaker
- local: in_translation
title: Convertir depuis des checkpoints Tensorflow
- local: in_translation
title: Exporter vers ONNX
- local: in_translation
title: Exporter vers TorchScript
- local: in_translation
title: Aide au dรฉpannage
title: Usage gรฉnรฉral
- sections:
- local: in_translation
title: Utiliser les tokenizers de ๐ค Tokenizers
- local: in_translation
title: Infรฉrence avec les modรจles multilingues
- local: in_translation
title: Stratรฉgies de gรฉnรฉration de texte
- sections:
- isExpanded: false
local: in_translation
title: Classification de texte
- local: in_translation
title: Classification de token
- local: in_translation
title: Systรจme de question-rรฉponse
- local: in_translation
title: Modรฉlisation causale du langage
- local: in_translation
title: Modรฉlisation du langage avec masque
- local: in_translation
title: Traduction
- local: in_translation
title: Gรฉnรฉration de rรฉsumรฉ
- local: in_translation
title: Question ร choix multiple
title: Guides des tรขches
title: Traitement automatique des langues
- sections:
- local: in_translation
title: Classification audio
- local: in_translation
title: Reconnaissance automatique de la parole
title: Audio
- sections:
- local: in_translation
title: Classification d'images
- local: in_translation
title: Segmentation sรฉmantique
- local: in_translation
title: Classification de vidรฉos
- local: in_translation
title: Dรฉtection d'objets
title: Vision par ordinateur
- sections:
- local: in_translation
title: Performance et extensibilitรฉ
- sections:
- local: in_translation
title: Comment contribuer ร transformers?
- local: in_translation
title: Comment ajouter un modรจle ร ๐ค Transformers?
- local: in_translation
title: Comment convertir un modรจle ๐ค Transformers vers TensorFlow?
- local: in_translation
title: Comment ajouter un pipeline ร ๐ค Transformers?
- local: in_translation
title: Tester
- local: in_translation
title: Vรฉrification pour une Pull Request
title: Contribuer
- local: in_translation
title: ๐ค Transformers Notebooks
- local: in_translation
title: Ressources communautaires
- local: in_translation
title: Benchmarks
- local: in_translation
title: Migration ร partir de versions prรฉcรฉdentes
title: Guides d'utilisation
- sections:
- local: in_translation
title: Philosophie
- local: in_translation
title: Glossaire
- local: in_translation
title: Qu'est ce ๐ค Transformers peut faire ?
- local: in_translation
title: Quelles tรขches ๐ค Transformers peut rรฉsoudre ?
- local: in_translation
title: Rรฉsumรฉ des modรจles
- local: in_translation
title: Rรฉsumรฉ des tokenizers
- local: in_translation
title: Remplissage et troncature
- local: in_translation
title: BERTology
- local: in_translation
title: Perplexitรฉ des modรจles ร longueur fixe
- local: in_translation
title: Pipelines pour infรฉrence avec des serveurs web
title: Guides conceptuels
- sections:
- isExpanded: false
sections:
- local: in_translation
title: Classes principales
- local: in_translation
title: Modรจles textuels
- local: in_translation
title: Modรจles visuels
- local: in_translation
title: Modรจles audio
- local: in_translation
title: Modรจles multimodal
- local: in_translation
title: Modรจles d'apprentissage par renforcement
- local: in_translation
title: Modรจles de sรฉries temporelles
- local: in_translation
title: Graph models
title: Modรจles
- sections:
- local: in_translation
title: Utilitaires internes
title: API
| 0 |
hf_public_repos/transformers/docs/source | hf_public_repos/transformers/docs/source/fr/in_translation.md | <!--โ ๏ธ Note that this file is in Markdown but contain specific syntax for our doc-builder (similar to MDX) that may not be
rendered properly in your Markdown viewer.
-->
# Traduction en cours. | 0 |
hf_public_repos/transformers/docs/source | hf_public_repos/transformers/docs/source/fr/_config.py | # docstyle-ignore
INSTALL_CONTENT = """
# Installation de Transformers
! pip install transformers datasets
# Pour installer ร partir du code source au lieu de la derniรจre version, commentez la commande ci-dessus et dรฉcommentez la suivante.
# ! pip install git+https://github.com/huggingface/transformers.git
"""
notebook_first_cells = [{"type": "code", "content": INSTALL_CONTENT}]
black_avoid_patterns = {
"{processor_class}": "FakeProcessorClass",
"{model_class}": "FakeModelClass",
"{object_class}": "FakeObjectClass",
}
| 0 |
hf_public_repos/transformers/docs/source | hf_public_repos/transformers/docs/source/de/autoclass_tutorial.md | <!--Copyright 2022 The HuggingFace Team. All rights reserved.
Licensed under the Apache License, Version 2.0 (the "License"); you may not use this file except in compliance with
the License. You may obtain a copy of the License at
http://www.apache.org/licenses/LICENSE-2.0
Unless required by applicable law or agreed to in writing, software distributed under the License is distributed on
an "AS IS" BASIS, WITHOUT WARRANTIES OR CONDITIONS OF ANY KIND, either express or implied. See the License for the
specific language governing permissions and limitations under the License.
โ ๏ธ Note that this file is in Markdown but contain specific syntax for our doc-builder (similar to MDX) that may not be
rendered properly in your Markdown viewer.
-->
# Vortrainierte Instanzen mit einer AutoClass laden
Bei so vielen verschiedenen Transformator-Architekturen kann es eine Herausforderung sein, eine fรผr Ihren Checkpoint zu erstellen. Als Teil der ๐ค Transformers Kernphilosophie, die Bibliothek leicht, einfach und flexibel nutzbar zu machen, leitet eine `AutoClass` automatisch die richtige Architektur aus einem gegebenen Checkpoint ab und lรคdt sie. Mit der Methode `from_pretrained()` kann man schnell ein vortrainiertes Modell fรผr eine beliebige Architektur laden, so dass man keine Zeit und Ressourcen aufwenden muss, um ein Modell von Grund auf zu trainieren. Die Erstellung dieser Art von Checkpoint-agnostischem Code bedeutet, dass Ihr Code, wenn er fรผr einen Checkpoint funktioniert, auch mit einem anderen Checkpoint funktionieren wird - solange er fรผr eine รคhnliche Aufgabe trainiert wurde - selbst wenn die Architektur unterschiedlich ist.
<Tip>
Denken Sie daran, dass sich die Architektur auf das Skelett des Modells bezieht und die Checkpoints die Gewichte fรผr eine bestimmte Architektur sind. Zum Beispiel ist [BERT](https://huggingface.co/bert-base-uncased) eine Architektur, wรคhrend `bert-base-uncased` ein Checkpoint ist. Modell ist ein allgemeiner Begriff, der entweder Architektur oder Prรผfpunkt bedeuten kann.
</Tip>
In dieser Anleitung lernen Sie, wie man:
* Einen vortrainierten Tokenizer lรคdt.
* Einen vortrainierten Merkmalsextraktor lรคdt.
* Einen vortrainierten Prozessor lรคdt.
* Ein vortrainiertes Modell lรคdt.
## AutoTokenizer
Nahezu jede NLP-Aufgabe beginnt mit einem Tokenizer. Ein Tokenizer wandelt Ihre Eingabe in ein Format um, das vom Modell verarbeitet werden kann.
Laden Sie einen Tokenizer mit [`AutoTokenizer.from_pretrained`]:
```py
>>> from transformers import AutoTokenizer
>>> tokenizer = AutoTokenizer.from_pretrained("bert-base-uncased")
```
Dann tokenisieren Sie Ihre Eingabe wie unten gezeigt:
```py
>>> sequence = "In a hole in the ground there lived a hobbit."
>>> print(tokenizer(sequence))
{'input_ids': [101, 1999, 1037, 4920, 1999, 1996, 2598, 2045, 2973, 1037, 7570, 10322, 4183, 1012, 102],
'token_type_ids': [0, 0, 0, 0, 0, 0, 0, 0, 0, 0, 0, 0, 0, 0, 0],
'attention_mask': [1, 1, 1, 1, 1, 1, 1, 1, 1, 1, 1, 1, 1, 1, 1]}
```
## AutoFeatureExtractor
Fรผr Audio- und Bildverarbeitungsaufgaben verarbeitet ein Merkmalsextraktor das Audiosignal oder Bild in das richtige Eingabeformat.
Laden Sie einen Merkmalsextraktor mit [`AutoFeatureExtractor.from_pretrained`]:
```py
>>> from transformers import AutoFeatureExtractor
>>> feature_extractor = AutoFeatureExtractor.from_pretrained(
... "ehcalabres/wav2vec2-lg-xlsr-en-speech-emotion-recognition"
... )
```
## AutoProcessor
Multimodale Aufgaben erfordern einen Prozessor, der zwei Arten von Vorverarbeitungswerkzeugen kombiniert. Das Modell [LayoutLMV2](model_doc/layoutlmv2) beispielsweise benรถtigt einen Feature-Extraktor fรผr Bilder und einen Tokenizer fรผr Text; ein Prozessor kombiniert beide.
Laden Sie einen Prozessor mit [`AutoProcessor.from_pretrained`]:
```py
>>> from transformers import AutoProcessor
>>> processor = AutoProcessor.from_pretrained("microsoft/layoutlmv2-base-uncased")
```
## AutoModel
<frameworkcontent>
<pt>
Mit den `AutoModelFor`-Klassen kรถnnen Sie schlieรlich ein vortrainiertes Modell fรผr eine bestimmte Aufgabe laden (siehe [hier](model_doc/auto) fรผr eine vollstรคndige Liste der verfรผgbaren Aufgaben). Laden Sie zum Beispiel ein Modell fรผr die Sequenzklassifikation mit [`AutoModelForSequenceClassification.from_pretrained`]:
```py
>>> from transformers import AutoModelForSequenceClassification
>>> model = AutoModelForSequenceClassification.from_pretrained("distilbert-base-uncased")
```
Sie kรถnnen denselben Prรผfpunkt problemlos wiederverwenden, um eine Architektur fรผr eine andere Aufgabe zu laden:
```py
>>> from transformers import AutoModelForTokenClassification
>>> model = AutoModelForTokenClassification.from_pretrained("distilbert-base-uncased")
```
<Tip warning={true}>
Fรผr PyTorch-Modelle verwendet die Methode `from_pretrained()` `torch.load()`, die intern `pickle` verwendet und als unsicher bekannt ist. Generell sollte man niemals ein Modell laden, das aus einer nicht vertrauenswรผrdigen Quelle stammen kรถnnte, oder das manipuliert worden sein kรถnnte. Dieses Sicherheitsrisiko wird fรผr รถffentliche Modelle, die auf dem Hugging Face Hub gehostet werden, teilweise gemildert, da diese bei jeder รbertragung [auf Malware](https://huggingface.co/docs/hub/security-malware) gescannt werden. Siehe die [Hub-Dokumentation](https://huggingface.co/docs/hub/security) fรผr Best Practices wie [signierte Commit-Verifizierung](https://huggingface.co/docs/hub/security-gpg#signing-commits-with-gpg) mit GPG.
TensorFlow- und Flax-Checkpoints sind nicht betroffen und kรถnnen in PyTorch-Architekturen mit den Kwargs `from_tf` und `from_flax` fรผr die Methode `from_pretrained` geladen werden, um dieses Problem zu umgehen.
</Tip>
Im Allgemeinen empfehlen wir die Verwendung der Klasse "AutoTokenizer" und der Klasse "AutoModelFor", um trainierte Instanzen von Modellen zu laden. Dadurch wird sichergestellt, dass Sie jedes Mal die richtige Architektur laden. Im nรคchsten [Tutorial] (Vorverarbeitung) erfahren Sie, wie Sie Ihren neu geladenen Tokenizer, Feature Extractor und Prozessor verwenden, um einen Datensatz fรผr die Feinabstimmung vorzuverarbeiten.
</pt>
<tf>
Mit den Klassen `TFAutoModelFor` schlieรlich kรถnnen Sie ein vortrainiertes Modell fรผr eine bestimmte Aufgabe laden (siehe [hier](model_doc/auto) fรผr eine vollstรคndige Liste der verfรผgbaren Aufgaben). Laden Sie zum Beispiel ein Modell fรผr die Sequenzklassifikation mit [`TFAutoModelForSequenceClassification.from_pretrained`]:
```py
>>> from transformers import TFAutoModelForSequenceClassification
>>> model = TFAutoModelForSequenceClassification.from_pretrained("distilbert-base-uncased")
```
Sie kรถnnen denselben Prรผfpunkt problemlos wiederverwenden, um eine Architektur fรผr eine andere Aufgabe zu laden:
```py
>>> from transformers import TFAutoModelForTokenClassification
>>> model = TFAutoModelForTokenClassification.from_pretrained("distilbert-base-uncased")
```
Im Allgemeinen empfehlen wir, die Klasse "AutoTokenizer" und die Klasse "TFAutoModelFor" zu verwenden, um vortrainierte Instanzen von Modellen zu laden. Dadurch wird sichergestellt, dass Sie jedes Mal die richtige Architektur laden. Im nรคchsten [Tutorial] (Vorverarbeitung) erfahren Sie, wie Sie Ihren neu geladenen Tokenizer, Feature Extractor und Prozessor verwenden, um einen Datensatz fรผr die Feinabstimmung vorzuverarbeiten.
</tf>
</frameworkcontent>
| 0 |
hf_public_repos/transformers/docs/source | hf_public_repos/transformers/docs/source/de/quicktour.md | <!--Copyright 2022 The HuggingFace Team. All rights reserved.
Licensed under the Apache License, Version 2.0 (the "License"); you may not use this file except in compliance with
the License. You may obtain a copy of the License at
http://www.apache.org/licenses/LICENSE-2.0
Unless required by applicable law or agreed to in writing, software distributed under the License is distributed on
an "AS IS" BASIS, WITHOUT WARRANTIES OR CONDITIONS OF ANY KIND, either express or implied. See the License for the
specific language governing permissions and limitations under the License.
โ ๏ธ Note that this file is in Markdown but contain specific syntax for our doc-builder (similar to MDX) that may not be
rendered properly in your Markdown viewer.
-->
# Schnellstart
[[open-in-colab]]
Mit ๐ค Transformers kรถnnen Sie sofort loslegen! Verwenden Sie die [`pipeline`] fรผr schnelle Inferenz und laden Sie schnell ein vortrainiertes Modell und einen Tokenizer mit einer [AutoClass](./model_doc/auto), um Ihre Text-, Bild- oder Audioaufgabe zu lรถsen.
<Tip>
Alle in der Dokumentation vorgestellten Codebeispiele haben oben links einen Umschalter fรผr PyTorch und TensorFlow. Wenn
nicht, wird erwartet, dass der Code fรผr beide Backends ohne รnderungen funktioniert.
</Tip>
## Pipeline
[`pipeline`] ist der einfachste Weg, ein vortrainiertes Modell fรผr eine bestimmte Aufgabe zu verwenden.
<Youtube id="tiZFewofSLM"/>
Die [`pipeline`] unterstรผtzt viele gรคngige Aufgaben:
**Text**:
* Stimmungsanalyse: Klassifizierung der Polaritรคt eines gegebenen Textes.
* Textgenerierung (auf Englisch): Generierung von Text aus einer gegebenen Eingabe.
* Name-Entity-Recognition (NER): Kennzeichnung jedes Worts mit der Entitรคt, die es reprรคsentiert (Person, Datum, Ort usw.).
* Beantwortung von Fragen: Extrahieren der Antwort aus dem Kontext, wenn ein gewisser Kontext und eine Frage gegeben sind.
* Fill-mask: Ausfรผllen von Lรผcken in einem Text mit maskierten Wรถrtern.
* Zusammenfassung: Erstellung einer Zusammenfassung einer langen Text- oder Dokumentensequenz.
* รbersetzung: รbersetzen eines Textes in eine andere Sprache.
* Merkmalsextraktion: Erstellen einer Tensordarstellung des Textes.
**Bild**:
* Bildklassifizierung: Klassifizierung eines Bildes.
* Bildsegmentierung: Klassifizierung jedes Pixels in einem Bild.
* Objekterkennung: Erkennen von Objekten innerhalb eines Bildes.
**Audio**:
* Audioklassifizierung: Zuweisung eines Labels zu einem bestimmten Audiosegment.
* Automatische Spracherkennung (ASR): Transkription von Audiodaten in Text.
<Tip>
Fรผr mehr Details รผber die [`pipeline`] und assoziierte Aufgaben, schauen Sie in die Dokumentation [hier](./main_classes/pipelines).
</Tip>
### Verwendung der Pipeline
Im folgenden Beispiel werden Sie die [`pipeline`] fรผr die Stimmungsanalyse verwenden.
Installieren Sie die folgenden Abhรคngigkeiten, falls Sie dies nicht bereits getan haben:
<frameworkcontent>
<pt>
```bash
pip install torch
```
</pt>
<tf>
```bash
pip install tensorflow
```
</tf>
</frameworkcontent>
Importieren sie die [`pipeline`] und spezifizieren sie die Aufgabe, welche sie lรถsen mรถchten:
```py
>>> from transformers import pipeline
>>> classifier = pipeline("sentiment-analysis")
```
Die Pipeline lรคdt ein standardmรครiges [vortrainiertes Modell] (https://huggingface.co/distilbert-base-uncased-finetuned-sst-2-english) und einen Tokenizer fรผr die Stimmungs-Analyse herunter und speichert sie. Jetzt kรถnnen Sie den "Klassifikator" auf Ihren Zieltext anwenden:
```py
>>> classifier("We are very happy to show you the ๐ค Transformers library.")
[{'label': 'POSITIVE', 'score': 0.9998}]
```
For more than one sentence, pass a list of sentences to the [`pipeline`] which returns a list of dictionaries:
```py
>>> results = classifier(["We are very happy to show you the ๐ค Transformers library.", "We hope you don't hate it."])
>>> for result in results:
... print(f"label: {result['label']}, with score: {round(result['score'], 4)}")
label: POSITIVE, with score: 0.9998
label: NEGATIVE, with score: 0.5309
```
Die [`pipeline`] kann auch รผber einen ganzen Datensatz iterieren. Starten wir mit der Installation der [๐ค Datasets](https://huggingface.co/docs/datasets/) Bibliothek:
```bash
pip install datasets
```
Erstellen wir eine [`pipeline`] mit der Aufgabe die wir lรถsen und dem Modell welches wir nutzen mรถchten.
```py
>>> import torch
>>> from transformers import pipeline
>>> speech_recognizer = pipeline("automatic-speech-recognition", model="facebook/wav2vec2-base-960h")
```
Als nรคchstes laden wir den Datensatz (siehe ๐ค Datasets [Quick Start](https://huggingface.co/docs/datasets/quickstart.html) fรผr mehr Details) welches wir nutzen mรถchten. Zum Beispiel laden wir den [MInDS-14](https://huggingface.co/datasets/PolyAI/minds14) Datensatz:
```py
>>> from datasets import load_dataset, Audio
>>> dataset = load_dataset("PolyAI/minds14", name="en-US", split="train") # doctest: +IGNORE_RESULT
```
Wir mรผssen sicherstellen, dass die Abtastrate des Datensatzes der Abtastrate entspricht, mit der `facebook/wav2vec2-base-960h` trainiert wurde.
```py
>>> dataset = dataset.cast_column("audio", Audio(sampling_rate=speech_recognizer.feature_extractor.sampling_rate))
```
Audiodateien werden automatisch geladen und neu abgetastet, wenn die Spalte "audio" aufgerufen wird.
Extrahieren wir die rohen Wellenform-Arrays der ersten 4 Beispiele und รผbergeben wir sie als Liste an die Pipeline:
```py
>>> result = speech_recognizer(dataset[:4]["audio"])
>>> print([d["text"] for d in result])
['I WOULD LIKE TO SET UP A JOINT ACCOUNT WITH MY PARTNER HOW DO I PROCEED WITH DOING THAT', "FODING HOW I'D SET UP A JOIN TO HET WITH MY WIFE AND WHERE THE AP MIGHT BE", "I I'D LIKE TOY SET UP A JOINT ACCOUNT WITH MY PARTNER I'M NOT SEEING THE OPTION TO DO IT ON THE AP SO I CALLED IN TO GET SOME HELP CAN I JUST DO IT OVER THE PHONE WITH YOU AND GIVE YOU THE INFORMATION OR SHOULD I DO IT IN THE AP AND I'M MISSING SOMETHING UQUETTE HAD PREFERRED TO JUST DO IT OVER THE PHONE OF POSSIBLE THINGS", 'HOW DO I THURN A JOIN A COUNT']
```
Bei einem grรถรeren Datensatz mit vielen Eingaben (wie bei Sprache oder Bildverarbeitung) sollten Sie einen Generator anstelle einer Liste รผbergeben, der alle Eingaben in den Speicher lรคdt. Weitere Informationen finden Sie in der [Pipeline-Dokumentation](./main_classes/pipelines).
### Ein anderes Modell und einen anderen Tokenizer in der Pipeline verwenden
Die [`pipeline`] kann jedes Modell aus dem [Model Hub] (https://huggingface.co/models) verwenden, wodurch es einfach ist, die [`pipeline`] fรผr andere Anwendungsfรคlle anzupassen. Wenn Sie beispielsweise ein Modell wรผnschen, das franzรถsischen Text verarbeiten kann, verwenden Sie die Tags im Model Hub, um nach einem geeigneten Modell zu filtern. Das oberste gefilterte Ergebnis liefert ein mehrsprachiges [BERT-Modell](https://huggingface.co/nlptown/bert-base-multilingual-uncased-sentiment), das auf die Stimmungsanalyse abgestimmt ist. Groรartig, verwenden wir dieses Modell!
```py
>>> model_name = "nlptown/bert-base-multilingual-uncased-sentiment"
```
<frameworkcontent>
<pt>
Use the [`AutoModelForSequenceClassification`] and [`AutoTokenizer`] to load the pretrained model and it's associated tokenizer (more on an `AutoClass` below):
```py
>>> from transformers import AutoTokenizer, AutoModelForSequenceClassification
>>> model = AutoModelForSequenceClassification.from_pretrained(model_name)
>>> tokenizer = AutoTokenizer.from_pretrained(model_name)
```
</pt>
<tf>
Use the [`TFAutoModelForSequenceClassification`] and [`AutoTokenizer`] to load the pretrained model and it's associated tokenizer (more on an `TFAutoClass` below):
```py
>>> from transformers import AutoTokenizer, TFAutoModelForSequenceClassification
>>> model = TFAutoModelForSequenceClassification.from_pretrained(model_name)
>>> tokenizer = AutoTokenizer.from_pretrained(model_name)
```
</tf>
</frameworkcontent>
Dann kรถnnen Sie das Modell und den Tokenizer in der [`pipeline`] angeben und den `Klassifikator` auf Ihren Zieltext anwenden:
```py
>>> classifier = pipeline("sentiment-analysis", model=model, tokenizer=tokenizer)
>>> classifier("Nous sommes trรจs heureux de vous prรฉsenter la bibliothรจque ๐ค Transformers.")
[{'label': '5 stars', 'score': 0.7273}]
```
Wenn Sie kein Modell fรผr Ihren Anwendungsfall finden kรถnnen, mรผssen Sie ein vortrainiertes Modell auf Ihren Daten feinabstimmen. Schauen Sie sich unser [Feinabstimmungs-Tutorial](./training) an, um zu erfahren, wie das geht. Und schlieรlich, nachdem Sie Ihr trainiertes Modell verfeinert haben, sollten Sie es mit der Community im Model Hub teilen (siehe Tutorial [hier](./model_sharing)), um NLP fรผr alle zu demokratisieren! ๐ค
## AutoClass
<Youtube id="AhChOFRegn4"/>
Unter der Haube arbeiten die Klassen [`AutoModelForSequenceClassification`] und [`AutoTokenizer`] zusammen, um die [`pipeline`] zu betreiben. Eine [`AutoClass`](./model_doc/auto) ist eine Abkรผrzung, die automatisch die Architektur eines trainierten Modells aus dessen Namen oder Pfad abruft. Sie mรผssen nur die passende `AutoClass` fรผr Ihre Aufgabe und den zugehรถrigen Tokenizer mit [`AutoTokenizer`] auswรคhlen.
Kehren wir zu unserem Beispiel zurรผck und sehen wir uns an, wie Sie die `AutoClass` verwenden kรถnnen, um die Ergebnisse der [`pipeline`] zu replizieren.
### AutoTokenizer
Ein Tokenizer ist fรผr die Vorverarbeitung von Text in ein fรผr das Modell verstรคndliches Format zustรคndig. Zunรคchst zerlegt der Tokenisierer den Text in Wรถrter, die *Token* genannt werden. Es gibt mehrere Regeln fรผr den Tokenisierungsprozess, z. B. wie und auf welcher Ebene ein Wort aufgespalten wird (weitere Informationen รผber Tokenisierung [hier](./tokenizer_summary)). Das Wichtigste ist jedoch, dass Sie den Tokenizer mit demselben Modellnamen instanziieren mรผssen, um sicherzustellen, dass Sie dieselben Tokenisierungsregeln verwenden, mit denen ein Modell zuvor trainiert wurde.
Laden sie einen Tokenizer mit [`AutoTokenizer`]:
```py
>>> from transformers import AutoTokenizer
>>> model_name = "nlptown/bert-base-multilingual-uncased-sentiment"
>>> tokenizer = AutoTokenizer.from_pretrained(model_name)
```
Anschlieรend wandelt der Tokenizer die Token in Zahlen um, um einen Tensor als Eingabe fรผr das Modell zu konstruieren. Dieser wird als *Vokabular* des Modells bezeichnet.
รbergeben Sie Ihren Text an den Tokenizer:
```py
>>> encoding = tokenizer("We are very happy to show you the ๐ค Transformers library.")
>>> print(encoding)
{'input_ids': [101, 11312, 10320, 12495, 19308, 10114, 11391, 10855, 10103, 100, 58263, 13299, 119, 102],
'token_type_ids': [0, 0, 0, 0, 0, 0, 0, 0, 0, 0, 0, 0, 0, 0],
'attention_mask': [1, 1, 1, 1, 1, 1, 1, 1, 1, 1, 1, 1, 1, 1]}
```
Der Tokenizer gibt ein Wรถrterbuch zurรผck, das Folgendes enthรคlt:
* [input_ids](./glossary#input-ids): numerische Reprรคsentationen Ihrer Token.
* [atttention_mask](.glossary#attention-mask): gibt an, welche Token beachtet werden sollen.
Genau wie die [`pipeline`] akzeptiert der Tokenizer eine Liste von Eingaben. Darรผber hinaus kann der Tokenizer den Text auch auffรผllen und kรผrzen, um einen Stapel mit einheitlicher Lรคnge zurรผckzugeben:
<frameworkcontent>
<pt>
```py
>>> pt_batch = tokenizer(
... ["We are very happy to show you the ๐ค Transformers library.", "We hope you don't hate it."],
... padding=True,
... truncation=True,
... max_length=512,
... return_tensors="pt",
... )
```
</pt>
<tf>
```py
>>> tf_batch = tokenizer(
... ["We are very happy to show you the ๐ค Transformers library.", "We hope you don't hate it."],
... padding=True,
... truncation=True,
... max_length=512,
... return_tensors="tf",
... )
```
</tf>
</frameworkcontent>
Lesen Sie das Tutorial [preprocessing](./preprocessing) fรผr weitere Details zur Tokenisierung.
### AutoModel
<frameworkcontent>
<pt>
๐ค Transformers bietet eine einfache und einheitliche Mรถglichkeit, vortrainierte Instanzen zu laden. Das bedeutet, dass Sie ein [`AutoModel`] laden kรถnnen, wie Sie einen [`AutoTokenizer`] laden wรผrden. Der einzige Unterschied ist die Auswahl des richtigen [`AutoModel`] fรผr die Aufgabe. Da Sie eine Text- oder Sequenzklassifizierung vornehmen, laden Sie [`AutoModelForSequenceClassification`]:
```py
>>> from transformers import AutoModelForSequenceClassification
>>> model_name = "nlptown/bert-base-multilingual-uncased-sentiment"
>>> pt_model = AutoModelForSequenceClassification.from_pretrained(model_name)
```
<Tip>
In der [Aufgabenzusammenfassung](./task_summary) steht, welche [AutoModel]-Klasse fรผr welche Aufgabe zu verwenden ist.
</Tip>
Jetzt kรถnnen Sie Ihren vorverarbeiteten Stapel von Eingaben direkt an das Modell รผbergeben. Sie mรผssen nur das Wรถrterbuch entpacken, indem Sie `**` hinzufรผgen:
```py
>>> pt_outputs = pt_model(**pt_batch)
```
Das Modell gibt die endgรผltigen Aktivierungen in dem Attribut "logits" aus. Wenden Sie die Softmax-Funktion auf die "logits" an, um die Wahrscheinlichkeiten zu erhalten:
```py
>>> from torch import nn
>>> pt_predictions = nn.functional.softmax(pt_outputs.logits, dim=-1)
>>> print(pt_predictions)
tensor([[0.0021, 0.0018, 0.0115, 0.2121, 0.7725],
[0.2084, 0.1826, 0.1969, 0.1755, 0.2365]], grad_fn=<SoftmaxBackward0>)
```
</pt>
<tf>
๐ค Transformers bietet eine einfache und einheitliche Methode zum Laden von vortrainierten Instanzen. Das bedeutet, dass Sie ein [`TFAutoModel`] genauso laden kรถnnen, wie Sie einen [`AutoTokenizer`] laden wรผrden. Der einzige Unterschied ist die Auswahl des richtigen [`TFAutoModel`] fรผr die Aufgabe. Da Sie Text - oder Sequenz - Klassifizierung machen, laden Sie [`TFAutoModelForSequenceClassification`]:
```py
>>> from transformers import TFAutoModelForSequenceClassification
>>> model_name = "nlptown/bert-base-multilingual-uncased-sentiment"
>>> tf_model = TFAutoModelForSequenceClassification.from_pretrained(model_name)
```
<Tip>
In der [Aufgabenzusammenfassung](./task_summary) steht, welche [AutoModel]-Klasse fรผr welche Aufgabe zu verwenden ist.
</Tip>
Jetzt kรถnnen Sie Ihren vorverarbeiteten Stapel von Eingaben direkt an das Modell รผbergeben, indem Sie die Wรถrterbuchschlรผssel direkt an die Tensoren รผbergeben:
```py
>>> tf_outputs = tf_model(tf_batch)
```
Das Modell gibt die endgรผltigen Aktivierungen in dem Attribut "logits" aus. Wenden Sie die Softmax-Funktion auf die "logits" an, um die Wahrscheinlichkeiten zu erhalten:
```py
>>> import tensorflow as tf
>>> tf_predictions = tf.nn.softmax(tf_outputs.logits, axis=-1)
>>> tf_predictions # doctest: +IGNORE_RESULT
```
</tf>
</frameworkcontent>
<Tip>
Alle ๐ค Transformers-Modelle (PyTorch oder TensorFlow) geben die Tensoren *vor* der endgรผltigen Aktivierungsfunktion
Funktion (wie Softmax) aus, da die endgรผltige Aktivierungsfunktion oft mit dem Verlusten verschmolzen ist.
</Tip>
Modelle sind ein standardmรครiges [`torch.nn.Module`](https://pytorch.org/docs/stable/nn.html#torch.nn.Module) oder ein [`tf.keras.Model`](https://www.tensorflow.org/api_docs/python/tf/keras/Model), sodass Sie sie in Ihrer รผblichen Trainingsschleife verwenden kรถnnen. Um jedoch die Dinge einfacher zu machen, bietet ๐ค Transformers eine [`Trainer`]-Klasse fรผr PyTorch, die Funktionalitรคt fรผr verteiltes Training, gemischte Prรคzision und mehr bietet. Fรผr TensorFlow kรถnnen Sie die Methode `fit` aus [Keras](https://keras.io/) verwenden. Siehe das [training tutorial](./training) fรผr weitere Details.
<Tip>
Transformers-Modellausgaben sind spezielle Datenklassen, so dass ihre Attribute in einer IDE automatisch vervollstรคndigt werden.
Die Modellausgรคnge verhalten sich auch wie ein Tupel oder ein Wรถrterbuch (z.B. kรถnnen Sie mit einem Integer, einem Slice oder einem String indexieren), wobei die Attribute, die "None" sind, ignoriert werden.
</Tip>
### Modell speichern
<frameworkcontent>
<pt>
Sobald Ihr Modell feinabgestimmt ist, kรถnnen Sie es mit seinem Tokenizer speichern, indem Sie [`PreTrainedModel.save_pretrained`] verwenden:
```py
>>> pt_save_directory = "./pt_save_pretrained"
>>> tokenizer.save_pretrained(pt_save_directory) # doctest: +IGNORE_RESULT
>>> pt_model.save_pretrained(pt_save_directory)
```
Wenn Sie bereit sind, das Modell erneut zu verwenden, laden Sie es mit [`PreTrainedModel.from_pretrained`]:
```py
>>> pt_model = AutoModelForSequenceClassification.from_pretrained("./pt_save_pretrained")
```
</pt>
<tf>
Sobald Ihr Modell feinabgestimmt ist, kรถnnen Sie es mit seinem Tokenizer unter Verwendung von [`TFPreTrainedModel.save_pretrained`] speichern:
```py
>>> tf_save_directory = "./tf_save_pretrained"
>>> tokenizer.save_pretrained(tf_save_directory) # doctest: +IGNORE_RESULT
>>> tf_model.save_pretrained(tf_save_directory)
```
Wenn Sie bereit sind, das Modell wieder zu verwenden, laden Sie es mit [`TFPreTrainedModel.from_pretrained`]:
```py
>>> tf_model = TFAutoModelForSequenceClassification.from_pretrained("./tf_save_pretrained")
```
</tf>
</frameworkcontent>
Ein besonders cooles ๐ค Transformers-Feature ist die Mรถglichkeit, ein Modell zu speichern und es entweder als PyTorch- oder TensorFlow-Modell wieder zu laden. Der Parameter "from_pt" oder "from_tf" kann das Modell von einem Framework in das andere konvertieren:
<frameworkcontent>
<pt>
```py
>>> from transformers import AutoModel
>>> tokenizer = AutoTokenizer.from_pretrained(tf_save_directory)
>>> pt_model = AutoModelForSequenceClassification.from_pretrained(tf_save_directory, from_tf=True)
```
</pt>
<tf>
```py
>>> from transformers import TFAutoModel
>>> tokenizer = AutoTokenizer.from_pretrained(pt_save_directory)
>>> tf_model = TFAutoModelForSequenceClassification.from_pretrained(pt_save_directory, from_pt=True)
```
</tf>
</frameworkcontent>
## Custom model builds
Sie kรถnnen die Konfigurationsklasse des Modells รคndern, um zu bestimmen, wie ein Modell aufgebaut ist. Die Konfiguration legt die Attribute eines Modells fest, z. B. die Anzahl der verborgenen Schichten oder der Aufmerksamkeitskรถpfe. Wenn Sie ein Modell aus einer benutzerdefinierten Konfigurationsklasse initialisieren, beginnen Sie bei Null. Die Modellattribute werden zufรคllig initialisiert, und Sie mรผssen das Modell trainieren, bevor Sie es verwenden kรถnnen, um aussagekrรคftige Ergebnisse zu erhalten.
Beginnen Sie mit dem Import von [`AutoConfig`] und laden Sie dann das trainierte Modell, das Sie รคndern mรถchten. Innerhalb von [`AutoConfig.from_pretrained`] kรถnnen Sie das Attribut angeben, das Sie รคndern mรถchten, z. B. die Anzahl der Aufmerksamkeitskรถpfe:
```py
>>> from transformers import AutoConfig
>>> my_config = AutoConfig.from_pretrained("distilbert-base-uncased", n_heads=12)
```
<frameworkcontent>
<pt>
Create a model from your custom configuration with [`AutoModel.from_config`]:
```py
>>> from transformers import AutoModel
>>> my_model = AutoModel.from_config(my_config)
```
</pt>
<tf>
Create a model from your custom configuration with [`TFAutoModel.from_config`]:
```py
>>> from transformers import TFAutoModel
>>> my_model = TFAutoModel.from_config(my_config)
```
</tf>
</frameworkcontent>
Weitere Informationen zur Erstellung von benutzerdefinierten Konfigurationen finden Sie in der Anleitung [Erstellen einer benutzerdefinierten Architektur](./create_a_model).
## Wie geht es weiter?
Nachdem Sie nun die ๐ค Transformers-Kurztour abgeschlossen haben, schauen Sie sich unsere Anleitungen an und erfahren Sie, wie Sie spezifischere Dinge tun kรถnnen, wie das Schreiben eines benutzerdefinierten Modells, die Feinabstimmung eines Modells fรผr eine Aufgabe und wie man ein Modell mit einem Skript trainiert. Wenn Sie mehr รผber die Kernkonzepte von ๐ค Transformers erfahren mรถchten, nehmen Sie sich eine Tasse Kaffee und werfen Sie einen Blick auf unsere konzeptionellen Leitfรคden!
| 0 |
hf_public_repos/transformers/docs/source | hf_public_repos/transformers/docs/source/de/index.md | <!--Copyright 2020 The HuggingFace Team. All rights reserved.
Licensed under the Apache License, Version 2.0 (the "License"); you may not use this file except in compliance with
the License. You may obtain a copy of the License at
http://www.apache.org/licenses/LICENSE-2.0
Unless required by applicable law or agreed to in writing, software distributed under the License is distributed on
an "AS IS" BASIS, WITHOUT WARRANTIES OR CONDITIONS OF ANY KIND, either express or implied. See the License for the
specific language governing permissions and limitations under the License.
โ ๏ธ Note that this file is in Markdown but contain specific syntax for our doc-builder (similar to MDX) that may not be
rendered properly in your Markdown viewer.
-->
# ๐ค Transformers
Maschinelles Lernen auf dem neuesten Stand der Technik fรผr PyTorch, TensorFlow und JAX.
๐ค Transformers bietet APIs zum einfachen Herunterladen und Trainieren von vortrainierten Modellen auf dem neuesten Stand der Technik. Die Verwendung von vortrainierten Modellen kann Rechenkosten sparen und den CO2-Fuรabdruck reduzieren und Zeit sparen, die fรผr das Training eines Modells von Grund auf benรถtigt wird. Die Modelle kรถnnen fรผr verschiedene Modalitรคten verwendet werden, wie z. B.:
* ๐ Text: Textklassifizierung, Informationsextrahierung, Beantwortung von Fragen, Zusammenfassung, รbersetzung und Texterstellung in รผber 100 Sprachen.
* ๐ผ๏ธ Bilder: Bildklassifizierung, Objekterkennung und Segmentierung.
* ๐ฃ๏ธ Audio: Spracherkennung und Audioklassifizierung.
* ๐ Multimodal: Beantwortung von Tabellenfragen, optische Zeichenerkennung, Informationsextraktion aus gescannten Dokumenten, Videoklassifizierung und Beantwortung visueller Fragen.
Unsere Bibliothek unterstรผtzt die nahtlose Integration von drei der beliebtesten Deep-Learning-Bibliotheken: [PyTorch](https://pytorch.org/), [TensorFlow](https://www.tensorflow.org/) und [JAX](https://jax.readthedocs.io/en/latest/). Trainieren Sie Ihr Modell in drei Codezeilen in einem Framework und laden Sie es zur Inferenz mit einem anderen.
Jede ๐ค Transformers-Architektur ist in einem eigenstรคndigen Python-Modul definiert, so dass sie leicht fรผr Forschung und Experimente angepasst werden kann.
## Wenn Sie auf der Suche nach individueller Unterstรผtzung durch das Hugging Face-Team sind
<a target="_blank" href="https://huggingface.co/support">
<img alt="HuggingFace Expert Acceleration Program" src="https://cdn-media.huggingface.co/marketing/transformers/new-support-improved.png" style="width: 100%; max-width: 600px; border: 1px solid #eee; border-radius: 4px; box-shadow: 0 1px 2px 0 rgba(0, 0, 0, 0.05);">
</a>
## Inhalt
Die Dokumentation ist in fรผnf Teile gegliedert:
- **GET STARTED** enthรคlt eine kurze Tour und Installationsanweisungen, um mit ๐ค Transformers loszulegen.
- **TUTORIALS** sind ein hervorragender Ausgangspunkt, wenn Sie neu in unserer Bibliothek sind. Dieser Abschnitt hilft Ihnen, die grundlegenden Fรคhigkeiten zu erlangen, die Sie benรถtigen, um mit ๐ค Transformers zu arbeiten.
- **HOW-TO GUIDES** zeigen Ihnen, wie Sie ein bestimmtes Ziel erreichen kรถnnen, z. B. die Feinabstimmung eines vortrainierten Modells fรผr die Sprachmodellierung oder die Erstellung eines benutzerdefinierten Modellkopfs.
- **KONZEPTUELLE ANLEITUNGEN** bietet weitere Diskussionen und Erklรคrungen zu den zugrunde liegenden Konzepten und Ideen hinter Modellen, Aufgaben und der Designphilosophie von ๐ค Transformers.
- **API** beschreibt jede Klasse und Funktion, gruppiert in:
- **MAIN CLASSES** fรผr die Hauptklassen, die die wichtigsten APIs der Bibliothek darstellen.
- MODELLE** fรผr die Klassen und Funktionen, die zu jedem in der Bibliothek implementierten Modell gehรถren.
- **INTERNAL HELPERS** fรผr die Klassen und Funktionen, die wir intern verwenden.
Die Bibliothek enthรคlt derzeit JAX-, PyTorch- und TensorFlow-Implementierungen, vortrainierte Modellgewichte, Nutzungsskripte und Konvertierungsprogramme fรผr die folgenden Modelle.
### Unterstรผtze Modelle
<!--This list is updated automatically from the README with _make fix-copies_. Do not update manually! -->
1. **[ALBERT](model_doc/albert)** (from Google Research and the Toyota Technological Institute at Chicago) released with the paper [ALBERT: A Lite BERT for Self-supervised Learning of Language Representations](https://arxiv.org/abs/1909.11942), by Zhenzhong Lan, Mingda Chen, Sebastian Goodman, Kevin Gimpel, Piyush Sharma, Radu Soricut.
1. **[ALIGN](model_doc/align)** (from Google Research) released with the paper [Scaling Up Visual and Vision-Language Representation Learning With Noisy Text Supervision](https://arxiv.org/abs/2102.05918) by Chao Jia, Yinfei Yang, Ye Xia, Yi-Ting Chen, Zarana Parekh, Hieu Pham, Quoc V. Le, Yunhsuan Sung, Zhen Li, Tom Duerig.
1. **[BART](model_doc/bart)** (from Facebook) released with the paper [BART: Denoising Sequence-to-Sequence Pre-training for Natural Language Generation, Translation, and Comprehension](https://arxiv.org/abs/1910.13461) by Mike Lewis, Yinhan Liu, Naman Goyal, Marjan Ghazvininejad, Abdelrahman Mohamed, Omer Levy, Ves Stoyanov and Luke Zettlemoyer.
1. **[BARThez](model_doc/barthez)** (from รcole polytechnique) released with the paper [BARThez: a Skilled Pretrained French Sequence-to-Sequence Model](https://arxiv.org/abs/2010.12321) by Moussa Kamal Eddine, Antoine J.-P. Tixier, Michalis Vazirgiannis.
1. **[BARTpho](model_doc/bartpho)** (from VinAI Research) released with the paper [BARTpho: Pre-trained Sequence-to-Sequence Models for Vietnamese](https://arxiv.org/abs/2109.09701) by Nguyen Luong Tran, Duong Minh Le and Dat Quoc Nguyen.
1. **[BEiT](model_doc/beit)** (from Microsoft) released with the paper [BEiT: BERT Pre-Training of Image Transformers](https://arxiv.org/abs/2106.08254) by Hangbo Bao, Li Dong, Furu Wei.
1. **[BERT](model_doc/bert)** (from Google) released with the paper [BERT: Pre-training of Deep Bidirectional Transformers for Language Understanding](https://arxiv.org/abs/1810.04805) by Jacob Devlin, Ming-Wei Chang, Kenton Lee and Kristina Toutanova.
1. **[BERT For Sequence Generation](model_doc/bert-generation)** (from Google) released with the paper [Leveraging Pre-trained Checkpoints for Sequence Generation Tasks](https://arxiv.org/abs/1907.12461) by Sascha Rothe, Shashi Narayan, Aliaksei Severyn.
1. **[BERTweet](model_doc/bertweet)** (from VinAI Research) released with the paper [BERTweet: A pre-trained language model for English Tweets](https://aclanthology.org/2020.emnlp-demos.2/) by Dat Quoc Nguyen, Thanh Vu and Anh Tuan Nguyen.
1. **[BigBird-Pegasus](model_doc/bigbird_pegasus)** (from Google Research) released with the paper [Big Bird: Transformers for Longer Sequences](https://arxiv.org/abs/2007.14062) by Manzil Zaheer, Guru Guruganesh, Avinava Dubey, Joshua Ainslie, Chris Alberti, Santiago Ontanon, Philip Pham, Anirudh Ravula, Qifan Wang, Li Yang, Amr Ahmed.
1. **[BigBird-RoBERTa](model_doc/big_bird)** (from Google Research) released with the paper [Big Bird: Transformers for Longer Sequences](https://arxiv.org/abs/2007.14062) by Manzil Zaheer, Guru Guruganesh, Avinava Dubey, Joshua Ainslie, Chris Alberti, Santiago Ontanon, Philip Pham, Anirudh Ravula, Qifan Wang, Li Yang, Amr Ahmed.
1. **[Blenderbot](model_doc/blenderbot)** (from Facebook) released with the paper [Recipes for building an open-domain chatbot](https://arxiv.org/abs/2004.13637) by Stephen Roller, Emily Dinan, Naman Goyal, Da Ju, Mary Williamson, Yinhan Liu, Jing Xu, Myle Ott, Kurt Shuster, Eric M. Smith, Y-Lan Boureau, Jason Weston.
1. **[BlenderbotSmall](model_doc/blenderbot-small)** (from Facebook) released with the paper [Recipes for building an open-domain chatbot](https://arxiv.org/abs/2004.13637) by Stephen Roller, Emily Dinan, Naman Goyal, Da Ju, Mary Williamson, Yinhan Liu, Jing Xu, Myle Ott, Kurt Shuster, Eric M. Smith, Y-Lan Boureau, Jason Weston.
1. **[BLOOM](model_doc/bloom)** (from BigScience workshop) released by the [BigScience Workshop](https://bigscience.huggingface.co/).
1. **[BORT](model_doc/bort)** (from Alexa) released with the paper [Optimal Subarchitecture Extraction For BERT](https://arxiv.org/abs/2010.10499) by Adrian de Wynter and Daniel J. Perry.
1. **[ByT5](model_doc/byt5)** (from Google Research) released with the paper [ByT5: Towards a token-free future with pre-trained byte-to-byte models](https://arxiv.org/abs/2105.13626) by Linting Xue, Aditya Barua, Noah Constant, Rami Al-Rfou, Sharan Narang, Mihir Kale, Adam Roberts, Colin Raffel.
1. **[CamemBERT](model_doc/camembert)** (from Inria/Facebook/Sorbonne) released with the paper [CamemBERT: a Tasty French Language Model](https://arxiv.org/abs/1911.03894) by Louis Martin*, Benjamin Muller*, Pedro Javier Ortiz Suรกrez*, Yoann Dupont, Laurent Romary, รric Villemonte de la Clergerie, Djamรฉ Seddah and Benoรฎt Sagot.
1. **[CANINE](model_doc/canine)** (from Google Research) released with the paper [CANINE: Pre-training an Efficient Tokenization-Free Encoder for Language Representation](https://arxiv.org/abs/2103.06874) by Jonathan H. Clark, Dan Garrette, Iulia Turc, John Wieting.
1. **[CLIP](model_doc/clip)** (from OpenAI) released with the paper [Learning Transferable Visual Models From Natural Language Supervision](https://arxiv.org/abs/2103.00020) by Alec Radford, Jong Wook Kim, Chris Hallacy, Aditya Ramesh, Gabriel Goh, Sandhini Agarwal, Girish Sastry, Amanda Askell, Pamela Mishkin, Jack Clark, Gretchen Krueger, Ilya Sutskever.
1. **[CodeGen](model_doc/codegen)** (from Salesforce) released with the paper [A Conversational Paradigm for Program Synthesis](https://arxiv.org/abs/2203.13474) by Erik Nijkamp, Bo Pang, Hiroaki Hayashi, Lifu Tu, Huan Wang, Yingbo Zhou, Silvio Savarese, Caiming Xiong.
1. **[ConvBERT](model_doc/convbert)** (from YituTech) released with the paper [ConvBERT: Improving BERT with Span-based Dynamic Convolution](https://arxiv.org/abs/2008.02496) by Zihang Jiang, Weihao Yu, Daquan Zhou, Yunpeng Chen, Jiashi Feng, Shuicheng Yan.
1. **[ConvNeXT](model_doc/convnext)** (from Facebook AI) released with the paper [A ConvNet for the 2020s](https://arxiv.org/abs/2201.03545) by Zhuang Liu, Hanzi Mao, Chao-Yuan Wu, Christoph Feichtenhofer, Trevor Darrell, Saining Xie.
1. **[ConvNeXTV2](model_doc/convnextv2)** (from Facebook AI) released with the paper [ConvNeXt V2: Co-designing and Scaling ConvNets with Masked Autoencoders](https://arxiv.org/abs/2301.00808) by Sanghyun Woo, Shoubhik Debnath, Ronghang Hu, Xinlei Chen, Zhuang Liu, In So Kweon, Saining Xie.
1. **[CPM](model_doc/cpm)** (from Tsinghua University) released with the paper [CPM: A Large-scale Generative Chinese Pre-trained Language Model](https://arxiv.org/abs/2012.00413) by Zhengyan Zhang, Xu Han, Hao Zhou, Pei Ke, Yuxian Gu, Deming Ye, Yujia Qin, Yusheng Su, Haozhe Ji, Jian Guan, Fanchao Qi, Xiaozhi Wang, Yanan Zheng, Guoyang Zeng, Huanqi Cao, Shengqi Chen, Daixuan Li, Zhenbo Sun, Zhiyuan Liu, Minlie Huang, Wentao Han, Jie Tang, Juanzi Li, Xiaoyan Zhu, Maosong Sun.
1. **[CTRL](model_doc/ctrl)** (from Salesforce) released with the paper [CTRL: A Conditional Transformer Language Model for Controllable Generation](https://arxiv.org/abs/1909.05858) by Nitish Shirish Keskar*, Bryan McCann*, Lav R. Varshney, Caiming Xiong and Richard Socher.
1. **[CvT](model_doc/cvt)** (from Microsoft) released with the paper [CvT: Introducing Convolutions to Vision Transformers](https://arxiv.org/abs/2103.15808) by Haiping Wu, Bin Xiao, Noel Codella, Mengchen Liu, Xiyang Dai, Lu Yuan, Lei Zhang.
1. **[Data2Vec](model_doc/data2vec)** (from Facebook) released with the paper [Data2Vec: A General Framework for Self-supervised Learning in Speech, Vision and Language](https://arxiv.org/abs/2202.03555) by Alexei Baevski, Wei-Ning Hsu, Qiantong Xu, Arun Babu, Jiatao Gu, Michael Auli.
1. **[DeBERTa](model_doc/deberta)** (from Microsoft) released with the paper [DeBERTa: Decoding-enhanced BERT with Disentangled Attention](https://arxiv.org/abs/2006.03654) by Pengcheng He, Xiaodong Liu, Jianfeng Gao, Weizhu Chen.
1. **[DeBERTa-v2](model_doc/deberta-v2)** (from Microsoft) released with the paper [DeBERTa: Decoding-enhanced BERT with Disentangled Attention](https://arxiv.org/abs/2006.03654) by Pengcheng He, Xiaodong Liu, Jianfeng Gao, Weizhu Chen.
1. **[Decision Transformer](model_doc/decision_transformer)** (from Berkeley/Facebook/Google) released with the paper [Decision Transformer: Reinforcement Learning via Sequence Modeling](https://arxiv.org/abs/2106.01345) by Lili Chen, Kevin Lu, Aravind Rajeswaran, Kimin Lee, Aditya Grover, Michael Laskin, Pieter Abbeel, Aravind Srinivas, Igor Mordatch.
1. **[DeiT](model_doc/deit)** (from Facebook) released with the paper [Training data-efficient image transformers & distillation through attention](https://arxiv.org/abs/2012.12877) by Hugo Touvron, Matthieu Cord, Matthijs Douze, Francisco Massa, Alexandre Sablayrolles, Hervรฉ Jรฉgou.
1. **[DETR](model_doc/detr)** (from Facebook) released with the paper [End-to-End Object Detection with Transformers](https://arxiv.org/abs/2005.12872) by Nicolas Carion, Francisco Massa, Gabriel Synnaeve, Nicolas Usunier, Alexander Kirillov, Sergey Zagoruyko.
1. **[DialoGPT](model_doc/dialogpt)** (from Microsoft Research) released with the paper [DialoGPT: Large-Scale Generative Pre-training for Conversational Response Generation](https://arxiv.org/abs/1911.00536) by Yizhe Zhang, Siqi Sun, Michel Galley, Yen-Chun Chen, Chris Brockett, Xiang Gao, Jianfeng Gao, Jingjing Liu, Bill Dolan.
1. **[DistilBERT](model_doc/distilbert)** (from HuggingFace), released together with the paper [DistilBERT, a distilled version of BERT: smaller, faster, cheaper and lighter](https://arxiv.org/abs/1910.01108) by Victor Sanh, Lysandre Debut and Thomas Wolf. The same method has been applied to compress GPT2 into [DistilGPT2](https://github.com/huggingface/transformers/tree/main/examples/research_projects/distillation), RoBERTa into [DistilRoBERTa](https://github.com/huggingface/transformers/tree/main/examples/research_projects/distillation), Multilingual BERT into [DistilmBERT](https://github.com/huggingface/transformers/tree/main/examples/research_projects/distillation) and a German version of DistilBERT.
1. **[DiT](model_doc/dit)** (from Microsoft Research) released with the paper [DiT: Self-supervised Pre-training for Document Image Transformer](https://arxiv.org/abs/2203.02378) by Junlong Li, Yiheng Xu, Tengchao Lv, Lei Cui, Cha Zhang, Furu Wei.
1. **[DPR](model_doc/dpr)** (from Facebook) released with the paper [Dense Passage Retrieval for Open-Domain Question Answering](https://arxiv.org/abs/2004.04906) by Vladimir Karpukhin, Barlas Oฤuz, Sewon Min, Patrick Lewis, Ledell Wu, Sergey Edunov, Danqi Chen, and Wen-tau Yih.
1. **[DPT](master/model_doc/dpt)** (from Intel Labs) released with the paper [Vision Transformers for Dense Prediction](https://arxiv.org/abs/2103.13413) by Renรฉ Ranftl, Alexey Bochkovskiy, Vladlen Koltun.
1. **[EfficientNet](model_doc/efficientnet)** (from Google Research) released with the paper [EfficientNet: Rethinking Model Scaling for Convolutional Neural Networks](https://arxiv.org/abs/1905.11946) by Mingxing Tan and Quoc V. Le.
1. **[ELECTRA](model_doc/electra)** (from Google Research/Stanford University) released with the paper [ELECTRA: Pre-training text encoders as discriminators rather than generators](https://arxiv.org/abs/2003.10555) by Kevin Clark, Minh-Thang Luong, Quoc V. Le, Christopher D. Manning.
1. **[EncoderDecoder](model_doc/encoder-decoder)** (from Google Research) released with the paper [Leveraging Pre-trained Checkpoints for Sequence Generation Tasks](https://arxiv.org/abs/1907.12461) by Sascha Rothe, Shashi Narayan, Aliaksei Severyn.
1. **[FlauBERT](model_doc/flaubert)** (from CNRS) released with the paper [FlauBERT: Unsupervised Language Model Pre-training for French](https://arxiv.org/abs/1912.05372) by Hang Le, Loรฏc Vial, Jibril Frej, Vincent Segonne, Maximin Coavoux, Benjamin Lecouteux, Alexandre Allauzen, Benoรฎt Crabbรฉ, Laurent Besacier, Didier Schwab.
1. **[FLAVA](model_doc/flava)** (from Facebook AI) released with the paper [FLAVA: A Foundational Language And Vision Alignment Model](https://arxiv.org/abs/2112.04482) by Amanpreet Singh, Ronghang Hu, Vedanuj Goswami, Guillaume Couairon, Wojciech Galuba, Marcus Rohrbach, and Douwe Kiela.
1. **[FNet](model_doc/fnet)** (from Google Research) released with the paper [FNet: Mixing Tokens with Fourier Transforms](https://arxiv.org/abs/2105.03824) by James Lee-Thorp, Joshua Ainslie, Ilya Eckstein, Santiago Ontanon.
1. **[Funnel Transformer](model_doc/funnel)** (from CMU/Google Brain) released with the paper [Funnel-Transformer: Filtering out Sequential Redundancy for Efficient Language Processing](https://arxiv.org/abs/2006.03236) by Zihang Dai, Guokun Lai, Yiming Yang, Quoc V. Le.
1. **[GLPN](model_doc/glpn)** (from KAIST) released with the paper [Global-Local Path Networks for Monocular Depth Estimation with Vertical CutDepth](https://arxiv.org/abs/2201.07436) by Doyeon Kim, Woonghyun Ga, Pyungwhan Ahn, Donggyu Joo, Sehwan Chun, Junmo Kim.
1. **[GPT](model_doc/openai-gpt)** (from OpenAI) released with the paper [Improving Language Understanding by Generative Pre-Training](https://blog.openai.com/language-unsupervised/) by Alec Radford, Karthik Narasimhan, Tim Salimans and Ilya Sutskever.
1. **[GPT Neo](model_doc/gpt_neo)** (from EleutherAI) released in the repository [EleutherAI/gpt-neo](https://github.com/EleutherAI/gpt-neo) by Sid Black, Stella Biderman, Leo Gao, Phil Wang and Connor Leahy.
1. **[GPT NeoX](model_doc/gpt_neox)** (from EleutherAI) released with the paper [GPT-NeoX-20B: An Open-Source Autoregressive Language Model](https://arxiv.org/abs/2204.06745) by Sid Black, Stella Biderman, Eric Hallahan, Quentin Anthony, Leo Gao, Laurence Golding, Horace He, Connor Leahy, Kyle McDonell, Jason Phang, Michael Pieler, USVSN Sai Prashanth, Shivanshu Purohit, Laria Reynolds, Jonathan Tow, Ben Wang, Samuel Weinbach
1. **[GPT-2](model_doc/gpt2)** (from OpenAI) released with the paper [Language Models are Unsupervised Multitask Learners](https://blog.openai.com/better-language-models/) by Alec Radford*, Jeffrey Wu*, Rewon Child, David Luan, Dario Amodei** and Ilya Sutskever**.
1. **[GPT-J](model_doc/gptj)** (from EleutherAI) released in the repository [kingoflolz/mesh-transformer-jax](https://github.com/kingoflolz/mesh-transformer-jax/) by Ben Wang and Aran Komatsuzaki.
1. **[GPTSAN-japanese](model_doc/gptsan-japanese)** released in the repository [tanreinama/GPTSAN](https://github.com/tanreinama/GPTSAN/blob/main/report/model.md) by Toshiyuki Sakamoto(tanreinama).
1. **[GroupViT](model_doc/groupvit)** (from UCSD, NVIDIA) released with the paper [GroupViT: Semantic Segmentation Emerges from Text Supervision](https://arxiv.org/abs/2202.11094) by Jiarui Xu, Shalini De Mello, Sifei Liu, Wonmin Byeon, Thomas Breuel, Jan Kautz, Xiaolong Wang.
1. **[Hubert](model_doc/hubert)** (from Facebook) released with the paper [HuBERT: Self-Supervised Speech Representation Learning by Masked Prediction of Hidden Units](https://arxiv.org/abs/2106.07447) by Wei-Ning Hsu, Benjamin Bolte, Yao-Hung Hubert Tsai, Kushal Lakhotia, Ruslan Salakhutdinov, Abdelrahman Mohamed.
1. **[I-BERT](model_doc/ibert)** (from Berkeley) released with the paper [I-BERT: Integer-only BERT Quantization](https://arxiv.org/abs/2101.01321) by Sehoon Kim, Amir Gholami, Zhewei Yao, Michael W. Mahoney, Kurt Keutzer.
1. **[ImageGPT](model_doc/imagegpt)** (from OpenAI) released with the paper [Generative Pretraining from Pixels](https://openai.com/blog/image-gpt/) by Mark Chen, Alec Radford, Rewon Child, Jeffrey Wu, Heewoo Jun, David Luan, Ilya Sutskever.
1. **[LayoutLM](model_doc/layoutlm)** (from Microsoft Research Asia) released with the paper [LayoutLM: Pre-training of Text and Layout for Document Image Understanding](https://arxiv.org/abs/1912.13318) by Yiheng Xu, Minghao Li, Lei Cui, Shaohan Huang, Furu Wei, Ming Zhou.
1. **[LayoutLMv2](model_doc/layoutlmv2)** (from Microsoft Research Asia) released with the paper [LayoutLMv2: Multi-modal Pre-training for Visually-Rich Document Understanding](https://arxiv.org/abs/2012.14740) by Yang Xu, Yiheng Xu, Tengchao Lv, Lei Cui, Furu Wei, Guoxin Wang, Yijuan Lu, Dinei Florencio, Cha Zhang, Wanxiang Che, Min Zhang, Lidong Zhou.
1. **[LayoutLMv3](model_doc/layoutlmv3)** (from Microsoft Research Asia) released with the paper [LayoutLMv3: Pre-training for Document AI with Unified Text and Image Masking](https://arxiv.org/abs/2204.08387) by Yupan Huang, Tengchao Lv, Lei Cui, Yutong Lu, Furu Wei.
1. **[LayoutXLM](model_doc/layoutxlm)** (from Microsoft Research Asia) released with the paper [LayoutXLM: Multimodal Pre-training for Multilingual Visually-rich Document Understanding](https://arxiv.org/abs/2104.08836) by Yiheng Xu, Tengchao Lv, Lei Cui, Guoxin Wang, Yijuan Lu, Dinei Florencio, Cha Zhang, Furu Wei.
1. **[LED](model_doc/led)** (from AllenAI) released with the paper [Longformer: The Long-Document Transformer](https://arxiv.org/abs/2004.05150) by Iz Beltagy, Matthew E. Peters, Arman Cohan.
1. **[LeViT](model_doc/levit)** (from Meta AI) released with the paper [LeViT: A Vision Transformer in ConvNet's Clothing for Faster Inference](https://arxiv.org/abs/2104.01136) by Ben Graham, Alaaeldin El-Nouby, Hugo Touvron, Pierre Stock, Armand Joulin, Hervรฉ Jรฉgou, Matthijs Douze.
1. **[Longformer](model_doc/longformer)** (from AllenAI) released with the paper [Longformer: The Long-Document Transformer](https://arxiv.org/abs/2004.05150) by Iz Beltagy, Matthew E. Peters, Arman Cohan.
1. **[LongT5](model_doc/longt5)** (from Google AI) released with the paper [LongT5: Efficient Text-To-Text Transformer for Long Sequences](https://arxiv.org/abs/2112.07916) by Mandy Guo, Joshua Ainslie, David Uthus, Santiago Ontanon, Jianmo Ni, Yun-Hsuan Sung, Yinfei Yang.
1. **[LUKE](model_doc/luke)** (from Studio Ousia) released with the paper [LUKE: Deep Contextualized Entity Representations with Entity-aware Self-attention](https://arxiv.org/abs/2010.01057) by Ikuya Yamada, Akari Asai, Hiroyuki Shindo, Hideaki Takeda, Yuji Matsumoto.
1. **[LXMERT](model_doc/lxmert)** (from UNC Chapel Hill) released with the paper [LXMERT: Learning Cross-Modality Encoder Representations from Transformers for Open-Domain Question Answering](https://arxiv.org/abs/1908.07490) by Hao Tan and Mohit Bansal.
1. **[M-CTC-T](model_doc/mctct)** (from Facebook) released with the paper [Pseudo-Labeling For Massively Multilingual Speech Recognition](https://arxiv.org/abs/2111.00161) by Loren Lugosch, Tatiana Likhomanenko, Gabriel Synnaeve, and Ronan Collobert.
1. **[M2M100](model_doc/m2m_100)** (from Facebook) released with the paper [Beyond English-Centric Multilingual Machine Translation](https://arxiv.org/abs/2010.11125) by Angela Fan, Shruti Bhosale, Holger Schwenk, Zhiyi Ma, Ahmed El-Kishky, Siddharth Goyal, Mandeep Baines, Onur Celebi, Guillaume Wenzek, Vishrav Chaudhary, Naman Goyal, Tom Birch, Vitaliy Liptchinsky, Sergey Edunov, Edouard Grave, Michael Auli, Armand Joulin.
1. **[MarianMT](model_doc/marian)** Machine translation models trained using [OPUS](http://opus.nlpl.eu/) data by Jรถrg Tiedemann. The [Marian Framework](https://marian-nmt.github.io/) is being developed by the Microsoft Translator Team.
1. **[Mask2Former](model_doc/mask2former)** (from FAIR and UIUC) released with the paper [Masked-attention Mask Transformer for Universal Image Segmentation](https://arxiv.org/abs/2112.01527) by Bowen Cheng, Ishan Misra, Alexander G. Schwing, Alexander Kirillov, Rohit Girdhar.
1. **[MaskFormer](model_doc/maskformer)** (from Meta and UIUC) released with the paper [Per-Pixel Classification is Not All You Need for Semantic Segmentation](https://arxiv.org/abs/2107.06278) by Bowen Cheng, Alexander G. Schwing, Alexander Kirillov.
1. **[mBART](model_doc/mbart)** (from Facebook) released with the paper [Multilingual Denoising Pre-training for Neural Machine Translation](https://arxiv.org/abs/2001.08210) by Yinhan Liu, Jiatao Gu, Naman Goyal, Xian Li, Sergey Edunov, Marjan Ghazvininejad, Mike Lewis, Luke Zettlemoyer.
1. **[mBART-50](model_doc/mbart)** (from Facebook) released with the paper [Multilingual Translation with Extensible Multilingual Pretraining and Finetuning](https://arxiv.org/abs/2008.00401) by Yuqing Tang, Chau Tran, Xian Li, Peng-Jen Chen, Naman Goyal, Vishrav Chaudhary, Jiatao Gu, Angela Fan.
1. **[Megatron-BERT](model_doc/megatron-bert)** (from NVIDIA) released with the paper [Megatron-LM: Training Multi-Billion Parameter Language Models Using Model Parallelism](https://arxiv.org/abs/1909.08053) by Mohammad Shoeybi, Mostofa Patwary, Raul Puri, Patrick LeGresley, Jared Casper and Bryan Catanzaro.
1. **[Megatron-GPT2](model_doc/megatron_gpt2)** (from NVIDIA) released with the paper [Megatron-LM: Training Multi-Billion Parameter Language Models Using Model Parallelism](https://arxiv.org/abs/1909.08053) by Mohammad Shoeybi, Mostofa Patwary, Raul Puri, Patrick LeGresley, Jared Casper and Bryan Catanzaro.
1. **[mLUKE](model_doc/mluke)** (from Studio Ousia) released with the paper [mLUKE: The Power of Entity Representations in Multilingual Pretrained Language Models](https://arxiv.org/abs/2110.08151) by Ryokan Ri, Ikuya Yamada, and Yoshimasa Tsuruoka.
1. **[MobileBERT](model_doc/mobilebert)** (from CMU/Google Brain) released with the paper [MobileBERT: a Compact Task-Agnostic BERT for Resource-Limited Devices](https://arxiv.org/abs/2004.02984) by Zhiqing Sun, Hongkun Yu, Xiaodan Song, Renjie Liu, Yiming Yang, and Denny Zhou.
1. **[MobileViT](model_doc/mobilevit)** (from Apple) released with the paper [MobileViT: Light-weight, General-purpose, and Mobile-friendly Vision Transformer](https://arxiv.org/abs/2110.02178) by Sachin Mehta and Mohammad Rastegari.
1. **[MPNet](model_doc/mpnet)** (from Microsoft Research) released with the paper [MPNet: Masked and Permuted Pre-training for Language Understanding](https://arxiv.org/abs/2004.09297) by Kaitao Song, Xu Tan, Tao Qin, Jianfeng Lu, Tie-Yan Liu.
1. **[MT5](model_doc/mt5)** (from Google AI) released with the paper [mT5: A massively multilingual pre-trained text-to-text transformer](https://arxiv.org/abs/2010.11934) by Linting Xue, Noah Constant, Adam Roberts, Mihir Kale, Rami Al-Rfou, Aditya Siddhant, Aditya Barua, Colin Raffel.
1. **[MVP](model_doc/mvp)** (from RUC AI Box) released with the paper [MVP: Multi-task Supervised Pre-training for Natural Language Generation](https://arxiv.org/abs/2206.12131) by Tianyi Tang, Junyi Li, Wayne Xin Zhao and Ji-Rong Wen.
1. **[Nezha](model_doc/nezha)** (from Huawei Noahโs Ark Lab) released with the paper [NEZHA: Neural Contextualized Representation for Chinese Language Understanding](https://arxiv.org/abs/1909.00204) by Junqiu Wei, Xiaozhe Ren, Xiaoguang Li, Wenyong Huang, Yi Liao, Yasheng Wang, Jiashu Lin, Xin Jiang, Xiao Chen and Qun Liu.
1. **[NLLB](model_doc/nllb)** (from Meta) released with the paper [No Language Left Behind: Scaling Human-Centered Machine Translation](https://arxiv.org/abs/2207.04672) by the NLLB team.
1. **[Nystrรถmformer](model_doc/nystromformer)** (from the University of Wisconsin - Madison) released with the paper [Nystrรถmformer: A Nystrรถm-Based Algorithm for Approximating Self-Attention](https://arxiv.org/abs/2102.03902) by Yunyang Xiong, Zhanpeng Zeng, Rudrasis Chakraborty, Mingxing Tan, Glenn Fung, Yin Li, Vikas Singh.
1. **[OneFormer](model_doc/oneformer)** (from SHI Labs) released with the paper [OneFormer: One Transformer to Rule Universal Image Segmentation](https://arxiv.org/abs/2211.06220) by Jitesh Jain, Jiachen Li, MangTik Chiu, Ali Hassani, Nikita Orlov, Humphrey Shi.
1. **[OPT](master/model_doc/opt)** (from Meta AI) released with the paper [OPT: Open Pre-trained Transformer Language Models](https://arxiv.org/abs/2205.01068) by Susan Zhang, Stephen Roller, Naman Goyal, Mikel Artetxe, Moya Chen, Shuohui Chen et al.
1. **[OWL-ViT](model_doc/owlvit)** (from Google AI) released with the paper [Simple Open-Vocabulary Object Detection with Vision Transformers](https://arxiv.org/abs/2205.06230) by Matthias Minderer, Alexey Gritsenko, Austin Stone, Maxim Neumann, Dirk Weissenborn, Alexey Dosovitskiy, Aravindh Mahendran, Anurag Arnab, Mostafa Dehghani, Zhuoran Shen, Xiao Wang, Xiaohua Zhai, Thomas Kipf, and Neil Houlsby.
1. **[Pegasus](model_doc/pegasus)** (from Google) released with the paper [PEGASUS: Pre-training with Extracted Gap-sentences for Abstractive Summarization](https://arxiv.org/abs/1912.08777) by Jingqing Zhang, Yao Zhao, Mohammad Saleh and Peter J. Liu.
1. **[Perceiver IO](model_doc/perceiver)** (from Deepmind) released with the paper [Perceiver IO: A General Architecture for Structured Inputs & Outputs](https://arxiv.org/abs/2107.14795) by Andrew Jaegle, Sebastian Borgeaud, Jean-Baptiste Alayrac, Carl Doersch, Catalin Ionescu, David Ding, Skanda Koppula, Daniel Zoran, Andrew Brock, Evan Shelhamer, Olivier Hรฉnaff, Matthew M. Botvinick, Andrew Zisserman, Oriol Vinyals, Joรฃo Carreira.
1. **[PhoBERT](model_doc/phobert)** (from VinAI Research) released with the paper [PhoBERT: Pre-trained language models for Vietnamese](https://www.aclweb.org/anthology/2020.findings-emnlp.92/) by Dat Quoc Nguyen and Anh Tuan Nguyen.
1. **[PLBart](model_doc/plbart)** (from UCLA NLP) released with the paper [Unified Pre-training for Program Understanding and Generation](https://arxiv.org/abs/2103.06333) by Wasi Uddin Ahmad, Saikat Chakraborty, Baishakhi Ray, Kai-Wei Chang.
1. **[PoolFormer](model_doc/poolformer)** (from Sea AI Labs) released with the paper [MetaFormer is Actually What You Need for Vision](https://arxiv.org/abs/2111.11418) by Yu, Weihao and Luo, Mi and Zhou, Pan and Si, Chenyang and Zhou, Yichen and Wang, Xinchao and Feng, Jiashi and Yan, Shuicheng.
1. **[ProphetNet](model_doc/prophetnet)** (from Microsoft Research) released with the paper [ProphetNet: Predicting Future N-gram for Sequence-to-Sequence Pre-training](https://arxiv.org/abs/2001.04063) by Yu Yan, Weizhen Qi, Yeyun Gong, Dayiheng Liu, Nan Duan, Jiusheng Chen, Ruofei Zhang and Ming Zhou.
1. **[QDQBert](model_doc/qdqbert)** (from NVIDIA) released with the paper [Integer Quantization for Deep Learning Inference: Principles and Empirical Evaluation](https://arxiv.org/abs/2004.09602) by Hao Wu, Patrick Judd, Xiaojie Zhang, Mikhail Isaev and Paulius Micikevicius.
1. **[RAG](model_doc/rag)** (from Facebook) released with the paper [Retrieval-Augmented Generation for Knowledge-Intensive NLP Tasks](https://arxiv.org/abs/2005.11401) by Patrick Lewis, Ethan Perez, Aleksandara Piktus, Fabio Petroni, Vladimir Karpukhin, Naman Goyal, Heinrich Kรผttler, Mike Lewis, Wen-tau Yih, Tim Rocktรคschel, Sebastian Riedel, Douwe Kiela.
1. **[REALM](model_doc/realm.html)** (from Google Research) released with the paper [REALM: Retrieval-Augmented Language Model Pre-Training](https://arxiv.org/abs/2002.08909) by Kelvin Guu, Kenton Lee, Zora Tung, Panupong Pasupat and Ming-Wei Chang.
1. **[Reformer](model_doc/reformer)** (from Google Research) released with the paper [Reformer: The Efficient Transformer](https://arxiv.org/abs/2001.04451) by Nikita Kitaev, ลukasz Kaiser, Anselm Levskaya.
1. **[RegNet](model_doc/regnet)** (from META Platforms) released with the paper [Designing Network Design Space](https://arxiv.org/abs/2003.13678) by Ilija Radosavovic, Raj Prateek Kosaraju, Ross Girshick, Kaiming He, Piotr Dollรกr.
1. **[RemBERT](model_doc/rembert)** (from Google Research) released with the paper [Rethinking embedding coupling in pre-trained language models](https://arxiv.org/abs/2010.12821) by Hyung Won Chung, Thibault Fรฉvry, Henry Tsai, M. Johnson, Sebastian Ruder.
1. **[ResNet](model_doc/resnet)** (from Microsoft Research) released with the paper [Deep Residual Learning for Image Recognition](https://arxiv.org/abs/1512.03385) by Kaiming He, Xiangyu Zhang, Shaoqing Ren, Jian Sun.
1. **[RoBERTa](model_doc/roberta)** (from Facebook), released together with the paper [RoBERTa: A Robustly Optimized BERT Pretraining Approach](https://arxiv.org/abs/1907.11692) by Yinhan Liu, Myle Ott, Naman Goyal, Jingfei Du, Mandar Joshi, Danqi Chen, Omer Levy, Mike Lewis, Luke Zettlemoyer, Veselin Stoyanov.
1. **[RoFormer](model_doc/roformer)** (from ZhuiyiTechnology), released together with the paper [RoFormer: Enhanced Transformer with Rotary Position Embedding](https://arxiv.org/abs/2104.09864) by Jianlin Su and Yu Lu and Shengfeng Pan and Bo Wen and Yunfeng Liu.
1. **[SegFormer](model_doc/segformer)** (from NVIDIA) released with the paper [SegFormer: Simple and Efficient Design for Semantic Segmentation with Transformers](https://arxiv.org/abs/2105.15203) by Enze Xie, Wenhai Wang, Zhiding Yu, Anima Anandkumar, Jose M. Alvarez, Ping Luo.
1. **[SEW](model_doc/sew)** (from ASAPP) released with the paper [Performance-Efficiency Trade-offs in Unsupervised Pre-training for Speech Recognition](https://arxiv.org/abs/2109.06870) by Felix Wu, Kwangyoun Kim, Jing Pan, Kyu Han, Kilian Q. Weinberger, Yoav Artzi.
1. **[SEW-D](model_doc/sew_d)** (from ASAPP) released with the paper [Performance-Efficiency Trade-offs in Unsupervised Pre-training for Speech Recognition](https://arxiv.org/abs/2109.06870) by Felix Wu, Kwangyoun Kim, Jing Pan, Kyu Han, Kilian Q. Weinberger, Yoav Artzi.
1. **[SpeechToTextTransformer](model_doc/speech_to_text)** (from Facebook), released together with the paper [fairseq S2T: Fast Speech-to-Text Modeling with fairseq](https://arxiv.org/abs/2010.05171) by Changhan Wang, Yun Tang, Xutai Ma, Anne Wu, Dmytro Okhonko, Juan Pino.
1. **[SpeechToTextTransformer2](model_doc/speech_to_text_2)** (from Facebook), released together with the paper [Large-Scale Self- and Semi-Supervised Learning for Speech Translation](https://arxiv.org/abs/2104.06678) by Changhan Wang, Anne Wu, Juan Pino, Alexei Baevski, Michael Auli, Alexis Conneau.
1. **[Splinter](model_doc/splinter)** (from Tel Aviv University), released together with the paper [Few-Shot Question Answering by Pretraining Span Selection](https://arxiv.org/abs/2101.00438) by Ori Ram, Yuval Kirstain, Jonathan Berant, Amir Globerson, Omer Levy.
1. **[SqueezeBERT](model_doc/squeezebert)** (from Berkeley) released with the paper [SqueezeBERT: What can computer vision teach NLP about efficient neural networks?](https://arxiv.org/abs/2006.11316) by Forrest N. Iandola, Albert E. Shaw, Ravi Krishna, and Kurt W. Keutzer.
1. **[Swin Transformer](model_doc/swin)** (from Microsoft) released with the paper [Swin Transformer: Hierarchical Vision Transformer using Shifted Windows](https://arxiv.org/abs/2103.14030) by Ze Liu, Yutong Lin, Yue Cao, Han Hu, Yixuan Wei, Zheng Zhang, Stephen Lin, Baining Guo.
1. **[Swin Transformer V2](model_doc/swinv2)** (from Microsoft) released with the paper [Swin Transformer V2: Scaling Up Capacity and Resolution](https://arxiv.org/abs/2111.09883) by Ze Liu, Han Hu, Yutong Lin, Zhuliang Yao, Zhenda Xie, Yixuan Wei, Jia Ning, Yue Cao, Zheng Zhang, Li Dong, Furu Wei, Baining Guo.
1. **[T5](model_doc/t5)** (from Google AI) released with the paper [Exploring the Limits of Transfer Learning with a Unified Text-to-Text Transformer](https://arxiv.org/abs/1910.10683) by Colin Raffel and Noam Shazeer and Adam Roberts and Katherine Lee and Sharan Narang and Michael Matena and Yanqi Zhou and Wei Li and Peter J. Liu.
1. **[T5v1.1](model_doc/t5v1.1)** (from Google AI) released in the repository [google-research/text-to-text-transfer-transformer](https://github.com/google-research/text-to-text-transfer-transformer/blob/main/released_checkpoints.md#t511) by Colin Raffel and Noam Shazeer and Adam Roberts and Katherine Lee and Sharan Narang and Michael Matena and Yanqi Zhou and Wei Li and Peter J. Liu.
1. **[TAPAS](model_doc/tapas)** (from Google AI) released with the paper [TAPAS: Weakly Supervised Table Parsing via Pre-training](https://arxiv.org/abs/2004.02349) by Jonathan Herzig, Paweล Krzysztof Nowak, Thomas Mรผller, Francesco Piccinno and Julian Martin Eisenschlos.
1. **[TAPEX](model_doc/tapex)** (from Microsoft Research) released with the paper [TAPEX: Table Pre-training via Learning a Neural SQL Executor](https://arxiv.org/abs/2107.07653) by Qian Liu, Bei Chen, Jiaqi Guo, Morteza Ziyadi, Zeqi Lin, Weizhu Chen, Jian-Guang Lou.
1. **[Trajectory Transformer](model_doc/trajectory_transformers)** (from the University of California at Berkeley) released with the paper [Offline Reinforcement Learning as One Big Sequence Modeling Problem](https://arxiv.org/abs/2106.02039) by Michael Janner, Qiyang Li, Sergey Levine
1. **[Transformer-XL](model_doc/transfo-xl)** (from Google/CMU) released with the paper [Transformer-XL: Attentive Language Models Beyond a Fixed-Length Context](https://arxiv.org/abs/1901.02860) by Zihang Dai*, Zhilin Yang*, Yiming Yang, Jaime Carbonell, Quoc V. Le, Ruslan Salakhutdinov.
1. **[TrOCR](model_doc/trocr)** (from Microsoft), released together with the paper [TrOCR: Transformer-based Optical Character Recognition with Pre-trained Models](https://arxiv.org/abs/2109.10282) by Minghao Li, Tengchao Lv, Lei Cui, Yijuan Lu, Dinei Florencio, Cha Zhang, Zhoujun Li, Furu Wei.
1. **[UL2](model_doc/ul2)** (from Google Research) released with the paper [Unifying Language Learning Paradigms](https://arxiv.org/abs/2205.05131v1) by Yi Tay, Mostafa Dehghani, Vinh Q. Tran, Xavier Garcia, Dara Bahri, Tal Schuster, Huaixiu Steven Zheng, Neil Houlsby, Donald Metzler
1. **[UMT5](model_doc/umt5)** (from Google Research) released with the paper [UniMax: Fairer and More Effective Language Sampling for Large-Scale Multilingual Pretraining](https://openreview.net/forum?id=kXwdL1cWOAi) by Hyung Won Chung, Xavier Garcia, Adam Roberts, Yi Tay, Orhan Firat, Sharan Narang, Noah Constant.
1. **[UniSpeech](model_doc/unispeech)** (from Microsoft Research) released with the paper [UniSpeech: Unified Speech Representation Learning with Labeled and Unlabeled Data](https://arxiv.org/abs/2101.07597) by Chengyi Wang, Yu Wu, Yao Qian, Kenichi Kumatani, Shujie Liu, Furu Wei, Michael Zeng, Xuedong Huang.
1. **[UniSpeechSat](model_doc/unispeech-sat)** (from Microsoft Research) released with the paper [UNISPEECH-SAT: UNIVERSAL SPEECH REPRESENTATION LEARNING WITH SPEAKER AWARE PRE-TRAINING](https://arxiv.org/abs/2110.05752) by Sanyuan Chen, Yu Wu, Chengyi Wang, Zhengyang Chen, Zhuo Chen, Shujie Liu, Jian Wu, Yao Qian, Furu Wei, Jinyu Li, Xiangzhan Yu.
1. **[VAN](model_doc/van)** (from Tsinghua University and Nankai University) released with the paper [Visual Attention Network](https://arxiv.org/abs/2202.09741) by Meng-Hao Guo, Cheng-Ze Lu, Zheng-Ning Liu, Ming-Ming Cheng, Shi-Min Hu.
1. **[VideoMAE](model_doc/videomae)** (from Multimedia Computing Group, Nanjing University) released with the paper [VideoMAE: Masked Autoencoders are Data-Efficient Learners for Self-Supervised Video Pre-Training](https://arxiv.org/abs/2203.12602) by Zhan Tong, Yibing Song, Jue Wang, Limin Wang.
1. **[ViLT](model_doc/vilt)** (from NAVER AI Lab/Kakao Enterprise/Kakao Brain) released with the paper [ViLT: Vision-and-Language Transformer Without Convolution or Region Supervision](https://arxiv.org/abs/2102.03334) by Wonjae Kim, Bokyung Son, Ildoo Kim.
1. **[Vision Transformer (ViT)](model_doc/vit)** (from Google AI) released with the paper [An Image is Worth 16x16 Words: Transformers for Image Recognition at Scale](https://arxiv.org/abs/2010.11929) by Alexey Dosovitskiy, Lucas Beyer, Alexander Kolesnikov, Dirk Weissenborn, Xiaohua Zhai, Thomas Unterthiner, Mostafa Dehghani, Matthias Minderer, Georg Heigold, Sylvain Gelly, Jakob Uszkoreit, Neil Houlsby.
1. **[VisualBERT](model_doc/visual_bert)** (from UCLA NLP) released with the paper [VisualBERT: A Simple and Performant Baseline for Vision and Language](https://arxiv.org/pdf/1908.03557) by Liunian Harold Li, Mark Yatskar, Da Yin, Cho-Jui Hsieh, Kai-Wei Chang.
1. **[ViTMAE](model_doc/vit_mae)** (from Meta AI) released with the paper [Masked Autoencoders Are Scalable Vision Learners](https://arxiv.org/abs/2111.06377) by Kaiming He, Xinlei Chen, Saining Xie, Yanghao Li, Piotr Dollรกr, Ross Girshick.
1. **[Wav2Vec2](model_doc/wav2vec2)** (from Facebook AI) released with the paper [wav2vec 2.0: A Framework for Self-Supervised Learning of Speech Representations](https://arxiv.org/abs/2006.11477) by Alexei Baevski, Henry Zhou, Abdelrahman Mohamed, Michael Auli.
1. **[Wav2Vec2-Conformer](model_doc/wav2vec2-conformer)** (from Facebook AI) released with the paper [FAIRSEQ S2T: Fast Speech-to-Text Modeling with FAIRSEQ](https://arxiv.org/abs/2010.05171) by Changhan Wang, Yun Tang, Xutai Ma, Anne Wu, Sravya Popuri, Dmytro Okhonko, Juan Pino.
1. **[Wav2Vec2Phoneme](model_doc/wav2vec2_phoneme)** (from Facebook AI) released with the paper [Simple and Effective Zero-shot Cross-lingual Phoneme Recognition](https://arxiv.org/abs/2109.11680) by Qiantong Xu, Alexei Baevski, Michael Auli.
1. **[WavLM](model_doc/wavlm)** (from Microsoft Research) released with the paper [WavLM: Large-Scale Self-Supervised Pre-Training for Full Stack Speech Processing](https://arxiv.org/abs/2110.13900) by Sanyuan Chen, Chengyi Wang, Zhengyang Chen, Yu Wu, Shujie Liu, Zhuo Chen, Jinyu Li, Naoyuki Kanda, Takuya Yoshioka, Xiong Xiao, Jian Wu, Long Zhou, Shuo Ren, Yanmin Qian, Yao Qian, Jian Wu, Michael Zeng, Furu Wei.
1. **[XGLM](model_doc/xglm)** (From Facebook AI) released with the paper [Few-shot Learning with Multilingual Language Models](https://arxiv.org/abs/2112.10668) by Xi Victoria Lin, Todor Mihaylov, Mikel Artetxe, Tianlu Wang, Shuohui Chen, Daniel Simig, Myle Ott, Naman Goyal, Shruti Bhosale, Jingfei Du, Ramakanth Pasunuru, Sam Shleifer, Punit Singh Koura, Vishrav Chaudhary, Brian O'Horo, Jeff Wang, Luke Zettlemoyer, Zornitsa Kozareva, Mona Diab, Veselin Stoyanov, Xian Li.
1. **[XLM](model_doc/xlm)** (from Facebook) released together with the paper [Cross-lingual Language Model Pretraining](https://arxiv.org/abs/1901.07291) by Guillaume Lample and Alexis Conneau.
1. **[XLM-ProphetNet](model_doc/xlm-prophetnet)** (from Microsoft Research) released with the paper [ProphetNet: Predicting Future N-gram for Sequence-to-Sequence Pre-training](https://arxiv.org/abs/2001.04063) by Yu Yan, Weizhen Qi, Yeyun Gong, Dayiheng Liu, Nan Duan, Jiusheng Chen, Ruofei Zhang and Ming Zhou.
1. **[XLM-RoBERTa](model_doc/xlm-roberta)** (from Facebook AI), released together with the paper [Unsupervised Cross-lingual Representation Learning at Scale](https://arxiv.org/abs/1911.02116) by Alexis Conneau*, Kartikay Khandelwal*, Naman Goyal, Vishrav Chaudhary, Guillaume Wenzek, Francisco Guzmรกn, Edouard Grave, Myle Ott, Luke Zettlemoyer and Veselin Stoyanov.
1. **[XLM-RoBERTa-XL](model_doc/xlm-roberta-xl)** (from Facebook AI), released together with the paper [Larger-Scale Transformers for Multilingual Masked Language Modeling](https://arxiv.org/abs/2105.00572) by Naman Goyal, Jingfei Du, Myle Ott, Giri Anantharaman, Alexis Conneau.
1. **[XLM-V](model_doc/xlm-v)** (from Meta AI) released with the paper [XLM-V: Overcoming the Vocabulary Bottleneck in Multilingual Masked Language Models](https://arxiv.org/abs/2301.10472) by Davis Liang, Hila Gonen, Yuning Mao, Rui Hou, Naman Goyal, Marjan Ghazvininejad, Luke Zettlemoyer, Madian Khabsa.
1. **[XLNet](model_doc/xlnet)** (from Google/CMU) released with the paper [โXLNet: Generalized Autoregressive Pretraining for Language Understanding](https://arxiv.org/abs/1906.08237) by Zhilin Yang*, Zihang Dai*, Yiming Yang, Jaime Carbonell, Ruslan Salakhutdinov, Quoc V. Le.
1. **[XLS-R](model_doc/xls_r)** (from Facebook AI) released with the paper [XLS-R: Self-supervised Cross-lingual Speech Representation Learning at Scale](https://arxiv.org/abs/2111.09296) by Arun Babu, Changhan Wang, Andros Tjandra, Kushal Lakhotia, Qiantong Xu, Naman Goyal, Kritika Singh, Patrick von Platen, Yatharth Saraf, Juan Pino, Alexei Baevski, Alexis Conneau, Michael Auli.
1. **[XLSR-Wav2Vec2](model_doc/xlsr_wav2vec2)** (from Facebook AI) released with the paper [Unsupervised Cross-Lingual Representation Learning For Speech Recognition](https://arxiv.org/abs/2006.13979) by Alexis Conneau, Alexei Baevski, Ronan Collobert, Abdelrahman Mohamed, Michael Auli.
1. **[YOLOS](model_doc/yolos)** (from Huazhong University of Science & Technology) released with the paper [You Only Look at One Sequence: Rethinking Transformer in Vision through Object Detection](https://arxiv.org/abs/2106.00666) by Yuxin Fang, Bencheng Liao, Xinggang Wang, Jiemin Fang, Jiyang Qi, Rui Wu, Jianwei Niu, Wenyu Liu.
1. **[YOSO](model_doc/yoso)** (from the University of Wisconsin - Madison) released with the paper [You Only Sample (Almost) Once: Linear Cost Self-Attention Via Bernoulli Sampling](https://arxiv.org/abs/2111.09714) by Zhanpeng Zeng, Yunyang Xiong, Sathya N. Ravi, Shailesh Acharya, Glenn Fung, Vikas Singh.
### Unterstรผtzte Frameworks
Die folgende Tabelle zeigt die derzeitige Unterstรผtzung in der Bibliothek fรผr jedes dieser Modelle, unabhรคngig davon, ob sie einen Python
Tokenizer haben (als "langsam" bezeichnet), ein "schneller" Tokenizer, der von der ๐ค Tokenizers Bibliothek unterstรผtzt wird, ob sie Unterstรผtzung in Jax (via
Flax), PyTorch, und/oder TensorFlow haben.
<!--This table is updated automatically from the auto modules with _make fix-copies_. Do not update manually!-->
| Model | Tokenizer slow | Tokenizer fast | PyTorch support | TensorFlow support | Flax Support |
|:---------------------------:|:--------------:|:--------------:|:---------------:|:------------------:|:------------:|
| ALBERT | โ
| โ
| โ
| โ
| โ
|
| BART | โ
| โ
| โ
| โ
| โ
|
| BEiT | โ | โ | โ
| โ | โ
|
| BERT | โ
| โ
| โ
| โ
| โ
|
| Bert Generation | โ
| โ | โ
| โ | โ |
| BigBird | โ
| โ
| โ
| โ | โ
|
| BigBird-Pegasus | โ | โ | โ
| โ | โ |
| Blenderbot | โ
| โ
| โ
| โ
| โ
|
| BlenderbotSmall | โ
| โ
| โ
| โ
| โ
|
| BLOOM | โ | โ
| โ
| โ | โ |
| CamemBERT | โ
| โ
| โ
| โ
| โ |
| CANINE | โ
| โ | โ
| โ | โ |
| CLIP | โ
| โ
| โ
| โ
| โ
|
| CodeGen | โ
| โ
| โ
| โ | โ |
| ConvBERT | โ
| โ
| โ
| โ
| โ |
| ConvNeXT | โ | โ | โ
| โ
| โ |
| CTRL | โ
| โ | โ
| โ
| โ |
| CvT | โ | โ | โ
| โ | โ |
| Data2VecAudio | โ | โ | โ
| โ | โ |
| Data2VecText | โ | โ | โ
| โ | โ |
| Data2VecVision | โ | โ | โ
| โ
| โ |
| DeBERTa | โ
| โ
| โ
| โ
| โ |
| DeBERTa-v2 | โ
| โ
| โ
| โ
| โ |
| Decision Transformer | โ | โ | โ
| โ | โ |
| DeiT | โ | โ | โ
| โ
| โ |
| DETR | โ | โ | โ
| โ | โ |
| DistilBERT | โ
| โ
| โ
| โ
| โ
|
| DPR | โ
| โ
| โ
| โ
| โ |
| DPT | โ | โ | โ
| โ | โ |
| ELECTRA | โ
| โ
| โ
| โ
| โ
|
| Encoder decoder | โ | โ | โ
| โ
| โ
|
| FairSeq Machine-Translation | โ
| โ | โ
| โ | โ |
| FlauBERT | โ
| โ | โ
| โ
| โ |
| FLAVA | โ | โ | โ
| โ | โ |
| FNet | โ
| โ
| โ
| โ | โ |
| Funnel Transformer | โ
| โ
| โ
| โ
| โ |
| GLPN | โ | โ | โ
| โ | โ |
| GPT Neo | โ | โ | โ
| โ | โ
|
| GPT NeoX | โ | โ
| โ
| โ | โ |
| GPT-J | โ | โ | โ
| โ
| โ
|
| GroupViT | โ | โ | โ
| โ | โ |
| Hubert | โ | โ | โ
| โ
| โ |
| I-BERT | โ | โ | โ
| โ | โ |
| ImageGPT | โ | โ | โ
| โ | โ |
| LayoutLM | โ
| โ
| โ
| โ
| โ |
| LayoutLMv2 | โ
| โ
| โ
| โ | โ |
| LayoutLMv3 | โ
| โ
| โ
| โ | โ |
| LED | โ
| โ
| โ
| โ
| โ |
| LeViT | โ | โ | โ
| โ | โ |
| Longformer | โ
| โ
| โ
| โ
| โ |
| LongT5 | โ | โ | โ
| โ | โ
|
| LUKE | โ
| โ | โ
| โ | โ |
| LXMERT | โ
| โ
| โ
| โ
| โ |
| M-CTC-T | โ | โ | โ
| โ | โ |
| M2M100 | โ
| โ | โ
| โ | โ |
| Marian | โ
| โ | โ
| โ
| โ
|
| MaskFormer | โ | โ | โ
| โ | โ |
| mBART | โ
| โ
| โ
| โ
| โ
|
| Megatron-BERT | โ | โ | โ
| โ | โ |
| MobileBERT | โ
| โ
| โ
| โ
| โ |
| MobileViT | โ | โ | โ
| โ | โ |
| MPNet | โ
| โ
| โ
| โ
| โ |
| MT5 | โ
| โ
| โ
| โ
| โ
|
| MVP | โ
| โ
| โ
| โ | โ |
| Nezha | โ | โ | โ
| โ | โ |
| Nystrรถmformer | โ | โ | โ
| โ | โ |
| OpenAI GPT | โ
| โ
| โ
| โ
| โ |
| OpenAI GPT-2 | โ
| โ
| โ
| โ
| โ
|
| OPT | โ | โ | โ
| โ
| โ
|
| OWL-ViT | โ | โ | โ
| โ | โ |
| Pegasus | โ
| โ
| โ
| โ
| โ
|
| Perceiver | โ
| โ | โ
| โ | โ |
| PLBart | โ
| โ | โ
| โ | โ |
| PoolFormer | โ | โ | โ
| โ | โ |
| ProphetNet | โ
| โ | โ
| โ | โ |
| QDQBert | โ | โ | โ
| โ | โ |
| RAG | โ
| โ | โ
| โ
| โ |
| REALM | โ
| โ
| โ
| โ | โ |
| Reformer | โ
| โ
| โ
| โ | โ |
| RegNet | โ | โ | โ
| โ
| โ
|
| RemBERT | โ
| โ
| โ
| โ
| โ |
| ResNet | โ | โ | โ
| โ
| โ
|
| RetriBERT | โ
| โ
| โ
| โ | โ |
| RoBERTa | โ
| โ
| โ
| โ
| โ
|
| RoFormer | โ
| โ
| โ
| โ
| โ
|
| SegFormer | โ | โ | โ
| โ
| โ |
| SEW | โ | โ | โ
| โ | โ |
| SEW-D | โ | โ | โ
| โ | โ |
| Speech Encoder decoder | โ | โ | โ
| โ | โ
|
| Speech2Text | โ
| โ | โ
| โ
| โ |
| Speech2Text2 | โ
| โ | โ | โ | โ |
| Splinter | โ
| โ
| โ
| โ | โ |
| SqueezeBERT | โ
| โ
| โ
| โ | โ |
| Swin Transformer | โ | โ | โ
| โ
| โ |
| Swin Transformer V2 | โ | โ | โ
| โ | โ |
| T5 | โ
| โ
| โ
| โ
| โ
|
| TAPAS | โ
| โ | โ
| โ
| โ |
| Trajectory Transformer | โ | โ | โ
| โ | โ |
| Transformer-XL | โ
| โ | โ
| โ
| โ |
| TrOCR | โ | โ | โ
| โ | โ |
| UniSpeech | โ | โ | โ
| โ | โ |
| UniSpeechSat | โ | โ | โ
| โ | โ |
| VAN | โ | โ | โ
| โ | โ |
| VideoMAE | โ | โ | โ
| โ | โ |
| ViLT | โ | โ | โ
| โ | โ |
| Vision Encoder decoder | โ | โ | โ
| โ
| โ
|
| VisionTextDualEncoder | โ | โ | โ
| โ | โ
|
| VisualBERT | โ | โ | โ
| โ | โ |
| ViT | โ | โ | โ
| โ
| โ
|
| ViTMAE | โ | โ | โ
| โ
| โ |
| Wav2Vec2 | โ
| โ | โ
| โ
| โ
|
| Wav2Vec2-Conformer | โ | โ | โ
| โ | โ |
| WavLM | โ | โ | โ
| โ | โ |
| XGLM | โ
| โ
| โ
| โ | โ
|
| XLM | โ
| โ | โ
| โ
| โ |
| XLM-ProphetNet | โ
| โ | โ
| โ | โ |
| XLM-RoBERTa | โ
| โ
| โ
| โ
| โ
|
| XLM-RoBERTa-XL | โ | โ | โ
| โ | โ |
| XLNet | โ
| โ
| โ
| โ
| โ |
| YOLOS | โ | โ | โ
| โ | โ |
| YOSO | โ | โ | โ
| โ | โ |
<!-- End table-->
| 0 |
hf_public_repos/transformers/docs/source | hf_public_repos/transformers/docs/source/de/installation.md | <!---
Copyright 2022 The HuggingFace Team. All rights reserved.
Licensed under the Apache License, Version 2.0 (the "License");
you may not use this file except in compliance with the License.
You may obtain a copy of the License at
http://www.apache.org/licenses/LICENSE-2.0
Unless required by applicable law or agreed to in writing, software
distributed under the License is distributed on an "AS IS" BASIS,
WITHOUT WARRANTIES OR CONDITIONS OF ANY KIND, either express or implied.
See the License for the specific language governing permissions and
limitations under the License.
โ ๏ธ Note that this file is in Markdown but contain specific syntax for our doc-builder (similar to MDX) that may not be
rendered properly in your Markdown viewer.
-->
# Installation
Installieren Sie ๐ค Transformers fรผr die Deep-Learning-Bibliothek, mit der Sie arbeiten, richten Sie Ihren Cache ein und konfigurieren Sie ๐ค Transformers optional fรผr den Offline-Betrieb.
๐ค Transformers wurde unter Python 3.6+, PyTorch 1.1.0+, TensorFlow 2.0+, und Flax getestet. Folgen Sie den Installationsanweisungen unten fรผr die von Ihnen verwendete Deep-Learning-Bibliothek:
* [PyTorch](https://pytorch.org/get-started/locally/) installation instructions.
* [TensorFlow 2.0](https://www.tensorflow.org/install/pip) installation instructions.
* [Flax](https://flax.readthedocs.io/en/latest/) installation instructions.
## Installation mit pip
Sie sollten ๐ค Transformers in einer [virtuellen Umgebung](https://docs.python.org/3/library/venv.html) installieren. Wenn Sie mit virtuellen Python-Umgebungen nicht vertraut sind, werfen Sie einen Blick auf diese [Anleitung](https://packaging.python.org/guides/installing-using-pip-and-virtual-environments/). Eine virtuelle Umgebung macht es einfacher, verschiedene Projekte zu verwalten und Kompatibilitรคtsprobleme zwischen Abhรคngigkeiten zu vermeiden.
Beginnen wir mit der Erstellung einer virtuellen Umgebung in Ihrem Projektverzeichnis:
```bash
python -m venv .env
```
Aktivieren wir die virtuelle Umgebung. Unter Linux und MacOs:
```bash
source .env/bin/activate
```
Aktivieren wir die virtuelle Umgebung unter Windows
```bash
.env/Scripts/activate
```
Jetzt kรถnnen wir die ๐ค Transformers mit dem folgenden Befehl installieren:
```bash
pip install transformers
```
Bei reiner CPU-Unterstรผtzung kรถnnen wir ๐ค Transformers und eine Deep-Learning-Bibliothek bequem in einer Zeile installieren. Installieren wir zum Beispiel ๐ค Transformers und PyTorch mit:
```bash
pip install transformers[torch]
```
๐ค Transformers und TensorFlow 2.0:
```bash
pip install transformers[tf-cpu]
```
๐ค Transformers und Flax:
```bash
pip install transformers[flax]
```
รberprรผfen wir abschlieรend, ob ๐ค Transformers ordnungsgemรคร installiert wurde, indem wir den folgenden Befehl ausfรผhren. Es wird ein vortrainiertes Modell heruntergeladen:
```bash
python -c "from transformers import pipeline; print(pipeline('sentiment-analysis')('we love you'))"
```
Dann wird die Kategorie und die Wahrscheinlichkeit ausgegeben:
```bash
[{'label': 'POSITIVE', 'score': 0.9998704791069031}]
```
## Installation aus dem Code
Installieren wir ๐ค Transformers aus dem Quellcode mit dem folgenden Befehl:
```bash
pip install git+https://github.com/huggingface/transformers
```
Dieser Befehl installiert die aktuelle `main` Version und nicht die neueste `stable` Version. Die `main`-Version ist nรผtzlich, um mit den neuesten Entwicklungen Schritt zu halten. Zum Beispiel, wenn ein Fehler seit der letzten offiziellen Version behoben wurde, aber eine neue Version noch nicht verรถffentlicht wurde. Das bedeutet jedoch, dass die "Hauptversion" nicht immer stabil ist. Wir bemรผhen uns, die Hauptversion einsatzbereit zu halten, und die meisten Probleme werden normalerweise innerhalb weniger Stunden oder eines Tages behoben. Wenn Sie auf ein Problem stoรen, รถffnen Sie bitte ein [Issue] (https://github.com/huggingface/transformers/issues), damit wir es noch schneller beheben kรถnnen!
รberprรผfen wir, ob ๐ค Transformers richtig installiert wurde, indem Sie den folgenden Befehl ausfรผhren:
```bash
python -c "from transformers import pipeline; print(pipeline('sentiment-analysis')('I love you'))"
```
## Editierbare Installation
Sie benรถtigen eine bearbeitbare Installation, wenn Sie:
* die "Haupt"-Version des Quellcodes verwenden mรถchten.
* Zu ๐ค Transformers beitragen und รnderungen am Code testen wollen.
Klonen Sie das Repository und installieren ๐ค Transformers mit den folgenden Befehlen:
```bash
git clone https://github.com/huggingface/transformers.git
cd transformers
pip install -e .
```
Diese Befehle verknรผpfen den Ordner, in den Sie das Repository geklont haben, mit den Pfaden Ihrer Python-Bibliotheken. Python wird nun in dem Ordner suchen, in den Sie geklont haben, zusรคtzlich zu den normalen Bibliothekspfaden. Wenn zum Beispiel Ihre Python-Pakete normalerweise in `~/anaconda3/envs/main/lib/python3.7/site-packages/` installiert sind, wird Python auch den Ordner durchsuchen, in den Sie geklont haben: `~/transformers/`.
<Tip warning={true}>
Sie mรผssen den Ordner `transformers` behalten, wenn Sie die Bibliothek weiter verwenden wollen.
</Tip>
Jetzt kรถnnen Sie Ihren Klon mit dem folgenden Befehl ganz einfach auf die neueste Version von ๐ค Transformers aktualisieren:
```bash
cd ~/transformers/
git pull
```
Ihre Python-Umgebung wird beim nรคchsten Ausfรผhren die `main`-Version von ๐ค Transformers finden.
## Installation mit conda
Installation von dem conda Kanal `huggingface`:
```bash
conda install -c huggingface transformers
```
## Cache Einrichtung
Vorgefertigte Modelle werden heruntergeladen und lokal zwischengespeichert unter: `~/.cache/huggingface/hub`. Dies ist das Standardverzeichnis, das durch die Shell-Umgebungsvariable "TRANSFORMERS_CACHE" vorgegeben ist. Unter Windows wird das Standardverzeichnis durch `C:\Benutzer\Benutzername\.cache\huggingface\hub` angegeben. Sie kรถnnen die unten aufgefรผhrten Shell-Umgebungsvariablen - in der Reihenfolge ihrer Prioritรคt - รคndern, um ein anderes Cache-Verzeichnis anzugeben:
1. Shell-Umgebungsvariable (Standard): `HUGGINGFACE_HUB_CACHE` oder `TRANSFORMERS_CACHE`.
2. Shell-Umgebungsvariable: `HF_HOME`.
3. Shell-Umgebungsvariable: `XDG_CACHE_HOME` + `/huggingface`.
<Tip>
Transformers verwendet die Shell-Umgebungsvariablen `PYTORCH_TRANSFORMERS_CACHE` oder `PYTORCH_PRETRAINED_BERT_CACHE`, wenn Sie von einer frรผheren Iteration dieser Bibliothek kommen und diese Umgebungsvariablen gesetzt haben, sofern Sie nicht die Shell-Umgebungsvariable `TRANSFORMERS_CACHE` angeben.
</Tip>
## Offline Modus
Transformers ist in der Lage, in einer Firewall- oder Offline-Umgebung zu laufen, indem es nur lokale Dateien verwendet. Setzen Sie die Umgebungsvariable `TRANSFORMERS_OFFLINE=1`, um dieses Verhalten zu aktivieren.
<Tip>
Fรผgen sie [๐ค Datasets](https://huggingface.co/docs/datasets/) zu Ihrem Offline-Trainingsworkflow hinzufรผgen, indem Sie die Umgebungsvariable `HF_DATASETS_OFFLINE=1` setzen.
</Tip>
So wรผrden Sie beispielsweise ein Programm in einem normalen Netzwerk mit einer Firewall fรผr externe Instanzen mit dem folgenden Befehl ausfรผhren:
```bash
python examples/pytorch/translation/run_translation.py --model_name_or_path t5-small --dataset_name wmt16 --dataset_config ro-en ...
```
Fรผhren Sie das gleiche Programm in einer Offline-Instanz mit aus:
```bash
HF_DATASETS_OFFLINE=1 TRANSFORMERS_OFFLINE=1 \
python examples/pytorch/translation/run_translation.py --model_name_or_path t5-small --dataset_name wmt16 --dataset_config ro-en ...
```
Das Skript sollte nun laufen, ohne sich aufzuhรคngen oder eine Zeitรผberschreitung abzuwarten, da es weiร, dass es nur nach lokalen Dateien suchen soll.
### Abrufen von Modellen und Tokenizern zur Offline-Verwendung
Eine andere Mรถglichkeit, ๐ค Transformers offline zu verwenden, besteht darin, die Dateien im Voraus herunterzuladen und dann auf ihren lokalen Pfad zu verweisen, wenn Sie sie offline verwenden mรผssen. Es gibt drei Mรถglichkeiten, dies zu tun:
* Laden Sie eine Datei รผber die Benutzeroberflรคche des [Model Hub](https://huggingface.co/models) herunter, indem Sie auf das โ-Symbol klicken.

* Verwenden Sie den [PreTrainedModel.from_pretrained] und [PreTrainedModel.save_pretrained] Workflow:
1. Laden Sie Ihre Dateien im Voraus mit [`PreTrainedModel.from_pretrained`] herunter:
```py
>>> from transformers import AutoTokenizer, AutoModelForSeq2SeqLM
>>> tokenizer = AutoTokenizer.from_pretrained("bigscience/T0_3B")
>>> model = AutoModelForSeq2SeqLM.from_pretrained("bigscience/T0_3B")
```
2. Speichern Sie Ihre Dateien in einem bestimmten Verzeichnis mit [`PreTrainedModel.save_pretrained`]:
```py
>>> tokenizer.save_pretrained("./your/path/bigscience_t0")
>>> model.save_pretrained("./your/path/bigscience_t0")
```
3. Wenn Sie nun offline sind, laden Sie Ihre Dateien mit [`PreTrainedModel.from_pretrained`] aus dem bestimmten Verzeichnis:
```py
>>> tokenizer = AutoTokenizer.from_pretrained("./your/path/bigscience_t0")
>>> model = AutoModel.from_pretrained("./your/path/bigscience_t0")
```
* Programmatisches Herunterladen von Dateien mit der [huggingface_hub](https://github.com/huggingface/huggingface_hub/tree/main/src/huggingface_hub) Bibliothek:
1. Installieren Sie die "huggingface_hub"-Bibliothek in Ihrer virtuellen Umgebung:
```bash
python -m pip install huggingface_hub
```
2. Verwenden Sie die Funktion [`hf_hub_download`](https://huggingface.co/docs/hub/adding-a-library#download-files-from-the-hub), um eine Datei in einen bestimmten Pfad herunterzuladen. Der folgende Befehl lรคdt zum Beispiel die Datei "config.json" aus dem Modell [T0](https://huggingface.co/bigscience/T0_3B) in den gewรผnschten Pfad herunter:
```py
>>> from huggingface_hub import hf_hub_download
>>> hf_hub_download(repo_id="bigscience/T0_3B", filename="config.json", cache_dir="./your/path/bigscience_t0")
```
Sobald Ihre Datei heruntergeladen und lokal zwischengespeichert ist, geben Sie den lokalen Pfad an, um sie zu laden und zu verwenden:
```py
>>> from transformers import AutoConfig
>>> config = AutoConfig.from_pretrained("./your/path/bigscience_t0/config.json")
```
<Tip>
Weitere Informationen zum Herunterladen von Dateien, die auf dem Hub gespeichert sind, finden Sie im Abschnitt [Wie man Dateien vom Hub herunterlรคdt] (https://huggingface.co/docs/hub/how-to-downstream).
</Tip>
| 0 |
hf_public_repos/transformers/docs/source | hf_public_repos/transformers/docs/source/de/_toctree.yml | - sections:
- local: index
title: ๐ค Transformers
- local: quicktour
title: Schnellstart
- local: installation
title: Installation
title: Erste Schritte
- sections:
- local: pipeline_tutorial
title: Pipelines fรผr Inferenzen
- local: autoclass_tutorial
title: Laden von vortrainierten Instanzen mit einer AutoClass
- local: preprocessing
title: Vorverarbeiten
- local: training
title: Optimierung eines vortrainierten Modells
- local: accelerate
title: Verteiltes Training mit ๐ค Accelerate
- local: model_sharing
title: Ein Modell teilen
title: Tutorials
| 0 |
hf_public_repos/transformers/docs/source | hf_public_repos/transformers/docs/source/de/preprocessing.md | <!--Copyright 2022 The HuggingFace Team. All rights reserved.
Licensed under the Apache License, Version 2.0 (the "License"); you may not use this file except in compliance with
the License. You may obtain a copy of the License at
http://www.apache.org/licenses/LICENSE-2.0
Unless required by applicable law or agreed to in writing, software distributed under the License is distributed on
an "AS IS" BASIS, WITHOUT WARRANTIES OR CONDITIONS OF ANY KIND, either express or implied. See the License for the
specific language governing permissions and limitations under the License.
โ ๏ธ Note that this file is in Markdown but contain specific syntax for our doc-builder (similar to MDX) that may not be
rendered properly in your Markdown viewer.
-->
# Vorverarbeiten
[[open-in-colab]]
Bevor Sie Ihre Daten in einem Modell verwenden kรถnnen, mรผssen die Daten in ein fรผr das Modell akzeptables Format gebracht werden. Ein Modell versteht keine Rohtexte, Bilder oder Audiodaten. Diese Eingaben mรผssen in Zahlen umgewandelt und zu Tensoren zusammengesetzt werden. In dieser Anleitung werden Sie:
* Textdaten mit einem Tokenizer vorverarbeiten.
* Bild- oder Audiodaten mit einem Feature Extractor vorverarbeiten.
* Daten fรผr eine multimodale Aufgabe mit einem Prozessor vorverarbeiten.
## NLP
<Youtube id="Yffk5aydLzg"/>
Das wichtigste Werkzeug zur Verarbeitung von Textdaten ist ein [Tokenizer](main_classes/tokenizer). Ein Tokenizer zerlegt Text zunรคchst nach einer Reihe von Regeln in *Token*. Die Token werden in Zahlen umgewandelt, die zum Aufbau von Tensoren als Eingabe fรผr ein Modell verwendet werden. Alle zusรคtzlichen Eingaben, die ein Modell benรถtigt, werden ebenfalls vom Tokenizer hinzugefรผgt.
<Tip>
Wenn Sie ein vortrainiertes Modell verwenden mรถchten, ist es wichtig, den zugehรถrigen vortrainierten Tokenizer zu verwenden. Dadurch wird sichergestellt, dass der Text auf die gleiche Weise aufgeteilt wird wie das Pretraining-Korpus und die gleichen entsprechenden Token-zu-Index (in der Regel als *vocab* bezeichnet) wรคhrend des Pretrainings verwendet werden.
</Tip>
Laden Sie einen vortrainierten Tokenizer mit der Klasse [AutoTokenizer], um schnell loszulegen. Damit wird das *vocab* heruntergeladen, das verwendet wird, wenn ein Modell vortrainiert wird.
### Tokenize
Laden Sie einen vortrainierten Tokenizer mit [`AutoTokenizer.from_pretrained`]:
```py
>>> from transformers import AutoTokenizer
>>> tokenizer = AutoTokenizer.from_pretrained("bert-base-cased")
```
Dann รผbergeben Sie Ihren Satz an den Tokenizer:
```py
>>> encoded_input = tokenizer("Do not meddle in the affairs of wizards, for they are subtle and quick to anger.")
>>> print(encoded_input)
{'input_ids': [101, 2079, 2025, 19960, 10362, 1999, 1996, 3821, 1997, 16657, 1010, 2005, 2027, 2024, 11259, 1998, 4248, 2000, 4963, 1012, 102],
'token_type_ids': [0, 0, 0, 0, 0, 0, 0, 0, 0, 0, 0, 0, 0, 0, 0, 0, 0, 0, 0, 0, 0],
'attention_mask': [1, 1, 1, 1, 1, 1, 1, 1, 1, 1, 1, 1, 1, 1, 1, 1, 1, 1, 1, 1, 1]}
```
Der Tokenizer gibt ein Wรถrterbuch mit drei wichtigen Elementen zurรผck:
* [input_ids](glossary#input-ids) sind die Indizes, die den einzelnen Token im Satz entsprechen.
* [attention_mask](glossary#attention-mask) gibt an, ob ein Token beachtet werden soll oder nicht.
* [token_type_ids](glossary#token-type-ids) gibt an, zu welcher Sequenz ein Token gehรถrt, wenn es mehr als eine Sequenz gibt.
Sie kรถnnen die `input_ids` dekodieren, um die ursprรผngliche Eingabe zurรผckzugeben:
```py
>>> tokenizer.decode(encoded_input["input_ids"])
'[CLS] Do not meddle in the affairs of wizards, for they are subtle and quick to anger. [SEP]'
```
Wie Sie sehen kรถnnen, hat der Tokenisierer zwei spezielle Token - `CLS` und `SEP` (Klassifikator und Separator) - zum Satz hinzugefรผgt. Nicht alle Modelle benรถtigen
spezielle Token, aber wenn dies der Fall ist, fรผgt der Tokenisierer sie automatisch fรผr Sie hinzu.
Wenn Sie mehrere Sรคtze verarbeiten wollen, รผbergeben Sie die Sรคtze als Liste an den Tokenizer:
```py
>>> batch_sentences = [
... "But what about second breakfast?",
... "Don't think he knows about second breakfast, Pip.",
... "What about elevensies?",
... ]
>>> encoded_inputs = tokenizer(batch_sentences)
>>> print(encoded_inputs)
{'input_ids': [[101, 1252, 1184, 1164, 1248, 6462, 136, 102],
[101, 1790, 112, 189, 1341, 1119, 3520, 1164, 1248, 6462, 117, 21902, 1643, 119, 102],
[101, 1327, 1164, 5450, 23434, 136, 102]],
'token_type_ids': [[0, 0, 0, 0, 0, 0, 0, 0],
[0, 0, 0, 0, 0, 0, 0, 0, 0, 0, 0, 0, 0, 0, 0],
[0, 0, 0, 0, 0, 0, 0]],
'attention_mask': [[1, 1, 1, 1, 1, 1, 1, 1],
[1, 1, 1, 1, 1, 1, 1, 1, 1, 1, 1, 1, 1, 1, 1],
[1, 1, 1, 1, 1, 1, 1]]}
```
### Pad
Dies bringt uns zu einem wichtigen Thema. Wenn Sie einen Haufen von Sรคtzen verarbeiten, sind diese nicht immer gleich lang. Das ist ein Problem, weil Tensoren, die Eingabe fรผr das Modell, eine einheitliche Form haben mรผssen. Padding ist eine Strategie, die sicherstellt, dass Tensoren rechteckig sind, indem ein spezielles *Padding-Token* zu Sรคtzen mit weniger Token hinzugefรผgt wird.
Setzen Sie den Parameter "padding" auf "true", um die kรผrzeren Sequenzen im Stapel so aufzufรผllen, dass sie der lรคngsten Sequenz entsprechen:
```py
>>> batch_sentences = [
... "But what about second breakfast?",
... "Don't think he knows about second breakfast, Pip.",
... "What about elevensies?",
... ]
>>> encoded_input = tokenizer(batch_sentences, padding=True)
>>> print(encoded_input)
{'input_ids': [[101, 1252, 1184, 1164, 1248, 6462, 136, 102, 0, 0, 0, 0, 0, 0, 0],
[101, 1790, 112, 189, 1341, 1119, 3520, 1164, 1248, 6462, 117, 21902, 1643, 119, 102],
[101, 1327, 1164, 5450, 23434, 136, 102, 0, 0, 0, 0, 0, 0, 0, 0]],
'token_type_ids': [[0, 0, 0, 0, 0, 0, 0, 0, 0, 0, 0, 0, 0, 0, 0],
[0, 0, 0, 0, 0, 0, 0, 0, 0, 0, 0, 0, 0, 0, 0],
[0, 0, 0, 0, 0, 0, 0, 0, 0, 0, 0, 0, 0, 0, 0]],
'attention_mask': [[1, 1, 1, 1, 1, 1, 1, 1, 0, 0, 0, 0, 0, 0, 0],
[1, 1, 1, 1, 1, 1, 1, 1, 1, 1, 1, 1, 1, 1, 1],
[1, 1, 1, 1, 1, 1, 1, 0, 0, 0, 0, 0, 0, 0, 0]]}
```
Beachten Sie, dass der Tokenizer den ersten und den dritten Satz mit einer "0" aufgefรผllt hat, weil sie kรผrzer sind!
### Kรผrzung
Auf der anderen Seite des Spektrums kann es vorkommen, dass eine Sequenz zu lang fรผr ein Modell ist. In diesem Fall mรผssen Sie die Sequenz auf eine kรผrzere Lรคnge kรผrzen.
Setzen Sie den Parameter "truncation" auf "true", um eine Sequenz auf die vom Modell akzeptierte Hรถchstlรคnge zu kรผrzen:
```py
>>> batch_sentences = [
... "But what about second breakfast?",
... "Don't think he knows about second breakfast, Pip.",
... "What about elevensies?",
... ]
>>> encoded_input = tokenizer(batch_sentences, padding=True, truncation=True)
>>> print(encoded_input)
{'input_ids': [[101, 1252, 1184, 1164, 1248, 6462, 136, 102, 0, 0, 0, 0, 0, 0, 0],
[101, 1790, 112, 189, 1341, 1119, 3520, 1164, 1248, 6462, 117, 21902, 1643, 119, 102],
[101, 1327, 1164, 5450, 23434, 136, 102, 0, 0, 0, 0, 0, 0, 0, 0]],
'token_type_ids': [[0, 0, 0, 0, 0, 0, 0, 0, 0, 0, 0, 0, 0, 0, 0],
[0, 0, 0, 0, 0, 0, 0, 0, 0, 0, 0, 0, 0, 0, 0],
[0, 0, 0, 0, 0, 0, 0, 0, 0, 0, 0, 0, 0, 0, 0]],
'attention_mask': [[1, 1, 1, 1, 1, 1, 1, 1, 0, 0, 0, 0, 0, 0, 0],
[1, 1, 1, 1, 1, 1, 1, 1, 1, 1, 1, 1, 1, 1, 1],
[1, 1, 1, 1, 1, 1, 1, 0, 0, 0, 0, 0, 0, 0, 0]]}
```
### Tensoren erstellen
Schlieรlich mรถchten Sie, dass der Tokenizer die tatsรคchlichen Tensoren zurรผckgibt, die dem Modell zugefรผhrt werden.
Setzen Sie den Parameter `return_tensors` entweder auf `pt` fรผr PyTorch, oder `tf` fรผr TensorFlow:
<frameworkcontent>
<pt>
```py
>>> batch_sentences = [
... "But what about second breakfast?",
... "Don't think he knows about second breakfast, Pip.",
... "What about elevensies?",
... ]
>>> encoded_input = tokenizer(batch_sentences, padding=True, truncation=True, return_tensors="pt")
>>> print(encoded_input)
{'input_ids': tensor([[101, 1252, 1184, 1164, 1248, 6462, 136, 102, 0, 0, 0, 0, 0, 0, 0],
[101, 1790, 112, 189, 1341, 1119, 3520, 1164, 1248, 6462, 117, 21902, 1643, 119, 102],
[101, 1327, 1164, 5450, 23434, 136, 102, 0, 0, 0, 0, 0, 0, 0, 0]]),
'token_type_ids': tensor([[0, 0, 0, 0, 0, 0, 0, 0, 0, 0, 0, 0, 0, 0, 0],
[0, 0, 0, 0, 0, 0, 0, 0, 0, 0, 0, 0, 0, 0, 0],
[0, 0, 0, 0, 0, 0, 0, 0, 0, 0, 0, 0, 0, 0, 0]]),
'attention_mask': tensor([[1, 1, 1, 1, 1, 1, 1, 1, 0, 0, 0, 0, 0, 0, 0],
[1, 1, 1, 1, 1, 1, 1, 1, 1, 1, 1, 1, 1, 1, 1],
[1, 1, 1, 1, 1, 1, 1, 0, 0, 0, 0, 0, 0, 0, 0]])}
```
</pt>
<tf>
```py
>>> batch_sentences = [
... "But what about second breakfast?",
... "Don't think he knows about second breakfast, Pip.",
... "What about elevensies?",
... ]
>>> encoded_input = tokenizer(batch_sentences, padding=True, truncation=True, return_tensors="tf")
>>> print(encoded_input)
{'input_ids': <tf.Tensor: shape=(2, 9), dtype=int32, numpy=
array([[101, 1252, 1184, 1164, 1248, 6462, 136, 102, 0, 0, 0, 0, 0, 0, 0],
[101, 1790, 112, 189, 1341, 1119, 3520, 1164, 1248, 6462, 117, 21902, 1643, 119, 102],
[101, 1327, 1164, 5450, 23434, 136, 102, 0, 0, 0, 0, 0, 0, 0, 0]],
dtype=int32)>,
'token_type_ids': <tf.Tensor: shape=(2, 9), dtype=int32, numpy=
array([[0, 0, 0, 0, 0, 0, 0, 0, 0, 0, 0, 0, 0, 0, 0],
[0, 0, 0, 0, 0, 0, 0, 0, 0, 0, 0, 0, 0, 0, 0],
[0, 0, 0, 0, 0, 0, 0, 0, 0, 0, 0, 0, 0, 0, 0]], dtype=int32)>,
'attention_mask': <tf.Tensor: shape=(2, 9), dtype=int32, numpy=
array([[1, 1, 1, 1, 1, 1, 1, 1, 0, 0, 0, 0, 0, 0, 0],
[1, 1, 1, 1, 1, 1, 1, 1, 1, 1, 1, 1, 1, 1, 1],
[1, 1, 1, 1, 1, 1, 1, 0, 0, 0, 0, 0, 0, 0, 0]], dtype=int32)>}
```
</tf>
</frameworkcontent>
## Audio
Audioeingaben werden anders vorverarbeitet als Texteingaben, aber das Endziel bleibt dasselbe: numerische Sequenzen zu erstellen, die das Modell verstehen kann. Ein [feature extractor](main_classes/feature_extractor) dient dem ausdrรผcklichen Zweck, Merkmale aus Rohbild- oder Audiodaten zu extrahieren und in Tensoren zu konvertieren. Bevor Sie beginnen, installieren Sie ๐ค Datasets, um einen Audio-Datensatz zu laden, mit dem Sie experimentieren kรถnnen:
```bash
pip install datasets
```
Laden Sie den [MInDS-14](https://huggingface.co/datasets/PolyAI/minds14) Datensatz (weitere Informationen zum Laden eines Datensatzes finden Sie im ๐ค [Datasets tutorial](https://huggingface.co/docs/datasets/load_hub.html)):
```py
>>> from datasets import load_dataset, Audio
>>> dataset = load_dataset("PolyAI/minds14", name="en-US", split="train")
```
Greifen Sie auf das erste Element der `audio`-Spalte zu, um einen Blick auf die Eingabe zu werfen. Durch den Aufruf der Spalte "audio" wird die Audiodatei automatisch geladen und neu gesampelt:
```py
>>> dataset[0]["audio"]
{'array': array([ 0. , 0.00024414, -0.00024414, ..., -0.00024414,
0. , 0. ], dtype=float32),
'path': '/root/.cache/huggingface/datasets/downloads/extracted/f14948e0e84be638dd7943ac36518a4cf3324e8b7aa331c5ab11541518e9368c/en-US~JOINT_ACCOUNT/602ba55abb1e6d0fbce92065.wav',
'sampling_rate': 8000}
```
Dies gibt drei Elemente zurรผck:
* "array" ist das Sprachsignal, das als 1D-Array geladen - und mรถglicherweise neu gesampelt - wurde.
* Pfad" zeigt auf den Speicherort der Audiodatei.
* `sampling_rate` bezieht sich darauf, wie viele Datenpunkte im Sprachsignal pro Sekunde gemessen werden.
### Resample
Fรผr dieses Tutorial werden Sie das Modell [Wav2Vec2](https://huggingface.co/facebook/wav2vec2-base) verwenden. Wie Sie aus der Modellkarte ersehen kรถnnen, ist das Wav2Vec2-Modell auf 16kHz abgetastetes Sprachaudio vortrainiert. Es ist wichtig, dass die Abtastrate Ihrer Audiodaten mit der Abtastrate des Datensatzes รผbereinstimmt, der fรผr das Pre-Training des Modells verwendet wurde. Wenn die Abtastrate Ihrer Daten nicht dieselbe ist, mรผssen Sie Ihre Audiodaten neu abtasten.
Der Datensatz [MInDS-14](https://huggingface.co/datasets/PolyAI/minds14) hat zum Beispiel eine Abtastrate von 8000 kHz. Um das Wav2Vec2-Modell mit diesem Datensatz verwenden zu kรถnnen, mรผssen Sie die Abtastrate auf 16 kHz erhรถhen:
```py
>>> dataset = load_dataset("PolyAI/minds14", name="en-US", split="train")
>>> dataset[0]["audio"]
{'array': array([ 0. , 0.00024414, -0.00024414, ..., -0.00024414,
0. , 0. ], dtype=float32),
'path': '/root/.cache/huggingface/datasets/downloads/extracted/f14948e0e84be638dd7943ac36518a4cf3324e8b7aa331c5ab11541518e9368c/en-US~JOINT_ACCOUNT/602ba55abb1e6d0fbce92065.wav',
'sampling_rate': 8000}
```
1. Verwenden Sie die Methode [~datasets.Dataset.cast_column] von ๐ค Datasets, um die Abtastrate auf 16kHz zu erhรถhen:
```py
>>> dataset = dataset.cast_column("audio", Audio(sampling_rate=16_000))
```
2. Laden Sie die Audiodatei:
```py
>>> dataset[0]["audio"]
{'array': array([ 2.3443763e-05, 2.1729663e-04, 2.2145823e-04, ...,
3.8356509e-05, -7.3497440e-06, -2.1754686e-05], dtype=float32),
'path': '/root/.cache/huggingface/datasets/downloads/extracted/f14948e0e84be638dd7943ac36518a4cf3324e8b7aa331c5ab11541518e9368c/en-US~JOINT_ACCOUNT/602ba55abb1e6d0fbce92065.wav',
'sampling_rate': 16000}
```
Wie Sie sehen kรถnnen, ist die Abtastrate jetzt 16kHz!
### Merkmalsextraktor
Der nรคchste Schritt ist das Laden eines Merkmalsextraktors, um die Eingabe zu normalisieren und aufzufรผllen. Beim Auffรผllen von Textdaten wird fรผr kรผrzere Sequenzen ein `0` hinzugefรผgt. Die gleiche Idee gilt fรผr Audiodaten, und der Audio-Feature-Extraktor fรผgt eine `0` - interpretiert als Stille - zu `array` hinzu.
Laden Sie den Merkmalsextraktor mit [`AutoFeatureExtractor.from_pretrained`]:
```py
>>> from transformers import AutoFeatureExtractor
>>> feature_extractor = AutoFeatureExtractor.from_pretrained("facebook/wav2vec2-base")
```
รbergeben Sie das Audio-"Array" an den Feature-Extraktor. Wir empfehlen auch, das Argument `sampling_rate` im Feature Extractor hinzuzufรผgen, um eventuell auftretende stille Fehler besser zu beheben.
```py
>>> audio_input = [dataset[0]["audio"]["array"]]
>>> feature_extractor(audio_input, sampling_rate=16000)
{'input_values': [array([ 3.8106556e-04, 2.7506407e-03, 2.8015103e-03, ...,
5.6335266e-04, 4.6588284e-06, -1.7142107e-04], dtype=float32)]}
```
### Auffรผllen und Kรผrzen
Genau wie beim Tokenizer kรถnnen Sie variable Sequenzen in einem Stapel durch Auffรผllen oder Abschneiden behandeln. Werfen Sie einen Blick auf die Sequenzlรคnge dieser beiden Audiobeispiele:
```py
>>> dataset[0]["audio"]["array"].shape
(173398,)
>>> dataset[1]["audio"]["array"].shape
(106496,)
```
Wie Sie sehen kรถnnen, hat das erste Beispiel eine lรคngere Sequenz als das zweite Beispiel. Lassen Sie uns eine Funktion erstellen, die den Datensatz vorverarbeitet. Geben Sie eine maximale Lรคnge der Probe an, und der Feature-Extraktor wird die Sequenzen entweder auffรผllen oder abschneiden, damit sie dieser Lรคnge entsprechen:
```py
>>> def preprocess_function(examples):
... audio_arrays = [x["array"] for x in examples["audio"]]
... inputs = feature_extractor(
... audio_arrays,
... sampling_rate=16000,
... padding=True,
... max_length=100000,
... truncation=True,
... )
... return inputs
```
Wenden Sie die Funktion auf die ersten paar Beispiele im Datensatz an:
```py
>>> processed_dataset = preprocess_function(dataset[:5])
```
Schauen Sie sich nun noch einmal die verarbeiteten Beispiel-Lรคngen an:
```py
>>> processed_dataset["input_values"][0].shape
(100000,)
>>> processed_dataset["input_values"][1].shape
(100000,)
```
Die Lรคnge der ersten beiden Beispiele entspricht nun der von Ihnen angegebenen Maximallรคnge.
## Bildverarbeitung
Ein Merkmalsextraktor wird auch verwendet, um Bilder fรผr Bildverarbeitungsaufgaben zu verarbeiten. Auch hier besteht das Ziel darin, das Rohbild in eine Reihe von Tensoren als Eingabe zu konvertieren.
Laden wir den [food101](https://huggingface.co/datasets/food101) Datensatz fรผr dieses Tutorial. Verwenden Sie den Parameter ๐ค Datasets `split`, um nur eine kleine Stichprobe aus dem Trainingssplit zu laden, da der Datensatz recht groร ist:
```py
>>> from datasets import load_dataset
>>> dataset = load_dataset("food101", split="train[:100]")
```
Als Nรคchstes sehen Sie sich das Bild mit dem Merkmal ๐ค Datensรคtze [Bild] (https://huggingface.co/docs/datasets/package_reference/main_classes.html?highlight=image#datasets.Image) an:
```py
>>> dataset[0]["image"]
```
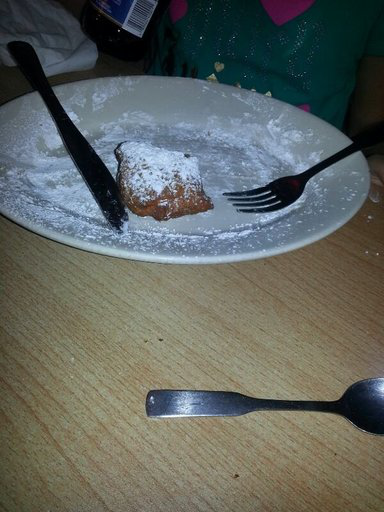
### Merkmalsextraktor
Laden Sie den Merkmalsextraktor mit [`AutoImageProcessor.from_pretrained`]:
```py
>>> from transformers import AutoImageProcessor
>>> image_processor = AutoImageProcessor.from_pretrained("google/vit-base-patch16-224")
```
### Datenerweiterung
Bei Bildverarbeitungsaufgaben ist es รผblich, den Bildern als Teil der Vorverarbeitung eine Art von Datenerweiterung hinzuzufรผgen. Sie kรถnnen Erweiterungen mit jeder beliebigen Bibliothek hinzufรผgen, aber in diesem Tutorial werden Sie das Modul [`transforms`](https://pytorch.org/vision/stable/transforms.html) von torchvision verwenden.
1. Normalisieren Sie das Bild und verwenden Sie [`Compose`](https://pytorch.org/vision/master/generated/torchvision.transforms.Compose.html), um einige Transformationen - [`RandomResizedCrop`](https://pytorch.org/vision/main/generated/torchvision.transforms.RandomResizedCrop.html) und [`ColorJitter`](https://pytorch.org/vision/main/generated/torchvision.transforms.ColorJitter.html) - miteinander zu verknรผpfen:
```py
>>> from torchvision.transforms import Compose, Normalize, RandomResizedCrop, ColorJitter, ToTensor
>>> normalize = Normalize(mean=image_processor.image_mean, std=image_processor.image_std)
>>> _transforms = Compose(
... [RandomResizedCrop(image_processor.size["height"]), ColorJitter(brightness=0.5, hue=0.5), ToTensor(), normalize]
... )
```
2. Das Modell akzeptiert [`pixel_values`](model_doc/visionencoderdecoder#transformers.VisionEncoderDecoderModel.forward.pixel_values) als Eingabe. Dieser Wert wird vom Merkmalsextraktor erzeugt. Erstellen Sie eine Funktion, die `pixel_values` aus den Transformationen erzeugt:
```py
>>> def transforms(examples):
... examples["pixel_values"] = [_transforms(image.convert("RGB")) for image in examples["image"]]
... return examples
```
3. Dann verwenden Sie ๐ค Datasets [`set_transform`](https://huggingface.co/docs/datasets/process.html#format-transform), um die Transformationen im laufenden Betrieb anzuwenden:
```py
>>> dataset.set_transform(transforms)
```
4. Wenn Sie nun auf das Bild zugreifen, werden Sie feststellen, dass der Feature Extractor die Modelleingabe "pixel_values" hinzugefรผgt hat:
```py
>>> dataset[0]["image"]
{'image': <PIL.JpegImagePlugin.JpegImageFile image mode=RGB size=384x512 at 0x7F1A7B0630D0>,
'label': 6,
'pixel_values': tensor([[[ 0.0353, 0.0745, 0.1216, ..., -0.9922, -0.9922, -0.9922],
[-0.0196, 0.0667, 0.1294, ..., -0.9765, -0.9843, -0.9922],
[ 0.0196, 0.0824, 0.1137, ..., -0.9765, -0.9686, -0.8667],
...,
[ 0.0275, 0.0745, 0.0510, ..., -0.1137, -0.1216, -0.0824],
[ 0.0667, 0.0824, 0.0667, ..., -0.0588, -0.0745, -0.0980],
[ 0.0353, 0.0353, 0.0431, ..., -0.0039, -0.0039, -0.0588]],
[[ 0.2078, 0.2471, 0.2863, ..., -0.9451, -0.9373, -0.9451],
[ 0.1608, 0.2471, 0.3098, ..., -0.9373, -0.9451, -0.9373],
[ 0.2078, 0.2706, 0.3020, ..., -0.9608, -0.9373, -0.8275],
...,
[-0.0353, 0.0118, -0.0039, ..., -0.2392, -0.2471, -0.2078],
[ 0.0196, 0.0353, 0.0196, ..., -0.1843, -0.2000, -0.2235],
[-0.0118, -0.0039, -0.0039, ..., -0.0980, -0.0980, -0.1529]],
[[ 0.3961, 0.4431, 0.4980, ..., -0.9216, -0.9137, -0.9216],
[ 0.3569, 0.4510, 0.5216, ..., -0.9059, -0.9137, -0.9137],
[ 0.4118, 0.4745, 0.5216, ..., -0.9137, -0.8902, -0.7804],
...,
[-0.2314, -0.1922, -0.2078, ..., -0.4196, -0.4275, -0.3882],
[-0.1843, -0.1686, -0.2000, ..., -0.3647, -0.3804, -0.4039],
[-0.1922, -0.1922, -0.1922, ..., -0.2941, -0.2863, -0.3412]]])}
```
Hier sehen Sie, wie das Bild nach der Vorverarbeitung aussieht. Wie von den angewandten Transformationen zu erwarten, wurde das Bild willkรผrlich beschnitten und seine Farbeigenschaften sind anders.
```py
>>> import numpy as np
>>> import matplotlib.pyplot as plt
>>> img = dataset[0]["pixel_values"]
>>> plt.imshow(img.permute(1, 2, 0))
```
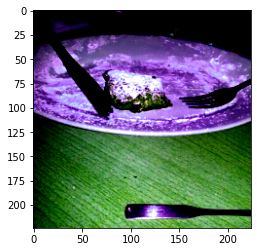
## Multimodal
Fรผr multimodale Aufgaben werden Sie eine Kombination aus allem, was Sie bisher gelernt haben, verwenden und Ihre Fรคhigkeiten auf eine Aufgabe der automatischen Spracherkennung (ASR) anwenden. Dies bedeutet, dass Sie einen:
* Feature Extractor zur Vorverarbeitung der Audiodaten.
* Tokenizer, um den Text zu verarbeiten.
Kehren wir zum [LJ Speech](https://huggingface.co/datasets/lj_speech) Datensatz zurรผck:
```py
>>> from datasets import load_dataset
>>> lj_speech = load_dataset("lj_speech", split="train")
```
Da Sie hauptsรคchlich an den Spalten "Audio" und "Text" interessiert sind, entfernen Sie die anderen Spalten:
```py
>>> lj_speech = lj_speech.map(remove_columns=["file", "id", "normalized_text"])
```
Schauen Sie sich nun die Spalten "Audio" und "Text" an:
```py
>>> lj_speech[0]["audio"]
{'array': array([-7.3242188e-04, -7.6293945e-04, -6.4086914e-04, ...,
7.3242188e-04, 2.1362305e-04, 6.1035156e-05], dtype=float32),
'path': '/root/.cache/huggingface/datasets/downloads/extracted/917ece08c95cf0c4115e45294e3cd0dee724a1165b7fc11798369308a465bd26/LJSpeech-1.1/wavs/LJ001-0001.wav',
'sampling_rate': 22050}
>>> lj_speech[0]["text"]
'Printing, in the only sense with which we are at present concerned, differs from most if not from all the arts and crafts represented in the Exhibition'
```
Erinnern Sie sich an den frรผheren Abschnitt รผber die Verarbeitung von Audiodaten: Sie sollten immer die Abtastrate Ihrer Audiodaten [resample](preprocessing#audio), damit sie mit der Abtastrate des Datensatzes รผbereinstimmt, der fรผr das Vortraining eines Modells verwendet wird:
```py
>>> lj_speech = lj_speech.cast_column("audio", Audio(sampling_rate=16_000))
```
### Prozessor
Ein Processor kombiniert einen Feature-Extraktor und einen Tokenizer. Laden Sie einen Processor mit [`AutoProcessor.from_pretrained]:
```py
>>> from transformers import AutoProcessor
>>> processor = AutoProcessor.from_pretrained("facebook/wav2vec2-base-960h")
```
1. Erstellen Sie eine Funktion, die die Audiodaten zu `input_values` verarbeitet und den Text zu `labels` tokenisiert. Dies sind Ihre Eingaben fรผr das Modell:
```py
>>> def prepare_dataset(example):
... audio = example["audio"]
... example.update(processor(audio=audio["array"], text=example["text"], sampling_rate=16000))
... return example
```
2. Wenden Sie die Funktion "prepare_dataset" auf ein Beispiel an:
```py
>>> prepare_dataset(lj_speech[0])
```
Beachten Sie, dass der Processor `input_values` und `labels` hinzugefรผgt hat. Auch die Abtastrate wurde korrekt auf 16kHz heruntergerechnet.
Toll, Sie sollten jetzt in der Lage sein, Daten fรผr jede Modalitรคt vorzuverarbeiten und sogar verschiedene Modalitรคten zu kombinieren! Im nรคchsten Kurs lernen Sie, wie Sie ein Modell mit Ihren neu aufbereiteten Daten feinabstimmen kรถnnen.
| 0 |
hf_public_repos/transformers/docs/source | hf_public_repos/transformers/docs/source/de/model_sharing.md | <!--Copyright 2022 The HuggingFace Team. All rights reserved.
Licensed under the Apache License, Version 2.0 (the "License"); you may not use this file except in compliance with
the License. You may obtain a copy of the License at
http://www.apache.org/licenses/LICENSE-2.0
Unless required by applicable law or agreed to in writing, software distributed under the License is distributed on
an "AS IS" BASIS, WITHOUT WARRANTIES OR CONDITIONS OF ANY KIND, either express or implied. See the License for the
specific language governing permissions and limitations under the License.
โ ๏ธ Note that this file is in Markdown but contain specific syntax for our doc-builder (similar to MDX) that may not be
rendered properly in your Markdown viewer.
-->
# Ein Modell teilen
Die letzten beiden Tutorials haben gezeigt, wie man ein Modell mit PyTorch, Keras und ๐ค Accelerate fรผr verteilte Setups feinabstimmen kann. Der nรคchste Schritt besteht darin, Ihr Modell mit der Community zu teilen! Bei Hugging Face glauben wir an den offenen Austausch von Wissen und Ressourcen, um kรผnstliche Intelligenz fรผr alle zu demokratisieren. Wir ermutigen Sie, Ihr Modell mit der Community zu teilen, um anderen zu helfen, Zeit und Ressourcen zu sparen.
In diesem Tutorial lernen Sie zwei Methoden kennen, wie Sie ein trainiertes oder verfeinertes Modell auf dem [Model Hub](https://huggingface.co/models) teilen kรถnnen:
- Programmgesteuertes รbertragen Ihrer Dateien auf den Hub.
- Ziehen Sie Ihre Dateien per Drag-and-Drop รผber die Weboberflรคche in den Hub.
<iframe width="560" height="315" src="https://www.youtube.com/embed/XvSGPZFEjDY" title="YouTube video player"
frameborder="0" allow="accelerometer; autoplay; clipboard-write; encrypted-media; gyroscope;
picture-in-picture" allowfullscreen></iframe>
<Tip>
Um ein Modell mit der รffentlichkeit zu teilen, benรถtigen Sie ein Konto auf [huggingface.co](https://huggingface.co/join). Sie kรถnnen auch einer bestehenden Organisation beitreten oder eine neue Organisation grรผnden.
</Tip>
## Repository-Funktionen
Jedes Repository im Model Hub verhรคlt sich wie ein typisches GitHub-Repository. Unsere Repositorys bieten Versionierung, Commit-Historie und die Mรถglichkeit, Unterschiede zu visualisieren.
Die integrierte Versionierung des Model Hub basiert auf Git und [git-lfs](https://git-lfs.github.com/). Mit anderen Worten: Sie kรถnnen ein Modell als ein Repository behandeln, was eine bessere Zugriffskontrolle und Skalierbarkeit ermรถglicht. Die Versionskontrolle ermรถglicht *Revisionen*, eine Methode zum Anheften einer bestimmten Version eines Modells mit einem Commit-Hash, Tag oder Branch.
Folglich kรถnnen Sie eine bestimmte Modellversion mit dem Parameter "Revision" laden:
```py
>>> model = AutoModel.from_pretrained(
... "julien-c/EsperBERTo-small", revision="v2.0.1" # tag name, or branch name, or commit hash
... )
```
Dateien lassen sich auch in einem Repository leicht bearbeiten, und Sie kรถnnen die Commit-Historie sowie die Unterschiede einsehen:

## Einrichtung
Bevor Sie ein Modell fรผr den Hub freigeben, benรถtigen Sie Ihre Hugging Face-Anmeldedaten. Wenn Sie Zugang zu einem Terminal haben, fรผhren Sie den folgenden Befehl in der virtuellen Umgebung aus, in der ๐ค Transformers installiert ist. Dadurch werden Ihre Zugangsdaten in Ihrem Hugging Face-Cache-Ordner (standardmรครig `~/.cache/`) gespeichert:
```bash
huggingface-cli login
```
Wenn Sie ein Notebook wie Jupyter oder Colaboratory verwenden, stellen Sie sicher, dass Sie die [`huggingface_hub`](https://huggingface.co/docs/hub/adding-a-library) Bibliothek installiert haben. Diese Bibliothek ermรถglicht Ihnen die programmatische Interaktion mit dem Hub.
```bash
pip install huggingface_hub
```
Verwenden Sie dann `notebook_login`, um sich beim Hub anzumelden, und folgen Sie dem Link [hier](https://huggingface.co/settings/token), um ein Token fรผr die Anmeldung zu generieren:
```py
>>> from huggingface_hub import notebook_login
>>> notebook_login()
```
## Ein Modell fรผr alle Frameworks konvertieren
Um sicherzustellen, dass Ihr Modell von jemandem verwendet werden kann, der mit einem anderen Framework arbeitet, empfehlen wir Ihnen, Ihr Modell sowohl mit PyTorch- als auch mit TensorFlow-Checkpoints zu konvertieren und hochzuladen. Wรคhrend Benutzer immer noch in der Lage sind, Ihr Modell von einem anderen Framework zu laden, wenn Sie diesen Schritt รผberspringen, wird es langsamer sein, weil ๐ค Transformers den Checkpoint on-the-fly konvertieren mรผssen.
Die Konvertierung eines Checkpoints fรผr ein anderes Framework ist einfach. Stellen Sie sicher, dass Sie PyTorch und TensorFlow installiert haben (siehe [hier](installation) fรผr Installationsanweisungen), und finden Sie dann das spezifische Modell fรผr Ihre Aufgabe in dem anderen Framework.
<frameworkcontent>
<pt>
Geben Sie `from_tf=True` an, um einen Prรผfpunkt von TensorFlow nach PyTorch zu konvertieren:
```py
>>> pt_model = DistilBertForSequenceClassification.from_pretrained("path/to/awesome-name-you-picked", from_tf=True)
>>> pt_model.save_pretrained("path/to/awesome-name-you-picked")
```
</pt>
<tf>
Geben Sie `from_pt=True` an, um einen Prรผfpunkt von PyTorch nach TensorFlow zu konvertieren:
```py
>>> tf_model = TFDistilBertForSequenceClassification.from_pretrained("path/to/awesome-name-you-picked", from_pt=True)
```
Dann kรถnnen Sie Ihr neues TensorFlow-Modell mit seinem neuen Checkpoint speichern:
```py
>>> tf_model.save_pretrained("path/to/awesome-name-you-picked")
```
</tf>
<jax>
Wenn ein Modell in Flax verfรผgbar ist, kรถnnen Sie auch einen Kontrollpunkt von PyTorch nach Flax konvertieren:
```py
>>> flax_model = FlaxDistilBertForSequenceClassification.from_pretrained(
... "path/to/awesome-name-you-picked", from_pt=True
... )
```
</jax>
</frameworkcontent>
## Ein Modell wรคhrend des Trainings hochladen
<frameworkcontent>
<pt>
<Youtube id="Z1-XMy-GNLQ"/>
Die Weitergabe eines Modells an den Hub ist so einfach wie das Hinzufรผgen eines zusรคtzlichen Parameters oder Rรผckrufs. Erinnern Sie sich an das [Feinabstimmungs-Tutorial](training), in der Klasse [`TrainingArguments`] geben Sie Hyperparameter und zusรคtzliche Trainingsoptionen an. Eine dieser Trainingsoptionen beinhaltet die Mรถglichkeit, ein Modell direkt an den Hub zu pushen. Setzen Sie `push_to_hub=True` in Ihrer [`TrainingArguments`]:
```py
>>> training_args = TrainingArguments(output_dir="my-awesome-model", push_to_hub=True)
```
รbergeben Sie Ihre Trainingsargumente wie gewohnt an [`Trainer`]:
```py
>>> trainer = Trainer(
... model=model,
... args=training_args,
... train_dataset=small_train_dataset,
... eval_dataset=small_eval_dataset,
... compute_metrics=compute_metrics,
... )
```
Nach der Feinabstimmung Ihres Modells rufen Sie [`~transformers.Trainer.push_to_hub`] auf [`Trainer`] auf, um das trainierte Modell an den Hub zu รผbertragen. Transformers fรผgt sogar automatisch Trainings-Hyperparameter, Trainingsergebnisse und Framework-Versionen zu Ihrer Modellkarte hinzu!
```py
>>> trainer.push_to_hub()
```
</pt>
<tf>
Geben Sie ein Modell mit [`PushToHubCallback`] an den Hub weiter. In der [`PushToHubCallback`] Funktion, fรผgen Sie hinzu:
- Ein Ausgabeverzeichnis fรผr Ihr Modell.
- Einen Tokenizer.
- Die `hub_model_id`, die Ihr Hub-Benutzername und Modellname ist.
```py
>>> from transformers import PushToHubCallback
>>> push_to_hub_callback = PushToHubCallback(
... output_dir="./your_model_save_path", tokenizer=tokenizer, hub_model_id="your-username/my-awesome-model"
... )
```
Fรผgen Sie den Callback zu [`fit`](https://keras.io/api/models/model_training_apis/) hinzu, und ๐ค Transformers wird das trainierte Modell an den Hub weiterleiten:
```py
>>> model.fit(tf_train_dataset, validation_data=tf_validation_dataset, epochs=3, callbacks=push_to_hub_callback)
```
</tf>
</frameworkcontent>
## Verwenden Sie die Funktion `push_to_hub`.
Sie kรถnnen `push_to_hub` auch direkt fรผr Ihr Modell aufrufen, um es in den Hub hochzuladen.
Geben Sie den Namen Ihres Modells in "push_to_hub" an:
```py
>>> pt_model.push_to_hub("my-awesome-model")
```
Dadurch wird ein Repository unter Ihrem Benutzernamen mit dem Modellnamen `my-awesome-model` erstellt. Benutzer kรถnnen nun Ihr Modell mit der Funktion `from_pretrained` laden:
```py
>>> from transformers import AutoModel
>>> model = AutoModel.from_pretrained("your_username/my-awesome-model")
```
Wenn Sie zu einer Organisation gehรถren und Ihr Modell stattdessen unter dem Namen der Organisation pushen wollen, fรผgen Sie diesen einfach zur `repo_id` hinzu:
```py
>>> pt_model.push_to_hub("my-awesome-org/my-awesome-model")
```
Die Funktion "push_to_hub" kann auch verwendet werden, um andere Dateien zu einem Modell-Repository hinzuzufรผgen. Zum Beispiel kann man einen Tokenizer zu einem Modell-Repository hinzufรผgen:
```py
>>> tokenizer.push_to_hub("my-awesome-model")
```
Oder vielleicht mรถchten Sie die TensorFlow-Version Ihres fein abgestimmten PyTorch-Modells hinzufรผgen:
```py
>>> tf_model.push_to_hub("my-awesome-model")
```
Wenn Sie nun zu Ihrem Hugging Face-Profil navigieren, sollten Sie Ihr neu erstelltes Modell-Repository sehen. Wenn Sie auf die Registerkarte **Dateien** klicken, werden alle Dateien angezeigt, die Sie in das Repository hochgeladen haben.
Weitere Einzelheiten zum Erstellen und Hochladen von Dateien in ein Repository finden Sie in der Hub-Dokumentation [hier](https://huggingface.co/docs/hub/how-to-upstream).
## Hochladen mit der Weboberflรคche
Benutzer, die einen no-code Ansatz bevorzugen, kรถnnen ein Modell รผber das Webinterface des Hubs hochladen. Besuchen Sie [huggingface.co/new](https://huggingface.co/new) um ein neues Repository zu erstellen:
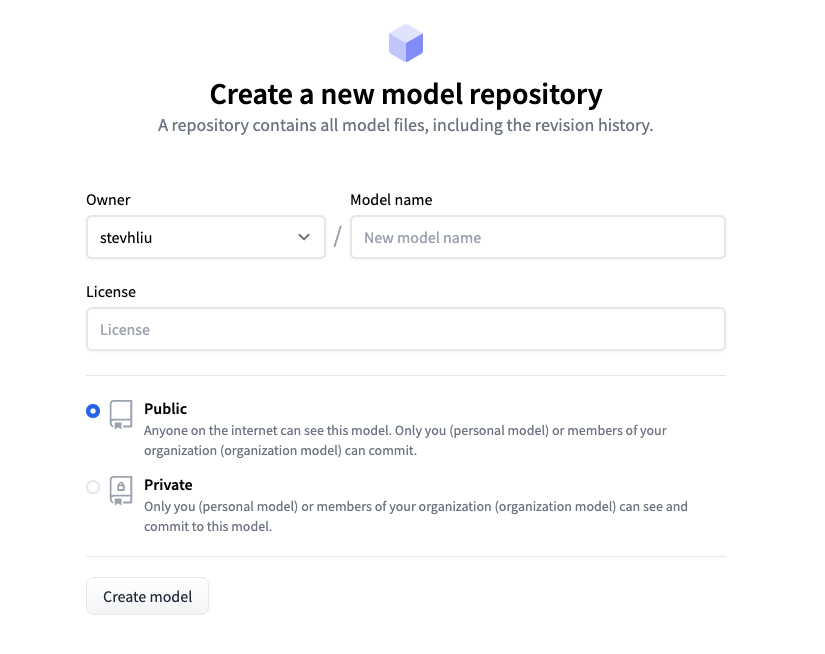
Fรผgen Sie von hier aus einige Informationen รผber Ihr Modell hinzu:
- Wรคhlen Sie den **Besitzer** des Repositorys. Dies kรถnnen Sie selbst oder eine der Organisationen sein, denen Sie angehรถren.
- Wรคhlen Sie einen Namen fรผr Ihr Modell, der auch der Name des Repositorys sein wird.
- Wรคhlen Sie, ob Ihr Modell รถffentlich oder privat ist.
- Geben Sie die Lizenzverwendung fรผr Ihr Modell an.
Klicken Sie nun auf die Registerkarte **Dateien** und klicken Sie auf die Schaltflรคche **Datei hinzufรผgen**, um eine neue Datei in Ihr Repository hochzuladen. Ziehen Sie dann eine Datei per Drag-and-Drop hoch und fรผgen Sie eine รbergabemeldung hinzu.
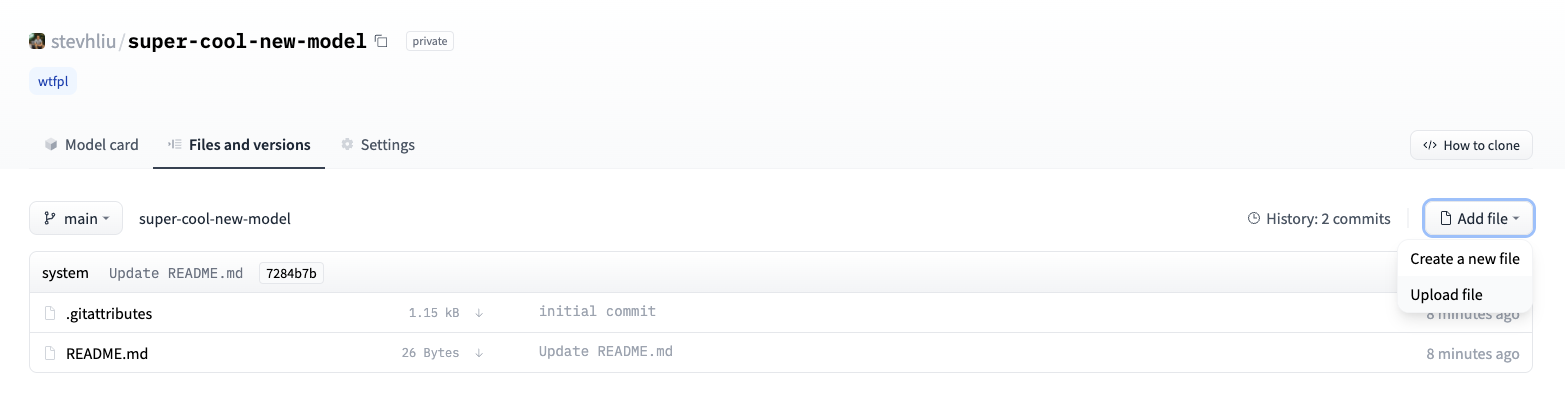
## Hinzufรผgen einer Modellkarte
Um sicherzustellen, dass die Benutzer die Fรคhigkeiten, Grenzen, mรถglichen Verzerrungen und ethischen Aspekte Ihres Modells verstehen, fรผgen Sie bitte eine Modellkarte zu Ihrem Repository hinzu. Die Modellkarte wird in der Datei `README.md` definiert. Sie kรถnnen eine Modellkarte hinzufรผgen, indem Sie:
* Manuelles Erstellen und Hochladen einer "README.md"-Datei.
* Klicken Sie auf die Schaltflรคche **Modellkarte bearbeiten** in Ihrem Modell-Repository.
Werfen Sie einen Blick auf die DistilBert [model card](https://huggingface.co/distilbert-base-uncased) als gutes Beispiel fรผr die Art von Informationen, die eine Modellkarte enthalten sollte. Weitere Details รผber andere Optionen, die Sie in der Datei "README.md" einstellen kรถnnen, wie z.B. den Kohlenstoff-Fuรabdruck eines Modells oder Beispiele fรผr Widgets, finden Sie in der Dokumentation [hier](https://huggingface.co/docs/hub/models-cards). | 0 |
hf_public_repos/transformers/docs/source | hf_public_repos/transformers/docs/source/de/pipeline_tutorial.md | <!--Copyright 2022 The HuggingFace Team. All rights reserved.
Licensed under the Apache License, Version 2.0 (the "License"); you may not use this file except in compliance with
the License. You may obtain a copy of the License at
http://www.apache.org/licenses/LICENSE-2.0
Unless required by applicable law or agreed to in writing, software distributed under the License is distributed on
an "AS IS" BASIS, WITHOUT WARRANTIES OR CONDITIONS OF ANY KIND, either express or implied. See the License for the
specific language governing permissions and limitations under the License.
โ ๏ธ Note that this file is in Markdown but contain specific syntax for our doc-builder (similar to MDX) that may not be
rendered properly in your Markdown viewer.
-->
# Pipelines fรผr Inferenzen
Die [`pipeline`] macht es einfach, jedes beliebige Modell aus dem [Hub](https://huggingface.co/models) fรผr die Inferenz auf jede Sprache, Computer Vision, Sprache und multimodale Aufgaben zu verwenden. Selbst wenn Sie keine Erfahrung mit einer bestimmten Modalitรคt haben oder nicht mit dem zugrundeliegenden Code hinter den Modellen vertraut sind, kรถnnen Sie sie mit der [`pipeline`] fรผr Inferenzen verwenden! In diesem Beispiel lernen Sie, wie:
* Eine [`pipeline`] fรผr Inferenz zu verwenden.
* Einen bestimmten Tokenizer oder ein bestimmtes Modell zu verwenden.
* Eine [`pipeline`] fรผr Audio-, Vision- und multimodale Aufgaben zu verwenden.
<Tip>
Eine vollstรคndige Liste der unterstรผtzten Aufgaben und verfรผgbaren Parameter finden Sie in der [`pipeline`]-Dokumentation.
</Tip>
## Verwendung von Pipelines
Obwohl jede Aufgabe eine zugehรถrige [`pipeline`] hat, ist es einfacher, die allgemeine [`pipeline`]-Abstraktion zu verwenden, die alle aufgabenspezifischen Pipelines enthรคlt. Die [`pipeline`] lรคdt automatisch ein Standardmodell und eine Vorverarbeitungsklasse, die fรผr Ihre Aufgabe inferenzfรคhig ist.
1. Beginnen Sie mit der Erstellung einer [`pipeline`] und geben Sie eine Inferenzaufgabe an:
```py
>>> from transformers import pipeline
>>> generator = pipeline(task="text-generation")
```
2. รbergeben Sie Ihren Eingabetext an die [`pipeline`]:
```py
>>> generator(
... "Three Rings for the Elven-kings under the sky, Seven for the Dwarf-lords in their halls of stone"
... ) # doctest: +SKIP
[{'generated_text': 'Three Rings for the Elven-kings under the sky, Seven for the Dwarf-lords in their halls of stone, Seven for the Iron-priests at the door to the east, and thirteen for the Lord Kings at the end of the mountain'}]
```
Wenn Sie mehr als eine Eingabe haben, รผbergeben Sie die Eingabe als Liste:
```py
>>> generator(
... [
... "Three Rings for the Elven-kings under the sky, Seven for the Dwarf-lords in their halls of stone",
... "Nine for Mortal Men, doomed to die, One for the Dark Lord on his dark throne",
... ]
... ) # doctest: +SKIP
```
Alle zusรคtzlichen Parameter fรผr Ihre Aufgabe kรถnnen auch in die [`pipeline`] aufgenommen werden. Die Aufgabe `Text-Generierung` hat eine [`~generation.GenerationMixin.generate`]-Methode mit mehreren Parametern zur Steuerung der Ausgabe. Wenn Sie zum Beispiel mehr als eine Ausgabe erzeugen wollen, setzen Sie den Parameter `num_return_sequences`:
```py
>>> generator(
... "Three Rings for the Elven-kings under the sky, Seven for the Dwarf-lords in their halls of stone",
... num_return_sequences=2,
... ) # doctest: +SKIP
```
### Wรคhlen Sie ein Modell und einen Tokenizer
Die [`pipeline`] akzeptiert jedes Modell aus dem [Hub] (https://huggingface.co/models). Auf dem Hub gibt es Tags, mit denen Sie nach einem Modell filtern kรถnnen, das Sie fรผr Ihre Aufgabe verwenden mรถchten. Sobald Sie ein passendes Modell ausgewรคhlt haben, laden Sie es mit der entsprechenden `AutoModelFor` und [`AutoTokenizer`] Klasse. Laden Sie zum Beispiel die Klasse [`AutoModelForCausalLM`] fรผr eine kausale Sprachmodellierungsaufgabe:
```py
>>> from transformers import AutoTokenizer, AutoModelForCausalLM
>>> tokenizer = AutoTokenizer.from_pretrained("distilgpt2")
>>> model = AutoModelForCausalLM.from_pretrained("distilgpt2")
```
Erstellen Sie eine [`pipeline`] fรผr Ihre Aufgabe, und geben Sie das Modell und den Tokenizer an, die Sie geladen haben:
```py
>>> from transformers import pipeline
>>> generator = pipeline(task="text-generation", model=model, tokenizer=tokenizer)
```
รbergeben Sie Ihren Eingabetext an die [`pipeline`] , um einen Text zu erzeugen:
```py
>>> generator(
... "Three Rings for the Elven-kings under the sky, Seven for the Dwarf-lords in their halls of stone"
... ) # doctest: +SKIP
[{'generated_text': 'Three Rings for the Elven-kings under the sky, Seven for the Dwarf-lords in their halls of stone, Seven for the Dragon-lords (for them to rule in a world ruled by their rulers, and all who live within the realm'}]
```
## Audio-Pipeline
Die [`pipeline`] unterstรผtzt auch Audioaufgaben wie Audioklassifizierung und automatische Spracherkennung.
Lassen Sie uns zum Beispiel die Emotion in diesem Audioclip klassifizieren:
```py
>>> from datasets import load_dataset
>>> import torch
>>> torch.manual_seed(42) # doctest: +IGNORE_RESULT
>>> ds = load_dataset("hf-internal-testing/librispeech_asr_demo", "clean", split="validation")
>>> audio_file = ds[0]["audio"]["path"]
```
Finden Sie ein [Audioklassifikation](https://huggingface.co/models?pipeline_tag=audio-classification) Modell auf dem Model Hub fรผr Emotionserkennung und laden Sie es in die [`pipeline`]:
```py
>>> from transformers import pipeline
>>> audio_classifier = pipeline(
... task="audio-classification", model="ehcalabres/wav2vec2-lg-xlsr-en-speech-emotion-recognition"
... )
```
รbergeben Sie die Audiodatei an die [`pipeline`]:
```py
>>> preds = audio_classifier(audio_file)
>>> preds = [{"score": round(pred["score"], 4), "label": pred["label"]} for pred in preds]
>>> preds
[{'score': 0.1315, 'label': 'calm'}, {'score': 0.1307, 'label': 'neutral'}, {'score': 0.1274, 'label': 'sad'}, {'score': 0.1261, 'label': 'fearful'}, {'score': 0.1242, 'label': 'happy'}]
```
## Bildverarbeitungs-Pipeline
Die Verwendung einer [`pipeline`] fรผr Bildverarbeitungsaufgaben ist praktisch identisch.
Geben Sie Ihre Aufgabe an und รผbergeben Sie Ihr Bild an den Klassifikator. Das Bild kann ein Link oder ein lokaler Pfad zu dem Bild sein. Zum Beispiel: Welche Katzenart ist unten abgebildet?

```py
>>> from transformers import pipeline
>>> vision_classifier = pipeline(task="image-classification")
>>> preds = vision_classifier(
... images="https://huggingface.co/datasets/huggingface/documentation-images/resolve/main/pipeline-cat-chonk.jpeg"
... )
>>> preds = [{"score": round(pred["score"], 4), "label": pred["label"]} for pred in preds]
>>> preds
[{'score': 0.4335, 'label': 'lynx, catamount'}, {'score': 0.0348, 'label': 'cougar, puma, catamount, mountain lion, painter, panther, Felis concolor'}, {'score': 0.0324, 'label': 'snow leopard, ounce, Panthera uncia'}, {'score': 0.0239, 'label': 'Egyptian cat'}, {'score': 0.0229, 'label': 'tiger cat'}]
```
## Multimodale Pipeline
Die [`pipeline`] unterstรผtzt mehr als eine Modalitรคt. Eine Aufgabe zur Beantwortung visueller Fragen (VQA) kombiniert zum Beispiel Text und Bild. Verwenden Sie einen beliebigen Bildlink und eine Frage, die Sie zu dem Bild stellen mรถchten. Das Bild kann eine URL oder ein lokaler Pfad zu dem Bild sein.
Wenn Sie zum Beispiel das gleiche Bild wie in der obigen Vision-Pipeline verwenden:
```py
>>> image = "https://huggingface.co/datasets/huggingface/documentation-images/resolve/main/pipeline-cat-chonk.jpeg"
>>> question = "Where is the cat?"
```
Erstellen Sie eine Pipeline fรผr "vqa" und รผbergeben Sie ihr das Bild und die Frage:
```py
>>> from transformers import pipeline
>>> vqa = pipeline(task="vqa")
>>> preds = vqa(image=image, question=question)
>>> preds = [{"score": round(pred["score"], 4), "answer": pred["answer"]} for pred in preds]
>>> preds
[{'score': 0.9112, 'answer': 'snow'}, {'score': 0.8796, 'answer': 'in snow'}, {'score': 0.6717, 'answer': 'outside'}, {'score': 0.0291, 'answer': 'on ground'}, {'score': 0.027, 'answer': 'ground'}]
```
| 0 |
hf_public_repos/transformers/docs/source | hf_public_repos/transformers/docs/source/de/_config.py | # docstyle-ignore
INSTALL_CONTENT = """
# Transformers installation
! pip install transformers datasets
# To install from source instead of the last release, comment the command above and uncomment the following one.
# ! pip install git+https://github.com/huggingface/transformers.git
"""
notebook_first_cells = [{"type": "code", "content": INSTALL_CONTENT}]
black_avoid_patterns = {
"{processor_class}": "FakeProcessorClass",
"{model_class}": "FakeModelClass",
"{object_class}": "FakeObjectClass",
}
| 0 |
hf_public_repos/transformers/docs/source | hf_public_repos/transformers/docs/source/de/accelerate.md | <!--Copyright 2022 The HuggingFace Team. All rights reserved.
Licensed under the Apache License, Version 2.0 (the "License"); you may not use this file except in compliance with
the License. You may obtain a copy of the License at
http://www.apache.org/licenses/LICENSE-2.0
Unless required by applicable law or agreed to in writing, software distributed under the License is distributed on
an "AS IS" BASIS, WITHOUT WARRANTIES OR CONDITIONS OF ANY KIND, either express or implied. See the License for the
specific language governing permissions and limitations under the License.
โ ๏ธ Note that this file is in Markdown but contain specific syntax for our doc-builder (similar to MDX) that may not be
rendered properly in your Markdown viewer.
-->
# Verteiltes Training mit ๐ค Accelerate
Da die Modelle immer grรถรer werden, hat sich die Parallelitรคt als Strategie zum Trainieren grรถรerer Modelle auf begrenzter Hardware und zur Beschleunigung der Trainingsgeschwindigkeit um mehrere Grรถรenordnungen erwiesen. Bei Hugging Face haben wir die Bibliothek [๐ค Accelerate](https://huggingface.co/docs/accelerate) entwickelt, um Nutzern zu helfen, ein ๐ค Transformers-Modell auf jeder Art von verteiltem Setup zu trainieren, egal ob es sich um mehrere GPUs auf einer Maschine oder mehrere GPUs auf mehreren Maschinen handelt. In diesem Tutorial lernen Sie, wie Sie Ihre native PyTorch-Trainingsschleife anpassen, um das Training in einer verteilten Umgebung zu ermรถglichen.
## Einrichtung
Beginnen Sie mit der Installation von ๐ค Accelerate:
```bash
pip install accelerate
```
Dann importieren und erstellen Sie ein [`~accelerate.Accelerator`]-Objekt. Der [`~accelerate.Accelerator`] wird automatisch Ihre Art der verteilten Einrichtung erkennen und alle notwendigen Komponenten fรผr das Training initialisieren. Sie mรผssen Ihr Modell nicht explizit auf einem Gerรคt platzieren.
```py
>>> from accelerate import Accelerator
>>> accelerator = Accelerator()
```
## Vorbereiten auf die Beschleunigung
Der nรคchste Schritt ist die รbergabe aller relevanten Trainingsobjekte an die Methode [`~accelerate.Accelerator.prepare`]. Dazu gehรถren Ihre Trainings- und Evaluierungs-DataLoader, ein Modell und ein Optimierer:
```py
>>> train_dataloader, eval_dataloader, model, optimizer = accelerator.prepare(
... train_dataloader, eval_dataloader, model, optimizer
... )
```
## Rรผckwรคrts
Die letzte Ergรคnzung besteht darin, das typische `loss.backward()` in der Trainingsschleife durch die ๐ค Accelerate-Methode [`~accelerate.Accelerator.backward`] zu ersetzen:
```py
>>> for epoch in range(num_epochs):
... for batch in train_dataloader:
... outputs = model(**batch)
... loss = outputs.loss
... accelerator.backward(loss)
... optimizer.step()
... lr_scheduler.step()
... optimizer.zero_grad()
... progress_bar.update(1)
```
Wie Sie im folgenden Code sehen kรถnnen, mรผssen Sie nur vier zusรคtzliche Codezeilen zu Ihrer Trainingsschleife hinzufรผgen, um verteiltes Training zu ermรถglichen!
```diff
+ from accelerate import Accelerator
from transformers import AdamW, AutoModelForSequenceClassification, get_scheduler
+ accelerator = Accelerator()
model = AutoModelForSequenceClassification.from_pretrained(checkpoint, num_labels=2)
optimizer = AdamW(model.parameters(), lr=3e-5)
- device = torch.device("cuda") if torch.cuda.is_available() else torch.device("cpu")
- model.to(device)
+ train_dataloader, eval_dataloader, model, optimizer = accelerator.prepare(
+ train_dataloader, eval_dataloader, model, optimizer
+ )
num_epochs = 3
num_training_steps = num_epochs * len(train_dataloader)
lr_scheduler = get_scheduler(
"linear",
optimizer=optimizer,
num_warmup_steps=0,
num_training_steps=num_training_steps
)
progress_bar = tqdm(range(num_training_steps))
model.train()
for epoch in range(num_epochs):
for batch in train_dataloader:
- batch = {k: v.to(device) for k, v in batch.items()}
outputs = model(**batch)
loss = outputs.loss
- loss.backward()
+ accelerator.backward(loss)
optimizer.step()
lr_scheduler.step()
optimizer.zero_grad()
progress_bar.update(1)
```
## Trainieren
Sobald Sie die entsprechenden Codezeilen hinzugefรผgt haben, starten Sie Ihr Training in einem Skript oder einem Notebook wie Colaboratory.
### Trainieren mit einem Skript
Wenn Sie Ihr Training mit einem Skript durchfรผhren, fรผhren Sie den folgenden Befehl aus, um eine Konfigurationsdatei zu erstellen und zu speichern:
```bash
accelerate config
```
Dann starten Sie Ihr Training mit:
```bash
accelerate launch train.py
```
### Trainieren mit einem Notebook
๐ค Accelerate kann auch in einem Notebook laufen, wenn Sie planen, die TPUs von Colaboratory zu verwenden. Verpacken Sie den gesamten Code, der fรผr das Training verantwortlich ist, in eine Funktion und รผbergeben Sie diese an [`~accelerate.notebook_launcher`]:
```py
>>> from accelerate import notebook_launcher
>>> notebook_launcher(training_function)
```
Weitere Informationen รผber ๐ค Accelerate und seine umfangreichen Funktionen finden Sie in der [Dokumentation](https://huggingface.co/docs/accelerate). | 0 |
hf_public_repos/transformers/docs/source | hf_public_repos/transformers/docs/source/de/training.md | <!--Copyright 2022 The HuggingFace Team. All rights reserved.
Licensed under the Apache License, Version 2.0 (the "License"); you may not use this file except in compliance with
the License. You may obtain a copy of the License at
http://www.apache.org/licenses/LICENSE-2.0
Unless required by applicable law or agreed to in writing, software distributed under the License is distributed on
an "AS IS" BASIS, WITHOUT WARRANTIES OR CONDITIONS OF ANY KIND, either express or implied. See the License for the
specific language governing permissions and limitations under the License.
โ ๏ธ Note that this file is in Markdown but contain specific syntax for our doc-builder (similar to MDX) that may not be
rendered properly in your Markdown viewer.
-->
# Optimierung eines vortrainierten Modells
[[open-in-colab]]
Die Verwendung eines vorab trainierten Modells hat erhebliche Vorteile. Es reduziert die Rechenkosten und den CO2-Fuรabdruck und ermรถglicht Ihnen die Verwendung von Modellen, die dem neuesten Stand der Technik entsprechen, ohne dass Sie ein Modell von Grund auf neu trainieren mรผssen. Transformers bietet Zugang zu Tausenden von vortrainierten Modellen fรผr eine Vielzahl von Aufgaben. Wenn Sie ein vorab trainiertes Modell verwenden, trainieren Sie es auf einem fรผr Ihre Aufgabe spezifischen Datensatz. Dies wird als Feinabstimmung bezeichnet und ist eine unglaublich leistungsfรคhige Trainingstechnik. In diesem Tutorial werden Sie ein vortrainiertes Modell mit einem Deep-Learning-Framework Ihrer Wahl feinabstimmen:
* Feinabstimmung eines vorab trainierten Modells mit ๐ค Transformers [`Trainer`].
* Feinabstimmung eines vorab trainierten Modells in TensorFlow mit Keras.
* Feinabstimmung eines vorab trainierten Modells in nativem PyTorch.
<a id='data-processing'></a>
## Vorbereitung eines Datensatzes
<Youtube id="_BZearw7f0w"/>
Bevor Sie die Feinabstimmung eines vortrainierten Modells vornehmen kรถnnen, mรผssen Sie einen Datensatz herunterladen und fรผr das Training vorbereiten. Im vorangegangenen Leitfaden haben Sie gelernt, wie man Daten fรผr das Training aufbereitet, und jetzt haben Sie die Gelegenheit, diese Fรคhigkeiten zu testen!
Laden Sie zunรคchst den Datensatz [Yelp Reviews](https://huggingface.co/datasets/yelp_review_full):
```py
>>> from datasets import load_dataset
>>> dataset = load_dataset("yelp_review_full")
>>> dataset["train"][100]
{'label': 0,
'text': 'My expectations for McDonalds are t rarely high. But for one to still fail so spectacularly...that takes something special!\\nThe cashier took my friends\'s order, then promptly ignored me. I had to force myself in front of a cashier who opened his register to wait on the person BEHIND me. I waited over five minutes for a gigantic order that included precisely one kid\'s meal. After watching two people who ordered after me be handed their food, I asked where mine was. The manager started yelling at the cashiers for \\"serving off their orders\\" when they didn\'t have their food. But neither cashier was anywhere near those controls, and the manager was the one serving food to customers and clearing the boards.\\nThe manager was rude when giving me my order. She didn\'t make sure that I had everything ON MY RECEIPT, and never even had the decency to apologize that I felt I was getting poor service.\\nI\'ve eaten at various McDonalds restaurants for over 30 years. I\'ve worked at more than one location. I expect bad days, bad moods, and the occasional mistake. But I have yet to have a decent experience at this store. It will remain a place I avoid unless someone in my party needs to avoid illness from low blood sugar. Perhaps I should go back to the racially biased service of Steak n Shake instead!'}
```
Wie Sie nun wissen, benรถtigen Sie einen Tokenizer, um den Text zu verarbeiten und eine Auffรผll- und Abschneidungsstrategie einzubauen, um mit variablen Sequenzlรคngen umzugehen. Um Ihren Datensatz in einem Schritt zu verarbeiten, verwenden Sie die ๐ค Methode Datasets [`map`](https://huggingface.co/docs/datasets/process.html#map), um eine Vorverarbeitungsfunktion auf den gesamten Datensatz anzuwenden:
```py
>>> from transformers import AutoTokenizer
>>> tokenizer = AutoTokenizer.from_pretrained("bert-base-cased")
>>> def tokenize_function(examples):
... return tokenizer(examples["text"], padding="max_length", truncation=True)
>>> tokenized_datasets = dataset.map(tokenize_function, batched=True)
```
Wenn Sie mรถchten, kรถnnen Sie eine kleinere Teilmenge des gesamten Datensatzes fรผr die Feinabstimmung erstellen, um den Zeitaufwand zu verringern:
```py
>>> small_train_dataset = tokenized_datasets["train"].shuffle(seed=42).select(range(1000))
>>> small_eval_dataset = tokenized_datasets["test"].shuffle(seed=42).select(range(1000))
```
<a id='trainer'></a>
## Training
An dieser Stelle sollten Sie dem Abschnitt folgen, der dem Rahmen entspricht, den Sie verwenden mรถchten. Sie kรถnnen รผber die Links
in der rechten Seitenleiste kรถnnen Sie zu dem gewรผnschten Abschnitt springen - und wenn Sie den gesamten Inhalt eines bestimmten Frameworks ausblenden mรถchten,
klicken Sie einfach auf die Schaltflรคche oben rechts im Block des jeweiligen Frameworks!
<frameworkcontent>
<pt>
<Youtube id="nvBXf7s7vTI"/>
## Trainieren mit PyTorch Trainer
๐ค Transformers bietet eine [`Trainer`]-Klasse, die fรผr das Training von ๐ค Transformers-Modellen optimiert ist und es einfacher macht, mit dem Training zu beginnen, ohne manuell eine eigene Trainingsschleife zu schreiben. Die [`Trainer`]-API unterstรผtzt eine breite Palette von Trainingsoptionen und Funktionen wie Logging, Gradientenakkumulation und gemischte Prรคzision.
Beginnen Sie mit dem Laden Ihres Modells und geben Sie die Anzahl der erwarteten Labels an. Aus dem Yelp Review [dataset card](https://huggingface.co/datasets/yelp_review_full#data-fields) wissen Sie, dass es fรผnf Labels gibt:
```py
>>> from transformers import AutoModelForSequenceClassification
>>> model = AutoModelForSequenceClassification.from_pretrained("bert-base-cased", num_labels=5)
```
<Tip>
Es wird eine Warnung angezeigt, dass einige der trainierten Parameter nicht verwendet werden und einige Parameter zufรคllig
initialisiert werden. Machen Sie sich keine Sorgen, das ist vรถllig normal! Der vorher trainierte Kopf des BERT-Modells wird verworfen und durch einen zufรคllig initialisierten Klassifikationskopf ersetzt. Sie werden diesen neuen Modellkopf in Ihrer Sequenzklassifizierungsaufgabe feinabstimmen, indem Sie das Wissen des vortrainierten Modells auf ihn รผbertragen.
</Tip>
### Hyperparameter fรผr das Training
Als Nรคchstes erstellen Sie eine Klasse [`TrainingArguments`], die alle Hyperparameter enthรคlt, die Sie einstellen kรถnnen, sowie Flags zur Aktivierung verschiedener Trainingsoptionen. Fรผr dieses Lernprogramm kรถnnen Sie mit den Standard- [Hyperparametern](https://huggingface.co/docs/transformers/main_classes/trainer#transformers.TrainingArguments) beginnen, aber Sie kรถnnen mit diesen experimentieren, um Ihre optimalen Einstellungen zu finden.
Geben Sie an, wo die Kontrollpunkte Ihres Trainings gespeichert werden sollen:
```py
>>> from transformers import TrainingArguments
>>> training_args = TrainingArguments(output_dir="test_trainer")
```
### Auswerten
Der [`Trainer`] wertet die Leistung des Modells wรคhrend des Trainings nicht automatisch aus. Sie mรผssen [`Trainer`] eine Funktion รผbergeben, um Metriken zu berechnen und zu berichten. Die [๐ค Evaluate](https://huggingface.co/docs/evaluate/index) Bibliothek bietet eine einfache [`accuracy`](https://huggingface.co/spaces/evaluate-metric/accuracy) Funktion, die Sie mit der [`evaluate.load`] Funktion laden kรถnnen (siehe diese [quicktour](https://huggingface.co/docs/evaluate/a_quick_tour) fรผr weitere Informationen):
```py
>>> import numpy as np
>>> import evaluate
>>> metric = evaluate.load("accuracy")
```
Rufen Sie [`~evaluate.compute`] auf `metric` auf, um die Genauigkeit Ihrer Vorhersagen zu berechnen. Bevor Sie Ihre Vorhersagen an `compute` รผbergeben, mรผssen Sie die Vorhersagen in Logits umwandeln (denken Sie daran, dass alle ๐ค Transformers-Modelle Logits zurรผckgeben):
```py
>>> def compute_metrics(eval_pred):
... logits, labels = eval_pred
... predictions = np.argmax(logits, axis=-1)
... return metric.compute(predictions=predictions, references=labels)
```
Wenn Sie Ihre Bewertungsmetriken wรคhrend der Feinabstimmung รผberwachen mรถchten, geben Sie den Parameter `evaluation_strategy` in Ihren Trainingsargumenten an, um die Bewertungsmetrik am Ende jeder Epoche zu ermitteln:
```py
>>> from transformers import TrainingArguments, Trainer
>>> training_args = TrainingArguments(output_dir="test_trainer", evaluation_strategy="epoch")
```
### Trainer
Erstellen Sie ein [`Trainer`]-Objekt mit Ihrem Modell, Trainingsargumenten, Trainings- und Testdatensรคtzen und einer Evaluierungsfunktion:
```py
>>> trainer = Trainer(
... model=model,
... args=training_args,
... train_dataset=small_train_dataset,
... eval_dataset=small_eval_dataset,
... compute_metrics=compute_metrics,
... )
```
Anschlieรend kรถnnen Sie Ihr Modell durch den Aufruf von [`~transformers.Trainer.train`] optimieren:
```py
>>> trainer.train()
```
</pt>
<tf>
<a id='keras'></a>
<Youtube id="rnTGBy2ax1c"/>
## Trainieren Sie ein TensorFlow-Modell mit Keras
Sie kรถnnen auch ๐ค Transformers Modelle in TensorFlow mit der Keras API trainieren!
### Laden von Daten fรผr Keras
Wenn Sie ein ๐ค Transformers Modell mit der Keras API trainieren wollen, mรผssen Sie Ihren Datensatz in ein Format konvertieren, das
Keras versteht. Wenn Ihr Datensatz klein ist, kรถnnen Sie das Ganze einfach in NumPy-Arrays konvertieren und an Keras รผbergeben.
Probieren wir das zuerst aus, bevor wir etwas Komplizierteres tun.
Laden Sie zunรคchst ein Dataset. Wir werden den CoLA-Datensatz aus dem [GLUE-Benchmark](https://huggingface.co/datasets/glue) verwenden,
da es sich um eine einfache Aufgabe zur Klassifizierung von binรคrem Text handelt, und nehmen vorerst nur den Trainingssplit.
```py
from datasets import load_dataset
dataset = load_dataset("glue", "cola")
dataset = dataset["train"] # Just take the training split for now
```
Als nรคchstes laden Sie einen Tokenizer und tokenisieren die Daten als NumPy-Arrays. Beachten Sie, dass die Beschriftungen bereits eine Liste von 0 und 1en sind,
Wir kรถnnen sie also ohne Tokenisierung direkt in ein NumPy-Array konvertieren!
```py
from transformers import AutoTokenizer
tokenizer = AutoTokenizer.from_pretrained("bert-base-cased")
tokenized_data = tokenizer(dataset["text"], return_tensors="np", padding=True)
# Tokenizer returns a BatchEncoding, but we convert that to a dict for Keras
tokenized_data = dict(tokenized_data)
labels = np.array(dataset["label"]) # Label is already an array of 0 and 1
```
Schlieรlich laden, [`compile`](https://keras.io/api/models/model_training_apis/#compile-method) und [`fit`](https://keras.io/api/models/model_training_apis/#fit-method) Sie das Modell:
```py
from transformers import TFAutoModelForSequenceClassification
from tensorflow.keras.optimizers import Adam
# Load and compile our model
model = TFAutoModelForSequenceClassification.from_pretrained("bert-base-cased")
# Lower learning rates are often better for fine-tuning transformers
model.compile(optimizer=Adam(3e-5))
model.fit(tokenized_data, labels)
```
<Tip>
Sie mรผssen Ihren Modellen kein Verlustargument รผbergeben, wenn Sie sie `compile()`! Hugging-Face-Modelle wรคhlen automatisch
einen Loss, der fรผr ihre Aufgabe und Modellarchitektur geeignet ist, wenn dieses Argument leer gelassen wird. Sie kรถnnen jederzeit auรer Kraft setzen, indem Sie selbst einen Loss angeben, wenn Sie das mรถchten!
</Tip>
Dieser Ansatz eignet sich hervorragend fรผr kleinere Datensรคtze, aber bei grรถรeren Datensรคtzen kann er zu einem Problem werden. Warum?
Weil das tokenisierte Array und die Beschriftungen vollstรคndig in den Speicher geladen werden mรผssten, und weil NumPy nicht mit
"gezackte" Arrays nicht verarbeiten kann, so dass jedes tokenisierte Sample auf die Lรคnge des lรคngsten Samples im gesamten Datensatz aufgefรผllt werden mรผsste.
Datensatzes aufgefรผllt werden. Dadurch wird das Array noch grรถรer, und all die aufgefรผllten Token verlangsamen auch das Training!
### Laden von Daten als tf.data.Dataset
Wenn Sie eine Verlangsamung des Trainings vermeiden wollen, kรถnnen Sie Ihre Daten stattdessen als `tf.data.Dataset` laden. Sie kรถnnen zwar Ihre eigene
tf.data"-Pipeline schreiben kรถnnen, wenn Sie wollen, haben wir zwei bequeme Methoden, um dies zu tun:
- [`~TFPreTrainedModel.prepare_tf_dataset`]: Dies ist die Methode, die wir in den meisten Fรคllen empfehlen. Da es sich um eine Methode
Ihres Modells ist, kann sie das Modell inspizieren, um automatisch herauszufinden, welche Spalten als Modelleingaben verwendet werden kรถnnen, und
verwirft die anderen, um einen einfacheren, leistungsfรคhigeren Datensatz zu erstellen.
- [~datasets.Dataset.to_tf_dataset`]: Diese Methode ist eher auf niedriger Ebene angesiedelt und ist nรผtzlich, wenn Sie genau kontrollieren wollen, wie
Dataset erstellt wird, indem man genau angibt, welche `columns` und `label_cols` einbezogen werden sollen.
Bevor Sie [~TFPreTrainedModel.prepare_tf_dataset`] verwenden kรถnnen, mรผssen Sie die Tokenizer-Ausgaben als Spalten zu Ihrem Datensatz hinzufรผgen, wie in
dem folgenden Codebeispiel:
```py
def tokenize_dataset(data):
# Keys of the returned dictionary will be added to the dataset as columns
return tokenizer(data["text"])
dataset = dataset.map(tokenize_dataset)
```
Denken Sie daran, dass Hugging Face-Datensรคtze standardmรครig auf der Festplatte gespeichert werden, so dass dies nicht zu einem erhรถhten Arbeitsspeicherbedarf fรผhren wird! Sobald die
Spalten hinzugefรผgt wurden, kรถnnen Sie Batches aus dem Datensatz streamen und zu jedem Batch Auffรผllungen hinzufรผgen, was die Anzahl der Auffรผllungs-Token im Vergleich zum Auffรผllen des gesamten Datensatzes reduziert.
```py
>>> tf_dataset = model.prepare_tf_dataset(dataset, batch_size=16, shuffle=True, tokenizer=tokenizer)
```
Beachten Sie, dass Sie im obigen Codebeispiel den Tokenizer an `prepare_tf_dataset` รผbergeben mรผssen, damit die Stapel beim Laden korrekt aufgefรผllt werden kรถnnen.
Wenn alle Stichproben in Ihrem Datensatz die gleiche Lรคnge haben und kein Auffรผllen erforderlich ist, kรถnnen Sie dieses Argument weglassen.
Wenn Sie etwas Komplexeres als nur das Auffรผllen von Stichproben benรถtigen (z. B. das Korrumpieren von Token fรผr die maskierte Sprachmodellierung), kรถnnen Sie das Argument
Modellierung), kรถnnen Sie stattdessen das Argument `collate_fn` verwenden, um eine Funktion zu รผbergeben, die aufgerufen wird, um die
Liste von Stichproben in einen Stapel umwandelt und alle gewรผnschten Vorverarbeitungen vornimmt. Siehe unsere
[examples](https://github.com/huggingface/transformers/tree/main/examples) oder
[notebooks](https://huggingface.co/docs/transformers/notebooks), um diesen Ansatz in Aktion zu sehen.
Sobald Sie einen `tf.data.Dataset` erstellt haben, kรถnnen Sie das Modell wie zuvor kompilieren und anpassen:
```py
model.compile(optimizer=Adam(3e-5))
model.fit(tf_dataset)
```
</tf>
</frameworkcontent>
<a id='pytorch_native'></a>
## Trainieren in nativem PyTorch
<frameworkcontent>
<pt>
<Youtube id="Dh9CL8fyG80"/>
[`Trainer`] kรผmmert sich um die Trainingsschleife und ermรถglicht die Feinabstimmung eines Modells in einer einzigen Codezeile. Fรผr Benutzer, die es vorziehen, ihre eigene Trainingsschleife zu schreiben, kรถnnen Sie auch eine Feinabstimmung eines ๐ค Transformers-Modells in nativem PyTorch vornehmen.
An diesem Punkt mรผssen Sie mรถglicherweise Ihr Notebook neu starten oder den folgenden Code ausfรผhren, um etwas Speicher freizugeben:
```py
del model
del pytorch_model
del trainer
torch.cuda.empty_cache()
```
Als Nรคchstes mรผssen Sie den Datensatz `tokenized_dataset` manuell nachbearbeiten, um ihn fรผr das Training vorzubereiten.
1. Entfernen Sie die Spalte "Text", da das Modell keinen Rohtext als Eingabe akzeptiert:
```py
>>> tokenized_datasets = tokenized_datasets.remove_columns(["text"])
```
2. Benennen Sie die Spalte "Label" in "Labels" um, da das Modell erwartet, dass das Argument "Labels" genannt wird:
```py
>>> tokenized_datasets = tokenized_datasets.rename_column("label", "labels")
```
3. Stellen Sie das Format des Datensatzes so ein, dass PyTorch-Tensoren anstelle von Listen zurรผckgegeben werden:
```py
>>> tokenized_datasets.set_format("torch")
```
Erstellen Sie dann eine kleinere Teilmenge des Datensatzes, wie zuvor gezeigt, um die Feinabstimmung zu beschleunigen:
```py
>>> small_train_dataset = tokenized_datasets["train"].shuffle(seed=42).select(range(1000))
>>> small_eval_dataset = tokenized_datasets["test"].shuffle(seed=42).select(range(1000))
```
### DataLoader
Erstellen Sie einen `DataLoader` fรผr Ihre Trainings- und Testdatensรคtze, damit Sie รผber die Datenstapel iterieren kรถnnen:
```py
>>> from torch.utils.data import DataLoader
>>> train_dataloader = DataLoader(small_train_dataset, shuffle=True, batch_size=8)
>>> eval_dataloader = DataLoader(small_eval_dataset, batch_size=8)
```
Laden Sie Ihr Modell mit der Anzahl der erwarteten Kennzeichnungen:
```py
>>> from transformers import AutoModelForSequenceClassification
>>> model = AutoModelForSequenceClassification.from_pretrained("bert-base-cased", num_labels=5)
```
### Optimierer und Lernratensteuerung
Erstellen Sie einen Optimierer und einen Scheduler fรผr die Lernrate, um das Modell fein abzustimmen. Wir verwenden den Optimierer [`AdamW`](https://pytorch.org/docs/stable/generated/torch.optim.AdamW.html) aus PyTorch:
```py
>>> from torch.optim import AdamW
>>> optimizer = AdamW(model.parameters(), lr=5e-5)
```
Erstellen Sie den Standard-Lernratenplaner aus [`Trainer`]:
```py
>>> from transformers import get_scheduler
>>> num_epochs = 3
>>> num_training_steps = num_epochs * len(train_dataloader)
>>> lr_scheduler = get_scheduler(
... name="linear", optimizer=optimizer, num_warmup_steps=0, num_training_steps=num_training_steps
... )
```
Geben Sie schlieรlich `device` an, um einen Grafikprozessor zu verwenden, wenn Sie Zugang zu einem solchen haben. Andernfalls kann das Training auf einer CPU mehrere Stunden statt ein paar Minuten dauern.
```py
>>> import torch
>>> device = torch.device("cuda") if torch.cuda.is_available() else torch.device("cpu")
>>> model.to(device)
```
<Tip>
Holen Sie sich mit einem gehosteten Notebook wie [Colaboratory](https://colab.research.google.com/) oder [SageMaker StudioLab](https://studiolab.sagemaker.aws/) kostenlosen Zugang zu einem Cloud-GPU, wenn Sie noch keinen haben.
</Tip>
Groรartig, Sie sind bereit fรผr das Training! ๐ฅณ
### Trainingsschleife
Um Ihren Trainingsfortschritt zu verfolgen, verwenden Sie die [tqdm](https://tqdm.github.io/) Bibliothek, um einen Fortschrittsbalken รผber die Anzahl der Trainingsschritte hinzuzufรผgen:
```py
>>> from tqdm.auto import tqdm
>>> progress_bar = tqdm(range(num_training_steps))
>>> model.train()
>>> for epoch in range(num_epochs):
... for batch in train_dataloader:
... batch = {k: v.to(device) for k, v in batch.items()}
... outputs = model(**batch)
... loss = outputs.loss
... loss.backward()
... optimizer.step()
... lr_scheduler.step()
... optimizer.zero_grad()
... progress_bar.update(1)
```
### Auswertung
Genauso wie Sie eine Bewertungsfunktion zu [`Trainer`] hinzugefรผgt haben, mรผssen Sie dasselbe tun, wenn Sie Ihre eigene Trainingsschleife schreiben. Aber anstatt die Metrik am Ende jeder Epoche zu berechnen und zu melden, werden Sie dieses Mal alle Stapel mit [`~evaluate.add_batch`] akkumulieren und die Metrik ganz am Ende berechnen.
```py
>>> import evaluate
>>> metric = evaluate.load("accuracy")
>>> model.eval()
>>> for batch in eval_dataloader:
... batch = {k: v.to(device) for k, v in batch.items()}
... with torch.no_grad():
... outputs = model(**batch)
... logits = outputs.logits
... predictions = torch.argmax(logits, dim=-1)
... metric.add_batch(predictions=predictions, references=batch["labels"])
>>> metric.compute()
```
</pt>
</frameworkcontent>
<a id='additional-resources'></a>
## Zusรคtzliche Ressourcen
Weitere Beispiele fรผr die Feinabstimmung finden Sie unter:
- [๐ค Transformers Examples](https://github.com/huggingface/transformers/tree/main/examples) enthรคlt Skripte
um gรคngige NLP-Aufgaben in PyTorch und TensorFlow zu trainieren.
- [๐ค Transformers Notebooks](notebooks) enthรคlt verschiedene Notebooks zur Feinabstimmung eines Modells fรผr bestimmte Aufgaben in PyTorch und TensorFlow. | 0 |
hf_public_repos/transformers/docs/source | hf_public_repos/transformers/docs/source/it/autoclass_tutorial.md | <!--Copyright 2022 The HuggingFace Team. All rights reserved.
Licensed under the Apache License, Version 2.0 (the "License"); you may not use this file except in compliance with
the License. You may obtain a copy of the License at
http://www.apache.org/licenses/LICENSE-2.0
Unless required by applicable law or agreed to in writing, software distributed under the License is distributed on
an "AS IS" BASIS, WITHOUT WARRANTIES OR CONDITIONS OF ANY KIND, either express or implied. See the License for the
specific language governing permissions and limitations under the License.
โ ๏ธ Note that this file is in Markdown but contain specific syntax for our doc-builder (similar to MDX) that may not be
rendered properly in your Markdown viewer.
-->
# Carica istanze pre-allenate con AutoClass
Con cosรฌ tante architetture Transformer differenti, puรฒ essere sfidante crearne una per il tuo checkpoint. Come parte della filosofia centrale di ๐ค Transformers per rendere la libreria facile, semplice e flessibile da utilizzare, una `AutoClass` inferisce e carica automaticamente l'architettura corretta da un dato checkpoint. Il metodo `from_pretrained` ti permette di caricare velocemente un modello pre-allenato per qualsiasi architettura, cosรฌ non devi utilizzare tempo e risorse per allenare un modello da zero. Produrre questo codice agnostico ai checkpoint significa che se il tuo codice funziona per un checkpoint, funzionerร anche per un altro checkpoint, purchรฉ sia stato allenato per un compito simile, anche se l'architettura รจ differente.
<Tip>
Ricorda, con architettura ci si riferisce allo scheletro del modello e con checkpoint ai pesi di una determinata architettura. Per esempio, [BERT](https://huggingface.co/bert-base-uncased) รจ un'architettura, mentre `bert-base-uncased` รจ un checkpoint. Modello รจ un termine generale che puรฒ significare sia architettura che checkpoint.
</Tip>
In questo tutorial, imparerai a:
* Caricare un tokenizer pre-allenato.
* Caricare un estrattore di caratteristiche (feature extractor, in inglese) pre-allenato.
* Caricare un processore pre-allenato.
* Caricare un modello pre-allenato.
## AutoTokenizer
Quasi tutti i compiti di NLP iniziano con un tokenizer. Un tokenizer converte il tuo input in un formato che possa essere elaborato dal modello.
Carica un tokenizer con [`AutoTokenizer.from_pretrained`]:
```py
>>> from transformers import AutoTokenizer
>>> tokenizer = AutoTokenizer.from_pretrained("xlm-roberta-base")
```
Poi tokenizza il tuo input come mostrato in seguito:
```py
>>> sequenza = "In un buco nel terreno viveva uno Hobbit."
>>> print(tokenizer(sequenza))
{'input_ids': [0, 360, 51, 373, 587, 1718, 54644, 22597, 330, 3269, 2291, 22155, 18, 5, 2],
'attention_mask': [1, 1, 1, 1, 1, 1, 1, 1, 1, 1, 1, 1, 1, 1, 1]}
```
## AutoFeatureExtractor
Per compiti inerenti a audio e video, un feature extractor processa il segnale audio o l'immagine nel formato di input corretto.
Carica un feature extractor con [`AutoFeatureExtractor.from_pretrained`]:
```py
>>> from transformers import AutoFeatureExtractor
>>> feature_extractor = AutoFeatureExtractor.from_pretrained(
... "ehcalabres/wav2vec2-lg-xlsr-en-speech-emotion-recognition"
... )
```
## AutoProcessor
Compiti multimodali richiedono un processore che combini i due tipi di strumenti di elaborazione. Per esempio, il modello [LayoutLMV2](model_doc/layoutlmv2) richiede un feature extractor per gestire le immagine e un tokenizer per gestire il testo; un processore li combina entrambi.
Carica un processore con [`AutoProcessor.from_pretrained`]:
```py
>>> from transformers import AutoProcessor
>>> processor = AutoProcessor.from_pretrained("microsoft/layoutlmv2-base-uncased")
```
## AutoModel
<frameworkcontent>
<pt>
Infine, le classi `AutoModelFor` ti permettono di caricare un modello pre-allenato per un determinato compito (guarda [qui](model_doc/auto) per una lista completa di compiti presenti). Per esempio, carica un modello per la classificazione di sequenze con [`AutoModelForSequenceClassification.from_pretrained`]:
```py
>>> from transformers import AutoModelForSequenceClassification
>>> model = AutoModelForSequenceClassification.from_pretrained("distilbert-base-uncased")
```
Semplicemente utilizza lo stesso checkpoint per caricare un'architettura per un task differente:
```py
>>> from transformers import AutoModelForTokenClassification
>>> model = AutoModelForTokenClassification.from_pretrained("distilbert-base-uncased")
```
Generalmente, raccomandiamo di utilizzare la classe `AutoTokenizer` e la classe `AutoModelFor` per caricare istanze pre-allenate dei modelli. Questo ti assicurerร di aver caricato la corretta architettura ogni volta. Nel prossimo [tutorial](preprocessing), imparerai come utilizzare il tokenizer, il feature extractor e il processore per elaborare un dataset per il fine-tuning.
</pt>
<tf>
Infine, le classi `TFAutoModelFor` ti permettono di caricare un modello pre-allenato per un determinato compito (guarda [qui](model_doc/auto) per una lista completa di compiti presenti). Per esempio, carica un modello per la classificazione di sequenze con [`TFAutoModelForSequenceClassification.from_pretrained`]:
```py
>>> from transformers import TFAutoModelForSequenceClassification
>>> model = TFAutoModelForSequenceClassification.from_pretrained("distilbert-base-uncased")
```
Semplicemente utilizza lo stesso checkpoint per caricare un'architettura per un task differente:
```py
>>> from transformers import TFAutoModelForTokenClassification
>>> model = TFAutoModelForTokenClassification.from_pretrained("distilbert-base-uncased")
```
Generalmente, raccomandiamo di utilizzare la classe `AutoTokenizer` e la classe `TFAutoModelFor` per caricare istanze pre-allenate dei modelli. Questo ti assicurerร di aver caricato la corretta architettura ogni volta. Nel prossimo [tutorial](preprocessing), imparerai come utilizzare il tokenizer, il feature extractor e il processore per elaborare un dataset per il fine-tuning.
</tf>
</frameworkcontent>
| 0 |
hf_public_repos/transformers/docs/source | hf_public_repos/transformers/docs/source/it/converting_tensorflow_models.md | <!--Copyright 2020 The HuggingFace Team. All rights reserved.
Licensed under the Apache License, Version 2.0 (the "License"); you may not use this file except in compliance with
the License. You may obtain a copy of the License at
http://www.apache.org/licenses/LICENSE-2.0
Unless required by applicable law or agreed to in writing, software distributed under the License is distributed on
an "AS IS" BASIS, WITHOUT WARRANTIES OR CONDITIONS OF ANY KIND, either express or implied. See the License for the
specific language governing permissions and limitations under the License.
โ ๏ธ Note that this file is in Markdown but contain specific syntax for our doc-builder (similar to MDX) that may not be
rendered properly in your Markdown viewer.
-->
# Convertire checkpoint di Tensorflow
ร disponibile un'interfaccia a linea di comando per convertire gli originali checkpoint di Bert/GPT/GPT-2/Transformer-XL/XLNet/XLM
in modelli che possono essere caricati utilizzando i metodi `from_pretrained` della libreria.
<Tip>
A partire dalla versione 2.3.0 lo script di conversione รจ parte di transformers CLI (**transformers-cli**), disponibile in ogni installazione
di transformers >=2.3.0.
La seguente documentazione riflette il formato dei comandi di **transformers-cli convert**.
</Tip>
## BERT
Puoi convertire qualunque checkpoint Tensorflow di BERT (in particolare
[i modeli pre-allenati rilasciati da Google](https://github.com/google-research/bert#pre-trained-models))
in un file di salvataggio Pytorch utilizzando lo script
[convert_bert_original_tf_checkpoint_to_pytorch.py](https://github.com/huggingface/transformers/tree/main/src/transformers/models/bert/convert_bert_original_tf_checkpoint_to_pytorch.py).
Questo CLI prende come input un checkpoint di Tensorflow (tre files che iniziano con `bert_model.ckpt`) ed il relativo
file di configurazione (`bert_config.json`), crea un modello Pytorch per questa configurazione, carica i pesi dal
checkpoint di Tensorflow nel modello di Pytorch e salva il modello che ne risulta in un file di salvataggio standard di Pytorch che
puรฒ essere importato utilizzando `from_pretrained()` (vedi l'esempio nel
[quicktour](quicktour) , [run_glue.py](https://github.com/huggingface/transformers/tree/main/examples/pytorch/text-classification/run_glue.py) ).
Devi soltanto lanciare questo script di conversione **una volta** per ottenere un modello Pytorch. Dopodichรจ, potrai tralasciare
il checkpoint di Tensorflow (i tre files che iniziano con `bert_model.ckpt`), ma assicurati di tenere il file di configurazione
(`bert_config.json`) ed il file di vocabolario (`vocab.txt`) in quanto queste componenti sono necessarie anche per il modello di Pytorch.
Per lanciare questo specifico script di conversione avrai bisogno di un'installazione di Tensorflow e di Pytorch
(`pip install tensorflow`). Il resto della repository richiede soltanto Pytorch.
Questo รจ un esempio del processo di conversione per un modello `BERT-Base Uncased` pre-allenato:
```bash
export BERT_BASE_DIR=/path/to/bert/uncased_L-12_H-768_A-12
transformers-cli convert --model_type bert \
--tf_checkpoint $BERT_BASE_DIR/bert_model.ckpt \
--config $BERT_BASE_DIR/bert_config.json \
--pytorch_dump_output $BERT_BASE_DIR/pytorch_model.bin
```
Puoi scaricare i modelli pre-allenati di Google per la conversione [qua](https://github.com/google-research/bert#pre-trained-models).
## ALBERT
Per il modello ALBERT, converti checkpoint di Tensoflow in Pytorch utilizzando lo script
[convert_albert_original_tf_checkpoint_to_pytorch.py](https://github.com/huggingface/transformers/tree/main/src/transformers/models/albert/convert_albert_original_tf_checkpoint_to_pytorch.py).
Il CLI prende come input un checkpoint di Tensorflow (tre files che iniziano con `model.ckpt-best`) e i relativi file di
configurazione (`albert_config.json`), dopodichรจ crea e salva un modello Pytorch. Per lanciare questa conversione
avrai bisogno di un'installazione di Tensorflow e di Pytorch.
Ecco un esempio del procedimento di conversione di un modello `ALBERT Base` pre-allenato:
```bash
export ALBERT_BASE_DIR=/path/to/albert/albert_base
transformers-cli convert --model_type albert \
--tf_checkpoint $ALBERT_BASE_DIR/model.ckpt-best \
--config $ALBERT_BASE_DIR/albert_config.json \
--pytorch_dump_output $ALBERT_BASE_DIR/pytorch_model.bin
```
Puoi scaricare i modelli pre-allenati di Google per la conversione [qui](https://github.com/google-research/albert#pre-trained-models).
## OpenAI GPT
Ecco un esempio del processo di conversione di un modello OpenAI GPT pre-allenato, assumendo che il tuo checkpoint di NumPy
sia salvato nello stesso formato dei modelli pre-allenati OpenAI (vedi [qui](https://github.com/openai/finetune-transformer-lm)):
```bash
export OPENAI_GPT_CHECKPOINT_FOLDER_PATH=/path/to/openai/pretrained/numpy/weights
transformers-cli convert --model_type gpt \
--tf_checkpoint $OPENAI_GPT_CHECKPOINT_FOLDER_PATH \
--pytorch_dump_output $PYTORCH_DUMP_OUTPUT \
[--config OPENAI_GPT_CONFIG] \
[--finetuning_task_name OPENAI_GPT_FINETUNED_TASK] \
```
## OpenAI GPT-2
Ecco un esempio del processo di conversione di un modello OpenAI GPT-2 pre-allenato (vedi [qui](https://github.com/openai/gpt-2)):
```bash
export OPENAI_GPT2_CHECKPOINT_PATH=/path/to/gpt2/pretrained/weights
transformers-cli convert --model_type gpt2 \
--tf_checkpoint $OPENAI_GPT2_CHECKPOINT_PATH \
--pytorch_dump_output $PYTORCH_DUMP_OUTPUT \
[--config OPENAI_GPT2_CONFIG] \
[--finetuning_task_name OPENAI_GPT2_FINETUNED_TASK]
```
## Transformer-XL
Ecco un esempio del processo di conversione di un modello Transformer-XL pre-allenato
(vedi [qui](https://github.com/kimiyoung/transformer-xl/tree/master/tf#obtain-and-evaluate-pretrained-sota-models)):
```bash
export TRANSFO_XL_CHECKPOINT_FOLDER_PATH=/path/to/transfo/xl/checkpoint
transformers-cli convert --model_type transfo_xl \
--tf_checkpoint $TRANSFO_XL_CHECKPOINT_FOLDER_PATH \
--pytorch_dump_output $PYTORCH_DUMP_OUTPUT \
[--config TRANSFO_XL_CONFIG] \
[--finetuning_task_name TRANSFO_XL_FINETUNED_TASK]
```
## XLNet
Ecco un esempio del processo di conversione di un modello XLNet pre-allenato:
```bash
export TRANSFO_XL_CHECKPOINT_PATH=/path/to/xlnet/checkpoint
export TRANSFO_XL_CONFIG_PATH=/path/to/xlnet/config
transformers-cli convert --model_type xlnet \
--tf_checkpoint $TRANSFO_XL_CHECKPOINT_PATH \
--config $TRANSFO_XL_CONFIG_PATH \
--pytorch_dump_output $PYTORCH_DUMP_OUTPUT \
[--finetuning_task_name XLNET_FINETUNED_TASK] \
```
## XLM
Ecco un esempio del processo di conversione di un modello XLM pre-allenato:
```bash
export XLM_CHECKPOINT_PATH=/path/to/xlm/checkpoint
transformers-cli convert --model_type xlm \
--tf_checkpoint $XLM_CHECKPOINT_PATH \
--pytorch_dump_output $PYTORCH_DUMP_OUTPUT
[--config XML_CONFIG] \
[--finetuning_task_name XML_FINETUNED_TASK]
```
## T5
Ecco un esempio del processo di conversione di un modello T5 pre-allenato:
```bash
export T5=/path/to/t5/uncased_L-12_H-768_A-12
transformers-cli convert --model_type t5 \
--tf_checkpoint $T5/t5_model.ckpt \
--config $T5/t5_config.json \
--pytorch_dump_output $T5/pytorch_model.bin
```
| 0 |
hf_public_repos/transformers/docs/source | hf_public_repos/transformers/docs/source/it/add_new_model.md | <!--Copyright 2020 The HuggingFace Team. All rights reserved.
Licensed under the Apache License, Version 2.0 (the "License"); you may not use this file except in compliance with
the License. You may obtain a copy of the License at
http://www.apache.org/licenses/LICENSE-2.0
Unless required by applicable law or agreed to in writing, software distributed under the License is distributed on
an "AS IS" BASIS, WITHOUT WARRANTIES OR CONDITIONS OF ANY KIND, either express or implied. See the License for the
โ ๏ธ Note that this file is in Markdown but contain specific syntax for our doc-builder (similar to MDX) that may not be
rendered properly in your Markdown viewer.
-->
# Come aggiungere un modello a ๐ค Transformers?
Aggiungere un nuovo modello รฉ spesso difficile e richiede una profonda conoscenza della libreria ๐ค Transformers e anche
della repository originale del modello. A Hugging Face cerchiamo di dare alla community sempre piรบ poteri per aggiungere
modelli independentemente. Quindi, per alcuni nuovi modelli che la community vuole aggiungere a ๐ค Transformers, abbiamo
creato una specifica *call-for-model-addition* che spiega passo dopo passo come aggiungere il modello richiesto. Con
questo *call-for-model-addition* vogliamo insegnare a volenterosi e esperti collaboratori della community come implementare
un modello in ๐ค Transformers.
Se questo รฉ qualcosa che puรฒ interessarvi, siete liberi di controllare l'attuale โcalls-for-model-additionโ [qui](https://github.com/huggingface/transformers/tree/main/templates/adding_a_new_model/open_model_proposals/README.md)
e contattarci.
Se il modello sarร selezionato, allora potrete lavorare insieme a un membro di Hugging Face per integrare il modello in ๐ค
Transformers. Cosรฌ facendo, ci guadagnerai in una comprensione totale, sia teorica che pratica, del modello proposto. Inoltre,
sarai l'artefice di un importante contributo open-source a ๐ค Transformers. Durante l'implementazione avrai l'opportunitร di:
- ottenere piรน comprensione delle best practices in open-source
- capire i principi di design di una della librerie NLP piรน popolari
- capire come efficientemente testare complessi modelli NLP
- capire come integrare utilit Python come `black`, `ruff`, `make fix-copies` in una libreria per garantire sempre di avere un codice leggibile e pulito
Siamo anche contenti se vuoi aggiungere un modello che non puรฒ essere trovato nella cartella โcalls-for-model-additionโ.
Le seguenti sezioni spiegano in dettaglio come aggiungere un nuovo modello. Puรฒ anche essere molto utile controllare modelli
giร aggiunti [qui](https://github.com/huggingface/transformers/pulls?q=is%3Apr+label%3A%22PR+for+Model+Addition%22+is%3Aclosed),
per capire se richiamano il modello che vorreste aggiungere.
Per cominciare, vediamo una panoramica general della libreria Transformers.
## Panoramica generale su ๐ค Transformers
Prima di tutto, vediamo in generale ๐ค Transformers. ๐ค Transformers รฉ una libreria molto strutturata, quindi
puร essere che a volte ci sia un disaccordo con alcune filosofie della libreria o scelte di design. Dalla nostra esperienza,
tuttavia, abbiamo trovato che le scelte fondamentali di design della libreria sono cruciali per usare ๐ค Transformers efficacemente
su larga scala, mantenendo i costi a un livello accettabile.
Un buon primo punto di partenza per capire al meglio la libreria รฉ leggere la [documentazione sulla nostra filosofia](filosofia)
Da qui, ci sono alcune scelte sul modo di lavorare che cerchiamo di applicare a tutti i modelli:
- La composizione รฉ generalmente favorita sulla sovra-astrazione
- Duplicare il codice non รฉ sempre male, soprattutto se migliora notevolmente la leggibilitร e accessibilitร del modello
- Tutti i files creati per il nuovo modello devono il piu possibile "compatti". Questo vuol dire che quando qualcuno leggerรก il codice
di uno specifico modello, potrรก vedere solo il corrispettivo file `modeling_....py` senza avere multiple dipendenze.
La cosa piรบ importante, รฉ che consideriamo la libreria non solo un mezzo per dare un prodotto, *per esempio* dare la possibilitร
di usare BERT per inferenza, ma รฉ anche il prodotto reale che noi vogliamo migliorare sempre piรน. Quindi, quando aggiungi
un modello, non sei solo la persona che userร il modello, ma rappresenti anche tutti coloro che leggeranno,
cercheranno di capire e modificare il tuo modello.
Tenendo questi principi in mente, immergiamoci nel design generale della libreria.
### Panoramica sui modelli
Per aggiungere con successo un modello, รฉ importante capire l'interazione tra il tuo modello e la sua configurazione,
[`PreTrainedModel`], e [`PretrainedConfig`]. Per dare un esempio, chiameremo il modello da aggiungere a ๐ค Transformers
`BrandNewBert`.
Diamo un'occhiata:
<img src="https://huggingface.co/datasets/huggingface/documentation-images/resolve/main/transformers_overview.png"/>
Come potete vedere, ci basiamo sull'ereditarietร in ๐ค Transformers, tenendo perรฒ il livello di astrazione a un minimo
assoluto. Non ci sono mai piรน di due livelli di astrazione per ogni modello nella libreria. `BrandNewBertModel` eredita
da `BrandNewBertPreTrainedModel` che, a sua volta, eredita da [`PreTrainedModel`] - semplice no?
Come regola generale, vogliamo essere sicuri che un nuovo modello dipenda solo da [`PreTrainedModel`]. Le funzionalitร
importanti che sono automaticamente conferite a ogni nuovo modello sono [`~PreTrainedModel.from_pretrained`]
e [`~PreTrainedModel.save_pretrained`], che sono usate per serializzazione e deserializzazione. Tutte le altre importanti
funzionalitร , come ad esempio `BrandNewBertModel.forward` devono essere definite completamente nel nuovo script
`modeling_brand_new_bert.py`. Inoltre, vogliamo essere sicuri che un modello con uno specifico head layer, come
`BrandNewBertForMaskedLM` non erediti da `BrandNewBertModel`, ma piuttosto usi `BrandNewBertModel`
come componente che puรฒ essere chiamata nel passaggio forward per mantenere il livello di astrazione basso. Ogni
nuovo modello richieste una classe di configurazione, chiamata `BrandNewBertConfig`. Questa configurazione รฉ sempre
mantenuta come un attributo in [`PreTrainedModel`], e quindi puรฒ essere accessibile tramite l'attributo `config`
per tutte le classi che ereditano da `BrandNewBertPreTrainedModel`:
```python
model = BrandNewBertModel.from_pretrained("brandy/brand_new_bert")
model.config # il modello ha accesso al suo config
```
Analogamente al modello, la configurazione eredita le funzionalitร base di serializzazione e deserializzazione da
[`PretrainedConfig`]. ร da notare che la configurazione e il modello sono sempre serializzati in due formati differenti -
il modello รฉ serializzato in un file *pytorch_model.bin* mentre la configurazione con *config.json*. Chiamando
[`~PreTrainedModel.save_pretrained`] automaticamente chiamerร [`~PretrainedConfig.save_pretrained`], cosicchรฉ sia il
modello che la configurazione siano salvati.
### Stile per il codice
Quando codifichi un nuovo modello, tieni presente che Transformers ha una sua struttura di fondo come libreria, perciรฒ
ci sono alcuni fatti da considerare su come scrivere un codice :-)
1. Il forward pass del tuo modello dev'essere scritto completamente nel file del modello, mentre dev'essere indipendente
da altri modelli nella libreria. Se vuoi riutilizzare un blocco di codice da un altro modello, copia e incolla il codice con un commento `# Copied from` in cima al codice (guarda [qui](https://github.com/huggingface/transformers/blob/v4.17.0/src/transformers/models/roberta/modeling_roberta.py#L160)
per un ottimo esempio).
2. Il codice dev'essere interamente comprensibile, anche da persone che non parlano in inglese. Questo significa che le
variabili devono avere un nome descrittivo e bisogna evitare abbreviazioni. Per esempio, `activation` รฉ molto meglio
che `act`. Le variabili con una lettera sono da evitare fortemente, almeno che non sia per un indce in un for loop.
3. Generamente รฉ meglio avere un codice esplicito e piรบ lungo che un codice corto e magico.
4. Evita di subclassare `nn.Sequential` in Pytorch, puoi subclassare `nn.Module` e scrivere il forward pass, cosicchรฉ
chiunque puรฒ effettuare debug sul tuo codice, aggiungendo print o breaking points.
5. La tua function-signature dev'essere type-annoted. Per il resto, รฉ meglio preferire variabili con un nome accettabile
piuttosto che annotazioni per aumentare la comprensione e leggibilitร del codice.
### Panoramica sui tokenizers
Questa sezione sarร creata al piu presto :-(
## Aggiungere un modello a ๐ค Transformers passo dopo passo
Ci sono differenti modi per aggiungere un modello a Hugging Face. Qui trovi una lista di blog posts da parte della community su come aggiungere un modello:
1. [Aggiungere GPT2](https://medium.com/huggingface/from-tensorflow-to-pytorch-265f40ef2a28) scritto da [Thomas](https://huggingface.co/thomwolf)
2. [Aggiungere WMT19 MT](https://huggingface.co/blog/porting-fsmt) scritto da [Stas](https://huggingface.co/stas)
Per esperienza, possiamo dirti che quando si aggiunge un modello รฉ meglio tenere a mente le seguenti considerazioni:
- Non sfondare una porta giรก aperta! La maggior parte del codice che aggiungerai per un nuovo modello ๐ค Transformers
esiste giร da qualche parte in ๐ค Transformers. Prendi un po' di tempo per trovare codici simili in modelli e tokenizers esistenti e fare un copia-incolla. Ricorda che [grep](https://www.gnu.org/software/grep/) e [rg](https://github.com/BurntSushi/ripgrep) sono tuoi buoni amici. Inoltre, ricorda che puรณ essere molto probabile che il tokenizer per il tuo modello sia basato sull'implementazione di un altro modello, e il codice del tuo modello stesso su un altro ancora. *Per esempio* il modello FSMT รฉ basato su BART, mentre il tokenizer di FSMT รฉ basato su XLM.
- Ricorda che qui รฉ piu una sfida ingegneristica che scientifica. Spendi piรบ tempo per create un efficiente ambiente di debugging piuttosto che cercare di capire tutti gli aspetti teorici dell'articolo del modello.
- Chiedi aiuto se sei in panne! I modelli sono la parte principale di ๐ค Transformers, perciรฒ qui a Hugging Face siamo piรน che contenti di aiutarti in ogni passo per aggiungere il tuo modello. Non esitare a chiedere se vedi che non riesci a progredire.
Di seguito, diamo una ricetta generale per aiutare a portare un modello in ๐ค Transformers.
La lista seguente รฉ un sommario di tutto quello che รฉ stato fatto per aggiungere un modello, e puรฒ essere usata come To-Do List:
- 1. โ (Opzionale) Capire gli aspetti teorici del modello
- 2. โ Preparare l'ambiente dev per transformers
- 3. โ Preparare l'ambiente debugging della repository originale
- 4. โ Create uno script che gestisca con successo il forward pass usando la repository originale e checkpoint
- 5. โ Aggiungere con successo lo scheletro del modello a Transformers
- 6. โ Convertire i checkpoint original a Transformers checkpoint
- 7. โ Effettuare con successo la forward pass in Transformers, di modo che dia un output identico al checkpoint originale
- 8. โ Finire i tests per il modello in Transformers
- 9. โ Aggiungere con successo Tokenizer in Transformers
- 10. โ Testare e provare gli integration tests da capo a fine
- 11. โ Completare i docs
- 12. โ Caricare i moedl weights all'hub
- 13. โ Sottomettere una pull request
- 14. โ (Opzionale) Aggiungere un notebook con una demo
Per cominciare di solito consigliamo `BrandNewBert`, partendo dalla teoria, di modo da avere una buona comprensione della teoria generale. TUttavia, se preferisci imparare l'aspetto teorico del modello mentre *lavori* sul modello รฉ ok immergersi direttamente nel codice di `BrandNewBert`. Questa opzione puรณ essere buona se le tue skills ingegneristiche sono meglio che quelle teoriche, o se il paper `BrandNewBert` ti dรก problemi, o se semplicemente ti piace programmare piรบ che leggere articoli scientifici.
### 1. (Opzionale) Aspetti teorici di BrandNewBert
Allora con calma, prendi un po' di tempo per leggere l'articolo su *BrandNewBert* . Sicuramente, alcune sezioni dell'articolo sono molto complesse, ma non preoccuparti! L'obiettivo non รฉ avere una compresione immensa della teoria alla base, ma estrarre le informazioni necessarie per re-implementare con successo il modello in ๐ค Transformers. Quindi, non impazzire sugli aspetti teorici, ma piuttosto focalizzati su quelli pratici, ossia:
- Che tipo di modello รฉ *brand_new_bert*? ร solo un encoder in stile BERT? O tipo decoder come GPT2? O encoder e decoder stile BART? Dai un'occhiata a [model_summary](model_summary) se non sei famigliare con le differenze tra questi modelli
- Quali sono le applicazioni di *brand_new_bert*? Classificazione di testo? Generazione di testo? O per tasks del genere seq2seq?
- Quali sono le nuove aggiunte al modello che lo rendono diverso da BERT/GPT-2/BART?
- Quali modelli estistenti in [๐ค Transformers models](https://huggingface.co/transformers/#contents) sono molto simili a *brand_new_bert*?
- Che tipo di tokenizer si usa in questo caso? Un sentencepiece tokenizer? O un word piece tokenizer? Il tokenizer รฉ lo stesso di BERT o BART?
Una volta che senti che hai avuto una bella overview dell'architettura del modello, puoi scrivere senza problemi al team di Hugging Face per ogni domanda che tu hai. Questo puรณ includere domande sull'architettura del modello, o sull'attention layer, etc. Saremo molto felici di aiutarti :)
### 2. Prepare il tuo ambiente
1. Forka la [repository](https://github.com/huggingface/transformers) cliccando sul tasto โFork' nella pagina della repository. Questo crea una copia del codice nel tuo account GitHub
2. Clona il tuo fork `transfomers` sul tuo dico locale, e aggiungi la repository base come remota:
```bash
git clone https://github.com/[your Github handle]/transformers.git
cd transformers
git remote add upstream https://github.com/huggingface/transformers.git
```
3. Crea un ambiente di sviluppo, per esempio tramite questo comando:
```bash
python -m venv .env
source .env/bin/activate
pip install -e ".[dev]"
```
quindi torna alla directory principale:
```bash
cd ..
```
4. Attenzione, raccomandiamo di aggiungere la versione di PyTorch di *brand_new_bert* a Transfomers. Per installare PyTorch, basta seguire queste istruzioni https://pytorch.org/get-started/locally/.
**Nota bene:** Non c'รฉ bisogno di installare o avere installato CUDA. Il nuovo modello puรฒ funzionare senza problemi su una CPU.
5. Per trasferire *brand_new_bert* To port *brand_new_bert* avrai bisogno anche accesso alla sua repository originale:
```bash
git clone https://github.com/org_that_created_brand_new_bert_org/brand_new_bert.git
cd brand_new_bert
pip install -e .
```
Ok, ora hai un ambiente di sviluppo per portare *brand_new_bert* in ๐ค Transformers.
### 3.-4. Provare un pretrained checkpoint usando la repo originale
Per cominciare, comincerai a lavorare sulla repo originale di *brand_new_bert*. Come spesso accade, l'implementazione originale รฉ molto sullo stile "ricerca". Questo significa che a volte la documentazione non รฉ al top, magari manca qualche cosa e il codice puรณ essere difficile da capire. Tuttavia, questa รฉ e dev'essere la motivazione per reimplementare *brand_new_bert*. In Hugging Face, uno degli obiettivi principali รฉ di *mettere le persone sulle spalle dei giganti*, il che si traduce, in questo contesto, di prendere un modello funzionante e riscriverlo e renderlo il piรบ possibile **accessibile, user-friendly, e leggibile**. Questa รฉ la top motivazione per re-implementare modelli in ๐ค Transformers - cercare di creare nuove complesse tecnologie NLP accessibili a **chiunque**.
Riuscire a far girare il modello pretrained originale dalla repository ufficiale รฉ spesso il passo **piu arduo**. Dalla nostra esperienza, รฉ molto importante spendere un p' di tempo per diventare familiari con il codice base originale. Come test, prova a capire i seguenti punti:
- Dove si trovano i pretrained weights?
- Come caricare i pretrained weights nel modello corrispondente?
- Come girare un tokenizer independentemente dal modello?
- Prova a tracciare un singolo forward pass, cosicchรฉ potrai sapere che classi e funzioni sono richieste per un semplice forward pass. Di solito, dovrai reimplementare queste funzioni e basta
- Prova a localizzare i componenti importanti del modello: Dove si trova la classe del modello? Ci sono sotto classi nel modello *per esempio* EngoderModel, DecoderMOdel? Dove si trova il self-attention layer? Ci sono molteplici differenti layer di attention, *per esempio * *self-attention*, *cross-attention*...?
- Come puoi fare debug sul modello nell'ambiente originale della repo? Devi aggiungere dei *print* o puoi usare *ipdb* come debugger interattivo, o vabene anche un IDE efficiente per debug come PyCharm?
ร molto importante che prima di cominciare a trasferire il modello nuovo tu spenda tempo a fare debug del codice originale in maniera **efficiente**! Inoltre, ricorda che tutta la library รฉ open-soruce, quindi non temere di aprire issue o fare una pull request nella repo originale. Tutti coloro che mantengono la repository saranno piรบ che felici di avere qualcuno che guarda e gioca con i loro codici!
A questo punto, sta a te decidere quale ambiente per debug vuoi usare. Noi consilgiamo di evitare setup con GPU, che potrebbero costare assai, lavorare su una CPU puรณ essere un ottimo punto di partenza per indagare la repository originale e per cominciare a scrivere il codice per ๐ค Transformers. Solo alla fine, quando il modello รฉ stato portato con successo in ๐ค Transformers, allora si potrรก verificare il suo funzionamento su GPU.
In generale ci sono due possibili ambienti di debug per il testare il modello originale:
- [Jupyter notebooks](https://jupyter.org/) / [google colab](https://colab.research.google.com/notebooks/intro.ipynb)
- Scripts locali in Python
Il vantaggio dei Jupyter notebooks รฉ la possibilitร di eseguire cella per cella, il che puรฒ essere utile per decomporre tutte le componenti logiche, cosi da a vere un ciclo di debug piรน rapido, siccome si possono salvare i risultati da steps intermedi. Inoltre, i notebooks spesso sono molto facili da condividere con altri contributors, il che puรฒ essere molto utile se vuoi chiedere aiuto al team di Hugging Face. Se sei famigliare con Jupyter notebooks allora racommandiamo di lavorare in questa maniera.
Ovviamente se non siete abituati a lavorare con i notebook, questo puรฒ essere uno svantaggio nell'usare questa tecnologia, sprecando un sacco di tempo per setup e portare tutto al nuovo ambiente, siccome non potreste neanche usare dei tools di debug come `ipdb`.
Per ogni pratica code-base, รฉ sempre meglio come primo step caricare un **piccolo** checkpoint pretrained e cercare di riprodurre un singolo forward pass usando un vettore fittizio di IDs fatti da numeri interi. Un esempio per uno script simile, in pseudocodice รฉ:
```python
model = BrandNewBertModel.load_pretrained_checkpoint("/path/to/checkpoint/")
input_ids = [0, 4, 5, 2, 3, 7, 9] # vector of input ids
original_output = model.predict(input_ids)
```
Per quanto riguarda la strategia di debugging, si puรฒ scegliere tra:
- Decomporre il modello originario in piccole componenenti e testare ognuna di esse
- Decomporre il modello originario nel *tokenizer* originale e nel *modello* originale, testare un forward pass su questi,
e usare dei print statement o breakpoints intermedi per verificare
Ancora una volta, siete liberi di scegliere quale strategia sia ottimale per voi. Spesso una strategia รฉ piu
avvantaggiosa di un'altra, ma tutto dipende dall'code-base originario.
Se il code-base vi permette di decomporre il modello in piccole sub-componenenti, *per esempio* se il code-base
originario puรฒ essere facilmente testato in eager mode, allora vale la pena effettuare un debugging di questo genere.
Ricordate che ci sono dei vantaggi nel decidere di prendere la strada piu impegnativa sin da subito:
- negli stage piu finali, quando bisognerร comparare il modello originario all'implementazione in Hugging Face, potrete verificare
automaticamente ogni componente, individualmente, di modo che ci sia una corrispondenza 1:1
- avrete l'opportunitร di decomporre un problema molto grande in piccoli passi, cosรฌ da strutturare meglio il vostro lavoro
- separare il modello in componenti logiche vi aiuterร ad avere un'ottima overview sul design del modello, quindi una migliore
comprensione del modello stesso
- verso gli stage finali i test fatti componente per componente vi aiuterร ad essere sicuri di non andare avanti e indietro
nell'implementazione, cosรฌ da continuare la modifica del codice senza interruzione
Un ottimo esempio di come questo puรฒ essere fatto รฉ dato da [Lysandre](https://gist.github.com/LysandreJik/db4c948f6b4483960de5cbac598ad4ed)
per il modello ELECTRA
Tuttavia, se il code-base originale รฉ molto complesso o le componenti intermedie possono essere testate solo in tramite
compilazione, potrebbe richiedere parecchio tempo o addirittura essere impossibile separare il modello in piccole sotto-componenti.
Un buon esempio รฉ [MeshTensorFlow di T5](https://github.com/tensorflow/mesh/tree/master/mesh_tensorflow). Questa libreria
รฉ molto complessa e non offre un metodo semplice di decomposizione in sotto-componenti. Per simili librerie, potrete fare
affidamento ai print statements.
In ogni caso, indipendentemente da quale strategia scegliete, la procedura raccomandata รฉ di cominciare a fare debug dal
primo layer al layer finale.
ร consigliato recuperare gli output dai layers, tramite print o sotto-componenti, nel seguente ordine:
1. Recuperare gli IDs di input dati al modello
2. Recuperare i word embeddings
3. Recuperare l'input del primo Transformer layer
4. Recuperare l'output del primo Transformer layer
5. Recuperare l'output dei seguenti `n - 1` Transformer layers
6. Recuperare l'output dell'intero BrandNewBert Model
Gli IDs in input dovrebbero essere un arrary di interi, *per esempio* `input_ids = [0, 4, 4, 3, 2, 4, 1, 7, 19]`
Gli output dei seguenti layer di solito dovrebbero essere degli array di float multi-dimensionali come questo:
```
[[
[-0.1465, -0.6501, 0.1993, ..., 0.1451, 0.3430, 0.6024],
[-0.4417, -0.5920, 0.3450, ..., -0.3062, 0.6182, 0.7132],
[-0.5009, -0.7122, 0.4548, ..., -0.3662, 0.6091, 0.7648],
...,
[-0.5613, -0.6332, 0.4324, ..., -0.3792, 0.7372, 0.9288],
[-0.5416, -0.6345, 0.4180, ..., -0.3564, 0.6992, 0.9191],
[-0.5334, -0.6403, 0.4271, ..., -0.3339, 0.6533, 0.8694]]],
```
Ci aspettiamo che ogni modello aggiunto a ๐ค Transformers passi con successo un paio di test d'integrazione. Questo
significa che il modello originale e la sua implementazione in ๐ค Transformers abbiano lo stesso output con una precisione
di 0.001! Siccome รฉ normale che lo stesso esatto modello, scritto in librerie diverse, possa dare output leggermente
diversi, la tolleranza accettata รฉ 1e-3 (0.001). Ricordate che i due modelli devono dare output quasi identici. Dunque,
รฉ molto conveniente comparare gli output intermedi di ๐ค Transformers molteplici volte con gli output intermedi del
modello originale di *brand_new_bert*. Di seguito vi diamo alcuni consigli per avere un ambiente di debug il piu efficiente
possibile:
- Trovate la migliore strategia per fare debug dei risultati intermedi. Per esempio, รฉ la repository originale scritta in PyTorch?
Se si, molto probabilmente dovrete dedicare un po' di tempo per scrivere degli script piu lunghi, cosรฌ da decomporre il
modello originale in piccole sotto-componenti, in modo da poter recuperare i valori intermedi. Oppure, la repo originale
รฉ scritta in Tensorflow 1? Se รฉ cosรฌ dovrete fare affidamento ai print di Tensorflow [tf.print](https://www.tensorflow.org/api_docs/python/tf/print)
per avere i valori intermedi. Altro caso, la repo รฉ scritta in Jax? Allora assicuratevi che il modello non sia in **jit**
quanto testate il foward pass, *per esempio* controllate [questo link](https://github.com/google/jax/issues/196).
- Usate i piรน piccoli pretrained checkpoint che potete trovare. Piu piccolo รฉ il checkpoint, piu velocemente sarร il vostro
ciclo di debug. Non รฉ efficiente avere un pretrained model cosรฌ gigante che per il forward pass impieghi piu di 10 secondi.
Nel caso in cui i checkpoints siano molto grandi, e non si possa trovare di meglio, allora รฉ buona consuetudine ricorrere
a fare un dummy model nel nuovo ambiente, con weights inizializzati random e salvare quei weights per comprare la versione ๐ค Transformers
con il vostro modello
- Accertatevi di usare la via piu semplice per chiamare il forward pass nella repo originale. Sarebbe opportuno trovare
la funzione originaria che chiami **solo** un singolo forward pass, *per esempio* questa funzione spesso viene chiamata
`predict`, `evaluate`, `forward` o `__call__`. Siate sicuri di non fare debug su una funzione che chiami `forward` molteplici
volte, *per esempio* per generare testo, come `autoregressive_sample`, `generate`.
- Cercate di separare la tokenization dal forward pass del modello. Se la repo originaria mostra esempio dove potete dare
come input una stringa, provate a cercare dove nella forward call la stringa viene cambiata in input ids e cominciate il
debug da questo punto. Questo vi garantisce un ottimo punto di partenza per scrivere un piccolo script personale dove dare
gli input al modello, anziche delle stringhe in input.
- Assicuratevi che il debugging **non** sia in training mode. Spesso questo potra il modello a dare degli output random, per
via dei molteplici dropout layers. Assicuratevi che il forward pass nell'ambiente di debug sia **deterministico**, cosicche
i dropout non siano usati. Alternativamente, potete usare *transformers.utils.set_seed* se la vecchia e nuova implementazione
sono nello stesso framework.
La seguente sezione vi da ulteriori dettagli e accorgimenti su come potete fare tutto questo per *brand_new_bert*.
### 5.-14. Trasferire BrandNewBert in ๐ค Transformers
Allora cominciamo ad aggiungere un nuovo codice in ๐ค Transformers. Andate nel vostro fork clone di ๐ค Transformers:
```bash
cd transformers
```
Nel caso speciale in cui stiate aggiungendo un modello, la cui architettura sia identica a una di un modello giร esistente,
dovrete solo aggiugnere uno script di conversione, come descritto [qui](#write-a-conversion-script).
In questo caso, potete riutilizzare l'intera architettura del modello gia esistente.
Se questo non รฉ il caso, cominciamo con il generare un nuovo modello. Avrete due opzioni:
- `transformers-cli add-new-model-like` per aggiungere un nuovo modello come uno che gia esiste
- `transformers-cli add-new-model` per aggiungere un nuovo modello da un nostro template (questo assomigliera a BERT o Bart, in base al modello che selezionerete)
In entrambi i casi, l'output vi darร un questionario da riempire con informazioni basi sul modello. Il secondo comando richiede di installare
un `cookiecutter` - maggiori informazioni [qui](https://github.com/huggingface/transformers/tree/main/templates/adding_a_new_model).
**Aprire una Pull Request in main huggingface/transformers repo**
Prime di cominciare ad adattare il codice automaticamente generato, aprite una nuova PR come "Work in progress (WIP)",
*per esempio* "[WIP] Aggiungere *brand_new_bert*", cosicchรฉ il team di Hugging Face possa lavorare al vostro fianco nell'
integrare il modello in ๐ค Transformers.
Questi sarebbero gli step generali da seguire:
1. Creare un branch dal main branch con un nome descrittivo
```bash
git checkout -b add_brand_new_bert
```
2. Commit del codice automaticamente generato
```bash
git add .
git commit
```
3. Fare fetch e rebase del main esistente
```bash
git fetch upstream
git rebase upstream/main
```
4. Push dei cambiamenti al proprio account:
```bash
git push -u origin a-descriptive-name-for-my-changes
```
5. Una volte che siete soddisfatti dei nuovi cambiamenti, andate sulla webpage del vostro fork su GitHub. Cliccate "Pull request".
Assiuratevi di aggiungere alcuni membri di Hugging Face come reviewers, nel riguardo alla destra della pagina della PR, cosicche il team
Hugging Face verrร notificato anche per i futuri cambiamenti.
6. Cambiare la PR a draft, cliccando su "Convert to draft" alla destra della pagina della PR
Da quel punto in poi, ricordate di fare commit di ogni progresso e cambiamento, cosicche venga mostrato nella PR. Inoltre,
ricordatevi di tenere aggiornato il vostro lavoro con il main esistente:
```bash
git fetch upstream
git merge upstream/main
```
In generale, tutte le domande che avrete riguardo al modello o l'implementazione dovranno essere fatte nella vostra PR
e discusse/risolte nella PR stessa. In questa maniera, il team di Hugging Face sarร sempre notificato quando farete commit
di un nuovo codice o se avrete qualche domanda. ร molto utile indicare al team di Hugging Face il codice a cui fate riferimento
nella domanda, cosicche il team potra facilmente capire il problema o la domanda.
Per fare questo andate sulla tab "Files changed", dove potrete vedere tutti i vostri cambiamenti al codice, andate sulla linea
dove volete chiedere una domanda, e cliccate sul simbolo "+" per aggiungere un commento. Ogni volta che una domanda o problema
รฉ stato risolto, cliccate sul bottone "Resolve".
In questa stessa maniera, Hugging Face aprirร domande o commenti nel rivedere il vostro codice. Mi raccomando, chiedete piรน
domande possibili nella pagina della vostra PR. Se avete domande molto generali, non molto utili per il pubblico, siete liberi
di chiedere al team Hugging Face direttamente su slack o email.
**5. Adattare i codici per brand_new_bert**
Per prima cosa, ci focalizzeremo sul modello e non sui tokenizer. Tutto il codice relative dovrebbe trovarsi in
`src/transformers/models/brand_new_bert/modeling_brand_new_bert.py` e
`src/transformers/models/brand_new_bert/configuration_brand_new_bert.py`.
Ora potete finalmente cominciare il codice :). Il codice generato in
`src/transformers/models/brand_new_bert/modeling_brand_new_bert.py` avrร sia la stessa architettura di BERT se รฉ un
modello encoder-only o BART se รฉ encoder-decoder. A questo punto, ricordatevi cio che avete imparato all'inizio, riguardo
agli aspetti teorici del modello: *In che maniera il modello che sto implmementando รฉ diverso da BERT o BART?*. Implementare
questi cambi spesso vuol dire cambiare il layer *self-attention*, l'ordine dei layer di normalizzazione e cosรฌ via...
Ancora una volta ripetiamo, รฉ molto utile vedere architetture simili di modelli gia esistenti in Transformers per avere
un'idea migliore su come implementare il modello.
**Notate** che a questo punto non dovete avere subito un codice tutto corretto o pulito. Piuttosto, รฉ consigliato cominciare con un
codice poco pulito, con copia-incolla del codice originale in `src/transformers/models/brand_new_bert/modeling_brand_new_bert.py`
fino a che non avrete tutto il codice necessario. In base alla nostra esperienza, รฉ molto meglio aggiungere una prima bozza
del codice richiesto e poi correggere e migliorare iterativamente. L'unica cosa essenziale che deve funzionare qui รฉ la seguente
instanza:
```python
from transformers import BrandNewBertModel, BrandNewBertConfig
model = BrandNewBertModel(BrandNewBertConfig())
```
Questo comando creerร un modello con i parametri di default definiti in `BrandNewBergConfig()` e weights random. Questo garantisce
che `init()` di tutte le componenti funzioni correttamente.
**6. Scrivere uno script di conversione**
Il prossimo step รฉ scrivere uno script per convertire il checkpoint che avete usato per fare debug su *brand_new_berts* nella
repo originale in un checkpoint per la nuova implementazione di *brand_new_bert* in ๐ค Transformers. Non รฉ consigliato scrivere
lo script di conversione da zero, ma piuttosto cercate e guardate script gia esistenti in ๐ค Transformers, cosรฌ da trovarne
uno simile al vostro modello. Di solito basta fare una copia di uno script gia esistente e adattarlo al vostro caso.
Non esistate a chiedre al team di Hugging Face a riguardo.
- Se state convertendo un modello da TensorFlow a PyTorch, un ottimo inizio รฉ vedere [questo script di conversione per BERT](https://github.com/huggingface/transformers/blob/7acfa95afb8194f8f9c1f4d2c6028224dbed35a2/src/transformers/models/bert/modeling_bert.py#L91)
- Se state convertendo un modello da PyTorch a PyTorch, [lo script di conversione di BART puรฒ esservi utile](https://github.com/huggingface/transformers/blob/main/src/transformers/models/bart/convert_bart_original_pytorch_checkpoint_to_pytorch.py)
Qui di seguito spiegheremo come i modelli PyTorch salvano i weights per ogni layer e come i nomi dei layer sono definiti. In PyTorch,
il nomde del layer รฉ definito dal nome della class attribute che date al layer. Definiamo un modello dummy in PyTorch,
chiamato `SimpleModel`:
```python
from torch import nn
class SimpleModel(nn.Module):
def __init__(self):
super().__init__()
self.dense = nn.Linear(10, 10)
self.intermediate = nn.Linear(10, 10)
self.layer_norm = nn.LayerNorm(10)
```
Ora possiamo creare un'instanza di questa definizione di modo da inizializzare a random weights: `dense`, `intermediate`, `layer_norm`.
Possiamo usare print per vedere l'architettura del modello:
```python
model = SimpleModel()
print(model)
```
Da cui si ottiene:
```
SimpleModel(
(dense): Linear(in_features=10, out_features=10, bias=True)
(intermediate): Linear(in_features=10, out_features=10, bias=True)
(layer_norm): LayerNorm((10,), eps=1e-05, elementwise_affine=True)
)
```
Si puรฒ vedere come i nomi dei layers siano definiti dal nome della class attribute in PyTorch. I valori dei weights di uno
specifico layer possono essere visualizzati:
```python
print(model.dense.weight.data)
```
ad esempio:
```
tensor([[-0.0818, 0.2207, -0.0749, -0.0030, 0.0045, -0.1569, -0.1598, 0.0212,
-0.2077, 0.2157],
[ 0.1044, 0.0201, 0.0990, 0.2482, 0.3116, 0.2509, 0.2866, -0.2190,
0.2166, -0.0212],
[-0.2000, 0.1107, -0.1999, -0.3119, 0.1559, 0.0993, 0.1776, -0.1950,
-0.1023, -0.0447],
[-0.0888, -0.1092, 0.2281, 0.0336, 0.1817, -0.0115, 0.2096, 0.1415,
-0.1876, -0.2467],
[ 0.2208, -0.2352, -0.1426, -0.2636, -0.2889, -0.2061, -0.2849, -0.0465,
0.2577, 0.0402],
[ 0.1502, 0.2465, 0.2566, 0.0693, 0.2352, -0.0530, 0.1859, -0.0604,
0.2132, 0.1680],
[ 0.1733, -0.2407, -0.1721, 0.1484, 0.0358, -0.0633, -0.0721, -0.0090,
0.2707, -0.2509],
[-0.1173, 0.1561, 0.2945, 0.0595, -0.1996, 0.2988, -0.0802, 0.0407,
0.1829, -0.1568],
[-0.1164, -0.2228, -0.0403, 0.0428, 0.1339, 0.0047, 0.1967, 0.2923,
0.0333, -0.0536],
[-0.1492, -0.1616, 0.1057, 0.1950, -0.2807, -0.2710, -0.1586, 0.0739,
0.2220, 0.2358]]).
```
Nello script di conversione, dovreste riempire quei valori di inizializzazione random con gli stessi weights del corrispondente
layer nel checkpoint. *Per esempio*
```python
# retrieve matching layer weights, e.g. by
# recursive algorithm
layer_name = "dense"
pretrained_weight = array_of_dense_layer
model_pointer = getattr(model, "dense")
model_pointer.weight.data = torch.from_numpy(pretrained_weight)
```
Cosรฌ facendo, dovete verificare che ogni inizializzazione random di un peso del modello PyTorch e il suo corrispondente peso nel pretrained checkpoint
siano esattamente gli stessi e uguali in **dimensione/shape e nome**. Per fare questo, รฉ **necessario** aggiungere un `assert`
per la dimensione/shape e nome:
```python
assert (
model_pointer.weight.shape == pretrained_weight.shape
), f"Pointer shape of random weight {model_pointer.shape} and array shape of checkpoint weight {pretrained_weight.shape} mismatched"
```
Inoltre, dovrete fare il print sia dei nomi che dei weights per essere sicuri che siano gli stessi:
```python
logger.info(f"Initialize PyTorch weight {layer_name} from {pretrained_weight.name}")
```
Se la dimensione o il nome non sono uguali, probabilmente avete sbagliato ad assegnare il peso nel checkpoint o nel layer costrutture di
๐ค Transformers.
Una dimensione sbagliata puรฒ essere dovuta ad un errore nei parameteri in `BrandNewBertConfig()`. Tuttavia, puรฒ essere anche
che l'implementazione del layer in PyTorch richieda di fare una transposizione della matrice dei weights.
Infine, controllate **tutti** che tutti i weights inizializzati e fate print di tutti i weights del checkpoint che non sono stati
usati per l'inizializzazione, di modo da essere sicuri che il modello sia correttamente convertito. ร normale che ci siano
errori nel test di conversione, fai per un errore in `BrandNewBertConfig()`, o un errore nell'architettura in ๐ค Transformers,
o un bug in `init()`.
Questo step dev'essere fatto tramite iterazioni fino a che non si raggiungano gli stessi valori per i weights. Una volta che
il checkpoint รฉ stato correttamente caricato in ๐ค Transformers, potete salvare il modello in una cartella di vostra scelta
`/path/to/converted/checkpoint/folder` che contenga sia
`pytorch_model.bin` che `config.json`:
```python
model.save_pretrained("/path/to/converted/checkpoint/folder")
```
**7. Implementare il forward pass**
Una volta che i weights pretrained sono stati correttamente caricati in ๐ค Transformers, dovrete assicurarvi che il forward pass
sia correttamente implementato. [Qui](#provare-un-pretrained-checkpoint-usando-la-repo-originale), avete give creato e provato
uno script che testi il forward pass del modello usando la repo originaria. Ora dovrete fare lo stesso con uno script analogo
usando l'implementazione in ๐ค Transformers anzichรฉ l'originale. Piu o meno lo script dovrebbe essere:
```python
model = BrandNewBertModel.from_pretrained("/path/to/converted/checkpoint/folder")
input_ids = [0, 4, 4, 3, 2, 4, 1, 7, 19]
output = model(input_ids).last_hidden_states
```
Di solito l'output da ๐ค Transformers non รฉ uguale uguale all'output originario, sopratto la prima volta. Non vi abbattete -
รฉ normale! Prima di tutto assicuratevi che non ci siano errori o che non vengano segnalati degli errori nella forward pass.
Spesso capita che ci siano dimensioni sbagliate o data type sbagliati, *ad esempio* `torch.long` anziche `torch.float32`.
Non esistate a chiedere al team Hugging Face!
Nella parte finale assicuratevi che l'implementazione ๐ค Transformers funzioni correttamente cosi da testare che gli output
siano equivalenti a una precisione di `1e-3`. Controllate che `outputs.shape` siano le stesse tra ๐ค Transformers e l'implementazione
originaria. Poi, controllate che i valori in output siano identici. Questa รฉ sicuramente la parte piรน difficile, qui una serie
di errori comuni quando gli output non sono uguali:
- Alcuni layers non sono stati aggiunti, *ad esempio* un *activation* layer non รฉ stato aggiunto, o ci si รฉ scordati di una connessione
- La matrice del word embedding non รฉ stata ripareggiata
- Ci sono degli embeddings posizionali sbagliati perchรฉ l'implementazione originaria ha un offset
- Il dropout รฉ in azione durante il forward pass. Per sistemare questo errore controllate che *model.training = False* e che
il dropout non sia stato attivato nel forward pass, * per esempio * passate *self.training* a [PyTorch's functional dropout](https://pytorch.org/docs/stable/nn.functional.html?highlight=dropout#torch.nn.functional.dropout)
La miglior maniera per sistemare il problema รฉ di vedere all'implementazione originaria del forward pass e in ๐ค Transformers
fianco a fianco e vedere se ci sono delle differenze. In teoria, con debug e print degli output intermedie di entrambe le
implementazioni nel forward pass nell'esatta posizione del network dovrebbe aiutarvi a vedere dove ci sono differenze tra
i due frameworks. Come prima mossa controllate che `input_ids` siano identici in entrambi gli scripts. Da lรฌ andate fino
all'ultimo layer. Potrete notare una differenza tra le due implementazioni a quel punto.
Una volta che lo stesso output รฉ stato ragguingi, verificate gli output con `torch.allclose(original_output, output, atol=1e-3)`.
A questo punto se รฉ tutto a posto: complimenti! Le parti seguenti saranno una passeggiata ๐.
**8. Aggiungere i test necessari per il modello**
A questo punto avete aggiunto con successo il vostro nuovo modello. Tuttavia, รฉ molto probabile che il modello non sia
del tutto ok con il design richiesto. Per essere sicuri che l'implementazione sia consona e compatibile con ๐ค Transformers รฉ
necessario implementare dei tests. Il Cookiecutter dovrebbe fornire automaticamente dei file per test per il vostro modello,
di solito nella folder `tests/test_modeling_brand_new_bert.py`. Provate questo per verificare l'ok nei test piu comuni:
```bash
pytest tests/test_modeling_brand_new_bert.py
```
Una volta sistemati i test comuni, bisogna assicurarsi che il vostro lavoro sia correttamente testato cosicchรจ:
- a) La community puo capire in maniera semplice il vostro lavoro controllando tests specifici del modello *brand_new_bert*,
- b) Implementazioni future del vostro modello non rompano alcune feature importante del modello.
Per prima cosa agguingete dei test d'integrazione. Questi sono essenziali perche fanno la stessa funzione degli scripts di
debug usati precedentemente. Un template per questi tests esiste gia nel Cookiecutter ed รฉ sotto il nome di `BrandNewBertModelIntegrationTests`,
voi dovrete solo completarlo. Una volta che questi tests sono OK, provate:
```bash
RUN_SLOW=1 pytest -sv tests/test_modeling_brand_new_bert.py::BrandNewBertModelIntegrationTests
```
<Tip>
Nel caso siate su Windows, sostituite `RUN_SLOW=1` con `SET RUN_SLOW=1`
</Tip>
Di seguito, tutte le features che sono utili e necessarire per *brand_new_bert* devono essere testate in test separati,
contenuti in `BrandNewBertModelTester`/ `BrandNewBertModelTest`. spesso la gente si scorda questi test, ma ricordate che sono utili per:
- Aiuta gli utenti a capire il vostro codice meglio, richiamando l'attenzione su queste nuove features
- Developers e contributors futuri potranno velocemente testare nuove implementazioni del modello testanto questi casi speciali.
**9. Implementare il tokenizer**
A questo punto avremo bisogno un tokenizer per *brand_new_bert*. Di solito il tokenizer รฉ uguale ad altri modelli in ๐ค Transformers.
ร importante che troviate il file con il tokenizer originale e che lo carichiate in ๐ค Transformers.
Per controllare che il tokenizer funzioni in modo corretto, create uno script nella repo originaria che riceva come input
una stringa e ritorni gli `input_ids`. Piu o meno questo potrebbe essere il codice:
```python
input_str = "This is a long example input string containing special characters .$?-, numbers 2872 234 12 and words."
model = BrandNewBertModel.load_pretrained_checkpoint("/path/to/checkpoint/")
input_ids = model.tokenize(input_str)
```
Potrebbe richiedere un po' di tempo, ma guardate ancora alla repo originaria per trovare la funzione corretta del tokenizer.
A volte capita di dover riscrivere il tokenizer nella repo originaria, di modo da avere come output gli `input_ids`.
A quel punto uno script analogo รฉ necessario in ๐ค Transformers:
```python
from transformers import BrandNewBertTokenizer
input_str = "This is a long example input string containing special characters .$?-, numbers 2872 234 12 and words."
tokenizer = BrandNewBertTokenizer.from_pretrained("/path/to/tokenizer/folder/")
input_ids = tokenizer(input_str).input_ids
```
Una volta che `input_ids` sono uguali, bisogna aggiungere un test per il tokenizer.
Il file test per tokenizer di *brand_new_brand* dovrebbe avere un paio di hard-coded test d'integrazione.
**10. Test end-to-end**
Ora che avete il tokenizer, dovrete aggiungere dei test d'integrazione per l'intero workflow in `tests/test_modeling_brand_new_bert.py` in ๐ค Transformer.
Questi test devono mostrare che un significante campione text-to-text funzioni come ci si aspetta nell'implementazione di ๐ค Transformers.
*Per esempio* potreste usare dei source-to-target-translation, o un sommario di un articolo, o un domanda-risposta e cosi via.
Se nessuno dei checkpoints รฉ stato ultra parametrizzato per task simili, allora i tests per il modello sono piu che sufficienti.
Nello step finale dovete assicurarvi che il modello sia totalmente funzionale, e consigliamo anche di provare a testare su GPU.
Puo succedere che ci si scordi un `.to(self.device)` ad esempio. Se non avete accesso a GPU, il team Hugging Face puo provvedere
a testare questo aspetto per voi.
**11. Aggiungere una Docstring**
Siete quasi alla fine! L'ultima cosa rimasta รฉ avere una bella docstring e una pagina doc. Il Cookiecutter dovrebbe provvedere giร
un template chiamato `docs/source/model_doc/brand_new_bert.rst`, che dovrete compilare. La prima cosa che un utente farร
per usare il vostro modello sarร dare una bella lettura al doc. Quindi proponete una documentazione chiara e concisa. ร molto
utile per la community avere anche delle *Tips* per mostrare come il modello puo' essere usato. Non esitate a chiedere a Hugging Face
riguardo alle docstirng.
Quindi, assicuratevi che la docstring sia stata aggiunta a `src/transformers/models/brand_new_bert/modeling_brand_new_bert.py`.
Assicuratevi che la docstring sia corretta e che includa tutti i necessari input e output. Abbiamo una guida dettagliata per
scrivere la documentazione e docstring.
**Rifattorizzare il codice**
Perfetto! Ora che abbiamo tutto per *brand_new_bert* controllate che lo stile del codice sia ok:
```bash
make style
```
E che il codice passi i quality check:
```bash
make quality
```
A volte capita che manchino delle informazioninella docstring o alcuni nomi sbagliati, questo farร fallire i tests sopra.
Ripetiamo: chiedete pure a Hugging Face, saremo lieti di aiutarvi.
Per ultimo, fare del refactoring del codice una volta che รฉ stato creato.
Avete finito con il codice, congratulazioni! ๐ Siete fantasticiiiiiii! ๐
**12. Caricare il modello sul model hub**
In questa ultima parte dovrete convertire e caricare il modello, con tutti i checkpoints, nel model hub e aggiungere una
model card per ogni checkpoint caricato. Leggete la nostra guida [Model sharing and uploading Page](model_sharing) per
avere familiaritร con l'hub. Di solito in questa parte lavorate a fianco di Hugging face per decidere un nome che sia ok
per ogni checkpoint, per ottenere i permessi necessari per caricare il modello nell'organizzazione dell'autore di *brand_new_bert*.
Il metodo `push_to_hub`, presente in tutti i modelli `transformers`, รฉ una maniera rapida e indolore per caricare il vostro checkpoint sull'hub:
```python
brand_new_bert.push_to_hub(
repo_path_or_name="brand_new_bert",
# Uncomment the following line to push to an organization
# organization="<ORGANIZATION>",
commit_message="Add model",
use_temp_dir=True,
)
```
Vale la pena spendere un po' di tempo per creare una model card ad-hoc per ogni checkpoint. Le model cards dovrebbero
suggerire le caratteristiche specifiche del checkpoint, *per esempio* su che dataset il checkpoint รฉ stato pretrained o fine-tuned.
O che su che genere di task il modello lavoro? E anche buona pratica includere del codice su come usare il modello correttamente.
**13. (Opzionale) Aggiungere un notebook**
ร molto utile aggiungere un notebook, che dimostri in dettaglio come *brand_new_bert* si utilizzi per fare inferenza e/o
fine-tuned su specifiche task. Non รฉ una cosa obbligatoria da avere nella vostra PR, ma รฉ molto utile per la community.
**14. Sottomettere la PR**
L'ultimissimo step! Ovvero il merge della PR nel main. Di solito il team Hugging face a questo punto vi avrร gia aiutato,
ma รฉ ok prendere un po' di tempo per pulire la descirzione e commenti nel codice.
### Condividete il vostro lavoro!!
ร ora tempo di prendere un po' di credito dalla communitร per il vostro lavoro! Caricare e implementare un nuovo modello
รฉ un grandissimo contributo per Transformers e l'intera community NLP. Il codice e la conversione dei modelli pre-trained sara
sicuramente utilizzato da centinaia o migliaia di sviluppatori e ricercatori. Siate fieri e orgogliosi di condividere il vostro
traguardo con l'intera community :)
** Avete create un altro modello che รฉ super facile da usare per tutti quanti nella community! ๐คฏ**
| 0 |
hf_public_repos/transformers/docs/source | hf_public_repos/transformers/docs/source/it/custom_models.md | <!--Copyright 2020 The HuggingFace Team. All rights reserved.
Licensed under the Apache License, Version 2.0 (the "License"); you may not use this file except in compliance with
the License. You may obtain a copy of the License at
http://www.apache.org/licenses/LICENSE-2.0
Unless required by applicable law or agreed to in writing, software distributed under the License is distributed on
an "AS IS" BASIS, WITHOUT WARRANTIES OR CONDITIONS OF ANY KIND, either express or implied. See the License for the
specific language governing permissions and limitations under the License.
โ ๏ธ Note that this file is in Markdown but contain specific syntax for our doc-builder (similar to MDX) that may not be
rendered properly in your Markdown viewer.
-->
# Condividere modelli personalizzati
La libreria ๐ค Transformers รจ studiata per essere facilmente estendibile. Il codice di ogni modello รจ interamente
situato in una sottocartella del repository senza alcuna astrazione, perciรฒ puoi facilmente copiare il file di un
modello e modificarlo in base ai tuoi bisogni.
Se stai scrivendo un nuovo modello, potrebbe essere piรน semplice iniziare da zero. In questo tutorial, ti mostreremo
come scrivere un modello personalizzato e la sua configurazione in modo che possa essere utilizzato allโinterno di
Transformers, e come condividerlo con la community (assieme al relativo codice) cosรฌ che tutte le persone possano usarlo, anche
se non presente nella libreria ๐ค Transformers.
Illustriamo tutto questo su un modello ResNet, avvolgendo la classe ResNet della
[libreria timm](https://github.com/rwightman/pytorch-image-models) in un [`PreTrainedModel`].
## Scrivere una configurazione personalizzata
Prima di iniziare a lavorare al modello, scriviamone la configurazione. La configurazione di un modello รจ un oggetto
che contiene tutte le informazioni necessarie per la build del modello. Come vedremo nella prossima sezione, il
modello puรฒ soltanto essere inizializzato tramite `config`, per cui dovremo rendere tale oggetto piรน completo possibile.
Nel nostro esempio, prenderemo un paio di argomenti della classe ResNet che potremmo voler modificare.
Configurazioni differenti ci daranno quindi i differenti possibili tipi di ResNet. Salveremo poi questi argomenti,
dopo averne controllato la validitร .
```python
from transformers import PretrainedConfig
from typing import List
class ResnetConfig(PretrainedConfig):
model_type = "resnet"
def __init__(
self,
block_type="bottleneck",
layers: List[int] = [3, 4, 6, 3],
num_classes: int = 1000,
input_channels: int = 3,
cardinality: int = 1,
base_width: int = 64,
stem_width: int = 64,
stem_type: str = "",
avg_down: bool = False,
**kwargs,
):
if block_type not in ["basic", "bottleneck"]:
raise ValueError(f"`block_type` must be 'basic' or bottleneck', got {block_type}.")
if stem_type not in ["", "deep", "deep-tiered"]:
raise ValueError(f"`stem_type` must be '', 'deep' or 'deep-tiered', got {stem_type}.")
self.block_type = block_type
self.layers = layers
self.num_classes = num_classes
self.input_channels = input_channels
self.cardinality = cardinality
self.base_width = base_width
self.stem_width = stem_width
self.stem_type = stem_type
self.avg_down = avg_down
super().__init__(**kwargs)
```
Le tre cose piรน importanti da ricordare quando scrivi le tue configurazioni sono le seguenti:
- Devi ereditare da `Pretrainedconfig`,
- Il metodo `__init__` del tuo `Pretrainedconfig` deve accettare i kwargs,
- I `kwargs` devono essere passati alla superclass `__init__`
Lโereditร รจ importante per assicurarsi di ottenere tutte le funzionalitร della libreria ๐ค transformers,
mentre gli altri due vincoli derivano dal fatto che un `Pretrainedconfig` ha piรน campi di quelli che stai settando.
Quando ricarichi una config da un metodo `from_pretrained`, questi campi devono essere accettati dalla tua config e
poi inviati alla superclasse.
Definire un `model_type` per la tua configurazione (qua `model_type = โresnetโ`) non รจ obbligatorio, a meno che tu
non voglia registrare il modello con le classi Auto (vedi l'ultima sezione).
Una volta completato, puoi facilmente creare e salvare la tua configurazione come faresti con ogni altra configurazione
di modelli della libreria. Ecco come possiamo creare la config di un resnet50d e salvarlo:
```py
resnet50d_config = ResnetConfig(block_type="bottleneck", stem_width=32, stem_type="deep", avg_down=True)
resnet50d_config.save_pretrained("custom-resnet")
```
Questo salverร un file chiamato `config.json` all'interno della cartella `custom-resnet`. Potrai poi ricaricare la tua
config con il metodo `from_pretrained`.
```py
resnet50d_config = ResnetConfig.from_pretrained("custom-resnet")
```
Puoi anche usare qualunque altro metodo della classe [`PretrainedConfig`], come [`~PretrainedConfig.push_to_hub`]
per caricare direttamente la tua configurazione nell'hub.
## Scrivere un modello personalizzato
Ora che abbiamo la nostra configurazione ResNet, possiamo continuare a scrivere il modello. In realtร , ne scriveremo
due: uno che estrae le features nascoste da una batch di immagini (come [`BertModel`]) e uno che รจ utilizzabile per
la classificazione di immagini (come [`BertModelForSequenceClassification`]).
Come abbiamo menzionato in precedenza, scriveremo soltanto un wrapper del modello, per mantenerlo semplice ai fini di
questo esempio. L'unica cosa che dobbiamo fare prima di scrivere questa classe รจ una mappatura fra i tipi di blocco e
le vere classi dei blocchi. Successivamente il modello รจ definito tramite la configurazione, passando tutto quanto alla
classe `ResNet`.
```py
from transformers import PreTrainedModel
from timm.models.resnet import BasicBlock, Bottleneck, ResNet
from .configuration_resnet import ResnetConfig
BLOCK_MAPPING = {"basic": BasicBlock, "bottleneck": Bottleneck}
class ResnetModel(PreTrainedModel):
config_class = ResnetConfig
def __init__(self, config):
super().__init__(config)
block_layer = BLOCK_MAPPING[config.block_type]
self.model = ResNet(
block_layer,
config.layers,
num_classes=config.num_classes,
in_chans=config.input_channels,
cardinality=config.cardinality,
base_width=config.base_width,
stem_width=config.stem_width,
stem_type=config.stem_type,
avg_down=config.avg_down,
)
def forward(self, tensor):
return self.model.forward_features(tensor)
```
Per il modello che classificherร le immagini, cambiamo soltanto il metodo forward:
```py
import torch
class ResnetModelForImageClassification(PreTrainedModel):
config_class = ResnetConfig
def __init__(self, config):
super().__init__(config)
block_layer = BLOCK_MAPPING[config.block_type]
self.model = ResNet(
block_layer,
config.layers,
num_classes=config.num_classes,
in_chans=config.input_channels,
cardinality=config.cardinality,
base_width=config.base_width,
stem_width=config.stem_width,
stem_type=config.stem_type,
avg_down=config.avg_down,
)
def forward(self, tensor, labels=None):
logits = self.model(tensor)
if labels is not None:
loss = torch.nn.cross_entropy(logits, labels)
return {"loss": loss, "logits": logits}
return {"logits": logits}
```
Nota come, in entrambi i casi, ereditiamo da `PreTrainedModel` e chiamiamo l'inizializzazione della superclasse
con il metodo `config` (un po' come quando scrivi un normale `torch.nn.Module`). La riga che imposta la `config_class`
non รจ obbligatoria, a meno che tu non voglia registrare il modello con le classi Auto (vedi l'ultima sezione).
<Tip>
Se il tuo modello รจ molto simile a un modello all'interno della libreria, puoi ri-usare la stessa configurazione di quel modello.
</Tip>
Puoi fare in modo che il tuo modello restituisca in output qualunque cosa tu voglia, ma far restituire un dizionario
come abbiamo fatto per `ResnetModelForImageClassification`, con la funzione di perdita inclusa quando vengono passate le labels,
renderร il tuo modello direttamente utilizzabile all'interno della classe [`Trainer`]. Utilizzare altri formati di output va bene
se hai in progetto di utilizzare un tuo loop di allenamento, o se utilizzerai un'altra libreria per l'addestramento.
Ora che abbiamo la classe del nostro modello, creiamone uno:
```py
resnet50d = ResnetModelForImageClassification(resnet50d_config)
```
Ribadiamo, puoi usare qualunque metodo dei [`PreTrainedModel`], come [`~PreTrainedModel.save_pretrained`] o
[`~PreTrainedModel.push_to_hub`]. Utilizzeremo quest'ultimo nella prossima sezione, e vedremo come caricare i pesi del
modello assieme al codice del modello stesso. Ma prima, carichiamo alcuni pesi pre-allenati all'interno del nostro modello.
Nel tuo caso specifico, probabilmente allenerai il tuo modello sui tuoi dati. Per velocizzare in questo tutorial,
utilizzeremo la versione pre-allenata del resnet50d. Dato che il nostro modello รจ soltanto un wrapper attorno a quel modello,
sarร facile trasferirne i pesi:
```py
import timm
pretrained_model = timm.create_model("resnet50d", pretrained=True)
resnet50d.model.load_state_dict(pretrained_model.state_dict())
```
Vediamo adesso come assicurarci che quando facciamo [`~PreTrainedModel.save_pretrained`] o [`~PreTrainedModel.push_to_hub`],
il codice del modello venga salvato.
## Inviare il codice all'Hub
<Tip warning={true}>
Questa API รจ sperimentale e potrebbe avere alcuni cambiamenti nei prossimi rilasci.
</Tip>
Innanzitutto, assicurati che il tuo modello sia completamente definito in un file `.py`. Puรฒ sfruttare import relativi
ad altri file, purchรจ questi siano nella stessa directory (non supportiamo ancora sotto-moduli per questa funzionalitร ).
Per questo esempio, definiremo un file `modeling_resnet.py` e un file `configuration_resnet.py` in una cartella dell'attuale
working directory chiamata `resnet_model`. Il file configuration contiene il codice per `ResnetConfig` e il file modeling
contiene il codice di `ResnetModel` e `ResnetModelForImageClassification`.
```
.
โโโ resnet_model
โโโ __init__.py
โโโ configuration_resnet.py
โโโ modeling_resnet.py
```
Il file `__init__.py` puรฒ essere vuoto, serve solo perchรจ Python capisca che `resnet_model` puรฒ essere utilizzato come un modulo.
<Tip warning={true}>
Se stai copiando i file relativi alla modellazione della libreria, dovrai sostituire tutti gli import relativi in cima al file con import del
pacchetto `transformers`.
</Tip>
Nota che puoi ri-utilizzare (o usare come sottoclassi) un modello/configurazione esistente.
Per condividere il tuo modello con la community, segui questi passi: prima importa il modello ResNet e la sua configurazione
dai nuovi file creati:
```py
from resnet_model.configuration_resnet import ResnetConfig
from resnet_model.modeling_resnet import ResnetModel, ResnetModelForImageClassification
```
Dopodichรจ dovrai dire alla libreria che vuoi copiare i file con il codice di quegli oggetti quando utilizzi il metodo
`save_pretrained` e registrarli in modo corretto con una Auto classe (specialmente per i modelli). Utilizza semplicemente:
```py
ResnetConfig.register_for_auto_class()
ResnetModel.register_for_auto_class("AutoModel")
ResnetModelForImageClassification.register_for_auto_class("AutoModelForImageClassification")
```
Nota che non c'รจ bisogno di specificare una Auto classe per la configurazione (c'รจ solo una Auto classe per le configurazioni,
[`AutoConfig`], ma รจ diversa per i modelli). Il tuo modello personalizato potrebbe essere utilizzato per diverse tasks,
per cui devi specificare quale delle classi Auto รจ quella corretta per il tuo modello.
Successivamente, creiamo i modelli e la config come abbiamo fatto in precedenza:
```py
resnet50d_config = ResnetConfig(block_type="bottleneck", stem_width=32, stem_type="deep", avg_down=True)
resnet50d = ResnetModelForImageClassification(resnet50d_config)
pretrained_model = timm.create_model("resnet50d", pretrained=True)
resnet50d.model.load_state_dict(pretrained_model.state_dict())
```
Adesso, per inviare il modello all'Hub, assicurati di aver effettuato l'accesso. Lancia dal tuo terminale:
```bash
huggingface-cli login
```
O da un notebook:
```py
from huggingface_hub import notebook_login
notebook_login()
```
Potrai poi inviare il tutto sul tuo profilo (o di un'organizzazione di cui fai parte) in questo modo:
```py
resnet50d.push_to_hub("custom-resnet50d")
```
Oltre ai pesi del modello e alla configurazione in formato json, questo ha anche copiato i file `.py` modeling e
configuration all'interno della cartella `custom-resnet50d` e ha caricato i risultati sull'Hub. Puoi controllare
i risultati in questa [model repo](https://huggingface.co/sgugger/custom-resnet50d).
Puoi controllare il tutorial di condivisione [tutorial di condivisione](model_sharing) per piรน informazioni sul
metodo con cui inviare all'Hub.
## Usare un modello con codice personalizzato
Puoi usare ogni configurazione, modello o tokenizer con file di codice personalizzati nella sua repository
con le classi Auto e il metodo `from_pretrained`. Tutti i files e il codice caricati sull'Hub sono scansionati da malware
(fai riferimento alla documentazione [Hub security](https://huggingface.co/docs/hub/security#malware-scanning) per piรน informazioni),
ma dovresti comunque assicurarti dell'affidabilitร del codice e dell'autore per evitare di eseguire codice dannoso sulla tua macchina.
Imposta `trust_remote_code=True` per usare un modello con codice personalizzato:
```py
from transformers import AutoModelForImageClassification
model = AutoModelForImageClassification.from_pretrained("sgugger/custom-resnet50d", trust_remote_code=True)
```
Inoltre, raccomandiamo fortemente di passare un hash del commit come `revision` per assicurarti che le autrici o gli autori del modello
non abbiano modificato il codice con alcune nuove righe dannose (a meno che non ti fidi completamente della fonte):
```py
commit_hash = "ed94a7c6247d8aedce4647f00f20de6875b5b292"
model = AutoModelForImageClassification.from_pretrained(
"sgugger/custom-resnet50d", trust_remote_code=True, revision=commit_hash
)
```
Nota che quando cerchi la storia dei commit della repo del modello sull'Hub, c'รจ un bottone con cui facilmente copiare il
commit hash di ciascun commit.
## Registrare un modello con codice personalizzato nelle classi Auto
Se stai scrivendo una libreria che estende ๐ค Transformers, potresti voler estendere le classi Auto per includere il tuo modello.
Questo รจ diverso dall'inviare codice nell'Hub: gli utenti dovranno importare la tua libreria per ottenere il modello personalizzato
(anzichรจ scaricare automaticamente il modello dall'Hub).
Finchรจ il tuo file di configurazione ha un attributo `model_type` diverso dai model types esistenti, e finchรจ le tue
classi modello hanno i corretti attributi `config_class`, potrai semplicemente aggiungerli alle classi Auto come segue:
```py
from transformers import AutoConfig, AutoModel, AutoModelForImageClassification
AutoConfig.register("resnet", ResnetConfig)
AutoModel.register(ResnetConfig, ResnetModel)
AutoModelForImageClassification.register(ResnetConfig, ResnetModelForImageClassification)
```
Nota che il primo argomento utilizzato quando registri la configurazione di un modello personalizzato con [`AutoConfig`]
deve corrispondere al `model_type` della tua configurazione personalizzata, ed il primo argomento utilizzato quando
registri i tuoi modelli personalizzati in una qualunque classe Auto del modello deve corrispondere alla `config_class`
di quei modelli.
| 0 |
hf_public_repos/transformers/docs/source | hf_public_repos/transformers/docs/source/it/perf_hardware.md | <!---
Copyright 2022 The HuggingFace Team. All rights reserved.
Licensed under the Apache License, Version 2.0 (the "License");
you may not use this file except in compliance with the License.
You may obtain a copy of the License at
http://www.apache.org/licenses/LICENSE-2.0
Unless required by applicable law or agreed to in writing, software
distributed under the License is distributed on an "AS IS" BASIS,
WITHOUT WARRANTIES OR CONDITIONS OF ANY KIND, either express or implied.
See the License for the specific language governing permissions and
limitations under the License.
โ ๏ธ Note that this file is in Markdown but contain specific syntax for our doc-builder (similar to MDX) that may not be
rendered properly in your Markdown viewer.
-->
# Hardware ottimizzato per l'addestramento
L'hardware utilizzato per eseguire l'addestramento del modello e l'inferenza puรฒ avere un grande effetto sulle prestazioni. Per un analisi approfondita delle GPUs, assicurati di dare un'occhiata all'eccellente [blog post](https://timdettmers.com/2020/09/07/which-gpu-for-deep-learning/) di Tim Dettmer.
Diamo un'occhiata ad alcuni consigli pratici per la configurazione della GPU.
## GPU
Quando si addestrano modelli piรน grandi ci sono essenzialmente tre opzioni:
- GPUs piu' grandi
- Piu' GPUs
- Piu' CPU e piu' NVMe (scaricato da [DeepSpeed-Infinity](main_classes/deepspeed#nvme-support))
Iniziamo dal caso in cui ci sia una singola GPU.
### Potenza e Raffreddamento
Se hai acquistato una costosa GPU di fascia alta, assicurati di darle la potenza corretta e un raffreddamento sufficiente.
**Potenza**:
Alcune schede GPU consumer di fascia alta hanno 2 e talvolta 3 prese di alimentazione PCI-E a 8 pin. Assicurati di avere tanti cavi PCI-E a 8 pin indipendenti da 12 V collegati alla scheda quante sono le prese. Non utilizzare le 2 fessure a un'estremitร dello stesso cavo (noto anche come cavo a spirale). Cioรจ se hai 2 prese sulla GPU, vuoi 2 cavi PCI-E a 8 pin che vanno dall'alimentatore alla scheda e non uno che abbia 2 connettori PCI-E a 8 pin alla fine! In caso contrario, non otterrai tutte le prestazioni ufficiali.
Ciascun cavo di alimentazione PCI-E a 8 pin deve essere collegato a una guida da 12 V sul lato dell'alimentatore e puรฒ fornire fino a 150 W di potenza.
Alcune altre schede possono utilizzare connettori PCI-E a 12 pin e questi possono fornire fino a 500-600 W di potenza.
Le schede di fascia bassa possono utilizzare connettori a 6 pin, che forniscono fino a 75 W di potenza.
Inoltre vuoi un alimentatore (PSU) di fascia alta che abbia una tensione stabile. Alcuni PSU di qualitร inferiore potrebbero non fornire alla scheda la tensione stabile di cui ha bisogno per funzionare al massimo.
E ovviamente l'alimentatore deve avere abbastanza Watt inutilizzati per alimentare la scheda.
**Raffreddamento**:
Quando una GPU si surriscalda, inizierร a rallentare e non fornirร le prestazioni mssimali e potrebbe persino spegnersi se diventasse troppo calda.
ร difficile dire l'esatta temperatura migliore a cui aspirare quando una GPU รจ molto caricata, ma probabilmente qualsiasi cosa al di sotto di +80ยฐC va bene, ma piรน bassa รจ meglio - forse 70-75ยฐC รจ un intervallo eccellente in cui trovarsi. ร probabile che il rallentamento inizi a circa 84-90ยฐC. Ma oltre alla limitazione delle prestazioni, una temperatura molto elevata prolungata รจ probabile che riduca la durata di una GPU.
Diamo quindi un'occhiata a uno degli aspetti piรน importanti quando si hanno piรน GPU: la connettivitร .
### Connettivitร multi-GPU
Se utilizzi piรน GPU, il modo in cui le schede sono interconnesse puรฒ avere un enorme impatto sul tempo totale di allenamento. Se le GPU si trovano sullo stesso nodo fisico, puoi eseguire:
```
nvidia-smi topo -m
```
e ti dirร come sono interconnesse le GPU. Su una macchina con doppia GPU e collegata a NVLink, molto probabilmente vedrai qualcosa del tipo:
```
GPU0 GPU1 CPU Affinity NUMA Affinity
GPU0 X NV2 0-23 N/A
GPU1 NV2 X 0-23 N/A
```
su una macchina diversa senza NVLink potremmo vedere:
```
GPU0 GPU1 CPU Affinity NUMA Affinity
GPU0 X PHB 0-11 N/A
GPU1 PHB X 0-11 N/A
```
Il rapporto include questa legenda:
```
X = Self
SYS = Connection traversing PCIe as well as the SMP interconnect between NUMA nodes (e.g., QPI/UPI)
NODE = Connection traversing PCIe as well as the interconnect between PCIe Host Bridges within a NUMA node
PHB = Connection traversing PCIe as well as a PCIe Host Bridge (typically the CPU)
PXB = Connection traversing multiple PCIe bridges (without traversing the PCIe Host Bridge)
PIX = Connection traversing at most a single PCIe bridge
NV# = Connection traversing a bonded set of # NVLinks
```
Quindi il primo rapporto `NV2` ci dice che le GPU sono interconnesse con 2 NVLinks e nel secondo report `PHB` abbiamo una tipica configurazione PCIe+Bridge a livello di consumatore.
Controlla che tipo di connettivitร hai sulla tua configurazione. Alcuni di questi renderanno la comunicazione tra le carte piรน veloce (es. NVLink), altri piรน lenta (es. PHB).
A seconda del tipo di soluzione di scalabilitร utilizzata, la velocitร di connettivitร potrebbe avere un impatto maggiore o minore. Se le GPU devono sincronizzarsi raramente, come in DDP, l'impatto di una connessione piรน lenta sarร meno significativo. Se le GPU devono scambiarsi messaggi spesso, come in ZeRO-DP, una connettivitร piรน veloce diventa estremamente importante per ottenere un addestramento piรน veloce.
#### NVlink
[NVLink](https://en.wikipedia.org/wiki/NVLink) รจ un collegamento di comunicazione a corto raggio multilinea seriale basato su cavo sviluppato da Nvidia.
Ogni nuova generazione fornisce una larghezza di banda piรน veloce, ad es. ecco una citazione da [Nvidia Ampere GA102 GPU Architecture](https://www.nvidia.com/content/dam/en-zz/Solutions/geforce/ampere/pdf/NVIDIA-ampere-GA102-GPU-Architecture-Whitepaper-V1.pdf):
> Third-Generation NVLinkยฎ
> GA102 GPUs utilize NVIDIAโs third-generation NVLink interface, which includes four x4 links,
> with each link providing 14.0625 GB/sec bandwidth in each direction between two GPUs. Four
> links provide 56.25 GB/sec bandwidth in each direction, and 112.5 GB/sec total bandwidth
> between two GPUs. Two RTX 3090 GPUs can be connected together for SLI using NVLink.
> (Note that 3-Way and 4-Way SLI configurations are not supported.)
Quindi piรน `X` si ottiene nel rapporto di `NVX` nell'output di `nvidia-smi topo -m`, meglio รจ. La generazione dipenderร dall'architettura della tua GPU.
Confrontiamo l'esecuzione di un training del modello di linguaggio gpt2 su un piccolo campione di wikitext
I risultati sono:
| NVlink | Time |
| ----- | ---: |
| Y | 101s |
| N | 131s |
Puoi vedere che NVLink completa l'addestramento circa il 23% piรน velocemente. Nel secondo benchmark utilizziamo `NCCL_P2P_DISABLE=1` per dire alle GPU di non utilizzare NVLink.
Ecco il codice benchmark completo e gli output:
```bash
# DDP w/ NVLink
rm -r /tmp/test-clm; CUDA_VISIBLE_DEVICES=0,1 python -m torch.distributed.launch \
--nproc_per_node 2 examples/pytorch/language-modeling/run_clm.py --model_name_or_path gpt2 \
--dataset_name wikitext --dataset_config_name wikitext-2-raw-v1 --do_train \
--output_dir /tmp/test-clm --per_device_train_batch_size 4 --max_steps 200
{'train_runtime': 101.9003, 'train_samples_per_second': 1.963, 'epoch': 0.69}
# DDP w/o NVLink
rm -r /tmp/test-clm; CUDA_VISIBLE_DEVICES=0,1 NCCL_P2P_DISABLE=1 python -m torch.distributed.launch \
--nproc_per_node 2 examples/pytorch/language-modeling/run_clm.py --model_name_or_path gpt2 \
--dataset_name wikitext --dataset_config_name wikitext-2-raw-v1 --do_train
--output_dir /tmp/test-clm --per_device_train_batch_size 4 --max_steps 200
{'train_runtime': 131.4367, 'train_samples_per_second': 1.522, 'epoch': 0.69}
```
Hardware: 2x TITAN RTX 24GB each + NVlink with 2 NVLinks (`NV2` in `nvidia-smi topo -m`)
Software: `pytorch-1.8-to-be` + `cuda-11.0` / `transformers==4.3.0.dev0` | 0 |
hf_public_repos/transformers/docs/source | hf_public_repos/transformers/docs/source/it/perf_train_cpu_many.md | <!--Copyright 2022 The HuggingFace Team. All rights reserved.
Licensed under the Apache License, Version 2.0 (the "License"); you may not use this file except in compliance with
the License. You may obtain a copy of the License at
http://www.apache.org/licenses/LICENSE-2.0
Unless required by applicable law or agreed to in writing, software distributed under the License is distributed on
an "AS IS" BASIS, WITHOUT WARRANTIES OR CONDITIONS OF ANY KIND, either express or implied. See the License for the
โ ๏ธ Note that this file is in Markdown but contain specific syntax for our doc-builder (similar to MDX) that may not be
rendered properly in your Markdown viewer.
-->
# Addestramento effciente su multiple CPU
Quando l'addestramento su una singola CPU รจ troppo lento, possiamo usare CPU multiple. Quasta guida si concentra su DDP basato su PyTorch abilitando l'addetramento distribuito su CPU in maniera efficiente.
## Intelยฎ oneCCL Bindings per PyTorch
[Intelยฎ oneCCL](https://github.com/oneapi-src/oneCCL) (collective communications library) รจ una libreria per l'addestramento efficiente del deep learning in distribuito e implementa collettivi come allreduce, allgather, alltoall. Per maggiori informazioni su oneCCL, fai riferimento a [oneCCL documentation](https://spec.oneapi.com/versions/latest/elements/oneCCL/source/index.html) e [oneCCL specification](https://spec.oneapi.com/versions/latest/elements/oneCCL/source/index.html).
Il modulo `oneccl_bindings_for_pytorch` (`torch_ccl` precedentemente alla versione 1.12) implementa PyTorch C10D ProcessGroup API e puรฒ essere caricato dinamicamente com external ProcessGroup e funziona solo su piattaforma Linux al momento.
Qui trovi informazioni piรน dettagliate per [oneccl_bind_pt](https://github.com/intel/torch-ccl).
### Intelยฎ oneCCL Bindings per l'installazione PyTorch:
I file wheel sono disponibili per le seguenti versioni di Python:
| Extension Version | Python 3.6 | Python 3.7 | Python 3.8 | Python 3.9 | Python 3.10 |
| :---------------: | :--------: | :--------: | :--------: | :--------: | :---------: |
| 1.13.0 | | โ | โ | โ | โ |
| 1.12.100 | | โ | โ | โ | โ |
| 1.12.0 | | โ | โ | โ | โ |
| 1.11.0 | | โ | โ | โ | โ |
| 1.10.0 | โ | โ | โ | โ | |
```bash
pip install oneccl_bind_pt=={pytorch_version} -f https://developer.intel.com/ipex-whl-stable-cpu
```
dove `{pytorch_version}` deve essere la tua versione di PyTorch, per l'stanza 1.13.0.
Verifica altri approcci per [oneccl_bind_pt installation](https://github.com/intel/torch-ccl).
Le versioni di oneCCL e PyTorch devono combaciare.
<Tip warning={true}>
oneccl_bindings_for_pytorch 1.12.0 prebuilt wheel does not work with PyTorch 1.12.1 (it is for PyTorch 1.12.0)
PyTorch 1.12.1 should work with oneccl_bindings_for_pytorch 1.12.100
</Tip>
## Intelยฎ MPI library
Usa questa implementazione basata su standard MPI per fornire una architettura flessibile, efficiente, scalabile su cluster per Intelยฎ. Questo componente รจ parte di Intelยฎ oneAPI HPC Toolkit.
oneccl_bindings_for_pytorch รจ installato insieme al set di strumenti MPI. Necessitร di reperire l'ambiente prima di utilizzarlo.
per Intelยฎ oneCCL >= 1.12.0
```bash
oneccl_bindings_for_pytorch_path=$(python -c "from oneccl_bindings_for_pytorch import cwd; print(cwd)")
source $oneccl_bindings_for_pytorch_path/env/setvars.sh
```
per Intelยฎ oneCCL con versione < 1.12.0
```bash
torch_ccl_path=$(python -c "import torch; import torch_ccl; import os; print(os.path.abspath(os.path.dirname(torch_ccl.__file__)))")
source $torch_ccl_path/env/setvars.sh
```
#### Installazione IPEX:
IPEX fornisce ottimizzazioni delle prestazioni per l'addestramento della CPU sia con Float32 che con BFloat16; puoi fare riferimento a [single CPU section](./perf_train_cpu).
Il seguente "Utilizzo in Trainer" prende come esempio mpirun nella libreria Intelยฎ MPI.
## Utilizzo in Trainer
Per abilitare l'addestramento distribuito multi CPU nel Trainer con il ccl backend, gli utenti devono aggiungere **`--ddp_backend ccl`** negli argomenti del comando.
Vediamo un esempio per il [question-answering example](https://github.com/huggingface/transformers/tree/main/examples/pytorch/question-answering)
Il seguente comando abilita due processi sul nodo Xeon, con un processo in esecuzione per ogni socket. Le variabili OMP_NUM_THREADS/CCL_WORKER_COUNT possono essere impostate per una prestazione ottimale.
```shell script
export CCL_WORKER_COUNT=1
export MASTER_ADDR=127.0.0.1
mpirun -n 2 -genv OMP_NUM_THREADS=23 \
python3 run_qa.py \
--model_name_or_path bert-large-uncased \
--dataset_name squad \
--do_train \
--do_eval \
--per_device_train_batch_size 12 \
--learning_rate 3e-5 \
--num_train_epochs 2 \
--max_seq_length 384 \
--doc_stride 128 \
--output_dir /tmp/debug_squad/ \
--no_cuda \
--ddp_backend ccl \
--use_ipex
```
Il seguente comando abilita l'addestramento per un totale di quattro processi su due Xeon (node0 e node1, prendendo node0 come processo principale), ppn (processes per node) รจ impostato a 2, on un processo in esecuzione per ogni socket. Le variabili OMP_NUM_THREADS/CCL_WORKER_COUNT possono essere impostate per una prestazione ottimale.
In node0, รจ necessario creare un file di configurazione che contenga gli indirizzi IP di ciascun nodo (per esempio hostfile) e passare il percorso del file di configurazione come parametro.
```shell script
cat hostfile
xxx.xxx.xxx.xxx #node0 ip
xxx.xxx.xxx.xxx #node1 ip
```
A questo punto, esegui il seguente comando nel nodo0 e **4DDP** sarร abilitato in node0 e node1 con BF16 auto mixed precision:
```shell script
export CCL_WORKER_COUNT=1
export MASTER_ADDR=xxx.xxx.xxx.xxx #node0 ip
mpirun -f hostfile -n 4 -ppn 2 \
-genv OMP_NUM_THREADS=23 \
python3 run_qa.py \
--model_name_or_path bert-large-uncased \
--dataset_name squad \
--do_train \
--do_eval \
--per_device_train_batch_size 12 \
--learning_rate 3e-5 \
--num_train_epochs 2 \
--max_seq_length 384 \
--doc_stride 128 \
--output_dir /tmp/debug_squad/ \
--no_cuda \
--ddp_backend ccl \
--use_ipex \
--bf16
```
| 0 |
hf_public_repos/transformers/docs/source | hf_public_repos/transformers/docs/source/it/perf_infer_gpu_one.md | <!--Copyright 2022 The HuggingFace Team. All rights reserved.
Licensed under the Apache License, Version 2.0 (the "License"); you may not use this file except in compliance with
the License. You may obtain a copy of the License at
http://www.apache.org/licenses/LICENSE-2.0
Unless required by applicable law or agreed to in writing, software distributed under the License is distributed on
an "AS IS" BASIS, WITHOUT WARRANTIES OR CONDITIONS OF ANY KIND, either express or implied. See the License for the
โ ๏ธ Note that this file is in Markdown but contain specific syntax for our doc-builder (similar to MDX) that may not be
rendered properly in your Markdown viewer.
-->
# Inferenza efficiente su GPU singola
Questo documento sarร presto completato con informazioni su come effetture l'inferenza su una singola GPU. Nel frattempo รจ possibile consultare [la guida per l'addestramento su una singola GPU](perf_train_gpu_one) e [la guida per l'inferenza su CPU](perf_infer_cpu).
## `BetterTransformer` per l'inferenza piรน veloce
Abbiamo recentemente integrato `BetterTransformer` per velocizzare l'inferenza su GPU per modelli di testo, immagini e audio. Per maggiori dettagli, consultare la documentazione su questa integrazione [qui](https://huggingface.co/docs/optimum/bettertransformer/overview).
## Integrazione di `bitsandbytes` per Int8 mixed-precision matrix decomposition
<Tip>
Nota che questa funzione puรฒ essere utilizzata anche nelle configurazioni multi GPU.
</Tip>
Dal paper [`LLM.int8() : 8-bit Matrix Multiplication for Transformers at Scale`](https://arxiv.org/abs/2208.07339), noi supportiamo l'integrazione di Hugging Face per tutti i modelli dell'Hub con poche righe di codice.
Il metodo `nn.Linear` riduce la dimensione di 2 per i pesi `float16` e `bfloat16` e di 4 per i pesi `float32`, con un impatto quasi nullo sulla qualitร , operando sugli outlier in half-precision.

Il metodo Int8 mixed-precision matrix decomposition funziona separando la moltiplicazione tra matrici in due flussi: (1) una matrice di flusso di outlier di caratteristiche sistematiche moltiplicata in fp16, (2) in flusso regolare di moltiplicazione di matrici int8 (99,9%). Con questo metodo, รจ possibile effettutare inferenza int8 per modelli molto grandi senza degrado predittivo.
Per maggiori dettagli sul metodo, consultare il [paper](https://arxiv.org/abs/2208.07339) o il nostro [blogpost sull'integrazione](https://huggingface.co/blog/hf-bitsandbytes-integration).

Nota che รจ necessaria una GPU per eseguire modelli di tipo mixed-8bit, poichรฉ i kernel sono stati compilati solo per le GPU. Prima di utilizzare questa funzione, assicurarsi di disporre di memoria sufficiente sulla GPU per memorizzare un quarto del modello (o la metร se i pesi del modello sono in mezza precisione).
Di seguito sono riportate alcune note per aiutarvi a utilizzare questo modulo, oppure seguite le dimostrazioni su [Google colab](#colab-demos).
### Requisiti
- Se si dispone di `bitsandbytes<0.37.0`, assicurarsi di eseguire su GPU NVIDIA che supportano tensor cores a 8 bit (Turing, Ampere o architetture piรน recenti - ad esempio T4, RTX20s RTX30s, A40-A100). Per `bitsandbytes>=0.37.0`, tutte le GPU dovrebbero essere supportate.
- Installare la versione corretta di `bitsandbytes` eseguendo:
`pip install bitsandbytes>=0.31.5`.
- Installare `accelerate`
`pip install accelerate>=0.12.0`
### Esecuzione di modelli mixed-Int8 - configurazione per singola GPU
Dopo aver installato le librerie necessarie, per caricare il tuo modello mixed 8-bit รจ il seguente:
```py
from transformers import AutoModelForCausalLM
model_name = "bigscience/bloom-2b5"
model_8bit = AutoModelForCausalLM.from_pretrained(model_name, device_map="auto", load_in_8bit=True)
```
Per la generazione di testo, si consiglia di:
* utilizzare il metodo `generate()` del modello invece della funzione `pipeline()`. Sebbene l'inferenza sia possibile con la funzione `pipeline()`, essa non รจ ottimizzata per i modelli mixed-8bit e sarร piรน lenta rispetto all'uso del metodo `generate()`. Inoltre, alcune strategie di campionamento, come il campionamento nucleaus, non sono supportate dalla funzione `pipeline()` per i modelli mixed-8bit.
* collocare tutti gli ingressi sullo stesso dispositivo del modello.
Ecco un semplice esempio:
```py
from transformers import AutoModelForCausalLM, AutoTokenizer
model_name = "bigscience/bloom-2b5"
tokenizer = AutoTokenizer.from_pretrained(model_name)
model_8bit = AutoModelForCausalLM.from_pretrained(model_name, device_map="auto", load_in_8bit=True)
text = "Hello, my llama is cute"
inputs = tokenizer(prompt, return_tensors="pt").to("cuda")
generated_ids = model.generate(**inputs)
outputs = tokenizer.batch_decode(generated_ids, skip_special_tokens=True)
```
### Esecuzione di modelli mixed-8bit - configurazione multi GPU
Usare il seguente modo caricare il modello mixed-8bit su piรน GPU (stesso comando della configurazione a GPU singola):
```py
model_name = "bigscience/bloom-2b5"
model_8bit = AutoModelForCausalLM.from_pretrained(model_name, device_map="auto", load_in_8bit=True)
```
Puoi controllare la RAM della GPU che si vuole allocare su ogni GPU usando `accelerate`. Utilizzare l'argomento `max_memory` come segue:
```py
max_memory_mapping = {0: "1GB", 1: "2GB"}
model_name = "bigscience/bloom-3b"
model_8bit = AutoModelForCausalLM.from_pretrained(
model_name, device_map="auto", load_in_8bit=True, max_memory=max_memory_mapping
)
```
In questo esempio, la prima GPU utilizzerร 1 GB di memoria e la seconda 2 GB.
### Colab demos
Con questo metodo รจ possibile inferire modelli che prima non era possibile inferire su Google Colab.
Guardate la demo per l'esecuzione di T5-11b (42GB in fp32)! Utilizzo la quantizzazione a 8 bit su Google Colab:
[](https://colab.research.google.com/drive/1YORPWx4okIHXnjW7MSAidXN29mPVNT7F?usp=sharing)
Oppure questa demo di BLOOM-3B:
[](https://colab.research.google.com/drive/1qOjXfQIAULfKvZqwCen8-MoWKGdSatZ4?usp=sharing) | 0 |
hf_public_repos/transformers/docs/source | hf_public_repos/transformers/docs/source/it/quicktour.md | <!--Copyright 2022 The HuggingFace Team. All rights reserved.
Licensed under the Apache License, Version 2.0 (the "License"); you may not use this file except in compliance with
the License. You may obtain a copy of the License at
http://www.apache.org/licenses/LICENSE-2.0
Unless required by applicable law or agreed to in writing, software distributed under the License is distributed on
an "AS IS" BASIS, WITHOUT WARRANTIES OR CONDITIONS OF ANY KIND, either express or implied. See the License for the
specific language governing permissions and limitations under the License.
โ ๏ธ Note that this file is in Markdown but contain specific syntax for our doc-builder (similar to MDX) that may not be
rendered properly in your Markdown viewer.
-->
# Quick tour
[[open-in-colab]]
Entra in azione con ๐ค Transformers! Inizia utilizzando [`pipeline`] per un'inferenza veloce, carica un modello pre-allenato e un tokenizer con una [AutoClass](./model_doc/auto) per risolvere i tuoi compiti legati a testo, immagini o audio.
<Tip>
Tutti gli esempi di codice presenti in questa documentazione hanno un pulsante in alto a sinistra che permette di selezionare tra PyTorch e TensorFlow. Se
questo non รจ presente, ci si aspetta che il codice funzioni per entrambi i backend senza alcun cambiamento.
</Tip>
## Pipeline
[`pipeline`] รจ il modo piรน semplice per utilizzare un modello pre-allenato per un dato compito.
<Youtube id="tiZFewofSLM"/>
La [`pipeline`] supporta molti compiti comuni:
**Testo**:
* Analisi del Sentimento (Sentiment Analysis, in inglese): classifica la polaritร di un testo dato.
* Generazione del Testo (Text Generation, in inglese): genera del testo a partire da un dato input.
* Riconoscimento di Entitร (Name Entity Recognition o NER, in inglese): etichetta ogni parola con l'entitร che questa rappresenta (persona, data, luogo, ecc.).
* Rispondere a Domande (Question answering, in inglese): estrae la risposta da un contesto, dato del contesto e una domanda.
* Riempimento di Maschere (Fill-mask, in inglese): riempie gli spazi mancanti in un testo che ha parole mascherate.
* Riassumere (Summarization, in inglese): genera una sintesi di una lunga sequenza di testo o di un documento.
* Traduzione (Translation, in inglese): traduce un testo in un'altra lingua.
* Estrazione di Caratteristiche (Feature Extraction, in inglese): crea un tensore che rappresenta un testo.
**Immagini**:
* Classificazione di Immagini (Image Classification, in inglese): classifica un'immagine.
* Segmentazione di Immagini (Image Segmentation, in inglese): classifica ogni pixel di un'immagine.
* Rilevazione di Oggetti (Object Detection, in inglese): rileva oggetti all'interno di un'immagine.
**Audio**:
* Classificazione di Audio (Audio Classification, in inglese): assegna un'etichetta ad un segmento di audio dato.
* Riconoscimento Vocale Automatico (Automatic Speech Recognition o ASR, in inglese): trascrive il contenuto di un audio dato in un testo.
<Tip>
Per maggiori dettagli legati alla [`pipeline`] e ai compiti ad essa associati, fai riferimento alla documentazione [qui](./main_classes/pipelines).
</Tip>
### Utilizzo della Pipeline
Nel seguente esempio, utilizzerai la [`pipeline`] per l'analisi del sentimento.
Installa le seguenti dipendenze se non lo hai giร fatto:
<frameworkcontent>
<pt>
```bash
pip install torch
```
</pt>
<tf>
```bash
pip install tensorflow
```
</tf>
</frameworkcontent>
Importa [`pipeline`] e specifica il compito che vuoi completare:
```py
>>> from transformers import pipeline
>>> classificatore = pipeline("sentiment-analysis", model="MilaNLProc/feel-it-italian-sentiment")
```
La pipeline scarica e salva il [modello pre-allenato](https://huggingface.co/MilaNLProc/feel-it-italian-sentiment) e il tokenizer per l'analisi del sentimento. Se non avessimo scelto un modello, la pipeline ne avrebbe scelto uno di default. Ora puoi utilizzare il `classifier` sul tuo testo obiettivo:
```py
>>> classificatore("Siamo molto felici di mostrarti la libreria ๐ค Transformers.")
[{'label': 'positive', 'score': 0.9997}]
```
Per piรน di una frase, passa una lista di frasi alla [`pipeline`] la quale restituirร una lista di dizionari:
```py
>>> risultati = classificatore(
... ["Siamo molto felici di mostrarti la libreria ๐ค Transformers.", "Speriamo te non la odierai."]
... )
>>> for risultato in risultati:
... print(f"etichetta: {risultato['label']}, con punteggio: {round(risultato['score'], 4)}")
etichetta: positive, con punteggio: 0.9998
etichetta: negative, con punteggio: 0.9998
```
La [`pipeline`] puรฒ anche iterare su un dataset intero. Inizia installando la libreria [๐ค Datasets](https://huggingface.co/docs/datasets/):
```bash
pip install datasets
```
Crea una [`pipeline`] con il compito che vuoi risolvere e con il modello che vuoi utilizzare.
```py
>>> import torch
>>> from transformers import pipeline
>>> riconoscitore_vocale = pipeline(
... "automatic-speech-recognition", model="radiogroup-crits/wav2vec2-xls-r-1b-italian-doc4lm-5gram"
... )
```
Poi, carica un dataset (vedi ๐ค Datasets [Quick Start](https://huggingface.co/docs/datasets/quickstart.html) per maggiori dettagli) sul quale vuoi iterare. Per esempio, carichiamo il dataset [MInDS-14](https://huggingface.co/datasets/PolyAI/minds14):
```py
>>> from datasets import load_dataset, Audio
>>> dataset = load_dataset("PolyAI/minds14", name="it-IT", split="train") # doctest: +IGNORE_RESULT
```
Dobbiamo assicurarci che la frequenza di campionamento del set di dati corrisponda alla frequenza di campionamento con cui รจ stato addestrato `radiogroup-crits/wav2vec2-xls-r-1b-italian-doc4lm-5gram`.
```py
>>> dataset = dataset.cast_column("audio", Audio(sampling_rate=riconoscitore_vocale.feature_extractor.sampling_rate))
```
I file audio vengono caricati automaticamente e ri-campionati quando chiamiamo la colonna "audio".
Estraiamo i vettori delle forme d'onda grezze delle prime 4 osservazioni e passiamoli come lista alla pipeline:
```py
>>> risultato = riconoscitore_vocale(dataset[:4]["audio"])
>>> print([d["text"] for d in risultato])
['dovrei caricare dei soldi sul mio conto corrente', 'buongiorno e senza vorrei depositare denaro sul mio conto corrente come devo fare per cortesia', 'sรฌ salve vorrei depositare del denaro sul mio conto', 'e buon pomeriggio vorrei depositare dei soldi sul mio conto bancario volleo sapere come posso fare se e posso farlo online ed un altro conto o andandoo tramite bancomut']
```
Per un dataset piรน grande dove gli input sono di dimensione maggiore (come nel parlato/audio o nella visione), dovrai passare un generatore al posto di una lista che carica tutti gli input in memoria. Guarda la [documentazione della pipeline](./main_classes/pipelines) per maggiori informazioni.
### Utilizzare un altro modello e tokenizer nella pipeline
La [`pipeline`] puรฒ ospitare qualsiasi modello del [Model Hub](https://huggingface.co/models), rendendo semplice l'adattamento della [`pipeline`] per altri casi d'uso. Per esempio, se si vuole un modello capace di trattare testo in francese, usa i tag presenti nel Model Hub in modo da filtrare per ottenere un modello appropriato. Il miglior risultato filtrato restituisce un modello multi-lingua [BERT model](https://huggingface.co/nlptown/bert-base-multilingual-uncased-sentiment) fine-tuned per l'analisi del sentimento. Ottimo, utilizziamo questo modello!
```py
>>> model_name = "nlptown/bert-base-multilingual-uncased-sentiment"
```
<frameworkcontent>
<pt>
Usa [`AutoModelForSequenceClassification`] e [`AutoTokenizer`] per caricare il modello pre-allenato e il suo tokenizer associato (maggiori informazioni su una `AutoClass` in seguito):
```py
>>> from transformers import AutoTokenizer, AutoModelForSequenceClassification
>>> model = AutoModelForSequenceClassification.from_pretrained(model_name)
>>> tokenizer = AutoTokenizer.from_pretrained(model_name)
```
</pt>
<tf>
Usa [`TFAutoModelForSequenceClassification`] e [`AutoTokenizer`] per caricare il modello pre-allenato e il suo tokenizer associato (maggiori informazioni su una `TFAutoClass` in seguito):
```py
>>> from transformers import AutoTokenizer, TFAutoModelForSequenceClassification
>>> model = TFAutoModelForSequenceClassification.from_pretrained(model_name)
>>> tokenizer = AutoTokenizer.from_pretrained(model_name)
```
</tf>
</frameworkcontent>
Poi puoi specificare il modello e il tokenizer nella [`pipeline`], e applicare il `classifier` sul tuo testo obiettivo:
```py
>>> classifier = pipeline("sentiment-analysis", model=model, tokenizer=tokenizer)
>>> classifier("Nous sommes trรจs heureux de vous prรฉsenter la bibliothรจque ๐ค Transformers.")
[{'label': '5 stars', 'score': 0.7273}]
```
Se non riesci a trovare un modello per il tuo caso d'uso, dovrai fare fine-tuning di un modello pre-allenato sui tuoi dati. Dai un'occhiata al nostro tutorial [fine-tuning tutorial](./training) per imparare come. Infine, dopo che hai completato il fine-tuning del tuo modello pre-allenato, considera per favore di condividerlo (vedi il tutorial [qui](./model_sharing)) con la comunitร sul Model Hub per democratizzare l'NLP! ๐ค
## AutoClass
<Youtube id="AhChOFRegn4"/>
Al suo interno, le classi [`AutoModelForSequenceClassification`] e [`AutoTokenizer`] lavorano assieme per dare potere alla [`pipeline`]. Una [AutoClass](./model_doc/auto) รจ una scorciatoia che automaticamente recupera l'architettura di un modello pre-allenato a partire dal suo nome o path. Hai solo bisogno di selezionare la `AutoClass` appropriata per il tuo compito e il suo tokenizer associato con [`AutoTokenizer`].
Ritorniamo al nostro esempio e vediamo come puoi utilizzare la `AutoClass` per replicare i risultati della [`pipeline`].
### AutoTokenizer
Un tokenizer รจ responsabile dell'elaborazione del testo in modo da trasformarlo in un formato comprensibile dal modello. Per prima cosa, il tokenizer dividerร il testo in parole chiamate *token*. Ci sono diverse regole che governano il processo di tokenizzazione, tra cui come dividere una parola e a quale livello (impara di piรน sulla tokenizzazione [qui](./tokenizer_summary)). La cosa piรน importante da ricordare comunque รจ che hai bisogno di inizializzare il tokenizer con lo stesso nome del modello in modo da assicurarti che stai utilizzando le stesse regole di tokenizzazione con cui il modello รจ stato pre-allenato.
Carica un tokenizer con [`AutoTokenizer`]:
```py
>>> from transformers import AutoTokenizer
>>> nome_del_modello = "nlptown/bert-base-multilingual-uncased-sentiment"
>>> tokenizer = AutoTokenizer.from_pretrained(nome_del_modello)
```
Dopodichรฉ, il tokenizer converte i token in numeri in modo da costruire un tensore come input del modello. Questo รจ conosciuto come il *vocabolario* del modello.
Passa il tuo testo al tokenizer:
```py
>>> encoding = tokenizer("Siamo molto felici di mostrarti la libreria ๐ค Transformers.")
>>> print(encoding)
{'input_ids': [101, 56821, 10132, 14407, 13019, 13007, 10120, 47201, 10330, 10106, 91686, 100, 58263, 119, 102],
'token_type_ids': [0, 0, 0, 0, 0, 0, 0, 0, 0, 0, 0, 0, 0, 0, 0],
'attention_mask': [1, 1, 1, 1, 1, 1, 1, 1, 1, 1, 1, 1, 1, 1, 1]}
```
Il tokenizer restituirร un dizionario contenente:
* [input_ids](./glossary#input-ids): rappresentazioni numeriche dei tuoi token.
* [attention_mask](.glossary#attention-mask): indica quali token devono essere presi in considerazione.
Come con la [`pipeline`], il tokenizer accetterร una lista di input. In piรน, il tokenizer puรฒ anche completare (pad, in inglese) e troncare il testo in modo da restituire un lotto (batch, in inglese) di lunghezza uniforme:
<frameworkcontent>
<pt>
```py
>>> pt_batch = tokenizer(
... ["Siamo molto felici di mostrarti la libreria ๐ค Transformers.", "Speriamo te non la odierai."],
... padding=True,
... truncation=True,
... max_length=512,
... return_tensors="pt",
... )
```
</pt>
<tf>
```py
>>> tf_batch = tokenizer(
... ["Siamo molto felici di mostrarti la libreria ๐ค Transformers.", "Speriamo te non la odierai."],
... padding=True,
... truncation=True,
... max_length=512,
... return_tensors="tf",
... )
```
</tf>
</frameworkcontent>
Leggi il tutorial sul [preprocessing](./preprocessing) per maggiori dettagli sulla tokenizzazione.
### AutoModel
<frameworkcontent>
<pt>
๐ค Transformers fornisce un metodo semplice e unificato per caricare istanze pre-allenate. Questo significa che puoi caricare un [`AutoModel`] come caricheresti un [`AutoTokenizer`]. L'unica differenza รจ selezionare l'[`AutoModel`] corretto per il compito di interesse. Dato che stai facendo classificazione di testi, o sequenze, carica [`AutoModelForSequenceClassification`]:
```py
>>> from transformers import AutoModelForSequenceClassification
>>> model_name = "nlptown/bert-base-multilingual-uncased-sentiment"
>>> pt_model = AutoModelForSequenceClassification.from_pretrained(model_name)
```
<Tip>
Guarda il [task summary](./task_summary) per sapere quale classe di [`AutoModel`] utilizzare per quale compito.
</Tip>
Ora puoi passare il tuo lotto di input pre-processati direttamente al modello. Devi solo spacchettare il dizionario aggiungendo `**`:
```py
>>> pt_outputs = pt_model(**pt_batch)
```
Il modello produrrร le attivazioni finali nell'attributo `logits`. Applica la funzione softmax a `logits` per ottenere le probabilitร :
```py
>>> from torch import nn
>>> pt_predictions = nn.functional.softmax(pt_outputs.logits, dim=-1)
>>> print(pt_predictions)
tensor([[0.0041, 0.0037, 0.0203, 0.2005, 0.7713],
[0.3766, 0.3292, 0.1832, 0.0558, 0.0552]], grad_fn=<SoftmaxBackward0>)
```
</pt>
<tf>
๐ค Transformers fornisce un metodo semplice e unificato per caricare istanze pre-allenate. Questo significa che puoi caricare un [`TFAutoModel`] come caricheresti un [`AutoTokenizer`]. L'unica differenza รจ selezionare il [`TFAutoModel`] corretto per il compito di interesse. Dato che stai facendo classificazione di testi, o sequenze, carica [`TFAutoModelForSequenceClassification`]:
```py
>>> from transformers import TFAutoModelForSequenceClassification
>>> nome_del_modello = "nlptown/bert-base-multilingual-uncased-sentiment"
>>> tf_model = TFAutoModelForSequenceClassification.from_pretrained(nome_del_modello)
```
<Tip>
Guarda il [task summary](./task_summary) per sapere quale classe di [`AutoModel`] utilizzare per quale compito.
</Tip>
Ora puoi passare il tuo lotto di input pre-processati direttamente al modello passando le chiavi del dizionario al tensore:
```py
>>> tf_outputs = tf_model(tf_batch)
```
Il modello produrrร le attivazioni finali nell'attributo `logits`. Applica la funzione softmax a `logits` per ottenere le probabilitร :
```py
>>> import tensorflow as tf
>>> tf_predictions = tf.nn.softmax(tf_outputs.logits, axis=-1)
>>> tf_predictions # doctest: +IGNORE_RESULT
```
</tf>
</frameworkcontent>
<Tip>
Tutti i modelli di ๐ค Transformers (PyTorch e TensorFlow) restituiscono i tensori *prima* della funzione finale
di attivazione (come la softmax) perchรฉ la funzione di attivazione finale viene spesso unita a quella di perdita.
</Tip>
I modelli sono [`torch.nn.Module`](https://pytorch.org/docs/stable/nn.html#torch.nn.Module) o [`tf.keras.Model`](https://www.tensorflow.org/api_docs/python/tf/keras/Model) standard cosรฌ puoi utilizzarli all'interno del tuo training loop usuale. Tuttavia, per rendere le cose piรน semplici, ๐ค Transformers fornisce una classe [`Trainer`] per PyTorch che aggiunge delle funzionalitร per l'allenamento distribuito, precisione mista, e altro ancora. Per TensorFlow, puoi utilizzare il metodo `fit` di [Keras](https://keras.io/). Fai riferimento al [tutorial per il training](./training) per maggiori dettagli.
<Tip>
Gli output del modello di ๐ค Transformers sono delle dataclasses speciali in modo che i loro attributi vengano auto-completati all'interno di un IDE.
Gli output del modello si comportano anche come una tupla o un dizionario (ad esempio, puoi indicizzare con un intero, una slice o una stringa) nel qual caso gli attributi che sono `None` vengono ignorati.
</Tip>
### Salva un modello
<frameworkcontent>
<pt>
Una volta completato il fine-tuning del tuo modello, puoi salvarlo con il suo tokenizer utilizzando [`PreTrainedModel.save_pretrained`]:
```py
>>> pt_save_directory = "./pt_save_pretrained"
>>> tokenizer.save_pretrained(pt_save_directory) # doctest: +IGNORE_RESULT
>>> pt_model.save_pretrained(pt_save_directory)
```
Quando desideri utilizzare il tuo modello nuovamente, puoi ri-caricarlo con [`PreTrainedModel.from_pretrained`]:
```py
>>> pt_model = AutoModelForSequenceClassification.from_pretrained("./pt_save_pretrained")
```
</pt>
<tf>
Una volta completato il fine-tuning del tuo modello, puoi salvarlo con il suo tokenizer utilizzando [`TFPreTrainedModel.save_pretrained`]:
```py
>>> tf_save_directory = "./tf_save_pretrained"
>>> tokenizer.save_pretrained(tf_save_directory) # doctest: +IGNORE_RESULT
>>> tf_model.save_pretrained(tf_save_directory)
```
Quando desideri utilizzare il tuo modello nuovamente, puoi ri-caricarlo con [`TFPreTrainedModel.from_pretrained`]:
```py
>>> tf_model = TFAutoModelForSequenceClassification.from_pretrained("./tf_save_pretrained")
```
</tf>
</frameworkcontent>
Una caratteristica particolarmente interessante di ๐ค Transformers รจ la sua abilitร di salvare un modello e ri-caricarlo sia come modello di PyTorch che di TensorFlow. I parametri `from_pt` o `from_tf` possono convertire un modello da un framework all'altro:
<frameworkcontent>
<pt>
```py
>>> from transformers import AutoModel
>>> tokenizer = AutoTokenizer.from_pretrained(tf_save_directory)
>>> pt_model = AutoModelForSequenceClassification.from_pretrained(tf_save_directory, from_tf=True)
```
</pt>
<tf>
```py
>>> from transformers import TFAutoModel
>>> tokenizer = AutoTokenizer.from_pretrained(pt_save_directory)
>>> tf_model = TFAutoModelForSequenceClassification.from_pretrained(pt_save_directory, from_pt=True)
```
</tf>
</frameworkcontent>
| 0 |
hf_public_repos/transformers/docs/source | hf_public_repos/transformers/docs/source/it/run_scripts.md | <!--Copyright 2022 The HuggingFace Team. All rights reserved.
Licensed under the Apache License, Version 2.0 (the "License"); you may not use this file except in compliance with
the License. You may obtain a copy of the License at
http://www.apache.org/licenses/LICENSE-2.0
Unless required by applicable law or agreed to in writing, software distributed under the License is distributed on
an "AS IS" BASIS, WITHOUT WARRANTIES OR CONDITIONS OF ANY KIND, either express or implied. See the License for the
specific language governing permissions and limitations under the License.
โ ๏ธ Note that this file is in Markdown but contain specific syntax for our doc-builder (similar to MDX) that may not be
rendered properly in your Markdown viewer.
-->
# Addestramento con script
Insieme ai [notebooks](./noteboks/README) ๐ค Transformers, ci sono anche esempi di script che dimostrano come addestrare un modello per un task con [PyTorch](https://github.com/huggingface/transformers/tree/main/examples/pytorch), [TensorFlow](https://github.com/huggingface/transformers/tree/main/examples/tensorflow), o [JAX/Flax](https://github.com/huggingface/transformers/tree/main/examples/flax).
Troverai anche script che abbiamo usato nei nostri [progetti di ricerca](https://github.com/huggingface/transformers/tree/main/examples/research_projects) e [precedenti esempi](https://github.com/huggingface/transformers/tree/main/examples/legacy) a cui contribuisce per lo piรน la comunitร . Questi script non sono attivamente mantenuti e richiedono una specifica versione di ๐ค Transformers che sarร molto probabilmente incompatibile con l'ultima versione della libreria.
Non รจ dato per scontato che gli script di esempio funzionino senza apportare modifiche per ogni problema, bensรฌ potrebbe essere necessario adattare lo script al tuo caso specifico. Per aiutarti in ciรฒ, la maggioranza degli script espone le modalitร di pre-processamento dei dati, consentendoti di modificare lo script come preferisci.
Per qualsiasi feature che vorresti implementare in uno script d'esempio, per favore discutine nel [forum](https://discuss.huggingface.co/) o in un'[issue](https://github.com/huggingface/transformers/issues) prima di inviare una Pull Request. Mentre accogliamo con piacere la correzione di bug, รจ piรน improbabile che faremo la stessa con una PR che aggiunge funzionalitร sacrificando la leggibilitร .
Questa guida ti mostrerร come eseguire uno script di esempio relativo al task di summarization in [PyTorch](https://github.com/huggingface/transformers/tree/main/examples/pytorch/summarization) e [TensorFlow](https://github.com/huggingface/transformers/tree/main/examples/tensorflow/summarization). Tutti gli esempi funzioneranno con entrambi i framework a meno che non sia specificato altrimenti.
## Installazione
Per eseguire con successo l'ultima versione degli script di esempio, devi **installare ๐ค Transformers dalla fonte** in un nuovo ambiente virtuale:
```bash
git clone https://github.com/huggingface/transformers
cd transformers
pip install .
```
Per le precedenti versioni degli script di esempio, clicca sul pulsante di seguito:
<details>
<summary>Esempi per versioni precedenti di ๐ค Transformers</summary>
<ul>
<li><a href="https://github.com/huggingface/transformers/tree/v4.5.1/examples">v4.5.1</a></li>
<li><a href="https://github.com/huggingface/transformers/tree/v4.4.2/examples">v4.4.2</a></li>
<li><a href="https://github.com/huggingface/transformers/tree/v4.3.3/examples">v4.3.3</a></li>
<li><a href="https://github.com/huggingface/transformers/tree/v4.2.2/examples">v4.2.2</a></li>
<li><a href="https://github.com/huggingface/transformers/tree/v4.1.1/examples">v4.1.1</a></li>
<li><a href="https://github.com/huggingface/transformers/tree/v4.0.1/examples">v4.0.1</a></li>
<li><a href="https://github.com/huggingface/transformers/tree/v3.5.1/examples">v3.5.1</a></li>
<li><a href="https://github.com/huggingface/transformers/tree/v3.4.0/examples">v3.4.0</a></li>
<li><a href="https://github.com/huggingface/transformers/tree/v3.3.1/examples">v3.3.1</a></li>
<li><a href="https://github.com/huggingface/transformers/tree/v3.2.0/examples">v3.2.0</a></li>
<li><a href="https://github.com/huggingface/transformers/tree/v3.1.0/examples">v3.1.0</a></li>
<li><a href="https://github.com/huggingface/transformers/tree/v3.0.2/examples">v3.0.2</a></li>
<li><a href="https://github.com/huggingface/transformers/tree/v2.11.0/examples">v2.11.0</a></li>
<li><a href="https://github.com/huggingface/transformers/tree/v2.10.0/examples">v2.10.0</a></li>
<li><a href="https://github.com/huggingface/transformers/tree/v2.9.1/examples">v2.9.1</a></li>
<li><a href="https://github.com/huggingface/transformers/tree/v2.8.0/examples">v2.8.0</a></li>
<li><a href="https://github.com/huggingface/transformers/tree/v2.7.0/examples">v2.7.0</a></li>
<li><a href="https://github.com/huggingface/transformers/tree/v2.6.0/examples">v2.6.0</a></li>
<li><a href="https://github.com/huggingface/transformers/tree/v2.5.1/examples">v2.5.1</a></li>
<li><a href="https://github.com/huggingface/transformers/tree/v2.4.0/examples">v2.4.0</a></li>
<li><a href="https://github.com/huggingface/transformers/tree/v2.3.0/examples">v2.3.0</a></li>
<li><a href="https://github.com/huggingface/transformers/tree/v2.2.0/examples">v2.2.0</a></li>
<li><a href="https://github.com/huggingface/transformers/tree/v2.1.0/examples">v2.1.1</a></li>
<li><a href="https://github.com/huggingface/transformers/tree/v2.0.0/examples">v2.0.0</a></li>
<li><a href="https://github.com/huggingface/transformers/tree/v1.2.0/examples">v1.2.0</a></li>
<li><a href="https://github.com/huggingface/transformers/tree/v1.1.0/examples">v1.1.0</a></li>
<li><a href="https://github.com/huggingface/transformers/tree/v1.0.0/examples">v1.0.0</a></li>
</ul>
</details>
Successivamente, cambia la tua attuale copia di ๐ค Transformers specificandone la versione, ad esempio v3.5.1:
```bash
git checkout tags/v3.5.1
```
Dopo aver configurato correttamente la versione della libreria, naviga nella cartella degli esempi di tua scelta e installa i requisiti:
```bash
pip install -r requirements.txt
```
## Esegui uno script
<frameworkcontent>
<pt>
Lo script di esempio scarica e pre-processa un dataset dalla libreria ๐ค [Datasets](https://huggingface.co/docs/datasets/). Successivamente, lo script esegue il fine-tuning su un dataset usando il [Trainer](https://huggingface.co/docs/transformers/main_classes/trainer) su un'architettura che supporta la summarization. Il seguente esempio mostra come eseguire il fine-tuning di [T5-small](https://huggingface.co/t5-small) sul dataset [CNN/DailyMail](https://huggingface.co/datasets/cnn_dailymail). Il modello T5 richiede un parametro addizionale `source_prefix` a causa del modo in cui รจ stato addestrato. Questo prefisso permette a T5 di sapere che si tratta di un task di summarization.
```bash
python examples/pytorch/summarization/run_summarization.py \
--model_name_or_path t5-small \
--do_train \
--do_eval \
--dataset_name cnn_dailymail \
--dataset_config "3.0.0" \
--source_prefix "summarize: " \
--output_dir /tmp/tst-summarization \
--per_device_train_batch_size=4 \
--per_device_eval_batch_size=4 \
--overwrite_output_dir \
--predict_with_generate
```
</pt>
<tf>
Lo script di esempio scarica e pre-processa un dataset dalla libreria ๐ค [Datasets](https://huggingface.co/docs/datasets/). Successivamente, lo script esegue il fine-tuning su un dataset usando Keras su un'architettura che supporta la summarization. Il seguente esempio mostra come eseguire il fine-tuning di [T5-small](https://huggingface.co/t5-small) sul dataset [CNN/DailyMail](https://huggingface.co/datasets/cnn_dailymail). Il modello T5 richiede un parametro addizionale `source_prefix` a causa del modo in cui รจ stato addestrato. Questo prefisso permette a T5 di sapere che si tratta di un task di summarization.
```bash
python examples/tensorflow/summarization/run_summarization.py \
--model_name_or_path t5-small \
--dataset_name cnn_dailymail \
--dataset_config "3.0.0" \
--output_dir /tmp/tst-summarization \
--per_device_train_batch_size 8 \
--per_device_eval_batch_size 16 \
--num_train_epochs 3 \
--do_train \
--do_eval
```
</tf>
</frameworkcontent>
## Addestramento distribuito e precisione mista
Il [Trainer](https://huggingface.co/docs/transformers/main_classes/trainer) supporta l'addestramento distribuito e la precisione mista, che significa che puoi anche usarla in uno script. Per abilitare entrambe le funzionalitร :
- Aggiunto l'argomento `fp16` per abilitare la precisione mista.
- Imposta un numero di GPU da usare con l'argomento `nproc_per_node`.
```bash
python -m torch.distributed.launch \
--nproc_per_node 8 pytorch/summarization/run_summarization.py \
--fp16 \
--model_name_or_path t5-small \
--do_train \
--do_eval \
--dataset_name cnn_dailymail \
--dataset_config "3.0.0" \
--source_prefix "summarize: " \
--output_dir /tmp/tst-summarization \
--per_device_train_batch_size=4 \
--per_device_eval_batch_size=4 \
--overwrite_output_dir \
--predict_with_generate
```
Gli script TensorFlow utilizzano una [`MirroredStrategy`](https://www.tensorflow.org/guide/distributed_training#mirroredstrategy) per il training distribuito e non devi aggiungere alcun argomento addizionale allo script di training. Lo script TensorFlow userร multiple GPU in modo predefinito se quest'ultime sono disponibili:
## Esegui uno script su TPU
<frameworkcontent>
<pt>
Le Tensor Processing Units (TPU) sono state progettate per migliorare le prestazioni. PyTorch supporta le TPU con il compilatore per deep learning [XLA](https://www.tensorflow.org/xla) (guarda [questo link](https://github.com/pytorch/xla/blob/master/README.md) per maggiori dettagli). Per usare una TPU, avvia lo script `xla_spawn.py` e usa l'argomento `num_cores` per impostare il numero di core TPU che intendi usare.
```bash
python xla_spawn.py --num_cores 8 \
summarization/run_summarization.py \
--model_name_or_path t5-small \
--do_train \
--do_eval \
--dataset_name cnn_dailymail \
--dataset_config "3.0.0" \
--source_prefix "summarize: " \
--output_dir /tmp/tst-summarization \
--per_device_train_batch_size=4 \
--per_device_eval_batch_size=4 \
--overwrite_output_dir \
--predict_with_generate
```
</pt>
<tf>
Le Tensor Processing Units (TPU) sono state progettate per migliorare le prestazioni. Gli script TensorFlow utilizzano una [`TPUStrategy`](https://www.tensorflow.org/guide/distributed_training#tpustrategy) per eseguire l'addestramento su TPU. Per usare una TPU, passa il nome della risorsa TPU all'argomento `tpu`.
```bash
python run_summarization.py \
--tpu name_of_tpu_resource \
--model_name_or_path t5-small \
--dataset_name cnn_dailymail \
--dataset_config "3.0.0" \
--output_dir /tmp/tst-summarization \
--per_device_train_batch_size 8 \
--per_device_eval_batch_size 16 \
--num_train_epochs 3 \
--do_train \
--do_eval
```
</tf>
</frameworkcontent>
## Esegui uno script con ๐ค Accelerate
๐ค [Accelerate](https://huggingface.co/docs/accelerate) รจ una libreria compatibile solo con PyTorch che offre un metodo unificato per addestrare modelli su diverse tipologie di configurazioni (CPU, multiple GPU, TPU) mantenendo una completa visibilitร rispetto al ciclo di training di PyTorch. Assicurati di aver effettuato l'installazione di ๐ค Accelerate, nel caso non lo avessi fatto:
> Nota: dato che Accelerate รจ in rapido sviluppo, รจ necessario installare la versione proveniente da git per eseguire gli script:
```bash
pip install git+https://github.com/huggingface/accelerate
```
Invece che usare lo script `run_summarization.py`, devi usare lo script `run_summarization_no_trainer.py`. Gli script supportati in ๐ค Accelerate avranno un file chiamato `task_no_trainer.py` nella rispettiva cartella. Per iniziare, esegui il seguente comando per creare e salvare un file di configurazione:
```bash
accelerate config
```
Testa la tua configurazione per assicurarti della sua correttezza:
```bash
accelerate test
```
Ora sei pronto per avviare l'addestramento:
```bash
accelerate launch run_summarization_no_trainer.py \
--model_name_or_path t5-small \
--dataset_name cnn_dailymail \
--dataset_config "3.0.0" \
--source_prefix "summarize: " \
--output_dir ~/tmp/tst-summarization
```
## Uso di un dataset personalizzato
Lo script di summarization supporta dataset personalizzati purchรฉ siano file CSV o JSON Line. Quando usi il tuo dataset, devi specificare diversi argomenti aggiuntivi:
- `train_file` e `validation_file` specificano dove si trovano i file di addestramento e validazione.
- `text_column` รจ il file di input da riassumere.
- `summary_column` รจ il file di destinazione per l'output.
Uno script di summarization usando un dataset personalizzato sarebbe simile a questo:
```bash
python examples/pytorch/summarization/run_summarization.py \
--model_name_or_path t5-small \
--do_train \
--do_eval \
--train_file path_to_csv_or_jsonlines_file \
--validation_file path_to_csv_or_jsonlines_file \
--text_column text_column_name \
--summary_column summary_column_name \
--source_prefix "summarize: " \
--output_dir /tmp/tst-summarization \
--overwrite_output_dir \
--per_device_train_batch_size=4 \
--per_device_eval_batch_size=4 \
--predict_with_generate
```
## Testare uno script
ร spesso una buona idea avviare il tuo script su un numero inferiore di esempi tratti dal dataset, per assicurarti che tutto funzioni come previsto prima di eseguire lo script sull'intero dataset, che potrebbe necessitare di ore. Usa i seguenti argomenti per limitare il dataset ad un massimo numero di esempi:
- `max_train_samples`
- `max_eval_samples`
- `max_predict_samples`
```bash
python examples/pytorch/summarization/run_summarization.py \
--model_name_or_path t5-small \
--max_train_samples 50 \
--max_eval_samples 50 \
--max_predict_samples 50 \
--do_train \
--do_eval \
--dataset_name cnn_dailymail \
--dataset_config "3.0.0" \
--source_prefix "summarize: " \
--output_dir /tmp/tst-summarization \
--per_device_train_batch_size=4 \
--per_device_eval_batch_size=4 \
--overwrite_output_dir \
--predict_with_generate
```
Non tutti gli esempi di script supportano l'argomento `max_predict_samples`. Se non sei sicuro circa il supporto di questo argomento da parte del tuo script, aggiungi l'argomento `-h` per controllare:
```bash
examples/pytorch/summarization/run_summarization.py -h
```
## Riavviare addestramento da un checkpoint
Un'altra utile opzione รจ riavviare un addestramento da un checkpoint precedente. Questo garantirร che tu possa riprendere da dove hai interrotto senza ricominciare se l'addestramento viene interrotto. Ci sono due metodi per riavviare l'addestramento da un checkpoint:
Il primo metodo usa l'argomento `output_dir previous_output_dir` per riavviare l'addestramento dall'ultima versione del checkpoint contenuto in `output_dir`. In questo caso, dovresti rimuovere `overwrite_output_dir`:
```bash
python examples/pytorch/summarization/run_summarization.py
--model_name_or_path t5-small \
--do_train \
--do_eval \
--dataset_name cnn_dailymail \
--dataset_config "3.0.0" \
--source_prefix "summarize: " \
--output_dir /tmp/tst-summarization \
--per_device_train_batch_size=4 \
--per_device_eval_batch_size=4 \
--output_dir previous_output_dir \
--predict_with_generate
```
Il secondo metodo usa l'argomento `resume_from_checkpoint path_to_specific_checkpoint` per riavviare un addestramento da una specifica cartella di checkpoint.
```bash
python examples/pytorch/summarization/run_summarization.py
--model_name_or_path t5-small \
--do_train \
--do_eval \
--dataset_name cnn_dailymail \
--dataset_config "3.0.0" \
--source_prefix "summarize: " \
--output_dir /tmp/tst-summarization \
--per_device_train_batch_size=4 \
--per_device_eval_batch_size=4 \
--overwrite_output_dir \
--resume_from_checkpoint path_to_specific_checkpoint \
--predict_with_generate
```
## Condividi il tuo modello
Tutti gli script possono caricare il tuo modello finale al [Model Hub](https://huggingface.co/models). Prima di iniziare, assicurati di aver effettuato l'accesso su Hugging Face:
```bash
huggingface-cli login
```
Poi, aggiungi l'argomento `push_to_hub` allo script. Questo argomento consentirร di creare un repository con il tuo username Hugging Face e la cartella specificata in `output_dir`.
Per dare uno specifico nome al repository, usa l'argomento `push_to_hub_model_id`. Il repository verrร automaticamente elencata sotto al tuo namespace.
Il seguente esempio mostra come caricare un modello specificando il nome del repository:
```bash
python examples/pytorch/summarization/run_summarization.py
--model_name_or_path t5-small \
--do_train \
--do_eval \
--dataset_name cnn_dailymail \
--dataset_config "3.0.0" \
--source_prefix "summarize: " \
--push_to_hub \
--push_to_hub_model_id finetuned-t5-cnn_dailymail \
--output_dir /tmp/tst-summarization \
--per_device_train_batch_size=4 \
--per_device_eval_batch_size=4 \
--overwrite_output_dir \
--predict_with_generate
```
| 0 |
hf_public_repos/transformers/docs/source | hf_public_repos/transformers/docs/source/it/index.md | <!--Copyright 2020 The HuggingFace Team. All rights reserved.
Licensed under the Apache License, Version 2.0 (the "License"); you may not use this file except in compliance with
the License. You may obtain a copy of the License at
http://www.apache.org/licenses/LICENSE-2.0
Unless required by applicable law or agreed to in writing, software distributed under the License is distributed on
an "AS IS" BASIS, WITHOUT WARRANTIES OR CONDITIONS OF ANY KIND, either express or implied. See the License for the
specific language governing permissions and limitations under the License.
โ ๏ธ Note that this file is in Markdown but contain specific syntax for our doc-builder (similar to MDX) that may not be
rendered properly in your Markdown viewer.
-->
# ๐ค Transformers
Machine Learning allo stato dell'arte per PyTorch, TensorFlow e JAX.
๐ค Transformers fornisce delle API per scaricare in modo semplice e allenare modelli pre-allenati allo stato dell'arte. L'utilizzo di modelli pre-allenati puรฒ ridurre i tuoi costi computazionali, l'impatto ambientale, e farti risparmiare il tempo che utilizzeresti per allenare un modello da zero. I modelli possono essere utilizzati in diverse modalitร come ad esempio:
* ๐ Testo: classificazione del testo, estrazione delle informazioni, rispondere a domande, riassumere, traduzione e generazione del testo in piรน di 100 lingue.
* ๐ผ๏ธ Immagini: classificazione di immagini, rilevazione di oggetti e segmentazione.
* ๐ฃ๏ธ Audio: riconoscimento vocale e classificazione dell'audio.
* ๐ Multimodale: rispondere a domande inerenti dati tabulari, riconoscimento ottico dei caratteri, estrazione di informazioni a partire da documenti scannerizzati, classificazione di video e risposta visuale a domande.
La nostra libreria supporta un'integrazione perfetta tra tre delle librerie per il deep learning piรน popolari: [PyTorch](https://pytorch.org/), [TensorFlow](https://www.tensorflow.org/) e [JAX](https://jax.readthedocs.io/en/latest/). Allena il tuo modello in tre righe di codice in un framework, e caricalo per l'inferenza in un altro.
Ogni architettura di ๐ค Transformers รจ definita in un modulo Python indipendente cosรฌ da poter essere personalizzata in modo semplice per la ricerca e gli esperimenti.
## Se stai cercando supporto personalizzato dal team di Hugging Face
<a target="_blank" href="https://huggingface.co/support">
<img alt="HuggingFace Expert Acceleration Program" src="https://huggingface.co/front/thumbnails/support.png" style="width: 100%; max-width: 600px; border: 1px solid #eee; border-radius: 4px; box-shadow: 0 1px 2px 0 rgba(0, 0, 0, 0.05);">
</a>
## Contenuti
La documentazione รจ organizzata in cinque parti:
- **INIZIARE** contiene un tour rapido e le istruzioni di installazione per cominciare ad utilizzare ๐ค Transformers.
- **TUTORIALS** รจ un buon posto da cui iniziare se per te la nostra libreria รจ nuova. Questa sezione ti aiuterร ad acquisire le competenze basilari di cui hai bisogno per iniziare ad utilizzare ๐ค Transformers.
- **GUIDE PRATICHE** ti mostrerร come raggiungere obiettivi specifici come fare fine-tuning di un modello pre-allenato per la modellizzazione del linguaggio o come creare una testa per un modello personalizzato.
- **GUIDE CONCETTUALI** fornisce discussioni e spiegazioni dei concetti sottostanti alle idee dietro ai modelli, compiti, e la filosofia di progettazione di ๐ค Transformers.
- **API** descrive ogni classe e funzione, raggruppate in:
- **CLASSI PRINCIPALI** per le classi principali che espongono le API importanti della libreria.
- **MODELLI** per le classi e le funzioni relative ad ogni modello implementato all'interno della libreria.
- **HELPERS INTERNI** per le classi e le funzioni che utilizziamo internamente.
La libreria attualmente contiene implementazioni in JAX, PyTorch e TensorFlow, pesi di modelli pre-allenati, script di utilizzo e strumenti di conversione per i seguenti modelli.
### Modelli supportati
<!--This list is updated automatically from the README with _make fix-copies_. Do not update manually! -->
1. **[ALBERT](model_doc/albert)** (da Google Research e l'Istituto Tecnologico di Chicago) rilasciato con il paper [ALBERT: A Lite BERT for Self-supervised Learning of Language Representations](https://arxiv.org/abs/1909.11942), da Zhenzhong Lan, Mingda Chen, Sebastian Goodman, Kevin Gimpel, Piyush Sharma, Radu Soricut.
1. **[ALIGN](model_doc/align)** (from Google Research) rilasciato con il paper [Scaling Up Visual and Vision-Language Representation Learning With Noisy Text Supervision](https://arxiv.org/abs/2102.05918) da Chao Jia, Yinfei Yang, Ye Xia, Yi-Ting Chen, Zarana Parekh, Hieu Pham, Quoc V. Le, Yunhsuan Sung, Zhen Li, Tom Duerig.
1. **[BART](model_doc/bart)** (da Facebook) rilasciato con il paper [BART: Denoising Sequence-to-Sequence Pre-training for Natural Language Generation, Translation, and Comprehension](https://arxiv.org/abs/1910.13461) da Mike Lewis, Yinhan Liu, Naman Goyal, Marjan Ghazvininejad, Abdelrahman Mohamed, Omer Levy, Ves Stoyanov e Luke Zettlemoyer.
1. **[BARThez](model_doc/barthez)** (da politecnico di รcole) rilasciato con il paper [BARThez: a Skilled Pretrained French Sequence-to-Sequence Model](https://arxiv.org/abs/2010.12321) da Moussa Kamal Eddine, Antoine J.-P. Tixier, Michalis Vazirgiannis.
1. **[BARTpho](model_doc/bartpho)** (da VinAI Research) rilasciato con il paper [BARTpho: Pre-trained Sequence-to-Sequence Models for Vietnamese](https://arxiv.org/abs/2109.09701) da Nguyen Luong Tran, Duong Minh Le e Dat Quoc Nguyen.
1. **[BEiT](model_doc/beit)** (da Microsoft) rilasciato con il paper [BEiT: BERT Pre-Training of Image Transformers](https://arxiv.org/abs/2106.08254) da Hangbo Bao, Li Dong, Furu Wei.
1. **[BERT](model_doc/bert)** (da Google) rilasciato con il paper [BERT: Pre-training of Deep Bidirectional Transformers for Language Understanding](https://arxiv.org/abs/1810.04805) da Jacob Devlin, Ming-Wei Chang, Kenton Lee e Kristina Toutanova.
1. **[BERTweet](model_doc/bertweet)** (da VinAI Research) rilasciato con il paper [BERTweet: A pre-trained language model for English Tweets](https://aclanthology.org/2020.emnlp-demos.2/) da Dat Quoc Nguyen, Thanh Vu e Anh Tuan Nguyen.
1. **[BERT For Sequence Generation](model_doc/bert-generation)** (da Google) rilasciato con il paper [Leveraging Pre-trained Checkpoints for Sequence Generation Tasks](https://arxiv.org/abs/1907.12461) da Sascha Rothe, Shashi Narayan, Aliaksei Severyn.
1. **[BigBird-RoBERTa](model_doc/big_bird)** (da Google Research) rilasciato con il paper [Big Bird: Transformers for Longer Sequences](https://arxiv.org/abs/2007.14062) da Manzil Zaheer, Guru Guruganesh, Avinava Dubey, Joshua Ainslie, Chris Alberti, Santiago Ontanon, Philip Pham, Anirudh Ravula, Qifan Wang, Li Yang, Amr Ahmed.
1. **[BigBird-Pegasus](model_doc/bigbird_pegasus)** (v Google Research) rilasciato con il paper [Big Bird: Transformers for Longer Sequences](https://arxiv.org/abs/2007.14062) da Manzil Zaheer, Guru Guruganesh, Avinava Dubey, Joshua Ainslie, Chris Alberti, Santiago Ontanon, Philip Pham, Anirudh Ravula, Qifan Wang, Li Yang, Amr Ahmed.
1. **[Blenderbot](model_doc/blenderbot)** (da Facebook) rilasciato con il paper [Recipes for building an open-domain chatbot](https://arxiv.org/abs/2004.13637) da Stephen Roller, Emily Dinan, Naman Goyal, Da Ju, Mary Williamson, Yinhan Liu, Jing Xu, Myle Ott, Kurt Shuster, Eric M. Smith, Y-Lan Boureau, Jason Weston.
1. **[BlenderbotSmall](model_doc/blenderbot-small)** (da Facebook) rilasciato con il paper [Recipes for building an open-domain chatbot](https://arxiv.org/abs/2004.13637) da Stephen Roller, Emily Dinan, Naman Goyal, Da Ju, Mary Williamson, Yinhan Liu, Jing Xu, Myle Ott, Kurt Shuster, Eric M. Smith, Y-Lan Boureau, Jason Weston.
1. **[BORT](model_doc/bort)** (da Alexa) rilasciato con il paper [Optimal Subarchitecture Extraction For BERT](https://arxiv.org/abs/2010.10499) da Adrian de Wynter e Daniel J. Perry.
1. **[ByT5](model_doc/byt5)** (da Google Research) rilasciato con il paper [ByT5: Towards a token-free future with pre-trained byte-to-byte models](https://arxiv.org/abs/2105.13626) da Linting Xue, Aditya Barua, Noah Constant, Rami Al-Rfou, Sharan Narang, Mihir Kale, Adam Roberts, Colin Raffel.
1. **[CamemBERT](model_doc/camembert)** (da Inria/Facebook/Sorbonne) rilasciato con il paper [CamemBERT: a Tasty French Language Model](https://arxiv.org/abs/1911.03894) da Louis Martin*, Benjamin Muller*, Pedro Javier Ortiz Suรกrez*, Yoann Dupont, Laurent Romary, รric Villemonte de la Clergerie, Djamรฉ Seddah e Benoรฎt Sagot.
1. **[CANINE](model_doc/canine)** (da Google Research) rilasciato con il paper [CANINE: Pre-training an Efficient Tokenization-Free Encoder for Language Representation](https://arxiv.org/abs/2103.06874) da Jonathan H. Clark, Dan Garrette, Iulia Turc, John Wieting.
1. **[ConvNeXT](model_doc/convnext)** (da Facebook AI) rilasciato con il paper [A ConvNet for the 2020s](https://arxiv.org/abs/2201.03545) da Zhuang Liu, Hanzi Mao, Chao-Yuan Wu, Christoph Feichtenhofer, Trevor Darrell, Saining Xie.
1. **[ConvNeXTV2](model_doc/convnextv2)** (da Facebook AI) rilasciato con il paper [ConvNeXt V2: Co-designing and Scaling ConvNets with Masked Autoencoders](https://arxiv.org/abs/2301.00808) da Sanghyun Woo, Shoubhik Debnath, Ronghang Hu, Xinlei Chen, Zhuang Liu, In So Kweon, Saining Xie.
1. **[CLIP](model_doc/clip)** (da OpenAI) rilasciato con il paper [Learning Transferable Visual Models From Natural Language Supervision](https://arxiv.org/abs/2103.00020) da Alec Radford, Jong Wook Kim, Chris Hallacy, Aditya Ramesh, Gabriel Goh, Sandhini Agarwal, Girish Sastry, Amanda Askell, Pamela Mishkin, Jack Clark, Gretchen Krueger, Ilya Sutskever.
1. **[ConvBERT](model_doc/convbert)** (da YituTech) rilasciato con il paper [ConvBERT: Improving BERT with Span-based Dynamic Convolution](https://arxiv.org/abs/2008.02496) da Zihang Jiang, Weihao Yu, Daquan Zhou, Yunpeng Chen, Jiashi Feng, Shuicheng Yan.
1. **[CPM](model_doc/cpm)** (dalla Universitร di Tsinghua) rilasciato con il paper [CPM: A Large-scale Generative Chinese Pre-trained Language Model](https://arxiv.org/abs/2012.00413) da Zhengyan Zhang, Xu Han, Hao Zhou, Pei Ke, Yuxian Gu, Deming Ye, Yujia Qin, Yusheng Su, Haozhe Ji, Jian Guan, Fanchao Qi, Xiaozhi Wang, Yanan Zheng, Guoyang Zeng, Huanqi Cao, Shengqi Chen, Daixuan Li, Zhenbo Sun, Zhiyuan Liu, Minlie Huang, Wentao Han, Jie Tang, Juanzi Li, Xiaoyan Zhu, Maosong Sun.
1. **[CTRL](model_doc/ctrl)** (da Salesforce) rilasciato con il paper [CTRL: A Conditional Transformer Language Model for Controllable Generation](https://arxiv.org/abs/1909.05858) da Nitish Shirish Keskar*, Bryan McCann*, Lav R. Varshney, Caiming Xiong e Richard Socher.
1. **[CvT](model_doc/cvt)** (da Microsoft) rilasciato con il paper [CvT: Introducing Convolutions to Vision Transformers](https://arxiv.org/abs/2103.15808) da Haiping Wu, Bin Xiao, Noel Codella, Mengchen Liu, Xiyang Dai, Lu Yuan, Lei Zhang.
1. **[Data2Vec](model_doc/data2vec)** (da Facebook) rilasciato con il paper [Data2Vec: A General Framework for Self-supervised Learning in Speech, Vision and Language](https://arxiv.org/abs/2202.03555) da Alexei Baevski, Wei-Ning Hsu, Qiantong Xu, Arun Babu, Jiatao Gu, Michael Auli.
1. **[DeBERTa](model_doc/deberta)** (da Microsoft) rilasciato con il paper [DeBERTa: Decoding-enhanced BERT with Disentangled Attention](https://arxiv.org/abs/2006.03654) da Pengcheng He, Xiaodong Liu, Jianfeng Gao, Weizhu Chen.
1. **[DeBERTa-v2](model_doc/deberta-v2)** (da Microsoft) rilasciato con il paper [DeBERTa: Decoding-enhanced BERT with Disentangled Attention](https://arxiv.org/abs/2006.03654) da Pengcheng He, Xiaodong Liu, Jianfeng Gao, Weizhu Chen.
1. **[Decision Transformer](model_doc/decision_transformer)** (da Berkeley/Facebook/Google) rilasciato con il paper [Decision Transformer: Reinforcement Learning via Sequence Modeling](https://arxiv.org/abs/2106.01345) da Lili Chen, Kevin Lu, Aravind Rajeswaran, Kimin Lee, Aditya Grover, Michael Laskin, Pieter Abbeel, Aravind Srinivas, Igor Mordatch.
1. **[DiT](model_doc/dit)** (da Microsoft Research) rilasciato con il paper [DiT: Self-supervised Pre-training for Document Image Transformer](https://arxiv.org/abs/2203.02378) da Junlong Li, Yiheng Xu, Tengchao Lv, Lei Cui, Cha Zhang, Furu Wei.
1. **[DeiT](model_doc/deit)** (da Facebook) rilasciato con il paper [Training data-efficient image transformers & distillation through attention](https://arxiv.org/abs/2012.12877) da Hugo Touvron, Matthieu Cord, Matthijs Douze, Francisco Massa, Alexandre Sablayrolles, Hervรฉ Jรฉgou.
1. **[DETR](model_doc/detr)** (da Facebook) rilasciato con il paper [End-to-End Object Detection with Transformers](https://arxiv.org/abs/2005.12872) da Nicolas Carion, Francisco Massa, Gabriel Synnaeve, Nicolas Usunier, Alexander Kirillov, Sergey Zagoruyko.
1. **[DialoGPT](model_doc/dialogpt)** (da Microsoft Research) rilasciato con il paper [DialoGPT: Large-Scale Generative Pre-training for Conversational Response Generation](https://arxiv.org/abs/1911.00536) da Yizhe Zhang, Siqi Sun, Michel Galley, Yen-Chun Chen, Chris Brockett, Xiang Gao, Jianfeng Gao, Jingjing Liu, Bill Dolan.
1. **[DistilBERT](model_doc/distilbert)** (da HuggingFace), rilasciato assieme al paper [DistilBERT, a distilled version of BERT: smaller, faster, cheaper and lighter](https://arxiv.org/abs/1910.01108) da Victor Sanh, Lysandre Debut e Thomas Wolf. La stessa tecnica รจ stata applicata per comprimere GPT2 in [DistilGPT2](https://github.com/huggingface/transformers/tree/main/examples/research_projects/distillation), RoBERTa in [DistilRoBERTa](https://github.com/huggingface/transformers/tree/main/examples/research_projects/distillation), Multilingual BERT in [DistilmBERT](https://github.com/huggingface/transformers/tree/main/examples/research_projects/distillation) and a German version of DistilBERT.
1. **[DPR](model_doc/dpr)** (da Facebook) rilasciato con il paper [Dense Passage Retrieval for Open-Domain Question Answering](https://arxiv.org/abs/2004.04906) da Vladimir Karpukhin, Barlas Oฤuz, Sewon Min, Patrick Lewis, Ledell Wu, Sergey Edunov, Danqi Chen, e Wen-tau Yih.
1. **[DPT](master/model_doc/dpt)** (da Intel Labs) rilasciato con il paper [Vision Transformers for Dense Prediction](https://arxiv.org/abs/2103.13413) da Renรฉ Ranftl, Alexey Bochkovskiy, Vladlen Koltun.
1. **[EfficientNet](model_doc/efficientnet)** (from Google Research) released with the paper [EfficientNet: Rethinking Model Scaling for Convolutional Neural Networks](https://arxiv.org/abs/1905.11946) by Mingxing Tan and Quoc V. Le.
1. **[EncoderDecoder](model_doc/encoder-decoder)** (da Google Research) rilasciato con il paper [Leveraging Pre-trained Checkpoints for Sequence Generation Tasks](https://arxiv.org/abs/1907.12461) da Sascha Rothe, Shashi Narayan, Aliaksei Severyn.
1. **[ELECTRA](model_doc/electra)** (da Google Research/Stanford University) rilasciato con il paper [ELECTRA: Pre-training text encoders as discriminators rather than generators](https://arxiv.org/abs/2003.10555) da Kevin Clark, Minh-Thang Luong, Quoc V. Le, Christopher D. Manning.
1. **[FlauBERT](model_doc/flaubert)** (da CNRS) rilasciato con il paper [FlauBERT: Unsupervised Language Model Pre-training for French](https://arxiv.org/abs/1912.05372) da Hang Le, Loรฏc Vial, Jibril Frej, Vincent Segonne, Maximin Coavoux, Benjamin Lecouteux, Alexandre Allauzen, Benoรฎt Crabbรฉ, Laurent Besacier, Didier Schwab.
1. **[FLAVA](model_doc/flava)** (da Facebook AI) rilasciato con il paper [FLAVA: A Foundational Language And Vision Alignment Model](https://arxiv.org/abs/2112.04482) da Amanpreet Singh, Ronghang Hu, Vedanuj Goswami, Guillaume Couairon, Wojciech Galuba, Marcus Rohrbach, e Douwe Kiela.
1. **[FNet](model_doc/fnet)** (da Google Research) rilasciato con il paper [FNet: Mixing Tokens with Fourier Transforms](https://arxiv.org/abs/2105.03824) da James Lee-Thorp, Joshua Ainslie, Ilya Eckstein, Santiago Ontanon.
1. **[Funnel Transformer](model_doc/funnel)** (da CMU/Google Brain) rilasciato con il paper [Funnel-Transformer: Filtering out Sequential Redundancy for Efficient Language Processing](https://arxiv.org/abs/2006.03236) da Zihang Dai, Guokun Lai, Yiming Yang, Quoc V. Le.
1. **[GLPN](model_doc/glpn)** (da KAIST) rilasciato con il paper [Global-Local Path Networks for Monocular Depth Estimation with Vertical CutDepth](https://arxiv.org/abs/2201.07436) da Doyeon Kim, Woonghyun Ga, Pyungwhan Ahn, Donggyu Joo, Sehwan Chun, Junmo Kim.
1. **[GPT](model_doc/openai-gpt)** (da OpenAI) rilasciato con il paper [Improving Language Understanding by Generative Pre-Training](https://blog.openai.com/language-unsupervised/) da Alec Radford, Karthik Narasimhan, Tim Salimans e Ilya Sutskever.
1. **[GPT-2](model_doc/gpt2)** (da OpenAI) rilasciato con il paper [Language Models are Unsupervised Multitask Learners](https://blog.openai.com/better-language-models/) da Alec Radford*, Jeffrey Wu*, Rewon Child, David Luan, Dario Amodei** e Ilya Sutskever**.
1. **[GPT-J](model_doc/gptj)** (da EleutherAI) rilasciato nel repository [kingoflolz/mesh-transformer-jax](https://github.com/kingoflolz/mesh-transformer-jax/) da Ben Wang e Aran Komatsuzaki.
1. **[GPT Neo](model_doc/gpt_neo)** (da EleutherAI) rilasciato nel repository [EleutherAI/gpt-neo](https://github.com/EleutherAI/gpt-neo) da Sid Black, Stella Biderman, Leo Gao, Phil Wang e Connor Leahy.
1. **[GPT NeoX](model_doc/gpt_neox)** (da EleutherAI) rilasciato con il paper [GPT-NeoX-20B: An Open-Source Autoregressive Language Model](https://arxiv.org/abs/2204.06745) da Sid Black, Stella Biderman, Eric Hallahan, Quentin Anthony, Leo Gao, Laurence Golding, Horace He, Connor Leahy, Kyle McDonell, Jason Phang, Michael Pieler, USVSN Sai Prashanth, Shivanshu Purohit, Laria Reynolds, Jonathan Tow, Ben Wang, Samuel Weinbach
1. **[Hubert](model_doc/hubert)** (da Facebook) rilasciato con il paper [HuBERT: Self-Supervised Speech Representation Learning by Masked Prediction of Hidden Units](https://arxiv.org/abs/2106.07447) da Wei-Ning Hsu, Benjamin Bolte, Yao-Hung Hubert Tsai, Kushal Lakhotia, Ruslan Salakhutdinov, Abdelrahman Mohamed.
1. **[I-BERT](model_doc/ibert)** (da Berkeley) rilasciato con il paper [I-BERT: Integer-only BERT Quantization](https://arxiv.org/abs/2101.01321) da Sehoon Kim, Amir Gholami, Zhewei Yao, Michael W. Mahoney, Kurt Keutzer.
1. **[ImageGPT](model_doc/imagegpt)** (da OpenAI) rilasciato con il paper [Generative Pretraining from Pixels](https://openai.com/blog/image-gpt/) da Mark Chen, Alec Radford, Rewon Child, Jeffrey Wu, Heewoo Jun, David Luan, Ilya Sutskever.
1. **[LayoutLM](model_doc/layoutlm)** (da Microsoft Research Asia) rilasciato con il paper [LayoutLM: Pre-training of Text and Layout for Document Image Understanding](https://arxiv.org/abs/1912.13318) da Yiheng Xu, Minghao Li, Lei Cui, Shaohan Huang, Furu Wei, Ming Zhou.
1. **[LayoutLMv2](model_doc/layoutlmv2)** (da Microsoft Research Asia) rilasciato con il paper [LayoutLMv2: Multi-modal Pre-training for Visually-Rich Document Understanding](https://arxiv.org/abs/2012.14740) da Yang Xu, Yiheng Xu, Tengchao Lv, Lei Cui, Furu Wei, Guoxin Wang, Yijuan Lu, Dinei Florencio, Cha Zhang, Wanxiang Che, Min Zhang, Lidong Zhou.
1. **[LayoutLMv3](model_doc/layoutlmv3)** (da Microsoft Research Asia) rilasciato con il paper [LayoutLMv3: Pre-training for Document AI with Unified Text and Image Masking](https://arxiv.org/abs/2204.08387) da Yupan Huang, Tengchao Lv, Lei Cui, Yutong Lu, Furu Wei.
1. **[LayoutXLM](model_doc/layoutlxlm)** (da Microsoft Research Asia) rilasciato con il paper [LayoutXLM: Multimodal Pre-training for Multilingual Visually-rich Document Understanding](https://arxiv.org/abs/2104.08836) da Yiheng Xu, Tengchao Lv, Lei Cui, Guoxin Wang, Yijuan Lu, Dinei Florencio, Cha Zhang, Furu Wei.
1. **[LED](model_doc/led)** (da AllenAI) rilasciato con il paper [Longformer: The Long-Document Transformer](https://arxiv.org/abs/2004.05150) da Iz Beltagy, Matthew E. Peters, Arman Cohan.
1. **[Longformer](model_doc/longformer)** (da AllenAI) rilasciato con il paper [Longformer: The Long-Document Transformer](https://arxiv.org/abs/2004.05150) da Iz Beltagy, Matthew E. Peters, Arman Cohan.
1. **[LUKE](model_doc/luke)** (da Studio Ousia) rilasciato con il paper [LUKE: Deep Contextualized Entity Representations with Entity-aware Self-attention](https://arxiv.org/abs/2010.01057) da Ikuya Yamada, Akari Asai, Hiroyuki Shindo, Hideaki Takeda, Yuji Matsumoto.
1. **[mLUKE](model_doc/mluke)** (da Studio Ousia) rilasciato con il paper [mLUKE: The Power of Entity Representations in Multilingual Pretrained Language Models](https://arxiv.org/abs/2110.08151) da Ryokan Ri, Ikuya Yamada, e Yoshimasa Tsuruoka.
1. **[LXMERT](model_doc/lxmert)** (da UNC Chapel Hill) rilasciato con il paper [LXMERT: Learning Cross-Modality Encoder Representations from Transformers for Open-Domain Question Answering](https://arxiv.org/abs/1908.07490) da Hao Tan e Mohit Bansal.
1. **[M2M100](model_doc/m2m_100)** (da Facebook) rilasciato con il paper [Beyond English-Centric Multilingual Machine Translation](https://arxiv.org/abs/2010.11125) da Angela Fan, Shruti Bhosale, Holger Schwenk, Zhiyi Ma, Ahmed El-Kishky, Siddharth Goyal, Mandeep Baines, Onur Celebi, Guillaume Wenzek, Vishrav Chaudhary, Naman Goyal, Tom Birch, Vitaliy Liptchinsky, Sergey Edunov, Edouard Grave, Michael Auli, Armand Joulin.
1. **[MarianMT](model_doc/marian)** Modello di machine learning per le traduzioni allenato utilizzando i dati [OPUS](http://opus.nlpl.eu/) di Jรถrg Tiedemann. Il [Framework Marian](https://marian-nmt.github.io/) รจ stato sviluppato dal Microsoft Translator Team.
1. **[Mask2Former](model_doc/mask2former)** (da FAIR e UIUC) rilasciato con il paper [Masked-attention Mask Transformer for Universal Image Segmentation](https://arxiv.org/abs/2112.01527) da Bowen Cheng, Ishan Misra, Alexander G. Schwing, Alexander Kirillov, Rohit Girdhar.
1. **[MaskFormer](model_doc/maskformer)** (da Meta e UIUC) rilasciato con il paper [Per-Pixel Classification is Not All You Need for Semantic Segmentation](https://arxiv.org/abs/2107.06278) da Bowen Cheng, Alexander G. Schwing, Alexander Kirillov.
1. **[MBart](model_doc/mbart)** (da Facebook) rilasciato con il paper [Multilingual Denoising Pre-training for Neural Machine Translation](https://arxiv.org/abs/2001.08210) da Yinhan Liu, Jiatao Gu, Naman Goyal, Xian Li, Sergey Edunov, Marjan Ghazvininejad, Mike Lewis, Luke Zettlemoyer.
1. **[MBart-50](model_doc/mbart)** (da Facebook) rilasciato con il paper [Multilingual Translation with Extensible Multilingual Pretraining and Finetuning](https://arxiv.org/abs/2008.00401) da Yuqing Tang, Chau Tran, Xian Li, Peng-Jen Chen, Naman Goyal, Vishrav Chaudhary, Jiatao Gu, Angela Fan.
1. **[Megatron-BERT](model_doc/megatron-bert)** (da NVIDIA) rilasciato con il paper [Megatron-LM: Training Multi-Billion Parameter Language Models Using Model Parallelism](https://arxiv.org/abs/1909.08053) da Mohammad Shoeybi, Mostofa Patwary, Raul Puri, Patrick LeGresley, Jared Casper e Bryan Catanzaro.
1. **[Megatron-GPT2](model_doc/megatron_gpt2)** (da NVIDIA) rilasciato con il paper [Megatron-LM: Training Multi-Billion Parameter Language Models Using Model Parallelism](https://arxiv.org/abs/1909.08053) da Mohammad Shoeybi, Mostofa Patwary, Raul Puri, Patrick LeGresley, Jared Casper e Bryan Catanzaro.
1. **[MPNet](model_doc/mpnet)** (da Microsoft Research) rilasciato con il paper [MPNet: Masked and Permuted Pre-training for Language Understanding](https://arxiv.org/abs/2004.09297) da Kaitao Song, Xu Tan, Tao Qin, Jianfeng Lu, Tie-Yan Liu.
1. **[MT5](model_doc/mt5)** (da Google AI) rilasciato con il paper [mT5: A massively multilingual pre-trained text-to-text transformer](https://arxiv.org/abs/2010.11934) da Linting Xue, Noah Constant, Adam Roberts, Mihir Kale, Rami Al-Rfou, Aditya Siddhant, Aditya Barua, Colin Raffel.
1. **[Nystrรถmformer](model_doc/nystromformer)** (dalla Universitร del Wisconsin - Madison) rilasciato con il paper [Nystrรถmformer: A Nystrรถm-Based Algorithm for Approximating Self-Attention](https://arxiv.org/abs/2102.03902) da Yunyang Xiong, Zhanpeng Zeng, Rudrasis Chakraborty, Mingxing Tan, Glenn Fung, Yin Li, Vikas Singh.
1. **[OneFormer](model_doc/oneformer)** (da SHI Labs) rilasciato con il paper [OneFormer: One Transformer to Rule Universal Image Segmentation](https://arxiv.org/abs/2211.06220) da Jitesh Jain, Jiachen Li, MangTik Chiu, Ali Hassani, Nikita Orlov, Humphrey Shi.
1. **[OPT](master/model_doc/opt)** (da Meta AI) rilasciato con il paper [OPT: Open Pre-trained Transformer Language Models](https://arxiv.org/abs/2205.01068) da Susan Zhang, Stephen Roller, Naman Goyal, Mikel Artetxe, Moya Chen, Shuohui Chen et al.
1. **[Pegasus](model_doc/pegasus)** (da Google) rilasciato con il paper [PEGASUS: Pre-training with Extracted Gap-sentences for Abstractive Summarization](https://arxiv.org/abs/1912.08777) da Jingqing Zhang, Yao Zhao, Mohammad Saleh e Peter J. Liu.
1. **[Perceiver IO](model_doc/perceiver)** (da Deepmind) rilasciato con il paper [Perceiver IO: A General Architecture for Structured Inputs & Outputs](https://arxiv.org/abs/2107.14795) da Andrew Jaegle, Sebastian Borgeaud, Jean-Baptiste Alayrac, Carl Doersch, Catalin Ionescu, David Ding, Skanda Koppula, Daniel Zoran, Andrew Brock, Evan Shelhamer, Olivier Hรฉnaff, Matthew M. Botvinick, Andrew Zisserman, Oriol Vinyals, Joรฃo Carreira.
1. **[PhoBERT](model_doc/phobert)** (da VinAI Research) rilasciato con il paper [PhoBERT: Pre-trained language models for Vietnamese](https://www.aclweb.org/anthology/2020.findings-emnlp.92/) da Dat Quoc Nguyen e Anh Tuan Nguyen.
1. **[PLBart](model_doc/plbart)** (da UCLA NLP) rilasciato con il paper [Unified Pre-training for Program Understanding and Generation](https://arxiv.org/abs/2103.06333) da Wasi Uddin Ahmad, Saikat Chakraborty, Baishakhi Ray, Kai-Wei Chang.
1. **[PoolFormer](model_doc/poolformer)** (da Sea AI Labs) rilasciato con il paper [MetaFormer is Actually What You Need for Vision](https://arxiv.org/abs/2111.11418) da Yu, Weihao e Luo, Mi e Zhou, Pan e Si, Chenyang e Zhou, Yichen e Wang, Xinchao e Feng, Jiashi e Yan, Shuicheng.
1. **[ProphetNet](model_doc/prophetnet)** (da Microsoft Research) rilasciato con il paper [ProphetNet: Predicting Future N-gram for Sequence-to-Sequence Pre-training](https://arxiv.org/abs/2001.04063) da Yu Yan, Weizhen Qi, Yeyun Gong, Dayiheng Liu, Nan Duan, Jiusheng Chen, Ruofei Zhang e Ming Zhou.
1. **[QDQBert](model_doc/qdqbert)** (da NVIDIA) rilasciato con il paper [Integer Quantization for Deep Learning Inference: Principles and Empirical Evaluation](https://arxiv.org/abs/2004.09602) da Hao Wu, Patrick Judd, Xiaojie Zhang, Mikhail Isaev e Paulius Micikevicius.
1. **[REALM](model_doc/realm.html)** (da Google Research) rilasciato con il paper [REALM: Retrieval-Augmented Language Model Pre-Training](https://arxiv.org/abs/2002.08909) da Kelvin Guu, Kenton Lee, Zora Tung, Panupong Pasupat e Ming-Wei Chang.
1. **[Reformer](model_doc/reformer)** (da Google Research) rilasciato con il paper [Reformer: The Efficient Transformer](https://arxiv.org/abs/2001.04451) da Nikita Kitaev, ลukasz Kaiser, Anselm Levskaya.
1. **[RemBERT](model_doc/rembert)** (da Google Research) rilasciato con il paper [Rethinking embedding coupling in pre-trained language models](https://arxiv.org/abs/2010.12821) da Hyung Won Chung, Thibault Fรฉvry, Henry Tsai, M. Johnson, Sebastian Ruder.
1. **[RegNet](model_doc/regnet)** (da META Platforms) rilasciato con il paper [Designing Network Design Space](https://arxiv.org/abs/2003.13678) da Ilija Radosavovic, Raj Prateek Kosaraju, Ross Girshick, Kaiming He, Piotr Dollรกr.
1. **[ResNet](model_doc/resnet)** (da Microsoft Research) rilasciato con il paper [Deep Residual Learning for Image Recognition](https://arxiv.org/abs/1512.03385) da Kaiming He, Xiangyu Zhang, Shaoqing Ren, Jian Sun.
1. **[RoBERTa](model_doc/roberta)** (da Facebook), rilasciato assieme al paper [RoBERTa: A Robustly Optimized BERT Pretraining Approach](https://arxiv.org/abs/1907.11692) da Yinhan Liu, Myle Ott, Naman Goyal, Jingfei Du, Mandar Joshi, Danqi Chen, Omer Levy, Mike Lewis, Luke Zettlemoyer, Veselin Stoyanov.
1. **[RoFormer](model_doc/roformer)** (da ZhuiyiTechnology), rilasciato assieme al paper [RoFormer: Enhanced Transformer with Rotary Position Embedding](https://arxiv.org/abs/2104.09864) da Jianlin Su e Yu Lu e Shengfeng Pan e Bo Wen e Yunfeng Liu.
1. **[SegFormer](model_doc/segformer)** (da NVIDIA) rilasciato con il paper [SegFormer: Simple and Efficient Design for Semantic Segmentation with Transformers](https://arxiv.org/abs/2105.15203) da Enze Xie, Wenhai Wang, Zhiding Yu, Anima Anandkumar, Jose M. Alvarez, Ping Luo.
1. **[SEW](model_doc/sew)** (da ASAPP) rilasciato con il paper [Performance-Efficiency Trade-offs in Unsupervised Pre-training for Speech Recognition](https://arxiv.org/abs/2109.06870) da Felix Wu, Kwangyoun Kim, Jing Pan, Kyu Han, Kilian Q. Weinberger, Yoav Artzi.
1. **[SEW-D](model_doc/sew_d)** (da ASAPP) rilasciato con il paper [Performance-Efficiency Trade-offs in Unsupervised Pre-training for Speech Recognition](https://arxiv.org/abs/2109.06870) da Felix Wu, Kwangyoun Kim, Jing Pan, Kyu Han, Kilian Q. Weinberger, Yoav Artzi.
1. **[SpeechToTextTransformer](model_doc/speech_to_text)** (da Facebook), rilasciato assieme al paper [fairseq S2T: Fast Speech-to-Text Modeling with fairseq](https://arxiv.org/abs/2010.05171) da Changhan Wang, Yun Tang, Xutai Ma, Anne Wu, Dmytro Okhonko, Juan Pino.
1. **[SpeechToTextTransformer2](model_doc/speech_to_text_2)** (da Facebook), rilasciato assieme al paper [Large-Scale Self- and Semi-Supervised Learning for Speech Translation](https://arxiv.org/abs/2104.06678) da Changhan Wang, Anne Wu, Juan Pino, Alexei Baevski, Michael Auli, Alexis Conneau.
1. **[Splinter](model_doc/splinter)** (dalla Universitร di Tel Aviv), rilasciato assieme al paper [Few-Shot Question Answering by Pretraining Span Selection](https://arxiv.org/abs/2101.00438) da Ori Ram, Yuval Kirstain, Jonathan Berant, Amir Globerson, Omer Levy.
1. **[SqueezeBert](model_doc/squeezebert)** (da Berkeley) rilasciato con il paper [SqueezeBERT: What can computer vision teach NLP about efficient neural networks?](https://arxiv.org/abs/2006.11316) da Forrest N. Iandola, Albert E. Shaw, Ravi Krishna, e Kurt W. Keutzer.
1. **[Swin Transformer](model_doc/swin)** (da Microsoft) rilasciato con il paper [Swin Transformer: Hierarchical Vision Transformer using Shifted Windows](https://arxiv.org/abs/2103.14030) da Ze Liu, Yutong Lin, Yue Cao, Han Hu, Yixuan Wei, Zheng Zhang, Stephen Lin, Baining Guo.
1. **[T5](model_doc/t5)** (da Google AI) rilasciato con il paper [Exploring the Limits of Transfer Learning with a Unified Text-to-Text Transformer](https://arxiv.org/abs/1910.10683) da Colin Raffel e Noam Shazeer e Adam Roberts e Katherine Lee e Sharan Narang e Michael Matena e Yanqi Zhou e Wei Li e Peter J. Liu.
1. **[T5v1.1](model_doc/t5v1.1)** (da Google AI) rilasciato nel repository [google-research/text-to-text-transfer-transformer](https://github.com/google-research/text-to-text-transfer-transformer/blob/main/released_checkpoints.md#t511) da Colin Raffel e Noam Shazeer e Adam Roberts e Katherine Lee e Sharan Narang e Michael Matena e Yanqi Zhou e Wei Li e Peter J. Liu.
1. **[TAPAS](model_doc/tapas)** (da Google AI) rilasciato con il paper [TAPAS: Weakly Supervised Table Parsing via Pre-training](https://arxiv.org/abs/2004.02349) da Jonathan Herzig, Paweล Krzysztof Nowak, Thomas Mรผller, Francesco Piccinno e Julian Martin Eisenschlos.
1. **[TAPEX](model_doc/tapex)** (da Microsoft Research) rilasciato con il paper [TAPEX: Table Pre-training via Learning a Neural SQL Executor](https://arxiv.org/abs/2107.07653) da Qian Liu, Bei Chen, Jiaqi Guo, Morteza Ziyadi, Zeqi Lin, Weizhu Chen, Jian-Guang Lou.
1. **[Trajectory Transformer](model_doc/trajectory_transformers)** (dall'Universitร della California a Berkeley) rilasciato con il paper [Offline Reinforcement Learning as One Big Sequence Modeling Problem](https://arxiv.org/abs/2106.02039) da Michael Janner, Qiyang Li, Sergey Levine
1. **[Transformer-XL](model_doc/transfo-xl)** (da Google/CMU) rilasciato con il paper [Transformer-XL: Attentive Language Models Beyond a Fixed-Length Context](https://arxiv.org/abs/1901.02860) da Zihang Dai*, Zhilin Yang*, Yiming Yang, Jaime Carbonell, Quoc V. Le, Ruslan Salakhutdinov.
1. **[TrOCR](model_doc/trocr)** (da Microsoft), rilasciato assieme al paper [TrOCR: Transformer-based Optical Character Recognition with Pre-trained Models](https://arxiv.org/abs/2109.10282) da Minghao Li, Tengchao Lv, Lei Cui, Yijuan Lu, Dinei Florencio, Cha Zhang, Zhoujun Li, Furu Wei.
1. **[UniSpeech](model_doc/unispeech)** (da Microsoft Research) rilasciato con il paper [UniSpeech: Unified Speech Representation Learning with Labeled and Unlabeled Data](https://arxiv.org/abs/2101.07597) da Chengyi Wang, Yu Wu, Yao Qian, Kenichi Kumatani, Shujie Liu, Furu Wei, Michael Zeng, Xuedong Huang.
1. **[UniSpeechSat](model_doc/unispeech-sat)** (da Microsoft Research) rilasciato con il paper [UNISPEECH-SAT: UNIVERSAL SPEECH REPRESENTATION LEARNING WITH SPEAKER AWARE PRE-TRAINING](https://arxiv.org/abs/2110.05752) da Sanyuan Chen, Yu Wu, Chengyi Wang, Zhengyang Chen, Zhuo Chen, Shujie Liu, Jian Wu, Yao Qian, Furu Wei, Jinyu Li, Xiangzhan Yu.
1. **[VAN](model_doc/van)** (dalle Universitร di Tsinghua e Nankai) rilasciato con il paper [Visual Attention Network](https://arxiv.org/abs/2202.09741) da Meng-Hao Guo, Cheng-Ze Lu, Zheng-Ning Liu, Ming-Ming Cheng, Shi-Min Hu.
1. **[ViLT](model_doc/vilt)** (da NAVER AI Lab/Kakao Enterprise/Kakao Brain) rilasciato con il paper [ViLT: Vision-and-Language Transformer Without Convolution or Region Supervision](https://arxiv.org/abs/2102.03334) da Wonjae Kim, Bokyung Son, Ildoo Kim.
1. **[Vision Transformer (ViT)](model_doc/vit)** (da Google AI) rilasciato con il paper [An Image is Worth 16x16 Words: Transformers for Image Recognition at Scale](https://arxiv.org/abs/2010.11929) da Alexey Dosovitskiy, Lucas Beyer, Alexander Kolesnikov, Dirk Weissenborn, Xiaohua Zhai, Thomas Unterthiner, Mostafa Dehghani, Matthias Minderer, Georg Heigold, Sylvain Gelly, Jakob Uszkoreit, Neil Houlsby.
1. **[ViTMAE](model_doc/vit_mae)** (da Meta AI) rilasciato con il paper [Masked Autoencoders Are Scalable Vision Learners](https://arxiv.org/abs/2111.06377) da Kaiming He, Xinlei Chen, Saining Xie, Yanghao Li, Piotr Dollรกr, Ross Girshick.
1. **[VisualBERT](model_doc/visual_bert)** (da UCLA NLP) rilasciato con il paper [VisualBERT: A Simple and Performant Baseline for Vision and Language](https://arxiv.org/pdf/1908.03557) da Liunian Harold Li, Mark Yatskar, Da Yin, Cho-Jui Hsieh, Kai-Wei Chang.
1. **[WavLM](model_doc/wavlm)** (da Microsoft Research) rilasciato con il paper [WavLM: Large-Scale Self-Supervised Pre-Training for Full Stack Speech Processing](https://arxiv.org/abs/2110.13900) da Sanyuan Chen, Chengyi Wang, Zhengyang Chen, Yu Wu, Shujie Liu, Zhuo Chen, Jinyu Li, Naoyuki Kanda, Takuya Yoshioka, Xiong Xiao, Jian Wu, Long Zhou, Shuo Ren, Yanmin Qian, Yao Qian, Jian Wu, Michael Zeng, Furu Wei.
1. **[Wav2Vec2](model_doc/wav2vec2)** (da Facebook AI) rilasciato con il paper [wav2vec 2.0: A Framework for Self-Supervised Learning of Speech Representations](https://arxiv.org/abs/2006.11477) da Alexei Baevski, Henry Zhou, Abdelrahman Mohamed, Michael Auli.
1. **[Wav2Vec2Phoneme](model_doc/wav2vec2_phoneme)** (da Facebook AI) rilasciato con il paper [Simple and Effective Zero-shot Cross-lingual Phoneme Recognition](https://arxiv.org/abs/2109.11680) da Qiantong Xu, Alexei Baevski, Michael Auli.
1. **[XGLM](model_doc/xglm)** (da Facebook AI) rilasciato con il paper [Few-shot Learning with Multilingual Language Models](https://arxiv.org/abs/2112.10668) da Xi Victoria Lin, Todor Mihaylov, Mikel Artetxe, Tianlu Wang, Shuohui Chen, Daniel Simig, Myle Ott, Naman Goyal, Shruti Bhosale, Jingfei Du, Ramakanth Pasunuru, Sam Shleifer, Punit Singh Koura, Vishrav Chaudhary, Brian O'Horo, Jeff Wang, Luke Zettlemoyer, Zornitsa Kozareva, Mona Diab, Veselin Stoyanov, Xian Li.
1. **[XLM](model_doc/xlm)** (v Facebook) rilasciato assieme al paper [Cross-lingual Language Model Pretraining](https://arxiv.org/abs/1901.07291) da Guillaume Lample e Alexis Conneau.
1. **[XLM-ProphetNet](model_doc/xlm-prophetnet)** (da Microsoft Research) rilasciato con il paper [ProphetNet: Predicting Future N-gram for Sequence-to-Sequence Pre-training](https://arxiv.org/abs/2001.04063) da Yu Yan, Weizhen Qi, Yeyun Gong, Dayiheng Liu, Nan Duan, Jiusheng Chen, Ruofei Zhang e Ming Zhou.
1. **[XLM-RoBERTa](model_doc/xlm-roberta)** (da Facebook AI), rilasciato assieme al paper [Unsupervised Cross-lingual Representation Learning at Scale](https://arxiv.org/abs/1911.02116) da Alexis Conneau*, Kartikay Khandelwal*, Naman Goyal, Vishrav Chaudhary, Guillaume Wenzek, Francisco Guzmรกn, Edouard Grave, Myle Ott, Luke Zettlemoyer e Veselin Stoyanov.
1. **[XLM-RoBERTa-XL](model_doc/xlm-roberta-xl)** (da Facebook AI), rilasciato assieme al paper [Larger-Scale Transformers for Multilingual Masked Language Modeling](https://arxiv.org/abs/2105.00572) da Naman Goyal, Jingfei Du, Myle Ott, Giri Anantharaman, Alexis Conneau.
1. **[XLNet](model_doc/xlnet)** (da Google/CMU) rilasciato con il paper [โXLNet: Generalized Autoregressive Pretraining for Language Understanding](https://arxiv.org/abs/1906.08237) da Zhilin Yang*, Zihang Dai*, Yiming Yang, Jaime Carbonell, Ruslan Salakhutdinov, Quoc V. Le.
1. **[XLSR-Wav2Vec2](model_doc/xlsr_wav2vec2)** (da Facebook AI) rilasciato con il paper [Unsupervised Cross-Lingual Representation Learning For Speech Recognition](https://arxiv.org/abs/2006.13979) da Alexis Conneau, Alexei Baevski, Ronan Collobert, Abdelrahman Mohamed, Michael Auli.
1. **[XLS-R](model_doc/xls_r)** (da Facebook AI) rilasciato con il paper [XLS-R: Self-supervised Cross-lingual Speech Representation Learning at Scale](https://arxiv.org/abs/2111.09296) da Arun Babu, Changhan Wang, Andros Tjandra, Kushal Lakhotia, Qiantong Xu, Naman Goyal, Kritika Singh, Patrick von Platen, Yatharth Saraf, Juan Pino, Alexei Baevski, Alexis Conneau, Michael Auli.
1. **[YOLOS](model_doc/yolos)** (dalla Universitร della scienza e tecnologia di Huazhong) rilasciato con il paper [You Only Look at One Sequence: Rethinking Transformer in Vision through Object Detection](https://arxiv.org/abs/2106.00666) da Yuxin Fang, Bencheng Liao, Xinggang Wang, Jiemin Fang, Jiyang Qi, Rui Wu, Jianwei Niu, Wenyu Liu.
1. **[YOSO](model_doc/yoso)** (dall'Universitร del Wisconsin - Madison) rilasciato con il paper [You Only Sample (Almost) Once: Linear Cost Self-Attention Via Bernoulli Sampling](https://arxiv.org/abs/2111.09714) da Zhanpeng Zeng, Yunyang Xiong, Sathya N. Ravi, Shailesh Acharya, Glenn Fung, Vikas Singh.
### Framework supportati
La tabella seguente rappresenta il supporto attuale nella libreria per ognuno di questi modelli, si puรฒ identificare se questi hanno un Python
tokenizer (chiamato "slow"). Un tokenizer "fast" supportato dalla libreria ๐ค Tokenizers, e se hanno supporto in Jax (via Flax), PyTorch, e/o TensorFlow.
<!--This table is updated automatically from the auto modules with _make fix-copies_. Do not update manually!-->
| Model | Tokenizer slow | Tokenizer fast | PyTorch support | TensorFlow support | Flax Support |
|:---------------------------:|:--------------:|:--------------:|:---------------:|:------------------:|:------------:|
| ALBERT | โ
| โ
| โ
| โ
| โ
|
| BART | โ
| โ
| โ
| โ
| โ
|
| BEiT | โ | โ | โ
| โ | โ
|
| BERT | โ
| โ
| โ
| โ
| โ
|
| Bert Generation | โ
| โ | โ
| โ | โ |
| BigBird | โ
| โ
| โ
| โ | โ
|
| BigBirdPegasus | โ | โ | โ
| โ | โ |
| Blenderbot | โ
| โ
| โ
| โ
| โ
|
| BlenderbotSmall | โ
| โ
| โ
| โ
| โ
|
| CamemBERT | โ
| โ
| โ
| โ
| โ |
| Canine | โ
| โ | โ
| โ | โ |
| CLIP | โ
| โ
| โ
| โ
| โ
|
| ConvBERT | โ
| โ
| โ
| โ
| โ |
| ConvNext | โ | โ | โ
| โ
| โ |
| CTRL | โ
| โ | โ
| โ
| โ |
| CvT | โ | โ | โ
| โ | โ |
| Data2VecAudio | โ | โ | โ
| โ | โ |
| Data2VecText | โ | โ | โ
| โ | โ |
| Data2VecVision | โ | โ | โ
| โ
| โ |
| DeBERTa | โ
| โ
| โ
| โ
| โ |
| DeBERTa-v2 | โ
| โ
| โ
| โ
| โ |
| Decision Transformer | โ | โ | โ
| โ | โ |
| DeiT | โ | โ | โ
| โ | โ |
| DETR | โ | โ | โ
| โ | โ |
| DistilBERT | โ
| โ
| โ
| โ
| โ
|
| DPR | โ
| โ
| โ
| โ
| โ |
| DPT | โ | โ | โ
| โ | โ |
| ELECTRA | โ
| โ
| โ
| โ
| โ
|
| Encoder decoder | โ | โ | โ
| โ
| โ
|
| FairSeq Machine-Translation | โ
| โ | โ
| โ | โ |
| FlauBERT | โ
| โ | โ
| โ
| โ |
| Flava | โ | โ | โ
| โ | โ |
| FNet | โ
| โ
| โ
| โ | โ |
| Funnel Transformer | โ
| โ
| โ
| โ
| โ |
| GLPN | โ | โ | โ
| โ | โ |
| GPT Neo | โ | โ | โ
| โ | โ
|
| GPT NeoX | โ | โ
| โ
| โ | โ |
| GPT-J | โ | โ | โ
| โ
| โ
|
| Hubert | โ | โ | โ
| โ
| โ |
| I-BERT | โ | โ | โ
| โ | โ |
| ImageGPT | โ | โ | โ
| โ | โ |
| LayoutLM | โ
| โ
| โ
| โ
| โ |
| LayoutLMv2 | โ
| โ
| โ
| โ | โ |
| LayoutLMv3 | โ
| โ
| โ
| โ
| โ |
| LED | โ
| โ
| โ
| โ
| โ |
| Longformer | โ
| โ
| โ
| โ
| โ |
| LUKE | โ
| โ | โ
| โ | โ |
| LXMERT | โ
| โ
| โ
| โ
| โ |
| M2M100 | โ
| โ | โ
| โ | โ |
| Marian | โ
| โ | โ
| โ
| โ
|
| MaskFormer | โ | โ | โ
| โ | โ |
| mBART | โ
| โ
| โ
| โ
| โ
|
| MegatronBert | โ | โ | โ
| โ | โ |
| MobileBERT | โ
| โ
| โ
| โ
| โ |
| MPNet | โ
| โ
| โ
| โ
| โ |
| mT5 | โ
| โ
| โ
| โ
| โ
|
| Nystromformer | โ | โ | โ
| โ | โ |
| OpenAI GPT | โ
| โ
| โ
| โ
| โ |
| OpenAI GPT-2 | โ
| โ
| โ
| โ
| โ
|
| OPT | โ | โ | โ
| โ | โ |
| Pegasus | โ
| โ
| โ
| โ
| โ
|
| Perceiver | โ
| โ | โ
| โ | โ |
| PLBart | โ
| โ | โ
| โ | โ |
| PoolFormer | โ | โ | โ
| โ | โ |
| ProphetNet | โ
| โ | โ
| โ | โ |
| QDQBert | โ | โ | โ
| โ | โ |
| RAG | โ
| โ | โ
| โ
| โ |
| Realm | โ
| โ
| โ
| โ | โ |
| Reformer | โ
| โ
| โ
| โ | โ |
| RegNet | โ | โ | โ
| โ
| โ
|
| RemBERT | โ
| โ
| โ
| โ
| โ |
| ResNet | โ | โ | โ
| โ
| โ
|
| RetriBERT | โ
| โ
| โ
| โ | โ |
| RoBERTa | โ
| โ
| โ
| โ
| โ
|
| RoFormer | โ
| โ
| โ
| โ
| โ
|
| SegFormer | โ | โ | โ
| โ | โ |
| SEW | โ | โ | โ
| โ | โ |
| SEW-D | โ | โ | โ
| โ | โ |
| Speech Encoder decoder | โ | โ | โ
| โ | โ
|
| Speech2Text | โ
| โ | โ
| โ
| โ |
| Speech2Text2 | โ
| โ | โ | โ | โ |
| Splinter | โ
| โ
| โ
| โ | โ |
| SqueezeBERT | โ
| โ
| โ
| โ | โ |
| Swin | โ | โ | โ
| โ
| โ |
| T5 | โ
| โ
| โ
| โ
| โ
|
| TAPAS | โ
| โ | โ
| โ
| โ |
| Trajectory Transformer | โ | โ | โ
| โ | โ |
| Transformer-XL | โ
| โ | โ
| โ
| โ |
| TrOCR | โ | โ | โ
| โ | โ |
| UniSpeech | โ | โ | โ
| โ | โ |
| UniSpeechSat | โ | โ | โ
| โ | โ |
| VAN | โ | โ | โ
| โ | โ |
| ViLT | โ | โ | โ
| โ | โ |
| Vision Encoder decoder | โ | โ | โ
| โ
| โ
|
| VisionTextDualEncoder | โ | โ | โ
| โ | โ
|
| VisualBert | โ | โ | โ
| โ | โ |
| ViT | โ | โ | โ
| โ
| โ
|
| ViTMAE | โ | โ | โ
| โ
| โ |
| Wav2Vec2 | โ
| โ | โ
| โ
| โ
|
| Wav2Vec2-Conformer | โ | โ | โ
| โ | โ |
| WavLM | โ | โ | โ
| โ | โ |
| XGLM | โ
| โ
| โ
| โ | โ
|
| XLM | โ
| โ | โ
| โ
| โ |
| XLM-RoBERTa | โ
| โ
| โ
| โ
| โ
|
| XLM-RoBERTa-XL | โ | โ | โ
| โ | โ |
| XLMProphetNet | โ
| โ | โ
| โ | โ |
| XLNet | โ
| โ
| โ
| โ
| โ |
| YOLOS | โ | โ | โ
| โ | โ |
| YOSO | โ | โ | โ
| โ | โ |
<!-- End table-->
| 0 |
hf_public_repos/transformers/docs/source | hf_public_repos/transformers/docs/source/it/migration.md | <!---
Copyright 2020 The HuggingFace Team. Tutti i diritti riservati.
Concesso in licenza in base alla Licenza Apache, Versione 2.0 (la "Licenza");
non รจ possibile utilizzare questo file se non in conformitร con la Licenza.
ร possibile ottenere una copia della Licenza all'indirizzo
http://www.apache.org/licenses/LICENSE-2.0
A meno che non sia richiesto dalla legge applicabile o concordato per iscritto, il software
distribuito con la Licenza รจ distribuito su BASE "COSร COM'ร",
SENZA GARANZIE O CONDIZIONI DI ALCUN TIPO, espresse o implicite.
Per la lingua specifica vedi la Licenza che regola le autorizzazioni e
le limitazioni ai sensi della STESSA.
โ ๏ธ Note that this file is in Markdown but contain specific syntax for our doc-builder (similar to MDX) that may not be
rendered properly in your Markdown viewer.
-->
# Migrazione da pacchetti precedenti
## Migrazione da transformers `v3.x` a `v4.x`
Un paio di modifiche sono state introdotte nel passaggio dalla versione 3 alla versione 4. Di seguito รจ riportato un riepilogo delle
modifiche previste:
#### 1. AutoTokenizer e pipeline ora utilizzano tokenizer veloci (rust) per impostazione predefinita.
I tokenizer python e rust hanno all'incirca le stesse API, ma i tokenizer rust hanno un set di funzionalitร piรน completo.
Ciรฒ introduce due modifiche sostanziali:
- La gestione dei token in overflow tra i tokenizer Python e Rust รจ diversa.
- I tokenizers di rust non accettano numeri interi nei metodi di codifica.
##### Come ottenere lo stesso comportamento di v3.x in v4.x
- Le pipeline ora contengono funzionalitร aggiuntive pronte all'uso. Vedi la [pipeline di classificazione dei token con il flag `grouped_entities`](main_classes/pipelines#transformers.TokenClassificationPipeline).
- Gli auto-tokenizer ora restituiscono tokenizer rust. Per ottenere invece i tokenizer python, l'utente deve usare il flag `use_fast` impostandolo `False`:
Nella versione `v3.x`:
```py
from transformers import AutoTokenizer
tokenizer = AutoTokenizer.from_pretrained("bert-base-cased")
```
per ottenere lo stesso nella versione `v4.x`:
```py
from transformers import AutoTokenizer
tokenizer = AutoTokenizer.from_pretrained("bert-base-cased", use_fast=False)
```
#### 2. SentencePiece รจ stato rimosso dalle dipendenze richieste
Il requisito sulla dipendenza SentencePiece รจ stato rimosso da `setup.py`. ร stato fatto per avere un canale su anaconda cloud senza basarsi su `conda-forge`. Ciรฒ significa che i tokenizer che dipendono dalla libreria SentencePiece non saranno disponibili con un'installazione standard di `transformers`.
Ciรฒ include le versioni **lente** di:
- `XLNetTokenizer`
- `AlbertTokenizer`
- `CamembertTokenizer`
- `MBartTokenizer`
- `PegasusTokenizer`
- `T5Tokenizer`
- `ReformerTokenizer`
- `XLMRobertaTokenizer`
##### Come ottenere lo stesso comportamento della v3.x nella v4.x
Per ottenere lo stesso comportamento della versione `v3.x`, devi installare anche `sentencepiece`:
Nella versione `v3.x`:
```bash
pip install transformers
```
per ottenere lo stesso nella versione `v4.x`:
```bash
pip install transformers[sentencepiece]
```
o
```bash
pip install transformers stentencepiece
```
#### 3. L'architettura delle repo รจ stato aggiornata in modo che ogni modello abbia la propria cartella
Con lโaggiunta di nuovi modelli, il numero di file nella cartella `src/transformers` continua a crescere e diventa piรน difficile navigare e capire. Abbiamo fatto la scelta di inserire ogni modello e i file che lo accompagnano nelle proprie sottocartelle.
Si tratta di una modifica sostanziale in quanto l'importazione di layer intermedi utilizzando direttamente il modulo di un modello deve essere eseguita tramite un percorso diverso.
##### Come ottenere lo stesso comportamento della v3.x nella v4.x
Per ottenere lo stesso comportamento della versione `v3.x`, devi aggiornare il percorso utilizzato per accedere ai layer.
Nella versione `v3.x`:
```bash
from transformers.modeling_bert import BertLayer
```
per ottenere lo stesso nella versione `v4.x`:
```bash
from transformers.models.bert.modeling_bert import BertLayer
```
#### 4. Impostare l'argomento `return_dict` su `True` per impostazione predefinita
L'[argomento `return_dict`](main_classes/output) abilita la restituzione di oggetti python dict-like contenenti gli output del modello, invece delle tuple standard. Questo oggetto รจ self-documented poichรฉ le chiavi possono essere utilizzate per recuperare valori, comportandosi anche come una tupla e gli utenti possono recuperare oggetti per indexing o slicing.
Questa รจ una modifica sostanziale poichรฉ la tupla non puรฒ essere decompressa: `value0, value1 = outputs` non funzionerร .
##### Come ottenere lo stesso comportamento della v3.x nella v4.x
Per ottenere lo stesso comportamento della versione `v3.x`, specifica l'argomento `return_dict` come `False`, sia nella configurazione del modello che nel passaggio successivo.
Nella versione `v3.x`:
```bash
model = BertModel.from_pretrained("bert-base-cased")
outputs = model(**inputs)
```
per ottenere lo stesso nella versione `v4.x`:
```bash
model = BertModel.from_pretrained("bert-base-cased")
outputs = model(**inputs, return_dict=False)
```
o
```bash
model = BertModel.from_pretrained("bert-base-cased", return_dict=False)
outputs = model(**inputs)
```
#### 5. Rimozione di alcuni attributi deprecati
Gli attributi sono stati rimossi se deprecati da almeno un mese. L'elenco completo degli attributi obsoleti รจ disponibile in [#8604](https://github.com/huggingface/transformers/pull/8604).
Ecco un elenco di questi attributi/metodi/argomenti e quali dovrebbero essere le loro sostituzioni:
In diversi modelli, le etichette diventano coerenti con gli altri modelli:
- `masked_lm_labels` diventa `labels` in `AlbertForMaskedLM` e `AlbertForPreTraining`.
- `masked_lm_labels` diventa `labels` in `BertForMaskedLM` e `BertForPreTraining`.
- `masked_lm_labels` diventa `labels` in `DistilBertForMaskedLM`.
- `masked_lm_labels` diventa `labels` in `ElectraForMaskedLM`.
- `masked_lm_labels` diventa `labels` in `LongformerForMaskedLM`.
- `masked_lm_labels` diventa `labels` in `MobileBertForMaskedLM`.
- `masked_lm_labels` diventa `labels` in `RobertaForMaskedLM`.
- `lm_labels` diventa `labels` in `BartForConditionalGeneration`.
- `lm_labels` diventa `labels` in `GPT2DoubleHeadsModel`.
- `lm_labels` diventa `labels` in `OpenAIGPTDoubleHeadsModel`.
- `lm_labels` diventa `labels` in `T5ForConditionalGeneration`.
In diversi modelli, il meccanismo di memorizzazione nella cache diventa coerente con gli altri:
- `decoder_cached_states` diventa `past_key_values` in tutti i modelli BART-like, FSMT e T5.
- `decoder_past_key_values` diventa `past_key_values` in tutti i modelli BART-like, FSMT e T5.
- `past` diventa `past_key_values` in tutti i modelli CTRL.
- `past` diventa `past_key_values` in tutti i modelli GPT-2.
Per quanto riguarda le classi tokenizer:
- L'attributo tokenizer `max_len` diventa `model_max_length`.
- L'attributo tokenizer `return_lengths` diventa `return_length`.
- L'argomento di codifica del tokenizer `is_pretokenized` diventa `is_split_into_words`.
Per quanto riguarda la classe `Trainer`:
- L'argomento `tb_writer` di `Trainer` รจ stato rimosso in favore della funzione richiamabile `TensorBoardCallback(tb_writer=...)`.
- L'argomento `prediction_loss_only` di `Trainer` รจ stato rimosso in favore dell'argomento di classe `args.prediction_loss_only`.
- L'attributo `data_collator` di `Trainer` sarร richiamabile.
- Il metodo `_log` di `Trainer` รจ deprecato a favore di `log`.
- Il metodo `_training_step` di `Trainer` รจ deprecato a favore di `training_step`.
- Il metodo `_prediction_loop` di `Trainer` รจ deprecato a favore di `prediction_loop`.
- Il metodo `is_local_master` di `Trainer` รจ deprecato a favore di `is_local_process_zero`.
- Il metodo `is_world_master` di `Trainer` รจ deprecato a favore di `is_world_process_zero`.
Per quanto riguarda la classe `TFTrainer`:
- L'argomento `prediction_loss_only` di `TFTrainer` รจ stato rimosso a favore dell'argomento di classe `args.prediction_loss_only`.
- Il metodo `_log` di `Trainer` รจ deprecato a favore di `log`.
- Il metodo `_prediction_loop` di `TFTrainer` รจ deprecato a favore di `prediction_loop`.
- Il metodo `_setup_wandb` di `TFTrainer` รจ deprecato a favore di `setup_wandb`.
- Il metodo `_run_model` di `TFTrainer` รจ deprecato a favore di `run_model`.
Per quanto riguarda la classe `TrainingArguments`:
- L'argomento `evaluate_during_training` di `TrainingArguments` รจ deprecato a favore di `evaluation_strategy`.
Per quanto riguarda il modello Transfo-XL:
- L'attributo di configurazione `tie_weight` di Transfo-XL diventa `tie_words_embeddings`.
- Il metodo di modellazione `reset_length` di Transfo-XL diventa `reset_memory_length`.
Per quanto riguarda le pipeline:
- L'argomento `topk` di `FillMaskPipeline` diventa `top_k`.
## Passaggio da pytorch-transformers a ๐ค Transformers
Ecco un breve riepilogo di ciรฒ a cui prestare attenzione durante il passaggio da `pytorch-transformers` a ๐ค Transformers.
### Lโordine posizionale di alcune parole chiave di input dei modelli (`attention_mask`, `token_type_ids`...) รจ cambiato
Per usare Torchscript (vedi #1010, #1204 e #1195) l'ordine specifico delle **parole chiave di input** di alcuni modelli (`attention_mask`, `token_type_ids`...) รจ stato modificato.
Se inizializzavi i modelli usando parole chiave per gli argomenti, ad esempio `model(inputs_ids, attention_mask=attention_mask, token_type_ids=token_type_ids)`, questo non dovrebbe causare alcun cambiamento.
Se inizializzavi i modelli con input posizionali per gli argomenti, ad esempio `model(inputs_ids, attention_mask, token_type_ids)`, potrebbe essere necessario ricontrollare l'ordine esatto degli argomenti di input.
## Migrazione da pytorch-pretrained-bert
Ecco un breve riepilogo di ciรฒ a cui prestare attenzione durante la migrazione da `pytorch-pretrained-bert` a ๐ค Transformers
### I modelli restituiscono sempre `tuple`
La principale modifica di rilievo durante la migrazione da `pytorch-pretrained-bert` a ๐ค Transformers รจ che il metodo dei modelli di previsione dร sempre una `tupla` con vari elementi a seconda del modello e dei parametri di configurazione.
Il contenuto esatto delle tuple per ciascun modello รจ mostrato in dettaglio nelle docstring dei modelli e nella [documentazione](https://huggingface.co/transformers/).
In quasi tutti i casi, andrร bene prendendo il primo elemento dell'output come quello che avresti precedentemente utilizzato in `pytorch-pretrained-bert`.
Ecco un esempio di conversione da `pytorch-pretrained-bert`
a ๐ค Transformers per un modello di classificazione `BertForSequenceClassification`:
```python
# Carichiamo il nostro modello
model = BertForSequenceClassification.from_pretrained("bert-base-uncased")
# Se usavi questa riga in pytorch-pretrained-bert :
loss = model(input_ids, labels=labels)
# Ora usa questa riga in ๐ค Transformers per estrarre la perdita dalla tupla di output:
outputs = model(input_ids, labels=labels)
loss = outputs[0]
# In ๐ค Transformers puoi anche avere accesso ai logit:
loss, logits = outputs[:2]
# Ed anche agli attention weight se configuri il modello per restituirli (e anche altri output, vedi le docstring e la documentazione)
model = BertForSequenceClassification.from_pretrained(" bert-base-uncased", output_attentions=True)
outputs = model(input_ids, labels=labels)
loss, logits, attentions = outputs
```
### Serializzazione
Modifica sostanziale nel metodo `from_pretrained()`:
1. I modelli sono ora impostati in modalitร di valutazione in maniera predefinita quando usi il metodo `from_pretrained()`. Per addestrarli non dimenticare di riportarli in modalitร di addestramento (`model.train()`) per attivare i moduli di dropout.
2. Gli argomenti aggiuntivi `*inputs` e `**kwargs` forniti al metodo `from_pretrained()` venivano passati direttamente al metodo `__init__()` della classe sottostante del modello. Ora sono usati per aggiornare prima l'attributo di configurazione del modello, che puรฒ non funzionare con le classi del modello derivate costruite basandosi sui precedenti esempi di `BertForSequenceClassification`. Piรน precisamente, gli argomenti posizionali `*inputs` forniti a `from_pretrained()` vengono inoltrati direttamente al metodo `__init__()` del modello mentre gli argomenti keyword `**kwargs` (i) che corrispondono agli attributi della classe di configurazione, vengono utilizzati per aggiornare tali attributi (ii) che non corrispondono ad alcun attributo della classe di configurazione, vengono inoltrati al metodo `__init__()`.
Inoltre, sebbene non si tratti di una modifica sostanziale, i metodi di serializzazione sono stati standardizzati e probabilmente dovresti passare al nuovo metodo `save_pretrained(save_directory)` se prima usavi qualsiasi altro metodo di serializzazione.
Ecco un esempio:
```python
### Carichiamo un modello e un tokenizer
model = BertForSequenceClassification.from_pretrained("bert-base-uncased")
tokenizer = BertTokenizer.from_pretrained("bert-base-uncased")
### Facciamo fare alcune cose al nostro modello e tokenizer
# Es: aggiungiamo nuovi token al vocabolario e agli embending del nostro modello
tokenizer.add_tokens(["[SPECIAL_TOKEN_1]", "[SPECIAL_TOKEN_2]"])
model.resize_token_embeddings(len(tokenizer))
# Alleniamo il nostro modello
train(model)
### Ora salviamo il nostro modello e il tokenizer in una cartella
model.save_pretrained("./my_saved_model_directory/")
tokenizer.save_pretrained("./my_saved_model_directory/")
### Ricarichiamo il modello e il tokenizer
model = BertForSequenceClassification.from_pretrained("./my_saved_model_directory/")
tokenizer = BertTokenizer.from_pretrained("./my_saved_model_directory/")
```
### Ottimizzatori: BertAdam e OpenAIAdam ora sono AdamW, lo scheduling รจ quello standard PyTorch
I due ottimizzatori precedenti inclusi, `BertAdam` e `OpenAIAdam`, sono stati sostituiti da un singolo `AdamW` che presenta alcune differenze:
- implementa solo la correzione del weights decay,
- lo scheduling ora รจ esterno (vedi sotto),
- anche il gradient clipping ora รจ esterno (vedi sotto).
Il nuovo ottimizzatore `AdamW` corrisponde alle API di `Adam` di PyTorch e ti consente di utilizzare metodi PyTorch o apex per lo scheduling e il clipping.
Lo scheduling รจ ora standard [PyTorch learning rate schedulers](https://pytorch.org/docs/stable/optim.html#how-to-adjust-learning-rate) e non fanno piรน parte dell'ottimizzatore.
Ecco un esempio di linear warmup e decay con `BertAdam` e con `AdamW`:
```python
# Parametri:
lr = 1e-3
max_grad_norm = 1.0
num_training_steps = 1000
num_warmup_steps = 100
warmup_proportion = float( num_warmup_steps) / float(num_training_steps) # 0.1
### In precedenza l'ottimizzatore BertAdam veniva istanziato in questo modo:
optimizer = BertAdam(
model.parameters(),
lr=lr,
schedule="warmup_linear",
warmup=warmup_proportion,
num_training_steps=num_training_steps,
)
### e usato in questo modo:
for batch in train_data:
loss = model(batch)
loss.backward()
optimizer.step()
### In ๐ค Transformers, ottimizzatore e schedule sono divisi e usati in questo modo:
optimizer = AdamW(
model.parameters(), lr=lr, correct_bias=False
) # Per riprodurre il comportamento specifico di BertAdam impostare correct_bias=False
scheduler = get_linear_schedule_with_warmup(
optimizer, num_warmup_steps=num_warmup_steps, num_training_steps=num_training_steps
) # PyTorch scheduler
### e va usato cosรฌ:
for batch in train_data:
loss = model(batch)
loss.backward()
torch.nn.utils.clip_grad_norm_(
model.parameters(), max_grad_norm
) # Gradient clipping non รจ piรน in AdamW (quindi puoi usare amp senza problemi)
optimizer.step()
scheduler.step()
```
| 0 |
hf_public_repos/transformers/docs/source | hf_public_repos/transformers/docs/source/it/installation.md | <!---
Copyright 2022 The HuggingFace Team. All rights reserved.
Licensed under the Apache License, Version 2.0 (the "License");
you may not use this file except in compliance with the License.
You may obtain a copy of the License at
http://www.apache.org/licenses/LICENSE-2.0
Unless required by applicable law or agreed to in writing, software
distributed under the License is distributed on an "AS IS" BASIS,
WITHOUT WARRANTIES OR CONDITIONS OF ANY KIND, either express or implied.
See the License for the specific language governing permissions and
limitations under the License.
โ ๏ธ Note that this file is in Markdown but contain specific syntax for our doc-builder (similar to MDX) that may not be
rendered properly in your Markdown viewer.
-->
# Installazione
Installa ๐ค Transformers per qualsiasi libreria di deep learning con cui stai lavorando, imposta la tua cache, e opzionalmente configura ๐ค Transformers per l'esecuzione offline.
๐ค Transformers รจ testato su Python 3.6+, PyTorch 1.1.0+, TensorFlow 2.0+, e Flax. Segui le istruzioni di installazione seguenti per la libreria di deep learning che stai utilizzando:
* [PyTorch](https://pytorch.org/get-started/locally/) istruzioni di installazione.
* [TensorFlow 2.0](https://www.tensorflow.org/install/pip) istruzioni di installazione.
* [Flax](https://flax.readthedocs.io/en/latest/) istruzioni di installazione.
## Installazione con pip
Puoi installare ๐ค Transformers in un [ambiente virtuale](https://docs.python.org/3/library/venv.html). Se non sei familiare con gli ambienti virtuali in Python, dai un'occhiata a questa [guida](https://packaging.python.org/guides/installing-using-pip-and-virtual-environments/). Un ambiente virtuale rende piรน semplice la gestione di progetti differenti, evitando problemi di compatibilitร tra dipendenze.
Inizia creando un ambiente virtuale nella directory del tuo progetto:
```bash
python -m venv .env
```
Attiva l'ambiente virtuale:
```bash
source .env/bin/activate
```
Ora puoi procedere con l'installazione di ๐ค Transformers eseguendo il comando seguente:
```bash
pip install transformers
```
Per il solo supporto della CPU, puoi installare facilmente ๐ค Transformers e una libreria di deep learning in solo una riga. Ad esempio, installiamo ๐ค Transformers e PyTorch con:
```bash
pip install transformers[torch]
```
๐ค Transformers e TensorFlow 2.0:
```bash
pip install transformers[tf-cpu]
```
๐ค Transformers e Flax:
```bash
pip install transformers[flax]
```
Infine, verifica se ๐ค Transformers รจ stato installato in modo appropriato eseguendo il seguente comando. Questo scaricherร un modello pre-allenato:
```bash
python -c "from transformers import pipeline; print(pipeline('sentiment-analysis')('we love you'))"
```
Dopodichรฉ stampa l'etichetta e il punteggio:
```bash
[{'label': 'POSITIVE', 'score': 0.9998704791069031}]
```
## Installazione dalla fonte
Installa ๐ค Transformers dalla fonte con il seguente comando:
```bash
pip install git+https://github.com/huggingface/transformers
```
Questo comando installa la versione `main` piรน attuale invece dell'ultima versione stabile. Questo รจ utile per stare al passo con gli ultimi sviluppi. Ad esempio, se un bug รจ stato sistemato da quando รจ uscita l'ultima versione ufficiale ma non รจ stata ancora rilasciata una nuova versione. Tuttavia, questo significa che questa versione `main` puรฒ non essere sempre stabile. Ci sforziamo per mantenere la versione `main` operativa, e la maggior parte dei problemi viene risolta in poche ore o in un giorno. Se riscontri un problema, per favore apri una [Issue](https://github.com/huggingface/transformers/issues) cosรฌ possiamo sistemarlo ancora piรน velocemente!
Controlla se ๐ค Transformers รจ stata installata in modo appropriato con il seguente comando:
```bash
python -c "from transformers import pipeline; print(pipeline('sentiment-analysis')('I love you'))"
```
## Installazione modificabile
Hai bisogno di un'installazione modificabile se vuoi:
* Usare la versione `main` del codice dalla fonte.
* Contribuire a ๐ค Transformers e hai bisogno di testare i cambiamenti nel codice.
Clona il repository e installa ๐ค Transformers con i seguenti comandi:
```bash
git clone https://github.com/huggingface/transformers.git
cd transformers
pip install -e .
```
Questi comandi collegheranno la cartella in cui รจ stato clonato il repository e i path delle librerie Python. Python guarderร ora all'interno della cartella clonata, oltre ai normali path delle librerie. Per esempio, se i tuoi pacchetti Python sono installati tipicamente in `~/anaconda3/envs/main/lib/python3.7/site-packages/`, Python cercherร anche nella cartella clonata: `~/transformers/`.
<Tip warning={true}>
Devi tenere la cartella `transformers` se vuoi continuare ad utilizzare la libreria.
</Tip>
Ora puoi facilmente aggiornare il tuo clone all'ultima versione di ๐ค Transformers con il seguente comando:
```bash
cd ~/transformers/
git pull
```
Il tuo ambiente Python troverร la versione `main` di ๐ค Transformers alla prossima esecuzione.
## Installazione con conda
Installazione dal canale conda `huggingface`:
```bash
conda install -c huggingface transformers
```
## Impostazione della cache
I modelli pre-allenati sono scaricati e memorizzati localmente nella cache in: `~/.cache/huggingface/transformers/`. Questa รจ la directory di default data dalla variabile d'ambiente della shell `TRANSFORMERS_CACHE`. Su Windows, la directory di default รจ data da `C:\Users\username\.cache\huggingface\transformers`. Puoi cambiare le variabili d'ambiente della shell indicate in seguito, in ordine di prioritร , per specificare una directory differente per la cache:
1. Variabile d'ambiente della shell (default): `TRANSFORMERS_CACHE`.
2. Variabile d'ambiente della shell: `HF_HOME` + `transformers/`.
3. Variabile d'ambiente della shell: `XDG_CACHE_HOME` + `/huggingface/transformers`.
<Tip>
๐ค Transformers utilizzerร le variabili d'ambiente della shell `PYTORCH_TRANSFORMERS_CACHE` o `PYTORCH_PRETRAINED_BERT_CACHE` se si proviene da un'iterazione precedente di questa libreria e sono state impostate queste variabili d'ambiente, a meno che non si specifichi la variabile d'ambiente della shell `TRANSFORMERS_CACHE`.
</Tip>
## Modalitร Offline
๐ค Transformers puรฒ essere eseguita in un ambiente firewalled o offline utilizzando solo file locali. Imposta la variabile d'ambiente `TRANSFORMERS_OFFLINE=1` per abilitare questo comportamento.
<Tip>
Aggiungi [๐ค Datasets](https://huggingface.co/docs/datasets/) al tuo flusso di lavoro offline di training impostando la variabile d'ambiente `HF_DATASETS_OFFLINE=1`.
</Tip>
Ad esempio, in genere si esegue un programma su una rete normale, protetta da firewall per le istanze esterne, con il seguente comando:
```bash
python examples/pytorch/translation/run_translation.py --model_name_or_path t5-small --dataset_name wmt16 --dataset_config ro-en ...
```
Esegui lo stesso programma in un'istanza offline con:
```bash
HF_DATASETS_OFFLINE=1 TRANSFORMERS_OFFLINE=1 \
python examples/pytorch/translation/run_translation.py --model_name_or_path t5-small --dataset_name wmt16 --dataset_config ro-en ...
```
Lo script viene ora eseguito senza bloccarsi o attendere il timeout, perchรฉ sa di dover cercare solo file locali.
### Ottenere modelli e tokenizer per l'uso offline
Un'altra opzione per utilizzare offline ๐ค Transformers รจ scaricare i file in anticipo, e poi puntare al loro path locale quando hai la necessitร di utilizzarli offline. Ci sono tre modi per fare questo:
* Scarica un file tramite l'interfaccia utente sul [Model Hub](https://huggingface.co/models) premendo sull'icona โ.

* Utilizza il flusso [`PreTrainedModel.from_pretrained`] e [`PreTrainedModel.save_pretrained`]:
1. Scarica i tuoi file in anticipo con [`PreTrainedModel.from_pretrained`]:
```py
>>> from transformers import AutoTokenizer, AutoModelForSeq2SeqLM
>>> tokenizer = AutoTokenizer.from_pretrained("bigscience/T0_3B")
>>> model = AutoModelForSeq2SeqLM.from_pretrained("bigscience/T0_3B")
```
2. Salva i tuoi file in una directory specificata con [`PreTrainedModel.save_pretrained`]:
```py
>>> tokenizer.save_pretrained("./il/tuo/path/bigscience_t0")
>>> model.save_pretrained("./il/tuo/path/bigscience_t0")
```
3. Ora quando sei offline, carica i tuoi file con [`PreTrainedModel.from_pretrained`] dalla directory specificata:
```py
>>> tokenizer = AutoTokenizer.from_pretrained("./il/tuo/path/bigscience_t0")
>>> model = AutoModel.from_pretrained("./il/tuo/path/bigscience_t0")
```
* Scarica in maniera programmatica i file con la libreria [huggingface_hub](https://github.com/huggingface/huggingface_hub/tree/main/src/huggingface_hub):
1. Installa la libreria `huggingface_hub` nel tuo ambiente virtuale:
```bash
python -m pip install huggingface_hub
```
2. Utilizza la funzione [`hf_hub_download`](https://huggingface.co/docs/hub/adding-a-library#download-files-from-the-hub) per scaricare un file in un path specifico. Per esempio, il seguente comando scarica il file `config.json` dal modello [T0](https://huggingface.co/bigscience/T0_3B) nel path che desideri:
```py
>>> from huggingface_hub import hf_hub_download
>>> hf_hub_download(repo_id="bigscience/T0_3B", filename="config.json", cache_dir="./il/tuo/path/bigscience_t0")
```
Una volta che il tuo file รจ scaricato e salvato in cache localmente, specifica il suo path locale per caricarlo e utilizzarlo:
```py
>>> from transformers import AutoConfig
>>> config = AutoConfig.from_pretrained("./il/tuo/path/bigscience_t0/config.json")
```
<Tip>
Fai riferimento alla sezione [How to download files from the Hub](https://huggingface.co/docs/hub/how-to-downstream) per avere maggiori dettagli su come scaricare modelli presenti sull Hub.
</Tip> | 0 |
hf_public_repos/transformers/docs/source | hf_public_repos/transformers/docs/source/it/_toctree.yml | - sections:
- local: index
title: ๐ค Transformers
- local: quicktour
title: Tour rapido
- local: installation
title: Installazione
title: Iniziare
- sections:
- local: pipeline_tutorial
title: Pipeline per l'inferenza
- local: autoclass_tutorial
title: Carica istanze pre-allenate con AutoClass
- local: preprocessing
title: Preprocess
- local: training
title: Fine-tuning di un modello pre-addestrato
- local: accelerate
title: Allenamento distribuito con ๐ค Accelerate
- local: model_sharing
title: Condividere un modello
title: Esercitazione
- sections:
- local: create_a_model
title: Crea un'architettura personalizzata
- local: custom_models
title: Condividere modelli personalizzati
- local: run_scripts
title: Addestramento con script
- local: multilingual
title: Modelli multilingua per l'inferenza
- local: converting_tensorflow_models
title: Convertire modelli tensorflow
- local: serialization
title: Esporta modelli Transformers
- local: perf_train_cpu
title: Addestramento efficiente su CPU
- local: perf_train_cpu_many
title: Addestramento efficiente su multiple CPU
- local: perf_train_tpu
title: Addestramento su TPU
- local: perf_train_special
title: Addestramento su Hardware Specializzato
- local: perf_infer_cpu
title: Inferenza Efficiente su CPU
- local: perf_infer_gpu_one
title: Inferenza su una GPU
- local: perf_infer_gpu_many
title: Inferenza Efficiente su GPU Multiple
- local: perf_infer_special
title: Inferenza su Hardware Specializzato
- local: big_models
title: Istanziare un big model
- local: migration
title: Passaggio da pacchetti precedenti
- local: debugging
title: Debugging
title: Guide pratiche
- sections:
- local: add_new_pipeline
title: Come aggiungere una pipeline a ๐ค Transformers?
- local: add_new_model
title: Come aggiungere un modello a ๐ค Transformers?
- local: perf_hardware
title: Hardware ottimizzato per l'addestramento
- local: community
title: Risorse della comunitร
- local: pr_checks
title: Controlli su una Pull Request
title: Guide How-to
| 0 |
hf_public_repos/transformers/docs/source | hf_public_repos/transformers/docs/source/it/multilingual.md | <!--Copyright 2022 The HuggingFace Team. All rights reserved.
Licensed under the Apache License, Version 2.0 (the "License"); you may not use this file except in compliance with
the License. You may obtain a copy of the License at
http://www.apache.org/licenses/LICENSE-2.0
Unless required by applicable law or agreed to in writing, software distributed under the License is distributed on
an "AS IS" BASIS, WITHOUT WARRANTIES OR CONDITIONS OF ANY KIND, either express or implied. See the License for the
specific language governing permissions and limitations under the License.
โ ๏ธ Note that this file is in Markdown but contain specific syntax for our doc-builder (similar to MDX) that may not be
rendered properly in your Markdown viewer.
-->
# Modelli multilingue per l'inferenza
[[open-in-colab]]
Ci sono diversi modelli multilingue in ๐ค Transformers, e il loro utilizzo per l'inferenza differisce da quello dei modelli monolingua. Non *tutti* gli utilizzi dei modelli multilingue sono perรฒ diversi. Alcuni modelli, come [bert-base-multilingual-uncased](https://huggingface.co/bert-base-multilingual-uncased), possono essere usati come un modello monolingua. Questa guida ti mostrerร come utilizzare modelli multilingue che utilizzano un modo diverso per fare l'inferenza.
## XLM
XLM ha dieci diversi checkpoint, di cui solo uno รจ monolingua. I nove checkpoint rimanenti possono essere suddivisi in due categorie: i checkpoint che utilizzano i language embeddings e quelli che non li utilizzano.
### XLM con language embeddings
I seguenti modelli XLM utilizzano gli embeddings linguistici per specificare la lingua utilizzata per l'inferenza:
- `xlm-mlm-ende-1024` (Modellazione mascherata del linguaggio (Masked language modeling, in inglese), Inglese-Tedesco)
- `xlm-mlm-enfr-1024` (Modellazione mascherata del linguaggio, Inglese-Francese)
- `xlm-mlm-enro-1024` (Modellazione mascherata del linguaggio, Inglese-Rumeno)
- `xlm-mlm-xnli15-1024` (Modellazione mascherata del linguaggio, lingue XNLI)
- `xlm-mlm-tlm-xnli15-1024` (Modellazione mascherata del linguaggio + traduzione, lingue XNLI)
- `xlm-clm-enfr-1024` (Modellazione causale del linguaggio, Inglese-Francese)
- `xlm-clm-ende-1024` (Modellazione causale del linguaggio, Inglese-Tedesco)
Gli embeddings linguistici sono rappresentati come un tensore delle stesse dimensioni dell' `input_ids` passato al modello. I valori in questi tensori dipendono dal linguaggio usato e sono identificati dagli attributi `lang2id` e `id2lang` del tokenizer.
In questo esempio, carica il checkpoint `xlm-clm-enfr-1024` (Modellazione causale del linguaggio, Inglese-Francese):
```py
>>> import torch
>>> from transformers import XLMTokenizer, XLMWithLMHeadModel
>>> tokenizer = XLMTokenizer.from_pretrained("xlm-clm-enfr-1024")
>>> model = XLMWithLMHeadModel.from_pretrained("xlm-clm-enfr-1024")
```
L'attributo `lang2id` del tokenizer mostra il linguaggio del modello e il suo ids:
```py
>>> print(tokenizer.lang2id)
{'en': 0, 'fr': 1}
```
Poi, crea un esempio di input:
```py
>>> input_ids = torch.tensor([tokenizer.encode("Wikipedia was used to")]) # batch size of 1
```
Imposta l'id del linguaggio a `"en"` e usalo per definire il language embedding. Il language embedding รจ un tensore riempito con `0` perchรฉ questo รจ il language id per l'inglese. Questo tensore dovrebbe avere la stessa dimensione di `input_ids`.
```py
>>> language_id = tokenizer.lang2id["en"] # 0
>>> langs = torch.tensor([language_id] * input_ids.shape[1]) # torch.tensor([0, 0, 0, ..., 0])
>>> # We reshape it to be of size (batch_size, sequence_length)
>>> langs = langs.view(1, -1) # is now of shape [1, sequence_length] (we have a batch size of 1)
```
Adesso puoi inserire `input_ids` e language embedding nel modello:
```py
>>> outputs = model(input_ids, langs=langs)
```
Lo script [run_generation.py](https://github.com/huggingface/transformers/tree/main/examples/pytorch/text-generation/run_generation.py) puรฒ generare testo tramite i language embeddings usando i checkpoints `xlm-clm`.
### XLM senza language embeddings
I seguenti modelli XLM non richiedono l'utilizzo dei language embeddings per fare inferenza:
- `xlm-mlm-17-1280` (Modellazione mascherata del linguaggio, 17 lingue)
- `xlm-mlm-100-1280` (Modellazione mascherata del linguaggio, 100 lingue)
Questi modelli sono utilizzati per rappresentazioni generiche di frasi, a differenza dei precedenti checkpoints XML.
## BERT
Il seguente modello BERT puรฒ essere usato per compiti multilingue:
- `bert-base-multilingual-uncased` (Modellazione mascherata del linguaggio + Previsione della prossima frase, 102 lingue)
- `bert-base-multilingual-cased` (Modellazione mascherata del linguaggio + Previsione della prossima frase, 104 lingue)
Questi modelli non richiedono language embeddings per fare inferenza. Riescono ad identificare il linguaggio dal contesto e inferire di conseguenza.
## XLM-RoBERTa
Il seguente modello XLM-RoBERTa puรฒ essere usato per compiti multilingue:
- `xlm-roberta-base` (Modellazione mascherata del linguaggio, 100 lingue)
- `xlm-roberta-large` (Modellazione mascherata del linguaggio, 100 lingue)
XLM-RoBERTa รจ stato addestrato su 2.5TB di dati CommonCrawl appena creati e puliti in 100 lingue. Offre notevoli vantaggi rispetto ai modelli multilingue rilasciati in precedenza, come mBERT o XLM, in compiti come la classificazione, l'etichettatura delle sequenze e la risposta alle domande.
## M2M100
Il seguente modello M2M100 puรฒ essere usato per compiti multilingue:
- `facebook/m2m100_418M` (Traduzione)
- `facebook/m2m100_1.2B` (Traduzione)
In questo esempio, carica il checkpoint `facebook/m2m100_418M` per tradurre dal cinese all'inglese. Puoi impostare la lingua di partenza nel tokenizer:
```py
>>> from transformers import M2M100ForConditionalGeneration, M2M100Tokenizer
>>> en_text = "Do not meddle in the affairs of wizards, for they are subtle and quick to anger."
>>> chinese_text = "ไธ่ฆๆๆๅทซๅธซ็ไบๅ, ๅ ็บไปๅๆฏๅพฎๅฆ็, ๅพๅฟซๅฐฑๆ็ผๆ."
>>> tokenizer = M2M100Tokenizer.from_pretrained("facebook/m2m100_418M", src_lang="zh")
>>> model = M2M100ForConditionalGeneration.from_pretrained("facebook/m2m100_418M")
```
Applica il tokenizer al testo:
```py
>>> encoded_zh = tokenizer(chinese_text, return_tensors="pt")
```
M2M100 forza l'id della lingua obiettivo come primo token generato per tradurre nella lingua obiettivo. Imposta il parametro `forced_bos_token_id` a `en` nel metodo `generate` per tradurre in inglese:
```py
>>> generated_tokens = model.generate(**encoded_zh, forced_bos_token_id=tokenizer.get_lang_id("en"))
>>> tokenizer.batch_decode(generated_tokens, skip_special_tokens=True)
'Do not interfere with the matters of the witches, because they are delicate and will soon be angry.'
```
## MBart
Il seguente modello MBart puรฒ essere usato per compiti multilingue:
- `facebook/mbart-large-50-one-to-many-mmt` (Traduzione automatica multilingue uno-a-molti, 50 lingue)
- `facebook/mbart-large-50-many-to-many-mmt` (Traduzione automatica multilingue molti-a-molti, 50 lingue)
- `facebook/mbart-large-50-many-to-one-mmt` (Traduzione automatica multilingue molti-a-uno, 50 lingue)
- `facebook/mbart-large-50` (Traduzione multilingue, 50 lingue)
- `facebook/mbart-large-cc25`
In questo esempio, carica il checkpoint `facebook/mbart-large-50-many-to-many-mmt` per tradurre dal finlandese all'inglese. Puoi impostare la lingua di partenza nel tokenizer:
```py
>>> from transformers import AutoTokenizer, AutoModelForSeq2SeqLM
>>> en_text = "Do not meddle in the affairs of wizards, for they are subtle and quick to anger."
>>> fi_text = "รlรค sekaannu velhojen asioihin, sillรค ne ovat hienovaraisia ja nopeasti vihaisia."
>>> tokenizer = AutoTokenizer.from_pretrained("facebook/mbart-large-50-many-to-many-mmt", src_lang="fi_FI")
>>> model = AutoModelForSeq2SeqLM.from_pretrained("facebook/mbart-large-50-many-to-many-mmt")
```
Applica il tokenizer sul testo:
```py
>>> encoded_en = tokenizer(en_text, return_tensors="pt")
```
MBart forza l'id della lingua obiettivo come primo token generato per tradurre nella lingua obiettivo. Imposta il parametro `forced_bos_token_id` a `en` nel metodo `generate` per tradurre in inglese:
```py
>>> generated_tokens = model.generate(**encoded_en, forced_bos_token_id=tokenizer.lang_code_to_id("en_XX"))
>>> tokenizer.batch_decode(generated_tokens, skip_special_tokens=True)
"Don't interfere with the wizard's affairs, because they are subtle, will soon get angry."
```
Se stai usando il checkpoint `facebook/mbart-large-50-many-to-one-mmt`, non hai bisogno di forzare l'id della lingua obiettivo come primo token generato altrimenti l'uso รจ lo stesso. | 0 |
hf_public_repos/transformers/docs/source | hf_public_repos/transformers/docs/source/it/preprocessing.md | <!--Copyright 2022 The HuggingFace Team. All rights reserved.
Licensed under the Apache License, Version 2.0 (the "License"); you may not use this file except in compliance with
the License. You may obtain a copy of the License at
http://www.apache.org/licenses/LICENSE-2.0
Unless required by applicable law or agreed to in writing, software distributed under the License is distributed on
an "AS IS" BASIS, WITHOUT WARRANTIES OR CONDITIONS OF ANY KIND, either express or implied. See the License for the
specific language governing permissions and limitations under the License.
โ ๏ธ Note that this file is in Markdown but contain specific syntax for our doc-builder (similar to MDX) that may not be
rendered properly in your Markdown viewer.
-->
# Preprocess
[[open-in-colab]]
Prima di poter usare i dati in un modello, bisogna processarli in un formato accettabile per quest'ultimo. Un modello non comprende il testo grezzo, le immagini o l'audio. Bisogna convertire questi input in numeri e assemblarli all'interno di tensori. In questa esercitazione, tu potrai:
* Preprocessare dati testuali con un tokenizer.
* Preprocessare immagini o dati audio con un estrattore di caratteristiche.
* Preprocessare dati per attivitร multimodali mediante un processore.
## NLP
<Youtube id="Yffk5aydLzg"/>
Lo strumento principale per processare dati testuali รจ un [tokenizer](main_classes/tokenizer). Un tokenizer inizia separando il testo in *tokens* secondo una serie di regole. I tokens sono convertiti in numeri, questi vengono utilizzati per costruire i tensori di input del modello. Anche altri input addizionali se richiesti dal modello vengono aggiunti dal tokenizer.
<Tip>
Se stai pensando si utilizzare un modello preaddestrato, รจ importante utilizzare il tokenizer preaddestrato associato. Questo assicura che il testo sia separato allo stesso modo che nel corpus usato per l'addestramento, e venga usata la stessa mappatura tokens-to-index (solitamente indicato come il *vocabolario*) come nel preaddestramento.
</Tip>
Iniziamo subito caricando un tokenizer preaddestrato con la classe [`AutoTokenizer`]. Questo scarica il *vocabolario* usato quando il modello รจ stato preaddestrato.
### Tokenize
Carica un tokenizer preaddestrato con [`AutoTokenizer.from_pretrained`]:
```py
>>> from transformers import AutoTokenizer
>>> tokenizer = AutoTokenizer.from_pretrained("bert-base-cased")
```
Poi inserisci le tue frasi nel tokenizer:
```py
>>> encoded_input = tokenizer("Do not meddle in the affairs of wizards, for they are subtle and quick to anger.")
>>> print(encoded_input)
{'input_ids': [101, 2079, 2025, 19960, 10362, 1999, 1996, 3821, 1997, 16657, 1010, 2005, 2027, 2024, 11259, 1998, 4248, 2000, 4963, 1012, 102],
'token_type_ids': [0, 0, 0, 0, 0, 0, 0, 0, 0, 0, 0, 0, 0, 0, 0, 0, 0, 0, 0, 0, 0],
'attention_mask': [1, 1, 1, 1, 1, 1, 1, 1, 1, 1, 1, 1, 1, 1, 1, 1, 1, 1, 1, 1, 1]}
```
Il tokenizer restituisce un dizionario contenente tre oggetti importanti:
* [input_ids](glossary#input-ids) sono gli indici che corrispondono ad ogni token nella frase.
* [attention_mask](glossary#attention-mask) indicata se un token deve essere elaborato o no.
* [token_type_ids](glossary#token-type-ids) identifica a quale sequenza appartiene un token se รจ presente piรน di una sequenza.
Si possono decodificare gli `input_ids` per farsi restituire l'input originale:
```py
>>> tokenizer.decode(encoded_input["input_ids"])
'[CLS] Do not meddle in the affairs of wizards, for they are subtle and quick to anger. [SEP]'
```
Come si puรฒ vedere, il tokenizer aggiunge due token speciali - `CLS` e `SEP` (classificatore e separatore) - alla frase. Non tutti i modelli hanno bisogno dei token speciali, ma se servono, il tokenizer li aggiungerร automaticamente.
Se ci sono piรน frasi che vuoi processare, passale come una lista al tokenizer:
```py
>>> batch_sentences = [
... "But what about second breakfast?",
... "Don't think he knows about second breakfast, Pip.",
... "What about elevensies?",
... ]
>>> encoded_inputs = tokenizer(batch_sentences)
>>> print(encoded_inputs)
{'input_ids': [[101, 1252, 1184, 1164, 1248, 6462, 136, 102],
[101, 1790, 112, 189, 1341, 1119, 3520, 1164, 1248, 6462, 117, 21902, 1643, 119, 102],
[101, 1327, 1164, 5450, 23434, 136, 102]],
'token_type_ids': [[0, 0, 0, 0, 0, 0, 0, 0],
[0, 0, 0, 0, 0, 0, 0, 0, 0, 0, 0, 0, 0, 0, 0],
[0, 0, 0, 0, 0, 0, 0]],
'attention_mask': [[1, 1, 1, 1, 1, 1, 1, 1],
[1, 1, 1, 1, 1, 1, 1, 1, 1, 1, 1, 1, 1, 1, 1],
[1, 1, 1, 1, 1, 1, 1]]}
```
### Pad
Questo รจ un argomento importante. Quando processi un insieme di frasi potrebbero non avere tutte la stessa lunghezza. Questo รจ un problema perchรจ i tensori, in input del modello, devono avere dimensioni uniformi. Il padding รจ una strategia per assicurarsi che i tensori siano rettangolari aggiungendo uno speciale *padding token* alle frasi piรน corte.
Imposta il parametro `padding` a `True` per imbottire le frasi piรน corte nel gruppo in modo che combacino con la massima lunghezza presente:
```py
>>> batch_sentences = [
... "But what about second breakfast?",
... "Don't think he knows about second breakfast, Pip.",
... "What about elevensies?",
... ]
>>> encoded_input = tokenizer(batch_sentences, padding=True)
>>> print(encoded_input)
{'input_ids': [[101, 1252, 1184, 1164, 1248, 6462, 136, 102, 0, 0, 0, 0, 0, 0, 0],
[101, 1790, 112, 189, 1341, 1119, 3520, 1164, 1248, 6462, 117, 21902, 1643, 119, 102],
[101, 1327, 1164, 5450, 23434, 136, 102, 0, 0, 0, 0, 0, 0, 0, 0]],
'token_type_ids': [[0, 0, 0, 0, 0, 0, 0, 0, 0, 0, 0, 0, 0, 0, 0],
[0, 0, 0, 0, 0, 0, 0, 0, 0, 0, 0, 0, 0, 0, 0],
[0, 0, 0, 0, 0, 0, 0, 0, 0, 0, 0, 0, 0, 0, 0]],
'attention_mask': [[1, 1, 1, 1, 1, 1, 1, 1, 0, 0, 0, 0, 0, 0, 0],
[1, 1, 1, 1, 1, 1, 1, 1, 1, 1, 1, 1, 1, 1, 1],
[1, 1, 1, 1, 1, 1, 1, 0, 0, 0, 0, 0, 0, 0, 0]]}
```
Nota che il tokenizer aggiunge alle sequenze degli `0` perchรจ sono troppo corte!
### Truncation
L'altra faccia della medaglia รจ che avolte le sequenze possono essere troppo lunghe per essere gestite dal modello. In questo caso, avrai bisogno di troncare la sequenza per avere una lunghezza minore.
Imposta il parametro `truncation` a `True` per troncare una sequenza alla massima lunghezza accettata dal modello:
```py
>>> batch_sentences = [
... "But what about second breakfast?",
... "Don't think he knows about second breakfast, Pip.",
... "What about elevensies?",
... ]
>>> encoded_input = tokenizer(batch_sentences, padding=True, truncation=True)
>>> print(encoded_input)
{'input_ids': [[101, 1252, 1184, 1164, 1248, 6462, 136, 102, 0, 0, 0, 0, 0, 0, 0],
[101, 1790, 112, 189, 1341, 1119, 3520, 1164, 1248, 6462, 117, 21902, 1643, 119, 102],
[101, 1327, 1164, 5450, 23434, 136, 102, 0, 0, 0, 0, 0, 0, 0, 0]],
'token_type_ids': [[0, 0, 0, 0, 0, 0, 0, 0, 0, 0, 0, 0, 0, 0, 0],
[0, 0, 0, 0, 0, 0, 0, 0, 0, 0, 0, 0, 0, 0, 0],
[0, 0, 0, 0, 0, 0, 0, 0, 0, 0, 0, 0, 0, 0, 0]],
'attention_mask': [[1, 1, 1, 1, 1, 1, 1, 1, 0, 0, 0, 0, 0, 0, 0],
[1, 1, 1, 1, 1, 1, 1, 1, 1, 1, 1, 1, 1, 1, 1],
[1, 1, 1, 1, 1, 1, 1, 0, 0, 0, 0, 0, 0, 0, 0]]}
```
### Costruire i tensori
Infine, vuoi che il tokenizer restituisca i tensori prodotti dal modello.
Imposta il parametro `return_tensors` su `pt` per PyTorch, o `tf` per TensorFlow:
```py
>>> batch_sentences = [
... "But what about second breakfast?",
... "Don't think he knows about second breakfast, Pip.",
... "What about elevensies?",
... ]
>>> encoded_input = tokenizer(batch, padding=True, truncation=True, return_tensors="pt")
>>> print(encoded_input)
{'input_ids': tensor([[ 101, 153, 7719, 21490, 1122, 1114, 9582, 1623, 102],
[ 101, 5226, 1122, 9649, 1199, 2610, 1236, 102, 0]]),
'token_type_ids': tensor([[0, 0, 0, 0, 0, 0, 0, 0, 0],
[0, 0, 0, 0, 0, 0, 0, 0, 0]]),
'attention_mask': tensor([[1, 1, 1, 1, 1, 1, 1, 1, 1],
[1, 1, 1, 1, 1, 1, 1, 1, 0]])}
===PT-TF-SPLIT===
>>> batch_sentences = [
... "But what about second breakfast?",
... "Don't think he knows about second breakfast, Pip.",
... "What about elevensies?",
... ]
>>> encoded_input = tokenizer(batch, padding=True, truncation=True, return_tensors="tf")
>>> print(encoded_input)
{'input_ids': <tf.Tensor: shape=(2, 9), dtype=int32, numpy=
array([[ 101, 153, 7719, 21490, 1122, 1114, 9582, 1623, 102],
[ 101, 5226, 1122, 9649, 1199, 2610, 1236, 102, 0]],
dtype=int32)>,
'token_type_ids': <tf.Tensor: shape=(2, 9), dtype=int32, numpy=
array([[0, 0, 0, 0, 0, 0, 0, 0, 0],
[0, 0, 0, 0, 0, 0, 0, 0, 0]], dtype=int32)>,
'attention_mask': <tf.Tensor: shape=(2, 9), dtype=int32, numpy=
array([[1, 1, 1, 1, 1, 1, 1, 1, 1],
[1, 1, 1, 1, 1, 1, 1, 1, 0]], dtype=int32)>}
```
## Audio
Gli input audio sono processati in modo differente rispetto al testo, ma l'obiettivo rimane lo stesso: creare sequenze numeriche che il modello puรฒ capire. Un [estrattore di caratteristiche](main_classes/feature_extractor) รจ progettato con lo scopo preciso di estrarre caratteristiche da immagini o dati audio grezzi e convertirli in tensori. Prima di iniziare, installa ๐ค Datasets per caricare un dataset audio e sperimentare:
```bash
pip install datasets
```
Carica il dataset [MInDS-14](https://huggingface.co/datasets/PolyAI/minds14) (vedi il ๐ค [Datasets tutorial](https://huggingface.co/docs/datasets/load_hub.html) per avere maggiori dettagli su come caricare un dataset):
```py
>>> from datasets import load_dataset, Audio
>>> dataset = load_dataset("PolyAI/minds14", name="en-US", split="train")
```
Accedi al primo elemento della colonna `audio` per dare uno sguardo all'input. Richiamando la colonna `audio` sarร caricato automaticamente e ricampionato il file audio:
```py
>>> dataset[0]["audio"]
{'array': array([ 0. , 0.00024414, -0.00024414, ..., -0.00024414,
0. , 0. ], dtype=float32),
'path': '/root/.cache/huggingface/datasets/downloads/extracted/f14948e0e84be638dd7943ac36518a4cf3324e8b7aa331c5ab11541518e9368c/en-US~JOINT_ACCOUNT/602ba55abb1e6d0fbce92065.wav',
'sampling_rate': 8000}
```
Questo restituisce tre oggetti:
* `array` รจ il segnale vocale caricato - e potenzialmente ricampionato - come vettore 1D.
* `path` il percorso del file audio.
* `sampling_rate` si riferisce al numero di campioni del segnale vocale misurati al secondo.
### Ricampionamento
Per questo tutorial, puoi usare il modello [Wav2Vec2](https://huggingface.co/facebook/wav2vec2-base). Come puoi vedere dalla model card, il modello Wav2Vec2 รจ preaddestrato su un campionamento vocale a 16kHz.ร importante che la frequenza di campionamento dei tuoi dati audio combaci con la frequenza di campionamento del dataset usato per preaddestrare il modello. Se la frequenza di campionamento dei tuoi dati non รจ uguale dovrai ricampionare i tuoi dati audio.
Per esempio, il dataset [MInDS-14](https://huggingface.co/datasets/PolyAI/minds14) ha una frequenza di campionamento di 8000kHz. Utilizzando il modello Wav2Vec2 su questo dataset, alzala a 16kHz:
```py
>>> dataset = load_dataset("PolyAI/minds14", name="en-US", split="train")
>>> dataset[0]["audio"]
{'array': array([ 0. , 0.00024414, -0.00024414, ..., -0.00024414,
0. , 0. ], dtype=float32),
'path': '/root/.cache/huggingface/datasets/downloads/extracted/f14948e0e84be638dd7943ac36518a4cf3324e8b7aa331c5ab11541518e9368c/en-US~JOINT_ACCOUNT/602ba55abb1e6d0fbce92065.wav',
'sampling_rate': 8000}
```
1. Usa il metodo di ๐ค Datasets' [`cast_column`](https://huggingface.co/docs/datasets/package_reference/main_classes.html#datasets.Dataset.cast_column) per alzare la frequenza di campionamento a 16kHz:
```py
>>> dataset = dataset.cast_column("audio", Audio(sampling_rate=16_000))
```
2. Carica il file audio:
```py
>>> dataset[0]["audio"]
{'array': array([ 2.3443763e-05, 2.1729663e-04, 2.2145823e-04, ...,
3.8356509e-05, -7.3497440e-06, -2.1754686e-05], dtype=float32),
'path': '/root/.cache/huggingface/datasets/downloads/extracted/f14948e0e84be638dd7943ac36518a4cf3324e8b7aa331c5ab11541518e9368c/en-US~JOINT_ACCOUNT/602ba55abb1e6d0fbce92065.wav',
'sampling_rate': 16000}
```
Come puoi notare, la `sampling_rate` adesso รจ 16kHz!
### Feature extractor
Il prossimo passo รจ caricare un estrattore di caratteristiche per normalizzare e fare padding sull'input. Quando applichiamo il padding sui dati testuali, uno `0` รจ aggiunto alle sequenze piรน brevi. La stessa idea si applica ai dati audio, l'estrattore di caratteristiche per gli audio aggiungerร uno `0` - interpretato come silenzio - agli `array`.
Carica l'estrattore delle caratteristiche con [`AutoFeatureExtractor.from_pretrained`]:
```py
>>> from transformers import AutoFeatureExtractor
>>> feature_extractor = AutoFeatureExtractor.from_pretrained("facebook/wav2vec2-base")
```
Inserisci l' `array` audio nell'estrattore delle caratteristiche. Noi raccomandiamo sempre di aggiungere il parametro `sampling_rate` nell'estrattore delle caratteristiche per correggere meglio qualche errore, dovuto ai silenzi, che potrebbe verificarsi.
```py
>>> audio_input = [dataset[0]["audio"]["array"]]
>>> feature_extractor(audio_input, sampling_rate=16000)
{'input_values': [array([ 3.8106556e-04, 2.7506407e-03, 2.8015103e-03, ...,
5.6335266e-04, 4.6588284e-06, -1.7142107e-04], dtype=float32)]}
```
### Pad e truncate
Come per il tokenizer, puoi applicare le operazioni padding o truncation per manipolare sequenze di variabili a lotti. Dai uno sguaro alla lunghezza delle sequenze di questi due campioni audio:
```py
>>> dataset[0]["audio"]["array"].shape
(173398,)
>>> dataset[1]["audio"]["array"].shape
(106496,)
```
Come puoi vedere, il primo campione ha una sequenza piรน lunga del secondo. Crea una funzione che preprocesserร il dataset. Specifica una lunghezza massima del campione, e l'estrattore di features si occuperร di riempire o troncare la sequenza per coincidervi:
```py
>>> def preprocess_function(examples):
... audio_arrays = [x["array"] for x in examples["audio"]]
... inputs = feature_extractor(
... audio_arrays,
... sampling_rate=16000,
... padding=True,
... max_length=100000,
... truncation=True,
... )
... return inputs
```
Applica la funzione ai primi esempi nel dataset:
```py
>>> processed_dataset = preprocess_function(dataset[:5])
```
Adesso guarda la lunghezza dei campioni elaborati:
```py
>>> processed_dataset["input_values"][0].shape
(100000,)
>>> processed_dataset["input_values"][1].shape
(100000,)
```
La lunghezza dei campioni adesso coincide con la massima lunghezza impostata nelle funzione.
## Vision
Un estrattore di caratteristiche si puรฒ usare anche per processare immagini e per compiti di visione. Ancora una volta, l'obiettivo รจ convertire l'immagine grezza in un lotto di tensori come input.
Carica il dataset [food101](https://huggingface.co/datasets/food101) per questa esercitazione. Usa il parametro `split` di ๐ค Datasets per caricare solo un piccolo campione dal dataset di addestramento poichรจ il set di dati รจ molto grande:
```py
>>> from datasets import load_dataset
>>> dataset = load_dataset("food101", split="train[:100]")
```
Secondo passo, dai uno sguardo alle immagini usando la caratteristica [`Image`](https://huggingface.co/docs/datasets/package_reference/main_classes.html?highlight=image#datasets.Image) di ๐ค Datasets:
```py
>>> dataset[0]["image"]
```
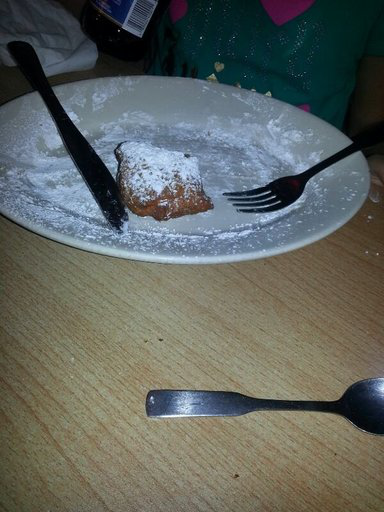
### Feature extractor
Carica l'estrattore di caratteristiche [`AutoFeatureExtractor.from_pretrained`]:
```py
>>> from transformers import AutoFeatureExtractor
>>> feature_extractor = AutoFeatureExtractor.from_pretrained("google/vit-base-patch16-224")
```
### Data augmentation
Per le attivitร di visione, รจ usuale aggiungere alcuni tipi di data augmentation alle immagini come parte del preprocessing. Puoi aggiungere augmentations con qualsiasi libreria che preferisci, ma in questa esercitazione, userai il modulo [`transforms`](https://pytorch.org/vision/stable/transforms.html) di torchvision.
1. Normalizza l'immagine e usa [`Compose`](https://pytorch.org/vision/master/generated/torchvision.transforms.Compose.html) per concatenare alcune trasformazioni - [`RandomResizedCrop`](https://pytorch.org/vision/main/generated/torchvision.transforms.RandomResizedCrop.html) e [`ColorJitter`](https://pytorch.org/vision/main/generated/torchvision.transforms.ColorJitter.html) - insieme:
```py
>>> from torchvision.transforms import Compose, Normalize, RandomResizedCrop, ColorJitter, ToTensor
>>> normalize = Normalize(mean=feature_extractor.image_mean, std=feature_extractor.image_std)
>>> _transforms = Compose(
... [RandomResizedCrop(feature_extractor.size), ColorJitter(brightness=0.5, hue=0.5), ToTensor(), normalize]
... )
```
2. Il modello accetta [`pixel_values`](model_doc/visionencoderdecoder#transformers.VisionEncoderDecoderModel.forward.pixel_values) come input. Questo valore รจ generato dall'estrattore di caratteristiche. Crea una funzione che genera `pixel_values` dai transforms:
```py
>>> def transforms(examples):
... examples["pixel_values"] = [_transforms(image.convert("RGB")) for image in examples["image"]]
... return examples
```
3. Poi utilizza ๐ค Datasets [`set_transform`](https://huggingface.co/docs/datasets/process.html#format-transform)per applicare al volo la trasformazione:
```py
>>> dataset.set_transform(transforms)
```
4. Adesso quando accedi all'immagine, puoi notare che l'estrattore di caratteristiche ha aggiunto `pixel_values` allo schema di input:
```py
>>> dataset[0]["image"]
{'image': <PIL.JpegImagePlugin.JpegImageFile image mode=RGB size=384x512 at 0x7F1A7B0630D0>,
'label': 6,
'pixel_values': tensor([[[ 0.0353, 0.0745, 0.1216, ..., -0.9922, -0.9922, -0.9922],
[-0.0196, 0.0667, 0.1294, ..., -0.9765, -0.9843, -0.9922],
[ 0.0196, 0.0824, 0.1137, ..., -0.9765, -0.9686, -0.8667],
...,
[ 0.0275, 0.0745, 0.0510, ..., -0.1137, -0.1216, -0.0824],
[ 0.0667, 0.0824, 0.0667, ..., -0.0588, -0.0745, -0.0980],
[ 0.0353, 0.0353, 0.0431, ..., -0.0039, -0.0039, -0.0588]],
[[ 0.2078, 0.2471, 0.2863, ..., -0.9451, -0.9373, -0.9451],
[ 0.1608, 0.2471, 0.3098, ..., -0.9373, -0.9451, -0.9373],
[ 0.2078, 0.2706, 0.3020, ..., -0.9608, -0.9373, -0.8275],
...,
[-0.0353, 0.0118, -0.0039, ..., -0.2392, -0.2471, -0.2078],
[ 0.0196, 0.0353, 0.0196, ..., -0.1843, -0.2000, -0.2235],
[-0.0118, -0.0039, -0.0039, ..., -0.0980, -0.0980, -0.1529]],
[[ 0.3961, 0.4431, 0.4980, ..., -0.9216, -0.9137, -0.9216],
[ 0.3569, 0.4510, 0.5216, ..., -0.9059, -0.9137, -0.9137],
[ 0.4118, 0.4745, 0.5216, ..., -0.9137, -0.8902, -0.7804],
...,
[-0.2314, -0.1922, -0.2078, ..., -0.4196, -0.4275, -0.3882],
[-0.1843, -0.1686, -0.2000, ..., -0.3647, -0.3804, -0.4039],
[-0.1922, -0.1922, -0.1922, ..., -0.2941, -0.2863, -0.3412]]])}
```
Di seguito come si vede l'immagine dopo la fase di preprocessing. Come ci si aspetterebbe dalle trasformazioni applicate, l'immagine รจ stata ritagliata in modo casuale e le proprietร del colore sono diverse.
```py
>>> import numpy as np
>>> import matplotlib.pyplot as plt
>>> img = dataset[0]["pixel_values"]
>>> plt.imshow(img.permute(1, 2, 0))
```
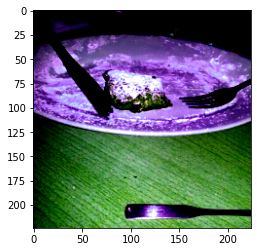
## Multimodal
Per attivitร multimodali userai una combinazione di tutto quello che hai imparato poco fa e applicherai le tue competenze alla comprensione automatica del parlato (Automatic Speech Recognition - ASR). Questo significa che avrai bisogno di:
* Un estrattore delle caratteristiche per processare i dati audio.
* Il Tokenizer per processare i testi.
Ritorna sul datasere [LJ Speech](https://huggingface.co/datasets/lj_speech):
```py
>>> from datasets import load_dataset
>>> lj_speech = load_dataset("lj_speech", split="train")
```
Visto che sei interessato solo alle colonne `audio` e `text`, elimina tutte le altre:
```py
>>> lj_speech = lj_speech.map(remove_columns=["file", "id", "normalized_text"])
```
Adesso guarda le colonne `audio` e `text`:
```py
>>> lj_speech[0]["audio"]
{'array': array([-7.3242188e-04, -7.6293945e-04, -6.4086914e-04, ...,
7.3242188e-04, 2.1362305e-04, 6.1035156e-05], dtype=float32),
'path': '/root/.cache/huggingface/datasets/downloads/extracted/917ece08c95cf0c4115e45294e3cd0dee724a1165b7fc11798369308a465bd26/LJSpeech-1.1/wavs/LJ001-0001.wav',
'sampling_rate': 22050}
>>> lj_speech[0]["text"]
'Printing, in the only sense with which we are at present concerned, differs from most if not from all the arts and crafts represented in the Exhibition'
```
Ricorda dalla sezione precedente sull'elaborazione dei dati audio, tu dovresti sempre [ricampionare](preprocessing#audio) la frequenza di campionamento dei tuoi dati audio per farla coincidere con quella del dataset usato dal modello preaddestrato:
```py
>>> lj_speech = lj_speech.cast_column("audio", Audio(sampling_rate=16_000))
```
### Processor
Un processor combina un estrattore di caratteristiche e un tokenizer. Carica un processor con [`AutoProcessor.from_pretrained]:
```py
>>> from transformers import AutoProcessor
>>> processor = AutoProcessor.from_pretrained("facebook/wav2vec2-base-960h")
```
1. Crea una funzione che processi i dati audio in `input_values`, e tokenizza il testo in `labels`. Questi sono i tuoi input per il modello:
```py
>>> def prepare_dataset(example):
... audio = example["audio"]
... example.update(processor(audio=audio["array"], text=example["text"], sampling_rate=16000))
... return example
```
2. Applica la funzione `prepare_dataset` ad un campione:
```py
>>> prepare_dataset(lj_speech[0])
```
Nota che il processor ha aggiunto `input_values` e `labels`. La frequenza di campionamento รจ stata corretta riducendola a 16kHz.
Fantastico, ora dovresti essere in grado di preelaborare i dati per qualsiasi modalitร e persino di combinare modalitร diverse! Nella prossima esercitazione, impareremo a mettere a punto un modello sui dati appena pre-elaborati. | 0 |
hf_public_repos/transformers/docs/source | hf_public_repos/transformers/docs/source/it/perf_train_special.md | <!--Copyright 2022 The HuggingFace Team. All rights reserved.
Licensed under the Apache License, Version 2.0 (the "License"); you may not use this file except in compliance with
the License. You may obtain a copy of the License at
http://www.apache.org/licenses/LICENSE-2.0
Unless required by applicable law or agreed to in writing, software distributed under the License is distributed on
an "AS IS" BASIS, WITHOUT WARRANTIES OR CONDITIONS OF ANY KIND, either express or implied. See the License for the
โ ๏ธ Note that this file is in Markdown but contain specific syntax for our doc-builder (similar to MDX) that may not be
rendered properly in your Markdown viewer.
-->
# Addestramento su Hardware Specializzato
<Tip>
Nota: Molte delle strategie introdotte nella [sezione sulla GPU singola](perf_train_gpu_one) (come mixed precision training o gradient accumulation) e [sezione multi-GPU](perf_train_gpu_many) sono generiche e applicabili all'addestramento di modelli in generale quindi assicurati di dargli un'occhiata prima di immergerti in questa sezione.
</Tip>
Questo documento sarร presto completato con informazioni su come effettuare la formazione su hardware specializzato.
| 0 |
hf_public_repos/transformers/docs/source | hf_public_repos/transformers/docs/source/it/perf_infer_gpu_many.md | <!--Copyright 2022 The HuggingFace Team. All rights reserved.
Licensed under the Apache License, Version 2.0 (the "License"); you may not use this file except in compliance with
the License. You may obtain a copy of the License at
http://www.apache.org/licenses/LICENSE-2.0
Unless required by applicable law or agreed to in writing, software distributed under the License is distributed on
an "AS IS" BASIS, WITHOUT WARRANTIES OR CONDITIONS OF ANY KIND, either express or implied. See the License for the
โ ๏ธ Note that this file is in Markdown but contain specific syntax for our doc-builder (similar to MDX) that may not be
rendered properly in your Markdown viewer.
-->
# Inferenza Efficiente su GPU Multiple
Questo documento contiene informazioni su come fare inferenza in maniera efficiente su GPU multiple.
<Tip>
Nota: Un setup con GPU multiple puรฒ utilizzare la maggior parte delle strategie descritte nella [sezione con GPU singola](./perf_infer_gpu_one). Tuttavia, รจ necessario conoscere delle tecniche semplici che possono essere utilizzate per un risultato migliore.
</Tip>
## `BetterTransformer` per inferenza piรน rapida
Abbiamo recentemente integrato `BetterTransformer` per inferenza piรน rapida su multi-GPU per modelli su testo, immagini e audio. Controlla il documento con queste integrazioni [qui](https://huggingface.co/docs/optimum/bettertransformer/overview) per maggiori dettagli.
| 0 |
hf_public_repos/transformers/docs/source | hf_public_repos/transformers/docs/source/it/perf_infer_cpu.md | <!--Copyright 2022 The HuggingFace Team. All rights reserved.
Licensed under the Apache License, Version 2.0 (the "License"); you may not use this file except in compliance with
the License. You may obtain a copy of the License at
http://www.apache.org/licenses/LICENSE-2.0
Unless required by applicable law or agreed to in writing, software distributed under the License is distributed on
an "AS IS" BASIS, WITHOUT WARRANTIES OR CONDITIONS OF ANY KIND, either express or implied. See the License for the
โ ๏ธ Note that this file is in Markdown but contain specific syntax for our doc-builder (similar to MDX) that may not be
rendered properly in your Markdown viewer.
-->
# Inferenza Efficiente su CPU
Questa guida si concentra sull'inferenza di modelli di grandi dimensioni in modo efficiente sulla CPU.
## `BetterTransformer` per inferenza piรน rapida
Abbiamo integrato di recente `BetterTransformer` per fare inferenza piรน rapidamente con modelli per testi, immagini e audio. Visualizza la documentazione sull'integrazione [qui](https://huggingface.co/docs/optimum/bettertransformer/overview) per maggiori dettagli.
## PyTorch JIT-mode (TorchScript)
TorchScript รจ un modo di creare modelli serializzabili e ottimizzabili da codice PyTorch. Ogni programmma TorchScript puรฒ esere salvato da un processo Python e caricato in un processo dove non ci sono dipendenze Python.
Comparandolo con l'eager mode di default, jit mode in PyTorch normalmente fornisce prestazioni migliori per l'inferenza del modello da parte di metodologie di ottimizzazione come la operator fusion.
Per una prima introduzione a TorchScript, vedi la Introduction to [PyTorch TorchScript tutorial](https://pytorch.org/tutorials/beginner/Intro_to_TorchScript_tutorial.html#tracing-modules).
### IPEX Graph Optimization con JIT-mode
Intelยฎ Extension per PyTorch fornnisce ulteriori ottimizzazioni in jit mode per i modelli della serie Transformers. Consigliamo vivamente agli utenti di usufruire dei vantaggi di Intelยฎ Extension per PyTorch con jit mode. Alcuni operator patterns usati fequentemente dai modelli Transformers models sono giร supportati in Intelยฎ Extension per PyTorch con jit mode fusions. Questi fusion patterns come Multi-head-attention fusion, Concat Linear, Linear+Add, Linear+Gelu, Add+LayerNorm fusion and etc. sono abilitati e hanno buone performance. I benefici della fusion รจ fornito agli utenti in modo trasparente. In base alle analisi, il ~70% dei problemi piรน popolari in NLP question-answering, text-classification, and token-classification possono avere benefici sulle performance grazie ai fusion patterns sia per Float32 precision che per BFloat16 Mixed precision.
Vedi maggiori informazioni per [IPEX Graph Optimization](https://intel.github.io/intel-extension-for-pytorch/cpu/latest/tutorials/features/graph_optimization.html).
#### Installazione di IPEX
I rilasci di IPEX seguono PyTorch, verifica i vari approcci per [IPEX installation](https://intel.github.io/intel-extension-for-pytorch/).
### Utilizzo del JIT-mode
Per abilitare JIT-mode in Trainer per evaluation e prediction, devi aggiungere `jit_mode_eval` negli argomenti di Trainer.
<Tip warning={true}>
per PyTorch >= 1.14.0. JIT-mode potrebe giovare a qualsiasi modello di prediction e evaluaion visto che il dict input รจ supportato in jit.trace
per PyTorch < 1.14.0. JIT-mode potrebbe giovare ai modelli il cui ordine dei parametri corrisponde all'ordine delle tuple in ingresso in jit.trace, come i modelli per question-answering.
Nel caso in cui l'ordine dei parametri seguenti non corrisponda all'ordine delle tuple in ingresso in jit.trace, come nei modelli di text-classification, jit.trace fallirร e lo cattureremo con una eccezione al fine di renderlo un fallback. Il logging รจ usato per notificare gli utenti.
</Tip>
Trovi un esempo con caso d'uso in [Transformers question-answering](https://github.com/huggingface/transformers/tree/main/examples/pytorch/question-answering)
- Inference using jit mode on CPU:
<pre>python run_qa.py \
--model_name_or_path csarron/bert-base-uncased-squad-v1 \
--dataset_name squad \
--do_eval \
--max_seq_length 384 \
--doc_stride 128 \
--output_dir /tmp/ \
--no_cuda \
<b>--jit_mode_eval </b></pre>
- Inference with IPEX using jit mode on CPU:
<pre>python run_qa.py \
--model_name_or_path csarron/bert-base-uncased-squad-v1 \
--dataset_name squad \
--do_eval \
--max_seq_length 384 \
--doc_stride 128 \
--output_dir /tmp/ \
--no_cuda \
<b>--use_ipex \</b>
<b>--jit_mode_eval</b></pre>
| 0 |
hf_public_repos/transformers/docs/source | hf_public_repos/transformers/docs/source/it/model_sharing.md | <!--Copyright 2022 The HuggingFace Team. All rights reserved.
Licensed under the Apache License, Version 2.0 (the "License"); you may not use this file except in compliance with
the License. You may obtain a copy of the License at
http://www.apache.org/licenses/LICENSE-2.0
Unless required by applicable law or agreed to in writing, software distributed under the License is distributed on
an "AS IS" BASIS, WITHOUT WARRANTIES OR CONDITIONS OF ANY KIND, either express or implied. See the License for the
specific language governing permissions and limitations under the License.
โ ๏ธ Note that this file is in Markdown but contain specific syntax for our doc-builder (similar to MDX) that may not be
rendered properly in your Markdown viewer.
-->
# Condividi un modello
Gli ultimi due tutorial ti hanno mostrato come puoi fare fine-tuning di un modello con PyTorch, Keras e ๐ค Accelerate per configurazioni distribuite. Il prossimo passo รจ quello di condividere il tuo modello con la community! In Hugging Face, crediamo nella condivisione della conoscenza e delle risorse in modo da democratizzare l'intelligenza artificiale per chiunque. Ti incoraggiamo a considerare di condividere il tuo modello con la community per aiutare altre persone a risparmiare tempo e risorse.
In questo tutorial, imparerai due metodi per la condivisione di un modello trained o fine-tuned nel [Model Hub](https://huggingface.co/models):
- Condividi in modo programmatico i tuoi file nell'Hub.
- Trascina i tuoi file nell'Hub mediante interfaccia grafica.
<iframe width="560" height="315" src="https://www.youtube.com/embed/XvSGPZFEjDY" title="YouTube video player"
frameborder="0" allow="accelerometer; autoplay; clipboard-write; encrypted-media; gyroscope;
picture-in-picture" allowfullscreen></iframe>
<Tip>
Per condividere un modello con la community, hai bisogno di un account su [huggingface.co](https://huggingface.co/join). Puoi anche unirti ad un'organizzazione esistente o crearne una nuova.
</Tip>
## Caratteristiche dei repository
Ogni repository nel Model Hub si comporta come un tipico repository di GitHub. I nostri repository offrono il versionamento, la cronologia dei commit, e la possibilitร di visualizzare le differenze.
Il versionamento all'interno del Model Hub รจ basato su git e [git-lfs](https://git-lfs.github.com/). In altre parole, puoi trattare un modello come un unico repository, consentendo un maggiore controllo degli accessi e maggiore scalabilitร . Il controllo delle versioni consente *revisions*, un metodo per appuntare una versione specifica di un modello con un hash di commit, un tag o un branch.
Come risultato, puoi caricare una specifica versione di un modello con il parametro `revision`:
```py
>>> model = AutoModel.from_pretrained(
... "julien-c/EsperBERTo-small", revision="v2.0.1" # nome di un tag, di un branch, o commit hash
... )
```
Anche i file possono essere modificati facilmente in un repository ed รจ possibile visualizzare la cronologia dei commit e le differenze:

## Configurazione
Prima di condividere un modello nell'Hub, hai bisogno delle tue credenziali di Hugging Face. Se hai accesso ad un terminale, esegui il seguente comando nell'ambiente virtuale in cui รจ installata la libreria ๐ค Transformers. Questo memorizzerร il tuo token di accesso nella cartella cache di Hugging Face (di default `~/.cache/`):
```bash
huggingface-cli login
```
Se stai usando un notebook come Jupyter o Colaboratory, assicurati di avere la libreria [`huggingface_hub`](https://huggingface.co/docs/hub/adding-a-library) installata. Questa libreria ti permette di interagire in maniera programmatica con l'Hub.
```bash
pip install huggingface_hub
```
Utilizza `notebook_login` per accedere all'Hub, e segui il link [qui](https://huggingface.co/settings/token) per generare un token con cui effettuare il login:
```py
>>> from huggingface_hub import notebook_login
>>> notebook_login()
```
## Converti un modello per tutti i framework
Per assicurarti che il tuo modello possa essere utilizzato da persone che lavorano con un framework differente, ti raccomandiamo di convertire e caricare il tuo modello sia con i checkpoint di PyTorch che con quelli di TensorFlow. Anche se รจ possibile caricare il modello da un framework diverso, se si salta questo passaggio, il caricamento sarร piรน lento perchรฉ ๐ค Transformers ha bisogno di convertire i checkpoint al momento.
Convertire un checkpoint per un altro framework รจ semplice. Assicurati di avere PyTorch e TensorFlow installati (vedi [qui](installation) per le istruzioni d'installazione), e poi trova il modello specifico per il tuo compito nell'altro framework.
<frameworkcontent>
<pt>
Specifica `from_tf=True` per convertire un checkpoint da TensorFlow a PyTorch:
```py
>>> pt_model = DistilBertForSequenceClassification.from_pretrained(
... "path/verso/il-nome-magnifico-che-hai-scelto", from_tf=True
... )
>>> pt_model.save_pretrained("path/verso/il-nome-magnifico-che-hai-scelto")
```
</pt>
<tf>
Specifica `from_pt=True` per convertire un checkpoint da PyTorch a TensorFlow:
```py
>>> tf_model = TFDistilBertForSequenceClassification.from_pretrained(
... "path/verso/il-nome-magnifico-che-hai-scelto", from_pt=True
... )
```
Poi puoi salvare il tuo nuovo modello in TensorFlow con il suo nuovo checkpoint:
```py
>>> tf_model.save_pretrained("path/verso/il-nome-magnifico-che-hai-scelto")
```
</tf>
<jax>
Se un modello รจ disponibile in Flax, puoi anche convertire un checkpoint da PyTorch a Flax:
```py
>>> flax_model = FlaxDistilBertForSequenceClassification.from_pretrained(
... "path/verso/il-nome-magnifico-che-hai-scelto", from_pt=True
... )
```
</jax>
</frameworkcontent>
## Condividi un modello durante il training
<frameworkcontent>
<pt>
<Youtube id="Z1-XMy-GNLQ"/>
Condividere un modello nell'Hub รจ tanto semplice quanto aggiungere un parametro extra o un callback. Ricorda dal [tutorial sul fine-tuning](training), la classe [`TrainingArguments`] รจ dove specifichi gli iperparametri e le opzioni addizionali per l'allenamento. Una di queste opzioni di training include l'abilitร di condividere direttamente un modello nell'Hub. Imposta `push_to_hub=True` in [`TrainingArguments`]:
```py
>>> training_args = TrainingArguments(output_dir="il-mio-bellissimo-modello", push_to_hub=True)
```
Passa gli argomenti per il training come di consueto al [`Trainer`]:
```py
>>> trainer = Trainer(
... model=model,
... args=training_args,
... train_dataset=small_train_dataset,
... eval_dataset=small_eval_dataset,
... compute_metrics=compute_metrics,
... )
```
Dopo aver effettuato il fine-tuning del tuo modello, chiama [`~transformers.Trainer.push_to_hub`] sul [`Trainer`] per condividere il modello allenato nell'Hub. ๐ค Transformers aggiungerร in modo automatico persino gli iperparametri, i risultati del training e le versioni del framework alla scheda del tuo modello (model card, in inglese)!
```py
>>> trainer.push_to_hub()
```
</pt>
<tf>
Condividi un modello nell'Hub con [`PushToHubCallback`]. Nella funzione [`PushToHubCallback`], aggiungi:
- Una directory di output per il tuo modello.
- Un tokenizer.
- L'`hub_model_id`, che รจ il tuo username sull'Hub e il nome del modello.
```py
>>> from transformers import PushToHubCallback
>>> push_to_hub_callback = PushToHubCallback(
... output_dir="./il_path_dove_salvare_il_tuo_modello",
... tokenizer=tokenizer,
... hub_model_id="il-tuo-username/il-mio-bellissimo-modello",
... )
```
Aggiungi il callback a [`fit`](https://keras.io/api/models/model_training_apis/), e ๐ค Transformers caricherร il modello allenato nell'Hub:
```py
>>> model.fit(tf_train_dataset, validation_data=tf_validation_dataset, epochs=3, callbacks=push_to_hub_callback)
```
</tf>
</frameworkcontent>
## Utilizzare la funzione `push_to_hub`
Puoi anche chiamare `push_to_hub` direttamente sul tuo modello per caricarlo nell'Hub.
Specifica il nome del tuo modello in `push_to_hub`:
```py
>>> pt_model.push_to_hub("il-mio-bellissimo-modello")
```
Questo crea un repository sotto il proprio username con il nome del modello `il-mio-bellissimo-modello`. Ora chiunque puรฒ caricare il tuo modello con la funzione `from_pretrained`:
```py
>>> from transformers import AutoModel
>>> model = AutoModel.from_pretrained("il-tuo-username/il-mio-bellissimo-modello")
```
Se fai parte di un'organizzazione e vuoi invece condividere un modello sotto il nome dell'organizzazione, aggiungi il parametro `organization`:
```py
>>> pt_model.push_to_hub("il-mio-bellissimo-modello", organization="la-mia-fantastica-org")
```
La funzione `push_to_hub` puรฒ essere anche utilizzata per aggiungere altri file al repository del modello. Per esempio, aggiungi un tokenizer ad un repository di un modello:
```py
>>> tokenizer.push_to_hub("il-mio-bellissimo-modello")
```
O magari potresti voler aggiungere la versione di TensorFlow del tuo modello PyTorch a cui hai fatto fine-tuning:
```py
>>> tf_model.push_to_hub("il-mio-bellissimo-modello")
```
Ora quando navighi nel tuo profilo Hugging Face, dovresti vedere il tuo repository del modello appena creato. Premendo sulla scheda **Files** vengono visualizzati tutti i file caricati nel repository.
Per maggiori dettagli su come creare e caricare file ad un repository, fai riferimento alla documentazione [qui](https://huggingface.co/docs/hub/how-to-upstream).
## Carica un modello utilizzando l'interfaccia web
Chi preferisce un approccio senza codice puรฒ caricare un modello tramite l'interfaccia web dell'hub. Visita [huggingface.co/new](https://huggingface.co/new) per creare un nuovo repository:
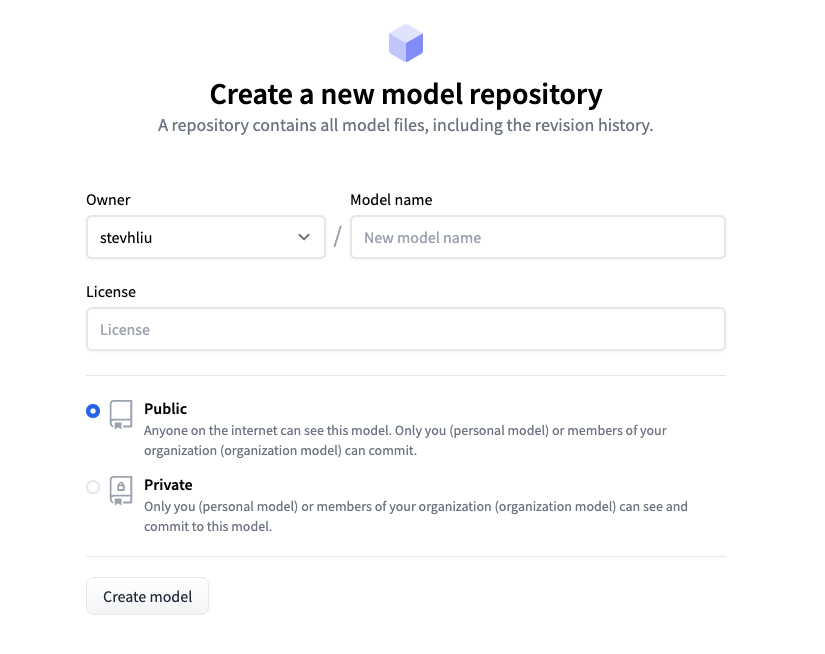
Da qui, aggiungi alcune informazioni sul tuo modello:
- Seleziona il/la **owner** del repository. Puoi essere te o qualunque organizzazione di cui fai parte.
- Scegli un nome per il tuo modello, il quale sarร anche il nome del repository.
- Scegli se il tuo modello รจ pubblico o privato.
- Specifica la licenza utilizzata per il tuo modello.
Ora premi sulla scheda **Files** e premi sul pulsante **Add file** per caricare un nuovo file al tuo repository. Trascina poi un file per caricarlo e aggiungere un messaggio di commit.
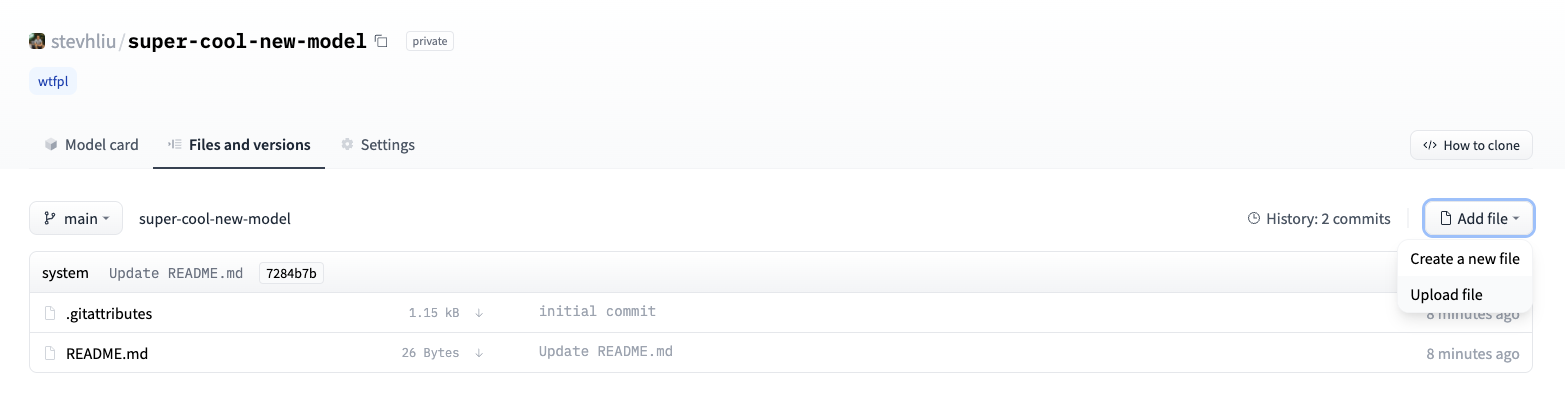
## Aggiungi una scheda del modello
Per assicurarti che chiunque possa comprendere le abilitร , limitazioni, i potenziali bias e le considerazioni etiche del tuo modello, per favore aggiungi una scheda del modello (model card, in inglese) al tuo repository. La scheda del modello รจ definita nel file `README.md`. Puoi aggiungere una scheda del modello:
* Creando manualmente e caricando un file `README.md`.
* Premendo sul pulsante **Edit model card** nel repository del tuo modello.
Dai un'occhiata alla [scheda del modello](https://huggingface.co/distilbert-base-uncased) di DistilBert per avere un buon esempio del tipo di informazioni che una scheda di un modello deve includere. Per maggiori dettagli legati ad altre opzioni che puoi controllare nel file `README.md`, come l'impatto ambientale o widget di esempio, fai riferimento alla documentazione [qui](https://huggingface.co/docs/hub/models-cards).
| 0 |
hf_public_repos/transformers/docs/source | hf_public_repos/transformers/docs/source/it/pipeline_tutorial.md | <!--Copyright 2022 The HuggingFace Team. All rights reserved.
Licensed under the Apache License, Version 2.0 (the "License"); you may not use this file except in compliance with
the License. You may obtain a copy of the License at
http://www.apache.org/licenses/LICENSE-2.0
Unless required by applicable law or agreed to in writing, software distributed under the License is distributed on
an "AS IS" BASIS, WITHOUT WARRANTIES OR CONDITIONS OF ANY KIND, either express or implied. See the License for the
specific language governing permissions and limitations under the License.
โ ๏ธ Note that this file is in Markdown but contain specific syntax for our doc-builder (similar to MDX) that may not be
rendered properly in your Markdown viewer.
-->
# Pipeline per l'inferenza
La [`pipeline`] rende semplice usare qualsiasi modello dal [Model Hub](https://huggingface.co/models) per fare inferenza su diversi compiti come generazione del testo, segmentazione di immagini e classificazione di audio. Anche se non hai esperienza con una modalitร specifica o non comprendi bene il codice che alimenta i modelli, รจ comunque possibile utilizzarli con l'opzione [`pipeline`]! Questa esercitazione ti insegnerร a:
* Usare una [`pipeline`] per fare inferenza.
* Usare uno specifico tokenizer o modello.
* Usare una [`pipeline`] per compiti che riguardano audio e video.
<Tip>
Dai un'occhiata alla documentazione di [`pipeline`] per una lista completa dei compiti supportati.
</Tip>
## Utilizzo della Pipeline
Nonostante ogni compito abbia una [`pipeline`] associata, รจ piรน semplice utilizzare l'astrazione generica della [`pipeline`] che contiene tutte quelle specifiche per ogni mansione. La [`pipeline`] carica automaticamente un modello predefinito e un tokenizer in grado di fare inferenza per il tuo compito.
1. Inizia creando una [`pipeline`] e specificando il compito su cui fare inferenza:
```py
>>> from transformers import pipeline
>>> generator = pipeline(task="text-generation")
```
2. Inserisci il testo in input nella [`pipeline`]:
```py
>>> generator(
... "Three Rings for the Elven-kings under the sky, Seven for the Dwarf-lords in their halls of stone"
... ) # doctest: +SKIP
[{'generated_text': 'Three Rings for the Elven-kings under the sky, Seven for the Dwarf-lords in their halls of stone, Seven for the Iron-priests at the door to the east, and thirteen for the Lord Kings at the end of the mountain'}]
```
Se hai piรน di un input, inseriscilo in una lista:
```py
>>> generator(
... [
... "Three Rings for the Elven-kings under the sky, Seven for the Dwarf-lords in their halls of stone",
... "Nine for Mortal Men, doomed to die, One for the Dark Lord on his dark throne",
... ]
... ) # doctest: +SKIP
```
Qualsiasi parametro addizionale per il tuo compito puรฒ essere incluso nella [`pipeline`]. La mansione `text-generation` ha un metodo [`~generation.GenerationMixin.generate`] con diversi parametri per controllare l'output. Ad esempio, se desideri generare piรน di un output, utilizza il parametro `num_return_sequences`:
```py
>>> generator(
... "Three Rings for the Elven-kings under the sky, Seven for the Dwarf-lords in their halls of stone",
... num_return_sequences=2,
... ) # doctest: +SKIP
```
### Scegliere modello e tokenizer
La [`pipeline`] accetta qualsiasi modello dal [Model Hub](https://huggingface.co/models). Ci sono tag nel Model Hub che consentono di filtrare i modelli per attivitร . Una volta che avrai scelto il modello appropriato, caricalo usando la corrispondente classe `AutoModelFor` e [`AutoTokenizer`]. Ad esempio, carica la classe [`AutoModelForCausalLM`] per un compito di causal language modeling:
```py
>>> from transformers import AutoTokenizer, AutoModelForCausalLM
>>> tokenizer = AutoTokenizer.from_pretrained("distilgpt2")
>>> model = AutoModelForCausalLM.from_pretrained("distilgpt2")
```
Crea una [`pipeline`] per il tuo compito, specificando il modello e il tokenizer che hai caricato:
```py
>>> from transformers import pipeline
>>> generator = pipeline(task="text-generation", model=model, tokenizer=tokenizer)
```
Inserisci il testo di input nella [`pipeline`] per generare del testo:
```py
>>> generator(
... "Three Rings for the Elven-kings under the sky, Seven for the Dwarf-lords in their halls of stone"
... ) # doctest: +SKIP
[{'generated_text': 'Three Rings for the Elven-kings under the sky, Seven for the Dwarf-lords in their halls of stone, Seven for the Dragon-lords (for them to rule in a world ruled by their rulers, and all who live within the realm'}]
```
## Audio pipeline
La flessibilitร della [`pipeline`] fa si che possa essere estesa ad attivitร sugli audio.
Per esempio, classifichiamo le emozioni in questo clip audio:
```py
>>> from datasets import load_dataset
>>> import torch
>>> torch.manual_seed(42) # doctest: +IGNORE_RESULT
>>> ds = load_dataset("hf-internal-testing/librispeech_asr_demo", "clean", split="validation")
>>> audio_file = ds[0]["audio"]["path"]
```
Trova un modello per la [classificazione audio](https://huggingface.co/models?pipeline_tag=audio-classification) sul Model Hub per eseguire un compito di riconoscimento automatico delle emozioni e caricalo nella [`pipeline`]:
```py
>>> from transformers import pipeline
>>> audio_classifier = pipeline(
... task="audio-classification", model="ehcalabres/wav2vec2-lg-xlsr-en-speech-emotion-recognition"
... )
```
Inserisci il file audio nella [`pipeline`]:
```py
>>> preds = audio_classifier(audio_file)
>>> preds = [{"score": round(pred["score"], 4), "label": pred["label"]} for pred in preds]
>>> preds
[{'score': 0.1315, 'label': 'calm'}, {'score': 0.1307, 'label': 'neutral'}, {'score': 0.1274, 'label': 'sad'}, {'score': 0.1261, 'label': 'fearful'}, {'score': 0.1242, 'label': 'happy'}]
```
## Vision pipeline
Infine, usare la [`pipeline`] per le attivitร sulle immagini รจ praticamente la stessa cosa.
Specifica la tua attivitร e inserisci l'immagine nel classificatore. L'immagine puรฒ essere sia un link che un percorso sul tuo pc in locale. Per esempio, quale specie di gatto รจ raffigurata qui sotto?

```py
>>> from transformers import pipeline
>>> vision_classifier = pipeline(task="image-classification")
>>> preds = vision_classifier(
... images="https://huggingface.co/datasets/huggingface/documentation-images/resolve/main/pipeline-cat-chonk.jpeg"
... )
>>> preds = [{"score": round(pred["score"], 4), "label": pred["label"]} for pred in preds]
>>> preds
[{'score': 0.4335, 'label': 'lynx, catamount'}, {'score': 0.0348, 'label': 'cougar, puma, catamount, mountain lion, painter, panther, Felis concolor'}, {'score': 0.0324, 'label': 'snow leopard, ounce, Panthera uncia'}, {'score': 0.0239, 'label': 'Egyptian cat'}, {'score': 0.0229, 'label': 'tiger cat'}]
```
| 0 |
hf_public_repos/transformers/docs/source | hf_public_repos/transformers/docs/source/it/_config.py | # docstyle-ignore
INSTALL_CONTENT = """
# Installazione di Transformers
! pip install transformers datasets
# Per installare dalla fonte invece dell'ultima versione rilasciata, commenta il comando sopra e
# rimuovi la modalitร commento al comando seguente.
# ! pip install git+https://github.com/huggingface/transformers.git
"""
notebook_first_cells = [{"type": "code", "content": INSTALL_CONTENT}]
black_avoid_patterns = {
"{processor_class}": "FakeProcessorClass",
"{model_class}": "FakeModelClass",
"{object_class}": "FakeObjectClass",
}
| 0 |
hf_public_repos/transformers/docs/source | hf_public_repos/transformers/docs/source/it/serialization.md | <!--Copyright 2020 The HuggingFace Team. All rights reserved.
Licensed under the Apache License, Version 2.0 (the "License"); you may not use this file except in compliance with
the License. You may obtain a copy of the License at
http://www.apache.org/licenses/LICENSE-2.0
Unless required by applicable law or agreed to in writing, software distributed under the License is distributed on
an "AS IS" BASIS, WITHOUT WARRANTIES OR CONDITIONS OF ANY KIND, either express or implied. See the License for the
specific language governing permissions and limitations under the License.
โ ๏ธ Note that this file is in Markdown but contain specific syntax for our doc-builder (similar to MDX) that may not be
rendered properly in your Markdown viewer.
-->
# Esporta modelli ๐ค Transformers
Se devi implementare ๐ค modelli Transformers in ambienti di produzione, noi
consigliamo di esportarli in un formato serializzato che puรฒ essere caricato ed eseguito
su runtime e hardware specializzati. In questa guida ti mostreremo come farlo
esporta ๐ค Modelli Transformers in due formati ampiamente utilizzati: ONNX e TorchScript.
Una volta esportato, un modello puรฒ essere ottimizato per l'inferenza tramite tecniche come
la quantizzazione e soppressione. Se sei interessato a ottimizzare i tuoi modelli per l'esecuzione
con la massima efficienza, dai un'occhiata a [๐ค Optimum
library](https://github.com/huggingface/optimum).
## ONNX
Il progetto [ONNX (Open Neural Network eXchange)](http://onnx.ai) Il progetto onnx รจ un open
standard che definisce un insieme comune di operatori e un formato di file comune a
rappresentano modelli di deep learning in un'ampia varietร di framework, tra cui
PyTorch e TensorFlow. Quando un modello viene esportato nel formato ONNX, questi
operatori sono usati per costruire un grafico computazionale (often called an
_intermediate representation_) che rappresenta il flusso di dati attraverso la
rete neurale.
Esponendo un grafico con operatori e tipi di dati standardizzati, ONNX rende
piรน facile passare da un framework all'altro. Ad esempio, un modello allenato in PyTorch puรฒ
essere esportato in formato ONNX e quindi importato in TensorFlow (e viceversa).
๐ค Transformers fornisce un pacchetto `transformers.onnx` che ti consente di
convertire i checkpoint del modello in un grafico ONNX sfruttando gli oggetti di configurazione.
Questi oggetti di configurazione sono giร pronti per una serie di architetture di modelli,
e sono progettati per essere facilmente estensibili ad altre architetture.
Le configurazioni pronte includono le seguenti architetture:
<!--This table is automatically generated by `make fix-copies`, do not fill manually!-->
- ALBERT
- BART
- BEiT
- BERT
- BigBird
- BigBird-Pegasus
- Blenderbot
- BlenderbotSmall
- CamemBERT
- ConvBERT
- Data2VecText
- Data2VecVision
- DeiT
- DistilBERT
- ELECTRA
- FlauBERT
- GPT Neo
- GPT-J
- I-BERT
- LayoutLM
- M2M100
- Marian
- mBART
- MobileBERT
- OpenAI GPT-2
- Perceiver
- PLBart
- RoBERTa
- RoFormer
- SqueezeBERT
- T5
- ViT
- XLM
- XLM-RoBERTa
- XLM-RoBERTa-XL
Nelle prossime due sezioni, ti mostreremo come:
* Esporta un modello supportato usando il pacchetto `transformers.onnx`.
* Esporta un modello personalizzato per un'architettura non supportata.
### Esportazione di un modello in ONNX
Per esportare un modello ๐ค Transformers in ONNX, dovrai prima installarne alcune
dipendenze extra:
```bash
pip install transformers[onnx]
```
Il pacchetto `transformers.onnx` puรฒ essere usato come modulo Python:
```bash
python -m transformers.onnx --help
usage: Hugging Face Transformers ONNX exporter [-h] -m MODEL [--feature {causal-lm, ...}] [--opset OPSET] [--atol ATOL] output
positional arguments:
output Path indicating where to store generated ONNX model.
optional arguments:
-h, --help show this help message and exit
-m MODEL, --model MODEL
Model ID on huggingface.co or path on disk to load model from.
--feature {causal-lm, ...}
The type of features to export the model with.
--opset OPSET ONNX opset version to export the model with.
--atol ATOL Absolute difference tolerance when validating the model.
```
L'esportazione di un checkpoint utilizzando una configurazione giร pronta puรฒ essere eseguita come segue:
```bash
python -m transformers.onnx --model=distilbert-base-uncased onnx/
```
che dovrebbe mostrare i seguenti log:
```bash
Validating ONNX model...
-[โ] ONNX model output names match reference model ({'last_hidden_state'})
- Validating ONNX Model output "last_hidden_state":
-[โ] (2, 8, 768) matches (2, 8, 768)
-[โ] all values close (atol: 1e-05)
All good, model saved at: onnx/model.onnx
```
Questo esporta un grafico ONNX del checkpoint definito dall'argomento `--model`.
In questo esempio รจ `distilbert-base-uncased`, ma puรฒ essere qualsiasi checkpoint
Hugging Face Hub o uno memorizzato localmente.
Il file risultante `model.onnx` puรฒ quindi essere eseguito su uno dei [tanti
acceleratori](https://onnx.ai/supported-tools.html#deployModel) che supportano il
lo standard ONNX. Ad esempio, possiamo caricare ed eseguire il modello con [ONNX
Runtime](https://onnxruntime.ai/) come segue:
```python
>>> from transformers import AutoTokenizer
>>> from onnxruntime import InferenceSession
>>> tokenizer = AutoTokenizer.from_pretrained("distilbert-base-uncased")
>>> session = InferenceSession("onnx/model.onnx")
>>> # ONNX Runtime expects NumPy arrays as input
>>> inputs = tokenizer("Using DistilBERT with ONNX Runtime!", return_tensors="np")
>>> outputs = session.run(output_names=["last_hidden_state"], input_feed=dict(inputs))
```
I nomi di output richiesti (cioรจ `["last_hidden_state"]`) possono essere ottenuti
dando un'occhiata alla configurazione ONNX di ogni modello. Ad esempio, per
DistilBERT abbiamo:
```python
>>> from transformers.models.distilbert import DistilBertConfig, DistilBertOnnxConfig
>>> config = DistilBertConfig()
>>> onnx_config = DistilBertOnnxConfig(config)
>>> print(list(onnx_config.outputs.keys()))
["last_hidden_state"]
```
Il processo รจ identico per i checkpoint TensorFlow sull'hub. Ad esempio, noi
possiamo esportare un checkpoint TensorFlow puro da [Keras
organizzazione](https://huggingface.co/keras-io) come segue:
```bash
python -m transformers.onnx --model=keras-io/transformers-qa onnx/
```
Per esportare un modello memorizzato localmente, devi disporre dei pesi del modello
e file tokenizer memorizzati in una directory. Ad esempio, possiamo caricare e salvare un
checkpoint come segue:
<frameworkcontent>
<pt>
```python
>>> from transformers import AutoTokenizer, AutoModelForSequenceClassification
>>> # Load tokenizer and PyTorch weights form the Hub
>>> tokenizer = AutoTokenizer.from_pretrained("distilbert-base-uncased")
>>> pt_model = AutoModelForSequenceClassification.from_pretrained("distilbert-base-uncased")
>>> # Save to disk
>>> tokenizer.save_pretrained("local-pt-checkpoint")
>>> pt_model.save_pretrained("local-pt-checkpoint")
```
Una volta salvato il checkpoint, possiamo esportarlo su ONNX puntando l'argomento `--model`
del pacchetto `transformers.onnx` nella directory desiderata:
```bash
python -m transformers.onnx --model=local-pt-checkpoint onnx/
```
</pt>
<tf>
```python
>>> from transformers import AutoTokenizer, TFAutoModelForSequenceClassification
>>> # Load tokenizer and TensorFlow weights from the Hub
>>> tokenizer = AutoTokenizer.from_pretrained("distilbert-base-uncased")
>>> tf_model = TFAutoModelForSequenceClassification.from_pretrained("distilbert-base-uncased")
>>> # Save to disk
>>> tokenizer.save_pretrained("local-tf-checkpoint")
>>> tf_model.save_pretrained("local-tf-checkpoint")
```
Once the checkpoint is saved, we can export it to ONNX by pointing the `--model`
argument of the `transformers.onnx` package to the desired directory:
```bash
python -m transformers.onnx --model=local-tf-checkpoint onnx/
```
</tf>
</frameworkcontent>
### Selezione delle caratteristiche per diverse topologie di modello
Ogni configurazione giร pronta viene fornita con una serie di _caratteristiche_ che ti consentono di
esportare modelli per diversi tipi di topologie o attivitร . Come mostrato nella tabella
di seguito, ogni caratteristica รจ associata a una diversa Auto Class:
| Caratteristica | Auto Class |
| ------------------------------------ | ------------------------------------ |
| `causal-lm`, `causal-lm-with-past` | `AutoModelForCausalLM` |
| `default`, `default-with-past` | `AutoModel` |
| `masked-lm` | `AutoModelForMaskedLM` |
| `question-answering` | `AutoModelForQuestionAnswering` |
| `seq2seq-lm`, `seq2seq-lm-with-past` | `AutoModelForSeq2SeqLM` |
| `sequence-classification` | `AutoModelForSequenceClassification` |
| `token-classification` | `AutoModelForTokenClassification` |
Per ciascuna configurazione, puoi trovare l'elenco delle funzionalitร supportate tramite il
`FeaturesManager`. Ad esempio, per DistilBERT abbiamo:
```python
>>> from transformers.onnx.features import FeaturesManager
>>> distilbert_features = list(FeaturesManager.get_supported_features_for_model_type("distilbert").keys())
>>> print(distilbert_features)
["default", "masked-lm", "causal-lm", "sequence-classification", "token-classification", "question-answering"]
```
Puoi quindi passare una di queste funzionalitร all'argomento `--feature` nel
pacchetto `transformers.onnx`. Ad esempio, per esportare un modello di classificazione del testo
possiamo scegliere un modello ottimizzato dall'Hub ed eseguire:
```bash
python -m transformers.onnx --model=distilbert-base-uncased-finetuned-sst-2-english \
--feature=sequence-classification onnx/
```
che visualizzerร i seguenti registri:
```bash
Validating ONNX model...
-[โ] ONNX model output names match reference model ({'logits'})
- Validating ONNX Model output "logits":
-[โ] (2, 2) matches (2, 2)
-[โ] all values close (atol: 1e-05)
All good, model saved at: onnx/model.onnx
```
Puoi notare che in questo caso, i nomi di output del modello ottimizzato sono
`logits` invece di `last_hidden_state` che abbiamo visto con il
checkpoint `distilbert-base-uncased` precedente. Questo รจ previsto dal
modello ottimizato visto che ha una testa di e.
<Tip>
Le caratteristiche che hanno un suffisso `wtih-past` (ad es. `causal-lm-with-past`)
corrispondono a topologie di modello con stati nascosti precalcolati (chiave e valori
nei blocchi di attenzione) che possono essere utilizzati per la decodifica autoregressiva veloce.
</Tip>
### Esportazione di un modello per un'architettura non supportata
Se desideri esportare un modello la cui architettura non รจ nativamente supportata dalla
libreria, ci sono tre passaggi principali da seguire:
1. Implementare una configurazione ONNX personalizzata.
2. Esportare il modello in ONNX.
3. Convalidare gli output di PyTorch e dei modelli esportati.
In questa sezione, vedremo come DistilBERT รจ stato implementato per mostrare cosa รจ
coinvolto in ogni passaggio.
#### Implementazione di una configurazione ONNX personalizzata
Iniziamo con l'oggetto di configurazione ONNX. Forniamo tre classi
astratte da cui ereditare, a seconda del tipo di archittettura
del modello che desideri esportare:
* I modelli basati su encoder ereditano da [`~onnx.config.OnnxConfig`]
* I modelli basati su decoder ereditano da [`~onnx.config.OnnxConfigWithPast`]
* I modelli encoder-decoder ereditano da[`~onnx.config.OnnxSeq2SeqConfigWithPast`]
<Tip>
Un buon modo per implementare una configurazione ONNX personalizzata รจ guardare l'implementazione
esistente nel file `configuration_<model_name>.py` di un'architettura simile.
</Tip>
Poichรฉ DistilBERT รจ un modello basato su encoder, la sua configurazione eredita da
`OnnxConfig`:
```python
>>> from typing import Mapping, OrderedDict
>>> from transformers.onnx import OnnxConfig
>>> class DistilBertOnnxConfig(OnnxConfig):
... @property
... def inputs(self) -> Mapping[str, Mapping[int, str]]:
... return OrderedDict(
... [
... ("input_ids", {0: "batch", 1: "sequence"}),
... ("attention_mask", {0: "batch", 1: "sequence"}),
... ]
... )
```
Ogni oggetto di configurazione deve implementare la proprietร `inputs` e restituire una
mappatura, dove ogni chiave corrisponde a un input previsto e ogni valore
indica l'asse di quell'input. Per DistilBERT, possiamo vedere che sono richiesti
due input: `input_ids` e `attention_mask`. Questi inputs hanno la stessa forma di
`(batch_size, sequence_length)` per questo motivo vediamo gli stessi assi usati nella
configurazione.
<Tip>
Puoi notare che la proprietร `inputs` per `DistilBertOnnxConfig` restituisce un
`OrdinatoDict`. Ciรฒ garantisce che gli input corrispondano alla loro posizione
relativa all'interno del metodo `PreTrainedModel.forward()` durante il tracciamento del grafico.
Raccomandiamo di usare un `OrderedDict` per le proprietร `inputs` e `outputs`
quando si implementano configurazioni ONNX personalizzate.
</Tip>
Dopo aver implementato una configurazione ONNX, รจ possibile istanziarla
fornendo alla configurazione del modello base come segue:
```python
>>> from transformers import AutoConfig
>>> config = AutoConfig.from_pretrained("distilbert-base-uncased")
>>> onnx_config = DistilBertOnnxConfig(config)
```
L'oggetto risultante ha diverse proprietร utili. Ad esempio รจ possibile visualizzare il
Set operatore ONNX che verrร utilizzato durante l'esportazione:
```python
>>> print(onnx_config.default_onnx_opset)
11
```
ร inoltre possibile visualizzare gli output associati al modello come segue:
```python
>>> print(onnx_config.outputs)
OrderedDict([("last_hidden_state", {0: "batch", 1: "sequence"})])
```
Puoi notare che la proprietร degli output segue la stessa struttura degli input; esso
restituisce un `OrderedDict` di output con nome e le loro forme. La struttura di output
รจ legato alla scelta della funzione con cui viene inizializzata la configurazione.
Per impostazione predefinita, la configurazione ONNX viene inizializzata con la funzione 'predefinita'
che corrisponde all'esportazione di un modello caricato con la classe `AutoModel`. Se tu
desideri esportare una topologia di modello diversa, รจ sufficiente fornire una funzionalitร diversa a
l'argomento `task` quando inizializzi la configurazione ONNX. Ad esempio, se
volevamo esportare DistilBERT con una testa di classificazione per sequenze, potremmo
usare:
```python
>>> from transformers import AutoConfig
>>> config = AutoConfig.from_pretrained("distilbert-base-uncased")
>>> onnx_config_for_seq_clf = DistilBertOnnxConfig(config, task="sequence-classification")
>>> print(onnx_config_for_seq_clf.outputs)
OrderedDict([('logits', {0: 'batch'})])
```
<Tip>
Tutte le proprietร e i metodi di base associati a [`~onnx.config.OnnxConfig`] e le
altre classi di configurazione possono essere sovrascritte se necessario. Guarda
[`BartOnnxConfig`] per un esempio avanzato.
</Tip>
#### Esportazione del modello
Una volta implementata la configurazione ONNX, il passaggio successivo consiste nell'esportare il
modello. Qui possiamo usare la funzione `export()` fornita dal
pacchetto `transformers.onnx`. Questa funzione prevede la configurazione ONNX, insieme
con il modello base e il tokenizer e il percorso per salvare il file esportato:
```python
>>> from pathlib import Path
>>> from transformers.onnx import export
>>> from transformers import AutoTokenizer, AutoModel
>>> onnx_path = Path("model.onnx")
>>> model_ckpt = "distilbert-base-uncased"
>>> base_model = AutoModel.from_pretrained(model_ckpt)
>>> tokenizer = AutoTokenizer.from_pretrained(model_ckpt)
>>> onnx_inputs, onnx_outputs = export(tokenizer, base_model, onnx_config, onnx_config.default_onnx_opset, onnx_path)
```
Gli `onnx_inputs` e `onnx_outputs` restituiti dalla funzione `export()` sono
liste di chiavi definite nelle proprietร di `input` e `output` della
configurazione. Una volta esportato il modello, puoi verificare che il modello sia ben
formato come segue:
```python
>>> import onnx
>>> onnx_model = onnx.load("model.onnx")
>>> onnx.checker.check_model(onnx_model)
```
<Tip>
Se il tuo modello รจ piรน largo di 2 GB, vedrai che molti file aggiuntivi sono
creati durante l'esportazione. Questo รจ _previsto_ perchรฉ ONNX utilizza [Protocol
Buffer](https://developers.google.com/protocol-buffers/) per memorizzare il modello e
questi hanno un limite di dimensione 2 GB. Vedi la [Documentazione
ONNX](https://github.com/onnx/onnx/blob/master/docs/ExternalData.md)
per istruzioni su come caricare modelli con dati esterni.
</Tip>
#### Convalida degli output del modello
Il passaggio finale consiste nel convalidare gli output dal modello di base e quello esportato
corrispondere entro una soglia di tolleranza assoluta. Qui possiamo usare la
Funzione `validate_model_outputs()` fornita dal pacchetto `transformers.onnx`
come segue:
```python
>>> from transformers.onnx import validate_model_outputs
>>> validate_model_outputs(
... onnx_config, tokenizer, base_model, onnx_path, onnx_outputs, onnx_config.atol_for_validation
... )
```
Questa funzione usa il metodo `OnnxConfig.generate_dummy_inputs()` per generare
input per il modello di base e quello esportato e la tolleranza assoluta puรฒ essere
definita nella configurazione. Generalmente troviamo una corrispondenza numerica nell'intervallo da 1e-6
a 1e-4, anche se รจ probabile che qualsiasi cosa inferiore a 1e-3 vada bene.
### Contribuire con una nuova configurazione a ๐ค Transformers
Stiamo cercando di espandere l'insieme di configurazioni giร pronte e di accettare
contributi della community! Se vuoi contribuire con la tua aggiunta
nella libreria, dovrai:
* Implementare la configurazione ONNX nella corrispondente `configuration file
_<model_name>.py`
* Includere l'architettura del modello e le funzioni corrispondenti in [`~onnx.features.FeatureManager`]
* Aggiungere la tua architettura del modello ai test in `test_onnx_v2.py`
Scopri come stato contribuito la configurazione per [IBERT]
(https://github.com/huggingface/transformers/pull/14868/files) per
avere un'idea di cosa รจ coinvolto.
## TorchScript
<Tip>
Questo รจ l'inizio dei nostri esperimenti con TorchScript e stiamo ancora esplorando le sue capacitร con
modelli con variable-input-size. ร una nostra prioritร e approfondiremo le nostre analisi nelle prossime versioni,
con piรน esempi di codici, un'implementazione piรน flessibile e benchmark che confrontano i codici basati su Python con quelli compilati con
TorchScript.
</Tip>
Secondo la documentazione di Pytorch: "TorchScript รจ un modo per creare modelli serializzabili e ottimizzabili da codice
Pytorch". I due moduli di Pytorch [JIT e TRACE](https://pytorch.org/docs/stable/jit.html) consentono allo sviluppatore di esportare
il loro modello da riutilizzare in altri programmi, come i programmi C++ orientati all'efficienza.
Abbiamo fornito un'interfaccia che consente l'esportazione di modelli ๐ค Transformers in TorchScript in modo che possano essere riutilizzati
in un ambiente diverso rispetto a un programma Python basato su Pytorch. Qui spieghiamo come esportare e utilizzare i nostri modelli utilizzando
TorchScript.
Esportare un modello richiede due cose:
- Un passaggio in avanti con input fittizzi.
- Istanziazione del modello con flag `torchscript`.
Queste necessitร implicano diverse cose a cui gli sviluppatori dovrebbero prestare attenzione. Questi dettagli mostrati sotto.
### Flag TorchScript e pesi legati
Questo flag รจ necessario perchรฉ la maggior parte dei modelli linguistici in questo repository hanno pesi legati tra il loro
strato "Embedding" e lo strato "Decoding". TorchScript non consente l'esportazione di modelli che hanno pesi
legati, quindi รจ necessario prima slegare e clonare i pesi.
Ciรฒ implica che i modelli istanziati con il flag `torchscript` hanno il loro strato `Embedding` e strato `Decoding`
separato, il che significa che non dovrebbero essere addestrati in futuro. L'allenamento de-sincronizza i due
strati, portando a risultati inaspettati.
Questo non รจ il caso per i modelli che non hanno una testa del modello linguistico, poichรฉ quelli non hanno pesi legati. Questi modelli
puรฒ essere esportato in sicurezza senza il flag `torchscript`.
### Input fittizi e standard lengths
Gli input fittizzi sono usati per fare un modello passaggio in avanti . Mentre i valori degli input si propagano attraverso i strati,
Pytorch tiene traccia delle diverse operazioni eseguite su ciascun tensore. Queste operazioni registrate vengono quindi utilizzate per
creare la "traccia" del modello.
La traccia viene creata relativamente alle dimensioni degli input. ร quindi vincolato dalle dimensioni dell'input
fittizio e non funzionerร per altre lunghezze di sequenza o dimensioni batch. Quando si proverร con una dimensione diversa, ci sarร errore
come:
`La dimensione espansa del tensore (3) deve corrispondere alla dimensione esistente (7) nella dimensione non singleton 2`
will be raised. Si consiglia pertanto di tracciare il modello con una dimensione di input fittizia grande almeno quanto il piรน grande
input che verrร fornito al modello durante l'inferenza. ร possibile eseguire il padding per riempire i valori mancanti. Il modello
sarร tracciato con una grande dimensione di input, tuttavia, anche le dimensioni della diverse matrici saranno grandi,
risultando in piรน calcoli.
Si raccomanda di prestare attenzione al numero totale di operazioni eseguite su ciascun input e di seguire da vicino le prestazioni
durante l'esportazione di modelli di sequenza-lunghezza variabili.
### Usare TorchSscript in Python
Di seguito รจ riportato un esempio, che mostra come salvare, caricare modelli e come utilizzare la traccia per l'inferenza.
#### Salvare un modello
Questo frammento di codice mostra come usare TorchScript per esportare un `BertModel`. Qui il `BertModel` รจ istanziato secondo
una classe `BertConfig` e quindi salvato su disco con il nome del file `traced_bert.pt`
```python
from transformers import BertModel, BertTokenizer, BertConfig
import torch
enc = BertTokenizer.from_pretrained("bert-base-uncased")
# Tokenizing input text
text = "[CLS] Who was Jim Henson ? [SEP] Jim Henson was a puppeteer [SEP]"
tokenized_text = enc.tokenize(text)
# Masking one of the input tokens
masked_index = 8
tokenized_text[masked_index] = "[MASK]"
indexed_tokens = enc.convert_tokens_to_ids(tokenized_text)
segments_ids = [0, 0, 0, 0, 0, 0, 0, 1, 1, 1, 1, 1, 1, 1]
# Creating a dummy input
tokens_tensor = torch.tensor([indexed_tokens])
segments_tensors = torch.tensor([segments_ids])
dummy_input = [tokens_tensor, segments_tensors]
# Initializing the model with the torchscript flag
# Flag set to True even though it is not necessary as this model does not have an LM Head.
config = BertConfig(
vocab_size_or_config_json_file=32000,
hidden_size=768,
num_hidden_layers=12,
num_attention_heads=12,
intermediate_size=3072,
torchscript=True,
)
# Instantiating the model
model = BertModel(config)
# The model needs to be in evaluation mode
model.eval()
# If you are instantiating the model with *from_pretrained* you can also easily set the TorchScript flag
model = BertModel.from_pretrained("bert-base-uncased", torchscript=True)
# Creating the trace
traced_model = torch.jit.trace(model, [tokens_tensor, segments_tensors])
torch.jit.save(traced_model, "traced_bert.pt")
```
#### Caricare un modello
Questo frammento di codice mostra come caricare il `BertModel` che era stato precedentemente salvato su disco con il nome `traced_bert.pt`.
Stiamo riutilizzando il `dummy_input` precedentemente inizializzato.
```python
loaded_model = torch.jit.load("traced_bert.pt")
loaded_model.eval()
all_encoder_layers, pooled_output = loaded_model(*dummy_input)
```
#### Utilizzare un modello tracciato per l'inferenza
Usare il modello tracciato per l'inferenza รจ semplice come usare il suo metodo dunder `__call__`:
```python
traced_model(tokens_tensor, segments_tensors)
```
###Implementare modelli HuggingFace TorchScript su AWS utilizzando Neuron SDK
AWS ha introdotto [Amazon EC2 Inf1](https://aws.amazon.com/ec2/instance-types/inf1/)
famiglia di istanze per l'inferenza di machine learning a basso costo e ad alte prestazioni nel cloud.
Le istanze Inf1 sono alimentate dal chip AWS Inferentia, un acceleratore hardware personalizzato,
specializzato in carichi di lavoro di inferenza di deep learning.
[AWS Neuron](https://awsdocs-neuron.readthedocs-hosted.com/en/latest/#)
รจ l'SDK per Inferentia che supporta il tracciamento e l'ottimizzazione dei modelli transformers per
distribuzione su Inf1. L'SDK Neuron fornisce:
1. API di facile utilizzo con una riga di modifica del codice per tracciare e ottimizzare un modello TorchScript per l'inferenza nel cloud.
2. Ottimizzazioni delle prestazioni pronte all'uso per [miglioramento dei costi-prestazioni](https://awsdocs-neuron.readthedocs-hosted.com/en/latest/neuron-guide/benchmark/>)
3. Supporto per i modelli di trasformatori HuggingFace costruiti con [PyTorch](https://awsdocs-neuron.readthedocs-hosted.com/en/latest/src/examples/pytorch/bert_tutorial/tutorial_pretrained_bert.html)
o [TensorFlow](https://awsdocs-neuron.readthedocs-hosted.com/en/latest/src/examples/tensorflow/huggingface_bert/huggingface_bert.html).
#### Implicazioni
Modelli Transformers basati su architettura [BERT (Bidirectional Encoder Representations from Transformers)](https://huggingface.co/docs/transformers/main/model_doc/bert),
o sue varianti come [distilBERT](https://huggingface.co/docs/transformers/main/model_doc/distilbert)
e [roBERTa](https://huggingface.co/docs/transformers/main/model_doc/roberta)
funzioneranno meglio su Inf1 per attivitร non generative come la question answering estrattive,
Classificazione della sequenza, Classificazione dei token. In alternativa, generazione di testo
le attivitร possono essere adattate per essere eseguite su Inf1, secondo questo [tutorial AWS Neuron MarianMT](https://awsdocs-neuron.readthedocs-hosted.com/en/latest/src/examples/pytorch/transformers-marianmt.html).
Ulteriori informazioni sui modelli che possono essere convertiti fuori dagli schemi su Inferentia possono essere
trovati nella [sezione Model Architecture Fit della documentazione Neuron](https://awsdocs-neuron.readthedocs-hosted.com/en/latest/neuron-guide/models/models-inferentia.html#models-inferentia).
#### Dipendenze
L'utilizzo di AWS Neuron per convertire i modelli richiede le seguenti dipendenze e l'ambiente:
* A [Neuron SDK environment](https://awsdocs-neuron.readthedocs-hosted.com/en/latest/neuron-guide/neuron-frameworks/pytorch-neuron/index.html#installation-guide),
which comes pre-configured on [AWS Deep Learning AMI](https://docs.aws.amazon.com/dlami/latest/devguide/tutorial-inferentia-launching.html).
#### Convertire un modello per AWS Neuron
Usando lo stesso script come in [Usando TorchScipt in Python](https://huggingface.co/docs/transformers/main/en/serialization#using-torchscript-in-python)
per tracciare un "BertModel", importi l'estensione del framework `torch.neuron` per accedere
i componenti di Neuron SDK tramite un'API Python.
```python
from transformers import BertModel, BertTokenizer, BertConfig
import torch
import torch.neuron
```
E modificare solo la riga di codice di traccia
Da:
```python
torch.jit.trace(model, [tokens_tensor, segments_tensors])
```
A:
```python
torch.neuron.trace(model, [token_tensor, segments_tensors])
```
Questa modifica consente a Neuron SDK di tracciare il modello e ottimizzarlo per l'esecuzione nelle istanze Inf1.
Per ulteriori informazioni sulle funzionalitร , gli strumenti, i tutorial di esempi e gli ultimi aggiornamenti di AWS Neuron SDK,
consultare la [documentazione AWS NeuronSDK](https://awsdocs-neuron.readthedocs-hosted.com/en/latest/index.html). | 0 |
hf_public_repos/transformers/docs/source | hf_public_repos/transformers/docs/source/it/big_models.md | <!--Copyright 2022 The HuggingFace Team. All rights reserved.
Licensed under the Apache License, Version 2.0 (the "License"); you may not use this file except in compliance with
the License. You may obtain a copy of the License at
http://www.apache.org/licenses/LICENSE-2.0
Unless required by applicable law or agreed to in writing, software distributed under the License is distributed on
an "AS IS" BASIS, WITHOUT WARRANTIES OR CONDITIONS OF ANY KIND, either express or implied. See the License for the
specific language governing permissions and limitations under the License.
โ ๏ธ Note that this file is in Markdown but contain specific syntax for our doc-builder (similar to MDX) that may not be
rendered properly in your Markdown viewer.
-->
# Istanziare un big model
Quando vuoi utilizzare un modello preaddestrato (pretrained) molto grande, una sfida รจ minimizzare l'uso della RAM. Il workflow classico
in PyTorch รจ:
1. Crea il tuo modello con pesi casuali (random weights).
2. Carica i tuoi pesi preaddestrati.
3. Inserisci i pesi preaddestrati nel tuo modello casuale.
I passi 1 e 2 una versione completa del modello in memoria, in molti casi non รจ un problema, ma se il modello inizia a pesare diversi GigaBytes, queste due copie possono sturare la nostra RAM. Ancora peggio, se stai usando `torch.distributed` per seguire l'addestramento (training) in distribuito, ogni processo caricherร il modello preaddestrato e memorizzerร queste due copie nella RAM.
<Tip>
Nota che il modello creato casualmente รจ inizializzato con tensori "vuoti", che occupano spazio in memoria ma senza riempirlo (quindi i valori casuali sono quelli che si trovavano in questa porzione di memoria in un determinato momento). L'inizializzazione casuale che segue la distribuzione appropriata per il tipo di modello/parametri istanziato (come la distribuzione normale per le istanze) รจ eseguito solo dopo il passaggio 3 sui pesi non inizializzati, per essere piรน rapido possibile!
</Tip>
In questa guida, esploreremo le soluzioni che Transformers offre per affrontare questo problema. C'รจ da tenere in conto che questa รจ un'area in cui si sta attualmente sviluppando, quindi le API spiegate qui possono variare velocemente in futuro.
## Checkpoints condivisi
Dalla versione 4.18.0, i checkpoints dei modelli che occupano piรน di 10GB di spazio vengono automaticamente frammentati in piรน parti. Per quanto riguarda la possibilitร di avere un unico checkpoint quando si utilizza `model.save_pretrained(save_dir)`, si hanno diversi checkpoint parziali (ognuno con dimensione < 10GB) e un indice che mappa i nomi dei parametri ai file in cui sono memorizzati.
Puoi controllare la dimensione massima dopo la frammentazione con il parametro `max_shard_size`, nel prossimo esempio, useremo modelli di dimensioni normali con frammenti di piccoli dimensioni: prendiamo un modello BERT classico.
```py
from transformers import AutoModel
model = AutoModel.from_pretrained("bert-base-cased")
```
Se tu salvi usando [`~PreTrainedModel.save_pretrained`], avrai una nuova cartella con due file: il config del modello e i suoi pesi:
```py
>>> import os
>>> import tempfile
>>> with tempfile.TemporaryDirectory() as tmp_dir:
... model.save_pretrained(tmp_dir)
... print(sorted(os.listdir(tmp_dir)))
['config.json', 'pytorch_model.bin']
```
Adesso usiamo una dimensione massima di frammentazione di 200MB:
```py
>>> with tempfile.TemporaryDirectory() as tmp_dir:
... model.save_pretrained(tmp_dir, max_shard_size="200MB")
... print(sorted(os.listdir(tmp_dir)))
['config.json', 'pytorch_model-00001-of-00003.bin', 'pytorch_model-00002-of-00003.bin', 'pytorch_model-00003-of-00003.bin', 'pytorch_model.bin.index.json']
```
In aggiunta alla configurazione del modello, vediamo tre differenti file dei pesi, e un file `index.json` che รจ il nostro indice. Un checkpoint puรฒ essere ricaricato totalmente usando il metodo [`~PreTrainedModel.from_pretrained`]:
```py
>>> with tempfile.TemporaryDirectory() as tmp_dir:
... model.save_pretrained(tmp_dir, max_shard_size="200MB")
... new_model = AutoModel.from_pretrained(tmp_dir)
```
Il vantaggio principale di applicare questo metodo per modelli grandi รจ che durante il passo 2 del workflow illustrato in precedenza, ogni frammento del checkpoint viene caricato dopo il precedente, limitando l'utilizzo della RAM alla dimensione del modello piรน la dimensione del frammento piรน grande.
Dietro le quinte, il file indice รจ utilizzato per determinare quali chiavi sono nel checkpoint, e dove i corrispondenti pesi sono memorizzati. Possiamo caricare l'indice come un qualsiasi json e ottenere un dizionario:
```py
>>> import json
>>> with tempfile.TemporaryDirectory() as tmp_dir:
... model.save_pretrained(tmp_dir, max_shard_size="200MB")
... with open(os.path.join(tmp_dir, "pytorch_model.bin.index.json"), "r") as f:
... index = json.load(f)
>>> print(index.keys())
dict_keys(['metadata', 'weight_map'])
```
I metadati consistono solo nella dimensione totale del modello per ora. Abbiamo in programma di aggiungere altre informazioni in futuro:
```py
>>> index["metadata"]
{'total_size': 433245184}
```
La mappa dei pesi รจ la parte principale di questo indice, che mappa ogni nome dei parametri (si trova solitamente nei modelli PyTorch come `state_dict`) al file in cui รจ memorizzato:
```py
>>> index["weight_map"]
{'embeddings.LayerNorm.bias': 'pytorch_model-00001-of-00003.bin',
'embeddings.LayerNorm.weight': 'pytorch_model-00001-of-00003.bin',
...
```
Se vuoi caricare direttamente un checkpoint frammentato in un modello senza usare [`~PreTrainedModel.from_pretrained`] (come si farebbe con `model.load_state_dict()` per un checkpoint completo) devi usare [`~modeling_utils.load_sharded_checkpoint`]:
```py
>>> from transformers.modeling_utils import load_sharded_checkpoint
>>> with tempfile.TemporaryDirectory() as tmp_dir:
... model.save_pretrained(tmp_dir, max_shard_size="200MB")
... load_sharded_checkpoint(model, tmp_dir)
```
## Caricamento low memory
Frammentare i checkpoint l'utilizzo di memoria al passo 2 del workflow citato in precedenza, ma per utilizzare questo modello in un ambiente con poca memoria, consigliamo di utilizzare i nostri strumenti basati sulla libreria Accelerate.
Per ulteriori informazioni, leggere la seguente guida: [Large model loading using Accelerate](./main_classes/model#large-model-loading) | 0 |
hf_public_repos/transformers/docs/source | hf_public_repos/transformers/docs/source/it/perf_train_cpu.md | <!--Copyright 2022 The HuggingFace Team. All rights reserved.
Licensed under the Apache License, Version 2.0 (the "License"); you may not use this file except in compliance with
the License. You may obtain a copy of the License at
http://www.apache.org/licenses/LICENSE-2.0
Unless required by applicable law or agreed to in writing, software distributed under the License is distributed on
an "AS IS" BASIS, WITHOUT WARRANTIES OR CONDITIONS OF ANY KIND, either express or implied. See the License for the
โ ๏ธ Note that this file is in Markdown but contain specific syntax for our doc-builder (similar to MDX) that may not be
rendered properly in your Markdown viewer.
-->
# Addestramento efficiente su CPU
Questa guida si concentra su come addestrare in maniera efficiente grandi modelli su CPU.
## Mixed precision con IPEX
IPEX รจ ottimizzato per CPU con AVX-512 o superiore, e funziona per le CPU con solo AVX2. Pertanto, si prevede che le prestazioni saranno piรน vantaggiose per le le CPU Intel con AVX-512 o superiori, mentre le CPU con solo AVX2 (ad esempio, le CPU AMD o le CPU Intel piรน vecchie) potrebbero ottenere prestazioni migliori con IPEX, ma non sono garantite. IPEX offre ottimizzazioni delle prestazioni per l'addestramento della CPU sia con Float32 che con BFloat16. L'uso di BFloat16 รจ l'argomento principale delle seguenti sezioni.
Il tipo di dati a bassa precisione BFloat16 รจ stato supportato in modo nativo su 3rd Generation Xeonยฎ Scalable Processors (aka Cooper Lake) con AVX512 e sarร supportata dalla prossima generazione di Intelยฎ Xeonยฎ Scalable Processors con Intelยฎ Advanced Matrix Extensions (Intelยฎ AMX) instruction set con prestazioni ulteriormente migliorate. L'Auto Mixed Precision per il backende della CPU รจ stato abilitato da PyTorch-1.10. allo stesso tempo, il supporto di Auto Mixed Precision con BFloat16 per CPU e l'ottimizzazione degli operatori BFloat16 รจ stata abilitata in modo massiccio in Intelยฎ Extension per PyTorch, and parzialmente aggiornato al branch master di PyTorch. Gli utenti possono ottenere prestazioni migliori ed users experience con IPEX Auto Mixed Precision..
Vedi informazioni piรน dettagliate su [Auto Mixed Precision](https://intel.github.io/intel-extension-for-pytorch/cpu/latest/tutorials/features/amp.html).
### Installazione di IPEX:
Il rilascio di IPEX segue quello di PyTorch, da installare via pip:
| PyTorch Version | IPEX version |
| :---------------: | :----------: |
| 1.13 | 1.13.0+cpu |
| 1.12 | 1.12.300+cpu |
| 1.11 | 1.11.200+cpu |
| 1.10 | 1.10.100+cpu |
```bash
pip install intel_extension_for_pytorch==<version_name> -f https://developer.intel.com/ipex-whl-stable-cpu
```
Vedi altri approcci per [IPEX installation](https://intel.github.io/intel-extension-for-pytorch/cpu/latest/tutorials/installation.html).
### Utilizzo nel Trainer
Per abilitare la auto mixed precision con IPEX in Trainer, l'utende dovrebbe aggiungere `use_ipex`, `bf16` e `no_cuda` negli argomenti del comando di addestramento.
Vedi un sempio di un caso d'uso [Transformers question-answering](https://github.com/huggingface/transformers/tree/main/examples/pytorch/question-answering)
- Training with IPEX using BF16 auto mixed precision on CPU:
<pre> python run_qa.py \
--model_name_or_path bert-base-uncased \
--dataset_name squad \
--do_train \
--do_eval \
--per_device_train_batch_size 12 \
--learning_rate 3e-5 \
--num_train_epochs 2 \
--max_seq_length 384 \
--doc_stride 128 \
--output_dir /tmp/debug_squad/ \
<b>--use_ipex \</b>
<b>--bf16 --no_cuda</b></pre>
### Esempi pratici
Blog: [Accelerating PyTorch Transformers with Intel Sapphire Rapids](https://huggingface.co/blog/intel-sapphire-rapids)
| 0 |
hf_public_repos/transformers/docs/source | hf_public_repos/transformers/docs/source/it/community.md | <!--โ ๏ธ Note that this file is in Markdown but contain specific syntax for our doc-builder (similar to MDX) that may not be
rendered properly in your Markdown viewer.
-->
# Comunitร
Questa pagina raggruppa le risorse sviluppate dalla comunitร riguardo ๐ค Transformers.
## Risorse della comunitร :
| Risorsa | Descrizione | Autore |
|:----------|:-------------|------:|
| [Glossario delle Flashcards di Transformers](https://www.darigovresearch.com/huggingface-transformers-glossary-flashcards) | Un insieme di flashcards basate sul [glossario della documentazione di Transformers](glossary), creato in un formato tale da permettere un facile apprendimento e revisione usando [Anki](https://apps.ankiweb.net/), un'applicazione open-source e multi-piattaforma, specificatamente progettata per ricordare informazioni nel lungo termine. Guarda questo [video introduttivo su come usare le flashcards](https://www.youtube.com/watch?v=Dji_h7PILrw). | [Darigov Research](https://www.darigovresearch.com/) |
## Notebook della comunitร :
| Notebook | Descrizione | Autore | |
|:----------|:-------------|:-------------|------:|
| [Fine-tuning di un Transformer pre-addestrato, al fine di generare testi di canzoni](https://github.com/AlekseyKorshuk/huggingartists) | Come generare testi di canzoni nello stile del vostro artista preferito attraverso il fine-tuning di un modello GPT-2. | [Aleksey Korshuk](https://github.com/AlekseyKorshuk) | [](https://colab.research.google.com/github/AlekseyKorshuk/huggingartists/blob/master/huggingartists-demo.ipynb) |
| [Addestramento di T5 in Tensorflow 2 ](https://github.com/snapthat/TF-T5-text-to-text) | Come addestrare T5 per qualsiasi attivitร usando Tensorflow 2. Questo notebook mostra come risolvere l'attivitร di "Question Answering" usando Tensorflow 2 e SQUAD. | [Muhammad Harris](https://github.com/HarrisDePerceptron) |[](https://colab.research.google.com/github/snapthat/TF-T5-text-to-text/blob/master/snapthatT5/notebooks/TF-T5-Datasets%20Training.ipynb) |
| [Addestramento di T5 con TPU](https://github.com/patil-suraj/exploring-T5/blob/master/T5_on_TPU.ipynb) | Come addestrare T5 su SQUAD con Transformers e NLP. | [Suraj Patil](https://github.com/patil-suraj) |[](https://colab.research.google.com/github/patil-suraj/exploring-T5/blob/master/T5_on_TPU.ipynb#scrollTo=QLGiFCDqvuil) |
| [Fine-tuning di T5 per la classificazione e scelta multipla](https://github.com/patil-suraj/exploring-T5/blob/master/t5_fine_tuning.ipynb) | Come effettuare il fine-tuning di T5 per le attivitร di classificazione a scelta multipla - usando un formato testo-a-testo - con PyTorch Lightning. | [Suraj Patil](https://github.com/patil-suraj) | [](https://colab.research.google.com/github/patil-suraj/exploring-T5/blob/master/t5_fine_tuning.ipynb) |
| [Fine-tuning di DialoGPT su nuovi dataset e lingue](https://github.com/ncoop57/i-am-a-nerd/blob/master/_notebooks/2020-05-12-chatbot-part-1.ipynb) | Come effettuare il fine-tuning di un modello DialoGPT su un nuovo dataset per chatbots conversazionali open-dialog. | [Nathan Cooper](https://github.com/ncoop57) | [](https://colab.research.google.com/github/ncoop57/i-am-a-nerd/blob/master/_notebooks/2020-05-12-chatbot-part-1.ipynb) |
| [Modellamento di una lunga sequenza con Reformer](https://github.com/patrickvonplaten/notebooks/blob/master/PyTorch_Reformer.ipynb) | Come addestrare su sequenze di lunghezza fino a 500 mila token con Reformer. | [Patrick von Platen](https://github.com/patrickvonplaten) | [](https://colab.research.google.com/github/patrickvonplaten/notebooks/blob/master/PyTorch_Reformer.ipynb) |
| [Fine-tuning di BART per riassumere testi](https://github.com/ohmeow/ohmeow_website/blob/master/_notebooks/2020-05-23-text-generation-with-blurr.ipynb) | Come effettuare il fine-tuning di BART per riassumere testi con fastai usando blurr. | [Wayde Gilliam](https://ohmeow.com/) | [](https://colab.research.google.com/github/ohmeow/ohmeow_website/blob/master/_notebooks/2020-05-23-text-generation-with-blurr.ipynb) |
| [Fine-tuning di un Transformer pre-addestrato su tweet](https://colab.research.google.com/github/borisdayma/huggingtweets/blob/master/huggingtweets-demo.ipynb) | Come generare tweet nello stile del tuo account Twitter preferito attraverso il fine-tuning di un modello GPT-2. | [Boris Dayma](https://github.com/borisdayma) | [](https://colab.research.google.com/github/borisdayma/huggingtweets/blob/master/huggingtweets-demo.ipynb) |
| [Ottimizzazione di modelli ๐ค Hugging Face con Weights & Biases](https://colab.research.google.com/github/wandb/examples/blob/master/colabs/huggingface/Optimize_Hugging_Face_models_with_Weights_%26_Biases.ipynb) | Un tutorial completo che mostra l'integrazione di W&B con Hugging Face. | [Boris Dayma](https://github.com/borisdayma) | [](https://colab.research.google.com/github/wandb/examples/blob/master/colabs/huggingface/Optimize_Hugging_Face_models_with_Weights_%26_Biases.ipynb) |
| [Longformer pre-addestrato](https://github.com/allenai/longformer/blob/master/scripts/convert_model_to_long.ipynb) | Come costruire una versione "long" degli esistenti modelli pre-addestrati. | [Iz Beltagy](https://beltagy.net) | [](https://colab.research.google.com/github/allenai/longformer/blob/master/scripts/convert_model_to_long.ipynb) |
| [Fine-tuning di Longformer per QA](https://github.com/patil-suraj/Notebooks/blob/master/longformer_qa_training.ipynb) | Come effettuare il fine-tuning di un modello longformer per un task di QA.| [Suraj Patil](https://github.com/patil-suraj) | [](https://colab.research.google.com/github/patil-suraj/Notebooks/blob/master/longformer_qa_training.ipynb) |
| [Valutazione di modelli con ๐คNLP](https://github.com/patrickvonplaten/notebooks/blob/master/How_to_evaluate_Longformer_on_TriviaQA_using_NLP.ipynb) | Come valutare longformer su TriviaQA con `NLP`. | [Patrick von Platen](https://github.com/patrickvonplaten) | [](https://colab.research.google.com/drive/1m7eTGlPmLRgoPkkA7rkhQdZ9ydpmsdLE?usp=sharing) |
| [Fine-tuning di T5 per Sentiment Span Extraction](https://github.com/enzoampil/t5-intro/blob/master/t5_qa_training_pytorch_span_extraction.ipynb) | Come effettuare il fine-tuning di T5 per la sentiment span extraction - usando un formato testo-a-testo - con PyTorch Lightning. | [Lorenzo Ampil](https://github.com/enzoampil) | [](https://colab.research.google.com/github/enzoampil/t5-intro/blob/master/t5_qa_training_pytorch_span_extraction.ipynb) |
| [Fine-tuning di DistilBert per la classificazione multi-classe](https://github.com/abhimishra91/transformers-tutorials/blob/master/transformers_multiclass_classification.ipynb) | Come effettuare il fine-tuning di DistilBert per la classificazione multi-classe con PyTorch. | [Abhishek Kumar Mishra](https://github.com/abhimishra91) | [](https://colab.research.google.com/github/abhimishra91/transformers-tutorials/blob/master/transformers_multiclass_classification.ipynb)|
|[Fine-tuning di BERT per la classificazione multi-etichetta](https://github.com/abhimishra91/transformers-tutorials/blob/master/transformers_multi_label_classification.ipynb)|Come effettuare il fine-tuning di BERT per la classificazione multi-etichetta con PyTorch. |[Abhishek Kumar Mishra](https://github.com/abhimishra91) |[](https://colab.research.google.com/github/abhimishra91/transformers-tutorials/blob/master/transformers_multi_label_classification.ipynb)|
|[Accelerazione del fine-tuning con il Dynamic Padding / Bucketing](https://github.com/ELS-RD/transformers-notebook/blob/master/Divide_Hugging_Face_Transformers_training_time_by_2_or_more.ipynb)| Come velocizzare il fine-tuning di un fattore 2X usando il dynamic padding / bucketing. |[Michael Benesty](https://github.com/pommedeterresautee) |[](https://colab.research.google.com/drive/1CBfRU1zbfu7-ijiOqAAQUA-RJaxfcJoO?usp=sharing)|
|[Pre-addestramento di Reformer per Masked Language Modeling](https://github.com/patrickvonplaten/notebooks/blob/master/Reformer_For_Masked_LM.ipynb)| Come addestrare un modello Reformer usando livelli di self-attention bi-direzionali.| [Patrick von Platen](https://github.com/patrickvonplaten) | [](https://colab.research.google.com/drive/1tzzh0i8PgDQGV3SMFUGxM7_gGae3K-uW?usp=sharing)|
|[Espansione e fine-tuning di Sci-BERT](https://github.com/lordtt13/word-embeddings/blob/master/COVID-19%20Research%20Data/COVID-SciBERT.ipynb)| Come incrementare il vocabolario di un modello SciBERT - pre-addestrato da AllenAI sul dataset CORD - e crearne una pipeline. | [Tanmay Thakur](https://github.com/lordtt13) | [](https://colab.research.google.com/drive/1rqAR40goxbAfez1xvF3hBJphSCsvXmh8)|
|[Fine-tuning di BlenderBotSmall per riassumere testi usando Trainer API](https://github.com/lordtt13/transformers-experiments/blob/master/Custom%20Tasks/fine-tune-blenderbot_small-for-summarization.ipynb)| Come effettuare il fine-tuning di BlenderBotSmall per riassumere testi su un dataset personalizzato, usando Trainer API. | [Tanmay Thakur](https://github.com/lordtt13) | [](https://colab.research.google.com/drive/19Wmupuls7mykSGyRN_Qo6lPQhgp56ymq?usp=sharing)|
|[Fine-tuning di Electra e interpretazione con Integrated Gradients](https://github.com/elsanns/xai-nlp-notebooks/blob/master/electra_fine_tune_interpret_captum_ig.ipynb) | Come effettuare il fine-tuning di Electra per l'analisi dei sentimenti e intepretare le predizioni con Captum Integrated Gradients. | [Eliza Szczechla](https://elsanns.github.io) | [](https://colab.research.google.com/github/elsanns/xai-nlp-notebooks/blob/master/electra_fine_tune_interpret_captum_ig.ipynb)|
|[Fine-tuning di un modello GPT-2 non inglese con la classe Trainer](https://github.com/philschmid/fine-tune-GPT-2/blob/master/Fine_tune_a_non_English_GPT_2_Model_with_Huggingface.ipynb) | Come effettuare il fine-tuning di un modello GPT-2 non inglese con la classe Trainer. | [Philipp Schmid](https://www.philschmid.de) | [](https://colab.research.google.com/github/philschmid/fine-tune-GPT-2/blob/master/Fine_tune_a_non_English_GPT_2_Model_with_Huggingface.ipynb)|
|[Fine-tuning di un modello DistilBERT per la classficazione multi-etichetta](https://github.com/DhavalTaunk08/Transformers_scripts/blob/master/Transformers_multilabel_distilbert.ipynb) | Come effettuare il fine-tuning di un modello DistilBERT per l'attivitร di classificazione multi-etichetta. | [Dhaval Taunk](https://github.com/DhavalTaunk08) | [](https://colab.research.google.com/github/DhavalTaunk08/Transformers_scripts/blob/master/Transformers_multilabel_distilbert.ipynb)|
|[Fine-tuning di ALBERT per la classifcazione di coppie di frasi](https://github.com/NadirEM/nlp-notebooks/blob/master/Fine_tune_ALBERT_sentence_pair_classification.ipynb) | Come effettuare il fine-tuning di un modello ALBERT - o un altro modello BERT-based - per l'attivitร di classificazione di coppie di frasi. | [Nadir El Manouzi](https://github.com/NadirEM) | [](https://colab.research.google.com/github/NadirEM/nlp-notebooks/blob/master/Fine_tune_ALBERT_sentence_pair_classification.ipynb)|
|[Fine-tuning di Roberta per l'analisi di sentimenti](https://github.com/DhavalTaunk08/NLP_scripts/blob/master/sentiment_analysis_using_roberta.ipynb) | Come effettuare il fine-tuning di un modello Roberta per l'analisi di sentimenti. | [Dhaval Taunk](https://github.com/DhavalTaunk08) | [](https://colab.research.google.com/github/DhavalTaunk08/NLP_scripts/blob/master/sentiment_analysis_using_roberta.ipynb)|
|[Valutazione di modelli che generano domande](https://github.com/flexudy-pipe/qugeev) | Quanto sono accurante le risposte alle domande generate dal tuo modello transformer seq2seq? | [Pascal Zoleko](https://github.com/zolekode) | [](https://colab.research.google.com/drive/1bpsSqCQU-iw_5nNoRm_crPq6FRuJthq_?usp=sharing)|
|[Classificazione di testo con DistilBERT e Tensorflow](https://github.com/peterbayerle/huggingface_notebook/blob/main/distilbert_tf.ipynb) | Come effettuare il fine-tuning di DistilBERT per la classificazione di testo in TensorFlow. | [Peter Bayerle](https://github.com/peterbayerle) | [](https://colab.research.google.com/github/peterbayerle/huggingface_notebook/blob/main/distilbert_tf.ipynb)|
|[Utilizzo di BERT per riassumere testi con un modello Encoder-Decoder su CNN/Dailymail](https://github.com/patrickvonplaten/notebooks/blob/master/BERT2BERT_for_CNN_Dailymail.ipynb) | Come avviare "a caldo" un *EncoderDecoderModel* attraverso l'utilizzo di un checkpoint *bert-base-uncased* per riassumere testi su CNN/Dailymail. | [Patrick von Platen](https://github.com/patrickvonplaten) | [](https://colab.research.google.com/github/patrickvonplaten/notebooks/blob/master/BERT2BERT_for_CNN_Dailymail.ipynb)|
|[Utilizzo di RoBERTa per riassumere testi con un modello Encoder-Decoder su BBC XSum](https://github.com/patrickvonplaten/notebooks/blob/master/RoBERTaShared_for_BBC_XSum.ipynb) | Come avviare "a caldo" un *EncoderDecoderModel* (condiviso) attraverso l'utilizzo di un checkpoint *roberta-base* per riassumere testi su BBC/XSum. | [Patrick von Platen](https://github.com/patrickvonplaten) | [](https://colab.research.google.com/github/patrickvonplaten/notebooks/blob/master/RoBERTaShared_for_BBC_XSum.ipynb)|
|[Fine-tuning di TAPAS su Sequential Question Answering (SQA)](https://github.com/NielsRogge/Transformers-Tutorials/blob/master/TAPAS/Fine_tuning_TapasForQuestionAnswering_on_SQA.ipynb) | Come effettuare il fine-tuning di un modello *TapasForQuestionAnswering* attraverso l'utilizzo di un checkpoint *tapas-base* sul dataset Sequential Question Answering (SQA). | [Niels Rogge](https://github.com/nielsrogge) | [](https://colab.research.google.com/github/NielsRogge/Transformers-Tutorials/blob/master/TAPAS/Fine_tuning_TapasForQuestionAnswering_on_SQA.ipynb)|
|[Valutazione di TAPAS su Table Fact Checking (TabFact)](https://github.com/NielsRogge/Transformers-Tutorials/blob/master/TAPAS/Evaluating_TAPAS_on_the_Tabfact_test_set.ipynb) | Come valutare un modello *TapasForSequenceClassification* - fine-tuned con un checkpoint *tapas-base-finetuned-tabfact* - usando una combinazione delle librerie ๐ค datasets e ๐ค transformers. | [Niels Rogge](https://github.com/nielsrogge) | [](https://colab.research.google.com/github/NielsRogge/Transformers-Tutorials/blob/master/TAPAS/Evaluating_TAPAS_on_the_Tabfact_test_set.ipynb)|
|[Fine-tuning di mBART per la traduzione](https://colab.research.google.com/github/vasudevgupta7/huggingface-tutorials/blob/main/translation_training.ipynb) | Come effettuare il fine-tuning di mBART usando Seq2SeqTrainer per la traduzione da hindi a inglese.| [Vasudev Gupta](https://github.com/vasudevgupta7) | [](https://colab.research.google.com/github/vasudevgupta7/huggingface-tutorials/blob/main/translation_training.ipynb)|
|[Fine-tuning di LayoutLM su FUNSD (un dataset per la comprensione della forma)](https://github.com/NielsRogge/Transformers-Tutorials/blob/master/LayoutLM/Fine_tuning_LayoutLMForTokenClassification_on_FUNSD.ipynb) | Come effettuare il fine-tuning di un modello *LayoutLMForTokenClassification* sul dataset FUNSD per l'estrazione di informazioni da documenti scannerizzati.| [Niels Rogge](https://github.com/nielsrogge) | [](https://colab.research.google.com/github/NielsRogge/Transformers-Tutorials/blob/master/LayoutLM/Fine_tuning_LayoutLMForTokenClassification_on_FUNSD.ipynb)|
|[Fine-tuning di DistilGPT2 e generazione di testo](https://colab.research.google.com/github/tripathiaakash/DistilGPT2-Tutorial/blob/main/distilgpt2_fine_tuning.ipynb) | Come effettuare il fine-tuning di DistilGPT2 e generare testo. | [Aakash Tripathi](https://github.com/tripathiaakash) | [](https://colab.research.google.com/github/tripathiaakash/DistilGPT2-Tutorial/blob/main/distilgpt2_fine_tuning.ipynb)|
|[Fine-tuning di LED fino a 8 mila token](https://github.com/patrickvonplaten/notebooks/blob/master/Fine_tune_Longformer_Encoder_Decoder_(LED)_for_Summarization_on_pubmed.ipynb) | Come effettuare il fine-tuning di LED su PubMed per riassumere "lunghi" testi. | [Patrick von Platen](https://github.com/patrickvonplaten) | [](https://colab.research.google.com/github/patrickvonplaten/notebooks/blob/master/Fine_tune_Longformer_Encoder_Decoder_(LED)_for_Summarization_on_pubmed.ipynb)|
|[Valutazione di LED su Arxiv](https://github.com/patrickvonplaten/notebooks/blob/master/LED_on_Arxiv.ipynb) | Come valutare efficacemente LED sull'attivitร di riassumere "lunghi" testi. | [Patrick von Platen](https://github.com/patrickvonplaten) | [](https://colab.research.google.com/github/patrickvonplaten/notebooks/blob/master/LED_on_Arxiv.ipynb)|
|[Fine-tuning di LayoutLM su RVL-CDIP, un dataset per la classificazione di documenti (immagini)](https://github.com/NielsRogge/Transformers-Tutorials/blob/master/LayoutLM/Fine_tuning_LayoutLMForSequenceClassification_on_RVL_CDIP.ipynb) | Come effettuare il fine-tuning di un modello *LayoutLMForSequenceClassification* sul dataset RVL-CDIP per la classificazione di documenti scannerizzati. | [Niels Rogge](https://github.com/nielsrogge) | [](https://colab.research.google.com/github/NielsRogge/Transformers-Tutorials/blob/master/LayoutLM/Fine_tuning_LayoutLMForSequenceClassification_on_RVL_CDIP.ipynb)|
|[Decodifica Wav2Vec2 CTC con variazioni di GPT2](https://github.com/voidful/huggingface_notebook/blob/main/xlsr_gpt.ipynb) | Come decodificare sequenze CTC, variate da modelli di linguaggio. | [Eric Lam](https://github.com/voidful) | [](https://colab.research.google.com/drive/1e_z5jQHYbO2YKEaUgzb1ww1WwiAyydAj?usp=sharing)
|[Fine-tuning di BART per riassumere testi in due lingue con la classe Trainer](https://github.com/elsanns/xai-nlp-notebooks/blob/master/fine_tune_bart_summarization_two_langs.ipynb) | Come effettuare il fine-tuning di BART per riassumere testi in due lingue usando la classe Trainer. | [Eliza Szczechla](https://github.com/elsanns) | [](https://colab.research.google.com/github/elsanns/xai-nlp-notebooks/blob/master/fine_tune_bart_summarization_two_langs.ipynb)|
|[Valutazione di Big Bird su Trivia QA](https://github.com/patrickvonplaten/notebooks/blob/master/Evaluating_Big_Bird_on_TriviaQA.ipynb) | Come valutare BigBird su question answering di "lunghi" documenti attraverso Trivia QA. | [Patrick von Platen](https://github.com/patrickvonplaten) | [](https://colab.research.google.com/github/patrickvonplaten/notebooks/blob/master/Evaluating_Big_Bird_on_TriviaQA.ipynb)|
| [Creazione di sottotitoli per video usando Wav2Vec2](https://github.com/Muennighoff/ytclipcc/blob/main/wav2vec_youtube_captions.ipynb) | Come creare sottotitoli per qualsiasi video di YouTube trascrivendo l'audio con Wav2Vec. | [Niklas Muennighoff](https://github.com/Muennighoff) |[](https://colab.research.google.com/github/Muennighoff/ytclipcc/blob/main/wav2vec_youtube_captions.ipynb) |
| [Fine-tuning di Vision Transformer su CIFAR-10 usando PyTorch Lightning](https://github.com/NielsRogge/Transformers-Tutorials/blob/master/VisionTransformer/Fine_tuning_the_Vision_Transformer_on_CIFAR_10_with_PyTorch_Lightning.ipynb) | Come effettuare il fine-tuning di Vision Transformer (ViT) su CIFAR-10 usando HuggingFace Transformers, Datasets e PyTorch Lightning.| [Niels Rogge](https://github.com/nielsrogge) |[](https://colab.research.google.com/github/NielsRogge/Transformers-Tutorials/blob/master/VisionTransformer/Fine_tuning_the_Vision_Transformer_on_CIFAR_10_with_PyTorch_Lightning.ipynb) |
| [Fine-tuning di Vision Transformer su CIFAR-10 usando ๐ค Trainer](https://github.com/NielsRogge/Transformers-Tutorials/blob/master/VisionTransformer/Fine_tuning_the_Vision_Transformer_on_CIFAR_10_with_the_%F0%9F%A4%97_Trainer.ipynb) | Come effettuare il fine-tuning di Vision Transformer (ViT) su CIFAR-10 usando HuggingFace Transformers, Datasets e ๐ค Trainer. | [Niels Rogge](https://github.com/nielsrogge) |[](https://colab.research.google.com/github/NielsRogge/Transformers-Tutorials/blob/master/VisionTransformer/Fine_tuning_the_Vision_Transformer_on_CIFAR_10_with_the_%F0%9F%A4%97_Trainer.ipynb) |
| [Valutazione di LUKE su Open Entity, un dataset di entity typing](https://github.com/studio-ousia/luke/blob/master/notebooks/huggingface_open_entity.ipynb) | Come valutare un modello *LukeForEntityClassification* sul dataset Open Entity. | [Ikuya Yamada](https://github.com/ikuyamada) |[](https://colab.research.google.com/github/studio-ousia/luke/blob/master/notebooks/huggingface_open_entity.ipynb) |
| [Valutazione di LUKE su TACRED, un dataset per l'estrazione di relazioni](https://github.com/studio-ousia/luke/blob/master/notebooks/huggingface_tacred.ipynb) | Come valutare un modello *LukeForEntityPairClassification* sul dataset TACRED. | [Ikuya Yamada](https://github.com/ikuyamada) |[](https://colab.research.google.com/github/studio-ousia/luke/blob/master/notebooks/huggingface_tacred.ipynb) |
| [Valutazione di LUKE su CoNLL-2003, un importante benchmark NER](https://github.com/studio-ousia/luke/blob/master/notebooks/huggingface_conll_2003.ipynb) | Come valutare un modello *LukeForEntitySpanClassification* sul dataset CoNLL-2003. | [Ikuya Yamada](https://github.com/ikuyamada) |[](https://colab.research.google.com/github/studio-ousia/luke/blob/master/notebooks/huggingface_conll_2003.ipynb) |
| [Valutazione di BigBird-Pegasus su dataset PubMed](https://github.com/vasudevgupta7/bigbird/blob/main/notebooks/bigbird_pegasus_evaluation.ipynb) | Come valutare un modello *BigBirdPegasusForConditionalGeneration* su dataset PubMed. | [Vasudev Gupta](https://github.com/vasudevgupta7) | [](https://colab.research.google.com/github/vasudevgupta7/bigbird/blob/main/notebooks/bigbird_pegasus_evaluation.ipynb) |
| [Classificazione di emozioni dal discorso con Wav2Vec2](https://github/m3hrdadfi/soxan/blob/main/notebooks/Emotion_recognition_in_Greek_speech_using_Wav2Vec2.ipynb) | Come utilizzare un modello pre-addestrato Wav2Vec2 per la classificazione di emozioni sul dataset MEGA. | [Mehrdad Farahani](https://github.com/m3hrdadfi) | [](https://colab.research.google.com/github/m3hrdadfi/soxan/blob/main/notebooks/Emotion_recognition_in_Greek_speech_using_Wav2Vec2.ipynb) |
| [Rilevamento oggetti in un'immagine con DETR](https://github.com/NielsRogge/Transformers-Tutorials/blob/master/DETR/DETR_minimal_example_(with_DetrFeatureExtractor).ipynb) | Come usare un modello addestrato *DetrForObjectDetection* per rilevare oggetti in un'immagine e visualizzare l'attention. | [Niels Rogge](https://github.com/NielsRogge) | [](https://colab.research.google.com/github/NielsRogge/Transformers-Tutorials/blob/master/DETR/DETR_minimal_example_(with_DetrFeatureExtractor).ipynb) |
| [Fine-tuning di DETR su un dataset personalizzato per rilevare oggetti](https://github.com/NielsRogge/Transformers-Tutorials/blob/master/DETR/Fine_tuning_DetrForObjectDetection_on_custom_dataset_(balloon).ipynb) | Come effettuare fine-tuning di un modello *DetrForObjectDetection* su un dataset personalizzato per rilevare oggetti. | [Niels Rogge](https://github.com/NielsRogge) | [](https://colab.research.google.com/github/NielsRogge/Transformers-Tutorials/blob/master/DETR/Fine_tuning_DetrForObjectDetection_on_custom_dataset_(balloon).ipynb) |
| [Fine-tuning di T5 per Named Entity Recognition](https://github.com/ToluClassics/Notebooks/blob/main/T5_Ner_Finetuning.ipynb) | Come effettuare fine-tunining di *T5* per un'attivitร di Named Entity Recognition. | [Ogundepo Odunayo](https://github.com/ToluClassics) | [](https://colab.research.google.com/drive/1obr78FY_cBmWY5ODViCmzdY6O1KB65Vc?usp=sharing) |
| 0 |
hf_public_repos/transformers/docs/source | hf_public_repos/transformers/docs/source/it/add_new_pipeline.md | <!--Copyright 2020 The HuggingFace Team. All rights reserved.
Licensed under the Apache License, Version 2.0 (the "License"); you may not use this file except in compliance with
the License. You may obtain a copy of the License at
http://www.apache.org/licenses/LICENSE-2.0
Unless required by applicable law or agreed to in writing, software distributed under the License is distributed on
an "AS IS" BASIS, WITHOUT WARRANTIES OR CONDITIONS OF ANY KIND, either express or implied. See the License for the
โ ๏ธ Note that this file is in Markdown but contain specific syntax for our doc-builder (similar to MDX) that may not be
rendered properly in your Markdown viewer.
-->
# Come creare una pipeline personalizzata?
In questa guida, scopriremo come creare una pipeline personalizzata e condividerla sull' [Hub](hf.co/models) o aggiungerla nella libreria
Transformers.
Innanzitutto, รจ necessario decidere gli input grezzi che la pipeline sarร in grado di accettare. Possono essere strings, raw bytes,
dictionaries o qualsiasi cosa sia l'input desiderato piรน probabile. Cerca di mantenere questi input il piรน possibile in Python
in quanto facilita la compatibilitร (anche con altri linguaggi tramite JSON). Questi saranno gli `inputs` della
pipeline (`preprocess`).
Poi definire gli `outputs`. Stessa strategia degli `inputs`. Piรน รจ seplice e meglio รจ. Questi saranno gli output del metodo
`postprocess`.
Si parte ereditando la classe base `Pipeline`. con i 4 metodi che bisogna implementare `preprocess`,
`_forward`, `postprocess` e `_sanitize_parameters`.
```python
from transformers import Pipeline
class MyPipeline(Pipeline):
def _sanitize_parameters(self, **kwargs):
preprocess_kwargs = {}
if "maybe_arg" in kwargs:
preprocess_kwargs["maybe_arg"] = kwargs["maybe_arg"]
return preprocess_kwargs, {}, {}
def preprocess(self, inputs, maybe_arg=2):
model_input = Tensor(inputs["input_ids"])
return {"model_input": model_input}
def _forward(self, model_inputs):
# model_inputs == {"model_input": model_input}
outputs = self.model(**model_inputs)
# Maybe {"logits": Tensor(...)}
return outputs
def postprocess(self, model_outputs):
best_class = model_outputs["logits"].softmax(-1)
return best_class
```
La struttura di questa suddivisione consiste nel supportare in modo relativamente continuo CPU/GPU, supportando allo stesso tempo l'esecuzione di
pre/postelaborazione sulla CPU su thread diversi.
`preprocess` prenderร gli input originariamente definiti e li trasformerร in qualcosa di alimentabile dal modello. Potrebbe
contenere piรน informazioni e di solito รจ un `Dict`.
`_forward` รจ il dettaglio dell'implementazione e non รจ destinato a essere chiamato direttamente. `forward` รจ il metodo preferito per assicurarsi che tutto funzioni correttamente perchรจ contiene delle slavaguardie. Se qualcosa รจ
รจ collegato a un modello reale, appartiene al metodo `_forward`, tutto il resto รจ nel preprocess/postprocess.
`postprocess` prende l'otput di `_forward` e lo trasforma nell'output finale che era stato deciso in precedenza.
`_sanitize_parameters` esiste per consentire agli utenti di passare i parametri ogni volta che desiderano sia a inizialization time `pipeline(...., maybe_arg=4)` che al call time `pipe = pipeline(...); output = pipe(...., maybe_arg=4)`.
`_sanitize_parameters` ritorna 3 dicts di kwargs che vengono passati direttamente a `preprocess`,
`_forward` e `postprocess`. Non riempire nulla se il chiamante non ha chiamato con alcun parametro aggiuntivo. Questo
consente di mantenere gli argomenti predefiniti nella definizione della funzione, che รจ sempre piรน "naturale".
Un esempio classico potrebbe essere l'argomento `top_k` nel post processing dei classification tasks.
```python
>>> pipe = pipeline("my-new-task")
>>> pipe("This is a test")
[{"label": "1-star", "score": 0.8}, {"label": "2-star", "score": 0.1}, {"label": "3-star", "score": 0.05}
{"label": "4-star", "score": 0.025}, {"label": "5-star", "score": 0.025}]
>>> pipe("This is a test", top_k=2)
[{"label": "1-star", "score": 0.8}, {"label": "2-star", "score": 0.1}]
```
In order to achieve that, we'll update our `postprocess` method with a default parameter to `5`. and edit
`_sanitize_parameters` to allow this new parameter.
```python
def postprocess(self, model_outputs, top_k=5):
best_class = model_outputs["logits"].softmax(-1)
# Add logic to handle top_k
return best_class
def _sanitize_parameters(self, **kwargs):
preprocess_kwargs = {}
if "maybe_arg" in kwargs:
preprocess_kwargs["maybe_arg"] = kwargs["maybe_arg"]
postprocess_kwargs = {}
if "top_k" in kwargs:
postprocess_kwargs["top_k"] = kwargs["top_k"]
return preprocess_kwargs, {}, postprocess_kwargs
```
Cercare di mantenere gli input/output molto semplici e idealmente serializzabili in JSON, in quanto ciรฒ rende l'uso della pipeline molto facile
senza richiedere agli utenti di comprendere nuovi tipi di oggetti. ร anche relativamente comune supportare molti tipi di argomenti
per facilitarne l'uso (ad esempio file audio, possono essere nomi di file, URL o byte puri).
## Aggiungilo alla lista dei tasks supportati
Per registrar il tuo `new-task` alla lista dei tasks supportati, devi aggiungerlo al `PIPELINE_REGISTRY`:
```python
from transformers.pipelines import PIPELINE_REGISTRY
PIPELINE_REGISTRY.register_pipeline(
"new-task",
pipeline_class=MyPipeline,
pt_model=AutoModelForSequenceClassification,
)
```
Puoi specificare il modello di default che desideri, in questo caso dovrebbe essere accompagnato da una revisione specifica (che puรฒ essere il nome di un branch o l'hash di un commit, in questo caso abbiamo preso `"abcdef"`) e anche dal type:
```python
PIPELINE_REGISTRY.register_pipeline(
"new-task",
pipeline_class=MyPipeline,
pt_model=AutoModelForSequenceClassification,
default={"pt": ("user/awesome_model", "abcdef")},
type="text", # current support type: text, audio, image, multimodal
)
```
## Condividi la tua pipeline sull'Hub
Per condividere la tua pipeline personalizzata sull'Hub, devi solo salvare il codice della tua sottoclasse `Pipeline` in un file
python. Per esempio, supponiamo di voler utilizzare una pipeline personalizzata per la classificazione delle coppie di frasi come la seguente:
```py
import numpy as np
from transformers import Pipeline
def softmax(outputs):
maxes = np.max(outputs, axis=-1, keepdims=True)
shifted_exp = np.exp(outputs - maxes)
return shifted_exp / shifted_exp.sum(axis=-1, keepdims=True)
class PairClassificationPipeline(Pipeline):
def _sanitize_parameters(self, **kwargs):
preprocess_kwargs = {}
if "second_text" in kwargs:
preprocess_kwargs["second_text"] = kwargs["second_text"]
return preprocess_kwargs, {}, {}
def preprocess(self, text, second_text=None):
return self.tokenizer(text, text_pair=second_text, return_tensors=self.framework)
def _forward(self, model_inputs):
return self.model(**model_inputs)
def postprocess(self, model_outputs):
logits = model_outputs.logits[0].numpy()
probabilities = softmax(logits)
best_class = np.argmax(probabilities)
label = self.model.config.id2label[best_class]
score = probabilities[best_class].item()
logits = logits.tolist()
return {"label": label, "score": score, "logits": logits}
```
L'implementazione รจ agnostica al framework, e lavorerร sia con modelli PyTorch che con TensorFlow. Se l'abbiamo salvato in un file chiamato `pair_classification.py`, puรฒ essere successivamente importato e registrato in questo modo:
```py
from pair_classification import PairClassificationPipeline
from transformers.pipelines import PIPELINE_REGISTRY
from transformers import AutoModelForSequenceClassification, TFAutoModelForSequenceClassification
PIPELINE_REGISTRY.register_pipeline(
"pair-classification",
pipeline_class=PairClassificationPipeline,
pt_model=AutoModelForSequenceClassification,
tf_model=TFAutoModelForSequenceClassification,
)
```
Una volta fatto, possiamo usarla con un modello pretrained. L'istanza `sgugger/finetuned-bert-mrpc` รจ stata
fine-tuned sul dataset MRPC, che classifica le coppie di frasi come parafrasi o no.
```py
from transformers import pipeline
classifier = pipeline("pair-classification", model="sgugger/finetuned-bert-mrpc")
```
Successivamente possiamo condividerlo sull'Hub usando il metodo `save_pretrained` in un `Repository`:
```py
from huggingface_hub import Repository
repo = Repository("test-dynamic-pipeline", clone_from="{your_username}/test-dynamic-pipeline")
classifier.save_pretrained("test-dynamic-pipeline")
repo.push_to_hub()
```
Questo codice copierร il file dove รจ stato definitp `PairClassificationPipeline` all'interno della cartella `"test-dynamic-pipeline"`,
insieme al salvataggio del modello e del tokenizer della pipeline, prima di pushare il tutto nel repository
`{your_username}/test-dynamic-pipeline`. Dopodichรฉ chiunque potrร utilizzarlo, purchรฉ fornisca l'opzione
`trust_remote_code=True`:
```py
from transformers import pipeline
classifier = pipeline(model="{your_username}/test-dynamic-pipeline", trust_remote_code=True)
```
## Aggiungere la pipeline a Transformers
Se vuoi contribuire con la tua pipeline a Transformers, dovrai aggiungere un modulo nel sottomodulo `pipelines`
con il codice della tua pipeline, quindi aggiungilo all'elenco dei tasks definiti in `pipelines/__init__.py`.
Poi hai bisogno di aggiungere i test. Crea un nuovo file `tests/test_pipelines_MY_PIPELINE.py` con esempi ed altri test.
La funzione `run_pipeline_test` sarร molto generica e su piccoli modelli casuali su ogni possibile
architettura, come definito da `model_mapping` e `tf_model_mapping`.
Questo รจ molto importante per testare la compatibilitร futura, nel senso che se qualcuno aggiunge un nuovo modello di
`XXXForQuestionAnswering` allora il test della pipeline tenterร di essere eseguito su di esso. Poichรฉ i modelli sono casuali, รจ
รจ impossibile controllare i valori effettivi, per questo esiste un aiuto `ANY` che tenterร solamente di far corrispondere l'output della pipeline TYPE.
Hai anche *bisogno* di implementare 2 (idealmente 4) test.
- `test_small_model_pt` : Definire 1 piccolo modello per questa pipeline (non importa se i risultati non hanno senso)
e testare i risultati della pipeline. I risultati dovrebbero essere gli stessi di `test_small_model_tf`.
- `test_small_model_tf` : Definire 1 piccolo modello per questa pipeline (non importa se i risultati non hanno senso)
e testare i risultati della pipeline. I risultati dovrebbero essere gli stessi di `test_small_model_pt`.
- `test_large_model_pt` (`optional`): Testare la pipeline su una pipeline reale in cui i risultati dovrebbero avere
senso. Questi test sono lenti e dovrebbero essere contrassegnati come tali. In questo caso l'obiettivo รจ mostrare la pipeline e assicurarsi che non ci siano derive nelle versioni future
- `test_large_model_tf` (`optional`): Testare la pipeline su una pipeline reale in cui i risultati dovrebbero avere
senso. Questi test sono lenti e dovrebbero essere contrassegnati come tali. In questo caso l'obiettivo รจ mostrare la pipeline e assicurarsi
che non ci siano derive nelle versioni future | 0 |
hf_public_repos/transformers/docs/source | hf_public_repos/transformers/docs/source/it/debugging.md | <!--Copyright 2021 The HuggingFace Team. All rights reserved.
Licensed under the Apache License, Version 2.0 (the "License"); you may not use this file except in compliance with
the License. You may obtain a copy of the License at
http://www.apache.org/licenses/LICENSE-2.0
Unless required by applicable law or agreed to in writing, software distributed under the License is distributed on
an "AS IS" BASIS, WITHOUT WARRANTIES OR CONDITIONS OF ANY KIND, either express or implied. See the License for the
specific language governing permissions and limitations under the License.
โ ๏ธ Note that this file is in Markdown but contain specific syntax for our doc-builder (similar to MDX) that may not be
rendered properly in your Markdown viewer.
-->
# Debugging
## Debug dei problemi di rete multi-GPU
Quando addestri o fai inferenza con `DistributedDataParallel` e GPU multiple, se si verificano problemi di intercomunicazione tra processi e/o nodi, puoi utilizzare il seguente script per diagnosticare i problemi della rete.
```bash
wget https://raw.githubusercontent.com/huggingface/transformers/main/scripts/distributed/torch-distributed-gpu-test.py
```
Per esempio per testare come 2 GPU interagiscono fai:
```bash
python -m torch.distributed.run --nproc_per_node 2 --nnodes 1 torch-distributed-gpu-test.py
```
Se entrambi i processi sono in grado di comunicare tra loro e di allocare la memoria della GPU, ciascuno di essi stamperร lo stato OK.
Per piรน GPU o nodi adatta gli argumenti nello script.
All'interno dello script di diagnostica troverai molti altri dettagli e anche una guida per eseguirlo in ambiente SLURM.
Un livello di debug superiore รจ aggiungere la variabile d'ambiente `NCCL_DEBUG=INFO` come di seguito:
```bash
NCCL_DEBUG=INFO python -m torch.distributed.run --nproc_per_node 2 --nnodes 1 torch-distributed-gpu-test.py
```
In questo modo si scaricano molte informazioni di debug relative a NCCL, che puoi cercare online in caso di problemi. Oppure, se non hai la sicurezza di come interpretare l'output, puoi condividere il file di log in una Issue.
## Rilevamento di Underflow e Overflow
<Tip>
Questa funzionalitร al momento รจ disponibile solo per PyTorch.
</Tip>
<Tip>
Per addestramento multi-GPU richiede DDP (`torch.distributed.launch`).
</Tip>
<Tip>
Questa funzionalitร puรฒ essere usata con modelli basati su `nn.Module`.
</Tip>
Se inizi a ottenere `loss=NaN` o il modello presenta qualche altro comportamento anomalo a causa di valori `inf` o `nan` in
attivazioni o nei pesi, รจ necessario scoprire dove si verifica il primo underflow o overflow e cosa lo ha determinato. Fortunatamente
รจ possibile farlo facilmente attivando un modulo speciale che effettuerร il rilevamento automaticamente.
Se stai usando [`Trainer`], hai bisogno di aggiungere solo:
```bash
--debug underflow_overflow
```
ai normali argomenti della riga di comando, o passa `debug="underflow_overflow"` quando viene creato l'oggetto
[`TrainingArguments`].
Se stai usando il tuo ciclo di allenamento o un altro trainer, puoi ottenere lo stesso risultato con:
```python
from .debug_utils import DebugUnderflowOverflow
debug_overflow = DebugUnderflowOverflow(model)
```
[`~debug_utils.DebugUnderflowOverflow`] inserisce dei ganci nel modello che dopo ogni chiamata
testeranno le variabili di ingresso e di uscita e anche i pesi del modulo corrispondente. Non appena viene rilevato `inf` o
o `nan` in almeno un elemento delle attivazioni o dei pesi, il programma lo notifica e stampa un rapporto come il seguente (questo รจ stato rilevato con `google/mt5-small` sotto fp16 mixed precision):
```
Detected inf/nan during batch_number=0
Last 21 forward frames:
abs min abs max metadata
encoder.block.1.layer.1.DenseReluDense.dropout Dropout
0.00e+00 2.57e+02 input[0]
0.00e+00 2.85e+02 output
[...]
encoder.block.2.layer.0 T5LayerSelfAttention
6.78e-04 3.15e+03 input[0]
2.65e-04 3.42e+03 output[0]
None output[1]
2.25e-01 1.00e+04 output[2]
encoder.block.2.layer.1.layer_norm T5LayerNorm
8.69e-02 4.18e-01 weight
2.65e-04 3.42e+03 input[0]
1.79e-06 4.65e+00 output
encoder.block.2.layer.1.DenseReluDense.wi_0 Linear
2.17e-07 4.50e+00 weight
1.79e-06 4.65e+00 input[0]
2.68e-06 3.70e+01 output
encoder.block.2.layer.1.DenseReluDense.wi_1 Linear
8.08e-07 2.66e+01 weight
1.79e-06 4.65e+00 input[0]
1.27e-04 2.37e+02 output
encoder.block.2.layer.1.DenseReluDense.dropout Dropout
0.00e+00 8.76e+03 input[0]
0.00e+00 9.74e+03 output
encoder.block.2.layer.1.DenseReluDense.wo Linear
1.01e-06 6.44e+00 weight
0.00e+00 9.74e+03 input[0]
3.18e-04 6.27e+04 output
encoder.block.2.layer.1.DenseReluDense T5DenseGatedGeluDense
1.79e-06 4.65e+00 input[0]
3.18e-04 6.27e+04 output
encoder.block.2.layer.1.dropout Dropout
3.18e-04 6.27e+04 input[0]
0.00e+00 inf output
```
L'output di esempio รจ stato tagliato al centro per brevitร .
La seconda colonna mostra il valore dell'elemento piรน grande in assoluto,cosรฌ se osserviamo da vicino gli ultimi istanti,
input e output sono nel range di `1e4`. Questo addestramento รจ stato eseguito con una mixed precision fp16 e l'ultimo passo usciva fuori (sotto `fp16` il valore piรน grande prima di `inf` รจ `64e3`). Per evitare overflows sotto `fp16` le attivazionioni devono rimanere molto al di sotto di `1e4`, perchรฉ `1e4 * 1e4 = 1e8` quindi qualsiasi moltiplicazione di matrice con grandi attivazioni porterร a una condizione di overflow numerico.
All'inizio della traccia รจ possibile scoprire a quale lotto si รจ verificato il problema (questo `Detected inf/nan during batch_number=0` significa che il problema si รจ verificato nel primo lotto).
Ogni frame segnalato inizia dichiarando la voce completamente qualificata per il modulo corrispondente per il quale il frame รจ stato segnalato.
Se osserviamo il seguente frame:
```
encoder.block.2.layer.1.layer_norm T5LayerNorm
8.69e-02 4.18e-01 weight
2.65e-04 3.42e+03 input[0]
1.79e-06 4.65e+00 output
```
Questo, `encoder.block.2.layer.1.layer_norm` indica che si tratta di un layer norm nel primo layer, del secondo blocco dell'encoder. E le chiamata specifica di `forward` รจ `T5LayerNorm`.
Osserviamo gli ultimi frame del report:
```
Detected inf/nan during batch_number=0
Last 21 forward frames:
abs min abs max metadata
[...]
encoder.block.2.layer.1.DenseReluDense.wi_0 Linear
2.17e-07 4.50e+00 weight
1.79e-06 4.65e+00 input[0]
2.68e-06 3.70e+01 output
encoder.block.2.layer.1.DenseReluDense.wi_1 Linear
8.08e-07 2.66e+01 weight
1.79e-06 4.65e+00 input[0]
1.27e-04 2.37e+02 output
encoder.block.2.layer.1.DenseReluDense.wo Linear
1.01e-06 6.44e+00 weight
0.00e+00 9.74e+03 input[0]
3.18e-04 6.27e+04 output
encoder.block.2.layer.1.DenseReluDense T5DenseGatedGeluDense
1.79e-06 4.65e+00 input[0]
3.18e-04 6.27e+04 output
encoder.block.2.layer.1.dropout Dropout
3.18e-04 6.27e+04 input[0]
0.00e+00 inf output
```
L'ultimo frame report per la funzione `Dropout.forward` con la prima voce per l'unico input e la seconda per l'unico output. Si puรฒ notare che รจ stato richiamato da un attibuto `dropout` dentro la classe `DenseReluDense`. Si puรฒ notare che ciรฒ รจ avvenuto durante il primo strato, del 2ยฐ blocco, durante il primissimo lotto. Infine, gli elementi di input piรน grandi in assoluto sono stati `6.27e+04` e l'equivalente per l'output era `inf`.
Puoi vedere qui, che `T5DenseGatedGeluDense.forward` risulta in output activations, il cui valore massimo assoluto era circa 62,7K, che รจ molto vicino al limite massimo di 64K di fp16. Nel prossimo frame abbiamo `Dropout` che rinormalizza i pesi, dopo aver azzerato alcuni elementi, il che spinge il valore massimo assoluto a piรน di 64K e si verifica un overflow.(`inf`).
Come puoi notare, รจ nei frames precedenti che occorre esaminare quando i numeri iniziano a diventare molto grandi per i valori fp16.
Confrontiamo il report al codice `models/t5/modeling_t5.py`:
```python
class T5DenseGatedGeluDense(nn.Module):
def __init__(self, config):
super().__init__()
self.wi_0 = nn.Linear(config.d_model, config.d_ff, bias=False)
self.wi_1 = nn.Linear(config.d_model, config.d_ff, bias=False)
self.wo = nn.Linear(config.d_ff, config.d_model, bias=False)
self.dropout = nn.Dropout(config.dropout_rate)
self.gelu_act = ACT2FN["gelu_new"]
def forward(self, hidden_states):
hidden_gelu = self.gelu_act(self.wi_0(hidden_states))
hidden_linear = self.wi_1(hidden_states)
hidden_states = hidden_gelu * hidden_linear
hidden_states = self.dropout(hidden_states)
hidden_states = self.wo(hidden_states)
return hidden_states
```
Ora รจ facile vedere la chiamata `dropout`, e tutte le chiamate precedenti.
Poichรฉ il rilevamento avviene in un avanzamento (forward hook in eng.), i rapporti vengono creati immeditamente dopo ogni rientro da `forward` (forward returns in eng.).
Tornando al rapporto completo, per agire e risolvere il problema, dobbiamo andare qualche frame piรน in alto, dove i numeri hanno iniziato a salire, e probabilmente passare alla modalitร `fp32`, in modo che i numeri non trabocchino quando vengono moltiplicati o sommati. Naturalmente, potrebbero esserci altre soluzioni. Per esempio, potremmo spegnere temporanemante `amp` se รจ abilitato, successivamente spostare `forward` in un helper wrapper, come:
```python
def _forward(self, hidden_states):
hidden_gelu = self.gelu_act(self.wi_0(hidden_states))
hidden_linear = self.wi_1(hidden_states)
hidden_states = hidden_gelu * hidden_linear
hidden_states = self.dropout(hidden_states)
hidden_states = self.wo(hidden_states)
return hidden_states
import torch
def forward(self, hidden_states):
if torch.is_autocast_enabled():
with torch.cuda.amp.autocast(enabled=False):
return self._forward(hidden_states)
else:
return self._forward(hidden_states)
```
Poichรฉ il rilevatore automatico riporta solo gli ingressi e le uscite di fotogrammi completi, una volta che si sa dove cercare, si puรฒ
analizzare anche le fasi intermedie di una specifica funzione `forward`. In alcuni casi puoi usare la funzione di supporto `detect_overflow` per indirizzare il rilevatore dove preferisci, ad esempio:
```python
from debug_utils import detect_overflow
class T5LayerFF(nn.Module):
[...]
def forward(self, hidden_states):
forwarded_states = self.layer_norm(hidden_states)
detect_overflow(forwarded_states, "after layer_norm")
forwarded_states = self.DenseReluDense(forwarded_states)
detect_overflow(forwarded_states, "after DenseReluDense")
return hidden_states + self.dropout(forwarded_states)
```
Si puรฒ vedere che abbiamo aggiunto 2 di questi e ora teniamo traccia se `inf` o `nan` per `forwarded_states` รจ stato rilevato
da qualche parte.
In realtร , il rilevatore li riporta giร , perchรฉ ciascuna delle chiamate nell'esempio precedente รจ un `nn.Module`, ma
diciamo che se avessimo dei calcoli diretti locali, questo รจ il modo in cui lo faremmo.
Inoltre, se si istanzia il debugger nel proprio codice, รจ possibile modificare il numero di fotogrammi stampati rispetto a
predefinito, ad esempio.:
```python
from .debug_utils import DebugUnderflowOverflow
debug_overflow = DebugUnderflowOverflow(model, max_frames_to_save=100)
```
### Tracciamento della mistura assoluta del lotto specifico e del valore massimo
La stessa classe di debug puรฒ essere utilizzata per il tracciamento per-batch con la funzione di rilevamento di underflow/overflow disattivata.
Supponiamo di voler osservare i valori minimi e massimi assoluti per tutti gli ingredienti di ogni chiamata `forward` di un dato lotto.
lotto, e che lo si voglia fare solo per i lotti 1 e 3. Si istanzia questa classe come:
```python
debug_overflow = DebugUnderflowOverflow(model, trace_batch_nums=[1, 3])
```
Ora i batch completi 1 e 3 saranno tracciati utilizzando lo stesso formato del rilevatore di underflow/overflow.
I batches sono 0-indexed.
Questo รจ utile se si sa che il programma inizia a comportarsi male dopo un certo numero di batch, in modo da poter avanzare velocemente fino a quell'area.
direttamente a quell'area. Ecco un esempio di output troncato per questa configurazione:
```
*** Starting batch number=1 ***
abs min abs max metadata
shared Embedding
1.01e-06 7.92e+02 weight
0.00e+00 2.47e+04 input[0]
5.36e-05 7.92e+02 output
[...]
decoder.dropout Dropout
1.60e-07 2.27e+01 input[0]
0.00e+00 2.52e+01 output
decoder T5Stack
not a tensor output
lm_head Linear
1.01e-06 7.92e+02 weight
0.00e+00 1.11e+00 input[0]
6.06e-02 8.39e+01 output
T5ForConditionalGeneration
not a tensor output
*** Starting batch number=3 ***
abs min abs max metadata
shared Embedding
1.01e-06 7.92e+02 weight
0.00e+00 2.78e+04 input[0]
5.36e-05 7.92e+02 output
[...]
```
Qui verrร scaricato un numero enorme di fotogrammi, tanti quanti sono le chiamate in avanti nel modello, quindi puรฒ essere o non essere quello che volete, ma a volte puรฒ essere piรน utile usarlo di un classico debugger. Per esempio, se il problema inizia a verificarsi a partire dal lotto numero 150. Quindi รจ possibile scaricare le tracce dei lotti 149 e 150 e confrontare i punti in cui i numeri hanno iniziato a divergere.
ร inoltre possibile specificare il numero di batch dopo il quale interrompere l'addestramento, con:
```python
debug_overflow = DebugUnderflowOverflow(model, trace_batch_nums=[1, 3], abort_after_batch_num=3)
```
| 0 |
Subsets and Splits
No community queries yet
The top public SQL queries from the community will appear here once available.