|
--- |
|
base_model: FacebookAI/xlm-roberta-large |
|
library_name: sentence-transformers |
|
metrics: |
|
- pearson_cosine |
|
- spearman_cosine |
|
- pearson_manhattan |
|
- spearman_manhattan |
|
- pearson_euclidean |
|
- spearman_euclidean |
|
- pearson_dot |
|
- spearman_dot |
|
- pearson_max |
|
- spearman_max |
|
pipeline_tag: sentence-similarity |
|
tags: |
|
- sentence-transformers |
|
- sentence-similarity |
|
- feature-extraction |
|
model-index: |
|
- name: SentenceTransformer based on FacebookAI/xlm-roberta-large |
|
results: |
|
- task: |
|
type: semantic-similarity |
|
name: Semantic Similarity |
|
dataset: |
|
name: sts dev |
|
type: sts-dev |
|
metrics: |
|
- type: pearson_cosine |
|
value: 0.8256350418804052 |
|
name: Pearson Cosine |
|
- type: spearman_cosine |
|
value: 0.827478494281667 |
|
name: Spearman Cosine |
|
- type: pearson_manhattan |
|
value: 0.8228224900306127 |
|
name: Pearson Manhattan |
|
- type: spearman_manhattan |
|
value: 0.8284011632112219 |
|
name: Spearman Manhattan |
|
- type: pearson_euclidean |
|
value: 0.8231973876582674 |
|
name: Pearson Euclidean |
|
- type: spearman_euclidean |
|
value: 0.8288613978074281 |
|
name: Spearman Euclidean |
|
- type: pearson_dot |
|
value: 0.8016573454999604 |
|
name: Pearson Dot |
|
- type: spearman_dot |
|
value: 0.8004396683364462 |
|
name: Spearman Dot |
|
- type: pearson_max |
|
value: 0.8256350418804052 |
|
name: Pearson Max |
|
- type: spearman_max |
|
value: 0.8288613978074281 |
|
name: Spearman Max |
|
license: apache-2.0 |
|
language: |
|
- ar |
|
--- |
|
|
|
# SentenceTransformer based on FacebookAI/xlm-roberta-large |
|
|
|
This is an **Arabic only** [sentence-transformers](https://www.SBERT.net) model finetuned from [FacebookAI/xlm-roberta-large](https://huggingface.co/FacebookAI/xlm-roberta-large). It maps sentences & paragraphs to a 1024-dimensional dense vector space and can be used for semantic textual similarity, semantic search, paraphrase mining, text classification, clustering, and more. |
|
|
|
The model is trained using the MatryoshkaLoss for embeddings of size 1024, 786, 512, 128, and 64 for storage optimization (See [Evaluation](#Evaluation)). |
|
|
|
## Model Details |
|
|
|
### Model Description |
|
- **Model Type:** Sentence Transformer |
|
- **Base model:** [FacebookAI/xlm-roberta-large](https://huggingface.co/FacebookAI/xlm-roberta-large) <!-- at revision c23d21b0620b635a76227c604d44e43a9f0ee389 --> |
|
- **Maximum Sequence Length:** 512 tokens |
|
- **Output Dimensionality:** 1024 tokens |
|
- **Similarity Function:** Cosine Similarity |
|
|
|
## Usage |
|
|
|
### Direct Usage (Sentence Transformers) |
|
|
|
First install the Sentence Transformers library: |
|
|
|
```bash |
|
pip install -U sentence-transformers |
|
``` |
|
|
|
Then you can load this model and run inference. |
|
```python |
|
from sentence_transformers import SentenceTransformer |
|
|
|
# Download from the 🤗 Hub |
|
matryoshka_dim = 786 |
|
model = SentenceTransformer("omarelshehy/Arabic-STS-Matryoshka", truncate_dim=matryoshka_dim) |
|
# Run inference |
|
sentences = [ |
|
'أحب قراءة الكتب في أوقات فراغي.', |
|
'أستمتع بقراءة القصص في المساء قبل النوم.', |
|
'القراءة تعزز معرفتي وتفتح أمامي آفاق جديدة.', |
|
] |
|
embeddings = model.encode(sentences) |
|
print(embeddings.shape) |
|
# [3, 1024] |
|
|
|
# Get the similarity scores for the embeddings |
|
similarities = model.similarity(embeddings, embeddings) |
|
print(similarities.shape) |
|
# [3, 3] |
|
``` |
|
|
|
## Evaluation |
|
|
|
### Metrics |
|
|
|
#### Semantic Similarity |
|
* Dataset: `sts-dev` |
|
* Evaluated with [<code>EmbeddingSimilarityEvaluator</code>](https://sbert.net/docs/package_reference/sentence_transformer/evaluation.html#sentence_transformers.evaluation.EmbeddingSimilarityEvaluator) |
|
|
|
| Metric | Value | |
|
|:--------------------|:-----------| |
|
| pearson_cosine | 0.8256 | |
|
| **spearman_cosine** | **0.8275** | |
|
| pearson_manhattan | 0.8228 | |
|
| spearman_manhattan | 0.8284 | |
|
| pearson_euclidean | 0.8232 | |
|
| spearman_euclidean | 0.8289 | |
|
| pearson_dot | 0.8017 | |
|
| spearman_dot | 0.8004 | |
|
| pearson_max | 0.8256 | |
|
| spearman_max | 0.8289 | |
|
|
|
#### Embedding Size and Performance |
|
|
|
This plot shows the slight degradation of performance qith smaller embedding sizes (worth investigating for your case since the benefits are huge compared to the slight loss in performance) |
|
|
|
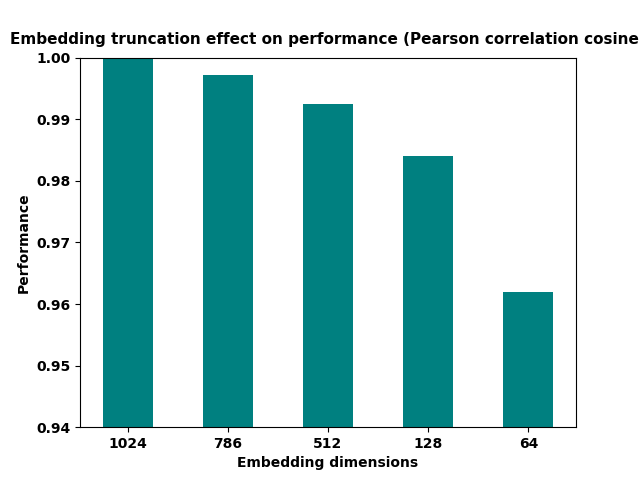 |
|
|
|
|
|
## Citation |
|
|
|
### BibTeX |
|
|
|
#### Sentence Transformers |
|
```bibtex |
|
@inproceedings{reimers-2019-sentence-bert, |
|
title = "Sentence-BERT: Sentence Embeddings using Siamese BERT-Networks", |
|
author = "Reimers, Nils and Gurevych, Iryna", |
|
booktitle = "Proceedings of the 2019 Conference on Empirical Methods in Natural Language Processing", |
|
month = "11", |
|
year = "2019", |
|
publisher = "Association for Computational Linguistics", |
|
url = "https://arxiv.org/abs/1908.10084", |
|
} |
|
``` |
|
|
|
#### MatryoshkaLoss |
|
```bibtex |
|
@misc{kusupati2024matryoshka, |
|
title={Matryoshka Representation Learning}, |
|
author={Aditya Kusupati and Gantavya Bhatt and Aniket Rege and Matthew Wallingford and Aditya Sinha and Vivek Ramanujan and William Howard-Snyder and Kaifeng Chen and Sham Kakade and Prateek Jain and Ali Farhadi}, |
|
year={2024}, |
|
eprint={2205.13147}, |
|
archivePrefix={arXiv}, |
|
primaryClass={cs.LG} |
|
} |
|
``` |
|
|
|
#### MultipleNegativesRankingLoss |
|
```bibtex |
|
@misc{henderson2017efficient, |
|
title={Efficient Natural Language Response Suggestion for Smart Reply}, |
|
author={Matthew Henderson and Rami Al-Rfou and Brian Strope and Yun-hsuan Sung and Laszlo Lukacs and Ruiqi Guo and Sanjiv Kumar and Balint Miklos and Ray Kurzweil}, |
|
year={2017}, |
|
eprint={1705.00652}, |
|
archivePrefix={arXiv}, |
|
primaryClass={cs.CL} |
|
} |
|
``` |
|
|
|
<!-- |
|
## Glossary |
|
|
|
*Clearly define terms in order to be accessible across audiences.* |
|
--> |
|
|
|
<!-- |
|
## Model Card Authors |
|
|
|
*Lists the people who create the model card, providing recognition and accountability for the detailed work that goes into its construction.* |
|
--> |
|
|
|
<!-- |
|
## Model Card Contact |
|
|
|
*Provides a way for people who have updates to the Model Card, suggestions, or questions, to contact the Model Card authors.* |
|
--> |