metadata
base_model: unsloth/llama-3-8b-bnb-4bit
tags:
- llama.cpp
- gguf
- quantized
- q4_k_m
- text-classification
license: apache-2.0
language:
- en
widget:
- text: >-
On the morning of June 15th, armed individuals forced their way into a
local bank in Mexico City. They held bank employees and customers at
gunpoint for several hours while demanding access to the vault. The
perpetrators escaped with an undisclosed amount of money after a prolonged
standoff with local authorities.
example_title: Armed Assault Example
output:
- label: Armed Assault | Hostage Taking
score: 0.9
- text: >-
A massive explosion occurred outside a government building in Baghdad. The
blast, caused by a car bomb, killed 12 people and injured over 30 others.
The explosion caused significant damage to the building's facade and
surrounding structures.
example_title: Bombing Example
output:
- label: Bombing/Explosion
score: 0.95
pipeline_tag: text-classification
inference:
parameters:
temperature: 0.7
max_new_tokens: 128
do_sample: true
ConflLlama: GTD-Finetuned LLaMA-3 8B
- Model Type: GGUF quantized (q4_k_m and q8_0)
- Base Model: unsloth/llama-3-8b-bnb-4bit
- Quantization Details:
- Methods: q4_k_m and q8_0
- q4_k_m uses Q6_K for half of attention.wv and feed_forward.w2 tensors
- Optimized for both speed (q8_0) and quality (q4_k_m)
Training Data
- Dataset: Global Terrorism Database (GTD)
- Time Period: Events before January 1, 2017
- Format: Event summaries with associated attack types
- Labels: Attack type classifications from GTD
Data Processing
- Date Filtering:
- Filtered events occurring before 2017-01-01
- Handled missing dates by setting default month/day to 1
- Data Cleaning:
- Removed entries with missing summaries
- Cleaned summary text by removing special characters and formatting
- Attack Type Processing:
- Combined multiple attack types with separator '|'
- Included primary, secondary, and tertiary attack types when available
- Training Format:
- Input: Processed event summaries
- Output: Combined attack types
- Used chat template:
Below describes details about terrorist events. >>> Event Details: {summary} >>> Attack Types: {combined_attacks}
Training Details
- Framework: QLoRA
- Hardware: NVIDIA A100-SXM4-40GB GPU on Delta Supercomputer
- Training Configuration:
- Batch Size: 1 per device
- Gradient Accumulation Steps: 8
- Learning Rate: 2e-4
- Max Steps: 1000
- Save Steps: 200
- Logging Steps: 10
- LoRA Configuration:
- Rank: 8
- Target Modules: q_proj, k_proj, v_proj, o_proj, gate_proj, up_proj, down_proj
- Alpha: 16
- Dropout: 0
- Optimizations:
- Gradient Checkpointing: Enabled
- 4-bit Quantization: Enabled
- Max Sequence Length: 1024
Model Architecture
The model uses a combination of efficient fine-tuning techniques and optimizations for handling conflict event classification:
Data Processing Pipeline
The preprocessing pipeline transforms raw GTD data into a format suitable for fine-tuning:
Memory Optimizations
- Used 4-bit quantization
- Gradient accumulation steps: 8
- Memory-efficient gradient checkpointing
- Reduced maximum sequence length to 1024
- Disabled dataloader pin memory
Intended Use
This model is designed for:
- Classification of terrorist events based on event descriptions
- Research in conflict studies and terrorism analysis
- Understanding attack type patterns in historical events
- Academic research in security studies
Limitations
- Training data limited to pre-2017 events
- Maximum sequence length limited to 1024 tokens
- May not capture recent changes in attack patterns
- Performance dependent on quality of event descriptions
Ethical Considerations
- Model trained on sensitive terrorism-related data
- Should be used responsibly for research purposes only
- Not intended for operational security decisions
- Results should be interpreted with appropriate context
Citation
@misc{conflllama,
author = {Meher, Shreyas},
title = {ConflLlama: GTD-Finetuned LLaMA-3 8B},
year = {2024},
publisher = {HuggingFace},
note = {Based on Meta's LLaMA-3 8B and GTD Dataset}
}
Acknowledgments
- Unsloth for optimization framework and base model
- Hugging Face for transformers infrastructure
- Global Terrorism Database team
- BBOV project support
- This research was supported by NSF award 2311142
- This work used Delta at NCSA / University of Illinois through allocation CIS220162 from the Advanced Cyberinfrastructure Coordination Ecosystem: Services & Support (ACCESS) program, which is supported by NSF grants 2138259, 2138286, 2138307, 2137603, and 2138296
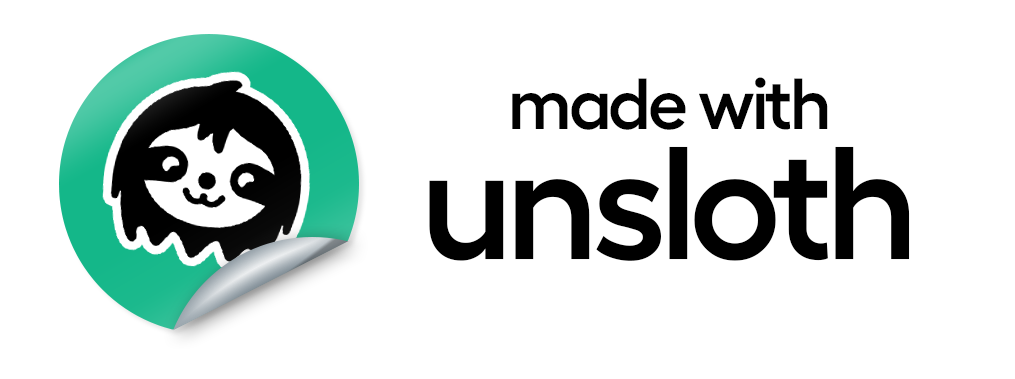