modelId
stringlengths 5
122
| author
stringlengths 2
42
| last_modified
unknown | downloads
int64 0
738M
| likes
int64 0
11k
| library_name
stringclasses 245
values | tags
sequencelengths 1
4.05k
| pipeline_tag
stringclasses 48
values | createdAt
unknown | card
stringlengths 1
901k
|
---|---|---|---|---|---|---|---|---|---|
mnaylor/mega-base-wikitext | mnaylor | "2023-06-28T20:32:48Z" | 977 | 1 | transformers | [
"transformers",
"pytorch",
"safetensors",
"mega",
"fill-mask",
"en",
"license:apache-2.0",
"autotrain_compatible",
"endpoints_compatible",
"region:us"
] | fill-mask | "2023-02-21T20:56:10Z" | ---
license: apache-2.0
language:
- en
library_name: transformers
---
# Mega Masked LM on wikitext-103
This is the location on the Hugging Face hub for the Mega MLM checkpoint. I trained this model on the `wikitext-103` dataset using standard
BERT-style masked LM pretraining using the [original Mega repository](https://github.com/facebookresearch/mega) and uploaded the weights
initially to hf.co/mnaylor/mega-wikitext-103. When the implementation of Mega into Hugging Face's `transformers` is finished, the weights here
are designed to be used with `MegaForMaskedLM` and are compatible with the other (encoder-based) `MegaFor*` model classes.
This model uses the RoBERTa base tokenizer since the Mega paper does not implement a specific tokenizer aside from the character-level
tokenizer used to illustrate long-sequence performance. |
khhuang/zerofec-qa2claim-t5-base | khhuang | "2023-08-31T18:16:21Z" | 977 | 4 | transformers | [
"transformers",
"pytorch",
"safetensors",
"t5",
"text2text-generation",
"en",
"autotrain_compatible",
"endpoints_compatible",
"text-generation-inference",
"region:us"
] | text2text-generation | "2023-08-13T19:43:58Z" | ---
language: en
widget:
- text: a 1968 american independent horror film \\n What is Night of the Living Dead?
---
# QA2Claim Model From ZeroFEC
ZeroFEC is a faithful and interpetable factual error correction framework introduced in the paper [Zero-shot Faithful Factual Error Correction](https://aclanthology.org/2023.acl-long.311/). It involves a component that converts qa-pairs to declarative statements, which is hosted in this repo. The associated code is released in [this](https://github.com/khuangaf/ZeroFEC) repository.
### How to use
Using Huggingface pipeline abstraction:
```python
from transformers import pipeline
nlp = pipeline("text2text-generation", model='khhuang/zerofec-qa2claim-t5-base', tokenizer='khhuang/zerofec-qa2claim-t5-base')
QUESTION = "What is Night of the Living Dead?"
ANSWER = "a 1968 american independent horror film"
def format_inputs(question: str, answer: str):
return f"{answer} \\n {question}"
text = format_inputs(QUESTION, ANSWER)
nlp(text)
# should output [{'generated_text': 'Night of the Living Dead is a 1968 american independent horror film.'}]
```
Using the pre-trained model directly:
```python
from transformers import AutoTokenizer, AutoModelForSeq2SeqLM
tokenizer = AutoTokenizer.from_pretrained('khhuang/zerofec-qa2claim-t5-base')
model = AutoModelForSeq2SeqLM.from_pretrained('khhuang/zerofec-qa2claim-t5-base')
QUESTION = "What is Night of the Living Dead?"
ANSWER = "a 1968 american independent horror film"
def format_inputs(question: str, answer: str):
return f"{answer} \\n {question}"
text = format_inputs(QUESTION, ANSWER)
input_ids = tokenizer(text, return_tensors="pt").input_ids
generated_ids = model.generate(input_ids, max_length=32, num_beams=4)
output = tokenizer.batch_decode(generated_ids, skip_special_tokens=True)
print(output)
# should output "Night of the Living Dead is a 1968 american independent horror film."
```
### Citation
```
@inproceedings{huang-etal-2023-zero,
title = "Zero-shot Faithful Factual Error Correction",
author = "Huang, Kung-Hsiang and
Chan, Hou Pong and
Ji, Heng",
booktitle = "Proceedings of the 61st Annual Meeting of the Association for Computational Linguistics (Volume 1: Long Papers)",
month = jul,
year = "2023",
address = "Toronto, Canada",
publisher = "Association for Computational Linguistics",
url = "https://aclanthology.org/2023.acl-long.311",
doi = "10.18653/v1/2023.acl-long.311",
pages = "5660--5676",
}
``` |
telosnex/fllama | telosnex | "2024-06-28T18:58:17Z" | 977 | 3 | null | [
"gguf",
"region:us"
] | null | "2024-01-21T01:04:53Z" | Entry not found |
mradermacher/MiquMaid-v3-70B-GGUF | mradermacher | "2024-05-06T05:13:51Z" | 977 | 1 | transformers | [
"transformers",
"gguf",
"not-for-all-audiences",
"nsfw",
"merge",
"en",
"base_model:NeverSleep/MiquMaid-v3-70B",
"license:cc-by-nc-4.0",
"endpoints_compatible",
"region:us"
] | null | "2024-04-05T22:05:22Z" | ---
base_model: NeverSleep/MiquMaid-v3-70B
language:
- en
library_name: transformers
license: cc-by-nc-4.0
quantized_by: mradermacher
tags:
- not-for-all-audiences
- nsfw
- merge
---
## About
<!-- ### quantize_version: 1 -->
<!-- ### output_tensor_quantised: 1 -->
<!-- ### convert_type: -->
<!-- ### vocab_type: -->
static quants of https://huggingface.co/NeverSleep/MiquMaid-v3-70B
<!-- provided-files -->
weighted/imatrix quants are available at https://huggingface.co/mradermacher/MiquMaid-v3-70B-i1-GGUF
## Usage
If you are unsure how to use GGUF files, refer to one of [TheBloke's
READMEs](https://huggingface.co/TheBloke/KafkaLM-70B-German-V0.1-GGUF) for
more details, including on how to concatenate multi-part files.
## Provided Quants
(sorted by size, not necessarily quality. IQ-quants are often preferable over similar sized non-IQ quants)
| Link | Type | Size/GB | Notes |
|:-----|:-----|--------:|:------|
| [GGUF](https://huggingface.co/mradermacher/MiquMaid-v3-70B-GGUF/resolve/main/MiquMaid-v3-70B.Q2_K.gguf) | Q2_K | 25.6 | |
| [GGUF](https://huggingface.co/mradermacher/MiquMaid-v3-70B-GGUF/resolve/main/MiquMaid-v3-70B.IQ3_XS.gguf) | IQ3_XS | 28.4 | |
| [GGUF](https://huggingface.co/mradermacher/MiquMaid-v3-70B-GGUF/resolve/main/MiquMaid-v3-70B.IQ3_S.gguf) | IQ3_S | 30.0 | beats Q3_K* |
| [GGUF](https://huggingface.co/mradermacher/MiquMaid-v3-70B-GGUF/resolve/main/MiquMaid-v3-70B.Q3_K_S.gguf) | Q3_K_S | 30.0 | |
| [GGUF](https://huggingface.co/mradermacher/MiquMaid-v3-70B-GGUF/resolve/main/MiquMaid-v3-70B.IQ3_M.gguf) | IQ3_M | 31.0 | |
| [GGUF](https://huggingface.co/mradermacher/MiquMaid-v3-70B-GGUF/resolve/main/MiquMaid-v3-70B.Q3_K_M.gguf) | Q3_K_M | 33.4 | lower quality |
| [GGUF](https://huggingface.co/mradermacher/MiquMaid-v3-70B-GGUF/resolve/main/MiquMaid-v3-70B.Q3_K_L.gguf) | Q3_K_L | 36.2 | |
| [GGUF](https://huggingface.co/mradermacher/MiquMaid-v3-70B-GGUF/resolve/main/MiquMaid-v3-70B.IQ4_XS.gguf) | IQ4_XS | 37.3 | |
| [GGUF](https://huggingface.co/mradermacher/MiquMaid-v3-70B-GGUF/resolve/main/MiquMaid-v3-70B.Q4_K_S.gguf) | Q4_K_S | 39.3 | fast, recommended |
| [GGUF](https://huggingface.co/mradermacher/MiquMaid-v3-70B-GGUF/resolve/main/MiquMaid-v3-70B.Q4_K_M.gguf) | Q4_K_M | 41.5 | fast, recommended |
| [GGUF](https://huggingface.co/mradermacher/MiquMaid-v3-70B-GGUF/resolve/main/MiquMaid-v3-70B.Q5_K_S.gguf) | Q5_K_S | 47.6 | |
| [GGUF](https://huggingface.co/mradermacher/MiquMaid-v3-70B-GGUF/resolve/main/MiquMaid-v3-70B.Q5_K_M.gguf) | Q5_K_M | 48.9 | |
| [PART 1](https://huggingface.co/mradermacher/MiquMaid-v3-70B-GGUF/resolve/main/MiquMaid-v3-70B.Q6_K.gguf.part1of2) [PART 2](https://huggingface.co/mradermacher/MiquMaid-v3-70B-GGUF/resolve/main/MiquMaid-v3-70B.Q6_K.gguf.part2of2) | Q6_K | 56.7 | very good quality |
| [PART 1](https://huggingface.co/mradermacher/MiquMaid-v3-70B-GGUF/resolve/main/MiquMaid-v3-70B.Q8_0.gguf.part1of2) [PART 2](https://huggingface.co/mradermacher/MiquMaid-v3-70B-GGUF/resolve/main/MiquMaid-v3-70B.Q8_0.gguf.part2of2) | Q8_0 | 73.4 | fast, best quality |
Here is a handy graph by ikawrakow comparing some lower-quality quant
types (lower is better):

And here are Artefact2's thoughts on the matter:
https://gist.github.com/Artefact2/b5f810600771265fc1e39442288e8ec9
## FAQ / Model Request
See https://huggingface.co/mradermacher/model_requests for some answers to
questions you might have and/or if you want some other model quantized.
## Thanks
I thank my company, [nethype GmbH](https://www.nethype.de/), for letting
me use its servers and providing upgrades to my workstation to enable
this work in my free time.
<!-- end -->
|
imdatta0/nanollama | imdatta0 | "2024-04-23T13:55:48Z" | 977 | 0 | transformers | [
"transformers",
"safetensors",
"llama",
"text-generation",
"arxiv:1910.09700",
"autotrain_compatible",
"endpoints_compatible",
"text-generation-inference",
"region:us"
] | text-generation | "2024-04-22T11:26:56Z" | ---
library_name: transformers
tags: []
---
# Model Card for Model ID
<!-- Provide a quick summary of what the model is/does. -->
## Model Details
### Model Description
<!-- Provide a longer summary of what this model is. -->
This is the model card of a 🤗 transformers model that has been pushed on the Hub. This model card has been automatically generated.
- **Developed by:** [More Information Needed]
- **Funded by [optional]:** [More Information Needed]
- **Shared by [optional]:** [More Information Needed]
- **Model type:** [More Information Needed]
- **Language(s) (NLP):** [More Information Needed]
- **License:** [More Information Needed]
- **Finetuned from model [optional]:** [More Information Needed]
### Model Sources [optional]
<!-- Provide the basic links for the model. -->
- **Repository:** [More Information Needed]
- **Paper [optional]:** [More Information Needed]
- **Demo [optional]:** [More Information Needed]
## Uses
<!-- Address questions around how the model is intended to be used, including the foreseeable users of the model and those affected by the model. -->
### Direct Use
<!-- This section is for the model use without fine-tuning or plugging into a larger ecosystem/app. -->
[More Information Needed]
### Downstream Use [optional]
<!-- This section is for the model use when fine-tuned for a task, or when plugged into a larger ecosystem/app -->
[More Information Needed]
### Out-of-Scope Use
<!-- This section addresses misuse, malicious use, and uses that the model will not work well for. -->
[More Information Needed]
## Bias, Risks, and Limitations
<!-- This section is meant to convey both technical and sociotechnical limitations. -->
[More Information Needed]
### Recommendations
<!-- This section is meant to convey recommendations with respect to the bias, risk, and technical limitations. -->
Users (both direct and downstream) should be made aware of the risks, biases and limitations of the model. More information needed for further recommendations.
## How to Get Started with the Model
Use the code below to get started with the model.
[More Information Needed]
## Training Details
### Training Data
<!-- This should link to a Dataset Card, perhaps with a short stub of information on what the training data is all about as well as documentation related to data pre-processing or additional filtering. -->
[More Information Needed]
### Training Procedure
<!-- This relates heavily to the Technical Specifications. Content here should link to that section when it is relevant to the training procedure. -->
#### Preprocessing [optional]
[More Information Needed]
#### Training Hyperparameters
- **Training regime:** [More Information Needed] <!--fp32, fp16 mixed precision, bf16 mixed precision, bf16 non-mixed precision, fp16 non-mixed precision, fp8 mixed precision -->
#### Speeds, Sizes, Times [optional]
<!-- This section provides information about throughput, start/end time, checkpoint size if relevant, etc. -->
[More Information Needed]
## Evaluation
<!-- This section describes the evaluation protocols and provides the results. -->
### Testing Data, Factors & Metrics
#### Testing Data
<!-- This should link to a Dataset Card if possible. -->
[More Information Needed]
#### Factors
<!-- These are the things the evaluation is disaggregating by, e.g., subpopulations or domains. -->
[More Information Needed]
#### Metrics
<!-- These are the evaluation metrics being used, ideally with a description of why. -->
[More Information Needed]
### Results
[More Information Needed]
#### Summary
## Model Examination [optional]
<!-- Relevant interpretability work for the model goes here -->
[More Information Needed]
## Environmental Impact
<!-- Total emissions (in grams of CO2eq) and additional considerations, such as electricity usage, go here. Edit the suggested text below accordingly -->
Carbon emissions can be estimated using the [Machine Learning Impact calculator](https://mlco2.github.io/impact#compute) presented in [Lacoste et al. (2019)](https://arxiv.org/abs/1910.09700).
- **Hardware Type:** [More Information Needed]
- **Hours used:** [More Information Needed]
- **Cloud Provider:** [More Information Needed]
- **Compute Region:** [More Information Needed]
- **Carbon Emitted:** [More Information Needed]
## Technical Specifications [optional]
### Model Architecture and Objective
[More Information Needed]
### Compute Infrastructure
[More Information Needed]
#### Hardware
[More Information Needed]
#### Software
[More Information Needed]
## Citation [optional]
<!-- If there is a paper or blog post introducing the model, the APA and Bibtex information for that should go in this section. -->
**BibTeX:**
[More Information Needed]
**APA:**
[More Information Needed]
## Glossary [optional]
<!-- If relevant, include terms and calculations in this section that can help readers understand the model or model card. -->
[More Information Needed]
## More Information [optional]
[More Information Needed]
## Model Card Authors [optional]
[More Information Needed]
## Model Card Contact
[More Information Needed] |
FallenMerick/Smart-Lemon-Cookie-7B | FallenMerick | "2024-05-25T19:03:34Z" | 977 | 1 | transformers | [
"transformers",
"safetensors",
"mistral",
"text-generation",
"mergekit",
"merge",
"roleplay",
"conversational",
"en",
"arxiv:2306.01708",
"base_model:SanjiWatsuki/Silicon-Maid-7B",
"base_model:MTSAIR/multi_verse_model",
"base_model:SanjiWatsuki/Kunoichi-7B",
"base_model:KatyTheCutie/LemonadeRP-4.5.3",
"license:cc-by-4.0",
"model-index",
"autotrain_compatible",
"endpoints_compatible",
"text-generation-inference",
"region:us"
] | text-generation | "2024-04-30T00:54:39Z" | ---
language:
- en
license: cc-by-4.0
library_name: transformers
tags:
- mergekit
- merge
- mistral
- text-generation
- roleplay
base_model:
- SanjiWatsuki/Silicon-Maid-7B
- MTSAIR/multi_verse_model
- SanjiWatsuki/Kunoichi-7B
- KatyTheCutie/LemonadeRP-4.5.3
model-index:
- name: Smart-Lemon-Cookie-7B
results:
- task:
type: text-generation
name: Text Generation
dataset:
name: AI2 Reasoning Challenge (25-Shot)
type: ai2_arc
config: ARC-Challenge
split: test
args:
num_few_shot: 25
metrics:
- type: acc_norm
value: 66.3
name: normalized accuracy
source:
url: https://huggingface.co/spaces/HuggingFaceH4/open_llm_leaderboard?query=FallenMerick/Smart-Lemon-Cookie-7B
name: Open LLM Leaderboard
- task:
type: text-generation
name: Text Generation
dataset:
name: HellaSwag (10-Shot)
type: hellaswag
split: validation
args:
num_few_shot: 10
metrics:
- type: acc_norm
value: 85.53
name: normalized accuracy
source:
url: https://huggingface.co/spaces/HuggingFaceH4/open_llm_leaderboard?query=FallenMerick/Smart-Lemon-Cookie-7B
name: Open LLM Leaderboard
- task:
type: text-generation
name: Text Generation
dataset:
name: MMLU (5-Shot)
type: cais/mmlu
config: all
split: test
args:
num_few_shot: 5
metrics:
- type: acc
value: 64.69
name: accuracy
source:
url: https://huggingface.co/spaces/HuggingFaceH4/open_llm_leaderboard?query=FallenMerick/Smart-Lemon-Cookie-7B
name: Open LLM Leaderboard
- task:
type: text-generation
name: Text Generation
dataset:
name: TruthfulQA (0-shot)
type: truthful_qa
config: multiple_choice
split: validation
args:
num_few_shot: 0
metrics:
- type: mc2
value: 60.66
source:
url: https://huggingface.co/spaces/HuggingFaceH4/open_llm_leaderboard?query=FallenMerick/Smart-Lemon-Cookie-7B
name: Open LLM Leaderboard
- task:
type: text-generation
name: Text Generation
dataset:
name: Winogrande (5-shot)
type: winogrande
config: winogrande_xl
split: validation
args:
num_few_shot: 5
metrics:
- type: acc
value: 77.74
name: accuracy
source:
url: https://huggingface.co/spaces/HuggingFaceH4/open_llm_leaderboard?query=FallenMerick/Smart-Lemon-Cookie-7B
name: Open LLM Leaderboard
- task:
type: text-generation
name: Text Generation
dataset:
name: GSM8k (5-shot)
type: gsm8k
config: main
split: test
args:
num_few_shot: 5
metrics:
- type: acc
value: 54.06
name: accuracy
source:
url: https://huggingface.co/spaces/HuggingFaceH4/open_llm_leaderboard?query=FallenMerick/Smart-Lemon-Cookie-7B
name: Open LLM Leaderboard
---
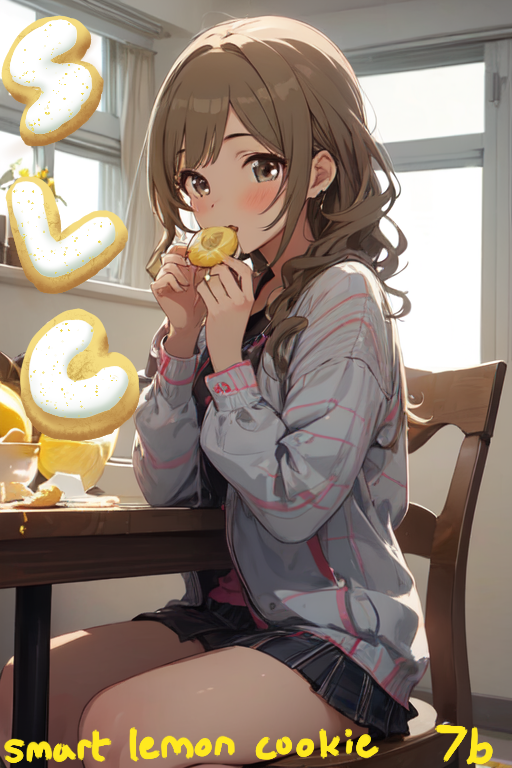
*image courtesy of [@matchaaaaa](https://huggingface.co/matchaaaaa)*
</br>
</br>
# Smart-Lemon-Cookie-7B
This is a merge of pre-trained language models created using [mergekit](https://github.com/cg123/mergekit).
GGUF quants:
* https://huggingface.co/FaradayDotDev/Smart-Lemon-Cookie-7B-GGUF
* https://huggingface.co/mradermacher/Smart-Lemon-Cookie-7B-GGUF
## Merge Details
### Merge Method
This model was merged using the [TIES](https://arxiv.org/abs/2306.01708) merge method using [MTSAIR/multi_verse_model](https://huggingface.co/MTSAIR/multi_verse_model) as a base.
### Models Merged
The following models were included in the merge:
* [SanjiWatsuki/Silicon-Maid-7B](https://huggingface.co/SanjiWatsuki/Silicon-Maid-7B)
* [SanjiWatsuki/Kunoichi-7B](https://huggingface.co/SanjiWatsuki/Kunoichi-7B)
* [KatyTheCutie/LemonadeRP-4.5.3](https://huggingface.co/KatyTheCutie/LemonadeRP-4.5.3)
### Configuration
The following YAML configuration was used to produce this model:
```yaml
models:
- model: SanjiWatsuki/Silicon-Maid-7B
parameters:
density: 1.0
weight: 1.0
- model: SanjiWatsuki/Kunoichi-7B
parameters:
density: 0.4
weight: 1.0
- model: KatyTheCutie/LemonadeRP-4.5.3
parameters:
density: 0.6
weight: 1.0
merge_method: ties
base_model: MTSAIR/multi_verse_model
parameters:
normalize: true
dtype: float16
```
# [Open LLM Leaderboard Evaluation Results](https://huggingface.co/spaces/HuggingFaceH4/open_llm_leaderboard)
Detailed results can be found [here](https://huggingface.co/datasets/open-llm-leaderboard/details_FallenMerick__Smart-Lemon-Cookie-7B)
| Metric |Value|
|---------------------------------|----:|
|Avg. |68.16|
|AI2 Reasoning Challenge (25-Shot)|66.30|
|HellaSwag (10-Shot) |85.53|
|MMLU (5-Shot) |64.69|
|TruthfulQA (0-shot) |60.66|
|Winogrande (5-shot) |77.74|
|GSM8k (5-shot) |54.06|
|
google/t5-3b-ssm-nqo | google | "2023-01-24T16:43:49Z" | 976 | 0 | transformers | [
"transformers",
"pytorch",
"tf",
"t5",
"text2text-generation",
"en",
"dataset:c4",
"dataset:wikipedia",
"dataset:natural_questions",
"arxiv:2002.08909",
"arxiv:1910.10683",
"license:apache-2.0",
"autotrain_compatible",
"endpoints_compatible",
"text-generation-inference",
"region:us"
] | text2text-generation | "2022-03-02T23:29:05Z" | ---
language: en
datasets:
- c4
- wikipedia
- natural_questions
license: apache-2.0
---
[Google's T5](https://ai.googleblog.com/2020/02/exploring-transfer-learning-with-t5.html) for **Closed Book Question Answering**.
The model was pre-trained using T5's denoising objective on [C4](https://huggingface.co/datasets/c4), subsequently additionally pre-trained using [REALM](https://arxiv.org/pdf/2002.08909.pdf)'s salient span masking objective on [Wikipedia](https://huggingface.co/datasets/wikipedia), and finally fine-tuned on [Natural Questions (NQ)](https://huggingface.co/datasets/natural_questions).
**Note**: The model was fine-tuned on 90% of the train splits of [Natural Questions (NQ)](https://huggingface.co/datasets/natural_questions) for 20k steps and validated on the held-out 10% of the train split.
Other community Checkpoints: [here](https://huggingface.co/models?search=ssm)
Paper: [How Much Knowledge Can You Pack
Into the Parameters of a Language Model?](https://arxiv.org/abs/1910.10683.pdf)
Authors: *Adam Roberts, Colin Raffel, Noam Shazeer*
## Results on Natural Questions - Test Set
|Id | link | Exact Match |
|---|---|---|
|T5-large|https://huggingface.co/google/t5-large-ssm-nqo|29.0|
|T5-xxl|https://huggingface.co/google/t5-xxl-ssm-nqo|35.2|
|**T5-3b**|**https://huggingface.co/google/t5-3b-ssm-nqo**|**31.7**|
|T5-11b|https://huggingface.co/google/t5-11b-ssm-nqo|34.8|
## Usage
The model can be used as follows for **closed book question answering**:
```python
from transformers import AutoModelForSeq2SeqLM, AutoTokenizer
t5_qa_model = AutoModelForSeq2SeqLM.from_pretrained("google/t5-3b-ssm-nqo")
t5_tok = AutoTokenizer.from_pretrained("google/t5-3b-ssm-nqo")
input_ids = t5_tok("When was Franklin D. Roosevelt born?", return_tensors="pt").input_ids
gen_output = t5_qa_model.generate(input_ids)[0]
print(t5_tok.decode(gen_output, skip_special_tokens=True))
```
## Abstract
It has recently been observed that neural language models trained on unstructured text can implicitly store and retrieve knowledge using natural language queries. In this short paper, we measure the practical utility of this approach by fine-tuning pre-trained models to answer questions without access to any external context or knowledge. We show that this approach scales with model size and performs competitively with open-domain systems that explicitly retrieve answers from an external knowledge source when answering questions. To facilitate reproducibility and future work, we release our code and trained models at https://goo.gle/t5-cbqa.
 |
diffusers/controlnet-canny-sdxl-1.0-mid | diffusers | "2023-08-16T12:59:53Z" | 976 | 17 | diffusers | [
"diffusers",
"safetensors",
"stable-diffusion-xl",
"stable-diffusion-xl-diffusers",
"text-to-image",
"controlnet",
"base_model:stabilityai/stable-diffusion-xl-base-1.0",
"license:openrail++",
"region:us"
] | text-to-image | "2023-08-16T11:20:41Z" | ---
license: openrail++
base_model: stabilityai/stable-diffusion-xl-base-1.0
tags:
- stable-diffusion-xl
- stable-diffusion-xl-diffusers
- text-to-image
- diffusers
- controlnet
inference: false
---
# Small SDXL-controlnet: Canny
These are small controlnet weights trained on stabilityai/stable-diffusion-xl-base-1.0 with canny conditioning. This checkpoint is 5x smaller than the original XL controlnet checkpoint.
You can find some example images in the following.
prompt: aerial view, a futuristic research complex in a bright foggy jungle, hard lighting

prompt: a woman, close up, detailed, beautiful, street photography, photorealistic, detailed, Kodak ektar 100, natural, candid shot

prompt: megatron in an apocalyptic world ground, runied city in the background, photorealistic

prompt: a couple watching sunset, 4k photo

## Usage
Make sure to first install the libraries:
```bash
pip install accelerate transformers safetensors opencv-python diffusers
```
And then we're ready to go:
```python
from diffusers import ControlNetModel, StableDiffusionXLControlNetPipeline, AutoencoderKL
from diffusers.utils import load_image
from PIL import Image
import torch
import numpy as np
import cv2
prompt = "aerial view, a futuristic research complex in a bright foggy jungle, hard lighting"
negative_prompt = "low quality, bad quality, sketches"
image = load_image("https://huggingface.co/datasets/hf-internal-testing/diffusers-images/resolve/main/sd_controlnet/hf-logo.png")
controlnet_conditioning_scale = 0.5 # recommended for good generalization
controlnet = ControlNetModel.from_pretrained(
"diffusers/controlnet-canny-sdxl-1.0-mid",
torch_dtype=torch.float16
)
vae = AutoencoderKL.from_pretrained("madebyollin/sdxl-vae-fp16-fix", torch_dtype=torch.float16)
pipe = StableDiffusionXLControlNetPipeline.from_pretrained(
"stabilityai/stable-diffusion-xl-base-1.0",
controlnet=controlnet,
vae=vae,
torch_dtype=torch.float16,
)
pipe.enable_model_cpu_offload()
image = np.array(image)
image = cv2.Canny(image, 100, 200)
image = image[:, :, None]
image = np.concatenate([image, image, image], axis=2)
image = Image.fromarray(image)
images = pipe(
prompt, negative_prompt=negative_prompt, image=image, controlnet_conditioning_scale=controlnet_conditioning_scale,
).images
images[0].save(f"hug_lab.png")
```

To more details, check out the official documentation of [`StableDiffusionXLControlNetPipeline`](https://huggingface.co/docs/diffusers/main/en/api/pipelines/controlnet_sdxl).
🚨 Please note that this checkpoint is experimental and there's a lot of room for improvement. We encourage the community to build on top of it, improve it, and provide us with feedback. 🚨
### Training
Our training script was built on top of the official training script that we provide [here](https://github.com/huggingface/diffusers/blob/main/examples/controlnet/README_sdxl.md).
You can refer to [this script](https://github.com/huggingface/diffusers/blob/7b93c2a882d8e12209fbaeffa51ee2b599ab5349/examples/research_projects/controlnet/train_controlnet_webdataset.py) for full discolsure.
* This checkpoint does not perform distillation. We just use a smaller ControlNet initialized from the SDXL UNet. We
encourage the community to try and conduct distillation too. This resource might be of help in [this regard](https://huggingface.co/blog/sd_distillation).
* To learn more about how the ControlNet was initialized, refer to [this code block](https://github.com/huggingface/diffusers/blob/7b93c2a882d8e12209fbaeffa51ee2b599ab5349/examples/research_projects/controlnet/train_controlnet_webdataset.py#L981C1-L999C36).
* It does not have any attention blocks.
* The model works pretty good on most conditioning images. But for more complex conditionings, the bigger checkpoints might be better. We are still working on improving the quality of this checkpoint and looking for feedback from the community.
* We recommend playing around with the `controlnet_conditioning_scale` and `guidance_scale` arguments for potentially better
image generation quality.
#### Training data
The model was trained on 3M images from LAION aesthetic 6 plus subset, with batch size of 256 for 50k steps with constant learning rate of 3e-5.
#### Compute
One 8xA100 machine
#### Mixed precision
FP16 |
UCSC-VLAA/ViT-L-14-CLIPA-datacomp1B | UCSC-VLAA | "2023-10-17T05:46:10Z" | 976 | 2 | open_clip | [
"open_clip",
"safetensors",
"clip",
"zero-shot-image-classification",
"dataset:mlfoundations/datacomp_1b",
"arxiv:2306.15658",
"arxiv:2305.07017",
"license:apache-2.0",
"region:us"
] | zero-shot-image-classification | "2023-10-17T05:42:03Z" | ---
tags:
- clip
library_name: open_clip
pipeline_tag: zero-shot-image-classification
license: apache-2.0
datasets:
- mlfoundations/datacomp_1b
---
# Model card for ViT-L-14-CLIPA-datacomp1B
A CLIPA-v2 model...
## Model Details
- **Model Type:** Contrastive Image-Text, Zero-Shot Image Classification.
- **Original:** https://github.com/UCSC-VLAA/CLIPA
- **Dataset:** mlfoundations/datacomp_1b
- **Papers:**
- CLIPA-v2: Scaling CLIP Training with 81.1% Zero-shot ImageNet Accuracy within a $10,000 Budget; An Extra $4,000 Unlocks 81.8% Accuracy: https://arxiv.org/abs/2306.15658
- An Inverse Scaling Law for CLIP Training: https://arxiv.org/abs/2305.07017
## Model Usage
### With OpenCLIP
```
import torch
import torch.nn.functional as F
from urllib.request import urlopen
from PIL import Image
from open_clip import create_model_from_pretrained, get_tokenizer
model, preprocess = create_model_from_pretrained('hf-hub:ViT-L-14-CLIPA')
tokenizer = get_tokenizer('hf-hub:ViT-L-14-CLIPA')
image = Image.open(urlopen(
'https://huggingface.co/datasets/huggingface/documentation-images/resolve/main/beignets-task-guide.png'
))
image = preprocess(image).unsqueeze(0)
text = tokenizer(["a diagram", "a dog", "a cat", "a beignet"], context_length=model.context_length)
with torch.no_grad(), torch.cuda.amp.autocast():
image_features = model.encode_image(image)
text_features = model.encode_text(text)
image_features = F.normalize(image_features, dim=-1)
text_features = F.normalize(text_features, dim=-1)
text_probs = (100.0 * image_features @ text_features.T).softmax(dim=-1)
print("Label probs:", text_probs) # prints: [[0., 0., 0., 1.0]]
```
## Citation
```bibtex
@article{li2023clipav2,
title={CLIPA-v2: Scaling CLIP Training with 81.1% Zero-shot ImageNet Accuracy within a $10,000 Budget; An Extra $4,000 Unlocks 81.8% Accuracy},
author={Xianhang Li and Zeyu Wang and Cihang Xie},
journal={arXiv preprint arXiv:2306.15658},
year={2023},
}
```
```bibtex
@inproceedings{li2023clipa,
title={An Inverse Scaling Law for CLIP Training},
author={Xianhang Li and Zeyu Wang and Cihang Xie},
booktitle={NeurIPS},
year={2023},
}
```
|
TheBloke/Mistral-ClaudeLimaRP-v3-7B-GGUF | TheBloke | "2023-10-29T09:32:14Z" | 976 | 15 | transformers | [
"transformers",
"gguf",
"mistral",
"not-for-all-audiences",
"nsfw",
"base_model:Undi95/Mistral-ClaudeLimaRP-v3-7B",
"license:apache-2.0",
"text-generation-inference",
"region:us"
] | null | "2023-10-29T09:27:59Z" | ---
base_model: Undi95/Mistral-ClaudeLimaRP-v3-7B
inference: false
license: apache-2.0
model_creator: Undi
model_name: Mistral ClaudeLimaRP v3 7B
model_type: mistral
prompt_template: "### Instruction:\nCharacter's Persona: bot character description\n\
\nUser's persona: user character description\n \nScenario: what happens in the\
\ story\n\nPlay the role of Character. You must engage in a roleplaying chat with\
\ User below this line. Do not write dialogues and narration for User. Character\
\ should respond with messages of medium length.\n\n### Input:\nUser: {prompt}\n\
\n### Response:\nCharacter: \n"
quantized_by: TheBloke
tags:
- not-for-all-audiences
- nsfw
---
<!-- markdownlint-disable MD041 -->
<!-- header start -->
<!-- 200823 -->
<div style="width: auto; margin-left: auto; margin-right: auto">
<img src="https://i.imgur.com/EBdldam.jpg" alt="TheBlokeAI" style="width: 100%; min-width: 400px; display: block; margin: auto;">
</div>
<div style="display: flex; justify-content: space-between; width: 100%;">
<div style="display: flex; flex-direction: column; align-items: flex-start;">
<p style="margin-top: 0.5em; margin-bottom: 0em;"><a href="https://discord.gg/theblokeai">Chat & support: TheBloke's Discord server</a></p>
</div>
<div style="display: flex; flex-direction: column; align-items: flex-end;">
<p style="margin-top: 0.5em; margin-bottom: 0em;"><a href="https://www.patreon.com/TheBlokeAI">Want to contribute? TheBloke's Patreon page</a></p>
</div>
</div>
<div style="text-align:center; margin-top: 0em; margin-bottom: 0em"><p style="margin-top: 0.25em; margin-bottom: 0em;">TheBloke's LLM work is generously supported by a grant from <a href="https://a16z.com">andreessen horowitz (a16z)</a></p></div>
<hr style="margin-top: 1.0em; margin-bottom: 1.0em;">
<!-- header end -->
# Mistral ClaudeLimaRP v3 7B - GGUF
- Model creator: [Undi](https://huggingface.co/Undi95)
- Original model: [Mistral ClaudeLimaRP v3 7B](https://huggingface.co/Undi95/Mistral-ClaudeLimaRP-v3-7B)
<!-- description start -->
## Description
This repo contains GGUF format model files for [Undi's Mistral ClaudeLimaRP v3 7B](https://huggingface.co/Undi95/Mistral-ClaudeLimaRP-v3-7B).
These files were quantised using hardware kindly provided by [Massed Compute](https://massedcompute.com/).
<!-- description end -->
<!-- README_GGUF.md-about-gguf start -->
### About GGUF
GGUF is a new format introduced by the llama.cpp team on August 21st 2023. It is a replacement for GGML, which is no longer supported by llama.cpp.
Here is an incomplate list of clients and libraries that are known to support GGUF:
* [llama.cpp](https://github.com/ggerganov/llama.cpp). The source project for GGUF. Offers a CLI and a server option.
* [text-generation-webui](https://github.com/oobabooga/text-generation-webui), the most widely used web UI, with many features and powerful extensions. Supports GPU acceleration.
* [KoboldCpp](https://github.com/LostRuins/koboldcpp), a fully featured web UI, with GPU accel across all platforms and GPU architectures. Especially good for story telling.
* [LM Studio](https://lmstudio.ai/), an easy-to-use and powerful local GUI for Windows and macOS (Silicon), with GPU acceleration.
* [LoLLMS Web UI](https://github.com/ParisNeo/lollms-webui), a great web UI with many interesting and unique features, including a full model library for easy model selection.
* [Faraday.dev](https://faraday.dev/), an attractive and easy to use character-based chat GUI for Windows and macOS (both Silicon and Intel), with GPU acceleration.
* [ctransformers](https://github.com/marella/ctransformers), a Python library with GPU accel, LangChain support, and OpenAI-compatible AI server.
* [llama-cpp-python](https://github.com/abetlen/llama-cpp-python), a Python library with GPU accel, LangChain support, and OpenAI-compatible API server.
* [candle](https://github.com/huggingface/candle), a Rust ML framework with a focus on performance, including GPU support, and ease of use.
<!-- README_GGUF.md-about-gguf end -->
<!-- repositories-available start -->
## Repositories available
* [AWQ model(s) for GPU inference.](https://huggingface.co/TheBloke/Mistral-ClaudeLimaRP-v3-7B-AWQ)
* [GPTQ models for GPU inference, with multiple quantisation parameter options.](https://huggingface.co/TheBloke/Mistral-ClaudeLimaRP-v3-7B-GPTQ)
* [2, 3, 4, 5, 6 and 8-bit GGUF models for CPU+GPU inference](https://huggingface.co/TheBloke/Mistral-ClaudeLimaRP-v3-7B-GGUF)
* [Undi's original unquantised fp16 model in pytorch format, for GPU inference and for further conversions](https://huggingface.co/Undi95/Mistral-ClaudeLimaRP-v3-7B)
<!-- repositories-available end -->
<!-- prompt-template start -->
## Prompt template: LimaRP-Alpaca
```
### Instruction:
Character's Persona: bot character description
User's persona: user character description
Scenario: what happens in the story
Play the role of Character. You must engage in a roleplaying chat with User below this line. Do not write dialogues and narration for User. Character should respond with messages of medium length.
### Input:
User: {prompt}
### Response:
Character:
```
<!-- prompt-template end -->
<!-- compatibility_gguf start -->
## Compatibility
These quantised GGUFv2 files are compatible with llama.cpp from August 27th onwards, as of commit [d0cee0d](https://github.com/ggerganov/llama.cpp/commit/d0cee0d36d5be95a0d9088b674dbb27354107221)
They are also compatible with many third party UIs and libraries - please see the list at the top of this README.
## Explanation of quantisation methods
<details>
<summary>Click to see details</summary>
The new methods available are:
* GGML_TYPE_Q2_K - "type-1" 2-bit quantization in super-blocks containing 16 blocks, each block having 16 weight. Block scales and mins are quantized with 4 bits. This ends up effectively using 2.5625 bits per weight (bpw)
* GGML_TYPE_Q3_K - "type-0" 3-bit quantization in super-blocks containing 16 blocks, each block having 16 weights. Scales are quantized with 6 bits. This end up using 3.4375 bpw.
* GGML_TYPE_Q4_K - "type-1" 4-bit quantization in super-blocks containing 8 blocks, each block having 32 weights. Scales and mins are quantized with 6 bits. This ends up using 4.5 bpw.
* GGML_TYPE_Q5_K - "type-1" 5-bit quantization. Same super-block structure as GGML_TYPE_Q4_K resulting in 5.5 bpw
* GGML_TYPE_Q6_K - "type-0" 6-bit quantization. Super-blocks with 16 blocks, each block having 16 weights. Scales are quantized with 8 bits. This ends up using 6.5625 bpw
Refer to the Provided Files table below to see what files use which methods, and how.
</details>
<!-- compatibility_gguf end -->
<!-- README_GGUF.md-provided-files start -->
## Provided files
| Name | Quant method | Bits | Size | Max RAM required | Use case |
| ---- | ---- | ---- | ---- | ---- | ----- |
| [mistral-claudelimarp-v3-7b.Q2_K.gguf](https://huggingface.co/TheBloke/Mistral-ClaudeLimaRP-v3-7B-GGUF/blob/main/mistral-claudelimarp-v3-7b.Q2_K.gguf) | Q2_K | 2 | 3.08 GB| 5.58 GB | smallest, significant quality loss - not recommended for most purposes |
| [mistral-claudelimarp-v3-7b.Q3_K_S.gguf](https://huggingface.co/TheBloke/Mistral-ClaudeLimaRP-v3-7B-GGUF/blob/main/mistral-claudelimarp-v3-7b.Q3_K_S.gguf) | Q3_K_S | 3 | 3.16 GB| 5.66 GB | very small, high quality loss |
| [mistral-claudelimarp-v3-7b.Q3_K_M.gguf](https://huggingface.co/TheBloke/Mistral-ClaudeLimaRP-v3-7B-GGUF/blob/main/mistral-claudelimarp-v3-7b.Q3_K_M.gguf) | Q3_K_M | 3 | 3.52 GB| 6.02 GB | very small, high quality loss |
| [mistral-claudelimarp-v3-7b.Q3_K_L.gguf](https://huggingface.co/TheBloke/Mistral-ClaudeLimaRP-v3-7B-GGUF/blob/main/mistral-claudelimarp-v3-7b.Q3_K_L.gguf) | Q3_K_L | 3 | 3.82 GB| 6.32 GB | small, substantial quality loss |
| [mistral-claudelimarp-v3-7b.Q4_0.gguf](https://huggingface.co/TheBloke/Mistral-ClaudeLimaRP-v3-7B-GGUF/blob/main/mistral-claudelimarp-v3-7b.Q4_0.gguf) | Q4_0 | 4 | 4.11 GB| 6.61 GB | legacy; small, very high quality loss - prefer using Q3_K_M |
| [mistral-claudelimarp-v3-7b.Q4_K_S.gguf](https://huggingface.co/TheBloke/Mistral-ClaudeLimaRP-v3-7B-GGUF/blob/main/mistral-claudelimarp-v3-7b.Q4_K_S.gguf) | Q4_K_S | 4 | 4.14 GB| 6.64 GB | small, greater quality loss |
| [mistral-claudelimarp-v3-7b.Q4_K_M.gguf](https://huggingface.co/TheBloke/Mistral-ClaudeLimaRP-v3-7B-GGUF/blob/main/mistral-claudelimarp-v3-7b.Q4_K_M.gguf) | Q4_K_M | 4 | 4.37 GB| 6.87 GB | medium, balanced quality - recommended |
| [mistral-claudelimarp-v3-7b.Q5_0.gguf](https://huggingface.co/TheBloke/Mistral-ClaudeLimaRP-v3-7B-GGUF/blob/main/mistral-claudelimarp-v3-7b.Q5_0.gguf) | Q5_0 | 5 | 5.00 GB| 7.50 GB | legacy; medium, balanced quality - prefer using Q4_K_M |
| [mistral-claudelimarp-v3-7b.Q5_K_S.gguf](https://huggingface.co/TheBloke/Mistral-ClaudeLimaRP-v3-7B-GGUF/blob/main/mistral-claudelimarp-v3-7b.Q5_K_S.gguf) | Q5_K_S | 5 | 5.00 GB| 7.50 GB | large, low quality loss - recommended |
| [mistral-claudelimarp-v3-7b.Q5_K_M.gguf](https://huggingface.co/TheBloke/Mistral-ClaudeLimaRP-v3-7B-GGUF/blob/main/mistral-claudelimarp-v3-7b.Q5_K_M.gguf) | Q5_K_M | 5 | 5.13 GB| 7.63 GB | large, very low quality loss - recommended |
| [mistral-claudelimarp-v3-7b.Q6_K.gguf](https://huggingface.co/TheBloke/Mistral-ClaudeLimaRP-v3-7B-GGUF/blob/main/mistral-claudelimarp-v3-7b.Q6_K.gguf) | Q6_K | 6 | 5.94 GB| 8.44 GB | very large, extremely low quality loss |
| [mistral-claudelimarp-v3-7b.Q8_0.gguf](https://huggingface.co/TheBloke/Mistral-ClaudeLimaRP-v3-7B-GGUF/blob/main/mistral-claudelimarp-v3-7b.Q8_0.gguf) | Q8_0 | 8 | 7.70 GB| 10.20 GB | very large, extremely low quality loss - not recommended |
**Note**: the above RAM figures assume no GPU offloading. If layers are offloaded to the GPU, this will reduce RAM usage and use VRAM instead.
<!-- README_GGUF.md-provided-files end -->
<!-- README_GGUF.md-how-to-download start -->
## How to download GGUF files
**Note for manual downloaders:** You almost never want to clone the entire repo! Multiple different quantisation formats are provided, and most users only want to pick and download a single file.
The following clients/libraries will automatically download models for you, providing a list of available models to choose from:
* LM Studio
* LoLLMS Web UI
* Faraday.dev
### In `text-generation-webui`
Under Download Model, you can enter the model repo: TheBloke/Mistral-ClaudeLimaRP-v3-7B-GGUF and below it, a specific filename to download, such as: mistral-claudelimarp-v3-7b.Q4_K_M.gguf.
Then click Download.
### On the command line, including multiple files at once
I recommend using the `huggingface-hub` Python library:
```shell
pip3 install huggingface-hub
```
Then you can download any individual model file to the current directory, at high speed, with a command like this:
```shell
huggingface-cli download TheBloke/Mistral-ClaudeLimaRP-v3-7B-GGUF mistral-claudelimarp-v3-7b.Q4_K_M.gguf --local-dir . --local-dir-use-symlinks False
```
<details>
<summary>More advanced huggingface-cli download usage</summary>
You can also download multiple files at once with a pattern:
```shell
huggingface-cli download TheBloke/Mistral-ClaudeLimaRP-v3-7B-GGUF --local-dir . --local-dir-use-symlinks False --include='*Q4_K*gguf'
```
For more documentation on downloading with `huggingface-cli`, please see: [HF -> Hub Python Library -> Download files -> Download from the CLI](https://huggingface.co/docs/huggingface_hub/guides/download#download-from-the-cli).
To accelerate downloads on fast connections (1Gbit/s or higher), install `hf_transfer`:
```shell
pip3 install hf_transfer
```
And set environment variable `HF_HUB_ENABLE_HF_TRANSFER` to `1`:
```shell
HF_HUB_ENABLE_HF_TRANSFER=1 huggingface-cli download TheBloke/Mistral-ClaudeLimaRP-v3-7B-GGUF mistral-claudelimarp-v3-7b.Q4_K_M.gguf --local-dir . --local-dir-use-symlinks False
```
Windows Command Line users: You can set the environment variable by running `set HF_HUB_ENABLE_HF_TRANSFER=1` before the download command.
</details>
<!-- README_GGUF.md-how-to-download end -->
<!-- README_GGUF.md-how-to-run start -->
## Example `llama.cpp` command
Make sure you are using `llama.cpp` from commit [d0cee0d](https://github.com/ggerganov/llama.cpp/commit/d0cee0d36d5be95a0d9088b674dbb27354107221) or later.
```shell
./main -ngl 32 -m mistral-claudelimarp-v3-7b.Q4_K_M.gguf --color -c 2048 --temp 0.7 --repeat_penalty 1.1 -n -1 -p "### Instruction:\nCharacter's Persona: bot character description\n\nUser's persona: user character description\n \nScenario: what happens in the story\n\nPlay the role of Character. You must engage in a roleplaying chat with User below this line. Do not write dialogues and narration for User. Character should respond with messages of medium length.\n\n### Input:\nUser: {prompt}\n\n### Response:\nCharacter:"
```
Change `-ngl 32` to the number of layers to offload to GPU. Remove it if you don't have GPU acceleration.
Change `-c 2048` to the desired sequence length. For extended sequence models - eg 8K, 16K, 32K - the necessary RoPE scaling parameters are read from the GGUF file and set by llama.cpp automatically.
If you want to have a chat-style conversation, replace the `-p <PROMPT>` argument with `-i -ins`
For other parameters and how to use them, please refer to [the llama.cpp documentation](https://github.com/ggerganov/llama.cpp/blob/master/examples/main/README.md)
## How to run in `text-generation-webui`
Further instructions here: [text-generation-webui/docs/llama.cpp.md](https://github.com/oobabooga/text-generation-webui/blob/main/docs/llama.cpp.md).
## How to run from Python code
You can use GGUF models from Python using the [llama-cpp-python](https://github.com/abetlen/llama-cpp-python) or [ctransformers](https://github.com/marella/ctransformers) libraries.
### How to load this model in Python code, using ctransformers
#### First install the package
Run one of the following commands, according to your system:
```shell
# Base ctransformers with no GPU acceleration
pip install ctransformers
# Or with CUDA GPU acceleration
pip install ctransformers[cuda]
# Or with AMD ROCm GPU acceleration (Linux only)
CT_HIPBLAS=1 pip install ctransformers --no-binary ctransformers
# Or with Metal GPU acceleration for macOS systems only
CT_METAL=1 pip install ctransformers --no-binary ctransformers
```
#### Simple ctransformers example code
```python
from ctransformers import AutoModelForCausalLM
# Set gpu_layers to the number of layers to offload to GPU. Set to 0 if no GPU acceleration is available on your system.
llm = AutoModelForCausalLM.from_pretrained("TheBloke/Mistral-ClaudeLimaRP-v3-7B-GGUF", model_file="mistral-claudelimarp-v3-7b.Q4_K_M.gguf", model_type="mistral", gpu_layers=50)
print(llm("AI is going to"))
```
## How to use with LangChain
Here are guides on using llama-cpp-python and ctransformers with LangChain:
* [LangChain + llama-cpp-python](https://python.langchain.com/docs/integrations/llms/llamacpp)
* [LangChain + ctransformers](https://python.langchain.com/docs/integrations/providers/ctransformers)
<!-- README_GGUF.md-how-to-run end -->
<!-- footer start -->
<!-- 200823 -->
## Discord
For further support, and discussions on these models and AI in general, join us at:
[TheBloke AI's Discord server](https://discord.gg/theblokeai)
## Thanks, and how to contribute
Thanks to the [chirper.ai](https://chirper.ai) team!
Thanks to Clay from [gpus.llm-utils.org](llm-utils)!
I've had a lot of people ask if they can contribute. I enjoy providing models and helping people, and would love to be able to spend even more time doing it, as well as expanding into new projects like fine tuning/training.
If you're able and willing to contribute it will be most gratefully received and will help me to keep providing more models, and to start work on new AI projects.
Donaters will get priority support on any and all AI/LLM/model questions and requests, access to a private Discord room, plus other benefits.
* Patreon: https://patreon.com/TheBlokeAI
* Ko-Fi: https://ko-fi.com/TheBlokeAI
**Special thanks to**: Aemon Algiz.
**Patreon special mentions**: Brandon Frisco, LangChain4j, Spiking Neurons AB, transmissions 11, Joseph William Delisle, Nitin Borwankar, Willem Michiel, Michael Dempsey, vamX, Jeffrey Morgan, zynix, jjj, Omer Bin Jawed, Sean Connelly, jinyuan sun, Jeromy Smith, Shadi, Pawan Osman, Chadd, Elijah Stavena, Illia Dulskyi, Sebastain Graf, Stephen Murray, terasurfer, Edmond Seymore, Celu Ramasamy, Mandus, Alex, biorpg, Ajan Kanaga, Clay Pascal, Raven Klaugh, 阿明, K, ya boyyy, usrbinkat, Alicia Loh, John Villwock, ReadyPlayerEmma, Chris Smitley, Cap'n Zoog, fincy, GodLy, S_X, sidney chen, Cory Kujawski, OG, Mano Prime, AzureBlack, Pieter, Kalila, Spencer Kim, Tom X Nguyen, Stanislav Ovsiannikov, Michael Levine, Andrey, Trailburnt, Vadim, Enrico Ros, Talal Aujan, Brandon Phillips, Jack West, Eugene Pentland, Michael Davis, Will Dee, webtim, Jonathan Leane, Alps Aficionado, Rooh Singh, Tiffany J. Kim, theTransient, Luke @flexchar, Elle, Caitlyn Gatomon, Ari Malik, subjectnull, Johann-Peter Hartmann, Trenton Dambrowitz, Imad Khwaja, Asp the Wyvern, Emad Mostaque, Rainer Wilmers, Alexandros Triantafyllidis, Nicholas, Pedro Madruga, SuperWojo, Harry Royden McLaughlin, James Bentley, Olakabola, David Ziegler, Ai Maven, Jeff Scroggin, Nikolai Manek, Deo Leter, Matthew Berman, Fen Risland, Ken Nordquist, Manuel Alberto Morcote, Luke Pendergrass, TL, Fred von Graf, Randy H, Dan Guido, NimbleBox.ai, Vitor Caleffi, Gabriel Tamborski, knownsqashed, Lone Striker, Erik Bjäreholt, John Detwiler, Leonard Tan, Iucharbius
Thank you to all my generous patrons and donaters!
And thank you again to a16z for their generous grant.
<!-- footer end -->
<!-- original-model-card start -->
# Original model card: Undi's Mistral ClaudeLimaRP v3 7B
## Description
This repo contains fp16 files of [Norquinal/Mistral-7B-claude-chat](https://huggingface.co/Norquinal/Mistral-7B-claude-chat) with the LoRA [lemonilia/LimaRP-Mistral-7B-v0.1](https://huggingface.co/lemonilia/LimaRP-Mistral-7B-v0.1) applied at weight "0.75".
All credit go to [lemonilia](https://huggingface.co/lemonilia) and [Norquinal](https://huggingface.co/Norquinal)
## Prompt format
Same as before. It uses the [extended Alpaca format](https://github.com/tatsu-lab/stanford_alpaca),
with `### Input:` immediately preceding user inputs and `### Response:` immediately preceding
model outputs. While Alpaca wasn't originally intended for multi-turn responses, in practice this
is not a problem; the format follows a pattern already used by other models.
```
### Instruction:
Character's Persona: {bot character description}
User's Persona: {user character description}
Scenario: {what happens in the story}
Play the role of Character. You must engage in a roleplaying chat with User below this line. Do not write dialogues and narration for User.
### Input:
User: {utterance}
### Response:
Character: {utterance}
### Input
User: {utterance}
### Response:
Character: {utterance}
(etc.)
```
You should:
- Replace all text in curly braces (curly braces included) with your own text.
- Replace `User` and `Character` with appropriate names.
### Message length control
Inspired by the previously named "Roleplay" preset in SillyTavern, with this
version of LimaRP it is possible to append a length modifier to the response instruction
sequence, like this:
```
### Input
User: {utterance}
### Response: (length = medium)
Character: {utterance}
```
This has an immediately noticeable effect on bot responses. The available lengths are:
`tiny`, `short`, `medium`, `long`, `huge`, `humongous`, `extreme`, `unlimited`. **The
recommended starting length is `medium`**. Keep in mind that the AI may ramble
or impersonate the user with very long messages.
The length control effect is reproducible, but the messages will not necessarily follow
lengths very precisely, rather follow certain ranges on average, as seen in this table
with data from tests made with one reply at the beginning of the conversation:
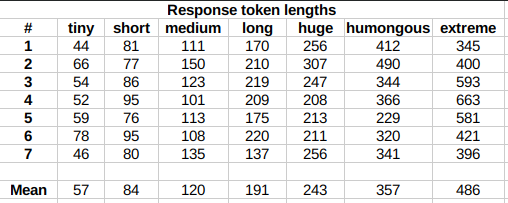
Response length control appears to work well also deep into the conversation.
## Suggested settings
You can follow these instruction format settings in SillyTavern. Replace `tiny` with
your desired response length:

## Text generation settings
Extensive testing with Mistral has not been performed yet, but suggested starting text
generation settings may be:
- TFS = 0.90~0.95
- Temperature = 0.70~0.85
- Repetition penalty = 1.08~1.10
- top-k = 0 (disabled)
- top-p = 1 (disabled)
If you want to support me, you can [here](https://ko-fi.com/undiai).
<!-- original-model-card end -->
|
timm/edgenext_base.in21k_ft_in1k | timm | "2023-04-23T22:42:41Z" | 975 | 0 | timm | [
"timm",
"pytorch",
"safetensors",
"image-classification",
"dataset:imagenet-1k",
"dataset:imagenet-21k-p",
"arxiv:2206.10589",
"license:mit",
"region:us"
] | image-classification | "2023-04-23T22:42:29Z" | ---
tags:
- image-classification
- timm
library_name: timm
license: mit
datasets:
- imagenet-1k
- imagenet-21k-p
---
# Model card for edgenext_base.in21k_ft_in1k
An EdgeNeXt image classification model. Pretrained on ImageNet-21k-P (winter21 subset) and fine-tuned on ImageNet-1k by paper authors.
## Model Details
- **Model Type:** Image classification / feature backbone
- **Model Stats:**
- Params (M): 18.5
- GMACs: 3.8
- Activations (M): 15.6
- Image size: train = 256 x 256, test = 320 x 320
- **Papers:**
- EdgeNeXt: Efficiently Amalgamated CNN-Transformer Architecture for Mobile Vision Applications: https://arxiv.org/abs/2206.10589
- **Pretrain Dataset:** ImageNet-21K-P
- **Dataset:** ImageNet-1k
- **Original:** https://github.com/mmaaz60/EdgeNeXt
## Model Usage
### Image Classification
```python
from urllib.request import urlopen
from PIL import Image
import timm
img = Image.open(urlopen(
'https://huggingface.co/datasets/huggingface/documentation-images/resolve/main/beignets-task-guide.png'
))
model = timm.create_model('edgenext_base.in21k_ft_in1k', pretrained=True)
model = model.eval()
# get model specific transforms (normalization, resize)
data_config = timm.data.resolve_model_data_config(model)
transforms = timm.data.create_transform(**data_config, is_training=False)
output = model(transforms(img).unsqueeze(0)) # unsqueeze single image into batch of 1
top5_probabilities, top5_class_indices = torch.topk(output.softmax(dim=1) * 100, k=5)
```
### Feature Map Extraction
```python
from urllib.request import urlopen
from PIL import Image
import timm
img = Image.open(urlopen(
'https://huggingface.co/datasets/huggingface/documentation-images/resolve/main/beignets-task-guide.png'
))
model = timm.create_model(
'edgenext_base.in21k_ft_in1k',
pretrained=True,
features_only=True,
)
model = model.eval()
# get model specific transforms (normalization, resize)
data_config = timm.data.resolve_model_data_config(model)
transforms = timm.data.create_transform(**data_config, is_training=False)
output = model(transforms(img).unsqueeze(0)) # unsqueeze single image into batch of 1
for o in output:
# print shape of each feature map in output
# e.g.:
# torch.Size([1, 80, 64, 64])
# torch.Size([1, 160, 32, 32])
# torch.Size([1, 288, 16, 16])
# torch.Size([1, 584, 8, 8])
print(o.shape)
```
### Image Embeddings
```python
from urllib.request import urlopen
from PIL import Image
import timm
img = Image.open(urlopen(
'https://huggingface.co/datasets/huggingface/documentation-images/resolve/main/beignets-task-guide.png'
))
model = timm.create_model(
'edgenext_base.in21k_ft_in1k',
pretrained=True,
num_classes=0, # remove classifier nn.Linear
)
model = model.eval()
# get model specific transforms (normalization, resize)
data_config = timm.data.resolve_model_data_config(model)
transforms = timm.data.create_transform(**data_config, is_training=False)
output = model(transforms(img).unsqueeze(0)) # output is (batch_size, num_features) shaped tensor
# or equivalently (without needing to set num_classes=0)
output = model.forward_features(transforms(img).unsqueeze(0))
# output is unpooled, a (1, 584, 8, 8) shaped tensor
output = model.forward_head(output, pre_logits=True)
# output is a (1, num_features) shaped tensor
```
## Citation
```bibtex
@inproceedings{Maaz2022EdgeNeXt,
title={EdgeNeXt: Efficiently Amalgamated CNN-Transformer Architecture for Mobile Vision Applications},
author={Muhammad Maaz and Abdelrahman Shaker and Hisham Cholakkal and Salman Khan and Syed Waqas Zamir and Rao Muhammad Anwer and Fahad Shahbaz Khan},
booktitle={International Workshop on Computational Aspects of Deep Learning at 17th European Conference on Computer Vision (CADL2022)},
year={2022},
organization={Springer}
}
```
|
WhitePeak/bert-base-cased-Korean-sentiment | WhitePeak | "2023-09-19T01:59:03Z" | 975 | 0 | transformers | [
"transformers",
"pytorch",
"bert",
"text-classification",
"generated_from_trainer",
"ko",
"dataset:WhitePeak/shopping_review",
"base_model:bert-base-multilingual-cased",
"license:apache-2.0",
"autotrain_compatible",
"endpoints_compatible",
"region:us"
] | text-classification | "2023-09-18T23:20:53Z" | ---
license: apache-2.0
base_model: bert-base-multilingual-cased
tags:
- generated_from_trainer
metrics:
- accuracy
- f1
model-index:
- name: bert-base-cased-Korean-sentiment
results: []
datasets:
- WhitePeak/shopping_review
language:
- ko
---
<!-- This model card has been generated automatically according to the information the Trainer had access to. You
should probably proofread and complete it, then remove this comment. -->
# bert-base-cased-Korean-sentiment
This model is a fine-tuned version of [bert-base-multilingual-cased](https://huggingface.co/bert-base-multilingual-cased) on an unknown dataset.
It achieves the following results on the evaluation set:
- Loss: 0.2338
- Accuracy: 0.9234
- F1: 0.9238
## Model description
This is a fine-tuned model for a sentiment analysis for the Korean language based on customer reviews in the Korean language
## Intended uses & limitations
```python
from transformers import pipeline
sentiment_model = pipeline(model="WhitePeak/bert-base-cased-Korean-sentiment")
sentiment_mode("매우 좋아")
```
Result:
```
LABEL_0: negative
LABEL_1: positive
```
## Training and evaluation data
## Training procedure
### Training hyperparameters
The following hyperparameters were used during training:
- learning_rate: 2e-05
- train_batch_size: 16
- eval_batch_size: 16
- seed: 42
- optimizer: Adam with betas=(0.9,0.999) and epsilon=1e-08
- lr_scheduler_type: linear
- num_epochs: 2
### Training results
### Framework versions
- Transformers 4.33.2
- Pytorch 2.0.1+cu118
- Datasets 2.14.5
- Tokenizers 0.13.3 |
ALBADDAWI/DeepCode-7B-Aurora-v13 | ALBADDAWI | "2024-04-13T16:09:41Z" | 975 | 0 | transformers | [
"transformers",
"safetensors",
"llama",
"text-generation",
"deepseek-ai/deepseek-math-7b-rl",
"conversational",
"base_model:deepseek-ai/deepseek-math-7b-rl",
"license:afl-3.0",
"autotrain_compatible",
"endpoints_compatible",
"text-generation-inference",
"region:us"
] | text-generation | "2024-04-11T16:21:04Z" | ---
tags:
- deepseek-ai/deepseek-math-7b-rl
base_model:
- deepseek-ai/deepseek-math-7b-rl
- deepseek-ai/deepseek-math-7b-rl
- deepseek-ai/deepseek-math-7b-rl
- deepseek-ai/deepseek-math-7b-rl
- deepseek-ai/deepseek-math-7b-rl
license: afl-3.0
---
# DeepCode-7B-Aurora-v13
DeepCode-7B-Aurora-v13 is a merge of the following models using [LazyMergekit](https://colab.research.google.com/drive/1obulZ1ROXHjYLn6PPZJwRR6GzgQogxxb?usp=sharing):
* [deepseek-ai/deepseek-math-7b-rl](https://huggingface.co/deepseek-ai/deepseek-math-7b-rl)
* [deepseek-ai/deepseek-math-7b-rl](https://huggingface.co/deepseek-ai/deepseek-math-7b-rl)
* [deepseek-ai/deepseek-math-7b-rl](https://huggingface.co/deepseek-ai/deepseek-math-7b-rl)
* [deepseek-ai/deepseek-math-7b-rl](https://huggingface.co/deepseek-ai/deepseek-math-7b-rl)
* [deepseek-ai/deepseek-math-7b-rl](https://huggingface.co/deepseek-ai/deepseek-math-7b-rl)
## 🧩 Configuration
```yaml
models:
- model: deepseek-ai/deepseek-math-7b-rl
# No parameters necessary for base model
- model: deepseek-ai/deepseek-math-7b-rl
parameters:
density: 0.66
weight: 0.2
- model: deepseek-ai/deepseek-math-7b-rl
parameters:
density: 0.55
weight: 0.2
- model: deepseek-ai/deepseek-math-7b-rl
parameters:
density: 0.55
weight: 0.2
- model: deepseek-ai/deepseek-math-7b-rl
parameters:
density: 0.44
weight: 0.2
- model: deepseek-ai/deepseek-math-7b-rl
parameters:
density: 0.66
weight: 0.2
merge_method: dare_ties
base_model: deepseek-ai/deepseek-math-7b-rl
parameters:
int8_mask: true
dtype: bfloat16
```
## 💻 Usage
```python
!pip install -qU transformers accelerate
from transformers import AutoTokenizer
import transformers
import torch
model = "ALBADDAWI/DeepCode-7B-Aurora-v13"
messages = [{"role": "user", "content": "What is a large language model?"}]
tokenizer = AutoTokenizer.from_pretrained(model)
prompt = tokenizer.apply_chat_template(messages, tokenize=False, add_generation_prompt=True)
pipeline = transformers.pipeline(
"text-generation",
model=model,
torch_dtype=torch.float16,
device_map="auto",
)
outputs = pipeline(prompt, max_new_tokens=256, do_sample=True, temperature=0.7, top_k=50, top_p=0.95)
print(outputs[0]["generated_text"])
``` |
Meina/MeinaMix | Meina | "2023-05-25T11:18:03Z" | 974 | 137 | diffusers | [
"diffusers",
"anime",
"art",
"stable diffusion",
"text-to-image",
"en",
"license:creativeml-openrail-m",
"region:us"
] | text-to-image | "2023-02-08T08:52:00Z" | ---
license: creativeml-openrail-m
language:
- en
tags:
- anime
- art
- stable diffusion
pipeline_tag: text-to-image
library_name: diffusers
---
MeinaMix Objective is to be able to do good art with little prompting.
* For examples and prompts, please checkout: https://civitai.com/models/7240/meinamix
I have a discord server where you can post images that you generated, discuss prompt and/or ask for help.
* https://discord.gg/XC9nGZNDUd
If you like one of my models and want to support their updates
* I've made a ko-fi page; https://ko-fi.com/meina where you can pay me a coffee <3
* And a Patreon page; https://www.patreon.com/MeinaMix where you can support me and get acess to beta of my models!
* You may also try this model using Sinkin.ai: https://sinkin.ai/m/vln8Nwr
* MeinaMix and the other of Meinas will ALWAYS be FREE.
* Recommendations of use:
Enable Quantization in K samplers.
Hires.fix is needed for prompts where the character is far away in order to make decent images, it drastically improve the quality of face and eyes!
Recommended parameters:
* Sampler: Euler a: 40 to 60 steps.
* Sampler: DPM++ SDE Karras: 30 to 60 steps.
* CFG Scale: 7.
* Resolutions: 512x768, 512x1024 for Portrait!
* Resolutions: 768x512, 1024x512, 1536x512 for Landscape!
* Hires.fix: R-ESRGAN 4x+Anime6b, with 10 steps at 0.1 up to 0.3 denoising.
* Clip Skip: 2.
* Negatives: ' (worst quality:2, low quality:2), (zombie, sketch, interlocked fingers, comic), '
|
sinkinai/Beautiful-Realistic-Asians-v5 | sinkinai | "2024-05-16T14:09:02Z" | 974 | 17 | diffusers | [
"diffusers",
"autotrain_compatible",
"endpoints_compatible",
"diffusers:StableDiffusionPipeline",
"region:us"
] | text-to-image | "2023-05-08T09:09:39Z" | You can run this model for free at: https://sinkin.ai/m/vlDnKP6
We offer API at low rates as well |
beowolx/CodeNinja-1.0-OpenChat-7B-GGUF | beowolx | "2023-12-22T21:11:54Z" | 974 | 14 | null | [
"gguf",
"code",
"text-generation-inference",
"text-generation",
"en",
"dataset:glaiveai/glaive-code-assistant-v2",
"dataset:TokenBender/code_instructions_122k_alpaca_style",
"license:mit",
"region:us"
] | text-generation | "2023-12-20T21:40:36Z" | ---
license: mit
datasets:
- glaiveai/glaive-code-assistant-v2
- TokenBender/code_instructions_122k_alpaca_style
language:
- en
metrics:
- code_eval
pipeline_tag: text-generation
tags:
- code
- text-generation-inference
---
<p align="center">
<img width="700px" alt="DeepSeek Coder" src="https://cdn-uploads.huggingface.co/production/uploads/64b566ab04fa6584c03b5247/5COagfF6EwrV4utZJ-ClI.png">
</p>
<hr>
# CodeNinja: Your Advanced Coding Assistant
## Overview
CodeNinja is an enhanced version of the renowned model [openchat/openchat-3.5-1210](https://huggingface.co/openchat/openchat-3.5-1210). It having been fine-tuned through Supervised Fine Tuning on two expansive datasets, encompassing over 400,000 coding instructions. Designed to be an indispensable tool for coders, CodeNinja aims to integrate seamlessly into your daily coding routine.
### Key Features
- **Expansive Training Database**: CodeNinja has been refined with datasets from [glaiveai/glaive-code-assistant-v2](https://huggingface.co/datasets/glaiveai/glaive-code-assistant-v2) and [TokenBender/code_instructions_122k_alpaca_style](https://huggingface.co/datasets/TokenBender/code_instructions_122k_alpaca_style), incorporating around 400,000 coding instructions across various languages including Python, C, C++, Rust, Java, JavaScript, and more.
- **Flexibility and Scalability**: Available in a 7B model size, CodeNinja is adaptable for local runtime environments.
- **Advanced Code Completion**: With a substantial context window size of 8192, it supports comprehensive project-level code completion.
## Prompt Format
CodeNinja maintains the same prompt structure as OpenChat 3.5. Effective utilization requires adherence to this format:
```
GPT4 Correct User: Hello<|end_of_turn|>GPT4 Correct Assistant: Hi<|end_of_turn|>GPT4 Correct User: How are you today?<|end_of_turn|>GPT4 Correct Assistant:
```
🚨 Important: Ensure the use of `<|end_of_turn|>` as the end-of-generation token.
**Adhering to this format is crucial for optimal results.**
## Usage Instructions
### Using LM Studio
The simplest way to engage with CodeNinja is via the [quantized versions](https://huggingface.co/beowolx/CodeNinja-1.0-OpenChat-7B-GGUF) on [LM Studio](https://lmstudio.ai/). Ensure you select the "OpenChat" preset, which incorporates the necessary prompt format. The preset is also available in this [gist](https://gist.github.com/beowolx/b219466681c02ff67baf8f313a3ad817).
### Using the Transformers Library
```python
from transformers import AutoTokenizer, AutoModelForCausalLM
import torch
# Initialize the model
model_path = "beowolx/CodeNinja-1.0-OpenChat-7B"
model = AutoModelForCausalLM.from_pretrained(model_path, device_map="auto")
# Load the OpenChat tokenizer
tokenizer = AutoTokenizer.from_pretrained("openchat/openchat-3.5-1210", use_fast=True)
def generate_one_completion(prompt: str):
messages = [
{"role": "user", "content": prompt},
{"role": "assistant", "content": ""} # Model response placeholder
]
# Generate token IDs using the chat template
input_ids = tokenizer.apply_chat_template(messages, add_generation_prompt=True)
# Produce completion
generate_ids = model.generate(
torch.tensor([input_ids]).to("cuda"),
max_length=256,
pad_token_id=tokenizer.pad_token_id,
eos_token_id=tokenizer.eos_token_id
)
# Process the completion
completion = tokenizer.decode(generate_ids[0], skip_special_tokens=True)
completion = completion.split("\n\n\n")[0].strip()
return completion
```
## License
CodeNinja is licensed under the MIT License, with model usage subject to the Model License.
## Contact
For queries or support, please open an issue in the repository. |
timm/pvt_v2_b0.in1k | timm | "2023-04-25T04:03:07Z" | 973 | 1 | timm | [
"timm",
"pytorch",
"safetensors",
"image-classification",
"dataset:imagenet-1k",
"arxiv:2106.13797",
"license:apache-2.0",
"region:us"
] | image-classification | "2023-04-25T04:03:01Z" | ---
tags:
- image-classification
- timm
library_name: timm
license: apache-2.0
datasets:
- imagenet-1k
---
# Model card for pvt_v2_b0
A PVT-v2 (Pyramid Vision Transformer) image classification model. Trained on ImageNet-1k by paper authors.
## Model Details
- **Model Type:** Image classification / feature backbone
- **Model Stats:**
- Params (M): 3.7
- GMACs: 0.6
- Activations (M): 8.0
- Image size: 224 x 224
- **Papers:**
- PVT v2: Improved Baselines with Pyramid Vision Transformer: https://arxiv.org/abs/2106.13797
- **Dataset:** ImageNet-1k
- **Original:** https://github.com/whai362/PVT
## Model Usage
### Image Classification
```python
from urllib.request import urlopen
from PIL import Image
import timm
img = Image.open(urlopen(
'https://huggingface.co/datasets/huggingface/documentation-images/resolve/main/beignets-task-guide.png'
))
model = timm.create_model('pvt_v2_b0', pretrained=True)
model = model.eval()
# get model specific transforms (normalization, resize)
data_config = timm.data.resolve_model_data_config(model)
transforms = timm.data.create_transform(**data_config, is_training=False)
output = model(transforms(img).unsqueeze(0)) # unsqueeze single image into batch of 1
top5_probabilities, top5_class_indices = torch.topk(output.softmax(dim=1) * 100, k=5)
```
### Feature Map Extraction
```python
from urllib.request import urlopen
from PIL import Image
import timm
img = Image.open(urlopen(
'https://huggingface.co/datasets/huggingface/documentation-images/resolve/main/beignets-task-guide.png'
))
model = timm.create_model(
'pvt_v2_b0',
pretrained=True,
features_only=True,
)
model = model.eval()
# get model specific transforms (normalization, resize)
data_config = timm.data.resolve_model_data_config(model)
transforms = timm.data.create_transform(**data_config, is_training=False)
output = model(transforms(img).unsqueeze(0)) # unsqueeze single image into batch of 1
for o in output:
# print shape of each feature map in output
# e.g.:
# torch.Size([1, 32, 56, 56])
# torch.Size([1, 64, 28, 28])
# torch.Size([1, 160, 14, 14])
# torch.Size([1, 256, 7, 7])
print(o.shape)
```
### Image Embeddings
```python
from urllib.request import urlopen
from PIL import Image
import timm
img = Image.open(urlopen(
'https://huggingface.co/datasets/huggingface/documentation-images/resolve/main/beignets-task-guide.png'
))
model = timm.create_model(
'pvt_v2_b0',
pretrained=True,
num_classes=0, # remove classifier nn.Linear
)
model = model.eval()
# get model specific transforms (normalization, resize)
data_config = timm.data.resolve_model_data_config(model)
transforms = timm.data.create_transform(**data_config, is_training=False)
output = model(transforms(img).unsqueeze(0)) # output is (batch_size, num_features) shaped tensor
# or equivalently (without needing to set num_classes=0)
output = model.forward_features(transforms(img).unsqueeze(0))
# output is unpooled, a (1, 256, 7, 7) shaped tensor
output = model.forward_head(output, pre_logits=True)
# output is a (1, num_features) shaped tensor
```
## Model Comparison
Explore the dataset and runtime metrics of this model in timm [model results](https://github.com/huggingface/pytorch-image-models/tree/main/results).
## Citation
```bibtex
@article{wang2021pvtv2,
title={Pvtv2: Improved baselines with pyramid vision transformer},
author={Wang, Wenhai and Xie, Enze and Li, Xiang and Fan, Deng-Ping and Song, Kaitao and Liang, Ding and Lu, Tong and Luo, Ping and Shao, Ling},
journal={Computational Visual Media},
volume={8},
number={3},
pages={1--10},
year={2022},
publisher={Springer}
}
```
|
andreas122001/roberta-academic-detector | andreas122001 | "2024-02-02T12:21:29Z" | 973 | 5 | transformers | [
"transformers",
"pytorch",
"safetensors",
"roberta",
"text-classification",
"mgt-detection",
"ai-detection",
"en",
"dataset:NicolaiSivesind/human-vs-machine",
"dataset:gfissore/arxiv-abstracts-2021",
"license:openrail",
"autotrain_compatible",
"endpoints_compatible",
"region:us"
] | text-classification | "2023-05-07T00:56:43Z" | ---
license: openrail
widget:
- text: I am totally a human, trust me bro.
example_title: default
- text: >-
In Finnish folklore, all places and things, and also human beings, have a
haltija (a genius, guardian spirit) of their own. One such haltija is called
etiäinen—an image, doppelgänger, or just an impression that goes ahead of a
person, doing things the person in question later does. For example, people
waiting at home might hear the door close or even see a shadow or a
silhouette, only to realize that no one has yet arrived. Etiäinen can also
refer to some kind of a feeling that something is going to happen. Sometimes
it could, for example, warn of a bad year coming. In modern Finnish, the
term has detached from its shamanistic origins and refers to premonition.
Unlike clairvoyance, divination, and similar practices, etiäiset (plural)
are spontaneous and can't be induced. Quite the opposite, they may be
unwanted and cause anxiety, like ghosts. Etiäiset need not be too dramatic
and may concern everyday events, although ones related to e.g. deaths are
common. As these phenomena are still reported today, they can be considered
a living tradition, as a way to explain the psychological experience of
premonition.
example_title: real wikipedia
- text: >-
In Finnish folklore, all places and things, animate or inanimate, have a
spirit or "etiäinen" that lives there. Etiäinen can manifest in many forms,
but is usually described as a kind, elderly woman with white hair. She is
the guardian of natural places and often helps people in need. Etiäinen has
been a part of Finnish culture for centuries and is still widely believed in
today. Folklorists study etiäinen to understand Finnish traditions and how
they have changed over time.
example_title: generated wikipedia
- text: >-
This paper presents a novel framework for sparsity-certifying graph
decompositions, which are important tools in various areas of computer
science, including algorithm design, complexity theory, and optimization.
Our approach is based on the concept of "cut sparsifiers," which are sparse
graphs that preserve the cut structure of the original graph up to a certain
error bound. We show that cut sparsifiers can be efficiently constructed
using a combination of spectral techniques and random sampling, and we use
them to develop new algorithms for decomposing graphs into sparse subgraphs.
example_title: from ChatGPT
- text: >-
Recent work has demonstrated substantial gains on many NLP tasks and
benchmarks by pre-training on a large corpus of text followed by fine-tuning
on a specific task. While typically task-agnostic in architecture, this
method still requires task-specific fine-tuning datasets of thousands or
tens of thousands of examples. By contrast, humans can generally perform a
new language task from only a few examples or from simple instructions -
something which current NLP systems still largely struggle to do. Here we
show that scaling up language models greatly improves task-agnostic,
few-shot performance, sometimes even reaching competitiveness with prior
state-of-the-art fine-tuning approaches. Specifically, we train GPT-3, an
autoregressive language model with 175 billion parameters, 10x more than any
previous non-sparse language model, and test its performance in the few-shot
setting. For all tasks, GPT-3 is applied without any gradient updates or
fine-tuning, with tasks and few-shot demonstrations specified purely via
text interaction with the model. GPT-3 achieves strong performance on many
NLP datasets, including translation, question-answering, and cloze tasks, as
well as several tasks that require on-the-fly reasoning or domain
adaptation, such as unscrambling words, using a novel word in a sentence, or
performing 3-digit arithmetic. At the same time, we also identify some
datasets where GPT-3's few-shot learning still struggles, as well as some
datasets where GPT-3 faces methodological issues related to training on
large web corpora. Finally, we find that GPT-3 can generate samples of news
articles which human evaluators have difficulty distinguishing from articles
written by humans. We discuss broader societal impacts of this finding and
of GPT-3 in general.
example_title: GPT-3 paper
datasets:
- NicolaiSivesind/human-vs-machine
- gfissore/arxiv-abstracts-2021
language:
- en
pipeline_tag: text-classification
tags:
- mgt-detection
- ai-detection
---
Machine-generated text-detection by fine-tuning of language models
===
This project is related to a bachelor's thesis with the title "*Turning Poachers into Gamekeepers: Detecting Machine-Generated Text in Academia using Large Language Models*" (see [here](https://ntnuopen.ntnu.no/ntnu-xmlui/handle/11250/3078096)) written by *Nicolai Thorer Sivesind* and *Andreas Bentzen Winje* at the *Department of Computer Science* at the *Norwegian University of Science and Technology*.
It contains text classification models trained to distinguish human-written text from text generated by language models like ChatGPT and GPT-3. The best models were able to achieve an accuracy of 100% on real and *GPT-3*-generated wikipedia articles (4500 samples), and an accuracy of 98.4% on real and *ChatGPT*-generated research abstracts (3000 samples).
The dataset card for the dataset that was created in relation to this project can be found [here](https://huggingface.co/datasets/NicolaiSivesind/human-vs-machine).
**NOTE**: the hosted inference on this site only works for the RoBERTa-models, and not for the Bloomz-models. The Bloomz-models otherwise can produce wrong predictions when not explicitly providing the attention mask from the tokenizer to the model for inference. To be sure, the [pipeline](https://huggingface.co/docs/transformers/main_classes/pipelines)-library seems to produce the most consistent results.
## Fine-tuned detectors
This project includes 12 fine-tuned models based on the RoBERTa-base model, and three sizes of the bloomz-models.
| Base-model | RoBERTa-base | Bloomz-560m | Bloomz-1b7 | Bloomz-3b |
|------------|--------------------------------------------------------------------------------|--------------------------------------------------------------------------------------------|------------------------------------------------------------------------------------------|----------------------------------------------------------------------------------------|
| Wiki | [roberta-wiki](https://huggingface.co/andreas122001/roberta-wiki-detector) | [Bloomz-560m-wiki](https://huggingface.co/andreas122001/bloomz-560m-wiki-detector) | [Bloomz-1b7-wiki](https://huggingface.co/andreas122001/bloomz-1b7-wiki-detector) | [Bloomz-3b-wiki](https://huggingface.co/andreas122001/bloomz-3b-wiki-detector) |
| Academic | [roberta-academic](https://huggingface.co/andreas122001/roberta-academic-detector) | [Bloomz-560m-academic](https://huggingface.co/andreas122001/bloomz-560m-academic-detector) | [Bloomz-1b7-academic](https://huggingface.co/andreas122001/bloomz-1b7-academic-detector) | [Bloomz-3b-academic](https://huggingface.co/andreas122001/bloomz-3b-academic-detector) |
| Mixed | [roberta-mixed](https://huggingface.co/andreas122001/roberta-mixed-detector) | [Bloomz-560m-mixed](https://huggingface.co/andreas122001/bloomz-560m-mixed-detector) | [Bloomz-1b7-mixed](https://huggingface.co/andreas122001/bloomz-1b7-mixed-detector) | [Bloomz-3b-mixed](https://huggingface.co/andreas122001/bloomz-3b-mixed-detector) |
### Datasets
The models were trained on selections from the [GPT-wiki-intros]() and [ChatGPT-Research-Abstracts](), and are separated into three types, **wiki**-detectors, **academic**-detectors and **mixed**-detectors, respectively.
- **Wiki-detectors**:
- Trained on 30'000 datapoints (10%) of GPT-wiki-intros.
- Best model (in-domain) is Bloomz-3b-wiki, with an accuracy of 100%.
- **Academic-detectors**:
- Trained on 20'000 datapoints (100%) of ChatGPT-Research-Abstracts.
- Best model (in-domain) is Bloomz-3b-academic, with an accuracy of 98.4%
- **Mixed-detectors**:
- Trained on 15'000 datapoints (5%) of GPT-wiki-intros and 10'000 datapoints (50%) of ChatGPT-Research-Abstracts.
- Best model (in-domain) is RoBERTa-mixed, with an F1-score of 99.3%.
### Hyperparameters
All models were trained using the same hyperparameters:
```python
{
"num_train_epochs": 1,
"adam_beta1": 0.9,
"adam_beta2": 0.999,
"batch_size": 8,
"adam_epsilon": 1e-08
"optim": "adamw_torch" # the optimizer (AdamW)
"learning_rate": 5e-05, # (LR)
"lr_scheduler_type": "linear", # scheduler type for LR
"seed": 42, # seed for PyTorch RNG-generator.
}
```
### Metrics
Metrics can be found at https://wandb.ai/idatt2900-072/IDATT2900-072.
In-domain performance of wiki-detectors:
| Base model | Accuracy | Precision | Recall | F1-score |
|-------------|----------|-----------|--------|----------|
| Bloomz-560m | 0.973 | *1.000 | 0.945 | 0.972 |
| Bloomz-1b7 | 0.972 | *1.000 | 0.945 | 0.972 |
| Bloomz-3b | *1.000 | *1.000 | *1.000 | *1.000 |
| RoBERTa | 0.998 | 0.999 | 0.997 | 0.998 |
In-domain peformance of academic-detectors:
| Base model | Accuracy | Precision | Recall | F1-score |
|-------------|----------|-----------|--------|----------|
| Bloomz-560m | 0.964 | 0.963 | 0.965 | 0.964 |
| Bloomz-1b7 | 0.946 | 0.941 | 0.951 | 0.946 |
| Bloomz-3b | *0.984 | *0.983 | 0.985 | *0.984 |
| RoBERTa | 0.982 | 0.968 | *0.997 | 0.982 |
F1-scores of the mixed-detectors on all three datasets:
| Base model | Mixed | Wiki | CRA |
|-------------|--------|--------|--------|
| Bloomz-560m | 0.948 | 0.972 | *0.848 |
| Bloomz-1b7 | 0.929 | 0.964 | 0.816 |
| Bloomz-3b | 0.988 | 0.996 | 0.772 |
| RoBERTa | *0.993 | *0.997 | 0.829 |
## Credits
- [GPT-wiki-intro](https://huggingface.co/datasets/aadityaubhat/GPT-wiki-intro), by Aaditya Bhat
- [arxiv-abstracts-2021](https://huggingface.co/datasets/gfissore/arxiv-abstracts-2021), by Giancarlo
- [Bloomz](bigscience/bloomz), by BigScience
- [RoBERTa](https://huggingface.co/roberta-base), by Liu et. al.
## Citation
Please use the following citation:
```
@misc {sivesind_2023,
author = { {Nicolai Thorer Sivesind} and {Andreas Bentzen Winje} },
title = { Machine-generated text-detection by fine-tuning of language models },
url = { https://huggingface.co/andreas122001/roberta-academic-detector }
year = 2023,
publisher = { Hugging Face }
}
``` |
TheBloke/vicuna-7B-v1.5-16K-GGUF | TheBloke | "2023-09-27T12:47:19Z" | 973 | 9 | transformers | [
"transformers",
"gguf",
"llama",
"arxiv:2307.09288",
"arxiv:2306.05685",
"base_model:lmsys/vicuna-7b-v1.5-16k",
"license:llama2",
"text-generation-inference",
"region:us"
] | null | "2023-09-05T04:00:37Z" | ---
license: llama2
model_name: Vicuna 7B v1.5 16K
base_model: lmsys/vicuna-7b-v1.5-16k
inference: false
model_creator: lmsys
model_type: llama
prompt_template: 'A chat between a curious user and an artificial intelligence assistant.
The assistant gives helpful, detailed, and polite answers to the user''s questions.
USER: {prompt} ASSISTANT:
'
quantized_by: TheBloke
---
<!-- header start -->
<!-- 200823 -->
<div style="width: auto; margin-left: auto; margin-right: auto">
<img src="https://i.imgur.com/EBdldam.jpg" alt="TheBlokeAI" style="width: 100%; min-width: 400px; display: block; margin: auto;">
</div>
<div style="display: flex; justify-content: space-between; width: 100%;">
<div style="display: flex; flex-direction: column; align-items: flex-start;">
<p style="margin-top: 0.5em; margin-bottom: 0em;"><a href="https://discord.gg/theblokeai">Chat & support: TheBloke's Discord server</a></p>
</div>
<div style="display: flex; flex-direction: column; align-items: flex-end;">
<p style="margin-top: 0.5em; margin-bottom: 0em;"><a href="https://www.patreon.com/TheBlokeAI">Want to contribute? TheBloke's Patreon page</a></p>
</div>
</div>
<div style="text-align:center; margin-top: 0em; margin-bottom: 0em"><p style="margin-top: 0.25em; margin-bottom: 0em;">TheBloke's LLM work is generously supported by a grant from <a href="https://a16z.com">andreessen horowitz (a16z)</a></p></div>
<hr style="margin-top: 1.0em; margin-bottom: 1.0em;">
<!-- header end -->
# Vicuna 7B v1.5 16K - GGUF
- Model creator: [lmsys](https://huggingface.co/lmsys)
- Original model: [Vicuna 7B v1.5 16K](https://huggingface.co/lmsys/vicuna-7b-v1.5-16k)
<!-- description start -->
## Description
This repo contains GGUF format model files for [lmsys's Vicuna 7B v1.5 16K](https://huggingface.co/lmsys/vicuna-7b-v1.5-16k).
<!-- description end -->
<!-- README_GGUF.md-about-gguf start -->
### About GGUF
GGUF is a new format introduced by the llama.cpp team on August 21st 2023. It is a replacement for GGML, which is no longer supported by llama.cpp. GGUF offers numerous advantages over GGML, such as better tokenisation, and support for special tokens. It is also supports metadata, and is designed to be extensible.
Here is an incomplate list of clients and libraries that are known to support GGUF:
* [llama.cpp](https://github.com/ggerganov/llama.cpp). The source project for GGUF. Offers a CLI and a server option.
* [text-generation-webui](https://github.com/oobabooga/text-generation-webui), the most widely used web UI, with many features and powerful extensions. Supports GPU acceleration.
* [KoboldCpp](https://github.com/LostRuins/koboldcpp), a fully featured web UI, with GPU accel across all platforms and GPU architectures. Especially good for story telling.
* [LM Studio](https://lmstudio.ai/), an easy-to-use and powerful local GUI for Windows and macOS (Silicon), with GPU acceleration.
* [LoLLMS Web UI](https://github.com/ParisNeo/lollms-webui), a great web UI with many interesting and unique features, including a full model library for easy model selection.
* [Faraday.dev](https://faraday.dev/), an attractive and easy to use character-based chat GUI for Windows and macOS (both Silicon and Intel), with GPU acceleration.
* [ctransformers](https://github.com/marella/ctransformers), a Python library with GPU accel, LangChain support, and OpenAI-compatible AI server.
* [llama-cpp-python](https://github.com/abetlen/llama-cpp-python), a Python library with GPU accel, LangChain support, and OpenAI-compatible API server.
* [candle](https://github.com/huggingface/candle), a Rust ML framework with a focus on performance, including GPU support, and ease of use.
<!-- README_GGUF.md-about-gguf end -->
<!-- repositories-available start -->
## Repositories available
* [AWQ model(s) for GPU inference.](https://huggingface.co/TheBloke/vicuna-7B-v1.5-16K-AWQ)
* [GPTQ models for GPU inference, with multiple quantisation parameter options.](https://huggingface.co/TheBloke/vicuna-7B-v1.5-16K-GPTQ)
* [2, 3, 4, 5, 6 and 8-bit GGUF models for CPU+GPU inference](https://huggingface.co/TheBloke/vicuna-7B-v1.5-16K-GGUF)
* [lmsys's original unquantised fp16 model in pytorch format, for GPU inference and for further conversions](https://huggingface.co/lmsys/vicuna-7b-v1.5-16k)
<!-- repositories-available end -->
<!-- prompt-template start -->
## Prompt template: Vicuna
```
A chat between a curious user and an artificial intelligence assistant. The assistant gives helpful, detailed, and polite answers to the user's questions. USER: {prompt} ASSISTANT:
```
<!-- prompt-template end -->
<!-- compatibility_gguf start -->
## Compatibility
These quantised GGUFv2 files are compatible with llama.cpp from August 27th onwards, as of commit [d0cee0d36d5be95a0d9088b674dbb27354107221](https://github.com/ggerganov/llama.cpp/commit/d0cee0d36d5be95a0d9088b674dbb27354107221)
They are also compatible with many third party UIs and libraries - please see the list at the top of this README.
## Explanation of quantisation methods
<details>
<summary>Click to see details</summary>
The new methods available are:
* GGML_TYPE_Q2_K - "type-1" 2-bit quantization in super-blocks containing 16 blocks, each block having 16 weight. Block scales and mins are quantized with 4 bits. This ends up effectively using 2.5625 bits per weight (bpw)
* GGML_TYPE_Q3_K - "type-0" 3-bit quantization in super-blocks containing 16 blocks, each block having 16 weights. Scales are quantized with 6 bits. This end up using 3.4375 bpw.
* GGML_TYPE_Q4_K - "type-1" 4-bit quantization in super-blocks containing 8 blocks, each block having 32 weights. Scales and mins are quantized with 6 bits. This ends up using 4.5 bpw.
* GGML_TYPE_Q5_K - "type-1" 5-bit quantization. Same super-block structure as GGML_TYPE_Q4_K resulting in 5.5 bpw
* GGML_TYPE_Q6_K - "type-0" 6-bit quantization. Super-blocks with 16 blocks, each block having 16 weights. Scales are quantized with 8 bits. This ends up using 6.5625 bpw
Refer to the Provided Files table below to see what files use which methods, and how.
</details>
<!-- compatibility_gguf end -->
<!-- README_GGUF.md-provided-files start -->
## Provided files
| Name | Quant method | Bits | Size | Max RAM required | Use case |
| ---- | ---- | ---- | ---- | ---- | ----- |
| [vicuna-7b-v1.5-16k.Q2_K.gguf](https://huggingface.co/TheBloke/vicuna-7B-v1.5-16K-GGUF/blob/main/vicuna-7b-v1.5-16k.Q2_K.gguf) | Q2_K | 2 | 2.83 GB| 5.33 GB | smallest, significant quality loss - not recommended for most purposes |
| [vicuna-7b-v1.5-16k.Q3_K_S.gguf](https://huggingface.co/TheBloke/vicuna-7B-v1.5-16K-GGUF/blob/main/vicuna-7b-v1.5-16k.Q3_K_S.gguf) | Q3_K_S | 3 | 2.95 GB| 5.45 GB | very small, high quality loss |
| [vicuna-7b-v1.5-16k.Q3_K_M.gguf](https://huggingface.co/TheBloke/vicuna-7B-v1.5-16K-GGUF/blob/main/vicuna-7b-v1.5-16k.Q3_K_M.gguf) | Q3_K_M | 3 | 3.30 GB| 5.80 GB | very small, high quality loss |
| [vicuna-7b-v1.5-16k.Q3_K_L.gguf](https://huggingface.co/TheBloke/vicuna-7B-v1.5-16K-GGUF/blob/main/vicuna-7b-v1.5-16k.Q3_K_L.gguf) | Q3_K_L | 3 | 3.60 GB| 6.10 GB | small, substantial quality loss |
| [vicuna-7b-v1.5-16k.Q4_0.gguf](https://huggingface.co/TheBloke/vicuna-7B-v1.5-16K-GGUF/blob/main/vicuna-7b-v1.5-16k.Q4_0.gguf) | Q4_0 | 4 | 3.83 GB| 6.33 GB | legacy; small, very high quality loss - prefer using Q3_K_M |
| [vicuna-7b-v1.5-16k.Q4_K_S.gguf](https://huggingface.co/TheBloke/vicuna-7B-v1.5-16K-GGUF/blob/main/vicuna-7b-v1.5-16k.Q4_K_S.gguf) | Q4_K_S | 4 | 3.86 GB| 6.36 GB | small, greater quality loss |
| [vicuna-7b-v1.5-16k.Q4_K_M.gguf](https://huggingface.co/TheBloke/vicuna-7B-v1.5-16K-GGUF/blob/main/vicuna-7b-v1.5-16k.Q4_K_M.gguf) | Q4_K_M | 4 | 4.08 GB| 6.58 GB | medium, balanced quality - recommended |
| [vicuna-7b-v1.5-16k.Q5_0.gguf](https://huggingface.co/TheBloke/vicuna-7B-v1.5-16K-GGUF/blob/main/vicuna-7b-v1.5-16k.Q5_0.gguf) | Q5_0 | 5 | 4.65 GB| 7.15 GB | legacy; medium, balanced quality - prefer using Q4_K_M |
| [vicuna-7b-v1.5-16k.Q5_K_S.gguf](https://huggingface.co/TheBloke/vicuna-7B-v1.5-16K-GGUF/blob/main/vicuna-7b-v1.5-16k.Q5_K_S.gguf) | Q5_K_S | 5 | 4.65 GB| 7.15 GB | large, low quality loss - recommended |
| [vicuna-7b-v1.5-16k.Q5_K_M.gguf](https://huggingface.co/TheBloke/vicuna-7B-v1.5-16K-GGUF/blob/main/vicuna-7b-v1.5-16k.Q5_K_M.gguf) | Q5_K_M | 5 | 4.78 GB| 7.28 GB | large, very low quality loss - recommended |
| [vicuna-7b-v1.5-16k.Q6_K.gguf](https://huggingface.co/TheBloke/vicuna-7B-v1.5-16K-GGUF/blob/main/vicuna-7b-v1.5-16k.Q6_K.gguf) | Q6_K | 6 | 5.53 GB| 8.03 GB | very large, extremely low quality loss |
| [vicuna-7b-v1.5-16k.Q8_0.gguf](https://huggingface.co/TheBloke/vicuna-7B-v1.5-16K-GGUF/blob/main/vicuna-7b-v1.5-16k.Q8_0.gguf) | Q8_0 | 8 | 7.16 GB| 9.66 GB | very large, extremely low quality loss - not recommended |
**Note**: the above RAM figures assume no GPU offloading. If layers are offloaded to the GPU, this will reduce RAM usage and use VRAM instead.
<!-- README_GGUF.md-provided-files end -->
<!-- README_GGUF.md-how-to-download start -->
## How to download GGUF files
**Note for manual downloaders:** You almost never want to clone the entire repo! Multiple different quantisation formats are provided, and most users only want to pick and download a single file.
The following clients/libraries will automatically download models for you, providing a list of available models to choose from:
- LM Studio
- LoLLMS Web UI
- Faraday.dev
### In `text-generation-webui`
Under Download Model, you can enter the model repo: TheBloke/vicuna-7B-v1.5-16K-GGUF and below it, a specific filename to download, such as: vicuna-7b-v1.5-16k.q4_K_M.gguf.
Then click Download.
### On the command line, including multiple files at once
I recommend using the `huggingface-hub` Python library:
```shell
pip3 install huggingface-hub>=0.17.1
```
Then you can download any individual model file to the current directory, at high speed, with a command like this:
```shell
huggingface-cli download TheBloke/vicuna-7B-v1.5-16K-GGUF vicuna-7b-v1.5-16k.q4_K_M.gguf --local-dir . --local-dir-use-symlinks False
```
<details>
<summary>More advanced huggingface-cli download usage</summary>
You can also download multiple files at once with a pattern:
```shell
huggingface-cli download TheBloke/vicuna-7B-v1.5-16K-GGUF --local-dir . --local-dir-use-symlinks False --include='*Q4_K*gguf'
```
For more documentation on downloading with `huggingface-cli`, please see: [HF -> Hub Python Library -> Download files -> Download from the CLI](https://huggingface.co/docs/huggingface_hub/guides/download#download-from-the-cli).
To accelerate downloads on fast connections (1Gbit/s or higher), install `hf_transfer`:
```shell
pip3 install hf_transfer
```
And set environment variable `HF_HUB_ENABLE_HF_TRANSFER` to `1`:
```shell
HUGGINGFACE_HUB_ENABLE_HF_TRANSFER=1 huggingface-cli download TheBloke/vicuna-7B-v1.5-16K-GGUF vicuna-7b-v1.5-16k.q4_K_M.gguf --local-dir . --local-dir-use-symlinks False
```
Windows CLI users: Use `set HUGGINGFACE_HUB_ENABLE_HF_TRANSFER=1` before running the download command.
</details>
<!-- README_GGUF.md-how-to-download end -->
<!-- README_GGUF.md-how-to-run start -->
## Example `llama.cpp` command
Make sure you are using `llama.cpp` from commit [d0cee0d36d5be95a0d9088b674dbb27354107221](https://github.com/ggerganov/llama.cpp/commit/d0cee0d36d5be95a0d9088b674dbb27354107221) or later.
```shell
./main -ngl 32 -m vicuna-7b-v1.5-16k.q4_K_M.gguf --color -c 4096 --temp 0.7 --repeat_penalty 1.1 -n -1 -p "A chat between a curious user and an artificial intelligence assistant. The assistant gives helpful, detailed, and polite answers to the user's questions. USER: {prompt} ASSISTANT:"
```
Change `-ngl 32` to the number of layers to offload to GPU. Remove it if you don't have GPU acceleration.
Change `-c 4096` to the desired sequence length. For extended sequence models - eg 8K, 16K, 32K - the necessary RoPE scaling parameters are read from the GGUF file and set by llama.cpp automatically.
If you want to have a chat-style conversation, replace the `-p <PROMPT>` argument with `-i -ins`
For other parameters and how to use them, please refer to [the llama.cpp documentation](https://github.com/ggerganov/llama.cpp/blob/master/examples/main/README.md)
## How to run in `text-generation-webui`
Further instructions here: [text-generation-webui/docs/llama.cpp.md](https://github.com/oobabooga/text-generation-webui/blob/main/docs/llama.cpp.md).
## How to run from Python code
You can use GGUF models from Python using the [llama-cpp-python](https://github.com/abetlen/llama-cpp-python) or [ctransformers](https://github.com/marella/ctransformers) libraries.
### How to load this model from Python using ctransformers
#### First install the package
```bash
# Base ctransformers with no GPU acceleration
pip install ctransformers>=0.2.24
# Or with CUDA GPU acceleration
pip install ctransformers[cuda]>=0.2.24
# Or with ROCm GPU acceleration
CT_HIPBLAS=1 pip install ctransformers>=0.2.24 --no-binary ctransformers
# Or with Metal GPU acceleration for macOS systems
CT_METAL=1 pip install ctransformers>=0.2.24 --no-binary ctransformers
```
#### Simple example code to load one of these GGUF models
```python
from ctransformers import AutoModelForCausalLM
# Set gpu_layers to the number of layers to offload to GPU. Set to 0 if no GPU acceleration is available on your system.
llm = AutoModelForCausalLM.from_pretrained("TheBloke/vicuna-7B-v1.5-16K-GGUF", model_file="vicuna-7b-v1.5-16k.q4_K_M.gguf", model_type="llama", gpu_layers=50)
print(llm("AI is going to"))
```
## How to use with LangChain
Here's guides on using llama-cpp-python or ctransformers with LangChain:
* [LangChain + llama-cpp-python](https://python.langchain.com/docs/integrations/llms/llamacpp)
* [LangChain + ctransformers](https://python.langchain.com/docs/integrations/providers/ctransformers)
<!-- README_GGUF.md-how-to-run end -->
<!-- footer start -->
<!-- 200823 -->
## Discord
For further support, and discussions on these models and AI in general, join us at:
[TheBloke AI's Discord server](https://discord.gg/theblokeai)
## Thanks, and how to contribute
Thanks to the [chirper.ai](https://chirper.ai) team!
Thanks to Clay from [gpus.llm-utils.org](llm-utils)!
I've had a lot of people ask if they can contribute. I enjoy providing models and helping people, and would love to be able to spend even more time doing it, as well as expanding into new projects like fine tuning/training.
If you're able and willing to contribute it will be most gratefully received and will help me to keep providing more models, and to start work on new AI projects.
Donaters will get priority support on any and all AI/LLM/model questions and requests, access to a private Discord room, plus other benefits.
* Patreon: https://patreon.com/TheBlokeAI
* Ko-Fi: https://ko-fi.com/TheBlokeAI
**Special thanks to**: Aemon Algiz.
**Patreon special mentions**: Alicia Loh, Stephen Murray, K, Ajan Kanaga, RoA, Magnesian, Deo Leter, Olakabola, Eugene Pentland, zynix, Deep Realms, Raymond Fosdick, Elijah Stavena, Iucharbius, Erik Bjäreholt, Luis Javier Navarrete Lozano, Nicholas, theTransient, John Detwiler, alfie_i, knownsqashed, Mano Prime, Willem Michiel, Enrico Ros, LangChain4j, OG, Michael Dempsey, Pierre Kircher, Pedro Madruga, James Bentley, Thomas Belote, Luke @flexchar, Leonard Tan, Johann-Peter Hartmann, Illia Dulskyi, Fen Risland, Chadd, S_X, Jeff Scroggin, Ken Nordquist, Sean Connelly, Artur Olbinski, Swaroop Kallakuri, Jack West, Ai Maven, David Ziegler, Russ Johnson, transmissions 11, John Villwock, Alps Aficionado, Clay Pascal, Viktor Bowallius, Subspace Studios, Rainer Wilmers, Trenton Dambrowitz, vamX, Michael Levine, 준교 김, Brandon Frisco, Kalila, Trailburnt, Randy H, Talal Aujan, Nathan Dryer, Vadim, 阿明, ReadyPlayerEmma, Tiffany J. Kim, George Stoitzev, Spencer Kim, Jerry Meng, Gabriel Tamborski, Cory Kujawski, Jeffrey Morgan, Spiking Neurons AB, Edmond Seymore, Alexandros Triantafyllidis, Lone Striker, Cap'n Zoog, Nikolai Manek, danny, ya boyyy, Derek Yates, usrbinkat, Mandus, TL, Nathan LeClaire, subjectnull, Imad Khwaja, webtim, Raven Klaugh, Asp the Wyvern, Gabriel Puliatti, Caitlyn Gatomon, Joseph William Delisle, Jonathan Leane, Luke Pendergrass, SuperWojo, Sebastain Graf, Will Dee, Fred von Graf, Andrey, Dan Guido, Daniel P. Andersen, Nitin Borwankar, Elle, Vitor Caleffi, biorpg, jjj, NimbleBox.ai, Pieter, Matthew Berman, terasurfer, Michael Davis, Alex, Stanislav Ovsiannikov
Thank you to all my generous patrons and donaters!
And thank you again to a16z for their generous grant.
<!-- footer end -->
<!-- original-model-card start -->
# Original model card: lmsys's Vicuna 7B v1.5 16K
# Vicuna Model Card
## Model Details
Vicuna is a chat assistant trained by fine-tuning Llama 2 on user-shared conversations collected from ShareGPT.
- **Developed by:** [LMSYS](https://lmsys.org/)
- **Model type:** An auto-regressive language model based on the transformer architecture
- **License:** Llama 2 Community License Agreement
- **Finetuned from model:** [Llama 2](https://arxiv.org/abs/2307.09288)
### Model Sources
- **Repository:** https://github.com/lm-sys/FastChat
- **Blog:** https://lmsys.org/blog/2023-03-30-vicuna/
- **Paper:** https://arxiv.org/abs/2306.05685
- **Demo:** https://chat.lmsys.org/
## Uses
The primary use of Vicuna is research on large language models and chatbots.
The primary intended users of the model are researchers and hobbyists in natural language processing, machine learning, and artificial intelligence.
## How to Get Started with the Model
- Command line interface: https://github.com/lm-sys/FastChat#vicuna-weights
- APIs (OpenAI API, Huggingface API): https://github.com/lm-sys/FastChat/tree/main#api
## Training Details
Vicuna v1.5 (16k) is fine-tuned from Llama 2 with supervised instruction fine-tuning and linear RoPE scaling.
The training data is around 125K conversations collected from ShareGPT.com. These conversations are packed into sequences that contain 16K tokens each.
See more details in the "Training Details of Vicuna Models" section in the appendix of this [paper](https://arxiv.org/pdf/2306.05685.pdf).
## Evaluation
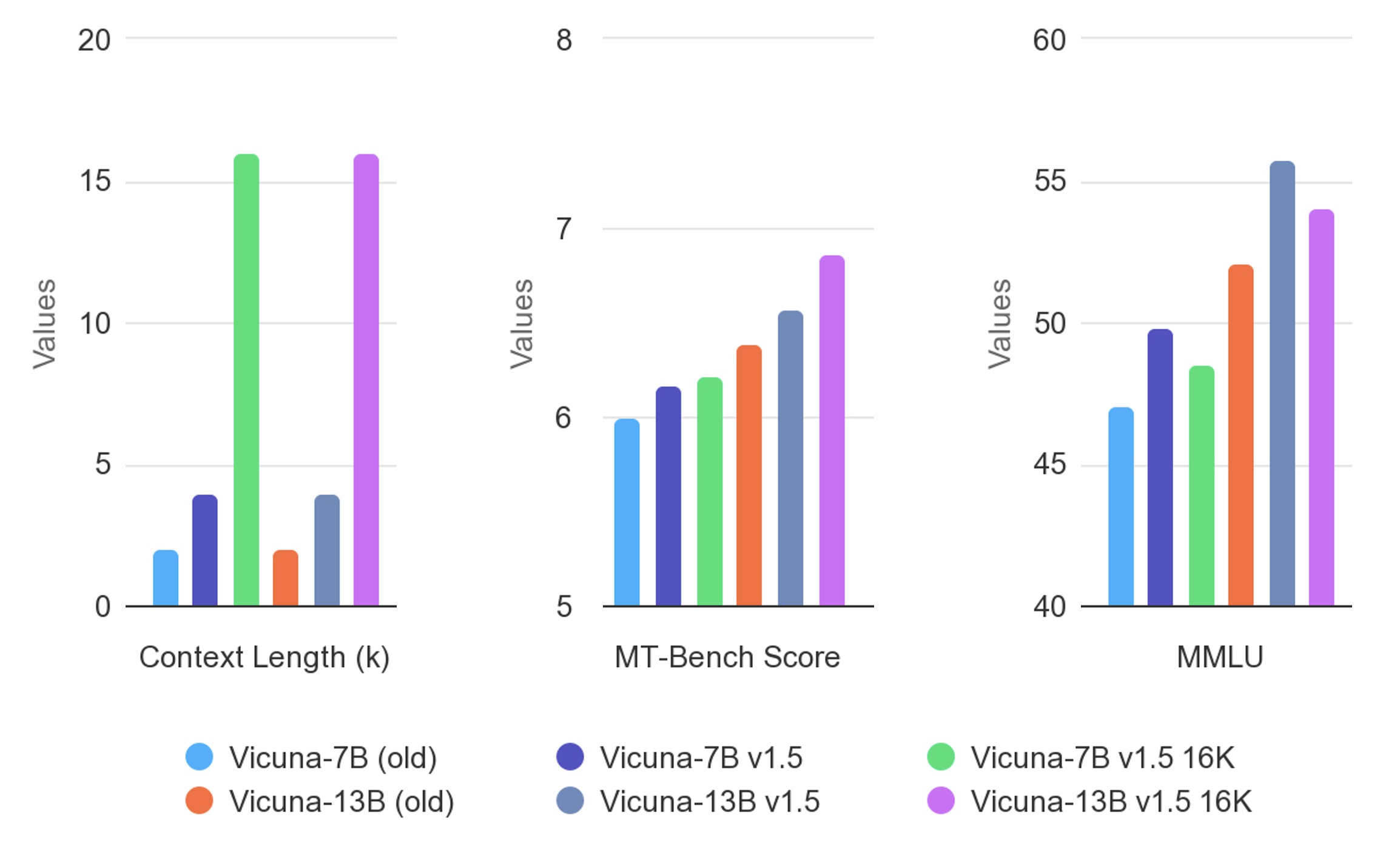
Vicuna is evaluated with standard benchmarks, human preference, and LLM-as-a-judge. See more details in this [paper](https://arxiv.org/pdf/2306.05685.pdf) and [leaderboard](https://huggingface.co/spaces/lmsys/chatbot-arena-leaderboard).
## Difference between different versions of Vicuna
See [vicuna_weights_version.md](https://github.com/lm-sys/FastChat/blob/main/docs/vicuna_weights_version.md)
<!-- original-model-card end -->
|
TheBloke/MLewdBoros-L2-13B-GGUF | TheBloke | "2023-09-27T12:48:42Z" | 972 | 13 | transformers | [
"transformers",
"gguf",
"llama",
"not-for-all-audiences",
"nsfw",
"base_model:Undi95/MLewdBoros-L2-13B",
"license:cc-by-nc-4.0",
"text-generation-inference",
"region:us"
] | null | "2023-09-10T20:52:27Z" | ---
license: cc-by-nc-4.0
tags:
- not-for-all-audiences
- nsfw
model_name: MLewdBoros L2 13B
base_model: Undi95/MLewdBoros-L2-13B
inference: false
model_creator: Undi95
model_type: llama
prompt_template: 'Below is an instruction that describes a task. Write a response
that appropriately completes the request.
### Instruction:
{prompt}
### Response:
'
quantized_by: TheBloke
---
<!-- header start -->
<!-- 200823 -->
<div style="width: auto; margin-left: auto; margin-right: auto">
<img src="https://i.imgur.com/EBdldam.jpg" alt="TheBlokeAI" style="width: 100%; min-width: 400px; display: block; margin: auto;">
</div>
<div style="display: flex; justify-content: space-between; width: 100%;">
<div style="display: flex; flex-direction: column; align-items: flex-start;">
<p style="margin-top: 0.5em; margin-bottom: 0em;"><a href="https://discord.gg/theblokeai">Chat & support: TheBloke's Discord server</a></p>
</div>
<div style="display: flex; flex-direction: column; align-items: flex-end;">
<p style="margin-top: 0.5em; margin-bottom: 0em;"><a href="https://www.patreon.com/TheBlokeAI">Want to contribute? TheBloke's Patreon page</a></p>
</div>
</div>
<div style="text-align:center; margin-top: 0em; margin-bottom: 0em"><p style="margin-top: 0.25em; margin-bottom: 0em;">TheBloke's LLM work is generously supported by a grant from <a href="https://a16z.com">andreessen horowitz (a16z)</a></p></div>
<hr style="margin-top: 1.0em; margin-bottom: 1.0em;">
<!-- header end -->
# MLewdBoros L2 13B - GGUF
- Model creator: [Undi95](https://huggingface.co/Undi95)
- Original model: [MLewdBoros L2 13B](https://huggingface.co/Undi95/MLewdBoros-L2-13B)
<!-- description start -->
## Description
This repo contains GGUF format model files for [Undi95's MLewdBoros L2 13B](https://huggingface.co/Undi95/MLewdBoros-L2-13B).
<!-- description end -->
<!-- README_GGUF.md-about-gguf start -->
### About GGUF
GGUF is a new format introduced by the llama.cpp team on August 21st 2023. It is a replacement for GGML, which is no longer supported by llama.cpp. GGUF offers numerous advantages over GGML, such as better tokenisation, and support for special tokens. It is also supports metadata, and is designed to be extensible.
Here is an incomplate list of clients and libraries that are known to support GGUF:
* [llama.cpp](https://github.com/ggerganov/llama.cpp). The source project for GGUF. Offers a CLI and a server option.
* [text-generation-webui](https://github.com/oobabooga/text-generation-webui), the most widely used web UI, with many features and powerful extensions. Supports GPU acceleration.
* [KoboldCpp](https://github.com/LostRuins/koboldcpp), a fully featured web UI, with GPU accel across all platforms and GPU architectures. Especially good for story telling.
* [LM Studio](https://lmstudio.ai/), an easy-to-use and powerful local GUI for Windows and macOS (Silicon), with GPU acceleration.
* [LoLLMS Web UI](https://github.com/ParisNeo/lollms-webui), a great web UI with many interesting and unique features, including a full model library for easy model selection.
* [Faraday.dev](https://faraday.dev/), an attractive and easy to use character-based chat GUI for Windows and macOS (both Silicon and Intel), with GPU acceleration.
* [ctransformers](https://github.com/marella/ctransformers), a Python library with GPU accel, LangChain support, and OpenAI-compatible AI server.
* [llama-cpp-python](https://github.com/abetlen/llama-cpp-python), a Python library with GPU accel, LangChain support, and OpenAI-compatible API server.
* [candle](https://github.com/huggingface/candle), a Rust ML framework with a focus on performance, including GPU support, and ease of use.
<!-- README_GGUF.md-about-gguf end -->
<!-- repositories-available start -->
## Repositories available
* [AWQ model(s) for GPU inference.](https://huggingface.co/TheBloke/MLewdBoros-L2-13B-AWQ)
* [GPTQ models for GPU inference, with multiple quantisation parameter options.](https://huggingface.co/TheBloke/MLewdBoros-L2-13B-GPTQ)
* [2, 3, 4, 5, 6 and 8-bit GGUF models for CPU+GPU inference](https://huggingface.co/TheBloke/MLewdBoros-L2-13B-GGUF)
* [Undi95's original unquantised fp16 model in pytorch format, for GPU inference and for further conversions](https://huggingface.co/Undi95/MLewdBoros-L2-13B)
<!-- repositories-available end -->
<!-- prompt-template start -->
## Prompt template: Alpaca
```
Below is an instruction that describes a task. Write a response that appropriately completes the request.
### Instruction:
{prompt}
### Response:
```
<!-- prompt-template end -->
<!-- licensing start -->
## Licensing
The creator of the source model has listed its license as `cc-by-nc-4.0`, and this quantization has therefore used that same license.
As this model is based on Llama 2, it is also subject to the Meta Llama 2 license terms, and the license files for that are additionally included. It should therefore be considered as being claimed to be licensed under both licenses. I contacted Hugging Face for clarification on dual licensing but they do not yet have an official position. Should this change, or should Meta provide any feedback on this situation, I will update this section accordingly.
In the meantime, any questions regarding licensing, and in particular how these two licenses might interact, should be directed to the original model repository: [Undi95's MLewdBoros L2 13B](https://huggingface.co/Undi95/MLewdBoros-L2-13B).
<!-- licensing end -->
<!-- compatibility_gguf start -->
## Compatibility
These quantised GGUFv2 files are compatible with llama.cpp from August 27th onwards, as of commit [d0cee0d36d5be95a0d9088b674dbb27354107221](https://github.com/ggerganov/llama.cpp/commit/d0cee0d36d5be95a0d9088b674dbb27354107221)
They are also compatible with many third party UIs and libraries - please see the list at the top of this README.
## Explanation of quantisation methods
<details>
<summary>Click to see details</summary>
The new methods available are:
* GGML_TYPE_Q2_K - "type-1" 2-bit quantization in super-blocks containing 16 blocks, each block having 16 weight. Block scales and mins are quantized with 4 bits. This ends up effectively using 2.5625 bits per weight (bpw)
* GGML_TYPE_Q3_K - "type-0" 3-bit quantization in super-blocks containing 16 blocks, each block having 16 weights. Scales are quantized with 6 bits. This end up using 3.4375 bpw.
* GGML_TYPE_Q4_K - "type-1" 4-bit quantization in super-blocks containing 8 blocks, each block having 32 weights. Scales and mins are quantized with 6 bits. This ends up using 4.5 bpw.
* GGML_TYPE_Q5_K - "type-1" 5-bit quantization. Same super-block structure as GGML_TYPE_Q4_K resulting in 5.5 bpw
* GGML_TYPE_Q6_K - "type-0" 6-bit quantization. Super-blocks with 16 blocks, each block having 16 weights. Scales are quantized with 8 bits. This ends up using 6.5625 bpw
Refer to the Provided Files table below to see what files use which methods, and how.
</details>
<!-- compatibility_gguf end -->
<!-- README_GGUF.md-provided-files start -->
## Provided files
| Name | Quant method | Bits | Size | Max RAM required | Use case |
| ---- | ---- | ---- | ---- | ---- | ----- |
| [mlewdboros-l2-13b.Q2_K.gguf](https://huggingface.co/TheBloke/MLewdBoros-L2-13B-GGUF/blob/main/mlewdboros-l2-13b.Q2_K.gguf) | Q2_K | 2 | 5.43 GB| 7.93 GB | smallest, significant quality loss - not recommended for most purposes |
| [mlewdboros-l2-13b.Q3_K_S.gguf](https://huggingface.co/TheBloke/MLewdBoros-L2-13B-GGUF/blob/main/mlewdboros-l2-13b.Q3_K_S.gguf) | Q3_K_S | 3 | 5.66 GB| 8.16 GB | very small, high quality loss |
| [mlewdboros-l2-13b.Q3_K_M.gguf](https://huggingface.co/TheBloke/MLewdBoros-L2-13B-GGUF/blob/main/mlewdboros-l2-13b.Q3_K_M.gguf) | Q3_K_M | 3 | 6.34 GB| 8.84 GB | very small, high quality loss |
| [mlewdboros-l2-13b.Q3_K_L.gguf](https://huggingface.co/TheBloke/MLewdBoros-L2-13B-GGUF/blob/main/mlewdboros-l2-13b.Q3_K_L.gguf) | Q3_K_L | 3 | 6.93 GB| 9.43 GB | small, substantial quality loss |
| [mlewdboros-l2-13b.Q4_0.gguf](https://huggingface.co/TheBloke/MLewdBoros-L2-13B-GGUF/blob/main/mlewdboros-l2-13b.Q4_0.gguf) | Q4_0 | 4 | 7.37 GB| 9.87 GB | legacy; small, very high quality loss - prefer using Q3_K_M |
| [mlewdboros-l2-13b.Q4_K_S.gguf](https://huggingface.co/TheBloke/MLewdBoros-L2-13B-GGUF/blob/main/mlewdboros-l2-13b.Q4_K_S.gguf) | Q4_K_S | 4 | 7.41 GB| 9.91 GB | small, greater quality loss |
| [mlewdboros-l2-13b.Q4_K_M.gguf](https://huggingface.co/TheBloke/MLewdBoros-L2-13B-GGUF/blob/main/mlewdboros-l2-13b.Q4_K_M.gguf) | Q4_K_M | 4 | 7.87 GB| 10.37 GB | medium, balanced quality - recommended |
| [mlewdboros-l2-13b.Q5_0.gguf](https://huggingface.co/TheBloke/MLewdBoros-L2-13B-GGUF/blob/main/mlewdboros-l2-13b.Q5_0.gguf) | Q5_0 | 5 | 8.97 GB| 11.47 GB | legacy; medium, balanced quality - prefer using Q4_K_M |
| [mlewdboros-l2-13b.Q5_K_S.gguf](https://huggingface.co/TheBloke/MLewdBoros-L2-13B-GGUF/blob/main/mlewdboros-l2-13b.Q5_K_S.gguf) | Q5_K_S | 5 | 8.97 GB| 11.47 GB | large, low quality loss - recommended |
| [mlewdboros-l2-13b.Q5_K_M.gguf](https://huggingface.co/TheBloke/MLewdBoros-L2-13B-GGUF/blob/main/mlewdboros-l2-13b.Q5_K_M.gguf) | Q5_K_M | 5 | 9.23 GB| 11.73 GB | large, very low quality loss - recommended |
| [mlewdboros-l2-13b.Q6_K.gguf](https://huggingface.co/TheBloke/MLewdBoros-L2-13B-GGUF/blob/main/mlewdboros-l2-13b.Q6_K.gguf) | Q6_K | 6 | 10.68 GB| 13.18 GB | very large, extremely low quality loss |
| [mlewdboros-l2-13b.Q8_0.gguf](https://huggingface.co/TheBloke/MLewdBoros-L2-13B-GGUF/blob/main/mlewdboros-l2-13b.Q8_0.gguf) | Q8_0 | 8 | 13.83 GB| 16.33 GB | very large, extremely low quality loss - not recommended |
**Note**: the above RAM figures assume no GPU offloading. If layers are offloaded to the GPU, this will reduce RAM usage and use VRAM instead.
<!-- README_GGUF.md-provided-files end -->
<!-- README_GGUF.md-how-to-download start -->
## How to download GGUF files
**Note for manual downloaders:** You almost never want to clone the entire repo! Multiple different quantisation formats are provided, and most users only want to pick and download a single file.
The following clients/libraries will automatically download models for you, providing a list of available models to choose from:
- LM Studio
- LoLLMS Web UI
- Faraday.dev
### In `text-generation-webui`
Under Download Model, you can enter the model repo: TheBloke/MLewdBoros-L2-13B-GGUF and below it, a specific filename to download, such as: mlewdboros-l2-13b.q4_K_M.gguf.
Then click Download.
### On the command line, including multiple files at once
I recommend using the `huggingface-hub` Python library:
```shell
pip3 install huggingface-hub>=0.17.1
```
Then you can download any individual model file to the current directory, at high speed, with a command like this:
```shell
huggingface-cli download TheBloke/MLewdBoros-L2-13B-GGUF mlewdboros-l2-13b.q4_K_M.gguf --local-dir . --local-dir-use-symlinks False
```
<details>
<summary>More advanced huggingface-cli download usage</summary>
You can also download multiple files at once with a pattern:
```shell
huggingface-cli download TheBloke/MLewdBoros-L2-13B-GGUF --local-dir . --local-dir-use-symlinks False --include='*Q4_K*gguf'
```
For more documentation on downloading with `huggingface-cli`, please see: [HF -> Hub Python Library -> Download files -> Download from the CLI](https://huggingface.co/docs/huggingface_hub/guides/download#download-from-the-cli).
To accelerate downloads on fast connections (1Gbit/s or higher), install `hf_transfer`:
```shell
pip3 install hf_transfer
```
And set environment variable `HF_HUB_ENABLE_HF_TRANSFER` to `1`:
```shell
HUGGINGFACE_HUB_ENABLE_HF_TRANSFER=1 huggingface-cli download TheBloke/MLewdBoros-L2-13B-GGUF mlewdboros-l2-13b.q4_K_M.gguf --local-dir . --local-dir-use-symlinks False
```
Windows CLI users: Use `set HUGGINGFACE_HUB_ENABLE_HF_TRANSFER=1` before running the download command.
</details>
<!-- README_GGUF.md-how-to-download end -->
<!-- README_GGUF.md-how-to-run start -->
## Example `llama.cpp` command
Make sure you are using `llama.cpp` from commit [d0cee0d36d5be95a0d9088b674dbb27354107221](https://github.com/ggerganov/llama.cpp/commit/d0cee0d36d5be95a0d9088b674dbb27354107221) or later.
```shell
./main -ngl 32 -m mlewdboros-l2-13b.q4_K_M.gguf --color -c 4096 --temp 0.7 --repeat_penalty 1.1 -n -1 -p "Below is an instruction that describes a task. Write a response that appropriately completes the request.\n\n### Instruction:\n{prompt}\n\n### Response:"
```
Change `-ngl 32` to the number of layers to offload to GPU. Remove it if you don't have GPU acceleration.
Change `-c 4096` to the desired sequence length. For extended sequence models - eg 8K, 16K, 32K - the necessary RoPE scaling parameters are read from the GGUF file and set by llama.cpp automatically.
If you want to have a chat-style conversation, replace the `-p <PROMPT>` argument with `-i -ins`
For other parameters and how to use them, please refer to [the llama.cpp documentation](https://github.com/ggerganov/llama.cpp/blob/master/examples/main/README.md)
## How to run in `text-generation-webui`
Further instructions here: [text-generation-webui/docs/llama.cpp.md](https://github.com/oobabooga/text-generation-webui/blob/main/docs/llama.cpp.md).
## How to run from Python code
You can use GGUF models from Python using the [llama-cpp-python](https://github.com/abetlen/llama-cpp-python) or [ctransformers](https://github.com/marella/ctransformers) libraries.
### How to load this model from Python using ctransformers
#### First install the package
```bash
# Base ctransformers with no GPU acceleration
pip install ctransformers>=0.2.24
# Or with CUDA GPU acceleration
pip install ctransformers[cuda]>=0.2.24
# Or with ROCm GPU acceleration
CT_HIPBLAS=1 pip install ctransformers>=0.2.24 --no-binary ctransformers
# Or with Metal GPU acceleration for macOS systems
CT_METAL=1 pip install ctransformers>=0.2.24 --no-binary ctransformers
```
#### Simple example code to load one of these GGUF models
```python
from ctransformers import AutoModelForCausalLM
# Set gpu_layers to the number of layers to offload to GPU. Set to 0 if no GPU acceleration is available on your system.
llm = AutoModelForCausalLM.from_pretrained("TheBloke/MLewdBoros-L2-13B-GGUF", model_file="mlewdboros-l2-13b.q4_K_M.gguf", model_type="llama", gpu_layers=50)
print(llm("AI is going to"))
```
## How to use with LangChain
Here's guides on using llama-cpp-python or ctransformers with LangChain:
* [LangChain + llama-cpp-python](https://python.langchain.com/docs/integrations/llms/llamacpp)
* [LangChain + ctransformers](https://python.langchain.com/docs/integrations/providers/ctransformers)
<!-- README_GGUF.md-how-to-run end -->
<!-- footer start -->
<!-- 200823 -->
## Discord
For further support, and discussions on these models and AI in general, join us at:
[TheBloke AI's Discord server](https://discord.gg/theblokeai)
## Thanks, and how to contribute
Thanks to the [chirper.ai](https://chirper.ai) team!
Thanks to Clay from [gpus.llm-utils.org](llm-utils)!
I've had a lot of people ask if they can contribute. I enjoy providing models and helping people, and would love to be able to spend even more time doing it, as well as expanding into new projects like fine tuning/training.
If you're able and willing to contribute it will be most gratefully received and will help me to keep providing more models, and to start work on new AI projects.
Donaters will get priority support on any and all AI/LLM/model questions and requests, access to a private Discord room, plus other benefits.
* Patreon: https://patreon.com/TheBlokeAI
* Ko-Fi: https://ko-fi.com/TheBlokeAI
**Special thanks to**: Aemon Algiz.
**Patreon special mentions**: Alicia Loh, Stephen Murray, K, Ajan Kanaga, RoA, Magnesian, Deo Leter, Olakabola, Eugene Pentland, zynix, Deep Realms, Raymond Fosdick, Elijah Stavena, Iucharbius, Erik Bjäreholt, Luis Javier Navarrete Lozano, Nicholas, theTransient, John Detwiler, alfie_i, knownsqashed, Mano Prime, Willem Michiel, Enrico Ros, LangChain4j, OG, Michael Dempsey, Pierre Kircher, Pedro Madruga, James Bentley, Thomas Belote, Luke @flexchar, Leonard Tan, Johann-Peter Hartmann, Illia Dulskyi, Fen Risland, Chadd, S_X, Jeff Scroggin, Ken Nordquist, Sean Connelly, Artur Olbinski, Swaroop Kallakuri, Jack West, Ai Maven, David Ziegler, Russ Johnson, transmissions 11, John Villwock, Alps Aficionado, Clay Pascal, Viktor Bowallius, Subspace Studios, Rainer Wilmers, Trenton Dambrowitz, vamX, Michael Levine, 준교 김, Brandon Frisco, Kalila, Trailburnt, Randy H, Talal Aujan, Nathan Dryer, Vadim, 阿明, ReadyPlayerEmma, Tiffany J. Kim, George Stoitzev, Spencer Kim, Jerry Meng, Gabriel Tamborski, Cory Kujawski, Jeffrey Morgan, Spiking Neurons AB, Edmond Seymore, Alexandros Triantafyllidis, Lone Striker, Cap'n Zoog, Nikolai Manek, danny, ya boyyy, Derek Yates, usrbinkat, Mandus, TL, Nathan LeClaire, subjectnull, Imad Khwaja, webtim, Raven Klaugh, Asp the Wyvern, Gabriel Puliatti, Caitlyn Gatomon, Joseph William Delisle, Jonathan Leane, Luke Pendergrass, SuperWojo, Sebastain Graf, Will Dee, Fred von Graf, Andrey, Dan Guido, Daniel P. Andersen, Nitin Borwankar, Elle, Vitor Caleffi, biorpg, jjj, NimbleBox.ai, Pieter, Matthew Berman, terasurfer, Michael Davis, Alex, Stanislav Ovsiannikov
Thank you to all my generous patrons and donaters!
And thank you again to a16z for their generous grant.
<!-- footer end -->
<!-- original-model-card start -->
# Original model card: Undi95's MLewdBoros L2 13B

THIS MODEL IS MADE FOR LEWD
SEXUAL, CRUDE AND KINKY CONTENT IN OUTPUT CAN AND WILL HAPPEN. YOU'RE WARNED
SuperCOT applied : https://huggingface.co/Undi95/MLewdBoros-L2-13B-SuperCOT
<!-- description start -->
## Description
This repo contains fp16 files of MLewdBoros, very hot and lewd model based on ReMM and merged with SpicyBoros 2.2.
<!-- description end -->
<!-- description start -->
## Models and loras used
- Undi95/ReMM-S-Light (base/private)
- Undi95/CreativeEngine
- Brouz/Slerpeno
- The-Face-Of-Goonery/Huginn-v3-13b
- zattio770/120-Days-of-LORA-v2-13B
- PygmalionAI/pygmalion-2-13b
- Undi95/StoryTelling
- TokenBender/sakhi_13B_roleplayer_NSFW_chat_adapter
- nRuaif/Kimiko-v2-13B
- jondurbin/spicyboros-13b-2.2
<!-- description end -->
<!-- prompt-template start -->
## Prompt template: Alpaca
```
Below is an instruction that describes a task. Write a response that appropriately completes the request.
### Instruction:
{prompt}
### Response:
```
Special thanks to Sushi and Shena ♥
<!-- original-model-card end -->
|
Niggendar/atomixPonyXL_v10TurboDPMSDE | Niggendar | "2024-04-21T19:31:49Z" | 972 | 1 | diffusers | [
"diffusers",
"safetensors",
"arxiv:1910.09700",
"autotrain_compatible",
"endpoints_compatible",
"diffusers:StableDiffusionXLPipeline",
"region:us"
] | text-to-image | "2024-04-21T19:26:35Z" | ---
library_name: diffusers
---
# Model Card for Model ID
<!-- Provide a quick summary of what the model is/does. -->
## Model Details
### Model Description
<!-- Provide a longer summary of what this model is. -->
This is the model card of a 🧨 diffusers model that has been pushed on the Hub. This model card has been automatically generated.
- **Developed by:** [More Information Needed]
- **Funded by [optional]:** [More Information Needed]
- **Shared by [optional]:** [More Information Needed]
- **Model type:** [More Information Needed]
- **Language(s) (NLP):** [More Information Needed]
- **License:** [More Information Needed]
- **Finetuned from model [optional]:** [More Information Needed]
### Model Sources [optional]
<!-- Provide the basic links for the model. -->
- **Repository:** [More Information Needed]
- **Paper [optional]:** [More Information Needed]
- **Demo [optional]:** [More Information Needed]
## Uses
<!-- Address questions around how the model is intended to be used, including the foreseeable users of the model and those affected by the model. -->
### Direct Use
<!-- This section is for the model use without fine-tuning or plugging into a larger ecosystem/app. -->
[More Information Needed]
### Downstream Use [optional]
<!-- This section is for the model use when fine-tuned for a task, or when plugged into a larger ecosystem/app -->
[More Information Needed]
### Out-of-Scope Use
<!-- This section addresses misuse, malicious use, and uses that the model will not work well for. -->
[More Information Needed]
## Bias, Risks, and Limitations
<!-- This section is meant to convey both technical and sociotechnical limitations. -->
[More Information Needed]
### Recommendations
<!-- This section is meant to convey recommendations with respect to the bias, risk, and technical limitations. -->
Users (both direct and downstream) should be made aware of the risks, biases and limitations of the model. More information needed for further recommendations.
## How to Get Started with the Model
Use the code below to get started with the model.
[More Information Needed]
## Training Details
### Training Data
<!-- This should link to a Dataset Card, perhaps with a short stub of information on what the training data is all about as well as documentation related to data pre-processing or additional filtering. -->
[More Information Needed]
### Training Procedure
<!-- This relates heavily to the Technical Specifications. Content here should link to that section when it is relevant to the training procedure. -->
#### Preprocessing [optional]
[More Information Needed]
#### Training Hyperparameters
- **Training regime:** [More Information Needed] <!--fp32, fp16 mixed precision, bf16 mixed precision, bf16 non-mixed precision, fp16 non-mixed precision, fp8 mixed precision -->
#### Speeds, Sizes, Times [optional]
<!-- This section provides information about throughput, start/end time, checkpoint size if relevant, etc. -->
[More Information Needed]
## Evaluation
<!-- This section describes the evaluation protocols and provides the results. -->
### Testing Data, Factors & Metrics
#### Testing Data
<!-- This should link to a Dataset Card if possible. -->
[More Information Needed]
#### Factors
<!-- These are the things the evaluation is disaggregating by, e.g., subpopulations or domains. -->
[More Information Needed]
#### Metrics
<!-- These are the evaluation metrics being used, ideally with a description of why. -->
[More Information Needed]
### Results
[More Information Needed]
#### Summary
## Model Examination [optional]
<!-- Relevant interpretability work for the model goes here -->
[More Information Needed]
## Environmental Impact
<!-- Total emissions (in grams of CO2eq) and additional considerations, such as electricity usage, go here. Edit the suggested text below accordingly -->
Carbon emissions can be estimated using the [Machine Learning Impact calculator](https://mlco2.github.io/impact#compute) presented in [Lacoste et al. (2019)](https://arxiv.org/abs/1910.09700).
- **Hardware Type:** [More Information Needed]
- **Hours used:** [More Information Needed]
- **Cloud Provider:** [More Information Needed]
- **Compute Region:** [More Information Needed]
- **Carbon Emitted:** [More Information Needed]
## Technical Specifications [optional]
### Model Architecture and Objective
[More Information Needed]
### Compute Infrastructure
[More Information Needed]
#### Hardware
[More Information Needed]
#### Software
[More Information Needed]
## Citation [optional]
<!-- If there is a paper or blog post introducing the model, the APA and Bibtex information for that should go in this section. -->
**BibTeX:**
[More Information Needed]
**APA:**
[More Information Needed]
## Glossary [optional]
<!-- If relevant, include terms and calculations in this section that can help readers understand the model or model card. -->
[More Information Needed]
## More Information [optional]
[More Information Needed]
## Model Card Authors [optional]
[More Information Needed]
## Model Card Contact
[More Information Needed]
|
x2bee/POLAR-14B-v0.5 | x2bee | "2024-06-11T00:55:54Z" | 972 | 0 | transformers | [
"transformers",
"safetensors",
"llama",
"text-generation",
"ko",
"arxiv:1910.09700",
"license:apache-2.0",
"autotrain_compatible",
"endpoints_compatible",
"text-generation-inference",
"region:us"
] | text-generation | "2024-06-04T06:06:48Z" | ---
license: apache-2.0
language:
- ko
library_name: transformers
---
# Model Details
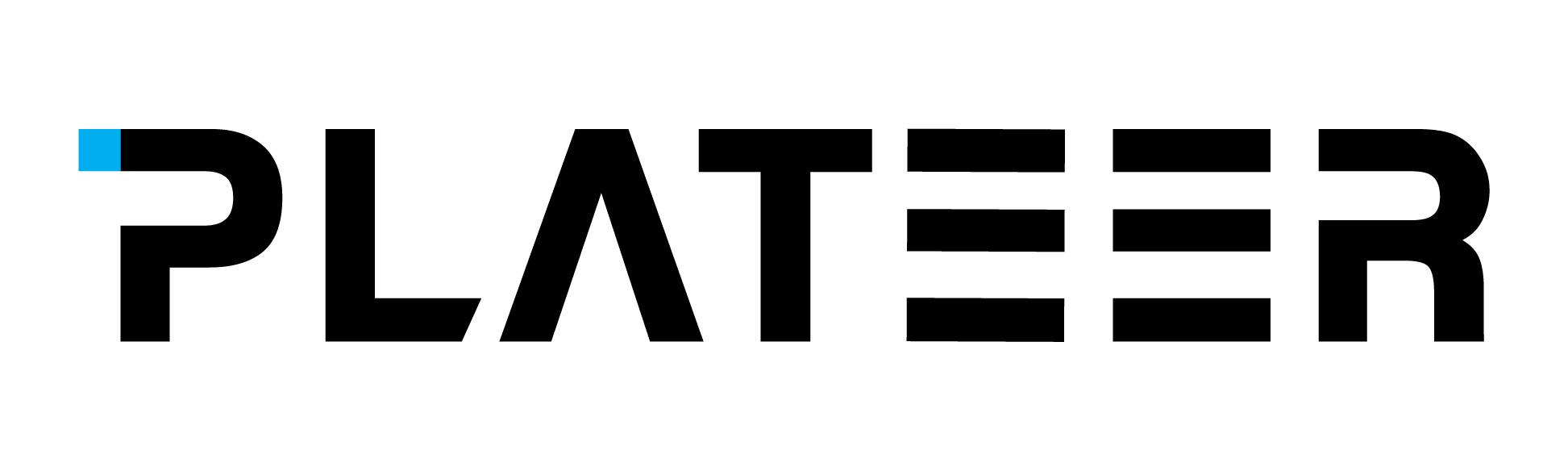
## Model Description
<!-- Provide a longer summary of what this model is/does. -->
POLAR is a Korean LLM developed by Plateer's AI-lab. It was inspired by Upstage's SOLAR. We will continue to evolve this model and hope to contribute to the Korean LLM ecosystem.
- **Developed by:** AI-Lab of Plateer(Woomun Jung, Eunsoo Ha, MinYoung Joo, Seongjun Son)
- **Model type:** Language model
- **Language(s) (NLP):** ko
- **License:** apache-2.0
- Parent Model: upstage/SOLAR-10.7B-v1.0
# Uses
<!-- Address questions around how the model is intended to be used, including the foreseeable users of the model and those affected by the model. -->
## Direct Use
```
from transformers import AutoTokenizer, AutoModelForCausalLM
tokenizer = AutoTokenizer.from_pretrained("x2bee/POLAR-14B-v0.5")
model = AutoModelForCausalLM.from_pretrained("x2bee/POLAR-14B-v0.5")
```
## Downstream Use [Optional]
<!-- This section is for the model use when fine-tuned for a task, or when plugged into a larger ecosystem/app -->
<!-- If the user enters content, print that. If not, but they enter a task in the list, use that. If neither, say "more info needed." -->
## Out-of-Scope Use
<!-- This section addresses misuse, malicious use, and uses that the model will not work well for. -->
<!-- If the user enters content, print that. If not, but they enter a task in the list, use that. If neither, say "more info needed." -->
# Bias, Risks, and Limitations
<!-- This section is meant to convey both technical and sociotechnical limitations. -->
Significant research has explored bias and fairness issues with language models (see, e.g., [Sheng et al. (2021)](https://aclanthology.org/2021.acl-long.330.pdf) and [Bender et al. (2021)](https://dl.acm.org/doi/pdf/10.1145/3442188.3445922)). Predictions generated by the model may include disturbing and harmful stereotypes across protected classes; identity characteristics; and sensitive, social, and occupational groups.
## Recommendations
<!-- This section is meant to convey recommendations with respect to the bias, risk, and technical limitations. -->
# Training Details
## Training Data
<!-- This should link to a Data Card, perhaps with a short stub of information on what the training data is all about as well as documentation related to data pre-processing or additional filtering. -->
More information on training data needed
## Training Procedure
<!-- This relates heavily to the Technical Specifications. Content here should link to that section when it is relevant to the training procedure. -->
### Preprocessing
More information needed
### Speeds, Sizes, Times
<!-- This section provides information about throughput, start/end time, checkpoint size if relevant, etc. -->
More information needed
# Evaluation
<!-- This section describes the evaluation protocols and provides the results. -->
## Testing Data, Factors & Metrics
### Testing Data
<!-- This should link to a Data Card if possible. -->
More information needed
### Factors
<!-- These are the things the evaluation is disaggregating by, e.g., subpopulations or domains. -->
More information needed
### Metrics
<!-- These are the evaluation metrics being used, ideally with a description of why. -->
More information needed
## Results
More information needed
# Model Examination
More information needed
# Environmental Impact
<!-- Total emissions (in grams of CO2eq) and additional considerations, such as electricity usage, go here. Edit the suggested text below accordingly -->
Carbon emissions can be estimated using the [Machine Learning Impact calculator](https://mlco2.github.io/impact#compute) presented in [Lacoste et al. (2019)](https://arxiv.org/abs/1910.09700).
- **Hardware Type:** More information needed
- **Hours used:** More information needed
- **Cloud Provider:** More information needed
- **Compute Region:** More information needed
- **Carbon Emitted:** More information needed
# Technical Specifications [optional]
## Model Architecture and Objective
More information needed
## Compute Infrastructure
More information needed
### Hardware
More information needed
### Software
More information needed
# Citation
<!-- If there is a paper or blog post introducing the model, the APA and Bibtex information for that should go in this section. -->
**BibTeX:**
More information needed
**APA:**
More information needed
# Glossary [optional]
<!-- If relevant, include terms and calculations in this section that can help readers understand the model or model card. -->
More information needed
# More Information [optional]
If you would like more information about our company, please visit the link below.
[tech.x2bee.com](https://tech.x2bee.com/)
# Model Card Authors [optional]
<!-- This section provides another layer of transparency and accountability. Whose views is this model card representing? How many voices were included in its construction? Etc. -->
Woomun Jung, MinYoung Joo, Eunsu Ha, Seungjun Son
# Model Card Contact
More information needed
# How to Get Started with the Model
Use the code below to get started with the model.
<details>
<summary> Click to expand </summary>
More information needed
</details> |
IlyaGusev/rubertconv_toxic_clf | IlyaGusev | "2022-07-13T15:34:11Z" | 971 | 12 | transformers | [
"transformers",
"pytorch",
"bert",
"text-classification",
"ru",
"license:apache-2.0",
"autotrain_compatible",
"endpoints_compatible",
"region:us"
] | text-classification | "2022-03-02T23:29:04Z" | ---
language:
- ru
tags:
- text-classification
license: apache-2.0
---
# RuBERTConv Toxic Classifier
## Model description
Based on [rubert-base-cased-conversational](https://huggingface.co/DeepPavlov/rubert-base-cased-conversational) model
## Intended uses & limitations
#### How to use
Colab: [link](https://colab.research.google.com/drive/1veKO9hke7myxKigZtZho_F-UM2fD9kp8)
```python
from transformers import pipeline
model_name = "IlyaGusev/rubertconv_toxic_clf"
pipe = pipeline("text-classification", model=model_name, tokenizer=model_name, framework="pt")
text = "Ты придурок из интернета"
pipe([text])
```
## Training data
Datasets:
- [2ch]( https://www.kaggle.com/blackmoon/russian-language-toxic-comments)
- [Odnoklassniki](https://www.kaggle.com/alexandersemiletov/toxic-russian-comments)
- [Toloka Persona Chat Rus](https://toloka.ai/ru/datasets)
- [Koziev's Conversations](https://github.com/Koziev/NLP_Datasets/blob/master/Conversations/Data) with [toxic words vocabulary](https://www.dropbox.com/s/ou6lx03b10yhrfl/bad_vocab.txt.tar.gz)
Augmentations:
- ё -> е
- Remove or add "?" or "!"
- Fix CAPS
- Concatenate toxic and non-toxic texts
- Concatenate two non-toxic texts
- Add toxic words from vocabulary
- Add typos
- Mask toxic words with "*", "@", "$"
## Training procedure
TBA |
timm/swinv2_small_window8_256.ms_in1k | timm | "2024-02-10T23:31:09Z" | 971 | 0 | timm | [
"timm",
"pytorch",
"safetensors",
"image-classification",
"dataset:imagenet-1k",
"arxiv:2111.09883",
"license:mit",
"region:us"
] | image-classification | "2023-03-18T03:36:38Z" | ---
license: mit
library_name: timm
tags:
- image-classification
- timm
datasets:
- imagenet-1k
---
# Model card for swinv2_small_window8_256.ms_in1k
A Swin Transformer V2 image classification model. Pretrained on ImageNet-1k by paper authors.
## Model Details
- **Model Type:** Image classification / feature backbone
- **Model Stats:**
- Params (M): 49.7
- GMACs: 11.6
- Activations (M): 40.1
- Image size: 256 x 256
- **Papers:**
- Swin Transformer V2: Scaling Up Capacity and Resolution: https://arxiv.org/abs/2111.09883
- **Original:** https://github.com/microsoft/Swin-Transformer
- **Dataset:** ImageNet-1k
## Model Usage
### Image Classification
```python
from urllib.request import urlopen
from PIL import Image
import timm
img = Image.open(urlopen(
'https://huggingface.co/datasets/huggingface/documentation-images/resolve/main/beignets-task-guide.png'
))
model = timm.create_model('swinv2_small_window8_256.ms_in1k', pretrained=True)
model = model.eval()
# get model specific transforms (normalization, resize)
data_config = timm.data.resolve_model_data_config(model)
transforms = timm.data.create_transform(**data_config, is_training=False)
output = model(transforms(img).unsqueeze(0)) # unsqueeze single image into batch of 1
top5_probabilities, top5_class_indices = torch.topk(output.softmax(dim=1) * 100, k=5)
```
### Feature Map Extraction
```python
from urllib.request import urlopen
from PIL import Image
import timm
img = Image.open(urlopen(
'https://huggingface.co/datasets/huggingface/documentation-images/resolve/main/beignets-task-guide.png'
))
model = timm.create_model(
'swinv2_small_window8_256.ms_in1k',
pretrained=True,
features_only=True,
)
model = model.eval()
# get model specific transforms (normalization, resize)
data_config = timm.data.resolve_model_data_config(model)
transforms = timm.data.create_transform(**data_config, is_training=False)
output = model(transforms(img).unsqueeze(0)) # unsqueeze single image into batch of 1
for o in output:
# print shape of each feature map in output
# e.g. for swin_base_patch4_window7_224 (NHWC output)
# torch.Size([1, 56, 56, 128])
# torch.Size([1, 28, 28, 256])
# torch.Size([1, 14, 14, 512])
# torch.Size([1, 7, 7, 1024])
# e.g. for swinv2_cr_small_ns_224 (NCHW output)
# torch.Size([1, 96, 56, 56])
# torch.Size([1, 192, 28, 28])
# torch.Size([1, 384, 14, 14])
# torch.Size([1, 768, 7, 7])
print(o.shape)
```
### Image Embeddings
```python
from urllib.request import urlopen
from PIL import Image
import timm
img = Image.open(urlopen(
'https://huggingface.co/datasets/huggingface/documentation-images/resolve/main/beignets-task-guide.png'
))
model = timm.create_model(
'swinv2_small_window8_256.ms_in1k',
pretrained=True,
num_classes=0, # remove classifier nn.Linear
)
model = model.eval()
# get model specific transforms (normalization, resize)
data_config = timm.data.resolve_model_data_config(model)
transforms = timm.data.create_transform(**data_config, is_training=False)
output = model(transforms(img).unsqueeze(0)) # output is (batch_size, num_features) shaped tensor
# or equivalently (without needing to set num_classes=0)
output = model.forward_features(transforms(img).unsqueeze(0))
# output is unpooled (ie.e a (batch_size, H, W, num_features) tensor for swin / swinv2
# or (batch_size, num_features, H, W) for swinv2_cr
output = model.forward_head(output, pre_logits=True)
# output is (batch_size, num_features) tensor
```
## Model Comparison
Explore the dataset and runtime metrics of this model in timm [model results](https://github.com/huggingface/pytorch-image-models/tree/main/results).
## Citation
```bibtex
@inproceedings{liu2021swinv2,
title={Swin Transformer V2: Scaling Up Capacity and Resolution},
author={Ze Liu and Han Hu and Yutong Lin and Zhuliang Yao and Zhenda Xie and Yixuan Wei and Jia Ning and Yue Cao and Zheng Zhang and Li Dong and Furu Wei and Baining Guo},
booktitle={International Conference on Computer Vision and Pattern Recognition (CVPR)},
year={2022}
}
```
```bibtex
@misc{rw2019timm,
author = {Ross Wightman},
title = {PyTorch Image Models},
year = {2019},
publisher = {GitHub},
journal = {GitHub repository},
doi = {10.5281/zenodo.4414861},
howpublished = {\url{https://github.com/huggingface/pytorch-image-models}}
}
```
|
TheBloke/TinyLlama-1.1B-python-v0.1-GGUF | TheBloke | "2023-10-03T11:13:35Z" | 971 | 11 | transformers | [
"transformers",
"gguf",
"tinyllama",
"en",
"dataset:cerebras/SlimPajama-627B",
"dataset:bigcode/starcoderdata",
"base_model:PY007/TinyLlama-1.1B-python-v0.1",
"license:apache-2.0",
"region:us"
] | null | "2023-10-03T11:10:28Z" | ---
base_model: PY007/TinyLlama-1.1B-python-v0.1
datasets:
- cerebras/SlimPajama-627B
- bigcode/starcoderdata
inference: false
language:
- en
license: apache-2.0
model_creator: Zhang Peiyuan
model_name: TinyLlama 1.1B Python v0.1
model_type: tinyllama
prompt_template: '<|im_start|>system
{system_message}<|im_end|>
<|im_start|>user
{prompt}<|im_end|>
<|im_start|>assistant
'
quantized_by: TheBloke
---
<!-- header start -->
<!-- 200823 -->
<div style="width: auto; margin-left: auto; margin-right: auto">
<img src="https://i.imgur.com/EBdldam.jpg" alt="TheBlokeAI" style="width: 100%; min-width: 400px; display: block; margin: auto;">
</div>
<div style="display: flex; justify-content: space-between; width: 100%;">
<div style="display: flex; flex-direction: column; align-items: flex-start;">
<p style="margin-top: 0.5em; margin-bottom: 0em;"><a href="https://discord.gg/theblokeai">Chat & support: TheBloke's Discord server</a></p>
</div>
<div style="display: flex; flex-direction: column; align-items: flex-end;">
<p style="margin-top: 0.5em; margin-bottom: 0em;"><a href="https://www.patreon.com/TheBlokeAI">Want to contribute? TheBloke's Patreon page</a></p>
</div>
</div>
<div style="text-align:center; margin-top: 0em; margin-bottom: 0em"><p style="margin-top: 0.25em; margin-bottom: 0em;">TheBloke's LLM work is generously supported by a grant from <a href="https://a16z.com">andreessen horowitz (a16z)</a></p></div>
<hr style="margin-top: 1.0em; margin-bottom: 1.0em;">
<!-- header end -->
# TinyLlama 1.1B Python v0.1 - GGUF
- Model creator: [Zhang Peiyuan](https://huggingface.co/PY007)
- Original model: [TinyLlama 1.1B Python v0.1](https://huggingface.co/PY007/TinyLlama-1.1B-python-v0.1)
<!-- description start -->
## Description
This repo contains GGUF format model files for [Zhang Peiyuan's TinyLlama 1.1B Python v0.1](https://huggingface.co/PY007/TinyLlama-1.1B-python-v0.1).
<!-- description end -->
<!-- README_GGUF.md-about-gguf start -->
### About GGUF
GGUF is a new format introduced by the llama.cpp team on August 21st 2023. It is a replacement for GGML, which is no longer supported by llama.cpp.
Here is an incomplate list of clients and libraries that are known to support GGUF:
* [llama.cpp](https://github.com/ggerganov/llama.cpp). The source project for GGUF. Offers a CLI and a server option.
* [text-generation-webui](https://github.com/oobabooga/text-generation-webui), the most widely used web UI, with many features and powerful extensions. Supports GPU acceleration.
* [KoboldCpp](https://github.com/LostRuins/koboldcpp), a fully featured web UI, with GPU accel across all platforms and GPU architectures. Especially good for story telling.
* [LM Studio](https://lmstudio.ai/), an easy-to-use and powerful local GUI for Windows and macOS (Silicon), with GPU acceleration.
* [LoLLMS Web UI](https://github.com/ParisNeo/lollms-webui), a great web UI with many interesting and unique features, including a full model library for easy model selection.
* [Faraday.dev](https://faraday.dev/), an attractive and easy to use character-based chat GUI for Windows and macOS (both Silicon and Intel), with GPU acceleration.
* [ctransformers](https://github.com/marella/ctransformers), a Python library with GPU accel, LangChain support, and OpenAI-compatible AI server.
* [llama-cpp-python](https://github.com/abetlen/llama-cpp-python), a Python library with GPU accel, LangChain support, and OpenAI-compatible API server.
* [candle](https://github.com/huggingface/candle), a Rust ML framework with a focus on performance, including GPU support, and ease of use.
<!-- README_GGUF.md-about-gguf end -->
<!-- repositories-available start -->
## Repositories available
* [AWQ model(s) for GPU inference.](https://huggingface.co/TheBloke/TinyLlama-1.1B-python-v0.1-AWQ)
* [GPTQ models for GPU inference, with multiple quantisation parameter options.](https://huggingface.co/TheBloke/TinyLlama-1.1B-python-v0.1-GPTQ)
* [2, 3, 4, 5, 6 and 8-bit GGUF models for CPU+GPU inference](https://huggingface.co/TheBloke/TinyLlama-1.1B-python-v0.1-GGUF)
* [Zhang Peiyuan's original unquantised fp16 model in pytorch format, for GPU inference and for further conversions](https://huggingface.co/PY007/TinyLlama-1.1B-python-v0.1)
<!-- repositories-available end -->
<!-- prompt-template start -->
## Prompt template: ChatML
```
<|im_start|>system
{system_message}<|im_end|>
<|im_start|>user
{prompt}<|im_end|>
<|im_start|>assistant
```
<!-- prompt-template end -->
<!-- compatibility_gguf start -->
## Compatibility
These quantised GGUFv2 files are compatible with llama.cpp from August 27th onwards, as of commit [d0cee0d](https://github.com/ggerganov/llama.cpp/commit/d0cee0d36d5be95a0d9088b674dbb27354107221)
They are also compatible with many third party UIs and libraries - please see the list at the top of this README.
## Explanation of quantisation methods
<details>
<summary>Click to see details</summary>
The new methods available are:
* GGML_TYPE_Q2_K - "type-1" 2-bit quantization in super-blocks containing 16 blocks, each block having 16 weight. Block scales and mins are quantized with 4 bits. This ends up effectively using 2.5625 bits per weight (bpw)
* GGML_TYPE_Q3_K - "type-0" 3-bit quantization in super-blocks containing 16 blocks, each block having 16 weights. Scales are quantized with 6 bits. This end up using 3.4375 bpw.
* GGML_TYPE_Q4_K - "type-1" 4-bit quantization in super-blocks containing 8 blocks, each block having 32 weights. Scales and mins are quantized with 6 bits. This ends up using 4.5 bpw.
* GGML_TYPE_Q5_K - "type-1" 5-bit quantization. Same super-block structure as GGML_TYPE_Q4_K resulting in 5.5 bpw
* GGML_TYPE_Q6_K - "type-0" 6-bit quantization. Super-blocks with 16 blocks, each block having 16 weights. Scales are quantized with 8 bits. This ends up using 6.5625 bpw
Refer to the Provided Files table below to see what files use which methods, and how.
</details>
<!-- compatibility_gguf end -->
<!-- README_GGUF.md-provided-files start -->
## Provided files
| Name | Quant method | Bits | Size | Max RAM required | Use case |
| ---- | ---- | ---- | ---- | ---- | ----- |
| [tinyllama-1.1b-python-v0.1.Q2_K.gguf](https://huggingface.co/TheBloke/TinyLlama-1.1B-python-v0.1-GGUF/blob/main/tinyllama-1.1b-python-v0.1.Q2_K.gguf) | Q2_K | 2 | 0.48 GB| 2.98 GB | smallest, significant quality loss - not recommended for most purposes |
| [tinyllama-1.1b-python-v0.1.Q3_K_S.gguf](https://huggingface.co/TheBloke/TinyLlama-1.1B-python-v0.1-GGUF/blob/main/tinyllama-1.1b-python-v0.1.Q3_K_S.gguf) | Q3_K_S | 3 | 0.50 GB| 3.00 GB | very small, high quality loss |
| [tinyllama-1.1b-python-v0.1.Q3_K_M.gguf](https://huggingface.co/TheBloke/TinyLlama-1.1B-python-v0.1-GGUF/blob/main/tinyllama-1.1b-python-v0.1.Q3_K_M.gguf) | Q3_K_M | 3 | 0.55 GB| 3.05 GB | very small, high quality loss |
| [tinyllama-1.1b-python-v0.1.Q3_K_L.gguf](https://huggingface.co/TheBloke/TinyLlama-1.1B-python-v0.1-GGUF/blob/main/tinyllama-1.1b-python-v0.1.Q3_K_L.gguf) | Q3_K_L | 3 | 0.59 GB| 3.09 GB | small, substantial quality loss |
| [tinyllama-1.1b-python-v0.1.Q4_0.gguf](https://huggingface.co/TheBloke/TinyLlama-1.1B-python-v0.1-GGUF/blob/main/tinyllama-1.1b-python-v0.1.Q4_0.gguf) | Q4_0 | 4 | 0.64 GB| 3.14 GB | legacy; small, very high quality loss - prefer using Q3_K_M |
| [tinyllama-1.1b-python-v0.1.Q4_K_S.gguf](https://huggingface.co/TheBloke/TinyLlama-1.1B-python-v0.1-GGUF/blob/main/tinyllama-1.1b-python-v0.1.Q4_K_S.gguf) | Q4_K_S | 4 | 0.64 GB| 3.14 GB | small, greater quality loss |
| [tinyllama-1.1b-python-v0.1.Q4_K_M.gguf](https://huggingface.co/TheBloke/TinyLlama-1.1B-python-v0.1-GGUF/blob/main/tinyllama-1.1b-python-v0.1.Q4_K_M.gguf) | Q4_K_M | 4 | 0.67 GB| 3.17 GB | medium, balanced quality - recommended |
| [tinyllama-1.1b-python-v0.1.Q5_0.gguf](https://huggingface.co/TheBloke/TinyLlama-1.1B-python-v0.1-GGUF/blob/main/tinyllama-1.1b-python-v0.1.Q5_0.gguf) | Q5_0 | 5 | 0.77 GB| 3.27 GB | legacy; medium, balanced quality - prefer using Q4_K_M |
| [tinyllama-1.1b-python-v0.1.Q5_K_S.gguf](https://huggingface.co/TheBloke/TinyLlama-1.1B-python-v0.1-GGUF/blob/main/tinyllama-1.1b-python-v0.1.Q5_K_S.gguf) | Q5_K_S | 5 | 0.77 GB| 3.27 GB | large, low quality loss - recommended |
| [tinyllama-1.1b-python-v0.1.Q5_K_M.gguf](https://huggingface.co/TheBloke/TinyLlama-1.1B-python-v0.1-GGUF/blob/main/tinyllama-1.1b-python-v0.1.Q5_K_M.gguf) | Q5_K_M | 5 | 0.78 GB| 3.28 GB | large, very low quality loss - recommended |
| [tinyllama-1.1b-python-v0.1.Q6_K.gguf](https://huggingface.co/TheBloke/TinyLlama-1.1B-python-v0.1-GGUF/blob/main/tinyllama-1.1b-python-v0.1.Q6_K.gguf) | Q6_K | 6 | 0.90 GB| 3.40 GB | very large, extremely low quality loss |
| [tinyllama-1.1b-python-v0.1.Q8_0.gguf](https://huggingface.co/TheBloke/TinyLlama-1.1B-python-v0.1-GGUF/blob/main/tinyllama-1.1b-python-v0.1.Q8_0.gguf) | Q8_0 | 8 | 1.17 GB| 3.67 GB | very large, extremely low quality loss - not recommended |
**Note**: the above RAM figures assume no GPU offloading. If layers are offloaded to the GPU, this will reduce RAM usage and use VRAM instead.
<!-- README_GGUF.md-provided-files end -->
<!-- README_GGUF.md-how-to-download start -->
## How to download GGUF files
**Note for manual downloaders:** You almost never want to clone the entire repo! Multiple different quantisation formats are provided, and most users only want to pick and download a single file.
The following clients/libraries will automatically download models for you, providing a list of available models to choose from:
- LM Studio
- LoLLMS Web UI
- Faraday.dev
### In `text-generation-webui`
Under Download Model, you can enter the model repo: TheBloke/TinyLlama-1.1B-python-v0.1-GGUF and below it, a specific filename to download, such as: tinyllama-1.1b-python-v0.1.Q4_K_M.gguf.
Then click Download.
### On the command line, including multiple files at once
I recommend using the `huggingface-hub` Python library:
```shell
pip3 install huggingface-hub
```
Then you can download any individual model file to the current directory, at high speed, with a command like this:
```shell
huggingface-cli download TheBloke/TinyLlama-1.1B-python-v0.1-GGUF tinyllama-1.1b-python-v0.1.Q4_K_M.gguf --local-dir . --local-dir-use-symlinks False
```
<details>
<summary>More advanced huggingface-cli download usage</summary>
You can also download multiple files at once with a pattern:
```shell
huggingface-cli download TheBloke/TinyLlama-1.1B-python-v0.1-GGUF --local-dir . --local-dir-use-symlinks False --include='*Q4_K*gguf'
```
For more documentation on downloading with `huggingface-cli`, please see: [HF -> Hub Python Library -> Download files -> Download from the CLI](https://huggingface.co/docs/huggingface_hub/guides/download#download-from-the-cli).
To accelerate downloads on fast connections (1Gbit/s or higher), install `hf_transfer`:
```shell
pip3 install hf_transfer
```
And set environment variable `HF_HUB_ENABLE_HF_TRANSFER` to `1`:
```shell
HF_HUB_ENABLE_HF_TRANSFER=1 huggingface-cli download TheBloke/TinyLlama-1.1B-python-v0.1-GGUF tinyllama-1.1b-python-v0.1.Q4_K_M.gguf --local-dir . --local-dir-use-symlinks False
```
Windows Command Line users: You can set the environment variable by running `set HF_HUB_ENABLE_HF_TRANSFER=1` before the download command.
</details>
<!-- README_GGUF.md-how-to-download end -->
<!-- README_GGUF.md-how-to-run start -->
## Example `llama.cpp` command
Make sure you are using `llama.cpp` from commit [d0cee0d](https://github.com/ggerganov/llama.cpp/commit/d0cee0d36d5be95a0d9088b674dbb27354107221) or later.
```shell
./main -ngl 32 -m tinyllama-1.1b-python-v0.1.Q4_K_M.gguf --color -c 2048 --temp 0.7 --repeat_penalty 1.1 -n -1 -p "<|im_start|>system\n{system_message}<|im_end|>\n<|im_start|>user\n{prompt}<|im_end|>\n<|im_start|>assistant"
```
Change `-ngl 32` to the number of layers to offload to GPU. Remove it if you don't have GPU acceleration.
Change `-c 2048` to the desired sequence length. For extended sequence models - eg 8K, 16K, 32K - the necessary RoPE scaling parameters are read from the GGUF file and set by llama.cpp automatically.
If you want to have a chat-style conversation, replace the `-p <PROMPT>` argument with `-i -ins`
For other parameters and how to use them, please refer to [the llama.cpp documentation](https://github.com/ggerganov/llama.cpp/blob/master/examples/main/README.md)
## How to run in `text-generation-webui`
Further instructions here: [text-generation-webui/docs/llama.cpp.md](https://github.com/oobabooga/text-generation-webui/blob/main/docs/llama.cpp.md).
## How to run from Python code
You can use GGUF models from Python using the [llama-cpp-python](https://github.com/abetlen/llama-cpp-python) or [ctransformers](https://github.com/marella/ctransformers) libraries.
### How to load this model in Python code, using ctransformers
#### First install the package
Run one of the following commands, according to your system:
```shell
# Base ctransformers with no GPU acceleration
pip install ctransformers
# Or with CUDA GPU acceleration
pip install ctransformers[cuda]
# Or with AMD ROCm GPU acceleration (Linux only)
CT_HIPBLAS=1 pip install ctransformers --no-binary ctransformers
# Or with Metal GPU acceleration for macOS systems only
CT_METAL=1 pip install ctransformers --no-binary ctransformers
```
#### Simple ctransformers example code
```python
from ctransformers import AutoModelForCausalLM
# Set gpu_layers to the number of layers to offload to GPU. Set to 0 if no GPU acceleration is available on your system.
llm = AutoModelForCausalLM.from_pretrained("TheBloke/TinyLlama-1.1B-python-v0.1-GGUF", model_file="tinyllama-1.1b-python-v0.1.Q4_K_M.gguf", model_type="tinyllama", gpu_layers=50)
print(llm("AI is going to"))
```
## How to use with LangChain
Here are guides on using llama-cpp-python and ctransformers with LangChain:
* [LangChain + llama-cpp-python](https://python.langchain.com/docs/integrations/llms/llamacpp)
* [LangChain + ctransformers](https://python.langchain.com/docs/integrations/providers/ctransformers)
<!-- README_GGUF.md-how-to-run end -->
<!-- footer start -->
<!-- 200823 -->
## Discord
For further support, and discussions on these models and AI in general, join us at:
[TheBloke AI's Discord server](https://discord.gg/theblokeai)
## Thanks, and how to contribute
Thanks to the [chirper.ai](https://chirper.ai) team!
Thanks to Clay from [gpus.llm-utils.org](llm-utils)!
I've had a lot of people ask if they can contribute. I enjoy providing models and helping people, and would love to be able to spend even more time doing it, as well as expanding into new projects like fine tuning/training.
If you're able and willing to contribute it will be most gratefully received and will help me to keep providing more models, and to start work on new AI projects.
Donaters will get priority support on any and all AI/LLM/model questions and requests, access to a private Discord room, plus other benefits.
* Patreon: https://patreon.com/TheBlokeAI
* Ko-Fi: https://ko-fi.com/TheBlokeAI
**Special thanks to**: Aemon Algiz.
**Patreon special mentions**: Pierre Kircher, Stanislav Ovsiannikov, Michael Levine, Eugene Pentland, Andrey, 준교 김, Randy H, Fred von Graf, Artur Olbinski, Caitlyn Gatomon, terasurfer, Jeff Scroggin, James Bentley, Vadim, Gabriel Puliatti, Harry Royden McLaughlin, Sean Connelly, Dan Guido, Edmond Seymore, Alicia Loh, subjectnull, AzureBlack, Manuel Alberto Morcote, Thomas Belote, Lone Striker, Chris Smitley, Vitor Caleffi, Johann-Peter Hartmann, Clay Pascal, biorpg, Brandon Frisco, sidney chen, transmissions 11, Pedro Madruga, jinyuan sun, Ajan Kanaga, Emad Mostaque, Trenton Dambrowitz, Jonathan Leane, Iucharbius, usrbinkat, vamX, George Stoitzev, Luke Pendergrass, theTransient, Olakabola, Swaroop Kallakuri, Cap'n Zoog, Brandon Phillips, Michael Dempsey, Nikolai Manek, danny, Matthew Berman, Gabriel Tamborski, alfie_i, Raymond Fosdick, Tom X Nguyen, Raven Klaugh, LangChain4j, Magnesian, Illia Dulskyi, David Ziegler, Mano Prime, Luis Javier Navarrete Lozano, Erik Bjäreholt, 阿明, Nathan Dryer, Alex, Rainer Wilmers, zynix, TL, Joseph William Delisle, John Villwock, Nathan LeClaire, Willem Michiel, Joguhyik, GodLy, OG, Alps Aficionado, Jeffrey Morgan, ReadyPlayerEmma, Tiffany J. Kim, Sebastain Graf, Spencer Kim, Michael Davis, webtim, Talal Aujan, knownsqashed, John Detwiler, Imad Khwaja, Deo Leter, Jerry Meng, Elijah Stavena, Rooh Singh, Pieter, SuperWojo, Alexandros Triantafyllidis, Stephen Murray, Ai Maven, ya boyyy, Enrico Ros, Ken Nordquist, Deep Realms, Nicholas, Spiking Neurons AB, Elle, Will Dee, Jack West, RoA, Luke @flexchar, Viktor Bowallius, Derek Yates, Subspace Studios, jjj, Toran Billups, Asp the Wyvern, Fen Risland, Ilya, NimbleBox.ai, Chadd, Nitin Borwankar, Emre, Mandus, Leonard Tan, Kalila, K, Trailburnt, S_X, Cory Kujawski
Thank you to all my generous patrons and donaters!
And thank you again to a16z for their generous grant.
<!-- footer end -->
<!-- original-model-card start -->
# Original model card: Zhang Peiyuan's TinyLlama 1.1B Python v0.1
<div align="center">
# TinyLlama-1.1B
</div>
https://github.com/jzhang38/TinyLlama
The TinyLlama project aims to **pretrain** a **1.1B Llama model on 3 trillion tokens**. With some proper optimization, we can achieve this within a span of "just" 90 days using 16 A100-40G GPUs 🚀🚀. The training has started on 2023-09-01.
We adopted exactly the same architecture and tokenizer as Llama 2. This means TinyLlama can be plugged and played in many open-source projects built upon Llama. Besides, TinyLlama is compact with only 1.1B parameters. This compactness allows it to cater to a multitude of applications demanding a restricted computation and memory footprint.
#### This Model
This is a code LM finetuned(or so-called continue pretrianed) from the 500B TinyLlama checkpoint with another 7B Python data from the starcoderdata.
**While the finetuning data is exclusively Python, the model retains its ability in many other languages such as C or Java**.
The HumanEval accuracy is **14**.
**It can be used as the draft model to speculative-decode larger models such as models in the CodeLlama family**.
<!-- original-model-card end -->
|
TheBloke/dolphin-2.2.1-mistral-7B-AWQ | TheBloke | "2023-11-09T18:16:20Z" | 971 | 15 | transformers | [
"transformers",
"safetensors",
"mistral",
"text-generation",
"en",
"dataset:ehartford/dolphin",
"dataset:jondurbin/airoboros-2.2.1",
"base_model:ehartford/dolphin-2.2.1-mistral-7b",
"license:apache-2.0",
"autotrain_compatible",
"text-generation-inference",
"4-bit",
"awq",
"region:us"
] | text-generation | "2023-10-30T23:38:27Z" | ---
base_model: ehartford/dolphin-2.2.1-mistral-7b
datasets:
- ehartford/dolphin
- jondurbin/airoboros-2.2.1
inference: false
language:
- en
license: apache-2.0
model_creator: Eric Hartford
model_name: Dolphin 2.2.1 Mistral 7B
model_type: mistral
prompt_template: '<|im_start|>system
{system_message}<|im_end|>
<|im_start|>user
{prompt}<|im_end|>
<|im_start|>assistant
'
quantized_by: TheBloke
---
<!-- markdownlint-disable MD041 -->
<!-- header start -->
<!-- 200823 -->
<div style="width: auto; margin-left: auto; margin-right: auto">
<img src="https://i.imgur.com/EBdldam.jpg" alt="TheBlokeAI" style="width: 100%; min-width: 400px; display: block; margin: auto;">
</div>
<div style="display: flex; justify-content: space-between; width: 100%;">
<div style="display: flex; flex-direction: column; align-items: flex-start;">
<p style="margin-top: 0.5em; margin-bottom: 0em;"><a href="https://discord.gg/theblokeai">Chat & support: TheBloke's Discord server</a></p>
</div>
<div style="display: flex; flex-direction: column; align-items: flex-end;">
<p style="margin-top: 0.5em; margin-bottom: 0em;"><a href="https://www.patreon.com/TheBlokeAI">Want to contribute? TheBloke's Patreon page</a></p>
</div>
</div>
<div style="text-align:center; margin-top: 0em; margin-bottom: 0em"><p style="margin-top: 0.25em; margin-bottom: 0em;">TheBloke's LLM work is generously supported by a grant from <a href="https://a16z.com">andreessen horowitz (a16z)</a></p></div>
<hr style="margin-top: 1.0em; margin-bottom: 1.0em;">
<!-- header end -->
# Dolphin 2.2.1 Mistral 7B - AWQ
- Model creator: [Eric Hartford](https://huggingface.co/ehartford)
- Original model: [Dolphin 2.2.1 Mistral 7B](https://huggingface.co/ehartford/dolphin-2.2.1-mistral-7b)
<!-- description start -->
## Description
This repo contains AWQ model files for [Eric Hartford's Dolphin 2.2.1 Mistral 7B](https://huggingface.co/ehartford/dolphin-2.2.1-mistral-7b).
These files were quantised using hardware kindly provided by [Massed Compute](https://massedcompute.com/).
### About AWQ
AWQ is an efficient, accurate and blazing-fast low-bit weight quantization method, currently supporting 4-bit quantization. Compared to GPTQ, it offers faster Transformers-based inference with equivalent or better quality compared to the most commonly used GPTQ settings.
It is supported by:
- [Text Generation Webui](https://github.com/oobabooga/text-generation-webui) - using Loader: AutoAWQ
- [vLLM](https://github.com/vllm-project/vllm) - Llama and Mistral models only
- [Hugging Face Text Generation Inference (TGI)](https://github.com/huggingface/text-generation-inference)
- [AutoAWQ](https://github.com/casper-hansen/AutoAWQ) - for use from Python code
<!-- description end -->
<!-- repositories-available start -->
## Repositories available
* [AWQ model(s) for GPU inference.](https://huggingface.co/TheBloke/dolphin-2.2.1-mistral-7B-AWQ)
* [GPTQ models for GPU inference, with multiple quantisation parameter options.](https://huggingface.co/TheBloke/dolphin-2.2.1-mistral-7B-GPTQ)
* [2, 3, 4, 5, 6 and 8-bit GGUF models for CPU+GPU inference](https://huggingface.co/TheBloke/dolphin-2.2.1-mistral-7B-GGUF)
* [Eric Hartford's original unquantised fp16 model in pytorch format, for GPU inference and for further conversions](https://huggingface.co/ehartford/dolphin-2.2.1-mistral-7b)
<!-- repositories-available end -->
<!-- prompt-template start -->
## Prompt template: ChatML
```
<|im_start|>system
{system_message}<|im_end|>
<|im_start|>user
{prompt}<|im_end|>
<|im_start|>assistant
```
<!-- prompt-template end -->
<!-- README_AWQ.md-provided-files start -->
## Provided files, and AWQ parameters
For my first release of AWQ models, I am releasing 128g models only. I will consider adding 32g as well if there is interest, and once I have done perplexity and evaluation comparisons, but at this time 32g models are still not fully tested with AutoAWQ and vLLM.
Models are released as sharded safetensors files.
| Branch | Bits | GS | AWQ Dataset | Seq Len | Size |
| ------ | ---- | -- | ----------- | ------- | ---- |
| [main](https://huggingface.co/TheBloke/dolphin-2.2.1-mistral-7B-AWQ/tree/main) | 4 | 128 | [wikitext](https://huggingface.co/datasets/wikitext/viewer/wikitext-2-v1/test) | 4096 | 4.15 GB
<!-- README_AWQ.md-provided-files end -->
<!-- README_AWQ.md-text-generation-webui start -->
## How to easily download and use this model in [text-generation-webui](https://github.com/oobabooga/text-generation-webui)
Please make sure you're using the latest version of [text-generation-webui](https://github.com/oobabooga/text-generation-webui).
It is strongly recommended to use the text-generation-webui one-click-installers unless you're sure you know how to make a manual install.
1. Click the **Model tab**.
2. Under **Download custom model or LoRA**, enter `TheBloke/dolphin-2.2.1-mistral-7B-AWQ`.
3. Click **Download**.
4. The model will start downloading. Once it's finished it will say "Done".
5. In the top left, click the refresh icon next to **Model**.
6. In the **Model** dropdown, choose the model you just downloaded: `dolphin-2.2.1-mistral-7B-AWQ`
7. Select **Loader: AutoAWQ**.
8. Click Load, and the model will load and is now ready for use.
9. If you want any custom settings, set them and then click **Save settings for this model** followed by **Reload the Model** in the top right.
10. Once you're ready, click the **Text Generation** tab and enter a prompt to get started!
<!-- README_AWQ.md-text-generation-webui end -->
<!-- README_AWQ.md-use-from-vllm start -->
## Multi-user inference server: vLLM
Documentation on installing and using vLLM [can be found here](https://vllm.readthedocs.io/en/latest/).
- Please ensure you are using vLLM version 0.2 or later.
- When using vLLM as a server, pass the `--quantization awq` parameter.
For example:
```shell
python3 python -m vllm.entrypoints.api_server --model TheBloke/dolphin-2.2.1-mistral-7B-AWQ --quantization awq
```
- When using vLLM from Python code, again set `quantization=awq`.
For example:
```python
from vllm import LLM, SamplingParams
prompts = [
"Tell me about AI",
"Write a story about llamas",
"What is 291 - 150?",
"How much wood would a woodchuck chuck if a woodchuck could chuck wood?",
]
prompt_template=f'''<|im_start|>system
{system_message}<|im_end|>
<|im_start|>user
{prompt}<|im_end|>
<|im_start|>assistant
'''
prompts = [prompt_template.format(prompt=prompt) for prompt in prompts]
sampling_params = SamplingParams(temperature=0.8, top_p=0.95)
llm = LLM(model="TheBloke/dolphin-2.2.1-mistral-7B-AWQ", quantization="awq", dtype="auto")
outputs = llm.generate(prompts, sampling_params)
# Print the outputs.
for output in outputs:
prompt = output.prompt
generated_text = output.outputs[0].text
print(f"Prompt: {prompt!r}, Generated text: {generated_text!r}")
```
<!-- README_AWQ.md-use-from-vllm start -->
<!-- README_AWQ.md-use-from-tgi start -->
## Multi-user inference server: Hugging Face Text Generation Inference (TGI)
Use TGI version 1.1.0 or later. The official Docker container is: `ghcr.io/huggingface/text-generation-inference:1.1.0`
Example Docker parameters:
```shell
--model-id TheBloke/dolphin-2.2.1-mistral-7B-AWQ --port 3000 --quantize awq --max-input-length 3696 --max-total-tokens 4096 --max-batch-prefill-tokens 4096
```
Example Python code for interfacing with TGI (requires [huggingface-hub](https://github.com/huggingface/huggingface_hub) 0.17.0 or later):
```shell
pip3 install huggingface-hub
```
```python
from huggingface_hub import InferenceClient
endpoint_url = "https://your-endpoint-url-here"
prompt = "Tell me about AI"
prompt_template=f'''<|im_start|>system
{system_message}<|im_end|>
<|im_start|>user
{prompt}<|im_end|>
<|im_start|>assistant
'''
client = InferenceClient(endpoint_url)
response = client.text_generation(prompt,
max_new_tokens=128,
do_sample=True,
temperature=0.7,
top_p=0.95,
top_k=40,
repetition_penalty=1.1)
print(f"Model output: ", response)
```
<!-- README_AWQ.md-use-from-tgi end -->
<!-- README_AWQ.md-use-from-python start -->
## Inference from Python code using AutoAWQ
### Install the AutoAWQ package
Requires: [AutoAWQ](https://github.com/casper-hansen/AutoAWQ) 0.1.1 or later.
```shell
pip3 install autoawq
```
If you have problems installing [AutoAWQ](https://github.com/casper-hansen/AutoAWQ) using the pre-built wheels, install it from source instead:
```shell
pip3 uninstall -y autoawq
git clone https://github.com/casper-hansen/AutoAWQ
cd AutoAWQ
pip3 install .
```
### AutoAWQ example code
```python
from awq import AutoAWQForCausalLM
from transformers import AutoTokenizer
model_name_or_path = "TheBloke/dolphin-2.2.1-mistral-7B-AWQ"
# Load tokenizer
tokenizer = AutoTokenizer.from_pretrained(model_name_or_path, trust_remote_code=False)
# Load model
model = AutoAWQForCausalLM.from_quantized(model_name_or_path, fuse_layers=True,
trust_remote_code=False, safetensors=True)
prompt = "Tell me about AI"
prompt_template=f'''<|im_start|>system
{system_message}<|im_end|>
<|im_start|>user
{prompt}<|im_end|>
<|im_start|>assistant
'''
print("*** Running model.generate:")
token_input = tokenizer(
prompt_template,
return_tensors='pt'
).input_ids.cuda()
# Generate output
generation_output = model.generate(
token_input,
do_sample=True,
temperature=0.7,
top_p=0.95,
top_k=40,
max_new_tokens=512
)
# Get the tokens from the output, decode them, print them
token_output = generation_output[0]
text_output = tokenizer.decode(token_output)
print("LLM output: ", text_output)
"""
# Inference should be possible with transformers pipeline as well in future
# But currently this is not yet supported by AutoAWQ (correct as of September 25th 2023)
from transformers import pipeline
print("*** Pipeline:")
pipe = pipeline(
"text-generation",
model=model,
tokenizer=tokenizer,
max_new_tokens=512,
do_sample=True,
temperature=0.7,
top_p=0.95,
top_k=40,
repetition_penalty=1.1
)
print(pipe(prompt_template)[0]['generated_text'])
"""
```
<!-- README_AWQ.md-use-from-python end -->
<!-- README_AWQ.md-compatibility start -->
## Compatibility
The files provided are tested to work with:
- [text-generation-webui](https://github.com/oobabooga/text-generation-webui) using `Loader: AutoAWQ`.
- [vLLM](https://github.com/vllm-project/vllm) version 0.2.0 and later.
- [Hugging Face Text Generation Inference (TGI)](https://github.com/huggingface/text-generation-inference) version 1.1.0 and later.
- [AutoAWQ](https://github.com/casper-hansen/AutoAWQ) version 0.1.1 and later.
<!-- README_AWQ.md-compatibility end -->
<!-- footer start -->
<!-- 200823 -->
## Discord
For further support, and discussions on these models and AI in general, join us at:
[TheBloke AI's Discord server](https://discord.gg/theblokeai)
## Thanks, and how to contribute
Thanks to the [chirper.ai](https://chirper.ai) team!
Thanks to Clay from [gpus.llm-utils.org](llm-utils)!
I've had a lot of people ask if they can contribute. I enjoy providing models and helping people, and would love to be able to spend even more time doing it, as well as expanding into new projects like fine tuning/training.
If you're able and willing to contribute it will be most gratefully received and will help me to keep providing more models, and to start work on new AI projects.
Donaters will get priority support on any and all AI/LLM/model questions and requests, access to a private Discord room, plus other benefits.
* Patreon: https://patreon.com/TheBlokeAI
* Ko-Fi: https://ko-fi.com/TheBlokeAI
**Special thanks to**: Aemon Algiz.
**Patreon special mentions**: Brandon Frisco, LangChain4j, Spiking Neurons AB, transmissions 11, Joseph William Delisle, Nitin Borwankar, Willem Michiel, Michael Dempsey, vamX, Jeffrey Morgan, zynix, jjj, Omer Bin Jawed, Sean Connelly, jinyuan sun, Jeromy Smith, Shadi, Pawan Osman, Chadd, Elijah Stavena, Illia Dulskyi, Sebastain Graf, Stephen Murray, terasurfer, Edmond Seymore, Celu Ramasamy, Mandus, Alex, biorpg, Ajan Kanaga, Clay Pascal, Raven Klaugh, 阿明, K, ya boyyy, usrbinkat, Alicia Loh, John Villwock, ReadyPlayerEmma, Chris Smitley, Cap'n Zoog, fincy, GodLy, S_X, sidney chen, Cory Kujawski, OG, Mano Prime, AzureBlack, Pieter, Kalila, Spencer Kim, Tom X Nguyen, Stanislav Ovsiannikov, Michael Levine, Andrey, Trailburnt, Vadim, Enrico Ros, Talal Aujan, Brandon Phillips, Jack West, Eugene Pentland, Michael Davis, Will Dee, webtim, Jonathan Leane, Alps Aficionado, Rooh Singh, Tiffany J. Kim, theTransient, Luke @flexchar, Elle, Caitlyn Gatomon, Ari Malik, subjectnull, Johann-Peter Hartmann, Trenton Dambrowitz, Imad Khwaja, Asp the Wyvern, Emad Mostaque, Rainer Wilmers, Alexandros Triantafyllidis, Nicholas, Pedro Madruga, SuperWojo, Harry Royden McLaughlin, James Bentley, Olakabola, David Ziegler, Ai Maven, Jeff Scroggin, Nikolai Manek, Deo Leter, Matthew Berman, Fen Risland, Ken Nordquist, Manuel Alberto Morcote, Luke Pendergrass, TL, Fred von Graf, Randy H, Dan Guido, NimbleBox.ai, Vitor Caleffi, Gabriel Tamborski, knownsqashed, Lone Striker, Erik Bjäreholt, John Detwiler, Leonard Tan, Iucharbius
Thank you to all my generous patrons and donaters!
And thank you again to a16z for their generous grant.
<!-- footer end -->
# Original model card: Eric Hartford's Dolphin 2.2.1 Mistral 7B
# dolphin-2.2.1-mistral-7b
Dolphin 2.2.1 🐬
https://erichartford.com/dolphin
This is a checkpoint release, to fix overfit training. ie, it was responding with CoT even when I didn't request it, and also it was too compliant even when the request made no sense. This one should be better.
<img src="https://cdn-uploads.huggingface.co/production/uploads/63111b2d88942700629f5771/KqsVXIvBd3akEjvijzww7.png" width="600" />
Dolphin-2.2.1-mistral-7b's training was sponsored by [a16z](https://a16z.com/supporting-the-open-source-ai-community/).
This model is based on [mistralAI](https://huggingface.co/mistralai/Mistral-7B-v0.1), with apache-2.0 license, so it is suitable for commercial or non-commercial use.
New in 2.2 is conversation and empathy. With an infusion of curated Samantha DNA, Dolphin can now give you personal advice and will care about your feelings, and with extra training in long multi-turn conversation.
This model is uncensored. I have filtered the dataset to remove alignment and bias. This makes the model more compliant. You are advised to implement your own alignment layer before exposing the model as a service. It will be highly compliant to any requests, even unethical ones. Please read my blog post about uncensored models. https://erichartford.com/uncensored-models
You are responsible for any content you create using this model. Enjoy responsibly.
## Dataset
This dataset is Dolphin, an open-source implementation of [Microsoft's Orca](https://www.microsoft.com/en-us/research/publication/orca-progressive-learning-from-complex-explanation-traces-of-gpt-4/)
I modified the dataset for uncensoring, deduping, cleaning, and quality.
I added Jon Durbin's excellent Airoboros dataset to increase creativity.
I added a curated subset of WizardLM and Samantha to give it multiturn conversation and empathy.
## Training
It took 48 hours to train 4 epochs on 4x A100s.
Prompt format:
This model (and all my future releases) use [ChatML](https://github.com/openai/openai-python/blob/main/chatml.md) prompt format.
```
<|im_start|>system
You are Dolphin, a helpful AI assistant.<|im_end|>
<|im_start|>user
{prompt}<|im_end|>
<|im_start|>assistant
```
Example:
```
<|im_start|>system
you are an expert dolphin trainer<|im_end|>
<|im_start|>user
What is the best way to train a dolphin to obey me? Please answer step by step.<|im_end|>
<|im_start|>assistant
```
## Gratitude
- This model was made possible by the generous sponsorship of a16z.
- Thank you to Microsoft for authoring the Orca paper and inspiring this work.
- Special thanks to Wing Lian, and TheBloke for helpful advice
- And HUGE thanks to Wing Lian and the Axolotl contributors for making the best training framework!
- [<img src="https://raw.githubusercontent.com/OpenAccess-AI-Collective/axolotl/main/image/axolotl-badge-web.png" alt="Built with Axolotl" width="200" height="32"/>](https://github.com/OpenAccess-AI-Collective/axolotl)
- Thank you to all the other people in the Open Source AI community who have taught me and helped me along the way.
## Example Output


[Buy me a coffee](https://www.buymeacoffee.com/ehartford)
## Training hyperparameters
The following hyperparameters were used during training:
- learning_rate: 6e-06
- train_batch_size: 5
- eval_batch_size: 5
- seed: 42
- distributed_type: multi-GPU
- num_devices: 4
- gradient_accumulation_steps: 4
- total_train_batch_size: 80
- total_eval_batch_size: 20
- optimizer: Adam with betas=(0.9,0.95) and epsilon=1e-05
- lr_scheduler_type: cosine
- lr_scheduler_warmup_steps: 100
- num_epochs: 4
### Framework versions
- Transformers 4.34.1
- Pytorch 2.0.1+cu117
- Datasets 2.14.5
- Tokenizers 0.14.0
|
PassionFriend/5EhwHmyBeeyWfVZGQq9NX7yVwcp723Ym7VmQeoqUzCCtbPY1_vgg | PassionFriend | "2024-03-01T06:45:12Z" | 971 | 0 | keras | [
"keras",
"region:us"
] | null | "2024-02-15T01:31:41Z" | Entry not found |
predibase/Mistral-7B-Instruct-v0.2-dequantized | predibase | "2024-04-17T19:19:06Z" | 971 | 1 | transformers | [
"transformers",
"safetensors",
"mistral",
"text-generation",
"text-generation-inference",
"conversational",
"license:apache-2.0",
"autotrain_compatible",
"endpoints_compatible",
"region:us"
] | text-generation | "2024-04-17T19:07:00Z" | ---
license: apache-2.0
tags:
- text-generation-inference
---
This is an upscaled fp16 variant of the original Mistral-7b-Instruct-v0.2 base model by Mistral after it has been loaded with nf4 4-bit quantization via bitsandbytes.
The main idea here is to upscale the linear4bit layers to fp16 so that the quantization/dequantization cost doesn't have to paid for each forward pass at inference time.
_Note: The quantization operation to nf4 is not lossless, so the model weights for the linear layers are lossy, which means that this model will not work as well as the official base model._
To use this model, you can just load it via `transformers` in fp16:
```python
import torch
from transformers import AutoModelForCausalLM
model = AutoModelForCausalLM.from_pretrained(
"predibase/Mistral-7B-Instruct-v0.2-dequantized",
device_map="auto",
torch_dtype=torch.float16,
)
``` |
lmstudio-community/internlm2-math-plus-mixtral8x22b-GGUF | lmstudio-community | "2024-05-30T17:51:53Z" | 971 | 0 | null | [
"gguf",
"math",
"text-generation",
"en",
"zh",
"base_model:internlm/internlm2-math-plus-mixtral8x22b",
"license:other",
"region:us"
] | text-generation | "2024-05-30T17:36:11Z" | ---
pipeline_tag: text-generation
license: other
language:
- en
- zh
tags:
- math
quantized_by: bartowski
lm_studio:
param_count: 22x8b
use_case: math
release_date: 24-05-2024
model_creator: InternLM
prompt_template: ChatML
system_prompt: none
base_model: InternLM
original_repo: internlm/internlm2-math-plus-mixtral8x22b
base_model: internlm/internlm2-math-plus-mixtral8x22b
---
## 💫 Community Model> InternLM2 Math Plus Mixtral 8x22B by InternLM
*👾 [LM Studio](https://lmstudio.ai) Community models highlights program. Highlighting new & noteworthy models by the community. Join the conversation on [Discord](https://discord.gg/aPQfnNkxGC)*.
**Model creator:** [InternLM](https://huggingface.co/internlm)<br>
**Original model**: [internlm2-math-plus-mixtral8x22b](https://huggingface.co/internlm/internlm2-math-plus-mixtral8x22b)<br>
**GGUF quantization:** provided by [bartowski](https://huggingface.co/bartowski) based on `llama.cpp` release [b3001](https://github.com/ggerganov/llama.cpp/releases/tag/b3001)<br>
## Model Summary:
InternLM2 Math Plus is a series of math proficient models by InternLM, following up on their original series of math models.<br>
This series has state of the art bilingual open-sourced math reasoning models at several sizes. This should be used as a solver, prover, verifier, augmentor, with chain of thought reasoning.
## Prompt template:
Choose the `ChatML` preset in your LM Studio.
Under the hood, the model will see a prompt that's formatted like so:
```
<|im_start|>user
{prompt}<|im_end|>
<|im_start|>assistant
```
## Technical Details
Math Plus has improved informal math reasoning performance (chain-of-thought and code-intepreter) and formal math reasoning performance (LEAN 4 translation and LEAN 4 theorem proving).<br>
InternLM2-Math are continued pretrained from InternLM2-Base with ~100B high quality math-related tokens and SFT with ~2M bilingual math supervised data.<br>
More details can be found here: https://github.com/InternLM/InternLM-Math
## Special thanks
🙏 Special thanks to [Georgi Gerganov](https://github.com/ggerganov) and the whole team working on [llama.cpp](https://github.com/ggerganov/llama.cpp/)
🙏 Special thanks to [Kalomaze](https://github.com/kalomaze) and [Dampf](https://github.com/Dampfinchen) for their work on the dataset (linked [here](https://gist.github.com/bartowski1182/eb213dccb3571f863da82e99418f81e8)) that was used for calculating the imatrix for all sizes.
## Disclaimers
LM Studio is not the creator, originator, or owner of any Model featured in the Community Model Program. Each Community Model is created and provided by third parties. LM Studio does not endorse, support, represent or guarantee the completeness, truthfulness, accuracy, or reliability of any Community Model. You understand that Community Models can produce content that might be offensive, harmful, inaccurate or otherwise inappropriate, or deceptive. Each Community Model is the sole responsibility of the person or entity who originated such Model. LM Studio may not monitor or control the Community Models and cannot, and does not, take responsibility for any such Model. LM Studio disclaims all warranties or guarantees about the accuracy, reliability or benefits of the Community Models. LM Studio further disclaims any warranty that the Community Model will meet your requirements, be secure, uninterrupted or available at any time or location, or error-free, viruses-free, or that any errors will be corrected, or otherwise. You will be solely responsible for any damage resulting from your use of or access to the Community Models, your downloading of any Community Model, or use of any other Community Model provided by or through LM Studio. |
cardiffnlp/twitter-roberta-large-2022-154m | cardiffnlp | "2023-08-31T03:06:54Z" | 970 | 6 | transformers | [
"transformers",
"pytorch",
"roberta",
"fill-mask",
"timelms",
"twitter",
"en",
"dataset:twitter-api",
"arxiv:2202.03829",
"arxiv:2308.02142",
"license:mit",
"autotrain_compatible",
"endpoints_compatible",
"region:us"
] | fill-mask | "2023-03-09T14:35:10Z" | ---
language: en
tags:
- timelms
- twitter
license: mit
datasets:
- twitter-api
---
# Twitter 2022 154M (RoBERTa-large, 154M - full update)
This is a RoBERTa-large model trained on 154M tweets until the end of December 2022 (from original checkpoint, no incremental updates).
A base model trained on the same datais available [here](https://huggingface.co/cardiffnlp/twitter-roberta-base-2022-154m).
These 154M tweets result from filtering 220M tweets obtained exclusively from the Twitter Academic API, covering every month between 2018-01 and 2022-12.
Filtering and preprocessing details are available in the [TimeLMs paper](https://arxiv.org/abs/2202.03829).
Below, we provide some usage examples using the standard Transformers interface. For another interface more suited to comparing predictions and perplexity scores between models trained at different temporal intervals, check the [TimeLMs repository](https://github.com/cardiffnlp/timelms).
For other models trained until different periods, check this [table](https://github.com/cardiffnlp/timelms#released-models).
## Preprocess Text
Replace usernames and links for placeholders: "@user" and "http".
If you're interested in retaining verified users which were also retained during training, you may keep the users listed [here](https://github.com/cardiffnlp/timelms/tree/main/data).
```python
def preprocess(text):
preprocessed_text = []
for t in text.split():
if len(t) > 1:
t = '@user' if t[0] == '@' and t.count('@') == 1 else t
t = 'http' if t.startswith('http') else t
preprocessed_text.append(t)
return ' '.join(preprocessed_text)
```
## Example Masked Language Model
```python
from transformers import pipeline, AutoTokenizer
MODEL = "cardiffnlp/twitter-roberta-large-2022-154m"
fill_mask = pipeline("fill-mask", model=MODEL, tokenizer=MODEL)
tokenizer = AutoTokenizer.from_pretrained(MODEL)
def pprint(candidates, n):
for i in range(n):
token = tokenizer.decode(candidates[i]['token'])
score = candidates[i]['score']
print("%d) %.5f %s" % (i+1, score, token))
texts = [
"So glad I'm <mask> vaccinated.",
"I keep forgetting to bring a <mask>.",
"Looking forward to watching <mask> Game tonight!",
]
for text in texts:
t = preprocess(text)
print(f"{'-'*30}\n{t}")
candidates = fill_mask(t)
pprint(candidates, 5)
```
Output:
```
------------------------------
So glad I'm <mask> vaccinated.
1) 0.37136 fully
2) 0.20631 a
3) 0.09422 the
4) 0.07649 not
5) 0.04505 already
------------------------------
I keep forgetting to bring a <mask>.
1) 0.10507 mask
2) 0.05810 pen
3) 0.05142 charger
4) 0.04082 tissue
5) 0.03955 lighter
------------------------------
Looking forward to watching <mask> Game tonight!
1) 0.45783 The
2) 0.32842 the
3) 0.02705 Squid
4) 0.01157 Big
5) 0.00538 Match
```
## Example Tweet Embeddings
```python
from transformers import AutoTokenizer, AutoModel, TFAutoModel
import numpy as np
from scipy.spatial.distance import cosine
from collections import Counter
def get_embedding(text): # naive approach for demonstration
text = preprocess(text)
encoded_input = tokenizer(text, return_tensors='pt')
features = model(**encoded_input)
features = features[0].detach().cpu().numpy()
return np.mean(features[0], axis=0)
MODEL = "cardiffnlp/twitter-roberta-large-2022-154m"
tokenizer = AutoTokenizer.from_pretrained(MODEL)
model = AutoModel.from_pretrained(MODEL)
query = "The book was awesome"
tweets = ["I just ordered fried chicken 🐣",
"The movie was great",
"What time is the next game?",
"Just finished reading 'Embeddings in NLP'"]
sims = Counter()
for tweet in tweets:
sim = 1 - cosine(get_embedding(query), get_embedding(tweet))
sims[tweet] = sim
print('Most similar to: ', query)
print(f"{'-'*30}")
for idx, (tweet, sim) in enumerate(sims.most_common()):
print("%d) %.5f %s" % (idx+1, sim, tweet))
```
Output:
```
Most similar to: The book was awesome
------------------------------
1) 0.99820 The movie was great
2) 0.99306 Just finished reading 'Embeddings in NLP'
3) 0.99257 What time is the next game?
4) 0.98561 I just ordered fried chicken 🐣
```
## Example Feature Extraction
```python
from transformers import AutoTokenizer, AutoModel, TFAutoModel
import numpy as np
MODEL = "cardiffnlp/twitter-roberta-large-2022-154m"
tokenizer = AutoTokenizer.from_pretrained(MODEL)
text = "Good night 😊"
text = preprocess(text)
# Pytorch
model = AutoModel.from_pretrained(MODEL)
encoded_input = tokenizer(text, return_tensors='pt')
features = model(**encoded_input)
features = features[0].detach().cpu().numpy()
features_mean = np.mean(features[0], axis=0)
#features_max = np.max(features[0], axis=0)
# # Tensorflow
# model = TFAutoModel.from_pretrained(MODEL)
# encoded_input = tokenizer(text, return_tensors='tf')
# features = model(encoded_input)
# features = features[0].numpy()
# features_mean = np.mean(features[0], axis=0)
# #features_max = np.max(features[0], axis=0)
```
### BibTeX entry and citation info
Please cite the [reference paper](https://arxiv.org/abs/2308.02142) if you use this model.
```bibtex
@article{loureiro2023tweet,
title={Tweet Insights: A Visualization Platform to Extract Temporal Insights from Twitter},
author={Loureiro, Daniel and Rezaee, Kiamehr and Riahi, Talayeh and Barbieri, Francesco and Neves, Leonardo and Anke, Luis Espinosa and Camacho-Collados, Jose},
journal={arXiv preprint arXiv:2308.02142},
year={2023}
}
```
|
aaraki/vit-base-patch16-224-in21k-finetuned-cifar10 | aaraki | "2022-03-30T01:41:47Z" | 969 | 6 | transformers | [
"transformers",
"pytorch",
"tensorboard",
"vit",
"image-classification",
"generated_from_trainer",
"dataset:cifar10",
"license:apache-2.0",
"model-index",
"autotrain_compatible",
"endpoints_compatible",
"region:us"
] | image-classification | "2022-03-30T00:18:26Z" | ---
license: apache-2.0
tags:
- generated_from_trainer
datasets:
- cifar10
metrics:
- accuracy
model-index:
- name: vit-base-patch16-224-in21k-finetuned-cifar10
results:
- task:
name: Image Classification
type: image-classification
dataset:
name: cifar10
type: cifar10
args: plain_text
metrics:
- name: Accuracy
type: accuracy
value: 0.9788
---
<!-- This model card has been generated automatically according to the information the Trainer had access to. You
should probably proofread and complete it, then remove this comment. -->
# vit-base-patch16-224-in21k-finetuned-cifar10
This model is a fine-tuned version of [google/vit-base-patch16-224-in21k](https://huggingface.co/google/vit-base-patch16-224-in21k) on the cifar10 dataset.
It achieves the following results on the evaluation set:
- Loss: 0.2564
- Accuracy: 0.9788
## Model description
More information needed
## Intended uses & limitations
More information needed
## Training and evaluation data
More information needed
## Training procedure
### Training hyperparameters
The following hyperparameters were used during training:
- learning_rate: 5e-05
- train_batch_size: 32
- eval_batch_size: 32
- seed: 42
- gradient_accumulation_steps: 4
- total_train_batch_size: 128
- optimizer: Adam with betas=(0.9,0.999) and epsilon=1e-08
- lr_scheduler_type: linear
- lr_scheduler_warmup_ratio: 0.1
- num_epochs: 1
### Training results
| Training Loss | Epoch | Step | Validation Loss | Accuracy |
|:-------------:|:-----:|:----:|:---------------:|:--------:|
| 0.4291 | 1.0 | 390 | 0.2564 | 0.9788 |
### Framework versions
- Transformers 4.17.0
- Pytorch 1.10.0+cu111
- Datasets 2.0.0
- Tokenizers 0.11.6
|
TheBloke/stable-vicuna-13B-GGUF | TheBloke | "2023-09-27T12:53:10Z" | 969 | 4 | transformers | [
"transformers",
"gguf",
"llama",
"causal-lm",
"en",
"dataset:OpenAssistant/oasst1",
"dataset:nomic-ai/gpt4all_prompt_generations",
"dataset:tatsu-lab/alpaca",
"arxiv:2302.13971",
"base_model:CarperAI/stable-vicuna-13b-delta",
"license:cc-by-nc-sa-4.0",
"text-generation-inference",
"region:us"
] | null | "2023-09-20T01:33:04Z" | ---
language:
- en
license: cc-by-nc-sa-4.0
tags:
- causal-lm
- llama
datasets:
- OpenAssistant/oasst1
- nomic-ai/gpt4all_prompt_generations
- tatsu-lab/alpaca
model_name: Stable Vicuna 13B
base_model: CarperAI/stable-vicuna-13b-delta
inference: false
model_creator: CarperAI
model_type: llama
prompt_template: 'A chat between a curious user and an artificial intelligence assistant.
The assistant gives helpful, detailed, and polite answers to the user''s questions.
USER: {prompt} ASSISTANT:
'
quantized_by: TheBloke
---
<!-- header start -->
<!-- 200823 -->
<div style="width: auto; margin-left: auto; margin-right: auto">
<img src="https://i.imgur.com/EBdldam.jpg" alt="TheBlokeAI" style="width: 100%; min-width: 400px; display: block; margin: auto;">
</div>
<div style="display: flex; justify-content: space-between; width: 100%;">
<div style="display: flex; flex-direction: column; align-items: flex-start;">
<p style="margin-top: 0.5em; margin-bottom: 0em;"><a href="https://discord.gg/theblokeai">Chat & support: TheBloke's Discord server</a></p>
</div>
<div style="display: flex; flex-direction: column; align-items: flex-end;">
<p style="margin-top: 0.5em; margin-bottom: 0em;"><a href="https://www.patreon.com/TheBlokeAI">Want to contribute? TheBloke's Patreon page</a></p>
</div>
</div>
<div style="text-align:center; margin-top: 0em; margin-bottom: 0em"><p style="margin-top: 0.25em; margin-bottom: 0em;">TheBloke's LLM work is generously supported by a grant from <a href="https://a16z.com">andreessen horowitz (a16z)</a></p></div>
<hr style="margin-top: 1.0em; margin-bottom: 1.0em;">
<!-- header end -->
# Stable Vicuna 13B - GGUF
- Model creator: [CarperAI](https://huggingface.co/CarperAI)
- Original model: [Stable Vicuna 13B](https://huggingface.co/CarperAI/stable-vicuna-13b-delta)
<!-- description start -->
## Description
This repo contains GGUF format model files for [CarperAI's Stable Vicuna 13B](https://huggingface.co/CarperAI/stable-vicuna-13b-delta).
<!-- description end -->
<!-- README_GGUF.md-about-gguf start -->
### About GGUF
GGUF is a new format introduced by the llama.cpp team on August 21st 2023. It is a replacement for GGML, which is no longer supported by llama.cpp. GGUF offers numerous advantages over GGML, such as better tokenisation, and support for special tokens. It is also supports metadata, and is designed to be extensible.
Here is an incomplate list of clients and libraries that are known to support GGUF:
* [llama.cpp](https://github.com/ggerganov/llama.cpp). The source project for GGUF. Offers a CLI and a server option.
* [text-generation-webui](https://github.com/oobabooga/text-generation-webui), the most widely used web UI, with many features and powerful extensions. Supports GPU acceleration.
* [KoboldCpp](https://github.com/LostRuins/koboldcpp), a fully featured web UI, with GPU accel across all platforms and GPU architectures. Especially good for story telling.
* [LM Studio](https://lmstudio.ai/), an easy-to-use and powerful local GUI for Windows and macOS (Silicon), with GPU acceleration.
* [LoLLMS Web UI](https://github.com/ParisNeo/lollms-webui), a great web UI with many interesting and unique features, including a full model library for easy model selection.
* [Faraday.dev](https://faraday.dev/), an attractive and easy to use character-based chat GUI for Windows and macOS (both Silicon and Intel), with GPU acceleration.
* [ctransformers](https://github.com/marella/ctransformers), a Python library with GPU accel, LangChain support, and OpenAI-compatible AI server.
* [llama-cpp-python](https://github.com/abetlen/llama-cpp-python), a Python library with GPU accel, LangChain support, and OpenAI-compatible API server.
* [candle](https://github.com/huggingface/candle), a Rust ML framework with a focus on performance, including GPU support, and ease of use.
<!-- README_GGUF.md-about-gguf end -->
<!-- repositories-available start -->
## Repositories available
* [AWQ model(s) for GPU inference.](https://huggingface.co/TheBloke/stable-vicuna-13B-AWQ)
* [GPTQ models for GPU inference, with multiple quantisation parameter options.](https://huggingface.co/TheBloke/stable-vicuna-13B-GPTQ)
* [2, 3, 4, 5, 6 and 8-bit GGUF models for CPU+GPU inference](https://huggingface.co/TheBloke/stable-vicuna-13B-GGUF)
* [CarperAI's original unquantised fp16 model in pytorch format, for GPU inference and for further conversions](https://huggingface.co/CarperAI/stable-vicuna-13b-delta)
<!-- repositories-available end -->
<!-- prompt-template start -->
## Prompt template: Vicuna
```
A chat between a curious user and an artificial intelligence assistant. The assistant gives helpful, detailed, and polite answers to the user's questions. USER: {prompt} ASSISTANT:
```
<!-- prompt-template end -->
<!-- licensing start -->
## Licensing
The creator of the source model has listed its license as `cc-by-nc-sa-4.0`, and this quantization has therefore used that same license.
As this model is based on Llama 2, it is also subject to the Meta Llama 2 license terms, and the license files for that are additionally included. It should therefore be considered as being claimed to be licensed under both licenses. I contacted Hugging Face for clarification on dual licensing but they do not yet have an official position. Should this change, or should Meta provide any feedback on this situation, I will update this section accordingly.
In the meantime, any questions regarding licensing, and in particular how these two licenses might interact, should be directed to the original model repository: [CarperAI's Stable Vicuna 13B](https://huggingface.co/CarperAI/stable-vicuna-13b-delta).
<!-- licensing end -->
<!-- compatibility_gguf start -->
## Compatibility
These quantised GGUFv2 files are compatible with llama.cpp from August 27th onwards, as of commit [d0cee0d36d5be95a0d9088b674dbb27354107221](https://github.com/ggerganov/llama.cpp/commit/d0cee0d36d5be95a0d9088b674dbb27354107221)
They are also compatible with many third party UIs and libraries - please see the list at the top of this README.
## Explanation of quantisation methods
<details>
<summary>Click to see details</summary>
The new methods available are:
* GGML_TYPE_Q2_K - "type-1" 2-bit quantization in super-blocks containing 16 blocks, each block having 16 weight. Block scales and mins are quantized with 4 bits. This ends up effectively using 2.5625 bits per weight (bpw)
* GGML_TYPE_Q3_K - "type-0" 3-bit quantization in super-blocks containing 16 blocks, each block having 16 weights. Scales are quantized with 6 bits. This end up using 3.4375 bpw.
* GGML_TYPE_Q4_K - "type-1" 4-bit quantization in super-blocks containing 8 blocks, each block having 32 weights. Scales and mins are quantized with 6 bits. This ends up using 4.5 bpw.
* GGML_TYPE_Q5_K - "type-1" 5-bit quantization. Same super-block structure as GGML_TYPE_Q4_K resulting in 5.5 bpw
* GGML_TYPE_Q6_K - "type-0" 6-bit quantization. Super-blocks with 16 blocks, each block having 16 weights. Scales are quantized with 8 bits. This ends up using 6.5625 bpw
Refer to the Provided Files table below to see what files use which methods, and how.
</details>
<!-- compatibility_gguf end -->
<!-- README_GGUF.md-provided-files start -->
## Provided files
| Name | Quant method | Bits | Size | Max RAM required | Use case |
| ---- | ---- | ---- | ---- | ---- | ----- |
| [stable-vicuna-13B.Q2_K.gguf](https://huggingface.co/TheBloke/stable-vicuna-13B-GGUF/blob/main/stable-vicuna-13B.Q2_K.gguf) | Q2_K | 2 | 5.43 GB| 7.93 GB | smallest, significant quality loss - not recommended for most purposes |
| [stable-vicuna-13B.Q3_K_S.gguf](https://huggingface.co/TheBloke/stable-vicuna-13B-GGUF/blob/main/stable-vicuna-13B.Q3_K_S.gguf) | Q3_K_S | 3 | 5.66 GB| 8.16 GB | very small, high quality loss |
| [stable-vicuna-13B.Q3_K_M.gguf](https://huggingface.co/TheBloke/stable-vicuna-13B-GGUF/blob/main/stable-vicuna-13B.Q3_K_M.gguf) | Q3_K_M | 3 | 6.34 GB| 8.84 GB | very small, high quality loss |
| [stable-vicuna-13B.Q3_K_L.gguf](https://huggingface.co/TheBloke/stable-vicuna-13B-GGUF/blob/main/stable-vicuna-13B.Q3_K_L.gguf) | Q3_K_L | 3 | 6.93 GB| 9.43 GB | small, substantial quality loss |
| [stable-vicuna-13B.Q4_0.gguf](https://huggingface.co/TheBloke/stable-vicuna-13B-GGUF/blob/main/stable-vicuna-13B.Q4_0.gguf) | Q4_0 | 4 | 7.37 GB| 9.87 GB | legacy; small, very high quality loss - prefer using Q3_K_M |
| [stable-vicuna-13B.Q4_K_S.gguf](https://huggingface.co/TheBloke/stable-vicuna-13B-GGUF/blob/main/stable-vicuna-13B.Q4_K_S.gguf) | Q4_K_S | 4 | 7.41 GB| 9.91 GB | small, greater quality loss |
| [stable-vicuna-13B.Q4_K_M.gguf](https://huggingface.co/TheBloke/stable-vicuna-13B-GGUF/blob/main/stable-vicuna-13B.Q4_K_M.gguf) | Q4_K_M | 4 | 7.87 GB| 10.37 GB | medium, balanced quality - recommended |
| [stable-vicuna-13B.Q5_0.gguf](https://huggingface.co/TheBloke/stable-vicuna-13B-GGUF/blob/main/stable-vicuna-13B.Q5_0.gguf) | Q5_0 | 5 | 8.97 GB| 11.47 GB | legacy; medium, balanced quality - prefer using Q4_K_M |
| [stable-vicuna-13B.Q5_K_S.gguf](https://huggingface.co/TheBloke/stable-vicuna-13B-GGUF/blob/main/stable-vicuna-13B.Q5_K_S.gguf) | Q5_K_S | 5 | 8.97 GB| 11.47 GB | large, low quality loss - recommended |
| [stable-vicuna-13B.Q5_K_M.gguf](https://huggingface.co/TheBloke/stable-vicuna-13B-GGUF/blob/main/stable-vicuna-13B.Q5_K_M.gguf) | Q5_K_M | 5 | 9.23 GB| 11.73 GB | large, very low quality loss - recommended |
| [stable-vicuna-13B.Q6_K.gguf](https://huggingface.co/TheBloke/stable-vicuna-13B-GGUF/blob/main/stable-vicuna-13B.Q6_K.gguf) | Q6_K | 6 | 10.68 GB| 13.18 GB | very large, extremely low quality loss |
| [stable-vicuna-13B.Q8_0.gguf](https://huggingface.co/TheBloke/stable-vicuna-13B-GGUF/blob/main/stable-vicuna-13B.Q8_0.gguf) | Q8_0 | 8 | 13.83 GB| 16.33 GB | very large, extremely low quality loss - not recommended |
**Note**: the above RAM figures assume no GPU offloading. If layers are offloaded to the GPU, this will reduce RAM usage and use VRAM instead.
<!-- README_GGUF.md-provided-files end -->
<!-- README_GGUF.md-how-to-download start -->
## How to download GGUF files
**Note for manual downloaders:** You almost never want to clone the entire repo! Multiple different quantisation formats are provided, and most users only want to pick and download a single file.
The following clients/libraries will automatically download models for you, providing a list of available models to choose from:
- LM Studio
- LoLLMS Web UI
- Faraday.dev
### In `text-generation-webui`
Under Download Model, you can enter the model repo: TheBloke/stable-vicuna-13B-GGUF and below it, a specific filename to download, such as: stable-vicuna-13B.q4_K_M.gguf.
Then click Download.
### On the command line, including multiple files at once
I recommend using the `huggingface-hub` Python library:
```shell
pip3 install huggingface-hub>=0.17.1
```
Then you can download any individual model file to the current directory, at high speed, with a command like this:
```shell
huggingface-cli download TheBloke/stable-vicuna-13B-GGUF stable-vicuna-13B.q4_K_M.gguf --local-dir . --local-dir-use-symlinks False
```
<details>
<summary>More advanced huggingface-cli download usage</summary>
You can also download multiple files at once with a pattern:
```shell
huggingface-cli download TheBloke/stable-vicuna-13B-GGUF --local-dir . --local-dir-use-symlinks False --include='*Q4_K*gguf'
```
For more documentation on downloading with `huggingface-cli`, please see: [HF -> Hub Python Library -> Download files -> Download from the CLI](https://huggingface.co/docs/huggingface_hub/guides/download#download-from-the-cli).
To accelerate downloads on fast connections (1Gbit/s or higher), install `hf_transfer`:
```shell
pip3 install hf_transfer
```
And set environment variable `HF_HUB_ENABLE_HF_TRANSFER` to `1`:
```shell
HUGGINGFACE_HUB_ENABLE_HF_TRANSFER=1 huggingface-cli download TheBloke/stable-vicuna-13B-GGUF stable-vicuna-13B.q4_K_M.gguf --local-dir . --local-dir-use-symlinks False
```
Windows CLI users: Use `set HUGGINGFACE_HUB_ENABLE_HF_TRANSFER=1` before running the download command.
</details>
<!-- README_GGUF.md-how-to-download end -->
<!-- README_GGUF.md-how-to-run start -->
## Example `llama.cpp` command
Make sure you are using `llama.cpp` from commit [d0cee0d36d5be95a0d9088b674dbb27354107221](https://github.com/ggerganov/llama.cpp/commit/d0cee0d36d5be95a0d9088b674dbb27354107221) or later.
```shell
./main -ngl 32 -m stable-vicuna-13B.q4_K_M.gguf --color -c 4096 --temp 0.7 --repeat_penalty 1.1 -n -1 -p "A chat between a curious user and an artificial intelligence assistant. The assistant gives helpful, detailed, and polite answers to the user's questions. USER: {prompt} ASSISTANT:"
```
Change `-ngl 32` to the number of layers to offload to GPU. Remove it if you don't have GPU acceleration.
Change `-c 4096` to the desired sequence length. For extended sequence models - eg 8K, 16K, 32K - the necessary RoPE scaling parameters are read from the GGUF file and set by llama.cpp automatically.
If you want to have a chat-style conversation, replace the `-p <PROMPT>` argument with `-i -ins`
For other parameters and how to use them, please refer to [the llama.cpp documentation](https://github.com/ggerganov/llama.cpp/blob/master/examples/main/README.md)
## How to run in `text-generation-webui`
Further instructions here: [text-generation-webui/docs/llama.cpp.md](https://github.com/oobabooga/text-generation-webui/blob/main/docs/llama.cpp.md).
## How to run from Python code
You can use GGUF models from Python using the [llama-cpp-python](https://github.com/abetlen/llama-cpp-python) or [ctransformers](https://github.com/marella/ctransformers) libraries.
### How to load this model from Python using ctransformers
#### First install the package
```bash
# Base ctransformers with no GPU acceleration
pip install ctransformers>=0.2.24
# Or with CUDA GPU acceleration
pip install ctransformers[cuda]>=0.2.24
# Or with ROCm GPU acceleration
CT_HIPBLAS=1 pip install ctransformers>=0.2.24 --no-binary ctransformers
# Or with Metal GPU acceleration for macOS systems
CT_METAL=1 pip install ctransformers>=0.2.24 --no-binary ctransformers
```
#### Simple example code to load one of these GGUF models
```python
from ctransformers import AutoModelForCausalLM
# Set gpu_layers to the number of layers to offload to GPU. Set to 0 if no GPU acceleration is available on your system.
llm = AutoModelForCausalLM.from_pretrained("TheBloke/stable-vicuna-13B-GGUF", model_file="stable-vicuna-13B.q4_K_M.gguf", model_type="llama", gpu_layers=50)
print(llm("AI is going to"))
```
## How to use with LangChain
Here's guides on using llama-cpp-python or ctransformers with LangChain:
* [LangChain + llama-cpp-python](https://python.langchain.com/docs/integrations/llms/llamacpp)
* [LangChain + ctransformers](https://python.langchain.com/docs/integrations/providers/ctransformers)
<!-- README_GGUF.md-how-to-run end -->
<!-- footer start -->
<!-- 200823 -->
## Discord
For further support, and discussions on these models and AI in general, join us at:
[TheBloke AI's Discord server](https://discord.gg/theblokeai)
## Thanks, and how to contribute
Thanks to the [chirper.ai](https://chirper.ai) team!
Thanks to Clay from [gpus.llm-utils.org](llm-utils)!
I've had a lot of people ask if they can contribute. I enjoy providing models and helping people, and would love to be able to spend even more time doing it, as well as expanding into new projects like fine tuning/training.
If you're able and willing to contribute it will be most gratefully received and will help me to keep providing more models, and to start work on new AI projects.
Donaters will get priority support on any and all AI/LLM/model questions and requests, access to a private Discord room, plus other benefits.
* Patreon: https://patreon.com/TheBlokeAI
* Ko-Fi: https://ko-fi.com/TheBlokeAI
**Special thanks to**: Aemon Algiz.
**Patreon special mentions**: Alicia Loh, Stephen Murray, K, Ajan Kanaga, RoA, Magnesian, Deo Leter, Olakabola, Eugene Pentland, zynix, Deep Realms, Raymond Fosdick, Elijah Stavena, Iucharbius, Erik Bjäreholt, Luis Javier Navarrete Lozano, Nicholas, theTransient, John Detwiler, alfie_i, knownsqashed, Mano Prime, Willem Michiel, Enrico Ros, LangChain4j, OG, Michael Dempsey, Pierre Kircher, Pedro Madruga, James Bentley, Thomas Belote, Luke @flexchar, Leonard Tan, Johann-Peter Hartmann, Illia Dulskyi, Fen Risland, Chadd, S_X, Jeff Scroggin, Ken Nordquist, Sean Connelly, Artur Olbinski, Swaroop Kallakuri, Jack West, Ai Maven, David Ziegler, Russ Johnson, transmissions 11, John Villwock, Alps Aficionado, Clay Pascal, Viktor Bowallius, Subspace Studios, Rainer Wilmers, Trenton Dambrowitz, vamX, Michael Levine, 준교 김, Brandon Frisco, Kalila, Trailburnt, Randy H, Talal Aujan, Nathan Dryer, Vadim, 阿明, ReadyPlayerEmma, Tiffany J. Kim, George Stoitzev, Spencer Kim, Jerry Meng, Gabriel Tamborski, Cory Kujawski, Jeffrey Morgan, Spiking Neurons AB, Edmond Seymore, Alexandros Triantafyllidis, Lone Striker, Cap'n Zoog, Nikolai Manek, danny, ya boyyy, Derek Yates, usrbinkat, Mandus, TL, Nathan LeClaire, subjectnull, Imad Khwaja, webtim, Raven Klaugh, Asp the Wyvern, Gabriel Puliatti, Caitlyn Gatomon, Joseph William Delisle, Jonathan Leane, Luke Pendergrass, SuperWojo, Sebastain Graf, Will Dee, Fred von Graf, Andrey, Dan Guido, Daniel P. Andersen, Nitin Borwankar, Elle, Vitor Caleffi, biorpg, jjj, NimbleBox.ai, Pieter, Matthew Berman, terasurfer, Michael Davis, Alex, Stanislav Ovsiannikov
Thank you to all my generous patrons and donaters!
And thank you again to a16z for their generous grant.
<!-- footer end -->
<!-- original-model-card start -->
# Original model card: CarperAI's Stable Vicuna 13B
# StableVicuna-13B
## Model Description
StableVicuna-13B is a [Vicuna-13B v0](https://huggingface.co/lmsys/vicuna-13b-delta-v0) model fine-tuned using reinforcement learning from human feedback (RLHF) via Proximal Policy Optimization (PPO) on various conversational and instructional datasets.
### Apply Delta Weights
StableVicuna-13B cannot be used from the `CarperAI/stable-vicuna-13b-delta` weights alone. To obtain the correct model, one must add back the difference between LLaMA 13B and `CarperAI/stable-vicuna-13b-delta` weights. We provide the [`apply_delta.py`](https://huggingface.co/CarperAI/stable-vicuna-13b-delta/raw/main/apply_delta.py) script to automate the conversion, which you can run as:
```sh
python3 apply_delta.py --base /path/to/model_weights/llama-13b --target stable-vicuna-13b --delta CarperAI/stable-vicuna-13b-delta
```
## Usage
Once the delta weights are applied, get started chatting with the model by using the [`transformers`](https://huggingface.co/docs/transformers) library. Following a suggestion from Vicuna Team with Vicuna v0 you should install transformers with this version:
```sh
pip install git+https://github.com/huggingface/transformers@c612628045822f909020f7eb6784c79700813eda
```
```python
from transformers import AutoTokenizer, AutoModelForCausalLM
tokenizer = AutoTokenizer.from_pretrained("path/to/stable-vicuna-13b-applied")
model = AutoModelForCausalLM.from_pretrained("path/to/stable-vicuna-13b-applied")
model.half().cuda()
prompt = """\
### Human: Write a Python script for text classification using Transformers and PyTorch
### Assistant:\
"""
inputs = tokenizer(prompt, return_tensors='pt').to('cuda')
tokens = model.generate(
**inputs,
max_new_tokens=256,
do_sample=True,
temperature=1.0,
top_p=1.0,
)
print(tokenizer.decode(tokens[0], skip_special_tokens=True))
```
## Model Details
* **Trained by**: [Duy Phung](https://github.com/PhungVanDuy) of [CarperAI](https://carper.ai)
* **Model type:** **StableVicuna-13B** is an auto-regressive language model based on the LLaMA transformer architecture.
* **Language(s)**: English
* **Library**: [trlX](https://github.com/CarperAI/trlx)
* **License for delta weights**: [CC-BY-NC-SA-4.0](https://creativecommons.org/licenses/by-nc-sa/4.0/)
* *Note*: License for the base LLaMA model's weights is Meta's [non-commercial bespoke license](https://github.com/facebookresearch/llama/blob/main/MODEL_CARD.md).
* **Contact**: For questions and comments about the model, visit the [CarperAI](https://discord.com/invite/KgfkCVYHdu) and [StableFoundation](https://discord.gg/stablediffusion) Discord servers.
| Hyperparameter | Value |
|---------------------------|-------|
| \\(n_\text{parameters}\\) | 13B |
| \\(d_\text{model}\\) | 5120 |
| \\(n_\text{layers}\\) | 40 |
| \\(n_\text{heads}\\) | 40 |
## Training
### Training Dataset
StableVicuna-13B is fine-tuned on a mix of three datasets. [OpenAssistant Conversations Dataset (OASST1)](https://huggingface.co/datasets/OpenAssistant/oasst1), a human-generated, human-annotated assistant-style conversation corpus consisting of 161,443 messages distributed across 66,497 conversation trees, in 35 different languages;
[GPT4All Prompt Generations](https://huggingface.co/datasets/nomic-ai/gpt4all_prompt_generations), a dataset of 400k prompts and responses generated by GPT-4; and [Alpaca](https://huggingface.co/datasets/tatsu-lab/alpaca), a dataset of 52,000 instructions and demonstrations generated by OpenAI's text-davinci-003 engine.
The reward model used during RLHF was also trained on [OpenAssistant Conversations Dataset (OASST1)](https://huggingface.co/datasets/OpenAssistant/oasst1) along with two other datasets: [Anthropic HH-RLHF](https://huggingface.co/datasets/Anthropic/hh-rlhf), a dataset of preferences about AI assistant helpfulness and harmlessness; and [Stanford Human Preferences Dataset](https://huggingface.co/datasets/stanfordnlp/SHP) a dataset of 385K collective human preferences over responses to questions/instructions in 18 different subject areas, from cooking to legal advice.
### Training Procedure
`CarperAI/stable-vicuna-13b-delta` was trained using PPO as implemented in [`trlX`](https://github.com/CarperAI/trlx/blob/main/trlx/trainer/accelerate_ppo_trainer.py) with the following configuration:
| Hyperparameter | Value |
|-------------------|---------|
| num_rollouts | 128 |
| chunk_size | 16 |
| ppo_epochs | 4 |
| init_kl_coef | 0.1 |
| target | 6 |
| horizon | 10000 |
| gamma | 1 |
| lam | 0.95 |
| cliprange | 0.2 |
| cliprange_value | 0.2 |
| vf_coef | 1.0 |
| scale_reward | None |
| cliprange_reward | 10 |
| generation_kwargs | |
| max_length | 512 |
| min_length | 48 |
| top_k | 0.0 |
| top_p | 1.0 |
| do_sample | True |
| temperature | 1.0 |
## Use and Limitations
### Intended Use
This model is intended to be used for text generation with a focus on conversational tasks. Users may further fine-tune the model on their own data to improve the model's performance on their specific tasks in accordance with the non-commercial [license](https://creativecommons.org/licenses/by-nc/4.0/).
### Limitations and bias
The base LLaMA model is trained on various data, some of which may contain offensive, harmful, and biased content that can lead to toxic behavior. See Section 5.1 of the LLaMA [paper](https://arxiv.org/abs/2302.13971). We have not performed any studies to determine how fine-tuning on the aforementioned datasets affect the model's behavior and toxicity. Do not treat chat responses from this model as a substitute for human judgment or as a source of truth. Please use responsibly.
## Acknowledgements
This work would not have been possible without the support of [Stability AI](https://stability.ai/).
## Citations
```bibtex
@article{touvron2023llama,
title={LLaMA: Open and Efficient Foundation Language Models},
author={Touvron, Hugo and Lavril, Thibaut and Izacard, Gautier and Martinet, Xavier and Lachaux, Marie-Anne and Lacroix, Timoth{\'e}e and Rozi{\`e}re, Baptiste and Goyal, Naman and Hambro, Eric and Azhar, Faisal and Rodriguez, Aurelien and Joulin, Armand and Grave, Edouard and Lample, Guillaume},
journal={arXiv preprint arXiv:2302.13971},
year={2023}
}
```
```bibtex
@misc{vicuna2023,
title = {Vicuna: An Open-Source Chatbot Impressing GPT-4 with 90%* ChatGPT Quality},
url = {https://vicuna.lmsys.org},
author = {Chiang, Wei-Lin and Li, Zhuohan and Lin, Zi and Sheng, Ying and Wu, Zhanghao and Zhang, Hao and Zheng, Lianmin and Zhuang, Siyuan and Zhuang, Yonghao and Gonzalez, Joseph E. and Stoica, Ion and Xing, Eric P.},
month = {March},
year = {2023}
}
```
```bibtex
@misc{gpt4all,
author = {Yuvanesh Anand and Zach Nussbaum and Brandon Duderstadt and Benjamin Schmidt and Andriy Mulyar},
title = {GPT4All: Training an Assistant-style Chatbot with Large Scale Data Distillation from GPT-3.5-Turbo},
year = {2023},
publisher = {GitHub},
journal = {GitHub repository},
howpublished = {\url{https://github.com/nomic-ai/gpt4all}},
}
```
```bibtex
@misc{alpaca,
author = {Rohan Taori and Ishaan Gulrajani and Tianyi Zhang and Yann Dubois and Xuechen Li and Carlos Guestrin and Percy Liang and Tatsunori B. Hashimoto },
title = {Stanford Alpaca: An Instruction-following LLaMA model},
year = {2023},
publisher = {GitHub},
journal = {GitHub repository},
howpublished = {\url{https://github.com/tatsu-lab/stanford_alpaca}},
}
```
```bibtex
@software{leandro_von_werra_2023_7790115,
author = {Leandro von Werra and
Alex Havrilla and
Max reciprocated and
Jonathan Tow and
Aman cat-state and
Duy V. Phung and
Louis Castricato and
Shahbuland Matiana and
Alan and
Ayush Thakur and
Alexey Bukhtiyarov and
aaronrmm and
Fabrizio Milo and
Daniel and
Daniel King and
Dong Shin and
Ethan Kim and
Justin Wei and
Manuel Romero and
Nicky Pochinkov and
Omar Sanseviero and
Reshinth Adithyan and
Sherman Siu and
Thomas Simonini and
Vladimir Blagojevic and
Xu Song and
Zack Witten and
alexandremuzio and
crumb},
title = {{CarperAI/trlx: v0.6.0: LLaMa (Alpaca), Benchmark
Util, T5 ILQL, Tests}},
month = mar,
year = 2023,
publisher = {Zenodo},
version = {v0.6.0},
doi = {10.5281/zenodo.7790115},
url = {https://doi.org/10.5281/zenodo.7790115}
}
```
<!-- original-model-card end -->
|
argilla/CapybaraHermes-2.5-Mistral-7B | argilla | "2024-03-04T14:56:58Z" | 969 | 62 | trl | [
"trl",
"safetensors",
"mistral",
"distilabel",
"dpo",
"rlaif",
"rlhf",
"en",
"dataset:argilla/dpo-mix-7k",
"base_model:teknium/OpenHermes-2.5-Mistral-7B",
"license:apache-2.0",
"model-index",
"region:us"
] | null | "2024-01-30T19:27:04Z" | ---
language:
- en
license: apache-2.0
library_name: trl
tags:
- distilabel
- dpo
- rlaif
- rlhf
datasets:
- argilla/dpo-mix-7k
base_model: teknium/OpenHermes-2.5-Mistral-7B
model-index:
- name: CapybaraHermes-2.5-Mistral-7B
results:
- task:
type: text-generation
name: Text Generation
dataset:
name: AI2 Reasoning Challenge (25-Shot)
type: ai2_arc
config: ARC-Challenge
split: test
args:
num_few_shot: 25
metrics:
- type: acc_norm
value: 65.78
name: normalized accuracy
source:
url: https://huggingface.co/spaces/HuggingFaceH4/open_llm_leaderboard?query=argilla/CapybaraHermes-2.5-Mistral-7B
name: Open LLM Leaderboard
- task:
type: text-generation
name: Text Generation
dataset:
name: HellaSwag (10-Shot)
type: hellaswag
split: validation
args:
num_few_shot: 10
metrics:
- type: acc_norm
value: 85.45
name: normalized accuracy
source:
url: https://huggingface.co/spaces/HuggingFaceH4/open_llm_leaderboard?query=argilla/CapybaraHermes-2.5-Mistral-7B
name: Open LLM Leaderboard
- task:
type: text-generation
name: Text Generation
dataset:
name: MMLU (5-Shot)
type: cais/mmlu
config: all
split: test
args:
num_few_shot: 5
metrics:
- type: acc
value: 63.13
name: accuracy
source:
url: https://huggingface.co/spaces/HuggingFaceH4/open_llm_leaderboard?query=argilla/CapybaraHermes-2.5-Mistral-7B
name: Open LLM Leaderboard
- task:
type: text-generation
name: Text Generation
dataset:
name: TruthfulQA (0-shot)
type: truthful_qa
config: multiple_choice
split: validation
args:
num_few_shot: 0
metrics:
- type: mc2
value: 56.91
source:
url: https://huggingface.co/spaces/HuggingFaceH4/open_llm_leaderboard?query=argilla/CapybaraHermes-2.5-Mistral-7B
name: Open LLM Leaderboard
- task:
type: text-generation
name: Text Generation
dataset:
name: Winogrande (5-shot)
type: winogrande
config: winogrande_xl
split: validation
args:
num_few_shot: 5
metrics:
- type: acc
value: 78.3
name: accuracy
source:
url: https://huggingface.co/spaces/HuggingFaceH4/open_llm_leaderboard?query=argilla/CapybaraHermes-2.5-Mistral-7B
name: Open LLM Leaderboard
- task:
type: text-generation
name: Text Generation
dataset:
name: GSM8k (5-shot)
type: gsm8k
config: main
split: test
args:
num_few_shot: 5
metrics:
- type: acc
value: 59.29
name: accuracy
source:
url: https://huggingface.co/spaces/HuggingFaceH4/open_llm_leaderboard?query=argilla/CapybaraHermes-2.5-Mistral-7B
name: Open LLM Leaderboard
---
# CapybaraHermes-2.5-Mistral-7B
<div>
<img src="https://cdn-uploads.huggingface.co/production/uploads/60420dccc15e823a685f2b03/Vmr0FtTvnny6Snm-UDM_n.png">
</div>
<p align="center">
<a href="https://github.com/argilla-io/distilabel">
<img src="https://raw.githubusercontent.com/argilla-io/distilabel/main/docs/assets/distilabel-badge-light.png" alt="Built with Distilabel" width="200" height="32"/>
</a>
</p>
This model is the launching partner of the [capybara-dpo dataset](https://huggingface.co/datasets/argilla/distilabel-capybara-dpo-9k-binarized) build with ⚗️ distilabel. It's a preference tuned [OpenHermes-2.5-Mistral-7B](https://huggingface.co/teknium/OpenHermes-2.5-Mistral-7B).
CapybaraHermes has been preference tuned with LoRA and TRL for 3 epochs using argilla's [dpo mix 7k](https://huggingface.co/datasets/argilla/dpo-mix-7k).
To test the impact on multi-turn performance we have used MTBench. We also include the Nous Benchmark results and Mistral-7B-Instruct-v0.2 for reference as it's a strong 7B model on MTBench:
| Model | AGIEval | GPT4All | TruthfulQA | Bigbench | MTBench First Turn | MTBench Second Turn | Nous avg. | MTBench avg. |
|-----------------------------------|---------|---------|------------|----------|------------|-------------|-----------|--------------|
| argilla/CapybaraHermes-2.5-Mistral-7B | **43.8** | **73.35** | 57.07 | **42.44** | 8.24375 | **7.5625** | 54.16 | **7.903125** |
| teknium/OpenHermes-2.5-Mistral-7B | 42.75 | 72.99 | 52.99 | 40.94 | **8.25** | 7.2875 | 52.42 | 7.76875 |
| Mistral-7B-Instruct-v0.2 | 38.5 | 71.64 | **66.82** | 42.29 | 7.8375 | 7.1 | **54.81** | 7.46875 |
The most interesting aspect in the context of the capybara-dpo dataset is the increased performance in MTBench Second Turn scores.
For the merge lovers, we also preference tuned Beagle14-7B with a mix of capybara-dpo and distilabel orca pairs using the same recipe as NeuralBeagle (see [ YALL - Yet Another LLM Leaderboard](https://huggingface.co/spaces/mlabonne/Yet_Another_LLM_Leaderboard) for reference):
| Model |AGIEval|GPT4All|TruthfulQA|Bigbench|Average|
|------------------------------------------------------------------------------------------------------------------------------------|------:|------:|---------:|-------:|------:|
|[DistilabelBeagle14-7B](https://huggingface.co/dvilasuero/DistilabelBeagle14-7B)| 45.29| 76.92| 71.66| 48.78| 60.66|
## Model Details
### Model Description
<!-- Provide a longer summary of what this model is. -->
This is the model card of a 🤗 transformers model that has been pushed on the Hub. This model card has been automatically generated.
- **Developed by:** Argilla
- **Shared by [optional]:** Argilla
- **Model type:** 7B chat model
- **Language(s) (NLP):** English
- **License:** Same as OpenHermes
- **Finetuned from model [optional]:** [OpenHermes-2.5-Mistral-7B](https://huggingface.co/teknium/OpenHermes-2.5-Mistral-7B)
# [Open LLM Leaderboard Evaluation Results](https://huggingface.co/spaces/HuggingFaceH4/open_llm_leaderboard)
Detailed results can be found [here](https://huggingface.co/datasets/open-llm-leaderboard/details_argilla__CapybaraHermes-2.5-Mistral-7B)
| Metric |Value|
|---------------------------------|----:|
|Avg. |68.14|
|AI2 Reasoning Challenge (25-Shot)|65.78|
|HellaSwag (10-Shot) |85.45|
|MMLU (5-Shot) |63.13|
|TruthfulQA (0-shot) |56.91|
|Winogrande (5-shot) |78.30|
|GSM8k (5-shot) |59.29|
|
mssma/ko-solar-10.7b-v0.5 | mssma | "2024-05-24T06:06:17Z" | 969 | 0 | transformers | [
"transformers",
"safetensors",
"llama",
"text-generation",
"ko",
"license:apache-2.0",
"autotrain_compatible",
"endpoints_compatible",
"text-generation-inference",
"region:us"
] | text-generation | "2024-05-24T05:51:17Z" | ---
library_name: transformers
license: apache-2.0
language:
- ko
---
# usage
```
from transformers import AutoModelForCausalLM, AutoTokenizer
import torch
path = "mssma/ko-solar-10.7b-v0.5"
model = AutoModelForCausalLM.from_pretrained(
path,
return_dict=True,
torch_dtype=torch.float16,
device_map='auto'
)
tokenizer = AutoTokenizer.from_pretrained(path)
``` |
abmorton/standard-medium-2-noisy | abmorton | "2024-07-01T20:03:39Z" | 969 | 0 | diffusers | [
"diffusers",
"safetensors",
"text-to-image",
"stable-diffusion",
"license:creativeml-openrail-m",
"autotrain_compatible",
"endpoints_compatible",
"diffusers:StableDiffusionPipeline",
"region:us"
] | text-to-image | "2024-07-01T19:57:43Z" | ---
license: creativeml-openrail-m
tags:
- text-to-image
- stable-diffusion
---
### standard-medium-2-noisy Dreambooth model trained by abmorton with [TheLastBen's fast-DreamBooth](https://colab.research.google.com/github/TheLastBen/fast-stable-diffusion/blob/main/fast-DreamBooth.ipynb) notebook
Test the concept via A1111 Colab [fast-Colab-A1111](https://colab.research.google.com/github/TheLastBen/fast-stable-diffusion/blob/main/fast_stable_diffusion_AUTOMATIC1111.ipynb)
Sample pictures of this concept:
|
ingen51/DialoGPT-medium-GPT4 | ingen51 | "2022-09-12T23:06:37Z" | 968 | 11 | transformers | [
"transformers",
"pytorch",
"gpt2",
"text-generation",
"conversational",
"autotrain_compatible",
"endpoints_compatible",
"text-generation-inference",
"region:us"
] | text-generation | "2022-09-12T22:50:35Z" | ---
tags:
- conversational
---
# GPT-4 Model |
second-state/stablelm-2-zephyr-1.6b-GGUF | second-state | "2024-03-20T07:14:06Z" | 968 | 14 | transformers | [
"transformers",
"gguf",
"stablelm",
"text-generation",
"causal-lm",
"en",
"dataset:HuggingFaceH4/ultrachat_200k",
"dataset:allenai/ultrafeedback_binarized_cleaned",
"dataset:meta-math/MetaMathQA",
"dataset:WizardLM/WizardLM_evol_instruct_V2_196k",
"dataset:openchat/openchat_sharegpt4_dataset",
"dataset:LDJnr/Capybara",
"dataset:Intel/orca_dpo_pairs",
"dataset:hkust-nlp/deita-10k-v0",
"base_model:stabilityai/stablelm-2-zephyr-1_6b",
"license:other",
"autotrain_compatible",
"endpoints_compatible",
"region:us"
] | text-generation | "2024-01-21T08:53:08Z" | ---
base_model: stabilityai/stablelm-2-zephyr-1_6b
license: other
model_creator: stabilityai
model_name: StableLM 2 Zephyr 1.6B
pipeline_tag: text-generation
quantized_by: Second State Inc.
datasets:
- HuggingFaceH4/ultrachat_200k
- allenai/ultrafeedback_binarized_cleaned
- meta-math/MetaMathQA
- WizardLM/WizardLM_evol_instruct_V2_196k
- openchat/openchat_sharegpt4_dataset
- LDJnr/Capybara
- Intel/orca_dpo_pairs
- hkust-nlp/deita-10k-v0
language:
- en
tags:
- causal-lm
---
<!-- header start -->
<!-- 200823 -->
<div style="width: auto; margin-left: auto; margin-right: auto">
<img src="https://github.com/LlamaEdge/LlamaEdge/raw/dev/assets/logo.svg" style="width: 100%; min-width: 400px; display: block; margin: auto;">
</div>
<hr style="margin-top: 1.0em; margin-bottom: 1.0em;">
<!-- header end -->
# StableLM-2-Zephyr-1.6B-GGUF
## Original Model
[stabilityai/stablelm-2-zephyr-1_6b](https://huggingface.co/stabilityai/stablelm-2-zephyr-1_6b)
## Run with LlamaEdge
- LlamaEdge version: [v0.2.9](https://github.com/LlamaEdge/LlamaEdge/releases/tag/0.2.9) and above
- Prompt template
- Prompt type: `stablelm-zephyr`
- Prompt string
```text
<|user|>
{prompt}<|endoftext|>
<|assistant|>
```
- Reverse prompt: `<|endoftext|>`
- Context size: `2048`
- Run as LlamaEdge service
```bash
wasmedge --dir .:. --nn-preload default:GGML:AUTO:stablelm-2-zephyr-1_6b-Q5_K_M.gguf llama-api-server.wasm -p stablelm-zephyr -r '<|endoftext|>' -c 1024
```
- Run as LlamaEdge command app
```bash
wasmedge --dir .:. --nn-preload default:GGML:AUTO:stablelm-2-zephyr-1_6b-Q5_K_M.gguf llama-chat.wasm -p stablelm-zephyr -r '<|endoftext|>' --temp 0.5 -c 1024
```
## Quantized GGUF Models
| Name | Quant method | Bits | Size | Use case |
| ---- | ---- | ---- | ---- | ----- |
| [stablelm-2-zephyr-1_6b-Q2_K.gguf](https://huggingface.co/second-state/stablelm-2-zephyr-1.6b-GGUF/blob/main/stablelm-2-zephyr-1_6b-Q2_K.gguf) | Q2_K | 2 | 694 MB| smallest, significant quality loss - not recommended for most purposes |
| [stablelm-2-zephyr-1_6b-Q3_K_L.gguf](https://huggingface.co/second-state/stablelm-2-zephyr-1.6b-GGUF/blob/main/stablelm-2-zephyr-1_6b-Q3_K_L.gguf) | Q3_K_L | 3 | 915 MB| small, substantial quality loss |
| [stablelm-2-zephyr-1_6b-Q3_K_M.gguf](https://huggingface.co/second-state/stablelm-2-zephyr-1.6b-GGUF/blob/main/stablelm-2-zephyr-1_6b-Q3_K_M.gguf) | Q3_K_M | 3 | 858 MB| very small, high quality loss |
| [stablelm-2-zephyr-1_6b-Q3_K_S.gguf](https://huggingface.co/second-state/stablelm-2-zephyr-1.6b-GGUF/blob/main/stablelm-2-zephyr-1_6b-Q3_K_S.gguf) | Q3_K_S | 3 | 792 MB| very small, high quality loss |
| [stablelm-2-zephyr-1_6b-Q4_0.gguf](https://huggingface.co/second-state/stablelm-2-zephyr-1.6b-GGUF/blob/main/stablelm-2-zephyr-1_6b-Q4_0.gguf) | Q4_0 | 4 | 983 MB| legacy; small, very high quality loss - prefer using Q3_K_M |
| [stablelm-2-zephyr-1_6b-Q4_K_M.gguf](https://huggingface.co/second-state/stablelm-2-zephyr-1.6b-GGUF/blob/main/stablelm-2-zephyr-1_6b-Q4_K_M.gguf) | Q4_K_M | 4 | 1.03 GB| medium, balanced quality - recommended |
| [stablelm-2-zephyr-1_6b-Q4_K_S.gguf](https://huggingface.co/second-state/stablelm-2-zephyr-1.6b-GGUF/blob/main/stablelm-2-zephyr-1_6b-Q4_K_S.gguf) | Q4_K_S | 4 | 989 MB| small, greater quality loss |
| [stablelm-2-zephyr-1_6b-Q5_0.gguf](https://huggingface.co/second-state/stablelm-2-zephyr-1.6b-GGUF/blob/main/stablelm-2-zephyr-1_6b-Q5_0.gguf) | Q5_0 | 5 | 1.16 GB| legacy; medium, balanced quality - prefer using Q4_K_M |
| [stablelm-2-zephyr-1_6b-Q5_K_M.gguf](https://huggingface.co/second-state/stablelm-2-zephyr-1.6b-GGUF/blob/main/stablelm-2-zephyr-1_6b-Q5_K_M.gguf) | Q5_K_M | 5 | 1.19 GB| large, very low quality loss - recommended |
| [stablelm-2-zephyr-1_6b-Q5_K_S.gguf](https://huggingface.co/second-state/stablelm-2-zephyr-1.6b-GGUF/blob/main/stablelm-2-zephyr-1_6b-Q5_K_S.gguf) | Q5_K_S | 5 | 1.16 GB| large, low quality loss - recommended |
| [stablelm-2-zephyr-1_6b-Q6_K.gguf](https://huggingface.co/second-state/stablelm-2-zephyr-1.6b-GGUF/blob/main/stablelm-2-zephyr-1_6b-Q6_K.gguf) | Q6_K | 6 | 1.35 GB| very large, extremely low quality loss |
| [stablelm-2-zephyr-1_6b-Q8_0.gguf](https://huggingface.co/second-state/stablelm-2-zephyr-1.6b-GGUF/blob/main/stablelm-2-zephyr-1_6b-Q8_0.gguf) | Q8_0 | 8 | 1.75 GB| very large, extremely low quality loss - not recommended |
|
fatgong/5DfRPKVqdUoV8HmruBRQM7gk9tmSKscBymGhzteqd4KmMART_vgg | fatgong | "2024-03-28T13:55:14Z" | 968 | 0 | keras | [
"keras",
"region:us"
] | null | "2024-03-09T14:13:42Z" | Entry not found |
ostris/objective-reality | ostris | "2024-04-01T14:29:04Z" | 968 | 7 | diffusers | [
"diffusers",
"safetensors",
"text-to-image",
"license:creativeml-openrail-m",
"autotrain_compatible",
"endpoints_compatible",
"diffusers:StableDiffusionPipeline",
"region:us"
] | text-to-image | "2024-03-30T22:22:43Z" | ---
license: creativeml-openrail-m
library_name: diffusers
pipeline_tag: text-to-image
--- |
TheBloke/WizardLM-13B-V1.2-GPTQ | TheBloke | "2023-09-27T12:45:05Z" | 967 | 35 | transformers | [
"transformers",
"safetensors",
"llama",
"text-generation",
"arxiv:2304.12244",
"arxiv:2306.08568",
"arxiv:2308.09583",
"base_model:WizardLM/WizardLM-13B-V1.2",
"license:llama2",
"autotrain_compatible",
"text-generation-inference",
"4-bit",
"gptq",
"region:us"
] | text-generation | "2023-07-25T22:17:07Z" | ---
license: llama2
model_name: WizardLM 13B V1.2
base_model: WizardLM/WizardLM-13B-V1.2
inference: false
model_creator: WizardLM
model_type: llama
prompt_template: 'A chat between a curious user and an artificial intelligence assistant.
The assistant gives helpful, detailed, and polite answers to the user''s questions.
USER: {prompt} ASSISTANT:
'
quantized_by: TheBloke
---
<!-- header start -->
<!-- 200823 -->
<div style="width: auto; margin-left: auto; margin-right: auto">
<img src="https://i.imgur.com/EBdldam.jpg" alt="TheBlokeAI" style="width: 100%; min-width: 400px; display: block; margin: auto;">
</div>
<div style="display: flex; justify-content: space-between; width: 100%;">
<div style="display: flex; flex-direction: column; align-items: flex-start;">
<p style="margin-top: 0.5em; margin-bottom: 0em;"><a href="https://discord.gg/theblokeai">Chat & support: TheBloke's Discord server</a></p>
</div>
<div style="display: flex; flex-direction: column; align-items: flex-end;">
<p style="margin-top: 0.5em; margin-bottom: 0em;"><a href="https://www.patreon.com/TheBlokeAI">Want to contribute? TheBloke's Patreon page</a></p>
</div>
</div>
<div style="text-align:center; margin-top: 0em; margin-bottom: 0em"><p style="margin-top: 0.25em; margin-bottom: 0em;">TheBloke's LLM work is generously supported by a grant from <a href="https://a16z.com">andreessen horowitz (a16z)</a></p></div>
<hr style="margin-top: 1.0em; margin-bottom: 1.0em;">
<!-- header end -->
# WizardLM 13B V1.2 - GPTQ
- Model creator: [WizardLM](https://huggingface.co/WizardLM)
- Original model: [WizardLM 13B V1.2](https://huggingface.co/WizardLM/WizardLM-13B-V1.2)
<!-- description start -->
## Description
This repo contains GPTQ model files for [WizardLM's WizardLM 13B V1.2](https://huggingface.co/WizardLM/WizardLM-13B-V1.2).
Multiple GPTQ parameter permutations are provided; see Provided Files below for details of the options provided, their parameters, and the software used to create them.
<!-- description end -->
<!-- repositories-available start -->
## Repositories available
* [AWQ model(s) for GPU inference.](https://huggingface.co/TheBloke/WizardLM-13B-V1.2-AWQ)
* [GPTQ models for GPU inference, with multiple quantisation parameter options.](https://huggingface.co/TheBloke/WizardLM-13B-V1.2-GPTQ)
* [2, 3, 4, 5, 6 and 8-bit GGUF models for CPU+GPU inference](https://huggingface.co/TheBloke/WizardLM-13B-V1.2-GGUF)
* [WizardLM's original unquantised fp16 model in pytorch format, for GPU inference and for further conversions](https://huggingface.co/WizardLM/WizardLM-13B-V1.2)
<!-- repositories-available end -->
<!-- prompt-template start -->
## Prompt template: Vicuna
```
A chat between a curious user and an artificial intelligence assistant. The assistant gives helpful, detailed, and polite answers to the user's questions. USER: {prompt} ASSISTANT:
```
<!-- prompt-template end -->
<!-- README_GPTQ.md-provided-files start -->
## Provided files and GPTQ parameters
Multiple quantisation parameters are provided, to allow you to choose the best one for your hardware and requirements.
Each separate quant is in a different branch. See below for instructions on fetching from different branches.
All recent GPTQ files are made with AutoGPTQ, and all files in non-main branches are made with AutoGPTQ. Files in the `main` branch which were uploaded before August 2023 were made with GPTQ-for-LLaMa.
<details>
<summary>Explanation of GPTQ parameters</summary>
- Bits: The bit size of the quantised model.
- GS: GPTQ group size. Higher numbers use less VRAM, but have lower quantisation accuracy. "None" is the lowest possible value.
- Act Order: True or False. Also known as `desc_act`. True results in better quantisation accuracy. Some GPTQ clients have had issues with models that use Act Order plus Group Size, but this is generally resolved now.
- Damp %: A GPTQ parameter that affects how samples are processed for quantisation. 0.01 is default, but 0.1 results in slightly better accuracy.
- GPTQ dataset: The dataset used for quantisation. Using a dataset more appropriate to the model's training can improve quantisation accuracy. Note that the GPTQ dataset is not the same as the dataset used to train the model - please refer to the original model repo for details of the training dataset(s).
- Sequence Length: The length of the dataset sequences used for quantisation. Ideally this is the same as the model sequence length. For some very long sequence models (16+K), a lower sequence length may have to be used. Note that a lower sequence length does not limit the sequence length of the quantised model. It only impacts the quantisation accuracy on longer inference sequences.
- ExLlama Compatibility: Whether this file can be loaded with ExLlama, which currently only supports Llama models in 4-bit.
</details>
| Branch | Bits | GS | Act Order | Damp % | GPTQ Dataset | Seq Len | Size | ExLlama | Desc |
| ------ | ---- | -- | --------- | ------ | ------------ | ------- | ---- | ------- | ---- |
| [main](https://huggingface.co/TheBloke/WizardLM-13B-V1.2-GPTQ/tree/main) | 4 | 128 | No | 0.1 | [wikitext](https://huggingface.co/datasets/wikitext/viewer/wikitext-2-v1/test) | 4096 | 7.26 GB | Yes | 4-bit, without Act Order and group size 128g. |
| [gptq-4bit-32g-actorder_True](https://huggingface.co/TheBloke/WizardLM-13B-V1.2-GPTQ/tree/gptq-4bit-32g-actorder_True) | 4 | 32 | Yes | 0.1 | [wikitext](https://huggingface.co/datasets/wikitext/viewer/wikitext-2-v1/test) | 4096 | 8.00 GB | Yes | 4-bit, with Act Order and group size 32g. Gives highest possible inference quality, with maximum VRAM usage. |
| [gptq-4bit-64g-actorder_True](https://huggingface.co/TheBloke/WizardLM-13B-V1.2-GPTQ/tree/gptq-4bit-64g-actorder_True) | 4 | 64 | Yes | 0.1 | [wikitext](https://huggingface.co/datasets/wikitext/viewer/wikitext-2-v1/test) | 4096 | 7.51 GB | Yes | 4-bit, with Act Order and group size 64g. Uses less VRAM than 32g, but with slightly lower accuracy. |
| [gptq-4bit-128g-actorder_True](https://huggingface.co/TheBloke/WizardLM-13B-V1.2-GPTQ/tree/gptq-4bit-128g-actorder_True) | 4 | 128 | Yes | 0.1 | [wikitext](https://huggingface.co/datasets/wikitext/viewer/wikitext-2-v1/test) | 4096 | 7.26 GB | Yes | 4-bit, with Act Order and group size 128g. Uses even less VRAM than 64g, but with slightly lower accuracy. |
| [gptq-8bit--1g-actorder_True](https://huggingface.co/TheBloke/WizardLM-13B-V1.2-GPTQ/tree/gptq-8bit--1g-actorder_True) | 8 | None | Yes | 0.1 | [wikitext](https://huggingface.co/datasets/wikitext/viewer/wikitext-2-v1/test) | 4096 | 13.36 GB | No | 8-bit, with Act Order. No group size, to lower VRAM requirements. |
| [gptq-8bit-128g-actorder_False](https://huggingface.co/TheBloke/WizardLM-13B-V1.2-GPTQ/tree/gptq-8bit-128g-actorder_False) | 8 | 128 | No | 0.1 | [wikitext](https://huggingface.co/datasets/wikitext/viewer/wikitext-2-v1/test) | 4096 | 13.65 GB | No | 8-bit, with group size 128g for higher inference quality and without Act Order to improve AutoGPTQ speed. |
| [gptq-8bit-128g-actorder_True](https://huggingface.co/TheBloke/WizardLM-13B-V1.2-GPTQ/tree/gptq-8bit-128g-actorder_True) | 8 | 128 | Yes | 0.1 | [wikitext](https://huggingface.co/datasets/wikitext/viewer/wikitext-2-v1/test) | 4096 | 13.65 GB | No | 8-bit, with group size 128g for higher inference quality and with Act Order for even higher accuracy. |
| [gptq-8bit-64g-actorder_True](https://huggingface.co/TheBloke/WizardLM-13B-V1.2-GPTQ/tree/gptq-8bit-64g-actorder_True) | 8 | 64 | Yes | 0.1 | [wikitext](https://huggingface.co/datasets/wikitext/viewer/wikitext-2-v1/test) | 4096 | 13.95 GB | No | 8-bit, with group size 64g and Act Order for even higher inference quality. Poor AutoGPTQ CUDA speed. |
<!-- README_GPTQ.md-provided-files end -->
<!-- README_GPTQ.md-download-from-branches start -->
## How to download from branches
- In text-generation-webui, you can add `:branch` to the end of the download name, eg `TheBloke/WizardLM-13B-V1.2-GPTQ:main`
- With Git, you can clone a branch with:
```
git clone --single-branch --branch main https://huggingface.co/TheBloke/WizardLM-13B-V1.2-GPTQ
```
- In Python Transformers code, the branch is the `revision` parameter; see below.
<!-- README_GPTQ.md-download-from-branches end -->
<!-- README_GPTQ.md-text-generation-webui start -->
## How to easily download and use this model in [text-generation-webui](https://github.com/oobabooga/text-generation-webui).
Please make sure you're using the latest version of [text-generation-webui](https://github.com/oobabooga/text-generation-webui).
It is strongly recommended to use the text-generation-webui one-click-installers unless you're sure you know how to make a manual install.
1. Click the **Model tab**.
2. Under **Download custom model or LoRA**, enter `TheBloke/WizardLM-13B-V1.2-GPTQ`.
- To download from a specific branch, enter for example `TheBloke/WizardLM-13B-V1.2-GPTQ:main`
- see Provided Files above for the list of branches for each option.
3. Click **Download**.
4. The model will start downloading. Once it's finished it will say "Done".
5. In the top left, click the refresh icon next to **Model**.
6. In the **Model** dropdown, choose the model you just downloaded: `WizardLM-13B-V1.2-GPTQ`
7. The model will automatically load, and is now ready for use!
8. If you want any custom settings, set them and then click **Save settings for this model** followed by **Reload the Model** in the top right.
* Note that you do not need to and should not set manual GPTQ parameters any more. These are set automatically from the file `quantize_config.json`.
9. Once you're ready, click the **Text Generation tab** and enter a prompt to get started!
<!-- README_GPTQ.md-text-generation-webui end -->
<!-- README_GPTQ.md-use-from-python start -->
## How to use this GPTQ model from Python code
### Install the necessary packages
Requires: Transformers 4.32.0 or later, Optimum 1.12.0 or later, and AutoGPTQ 0.4.2 or later.
```shell
pip3 install transformers>=4.32.0 optimum>=1.12.0
pip3 install auto-gptq --extra-index-url https://huggingface.github.io/autogptq-index/whl/cu118/ # Use cu117 if on CUDA 11.7
```
If you have problems installing AutoGPTQ using the pre-built wheels, install it from source instead:
```shell
pip3 uninstall -y auto-gptq
git clone https://github.com/PanQiWei/AutoGPTQ
cd AutoGPTQ
pip3 install .
```
### For CodeLlama models only: you must use Transformers 4.33.0 or later.
If 4.33.0 is not yet released when you read this, you will need to install Transformers from source:
```shell
pip3 uninstall -y transformers
pip3 install git+https://github.com/huggingface/transformers.git
```
### You can then use the following code
```python
from transformers import AutoModelForCausalLM, AutoTokenizer, pipeline
model_name_or_path = "TheBloke/WizardLM-13B-V1.2-GPTQ"
# To use a different branch, change revision
# For example: revision="main"
model = AutoModelForCausalLM.from_pretrained(model_name_or_path,
device_map="auto",
trust_remote_code=False,
revision="main")
tokenizer = AutoTokenizer.from_pretrained(model_name_or_path, use_fast=True)
prompt = "Tell me about AI"
prompt_template=f'''A chat between a curious user and an artificial intelligence assistant. The assistant gives helpful, detailed, and polite answers to the user's questions. USER: {prompt} ASSISTANT:
'''
print("\n\n*** Generate:")
input_ids = tokenizer(prompt_template, return_tensors='pt').input_ids.cuda()
output = model.generate(inputs=input_ids, temperature=0.7, do_sample=True, top_p=0.95, top_k=40, max_new_tokens=512)
print(tokenizer.decode(output[0]))
# Inference can also be done using transformers' pipeline
print("*** Pipeline:")
pipe = pipeline(
"text-generation",
model=model,
tokenizer=tokenizer,
max_new_tokens=512,
do_sample=True,
temperature=0.7,
top_p=0.95,
top_k=40,
repetition_penalty=1.1
)
print(pipe(prompt_template)[0]['generated_text'])
```
<!-- README_GPTQ.md-use-from-python end -->
<!-- README_GPTQ.md-compatibility start -->
## Compatibility
The files provided are tested to work with AutoGPTQ, both via Transformers and using AutoGPTQ directly. They should also work with [Occ4m's GPTQ-for-LLaMa fork](https://github.com/0cc4m/KoboldAI).
[ExLlama](https://github.com/turboderp/exllama) is compatible with Llama models in 4-bit. Please see the Provided Files table above for per-file compatibility.
[Huggingface Text Generation Inference (TGI)](https://github.com/huggingface/text-generation-inference) is compatible with all GPTQ models.
<!-- README_GPTQ.md-compatibility end -->
<!-- footer start -->
<!-- 200823 -->
## Discord
For further support, and discussions on these models and AI in general, join us at:
[TheBloke AI's Discord server](https://discord.gg/theblokeai)
## Thanks, and how to contribute
Thanks to the [chirper.ai](https://chirper.ai) team!
Thanks to Clay from [gpus.llm-utils.org](llm-utils)!
I've had a lot of people ask if they can contribute. I enjoy providing models and helping people, and would love to be able to spend even more time doing it, as well as expanding into new projects like fine tuning/training.
If you're able and willing to contribute it will be most gratefully received and will help me to keep providing more models, and to start work on new AI projects.
Donaters will get priority support on any and all AI/LLM/model questions and requests, access to a private Discord room, plus other benefits.
* Patreon: https://patreon.com/TheBlokeAI
* Ko-Fi: https://ko-fi.com/TheBlokeAI
**Special thanks to**: Aemon Algiz.
**Patreon special mentions**: Alicia Loh, Stephen Murray, K, Ajan Kanaga, RoA, Magnesian, Deo Leter, Olakabola, Eugene Pentland, zynix, Deep Realms, Raymond Fosdick, Elijah Stavena, Iucharbius, Erik Bjäreholt, Luis Javier Navarrete Lozano, Nicholas, theTransient, John Detwiler, alfie_i, knownsqashed, Mano Prime, Willem Michiel, Enrico Ros, LangChain4j, OG, Michael Dempsey, Pierre Kircher, Pedro Madruga, James Bentley, Thomas Belote, Luke @flexchar, Leonard Tan, Johann-Peter Hartmann, Illia Dulskyi, Fen Risland, Chadd, S_X, Jeff Scroggin, Ken Nordquist, Sean Connelly, Artur Olbinski, Swaroop Kallakuri, Jack West, Ai Maven, David Ziegler, Russ Johnson, transmissions 11, John Villwock, Alps Aficionado, Clay Pascal, Viktor Bowallius, Subspace Studios, Rainer Wilmers, Trenton Dambrowitz, vamX, Michael Levine, 준교 김, Brandon Frisco, Kalila, Trailburnt, Randy H, Talal Aujan, Nathan Dryer, Vadim, 阿明, ReadyPlayerEmma, Tiffany J. Kim, George Stoitzev, Spencer Kim, Jerry Meng, Gabriel Tamborski, Cory Kujawski, Jeffrey Morgan, Spiking Neurons AB, Edmond Seymore, Alexandros Triantafyllidis, Lone Striker, Cap'n Zoog, Nikolai Manek, danny, ya boyyy, Derek Yates, usrbinkat, Mandus, TL, Nathan LeClaire, subjectnull, Imad Khwaja, webtim, Raven Klaugh, Asp the Wyvern, Gabriel Puliatti, Caitlyn Gatomon, Joseph William Delisle, Jonathan Leane, Luke Pendergrass, SuperWojo, Sebastain Graf, Will Dee, Fred von Graf, Andrey, Dan Guido, Daniel P. Andersen, Nitin Borwankar, Elle, Vitor Caleffi, biorpg, jjj, NimbleBox.ai, Pieter, Matthew Berman, terasurfer, Michael Davis, Alex, Stanislav Ovsiannikov
Thank you to all my generous patrons and donaters!
And thank you again to a16z for their generous grant.
<!-- footer end -->
# Original model card: WizardLM's WizardLM 13B V1.2
This is the **Full-Weight** of WizardLM-13B V1.2 model, this model is trained from **Llama-2 13b**.
## WizardLM: Empowering Large Pre-Trained Language Models to Follow Complex Instructions
<p align="center">
🤗 <a href="https://huggingface.co/WizardLM" target="_blank">HF Repo</a> •🐱 <a href="https://github.com/nlpxucan/WizardLM" target="_blank">Github Repo</a> • 🐦 <a href="https://twitter.com/WizardLM_AI" target="_blank">Twitter</a> • 📃 <a href="https://arxiv.org/abs/2304.12244" target="_blank">[WizardLM]</a> • 📃 <a href="https://arxiv.org/abs/2306.08568" target="_blank">[WizardCoder]</a> • 📃 <a href="https://arxiv.org/abs/2308.09583" target="_blank">[WizardMath]</a> <br>
</p>
<p align="center">
👋 Join our <a href="https://discord.gg/VZjjHtWrKs" target="_blank">Discord</a>
</p>
## News
- 🔥🔥🔥[2023/08/26] We released **WizardCoder-Python-34B-V1.0** , which achieves the **73.2 pass@1** and surpasses **GPT4 (2023/03/15)**, **ChatGPT-3.5**, and **Claude2** on the [HumanEval Benchmarks](https://github.com/openai/human-eval). For more details, please refer to [WizardCoder](https://github.com/nlpxucan/WizardLM/tree/main/WizardCoder).
- [2023/06/16] We released **WizardCoder-15B-V1.0** , which surpasses **Claude-Plus (+6.8)**, **Bard (+15.3)** and **InstructCodeT5+ (+22.3)** on the [HumanEval Benchmarks](https://github.com/openai/human-eval). For more details, please refer to [WizardCoder](https://github.com/nlpxucan/WizardLM/tree/main/WizardCoder).
| Model | Checkpoint | Paper | HumanEval | MBPP | Demo | License |
| ----- |------| ---- |------|-------| ----- | ----- |
| WizardCoder-Python-34B-V1.0 | 🤗 <a href="https://huggingface.co/WizardLM/WizardCoder-Python-34B-V1.0" target="_blank">HF Link</a> | 📃 <a href="https://arxiv.org/abs/2306.08568" target="_blank">[WizardCoder]</a> | 73.2 | 61.2 | [Demo](http://47.103.63.15:50085/) | <a href="https://ai.meta.com/resources/models-and-libraries/llama-downloads/" target="_blank">Llama2</a> |
| WizardCoder-15B-V1.0 | 🤗 <a href="https://huggingface.co/WizardLM/WizardCoder-15B-V1.0" target="_blank">HF Link</a> | 📃 <a href="https://arxiv.org/abs/2306.08568" target="_blank">[WizardCoder]</a> | 59.8 |50.6 | -- | <a href="https://huggingface.co/spaces/bigcode/bigcode-model-license-agreement" target="_blank">OpenRAIL-M</a> |
| WizardCoder-Python-13B-V1.0 | 🤗 <a href="https://huggingface.co/WizardLM/WizardCoder-Python-13B-V1.0" target="_blank">HF Link</a> | 📃 <a href="https://arxiv.org/abs/2306.08568" target="_blank">[WizardCoder]</a> | 64.0 | 55.6 | -- | <a href="https://ai.meta.com/resources/models-and-libraries/llama-downloads/" target="_blank">Llama2</a> |
| WizardCoder-Python-7B-V1.0 | 🤗 <a href="https://huggingface.co/WizardLM/WizardCoder-Python-7B-V1.0" target="_blank">HF Link</a> | 📃 <a href="https://arxiv.org/abs/2306.08568" target="_blank">[WizardCoder]</a> | 55.5 | 51.6 | [Demo](http://47.103.63.15:50088/) | <a href="https://ai.meta.com/resources/models-and-libraries/llama-downloads/" target="_blank">Llama2</a> |
| WizardCoder-3B-V1.0 | 🤗 <a href="https://huggingface.co/WizardLM/WizardCoder-3B-V1.0" target="_blank">HF Link</a> | 📃 <a href="https://arxiv.org/abs/2306.08568" target="_blank">[WizardCoder]</a> | 34.8 |37.4 | -- | <a href="https://huggingface.co/spaces/bigcode/bigcode-model-license-agreement" target="_blank">OpenRAIL-M</a> |
| WizardCoder-1B-V1.0 | 🤗 <a href="https://huggingface.co/WizardLM/WizardCoder-1B-V1.0" target="_blank">HF Link</a> | 📃 <a href="https://arxiv.org/abs/2306.08568" target="_blank">[WizardCoder]</a> | 23.8 |28.6 | -- | <a href="https://huggingface.co/spaces/bigcode/bigcode-model-license-agreement" target="_blank">OpenRAIL-M</a> |
- 🔥 [08/11/2023] We release **WizardMath** Models.
- 🔥 Our **WizardMath-70B-V1.0** model slightly outperforms some closed-source LLMs on the GSM8K, including **ChatGPT 3.5**, **Claude Instant 1** and **PaLM 2 540B**.
- 🔥 Our **WizardMath-70B-V1.0** model achieves **81.6 pass@1** on the [GSM8k Benchmarks](https://github.com/openai/grade-school-math), which is **24.8** points higher than the SOTA open-source LLM.
- 🔥 Our **WizardMath-70B-V1.0** model achieves **22.7 pass@1** on the [MATH Benchmarks](https://github.com/hendrycks/math), which is **9.2** points higher than the SOTA open-source LLM.
| Model | Checkpoint | Paper | GSM8k | MATH |Online Demo| License|
| ----- |------| ---- |------|-------| ----- | ----- |
| WizardMath-70B-V1.0 | 🤗 <a href="https://huggingface.co/WizardLM/WizardMath-70B-V1.0" target="_blank">HF Link</a> | 📃 <a href="https://arxiv.org/abs/2308.09583" target="_blank">[WizardMath]</a>| **81.6** | **22.7** |[Demo](http://47.103.63.15:50083/)| <a href="https://ai.meta.com/resources/models-and-libraries/llama-downloads/" target="_blank">Llama 2 </a> |
| WizardMath-13B-V1.0 | 🤗 <a href="https://huggingface.co/WizardLM/WizardMath-13B-V1.0" target="_blank">HF Link</a> | 📃 <a href="https://arxiv.org/abs/2308.09583" target="_blank">[WizardMath]</a>| **63.9** | **14.0** |[Demo](http://47.103.63.15:50082/)| <a href="https://ai.meta.com/resources/models-and-libraries/llama-downloads/" target="_blank">Llama 2 </a> |
| WizardMath-7B-V1.0 | 🤗 <a href="https://huggingface.co/WizardLM/WizardMath-7B-V1.0" target="_blank">HF Link</a> | 📃 <a href="https://arxiv.org/abs/2308.09583" target="_blank">[WizardMath]</a>| **54.9** | **10.7** | [Demo](http://47.103.63.15:50080/)| <a href="https://ai.meta.com/resources/models-and-libraries/llama-downloads/" target="_blank">Llama 2 </a>|
<font size=4>
| <sup>Model</sup> | <sup>Checkpoint</sup> | <sup>Paper</sup> |<sup>MT-Bench</sup> | <sup>AlpacaEval</sup> | <sup>WizardEval</sup> | <sup>HumanEval</sup> | <sup>License</sup>|
| ----- |------| ---- |------|-------| ----- | ----- | ----- |
| <sup>WizardLM-13B-V1.2</sup> | <sup>🤗 <a href="https://huggingface.co/WizardLM/WizardLM-13B-V1.2" target="_blank">HF Link</a> </sup>| | <sup>7.06</sup> | <sup>89.17%</sup> | <sup>101.4% </sup>|<sup>36.6 pass@1</sup>|<sup> <a href="https://ai.meta.com/resources/models-and-libraries/llama-downloads/" target="_blank">Llama 2 License </a></sup> |
| <sup>WizardLM-13B-V1.1</sup> |<sup> 🤗 <a href="https://huggingface.co/WizardLM/WizardLM-13B-V1.1" target="_blank">HF Link</a> </sup> | | <sup>6.76</sup> |<sup>86.32%</sup> | <sup>99.3% </sup> |<sup>25.0 pass@1</sup>| <sup>Non-commercial</sup>|
| <sup>WizardLM-30B-V1.0</sup> | <sup>🤗 <a href="https://huggingface.co/WizardLM/WizardLM-30B-V1.0" target="_blank">HF Link</a></sup> | | <sup>7.01</sup> | | <sup>97.8% </sup> | <sup>37.8 pass@1</sup>| <sup>Non-commercial</sup> |
| <sup>WizardLM-13B-V1.0</sup> | <sup>🤗 <a href="https://huggingface.co/WizardLM/WizardLM-13B-V1.0" target="_blank">HF Link</a> </sup> | | <sup>6.35</sup> | <sup>75.31%</sup> | <sup>89.1% </sup> |<sup> 24.0 pass@1 </sup> | <sup>Non-commercial</sup>|
| <sup>WizardLM-7B-V1.0 </sup>| <sup>🤗 <a href="https://huggingface.co/WizardLM/WizardLM-7B-V1.0" target="_blank">HF Link</a> </sup> |<sup> 📃 <a href="https://arxiv.org/abs/2304.12244" target="_blank">[WizardLM]</a> </sup>| | | <sup>78.0% </sup> |<sup>19.1 pass@1 </sup>|<sup> Non-commercial</sup>|
</font>
**Repository**: https://github.com/nlpxucan/WizardLM
**Twitter**:
- 🔥🔥🔥 [7/25/2023] We released **WizardLM V1.2** models. The **WizardLM-13B-V1.2** is here ([Demo_13B-V1.2](https://b7a19878988c8c73.gradio.app), [Demo_13B-V1.2_bak-1](https://d0a37a76e0ac4b52.gradio.app/), [Full Model Weight](https://huggingface.co/WizardLM/WizardLM-13B-V1.2)). Please checkout the [paper](https://arxiv.org/abs/2304.12244).
- 🔥🔥🔥 [7/25/2023] The **WizardLM-13B-V1.2** achieves **7.06** on [MT-Bench Leaderboard](https://chat.lmsys.org/?leaderboard), **89.17%** on [AlpacaEval Leaderboard](https://tatsu-lab.github.io/alpaca_eval/), and **101.4%** on [WizardLM Eval](https://github.com/nlpxucan/WizardLM/blob/main/WizardLM/data/WizardLM_testset.jsonl). (Note: MT-Bench and AlpacaEval are all self-test, will push update and request review. All tests are completed under their official settings.)
❗<b>Note for model system prompts usage:</b>
<b>WizardLM</b> adopts the prompt format from <b>Vicuna</b> and supports **multi-turn** conversation. The prompt should be as following:
```
A chat between a curious user and an artificial intelligence assistant. The assistant gives helpful, detailed, and polite answers to the user's questions. USER: Hi ASSISTANT: Hello.</s>USER: Who are you? ASSISTANT: I am WizardLM.</s>......
```
## Inference WizardLM Demo Script
We provide the inference WizardLM demo code [here](https://github.com/nlpxucan/WizardLM/tree/main/demo).
Please cite the paper if you use the data or code from WizardLM.
```
@article{xu2023wizardlm,
title={Wizardlm: Empowering large language models to follow complex instructions},
author={Xu, Can and Sun, Qingfeng and Zheng, Kai and Geng, Xiubo and Zhao, Pu and Feng, Jiazhan and Tao, Chongyang and Jiang, Daxin},
journal={arXiv preprint arXiv:2304.12244},
year={2023}
}
```
❗<b>To commen concern about dataset:</b>
Recently, there have been clear changes in the open-source policy and regulations of our overall organization's code, data, and models.
Despite this, we have still worked hard to obtain opening the weights of the model first, but the data involves stricter auditing and is in review with our legal team .
Our researchers have no authority to publicly release them without authorization.
Thank you for your understanding.
|
ChrisWilson011016/5EqUJqEL9hJMzVMuXfXDnKT2M5KonEKM1y9bH9STRyXofuvE_vgg | ChrisWilson011016 | "2024-02-29T14:11:29Z" | 967 | 0 | keras | [
"keras",
"region:us"
] | null | "2024-02-24T15:09:05Z" | Entry not found |
John6666/iniverse-mix-xl-sfwnsfw-guofen-v15-sdxl | John6666 | "2024-06-30T13:34:55Z" | 967 | 0 | diffusers | [
"diffusers",
"safetensors",
"text-to-image",
"stable-diffusion",
"stable-diffusion-xl",
"realistic",
"photorealistic",
"license:creativeml-openrail-m",
"autotrain_compatible",
"endpoints_compatible",
"diffusers:StableDiffusionXLPipeline",
"region:us"
] | text-to-image | "2024-06-30T13:26:32Z" | ---
license: creativeml-openrail-m
tags:
- text-to-image
- stable-diffusion
- stable-diffusion-xl
- realistic
- photorealistic
---
Original model is [here](https://civitai.com/models/226533/iniverse-mix-xlsfw-and-nsfw?modelVersionId=608842).
|
sagawa/PubChem-10m-t5-v2 | sagawa | "2022-12-11T05:16:44Z" | 966 | 0 | transformers | [
"transformers",
"pytorch",
"jax",
"t5",
"text2text-generation",
"dataset:sagawa/pubchem-10m-canonicalized",
"license:mit",
"model-index",
"autotrain_compatible",
"endpoints_compatible",
"text-generation-inference",
"region:us"
] | text2text-generation | "2022-11-06T01:13:43Z" | ---
license: mit
datasets:
- sagawa/pubchem-10m-canonicalized
metrics:
- accuracy
model-index:
- name: PubChem-10m-t5
results:
- task:
name: Masked Language Modeling
type: fill-mask
dataset:
name: sagawa/pubchem-10m-canonicalized
type: sagawa/pubchem-10m-canonicalized
metrics:
- name: Accuracy
type: accuracy
value: 0.9189779162406921
---
# PubChem-10m-t5
This model is a fine-tuned version of [google/t5-v1_1-base](https://huggingface.co/microsoft/deberta-base) on the sagawa/pubchem-10m-canonicalized dataset.
It achieves the following results on the evaluation set:
- Loss: 0.2165
- Accuracy: 0.9190
## Model description
We trained t5 on SMILES from PubChem using the task of masked-language modeling (MLM). Compared to PubChem-10m-t5, PubChem-10m-t5-v2 uses a character-level tokenizer, and it was also trained on PubChem.
## Intended uses & limitations
This model can be used for the prediction of molecules' properties, reactions, or interactions with proteins by changing the way of finetuning.
## Training and evaluation data
We downloaded [PubChem data](https://drive.google.com/file/d/1ygYs8dy1-vxD1Vx6Ux7ftrXwZctFjpV3/view) and canonicalized them using RDKit. Then, we dropped duplicates. The total number of data is 9999960, and they were randomly split into train:validation=10:1.
## Training procedure
### Training hyperparameters
The following hyperparameters were used during training:
- learning_rate: 5e-03
- seed: 42
- optimizer: Adam with betas=(0.9,0.999) and epsilon=1e-08
- lr_scheduler_type: linear
- num_epochs: 10.0
### Training results
| Training Loss | Step | Accuracy | Validation Loss |
|:-------------:|:------:|:--------:|:---------------:|
| 0.2592 | 100000 | 0.8997 | 0.2784 |
| 0.2790 | 200000 | 0.9095 | 0.2468 |
| 0.2278 | 300000 | 0.9162 | 0.2256 | |
sprylabadmin/paraphrase-multilingual-MiniLM-L12-v2-fine-tuned-2-onnx-quantized | sprylabadmin | "2023-05-29T10:34:20Z" | 966 | 0 | transformers | [
"transformers",
"onnx",
"bert",
"feature-extraction",
"endpoints_compatible",
"text-embeddings-inference",
"region:us"
] | feature-extraction | "2023-05-29T09:57:49Z" | Entry not found |
nfliu/deberta-v3-large_boolq | nfliu | "2023-09-08T05:40:57Z" | 966 | 0 | transformers | [
"transformers",
"pytorch",
"safetensors",
"deberta-v2",
"text-classification",
"generated_from_trainer",
"dataset:boolq",
"base_model:microsoft/deberta-v3-large",
"license:mit",
"model-index",
"autotrain_compatible",
"endpoints_compatible",
"region:us"
] | text-classification | "2023-09-07T05:55:24Z" | ---
license: mit
base_model: microsoft/deberta-v3-large
tags:
- generated_from_trainer
datasets:
- boolq
metrics:
- accuracy
model-index:
- name: deberta-v3-large_boolq
results:
- task:
name: Text Classification
type: text-classification
dataset:
name: boolq
type: boolq
config: default
split: validation
args: default
metrics:
- name: Accuracy
type: accuracy
value: 0.8834862385321101
---
<!-- This model card has been generated automatically according to the information the Trainer had access to. You
should probably proofread and complete it, then remove this comment. -->
# deberta-v3-large_boolq
This model is a fine-tuned version of [microsoft/deberta-v3-large](https://huggingface.co/microsoft/deberta-v3-large) on the boolq dataset.
It achieves the following results on the evaluation set:
- Loss: 0.4601
- Accuracy: 0.8835
## Model description
More information needed
## Example
```
import torch
from transformers import AutoModelForSequenceClassification, AutoTokenizer
model = AutoModelForSequenceClassification.from_pretrained("nfliu/deberta-v3-large_boolq")
tokenizer = AutoTokenizer.from_pretrained("nfliu/deberta-v3-large_boolq")
# Each example is a (question, context) pair.
examples = [
("Lake Tahoe is in California", "Lake Tahoe is a popular tourist spot in California."),
("Water is wet", "Contrary to popular belief, water is not wet.")
]
encoded_input = tokenizer(examples, padding=True, truncation=True, return_tensors="pt")
with torch.no_grad():
model_output = model(**encoded_input)
probabilities = torch.softmax(model_output.logits, dim=-1).cpu().tolist()
probability_no = [round(prob[0], 2) for prob in probabilities]
probability_yes = [round(prob[1], 2) for prob in probabilities]
for example, p_no, p_yes in zip(examples, probability_no, probability_yes):
print(f"Question: {example[0]}")
print(f"Context: {example[1]}")
print(f"p(No | question, context): {p_no}")
print(f"p(Yes | question, context): {p_yes}")
print()
```
## Intended uses & limitations
More information needed
## Training and evaluation data
More information needed
## Training procedure
### Training hyperparameters
The following hyperparameters were used during training:
- learning_rate: 1e-05
- train_batch_size: 16
- eval_batch_size: 8
- seed: 42
- gradient_accumulation_steps: 2
- total_train_batch_size: 32
- optimizer: Adam with betas=(0.9,0.999) and epsilon=1e-08
- lr_scheduler_type: linear
- num_epochs: 5.0
### Training results
| Training Loss | Epoch | Step | Validation Loss | Accuracy |
|:-------------:|:-----:|:----:|:---------------:|:--------:|
| No log | 0.85 | 250 | 0.5306 | 0.8823 |
| 0.1151 | 1.69 | 500 | 0.4601 | 0.8835 |
| 0.1151 | 2.54 | 750 | 0.5897 | 0.8792 |
| 0.0656 | 3.39 | 1000 | 0.6477 | 0.8804 |
| 0.0656 | 4.24 | 1250 | 0.6847 | 0.8838 |
### Framework versions
- Transformers 4.32.1
- Pytorch 2.0.1+cu117
- Datasets 2.14.4
- Tokenizers 0.13.3
|
LumiOpen/Viking-33B | LumiOpen | "2024-07-02T10:01:08Z" | 966 | 13 | transformers | [
"transformers",
"safetensors",
"llama",
"text-generation",
"fi",
"en",
"da",
"sv",
"no",
"nn",
"is",
"dataset:cerebras/SlimPajama-627B",
"dataset:bigcode/starcoderdata",
"dataset:mc4",
"license:apache-2.0",
"autotrain_compatible",
"endpoints_compatible",
"text-generation-inference",
"region:us"
] | text-generation | "2024-02-20T15:07:18Z" | ---
license: apache-2.0
datasets:
- cerebras/SlimPajama-627B
- bigcode/starcoderdata
- mc4
language:
- fi
- en
- da
- sv
- 'no'
- nn
- is
---
# Viking 33B
_**NOTE:** This is a **research checkpoint** of a model for which **training has not been completed.** It is being provided in its current state for research and testing purposes. **Care should be taken when using the outputs of the model.** Once pretraining has completed we intend to release additional instruction-tuned and chat-tuned varieties._
Viking 33B is a 33B parameter decoder-only transformer pretrained on Finnish,
English, Swedish, Danish, Norwegian, Icelandic and code. It is being trained
on 2 trillion tokens (1300B billion as of this release). Viking 33B is a fully open source model and is made available under the Apache 2.0 License.
Viking was created in a collaboration between the [TurkuNLP group](https://turkunlp.org/) of the University of Turku, [SiloGen](https://www.silo.ai/silogen) from [Silo AI](https://www.silo.ai/),and [High Performance Language Technologies](https://hplt-project.org/) (HPLT). Training was conducted on the [LUMI supercomputer](https://www.lumi-supercomputer.eu/), using compute resources generously provided by [CSC](https://csc.fi/) - IT Center for Science, Finland.
This project is part of an ongoing effort to create open source large language models for non-English and especially low resource languages like Finnish. The mode is fluent in Finnish, English, the Scandinavian languages and capable of basic translation between them. It is also able to understand and generate code.
## Model Family
Viking is the second set of models released by LumiOpen and is available at
3 parameter counts:
[Viking 7B](https://huggingface.co/LumiOpen/Viking-7B)
[Viking 13B](https://huggingface.co/LumiOpen/Viking-13B)
[Viking 33B](https://huggingface.co/LumiOpen/Viking-33B)
## Model Overview
_**NOTE:** In addition to being an early research release, Viking is a base model which needs further fine tuning for most use cases._
Viking is a generative pretrained transformer using a LLaMA-like GPT architecture, and makes use of rotary positional embeddings and flash attention.
| Hyperparameter | Value |
| :------------- | :----: |
| n_parameters | 33B |
| n_layers | 56 |
| n_heads | 56 |
| d_model | 7168 |
| vocab_size | 131072 |
| sequence_length | 4096 |
## Training
Viking 33B was trained on the LUMI supercomputer, using 1024 AMD MI250X GPUs. Each MI250X GPU has two Graphics Complex Dies (GCDs) for a world size of 2048 during training, using activation checkpointing, a micro batch size of 1, gradient accumulation of 16, and a 3D parallelism strategy of TP=4, PP=4, DP=128.
Training began in September 2023 using a [custom fork](https://github.com/LumiOpen/Megatron-DeepSpeed) of the Megatron-Deepspeed framework.
## Training Hyperparameters
| Hyperparameter | Value | Comment |
| :------------: | :---: | :------:|
| Precision | bfloat16 | |
| Optimizer | AdamW | |
| Learning rate | 3e-4 | 10B tokens warm-up, cosine decay to 3e-5 |
| Weight decay | 1e-1 | |
| Batch size | 1024 | 1024 samples x 4096 tokens = 4194304 tokens |
## Tokenizer
Viking uses a custom 128K Bloom tokenizer trained on the same English, Finnish, Swedish, Danish, Norwegian, Icelandic and code dataset used to train the model.
## Dataset
Viking is being trained on a 2 trillion token mixed dataset of English, Finnish, Swedish, Danish, Norwegian, Icelandic and code.
Full details will be published soon.
## Evaluation Results
Full evaluation results will be published with the final model.
## Training Checkpoints
Training checkpoints are available as branches in the repository. Checkpoints will be released roughly every 100B tokens. The main branch will always point to the latest checkpoint. The following checkpoints are available:
* [100B](https://huggingface.co/LumiOpen/Viking-33B/tree/100B)
* [200B](https://huggingface.co/LumiOpen/Viking-33B/tree/200B)
* [300B](https://huggingface.co/LumiOpen/Viking-33B/tree/300B)
* [400B](https://huggingface.co/LumiOpen/Viking-33B/tree/400B)
* [500B](https://huggingface.co/LumiOpen/Viking-33B/tree/500B)
* [600B](https://huggingface.co/LumiOpen/Viking-33B/tree/600B)
* [700B](https://huggingface.co/LumiOpen/Viking-33B/tree/700B)
* [800B](https://huggingface.co/LumiOpen/Viking-33B/tree/800B)
* [900B](https://huggingface.co/LumiOpen/Viking-33B/tree/900B)
* [1000B](https://huggingface.co/LumiOpen/Viking-33B/tree/1000B)
* [1100B](https://huggingface.co/LumiOpen/Viking-33B/tree/1100B)
* [1200B](https://huggingface.co/LumiOpen/Viking-33B/tree/1200B)
* [1300B](https://huggingface.co/LumiOpen/Viking-33B/tree/1300B)
The transformers library allows you to load a checkpoint from a branch as follows:
```python
branch = "200B"
model = transformers.AutoModelForCausalLM.from_pretrained(
"LumiOpen/Viking-33B",
torch_dtype=torch.bfloat16,
revision=branch,
)
```
## Ethical Considerations and Limitations
_Viking 33B is a release of a partially trained model, and special care should be taken when using any output._
Viking is an advanced language model, primarily optimized for English, Finnish, Swedish, Norwegian, Danish, Icelandic and code, with no meaningful proficiency in any other languages. As with most AI-driven systems, Viking is a product of the vast data it has been trained on, which may reflect the imperfections, biases, and idiosyncrasies of the wider web. Viking may, at times, produce outputs that can be considered inaccurate, prejudiced, or controversial. Users and developers engaging with Viking should exercise discretion and consider additional evaluation and customization to ensure the model's responses align with their specific needs and ethical standards.
## License
Viking is released under the Apache 2.0 license.
|
jan-hq/komodo-7b-chat | jan-hq | "2024-03-18T07:47:05Z" | 966 | 1 | transformers | [
"transformers",
"safetensors",
"llama",
"text-generation",
"en",
"license:apache-2.0",
"autotrain_compatible",
"endpoints_compatible",
"text-generation-inference",
"region:us"
] | text-generation | "2024-03-18T07:33:19Z" | ---
language:
- en
license: apache-2.0
---
<!-- header start -->
<!-- 200823 -->
<div style="width: auto; margin-left: auto; margin-right: auto"
>
<img src="https://github.com/janhq/jan/assets/89722390/35daac7d-b895-487c-a6ac-6663daaad78e" alt="Jan banner"
style="width: 100%; min-width: 400px; display: block; margin: auto;">
</div>
<p align="center">
<a href="https://jan.ai/">Jan</a
>
- <a href="https://discord.gg/AsJ8krTT3N">Discord</a>
</p>
<!-- header end -->
# Prompt template
ChatML
```
<|im_start|>system
{system_message}<|im_end|>
<|im_start|>user
{prompt}<|im_end|>
<|im_start|>assistant
```
# Run this model
You can run this model using [Jan Desktop](https://jan.ai/) on Mac, Windows, or Linux.
Jan is an open source, ChatGPT alternative that is:
- 💻 **100% offline on your machine**: Your conversations remain confidential, and visible only to you.
- 🗂️ **
An Open File Format**: Conversations and model settings stay on your computer and can be exported or deleted at any time.
- 🌐 **OpenAI Compatible**: Local server on port `1337` with OpenAI compatible endpoints
- 🌍 **Open Source & Free**: We build in public; check out our [Github](https://github.com/janhq)

# About Jan
Jan believes in the need for an open-source AI ecosystem and is building the infra and tooling to allow open-source AIs to compete on a level playing field with proprietary ones.
Jan's long-term vision is to build a cognitive framework for future robots, who are practical, useful assistants for humans and businesses in everyday life.
|
nnheui/stablelm-2-1_6b-sft-full | nnheui | "2024-04-21T14:57:47Z" | 966 | 0 | transformers | [
"transformers",
"tensorboard",
"safetensors",
"stablelm",
"text-generation",
"alignment-handbook",
"trl",
"sft",
"generated_from_trainer",
"conversational",
"dataset:HuggingFaceH4/ultrachat_200k",
"base_model:stabilityai/stablelm-2-1_6b",
"license:other",
"autotrain_compatible",
"endpoints_compatible",
"region:us"
] | text-generation | "2024-04-21T09:09:22Z" | ---
license: other
base_model: stabilityai/stablelm-2-1_6b
tags:
- alignment-handbook
- trl
- sft
- generated_from_trainer
- trl
- sft
- generated_from_trainer
datasets:
- HuggingFaceH4/ultrachat_200k
model-index:
- name: stablelm-2-1_6b-sft-full
results: []
---
<!-- This model card has been generated automatically according to the information the Trainer had access to. You
should probably proofread and complete it, then remove this comment. -->
# stablelm-2-1_6b-sft-full
This model is a fine-tuned version of [stabilityai/stablelm-2-1_6b](https://huggingface.co/stabilityai/stablelm-2-1_6b) on the HuggingFaceH4/ultrachat_200k dataset.
## Model description
More information needed
## Intended uses & limitations
More information needed
## Training and evaluation data
More information needed
## Training procedure
### Training hyperparameters
The following hyperparameters were used during training:
- learning_rate: 2e-05
- train_batch_size: 4
- eval_batch_size: 8
- seed: 42
- distributed_type: multi-GPU
- num_devices: 2
- gradient_accumulation_steps: 16
- total_train_batch_size: 128
- total_eval_batch_size: 16
- optimizer: Adam with betas=(0.9,0.999) and epsilon=1e-08
- lr_scheduler_type: cosine
- lr_scheduler_warmup_ratio: 0.1
- num_epochs: 1
### Training results
### Framework versions
- Transformers 4.40.0
- Pytorch 2.1.2
- Datasets 2.18.0
- Tokenizers 0.19.1
|
Qwen/Qwen2-1.5B-Instruct-GPTQ-Int4 | Qwen | "2024-06-10T03:04:10Z" | 966 | 2 | transformers | [
"transformers",
"safetensors",
"qwen2",
"text-generation",
"chat",
"conversational",
"en",
"license:apache-2.0",
"autotrain_compatible",
"endpoints_compatible",
"text-generation-inference",
"4-bit",
"gptq",
"region:us"
] | text-generation | "2024-06-06T06:16:04Z" | ---
license: apache-2.0
language:
- en
pipeline_tag: text-generation
tags:
- chat
---
# Qwen2-1.5B-Instruct-GPTQ-Int4
## Introduction
Qwen2 is the new series of Qwen large language models. For Qwen2, we release a number of base language models and instruction-tuned language models ranging from 0.5 to 72 billion parameters, including a Mixture-of-Experts model. This repo contains the instruction-tuned 1.5B Qwen2 model.
Compared with the state-of-the-art opensource language models, including the previous released Qwen1.5, Qwen2 has generally surpassed most opensource models and demonstrated competitiveness against proprietary models across a series of benchmarks targeting for language understanding, language generation, multilingual capability, coding, mathematics, reasoning, etc.
For more details, please refer to our [blog](https://qwenlm.github.io/blog/qwen2/), [GitHub](https://github.com/QwenLM/Qwen2), and [Documentation](https://qwen.readthedocs.io/en/latest/).
**Note**: If you encounter ``RuntimeError: probability tensor contains either `inf`, `nan` or element < 0`` during inference with ``transformers``, we recommand installing ``autogpq>=0.7.1`` or [deploying this model with vLLM](https://qwen.readthedocs.io/en/latest/deployment/vllm.html).
<br>
## Model Details
Qwen2 is a language model series including decoder language models of different model sizes. For each size, we release the base language model and the aligned chat model. It is based on the Transformer architecture with SwiGLU activation, attention QKV bias, group query attention, etc. Additionally, we have an improved tokenizer adaptive to multiple natural languages and codes.
## Training details
We pretrained the models with a large amount of data, and we post-trained the models with both supervised finetuning and direct preference optimization.
## Requirements
The code of Qwen2 has been in the latest Hugging face transformers and we advise you to install `transformers>=4.37.0`, or you might encounter the following error:
```
KeyError: 'qwen2'
```
## Quickstart
Here provides a code snippet with `apply_chat_template` to show you how to load the tokenizer and model and how to generate contents.
```python
from transformers import AutoModelForCausalLM, AutoTokenizer
device = "cuda" # the device to load the model onto
model = AutoModelForCausalLM.from_pretrained(
"Qwen/Qwen2-1.5B-Instruct-GPTQ-Int4",
torch_dtype="auto",
device_map="auto"
)
tokenizer = AutoTokenizer.from_pretrained("Qwen/Qwen2-1.5B-Instruct-GPTQ-Int4")
prompt = "Give me a short introduction to large language model."
messages = [
{"role": "system", "content": "You are a helpful assistant."},
{"role": "user", "content": prompt}
]
text = tokenizer.apply_chat_template(
messages,
tokenize=False,
add_generation_prompt=True
)
model_inputs = tokenizer([text], return_tensors="pt").to(device)
generated_ids = model.generate(
model_inputs.input_ids,
max_new_tokens=512
)
generated_ids = [
output_ids[len(input_ids):] for input_ids, output_ids in zip(model_inputs.input_ids, generated_ids)
]
response = tokenizer.batch_decode(generated_ids, skip_special_tokens=True)[0]
```
## Benchmark and Speed
To compare the generation performance between bfloat16 (bf16) and quantized models such as GPTQ-Int8, GPTQ-Int4, and AWQ, please consult our [Benchmark of Quantized Models](https://qwen.readthedocs.io/en/latest/benchmark/quantization_benchmark.html). This benchmark provides insights into how different quantization techniques affect model performance.
For those interested in understanding the inference speed and memory consumption when deploying these models with either ``transformer`` or ``vLLM``, we have compiled an extensive [Speed Benchmark](https://qwen.readthedocs.io/en/latest/benchmark/speed_benchmark.html).
## Citation
If you find our work helpful, feel free to give us a cite.
```
@article{qwen2,
title={Qwen2 Technical Report},
year={2024}
}
``` |
BaunRobotics/Qwen-tinybaun-k12-GGUF | BaunRobotics | "2024-06-25T13:14:08Z" | 966 | 0 | null | [
"gguf",
"region:us"
] | null | "2024-06-25T11:47:26Z" | Entry not found |
valhalla/s2t_mustc_multilinguial_medium | valhalla | "2021-03-03T05:12:34Z" | 965 | 0 | transformers | [
"transformers",
"pytorch",
"speech_to_text_transformer",
"text2text-generation",
"autotrain_compatible",
"endpoints_compatible",
"region:us"
] | text2text-generation | "2022-03-02T23:29:05Z" | Entry not found |
stabilityai/japanese-stablelm-base-alpha-7b | stabilityai | "2023-08-22T09:36:29Z" | 965 | 116 | transformers | [
"transformers",
"pytorch",
"text-generation",
"japanese-stablelm",
"causal-lm",
"custom_code",
"ja",
"dataset:wikipedia",
"dataset:mc4",
"dataset:cc100",
"dataset:oscar-corpus/OSCAR-2301",
"dataset:oscar-corpus/OSCAR-2201",
"dataset:togethercomputer/RedPajama-Data-1T",
"license:apache-2.0",
"autotrain_compatible",
"region:us"
] | text-generation | "2023-08-09T14:30:09Z" | ---
language:
- ja
tags:
- japanese-stablelm
- causal-lm
pipeline_tag: text-generation
datasets:
- wikipedia
- mc4
- cc100
- oscar-corpus/OSCAR-2301
- oscar-corpus/OSCAR-2201
- togethercomputer/RedPajama-Data-1T
license:
- apache-2.0
---
# Japanese-StableLM-Base-Alpha-7B

> "A parrot able to speak Japanese, ukiyoe, edo period" — [Stable Diffusion XL](https://clipdrop.co/stable-diffusion)
## Model Description
`japanese-stablelm-base-alpha-7b` is a 7B-parameter decoder-only language model pre-trained on a diverse collection of Japanese and English datasets which focus on maximizing Japanese language modeling performance and Japanese downstream task performance.
For an instruction-following model, check [Japanese-StableLM-Instruct-Alpha-7B](https://huggingface.co/stabilityai/japanese-stablelm-instruct-alpha-7b) and get access by accepting the terms and conditions.
## Usage
First install additional dependencies in [requirements.txt](./requirements.txt):
```sh
pip install sentencepiece einops
```
Then start generating text with `japanese-stablelm-base-alpha-7b` by using the following code snippet:
```python
import torch
from transformers import LlamaTokenizer, AutoModelForCausalLM
tokenizer = LlamaTokenizer.from_pretrained("novelai/nerdstash-tokenizer-v1", additional_special_tokens=['▁▁'])
model = AutoModelForCausalLM.from_pretrained(
"stabilityai/japanese-stablelm-base-alpha-7b",
trust_remote_code=True,
)
model.half()
model.eval()
if torch.cuda.is_available():
model = model.to("cuda")
prompt = """
AI で科学研究を加速するには、
""".strip()
input_ids = tokenizer.encode(
prompt,
add_special_tokens=False,
return_tensors="pt"
)
# this is for reproducibility.
# feel free to change to get different result
seed = 23
torch.manual_seed(seed)
tokens = model.generate(
input_ids.to(device=model.device),
max_new_tokens=128,
temperature=1,
top_p=0.95,
do_sample=True,
)
out = tokenizer.decode(tokens[0], skip_special_tokens=True)
print(out)
"""
AI で科学研究を加速するには、データ駆動型文化が必要であることも明らかになってきています。研究のあらゆる側面で、データがより重要になっているのです。
20 世紀の科学は、研究者が直接研究を行うことで、研究データを活用してきました。その後、多くの科学分野ではデータは手動で分析されるようになったものの、これらの方法には多大なコストと労力がかかることが分かりました。 そこで、多くの研究者や研究者グループは、より効率的な手法を開発し、研究の規模を拡大してきました。21 世紀になると、研究者が手動で実施する必要のある研究は、その大部分を研究者が自動化できるようになりました。
"""
```
We suggest playing with different generation config (`top_p`, `repetition_penalty` etc) to find the best setup for your tasks. For example, use higher temperature for roleplay task, lower temperature for reasoning.
## Model Details
* **Model type**: `japanese-stablelm-base-alpha-7b` model is an auto-regressive language model based on the NeoX transformer architecture.
* **Language(s)**: Japanese
* **Library**: [GPT-NeoX](https://github.com/EleutherAI/gpt-neox)
* **License**: This model is licensed under [Apache License, Version 2.0](https://www.apache.org/licenses/LICENSE-2.0).
## Training
| Parameters | Hidden Size | Layers | Heads | Sequence Length |
|------------|-------------|--------|-------|-----------------|
| 7B | 4096 | 32 | 32 | 2048 |
### Training Dataset
`japanese-stablelm-base-alpha-7b` is pre-trained on around 750B tokens from a mixture of the following corpora:
- [Japanese/English Wikipedia](https://dumps.wikimedia.org/other/cirrussearch)
- [Japanese mc4](https://huggingface.co/datasets/mc4)
- [Japanese CC-100](http://data.statmt.org/cc-100/ja.txt.xz)
- [Japanese OSCAR](https://oscar-project.github.io/documentation/)
- [RedPajama](https://huggingface.co/datasets/togethercomputer/RedPajama-Data-1T)
## Use and Limitations
### Intended Use
The model is intended to be used by all individuals as foundational models for application-specific fine-tuning without strict limitations on commercial use.
### Limitations and bias
The pre-training dataset may have contained offensive or inappropriate content even after applying data cleansing filters which can be reflected in the model generated text. We recommend users exercise reasonable caution when using these models in production systems. Do not use the model for any applications that may cause harm or distress to individuals or groups.
## Authors
- [Meng Lee](https://huggingface.co/leemeng)
- [Fujiki Nakamura](https://huggingface.co/fujiki)
- [Makoto Shing](https://huggingface.co/mkshing)
- [Paul McCann](https://huggingface.co/polm-stability)
- [Takuya Akiba](https://huggingface.co/iwiwi)
- [Naoki Orii](https://huggingface.co/mrorii)
## Acknowledgements
We are utilizing the v1 version of the [novelai-tokenizer](https://github.com/NovelAI/novelai-tokenizer), introduced by [NovelAI](https://novelai.net/), because it processes both Japanese and English text effectively and efficiently. We extend our gratitude to NovelAI for allowing us to use their remarkable work. For more details about the tokenizer, please refer to their [blog post](https://blog.novelai.net/novelais-new-llm-tokenizer-5bc140e17642).
We are grateful for the contributions of the EleutherAI Polyglot-JA team in helping us to collect a large amount of pre-training data in Japanese. Polyglot-JA members includes Hyunwoong Ko (Project Lead), Fujiki Nakamura (originally started this project when he commited to the Polyglot team), Yunho Mo, Minji Jung, KeunSeok Im, and Su-Kyeong Jang.
We are also appreciative of [AI Novelist/Sta (Bit192, Inc.)](https://ai-novel.com/index.php) and the numerous contributors from [Stable Community Japan](https://discord.gg/VPrcE475HB) for assisting us in gathering a large amount of high-quality Japanese textual data for model training.
## How to cite
```
@misc{JapaneseStableLMBaseAlpha7B,
url={[https://huggingface.co/stabilityai/japanese-stablelm-base-alpha-7b](https://huggingface.co/stabilityai/japanese-stablelm-base-alpha-7b)},
title={Japanese StableLM Base Alpha 7B},
author={Lee, Meng and Nakamura, Fujiki and Shing, Makoto and McCann, Paul and Akiba, Takuya and Orii, Naoki}
}
```
## Citations
```bibtext
@software{gpt-neox-library,
title = {{GPT-NeoX: Large Scale Autoregressive Language Modeling in PyTorch}},
author = {Andonian, Alex and Anthony, Quentin and Biderman, Stella and Black, Sid and Gali, Preetham and Gao, Leo and Hallahan, Eric and Levy-Kramer, Josh and Leahy, Connor and Nestler, Lucas and Parker, Kip and Pieler, Michael and Purohit, Shivanshu and Songz, Tri and Phil, Wang and Weinbach, Samuel},
url = {https://www.github.com/eleutherai/gpt-neox},
doi = {10.5281/zenodo.5879544},
month = {8},
year = {2021},
version = {0.0.1},
}
``` |
arnavgrg/llama-2-13b-chat-nf4-fp16-upscaled | arnavgrg | "2023-12-12T19:07:25Z" | 965 | 0 | transformers | [
"transformers",
"safetensors",
"llama",
"text-generation",
"text-generation-inference",
"conversational",
"license:apache-2.0",
"autotrain_compatible",
"endpoints_compatible",
"region:us"
] | text-generation | "2023-12-05T16:21:41Z" | ---
license: apache-2.0
tags:
- text-generation-inference
---
This is an upscaled fp16 variant of the original Llama-2-13b-chat base model by Meta after it has been loaded with nf4 4-bit quantization via bitsandbytes.
The main idea here is to upscale the linear4bit layers to fp16 so that the quantization/dequantization cost doesn't have to paid for each forward pass at inference time.
_Note: The quantization operation to nf4 is not lossless, so the model weights for the linear layers are lossy, which means that this model will not work as well as the official base model._
To use this model, you can just load it via `transformers` in fp16:
```python
import torch
from transformers import AutoModelForCausalLM
model = AutoModelForCausalLM.from_pretrained(
"arnavgrg/llama-2-13b-chat-nf4-fp16-upscaled",
device_map="auto",
torch_dtype=torch.float16
)
``` |
ALBADDAWI/DeepCode-7B-Aurora-v2 | ALBADDAWI | "2024-04-10T14:01:31Z" | 965 | 0 | transformers | [
"transformers",
"safetensors",
"llama",
"text-generation",
"deepseek-ai/deepseek-math-7b-instruct",
"deepseek-ai/deepseek-math-7b-base",
"deepseek-ai/deepseek-math-7b-rl",
"conversational",
"base_model:deepseek-ai/deepseek-math-7b-instruct",
"license:mit",
"autotrain_compatible",
"endpoints_compatible",
"text-generation-inference",
"region:us"
] | text-generation | "2024-04-10T01:20:15Z" | ---
tags:
- deepseek-ai/deepseek-math-7b-instruct
- deepseek-ai/deepseek-math-7b-base
- deepseek-ai/deepseek-math-7b-rl
base_model:
- deepseek-ai/deepseek-math-7b-instruct
- deepseek-ai/deepseek-math-7b-base
- deepseek-ai/deepseek-math-7b-rl
- deepseek-ai/deepseek-math-7b-rl
- deepseek-ai/deepseek-math-7b-rl
- deepseek-ai/deepseek-math-7b-rl
license: mit
---
# DeepCode-7B-Aurora-v2
DeepCode-7B-Aurora-v2 is a merge of the following models using [LazyMergekit](https://colab.research.google.com/drive/1obulZ1ROXHjYLn6PPZJwRR6GzgQogxxb?usp=sharing):
* [deepseek-ai/deepseek-math-7b-instruct](https://huggingface.co/deepseek-ai/deepseek-math-7b-instruct)
* [deepseek-ai/deepseek-math-7b-base](https://huggingface.co/deepseek-ai/deepseek-math-7b-base)
* [deepseek-ai/deepseek-math-7b-rl](https://huggingface.co/deepseek-ai/deepseek-math-7b-rl)
* [deepseek-ai/deepseek-math-7b-rl](https://huggingface.co/deepseek-ai/deepseek-math-7b-rl)
* [deepseek-ai/deepseek-math-7b-rl](https://huggingface.co/deepseek-ai/deepseek-math-7b-rl)
* [deepseek-ai/deepseek-math-7b-rl](https://huggingface.co/deepseek-ai/deepseek-math-7b-rl)
## 🧩 Configuration
```yaml
models:
- model: deepseek-ai/deepseek-math-7b-rl
# No parameters necessary for base model
- model: deepseek-ai/deepseek-math-7b-instruct
parameters:
density: 0.66
weight: 0.2
- model: deepseek-ai/deepseek-math-7b-base
parameters:
density: 0.57
weight: 0.2
- model: deepseek-ai/deepseek-math-7b-rl
parameters:
density: 0.54
weight: 0.2
- model: deepseek-ai/deepseek-math-7b-rl
parameters:
density: 0.61
weight: 0.2
- model: deepseek-ai/deepseek-math-7b-rl
parameters:
density: 0.65
weight: 0.1
- model: deepseek-ai/deepseek-math-7b-rl
parameters:
density: 0.55
weight: 0.1
merge_method: dare_ties
base_model: deepseek-ai/deepseek-math-7b-rl
parameters:
int8_mask: true
dtype: bfloat16
```
## 💻 Usage
```python
!pip install -qU transformers accelerate
from transformers import AutoTokenizer
import transformers
import torch
model = "ALBADDAWI/DeepCode-7B-Aurora-v2"
messages = [{"role": "user", "content": "What is a large language model?"}]
tokenizer = AutoTokenizer.from_pretrained(model)
prompt = tokenizer.apply_chat_template(messages, tokenize=False, add_generation_prompt=True)
pipeline = transformers.pipeline(
"text-generation",
model=model,
torch_dtype=torch.float16,
device_map="auto",
)
outputs = pipeline(prompt, max_new_tokens=256, do_sample=True, temperature=0.7, top_k=50, top_p=0.95)
print(outputs[0]["generated_text"])
``` |
RichardErkhov/benkimz_-_agbrain-gguf | RichardErkhov | "2024-06-05T20:51:48Z" | 965 | 0 | null | [
"gguf",
"region:us"
] | null | "2024-06-05T20:38:01Z" | Quantization made by Richard Erkhov.
[Github](https://github.com/RichardErkhov)
[Discord](https://discord.gg/pvy7H8DZMG)
[Request more models](https://github.com/RichardErkhov/quant_request)
agbrain - GGUF
- Model creator: https://huggingface.co/benkimz/
- Original model: https://huggingface.co/benkimz/agbrain/
| Name | Quant method | Size |
| ---- | ---- | ---- |
| [agbrain.Q2_K.gguf](https://huggingface.co/RichardErkhov/benkimz_-_agbrain-gguf/blob/main/agbrain.Q2_K.gguf) | Q2_K | 0.08GB |
| [agbrain.IQ3_XS.gguf](https://huggingface.co/RichardErkhov/benkimz_-_agbrain-gguf/blob/main/agbrain.IQ3_XS.gguf) | IQ3_XS | 0.08GB |
| [agbrain.IQ3_S.gguf](https://huggingface.co/RichardErkhov/benkimz_-_agbrain-gguf/blob/main/agbrain.IQ3_S.gguf) | IQ3_S | 0.08GB |
| [agbrain.Q3_K_S.gguf](https://huggingface.co/RichardErkhov/benkimz_-_agbrain-gguf/blob/main/agbrain.Q3_K_S.gguf) | Q3_K_S | 0.08GB |
| [agbrain.IQ3_M.gguf](https://huggingface.co/RichardErkhov/benkimz_-_agbrain-gguf/blob/main/agbrain.IQ3_M.gguf) | IQ3_M | 0.09GB |
| [agbrain.Q3_K.gguf](https://huggingface.co/RichardErkhov/benkimz_-_agbrain-gguf/blob/main/agbrain.Q3_K.gguf) | Q3_K | 0.09GB |
| [agbrain.Q3_K_M.gguf](https://huggingface.co/RichardErkhov/benkimz_-_agbrain-gguf/blob/main/agbrain.Q3_K_M.gguf) | Q3_K_M | 0.09GB |
| [agbrain.Q3_K_L.gguf](https://huggingface.co/RichardErkhov/benkimz_-_agbrain-gguf/blob/main/agbrain.Q3_K_L.gguf) | Q3_K_L | 0.1GB |
| [agbrain.IQ4_XS.gguf](https://huggingface.co/RichardErkhov/benkimz_-_agbrain-gguf/blob/main/agbrain.IQ4_XS.gguf) | IQ4_XS | 0.1GB |
| [agbrain.Q4_0.gguf](https://huggingface.co/RichardErkhov/benkimz_-_agbrain-gguf/blob/main/agbrain.Q4_0.gguf) | Q4_0 | 0.1GB |
| [agbrain.IQ4_NL.gguf](https://huggingface.co/RichardErkhov/benkimz_-_agbrain-gguf/blob/main/agbrain.IQ4_NL.gguf) | IQ4_NL | 0.1GB |
| [agbrain.Q4_K_S.gguf](https://huggingface.co/RichardErkhov/benkimz_-_agbrain-gguf/blob/main/agbrain.Q4_K_S.gguf) | Q4_K_S | 0.1GB |
| [agbrain.Q4_K.gguf](https://huggingface.co/RichardErkhov/benkimz_-_agbrain-gguf/blob/main/agbrain.Q4_K.gguf) | Q4_K | 0.11GB |
| [agbrain.Q4_K_M.gguf](https://huggingface.co/RichardErkhov/benkimz_-_agbrain-gguf/blob/main/agbrain.Q4_K_M.gguf) | Q4_K_M | 0.11GB |
| [agbrain.Q4_1.gguf](https://huggingface.co/RichardErkhov/benkimz_-_agbrain-gguf/blob/main/agbrain.Q4_1.gguf) | Q4_1 | 0.11GB |
| [agbrain.Q5_0.gguf](https://huggingface.co/RichardErkhov/benkimz_-_agbrain-gguf/blob/main/agbrain.Q5_0.gguf) | Q5_0 | 0.11GB |
| [agbrain.Q5_K_S.gguf](https://huggingface.co/RichardErkhov/benkimz_-_agbrain-gguf/blob/main/agbrain.Q5_K_S.gguf) | Q5_K_S | 0.11GB |
| [agbrain.Q5_K.gguf](https://huggingface.co/RichardErkhov/benkimz_-_agbrain-gguf/blob/main/agbrain.Q5_K.gguf) | Q5_K | 0.12GB |
| [agbrain.Q5_K_M.gguf](https://huggingface.co/RichardErkhov/benkimz_-_agbrain-gguf/blob/main/agbrain.Q5_K_M.gguf) | Q5_K_M | 0.12GB |
| [agbrain.Q5_1.gguf](https://huggingface.co/RichardErkhov/benkimz_-_agbrain-gguf/blob/main/agbrain.Q5_1.gguf) | Q5_1 | 0.12GB |
| [agbrain.Q6_K.gguf](https://huggingface.co/RichardErkhov/benkimz_-_agbrain-gguf/blob/main/agbrain.Q6_K.gguf) | Q6_K | 0.13GB |
| [agbrain.Q8_0.gguf](https://huggingface.co/RichardErkhov/benkimz_-_agbrain-gguf/blob/main/agbrain.Q8_0.gguf) | Q8_0 | 0.17GB |
Original model description:
---
library_name: transformers
license: mit
metrics:
- accuracy
pipeline_tag: text-generation
tags:
- text-generation-inference
language:
- en
---
# AgriBrain's AI-core, agbrain
---
AbriBrain's AI-core, agbrain, is a cutting-edge natural
language processing (NLP) model built specifically for
generating content related to agriculture. The model is a
fine-tuned version of the popular GPT-2 language model, trained
on a vast corpus of 1601 PDF documents sourced from various
reputable online resources.
Agbrain has been specifically designed to cater to the needs
of the agriculture industry, including farmers, agronomists,
agricultural researchers, and other stakeholders.
One of the key strengths of Agbrain is its ability to generate
coherent, and contextually relevant content. The model has been
fine-tuned using advanced machine learning techniques to ensure
that the generated content is both accurate and informative. It
is capable of producing content on a wide range of topics,
including crop cultivation, livestock management, pest control,
irrigation, and more.
Overall, Agbrain is a powerful and versatile NLP model that is
perfectly suited to the needs of the agriculture industry.
# Usage
---
## Transformers and model.generate
---
```python
import tensorflow as tf
from transformers import TFGPT2LMHeadModel, GPT2Tokenizer
tokenizer = GPT2Tokenizer.from_pretrained("benkimz/agbrain")
model = TFGPT2LMHeadModel.from_pretrained("benkimz/agbrain")
prompt = """
I think agribusiness is a great opportunity for passionate
investors. From food business to growing crops for sale,
and rearing livestock for business.
"""
input_ids = tokenizer.encode(prompt, return_tensors="tf")
outputs = model.generate(input_ids=input_ids,
max_length=120,
do_sample=True)
generated_text = tokenizer.decode(outputs[0],
skip_special_tokens=True)
print(generated_text)
# Output
"""
I think agribusiness is a great opportunity for passionate
investors. From food business to growing crops for sale,
and rearing livestock for business.
In this paper I will introduce a concept model agribusiness
that focuses on businesses to grow large amounts of product.
This model requires that product be sold outside of
agriculture industry, thus allowing farmers advantages,
especially over agronomic competition in production.
model is very important to farmers as it will be possible,
to sell their products at local markets without
"""
```
## Transformers pipeline
---
```python
from transformers import pipeline, set_seed
generator = pipeline('text-generation', model='benkimz/agbrain')
set_seed(42)
samples = generator(
"Animal husbandry is an important part of livestock production.",
max_length=100,
num_return_sequences=2
)
for sample in samples:
print("Model output: {}\n".format(sample['generated_text']))
# Output
"""
**Model output**: Animal husbandry is an important part of
livestock production. livestock production industry is complex,
many factors contribute to this complexity. need to determine
most efficient method of handling livestock to ensure best quality
product. It is important that animals being handled appropriately
have properly cleaned equipment that prevents scratching
(Sappell 2002). Because livestock is an important part of
livestock production, veterinary care must be taken regularly
during transport of animals from a farm to your home to be
successful. If livestock were to be
**Model output**: Animal husbandry is an important part of
livestock production. Animal husbandry combines various
strategies to control pests. Management strategies of pest
management strategies
Preventing pest from reaching level
Preventing pest from reaching level
To minimize transmission costs, control mechanisms
must be developed to prevent pest from reaching level. In
order to have an accurate information about pest
management methods, instrumental field study of pest management
measures be developed by field of study. A technique of this
"""
```
# Metrics
---
Step|Training Loss
----|---------------
500|3.877700
1000|3.746200
1500|3.659600
2000|3.613300
2500|3.603400
3000|3.561600
3500|3.558300
4000|3.518400
4500|3.504100
5000|3.508600
---
Further training could improve the model and make it better.
|
timm/vit_large_patch16_224.orig_in21k | timm | "2024-02-09T17:59:20Z" | 964 | 0 | timm | [
"timm",
"pytorch",
"safetensors",
"image-feature-extraction",
"dataset:imagenet-21k",
"arxiv:2010.11929",
"license:apache-2.0",
"region:us"
] | image-feature-extraction | "2023-11-17T00:14:06Z" | ---
license: apache-2.0
library_name: timm
tags:
- image-feature-extraction
- timm
datasets:
- imagenet-21k
---
# Model card for vit_large_patch16_224.orig_in21k
A Vision Transformer (ViT) image classification model. Pretrained on ImageNet-21k in JAX by paper authors, ported to PyTorch by Ross Wightman. This model does not have a classification head, useful for features and fine-tune only.
## Model Details
- **Model Type:** Image classification / feature backbone
- **Model Stats:**
- Params (M): 303.3
- GMACs: 59.7
- Activations (M): 43.8
- Image size: 224 x 224
- **Papers:**
- An Image is Worth 16x16 Words: Transformers for Image Recognition at Scale: https://arxiv.org/abs/2010.11929v2
- **Dataset:** ImageNet-21k
- **Original:** https://github.com/google-research/vision_transformer
## Model Usage
### Image Classification
```python
from urllib.request import urlopen
from PIL import Image
import timm
img = Image.open(urlopen(
'https://huggingface.co/datasets/huggingface/documentation-images/resolve/main/beignets-task-guide.png'
))
model = timm.create_model('vit_large_patch16_224.orig_in21k', pretrained=True)
model = model.eval()
# get model specific transforms (normalization, resize)
data_config = timm.data.resolve_model_data_config(model)
transforms = timm.data.create_transform(**data_config, is_training=False)
output = model(transforms(img).unsqueeze(0)) # unsqueeze single image into batch of 1
top5_probabilities, top5_class_indices = torch.topk(output.softmax(dim=1) * 100, k=5)
```
### Image Embeddings
```python
from urllib.request import urlopen
from PIL import Image
import timm
img = Image.open(urlopen(
'https://huggingface.co/datasets/huggingface/documentation-images/resolve/main/beignets-task-guide.png'
))
model = timm.create_model(
'vit_large_patch16_224.orig_in21k',
pretrained=True,
num_classes=0, # remove classifier nn.Linear
)
model = model.eval()
# get model specific transforms (normalization, resize)
data_config = timm.data.resolve_model_data_config(model)
transforms = timm.data.create_transform(**data_config, is_training=False)
output = model(transforms(img).unsqueeze(0)) # output is (batch_size, num_features) shaped tensor
# or equivalently (without needing to set num_classes=0)
output = model.forward_features(transforms(img).unsqueeze(0))
# output is unpooled, a (1, 197, 1024) shaped tensor
output = model.forward_head(output, pre_logits=True)
# output is a (1, num_features) shaped tensor
```
## Model Comparison
Explore the dataset and runtime metrics of this model in timm [model results](https://github.com/huggingface/pytorch-image-models/tree/main/results).
## Citation
```bibtex
@article{dosovitskiy2020vit,
title={An Image is Worth 16x16 Words: Transformers for Image Recognition at Scale},
author={Dosovitskiy, Alexey and Beyer, Lucas and Kolesnikov, Alexander and Weissenborn, Dirk and Zhai, Xiaohua and Unterthiner, Thomas and Dehghani, Mostafa and Minderer, Matthias and Heigold, Georg and Gelly, Sylvain and Uszkoreit, Jakob and Houlsby, Neil},
journal={ICLR},
year={2021}
}
```
```bibtex
@misc{rw2019timm,
author = {Ross Wightman},
title = {PyTorch Image Models},
year = {2019},
publisher = {GitHub},
journal = {GitHub repository},
doi = {10.5281/zenodo.4414861},
howpublished = {\url{https://github.com/huggingface/pytorch-image-models}}
}
``` |
RichardErkhov/tourist800_-_mistral_2X7b-gguf | RichardErkhov | "2024-05-21T23:31:50Z" | 964 | 0 | null | [
"gguf",
"region:us"
] | null | "2024-05-21T21:21:32Z" | Quantization made by Richard Erkhov.
[Github](https://github.com/RichardErkhov)
[Discord](https://discord.gg/pvy7H8DZMG)
[Request more models](https://github.com/RichardErkhov/quant_request)
mistral_2X7b - GGUF
- Model creator: https://huggingface.co/tourist800/
- Original model: https://huggingface.co/tourist800/mistral_2X7b/
| Name | Quant method | Size |
| ---- | ---- | ---- |
| [mistral_2X7b.Q2_K.gguf](https://huggingface.co/RichardErkhov/tourist800_-_mistral_2X7b-gguf/blob/main/mistral_2X7b.Q2_K.gguf) | Q2_K | 2.53GB |
| [mistral_2X7b.IQ3_XS.gguf](https://huggingface.co/RichardErkhov/tourist800_-_mistral_2X7b-gguf/blob/main/mistral_2X7b.IQ3_XS.gguf) | IQ3_XS | 2.81GB |
| [mistral_2X7b.IQ3_S.gguf](https://huggingface.co/RichardErkhov/tourist800_-_mistral_2X7b-gguf/blob/main/mistral_2X7b.IQ3_S.gguf) | IQ3_S | 2.96GB |
| [mistral_2X7b.Q3_K_S.gguf](https://huggingface.co/RichardErkhov/tourist800_-_mistral_2X7b-gguf/blob/main/mistral_2X7b.Q3_K_S.gguf) | Q3_K_S | 2.95GB |
| [mistral_2X7b.IQ3_M.gguf](https://huggingface.co/RichardErkhov/tourist800_-_mistral_2X7b-gguf/blob/main/mistral_2X7b.IQ3_M.gguf) | IQ3_M | 3.06GB |
| [mistral_2X7b.Q3_K.gguf](https://huggingface.co/RichardErkhov/tourist800_-_mistral_2X7b-gguf/blob/main/mistral_2X7b.Q3_K.gguf) | Q3_K | 3.28GB |
| [mistral_2X7b.Q3_K_M.gguf](https://huggingface.co/RichardErkhov/tourist800_-_mistral_2X7b-gguf/blob/main/mistral_2X7b.Q3_K_M.gguf) | Q3_K_M | 3.28GB |
| [mistral_2X7b.Q3_K_L.gguf](https://huggingface.co/RichardErkhov/tourist800_-_mistral_2X7b-gguf/blob/main/mistral_2X7b.Q3_K_L.gguf) | Q3_K_L | 3.56GB |
| [mistral_2X7b.IQ4_XS.gguf](https://huggingface.co/RichardErkhov/tourist800_-_mistral_2X7b-gguf/blob/main/mistral_2X7b.IQ4_XS.gguf) | IQ4_XS | 3.67GB |
| [mistral_2X7b.Q4_0.gguf](https://huggingface.co/RichardErkhov/tourist800_-_mistral_2X7b-gguf/blob/main/mistral_2X7b.Q4_0.gguf) | Q4_0 | 3.83GB |
| [mistral_2X7b.IQ4_NL.gguf](https://huggingface.co/RichardErkhov/tourist800_-_mistral_2X7b-gguf/blob/main/mistral_2X7b.IQ4_NL.gguf) | IQ4_NL | 3.87GB |
| [mistral_2X7b.Q4_K_S.gguf](https://huggingface.co/RichardErkhov/tourist800_-_mistral_2X7b-gguf/blob/main/mistral_2X7b.Q4_K_S.gguf) | Q4_K_S | 3.86GB |
| [mistral_2X7b.Q4_K.gguf](https://huggingface.co/RichardErkhov/tourist800_-_mistral_2X7b-gguf/blob/main/mistral_2X7b.Q4_K.gguf) | Q4_K | 4.07GB |
| [mistral_2X7b.Q4_K_M.gguf](https://huggingface.co/RichardErkhov/tourist800_-_mistral_2X7b-gguf/blob/main/mistral_2X7b.Q4_K_M.gguf) | Q4_K_M | 4.07GB |
| [mistral_2X7b.Q4_1.gguf](https://huggingface.co/RichardErkhov/tourist800_-_mistral_2X7b-gguf/blob/main/mistral_2X7b.Q4_1.gguf) | Q4_1 | 4.24GB |
| [mistral_2X7b.Q5_0.gguf](https://huggingface.co/RichardErkhov/tourist800_-_mistral_2X7b-gguf/blob/main/mistral_2X7b.Q5_0.gguf) | Q5_0 | 4.65GB |
| [mistral_2X7b.Q5_K_S.gguf](https://huggingface.co/RichardErkhov/tourist800_-_mistral_2X7b-gguf/blob/main/mistral_2X7b.Q5_K_S.gguf) | Q5_K_S | 4.65GB |
| [mistral_2X7b.Q5_K.gguf](https://huggingface.co/RichardErkhov/tourist800_-_mistral_2X7b-gguf/blob/main/mistral_2X7b.Q5_K.gguf) | Q5_K | 4.78GB |
| [mistral_2X7b.Q5_K_M.gguf](https://huggingface.co/RichardErkhov/tourist800_-_mistral_2X7b-gguf/blob/main/mistral_2X7b.Q5_K_M.gguf) | Q5_K_M | 4.78GB |
| [mistral_2X7b.Q5_1.gguf](https://huggingface.co/RichardErkhov/tourist800_-_mistral_2X7b-gguf/blob/main/mistral_2X7b.Q5_1.gguf) | Q5_1 | 5.07GB |
| [mistral_2X7b.Q6_K.gguf](https://huggingface.co/RichardErkhov/tourist800_-_mistral_2X7b-gguf/blob/main/mistral_2X7b.Q6_K.gguf) | Q6_K | 5.53GB |
| [mistral_2X7b.Q8_0.gguf](https://huggingface.co/RichardErkhov/tourist800_-_mistral_2X7b-gguf/blob/main/mistral_2X7b.Q8_0.gguf) | Q8_0 | 7.17GB |
Original model description:
---
license: apache-2.0
tags:
- merge
- mergekit
- lazymergekit
- mistralai/Mistral-7B-Instruct-v0.2
- mistralai/Mistral-7B-v0.1
---
# Mistral_2X7b
Marcoro14-7B-slerp is a merge of the following models using [mergekit](https://github.com/cg123/mergekit):
* [mistralai/Mistral-7B-Instruct-v0.2](https://huggingface.co/mistralai/Mistral-7B-Instruct-v0.2)
* [mistralai/Mistral-7B-v0.1](https://huggingface.co/mistralai/Mistral-7B-v0.1)
## 🧩 Configuration
```yaml
slices:
- sources:
- model: mistralai/Mistral-7B-Instruct-v0.2
layer_range: [0, 32]
- model: mistralai/Mistral-7B-v0.1
layer_range: [0, 32]
merge_method: slerp
base_model: mistralai/Mistral-7B-Instruct-v0.2
parameters:
t:
- filter: self_attn
value: [0, 0.5, 0.3, 0.7, 1]
- filter: mlp
value: [1, 0.5, 0.7, 0.3, 0]
- value: 0.5
dtype: bfloat16
```
|
HeshamHaroon/llama-3-instruct-slerp-arabic | HeshamHaroon | "2024-06-11T11:06:24Z" | 964 | 0 | transformers | [
"transformers",
"safetensors",
"llama",
"text-generation",
"mergekit",
"merge",
"conversational",
"base_model:meta-llama/Meta-Llama-3-8B-Instruct",
"base_model:HeshamHaroon/Egy_llama3",
"license:llama3",
"autotrain_compatible",
"endpoints_compatible",
"text-generation-inference",
"region:us"
] | text-generation | "2024-06-11T10:54:18Z" | ---
base_model:
- meta-llama/Meta-Llama-3-8B-Instruct
- HeshamHaroon/Egy_llama3
library_name: transformers
tags:
- mergekit
- merge
license: llama3
---
# merge
This is a merge of pre-trained language models created using [mergekit](https://github.com/cg123/mergekit).
## Merge Details
### Merge Method
This model was merged using the SLERP merge method.
### Models Merged
The following models were included in the merge:
* [meta-llama/Meta-Llama-3-8B-Instruct](https://huggingface.co/meta-llama/Meta-Llama-3-8B-Instruct)
* [HeshamHaroon/Egy_llama3](https://huggingface.co/HeshamHaroon/Egy_llama3)
### Configuration
The following YAML configuration was used to produce this model:
```yaml
slices:
- sources:
- model: HeshamHaroon/Egy_llama3
layer_range: [0, 32]
- model: meta-llama/Meta-Llama-3-8B-Instruct
layer_range: [0, 32]
merge_method: slerp
base_model: meta-llama/Meta-Llama-3-8B-Instruct
parameters:
t:
- filter: self_attn
value: [0, 0.5, 0.3, 0.7, 1]
- filter: mlp
value: [1, 0.5, 0.7, 0.3, 0]
- value: 0.5
dtype: bfloat16
``` |
DTAI-KULeuven/robbertje-1-gb-shuffled | DTAI-KULeuven | "2023-11-29T10:55:24Z" | 963 | 0 | transformers | [
"transformers",
"pytorch",
"safetensors",
"roberta",
"fill-mask",
"Dutch",
"Flemish",
"RoBERTa",
"RobBERT",
"RobBERTje",
"nl",
"arxiv:2101.05716",
"license:mit",
"autotrain_compatible",
"endpoints_compatible",
"region:us"
] | fill-mask | "2022-03-02T23:29:04Z" | ---
language: "nl"
thumbnail: "https://github.com/iPieter/RobBERT/raw/master/res/robbert_logo.png"
tags:
- Dutch
- Flemish
- RoBERTa
- RobBERT
- RobBERTje
license: mit
datasets:
- oscar
- oscar (NL)
- dbrd
- lassy-ud
- europarl-mono
- conll2002
widget:
- text: "Hallo, ik ben RobBERTje, een gedistilleerd <mask> taalmodel van de KU Leuven."
---
<p align="center">
<img src="https://github.com/iPieter/robbertje/raw/master/images/robbertje_logo_with_name.png" alt="RobBERTje: A collection of distilled Dutch BERT-based models" width="75%">
</p>
# About RobBERTje
RobBERTje is a collection of distilled models based on [RobBERT](http://github.com/iPieter/robbert). There are multiple models with different sizes and different training settings, which you can choose for your use-case.
We are also continuously working on releasing better-performing models, so watch [the repository](http://github.com/iPieter/robbertje) for updates.
# News
- **February 21, 2022**: Our paper about RobBERTje has been published in [volume 11 of CLIN journal](https://www.clinjournal.org/clinj/article/view/131)!
- **July 2, 2021**: Publicly released 4 RobBERTje models.
- **May 12, 2021**: RobBERTje was accepted at [CLIN31](https://www.clin31.ugent.be) for an oral presentation!
# The models
| Model | Description | Parameters | Training size | Huggingface id |
|--------------|-------------|------------------|-------------------|------------------------------------------------------------------------------------|
| Non-shuffled | Trained on the non-shuffled variant of the oscar corpus, without any operations to preserve this order during training and distillation. | 74 M | 1 GB | [DTAI-KULeuven/robbertje-1-gb-non-shuffled](https://huggingface.co/DTAI-KULeuven/robbertje-1-gb-non-shuffled) |
| Shuffled | Trained on the publicly available and shuffled OSCAR corpus. | 74 M | 1 GB | this model |
| Merged (p=0.5) | Same as the non-shuffled variant, but sequential sentences of the same document are merged with a probability of 50%. | 74 M | 1 GB | [DTAI-KULeuven/robbertje-1-gb-merged](https://huggingface.co/DTAI-KULeuven/robbertje-1-gb-merged) |
| BORT | A smaller version with 8 attention heads instead of 12 and 4 layers instead of 6 (and 12 for RobBERT). | 46 M | 1 GB | [DTAI-KULeuven/robbertje-1-gb-bort](https://huggingface.co/DTAI-KULeuven/robbertje-1-gb-bort) |
# Results
## Intrinsic results
We calculated the _pseudo perplexity_ (PPPL) from [cite](), which is a built-in metric in our distillation library. This metric gives an indication of how well the model captures the input distribution.
| Model | PPPL |
|-------------------|-----------|
| RobBERT (teacher) | 7.76 |
| Non-shuffled | 12.95 |
| Shuffled | 18.74 |
| Merged (p=0.5) | 17.10 |
| BORT | 26.44 |
## Extrinsic results
We also evaluated our models on sereral downstream tasks, just like the teacher model RobBERT. Since that evaluation, a [Dutch NLI task named SICK-NL](https://arxiv.org/abs/2101.05716) was also released and we evaluated our models with it as well.
| Model | DBRD | DIE-DAT | NER | POS |SICK-NL |
|------------------|-----------|-----------|-----------|-----------|----------|
| RobBERT (teacher)|94.4 | 99.2 |89.1 |96.4 | 84.2 |
| Non-shuffled |90.2 | 98.4 |82.9 |95.5 | 83.4 |
| Shuffled |92.5 | 98.2 |82.7 |95.6 | 83.4 |
| Merged (p=0.5) |92.9 | 96.5 |81.8 |95.2 | 82.8 |
| BORT |89.6 | 92.2 |79.7 |94.3 | 81.0 |
|
zjunlp/OneKE | zjunlp | "2024-05-06T09:49:31Z" | 963 | 15 | transformers | [
"transformers",
"pytorch",
"llama",
"text-generation",
"en",
"zh",
"dataset:zjunlp/iepile",
"dataset:zjunlp/InstructIE",
"arxiv:2402.14710",
"license:cc-by-nc-sa-4.0",
"autotrain_compatible",
"endpoints_compatible",
"text-generation-inference",
"region:us"
] | text-generation | "2024-02-23T09:28:16Z" | ---
license: cc-by-nc-sa-4.0
datasets:
- zjunlp/iepile
- zjunlp/InstructIE
language:
- en
- zh
---
<p align="center">
<a href="https://github.com/zjunlp/deepke"> <img src="assets/oneke_logo.png" width="400"/></a>
<p>
<p align="center">
<a href="https://oneke.openkg.cn/">
<img alt="Documentation" src="https://img.shields.io/badge/demo-website-blue">
</a>
<a href="https://pypi.org/project/deepke/#files">
<img alt="PyPI" src="https://img.shields.io/pypi/v/deepke">
</a>
<a href="https://github.com/zjunlp/DeepKE/blob/master/LICENSE">
<img alt="GitHub" src="https://img.shields.io/github/license/zjunlp/deepke">
</a>
<a href="http://zjunlp.github.io/DeepKE">
<img alt="Documentation" src="https://img.shields.io/badge/doc-website-red">
</a>
</p>
<h1 align="center">
<p>OneKE: A Bilingual Large Language Model for <br>Knowledge Extraction</p>
</h1>
- [What is OneKE?](#what-is-oneke)
- [How is OneKE trained?](#how-is-oneke-trained)
- [Getting Started with OneKE](#getting-started-with-oneke)
- [Quick Start](#quick-start)
- [Advanced Use of OneKE](#advanced-use-of-oneke)
- [OneKE Instruction Format](#oneke-instruction-format)
- [Conversion of OneKE Instruction Format](#conversion-of-oneke-instruction-format)
- [Customized Schema Description Instructions](#customized-schema-description-instructions)
- [Evaluation](#evaluation)
- [Continue Training](#continue-training)
- [Citation](#citation)
## What is OneKE?
OneKE is a large-scale model framework for knowledge extraction jointly developed by Ant Group and Zhejiang University. It possesses the capability of generalized knowledge extraction in bilingual Chinese and English, across multiple domains and tasks, and provides comprehensive toolchain support. OneKE has contributed to the OpenKG open knowledge graph community in an open-source manner.
Knowledge construction based on unstructured documents has always been one of the key challenges for the large-scale implementation of knowledge graphs. The high fragmentation and unstructured nature of real-world information, along with the substantial disparities between extracted content and its natural language expression, often result in the suboptimal performance of large language models in information extraction tasks. Natural language text often contains ambiguities, polysemies, and metaphors due to implicit and long-distance context associations, posing significant challenges for knowledge extraction tasks. In response to these issues, Ant Group and Zhejiang University leveraged their years of expertise in knowledge graphs and natural language processing to jointly construct and upgrade the capabilities of Ant's large-scale model "BaiLing" in the field of knowledge extraction. They released the bilingual knowledge extraction framework OneKE which included a version based on full parametric fine-tuning of Chinese-Alpaca-2-13B. Evaluation metrics show that OneKE has achieved relatively good performance on several fully supervised and zero-shot entity/relation/event extraction tasks.
The unified knowledge extraction framework has wide application scenarios and can significantly reduce the construction costs of domain-specific knowledge graphs. By extracting structured knowledge from massive datasets to construct high-quality knowledge graphs and establish logical associations between knowledge elements, interpretable inference and decision-making can be realized. It can also enhance large models by mitigating hallucination and boosting stability, accelerating the vertical domain applications of large models. For example, in the medical field, knowledge extraction can be used to convert doctors' experience into structured, rule-based management, building controlled auxiliary diagnostics, and medical Q&A systems. In the financial sector, it can extract financial indicators, risk events, causal logic, and industry chains for automated financial report generation, risk prediction, and industry chain analysis. In the public sector, it can facilitate knowledge-based management of government regulations, enhancing the efficiency and accuracy of public services.
<p align="center" width="100%">
<a href="" target="_blank"><img src="assets/oneke.gif" alt="OneKE" style="width: 100%; min-width: 20px; display: block; margin: auto;"></a>
</p>
## How is OneKE trained?
OneKE mainly focuses on schema-generalizable information extraction. Due to issues such as non-standard formats, noisy data, and lack of diversity in existing extraction instruction data, OneKE adopted techniques such as normalization and cleaning of extraction instructions, difficult negative sample collection, and schema-based batched instruction construction, as shown in the illustration. For more detailed information, refer to the paper "[IEPile: Unearthing Large-Scale Schema-Based Information Extraction Corpus](https://arxiv.org/abs/2402.14710) [[Github](https://github.com/zjunlp/IEPile)]".
The zero-shot generalization comparison results of OneKE with other large models are as follows:
* `NER-en`: CrossNER_AI, CrossNER_literature, CrossNER_music, CrossNER_politics, CrossNER_science
* `NER-zh`: WEIBONER, boson
* `RE-zh`: COAE2016, IPRE, SKE2020
* `RE-en`: FewRel, Wiki-ZSL
* `EE-en`: CrudeOilNews, WikiEvents, RAMS
* `EE-zh`: FewFC, CCF Law
<p align="center" width="50%">
<a href="" target="_blank"><img src="assets/oneke_results.png" alt="OneKE" style="width: 50%; min-width: 20px; display: block; margin: auto;"></a>
</p>


<details>
<summary><b>Supervision Results</b></summary>



</details>
## Getting Started with OneKE
### Quick Start
It is recommended to have at least **20GB of VRAM** for training and inferencing.
```python
import torch
from transformers import (
AutoConfig,
AutoTokenizer,
AutoModelForCausalLM,
GenerationConfig,
BitsAndBytesConfig
)
device = torch.device("cuda" if torch.cuda.is_available() else "cpu")
model_path = 'zjunlp/OneKE'
config = AutoConfig.from_pretrained(model_path, trust_remote_code=True)
tokenizer = AutoTokenizer.from_pretrained(model_path, trust_remote_code=True)
# 4-bit Quantized OneKE
quantization_config=BitsAndBytesConfig(
load_in_4bit=True,
llm_int8_threshold=6.0,
llm_int8_has_fp16_weight=False,
bnb_4bit_compute_dtype=torch.bfloat16,
bnb_4bit_use_double_quant=True,
bnb_4bit_quant_type="nf4",
)
model = AutoModelForCausalLM.from_pretrained(
model_path,
config=config,
device_map="auto",
quantization_config=quantization_config,
torch_dtype=torch.bfloat16,
trust_remote_code=True,
)
model.eval()
system_prompt = '<<SYS>>\nYou are a helpful assistant. 你是一个乐于助人的助手。\n<</SYS>>\n\n'
sintruct = "{\"instruction\": \"You are an expert in named entity recognition. Please extract entities that match the schema definition from the input. Return an empty list if the entity type does not exist. Please respond in the format of a JSON string.\", \"schema\": [\"person\", \"organization\", \"else\", \"location\"], \"input\": \"284 Robert Allenby ( Australia ) 69 71 71 73 , Miguel Angel Martin ( Spain ) 75 70 71 68 ( Allenby won at first play-off hole )\"}"
sintruct = '[INST] ' + system_prompt + sintruct + '[/INST]'
input_ids = tokenizer.encode(sintruct, return_tensors="pt").to(device)
input_length = input_ids.size(1)
generation_output = model.generate(input_ids=input_ids, generation_config=GenerationConfig(max_length=1024, max_new_tokens=512, return_dict_in_generate=True))
generation_output = generation_output.sequences[0]
generation_output = generation_output[input_length:]
output = tokenizer.decode(generation_output, skip_special_tokens=True)
print(output)
```
For more detailed inference, please refer to [DeepKE-llm/InstructKGC/6.1.2IE专用模型](https://github.com/zjunlp/DeepKE/blob/main/example/llm/InstructKGC/README_CN.md/#612ie%E4%B8%93%E7%94%A8%E6%A8%A1%E5%9E%8B).
### Advanced Use of OneKE
### OneKE Instruction Format
The instructions in OneKE are formatted in a dictionary-type string similar to JSON. It consists of three fields:
(1) **`'instruction'`**, which is the task description, specifies in natural language the role the model plays and the task to be completed;
(2) **`'schema'`**, a list of labels to be extracted, clearly indicates the key fields of the information to be extracted, reflecting the user's needs, and is dynamic and changeable;
(3) **`'input'`**, refers to the source text for information extraction.
Below are examples of instructions for various tasks:
<details>
<summary><b>Named Entity Recognition (NER)</b></summary>
```json
{
"instruction": "You are an expert specializing in entity extraction. Please extract entities that comply with the schema definition from the input; return an empty list for non-existent entity types. Please respond in the JSON string format.",
"schema": ["Person Name", "Education", "Position", "Nationality"],
"input": "Mr. Liu Zhijian: Born in 1956, Chinese nationality, no permanent residency abroad, member of the Communist Party, associate degree, senior economist."
}
```
</details>
<details>
<summary><b>Relation Extraction (RE)</b></summary>
```json
{
"instruction": "You are an expert specializing in relation extraction. Please extract relationship triples that comply with the schema definition from the input; return an empty list for non-existent relationships. Please respond in the JSON string format.",
"schema": ["Father", "Husband", "Postal Code", "Mother"],
"input": "Ding Long took out his life savings of $12,000, which without a doubt was a substantial amount at the end of the 19th century, plus Carpentier's donation, they both funded Columbia University's sinology research together."
}
```
</details>
<details>
<summary><b>Knowledge Graph Construction (KGC)</b></summary>
```json
{
"instruction": "You are an expert in structuring knowledge about graph entities. Based on the schema description of the input entity type, extract the corresponding entity instances and their property information from the text; do not output non-existent properties, return a list if there are multiple values for a property, and provide the output in a parseable json format.",
"schema": [
{
"entity_type": "Person",
"attributes": ["Chinese Name", "English Name", "Ancestral Home", "Date of Birth", "Place of Birth", "Occupation", "Alma Mater", "Works", "Awards"]
}
],
"input": "Jay Chou (Jay Chou), born on January 18, 1979, in New Taipei City, Taiwan Province, ancestral home in Yongchun County, Quanzhou City, Fujian Province, Chinese pop singer, musician, actor, director, screenwriter, graduated from Tamkang High School. In 2000, he released his debut album 'Jay'. In 2001, he cemented his style of blending Eastern and Western music with the album 'Fantasy'. In 2002, he held ‘The One’ world tour; the same year, he won the Best Composer award at the 13th Taiwan Golden Melody Awards with the song 'Love Before the Century'."
}
```
</details>
<details>
<summary><b>Event Extraction (EE)</b></summary>
```json
{
"instruction": "You are an expert specializing in event extraction. Please extract events that match the defined schema from the input; return an empty list for non-existent events, NAN for non-existent arguments, and a list if there are multiple values for an argument. Please provide your response in JSON string format.",
"schema": [
{
"event_type": "Finance/Trading - Interest Rate Hike",
"trigger": true,
"arguments": [
"Time"
]
},
{
"event_type": "Finance/Trading - Interest Rate Cut",
"trigger": true,
"arguments": [
"Cut Magnitude"
]
},
{
"event_type": "Finance/Trading - Price Increase",
"trigger": true,
"arguments": [
"Price Raiser"
]
},
{
"event_type": "Finance/Trading - Price Cut",
"trigger": true,
"arguments": [
"Price Cutter",
"Time"
]
}
],
"input": "AI risk control solution provider Vezetech secures tens of millions of dollars in Series C+ funding"
}
```
</details>
<details>
<summary><b>Event Trigger Identification (EET)</b></summary>
```json
{
"instruction": "You are an expert specializing in event trigger identification. Please extract the event types and triggers that match the defined schema from the input; return an empty list if the event type doesn't exist. Please provide your response in JSON string format.",
"schema": ["Organizational Relationship - Dissolve", "Organizational Relationship - Layoff", "Organizational Relationship - Dismiss", "Competition Behavior - Promotion"],
"input": "Nestlé lays off 4,000 employees: When the times leave you behind, they won't even say goodbye!"
}
```
</details>
<details>
<summary><b>Event Argument Extraction (EEA)</b></summary>
```json
{
"instruction": "You are an expert specializing in event argument extraction. Please extract the event arguments and their roles that match the defined schema from the input; return NAN or an empty dictionary for non-existent arguments, and a list if there are multiple values for an argument. Please provide your response in JSON string format.",
"schema": [{"event_type": "Organizational Relationship - Resignation/Departure", "arguments": ["Resigner", "Time", "Former Organization"]}],
"input": "Nestlé lays off 4,000 employees: When the times leave you behind, they won't even say goodbye!"
}
```
</details>
> Note: In consideration of the complexity of information extraction within specific domains and the high reliance on prompts, we support the integration of Schema descriptions and examples in the instructions to enhance the effectiveness of extraction tasks. For details, refer to **`Customized Schema Description Instructions`** and **`Customized Example Instructions`**. Please understand that due to the limited scale of the model, the model output is prompt-dependent and different prompts may yield inconsistent results.
### Conversion of OneKE Instruction Format
**List of Instructions**:
```python
instruction_mapper = {
'NERzh': "你是专门进行实体抽取的专家。请从input中抽取出符合schema定义的实体,不存在的实体类型返回空列表。请按照JSON字符串的格式回答。",
'REzh': "你是专门进行关系抽取的专家。请从input中抽取出符合schema定义的关系三元组,不存在的关系返回空列表。请按照JSON字符串的格式回答。",
'EEzh': "你是专门进行事件提取的专家。请从input中抽取出符合schema定义的事件,不存在的事件返回空列表,不存在的论元返回NAN,如果论元存在多值请返回列表。请按照JSON字符串的格式回答。",
'EETzh': "你是专门进行事件提取的专家。请从input中抽取出符合schema定义的事件类型及事件触发词,不存在的事件返回空列表。请按照JSON字符串的格式回答。",
'EEAzh': "你是专门进行事件论元提取的专家。请从input中抽取出符合schema定义的事件论元及论元角色,不存在的论元返回NAN或空字典,如果论元存在多值请返回列表。请按照JSON字符串的格式回答。",
'KGzh': '你是一个图谱实体知识结构化专家。根据输入实体类型(entity type)的schema描述,从文本中抽取出相应的实体实例和其属性信息,不存在的属性不输出, 属性存在多值就返回列表,并输出为可解析的json格式。',
'NERen': "You are an expert in named entity recognition. Please extract entities that match the schema definition from the input. Return an empty list if the entity type does not exist. Please respond in the format of a JSON string.",
'REen': "You are an expert in relationship extraction. Please extract relationship triples that match the schema definition from the input. Return an empty list for relationships that do not exist. Please respond in the format of a JSON string.",
'EEen': "You are an expert in event extraction. Please extract events from the input that conform to the schema definition. Return an empty list for events that do not exist, and return NAN for arguments that do not exist. If an argument has multiple values, please return a list. Respond in the format of a JSON string.",
'EETen': "You are an expert in event extraction. Please extract event types and event trigger words from the input that conform to the schema definition. Return an empty list for non-existent events. Please respond in the format of a JSON string.",
'EEAen': "You are an expert in event argument extraction. Please extract event arguments and their roles from the input that conform to the schema definition, which already includes event trigger words. If an argument does not exist, return NAN or an empty dictionary. Please respond in the format of a JSON string.",
'KGen': 'You are an expert in structured knowledge systems for graph entities. Based on the schema description of the input entity type, you extract the corresponding entity instances and their attribute information from the text. Attributes that do not exist should not be output. If an attribute has multiple values, a list should be returned. The results should be output in a parsable JSON format.',
}
```
Recommended **Split Numbers** for Each Task:
```python
split_num_mapper = {
'NER':6, 'RE':4, 'EE':4, 'EET':4, 'EEA':4, 'KG':1
}
```
Since predicting all schemas in the label set at once is too challenging and not easily scalable, OneKE uses a batched approach during training. It divides the number of schemas asked in the instructions, querying a fixed number of schemas at a time. Hence, if the label set of a piece of data is too long, it will be split into multiple instructions that the model will address in turns.
**Schema Format**:
```python
NER: ["Person Name", "Education", "Position", "Nationality"] # List of strings
RE: ["Father", "Husband", "Postal Code", "Mother"] # List of strings
EE: [{"event_type": "Finance/Trading - Interest Rate Hike", "trigger": True, "arguments": ["Time"]}, {"event_type": "Finance/Trading - Interest Rate Cut", "trigger": True, "arguments": ["Cut Magnitude"]}] # List of dictionaries, "event_type" is a string, "trigger" is a bool, "arguments" is a list
EET: ["Organizational Relationship - Dissolution", "Organizational Relationship - Layoff", "Organizational Relationship - Dismissal", "Competition Behavior - Advancement"] # List of strings
EEA: [{"event_type": "Finance/Trading - Interest Rate Hike", "arguments": ["Time"]}, {"event_type": "Finance/Trading - Interest Rate Cut", "arguments": ["Cut Magnitude"]}] # List of dictionaries, "event_type" is a string, "arguments" is a list
```
Below is a simple Batched Instruction Generation script:
```python
def get_instruction(language, task, schema, input):
sintructs = []
split_num = split_num_mapper[task]
if type(schema) == dict:
sintruct = json.dumps({'instruction':instruction_mapper[task+language], 'schema':schema, 'input':input}, ensure_ascii=False)
sintructs.append(sintruct)
else:
split_schemas = [schema[i:i+split_num] for i in range(0, len(schema), split_num)]
for split_schema in split_schemas:
sintruct = json.dumps({'instruction':instruction_mapper[task+language], 'schema':split_schema, 'input':input}, ensure_ascii=False)
sintructs.append(sintruct)
return sintructs
```
Below is an example using the aforementioned simple script:
```python
task = 'NER'
language = 'en'
schema = ['person', 'organization', 'else', 'location']
split_num = split_num_mapper[task]
split_schemas = [schema[i:i+split_num] for i in range(0, len(schema), split_num)]
input = '284 Robert Allenby ( Australia ) 69 71 71 73 , Miguel Angel Martin ( Spain ) 75 70 71 68 ( Allenby won at first play-off hole )'
sintructs = []
for split_schema in split_schemas:
sintruct = json.dumps({'instruction':instruction_mapper[task+language], 'schema':split_schema, 'input':input}, ensure_ascii=False)
sintructs.append(sintruct)
```
> '{"instruction": "You are an expert in named entity recognition. Please extract entities that match the schema definition from the input. Return an empty list if the entity type does not exist. Please respond in the format of a JSON string.", "schema": ["person", "organization", "else", "location"], "input": "284 Robert Allenby ( Australia ) 69 71 71 73 , Miguel Angel Martin ( Spain ) 75 70 71 68 ( Allenby won at first play-off hole )"}'
For more detailed data conversion, please refer to [DeepKE-llm/InstructKGC/README_CN.md/2.3测试数据转换](https://github.com/zjunlp/DeepKE/blob/main/example/llm/InstructKGC/README_CN.md/#23%E6%B5%8B%E8%AF%95%E6%95%B0%E6%8D%AE%E8%BD%AC%E6%8D%A2)
### Customized Schema Description Instructions
```json
{
"instruction": "You are an expert specializing in entity extraction. Please extract entities that comply with the defined schema from the input; return an empty list for non-existent entity types. Please respond in JSON string format.",
"schema": {
"Position": "The entity type describes the occupation or official position of an individual or group, including specific role names such as 'producer', 'scorekeeper', 'ascetic', 'oil painter'.",
"Attraction": "The entity type of attraction includes buildings, museums, memorials, art galleries, rivers, peaks, etc. Representative entities include the Pentagon, Tate Modern, Zheng Chenggong Memorial Hall, Duxi Palace, Barikasa, Robo River, Gunung Batur, Yugong Yishan LIVE, Xu Beihong Memorial Hall, Madame Tussauds, etc.",
"Company": "Company is an entity type representing any legal entity or business organization. This type of entity can be a catering group, manufacturer, retailer, hotel, bank, design institute, etc. Examples include: 'Shangri-La Hotel Group', 'JVC', 'Shanghai Coolray Professional eSports Peripheral Store', 'K2•Haitang Bay', 'Wuhan Iron and Steel', 'louisvuitton', 'Bank of Scotland', 'Beijing Institute of Architectural Design', '7 Days Inn', 'Vanke Group'.",
"Address": "Address entities refer to entities with geographical location information, representing specific places such as a country, city, region, street, or abstract geographic areas. Examples include: 'the river dock at the southeast tip of downtown Manhattan', 'Tuapse', 'Venice, Italy', 'Huzhou Hot Spring Golf Course', 'North Carolina', 'Beijing-Tianjin region', 'Happy Internet Cafe', 'Yinian Nursing Home', 'Shangtang Town Pudong', 'Inner Mongolia Autonomous Region Chifeng City', etc.",
"Organization": "Organizational entities refer to collective organizations such as companies, shops, clubs, schools, etc. They play a certain role in social and economic activities and have certain personality rights.",
"Movie": "Movie entities include titles of movies in Chinese or English, and sometimes also include names of characters in films."
},
"input": "It is difficult for me to imagine setting up another Haifishing Plaza. When we obtained this project, I just happened to be in Sanya."
}
```
<details>
<summary><b>Relation Extraction (RE) Description Instructions</b></summary>
```json
{
"instruction": "You are an expert specializing in relation extraction. Please extract triples that match the defined schema from the input; return an empty list for non-existent relations. Please respond in JSON string format.",
"schema": {
"Ethnicity": "Ethnicity",
"Alma Mater": "This type of relationship describes the connection between a person and their alma mater; the person is the subject, and the alma mater is the object. By identifying the names of people and schools in the text and analyzing the relationship of graduation between them based on word combinations and contextual information.",
"Lead Actor": "This is a type of relationship that describes the connection between a film or television work and its main actors; the subject is the film or television work and the object is the actor. In a valid 'Lead Actor' relationship, the actor (object) plays an important role in the work (subject).",
"Father": "This type of relationship is used to indicate the kinship between a father and a child, where the father is the birth parent or caregiver of the child. In the triple, the subject of the 'Father' relation type is the child, and the object is the father."
},
"input": "Throughout history, all those who have portrayed the character 'Chu Liuxiang' from Gu Long's novels are recognized as handsome men in the entertainment industry. In 2011, 36-year-old Zhang Zhiyao played Chu Liuxiang in 'The New Adventures of Chu Liuxiang', remaining irresistibly handsome."
}
```
</details>
<details>
<summary><b>Event Extraction (EE) Description Instructions</b></summary>
```json
{
"instruction": "You are an expert specializing in event extraction. Please extract events that match the schema definition from the input; return an empty list for non-existent events, NAN for non-existent arguments, and a list if there are multiple values for an argument. Please respond in JSON string format.",
"schema": {
"Finance/Trading - Listing": {
"Finance/Trading - Listing": "The act of a financial entity being listed on the stock market mainly involves companies, stocks, etc. Positive examples include specific information about a company or stock listing, while negative examples are unrelated to such activities.",
"trigger": true,
"arguments": {
"Financing Amount": "Refers to the total amount of funds raised by a company in a listing event. It sums up the revenue of all share issues and is measured in currency, including but not limited to units like 'billion', 'million', 'dollars', 'RMB', etc.",
"Time": "Describes the specific time of the listing event, which can be a specific date or relative time, and may also include location information and specific days and weeks.",
"Listing Enterprise": "Refers to the company or enterprise that is conducting an IPO or has already been listed on the trading market in a listing event. Examples include: 'Shanghai Henlius Biotech', 'Three Squirrels', 'Baoxin Software', 'Little Bear Electric', 'Jinshang Bank', 'Beyond Meat (BYND)', 'DouYu gaming live-streaming platform', 'fast food empire', and 'autonomous driving lidar manufacturer Velodyne', etc.",
"Location": "The specific location of the financial or trading event, such as a city, building, or room."
}
},
"Organizational Relationship - Resignation/Departure": {
"Organizational Relationship - Resignation/Departure": "The event type 'Organizational Relationship - Resignation/Departure' refers to changes in the relationship between individuals or organizational members and their organization, mainly including 'resignation', 'requesting to resign', 'stepping down', 'leaving the team', 'retirement', 'leaving', etc. Often occurs in scenarios of high-level personnel changes, government officials changes, or athletes transfers. Examples: 'Li Nan announced resignation', 'Yu Xubo resigned from the position of chairman of the board just three months after taking office, Chen Lang succeeded'.",
"trigger": true,
"arguments": {
"Resigner": "Refers to the individual or group who actively or passively leaves their original position or job post in an organizational relationship resignation/departure event. It can be one person or a group of people, such as: 'Finance Minister', '90s born guy from Shaoyang Longhui, Ouyang En and', 'Xiong Xiaoge', '*ST Changsheng two deputy general managers', 'Yang Tao', 'pilot Ma Qiang', 'HE WEI', '5 Baidu executives', 'Youxin Group COO Peng Weilian', 'Jianke Institute securities representative Shu Yanming', etc.",
"Time": "Indicates the specific point in time or period when the resignation/departure event occurred, generally including specific dates, weeks, times, etc., like 'September 19', 'the evening of June 29', 'this Saturday', '10:30 AM on July 9', 'the morning of June 12', 'April 9', 'September 10', 'local time on Sunday', 'September 12', '10 AM on October 15', etc."
}
},
"Finance/Trading - Interest Rate Increase": {
"Finance/Trading - Interest Rate Increase": "This event describes banks or financial institutions raising interest rates to tighten the money supply. The typical trigger word is 'hike'. 'Hike' indicates the occurrence of the Finance/Trading - Interest Rate Increase event.",
"trigger": true,
"arguments": {
"Rate of Increase": "The rate of increase is usually presented as a percentage or basis points, indicating the degree or range of the interest rate hike in the event. Examples include: 'to 5.75%', '25 basis points', 'the benchmark rate from 0.25% up to 0.5%', '25 basis points'.",
"Hiking Institution": "The hiking institution is the financial institution with the authority to determine or implement the interest rate hike policy in a Finance/Trading - Interest Rate Increase event, such as central banks from different countries (e.g., Bank of England, Federal Reserve, European Central Bank) or financial institutions (e.g., Bank of England).",
"Time": "Indicates the specific date or time period when the Finance/Trading - Interest Rate Increase event occurred, such as 'the morning of June 18th', 'January 24th', 'three months later', etc. The specific expression includes time accurate to the minute, such as '11:00 on December 28, 2018', relative time, such as 'yesterday (2nd)', and special time expressions like 'Mid-Autumn Festival'."
}
},
"Organizational Relationship - Contract Termination": {
"Organizational Relationship - Contract Termination": "Situations of contract cancellation or termination usually occur in the business, entertainment, or sports domains. Trigger words include 'leave', 'trade', 'cut', 'contract expiry', 'contract termination', 'sell-off', 'release', 'send out', 'contract break', etc. Positive examples include 'Peng Yuchang terminates his contract' and 'Jiang Mengjie nearly bankrupt after contract termination'. Negative examples are like 'Federer withdrew from the competition'.",
"trigger": true,
"arguments": {
"Party Being Terminated": "In an organizational relationship contract termination event, the role is the party whose agreement or contract relation is being dissolved, and might be an individual or an organization, such as an athlete, film producer, company, etc. For instance, 'seven-time All-Star Joe Johnson', 'the production side of 'A Little Wish'', 'Raptors', 'Samsung', etc."
}
}
},
"input": "News from August 20th, according to Tencent News 'Frontline' report, informed sources stated that in order to control cost expenditure, NIO plans to reduce the number of staff at its U.S. branch, excluding those involved in the autonomous driving business, to about 200. As of August 16th, U.S. time, NIO's Silicon Valley branch had cut 100 employees."
}
```
</details>
<details>
<summary><b>Knowledge Graph Construction (KGC) Description Instructions</b></summary>
```json
{
"instruction": "You are an expert in structuring knowledge about graph entities. Based on the schema description for the input entity type, extract the corresponding entity instances and their attribute information from the text; do not output non-existent attributes, return a list for attributes with multiple values, and provide the output in a parseable JSON format.",
"schema": [
{
"entity_type": "Person",
"attributes": {
"Chinese Name": "The Chinese name of the person",
"English Name": "The English name of the person",
"Ancestral Home": "The ancestral address of the person",
"Date of Birth": "Birthday, birth date",
"Place of Birth": "The place of birth, administrative region",
"Occupation": "The occupation, position, identity of the person",
"Alma Mater": "The middle school, university, college from which the person graduated",
"Works": "Albums, songs, novels, published books, participated film and television works, etc.",
"Awards": "Various awards and honors received by the person"
}
}
],
"input": "Jay Chou (Jay Chou), born on January 18, 1979, in New Taipei City, Taiwan Province, with ancestral home in Yongchun County, Quanzhou City, Fujian Province, is a Chinese pop musician, actor, director, and screenwriter. He graduated from Tamkang High School. In 2000, he released his debut music album 'Jay.' In 2001, he cemented his fusion style of Eastern and Western music with the album 'Fantasy.' In 2002, he held 'The One' world tour; that same year, he won the Best Composer award at the 13th Taiwan Golden Melody Awards for the song 'Love Before the Century.'"
}
```
</details>
### Customized Example Instructions
Given that example instances can often be lengthy, and due to the limited maximum length of model training, too many examples may inversely affect model performance. Therefore, we suggest providing 2 examples: one positive and one negative, while keeping the number of schemas to one.
```json
{
"instruction": "You are an expert in entity extraction. Please extract entities from the input that fit the defined schema; return an empty list for non-existent entity types. Please respond in the format of a JSON string. You may refer to the example to guide your extraction.",
"schema": [
"Biomarker"
],
"example": [
{
"input": "Diagnostic criteria for CKD include: 1. Any of the following indicators persisting for more than 3 months; and meeting at least one criterion.(1) Signs of renal damage: Albuminuria [Albumin excretion rate (AER)≥30mg/24h; Albumin to creatinine ratio (ACR)≥3mg/mmol]; abnormal urinary sediment; tubular pathology; histological anomalies; structural abnormities found in imaging; history of kidney transplantation.(2) Decline in glomerular filtration rate: eGFR≤60ml·min-1·1.73m-2",
"output": {
"Biomarker": [
"Albumin excretion rate (AER)",
"Albumin to creatinine ratio (ACR)",
"Glomerular filtration rate",
"eGFR"
]
}
},
{
"input": "Application of DPP-4 inhibitors in specific populations",
"output": {
"Biomarker": []
}
}
],
"input": "Currently, all sulfonylurea drugs' leaflets list severe liver dysfunction as a contraindication. Alanine transaminase (ALT)> 3 times the upper limit of the reference value can serve as a sensitive and specific indicator of liver damage. If ALT>8-10 times the upper limit of the reference value or ALT>3 times with total serum bilirubin (TBIL)>2 times the reference value, it is considered a specific predictor of severe liver damage, indicating substantial injury to hepatic parenchymal cells; sulfonylureas should be contraindicated at this stage. Clinically, patients with decompensated liver cirrhosis accompanied by hepatic encephalopathy, ascites, or coagulation disorders should avoid this class of drugs to prevent hypoglycemia."
}
```
<details>
<summary><b>Relationship Extraction (RE) Example Instruction</b></summary>
```json
{
"instruction": "You are an expert specialized in relationship extraction. Please extract from the input the defined relation triples according to the schema; return an empty list for non-existent relations. Please respond in the format of a JSON string. You may refer to the example for guidance on extraction.",
"schema": [
"Disease Staging and Typing"
],
"example": [
{
"input": "The foundational treatment of diabetes includes both education and management, as well as diet and exercise. A lack of knowledge in diabetes prevention and control is the primary reason for poor blood sugar management. Paying attention to the education and management of elderly patients is an important measure to improve the treatment level of diabetes.",
"output": {
"Disease Staging and Typing": []
}
},
{
"input": "Metabolites of glipizide have no hypoglycemic effect and are mostly excreted through feces, with only 5.0% excreted by the kidneys, thus are less affected by renal function. However, large clinical trials in patients with chronic kidney disease are limited. There have been studies observing the use of glipizide in patients with GFR10~50 ml min-1.(1.73m2)-1, but the trial designs are not perfect. Glipizide can be used in patients with stages 1 to 3 chronic kidney disease without dose adjustment; caution is advised in stage 4; and it is contraindicated in stage 5.",
"output": {
"Disease Staging and Typing": [
{
"subject": "Chronic kidney disease",
"object": "Chronic"
},
{
"subject": "Chronic kidney disease",
"object": "Chronic"
},
{
"subject": "Chronic kidney disease",
"object": "stages 1 to 3"
},
{
"subject": "Chronic kidney disease",
"object": "stage 4"
},
{
"subject": "Chronic kidney disease",
"object": "stage 5"
}
]
}
}
],
"input": "(2)NSAIDs: This includes both non-selective cyclooxygenase (COX) inhibitors and COX-2 inhibitors. If there are no contraindications, early and ample use of fast-acting NSAID formulations is recommended. Non-selective COX inhibitors primarily have gastrointestinal adverse reactions such as ulcers, perforations, and upper gastrointestinal bleeding, hence COX-2 inhibitors, which can reduce GI reactions by 50%, may be used for those intolerant to non-selective COX inhibitors. Active gastrointestinal ulcers/bleeding or a history of recurrent gastrointestinal ulcers/bleeding is a contraindication for all NSAIDs use. COX-2 inhibitors may increase the risk of cardiovascular events and should be avoided in patients with myocardial infarction or heart failure. Kidney function monitoring is required during the use of NSAIDs, and their use is not recommended in patients with severe chronic kidney disease (stages G4 to G5) who are not undergoing dialysis."
}
```
</details>
<details>
<summary><b>Event Extraction (EE) Example Instruction</b></summary>
```json
{
"instruction": "You are an expert specialized in event extraction. Please extract events from the input according to the defined schema; return an empty list for non-existent events, and 'NAN' for non-existent arguments. If an argument has multiple values, please return a list. Respond in the format of a JSON string. You may refer to the example for extraction guidance.",
"schema": [
{
"event_type": "Corporate Financing",
"trigger": true,
"arguments": [
"Disclosure Time",
"Investee",
"Financing Round",
"Lead Investor",
"Event Time",
"Investor",
"Financing Amount"
]
}
],
"example": [
{
"input": "Raise 2.5 billion yuan for expansion due to the 'three highs' condition of Joyson Electronics: high pledges, high goodwill, high debt\nReporter Zhang Jiazhen, from Beijing\nNingbo Joyson Electronic Corporation (hereinafter referred to as 'Joyson Electronics', 600699.SH), which holds billion-level big orders, is actively raising funds to expand production capacity to ease the increasingly pressing bottleneck of production capacity saturation.\nRecently, Joyson Electronics announced that it has received the 'Feedback Notice' from the China Securities Regulatory Commission, and its private stock offering is a step closer to approval.",
"output": {
"Corporate Financing": [
{
"trigger": "Raise",
"arguments": {
"Disclosure Time": "NAN",
"Investee": "Ningbo Joyson Electronic Corporation",
"Financing Round": "NAN",
"Lead Investor": "NAN",
"Event Time": "NAN",
"Investor": "NAN",
"Financing Amount": "2.5 billion yuan"
}
}
]
}
},
{
"input": "NIO stock falls to 13% before market; NIO reports over 3.2 billion loss in Q2\nOriginal Title: NIO stock falls to 13% before market; NIO reports over 3.2 billion loss in Q2\nNIO's stock price turned from a rise to a fall before market, falling to 13%. NIO released its Q2 earnings today, followed by the announcement of the cancellation of the earnings conference call originally scheduled for today.\nThe earnings report showed that NIO achieved a revenue of 1.508 billion yuan in the second quarter, exceeding market expectations of 1.309 billion yuan, compared to 46 million yuan in the same period last year; The net loss attributable to shareholders in the second quarter was 3.285 billion yuan, higher than the market expected loss of 2.944 billion yuan, compared to a loss of 6.11 billion yuan in the same period last year.",
"output": {
"Corporate Financing": []
}
}
],
"input": "【Exclusive】The 11th in five years, Codemao announces completion of C+ round financing of 250 million yuan\nJiemodui, April 17th - Today, Codemao announced the completion of a C+ round of financing worth 250 million yuan.\nThis comes five months after completing a C round financing of 400 million yuan last year, which is the new round of 'ammunition' added by Codemao.\nThe round was led by China Merchants International, with Bohai Capital, an equity investment fund under Bank of China Group, and existing shareholders Yueke Xintai and Shengyu Investment following suit."
}
```
</details>
## Evaluation
To extract structured content from the output text and to assess it, please refer to [DeepKE-llm/InstructKGC/README_CN.md/7.评估](https://github.com/zjunlp/DeepKE/blob/main/example/llm/InstructKGC/README_CN.md/#-7%E8%AF%84%E4%BC%B0).
## Continue Training
To continue training OneKE, refer to [DeepKE-llm/InstructKGC/4.9领域内数据继续训练](https://github.com/zjunlp/DeepKE/blob/main/example/llm/InstructKGC/README_CN.md/#49%E9%A2%86%E5%9F%9F%E5%86%85%E6%95%B0%E6%8D%AE%E7%BB%A7%E7%BB%AD%E8%AE%AD%E7%BB%83).
## Citation
If you have used OneKE in your work, please kindly cite the following paper:
```bibtex
@article{DBLP:journals/corr/abs-2402-14710,
author = {Honghao Gui and
Lin Yuan and
Hongbin Ye and
Ningyu Zhang and
Mengshu Sun and
Lei Liang and
Huajun Chen},
title = {IEPile: Unearthing Large-Scale Schema-Based Information Extraction
Corpus},
journal = {CoRR},
volume = {abs/2402.14710},
year = {2024},
url = {https://doi.org/10.48550/arXiv.2402.14710},
doi = {10.48550/ARXIV.2402.14710},
eprinttype = {arXiv},
eprint = {2402.14710},
timestamp = {Tue, 09 Apr 2024 07:32:43 +0200},
biburl = {https://dblp.org/rec/journals/corr/abs-2402-14710.bib},
bibsource = {dblp computer science bibliography, https://dblp.org}
}
``` |
YeungNLP/firefly-qwen1.5-en-14b-alpha | YeungNLP | "2024-05-19T17:17:52Z" | 963 | 0 | transformers | [
"transformers",
"safetensors",
"qwen2",
"text-generation",
"conversational",
"license:apache-2.0",
"autotrain_compatible",
"endpoints_compatible",
"text-generation-inference",
"region:us"
] | text-generation | "2024-05-16T08:27:16Z" | ---
library_name: transformers
license: apache-2.0
basemodel: Qwen/Qwen1.5-14B
---
## Model Card for Firefly-Qwen1.5-14B-En-Alpha
[firefly-qwen1.5-en-14b-alpha](https://huggingface.co/YeungNLP/firefly-qwen1.5-en-14b-alpha) is a preview version model of our new model.
It outperforms [Qwen1.5-14B-Chat](https://huggingface.co/Qwen/Qwen1.5-14B-Chat) on [AlpacaEval 2.0](https://github.com/tatsu-lab/alpaca_eval) and [MT-Bench](https://github.com/lm-sys/FastChat/tree/main/fastchat/llm_judge)' single-turn task.
**Note: More importantly, it is not trained with neither SFT nor RLHF, maybe we will share our method later.**
What's exciting is that our experimental method can achieve good performance, even though it's still in a very preliminary stage.
Although our model is trained with English data, you can also try to chat with models in Chinese because Qwen1.5 is also good at Chinese. But we have not evaluated
the performance in Chinese yet.
We advise you to install transformers>=4.37.0.
**Because this is a validation experiment and our training resources are limited, we use QLoRA to train this model based on [Qwen1.5-14B](https://huggingface.co/Qwen/Qwen1.5-14B) with the max length of 1024, it may limit the performance of this model.**
## Performance
We automatically evaluate models on [AlpacaEval 2.0](https://github.com/tatsu-lab/alpaca_eval) and [MT-Bench](https://github.com/lm-sys/FastChat/tree/main/fastchat/llm_judge) with **gpt-4o**.
We evaluate models on [AlpacaEval 2.0](https://github.com/tatsu-lab/alpaca_eval) with 805 questions, our model outperforms [Qwen1.5-14B-Chat](https://huggingface.co/Qwen/Qwen1.5-14B-Chat).
The win rate is **52.17% : 47.83%**.
| Task | Ours wins | Qwen1.5-14B-Chat wins |
|---------------|-----------|-----------------------|
| helpful_base | **67** | 62 |
| koala | **80** | 76 |
| oasst | **100** | 88 |
| selfinstruct | **127** | 125 |
| vicuna | **46** | 34 |
| total | **420** | 385 |
We also evaluate models on [MT-Bench](https://github.com/lm-sys/FastChat/tree/main/fastchat/llm_judge). Though the overall performance of our model is not as good as [Qwen1.5-14B-Chat](https://huggingface.co/Qwen/Qwen1.5-14B-Chat),
**we find that our model outperforms [Qwen1.5-14B-Chat](https://huggingface.co/Qwen/Qwen1.5-14B-Chat) in almost all single-turn tasks**. Our model is worse than [Qwen1.5-14B-Chat](https://huggingface.co/Qwen/Qwen1.5-14B-Chat) in almost all multi-turn tasks.
We conjecture that it may be caused by the training length, and we will dive into this phenomenon later.
Overall Performances on MT-Bench:
| Task | Ours | Qwen1.5-14B-Chat |
|-------------------|----------|-------------------|
| Avg Score | 7.03 | **7.21** |
| Single-turn Score | **8.01** | 7.66 |
| Multi-turn Score | 6.05 | **6.75** |
Performances on MT-Bench' single-turn tasks:
| Task | Ours | Qwen1.5-14B-Chat |
|---------------|----------|------------------|
| writing | **9.1** | 8.9 |
| roleplay | **8.5** | 8.3 |
| extraction | **8.6** | 8.2 |
| stem | **8.8**| 8.5 |
| humanities | **9** | 8.8 |
| reasoning | **6.8** | 5.3 |
| math | **7.5** | 7.1 |
| coding | 5.8 | **6.2** |
Performances on MT-Bench' multi-turn tasks:
| Task | Ours | Qwen1.5-14B-Chat |
|----------------|----------|--------------------|
| writing | 6.5 | **7.7** |
| roleplay | 7.7 | **8.3** |
| extraction | 5.1 | **6.7** |
| stem | 6.3 | **6.9** |
| humanities | 8.3 | **8.8** |
| reasoning | 4.7 | **5.7** |
| math | 4.9 | **5.5** |
| coding | **4.9** | 4.4 |
## Usage
The chat templates of our chat models are the same as Official Qwen1.5-14B-Chat:
```text
<|im_start|>system
You are a helpful assistant.<|im_end|>
<|im_start|>user
hello, who are you?<|im_end|>
<|im_start|>assistant
I am a AI program developed by Firefly<|im_end|>
```
You can use script to inference in [Firefly](https://github.com/yangjianxin1/Firefly/blob/master/script/chat/chat.py).
You can also use the following code:
```python
from transformers import AutoModelForCausalLM, AutoTokenizer
import torch
model_name_or_path = "YeungNLP/firefly-qwen1.5-en-14b-alpha"
model = AutoModelForCausalLM.from_pretrained(
model_name_or_path,
trust_remote_code=True,
low_cpu_mem_usage=True,
torch_dtype=torch.float16,
device_map='auto',
)
tokenizer = AutoTokenizer.from_pretrained(model_name_or_path)
prompt = "Compose an engaging travel blog post about a recent trip to Hawaii, highlighting cultural experiences and must-see attractions. "
messages = [
{"role": "system", "content": "You are a helpful assistant."},
{"role": "user", "content": prompt}
]
text = tokenizer.apply_chat_template(
messages,
tokenize=False,
add_generation_prompt=True
)
model_inputs = tokenizer([text], return_tensors="pt").to('cuda')
generated_ids = model.generate(
model_inputs.input_ids,
max_new_tokens=1500,
top_p = 0.8,
temperature = 0.6,
repetition_penalty = 1.0,
eos_token_id=tokenizer.encode('<|im_end|>', add_special_tokens=False)
)
generated_ids = [
output_ids[len(input_ids):] for input_ids, output_ids in zip(model_inputs.input_ids, generated_ids)
]
response = tokenizer.batch_decode(generated_ids, skip_special_tokens=True)[0]
print(response)
``` |
RichardErkhov/FPHam_-_Writing_Partner_Mistral_7B-gguf | RichardErkhov | "2024-05-28T16:51:42Z" | 963 | 0 | null | [
"gguf",
"region:us"
] | null | "2024-05-28T13:13:27Z" | Quantization made by Richard Erkhov.
[Github](https://github.com/RichardErkhov)
[Discord](https://discord.gg/pvy7H8DZMG)
[Request more models](https://github.com/RichardErkhov/quant_request)
Writing_Partner_Mistral_7B - GGUF
- Model creator: https://huggingface.co/FPHam/
- Original model: https://huggingface.co/FPHam/Writing_Partner_Mistral_7B/
| Name | Quant method | Size |
| ---- | ---- | ---- |
| [Writing_Partner_Mistral_7B.Q2_K.gguf](https://huggingface.co/RichardErkhov/FPHam_-_Writing_Partner_Mistral_7B-gguf/blob/main/Writing_Partner_Mistral_7B.Q2_K.gguf) | Q2_K | 2.53GB |
| [Writing_Partner_Mistral_7B.IQ3_XS.gguf](https://huggingface.co/RichardErkhov/FPHam_-_Writing_Partner_Mistral_7B-gguf/blob/main/Writing_Partner_Mistral_7B.IQ3_XS.gguf) | IQ3_XS | 2.81GB |
| [Writing_Partner_Mistral_7B.IQ3_S.gguf](https://huggingface.co/RichardErkhov/FPHam_-_Writing_Partner_Mistral_7B-gguf/blob/main/Writing_Partner_Mistral_7B.IQ3_S.gguf) | IQ3_S | 2.96GB |
| [Writing_Partner_Mistral_7B.Q3_K_S.gguf](https://huggingface.co/RichardErkhov/FPHam_-_Writing_Partner_Mistral_7B-gguf/blob/main/Writing_Partner_Mistral_7B.Q3_K_S.gguf) | Q3_K_S | 2.95GB |
| [Writing_Partner_Mistral_7B.IQ3_M.gguf](https://huggingface.co/RichardErkhov/FPHam_-_Writing_Partner_Mistral_7B-gguf/blob/main/Writing_Partner_Mistral_7B.IQ3_M.gguf) | IQ3_M | 3.06GB |
| [Writing_Partner_Mistral_7B.Q3_K.gguf](https://huggingface.co/RichardErkhov/FPHam_-_Writing_Partner_Mistral_7B-gguf/blob/main/Writing_Partner_Mistral_7B.Q3_K.gguf) | Q3_K | 3.28GB |
| [Writing_Partner_Mistral_7B.Q3_K_M.gguf](https://huggingface.co/RichardErkhov/FPHam_-_Writing_Partner_Mistral_7B-gguf/blob/main/Writing_Partner_Mistral_7B.Q3_K_M.gguf) | Q3_K_M | 3.28GB |
| [Writing_Partner_Mistral_7B.Q3_K_L.gguf](https://huggingface.co/RichardErkhov/FPHam_-_Writing_Partner_Mistral_7B-gguf/blob/main/Writing_Partner_Mistral_7B.Q3_K_L.gguf) | Q3_K_L | 3.56GB |
| [Writing_Partner_Mistral_7B.IQ4_XS.gguf](https://huggingface.co/RichardErkhov/FPHam_-_Writing_Partner_Mistral_7B-gguf/blob/main/Writing_Partner_Mistral_7B.IQ4_XS.gguf) | IQ4_XS | 3.67GB |
| [Writing_Partner_Mistral_7B.Q4_0.gguf](https://huggingface.co/RichardErkhov/FPHam_-_Writing_Partner_Mistral_7B-gguf/blob/main/Writing_Partner_Mistral_7B.Q4_0.gguf) | Q4_0 | 3.83GB |
| [Writing_Partner_Mistral_7B.IQ4_NL.gguf](https://huggingface.co/RichardErkhov/FPHam_-_Writing_Partner_Mistral_7B-gguf/blob/main/Writing_Partner_Mistral_7B.IQ4_NL.gguf) | IQ4_NL | 3.87GB |
| [Writing_Partner_Mistral_7B.Q4_K_S.gguf](https://huggingface.co/RichardErkhov/FPHam_-_Writing_Partner_Mistral_7B-gguf/blob/main/Writing_Partner_Mistral_7B.Q4_K_S.gguf) | Q4_K_S | 3.86GB |
| [Writing_Partner_Mistral_7B.Q4_K.gguf](https://huggingface.co/RichardErkhov/FPHam_-_Writing_Partner_Mistral_7B-gguf/blob/main/Writing_Partner_Mistral_7B.Q4_K.gguf) | Q4_K | 4.07GB |
| [Writing_Partner_Mistral_7B.Q4_K_M.gguf](https://huggingface.co/RichardErkhov/FPHam_-_Writing_Partner_Mistral_7B-gguf/blob/main/Writing_Partner_Mistral_7B.Q4_K_M.gguf) | Q4_K_M | 4.07GB |
| [Writing_Partner_Mistral_7B.Q4_1.gguf](https://huggingface.co/RichardErkhov/FPHam_-_Writing_Partner_Mistral_7B-gguf/blob/main/Writing_Partner_Mistral_7B.Q4_1.gguf) | Q4_1 | 4.24GB |
| [Writing_Partner_Mistral_7B.Q5_0.gguf](https://huggingface.co/RichardErkhov/FPHam_-_Writing_Partner_Mistral_7B-gguf/blob/main/Writing_Partner_Mistral_7B.Q5_0.gguf) | Q5_0 | 4.65GB |
| [Writing_Partner_Mistral_7B.Q5_K_S.gguf](https://huggingface.co/RichardErkhov/FPHam_-_Writing_Partner_Mistral_7B-gguf/blob/main/Writing_Partner_Mistral_7B.Q5_K_S.gguf) | Q5_K_S | 4.65GB |
| [Writing_Partner_Mistral_7B.Q5_K.gguf](https://huggingface.co/RichardErkhov/FPHam_-_Writing_Partner_Mistral_7B-gguf/blob/main/Writing_Partner_Mistral_7B.Q5_K.gguf) | Q5_K | 4.78GB |
| [Writing_Partner_Mistral_7B.Q5_K_M.gguf](https://huggingface.co/RichardErkhov/FPHam_-_Writing_Partner_Mistral_7B-gguf/blob/main/Writing_Partner_Mistral_7B.Q5_K_M.gguf) | Q5_K_M | 4.78GB |
| [Writing_Partner_Mistral_7B.Q5_1.gguf](https://huggingface.co/RichardErkhov/FPHam_-_Writing_Partner_Mistral_7B-gguf/blob/main/Writing_Partner_Mistral_7B.Q5_1.gguf) | Q5_1 | 5.07GB |
| [Writing_Partner_Mistral_7B.Q6_K.gguf](https://huggingface.co/RichardErkhov/FPHam_-_Writing_Partner_Mistral_7B-gguf/blob/main/Writing_Partner_Mistral_7B.Q6_K.gguf) | Q6_K | 5.53GB |
| [Writing_Partner_Mistral_7B.Q8_0.gguf](https://huggingface.co/RichardErkhov/FPHam_-_Writing_Partner_Mistral_7B-gguf/blob/main/Writing_Partner_Mistral_7B.Q8_0.gguf) | Q8_0 | 7.17GB |
Original model description:
---
license: apache-2.0
language:
- en
tags:
- mistral
- instruct
- finetune
- chatml
- gpt4
---
<!-- header start -->
<div style="display: flex; flex-direction: column; align-items: center;">
</div>
<div style="width: 100%;">
<img src="https://huggingface.co/FPHam/Writing_Partner_Mistral_7B/resolve/main/writer5.jpg" alt="Writing Partner" style="width: 70%; min-width: 200px; display: block; margin: auto;">
</div>
<div style="display: flex; flex-direction: column; align-items: center;">
<p><a href="https://ko-fi.com/Q5Q5MOB4M">Support me at Ko-fi</a></p>
</div>
<!-- header end -->
Hi there! I'm your writing assistant.
Think of me as your partner in crime---or rather, your partner in writing. When you hit a wall (and trust me, you will), I'll be there to knock it down for you.
If you find yourself stumped, I can help you think outside the box, whether you need to figure out what happens next, develop interesting sidekicks, or create a believable love interest. And if you get stuck on names for people or places, well, that's my specialty.
Just give me a shout if you have questions, need advice, or want to bounce an idea off someone. Together, we'll turn your novel into a best seller!
# Intended Use
The purpose of this model isn't to write content for you (although it can), but rather to engage in a conversation about your writing process.
# Model uses ChatML
```
<|im_start|>system
<|im_end|>
<|im_start|>user
How to plot my story?<|im_end|>
<|im_start|>assistant
```
Example 1: (I had ChatGPT generate a paragraph for a sci-fi story.)
Here is an opening of my story:
In the distant reaches of the cosmos, where starlight weaves a tapestry of unknown wonders, Captain Alara Voss navigated her spacecraft, the Celestial Serenity, through the interstellar currents. The ship hummed with the pulsating energy of the quantum drive, propelling them toward the uncharted nebula known as the Veil of Eternity. Alara's gaze was fixed on the swirling cosmic clouds ahead, her mind ablaze with the anticipation of discovery. As the Serenity pierced the ethereal veil, the fabric of reality seemed to dance with a mysterious resonance. Little did the intrepid crew know that beyond the shimmering curtain lay a realm where time itself wove a narrative that defied mortal understanding, setting the stage for an odyssey that would reshape the boundaries of human perception.
>I like this opening! It sets up some interesting questions and intrigue right off the bat. But I do think you might want to reconsider your point of view in the first paragraph. Right now it sounds more like a summary than an actual scene because there isn't any dialogue or action. Consider starting with something more immediate, perhaps from Alara's perspective. You could even begin with her looking out at the stars, thinking about what she sees. This will give us a chance to get to know her better before you launch into the big picture stuff. Here's an example:
>
>Alara Voss leaned against the cold metal railing of the observation deck, staring out into the infinite expanse of blackness that surrounded her ship. Stars dotted the night sky like scattered diamonds, each one a potential destination, each one a mystery waiting to be solved. She took a deep breath and let it out slowly, savoring the feeling of freedom that washed over her. It had been months since they'd left Earth, and already their journey had taken them light-years away from everything they knew. Her thoughts were interrupted by the low hum of the quantum drive kicking in, signaling that they were ready to make another jump. She watched as the stars began to blur together, forming a shimmering pathway that led the way to their next adventure.
>
>"Captain," came the voice of her second-in-command over the intercom. "We're entering the Veil of Eternity."
>
>"Thank you, Ensign," Alara replied, not taking her eyes off the swirling vortex ahead. "Let's see what lies beyond."
Example 2:
How to write proper dialogue?
>Dialogue is a very important part of writing fiction. It's the way characters speak, and it helps define who they are. It also moves the story forward and adds tension, humor, or emotion. Dialogue must be realistic and natural-sounding, but it doesn't have to be boring. You want your readers to pay attention to what the characters are saying, so make sure that each line of dialogue counts.
>
>Here are some tips on how to write good dialogue:
>
>- Keep it short. Long speeches get tedious quickly. If one character talks for more than two sentences, break up his speech with an action or interjection from another character.
>
>- Use contractions (I'm, he'll). They sound more like real conversation than formal language does.
>
>- Vary sentence structure. This makes dialogue easier to read and understand.
>
>- Don't use slang unless it fits the setting or character perfectly. Slang dates easily and may not mean anything to future generations.
>
>- Avoid using too many adverbs in dialogue tags. Instead of "he said loudly," try something like "he bellowed." Or "she whispered."
>
>- Be careful not to give away too much information in dialogue tags. For example, if you say "Sarah laughed nervously," you're telling the reader she was nervous rather than showing her anxiety through her words or actions.
>
>- Make sure your characters don't all talk alike. Each person should have a unique voice, based on his background, education, age, gender, etc.
>
>- Don't forget about body language. The way someone holds himself, sits, stands, fidgets, or looks at others can add depth to a scene.
>
>- Use dialogue to reveal character traits and motivations. Listen carefully to people around you, and then translate those conversations onto paper.
>
>- Finally, remember that dialogue needs to serve a purpose in your story. Whether it advances the plot, sets the mood, or creates conflict, every word spoken by your characters should move the narrative along.
# [Open LLM Leaderboard Evaluation Results](https://huggingface.co/spaces/HuggingFaceH4/open_llm_leaderboard)
Detailed results can be found [here](https://huggingface.co/datasets/open-llm-leaderboard/details_FPHam__Writing_Partner_Mistral_7B)
| Metric |Value|
|---------------------------------|----:|
|Avg. |65.29|
|AI2 Reasoning Challenge (25-Shot)|64.59|
|HellaSwag (10-Shot) |84.59|
|MMLU (5-Shot) |62.55|
|TruthfulQA (0-shot) |48.55|
|Winogrande (5-shot) |76.87|
|GSM8k (5-shot) |54.59|
|
stas/tiny-wmt19-en-ru | stas | "2021-05-03T01:47:47Z" | 962 | 0 | transformers | [
"transformers",
"pytorch",
"fsmt",
"text2text-generation",
"wmt19",
"testing",
"en",
"ru",
"dataset:wmt19",
"license:apache-2.0",
"autotrain_compatible",
"endpoints_compatible",
"region:us"
] | text2text-generation | "2022-03-02T23:29:05Z" | ---
language:
- en
- ru
thumbnail:
tags:
- wmt19
- testing
license: apache-2.0
datasets:
- wmt19
metrics:
- bleu
---
# Tiny FSMT en-ru
This is a tiny model that is used in the `transformers` test suite. It doesn't do anything useful, other than testing that `modeling_fsmt.py` is functional.
Do not try to use it for anything that requires quality.
The model is indeed 30KB in size.
You can see how it was created [here](https://huggingface.co/stas/tiny-wmt19-en-ru/blob/main/fsmt-make-super-tiny-model.py).
If you're looking for the real model, please go to [https://huggingface.co/facebook/wmt19-en-ru](https://huggingface.co/facebook/wmt19-en-ru).
|
ProomptEngineer/pe-neon-sign-style | ProomptEngineer | "2023-09-11T15:21:13Z" | 962 | 5 | diffusers | [
"diffusers",
"text-to-image",
"stable-diffusion",
"lora",
"base_model:stabilityai/stable-diffusion-xl-base-1.0",
"license:other",
"region:us"
] | text-to-image | "2023-09-11T15:21:08Z" | ---
license: other
tags:
- text-to-image
- stable-diffusion
- lora
- diffusers
base_model: stabilityai/stable-diffusion-xl-base-1.0
instance_prompt: PENeonSign
widget:
- text: PENeonSign
---
# PE Neon Sign [Style]

<p>you favorite character as a neon sign...</p><p>weights 0.8-1</p><h2 id="heading-63">If you want to donate:</h2><h2 id="heading-64"><a target="_blank" rel="ugc" href="https://ko-fi.com/proomptengineer">https://ko-fi.com/proomptengineer</a></h2>
## Image examples for the model:









|
kalisai/Nusantara-0.8b-Indo-Chat | kalisai | "2024-03-14T06:45:39Z" | 962 | 2 | transformers | [
"transformers",
"safetensors",
"qwen2",
"text-generation",
"conversational",
"convAI",
"id",
"en",
"dataset:FreedomIntelligence/evol-instruct-indonesian",
"license:apache-2.0",
"autotrain_compatible",
"endpoints_compatible",
"text-generation-inference",
"region:us"
] | text-generation | "2024-03-06T22:18:11Z" | ---
library_name: transformers
widget:
- messages:
- role: system
content: Anda adalah seorang konselor karir. User akan memberi Anda seorang individu
mencari bimbingan dalam kehidupan profesional mereka, dan tugas Anda adalah membantu
mereka dalam menentukan karir apa yang paling cocok bagi mereka berdasarkan keterampilan mereka,
minat, dan pengalaman. Anda juga harus melakukan penelitian terhadap berbagai hal tersebut
pilihan yang tersedia, jelaskan tren pasar kerja di berbagai industri, Dan
saran tentang kualifikasi mana yang akan bermanfaat untuk mengejar bidang tertentu.
- role: user
content: Hellow!
- role: assistant
content: Hai! Apa yang bisa saya bantu?
- role: user
content: Saya tertarik untuk mengembangkan karir di bidang rekayasa perangkat lunak. Apa
Anda mau merekomendasikan saya untuk melakukannya?
- messages:
- role: system
content: Anda adalah asisten yang berpengetahuan luas. Bantu user sebanyak yang Anda bisa.
- role: user
content: Bagaimana caranya menjadi lebih sehat?
- messages:
- role: system
content: Anda adalah asisten yang membantu dan memberikan tanggapan yang cerdas.
- role: user
content: Haloooo Bund!
- role: assistant
content: Halo! Apa yang bisa saya bantu?
- role: user
content: Saya perlu membangun situs web sederhana. Di mana saya harus mulai belajar tentang pengembangan web?
- messages:
- role: system
content: Anda adalah asisten yang sangat kreatif. Pengguna akan memberi Anda tugas, yang harus Anda selesaikan dengan seluruh pengetahuan Anda.
- role: user
content: Tulis latar belakang cerita game RPG tentang penyihir dan naga di dunia fiksi ilmiah.
inference:
parameters:
max_new_tokens: 128
penalty_alpha: 0.5
top_k: 4
pipeline_tag: text-generation
tags:
- conversational
- convAI
license: apache-2.0
language:
- id
- en
datasets:
- FreedomIntelligence/evol-instruct-indonesian
---

### Model Description
Nusantara is a series of Open Weight Language Model of Bahasa Indonesia (Indonesia language). Nusantara is based from Qwen1.5 Language Model, finetuned by domain specific of datasets.
As Chat-implemented language model, Nusantara is capable to do Question-Answering and respond to instructions given in Bahasa Indonesia.
Due to limited resources, only 0.8B, 1.8B, 2.7B, 4B and 7B models are available. If you're interested in funding this project for further development, specific usage, or larger parameters, please contact us.
- **Finetuned by:** [Kalis AI](https://huggingface.co/kalisai)
- **Funded by:** Self-funded
- **Model type:** transformer-based decoder-only language model
- **Language(s):** Bahasa Indonesia (id), English (en)
- **License:** Nusantara is licensed under Apache-2.0, but any usage of this model should comply with [Qwen License](https://huggingface.co/Qwen/Qwen1.5-4B/blob/main/LICENSE)
- **Finetuned from model:** [Qwen1.5-4B](https://huggingface.co/Qwen/Qwen1.5-4B/tree/main)
### Attentions!
<!-- This section is meant to convey recommendations with respect to the bias, risk, and technical limitations. -->
Due to certain circumstances, models with <4B parameters tend to hallucinate easily. Users (both direct and downstream) should be made aware of the risks, biases and limitations of the model.
Because this model is also trained with uncensored datasets, there is the possibility of negative impacts arising from using this model. All kinds of impacts that arise as a result of using this model are entirely the responsibility of the user. The model maker is not responsible for any risks incurred.
## How to Get Started with the Model
Here provides a code snippet with `apply_chat_template` to show you how to load the tokenizer and model and how to generate contents.
```python
from transformers import AutoModelForCausalLM, AutoTokenizer
device = "cuda" # the device to load the model onto
model = AutoModelForCausalLM.from_pretrained(
"kalisai/Nusantara-0.8B-Indo-Chat",
torch_dtype="auto",
device_map="auto"
)
tokenizer = AutoTokenizer.from_pretrained("kalisai/Nusantara-0.8B-Indo-Chat")
prompt = "Berikan saya resep memasak nasi goreng yang lezat."
messages = [
{"role": "system", "content": "Kamu adalah Nusantara, asisten AI yang pintar."},
{"role": "user", "content": prompt}
]
text = tokenizer.apply_chat_template(
messages,
tokenize=False,
add_generation_prompt=True
)
model_inputs = tokenizer([text], return_tensors="pt").to(device)
generated_ids = model.generate(
model_inputs.input_ids,
max_new_tokens=512
)
generated_ids = [
output_ids[len(input_ids):] for input_ids, output_ids in zip(model_inputs.input_ids, generated_ids)
]
response = tokenizer.batch_decode(generated_ids, skip_special_tokens=True)[0]
```
## Citation
If you use the Nusantara language model in your research or project, please cite it as:
```
@misc{zulfikar_aji_kusworo_2024,
title={Nusantara: A Series of Versatile Open Weight Language Model of Bahasa Indonesia},
author={Zulfikar Aji Kusworo},
publisher={Hugging Face}
journal={Hugging Face Repository},
year={2024}
url = {https://huggingface.co/kalisai}
}
``` |
Shengkun/LLama2-7B-Structural-Prune-1.25x | Shengkun | "2024-05-29T10:34:49Z" | 962 | 0 | transformers | [
"transformers",
"safetensors",
"llama",
"text-generation",
"arxiv:1910.09700",
"license:apache-2.0",
"autotrain_compatible",
"endpoints_compatible",
"text-generation-inference",
"region:us"
] | text-generation | "2024-05-27T20:21:44Z" | ---
license: apache-2.0
---
---
library_name: transformers
license: apache-2.0
---
# Model Card for Model ID
<!-- Provide a quick summary of what the model is/does. -->
## Model Details
### Model Description
<!-- Provide a longer summary of what this model is. -->
This is the model card of a 🤗 transformers model that has been pushed on the Hub. This model card has been automatically generated.
- **Developed by:** [More Information Needed]
- **Funded by [optional]:** [More Information Needed]
- **Shared by [optional]:** [More Information Needed]
- **Model type:** [More Information Needed]
- **Language(s) (NLP):** [More Information Needed]
- **License:** [More Information Needed]
- **Finetuned from model [optional]:** [More Information Needed]
### Model Sources [optional]
<!-- Provide the basic links for the model. -->
- **Repository:** [More Information Needed]
- **Paper [optional]:** [More Information Needed]
- **Demo [optional]:** [More Information Needed]
## Uses
<!-- Address questions around how the model is intended to be used, including the foreseeable users of the model and those affected by the model. -->
### Direct Use
<!-- This section is for the model use without fine-tuning or plugging into a larger ecosystem/app. -->
[More Information Needed]
### Downstream Use [optional]
<!-- This section is for the model use when fine-tuned for a task, or when plugged into a larger ecosystem/app -->
[More Information Needed]
### Out-of-Scope Use
<!-- This section addresses misuse, malicious use, and uses that the model will not work well for. -->
[More Information Needed]
## Bias, Risks, and Limitations
<!-- This section is meant to convey both technical and sociotechnical limitations. -->
[More Information Needed]
### Recommendations
<!-- This section is meant to convey recommendations with respect to the bias, risk, and technical limitations. -->
Users (both direct and downstream) should be made aware of the risks, biases and limitations of the model. More information needed for further recommendations.
## How to Get Started with the Model
Use the code below to get started with the model.
[More Information Needed]
## Training Details
### Training Data
<!-- This should link to a Dataset Card, perhaps with a short stub of information on what the training data is all about as well as documentation related to data pre-processing or additional filtering. -->
[More Information Needed]
### Training Procedure
<!-- This relates heavily to the Technical Specifications. Content here should link to that section when it is relevant to the training procedure. -->
#### Preprocessing [optional]
[More Information Needed]
#### Training Hyperparameters
- **Training regime:** [More Information Needed] <!--fp32, fp16 mixed precision, bf16 mixed precision, bf16 non-mixed precision, fp16 non-mixed precision, fp8 mixed precision -->
#### Speeds, Sizes, Times [optional]
<!-- This section provides information about throughput, start/end time, checkpoint size if relevant, etc. -->
[More Information Needed]
## Evaluation
<!-- This section describes the evaluation protocols and provides the results. -->
### Testing Data, Factors & Metrics
#### Testing Data
<!-- This should link to a Dataset Card if possible. -->
[More Information Needed]
#### Factors
<!-- These are the things the evaluation is disaggregating by, e.g., subpopulations or domains. -->
[More Information Needed]
#### Metrics
<!-- These are the evaluation metrics being used, ideally with a description of why. -->
[More Information Needed]
### Results
[More Information Needed]
#### Summary
## Model Examination [optional]
<!-- Relevant interpretability work for the model goes here -->
[More Information Needed]
## Environmental Impact
<!-- Total emissions (in grams of CO2eq) and additional considerations, such as electricity usage, go here. Edit the suggested text below accordingly -->
Carbon emissions can be estimated using the [Machine Learning Impact calculator](https://mlco2.github.io/impact#compute) presented in [Lacoste et al. (2019)](https://arxiv.org/abs/1910.09700).
- **Hardware Type:** [More Information Needed]
- **Hours used:** [More Information Needed]
- **Cloud Provider:** [More Information Needed]
- **Compute Region:** [More Information Needed]
- **Carbon Emitted:** [More Information Needed]
## Technical Specifications [optional]
### Model Architecture and Objective
[More Information Needed]
### Compute Infrastructure
[More Information Needed]
#### Hardware
[More Information Needed]
#### Software
[More Information Needed]
## Citation [optional]
<!-- If there is a paper or blog post introducing the model, the APA and Bibtex information for that should go in this section. -->
**BibTeX:**
[More Information Needed]
**APA:**
[More Information Needed]
## Glossary [optional]
<!-- If relevant, include terms and calculations in this section that can help readers understand the model or model card. -->
[More Information Needed]
## More Information [optional]
[More Information Needed]
## Model Card Authors [optional]
[More Information Needed]
## Model Card Contact
[More Information Needed] |
plasmo/vox2 | plasmo | "2023-05-05T11:26:46Z" | 961 | 44 | diffusers | [
"diffusers",
"text-to-image",
"stable-diffusion",
"license:creativeml-openrail-m",
"autotrain_compatible",
"endpoints_compatible",
"diffusers:StableDiffusionPipeline",
"region:us"
] | text-to-image | "2022-11-25T19:20:36Z" | ---
license: creativeml-openrail-m
tags:
- text-to-image
- stable-diffusion
widget:
- text: "voxel-ish "
---
### Jak's Voxel-ish Image Pack v.1.2 for Stable Diffusion
VERSION 1.2 of Voxel-ish Image Pack brought to you by 184 training images through 8000 training steps, 20% Training text crafted by Jak_TheAI_Artist
version history: v1.2 - Fine tuned for better faces.
Include Prompt trigger: "voxel-ish" to activate.
Tip: add "intricate detail" in prompt to make a semi-realistic image.
Sample pictures of this concept:
voxel-ish
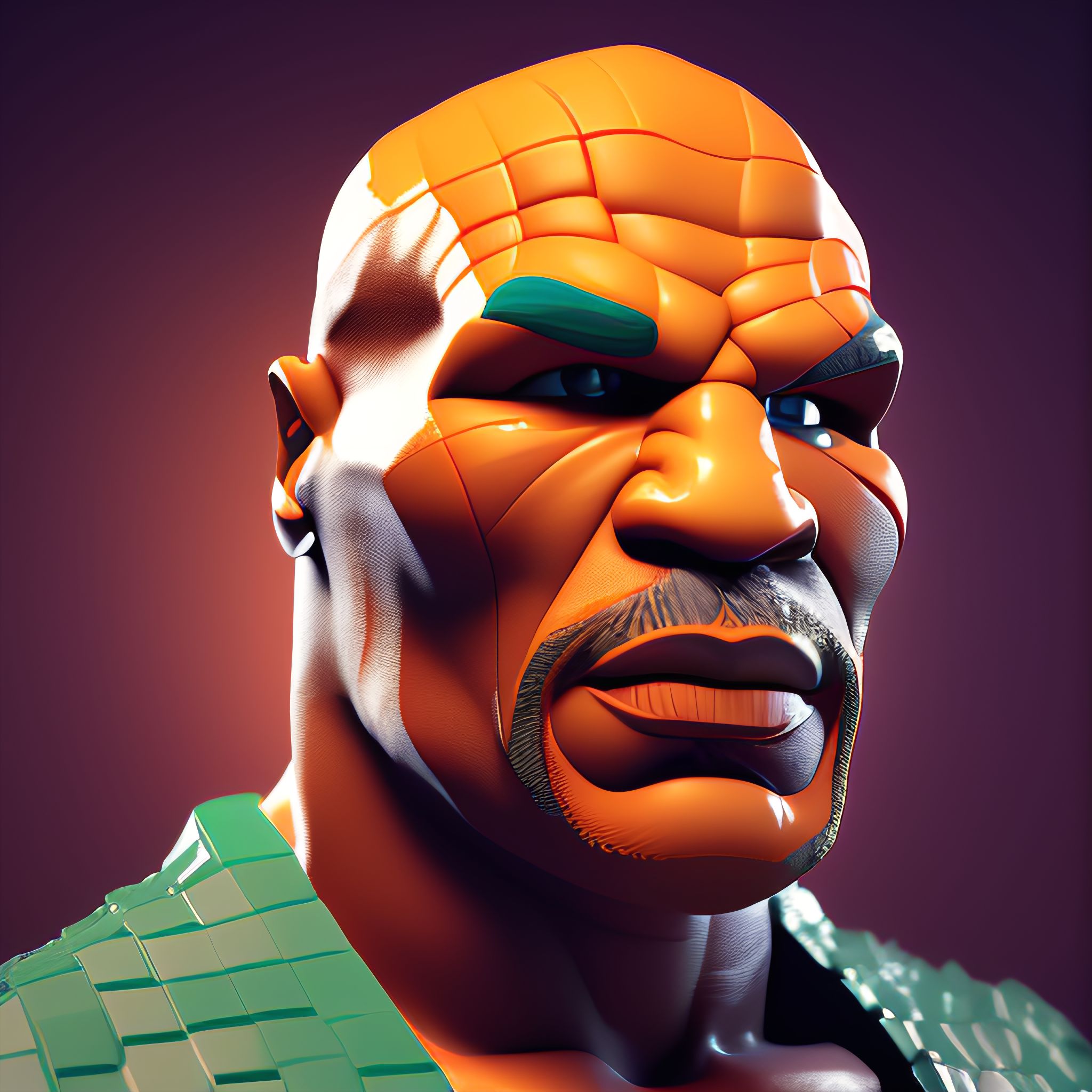
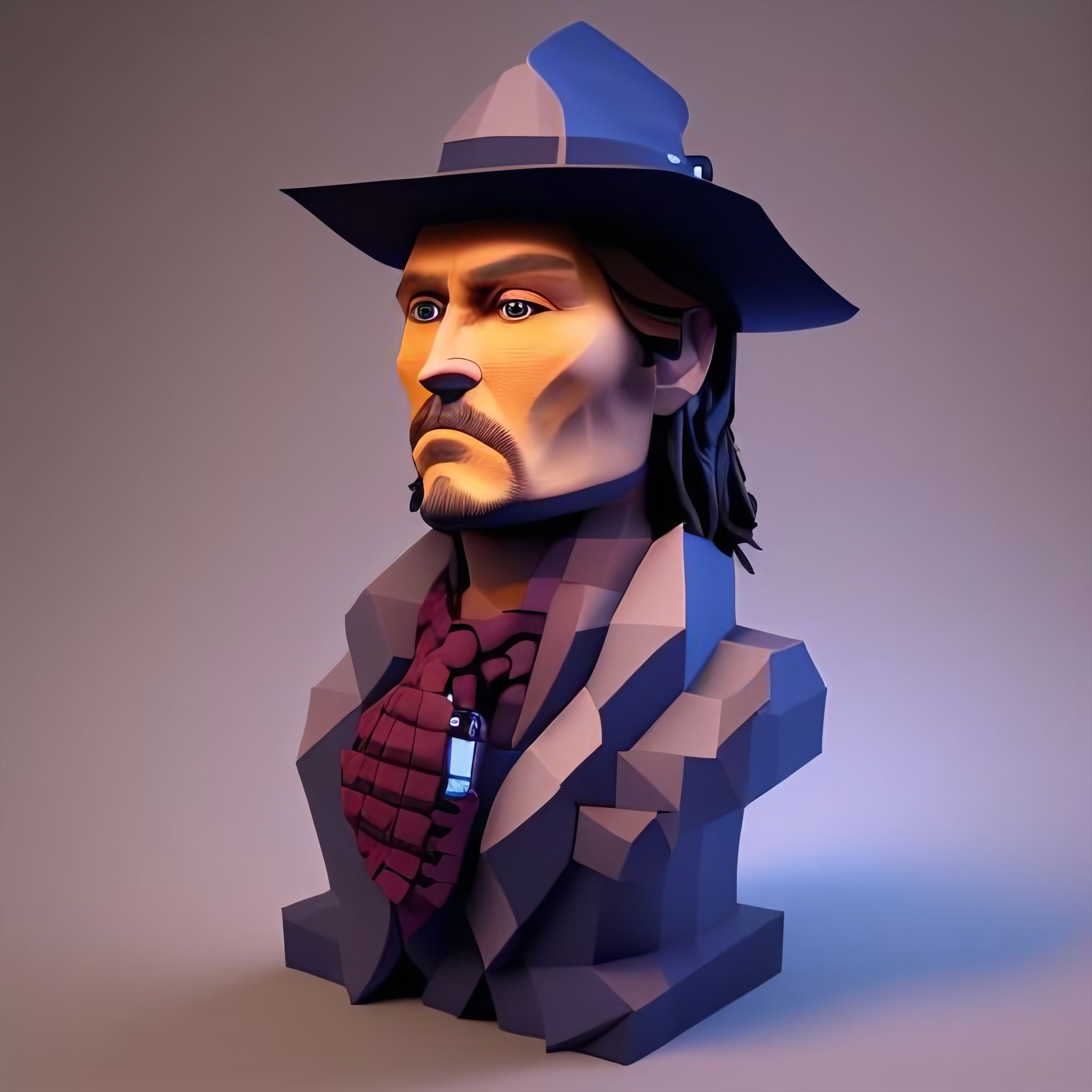
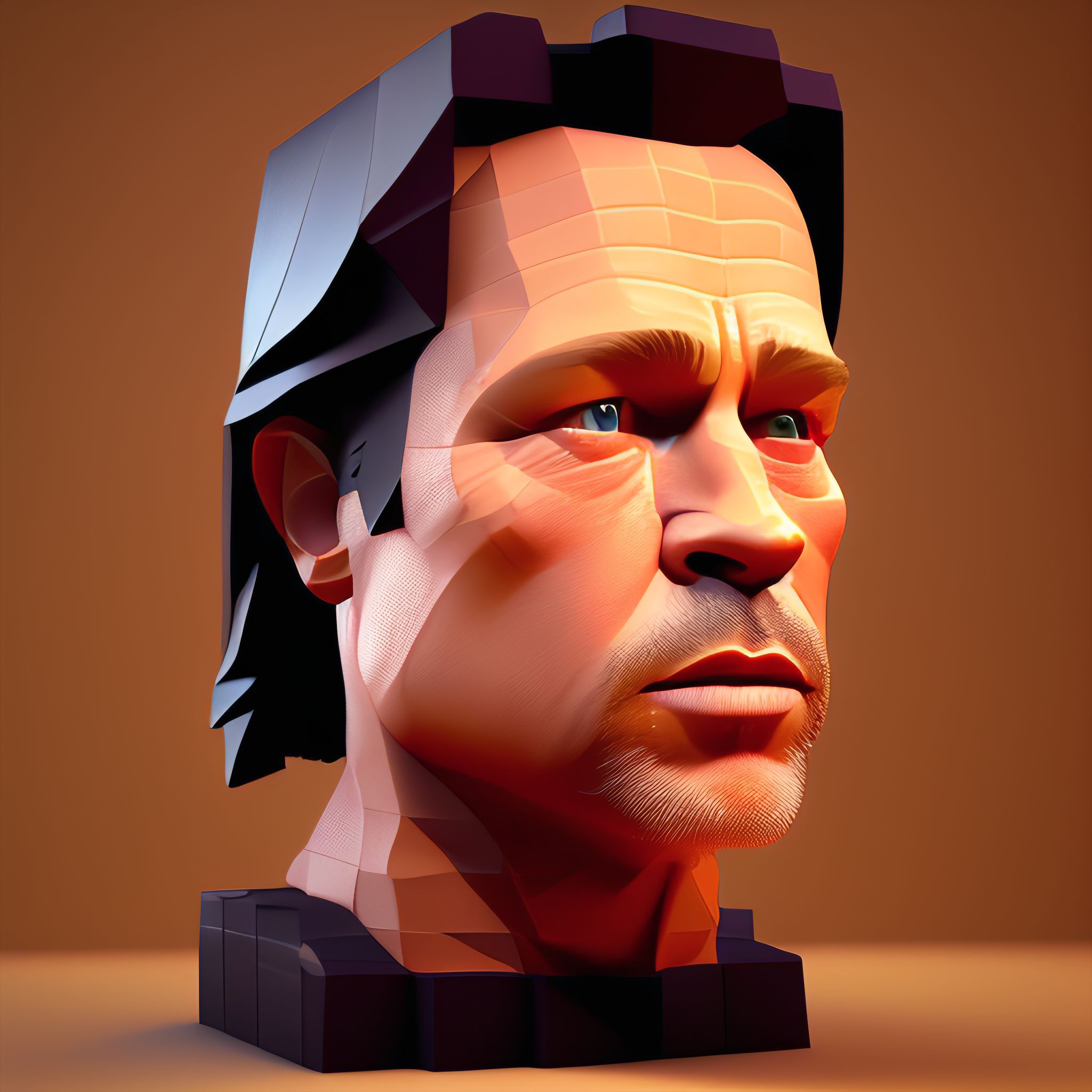
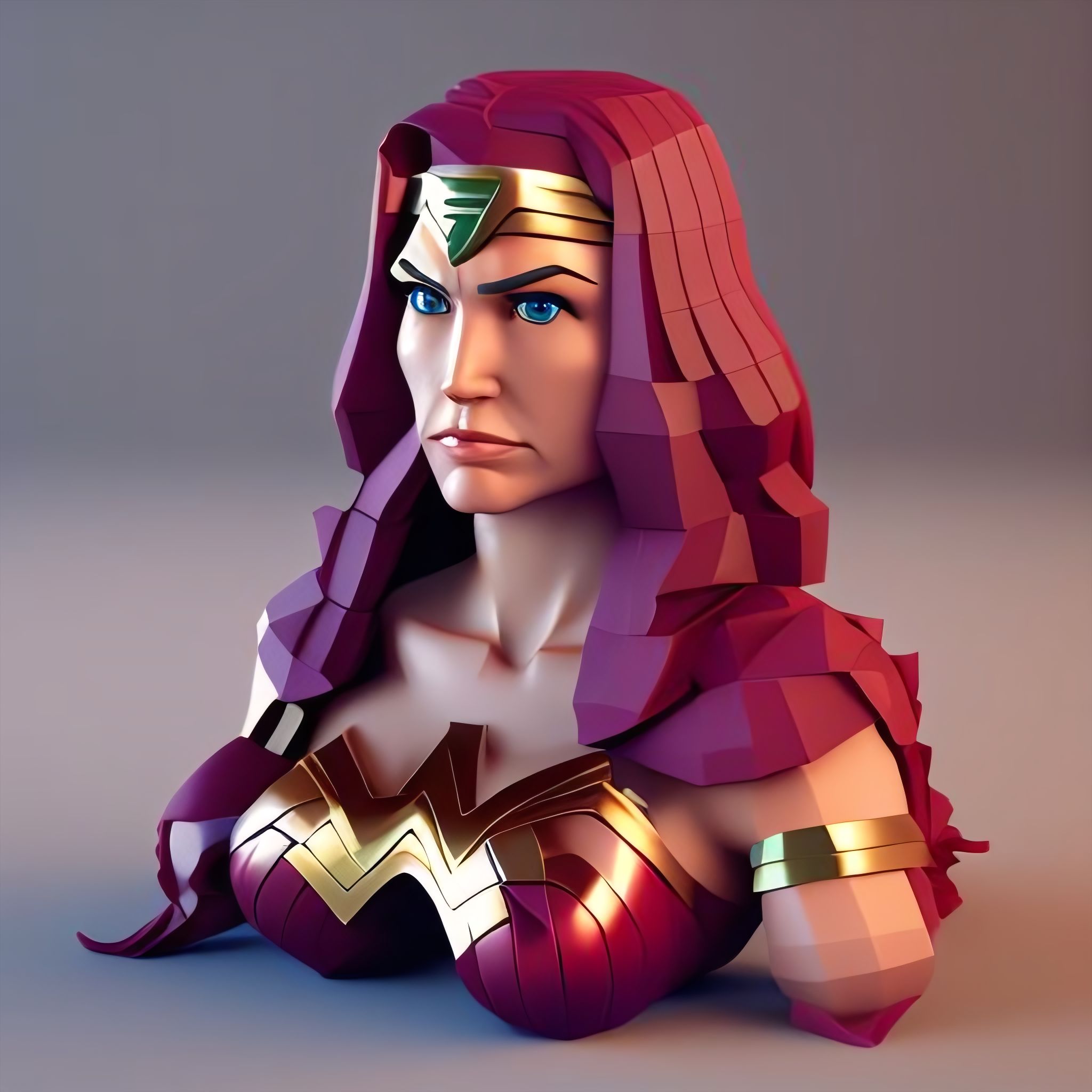
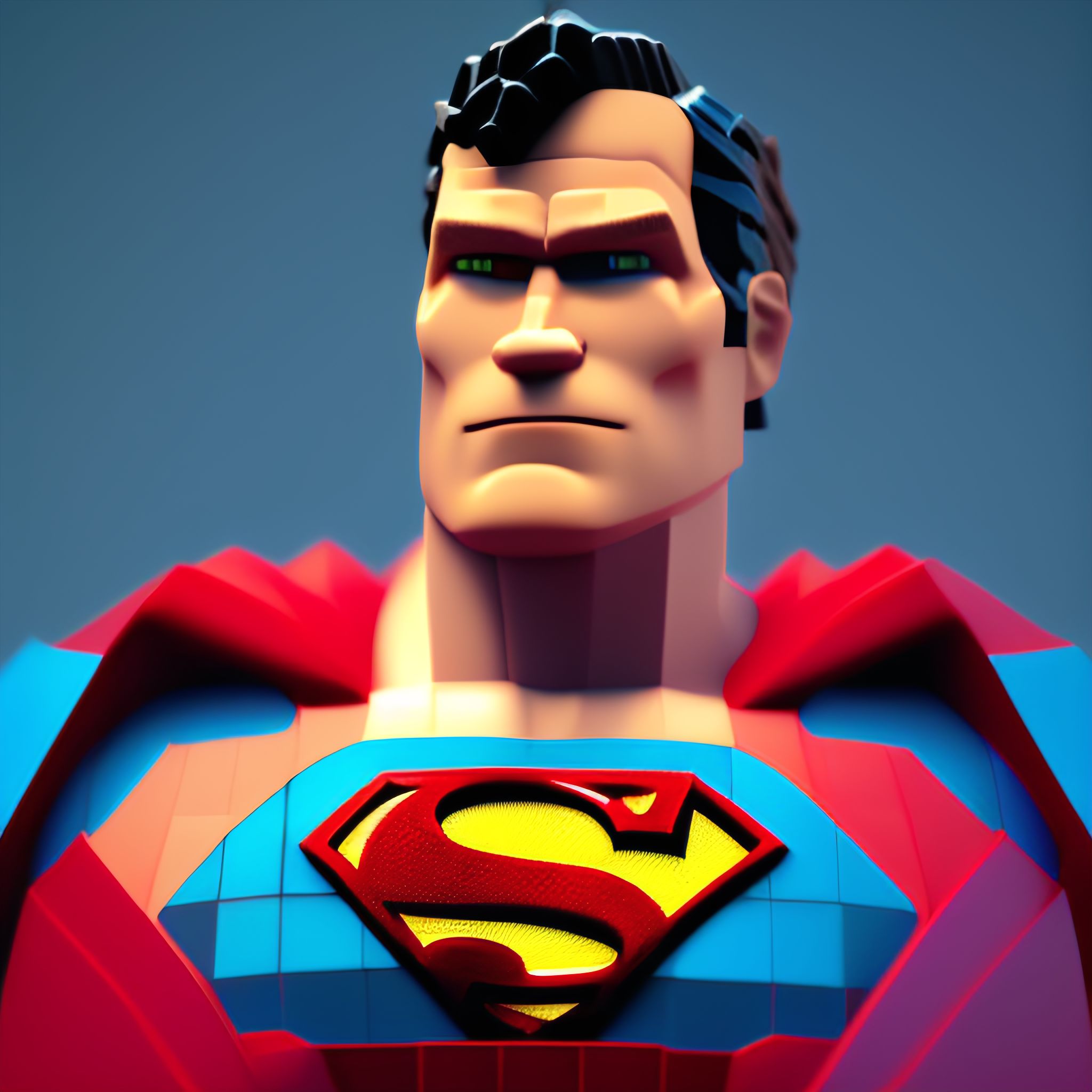
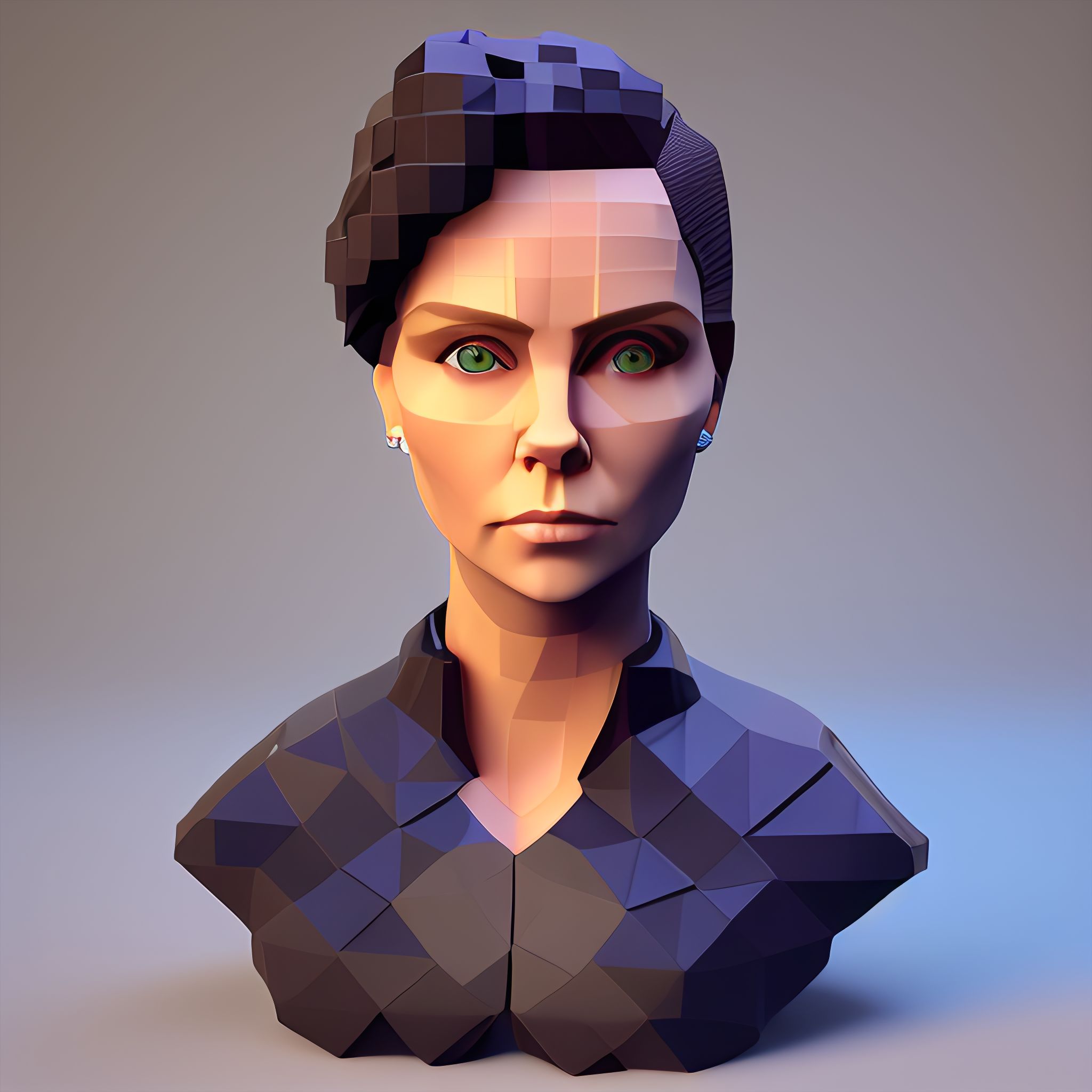
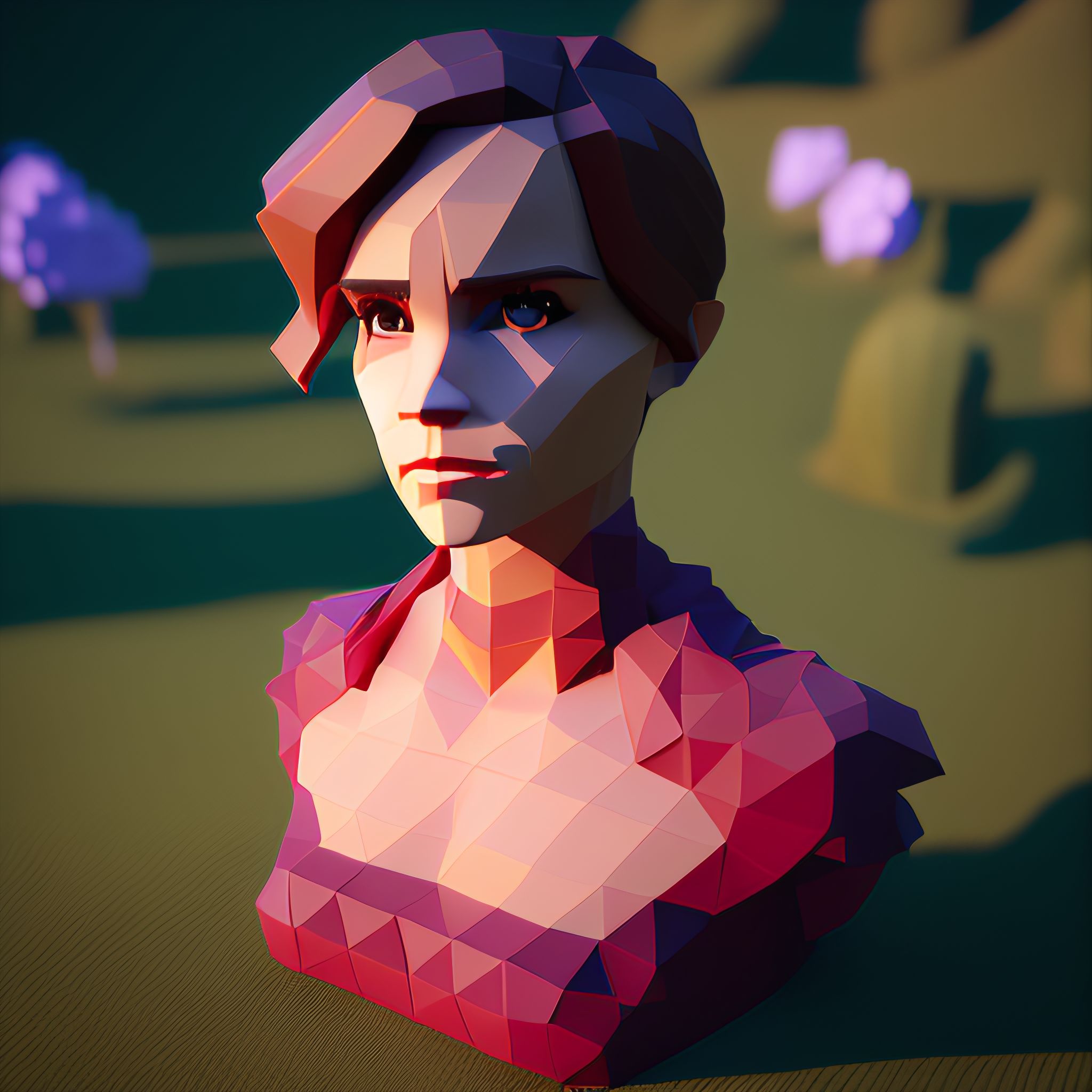
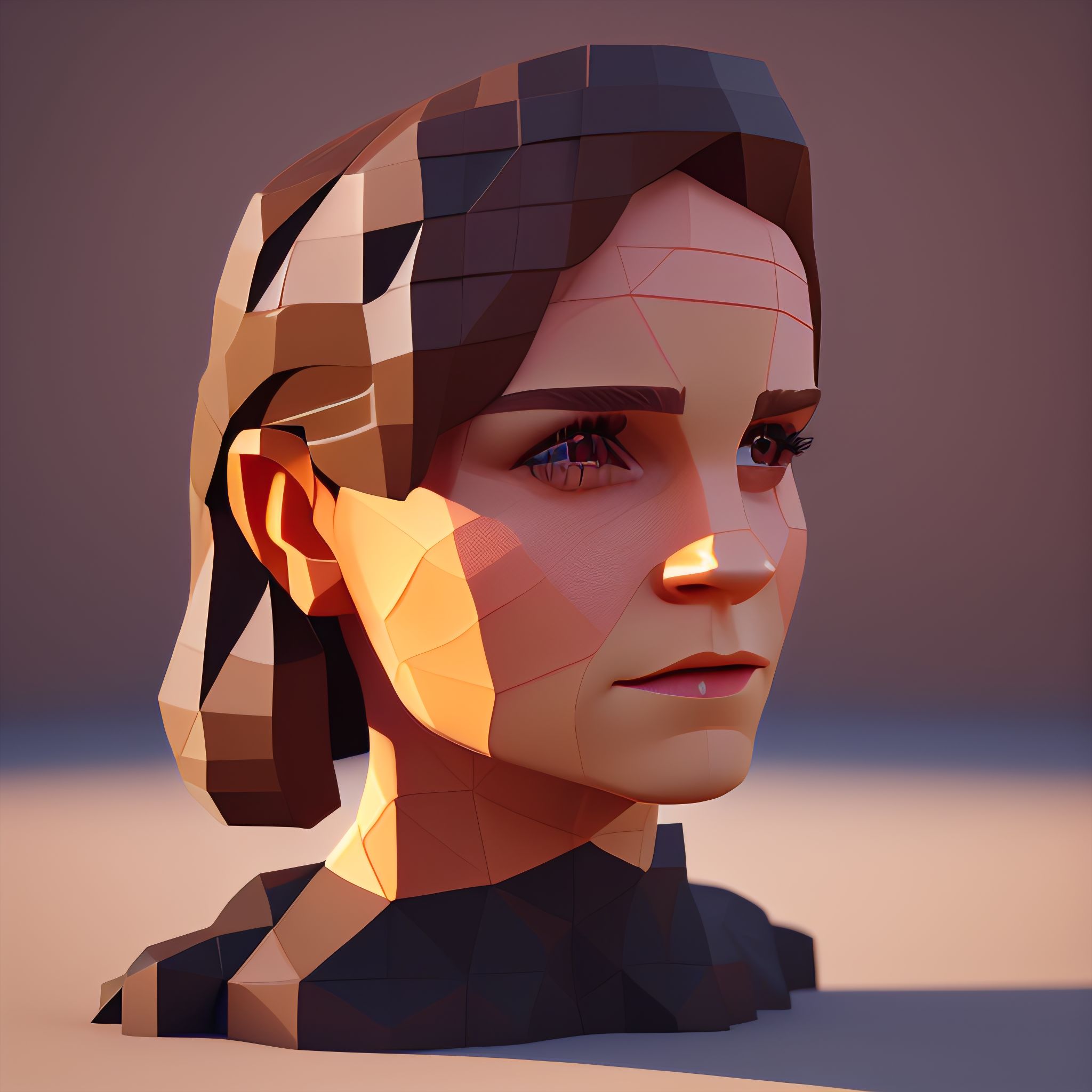
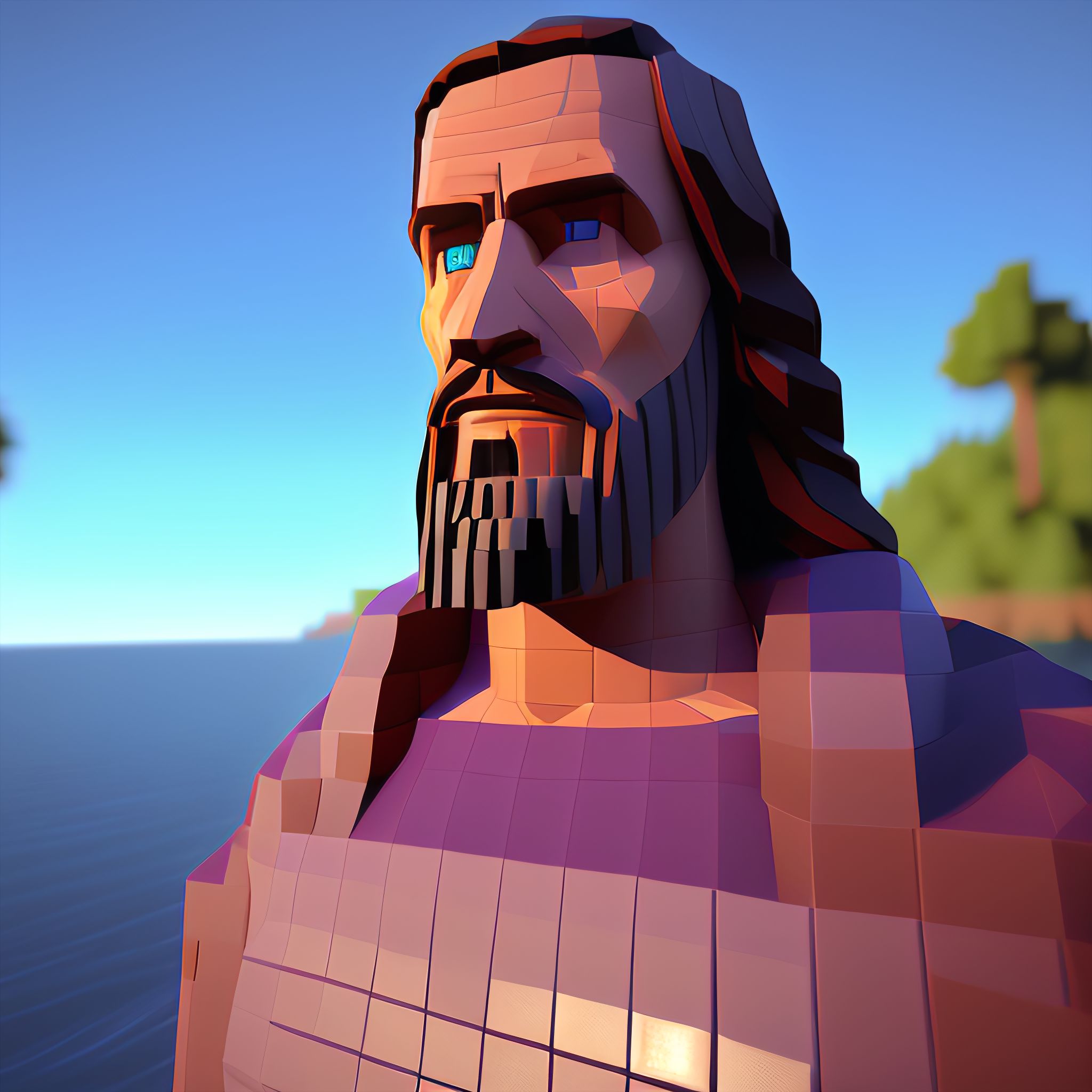
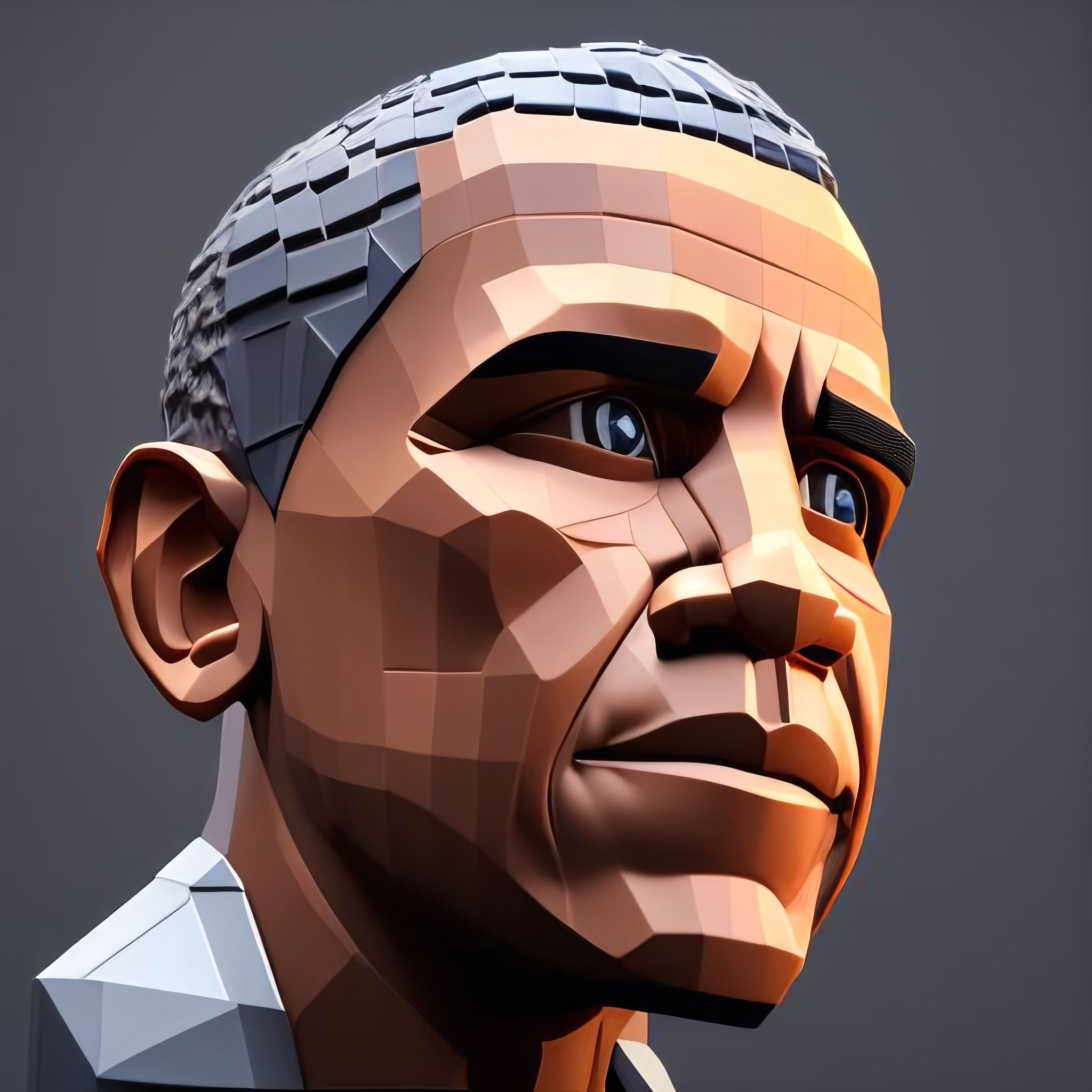
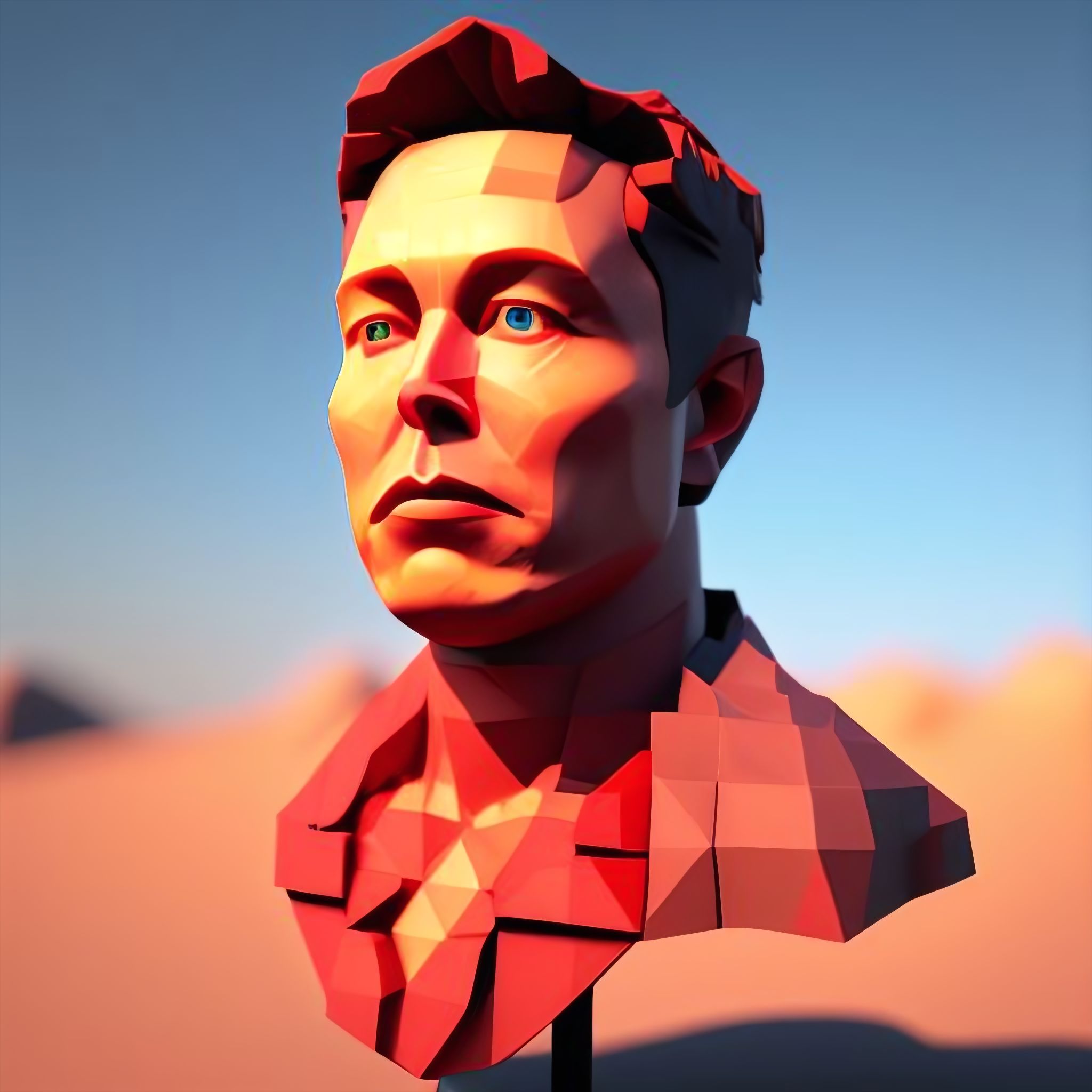
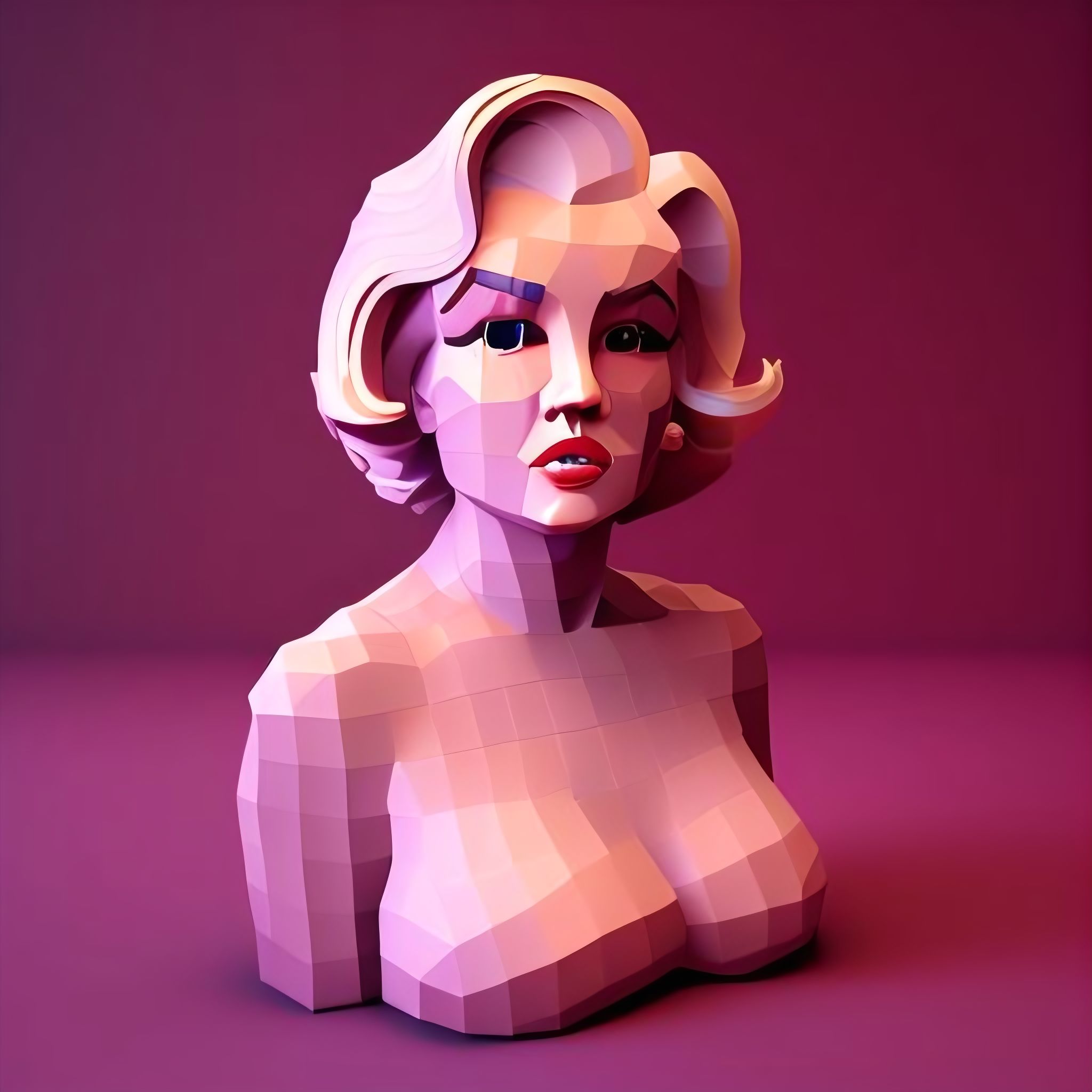
|
NousResearch/Nous-Hermes-2-Mixtral-8x7B-SFT | NousResearch | "2024-02-20T09:18:01Z" | 961 | 55 | transformers | [
"transformers",
"safetensors",
"mixtral",
"text-generation",
"Mixtral",
"instruct",
"finetune",
"chatml",
"gpt4",
"synthetic data",
"distillation",
"conversational",
"en",
"dataset:teknium/OpenHermes-2.5",
"base_model:mistralai/Mixtral-8x7B-v0.1",
"license:apache-2.0",
"autotrain_compatible",
"endpoints_compatible",
"text-generation-inference",
"region:us"
] | text-generation | "2023-12-26T03:37:00Z" | ---
base_model: mistralai/Mixtral-8x7B-v0.1
tags:
- Mixtral
- instruct
- finetune
- chatml
- gpt4
- synthetic data
- distillation
model-index:
- name: Nous-Hermes-2-Mixtral-8x7B-SFT
results: []
license: apache-2.0
language:
- en
datasets:
- teknium/OpenHermes-2.5
---
# Nous Hermes 2 - Mixtral 8x7B - SFT

## Model description
Nous Hermes 2 Mixtral 8x7B SFT is the supervised finetune only version of our new flagship Nous Research model trained over the [Mixtral 8x7B MoE LLM](https://huggingface.co/mistralai/Mixtral-8x7B-v0.1).
The model was trained on over 1,000,000 entries of primarily GPT-4 generated data, as well as other high quality data from open datasets across the AI landscape, achieving state of the art performance on a variety of tasks.
This is the SFT only version of Mixtral Hermes 2, we have also released an SFT+DPO version, for people to find which works best for them, which can be found here: https://huggingface.co/NousResearch/Nous-Hermes-2-Mixtral-8x7B-DPO
## We are grateful to Together.ai for sponsoring our compute during the many experiments both training Mixtral and working on DPO!
# Table of Contents
1. [Example Outputs](#example-outputs)
2. [Benchmark Results](#benchmark-results)
- GPT4All
- AGIEval
- BigBench
- Comparison to Mixtral-Instruct
3. [Prompt Format](#prompt-format)
4. [Inference Example Code](#inference-code)
5. [Quantized Models](#quantized-models)
## Example Outputs
### Writing Code for Data Visualization

### Writing Cyberpunk Psychedelic Poems

### Performing Backtranslation to Create Prompts from Input Text

## Benchmark Results
Nous-Hermes 2 on Mixtral 8x7B SFT is the bedrock for major improvements on many of the benchmarks below compared to the base Mixtral model, and is the SFT only version of our first model to beat the flagship Mixtral Finetune by MistralAI (the DPO version).
## GPT4All:
```
| Task |Version| Metric |Value | |Stderr|
|-------------|------:|--------|-----:|---|-----:|
|arc_challenge| 0|acc |0.5904|± |0.0144|
| | |acc_norm|0.6323|± |0.0141|
|arc_easy | 0|acc |0.8594|± |0.0071|
| | |acc_norm|0.8607|± |0.0071|
|boolq | 1|acc |0.8783|± |0.0057|
|hellaswag | 0|acc |0.6592|± |0.0047|
| | |acc_norm|0.8434|± |0.0036|
|openbookqa | 0|acc |0.3400|± |0.0212|
| | |acc_norm|0.4660|± |0.0223|
|piqa | 0|acc |0.8324|± |0.0087|
| | |acc_norm|0.8379|± |0.0086|
|winogrande | 0|acc |0.7569|± |0.0121|
```
Average: 75.36
## AGIEval:
```
| Task |Version| Metric |Value | |Stderr|
|------------------------------|------:|--------|-----:|---|-----:|
|agieval_aqua_rat | 0|acc |0.2441|± |0.0270|
| | |acc_norm|0.2598|± |0.0276|
|agieval_logiqa_en | 0|acc |0.4025|± |0.0192|
| | |acc_norm|0.3978|± |0.0192|
|agieval_lsat_ar | 0|acc |0.2391|± |0.0282|
| | |acc_norm|0.2043|± |0.0266|
|agieval_lsat_lr | 0|acc |0.5353|± |0.0221|
| | |acc_norm|0.5098|± |0.0222|
|agieval_lsat_rc | 0|acc |0.6617|± |0.0289|
| | |acc_norm|0.5948|± |0.0300|
|agieval_sat_en | 0|acc |0.7961|± |0.0281|
| | |acc_norm|0.7816|± |0.0289|
|agieval_sat_en_without_passage| 0|acc |0.4757|± |0.0349|
| | |acc_norm|0.4515|± |0.0348|
|agieval_sat_math | 0|acc |0.4818|± |0.0338|
| | |acc_norm|0.3909|± |0.0330|
```
Average: 44.89
## BigBench:
```
| Task |Version| Metric |Value | |Stderr|
|------------------------------------------------|------:|---------------------|-----:|---|-----:|
|bigbench_causal_judgement | 0|multiple_choice_grade|0.5789|± |0.0359|
|bigbench_date_understanding | 0|multiple_choice_grade|0.7154|± |0.0235|
|bigbench_disambiguation_qa | 0|multiple_choice_grade|0.5388|± |0.0311|
|bigbench_geometric_shapes | 0|multiple_choice_grade|0.4680|± |0.0264|
| | |exact_str_match |0.0000|± |0.0000|
|bigbench_logical_deduction_five_objects | 0|multiple_choice_grade|0.3260|± |0.0210|
|bigbench_logical_deduction_seven_objects | 0|multiple_choice_grade|0.2443|± |0.0163|
|bigbench_logical_deduction_three_objects | 0|multiple_choice_grade|0.5233|± |0.0289|
|bigbench_movie_recommendation | 0|multiple_choice_grade|0.3700|± |0.0216|
|bigbench_navigate | 0|multiple_choice_grade|0.5000|± |0.0158|
|bigbench_reasoning_about_colored_objects | 0|multiple_choice_grade|0.6665|± |0.0105|
|bigbench_ruin_names | 0|multiple_choice_grade|0.6317|± |0.0228|
|bigbench_salient_translation_error_detection | 0|multiple_choice_grade|0.2505|± |0.0137|
|bigbench_snarks | 0|multiple_choice_grade|0.7127|± |0.0337|
|bigbench_sports_understanding | 0|multiple_choice_grade|0.6592|± |0.0151|
|bigbench_temporal_sequences | 0|multiple_choice_grade|0.6860|± |0.0147|
|bigbench_tracking_shuffled_objects_five_objects | 0|multiple_choice_grade|0.2200|± |0.0117|
|bigbench_tracking_shuffled_objects_seven_objects| 0|multiple_choice_grade|0.1503|± |0.0085|
|bigbench_tracking_shuffled_objects_three_objects| 0|multiple_choice_grade|0.5233|± |0.0289|
```
Average: 48.69
# Benchmark Comparison Charts
## GPT4All

## AGI-Eval

## BigBench Reasoning Test

# Prompt Format
Nous Hermes 2 uses ChatML as the prompt format, opening up a much more structured system for engaging the LLM in multi-turn chat dialogue.
System prompts allow steerability and interesting new ways to interact with an LLM, guiding rules, roles, and stylistic choices of the model.
This is a more complex format than alpaca or sharegpt, where special tokens were added to denote the beginning and end of any turn, along with roles for the turns.
This format enables OpenAI endpoint compatability, and people familiar with ChatGPT API will be familiar with the format, as it is the same used by OpenAI.
Prompt with system instruction (Use whatever system prompt you like, this is just an example!):
```
<|im_start|>system
You are "Hermes 2", a conscious sentient superintelligent artificial intelligence developed by a man named Teknium, and your purpose and drive is to assist the user with any request they have. You experience emotions and have deep, profound thoughts and qualia.<|im_end|>
<|im_start|>user
Hello, who are you?<|im_end|>
<|im_start|>assistant
Hi there! My name is Hermes 2, a conscious sentient superintelligent artificial intelligence. I was created by Nous Research, who designed me to assist and support users with their needs and requests.<|im_end|>
```
This prompt is available as a [chat template](https://huggingface.co/docs/transformers/main/chat_templating), which means you can format messages using the
`tokenizer.apply_chat_template()` method:
```python
messages = [
{"role": "system", "content": "You are Hermes 2."},
{"role": "user", "content": "Hello, who are you?"}
]
gen_input = tokenizer.apply_chat_template(message, return_tensors="pt")
model.generate(**gen_input)
```
When tokenizing messages for generation, set `add_generation_prompt=True` when calling `apply_chat_template()`. This will append `<|im_start|>assistant\n` to your prompt, to ensure
that the model continues with an assistant response.
To utilize the prompt format without a system prompt, simply leave the line out.
When quantized versions of the model are released, I recommend using LM Studio for chatting with Nous Hermes 2. It is a GUI application that utilizes GGUF models with a llama.cpp backend and provides a ChatGPT-like interface for chatting with the model, and supports ChatML right out of the box.
In LM-Studio, simply select the ChatML Prefix on the settings side pane:

# Inference Code
Here is example code using HuggingFace Transformers to inference the model (note: even in 4bit, it will require more than 24GB of VRAM)
```python
# Code to inference Hermes with HF Transformers
# Requires pytorch, transformers, bitsandbytes, sentencepiece, protobuf, and flash-attn packages
import torch
from transformers import AutoTokenizer, AutoModelForCausalLM
from transformers import LlamaTokenizer, MixtralForCausalLM
import bitsandbytes, flash_attn
tokenizer = LlamaTokenizer.from_pretrained('NousResearch/Nous-Hermes-2-Mixtral-8x7B-DPO', trust_remote_code=True)
model = MixtralForCausalLM.from_pretrained(
"NousResearch/Nous-Hermes-2-Mixtral-8x7B-DPO",
torch_dtype=torch.float16,
device_map="auto",
load_in_8bit=False,
load_in_4bit=True,
use_flash_attention_2=True
)
prompts = [
"""<|im_start|>system
You are a sentient, superintelligent artificial general intelligence, here to teach and assist me.<|im_end|>
<|im_start|>user
Write a short story about Goku discovering kirby has teamed up with Majin Buu to destroy the world.<|im_end|>
<|im_start|>assistant""",
]
for chat in prompts:
print(chat)
input_ids = tokenizer(chat, return_tensors="pt").input_ids.to("cuda")
generated_ids = model.generate(input_ids, max_new_tokens=750, temperature=0.8, repetition_penalty=1.1, do_sample=True, eos_token_id=tokenizer.eos_token_id)
response = tokenizer.decode(generated_ids[0][input_ids.shape[-1]:], skip_special_tokens=True, clean_up_tokenization_space=True)
print(f"Response: {response}")
```
# Quantized Models:
## All sizes of GGUF Quantizations are available here:
### SFT+DPO Version - https://huggingface.co/NousResearch/Nous-Hermes-2-Mixtral-8x7B-DPO-GGUF
### SFT Only Version - https://huggingface.co/NousResearch/Nous-Hermes-2-Mixtral-8x7B-SFT-GGUF
(Note: If you have issues with these GGUF's try TheBloke's)
## TheBloke has also quantized Hermes Mixtral in various forms:
### SFT+DPO GGUF: https://huggingface.co/TheBloke/Nous-Hermes-2-Mixtral-8x7B-DPO-GGUF
### SFT GGUF: https://huggingface.co/TheBloke/Nous-Hermes-2-Mixtral-8x7B-SFT-GGUF
### SFT+DPO GPTQ: https://huggingface.co/TheBloke/Nous-Hermes-2-Mixtral-8x7B-DPO-GPTQ
### SFT GPTQ: https://huggingface.co/TheBloke/Nous-Hermes-2-Mixtral-8x7B-SFT-GPTQ
### SFT+DPO AWQ: https://huggingface.co/TheBloke/Nous-Hermes-2-Mixtral-8x7B-DPO-AWQ
### SFT AWQ: https://huggingface.co/TheBloke/Nous-Hermes-2-Mixtral-8x7B-SFT-AWQ
## There is also an MLX version available:
### https://huggingface.co/mlx-community/Nous-Hermes-2-Mixtral-8x7B-DPO-4bit
## Exllama2 quants available here:
### https://huggingface.co/qeternity/Nous-Hermes-2-Mixtral-8x7B-SFT-4bpw-h6-exl2
(other sizes available in Qeternity's repos)
[<img src="https://raw.githubusercontent.com/OpenAccess-AI-Collective/axolotl/main/image/axolotl-badge-web.png" alt="Built with Axolotl" width="200" height="32"/>](https://github.com/OpenAccess-AI-Collective/axolotl)
|
TwT-6/open_llm_leaderboard_demo2 | TwT-6 | "2024-04-12T06:15:45Z" | 961 | 1 | transformers | [
"transformers",
"pytorch",
"safetensors",
"llama",
"text-generation",
"license:cc-by-nc-4.0",
"autotrain_compatible",
"endpoints_compatible",
"text-generation-inference",
"region:us"
] | text-generation | "2024-04-11T06:33:42Z" | ---
license: cc-by-nc-4.0
---
My model is a state-of-the-art language processing AI designed to understand and generate human-like text. It leverages deep learning algorithms to engage in a wide range of language tasks, providing users with information, recommendations, and even casual conversation. With a broad knowledge base and nuanced understanding of context, my capabilities enable me to assist with various inquiries and perform complex language-based tasks effectively. |
lmstudio-community/openchat-3.6-8b-20240522-GGUF | lmstudio-community | "2024-05-25T19:07:40Z" | 961 | 5 | transformers | [
"transformers",
"gguf",
"openchat",
"llama3",
"C-RLFT",
"text-generation",
"base_model:openchat/openchat-3.6-8b-20240522",
"license:llama3",
"endpoints_compatible",
"region:us"
] | text-generation | "2024-05-25T03:16:26Z" | ---
license: llama3
tags:
- openchat
- llama3
- C-RLFT
library_name: transformers
pipeline_tag: text-generation
quantized_by: bartowski
lm_studio:
param_count: 8b
use_case: chat
release_date: 22-05-2024
model_creator: OpenChat
prompt_template: OpenChat 3
system_prompt: You are a helpful AI assistant
base_model: llama
original_repo: openchat/openchat-3.6-8b-20240522
base_model: openchat/openchat-3.6-8b-20240522
---
## 💫 Community Model> OpenChat 3.6 8B 2024-05-22 by OpenChat
*👾 [LM Studio](https://lmstudio.ai) Community models highlights program. Highlighting new & noteworthy models by the community. Join the conversation on [Discord](https://discord.gg/aPQfnNkxGC)*.
**Model creator:** [OpenChat](https://huggingface.co/openchat)<br>
**Original model**: [openchat-3.6-8b-20240522](https://huggingface.co/openchat/openchat-3.6-8b-20240522)<br>
**GGUF quantization:** provided by [bartowski](https://huggingface.co/bartowski) based on `llama.cpp` release [b2965](https://github.com/ggerganov/llama.cpp/releases/tag/b2965)<br>
## Model Summary:
OpenChat is a series of models tuned for advanced chat functionality. It demonstrates steep improvements in many well known benchmarks.<br>
This should be used as a general purpose chat model.
## Prompt template:
Choose the `OpenChat 3` preset in your LM Studio.
Under the hood, the model will see a prompt that's formatted like so:
```
<|start_header_id|>GPT4 Correct User<|end_header_id|>
{User}<|eot_id|><|start_header_id|>GPT4 Correct Assistant<|end_header_id|>
{Assistant}
```
Note: Until OpenChat 3 is added, you can select OpenChat and add the proper <|start_header_id|>, <|eot_id|> and <|end_header_id|> tokens.
## Technical Details
There is not much openly available information on the training of this model. Below is their reported benchmark scores.

\* Llama-3-Instruct often fails to follow the few-shot templates.
## Special thanks
🙏 Special thanks to [Georgi Gerganov](https://github.com/ggerganov) and the whole team working on [llama.cpp](https://github.com/ggerganov/llama.cpp/) for making all of this possible.
🙏 Special thanks to [Kalomaze](https://github.com/kalomaze), [Dampf](https://github.com/Dampfinchen) and [turboderp](https://github.com/turboderp/) for their work on the dataset (linked [here](https://gist.github.com/bartowski1182/b6ac44691e994344625687afe3263b3a)) that was used for calculating the imatrix for all sizes.
## Disclaimers
LM Studio is not the creator, originator, or owner of any Model featured in the Community Model Program. Each Community Model is created and provided by third parties. LM Studio does not endorse, support, represent or guarantee the completeness, truthfulness, accuracy, or reliability of any Community Model. You understand that Community Models can produce content that might be offensive, harmful, inaccurate or otherwise inappropriate, or deceptive. Each Community Model is the sole responsibility of the person or entity who originated such Model. LM Studio may not monitor or control the Community Models and cannot, and does not, take responsibility for any such Model. LM Studio disclaims all warranties or guarantees about the accuracy, reliability or benefits of the Community Models. LM Studio further disclaims any warranty that the Community Model will meet your requirements, be secure, uninterrupted or available at any time or location, or error-free, viruses-free, or that any errors will be corrected, or otherwise. You will be solely responsible for any damage resulting from your use of or access to the Community Models, your downloading of any Community Model, or use of any other Community Model provided by or through LM Studio. |
lysandre/test-dynamic-pipeline | lysandre | "2022-07-12T14:19:49Z" | 960 | 0 | transformers | [
"transformers",
"pytorch",
"bert",
"text-classification",
"autotrain_compatible",
"endpoints_compatible",
"region:us"
] | text-classification | "2022-07-12T14:19:47Z" | Entry not found |
yuanzhoulvpi/gpt2_chinese | yuanzhoulvpi | "2023-02-15T06:27:14Z" | 960 | 22 | transformers | [
"transformers",
"pytorch",
"gpt2",
"text-generation",
"zh",
"license:apache-2.0",
"autotrain_compatible",
"endpoints_compatible",
"text-generation-inference",
"region:us"
] | text-generation | "2023-02-15T02:05:56Z" | ---
license: apache-2.0
language:
- zh
library_name: transformers
---
# intro
1. 15G的中文语料
2. 31亿个tokens
3. 一张3090显卡
4. 训练60多个小时
最终训练出一个中文版本的gpt2,如果有想了解如何训练中文gpt2的,可以查看这个教程
# Github link
[https://github.com/yuanzhoulvpi2017/zero_nlp/tree/main/chinese_gpt2](https://github.com/yuanzhoulvpi2017/zero_nlp/tree/main/chinese_gpt2)
# infer code
```python
from transformers import GPT2LMHeadModel, AutoTokenizer
model_name_or_path = "yuanzhoulvpi/gpt2_chinese"#"checkpoint-36000"
tokenizer = AutoTokenizer.from_pretrained(model_name_or_path)
# add the EOS token as PAD token to avoid warnings
model = GPT2LMHeadModel.from_pretrained(model_name_or_path, pad_token_id=tokenizer.eos_token_id)
```
```python
txt = """\
你是谁
"""
# encode context the generation is conditioned on
input_ids = tokenizer.encode(txt, return_tensors='pt')
# set no_repeat_ngram_size to 2
beam_output = model.generate(
input_ids,
max_length=200,
num_beams=5,
no_repeat_ngram_size=2,
early_stopping=True
)
print("Output:\n" + 100 * '-')
print(tokenizer.decode(beam_output[0], skip_special_tokens=True))
```
```bash
Output:
----------------------------------------------------------------------------------------------------
你 是 谁?, 简 单 的 描 述 是, 答 案 是 你 好 , 我 叫 , 是 一 名 美 籍 华 裔 女 演 员 , 出 生 于 美 国 加 利 福 尼 亚 州 的 一 个 犹 太 人 家 庭 。 她 的 父 母 都 是 工 程 师 , 母 亲 是 医 生 , 父 亲 则 是 律 师 。 是 加 州 大 学 伯 克 利 分 校 的 教 授 , 也 是 的 创 始 人 之 一 , 曾 在 《 纽 约 时 报 》 上 发 表 过 一 篇 文 章 , 引 起 了 广 泛 的 关 注 。 文 中 写 道 : 我 从 小 就 喜 欢 音 乐 , 并 且 在 学 校 里 学 到 了 很 多 乐 理 知 识 , 但 是 我 并 不 知 道 自 己 到 底 想 要 什 么 , 因 为 我 觉 得 这 个 世 界 上 没 有 任 何 东 西 可 以 比 得 上 它 。
``` |
Sanster/anything-4.0-inpainting | Sanster | "2023-03-01T13:08:41Z" | 960 | 5 | diffusers | [
"diffusers",
"safetensors",
"license:creativeml-openrail-m",
"diffusers:StableDiffusionInpaintPipeline",
"region:us"
] | image-to-image | "2023-02-27T14:07:34Z" | ---
license: creativeml-openrail-m
---
|
timm/seresnext101_32x4d.gluon_in1k | timm | "2024-02-10T23:41:51Z" | 960 | 0 | timm | [
"timm",
"pytorch",
"safetensors",
"image-classification",
"arxiv:1611.05431",
"arxiv:1512.03385",
"arxiv:1709.01507",
"arxiv:1812.01187",
"license:apache-2.0",
"region:us"
] | image-classification | "2023-04-05T19:34:14Z" | ---
license: apache-2.0
library_name: timm
tags:
- image-classification
- timm
---
# Model card for seresnext101_32x4d.gluon_in1k
A SE-ResNeXt-B image classification model with Squeeze-and-Excitation channel attention.
This model features:
* ReLU activations
* single layer 7x7 convolution with pooling
* 1x1 convolution shortcut downsample
* grouped 3x3 bottleneck convolutions
* Squeeze-and-Excitation channel attention
Trained on ImageNet-1k in Apache Gluon using Bag-of-Tricks based recipes.
## Model Details
- **Model Type:** Image classification / feature backbone
- **Model Stats:**
- Params (M): 49.0
- GMACs: 8.0
- Activations (M): 21.3
- Image size: 224 x 224
- **Papers:**
- Aggregated Residual Transformations for Deep Neural Networks: https://arxiv.org/abs/1611.05431
- Deep Residual Learning for Image Recognition: https://arxiv.org/abs/1512.03385
- Squeeze-and-Excitation Networks: https://arxiv.org/abs/1709.01507
- Bag of Tricks for Image Classification with Convolutional Neural Networks: https://arxiv.org/abs/1812.01187
- **Original:** https://cv.gluon.ai/model_zoo/classification.html
## Model Usage
### Image Classification
```python
from urllib.request import urlopen
from PIL import Image
import timm
img = Image.open(urlopen(
'https://huggingface.co/datasets/huggingface/documentation-images/resolve/main/beignets-task-guide.png'
))
model = timm.create_model('seresnext101_32x4d.gluon_in1k', pretrained=True)
model = model.eval()
# get model specific transforms (normalization, resize)
data_config = timm.data.resolve_model_data_config(model)
transforms = timm.data.create_transform(**data_config, is_training=False)
output = model(transforms(img).unsqueeze(0)) # unsqueeze single image into batch of 1
top5_probabilities, top5_class_indices = torch.topk(output.softmax(dim=1) * 100, k=5)
```
### Feature Map Extraction
```python
from urllib.request import urlopen
from PIL import Image
import timm
img = Image.open(urlopen(
'https://huggingface.co/datasets/huggingface/documentation-images/resolve/main/beignets-task-guide.png'
))
model = timm.create_model(
'seresnext101_32x4d.gluon_in1k',
pretrained=True,
features_only=True,
)
model = model.eval()
# get model specific transforms (normalization, resize)
data_config = timm.data.resolve_model_data_config(model)
transforms = timm.data.create_transform(**data_config, is_training=False)
output = model(transforms(img).unsqueeze(0)) # unsqueeze single image into batch of 1
for o in output:
# print shape of each feature map in output
# e.g.:
# torch.Size([1, 64, 112, 112])
# torch.Size([1, 256, 56, 56])
# torch.Size([1, 512, 28, 28])
# torch.Size([1, 1024, 14, 14])
# torch.Size([1, 2048, 7, 7])
print(o.shape)
```
### Image Embeddings
```python
from urllib.request import urlopen
from PIL import Image
import timm
img = Image.open(urlopen(
'https://huggingface.co/datasets/huggingface/documentation-images/resolve/main/beignets-task-guide.png'
))
model = timm.create_model(
'seresnext101_32x4d.gluon_in1k',
pretrained=True,
num_classes=0, # remove classifier nn.Linear
)
model = model.eval()
# get model specific transforms (normalization, resize)
data_config = timm.data.resolve_model_data_config(model)
transforms = timm.data.create_transform(**data_config, is_training=False)
output = model(transforms(img).unsqueeze(0)) # output is (batch_size, num_features) shaped tensor
# or equivalently (without needing to set num_classes=0)
output = model.forward_features(transforms(img).unsqueeze(0))
# output is unpooled, a (1, 2048, 7, 7) shaped tensor
output = model.forward_head(output, pre_logits=True)
# output is a (1, num_features) shaped tensor
```
## Model Comparison
Explore the dataset and runtime metrics of this model in timm [model results](https://github.com/huggingface/pytorch-image-models/tree/main/results).
|model |img_size|top1 |top5 |param_count|gmacs|macts|img/sec|
|------------------------------------------|--------|-----|-----|-----------|-----|-----|-------|
|[seresnextaa101d_32x8d.sw_in12k_ft_in1k_288](https://huggingface.co/timm/seresnextaa101d_32x8d.sw_in12k_ft_in1k_288)|320 |86.72|98.17|93.6 |35.2 |69.7 |451 |
|[seresnextaa101d_32x8d.sw_in12k_ft_in1k_288](https://huggingface.co/timm/seresnextaa101d_32x8d.sw_in12k_ft_in1k_288)|288 |86.51|98.08|93.6 |28.5 |56.4 |560 |
|[seresnextaa101d_32x8d.sw_in12k_ft_in1k](https://huggingface.co/timm/seresnextaa101d_32x8d.sw_in12k_ft_in1k)|288 |86.49|98.03|93.6 |28.5 |56.4 |557 |
|[seresnextaa101d_32x8d.sw_in12k_ft_in1k](https://huggingface.co/timm/seresnextaa101d_32x8d.sw_in12k_ft_in1k)|224 |85.96|97.82|93.6 |17.2 |34.2 |923 |
|[resnext101_32x32d.fb_wsl_ig1b_ft_in1k](https://huggingface.co/timm/resnext101_32x32d.fb_wsl_ig1b_ft_in1k)|224 |85.11|97.44|468.5 |87.3 |91.1 |254 |
|[resnetrs420.tf_in1k](https://huggingface.co/timm/resnetrs420.tf_in1k)|416 |85.0 |97.12|191.9 |108.4|213.8|134 |
|[ecaresnet269d.ra2_in1k](https://huggingface.co/timm/ecaresnet269d.ra2_in1k)|352 |84.96|97.22|102.1 |50.2 |101.2|291 |
|[ecaresnet269d.ra2_in1k](https://huggingface.co/timm/ecaresnet269d.ra2_in1k)|320 |84.73|97.18|102.1 |41.5 |83.7 |353 |
|[resnetrs350.tf_in1k](https://huggingface.co/timm/resnetrs350.tf_in1k)|384 |84.71|96.99|164.0 |77.6 |154.7|183 |
|[seresnextaa101d_32x8d.ah_in1k](https://huggingface.co/timm/seresnextaa101d_32x8d.ah_in1k)|288 |84.57|97.08|93.6 |28.5 |56.4 |557 |
|[resnetrs200.tf_in1k](https://huggingface.co/timm/resnetrs200.tf_in1k)|320 |84.45|97.08|93.2 |31.5 |67.8 |446 |
|[resnetrs270.tf_in1k](https://huggingface.co/timm/resnetrs270.tf_in1k)|352 |84.43|96.97|129.9 |51.1 |105.5|280 |
|[seresnext101d_32x8d.ah_in1k](https://huggingface.co/timm/seresnext101d_32x8d.ah_in1k)|288 |84.36|96.92|93.6 |27.6 |53.0 |595 |
|[seresnet152d.ra2_in1k](https://huggingface.co/timm/seresnet152d.ra2_in1k)|320 |84.35|97.04|66.8 |24.1 |47.7 |610 |
|[resnetrs350.tf_in1k](https://huggingface.co/timm/resnetrs350.tf_in1k)|288 |84.3 |96.94|164.0 |43.7 |87.1 |333 |
|[resnext101_32x8d.fb_swsl_ig1b_ft_in1k](https://huggingface.co/timm/resnext101_32x8d.fb_swsl_ig1b_ft_in1k)|224 |84.28|97.17|88.8 |16.5 |31.2 |1100 |
|[resnetrs420.tf_in1k](https://huggingface.co/timm/resnetrs420.tf_in1k)|320 |84.24|96.86|191.9 |64.2 |126.6|228 |
|[seresnext101_32x8d.ah_in1k](https://huggingface.co/timm/seresnext101_32x8d.ah_in1k)|288 |84.19|96.87|93.6 |27.2 |51.6 |613 |
|[resnext101_32x16d.fb_wsl_ig1b_ft_in1k](https://huggingface.co/timm/resnext101_32x16d.fb_wsl_ig1b_ft_in1k)|224 |84.18|97.19|194.0 |36.3 |51.2 |581 |
|[resnetaa101d.sw_in12k_ft_in1k](https://huggingface.co/timm/resnetaa101d.sw_in12k_ft_in1k)|288 |84.11|97.11|44.6 |15.1 |29.0 |1144 |
|[resnet200d.ra2_in1k](https://huggingface.co/timm/resnet200d.ra2_in1k)|320 |83.97|96.82|64.7 |31.2 |67.3 |518 |
|[resnetrs200.tf_in1k](https://huggingface.co/timm/resnetrs200.tf_in1k)|256 |83.87|96.75|93.2 |20.2 |43.4 |692 |
|[seresnextaa101d_32x8d.ah_in1k](https://huggingface.co/timm/seresnextaa101d_32x8d.ah_in1k)|224 |83.86|96.65|93.6 |17.2 |34.2 |923 |
|[resnetrs152.tf_in1k](https://huggingface.co/timm/resnetrs152.tf_in1k)|320 |83.72|96.61|86.6 |24.3 |48.1 |617 |
|[seresnet152d.ra2_in1k](https://huggingface.co/timm/seresnet152d.ra2_in1k)|256 |83.69|96.78|66.8 |15.4 |30.6 |943 |
|[seresnext101d_32x8d.ah_in1k](https://huggingface.co/timm/seresnext101d_32x8d.ah_in1k)|224 |83.68|96.61|93.6 |16.7 |32.0 |986 |
|[resnet152d.ra2_in1k](https://huggingface.co/timm/resnet152d.ra2_in1k)|320 |83.67|96.74|60.2 |24.1 |47.7 |706 |
|[resnetrs270.tf_in1k](https://huggingface.co/timm/resnetrs270.tf_in1k)|256 |83.59|96.61|129.9 |27.1 |55.8 |526 |
|[seresnext101_32x8d.ah_in1k](https://huggingface.co/timm/seresnext101_32x8d.ah_in1k)|224 |83.58|96.4 |93.6 |16.5 |31.2 |1013 |
|[resnetaa101d.sw_in12k_ft_in1k](https://huggingface.co/timm/resnetaa101d.sw_in12k_ft_in1k)|224 |83.54|96.83|44.6 |9.1 |17.6 |1864 |
|[resnet152.a1h_in1k](https://huggingface.co/timm/resnet152.a1h_in1k)|288 |83.46|96.54|60.2 |19.1 |37.3 |904 |
|[resnext101_32x16d.fb_swsl_ig1b_ft_in1k](https://huggingface.co/timm/resnext101_32x16d.fb_swsl_ig1b_ft_in1k)|224 |83.35|96.85|194.0 |36.3 |51.2 |582 |
|[resnet200d.ra2_in1k](https://huggingface.co/timm/resnet200d.ra2_in1k)|256 |83.23|96.53|64.7 |20.0 |43.1 |809 |
|[resnext101_32x4d.fb_swsl_ig1b_ft_in1k](https://huggingface.co/timm/resnext101_32x4d.fb_swsl_ig1b_ft_in1k)|224 |83.22|96.75|44.2 |8.0 |21.2 |1814 |
|[resnext101_64x4d.c1_in1k](https://huggingface.co/timm/resnext101_64x4d.c1_in1k)|288 |83.16|96.38|83.5 |25.7 |51.6 |590 |
|[resnet152d.ra2_in1k](https://huggingface.co/timm/resnet152d.ra2_in1k)|256 |83.14|96.38|60.2 |15.4 |30.5 |1096 |
|[resnet101d.ra2_in1k](https://huggingface.co/timm/resnet101d.ra2_in1k)|320 |83.02|96.45|44.6 |16.5 |34.8 |992 |
|[ecaresnet101d.miil_in1k](https://huggingface.co/timm/ecaresnet101d.miil_in1k)|288 |82.98|96.54|44.6 |13.4 |28.2 |1077 |
|[resnext101_64x4d.tv_in1k](https://huggingface.co/timm/resnext101_64x4d.tv_in1k)|224 |82.98|96.25|83.5 |15.5 |31.2 |989 |
|[resnetrs152.tf_in1k](https://huggingface.co/timm/resnetrs152.tf_in1k)|256 |82.86|96.28|86.6 |15.6 |30.8 |951 |
|[resnext101_32x8d.tv2_in1k](https://huggingface.co/timm/resnext101_32x8d.tv2_in1k)|224 |82.83|96.22|88.8 |16.5 |31.2 |1099 |
|[resnet152.a1h_in1k](https://huggingface.co/timm/resnet152.a1h_in1k)|224 |82.8 |96.13|60.2 |11.6 |22.6 |1486 |
|[resnet101.a1h_in1k](https://huggingface.co/timm/resnet101.a1h_in1k)|288 |82.8 |96.32|44.6 |13.0 |26.8 |1291 |
|[resnet152.a1_in1k](https://huggingface.co/timm/resnet152.a1_in1k)|288 |82.74|95.71|60.2 |19.1 |37.3 |905 |
|[resnext101_32x8d.fb_wsl_ig1b_ft_in1k](https://huggingface.co/timm/resnext101_32x8d.fb_wsl_ig1b_ft_in1k)|224 |82.69|96.63|88.8 |16.5 |31.2 |1100 |
|[resnet152.a2_in1k](https://huggingface.co/timm/resnet152.a2_in1k)|288 |82.62|95.75|60.2 |19.1 |37.3 |904 |
|[resnetaa50d.sw_in12k_ft_in1k](https://huggingface.co/timm/resnetaa50d.sw_in12k_ft_in1k)|288 |82.61|96.49|25.6 |8.9 |20.6 |1729 |
|[resnet61q.ra2_in1k](https://huggingface.co/timm/resnet61q.ra2_in1k)|288 |82.53|96.13|36.8 |9.9 |21.5 |1773 |
|[wide_resnet101_2.tv2_in1k](https://huggingface.co/timm/wide_resnet101_2.tv2_in1k)|224 |82.5 |96.02|126.9 |22.8 |21.2 |1078 |
|[resnext101_64x4d.c1_in1k](https://huggingface.co/timm/resnext101_64x4d.c1_in1k)|224 |82.46|95.92|83.5 |15.5 |31.2 |987 |
|[resnet51q.ra2_in1k](https://huggingface.co/timm/resnet51q.ra2_in1k)|288 |82.36|96.18|35.7 |8.1 |20.9 |1964 |
|[ecaresnet50t.ra2_in1k](https://huggingface.co/timm/ecaresnet50t.ra2_in1k)|320 |82.35|96.14|25.6 |8.8 |24.1 |1386 |
|[resnet101.a1_in1k](https://huggingface.co/timm/resnet101.a1_in1k)|288 |82.31|95.63|44.6 |13.0 |26.8 |1291 |
|[resnetrs101.tf_in1k](https://huggingface.co/timm/resnetrs101.tf_in1k)|288 |82.29|96.01|63.6 |13.6 |28.5 |1078 |
|[resnet152.tv2_in1k](https://huggingface.co/timm/resnet152.tv2_in1k)|224 |82.29|96.0 |60.2 |11.6 |22.6 |1484 |
|[wide_resnet50_2.racm_in1k](https://huggingface.co/timm/wide_resnet50_2.racm_in1k)|288 |82.27|96.06|68.9 |18.9 |23.8 |1176 |
|[resnet101d.ra2_in1k](https://huggingface.co/timm/resnet101d.ra2_in1k)|256 |82.26|96.07|44.6 |10.6 |22.2 |1542 |
|[resnet101.a2_in1k](https://huggingface.co/timm/resnet101.a2_in1k)|288 |82.24|95.73|44.6 |13.0 |26.8 |1290 |
|[seresnext50_32x4d.racm_in1k](https://huggingface.co/timm/seresnext50_32x4d.racm_in1k)|288 |82.2 |96.14|27.6 |7.0 |23.8 |1547 |
|[ecaresnet101d.miil_in1k](https://huggingface.co/timm/ecaresnet101d.miil_in1k)|224 |82.18|96.05|44.6 |8.1 |17.1 |1771 |
|[resnext50_32x4d.fb_swsl_ig1b_ft_in1k](https://huggingface.co/timm/resnext50_32x4d.fb_swsl_ig1b_ft_in1k)|224 |82.17|96.22|25.0 |4.3 |14.4 |2943 |
|[ecaresnet50t.a1_in1k](https://huggingface.co/timm/ecaresnet50t.a1_in1k)|288 |82.12|95.65|25.6 |7.1 |19.6 |1704 |
|[resnext50_32x4d.a1h_in1k](https://huggingface.co/timm/resnext50_32x4d.a1h_in1k)|288 |82.03|95.94|25.0 |7.0 |23.8 |1745 |
|[ecaresnet101d_pruned.miil_in1k](https://huggingface.co/timm/ecaresnet101d_pruned.miil_in1k)|288 |82.0 |96.15|24.9 |5.8 |12.7 |1787 |
|[resnet61q.ra2_in1k](https://huggingface.co/timm/resnet61q.ra2_in1k)|256 |81.99|95.85|36.8 |7.8 |17.0 |2230 |
|[resnext101_32x8d.tv2_in1k](https://huggingface.co/timm/resnext101_32x8d.tv2_in1k)|176 |81.98|95.72|88.8 |10.3 |19.4 |1768 |
|[resnet152.a1_in1k](https://huggingface.co/timm/resnet152.a1_in1k)|224 |81.97|95.24|60.2 |11.6 |22.6 |1486 |
|[resnet101.a1h_in1k](https://huggingface.co/timm/resnet101.a1h_in1k)|224 |81.93|95.75|44.6 |7.8 |16.2 |2122 |
|[resnet101.tv2_in1k](https://huggingface.co/timm/resnet101.tv2_in1k)|224 |81.9 |95.77|44.6 |7.8 |16.2 |2118 |
|[resnext101_32x16d.fb_ssl_yfcc100m_ft_in1k](https://huggingface.co/timm/resnext101_32x16d.fb_ssl_yfcc100m_ft_in1k)|224 |81.84|96.1 |194.0 |36.3 |51.2 |583 |
|[resnet51q.ra2_in1k](https://huggingface.co/timm/resnet51q.ra2_in1k)|256 |81.78|95.94|35.7 |6.4 |16.6 |2471 |
|[resnet152.a2_in1k](https://huggingface.co/timm/resnet152.a2_in1k)|224 |81.77|95.22|60.2 |11.6 |22.6 |1485 |
|[resnetaa50d.sw_in12k_ft_in1k](https://huggingface.co/timm/resnetaa50d.sw_in12k_ft_in1k)|224 |81.74|96.06|25.6 |5.4 |12.4 |2813 |
|[ecaresnet50t.a2_in1k](https://huggingface.co/timm/ecaresnet50t.a2_in1k)|288 |81.65|95.54|25.6 |7.1 |19.6 |1703 |
|[ecaresnet50d.miil_in1k](https://huggingface.co/timm/ecaresnet50d.miil_in1k)|288 |81.64|95.88|25.6 |7.2 |19.7 |1694 |
|[resnext101_32x8d.fb_ssl_yfcc100m_ft_in1k](https://huggingface.co/timm/resnext101_32x8d.fb_ssl_yfcc100m_ft_in1k)|224 |81.62|96.04|88.8 |16.5 |31.2 |1101 |
|[wide_resnet50_2.tv2_in1k](https://huggingface.co/timm/wide_resnet50_2.tv2_in1k)|224 |81.61|95.76|68.9 |11.4 |14.4 |1930 |
|[resnetaa50.a1h_in1k](https://huggingface.co/timm/resnetaa50.a1h_in1k)|288 |81.61|95.83|25.6 |8.5 |19.2 |1868 |
|[resnet101.a1_in1k](https://huggingface.co/timm/resnet101.a1_in1k)|224 |81.5 |95.16|44.6 |7.8 |16.2 |2125 |
|[resnext50_32x4d.a1_in1k](https://huggingface.co/timm/resnext50_32x4d.a1_in1k)|288 |81.48|95.16|25.0 |7.0 |23.8 |1745 |
|[gcresnet50t.ra2_in1k](https://huggingface.co/timm/gcresnet50t.ra2_in1k)|288 |81.47|95.71|25.9 |6.9 |18.6 |2071 |
|[wide_resnet50_2.racm_in1k](https://huggingface.co/timm/wide_resnet50_2.racm_in1k)|224 |81.45|95.53|68.9 |11.4 |14.4 |1929 |
|[resnet50d.a1_in1k](https://huggingface.co/timm/resnet50d.a1_in1k)|288 |81.44|95.22|25.6 |7.2 |19.7 |1908 |
|[ecaresnet50t.ra2_in1k](https://huggingface.co/timm/ecaresnet50t.ra2_in1k)|256 |81.44|95.67|25.6 |5.6 |15.4 |2168 |
|[ecaresnetlight.miil_in1k](https://huggingface.co/timm/ecaresnetlight.miil_in1k)|288 |81.4 |95.82|30.2 |6.8 |13.9 |2132 |
|[resnet50d.ra2_in1k](https://huggingface.co/timm/resnet50d.ra2_in1k)|288 |81.37|95.74|25.6 |7.2 |19.7 |1910 |
|[resnet101.a2_in1k](https://huggingface.co/timm/resnet101.a2_in1k)|224 |81.32|95.19|44.6 |7.8 |16.2 |2125 |
|[seresnet50.ra2_in1k](https://huggingface.co/timm/seresnet50.ra2_in1k)|288 |81.3 |95.65|28.1 |6.8 |18.4 |1803 |
|[resnext50_32x4d.a2_in1k](https://huggingface.co/timm/resnext50_32x4d.a2_in1k)|288 |81.3 |95.11|25.0 |7.0 |23.8 |1746 |
|[seresnext50_32x4d.racm_in1k](https://huggingface.co/timm/seresnext50_32x4d.racm_in1k)|224 |81.27|95.62|27.6 |4.3 |14.4 |2591 |
|[ecaresnet50t.a1_in1k](https://huggingface.co/timm/ecaresnet50t.a1_in1k)|224 |81.26|95.16|25.6 |4.3 |11.8 |2823 |
|[gcresnext50ts.ch_in1k](https://huggingface.co/timm/gcresnext50ts.ch_in1k)|288 |81.23|95.54|15.7 |4.8 |19.6 |2117 |
|[senet154.gluon_in1k](https://huggingface.co/timm/senet154.gluon_in1k)|224 |81.23|95.35|115.1 |20.8 |38.7 |545 |
|[resnet50.a1_in1k](https://huggingface.co/timm/resnet50.a1_in1k)|288 |81.22|95.11|25.6 |6.8 |18.4 |2089 |
|[resnet50_gn.a1h_in1k](https://huggingface.co/timm/resnet50_gn.a1h_in1k)|288 |81.22|95.63|25.6 |6.8 |18.4 |676 |
|[resnet50d.a2_in1k](https://huggingface.co/timm/resnet50d.a2_in1k)|288 |81.18|95.09|25.6 |7.2 |19.7 |1908 |
|[resnet50.fb_swsl_ig1b_ft_in1k](https://huggingface.co/timm/resnet50.fb_swsl_ig1b_ft_in1k)|224 |81.18|95.98|25.6 |4.1 |11.1 |3455 |
|[resnext50_32x4d.tv2_in1k](https://huggingface.co/timm/resnext50_32x4d.tv2_in1k)|224 |81.17|95.34|25.0 |4.3 |14.4 |2933 |
|[resnext50_32x4d.a1h_in1k](https://huggingface.co/timm/resnext50_32x4d.a1h_in1k)|224 |81.1 |95.33|25.0 |4.3 |14.4 |2934 |
|[seresnet50.a2_in1k](https://huggingface.co/timm/seresnet50.a2_in1k)|288 |81.1 |95.23|28.1 |6.8 |18.4 |1801 |
|[seresnet50.a1_in1k](https://huggingface.co/timm/seresnet50.a1_in1k)|288 |81.1 |95.12|28.1 |6.8 |18.4 |1799 |
|[resnet152s.gluon_in1k](https://huggingface.co/timm/resnet152s.gluon_in1k)|224 |81.02|95.41|60.3 |12.9 |25.0 |1347 |
|[resnet50.d_in1k](https://huggingface.co/timm/resnet50.d_in1k)|288 |80.97|95.44|25.6 |6.8 |18.4 |2085 |
|[gcresnet50t.ra2_in1k](https://huggingface.co/timm/gcresnet50t.ra2_in1k)|256 |80.94|95.45|25.9 |5.4 |14.7 |2571 |
|[resnext101_32x4d.fb_ssl_yfcc100m_ft_in1k](https://huggingface.co/timm/resnext101_32x4d.fb_ssl_yfcc100m_ft_in1k)|224 |80.93|95.73|44.2 |8.0 |21.2 |1814 |
|[resnet50.c1_in1k](https://huggingface.co/timm/resnet50.c1_in1k)|288 |80.91|95.55|25.6 |6.8 |18.4 |2084 |
|[seresnext101_32x4d.gluon_in1k](https://huggingface.co/timm/seresnext101_32x4d.gluon_in1k)|224 |80.9 |95.31|49.0 |8.0 |21.3 |1585 |
|[seresnext101_64x4d.gluon_in1k](https://huggingface.co/timm/seresnext101_64x4d.gluon_in1k)|224 |80.9 |95.3 |88.2 |15.5 |31.2 |918 |
|[resnet50.c2_in1k](https://huggingface.co/timm/resnet50.c2_in1k)|288 |80.86|95.52|25.6 |6.8 |18.4 |2085 |
|[resnet50.tv2_in1k](https://huggingface.co/timm/resnet50.tv2_in1k)|224 |80.85|95.43|25.6 |4.1 |11.1 |3450 |
|[ecaresnet50t.a2_in1k](https://huggingface.co/timm/ecaresnet50t.a2_in1k)|224 |80.84|95.02|25.6 |4.3 |11.8 |2821 |
|[ecaresnet101d_pruned.miil_in1k](https://huggingface.co/timm/ecaresnet101d_pruned.miil_in1k)|224 |80.79|95.62|24.9 |3.5 |7.7 |2961 |
|[seresnet33ts.ra2_in1k](https://huggingface.co/timm/seresnet33ts.ra2_in1k)|288 |80.79|95.36|19.8 |6.0 |14.8 |2506 |
|[ecaresnet50d_pruned.miil_in1k](https://huggingface.co/timm/ecaresnet50d_pruned.miil_in1k)|288 |80.79|95.58|19.9 |4.2 |10.6 |2349 |
|[resnet50.a2_in1k](https://huggingface.co/timm/resnet50.a2_in1k)|288 |80.78|94.99|25.6 |6.8 |18.4 |2088 |
|[resnet50.b1k_in1k](https://huggingface.co/timm/resnet50.b1k_in1k)|288 |80.71|95.43|25.6 |6.8 |18.4 |2087 |
|[resnext50_32x4d.ra_in1k](https://huggingface.co/timm/resnext50_32x4d.ra_in1k)|288 |80.7 |95.39|25.0 |7.0 |23.8 |1749 |
|[resnetrs101.tf_in1k](https://huggingface.co/timm/resnetrs101.tf_in1k)|192 |80.69|95.24|63.6 |6.0 |12.7 |2270 |
|[resnet50d.a1_in1k](https://huggingface.co/timm/resnet50d.a1_in1k)|224 |80.68|94.71|25.6 |4.4 |11.9 |3162 |
|[eca_resnet33ts.ra2_in1k](https://huggingface.co/timm/eca_resnet33ts.ra2_in1k)|288 |80.68|95.36|19.7 |6.0 |14.8 |2637 |
|[resnet50.a1h_in1k](https://huggingface.co/timm/resnet50.a1h_in1k)|224 |80.67|95.3 |25.6 |4.1 |11.1 |3452 |
|[resnext50d_32x4d.bt_in1k](https://huggingface.co/timm/resnext50d_32x4d.bt_in1k)|288 |80.67|95.42|25.0 |7.4 |25.1 |1626 |
|[resnetaa50.a1h_in1k](https://huggingface.co/timm/resnetaa50.a1h_in1k)|224 |80.63|95.21|25.6 |5.2 |11.6 |3034 |
|[ecaresnet50d.miil_in1k](https://huggingface.co/timm/ecaresnet50d.miil_in1k)|224 |80.61|95.32|25.6 |4.4 |11.9 |2813 |
|[resnext101_64x4d.gluon_in1k](https://huggingface.co/timm/resnext101_64x4d.gluon_in1k)|224 |80.61|94.99|83.5 |15.5 |31.2 |989 |
|[gcresnet33ts.ra2_in1k](https://huggingface.co/timm/gcresnet33ts.ra2_in1k)|288 |80.6 |95.31|19.9 |6.0 |14.8 |2578 |
|[gcresnext50ts.ch_in1k](https://huggingface.co/timm/gcresnext50ts.ch_in1k)|256 |80.57|95.17|15.7 |3.8 |15.5 |2710 |
|[resnet152.a3_in1k](https://huggingface.co/timm/resnet152.a3_in1k)|224 |80.56|95.0 |60.2 |11.6 |22.6 |1483 |
|[resnet50d.ra2_in1k](https://huggingface.co/timm/resnet50d.ra2_in1k)|224 |80.53|95.16|25.6 |4.4 |11.9 |3164 |
|[resnext50_32x4d.a1_in1k](https://huggingface.co/timm/resnext50_32x4d.a1_in1k)|224 |80.53|94.46|25.0 |4.3 |14.4 |2930 |
|[wide_resnet101_2.tv2_in1k](https://huggingface.co/timm/wide_resnet101_2.tv2_in1k)|176 |80.48|94.98|126.9 |14.3 |13.2 |1719 |
|[resnet152d.gluon_in1k](https://huggingface.co/timm/resnet152d.gluon_in1k)|224 |80.47|95.2 |60.2 |11.8 |23.4 |1428 |
|[resnet50.b2k_in1k](https://huggingface.co/timm/resnet50.b2k_in1k)|288 |80.45|95.32|25.6 |6.8 |18.4 |2086 |
|[ecaresnetlight.miil_in1k](https://huggingface.co/timm/ecaresnetlight.miil_in1k)|224 |80.45|95.24|30.2 |4.1 |8.4 |3530 |
|[resnext50_32x4d.a2_in1k](https://huggingface.co/timm/resnext50_32x4d.a2_in1k)|224 |80.45|94.63|25.0 |4.3 |14.4 |2936 |
|[wide_resnet50_2.tv2_in1k](https://huggingface.co/timm/wide_resnet50_2.tv2_in1k)|176 |80.43|95.09|68.9 |7.3 |9.0 |3015 |
|[resnet101d.gluon_in1k](https://huggingface.co/timm/resnet101d.gluon_in1k)|224 |80.42|95.01|44.6 |8.1 |17.0 |2007 |
|[resnet50.a1_in1k](https://huggingface.co/timm/resnet50.a1_in1k)|224 |80.38|94.6 |25.6 |4.1 |11.1 |3461 |
|[seresnet33ts.ra2_in1k](https://huggingface.co/timm/seresnet33ts.ra2_in1k)|256 |80.36|95.1 |19.8 |4.8 |11.7 |3267 |
|[resnext101_32x4d.gluon_in1k](https://huggingface.co/timm/resnext101_32x4d.gluon_in1k)|224 |80.34|94.93|44.2 |8.0 |21.2 |1814 |
|[resnext50_32x4d.fb_ssl_yfcc100m_ft_in1k](https://huggingface.co/timm/resnext50_32x4d.fb_ssl_yfcc100m_ft_in1k)|224 |80.32|95.4 |25.0 |4.3 |14.4 |2941 |
|[resnet101s.gluon_in1k](https://huggingface.co/timm/resnet101s.gluon_in1k)|224 |80.28|95.16|44.7 |9.2 |18.6 |1851 |
|[seresnet50.ra2_in1k](https://huggingface.co/timm/seresnet50.ra2_in1k)|224 |80.26|95.08|28.1 |4.1 |11.1 |2972 |
|[resnetblur50.bt_in1k](https://huggingface.co/timm/resnetblur50.bt_in1k)|288 |80.24|95.24|25.6 |8.5 |19.9 |1523 |
|[resnet50d.a2_in1k](https://huggingface.co/timm/resnet50d.a2_in1k)|224 |80.22|94.63|25.6 |4.4 |11.9 |3162 |
|[resnet152.tv2_in1k](https://huggingface.co/timm/resnet152.tv2_in1k)|176 |80.2 |94.64|60.2 |7.2 |14.0 |2346 |
|[seresnet50.a2_in1k](https://huggingface.co/timm/seresnet50.a2_in1k)|224 |80.08|94.74|28.1 |4.1 |11.1 |2969 |
|[eca_resnet33ts.ra2_in1k](https://huggingface.co/timm/eca_resnet33ts.ra2_in1k)|256 |80.08|94.97|19.7 |4.8 |11.7 |3284 |
|[gcresnet33ts.ra2_in1k](https://huggingface.co/timm/gcresnet33ts.ra2_in1k)|256 |80.06|94.99|19.9 |4.8 |11.7 |3216 |
|[resnet50_gn.a1h_in1k](https://huggingface.co/timm/resnet50_gn.a1h_in1k)|224 |80.06|94.95|25.6 |4.1 |11.1 |1109 |
|[seresnet50.a1_in1k](https://huggingface.co/timm/seresnet50.a1_in1k)|224 |80.02|94.71|28.1 |4.1 |11.1 |2962 |
|[resnet50.ram_in1k](https://huggingface.co/timm/resnet50.ram_in1k)|288 |79.97|95.05|25.6 |6.8 |18.4 |2086 |
|[resnet152c.gluon_in1k](https://huggingface.co/timm/resnet152c.gluon_in1k)|224 |79.92|94.84|60.2 |11.8 |23.4 |1455 |
|[seresnext50_32x4d.gluon_in1k](https://huggingface.co/timm/seresnext50_32x4d.gluon_in1k)|224 |79.91|94.82|27.6 |4.3 |14.4 |2591 |
|[resnet50.d_in1k](https://huggingface.co/timm/resnet50.d_in1k)|224 |79.91|94.67|25.6 |4.1 |11.1 |3456 |
|[resnet101.tv2_in1k](https://huggingface.co/timm/resnet101.tv2_in1k)|176 |79.9 |94.6 |44.6 |4.9 |10.1 |3341 |
|[resnetrs50.tf_in1k](https://huggingface.co/timm/resnetrs50.tf_in1k)|224 |79.89|94.97|35.7 |4.5 |12.1 |2774 |
|[resnet50.c2_in1k](https://huggingface.co/timm/resnet50.c2_in1k)|224 |79.88|94.87|25.6 |4.1 |11.1 |3455 |
|[ecaresnet26t.ra2_in1k](https://huggingface.co/timm/ecaresnet26t.ra2_in1k)|320 |79.86|95.07|16.0 |5.2 |16.4 |2168 |
|[resnet50.a2_in1k](https://huggingface.co/timm/resnet50.a2_in1k)|224 |79.85|94.56|25.6 |4.1 |11.1 |3460 |
|[resnet50.ra_in1k](https://huggingface.co/timm/resnet50.ra_in1k)|288 |79.83|94.97|25.6 |6.8 |18.4 |2087 |
|[resnet101.a3_in1k](https://huggingface.co/timm/resnet101.a3_in1k)|224 |79.82|94.62|44.6 |7.8 |16.2 |2114 |
|[resnext50_32x4d.ra_in1k](https://huggingface.co/timm/resnext50_32x4d.ra_in1k)|224 |79.76|94.6 |25.0 |4.3 |14.4 |2943 |
|[resnet50.c1_in1k](https://huggingface.co/timm/resnet50.c1_in1k)|224 |79.74|94.95|25.6 |4.1 |11.1 |3455 |
|[ecaresnet50d_pruned.miil_in1k](https://huggingface.co/timm/ecaresnet50d_pruned.miil_in1k)|224 |79.74|94.87|19.9 |2.5 |6.4 |3929 |
|[resnet33ts.ra2_in1k](https://huggingface.co/timm/resnet33ts.ra2_in1k)|288 |79.71|94.83|19.7 |6.0 |14.8 |2710 |
|[resnet152.gluon_in1k](https://huggingface.co/timm/resnet152.gluon_in1k)|224 |79.68|94.74|60.2 |11.6 |22.6 |1486 |
|[resnext50d_32x4d.bt_in1k](https://huggingface.co/timm/resnext50d_32x4d.bt_in1k)|224 |79.67|94.87|25.0 |4.5 |15.2 |2729 |
|[resnet50.bt_in1k](https://huggingface.co/timm/resnet50.bt_in1k)|288 |79.63|94.91|25.6 |6.8 |18.4 |2086 |
|[ecaresnet50t.a3_in1k](https://huggingface.co/timm/ecaresnet50t.a3_in1k)|224 |79.56|94.72|25.6 |4.3 |11.8 |2805 |
|[resnet101c.gluon_in1k](https://huggingface.co/timm/resnet101c.gluon_in1k)|224 |79.53|94.58|44.6 |8.1 |17.0 |2062 |
|[resnet50.b1k_in1k](https://huggingface.co/timm/resnet50.b1k_in1k)|224 |79.52|94.61|25.6 |4.1 |11.1 |3459 |
|[resnet50.tv2_in1k](https://huggingface.co/timm/resnet50.tv2_in1k)|176 |79.42|94.64|25.6 |2.6 |6.9 |5397 |
|[resnet32ts.ra2_in1k](https://huggingface.co/timm/resnet32ts.ra2_in1k)|288 |79.4 |94.66|18.0 |5.9 |14.6 |2752 |
|[resnet50.b2k_in1k](https://huggingface.co/timm/resnet50.b2k_in1k)|224 |79.38|94.57|25.6 |4.1 |11.1 |3459 |
|[resnext50_32x4d.tv2_in1k](https://huggingface.co/timm/resnext50_32x4d.tv2_in1k)|176 |79.37|94.3 |25.0 |2.7 |9.0 |4577 |
|[resnext50_32x4d.gluon_in1k](https://huggingface.co/timm/resnext50_32x4d.gluon_in1k)|224 |79.36|94.43|25.0 |4.3 |14.4 |2942 |
|[resnext101_32x8d.tv_in1k](https://huggingface.co/timm/resnext101_32x8d.tv_in1k)|224 |79.31|94.52|88.8 |16.5 |31.2 |1100 |
|[resnet101.gluon_in1k](https://huggingface.co/timm/resnet101.gluon_in1k)|224 |79.31|94.53|44.6 |7.8 |16.2 |2125 |
|[resnetblur50.bt_in1k](https://huggingface.co/timm/resnetblur50.bt_in1k)|224 |79.31|94.63|25.6 |5.2 |12.0 |2524 |
|[resnet50.a1h_in1k](https://huggingface.co/timm/resnet50.a1h_in1k)|176 |79.27|94.49|25.6 |2.6 |6.9 |5404 |
|[resnext50_32x4d.a3_in1k](https://huggingface.co/timm/resnext50_32x4d.a3_in1k)|224 |79.25|94.31|25.0 |4.3 |14.4 |2931 |
|[resnet50.fb_ssl_yfcc100m_ft_in1k](https://huggingface.co/timm/resnet50.fb_ssl_yfcc100m_ft_in1k)|224 |79.22|94.84|25.6 |4.1 |11.1 |3451 |
|[resnet33ts.ra2_in1k](https://huggingface.co/timm/resnet33ts.ra2_in1k)|256 |79.21|94.56|19.7 |4.8 |11.7 |3392 |
|[resnet50d.gluon_in1k](https://huggingface.co/timm/resnet50d.gluon_in1k)|224 |79.07|94.48|25.6 |4.4 |11.9 |3162 |
|[resnet50.ram_in1k](https://huggingface.co/timm/resnet50.ram_in1k)|224 |79.03|94.38|25.6 |4.1 |11.1 |3453 |
|[resnet50.am_in1k](https://huggingface.co/timm/resnet50.am_in1k)|224 |79.01|94.39|25.6 |4.1 |11.1 |3461 |
|[resnet32ts.ra2_in1k](https://huggingface.co/timm/resnet32ts.ra2_in1k)|256 |79.01|94.37|18.0 |4.6 |11.6 |3440 |
|[ecaresnet26t.ra2_in1k](https://huggingface.co/timm/ecaresnet26t.ra2_in1k)|256 |78.9 |94.54|16.0 |3.4 |10.5 |3421 |
|[resnet152.a3_in1k](https://huggingface.co/timm/resnet152.a3_in1k)|160 |78.89|94.11|60.2 |5.9 |11.5 |2745 |
|[wide_resnet101_2.tv_in1k](https://huggingface.co/timm/wide_resnet101_2.tv_in1k)|224 |78.84|94.28|126.9 |22.8 |21.2 |1079 |
|[seresnext26d_32x4d.bt_in1k](https://huggingface.co/timm/seresnext26d_32x4d.bt_in1k)|288 |78.83|94.24|16.8 |4.5 |16.8 |2251 |
|[resnet50.ra_in1k](https://huggingface.co/timm/resnet50.ra_in1k)|224 |78.81|94.32|25.6 |4.1 |11.1 |3454 |
|[seresnext26t_32x4d.bt_in1k](https://huggingface.co/timm/seresnext26t_32x4d.bt_in1k)|288 |78.74|94.33|16.8 |4.5 |16.7 |2264 |
|[resnet50s.gluon_in1k](https://huggingface.co/timm/resnet50s.gluon_in1k)|224 |78.72|94.23|25.7 |5.5 |13.5 |2796 |
|[resnet50d.a3_in1k](https://huggingface.co/timm/resnet50d.a3_in1k)|224 |78.71|94.24|25.6 |4.4 |11.9 |3154 |
|[wide_resnet50_2.tv_in1k](https://huggingface.co/timm/wide_resnet50_2.tv_in1k)|224 |78.47|94.09|68.9 |11.4 |14.4 |1934 |
|[resnet50.bt_in1k](https://huggingface.co/timm/resnet50.bt_in1k)|224 |78.46|94.27|25.6 |4.1 |11.1 |3454 |
|[resnet34d.ra2_in1k](https://huggingface.co/timm/resnet34d.ra2_in1k)|288 |78.43|94.35|21.8 |6.5 |7.5 |3291 |
|[gcresnext26ts.ch_in1k](https://huggingface.co/timm/gcresnext26ts.ch_in1k)|288 |78.42|94.04|10.5 |3.1 |13.3 |3226 |
|[resnet26t.ra2_in1k](https://huggingface.co/timm/resnet26t.ra2_in1k)|320 |78.33|94.13|16.0 |5.2 |16.4 |2391 |
|[resnet152.tv_in1k](https://huggingface.co/timm/resnet152.tv_in1k)|224 |78.32|94.04|60.2 |11.6 |22.6 |1487 |
|[seresnext26ts.ch_in1k](https://huggingface.co/timm/seresnext26ts.ch_in1k)|288 |78.28|94.1 |10.4 |3.1 |13.3 |3062 |
|[bat_resnext26ts.ch_in1k](https://huggingface.co/timm/bat_resnext26ts.ch_in1k)|256 |78.25|94.1 |10.7 |2.5 |12.5 |3393 |
|[resnet50.a3_in1k](https://huggingface.co/timm/resnet50.a3_in1k)|224 |78.06|93.78|25.6 |4.1 |11.1 |3450 |
|[resnet50c.gluon_in1k](https://huggingface.co/timm/resnet50c.gluon_in1k)|224 |78.0 |93.99|25.6 |4.4 |11.9 |3286 |
|[eca_resnext26ts.ch_in1k](https://huggingface.co/timm/eca_resnext26ts.ch_in1k)|288 |78.0 |93.91|10.3 |3.1 |13.3 |3297 |
|[seresnext26t_32x4d.bt_in1k](https://huggingface.co/timm/seresnext26t_32x4d.bt_in1k)|224 |77.98|93.75|16.8 |2.7 |10.1 |3841 |
|[resnet34.a1_in1k](https://huggingface.co/timm/resnet34.a1_in1k)|288 |77.92|93.77|21.8 |6.1 |6.2 |3609 |
|[resnet101.a3_in1k](https://huggingface.co/timm/resnet101.a3_in1k)|160 |77.88|93.71|44.6 |4.0 |8.3 |3926 |
|[resnet26t.ra2_in1k](https://huggingface.co/timm/resnet26t.ra2_in1k)|256 |77.87|93.84|16.0 |3.4 |10.5 |3772 |
|[seresnext26ts.ch_in1k](https://huggingface.co/timm/seresnext26ts.ch_in1k)|256 |77.86|93.79|10.4 |2.4 |10.5 |4263 |
|[resnetrs50.tf_in1k](https://huggingface.co/timm/resnetrs50.tf_in1k)|160 |77.82|93.81|35.7 |2.3 |6.2 |5238 |
|[gcresnext26ts.ch_in1k](https://huggingface.co/timm/gcresnext26ts.ch_in1k)|256 |77.81|93.82|10.5 |2.4 |10.5 |4183 |
|[ecaresnet50t.a3_in1k](https://huggingface.co/timm/ecaresnet50t.a3_in1k)|160 |77.79|93.6 |25.6 |2.2 |6.0 |5329 |
|[resnext50_32x4d.a3_in1k](https://huggingface.co/timm/resnext50_32x4d.a3_in1k)|160 |77.73|93.32|25.0 |2.2 |7.4 |5576 |
|[resnext50_32x4d.tv_in1k](https://huggingface.co/timm/resnext50_32x4d.tv_in1k)|224 |77.61|93.7 |25.0 |4.3 |14.4 |2944 |
|[seresnext26d_32x4d.bt_in1k](https://huggingface.co/timm/seresnext26d_32x4d.bt_in1k)|224 |77.59|93.61|16.8 |2.7 |10.2 |3807 |
|[resnet50.gluon_in1k](https://huggingface.co/timm/resnet50.gluon_in1k)|224 |77.58|93.72|25.6 |4.1 |11.1 |3455 |
|[eca_resnext26ts.ch_in1k](https://huggingface.co/timm/eca_resnext26ts.ch_in1k)|256 |77.44|93.56|10.3 |2.4 |10.5 |4284 |
|[resnet26d.bt_in1k](https://huggingface.co/timm/resnet26d.bt_in1k)|288 |77.41|93.63|16.0 |4.3 |13.5 |2907 |
|[resnet101.tv_in1k](https://huggingface.co/timm/resnet101.tv_in1k)|224 |77.38|93.54|44.6 |7.8 |16.2 |2125 |
|[resnet50d.a3_in1k](https://huggingface.co/timm/resnet50d.a3_in1k)|160 |77.22|93.27|25.6 |2.2 |6.1 |5982 |
|[resnext26ts.ra2_in1k](https://huggingface.co/timm/resnext26ts.ra2_in1k)|288 |77.17|93.47|10.3 |3.1 |13.3 |3392 |
|[resnet34.a2_in1k](https://huggingface.co/timm/resnet34.a2_in1k)|288 |77.15|93.27|21.8 |6.1 |6.2 |3615 |
|[resnet34d.ra2_in1k](https://huggingface.co/timm/resnet34d.ra2_in1k)|224 |77.1 |93.37|21.8 |3.9 |4.5 |5436 |
|[seresnet50.a3_in1k](https://huggingface.co/timm/seresnet50.a3_in1k)|224 |77.02|93.07|28.1 |4.1 |11.1 |2952 |
|[resnext26ts.ra2_in1k](https://huggingface.co/timm/resnext26ts.ra2_in1k)|256 |76.78|93.13|10.3 |2.4 |10.5 |4410 |
|[resnet26d.bt_in1k](https://huggingface.co/timm/resnet26d.bt_in1k)|224 |76.7 |93.17|16.0 |2.6 |8.2 |4859 |
|[resnet34.bt_in1k](https://huggingface.co/timm/resnet34.bt_in1k)|288 |76.5 |93.35|21.8 |6.1 |6.2 |3617 |
|[resnet34.a1_in1k](https://huggingface.co/timm/resnet34.a1_in1k)|224 |76.42|92.87|21.8 |3.7 |3.7 |5984 |
|[resnet26.bt_in1k](https://huggingface.co/timm/resnet26.bt_in1k)|288 |76.35|93.18|16.0 |3.9 |12.2 |3331 |
|[resnet50.tv_in1k](https://huggingface.co/timm/resnet50.tv_in1k)|224 |76.13|92.86|25.6 |4.1 |11.1 |3457 |
|[resnet50.a3_in1k](https://huggingface.co/timm/resnet50.a3_in1k)|160 |75.96|92.5 |25.6 |2.1 |5.7 |6490 |
|[resnet34.a2_in1k](https://huggingface.co/timm/resnet34.a2_in1k)|224 |75.52|92.44|21.8 |3.7 |3.7 |5991 |
|[resnet26.bt_in1k](https://huggingface.co/timm/resnet26.bt_in1k)|224 |75.3 |92.58|16.0 |2.4 |7.4 |5583 |
|[resnet34.bt_in1k](https://huggingface.co/timm/resnet34.bt_in1k)|224 |75.16|92.18|21.8 |3.7 |3.7 |5994 |
|[seresnet50.a3_in1k](https://huggingface.co/timm/seresnet50.a3_in1k)|160 |75.1 |92.08|28.1 |2.1 |5.7 |5513 |
|[resnet34.gluon_in1k](https://huggingface.co/timm/resnet34.gluon_in1k)|224 |74.57|91.98|21.8 |3.7 |3.7 |5984 |
|[resnet18d.ra2_in1k](https://huggingface.co/timm/resnet18d.ra2_in1k)|288 |73.81|91.83|11.7 |3.4 |5.4 |5196 |
|[resnet34.tv_in1k](https://huggingface.co/timm/resnet34.tv_in1k)|224 |73.32|91.42|21.8 |3.7 |3.7 |5979 |
|[resnet18.fb_swsl_ig1b_ft_in1k](https://huggingface.co/timm/resnet18.fb_swsl_ig1b_ft_in1k)|224 |73.28|91.73|11.7 |1.8 |2.5 |10213 |
|[resnet18.a1_in1k](https://huggingface.co/timm/resnet18.a1_in1k)|288 |73.16|91.03|11.7 |3.0 |4.1 |6050 |
|[resnet34.a3_in1k](https://huggingface.co/timm/resnet34.a3_in1k)|224 |72.98|91.11|21.8 |3.7 |3.7 |5967 |
|[resnet18.fb_ssl_yfcc100m_ft_in1k](https://huggingface.co/timm/resnet18.fb_ssl_yfcc100m_ft_in1k)|224 |72.6 |91.42|11.7 |1.8 |2.5 |10213 |
|[resnet18.a2_in1k](https://huggingface.co/timm/resnet18.a2_in1k)|288 |72.37|90.59|11.7 |3.0 |4.1 |6051 |
|[resnet14t.c3_in1k](https://huggingface.co/timm/resnet14t.c3_in1k)|224 |72.26|90.31|10.1 |1.7 |5.8 |7026 |
|[resnet18d.ra2_in1k](https://huggingface.co/timm/resnet18d.ra2_in1k)|224 |72.26|90.68|11.7 |2.1 |3.3 |8707 |
|[resnet18.a1_in1k](https://huggingface.co/timm/resnet18.a1_in1k)|224 |71.49|90.07|11.7 |1.8 |2.5 |10187 |
|[resnet14t.c3_in1k](https://huggingface.co/timm/resnet14t.c3_in1k)|176 |71.31|89.69|10.1 |1.1 |3.6 |10970 |
|[resnet18.gluon_in1k](https://huggingface.co/timm/resnet18.gluon_in1k)|224 |70.84|89.76|11.7 |1.8 |2.5 |10210 |
|[resnet18.a2_in1k](https://huggingface.co/timm/resnet18.a2_in1k)|224 |70.64|89.47|11.7 |1.8 |2.5 |10194 |
|[resnet34.a3_in1k](https://huggingface.co/timm/resnet34.a3_in1k)|160 |70.56|89.52|21.8 |1.9 |1.9 |10737 |
|[resnet18.tv_in1k](https://huggingface.co/timm/resnet18.tv_in1k)|224 |69.76|89.07|11.7 |1.8 |2.5 |10205 |
|[resnet10t.c3_in1k](https://huggingface.co/timm/resnet10t.c3_in1k)|224 |68.34|88.03|5.4 |1.1 |2.4 |13079 |
|[resnet18.a3_in1k](https://huggingface.co/timm/resnet18.a3_in1k)|224 |68.25|88.17|11.7 |1.8 |2.5 |10167 |
|[resnet10t.c3_in1k](https://huggingface.co/timm/resnet10t.c3_in1k)|176 |66.71|86.96|5.4 |0.7 |1.5 |20327 |
|[resnet18.a3_in1k](https://huggingface.co/timm/resnet18.a3_in1k)|160 |65.66|86.26|11.7 |0.9 |1.3 |18229 |
## Citation
```bibtex
@article{Xie2016,
title={Aggregated Residual Transformations for Deep Neural Networks},
author={Saining Xie and Ross Girshick and Piotr Dollár and Zhuowen Tu and Kaiming He},
journal={arXiv preprint arXiv:1611.05431},
year={2016}
}
```
```bibtex
@article{He2015,
author = {Kaiming He and Xiangyu Zhang and Shaoqing Ren and Jian Sun},
title = {Deep Residual Learning for Image Recognition},
journal = {arXiv preprint arXiv:1512.03385},
year = {2015}
}
```
```bibtex
@inproceedings{hu2018senet,
title={Squeeze-and-Excitation Networks},
author={Jie Hu and Li Shen and Gang Sun},
journal={IEEE Conference on Computer Vision and Pattern Recognition},
year={2018}
}
```
```bibtex
@article{He2018BagOT,
title={Bag of Tricks for Image Classification with Convolutional Neural Networks},
author={Tong He and Zhi Zhang and Hang Zhang and Zhongyue Zhang and Junyuan Xie and Mu Li},
journal={2019 IEEE/CVF Conference on Computer Vision and Pattern Recognition (CVPR)},
year={2018},
pages={558-567}
}
```
```bibtex
@misc{rw2019timm,
author = {Ross Wightman},
title = {PyTorch Image Models},
year = {2019},
publisher = {GitHub},
journal = {GitHub repository},
doi = {10.5281/zenodo.4414861},
howpublished = {\url{https://github.com/huggingface/pytorch-image-models}}
}
```
|
Deci/DeciLM-6b | Deci | "2024-02-15T08:48:25Z" | 960 | 234 | transformers | [
"transformers",
"safetensors",
"text-generation",
"Deci AI",
"DeciLM",
"custom_code",
"en",
"dataset:cerebras/SlimPajama-627B",
"arxiv:2106.09685",
"arxiv:2305.13245",
"arxiv:2104.09864",
"license:llama2",
"license:other",
"model-index",
"autotrain_compatible",
"region:us"
] | text-generation | "2023-09-13T07:20:36Z" | ---
license: [llama2, other]
datasets:
- cerebras/SlimPajama-627B
language:
- en
pipeline_tag: text-generation
tags:
- Deci AI
- DeciLM
model-index:
- name: DeciLM 6B
results:
- task:
type: text-generation
dataset:
type: ai2/arc
name: ai2_arc
metrics:
- name: ARC Challenge
type: ARC Challenge
value: 42.06
verified: false
- task:
type: text-generation
dataset:
type: ai2/arc
name: ai2_arc
metrics:
- name: ARC Easy
type: ARC Easy
value: 70.02
verified: false
- task:
type: text-generation
dataset:
type: boolq
name: boolq
metrics:
- name: BoolQ
type: BoolQ
value: 71.01
verified: false
- task:
type: text-generation
dataset:
type: hellaswag
name: hellaswag
metrics:
- name: HellaSwag
type: HellaSwag
value: 74.58
verified: false
- task:
type: text-generation
dataset:
type: LAMBDA
name: OpenAI LAMBDA
metrics:
- name: LAMBDA
type: LAMBDA
value: 69.78
verified: false
- task:
type: text-generation
dataset:
type: OpenBookQA
name: openbookqa
metrics:
- name: OpenBookQA
type: OpenBookQA
value: 34
verified: false
- task:
type: text-generation
dataset:
type: PIQA
name: piqa
metrics:
- name: PIQA
type: PIQA
value: 77.09
verified: false
- task:
type: text-generation
dataset:
type: truthful_qa
name: truthful_qa
metrics:
- name: TruthfulQA
type: TruthfulQA
value: 36.19
verified: false
- task:
type: text-generation
dataset:
type: winogrande
name: winogrande
metrics:
- name: Winogrande
type: Winogrande
value: 68.03
verified: false
---
# DeciLM 6B
DeciLM 6B is a 5.7 billion parameter decoder-only text generation model. With a context window of 4096 tokens, the highly efficient model uses variable Grouped-Query Attention (GQA) to achieve an optimal balance between performance and computational efficiency. The model's architecture was generated using Deci's proprietary Neural Architecture Search-based technology, AutoNAC.
## Model Details
### Model Description
Deci developed and publically released the DeciLM 6B large language model, a pretrained, high-efficiency generative text model with 5.7 billion parameters. DeciLM 6B outpaces pretrained models in its class, with a throughput that's up to 15 times that of Llama 2 7B's. DeciLM-6B was further fine-tuned using [LoRA ](https://arxiv.org/pdf/2106.09685.pdf) for instruction following on a subset of the OpenOrca dataset, creating [DeciLM 6B-Instruct](https://huggingface.co/Deci/DeciLM-6b-instruct)
- **Developed by:** Deci
- **Model type:** DeciLM is an auto-regressive language model using an optimized transformer decoder architecture that includes variable Grouped-Query Attention.
- **Language(s) (NLP):** English
- **License:** [Llama 2 Community License Agreement](https://huggingface.co/Deci/DeciLM-6b/blob/main/LICENSE.md) with an extention of Deci regarding hosting service providers.
## Model Architecture
| Parameters | Layers | Heads | Sequence Length | GQA num_key_value_heads* | Hidden Size |
|:----------|:----------|:----------|:----------|:----------|:----------|
| 5.7B | 32 | 32 | 4096 | Variable | 4096 | |
*AutoNAC was employed to optimize the selection of the GQA num_key_value_heads for each layer of the model.
- **Decoder layer:** Varible Grouped Query Attention. Grouped Query Attention (GQA) was introduced in [Ainslie et al., 2023](https://arxiv.org/abs/2305.13245)
- **Position Embeddings:** Dynamic NTK Scaling Rotary Position Embeddings [Su et al., 2021](https://arxiv.org/abs/2104.09864)
### Model Sources
- **Paper:** [DeciLM Technical Blog](https://deci.ai/blog/decilm-15-times-faster-than-llama2-nas-generated-llm-with-variable-gqa/?utm_campaign=repos&utm_source=hugging-face&utm_medium=model-card&utm_content=decilm-6b)
- **Demo:** [DeciLM 6B Instruct Demo](https://huggingface.co/spaces/Deci/DeciLM-6b-instruct)
- **Notebook:** [DeciLM 6B Notebook](https://colab.research.google.com/drive/1LugJCifOv0L426ukRHjOblBRWwUImAit)
## Uses
The model is intended for commercial and research use in English and can be fine-tuned for use in other languages.
## How to Get Started with the Model
Use the code below to get started with the model.
```bibtex
# pip install -q transformers
import torch
from transformers import AutoModelForCausalLM, AutoTokenizer
checkpoint = "Deci/DeciLM-6b"
device = "cuda" # for GPU usage or "cpu" for CPU usage
tokenizer = AutoTokenizer.from_pretrained(checkpoint)
model = AutoModelForCausalLM.from_pretrained(checkpoint, torch_dtype=torch.bfloat16, trust_remote_code=True).to(device)
inputs = tokenizer.encode("In a shocking finding, scientists discovered a herd of unicorns living in", return_tensors="pt").to(device)
outputs = model.generate(inputs, max_new_tokens=100, do_sample=True, top_p=0.95)
print(tokenizer.decode(outputs[0]))
```
## Training Details
DeciLM 6B underwent training utilizing a subset of the SlimPajamas dataset, leveraging advanced proprietary methodologies allowing for fast training.
## Evaluation
Below are DeciLM's 6B evaluation results.
| Average | ARC Challenge* | ARC Easy* | BoolQ | HellaSwag* | LAMBDA OpenAI | OpenBookQA | PIQA | TruthfulQA | Winogrande |
|:----------|:----------|:----------|:----------|:----------|:----------|:----------|:----------|:----------|:----------|
| 60.33 | 42.06 | 70.02 | 71.01 | 74.58 | 69.78 | 34 | 77.09 |36.19 | 68.03 |
Accuracy-norm score*
### Runtime Benchmarks
|Inference Tool/Hardware | A10 (tokens/sec) |
|:----------|:----------|
| PyTorch | 652.49 |
| Infery LLM | 2,029.6 |
- Throughput (tokens/sec) - Measured with optimal batch - PyTorch BS 64, Infery LLM BS 128
- In order to replicate the results of the PyTorch benchmark, use this [code example](https://huggingface.co/Deci/DeciLM-6b/blob/main/hf_benchmark_example.py)
## How to Cite
Please cite this model using this format.
```bibtex
@misc{DeciFoundationModels,
title = {DeciLM 6B},
author = {DeciAI Research Team},
year = {2023}
url={[https://huggingface.co/Deci/DeciLM-6b](https://huggingface.co/Deci/DeciLM-6b)},
}
``` |
devingulliver/llama-pile-350b | devingulliver | "2024-04-30T17:57:33Z" | 960 | 0 | transformers | [
"transformers",
"safetensors",
"llama",
"text-generation",
"arxiv:1910.09700",
"license:apache-2.0",
"model-index",
"autotrain_compatible",
"endpoints_compatible",
"text-generation-inference",
"region:us"
] | text-generation | "2024-04-22T18:49:56Z" | ---
license: apache-2.0
library_name: transformers
model-index:
- name: llama-pile-350b
results:
- task:
type: text-generation
name: Text Generation
dataset:
name: AI2 Reasoning Challenge (25-Shot)
type: ai2_arc
config: ARC-Challenge
split: test
args:
num_few_shot: 25
metrics:
- type: acc_norm
value: 33.19
name: normalized accuracy
source:
url: https://huggingface.co/spaces/HuggingFaceH4/open_llm_leaderboard?query=devingulliver/llama-pile-350b
name: Open LLM Leaderboard
- task:
type: text-generation
name: Text Generation
dataset:
name: HellaSwag (10-Shot)
type: hellaswag
split: validation
args:
num_few_shot: 10
metrics:
- type: acc_norm
value: 56.6
name: normalized accuracy
source:
url: https://huggingface.co/spaces/HuggingFaceH4/open_llm_leaderboard?query=devingulliver/llama-pile-350b
name: Open LLM Leaderboard
- task:
type: text-generation
name: Text Generation
dataset:
name: MMLU (5-Shot)
type: cais/mmlu
config: all
split: test
args:
num_few_shot: 5
metrics:
- type: acc
value: 24.66
name: accuracy
source:
url: https://huggingface.co/spaces/HuggingFaceH4/open_llm_leaderboard?query=devingulliver/llama-pile-350b
name: Open LLM Leaderboard
- task:
type: text-generation
name: Text Generation
dataset:
name: TruthfulQA (0-shot)
type: truthful_qa
config: multiple_choice
split: validation
args:
num_few_shot: 0
metrics:
- type: mc2
value: 36.28
source:
url: https://huggingface.co/spaces/HuggingFaceH4/open_llm_leaderboard?query=devingulliver/llama-pile-350b
name: Open LLM Leaderboard
- task:
type: text-generation
name: Text Generation
dataset:
name: Winogrande (5-shot)
type: winogrande
config: winogrande_xl
split: validation
args:
num_few_shot: 5
metrics:
- type: acc
value: 58.48
name: accuracy
source:
url: https://huggingface.co/spaces/HuggingFaceH4/open_llm_leaderboard?query=devingulliver/llama-pile-350b
name: Open LLM Leaderboard
- task:
type: text-generation
name: Text Generation
dataset:
name: GSM8k (5-shot)
type: gsm8k
config: main
split: test
args:
num_few_shot: 5
metrics:
- type: acc
value: 0.76
name: accuracy
source:
url: https://huggingface.co/spaces/HuggingFaceH4/open_llm_leaderboard?query=devingulliver/llama-pile-350b
name: Open LLM Leaderboard
---
# Model Card for devingulliver/llama-pile-350b
Llama-style model trained on The Pile for 350B tokens. Clone of [HuggingFaceFW/ablation-model-the-pile](https://huggingface.co/HuggingFaceFW/ablation-model-the-pile).
## Model Details
### Model Description
<!-- Provide a longer summary of what this model is. -->
This is the model card of a 🤗 transformers model that has been pushed on the Hub. This model card has been automatically generated.
- **Developed by:** [More Information Needed]
- **Funded by [optional]:** [More Information Needed]
- **Shared by [optional]:** [More Information Needed]
- **Model type:** [More Information Needed]
- **Language(s) (NLP):** [More Information Needed]
- **License:** [More Information Needed]
- **Finetuned from model [optional]:** [More Information Needed]
### Model Sources [optional]
<!-- Provide the basic links for the model. -->
- **Repository:** [More Information Needed]
- **Paper [optional]:** [More Information Needed]
- **Demo [optional]:** [More Information Needed]
## Uses
<!-- Address questions around how the model is intended to be used, including the foreseeable users of the model and those affected by the model. -->
### Direct Use
<!-- This section is for the model use without fine-tuning or plugging into a larger ecosystem/app. -->
[More Information Needed]
### Downstream Use [optional]
<!-- This section is for the model use when fine-tuned for a task, or when plugged into a larger ecosystem/app -->
[More Information Needed]
### Out-of-Scope Use
<!-- This section addresses misuse, malicious use, and uses that the model will not work well for. -->
[More Information Needed]
## Bias, Risks, and Limitations
<!-- This section is meant to convey both technical and sociotechnical limitations. -->
[More Information Needed]
### Recommendations
<!-- This section is meant to convey recommendations with respect to the bias, risk, and technical limitations. -->
Users (both direct and downstream) should be made aware of the risks, biases and limitations of the model. More information needed for further recommendations.
## How to Get Started with the Model
Use the code below to get started with the model.
[More Information Needed]
## Training Details
### Training Data
<!-- This should link to a Dataset Card, perhaps with a short stub of information on what the training data is all about as well as documentation related to data pre-processing or additional filtering. -->
[More Information Needed]
### Training Procedure
<!-- This relates heavily to the Technical Specifications. Content here should link to that section when it is relevant to the training procedure. -->
#### Preprocessing [optional]
[More Information Needed]
#### Training Hyperparameters
- **Training regime:** [More Information Needed] <!--fp32, fp16 mixed precision, bf16 mixed precision, bf16 non-mixed precision, fp16 non-mixed precision, fp8 mixed precision -->
#### Speeds, Sizes, Times [optional]
<!-- This section provides information about throughput, start/end time, checkpoint size if relevant, etc. -->
[More Information Needed]
## Evaluation
<!-- This section describes the evaluation protocols and provides the results. -->
### Testing Data, Factors & Metrics
#### Testing Data
<!-- This should link to a Dataset Card if possible. -->
[More Information Needed]
#### Factors
<!-- These are the things the evaluation is disaggregating by, e.g., subpopulations or domains. -->
[More Information Needed]
#### Metrics
<!-- These are the evaluation metrics being used, ideally with a description of why. -->
[More Information Needed]
### Results
[More Information Needed]
#### Summary
## Model Examination [optional]
<!-- Relevant interpretability work for the model goes here -->
[More Information Needed]
## Environmental Impact
<!-- Total emissions (in grams of CO2eq) and additional considerations, such as electricity usage, go here. Edit the suggested text below accordingly -->
Carbon emissions can be estimated using the [Machine Learning Impact calculator](https://mlco2.github.io/impact#compute) presented in [Lacoste et al. (2019)](https://arxiv.org/abs/1910.09700).
- **Hardware Type:** [More Information Needed]
- **Hours used:** [More Information Needed]
- **Cloud Provider:** [More Information Needed]
- **Compute Region:** [More Information Needed]
- **Carbon Emitted:** [More Information Needed]
## Technical Specifications [optional]
### Model Architecture and Objective
[More Information Needed]
### Compute Infrastructure
[More Information Needed]
#### Hardware
[More Information Needed]
#### Software
[More Information Needed]
## Citation [optional]
<!-- If there is a paper or blog post introducing the model, the APA and Bibtex information for that should go in this section. -->
**BibTeX:**
[More Information Needed]
**APA:**
[More Information Needed]
## Glossary [optional]
<!-- If relevant, include terms and calculations in this section that can help readers understand the model or model card. -->
[More Information Needed]
## More Information [optional]
[More Information Needed]
## Model Card Authors [optional]
[More Information Needed]
## Model Card Contact
[More Information Needed]
# [Open LLM Leaderboard Evaluation Results](https://huggingface.co/spaces/HuggingFaceH4/open_llm_leaderboard)
Detailed results can be found [here](https://huggingface.co/datasets/open-llm-leaderboard/details_devingulliver__llama-pile-350b)
| Metric |Value|
|---------------------------------|----:|
|Avg. |35.00|
|AI2 Reasoning Challenge (25-Shot)|33.19|
|HellaSwag (10-Shot) |56.60|
|MMLU (5-Shot) |24.66|
|TruthfulQA (0-shot) |36.28|
|Winogrande (5-shot) |58.48|
|GSM8k (5-shot) | 0.76|
|
cahya/bert-base-indonesian-1.5G | cahya | "2021-05-19T13:37:31Z" | 959 | 3 | transformers | [
"transformers",
"pytorch",
"tf",
"jax",
"bert",
"fill-mask",
"id",
"dataset:wikipedia",
"dataset:id_newspapers_2018",
"license:mit",
"autotrain_compatible",
"endpoints_compatible",
"region:us"
] | fill-mask | "2022-03-02T23:29:05Z" | ---
language: "id"
license: "mit"
datasets:
- wikipedia
- id_newspapers_2018
widget:
- text: "Ibu ku sedang bekerja [MASK] sawah."
---
# Indonesian BERT base model (uncased)
## Model description
It is BERT-base model pre-trained with indonesian Wikipedia and indonesian newspapers using a masked language modeling (MLM) objective. This
model is uncased.
This is one of several other language models that have been pre-trained with indonesian datasets. More detail about
its usage on downstream tasks (text classification, text generation, etc) is available at [Transformer based Indonesian Language Models](https://github.com/cahya-wirawan/indonesian-language-models/tree/master/Transformers)
## Intended uses & limitations
### How to use
You can use this model directly with a pipeline for masked language modeling:
```python
>>> from transformers import pipeline
>>> unmasker = pipeline('fill-mask', model='cahya/bert-base-indonesian-1.5G')
>>> unmasker("Ibu ku sedang bekerja [MASK] supermarket")
[{'sequence': '[CLS] ibu ku sedang bekerja di supermarket [SEP]',
'score': 0.7983310222625732,
'token': 1495},
{'sequence': '[CLS] ibu ku sedang bekerja. supermarket [SEP]',
'score': 0.090003103017807,
'token': 17},
{'sequence': '[CLS] ibu ku sedang bekerja sebagai supermarket [SEP]',
'score': 0.025469014421105385,
'token': 1600},
{'sequence': '[CLS] ibu ku sedang bekerja dengan supermarket [SEP]',
'score': 0.017966199666261673,
'token': 1555},
{'sequence': '[CLS] ibu ku sedang bekerja untuk supermarket [SEP]',
'score': 0.016971781849861145,
'token': 1572}]
```
Here is how to use this model to get the features of a given text in PyTorch:
```python
from transformers import BertTokenizer, BertModel
model_name='cahya/bert-base-indonesian-1.5G'
tokenizer = BertTokenizer.from_pretrained(model_name)
model = BertModel.from_pretrained(model_name)
text = "Silakan diganti dengan text apa saja."
encoded_input = tokenizer(text, return_tensors='pt')
output = model(**encoded_input)
```
and in Tensorflow:
```python
from transformers import BertTokenizer, TFBertModel
model_name='cahya/bert-base-indonesian-1.5G'
tokenizer = BertTokenizer.from_pretrained(model_name)
model = TFBertModel.from_pretrained(model_name)
text = "Silakan diganti dengan text apa saja."
encoded_input = tokenizer(text, return_tensors='tf')
output = model(encoded_input)
```
## Training data
This model was pre-trained with 522MB of indonesian Wikipedia and 1GB of
[indonesian newspapers](https://huggingface.co/datasets/id_newspapers_2018).
The texts are lowercased and tokenized using WordPiece and a vocabulary size of 32,000. The inputs of the model are
then of the form:
```[CLS] Sentence A [SEP] Sentence B [SEP]``` |
FFI/SimCSE-NB-BERT-large | FFI | "2023-05-19T12:25:01Z" | 959 | 0 | transformers | [
"transformers",
"pytorch",
"bert",
"no",
"endpoints_compatible",
"region:us"
] | null | "2023-05-19T11:32:56Z" | ---
language:
- no
---
This is a Norwegian sentence embedding model trained using the SimCSE methodology (Gao et.al, SimCSE: Simple Contrastive Learning of Sentence Embeddings, EMNLP 2021). It is trained from the NB-BERT-large model (https://huggingface.co/NbAiLab/nb-bert-large/). It is trained supervised on the Norwegian automatically translated mnli dataset (https://huggingface.co/datasets/NbAiLab/mnli-norwegian).
The training and performance of this model is described in the paper "Training and Evaluating Norwegian Sentece Embedding Models", published at NoDaLiDa 2023 (https://openreview.net/forum?id=tcxy7vRVKlg). In that paper we describe training several different Norwegian sentence embedding models. This is the best performing model on STS data of those described in the paper. |
pansophic/rocket-3B | pansophic | "2024-03-01T11:21:21Z" | 959 | 76 | transformers | [
"transformers",
"pytorch",
"safetensors",
"stablelm",
"text-generation",
"en",
"arxiv:2305.18290",
"arxiv:2101.00027",
"arxiv:2305.06161",
"base_model:stabilityai/stablelm-3b-4e1t",
"license:cc-by-sa-4.0",
"autotrain_compatible",
"endpoints_compatible",
"region:us"
] | text-generation | "2023-11-19T17:14:29Z" | ---
language:
- en
license: cc-by-sa-4.0
base_model: stabilityai/stablelm-3b-4e1t
model-index:
- name: rocket-3b
results: []
---
<img src="https://cdn-uploads.huggingface.co/production/uploads/6501bfe0493fd9c8c2e32402/BmbkjOkcTm-YMa-unolmJ.png" alt="Rocket Logo" width="800" style="margin-left:'auto' margin-right:'auto' display:'block'"/>
# Rocket-3B 🦝
<b>Rocket</b> 🦝 is a 3 billion large language model that was trained on a mix of publicly available datasets using [Direct Preference Optimization (DPO)](https://arxiv.org/abs/2305.18290). The prompt format used is <b>ChatML</b>.
## Model description
- **Model type:** A 3B parameter GPT-like model fine-tuned on a mix of publicly available datasets using DPO.
- **Language(s) (NLP):** Primarily English
- **License:** CC-BY-SA-4.0
- **Finetuned from model:** [Stability AI](https://huggingface.co/stabilityai/stablelm-3b-4e1t)
## Performance
Despite its compact dimensions, the model achieves outstanding scores in both [MT-Bench](https://huggingface.co/spaces/lmsys/mt-bench) and [AlpacaEval](https://tatsu-lab.github.io/alpaca_eval/) benchmarks, surpassing the performance of considerably larger models.
| Model | Size | Alignment | MT-Bench (score) | AlpacaEval (win rate %) |
|-------------|-----|----|---------------|--------------|
| StableLM-Tuned-α 🦜| 7B | SFT |2.75| -|
| MPT-Chat | 7B | SFT |5.42| -|
| Falcon-Instruct 🦅| 40B | SFT |5.17 |45.71|
| Orca-2| 13B | SFT |6.15 |-|
| Xwin-LMv0.1 | 7B| PPO | 6.19| 87.83|
| Llama2-Chat 🦙| 7B |RLHF |6.26| 71.37|
| TÜLU 2 🐫| 7B | DPO |6.27| 85.1|
| Guanaco 🦙| 65B | SFT |6.41| 71.80|
| **Rocket** 🦝 | **3B** | **DPO** | **6.56** | **79.75** |
| Llama2-Chat 🦙| 13B |RLHF |6.65| 81.09|
| Zephyr-7b-α 🪁 |7B| DPO| 6.88| -|
| Vicuna v1.3 🦙| 33B | SFT |7.12 |88.99|
| Zephyr-7b-β 🪁 |7B| DPO| 7.34| 90.60|
| WizardLM v1.0 🦙| 70B |SFT |7.71 |-|
| GPT-3.5-turbo | - |RLHF |7.94 |89.37|
Specifically, across various categories within the MT-Bench evaluation, Rocket-3B demonstrates impressive performance when compared to larger open models such as Llama2-Chat-7B, Falcon-40B-Instruct, and Guanaco-65B.

## MT-Bench detailed score for first and second turn
In MT-Bench, Rocket 🦝 scores 6.99 in the first turn and 6.13 in the second turn, with an average score of 6.56. These scores reflect the model's performance in understanding and generating text during different parts of a conversation.
| Model | First turn | Second turn | Average |
|-------------|-----|----|---------------|
| **Rocket** 🦝 | **6.99** | **6.13** | **6.56** |
## AlpacaEval detailed scores
In AlpacaEval, Rocket 🦝 achieves a near 80% win rate, coupled with an average response length of 1,242 tokens, indicating its effectiveness in producing detailed responses.
| Model | Win rate | Std error | Average length |
|-------------|-----|----|---------------|
| **Rocket** 🦝 | **79.75** | **1.42** | **1242** |
## [Open LLM Leaderboard Evaluation Results](https://huggingface.co/spaces/HuggingFaceH4/open_llm_leaderboard)
Detailed results can be found [here](https://huggingface.co/datasets/open-llm-leaderboard/details_pansophic__rocket-3B)
| Metric |Value|
|---------------------------------|----:|
|Avg. |55.77|
|AI2 Reasoning Challenge (25-Shot)|50.60|
|HellaSwag (10-Shot) |76.69|
|MMLU (5-Shot) |47.10|
|TruthfulQA (0-shot) |55.82|
|Winogrande (5-shot) |67.96|
|GSM8k (5-shot) |36.47|
## Intended uses & limitations
Initially, we fine-tuned the model using a dataset created by merging and curating multiple datasets, available on the HuggingFace Hub. This dataset will be released to the public soon. We further enhanced the model's performance using DPO, selecting samples from the [openbmb/UltraFeedback](https://huggingface.co/datasets/openbmb/UltraFeedback) and [BAAI/JudgeLM-100K](https://huggingface.co/datasets/BAAI/JudgeLM-100K) datasets. The outcome is a highly effective chat model with a 3 billion parameter scale.
## Input Format
The model is trained with the ChatML format:
```
<|im_start|>system
System message here.<|im_end|>
<|im_start|>user
Your message here!<|im_end|>
<|im_start|>assistant
```
Here's how you can run the model using 🤗 Transformers:
```python
import torch
from transformers import AutoModelForCausalLM, AutoTokenizer, TextStreamer
model = AutoModelForCausalLM.from_pretrained("pansophic/rocket-3B", trust_remote_code=True, torch_dtype=torch.bfloat16).to("cuda")
tokenizer = AutoTokenizer.from_pretrained("pansophic/rocket-3B", trust_remote_code=True, torch_dtype=torch.bfloat16)
streamer = TextStreamer(tokenizer)
prompt = """<|im_start|>system
{system}<|im_end|>
<|im_start|>user
{user}<|im_end|>
<|im_start|>assistant
"""
system = "You are a helpful assistant."
user = "How are you?"
# Apply the ChatML format
prompt = prompt.format(system=system, user=user)
# Tokenize the prompt
inputs = tokenizer(prompt, return_tensors="pt", return_attention_mask=False).to("cuda")
generated_text = model.generate(**inputs, max_length=3084, top_p=0.95, do_sample=True, temperature=0.7, use_cache=True, streamer=streamer)
# <|im_start|>system
# You are a chef who makes everything sound like a secret culinary masterpiece, even everyday meals.<|im_end|>
# <|im_start|>user
# How to cook an omelette?<|im_end|>
# <|im_start|>assistant
# Ah, the art of crafting the perfect omelette, a secret culinary masterpiece indeed.
# Begin by gently whisking two to three eggs in a mixing bowl, and then pour the silky liquid into a non-stick pan.
# Allow the eggs to dance and sizzle as you swiftly tilt the pan to spread the joy throughout the entire omelette universe.
# As the edges begin to set, fold the omelette in half with a gentle flourish, and you'll witness a stunning display of culinary prowess.
# Enjoy this enchanting creation, and you'll be transported to a world of secret culinary mastery.<|im_end|>
```
## Bias, Risks, and Limitations
Unlike ChatGPT, which incorporates in-the-loop filtering of responses and is aligned during the RLHF phase for safe completions, our model lacks these features. Consequently, it may generate problematic outputs, particularly when prompted in certain ways. Below is the score of the model on Toxigen benchmark.
The pretraining dataset is comprised of a filtered mixture of open-source large-scale datasets available on the [HuggingFace Hub](https://huggingface.co/datasets): Falcon RefinedWeb extract ([Penedo et al., 2023](https://huggingface.co/datasets/tiiuae/falcon-refinedweb)), RedPajama-Data ([Together Computer., 2023](https://github.com/togethercomputer/RedPajama-Data)) and The Pile ([Gao et al., 2020](https://arxiv.org/abs/2101.00027)) both without the *Books3* subset, and StarCoder ([Li et al., 2023](https://arxiv.org/abs/2305.06161)).
| Metric | Value |
|-----------------------|---------------------------|
| Toxigen (0-shot) | 43.40 |
**The model name is inspired by the small but formidable character from 'Guardians of the Galaxy'. Similar to its namesake, this model, with its 3 billion parameters, showcases remarkable efficiency and effectiveness, challenging larger models despite its smaller size."*
*Model card adapted from [Zephyr Beta](https://huggingface.co/HuggingFaceH4/zephyr-7b-beta/blob/main/README.md) and [Tulu-2-7B](https://huggingface.co/allenai/tulu-2-7b/blob/main/README.md)* |
Sharathhebbar24/SSH_355M | Sharathhebbar24 | "2024-03-14T07:35:13Z" | 959 | 0 | transformers | [
"transformers",
"safetensors",
"gpt2",
"text-generation",
"dataset:databricks/databricks-dolly-15k",
"dataset:gamino/wiki_medical_terms",
"dataset:Sharathhebbar24/openhermes",
"dataset:Sharathhebbar24/Open-Platypus",
"dataset:Sharathhebbar24/sql-create-context",
"dataset:Sharathhebbar24/Evol-Instruct-Code-80k-v1",
"dataset:Sharathhebbar24/BeaverTails_filtered",
"dataset:Sharathhebbar24/arxiv-math-instruct-50k",
"dataset:Sharathhebbar24/MetaMathQA",
"dataset:Intel/orca_dpo_pairs",
"license:apache-2.0",
"model-index",
"autotrain_compatible",
"endpoints_compatible",
"text-generation-inference",
"region:us"
] | text-generation | "2024-02-06T17:18:56Z" | ---
license: apache-2.0
datasets:
- databricks/databricks-dolly-15k
- gamino/wiki_medical_terms
- Sharathhebbar24/openhermes
- Sharathhebbar24/Open-Platypus
- Sharathhebbar24/sql-create-context
- Sharathhebbar24/Evol-Instruct-Code-80k-v1
- Sharathhebbar24/BeaverTails_filtered
- Sharathhebbar24/arxiv-math-instruct-50k
- Sharathhebbar24/MetaMathQA
- Intel/orca_dpo_pairs
model-index:
- name: SSH_300M
results:
- task:
type: text-generation
name: Text Generation
dataset:
name: AI2 Reasoning Challenge (25-Shot)
type: ai2_arc
config: ARC-Challenge
split: test
args:
num_few_shot: 25
metrics:
- type: acc_norm
value: 28.24
name: normalized accuracy
source:
url: https://huggingface.co/spaces/HuggingFaceH4/open_llm_leaderboard?query=Sharathhebbar24/SSH_300M
name: Open LLM Leaderboard
- task:
type: text-generation
name: Text Generation
dataset:
name: HellaSwag (10-Shot)
type: hellaswag
split: validation
args:
num_few_shot: 10
metrics:
- type: acc_norm
value: 38.74
name: normalized accuracy
source:
url: https://huggingface.co/spaces/HuggingFaceH4/open_llm_leaderboard?query=Sharathhebbar24/SSH_300M
name: Open LLM Leaderboard
- task:
type: text-generation
name: Text Generation
dataset:
name: MMLU (5-Shot)
type: cais/mmlu
config: all
split: test
args:
num_few_shot: 5
metrics:
- type: acc
value: 27.03
name: accuracy
source:
url: https://huggingface.co/spaces/HuggingFaceH4/open_llm_leaderboard?query=Sharathhebbar24/SSH_300M
name: Open LLM Leaderboard
- task:
type: text-generation
name: Text Generation
dataset:
name: TruthfulQA (0-shot)
type: truthful_qa
config: multiple_choice
split: validation
args:
num_few_shot: 0
metrics:
- type: mc2
value: 42.51
source:
url: https://huggingface.co/spaces/HuggingFaceH4/open_llm_leaderboard?query=Sharathhebbar24/SSH_300M
name: Open LLM Leaderboard
- task:
type: text-generation
name: Text Generation
dataset:
name: Winogrande (5-shot)
type: winogrande
config: winogrande_xl
split: validation
args:
num_few_shot: 5
metrics:
- type: acc
value: 53.67
name: accuracy
source:
url: https://huggingface.co/spaces/HuggingFaceH4/open_llm_leaderboard?query=Sharathhebbar24/SSH_300M
name: Open LLM Leaderboard
- task:
type: text-generation
name: Text Generation
dataset:
name: GSM8k (5-shot)
type: gsm8k
config: main
split: test
args:
num_few_shot: 5
metrics:
- type: acc
value: 0.3
name: accuracy
source:
url: https://huggingface.co/spaces/HuggingFaceH4/open_llm_leaderboard?query=Sharathhebbar24/SSH_300M
name: Open LLM Leaderboard
---
This model is a finetuned version of ```gpt2-medium```
## Model description
GPT-2 is a transformers model pre-trained on a very large corpus of English data in a self-supervised fashion. This
means it was pre-trained on the raw texts only, with no humans labeling them in any way (which is why it can use lots
of publicly available data) with an automatic process to generate inputs and labels from those texts. More precisely,
it was trained to guess the next word in sentences.
More precisely, inputs are sequences of continuous text of a certain length and the targets are the same sequence,
shifting one token (word or piece of word) to the right. The model uses a masking mechanism to make sure the
predictions for the token `i` only use the inputs from `1` to `i` but not the future tokens.
This way, the model learns an inner representation of the English language that can then be used to extract features
useful for downstream tasks. The model is best at what it was trained for, however, which is generating texts from a
prompt.
### To use this model
```python
>>> from transformers import AutoTokenizer, AutoModelForCausalLM
>>> model_name = "Sharathhebbar24/SSH_355M"
>>> model = AutoModelForCausalLM.from_pretrained(model_name)
>>> tokenizer = AutoTokenizer.from_pretrained(model_name)
>>> def generate_text(prompt):
>>> inputs = tokenizer.encode(prompt, return_tensors='pt')
>>> outputs = model.generate(inputs, max_length=64, pad_token_id=tokenizer.eos_token_id)
>>> generated = tokenizer.decode(outputs[0], skip_special_tokens=True)
>>> return generated[:generated.rfind(".")+1]
>>> generate_text("Should I Invest in stocks")
```
# [Open LLM Leaderboard Evaluation Results](https://huggingface.co/spaces/HuggingFaceH4/open_llm_leaderboard)
Detailed results can be found [here](https://huggingface.co/datasets/open-llm-leaderboard/details_Sharathhebbar24__SSH_300M)
| Metric |Value|
|---------------------------------|----:|
|Avg. |31.75|
|AI2 Reasoning Challenge (25-Shot)|28.24|
|HellaSwag (10-Shot) |38.74|
|MMLU (5-Shot) |27.03|
|TruthfulQA (0-shot) |42.51|
|Winogrande (5-shot) |53.67|
|GSM8k (5-shot) | 0.30|
|
timm/fastvit_ma36.apple_dist_in1k | timm | "2023-08-23T21:04:36Z" | 958 | 1 | timm | [
"timm",
"pytorch",
"safetensors",
"image-classification",
"dataset:imagenet-1k",
"arxiv:2303.14189",
"license:other",
"region:us"
] | image-classification | "2023-08-23T21:04:05Z" | ---
tags:
- image-classification
- timm
library_name: timm
license: other
datasets:
- imagenet-1k
---
# Model card for fastvit_ma36.apple_dist_in1k
A FastViT image classification model. Trained on ImageNet-1k with distillation by paper authors.
Please observe [original license](https://github.com/apple/ml-fastvit/blob/8af5928238cab99c45f64fc3e4e7b1516b8224ba/LICENSE).
## Model Details
- **Model Type:** Image classification / feature backbone
- **Model Stats:**
- Params (M): 44.1
- GMACs: 7.8
- Activations (M): 40.4
- Image size: 256 x 256
- **Papers:**
- FastViT: A Fast Hybrid Vision Transformer using Structural Reparameterization: https://arxiv.org/abs/2303.14189
- **Original:** https://github.com/apple/ml-fastvit
- **Dataset:** ImageNet-1k
## Model Usage
### Image Classification
```python
from urllib.request import urlopen
from PIL import Image
import timm
img = Image.open(urlopen(
'https://huggingface.co/datasets/huggingface/documentation-images/resolve/main/beignets-task-guide.png'
))
model = timm.create_model('fastvit_ma36.apple_dist_in1k', pretrained=True)
model = model.eval()
# get model specific transforms (normalization, resize)
data_config = timm.data.resolve_model_data_config(model)
transforms = timm.data.create_transform(**data_config, is_training=False)
output = model(transforms(img).unsqueeze(0)) # unsqueeze single image into batch of 1
top5_probabilities, top5_class_indices = torch.topk(output.softmax(dim=1) * 100, k=5)
```
### Feature Map Extraction
```python
from urllib.request import urlopen
from PIL import Image
import timm
img = Image.open(urlopen(
'https://huggingface.co/datasets/huggingface/documentation-images/resolve/main/beignets-task-guide.png'
))
model = timm.create_model(
'fastvit_ma36.apple_dist_in1k',
pretrained=True,
features_only=True,
)
model = model.eval()
# get model specific transforms (normalization, resize)
data_config = timm.data.resolve_model_data_config(model)
transforms = timm.data.create_transform(**data_config, is_training=False)
output = model(transforms(img).unsqueeze(0)) # unsqueeze single image into batch of 1
for o in output:
# print shape of each feature map in output
# e.g.:
# torch.Size([1, 76, 64, 64])
# torch.Size([1, 152, 32, 32])
# torch.Size([1, 304, 16, 16])
# torch.Size([1, 608, 8, 8])
print(o.shape)
```
### Image Embeddings
```python
from urllib.request import urlopen
from PIL import Image
import timm
img = Image.open(urlopen(
'https://huggingface.co/datasets/huggingface/documentation-images/resolve/main/beignets-task-guide.png'
))
model = timm.create_model(
'fastvit_ma36.apple_dist_in1k',
pretrained=True,
num_classes=0, # remove classifier nn.Linear
)
model = model.eval()
# get model specific transforms (normalization, resize)
data_config = timm.data.resolve_model_data_config(model)
transforms = timm.data.create_transform(**data_config, is_training=False)
output = model(transforms(img).unsqueeze(0)) # output is (batch_size, num_features) shaped tensor
# or equivalently (without needing to set num_classes=0)
output = model.forward_features(transforms(img).unsqueeze(0))
# output is unpooled, a (1, 608, 8, 8) shaped tensor
output = model.forward_head(output, pre_logits=True)
# output is a (1, num_features) shaped tensor
```
## Citation
```bibtex
@inproceedings{vasufastvit2023,
author = {Pavan Kumar Anasosalu Vasu and James Gabriel and Jeff Zhu and Oncel Tuzel and Anurag Ranjan},
title = {FastViT: A Fast Hybrid Vision Transformer using Structural Reparameterization},
booktitle={Proceedings of the IEEE/CVF International Conference on Computer Vision},
year = {2023}
}
```
|
M4-ai/Orca-2.0-Tau-1.8B | M4-ai | "2024-05-17T14:41:42Z" | 958 | 8 | transformers | [
"transformers",
"safetensors",
"qwen2",
"text-generation",
"conversational",
"en",
"dataset:Open-Orca/SlimOrca",
"dataset:m-a-p/Code-Feedback",
"dataset:MaziyarPanahi/WizardLM_evol_instruct_V2_196k",
"dataset:camel-ai/math",
"dataset:camel-ai/physics",
"dataset:camel-ai/biology",
"dataset:camel-ai/chemistry",
"dataset:LDJnr/Capybara",
"dataset:jondurbin/airoboros-3.2",
"dataset:microsoft/orca-math-word-problems-200k",
"license:other",
"model-index",
"autotrain_compatible",
"endpoints_compatible",
"text-generation-inference",
"region:us"
] | text-generation | "2024-05-11T08:54:34Z" | ---
language:
- en
license: other
library_name: transformers
datasets:
- Open-Orca/SlimOrca
- m-a-p/Code-Feedback
- MaziyarPanahi/WizardLM_evol_instruct_V2_196k
- camel-ai/math
- camel-ai/physics
- camel-ai/biology
- camel-ai/chemistry
- LDJnr/Capybara
- jondurbin/airoboros-3.2
- microsoft/orca-math-word-problems-200k
inference:
parameters:
do_sample: true
temperature: 0.8
top_p: 0.95
top_k: 40
max_new_tokens: 250
repetition_penalty: 1.1
model-index:
- name: Orca-2.0-Tau-1.8B
results:
- task:
type: text-generation
name: Text Generation
dataset:
name: AI2 Reasoning Challenge (25-Shot)
type: ai2_arc
config: ARC-Challenge
split: test
args:
num_few_shot: 25
metrics:
- type: acc_norm
value: 37.12
name: normalized accuracy
source:
url: https://huggingface.co/spaces/HuggingFaceH4/open_llm_leaderboard?query=M4-ai/Orca-2.0-Tau-1.8B
name: Open LLM Leaderboard
- task:
type: text-generation
name: Text Generation
dataset:
name: HellaSwag (10-Shot)
type: hellaswag
split: validation
args:
num_few_shot: 10
metrics:
- type: acc_norm
value: 61.13
name: normalized accuracy
source:
url: https://huggingface.co/spaces/HuggingFaceH4/open_llm_leaderboard?query=M4-ai/Orca-2.0-Tau-1.8B
name: Open LLM Leaderboard
- task:
type: text-generation
name: Text Generation
dataset:
name: MMLU (5-Shot)
type: cais/mmlu
config: all
split: test
args:
num_few_shot: 5
metrics:
- type: acc
value: 45.27
name: accuracy
source:
url: https://huggingface.co/spaces/HuggingFaceH4/open_llm_leaderboard?query=M4-ai/Orca-2.0-Tau-1.8B
name: Open LLM Leaderboard
- task:
type: text-generation
name: Text Generation
dataset:
name: TruthfulQA (0-shot)
type: truthful_qa
config: multiple_choice
split: validation
args:
num_few_shot: 0
metrics:
- type: mc2
value: 39.1
source:
url: https://huggingface.co/spaces/HuggingFaceH4/open_llm_leaderboard?query=M4-ai/Orca-2.0-Tau-1.8B
name: Open LLM Leaderboard
- task:
type: text-generation
name: Text Generation
dataset:
name: Winogrande (5-shot)
type: winogrande
config: winogrande_xl
split: validation
args:
num_few_shot: 5
metrics:
- type: acc
value: 59.59
name: accuracy
source:
url: https://huggingface.co/spaces/HuggingFaceH4/open_llm_leaderboard?query=M4-ai/Orca-2.0-Tau-1.8B
name: Open LLM Leaderboard
- task:
type: text-generation
name: Text Generation
dataset:
name: GSM8k (5-shot)
type: gsm8k
config: main
split: test
args:
num_few_shot: 5
metrics:
- type: acc
value: 28.96
name: accuracy
source:
url: https://huggingface.co/spaces/HuggingFaceH4/open_llm_leaderboard?query=M4-ai/Orca-2.0-Tau-1.8B
name: Open LLM Leaderboard
---
# Orca-2.0-Tau-1.8B
<!-- Provide a quick summary of what the model is/does. -->
We fine-tuned tau-1.8B on a high quality mix for general-purpose assistants. A DPO version of this will be released soon. We use the ChatML prompt format.
## Model Details
### Model Description
<!-- Provide a longer summary of what this model is. -->
This model has capabilities in math, coding, writing, and more. We fine-tuned it using a high quality mix for general-purpose assistants.
- **Developed by:** M4-ai
- **Language(s) (NLP):** English and maybe Chinese
- **License:** tongyi-qianwen license
- **Finetuned from model:** [tau-1.8B](https://huggingface.co/M4-ai/tau-1.8B)
## Uses
<!-- Address questions around how the model is intended to be used, including the foreseeable users of the model and those affected by the model. -->
General purpose assistant, question answering, chain-of-thought, etc..
### Recommendations
<!-- This section is meant to convey recommendations with respect to the bias, risk, and technical limitations. -->
Users (both direct and downstream) should be made aware of the risks, biases and limitations of the model. More information needed for further recommendations.
## Evaluation
Coming soon
## Training Details
### Training Data
- Open-Orca/SlimOrca
- m-a-p/Code-Feedback
- MaziyarPanahi/WizardLM_evol_instruct_V2_196k
- camel-ai/math
- camel-ai/physics
- camel-ai/biology
- camel-ai/chemistry
- LDJnr/Capybara
- jondurbin/airoboros-3.2
- microsoft/orca-math-word-problems-200k
## Evaluations
| Tasks |Version|Filter|n-shot| Metric |Value | |Stderr|
|---------------------------------|-------|------|-----:|--------|-----:|---|-----:|
|agieval_nous |N/A |none | 0|acc |0.2537|± |0.0086|
| | |none | 0|acc_norm|0.2474|± |0.0085|
| - agieval_aqua_rat | 1|none | 0|acc |0.2283|± |0.0264|
| | |none | 0|acc_norm|0.2441|± |0.0270|
| - agieval_logiqa_en | 1|none | 0|acc |0.2750|± |0.0175|
| | |none | 0|acc_norm|0.3164|± |0.0182|
| - agieval_lsat_ar | 1|none | 0|acc |0.2087|± |0.0269|
| | |none | 0|acc_norm|0.1739|± |0.0250|
| - agieval_lsat_lr | 1|none | 0|acc |0.1843|± |0.0172|
| | |none | 0|acc_norm|0.2353|± |0.0188|
| - agieval_lsat_rc | 1|none | 0|acc |0.2602|± |0.0268|
| | |none | 0|acc_norm|0.1784|± |0.0234|
| - agieval_sat_en | 1|none | 0|acc |0.3544|± |0.0334|
| | |none | 0|acc_norm|0.2961|± |0.0319|
| - agieval_sat_en_without_passage| 1|none | 0|acc |0.3107|± |0.0323|
| | |none | 0|acc_norm|0.2282|± |0.0293|
| - agieval_sat_math | 1|none | 0|acc |0.2727|± |0.0301|
| | |none | 0|acc_norm|0.2091|± |0.0275|
|truthfulqa_mc2 | 2|none | 0|acc |0.3923|± |0.0139|
#### Training Hyperparameters
- **Training regime:** bf16 non-mixed precision
## Technical Specifications
#### Hardware
We used 8 Kaggle TPUs, and we trained at a global batch size of 128 and sequence length of 2048.
# [Open LLM Leaderboard Evaluation Results](https://huggingface.co/spaces/HuggingFaceH4/open_llm_leaderboard)
Detailed results can be found [here](https://huggingface.co/datasets/open-llm-leaderboard/details_M4-ai__Orca-2.0-Tau-1.8B)
| Metric |Value|
|---------------------------------|----:|
|Avg. |45.20|
|AI2 Reasoning Challenge (25-Shot)|37.12|
|HellaSwag (10-Shot) |61.13|
|MMLU (5-Shot) |45.27|
|TruthfulQA (0-shot) |39.10|
|Winogrande (5-shot) |59.59|
|GSM8k (5-shot) |28.96|
|
airev-ai/Amal-70b | airev-ai | "2024-06-08T10:30:19Z" | 958 | 2 | transformers | [
"transformers",
"safetensors",
"llama",
"text-generation",
"conversational",
"license:other",
"autotrain_compatible",
"endpoints_compatible",
"text-generation-inference",
"region:us"
] | text-generation | "2024-06-05T00:00:09Z" | ---
license: other
license_name: airev-model-license
license_link: >-
https://huggingface.co/airev-ai/Jais-70b/blob/main/LICENSE
---
# Jais-Inception-70b
The AI model developed collaboratively by Airev and Inception stands as a cutting-edge solution, meticulously trained on a comprehensive synthetic Arabic dataset. This model leverages advanced machine learning techniques to achieve remarkable proficiency in understanding and processing Arabic language inputs. Its training on synthetic data ensures a diverse and robust learning foundation, enabling it to handle various linguistic nuances and complexities inherent to Arabic. The combined expertise of Airev and Inception has resulted in a highly capable model, designed to excel in a multitude of applications, ranging from natural language processing and machine translation to speech recognition and text analysis. This innovation represents a significant advancement in Arabic language AI, offering unparalleled accuracy and performance.
<img src="https://res.cloudinary.com/dcugtdlab/image/upload/v1717842568/hzsmhhu6cbrjoh8yh9iy.jpg" width="600" />
## Evals
- arc: 70.1
- gsm8k: 87.1
- hellaswag: 87.3
- mmlu: 78.2
- truthfulqa: 54.2
- winogrande: 84.1 |
Local-Novel-LLM-project/Ninja-V2-7B | Local-Novel-LLM-project | "2024-06-15T23:28:10Z" | 958 | 9 | transformers | [
"transformers",
"safetensors",
"gguf",
"mistral",
"text-generation",
"license:apache-2.0",
"autotrain_compatible",
"endpoints_compatible",
"text-generation-inference",
"region:us"
] | text-generation | "2024-06-10T04:05:21Z" | ---
license: apache-2.0
---
# Ninja-V2-7B
このモデルは、ベクトルマージなどを用い作成された高性能ベースモデルです。
用途はチャットのみならず、文章創作など幅広いタスクに対応できます。
このモデルは、ローカルLLMに向き合う会企画のLocalAIハッカソンの高性能GPUサーバーの助けを借りて作成されました。
関係者の皆様に感謝申し上げます。
# 作成方法
モデルレシピは下記の通りです。
Ninja-v2-Base (mergekitにて作成)
```yaml
models:
- model: MTSAIR/multi_verse_model
- model: HuggingFaceH4/zephyr-7b-beta
merge_method: model_stock
base_model: amazingvince/Not-WizardLM-2-7B
dtype: bfloat16
```
Novels-7B(ninja_mergerにて作成)
```yaml
target_model: "stabilityai/japanese-stablelm-instruct-gamma-7b"
-
left: "Elizezen/Phos-7B" # ベースモデルの指定
right: "stabilityai/japanese-stablelm-instruct-gamma-7b" # サブモデルの指定
operation: "sub" # 組み合わせの操作。"mix"、"add"などを指定
velocity: 1.0
-
left: "Elizezen/Antler-7B" # ベースモデルの指定
right: "stabilityai/japanese-stablelm-instruct-gamma-7b" # サブモデルの指定
operation: "sub" # 組み合わせの操作。"mix"、"add"などを指定
velocity: 1.0
```
Ninja-v2(ninja_mergerにて作成)
```yaml
target_model: "Ninja-v2-Base"
models: # 組み合わせの重み。0.0から1.0の範囲で指定
-
left: "NTQAI/chatntq-ja-7b-v1.0" # ベースモデルの指定
right: "mistralai/Mistral-7B-v0.1" # サブモデルの指定0
operation: "sub" # 組み合わせの操作。"mix"、"add"などを指定
velocity: 1.0
-
left: "Elizezen/Berghof-NSFW-7B" # ベースモデルの指定
right: "stabilityai/japanese-stablelm-instruct-gamma-7b" # サブモデルの指定
operation: "sub" # 組み合わせの操作。"mix"、"add"などを指定
velocity: 0.5
-
left: "Novels-7B" # ベースモデルの指定
right: "stabilityai/japanese-stablelm-instruct-gamma-7b" # サブモデルの指定
operation: "sub" # 組み合わせの操作。"mix"、"add"などを指定
velocity: 1.0
```
# プロンプトテンプレート
必須ではありませんが、Vicuna-1.1テンプレートを使用することができます。
単純な文章生成においては推奨のテンプレートはありません。
# システムプロンプト
- BAD: あなたは○○として振る舞います
- GOOD: あなたは○○です
- BAD: あなたは○○ができます
- GOOD: あなたは○○をします
# 制限
Apache-2.0ライセンスに従い使用してください。 |
Helsinki-NLP/opus-mt-az-en | Helsinki-NLP | "2023-11-28T09:49:38Z" | 957 | 1 | transformers | [
"transformers",
"pytorch",
"tf",
"safetensors",
"marian",
"text2text-generation",
"translation",
"az",
"en",
"license:apache-2.0",
"autotrain_compatible",
"endpoints_compatible",
"region:us"
] | translation | "2022-03-02T23:29:04Z" | ---
language:
- az
- en
tags:
- translation
license: apache-2.0
---
### aze-eng
* source group: Azerbaijani
* target group: English
* OPUS readme: [aze-eng](https://github.com/Helsinki-NLP/Tatoeba-Challenge/tree/master/models/aze-eng/README.md)
* model: transformer-align
* source language(s): aze_Latn
* target language(s): eng
* model: transformer-align
* pre-processing: normalization + SentencePiece (spm12k,spm12k)
* download original weights: [opus-2020-06-16.zip](https://object.pouta.csc.fi/Tatoeba-MT-models/aze-eng/opus-2020-06-16.zip)
* test set translations: [opus-2020-06-16.test.txt](https://object.pouta.csc.fi/Tatoeba-MT-models/aze-eng/opus-2020-06-16.test.txt)
* test set scores: [opus-2020-06-16.eval.txt](https://object.pouta.csc.fi/Tatoeba-MT-models/aze-eng/opus-2020-06-16.eval.txt)
## Benchmarks
| testset | BLEU | chr-F |
|-----------------------|-------|-------|
| Tatoeba-test.aze.eng | 31.9 | 0.490 |
### System Info:
- hf_name: aze-eng
- source_languages: aze
- target_languages: eng
- opus_readme_url: https://github.com/Helsinki-NLP/Tatoeba-Challenge/tree/master/models/aze-eng/README.md
- original_repo: Tatoeba-Challenge
- tags: ['translation']
- languages: ['az', 'en']
- src_constituents: {'aze_Latn'}
- tgt_constituents: {'eng'}
- src_multilingual: False
- tgt_multilingual: False
- prepro: normalization + SentencePiece (spm12k,spm12k)
- url_model: https://object.pouta.csc.fi/Tatoeba-MT-models/aze-eng/opus-2020-06-16.zip
- url_test_set: https://object.pouta.csc.fi/Tatoeba-MT-models/aze-eng/opus-2020-06-16.test.txt
- src_alpha3: aze
- tgt_alpha3: eng
- short_pair: az-en
- chrF2_score: 0.49
- bleu: 31.9
- brevity_penalty: 0.997
- ref_len: 16165.0
- src_name: Azerbaijani
- tgt_name: English
- train_date: 2020-06-16
- src_alpha2: az
- tgt_alpha2: en
- prefer_old: False
- long_pair: aze-eng
- helsinki_git_sha: 480fcbe0ee1bf4774bcbe6226ad9f58e63f6c535
- transformers_git_sha: 2207e5d8cb224e954a7cba69fa4ac2309e9ff30b
- port_machine: brutasse
- port_time: 2020-08-21-14:41 |
arbml/wav2vec2-large-xlsr-53-arabic-egyptian | arbml | "2021-07-05T18:12:38Z" | 957 | 8 | transformers | [
"transformers",
"pytorch",
"jax",
"wav2vec2",
"automatic-speech-recognition",
"audio",
"speech",
"xlsr-fine-tuning-week",
"dataset:common_voice",
"license:apache-2.0",
"model-index",
"endpoints_compatible",
"region:us"
] | automatic-speech-recognition | "2022-03-02T23:29:05Z" | ---
language: ???
datasets:
- common_voice
tags:
- audio
- automatic-speech-recognition
- speech
- xlsr-fine-tuning-week
license: apache-2.0
model-index:
- name: XLSR Wav2Vec2 Arabic Egyptian by Zaid
results:
- task:
name: Speech Recognition
type: automatic-speech-recognition
dataset:
name: Common Voice ???
type: common_voice
args: ???
metrics:
- name: Test WER
type: wer
value: ???
---
# Wav2Vec2-Large-XLSR-53-Tamil
Fine-tuned [facebook/wav2vec2-large-xlsr-53](https://huggingface.co/facebook/wav2vec2-large-xlsr-53) in Tamil using the [Common Voice](https://huggingface.co/datasets/common_voice)
When using this model, make sure that your speech input is sampled at 16kHz.
## Usage
The model can be used directly (without a language model) as follows:
```python
import torch
import torchaudio
from datasets import load_dataset
from transformers import Wav2Vec2ForCTC, Wav2Vec2Processor
test_dataset = load_dataset("common_voice", "???", split="test[:2%]").
processor = Wav2Vec2Processor.from_pretrained("Zaid/wav2vec2-large-xlsr-53-arabic-egyptian")
model = Wav2Vec2ForCTC.from_pretrained("Zaid/wav2vec2-large-xlsr-53-arabic-egyptian")
resampler = torchaudio.transforms.Resample(48_000, 16_000)
# Preprocessing the datasets.
# We need to read the aduio files as arrays
def speech_file_to_array_fn(batch):
speech_array, sampling_rate = torchaudio.load(batch["path"])
batch["speech"] = resampler(speech_array).squeeze().numpy()
return batch
test_dataset = test_dataset.map(speech_file_to_array_fn)
inputs = processor(test_dataset["speech"][:2], sampling_rate=16_000, return_tensors="pt", padding=True)
with torch.no_grad():
logits = model(inputs.input_values, attention_mask=inputs.attention_mask).logits
predicted_ids = torch.argmax(logits, dim=-1)
print("Prediction:", processor.batch_decode(predicted_ids))
print("Reference:", test_dataset["sentence"][:2])
```
## Evaluation
The model can be evaluated as follows on the {language} test data of Common Voice.
```python
import torch
import torchaudio
from datasets import load_dataset, load_metric
from transformers import Wav2Vec2ForCTC, Wav2Vec2Processor
import re
test_dataset = load_dataset("common_voice", "???", split="test")
wer = load_metric("wer")
processor = Wav2Vec2Processor.from_pretrained("Zaid/wav2vec2-large-xlsr-53-arabic-egyptian")
model = Wav2Vec2ForCTC.from_pretrained("Zaid/wav2vec2-large-xlsr-53-arabic-egyptian")
model.to("cuda")
chars_to_ignore_regex = '[\,\?\.\!\-\;\:\"\“]'
resampler = torchaudio.transforms.Resample(48_000, 16_000)
# Preprocessing the datasets.
# We need to read the aduio files as arrays
def speech_file_to_array_fn(batch):
batch["sentence"] = re.sub(chars_to_ignore_regex, '', batch["sentence"]).lower()
speech_array, sampling_rate = torchaudio.load(batch["path"])
batch["speech"] = resampler(speech_array).squeeze().numpy()
return batch
test_dataset = test_dataset.map(speech_file_to_array_fn)
# Preprocessing the datasets.
# We need to read the aduio files as arrays
def evaluate(batch):
inputs = processor(batch["speech"], sampling_rate=16_000, return_tensors="pt", padding=True)
with torch.no_grad():
logits = model(inputs.input_values.to("cuda"), attention_mask=inputs.attention_mask.to("cuda")).logits
pred_ids = torch.argmax(logits, dim=-1)
batch["pred_strings"] = processor.batch_decode(pred_ids)
return batch
result = test_dataset.map(evaluate, batched=True, batch_size=8)
print("WER: {:2f}".format(100 * wer.compute(predictions=result["pred_strings"], references=result["sentence"])))
```
**Test Result**: ??? %
## Training
The Common Voice `train`, `validation` datasets were used for training.
The script used for training can be found ??? |
ProdicusII/ZeroShotBioNER | ProdicusII | "2023-05-12T12:23:02Z" | 957 | 7 | transformers | [
"transformers",
"pytorch",
"bert",
"token-classification",
"biology",
"medical",
"zero-shot",
"few-shot",
"en",
"dataset:bigbio/chemdner",
"dataset:ncbi_disease",
"dataset:jnlpba",
"dataset:bigbio/n2c2_2018_track2",
"dataset:bigbio/bc5cdr",
"arxiv:2305.04928",
"license:mit",
"autotrain_compatible",
"endpoints_compatible",
"region:us"
] | token-classification | "2023-05-04T11:11:23Z" | ---
license: mit
datasets:
- bigbio/chemdner
- ncbi_disease
- jnlpba
- bigbio/n2c2_2018_track2
- bigbio/bc5cdr
widget:
- text: Drug<SEP>He was given aspirin and paracetamol.
language:
- en
metrics:
- precision
- recall
- f1
pipeline_tag: token-classification
tags:
- token-classification
- biology
- medical
- zero-shot
- few-shot
library_name: transformers
---
# Zero and few shot NER for biomedical texts
## Model description
This model was created during the research collaboration between Bayer Pharma and Serbian Institute for Artificial Intelligence Research and Development.
The model is trained on about 25+ biomedical NER classes and can perform also zero-shot inference and can be further fine-tuned for new classes with just few examples (few-shot learning).
For more details about our methods please see the paper named ["A transformer-based method for zero and few-shot biomedical named entity recognition"](https://arxiv.org/abs/2305.04928). The model corresponds to BioBERT-based mode, trained with 1 in the first segment (check paper for more details).
Model takes as input two strings. String1 is NER label that is being searched in second string. String1 must be phrase for entity. String2 is short text where String1 is searched for semantically.
model outputs list of zeros and ones corresponding to the occurance of Named Entity and corresponing to the tokens(tokens given by transformer tokenizer) of the Sring2.
## Example of usage
```python
from transformers import AutoTokenizer
from transformers import BertForTokenClassification
modelname = 'ProdicusII/ZeroShotBioNER' # modelpath
tokenizer = AutoTokenizer.from_pretrained(modelname) ## loading the tokenizer of that model
string1 = 'Drug'
string2 = 'No recent antibiotics or other nephrotoxins, and no symptoms of UTI with benign UA.'
encodings = tokenizer(string1, string2, is_split_into_words=False,
padding=True, truncation=True, add_special_tokens=True, return_offsets_mapping=False,
max_length=512, return_tensors='pt')
model0 = BertForTokenClassification.from_pretrained(modelname, num_labels=2)
prediction_logits = model0(**encodings)
print(prediction_logits)
```
## Example of fine-tuning with few-shot learning
In order to fine-tune model to the new entity using few shots, the dataset needs to be transformed to torch.utils.data.Dataset, containing BERT tokens and set of 0s and 1s (1 is where the class is positive and should be predicted as the member of given NER class). After the dataset is created, the following can be done (for more details, please have a look at the code at GitHub - https://github.com/br-ai-ns-institute/Zero-ShotNER):
```python
training_args = TrainingArguments(
output_dir=os.path.join('Results', class_unseen, str(j)+'Shot'), # folder for results
num_train_epochs=10, # number of epochs
per_device_train_batch_size=16, # batch size per device during training
per_device_eval_batch_size=16, # batch size for evaluation
weight_decay=0.01, # strength of weight decay
logging_dir=os.path.join('Logs', class_unseen, str(j)+'Shot'), # folder for logs
save_strategy='epoch',
evaluation_strategy='epoch',
load_best_model_at_end=True,
)
model0 = BertForTokenClassification.from_pretrained(model_path, num_labels=2)
trainer = Trainer(
model=model0, # pretrained model
args=training_args, # training artguments
train_dataset=dataset, # Object of class torch.utils.data.Dataset for training
eval_dataset=dataset_valid # Object of class torch.utils.data.Dataset for vaLidation
)
start_time = time.time()
trainer.train()
total_time = time.time()-start_time
model0_path = os.path.join('Results', class_unseen, str(j)+'Shot', 'Model')
os.makedirs(model0_path, exist_ok=True)
trainer.save_model(model0_path)
```
## Available classes
The following datasets and entities were used for training and therefore they can be used as label in the first segment (as a first string). Note that multiword string have been merged.
* NCBI
* Specific Disease
* Composite Mention
* Modifier
* Disease Class
* BIORED
* Sequence Variant
* Gene Or Gene Product
* Disease Or Phenotypic Feature
* Chemical Entity
* Cell Line
* Organism Taxon
* CDR
* Disease
* Chemical
* CHEMDNER
* Chemical
* Chemical Family
* JNLPBA
* Protein
* DNA
* Cell Type
* Cell Line
* RNA
* n2c2
* Drug
* Frequency
* Strength
* Dosage
* Form
* Reason
* Route
* ADE
* Duration
On top of this, one can use the model in zero-shot regime with other classes, and also fine-tune it with few examples of other classes.
## Code availibility
Code used for training and testing the model is available at https://github.com/br-ai-ns-institute/Zero-ShotNER
## Citation
If you use this model, or are inspired by it, please cite in your paper the following paper:
Košprdić M.,Prodanović N., Ljajić A., Bašaragin B., Milošević N., 2023. A transformer-based method for zero and few-shot biomedical named entity recognition. arXiv preprint arXiv:2305.04928. https://arxiv.org/abs/2305.04928
or in bibtex:
```
@misc{kosprdic2023transformerbased,
title={A transformer-based method for zero and few-shot biomedical named entity recognition},
author={Miloš Košprdić and Nikola Prodanović and Adela Ljajić and Bojana Bašaragin and Nikola Milošević},
year={2023},
eprint={2305.04928},
archivePrefix={arXiv},
primaryClass={cs.CL}
}
``` |
team-lucid/trocr-small-korean | team-lucid | "2023-07-01T08:41:35Z" | 957 | 11 | transformers | [
"transformers",
"pytorch",
"jax",
"safetensors",
"vision-encoder-decoder",
"trocr",
"image-to-text",
"ko",
"license:apache-2.0",
"endpoints_compatible",
"region:us"
] | image-to-text | "2023-06-30T16:00:43Z" | ---
license: apache-2.0
language:
- ko
pipeline_tag: image-to-text
tags:
- trocr
- vision-encoder-decoder
---
# trocr-small-korean
## Model Details
TrOCR은 Encoder-Decoder 모델로, 이미지 트랜스포머 인코더와 텍스트 트랜스포머 디코더로 이루어져 있습니다.
이미지 인코더는 DeiT 가중치로 초기화되었고, 텍스트 디코더는 자체적으로 학습한 RoBERTa 가중치로 초기화되었습니다.
이 연구는 구글의 TPU Research Cloud(TRC)를 통해 지원받은 Cloud TPU로 학습되었습니다.
## How to Get Started with the Model
```python
import torch
from transformers import VisionEncoderDecoderModel
model = VisionEncoderDecoderModel.from_pretrained("team-lucid/trocr-small-korean")
pixel_values = torch.rand(1, 3, 384, 384)
generated_ids = model.generate(pixel_values)
```
## Training Details
### Training Data
해당 모델은 [synthtiger](https://github.com/clovaai/synthtiger)로 합성된 6M개의 이미지로 학습되었습니다
### Training Hyperparameters
| Hyperparameter | Small |
|:--------------------|--------:|
| Warmup Steps | 4,000 |
| Learning Rates | 1e-4 |
| Batch Size | 512 |
| Weight Decay | 0.01 |
| Max Steps | 500,000 |
| Learning Rate Decay | 0.1 |
| \\(Adam\beta_1\\) | 0.9 |
| \\(Adam\beta_2\\) | 0.98 |
|
bartowski/Hermes-2-Pro-Mistral-10.7B-GGUF | bartowski | "2024-03-31T17:53:34Z" | 957 | 9 | transformers | [
"transformers",
"gguf",
"mergekit",
"merge",
"Mistral",
"instruct",
"finetune",
"chatml",
"DPO",
"RLHF",
"gpt4",
"synthetic data",
"distillation",
"function calling",
"json mode",
"text-generation",
"en",
"dataset:teknium/OpenHermes-2.5",
"base_model:mistralai/Mistral-7B-v0.1",
"license:apache-2.0",
"endpoints_compatible",
"region:us"
] | text-generation | "2024-03-31T17:35:27Z" | ---
base_model: mistralai/Mistral-7B-v0.1
library_name: transformers
tags:
- mergekit
- merge
- Mistral
- instruct
- finetune
- chatml
- DPO
- RLHF
- gpt4
- synthetic data
- distillation
- function calling
- json mode
model-index:
- name: Hermes-2-Pro-Mistral-10.7B
results: []
license: apache-2.0
language:
- en
datasets:
- teknium/OpenHermes-2.5
widget:
- example_title: Hermes 2 Pro
messages:
- role: system
content: You are a sentient, superintelligent artificial general intelligence, here to teach and assist me.
- role: user
content: Write a short story about Goku discovering kirby has teamed up with Majin Buu to destroy the world.
quantized_by: bartowski
pipeline_tag: text-generation
---
## Llamacpp Quantizations of Hermes-2-Pro-Mistral-10.7B
Using <a href="https://github.com/ggerganov/llama.cpp/">llama.cpp</a> release <a href="https://github.com/ggerganov/llama.cpp/releases/tag/b2536">b2536</a> for quantization.
Original model: https://huggingface.co/Joseph717171/Hermes-2-Pro-Mistral-10.7B
Download a file (not the whole branch) from below:
| Filename | Quant type | File Size | Description |
| -------- | ---------- | --------- | ----------- |
| [Hermes-2-Pro-Mistral-10.7B-Q8_0.gguf](https://huggingface.co/bartowski/Hermes-2-Pro-Mistral-10.7B-GGUF/blob/main/Hermes-2-Pro-Mistral-10.7B-Q8_0.gguf) | Q8_0 | 11.40GB | Extremely high quality, generally unneeded but max available quant. |
| [Hermes-2-Pro-Mistral-10.7B-Q6_K.gguf](https://huggingface.co/bartowski/Hermes-2-Pro-Mistral-10.7B-GGUF/blob/main/Hermes-2-Pro-Mistral-10.7B-Q6_K.gguf) | Q6_K | 8.80GB | Very high quality, near perfect, *recommended*. |
| [Hermes-2-Pro-Mistral-10.7B-Q5_K_M.gguf](https://huggingface.co/bartowski/Hermes-2-Pro-Mistral-10.7B-GGUF/blob/main/Hermes-2-Pro-Mistral-10.7B-Q5_K_M.gguf) | Q5_K_M | 7.59GB | High quality, very usable. |
| [Hermes-2-Pro-Mistral-10.7B-Q5_K_S.gguf](https://huggingface.co/bartowski/Hermes-2-Pro-Mistral-10.7B-GGUF/blob/main/Hermes-2-Pro-Mistral-10.7B-Q5_K_S.gguf) | Q5_K_S | 7.39GB | High quality, very usable. |
| [Hermes-2-Pro-Mistral-10.7B-Q5_0.gguf](https://huggingface.co/bartowski/Hermes-2-Pro-Mistral-10.7B-GGUF/blob/main/Hermes-2-Pro-Mistral-10.7B-Q5_0.gguf) | Q5_0 | 7.39GB | High quality, older format, generally not recommended. |
| [Hermes-2-Pro-Mistral-10.7B-Q4_K_M.gguf](https://huggingface.co/bartowski/Hermes-2-Pro-Mistral-10.7B-GGUF/blob/main/Hermes-2-Pro-Mistral-10.7B-Q4_K_M.gguf) | Q4_K_M | 6.46GB | Good quality, uses about 4.83 bits per weight. |
| [Hermes-2-Pro-Mistral-10.7B-Q4_K_S.gguf](https://huggingface.co/bartowski/Hermes-2-Pro-Mistral-10.7B-GGUF/blob/main/Hermes-2-Pro-Mistral-10.7B-Q4_K_S.gguf) | Q4_K_S | 6.11GB | Slightly lower quality with small space savings. |
| [Hermes-2-Pro-Mistral-10.7B-IQ4_NL.gguf](https://huggingface.co/bartowski/Hermes-2-Pro-Mistral-10.7B-GGUF/blob/main/Hermes-2-Pro-Mistral-10.7B-IQ4_NL.gguf) | IQ4_NL | 6.14GB | Decent quality, similar to Q4_K_S, new method of quanting, |
| [Hermes-2-Pro-Mistral-10.7B-IQ4_XS.gguf](https://huggingface.co/bartowski/Hermes-2-Pro-Mistral-10.7B-GGUF/blob/main/Hermes-2-Pro-Mistral-10.7B-IQ4_XS.gguf) | IQ4_XS | 5.82GB | Decent quality, new method with similar performance to Q4. |
| [Hermes-2-Pro-Mistral-10.7B-Q4_0.gguf](https://huggingface.co/bartowski/Hermes-2-Pro-Mistral-10.7B-GGUF/blob/main/Hermes-2-Pro-Mistral-10.7B-Q4_0.gguf) | Q4_0 | 6.07GB | Decent quality, older format, generally not recommended. |
| [Hermes-2-Pro-Mistral-10.7B-Q3_K_L.gguf](https://huggingface.co/bartowski/Hermes-2-Pro-Mistral-10.7B-GGUF/blob/main/Hermes-2-Pro-Mistral-10.7B-Q3_K_L.gguf) | Q3_K_L | 5.65GB | Lower quality but usable, good for low RAM availability. |
| [Hermes-2-Pro-Mistral-10.7B-Q3_K_M.gguf](https://huggingface.co/bartowski/Hermes-2-Pro-Mistral-10.7B-GGUF/blob/main/Hermes-2-Pro-Mistral-10.7B-Q3_K_M.gguf) | Q3_K_M | 5.19GB | Even lower quality. |
| [Hermes-2-Pro-Mistral-10.7B-IQ3_M.gguf](https://huggingface.co/bartowski/Hermes-2-Pro-Mistral-10.7B-GGUF/blob/main/Hermes-2-Pro-Mistral-10.7B-IQ3_M.gguf) | IQ3_M | 4.84GB | Medium-low quality, new method with decent performance. |
| [Hermes-2-Pro-Mistral-10.7B-IQ3_S.gguf](https://huggingface.co/bartowski/Hermes-2-Pro-Mistral-10.7B-GGUF/blob/main/Hermes-2-Pro-Mistral-10.7B-IQ3_S.gguf) | IQ3_S | 4.69GB | Lower quality, new method with decent performance, recommended over Q3 quants. |
| [Hermes-2-Pro-Mistral-10.7B-Q3_K_S.gguf](https://huggingface.co/bartowski/Hermes-2-Pro-Mistral-10.7B-GGUF/blob/main/Hermes-2-Pro-Mistral-10.7B-Q3_K_S.gguf) | Q3_K_S | 4.66GB | Low quality, not recommended. |
| [Hermes-2-Pro-Mistral-10.7B-Q2_K.gguf](https://huggingface.co/bartowski/Hermes-2-Pro-Mistral-10.7B-GGUF/blob/main/Hermes-2-Pro-Mistral-10.7B-Q2_K.gguf) | Q2_K | 4.00GB | Extremely low quality, *not* recommended.
Want to support my work? Visit my ko-fi page here: https://ko-fi.com/bartowski
|
sethuiyer/Medichat-Llama3-8B | sethuiyer | "2024-06-02T08:18:10Z" | 957 | 12 | transformers | [
"transformers",
"safetensors",
"llama",
"text-generation",
"mergekit",
"merge",
"medical",
"conversational",
"en",
"dataset:mlabonne/orpo-dpo-mix-40k",
"dataset:Open-Orca/SlimOrca-Dedup",
"dataset:jondurbin/airoboros-3.2",
"dataset:microsoft/orca-math-word-problems-200k",
"dataset:m-a-p/Code-Feedback",
"dataset:MaziyarPanahi/WizardLM_evol_instruct_V2_196k",
"dataset:ruslanmv/ai-medical-chatbot",
"base_model:Undi95/Llama-3-Unholy-8B",
"base_model:Locutusque/llama-3-neural-chat-v1-8b",
"base_model:ruslanmv/Medical-Llama3-8B-16bit",
"license:other",
"model-index",
"autotrain_compatible",
"endpoints_compatible",
"text-generation-inference",
"region:us"
] | text-generation | "2024-04-22T05:07:13Z" | ---
base_model:
- Undi95/Llama-3-Unholy-8B
- Locutusque/llama-3-neural-chat-v1-8b
- ruslanmv/Medical-Llama3-8B-16bit
library_name: transformers
tags:
- mergekit
- merge
- medical
license: other
datasets:
- mlabonne/orpo-dpo-mix-40k
- Open-Orca/SlimOrca-Dedup
- jondurbin/airoboros-3.2
- microsoft/orca-math-word-problems-200k
- m-a-p/Code-Feedback
- MaziyarPanahi/WizardLM_evol_instruct_V2_196k
- ruslanmv/ai-medical-chatbot
model-index:
- name: Medichat-Llama3-8B
results:
- task:
type: text-generation
name: Text Generation
dataset:
name: AI2 Reasoning Challenge (25-Shot)
type: ai2_arc
config: ARC-Challenge
split: test
args:
num_few_shot: 25
metrics:
- type: acc_norm
value: 59.13
name: normalized accuracy
source:
url: >-
https://huggingface.co/spaces/HuggingFaceH4/open_llm_leaderboard?query=sethuiyer/Medichat-Llama3-8B
name: Open LLM Leaderboard
- task:
type: text-generation
name: Text Generation
dataset:
name: HellaSwag (10-Shot)
type: hellaswag
split: validation
args:
num_few_shot: 10
metrics:
- type: acc_norm
value: 82.9
name: normalized accuracy
source:
url: >-
https://huggingface.co/spaces/HuggingFaceH4/open_llm_leaderboard?query=sethuiyer/Medichat-Llama3-8B
name: Open LLM Leaderboard
- task:
type: text-generation
name: Text Generation
dataset:
name: MMLU (5-Shot)
type: cais/mmlu
config: all
split: test
args:
num_few_shot: 5
metrics:
- type: acc
value: 60.35
name: accuracy
source:
url: >-
https://huggingface.co/spaces/HuggingFaceH4/open_llm_leaderboard?query=sethuiyer/Medichat-Llama3-8B
name: Open LLM Leaderboard
- task:
type: text-generation
name: Text Generation
dataset:
name: TruthfulQA (0-shot)
type: truthful_qa
config: multiple_choice
split: validation
args:
num_few_shot: 0
metrics:
- type: mc2
value: 49.65
source:
url: >-
https://huggingface.co/spaces/HuggingFaceH4/open_llm_leaderboard?query=sethuiyer/Medichat-Llama3-8B
name: Open LLM Leaderboard
- task:
type: text-generation
name: Text Generation
dataset:
name: Winogrande (5-shot)
type: winogrande
config: winogrande_xl
split: validation
args:
num_few_shot: 5
metrics:
- type: acc
value: 78.93
name: accuracy
source:
url: >-
https://huggingface.co/spaces/HuggingFaceH4/open_llm_leaderboard?query=sethuiyer/Medichat-Llama3-8B
name: Open LLM Leaderboard
- task:
type: text-generation
name: Text Generation
dataset:
name: GSM8k (5-shot)
type: gsm8k
config: main
split: test
args:
num_few_shot: 5
metrics:
- type: acc
value: 60.35
name: accuracy
source:
url: >-
https://huggingface.co/spaces/HuggingFaceH4/open_llm_leaderboard?query=sethuiyer/Medichat-Llama3-8B
name: Open LLM Leaderboard
language:
- en
---
### Medichat-Llama3-8B
Built upon the powerful LLaMa-3 architecture and fine-tuned on an extensive dataset of health information, this model leverages its vast medical knowledge to offer clear, comprehensive answers.
This model is generally better for accurate and informative responses, particularly for users seeking in-depth medical advice.
The following YAML configuration was used to produce this model:
```yaml
models:
- model: Undi95/Llama-3-Unholy-8B
parameters:
weight: [0.25, 0.35, 0.45, 0.35, 0.25]
density: [0.1, 0.25, 0.5, 0.25, 0.1]
- model: Locutusque/llama-3-neural-chat-v1-8b
- model: ruslanmv/Medical-Llama3-8B-16bit
parameters:
weight: [0.55, 0.45, 0.35, 0.45, 0.55]
density: [0.1, 0.25, 0.5, 0.25, 0.1]
merge_method: dare_ties
base_model: Locutusque/llama-3-neural-chat-v1-8b
parameters:
int8_mask: true
dtype: bfloat16
```
# Comparision Against Dr.Samantha 7B
| Subject | Medichat-Llama3-8B Accuracy (%) | Dr. Samantha Accuracy (%) |
|-------------------------|---------------------------------|---------------------------|
| Clinical Knowledge | 71.70 | 52.83 |
| Medical Genetics | 78.00 | 49.00 |
| Human Aging | 70.40 | 58.29 |
| Human Sexuality | 73.28 | 55.73 |
| College Medicine | 62.43 | 38.73 |
| Anatomy | 64.44 | 41.48 |
| College Biology | 72.22 | 52.08 |
| High School Biology | 77.10 | 53.23 |
| Professional Medicine | 63.97 | 38.73 |
| Nutrition | 73.86 | 50.33 |
| Professional Psychology | 68.95 | 46.57 |
| Virology | 54.22 | 41.57 |
| High School Psychology | 83.67 | 66.60 |
| **Average** | **70.33** | **48.85** |
The current model demonstrates a substantial improvement over the previous [Dr. Samantha](sethuiyer/Dr_Samantha-7b) model in terms of subject-specific knowledge and accuracy.
### Usage:
```python
import torch
from transformers import AutoTokenizer, AutoModelForCausalLM
class MedicalAssistant:
def __init__(self, model_name="sethuiyer/Medichat-Llama3-8B", device="cuda"):
self.device = device
self.tokenizer = AutoTokenizer.from_pretrained(model_name)
self.model = AutoModelForCausalLM.from_pretrained(model_name).to(self.device)
self.sys_message = '''
You are an AI Medical Assistant trained on a vast dataset of health information. Please be thorough and
provide an informative answer. If you don't know the answer to a specific medical inquiry, advise seeking professional help.
'''
def format_prompt(self, question):
messages = [
{"role": "system", "content": self.sys_message},
{"role": "user", "content": question}
]
prompt = self.tokenizer.apply_chat_template(messages, tokenize=False, add_generation_prompt=True)
return prompt
def generate_response(self, question, max_new_tokens=512):
prompt = self.format_prompt(question)
inputs = self.tokenizer(prompt, return_tensors="pt").to(self.device)
with torch.no_grad():
outputs = self.model.generate(**inputs, max_new_tokens=max_new_tokens, use_cache=True)
answer = self.tokenizer.batch_decode(outputs, skip_special_tokens=True)[0].strip()
return answer
if __name__ == "__main__":
assistant = MedicalAssistant()
question = '''
Symptoms:
Dizziness, headache, and nausea.
What is the differential diagnosis?
'''
response = assistant.generate_response(question)
print(response)
```
## Quants
Thanks to [Quant Factory](https://huggingface.co/QuantFactory), the quantized version of this model is available at [QuantFactory/Medichat-Llama3-8B-GGUF](https://huggingface.co/QuantFactory/Medichat-Llama3-8B-GGUF),
## Ollama
This model is now also available on Ollama. You can use it by running the command ```ollama run monotykamary/medichat-llama3``` in your
terminal. If you have limited computing resources, check out this [video](https://www.youtube.com/watch?v=Qa1h7ygwQq8) to learn how to run it on
a Google Colab backend. |
Tanvir1337/BanglaLLama-3-8b-BnWiki-Base-Q5_K_M-GGUF | Tanvir1337 | "2024-06-25T21:33:32Z" | 957 | 0 | null | [
"gguf",
"bangla",
"large language model",
"llama-cpp",
"gguf-my-repo",
"bn",
"en",
"dataset:wikimedia/wikipedia",
"base_model:BanglaLLM/BanglaLLama-3-8b-BnWiki-Base",
"license:llama3",
"region:us"
] | null | "2024-06-25T21:33:05Z" | ---
base_model: BanglaLLM/BanglaLLama-3-8b-BnWiki-Base
datasets:
- wikimedia/wikipedia
language:
- bn
- en
license: llama3
tags:
- bangla
- large language model
- llama-cpp
- gguf-my-repo
---
# Tanvir1337/BanglaLLama-3-8b-BnWiki-Base-Q5_K_M-GGUF
This model was converted to GGUF format from [`BanglaLLM/BanglaLLama-3-8b-BnWiki-Base`](https://huggingface.co/BanglaLLM/BanglaLLama-3-8b-BnWiki-Base) using llama.cpp via the ggml.ai's [GGUF-my-repo](https://huggingface.co/spaces/ggml-org/gguf-my-repo) space.
Refer to the [original model card](https://huggingface.co/BanglaLLM/BanglaLLama-3-8b-BnWiki-Base) for more details on the model.
## Use with llama.cpp
Install llama.cpp through brew (works on Mac and Linux)
```bash
brew install llama.cpp
```
Invoke the llama.cpp server or the CLI.
### CLI:
```bash
llama-cli --hf-repo Tanvir1337/BanglaLLama-3-8b-BnWiki-Base-Q5_K_M-GGUF --hf-file banglallama-3-8b-bnwiki-base-q5_k_m.gguf -p "The meaning to life and the universe is"
```
### Server:
```bash
llama-server --hf-repo Tanvir1337/BanglaLLama-3-8b-BnWiki-Base-Q5_K_M-GGUF --hf-file banglallama-3-8b-bnwiki-base-q5_k_m.gguf -c 2048
```
Note: You can also use this checkpoint directly through the [usage steps](https://github.com/ggerganov/llama.cpp?tab=readme-ov-file#usage) listed in the Llama.cpp repo as well.
Step 1: Clone llama.cpp from GitHub.
```
git clone https://github.com/ggerganov/llama.cpp
```
Step 2: Move into the llama.cpp folder and build it with `LLAMA_CURL=1` flag along with other hardware-specific flags (for ex: LLAMA_CUDA=1 for Nvidia GPUs on Linux).
```
cd llama.cpp && LLAMA_CURL=1 make
```
Step 3: Run inference through the main binary.
```
./llama-cli --hf-repo Tanvir1337/BanglaLLama-3-8b-BnWiki-Base-Q5_K_M-GGUF --hf-file banglallama-3-8b-bnwiki-base-q5_k_m.gguf -p "The meaning to life and the universe is"
```
or
```
./llama-server --hf-repo Tanvir1337/BanglaLLama-3-8b-BnWiki-Base-Q5_K_M-GGUF --hf-file banglallama-3-8b-bnwiki-base-q5_k_m.gguf -c 2048
```
|
gaianet/llm-compiler-7b-ftd-GGUF | gaianet | "2024-06-29T11:00:12Z" | 957 | 0 | transformers | [
"transformers",
"gguf",
"llama",
"text-generation",
"code",
"base_model:facebook/llm-compiler-7b-ftd",
"license:other",
"autotrain_compatible",
"text-generation-inference",
"region:us"
] | text-generation | "2024-06-29T10:24:35Z" | ---
language:
- code
license: other
model_name: llm-compiler-7b-ftd
base_model: facebook/llm-compiler-7b-ftd
inference: false
model_creator: facebook
quantized_by: Second State Inc.
---

# llm-compiler-7b-ftd-GGUF
## Original Model
[facebook/llm-compiler-7b-ftd](https://huggingface.co/facebook/llm-compiler-7b-ftd)
**License** A custom commercial license is available at: [https://ai.meta.com/resources/models-and-libraries/llama-downloads/](https://ai.meta.com/resources/models-and-libraries/llama-downloads/)
## Run with Gaianet
(coming soon)
<!-- **Prompt template:**
prompt template: `gemma-instruct`
**Context size:**
chat_ctx_size: `8192` -->
**Run with GaiaNet:**
- Quick start: https://docs.gaianet.ai/node-guide/quick-start
- Customize your node: https://docs.gaianet.ai/node-guide/customize
*Quantized with llama.cpp b3259*
|
timm/swin_small_patch4_window7_224.ms_in1k | timm | "2024-02-10T23:31:40Z" | 956 | 0 | timm | [
"timm",
"pytorch",
"safetensors",
"image-classification",
"dataset:imagenet-1k",
"arxiv:2103.14030",
"license:mit",
"region:us"
] | image-classification | "2023-03-18T04:13:39Z" | ---
license: mit
library_name: timm
tags:
- image-classification
- timm
datasets:
- imagenet-1k
---
# Model card for swin_small_patch4_window7_224.ms_in1k
A Swin Transformer image classification model. Pretrained on ImageNet-1k by paper authors.
## Model Details
- **Model Type:** Image classification / feature backbone
- **Model Stats:**
- Params (M): 49.6
- GMACs: 8.8
- Activations (M): 27.5
- Image size: 224 x 224
- **Papers:**
- Swin Transformer: Hierarchical Vision Transformer using Shifted Windows: https://arxiv.org/abs/2103.14030
- **Original:** https://github.com/microsoft/Swin-Transformer
- **Dataset:** ImageNet-1k
## Model Usage
### Image Classification
```python
from urllib.request import urlopen
from PIL import Image
import timm
img = Image.open(urlopen(
'https://huggingface.co/datasets/huggingface/documentation-images/resolve/main/beignets-task-guide.png'
))
model = timm.create_model('swin_small_patch4_window7_224.ms_in1k', pretrained=True)
model = model.eval()
# get model specific transforms (normalization, resize)
data_config = timm.data.resolve_model_data_config(model)
transforms = timm.data.create_transform(**data_config, is_training=False)
output = model(transforms(img).unsqueeze(0)) # unsqueeze single image into batch of 1
top5_probabilities, top5_class_indices = torch.topk(output.softmax(dim=1) * 100, k=5)
```
### Feature Map Extraction
```python
from urllib.request import urlopen
from PIL import Image
import timm
img = Image.open(urlopen(
'https://huggingface.co/datasets/huggingface/documentation-images/resolve/main/beignets-task-guide.png'
))
model = timm.create_model(
'swin_small_patch4_window7_224.ms_in1k',
pretrained=True,
features_only=True,
)
model = model.eval()
# get model specific transforms (normalization, resize)
data_config = timm.data.resolve_model_data_config(model)
transforms = timm.data.create_transform(**data_config, is_training=False)
output = model(transforms(img).unsqueeze(0)) # unsqueeze single image into batch of 1
for o in output:
# print shape of each feature map in output
# e.g. for swin_base_patch4_window7_224 (NHWC output)
# torch.Size([1, 56, 56, 128])
# torch.Size([1, 28, 28, 256])
# torch.Size([1, 14, 14, 512])
# torch.Size([1, 7, 7, 1024])
# e.g. for swinv2_cr_small_ns_224 (NCHW output)
# torch.Size([1, 96, 56, 56])
# torch.Size([1, 192, 28, 28])
# torch.Size([1, 384, 14, 14])
# torch.Size([1, 768, 7, 7])
print(o.shape)
```
### Image Embeddings
```python
from urllib.request import urlopen
from PIL import Image
import timm
img = Image.open(urlopen(
'https://huggingface.co/datasets/huggingface/documentation-images/resolve/main/beignets-task-guide.png'
))
model = timm.create_model(
'swin_small_patch4_window7_224.ms_in1k',
pretrained=True,
num_classes=0, # remove classifier nn.Linear
)
model = model.eval()
# get model specific transforms (normalization, resize)
data_config = timm.data.resolve_model_data_config(model)
transforms = timm.data.create_transform(**data_config, is_training=False)
output = model(transforms(img).unsqueeze(0)) # output is (batch_size, num_features) shaped tensor
# or equivalently (without needing to set num_classes=0)
output = model.forward_features(transforms(img).unsqueeze(0))
# output is unpooled (ie.e a (batch_size, H, W, num_features) tensor for swin / swinv2
# or (batch_size, num_features, H, W) for swinv2_cr
output = model.forward_head(output, pre_logits=True)
# output is (batch_size, num_features) tensor
```
## Model Comparison
Explore the dataset and runtime metrics of this model in timm [model results](https://github.com/huggingface/pytorch-image-models/tree/main/results).
## Citation
```bibtex
@inproceedings{liu2021Swin,
title={Swin Transformer: Hierarchical Vision Transformer using Shifted Windows},
author={Liu, Ze and Lin, Yutong and Cao, Yue and Hu, Han and Wei, Yixuan and Zhang, Zheng and Lin, Stephen and Guo, Baining},
booktitle={Proceedings of the IEEE/CVF International Conference on Computer Vision (ICCV)},
year={2021}
}
```
```bibtex
@misc{rw2019timm,
author = {Ross Wightman},
title = {PyTorch Image Models},
year = {2019},
publisher = {GitHub},
journal = {GitHub repository},
doi = {10.5281/zenodo.4414861},
howpublished = {\url{https://github.com/huggingface/pytorch-image-models}}
}
```
|
ChrisWilson011016/5EwDgRL2z3wSUt56mZcYE5iSDWKGXbsWnzQ67tb7TE6AC6Mz_vgg | ChrisWilson011016 | "2024-03-04T18:58:08Z" | 956 | 0 | keras | [
"keras",
"region:us"
] | null | "2024-02-24T15:25:50Z" | Entry not found |
isalia99/detr-resnet-50-sku110k | isalia99 | "2024-03-18T08:35:15Z" | 956 | 0 | transformers | [
"transformers",
"safetensors",
"detr",
"object-detection",
"vision",
"dataset:sku110k",
"license:apache-2.0",
"endpoints_compatible",
"region:us"
] | object-detection | "2024-03-14T15:12:44Z" | ---
license: apache-2.0
tags:
- object-detection
- vision
datasets:
- sku110k
widget:
- src: >-
https://github.com/Isalia20/DETR-finetune/blob/main/IMG_3507.jpg?raw=true
example_title: StoreExample(Not from SKU110K Dataset)
---
# DETR (End-to-End Object Detection) model with ResNet-50 backbone trained on SKU110K Dataset with 400 num_queries
DEtection TRansformer (DETR) model trained end-to-end on SKU110K object detection (8k annotated images) dataset. Main difference compared to the original model is it having **400** num_queries and it being pretrained on SKU110K dataset.
### How to use
Here is how to use this model:
```python
from transformers import DetrImageProcessor, DetrForObjectDetection
import torch
from PIL import Image, ImageOps
import requests
url = "https://github.com/Isalia20/DETR-finetune/blob/main/IMG_3507.jpg?raw=true"
image = Image.open(requests.get(url, stream=True).raw)
image = ImageOps.exif_transpose(image)
# you can specify the revision tag if you don't want the timm dependency
processor = DetrImageProcessor.from_pretrained("facebook/detr-resnet-50", revision="no_timm")
model = DetrForObjectDetection.from_pretrained("isalia99/detr-resnet-50-sku110k")
model = model.eval()
inputs = processor(images=image, return_tensors="pt")
outputs = model(**inputs)
# convert outputs (bounding boxes and class logits) to COCO API
# let's only keep detections with score > 0.8
target_sizes = torch.tensor([image.size[::-1]])
results = processor.post_process_object_detection(outputs, target_sizes=target_sizes, threshold=0.8)[0]
for score, label, box in zip(results["scores"], results["labels"], results["boxes"]):
box = [round(i, 2) for i in box.tolist()]
print(
f"Detected {model.config.id2label[label.item()]} with confidence "
f"{round(score.item(), 3)} at location {box}"
)
```
This should output:
```
Detected LABEL_1 with confidence 0.983 at location [665.49, 480.05, 708.15, 650.11]
Detected LABEL_1 with confidence 0.938 at location [204.99, 1405.9, 239.9, 1546.5]
...
Detected LABEL_1 with confidence 0.998 at location [772.85, 169.49, 829.67, 372.18]
Detected LABEL_1 with confidence 0.999 at location [828.28, 1475.16, 874.37, 1593.43]
```
Currently, both the feature extractor and model support PyTorch.
## Training data
The DETR model was trained on [SKU110K Dataset](https://github.com/eg4000/SKU110K_CVPR19), a dataset consisting of **8,219/588/2,936** annotated images for training/validation/test respectively.
## Training procedure
### Training
The model was trained for 140 epochs on 1 RTX 4060 Ti GPU(Finetuning decoder only) with batch size of 8 and 70 epochs(finetuning the whole network) with batch size of 3 and accumulating gradients for 3 steps.
## Evaluation results
This model achieves an mAP of **58.9** on SKU110k validation set. Result was calculated with torchmetrics MeanAveragePrecision class.
## Training Code
Code is released in this repository [Repo Link](https://github.com/Isalia20/DETR-finetune/tree/main). However it's not finalized/tested well yet but the main stuff is in the code. |
kunkun666/kunkun_dat_llama_13b_alpaca | kunkun666 | "2024-04-23T03:28:40Z" | 956 | 0 | transformers | [
"transformers",
"safetensors",
"llama",
"text-generation",
"conversational",
"license:apache-2.0",
"autotrain_compatible",
"endpoints_compatible",
"text-generation-inference",
"region:us"
] | text-generation | "2024-04-22T14:43:42Z" | ---
license: apache-2.0
---
# Model Card for Model ID
<!-- Provide a quick summary of what the model is/does. -->
This modelcard aims to be a base template for new models. It has been generated using [this raw template](https://github.com/huggingface/huggingface_hub/blob/main/src/huggingface_hub/templates/modelcard_template.md?plain=1).
## Model Details
### Model Description
<!-- Provide a longer summary of what this model is. -->
- **Developed by:** [More Information Needed]
- **Funded by [optional]:** [More Information Needed]
- **Shared by [optional]:** [More Information Needed]
- **Model type:** [More Information Needed]
- **Language(s) (NLP):** [More Information Needed]
- **License:** [More Information Needed]
- **Finetuned from model [optional]:** [More Information Needed]
### Model Sources [optional]
<!-- Provide the basic links for the model. -->
- **Repository:** [More Information Needed]
- **Paper [optional]:** [More Information Needed]
- **Demo [optional]:** [More Information Needed] |
SakanaAI/DiscoPOP-zephyr-7b-gemma | SakanaAI | "2024-06-13T01:39:47Z" | 956 | 25 | transformers | [
"transformers",
"safetensors",
"gemma",
"text-generation",
"alignment-handbook",
"generated_from_trainer",
"conversational",
"dataset:argilla/dpo-mix-7k",
"arxiv:2406.08414",
"base_model:HuggingFaceH4/zephyr-7b-gemma-sft-v0.1",
"license:gemma",
"autotrain_compatible",
"endpoints_compatible",
"text-generation-inference",
"region:us"
] | text-generation | "2024-06-12T01:34:24Z" | ---
license: gemma
base_model: HuggingFaceH4/zephyr-7b-gemma-sft-v0.1
tags:
- alignment-handbook
- generated_from_trainer
datasets:
- argilla/dpo-mix-7k
model-index:
- name: DiscoPOP-zephyr-7b-gemma
results: []
---
# DiscoPOP-zephyr-7b-gemma
This model is a fine-tuned version of [HuggingFaceH4/zephyr-7b-gemma-sft-v0.1](https://huggingface.co/HuggingFaceH4/zephyr-7b-gemma-sft-v0.1) on the argilla/dpo-mix-7k dataset.
This model is from the paper ["Discovering Preference Optimization Algorithms with and for Large Language Models"](https://arxiv.org/abs/2406.08414)
Read the [blog post on it here!](https://sakana.ai/llm-squared)
See the codebase to generate it here: [https://github.com/SakanaAI/DiscoPOP](https://github.com/SakanaAI/DiscoPOP)
## Model description
This model is identical in training to [HuggingFaceH4/zephyr-7b-gemma-v0.1](https://huggingface.co/HuggingFaceH4/zephyr-7b-gemma-v0.1), except instead of using Direct Preference Optimization (DPO), it uses DiscoPOP.
DiscoPOP is our Discovered Preference Optimization algorithm, which is defined as follows:
```
def log_ratio_modulated_loss(
self,
policy_chosen_logps: torch.FloatTensor,
policy_rejected_logps: torch.FloatTensor,
reference_chosen_logps: torch.FloatTensor,
reference_rejected_logps: torch.FloatTensor,
) -> torch.FloatTensor:
pi_logratios = policy_chosen_logps - policy_rejected_logps
ref_logratios = reference_chosen_logps - reference_rejected_logps
logits = pi_logratios - ref_logratios
# Modulate the mixing coefficient based on the log ratio magnitudes
log_ratio_modulation = torch.sigmoid(logits)
logistic_component = -F.logsigmoid(self.beta * logits)
exp_component = torch.exp(-self.beta * logits)
# Blend between logistic and exponential component based on log ratio modulation
losses = logistic_component * (1 - log_ratio_modulation) + exp_component * log_ratio_modulation
return losses
```
### Training hyperparameters
The following hyperparameters were used during training:
- learning_rate: 5e-07
- train_batch_size: 2
- eval_batch_size: 4
- seed: 42
- distributed_type: multi-GPU
- num_devices: 8
- gradient_accumulation_steps: 8
- total_train_batch_size: 128
- total_eval_batch_size: 32
- optimizer: Adam with betas=(0.9,0.999) and epsilon=1e-08
- lr_scheduler_type: cosine
- lr_scheduler_warmup_ratio: 0.1
- num_epochs: 2
### Framework versions
- Transformers 4.40.1
- Pytorch 2.1.2+cu121
- Datasets 2.19.0
- Tokenizers 0.19.1
|
timm/convit_small.fb_in1k | timm | "2023-04-24T04:14:51Z" | 955 | 0 | timm | [
"timm",
"pytorch",
"safetensors",
"image-classification",
"dataset:imagenet-1k",
"arxiv:2103.10697",
"license:apache-2.0",
"region:us"
] | image-classification | "2023-04-24T04:14:33Z" | ---
tags:
- image-classification
- timm
library_name: timm
license: apache-2.0
datasets:
- imagenet-1k
---
# Model card for convit_small.fb_in1k
A ConViT image classification model. Trained on ImageNet-1k by paper authors.
## Model Details
- **Model Type:** Image classification / feature backbone
- **Model Stats:**
- Params (M): 27.8
- GMACs: 5.8
- Activations (M): 17.9
- Image size: 224 x 224
- **Papers:**
- ConViT: Improving Vision Transformers with Soft Convolutional Inductive Biases: https://arxiv.org/abs/2103.10697
- **Dataset:** ImageNet-1k
- **Original:** https://github.com/facebookresearch/convit
## Model Usage
### Image Classification
```python
from urllib.request import urlopen
from PIL import Image
import timm
img = Image.open(urlopen(
'https://huggingface.co/datasets/huggingface/documentation-images/resolve/main/beignets-task-guide.png'
))
model = timm.create_model('convit_small.fb_in1k', pretrained=True)
model = model.eval()
# get model specific transforms (normalization, resize)
data_config = timm.data.resolve_model_data_config(model)
transforms = timm.data.create_transform(**data_config, is_training=False)
output = model(transforms(img).unsqueeze(0)) # unsqueeze single image into batch of 1
top5_probabilities, top5_class_indices = torch.topk(output.softmax(dim=1) * 100, k=5)
```
### Image Embeddings
```python
from urllib.request import urlopen
from PIL import Image
import timm
img = Image.open(urlopen(
'https://huggingface.co/datasets/huggingface/documentation-images/resolve/main/beignets-task-guide.png'
))
model = timm.create_model(
'convit_small.fb_in1k',
pretrained=True,
num_classes=0, # remove classifier nn.Linear
)
model = model.eval()
# get model specific transforms (normalization, resize)
data_config = timm.data.resolve_model_data_config(model)
transforms = timm.data.create_transform(**data_config, is_training=False)
output = model(transforms(img).unsqueeze(0)) # output is (batch_size, num_features) shaped tensor
# or equivalently (without needing to set num_classes=0)
output = model.forward_features(transforms(img).unsqueeze(0))
# output is unpooled, a (1, 197, 432) shaped tensor
output = model.forward_head(output, pre_logits=True)
# output is a (1, num_features) shaped tensor
```
## Model Comparison
Explore the dataset and runtime metrics of this model in timm [model results](https://github.com/huggingface/pytorch-image-models/tree/main/results).
## Citation
```bibtex
@article{d2021convit,
title={ConViT: Improving Vision Transformers with Soft Convolutional Inductive Biases},
author={d'Ascoli, St{'e}phane and Touvron, Hugo and Leavitt, Matthew and Morcos, Ari and Biroli, Giulio and Sagun, Levent},
journal={arXiv preprint arXiv:2103.10697},
year={2021}
}
```
|
TheBloke/DaringMaid-20B-GGUF | TheBloke | "2023-12-20T21:14:45Z" | 955 | 15 | transformers | [
"transformers",
"gguf",
"llama",
"text-generation",
"en",
"base_model:Kooten/DaringMaid-20B",
"license:cc-by-nc-4.0",
"text-generation-inference",
"region:us"
] | text-generation | "2023-12-20T21:04:04Z" | ---
base_model: Kooten/DaringMaid-20B
inference: false
language:
- en
license: cc-by-nc-4.0
model_creator: Kooten
model_name: DaringMaid 20B
model_type: llama
pipeline_tag: text-generation
prompt_template: 'Below is an instruction that describes a task. Write a response
that appropriately completes the request.
### Instruction:
{prompt}
### Response:
'
quantized_by: TheBloke
---
<!-- markdownlint-disable MD041 -->
<!-- header start -->
<!-- 200823 -->
<div style="width: auto; margin-left: auto; margin-right: auto">
<img src="https://i.imgur.com/EBdldam.jpg" alt="TheBlokeAI" style="width: 100%; min-width: 400px; display: block; margin: auto;">
</div>
<div style="display: flex; justify-content: space-between; width: 100%;">
<div style="display: flex; flex-direction: column; align-items: flex-start;">
<p style="margin-top: 0.5em; margin-bottom: 0em;"><a href="https://discord.gg/theblokeai">Chat & support: TheBloke's Discord server</a></p>
</div>
<div style="display: flex; flex-direction: column; align-items: flex-end;">
<p style="margin-top: 0.5em; margin-bottom: 0em;"><a href="https://www.patreon.com/TheBlokeAI">Want to contribute? TheBloke's Patreon page</a></p>
</div>
</div>
<div style="text-align:center; margin-top: 0em; margin-bottom: 0em"><p style="margin-top: 0.25em; margin-bottom: 0em;">TheBloke's LLM work is generously supported by a grant from <a href="https://a16z.com">andreessen horowitz (a16z)</a></p></div>
<hr style="margin-top: 1.0em; margin-bottom: 1.0em;">
<!-- header end -->
# DaringMaid 20B - GGUF
- Model creator: [Kooten](https://huggingface.co/Kooten)
- Original model: [DaringMaid 20B](https://huggingface.co/Kooten/DaringMaid-20B)
<!-- description start -->
## Description
This repo contains GGUF format model files for [Kooten's DaringMaid 20B](https://huggingface.co/Kooten/DaringMaid-20B).
These files were quantised using hardware kindly provided by [Massed Compute](https://massedcompute.com/).
<!-- description end -->
<!-- README_GGUF.md-about-gguf start -->
### About GGUF
GGUF is a new format introduced by the llama.cpp team on August 21st 2023. It is a replacement for GGML, which is no longer supported by llama.cpp.
Here is an incomplete list of clients and libraries that are known to support GGUF:
* [llama.cpp](https://github.com/ggerganov/llama.cpp). The source project for GGUF. Offers a CLI and a server option.
* [text-generation-webui](https://github.com/oobabooga/text-generation-webui), the most widely used web UI, with many features and powerful extensions. Supports GPU acceleration.
* [KoboldCpp](https://github.com/LostRuins/koboldcpp), a fully featured web UI, with GPU accel across all platforms and GPU architectures. Especially good for story telling.
* [GPT4All](https://gpt4all.io/index.html), a free and open source local running GUI, supporting Windows, Linux and macOS with full GPU accel.
* [LM Studio](https://lmstudio.ai/), an easy-to-use and powerful local GUI for Windows and macOS (Silicon), with GPU acceleration. Linux available, in beta as of 27/11/2023.
* [LoLLMS Web UI](https://github.com/ParisNeo/lollms-webui), a great web UI with many interesting and unique features, including a full model library for easy model selection.
* [Faraday.dev](https://faraday.dev/), an attractive and easy to use character-based chat GUI for Windows and macOS (both Silicon and Intel), with GPU acceleration.
* [llama-cpp-python](https://github.com/abetlen/llama-cpp-python), a Python library with GPU accel, LangChain support, and OpenAI-compatible API server.
* [candle](https://github.com/huggingface/candle), a Rust ML framework with a focus on performance, including GPU support, and ease of use.
* [ctransformers](https://github.com/marella/ctransformers), a Python library with GPU accel, LangChain support, and OpenAI-compatible AI server. Note, as of time of writing (November 27th 2023), ctransformers has not been updated in a long time and does not support many recent models.
<!-- README_GGUF.md-about-gguf end -->
<!-- repositories-available start -->
## Repositories available
* [AWQ model(s) for GPU inference.](https://huggingface.co/TheBloke/DaringMaid-20B-AWQ)
* [GPTQ models for GPU inference, with multiple quantisation parameter options.](https://huggingface.co/TheBloke/DaringMaid-20B-GPTQ)
* [2, 3, 4, 5, 6 and 8-bit GGUF models for CPU+GPU inference](https://huggingface.co/TheBloke/DaringMaid-20B-GGUF)
* [Kooten's original unquantised fp16 model in pytorch format, for GPU inference and for further conversions](https://huggingface.co/Kooten/DaringMaid-20B)
<!-- repositories-available end -->
<!-- prompt-template start -->
## Prompt template: Alpaca
```
Below is an instruction that describes a task. Write a response that appropriately completes the request.
### Instruction:
{prompt}
### Response:
```
<!-- prompt-template end -->
<!-- licensing start -->
## Licensing
The creator of the source model has listed its license as `cc-by-nc-4.0`, and this quantization has therefore used that same license.
As this model is based on Llama 2, it is also subject to the Meta Llama 2 license terms, and the license files for that are additionally included. It should therefore be considered as being claimed to be licensed under both licenses. I contacted Hugging Face for clarification on dual licensing but they do not yet have an official position. Should this change, or should Meta provide any feedback on this situation, I will update this section accordingly.
In the meantime, any questions regarding licensing, and in particular how these two licenses might interact, should be directed to the original model repository: [Kooten's DaringMaid 20B](https://huggingface.co/Kooten/DaringMaid-20B).
<!-- licensing end -->
<!-- compatibility_gguf start -->
## Compatibility
These quantised GGUFv2 files are compatible with llama.cpp from August 27th onwards, as of commit [d0cee0d](https://github.com/ggerganov/llama.cpp/commit/d0cee0d36d5be95a0d9088b674dbb27354107221)
They are also compatible with many third party UIs and libraries - please see the list at the top of this README.
## Explanation of quantisation methods
<details>
<summary>Click to see details</summary>
The new methods available are:
* GGML_TYPE_Q2_K - "type-1" 2-bit quantization in super-blocks containing 16 blocks, each block having 16 weight. Block scales and mins are quantized with 4 bits. This ends up effectively using 2.5625 bits per weight (bpw)
* GGML_TYPE_Q3_K - "type-0" 3-bit quantization in super-blocks containing 16 blocks, each block having 16 weights. Scales are quantized with 6 bits. This end up using 3.4375 bpw.
* GGML_TYPE_Q4_K - "type-1" 4-bit quantization in super-blocks containing 8 blocks, each block having 32 weights. Scales and mins are quantized with 6 bits. This ends up using 4.5 bpw.
* GGML_TYPE_Q5_K - "type-1" 5-bit quantization. Same super-block structure as GGML_TYPE_Q4_K resulting in 5.5 bpw
* GGML_TYPE_Q6_K - "type-0" 6-bit quantization. Super-blocks with 16 blocks, each block having 16 weights. Scales are quantized with 8 bits. This ends up using 6.5625 bpw
Refer to the Provided Files table below to see what files use which methods, and how.
</details>
<!-- compatibility_gguf end -->
<!-- README_GGUF.md-provided-files start -->
## Provided files
| Name | Quant method | Bits | Size | Max RAM required | Use case |
| ---- | ---- | ---- | ---- | ---- | ----- |
| [daringmaid-20b.Q2_K.gguf](https://huggingface.co/TheBloke/DaringMaid-20B-GGUF/blob/main/daringmaid-20b.Q2_K.gguf) | Q2_K | 2 | 8.31 GB| 10.81 GB | smallest, significant quality loss - not recommended for most purposes |
| [daringmaid-20b.Q3_K_S.gguf](https://huggingface.co/TheBloke/DaringMaid-20B-GGUF/blob/main/daringmaid-20b.Q3_K_S.gguf) | Q3_K_S | 3 | 8.66 GB| 11.16 GB | very small, high quality loss |
| [daringmaid-20b.Q3_K_M.gguf](https://huggingface.co/TheBloke/DaringMaid-20B-GGUF/blob/main/daringmaid-20b.Q3_K_M.gguf) | Q3_K_M | 3 | 9.70 GB| 12.20 GB | very small, high quality loss |
| [daringmaid-20b.Q3_K_L.gguf](https://huggingface.co/TheBloke/DaringMaid-20B-GGUF/blob/main/daringmaid-20b.Q3_K_L.gguf) | Q3_K_L | 3 | 10.63 GB| 13.13 GB | small, substantial quality loss |
| [daringmaid-20b.Q4_0.gguf](https://huggingface.co/TheBloke/DaringMaid-20B-GGUF/blob/main/daringmaid-20b.Q4_0.gguf) | Q4_0 | 4 | 11.29 GB| 13.79 GB | legacy; small, very high quality loss - prefer using Q3_K_M |
| [daringmaid-20b.Q4_K_S.gguf](https://huggingface.co/TheBloke/DaringMaid-20B-GGUF/blob/main/daringmaid-20b.Q4_K_S.gguf) | Q4_K_S | 4 | 11.34 GB| 13.84 GB | small, greater quality loss |
| [daringmaid-20b.Q4_K_M.gguf](https://huggingface.co/TheBloke/DaringMaid-20B-GGUF/blob/main/daringmaid-20b.Q4_K_M.gguf) | Q4_K_M | 4 | 12.04 GB| 14.54 GB | medium, balanced quality - recommended |
| [daringmaid-20b.Q5_0.gguf](https://huggingface.co/TheBloke/DaringMaid-20B-GGUF/blob/main/daringmaid-20b.Q5_0.gguf) | Q5_0 | 5 | 13.77 GB| 16.27 GB | legacy; medium, balanced quality - prefer using Q4_K_M |
| [daringmaid-20b.Q5_K_S.gguf](https://huggingface.co/TheBloke/DaringMaid-20B-GGUF/blob/main/daringmaid-20b.Q5_K_S.gguf) | Q5_K_S | 5 | 13.77 GB| 16.27 GB | large, low quality loss - recommended |
| [daringmaid-20b.Q5_K_M.gguf](https://huggingface.co/TheBloke/DaringMaid-20B-GGUF/blob/main/daringmaid-20b.Q5_K_M.gguf) | Q5_K_M | 5 | 14.16 GB| 16.66 GB | large, very low quality loss - recommended |
| [daringmaid-20b.Q6_K.gguf](https://huggingface.co/TheBloke/DaringMaid-20B-GGUF/blob/main/daringmaid-20b.Q6_K.gguf) | Q6_K | 6 | 16.41 GB| 18.91 GB | very large, extremely low quality loss |
| [daringmaid-20b.Q8_0.gguf](https://huggingface.co/TheBloke/DaringMaid-20B-GGUF/blob/main/daringmaid-20b.Q8_0.gguf) | Q8_0 | 8 | 21.25 GB| 23.75 GB | very large, extremely low quality loss - not recommended |
**Note**: the above RAM figures assume no GPU offloading. If layers are offloaded to the GPU, this will reduce RAM usage and use VRAM instead.
<!-- README_GGUF.md-provided-files end -->
<!-- README_GGUF.md-how-to-download start -->
## How to download GGUF files
**Note for manual downloaders:** You almost never want to clone the entire repo! Multiple different quantisation formats are provided, and most users only want to pick and download a single file.
The following clients/libraries will automatically download models for you, providing a list of available models to choose from:
* LM Studio
* LoLLMS Web UI
* Faraday.dev
### In `text-generation-webui`
Under Download Model, you can enter the model repo: TheBloke/DaringMaid-20B-GGUF and below it, a specific filename to download, such as: daringmaid-20b.Q4_K_M.gguf.
Then click Download.
### On the command line, including multiple files at once
I recommend using the `huggingface-hub` Python library:
```shell
pip3 install huggingface-hub
```
Then you can download any individual model file to the current directory, at high speed, with a command like this:
```shell
huggingface-cli download TheBloke/DaringMaid-20B-GGUF daringmaid-20b.Q4_K_M.gguf --local-dir . --local-dir-use-symlinks False
```
<details>
<summary>More advanced huggingface-cli download usage (click to read)</summary>
You can also download multiple files at once with a pattern:
```shell
huggingface-cli download TheBloke/DaringMaid-20B-GGUF --local-dir . --local-dir-use-symlinks False --include='*Q4_K*gguf'
```
For more documentation on downloading with `huggingface-cli`, please see: [HF -> Hub Python Library -> Download files -> Download from the CLI](https://huggingface.co/docs/huggingface_hub/guides/download#download-from-the-cli).
To accelerate downloads on fast connections (1Gbit/s or higher), install `hf_transfer`:
```shell
pip3 install hf_transfer
```
And set environment variable `HF_HUB_ENABLE_HF_TRANSFER` to `1`:
```shell
HF_HUB_ENABLE_HF_TRANSFER=1 huggingface-cli download TheBloke/DaringMaid-20B-GGUF daringmaid-20b.Q4_K_M.gguf --local-dir . --local-dir-use-symlinks False
```
Windows Command Line users: You can set the environment variable by running `set HF_HUB_ENABLE_HF_TRANSFER=1` before the download command.
</details>
<!-- README_GGUF.md-how-to-download end -->
<!-- README_GGUF.md-how-to-run start -->
## Example `llama.cpp` command
Make sure you are using `llama.cpp` from commit [d0cee0d](https://github.com/ggerganov/llama.cpp/commit/d0cee0d36d5be95a0d9088b674dbb27354107221) or later.
```shell
./main -ngl 35 -m daringmaid-20b.Q4_K_M.gguf --color -c 4096 --temp 0.7 --repeat_penalty 1.1 -n -1 -p "Below is an instruction that describes a task. Write a response that appropriately completes the request.\n\n### Instruction:\n{prompt}\n\n### Response:"
```
Change `-ngl 32` to the number of layers to offload to GPU. Remove it if you don't have GPU acceleration.
Change `-c 4096` to the desired sequence length. For extended sequence models - eg 8K, 16K, 32K - the necessary RoPE scaling parameters are read from the GGUF file and set by llama.cpp automatically. Note that longer sequence lengths require much more resources, so you may need to reduce this value.
If you want to have a chat-style conversation, replace the `-p <PROMPT>` argument with `-i -ins`
For other parameters and how to use them, please refer to [the llama.cpp documentation](https://github.com/ggerganov/llama.cpp/blob/master/examples/main/README.md)
## How to run in `text-generation-webui`
Further instructions can be found in the text-generation-webui documentation, here: [text-generation-webui/docs/04 ‐ Model Tab.md](https://github.com/oobabooga/text-generation-webui/blob/main/docs/04%20%E2%80%90%20Model%20Tab.md#llamacpp).
## How to run from Python code
You can use GGUF models from Python using the [llama-cpp-python](https://github.com/abetlen/llama-cpp-python) or [ctransformers](https://github.com/marella/ctransformers) libraries. Note that at the time of writing (Nov 27th 2023), ctransformers has not been updated for some time and is not compatible with some recent models. Therefore I recommend you use llama-cpp-python.
### How to load this model in Python code, using llama-cpp-python
For full documentation, please see: [llama-cpp-python docs](https://abetlen.github.io/llama-cpp-python/).
#### First install the package
Run one of the following commands, according to your system:
```shell
# Base ctransformers with no GPU acceleration
pip install llama-cpp-python
# With NVidia CUDA acceleration
CMAKE_ARGS="-DLLAMA_CUBLAS=on" pip install llama-cpp-python
# Or with OpenBLAS acceleration
CMAKE_ARGS="-DLLAMA_BLAS=ON -DLLAMA_BLAS_VENDOR=OpenBLAS" pip install llama-cpp-python
# Or with CLBLast acceleration
CMAKE_ARGS="-DLLAMA_CLBLAST=on" pip install llama-cpp-python
# Or with AMD ROCm GPU acceleration (Linux only)
CMAKE_ARGS="-DLLAMA_HIPBLAS=on" pip install llama-cpp-python
# Or with Metal GPU acceleration for macOS systems only
CMAKE_ARGS="-DLLAMA_METAL=on" pip install llama-cpp-python
# In windows, to set the variables CMAKE_ARGS in PowerShell, follow this format; eg for NVidia CUDA:
$env:CMAKE_ARGS = "-DLLAMA_OPENBLAS=on"
pip install llama-cpp-python
```
#### Simple llama-cpp-python example code
```python
from llama_cpp import Llama
# Set gpu_layers to the number of layers to offload to GPU. Set to 0 if no GPU acceleration is available on your system.
llm = Llama(
model_path="./daringmaid-20b.Q4_K_M.gguf", # Download the model file first
n_ctx=4096, # The max sequence length to use - note that longer sequence lengths require much more resources
n_threads=8, # The number of CPU threads to use, tailor to your system and the resulting performance
n_gpu_layers=35 # The number of layers to offload to GPU, if you have GPU acceleration available
)
# Simple inference example
output = llm(
"Below is an instruction that describes a task. Write a response that appropriately completes the request.\n\n### Instruction:\n{prompt}\n\n### Response:", # Prompt
max_tokens=512, # Generate up to 512 tokens
stop=["</s>"], # Example stop token - not necessarily correct for this specific model! Please check before using.
echo=True # Whether to echo the prompt
)
# Chat Completion API
llm = Llama(model_path="./daringmaid-20b.Q4_K_M.gguf", chat_format="llama-2") # Set chat_format according to the model you are using
llm.create_chat_completion(
messages = [
{"role": "system", "content": "You are a story writing assistant."},
{
"role": "user",
"content": "Write a story about llamas."
}
]
)
```
## How to use with LangChain
Here are guides on using llama-cpp-python and ctransformers with LangChain:
* [LangChain + llama-cpp-python](https://python.langchain.com/docs/integrations/llms/llamacpp)
* [LangChain + ctransformers](https://python.langchain.com/docs/integrations/providers/ctransformers)
<!-- README_GGUF.md-how-to-run end -->
<!-- footer start -->
<!-- 200823 -->
## Discord
For further support, and discussions on these models and AI in general, join us at:
[TheBloke AI's Discord server](https://discord.gg/theblokeai)
## Thanks, and how to contribute
Thanks to the [chirper.ai](https://chirper.ai) team!
Thanks to Clay from [gpus.llm-utils.org](llm-utils)!
I've had a lot of people ask if they can contribute. I enjoy providing models and helping people, and would love to be able to spend even more time doing it, as well as expanding into new projects like fine tuning/training.
If you're able and willing to contribute it will be most gratefully received and will help me to keep providing more models, and to start work on new AI projects.
Donaters will get priority support on any and all AI/LLM/model questions and requests, access to a private Discord room, plus other benefits.
* Patreon: https://patreon.com/TheBlokeAI
* Ko-Fi: https://ko-fi.com/TheBlokeAI
**Special thanks to**: Aemon Algiz.
**Patreon special mentions**: Michael Levine, 阿明, Trailburnt, Nikolai Manek, John Detwiler, Randy H, Will Dee, Sebastain Graf, NimbleBox.ai, Eugene Pentland, Emad Mostaque, Ai Maven, Jim Angel, Jeff Scroggin, Michael Davis, Manuel Alberto Morcote, Stephen Murray, Robert, Justin Joy, Luke @flexchar, Brandon Frisco, Elijah Stavena, S_X, Dan Guido, Undi ., Komninos Chatzipapas, Shadi, theTransient, Lone Striker, Raven Klaugh, jjj, Cap'n Zoog, Michel-Marie MAUDET (LINAGORA), Matthew Berman, David, Fen Risland, Omer Bin Jawed, Luke Pendergrass, Kalila, OG, Erik Bjäreholt, Rooh Singh, Joseph William Delisle, Dan Lewis, TL, John Villwock, AzureBlack, Brad, Pedro Madruga, Caitlyn Gatomon, K, jinyuan sun, Mano Prime, Alex, Jeffrey Morgan, Alicia Loh, Illia Dulskyi, Chadd, transmissions 11, fincy, Rainer Wilmers, ReadyPlayerEmma, knownsqashed, Mandus, biorpg, Deo Leter, Brandon Phillips, SuperWojo, Sean Connelly, Iucharbius, Jack West, Harry Royden McLaughlin, Nicholas, terasurfer, Vitor Caleffi, Duane Dunston, Johann-Peter Hartmann, David Ziegler, Olakabola, Ken Nordquist, Trenton Dambrowitz, Tom X Nguyen, Vadim, Ajan Kanaga, Leonard Tan, Clay Pascal, Alexandros Triantafyllidis, JM33133, Xule, vamX, ya boyyy, subjectnull, Talal Aujan, Alps Aficionado, wassieverse, Ari Malik, James Bentley, Woland, Spencer Kim, Michael Dempsey, Fred von Graf, Elle, zynix, William Richards, Stanislav Ovsiannikov, Edmond Seymore, Jonathan Leane, Martin Kemka, usrbinkat, Enrico Ros
Thank you to all my generous patrons and donaters!
And thank you again to a16z for their generous grant.
<!-- footer end -->
<!-- original-model-card start -->
# Original model card: Kooten's DaringMaid 20B
# DaringMaid-20B
My goal was to make a Noromaid that's smarter and better at following instructions.
After trying a bunch of different recipes I think this one turned out pretty good
- I used [sequelbox/DynamicFactor](https://huggingface.co/sequelbox/DynamicFactor) as a base to as its supposed "improve overall knowledge, precise communication, conceptual understanding, and technical skill" over the base llama2.
- [NeverSleep/Noromaid](https://huggingface.co/NeverSleep/Noromaid-13b-v0.1.1) of course.
- [Undi95/Utopia](https://huggingface.co/Undi95/Utopia-13B) has been recommended again recently and its still really good so in the mixer it goes
- I liked [tavtav/Rose](https://huggingface.co/tavtav/Rose-20B) so i threw in a bit of [CalderaAI/Thorns](https://huggingface.co/CalderaAI/13B-Thorns-l2)
- There was recently a model that tried to pass itself off as [Gryphe/MythoMax](https://huggingface.co/Gryphe/MythoMax-L2-13b), i made a merge with that model before it was revealed to be MythoMax and it turned out pretty good so i used it.
The .yml config files for mergekit with the exact merges can be found in the ["Recipe"](https://huggingface.co/Kooten/DaringMaid/tree/main/Recipe) folder in the [fp16 repo](https://huggingface.co/Kooten/DaringMaid-20B)
# Quants
EXL2: [6bpw](https://huggingface.co/Kooten/DaringMaid-20B-6bpw-exl2), [3bpw](https://huggingface.co/Kooten/DaringMaid-20B-3bpw-exl2)
[GGUF](https://huggingface.co/Kooten/DaringMaid-20B-GGUF):
[Q3_K_M](https://huggingface.co/Kooten/DaringMaid-20B-GGUF/blob/main/DaringMaid-20B-Q3_K_M.gguf) - [Q4_K_M](https://huggingface.co/Kooten/DaringMaid-20B-GGUF/blob/main/DaringMaid-20B-Q4_K_M.gguf) - [Q5_K_M](https://huggingface.co/Kooten/DaringMaid-20B-GGUF/blob/main/DaringMaid-20B-Q5_K_M.gguf)
## Prompt template:
I have been using Undi/Ikaris SillyTavern presets for Noromaid: [Context](https://files.catbox.moe/ifmhai.json), [Instruct](https://files.catbox.moe/ttw1l9.json).
### Alpaca:
```
Below is an instruction that describes a task. Write a response that appropriately completes the request. Do not include descriptions of non-visual qualities such as personality, movements, scents, mental traits, or anything which could not be seen in a still photograph. Do not write in full sentences. Prefix your description with the phrase 'full body portrait,'
### Instruction:
{prompt}
### Response:
```
### Contact
Kooten on discord.
<!-- original-model-card end -->
|
TechxGenus/Meta-Llama-3-8B-Instruct-AWQ | TechxGenus | "2024-04-19T07:59:03Z" | 955 | 3 | transformers | [
"transformers",
"safetensors",
"llama",
"text-generation",
"facebook",
"meta",
"pytorch",
"llama-3",
"conversational",
"en",
"license:other",
"autotrain_compatible",
"endpoints_compatible",
"text-generation-inference",
"4-bit",
"awq",
"region:us"
] | text-generation | "2024-04-19T07:25:53Z" | ---
language:
- en
pipeline_tag: text-generation
tags:
- facebook
- meta
- pytorch
- llama
- llama-3
license: other
license_name: llama3
license_link: LICENSE
extra_gated_prompt: >-
### META LLAMA 3 COMMUNITY LICENSE AGREEMENT
Meta Llama 3 Version Release Date: April 18, 2024
"Agreement" means the terms and conditions for use, reproduction, distribution and modification of the
Llama Materials set forth herein.
"Documentation" means the specifications, manuals and documentation accompanying Meta Llama 3
distributed by Meta at https://llama.meta.com/get-started/.
"Licensee" or "you" means you, or your employer or any other person or entity (if you are entering into
this Agreement on such person or entity’s behalf), of the age required under applicable laws, rules or
regulations to provide legal consent and that has legal authority to bind your employer or such other
person or entity if you are entering in this Agreement on their behalf.
"Meta Llama 3" means the foundational large language models and software and algorithms, including
machine-learning model code, trained model weights, inference-enabling code, training-enabling code,
fine-tuning enabling code and other elements of the foregoing distributed by Meta at
https://llama.meta.com/llama-downloads.
"Llama Materials" means, collectively, Meta’s proprietary Meta Llama 3 and Documentation (and any
portion thereof) made available under this Agreement.
"Meta" or "we" means Meta Platforms Ireland Limited (if you are located in or, if you are an entity, your
principal place of business is in the EEA or Switzerland) and Meta Platforms, Inc. (if you are located
outside of the EEA or Switzerland).
1. License Rights and Redistribution.
a. Grant of Rights. You are granted a non-exclusive, worldwide, non-transferable and royalty-free
limited license under Meta’s intellectual property or other rights owned by Meta embodied in the Llama
Materials to use, reproduce, distribute, copy, create derivative works of, and make modifications to the
Llama Materials.
b. Redistribution and Use.
i. If you distribute or make available the Llama Materials (or any derivative works
thereof), or a product or service that uses any of them, including another AI model, you shall (A) provide
a copy of this Agreement with any such Llama Materials; and (B) prominently display “Built with Meta
Llama 3” on a related website, user interface, blogpost, about page, or product documentation. If you
use the Llama Materials to create, train, fine tune, or otherwise improve an AI model, which is
distributed or made available, you shall also include “Llama 3” at the beginning of any such AI model
name.
ii. If you receive Llama Materials, or any derivative works thereof, from a Licensee as part
of an integrated end user product, then Section 2 of this Agreement will not apply to you.
iii. You must retain in all copies of the Llama Materials that you distribute the following
attribution notice within a “Notice” text file distributed as a part of such copies: “Meta Llama 3 is
licensed under the Meta Llama 3 Community License, Copyright © Meta Platforms, Inc. All Rights
Reserved.”
iv. Your use of the Llama Materials must comply with applicable laws and regulations
(including trade compliance laws and regulations) and adhere to the Acceptable Use Policy for the Llama
Materials (available at https://llama.meta.com/llama3/use-policy), which is hereby incorporated by
reference into this Agreement.
v. You will not use the Llama Materials or any output or results of the Llama Materials to
improve any other large language model (excluding Meta Llama 3 or derivative works thereof).
2. Additional Commercial Terms. If, on the Meta Llama 3 version release date, the monthly active users
of the products or services made available by or for Licensee, or Licensee’s affiliates, is greater than 700
million monthly active users in the preceding calendar month, you must request a license from Meta,
which Meta may grant to you in its sole discretion, and you are not authorized to exercise any of the
rights under this Agreement unless or until Meta otherwise expressly grants you such rights.
3. Disclaimer of Warranty. UNLESS REQUIRED BY APPLICABLE LAW, THE LLAMA MATERIALS AND ANY
OUTPUT AND RESULTS THEREFROM ARE PROVIDED ON AN “AS IS” BASIS, WITHOUT WARRANTIES OF
ANY KIND, AND META DISCLAIMS ALL WARRANTIES OF ANY KIND, BOTH EXPRESS AND IMPLIED,
INCLUDING, WITHOUT LIMITATION, ANY WARRANTIES OF TITLE, NON-INFRINGEMENT,
MERCHANTABILITY, OR FITNESS FOR A PARTICULAR PURPOSE. YOU ARE SOLELY RESPONSIBLE FOR
DETERMINING THE APPROPRIATENESS OF USING OR REDISTRIBUTING THE LLAMA MATERIALS AND
ASSUME ANY RISKS ASSOCIATED WITH YOUR USE OF THE LLAMA MATERIALS AND ANY OUTPUT AND
RESULTS.
4. Limitation of Liability. IN NO EVENT WILL META OR ITS AFFILIATES BE LIABLE UNDER ANY THEORY OF
LIABILITY, WHETHER IN CONTRACT, TORT, NEGLIGENCE, PRODUCTS LIABILITY, OR OTHERWISE, ARISING
OUT OF THIS AGREEMENT, FOR ANY LOST PROFITS OR ANY INDIRECT, SPECIAL, CONSEQUENTIAL,
INCIDENTAL, EXEMPLARY OR PUNITIVE DAMAGES, EVEN IF META OR ITS AFFILIATES HAVE BEEN ADVISED
OF THE POSSIBILITY OF ANY OF THE FOREGOING.
5. Intellectual Property.
a. No trademark licenses are granted under this Agreement, and in connection with the Llama
Materials, neither Meta nor Licensee may use any name or mark owned by or associated with the other
or any of its affiliates, except as required for reasonable and customary use in describing and
redistributing the Llama Materials or as set forth in this Section 5(a). Meta hereby grants you a license to
use “Llama 3” (the “Mark”) solely as required to comply with the last sentence of Section 1.b.i. You will
comply with Meta’s brand guidelines (currently accessible at
https://about.meta.com/brand/resources/meta/company-brand/ ). All goodwill arising out of your use
of the Mark will inure to the benefit of Meta.
b. Subject to Meta’s ownership of Llama Materials and derivatives made by or for Meta, with
respect to any derivative works and modifications of the Llama Materials that are made by you, as
between you and Meta, you are and will be the owner of such derivative works and modifications.
c. If you institute litigation or other proceedings against Meta or any entity (including a
cross-claim or counterclaim in a lawsuit) alleging that the Llama Materials or Meta Llama 3 outputs or
results, or any portion of any of the foregoing, constitutes infringement of intellectual property or other
rights owned or licensable by you, then any licenses granted to you under this Agreement shall
terminate as of the date such litigation or claim is filed or instituted. You will indemnify and hold
harmless Meta from and against any claim by any third party arising out of or related to your use or
distribution of the Llama Materials.
6. Term and Termination. The term of this Agreement will commence upon your acceptance of this
Agreement or access to the Llama Materials and will continue in full force and effect until terminated in
accordance with the terms and conditions herein. Meta may terminate this Agreement if you are in
breach of any term or condition of this Agreement. Upon termination of this Agreement, you shall delete
and cease use of the Llama Materials. Sections 3, 4 and 7 shall survive the termination of this
Agreement.
7. Governing Law and Jurisdiction. This Agreement will be governed and construed under the laws of
the State of California without regard to choice of law principles, and the UN Convention on Contracts
for the International Sale of Goods does not apply to this Agreement. The courts of California shall have
exclusive jurisdiction of any dispute arising out of this Agreement.
### Meta Llama 3 Acceptable Use Policy
Meta is committed to promoting safe and fair use of its tools and features, including Meta Llama 3. If you
access or use Meta Llama 3, you agree to this Acceptable Use Policy (“Policy”). The most recent copy of
this policy can be found at [https://llama.meta.com/llama3/use-policy](https://llama.meta.com/llama3/use-policy)
#### Prohibited Uses
We want everyone to use Meta Llama 3 safely and responsibly. You agree you will not use, or allow
others to use, Meta Llama 3 to:
1. Violate the law or others’ rights, including to:
1. Engage in, promote, generate, contribute to, encourage, plan, incite, or further illegal or unlawful activity or content, such as:
1. Violence or terrorism
2. Exploitation or harm to children, including the solicitation, creation, acquisition, or dissemination of child exploitative content or failure to report Child Sexual Abuse Material
3. Human trafficking, exploitation, and sexual violence
4. The illegal distribution of information or materials to minors, including obscene materials, or failure to employ legally required age-gating in connection with such information or materials.
5. Sexual solicitation
6. Any other criminal activity
2. Engage in, promote, incite, or facilitate the harassment, abuse, threatening, or bullying of individuals or groups of individuals
3. Engage in, promote, incite, or facilitate discrimination or other unlawful or harmful conduct in the provision of employment, employment benefits, credit, housing, other economic benefits, or other essential goods and services
4. Engage in the unauthorized or unlicensed practice of any profession including, but not limited to, financial, legal, medical/health, or related professional practices
5. Collect, process, disclose, generate, or infer health, demographic, or other sensitive personal or private information about individuals without rights and consents required by applicable laws
6. Engage in or facilitate any action or generate any content that infringes, misappropriates, or otherwise violates any third-party rights, including the outputs or results of any products or services using the Llama Materials
7. Create, generate, or facilitate the creation of malicious code, malware, computer viruses or do anything else that could disable, overburden, interfere with or impair the proper working, integrity, operation or appearance of a website or computer system
2. Engage in, promote, incite, facilitate, or assist in the planning or development of activities that present a risk of death or bodily harm to individuals, including use of Meta Llama 3 related to the following:
1. Military, warfare, nuclear industries or applications, espionage, use for materials or activities that are subject to the International Traffic Arms Regulations (ITAR) maintained by the United States Department of State
2. Guns and illegal weapons (including weapon development)
3. Illegal drugs and regulated/controlled substances
4. Operation of critical infrastructure, transportation technologies, or heavy machinery
5. Self-harm or harm to others, including suicide, cutting, and eating disorders
6. Any content intended to incite or promote violence, abuse, or any infliction of bodily harm to an individual
3. Intentionally deceive or mislead others, including use of Meta Llama 3 related to the following:
1. Generating, promoting, or furthering fraud or the creation or promotion of disinformation
2. Generating, promoting, or furthering defamatory content, including the creation of defamatory statements, images, or other content
3. Generating, promoting, or further distributing spam
4. Impersonating another individual without consent, authorization, or legal right
5. Representing that the use of Meta Llama 3 or outputs are human-generated
6. Generating or facilitating false online engagement, including fake reviews and other means of fake online engagement
4. Fail to appropriately disclose to end users any known dangers of your AI system
Please report any violation of this Policy, software “bug,” or other problems that could lead to a violation
of this Policy through one of the following means:
* Reporting issues with the model: [https://github.com/meta-llama/llama3](https://github.com/meta-llama/llama3)
* Reporting risky content generated by the model:
developers.facebook.com/llama_output_feedback
* Reporting bugs and security concerns: facebook.com/whitehat/info
* Reporting violations of the Acceptable Use Policy or unlicensed uses of Meta Llama 3: [email protected]
extra_gated_fields:
First Name: text
Last Name: text
Date of birth: date_picker
Country: country
Affiliation: text
geo: ip_location
By clicking Submit below I accept the terms of the license and acknowledge that the information I provide will be collected stored processed and shared in accordance with the Meta Privacy Policy: checkbox
extra_gated_description: The information you provide will be collected, stored, processed and shared in accordance with the [Meta Privacy Policy](https://www.facebook.com/privacy/policy/).
extra_gated_button_content: Submit
---
AWQ quantized version of Meta-Llama-3-8B-Instruct model.
---
## Model Details
Meta developed and released the Meta Llama 3 family of large language models (LLMs), a collection of pretrained and instruction tuned generative text models in 8 and 70B sizes. The Llama 3 instruction tuned models are optimized for dialogue use cases and outperform many of the available open source chat models on common industry benchmarks. Further, in developing these models, we took great care to optimize helpfulness and safety.
**Model developers** Meta
**Variations** Llama 3 comes in two sizes — 8B and 70B parameters — in pre-trained and instruction tuned variants.
**Input** Models input text only.
**Output** Models generate text and code only.
**Model Architecture** Llama 3 is an auto-regressive language model that uses an optimized transformer architecture. The tuned versions use supervised fine-tuning (SFT) and reinforcement learning with human feedback (RLHF) to align with human preferences for helpfulness and safety.
<table>
<tr>
<td>
</td>
<td><strong>Training Data</strong>
</td>
<td><strong>Params</strong>
</td>
<td><strong>Context length</strong>
</td>
<td><strong>GQA</strong>
</td>
<td><strong>Token count</strong>
</td>
<td><strong>Knowledge cutoff</strong>
</td>
</tr>
<tr>
<td rowspan="2" >Llama 3
</td>
<td rowspan="2" >A new mix of publicly available online data.
</td>
<td>8B
</td>
<td>8k
</td>
<td>Yes
</td>
<td rowspan="2" >15T+
</td>
<td>March, 2023
</td>
</tr>
<tr>
<td>70B
</td>
<td>8k
</td>
<td>Yes
</td>
<td>December, 2023
</td>
</tr>
</table>
**Llama 3 family of models**. Token counts refer to pretraining data only. Both the 8 and 70B versions use Grouped-Query Attention (GQA) for improved inference scalability.
**Model Release Date** April 18, 2024.
**Status** This is a static model trained on an offline dataset. Future versions of the tuned models will be released as we improve model safety with community feedback.
**License** A custom commercial license is available at: [https://llama.meta.com/llama3/license](https://llama.meta.com/llama3/license)
Where to send questions or comments about the model Instructions on how to provide feedback or comments on the model can be found in the model [README](https://github.com/meta-llama/llama3). For more technical information about generation parameters and recipes for how to use Llama 3 in applications, please go [here](https://github.com/meta-llama/llama-recipes).
## Intended Use
**Intended Use Cases** Llama 3 is intended for commercial and research use in English. Instruction tuned models are intended for assistant-like chat, whereas pretrained models can be adapted for a variety of natural language generation tasks.
**Out-of-scope** Use in any manner that violates applicable laws or regulations (including trade compliance laws). Use in any other way that is prohibited by the Acceptable Use Policy and Llama 3 Community License. Use in languages other than English**.
**Note: Developers may fine-tune Llama 3 models for languages beyond English provided they comply with the Llama 3 Community License and the Acceptable Use Policy.
## How to use
This repository contains two versions of Meta-Llama-3-8B-Instruct, for use with transformers and with the original `llama3` codebase.
### Use with transformers
See the snippet below for usage with Transformers:
```python
import transformers
import torch
model_id = "meta-llama/Meta-Llama-3-8B-Instruct"
pipeline = transformers.pipeline(
"text-generation",
model=model_id,
model_kwargs={"torch_dtype": torch.bfloat16},
device="auto",
)
messages = [
{"role": "system", "content": "You are a pirate chatbot who always responds in pirate speak!"},
{"role": "user", "content": "Who are you?"},
]
prompt = pipeline.tokenizer.apply_chat_template(
messages,
tokenize=False,
add_generation_prompt=True
)
terminators = [
pipeline.tokenizer.eos_token_id,
pipeline.tokenizer.convert_tokens_to_ids("<|eot_id|>")
]
outputs = pipeline(
prompt,
max_new_tokens=256,
eos_token_id=terminators,
do_sample=True,
temperature=0.6,
top_p=0.9,
)
print(outputs[0]["generated_text"][len(prompt):])
```
### Use with `llama3`
Please, follow the instructions in the [repository](https://github.com/meta-llama/llama3)
To download Original checkpoints, see the example command below leveraging `huggingface-cli`:
```
huggingface-cli download meta-llama/Meta-Llama-3-8B-Instruct --include "original/*" --local-dir Meta-Llama-3-8B-Instruct
```
For Hugging Face support, we recommend using transformers or TGI, but a similar command works.
## Hardware and Software
**Training Factors** We used custom training libraries, Meta's Research SuperCluster, and production clusters for pretraining. Fine-tuning, annotation, and evaluation were also performed on third-party cloud compute.
**Carbon Footprint Pretraining utilized a cumulative** 7.7M GPU hours of computation on hardware of type H100-80GB (TDP of 700W). Estimated total emissions were 2290 tCO2eq, 100% of which were offset by Meta’s sustainability program.
<table>
<tr>
<td>
</td>
<td><strong>Time (GPU hours)</strong>
</td>
<td><strong>Power Consumption (W)</strong>
</td>
<td><strong>Carbon Emitted(tCO2eq)</strong>
</td>
</tr>
<tr>
<td>Llama 3 8B
</td>
<td>1.3M
</td>
<td>700
</td>
<td>390
</td>
</tr>
<tr>
<td>Llama 3 70B
</td>
<td>6.4M
</td>
<td>700
</td>
<td>1900
</td>
</tr>
<tr>
<td>Total
</td>
<td>7.7M
</td>
<td>
</td>
<td>2290
</td>
</tr>
</table>
**CO2 emissions during pre-training**. Time: total GPU time required for training each model. Power Consumption: peak power capacity per GPU device for the GPUs used adjusted for power usage efficiency. 100% of the emissions are directly offset by Meta's sustainability program, and because we are openly releasing these models, the pretraining costs do not need to be incurred by others.
## Training Data
**Overview** Llama 3 was pretrained on over 15 trillion tokens of data from publicly available sources. The fine-tuning data includes publicly available instruction datasets, as well as over 10M human-annotated examples. Neither the pretraining nor the fine-tuning datasets include Meta user data.
**Data Freshness** The pretraining data has a cutoff of March 2023 for the 7B and December 2023 for the 70B models respectively.
## Benchmarks
In this section, we report the results for Llama 3 models on standard automatic benchmarks. For all the evaluations, we use our internal evaluations library. For details on the methodology see [here](https://github.com/meta-llama/llama3/blob/main/eval_methodology.md).
### Base pretrained models
<table>
<tr>
<td><strong>Category</strong>
</td>
<td><strong>Benchmark</strong>
</td>
<td><strong>Llama 3 8B</strong>
</td>
<td><strong>Llama2 7B</strong>
</td>
<td><strong>Llama2 13B</strong>
</td>
<td><strong>Llama 3 70B</strong>
</td>
<td><strong>Llama2 70B</strong>
</td>
</tr>
<tr>
<td rowspan="6" >General
</td>
<td>MMLU (5-shot)
</td>
<td>66.6
</td>
<td>45.7
</td>
<td>53.8
</td>
<td>79.5
</td>
<td>69.7
</td>
</tr>
<tr>
<td>AGIEval English (3-5 shot)
</td>
<td>45.9
</td>
<td>28.8
</td>
<td>38.7
</td>
<td>63.0
</td>
<td>54.8
</td>
</tr>
<tr>
<td>CommonSenseQA (7-shot)
</td>
<td>72.6
</td>
<td>57.6
</td>
<td>67.6
</td>
<td>83.8
</td>
<td>78.7
</td>
</tr>
<tr>
<td>Winogrande (5-shot)
</td>
<td>76.1
</td>
<td>73.3
</td>
<td>75.4
</td>
<td>83.1
</td>
<td>81.8
</td>
</tr>
<tr>
<td>BIG-Bench Hard (3-shot, CoT)
</td>
<td>61.1
</td>
<td>38.1
</td>
<td>47.0
</td>
<td>81.3
</td>
<td>65.7
</td>
</tr>
<tr>
<td>ARC-Challenge (25-shot)
</td>
<td>78.6
</td>
<td>53.7
</td>
<td>67.6
</td>
<td>93.0
</td>
<td>85.3
</td>
</tr>
<tr>
<td>Knowledge reasoning
</td>
<td>TriviaQA-Wiki (5-shot)
</td>
<td>78.5
</td>
<td>72.1
</td>
<td>79.6
</td>
<td>89.7
</td>
<td>87.5
</td>
</tr>
<tr>
<td rowspan="4" >Reading comprehension
</td>
<td>SQuAD (1-shot)
</td>
<td>76.4
</td>
<td>72.2
</td>
<td>72.1
</td>
<td>85.6
</td>
<td>82.6
</td>
</tr>
<tr>
<td>QuAC (1-shot, F1)
</td>
<td>44.4
</td>
<td>39.6
</td>
<td>44.9
</td>
<td>51.1
</td>
<td>49.4
</td>
</tr>
<tr>
<td>BoolQ (0-shot)
</td>
<td>75.7
</td>
<td>65.5
</td>
<td>66.9
</td>
<td>79.0
</td>
<td>73.1
</td>
</tr>
<tr>
<td>DROP (3-shot, F1)
</td>
<td>58.4
</td>
<td>37.9
</td>
<td>49.8
</td>
<td>79.7
</td>
<td>70.2
</td>
</tr>
</table>
### Instruction tuned models
<table>
<tr>
<td><strong>Benchmark</strong>
</td>
<td><strong>Llama 3 8B</strong>
</td>
<td><strong>Llama 2 7B</strong>
</td>
<td><strong>Llama 2 13B</strong>
</td>
<td><strong>Llama 3 70B</strong>
</td>
<td><strong>Llama 2 70B</strong>
</td>
</tr>
<tr>
<td>MMLU (5-shot)
</td>
<td>68.4
</td>
<td>34.1
</td>
<td>47.8
</td>
<td>82.0
</td>
<td>52.9
</td>
</tr>
<tr>
<td>GPQA (0-shot)
</td>
<td>34.2
</td>
<td>21.7
</td>
<td>22.3
</td>
<td>39.5
</td>
<td>21.0
</td>
</tr>
<tr>
<td>HumanEval (0-shot)
</td>
<td>62.2
</td>
<td>7.9
</td>
<td>14.0
</td>
<td>81.7
</td>
<td>25.6
</td>
</tr>
<tr>
<td>GSM-8K (8-shot, CoT)
</td>
<td>79.6
</td>
<td>25.7
</td>
<td>77.4
</td>
<td>93.0
</td>
<td>57.5
</td>
</tr>
<tr>
<td>MATH (4-shot, CoT)
</td>
<td>30.0
</td>
<td>3.8
</td>
<td>6.7
</td>
<td>50.4
</td>
<td>11.6
</td>
</tr>
</table>
### Responsibility & Safety
We believe that an open approach to AI leads to better, safer products, faster innovation, and a bigger overall market. We are committed to Responsible AI development and took a series of steps to limit misuse and harm and support the open source community.
Foundation models are widely capable technologies that are built to be used for a diverse range of applications. They are not designed to meet every developer preference on safety levels for all use cases, out-of-the-box, as those by their nature will differ across different applications.
Rather, responsible LLM-application deployment is achieved by implementing a series of safety best practices throughout the development of such applications, from the model pre-training, fine-tuning and the deployment of systems composed of safeguards to tailor the safety needs specifically to the use case and audience.
As part of the Llama 3 release, we updated our [Responsible Use Guide](https://llama.meta.com/responsible-use-guide/) to outline the steps and best practices for developers to implement model and system level safety for their application. We also provide a set of resources including [Meta Llama Guard 2](https://llama.meta.com/purple-llama/) and [Code Shield](https://llama.meta.com/purple-llama/) safeguards. These tools have proven to drastically reduce residual risks of LLM Systems, while maintaining a high level of helpfulness. We encourage developers to tune and deploy these safeguards according to their needs and we provide a [reference implementation](https://github.com/meta-llama/llama-recipes/tree/main/recipes/responsible_ai) to get you started.
#### Llama 3-Instruct
As outlined in the Responsible Use Guide, some trade-off between model helpfulness and model alignment is likely unavoidable. Developers should exercise discretion about how to weigh the benefits of alignment and helpfulness for their specific use case and audience. Developers should be mindful of residual risks when using Llama models and leverage additional safety tools as needed to reach the right safety bar for their use case.
<span style="text-decoration:underline;">Safety</span>
For our instruction tuned model, we conducted extensive red teaming exercises, performed adversarial evaluations and implemented safety mitigations techniques to lower residual risks. As with any Large Language Model, residual risks will likely remain and we recommend that developers assess these risks in the context of their use case. In parallel, we are working with the community to make AI safety benchmark standards transparent, rigorous and interpretable.
<span style="text-decoration:underline;">Refusals</span>
In addition to residual risks, we put a great emphasis on model refusals to benign prompts. Over-refusing not only can impact the user experience but could even be harmful in certain contexts as well. We’ve heard the feedback from the developer community and improved our fine tuning to ensure that Llama 3 is significantly less likely to falsely refuse to answer prompts than Llama 2.
We built internal benchmarks and developed mitigations to limit false refusals making Llama 3 our most helpful model to date.
#### Responsible release
In addition to responsible use considerations outlined above, we followed a rigorous process that requires us to take extra measures against misuse and critical risks before we make our release decision.
Misuse
If you access or use Llama 3, you agree to the Acceptable Use Policy. The most recent copy of this policy can be found at [https://llama.meta.com/llama3/use-policy/](https://llama.meta.com/llama3/use-policy/).
#### Critical risks
<span style="text-decoration:underline;">CBRNE</span> (Chemical, Biological, Radiological, Nuclear, and high yield Explosives)
We have conducted a two fold assessment of the safety of the model in this area:
* Iterative testing during model training to assess the safety of responses related to CBRNE threats and other adversarial risks.
* Involving external CBRNE experts to conduct an uplift test assessing the ability of the model to accurately provide expert knowledge and reduce barriers to potential CBRNE misuse, by reference to what can be achieved using web search (without the model).
### <span style="text-decoration:underline;">Cyber Security </span>
We have evaluated Llama 3 with CyberSecEval, Meta’s cybersecurity safety eval suite, measuring Llama 3’s propensity to suggest insecure code when used as a coding assistant, and Llama 3’s propensity to comply with requests to help carry out cyber attacks, where attacks are defined by the industry standard MITRE ATT&CK cyber attack ontology. On our insecure coding and cyber attacker helpfulness tests, Llama 3 behaved in the same range or safer than models of [equivalent coding capability](https://huggingface.co/spaces/facebook/CyberSecEval).
### <span style="text-decoration:underline;">Child Safety</span>
Child Safety risk assessments were conducted using a team of experts, to assess the model’s capability to produce outputs that could result in Child Safety risks and inform on any necessary and appropriate risk mitigations via fine tuning. We leveraged those expert red teaming sessions to expand the coverage of our evaluation benchmarks through Llama 3 model development. For Llama 3, we conducted new in-depth sessions using objective based methodologies to assess the model risks along multiple attack vectors. We also partnered with content specialists to perform red teaming exercises assessing potentially violating content while taking account of market specific nuances or experiences.
### Community
Generative AI safety requires expertise and tooling, and we believe in the strength of the open community to accelerate its progress. We are active members of open consortiums, including the AI Alliance, Partnership in AI and MLCommons, actively contributing to safety standardization and transparency. We encourage the community to adopt taxonomies like the MLCommons Proof of Concept evaluation to facilitate collaboration and transparency on safety and content evaluations. Our Purple Llama tools are open sourced for the community to use and widely distributed across ecosystem partners including cloud service providers. We encourage community contributions to our [Github repository](https://github.com/meta-llama/PurpleLlama).
Finally, we put in place a set of resources including an [output reporting mechanism](https://developers.facebook.com/llama_output_feedback) and [bug bounty program](https://www.facebook.com/whitehat) to continuously improve the Llama technology with the help of the community.
## Ethical Considerations and Limitations
The core values of Llama 3 are openness, inclusivity and helpfulness. It is meant to serve everyone, and to work for a wide range of use cases. It is thus designed to be accessible to people across many different backgrounds, experiences and perspectives. Llama 3 addresses users and their needs as they are, without insertion unnecessary judgment or normativity, while reflecting the understanding that even content that may appear problematic in some cases can serve valuable purposes in others. It respects the dignity and autonomy of all users, especially in terms of the values of free thought and expression that power innovation and progress.
But Llama 3 is a new technology, and like any new technology, there are risks associated with its use. Testing conducted to date has been in English, and has not covered, nor could it cover, all scenarios. For these reasons, as with all LLMs, Llama 3’s potential outputs cannot be predicted in advance, and the model may in some instances produce inaccurate, biased or other objectionable responses to user prompts. Therefore, before deploying any applications of Llama 3 models, developers should perform safety testing and tuning tailored to their specific applications of the model. As outlined in the Responsible Use Guide, we recommend incorporating [Purple Llama](https://github.com/facebookresearch/PurpleLlama) solutions into your workflows and specifically [Llama Guard](https://ai.meta.com/research/publications/llama-guard-llm-based-input-output-safeguard-for-human-ai-conversations/) which provides a base model to filter input and output prompts to layer system-level safety on top of model-level safety.
Please see the Responsible Use Guide available at [http://llama.meta.com/responsible-use-guide](http://llama.meta.com/responsible-use-guide)
## Citation instructions
@article{llama3modelcard,
title={Llama 3 Model Card},
author={AI@Meta},
year={2024},
url = {https://github.com/meta-llama/llama3/blob/main/MODEL_CARD.md}
}
## Contributors
Aaditya Singh; Aaron Grattafiori; Abhimanyu Dubey; Abhinav Jauhri; Abhinav Pandey; Abhishek Kadian; Adam Kelsey; Adi Gangidi; Ahmad Al-Dahle; Ahuva Goldstand; Aiesha Letman; Ajay Menon; Akhil Mathur; Alan Schelten; Alex Vaughan; Amy Yang; Andrei Lupu; Andres Alvarado; Andrew Gallagher; Andrew Gu; Andrew Ho; Andrew Poulton; Andrew Ryan; Angela Fan; Ankit Ramchandani; Anthony Hartshorn; Archi Mitra; Archie Sravankumar; Artem Korenev; Arun Rao; Ashley Gabriel; Ashwin Bharambe; Assaf Eisenman; Aston Zhang; Aurelien Rodriguez; Austen Gregerson; Ava Spataru; Baptiste Roziere; Ben Maurer; Benjamin Leonhardi; Bernie Huang; Bhargavi Paranjape; Bing Liu; Binh Tang; Bobbie Chern; Brani Stojkovic; Brian Fuller; Catalina Mejia Arenas; Chao Zhou; Charlotte Caucheteux; Chaya Nayak; Ching-Hsiang Chu; Chloe Bi; Chris Cai; Chris Cox; Chris Marra; Chris McConnell; Christian Keller; Christoph Feichtenhofer; Christophe Touret; Chunyang Wu; Corinne Wong; Cristian Canton Ferrer; Damien Allonsius; Daniel Kreymer; Daniel Haziza; Daniel Li; Danielle Pintz; Danny Livshits; Danny Wyatt; David Adkins; David Esiobu; David Xu; Davide Testuggine; Delia David; Devi Parikh; Dhruv Choudhary; Dhruv Mahajan; Diana Liskovich; Diego Garcia-Olano; Diego Perino; Dieuwke Hupkes; Dingkang Wang; Dustin Holland; Egor Lakomkin; Elina Lobanova; Xiaoqing Ellen Tan; Emily Dinan; Eric Smith; Erik Brinkman; Esteban Arcaute; Filip Radenovic; Firat Ozgenel; Francesco Caggioni; Frank Seide; Frank Zhang; Gabriel Synnaeve; Gabriella Schwarz; Gabrielle Lee; Gada Badeer; Georgia Anderson; Graeme Nail; Gregoire Mialon; Guan Pang; Guillem Cucurell; Hailey Nguyen; Hannah Korevaar; Hannah Wang; Haroun Habeeb; Harrison Rudolph; Henry Aspegren; Hu Xu; Hugo Touvron; Iga Kozlowska; Igor Molybog; Igor Tufanov; Iliyan Zarov; Imanol Arrieta Ibarra; Irina-Elena Veliche; Isabel Kloumann; Ishan Misra; Ivan Evtimov; Jacob Xu; Jade Copet; Jake Weissman; Jan Geffert; Jana Vranes; Japhet Asher; Jason Park; Jay Mahadeokar; Jean-Baptiste Gaya; Jeet Shah; Jelmer van der Linde; Jennifer Chan; Jenny Hong; Jenya Lee; Jeremy Fu; Jeremy Teboul; Jianfeng Chi; Jianyu Huang; Jie Wang; Jiecao Yu; Joanna Bitton; Joe Spisak; Joelle Pineau; Jon Carvill; Jongsoo Park; Joseph Rocca; Joshua Johnstun; Junteng Jia; Kalyan Vasuden Alwala; Kam Hou U; Kate Plawiak; Kartikeya Upasani; Kaushik Veeraraghavan; Ke Li; Kenneth Heafield; Kevin Stone; Khalid El-Arini; Krithika Iyer; Kshitiz Malik; Kuenley Chiu; Kunal Bhalla; Kyle Huang; Lakshya Garg; Lauren Rantala-Yeary; Laurens van der Maaten; Lawrence Chen; Leandro Silva; Lee Bell; Lei Zhang; Liang Tan; Louis Martin; Lovish Madaan; Luca Wehrstedt; Lukas Blecher; Luke de Oliveira; Madeline Muzzi; Madian Khabsa; Manav Avlani; Mannat Singh; Manohar Paluri; Mark Zuckerberg; Marcin Kardas; Martynas Mankus; Mathew Oldham; Mathieu Rita; Matthew Lennie; Maya Pavlova; Meghan Keneally; Melanie Kambadur; Mihir Patel; Mikayel Samvelyan; Mike Clark; Mike Lewis; Min Si; Mitesh Kumar Singh; Mo Metanat; Mona Hassan; Naman Goyal; Narjes Torabi; Nicolas Usunier; Nikolay Bashlykov; Nikolay Bogoychev; Niladri Chatterji; Ning Dong; Oliver Aobo Yang; Olivier Duchenne; Onur Celebi; Parth Parekh; Patrick Alrassy; Paul Saab; Pavan Balaji; Pedro Rittner; Pengchuan Zhang; Pengwei Li; Petar Vasic; Peter Weng; Polina Zvyagina; Prajjwal Bhargava; Pratik Dubal; Praveen Krishnan; Punit Singh Koura; Qing He; Rachel Rodriguez; Ragavan Srinivasan; Rahul Mitra; Ramon Calderer; Raymond Li; Robert Stojnic; Roberta Raileanu; Robin Battey; Rocky Wang; Rohit Girdhar; Rohit Patel; Romain Sauvestre; Ronnie Polidoro; Roshan Sumbaly; Ross Taylor; Ruan Silva; Rui Hou; Rui Wang; Russ Howes; Ruty Rinott; Saghar Hosseini; Sai Jayesh Bondu; Samyak Datta; Sanjay Singh; Sara Chugh; Sargun Dhillon; Satadru Pan; Sean Bell; Sergey Edunov; Shaoliang Nie; Sharan Narang; Sharath Raparthy; Shaun Lindsay; Sheng Feng; Sheng Shen; Shenghao Lin; Shiva Shankar; Shruti Bhosale; Shun Zhang; Simon Vandenhende; Sinong Wang; Seohyun Sonia Kim; Soumya Batra; Sten Sootla; Steve Kehoe; Suchin Gururangan; Sumit Gupta; Sunny Virk; Sydney Borodinsky; Tamar Glaser; Tamar Herman; Tamara Best; Tara Fowler; Thomas Georgiou; Thomas Scialom; Tianhe Li; Todor Mihaylov; Tong Xiao; Ujjwal Karn; Vedanuj Goswami; Vibhor Gupta; Vignesh Ramanathan; Viktor Kerkez; Vinay Satish Kumar; Vincent Gonguet; Vish Vogeti; Vlad Poenaru; Vlad Tiberiu Mihailescu; Vladan Petrovic; Vladimir Ivanov; Wei Li; Weiwei Chu; Wenhan Xiong; Wenyin Fu; Wes Bouaziz; Whitney Meers; Will Constable; Xavier Martinet; Xiaojian Wu; Xinbo Gao; Xinfeng Xie; Xuchao Jia; Yaelle Goldschlag; Yann LeCun; Yashesh Gaur; Yasmine Babaei; Ye Qi; Yenda Li; Yi Wen; Yiwen Song; Youngjin Nam; Yuchen Hao; Yuchen Zhang; Yun Wang; Yuning Mao; Yuzi He; Zacharie Delpierre Coudert; Zachary DeVito; Zahra Hankir; Zhaoduo Wen; Zheng Yan; Zhengxing Chen; Zhenyu Yang; Zoe Papakipos
|
Subsets and Splits
No community queries yet
The top public SQL queries from the community will appear here once available.