file_path
stringlengths 21
207
| content
stringlengths 5
1.02M
| size
int64 5
1.02M
| lang
stringclasses 9
values | avg_line_length
float64 1.33
100
| max_line_length
int64 4
993
| alphanum_fraction
float64 0.27
0.93
|
---|---|---|---|---|---|---|
eliabntt/GRADE-RR/isaac_internals/kit/extscore/omni.syntheticdata/omni/syntheticdata/tests/sensors/test_bbox2d_loose.py | # NOTE:
# omni.kit.test - std python's unittest module with additional wrapping to add suport for async/await tests
# For most things refer to unittest docs: https://docs.python.org/3/library/unittest.html
import os
import math
import asyncio
from time import time
import unittest
import carb
import numpy as np
import omni.kit.test
from omni.kit.viewport.utility import get_active_viewport
from pxr import Gf, UsdGeom, Sdf
# Import extension python module we are testing with absolute import path, as if we are external user (other extension)
import omni.syntheticdata as syn
from ..utils import add_semantics
FILE_DIR = os.path.dirname(os.path.realpath(__file__))
TIMEOUT = 200
# Having a test class derived from omni.kit.test.AsyncTestCase declared on the root of module will make it auto-discoverable by omni.kit.test
class TestBBox2DLoose(omni.kit.test.AsyncTestCase):
# Before running each test
async def setUp(self):
np.random.seed(1234)
# Setup viewport
self.viewport = get_active_viewport()
# Initialize Sensor
await omni.usd.get_context().new_stage_async()
stage = omni.usd.get_context().get_stage()
await omni.kit.app.get_app().next_update_async()
await syn.sensors.create_or_retrieve_sensor_async(
self.viewport, syn._syntheticdata.SensorType.BoundingBox2DLoose
)
async def test_parsed_empty(self):
""" Test 2D bounding box on empty stage.
"""
bbox2d_data = syn.sensors.get_bounding_box_2d_loose(self.viewport)
assert not bool(bbox2d_data)
async def test_bbox_2d_loose_fields_exist(self):
stage = omni.usd.get_context().get_stage()
cube = stage.DefinePrim("/Cube", "Cube")
add_semantics(cube, "cube")
# Render one frame
await syn.sensors.next_sensor_data_async(self.viewport,True)
bbox2d_data = syn.sensors.get_bounding_box_2d_loose(self.viewport)
valid_dtype = [
("uniqueId", "<i4"),
("name", "O"),
("semanticLabel", "O"),
("metadata", "O"),
("instanceIds", "O"),
("semanticId", "<u4"),
("x_min", "<i4"),
("y_min", "<i4"),
("x_max", "<i4"),
("y_max", "<i4"),
]
assert bbox2d_data.dtype == np.dtype(valid_dtype)
async def test_bbox_2d_loose_cube(self):
""" Basic test for the sensor.
"""
stage = omni.usd.get_context().get_stage()
camera = stage.DefinePrim("/Camera", "Camera")
UsdGeom.Xformable(camera).AddTranslateOp().Set((0, 0, 0))
self.viewport.camera_path = camera.GetPath()
await omni.kit.app.get_app().next_update_async()
cube = stage.DefinePrim("/Cube", "Cube")
add_semantics(cube, "cube")
UsdGeom.XformCommonAPI(cube).SetTranslate((0, 0, -10))
# Render one frame
await syn.sensors.next_sensor_data_async(self.viewport,True)
bbox2d_data = syn.sensors.get_bounding_box_2d_loose(self.viewport)
assert bbox2d_data['x_min'] == 301
assert bbox2d_data['y_min'] == 21
assert bbox2d_data['x_max'] == 978
assert bbox2d_data['y_max'] == 698
async def test_cube_pathtracing(self):
""" Basic funtionality test of the sensor, but in path tracing mode.
"""
settings = carb.settings.get_settings()
settings.set_string("/rtx/rendermode", "PathTracing")
settings.set_int("/rtx/pathtracing/spp", 32)
settings.set_int("/persistent/app/viewport/displayOptions", 0)
stage = omni.usd.get_context().get_stage()
camera = stage.DefinePrim("/Camera", "Camera")
UsdGeom.Xformable(camera).AddTranslateOp().Set((0, 0, 0))
self.viewport.camera_path = camera.GetPath()
await omni.kit.app.get_app().next_update_async()
cube = stage.DefinePrim("/Cube", "Cube")
add_semantics(cube, "cube")
UsdGeom.XformCommonAPI(cube).SetTranslate((0, 0, -10))
# Render one frame
await syn.sensors.next_sensor_data_async(self.viewport,True)
bbox2d_data = syn.sensors.get_bounding_box_2d_loose(self.viewport)
assert bbox2d_data['x_min'] == 301
assert bbox2d_data['y_min'] == 21
assert bbox2d_data['x_max'] == 978
assert bbox2d_data['y_max'] == 698
async def test_cube_ray_traced_lighting(self):
""" Basic test for the sensor, but in ray traced lighting mode.
"""
# Set the rendering mode to be ray traced lighting.
settings_interface = carb.settings.get_settings()
settings_interface.set_string("/rtx/rendermode", "RayTracedLighting")
stage = omni.usd.get_context().get_stage()
camera = stage.DefinePrim("/Camera", "Camera")
UsdGeom.Xformable(camera).AddTranslateOp().Set((0, 0, 0))
self.viewport.camera_path = camera.GetPath()
await omni.kit.app.get_app().next_update_async()
cube = stage.DefinePrim("/Cube", "Cube")
add_semantics(cube, "cube")
UsdGeom.XformCommonAPI(cube).SetTranslate((0, 0, -10))
# Render one frame
await syn.sensors.next_sensor_data_async(self.viewport,True)
bbox2d_data = syn.sensors.get_bounding_box_2d_loose(self.viewport)
assert bbox2d_data['x_min'] == 301
assert bbox2d_data['y_min'] == 21
assert bbox2d_data['x_max'] == 978
assert bbox2d_data['y_max'] == 698
async def test_cube_ftheta(self):
""" Basic funtionality test of the sensor in ftheta camera.
"""
settings = carb.settings.get_settings()
settings.set_string("/rtx/rendermode", "PathTracing")
settings.set_int("/rtx/pathtracing/spp", 32)
settings.set_int("/persistent/app/viewport/displayOptions", 0)
stage = omni.usd.get_context().get_stage()
camera = stage.DefinePrim("/Camera", "Camera")
# Set the camera to be polynomial fish eye camera.
camera.CreateAttribute("cameraProjectionType", Sdf.ValueTypeNames.Token).Set("fisheyePolynomial")
UsdGeom.Xformable(camera).AddTranslateOp().Set((0, 0, 0))
self.viewport.camera_path = camera.GetPath()
await omni.kit.app.get_app().next_update_async()
cube = stage.DefinePrim("/Cube", "Cube")
add_semantics(cube, "cube")
UsdGeom.XformCommonAPI(cube).SetTranslate((0, 0, -10))
# Render one frame
await syn.sensors.next_sensor_data_async(self.viewport,True)
bbox2d_data = syn.sensors.get_bounding_box_2d_loose(self.viewport)
assert bbox2d_data['x_min'] == 612
assert bbox2d_data['y_min'] == 325
assert bbox2d_data['x_max'] == 671
assert bbox2d_data['y_max'] == 384
async def test_cube_spherical(self):
""" Basic funtionality test of the sensor in fisheye spherical camera.
"""
settings = carb.settings.get_settings()
settings.set_string("/rtx/rendermode", "PathTracing")
settings.set_int("/rtx/pathtracing/spp", 32)
settings.set_int("/persistent/app/viewport/displayOptions", 0)
stage = omni.usd.get_context().get_stage()
camera = stage.DefinePrim("/Camera", "Camera")
# Set the camera to be polynomial fish eye camera.
camera.CreateAttribute("cameraProjectionType", Sdf.ValueTypeNames.Token).Set("fisheyeSpherical")
UsdGeom.Xformable(camera).AddTranslateOp().Set((0, 0, 0))
self.viewport.camera_path = camera.GetPath()
await omni.kit.app.get_app().next_update_async()
cube = stage.DefinePrim("/Cube", "Cube")
add_semantics(cube, "cube")
UsdGeom.XformCommonAPI(cube).SetTranslate((0, 0, -10))
# Render one frame
await syn.sensors.next_sensor_data_async(self.viewport,True)
bbox2d_data = syn.sensors.get_bounding_box_2d_loose(self.viewport)
assert bbox2d_data['x_min'] == 617
assert bbox2d_data['y_min'] == 335
assert bbox2d_data['x_max'] == 662
assert bbox2d_data['y_max'] == 384
# After running each test
async def tearDown(self):
pass
| 8,240 | Python | 36.630137 | 141 | 0.622816 |
eliabntt/GRADE-RR/isaac_internals/kit/extscore/omni.syntheticdata/omni/syntheticdata/tests/sensors/test_instance_seg.py | # NOTE:
# omni.kit.test - std python's unittest module with additional wrapping to add suport for async/await tests
# For most things refer to unittest docs: https://docs.python.org/3/library/unittest.html
import os
import math
import asyncio
from time import time
from pathlib import Path
import unittest
import carb
import numpy as np
import omni.kit.test
from omni.kit.viewport.utility import get_active_viewport
from pxr import Gf, UsdGeom, Sdf
# Import extension python module we are testing with absolute import path, as if we are external user (other extension)
import omni.syntheticdata as syn
from ..utils import add_semantics
FILE_DIR = os.path.dirname(os.path.realpath(__file__))
TIMEOUT = 200
# Having a test class derived from omni.kit.test.AsyncTestCase declared on the root of module will make it auto-discoverable by omni.kit.test
class TestInstanceSeg(omni.kit.test.AsyncTestCase):
def __init__(self, methodName: str) -> None:
super().__init__(methodName=methodName)
self.golden_image_path = Path(os.path.dirname(os.path.abspath(__file__))) / ".." / "data" / "golden"
# Before running each test
async def setUp(self):
settings = carb.settings.get_settings()
settings.set_bool("syntheticdata/sensors/perSubsetSegmentation", False)
np.random.seed(1234)
# Setup viewport
self.viewport = get_active_viewport()
# Initialize Sensor
await omni.usd.get_context().new_stage_async()
stage = omni.usd.get_context().get_stage()
await omni.kit.app.get_app().next_update_async()
await syn.sensors.create_or_retrieve_sensor_async(
self.viewport, syn._syntheticdata.SensorType.InstanceSegmentation
)
# TODO
# async def test_parsed_empty(self):
# """ Test instance segmentation on empty stage.
# """
# data = syn.sensors.get_instance_segmentation(self.viewport, parsed=True)
# assert data.sum() == 0
async def test_parsed_dtype(self):
stage = omni.usd.get_context().get_stage()
cube = stage.DefinePrim("/Cube", "Cube")
add_semantics(cube, "cube")
await omni.kit.app.get_app().next_update_async()
# Render one frame
await syn.sensors.next_sensor_data_async(self.viewport,True)
data = syn.sensors.get_instance_segmentation(self.viewport, parsed=True)
assert data.dtype == np.uint32
async def test_cube(self):
stage = omni.usd.get_context().get_stage()
cube = stage.DefinePrim("/Cube", "Cube")
add_semantics(cube, "cube")
cube.GetAttribute("size").Set(100)
# Render one frame
await syn.sensors.next_sensor_data_async(self.viewport,True)
data = syn.sensors.get_instance_segmentation(self.viewport, return_mapping=False)
# np.savez_compressed(self.golden_image_path / 'instance_seg_cube.npz', array=data)
golden_image = np.load(self.golden_image_path / "instance_seg_cube.npz")["array"]
std_dev = np.sqrt(np.square(data - golden_image).astype(float).mean())
assert std_dev < 2
async def test_cube_sphere(self):
stage = omni.usd.get_context().get_stage()
cube = stage.DefinePrim("/Cube", "Cube")
add_semantics(cube, "cube")
cube.GetAttribute("size").Set(100)
sphere_prim = stage.DefinePrim("/Sphere", "Sphere")
UsdGeom.XformCommonAPI(sphere_prim).SetTranslate((300, 0, 0))
add_semantics(sphere_prim, "sphere")
sphere = UsdGeom.Sphere(sphere_prim)
sphere.GetRadiusAttr().Set(100)
# Render one frame
await syn.sensors.next_sensor_data_async(self.viewport,True)
data = syn.sensors.get_instance_segmentation(self.viewport)
# np.savez_compressed(self.golden_image_path / 'instance_seg_cube_sphere.npz', array=data)
golden_image = np.load(self.golden_image_path / "instance_seg_cube_sphere.npz")["array"]
std_dev = np.sqrt(np.square(data - golden_image).astype(float).mean())
assert std_dev < 2
async def test_cube_pathtracing(self):
""" Basic funtionality test of the sensor, but in path tracing mode.
"""
settings = carb.settings.get_settings()
settings.set_string("/rtx/rendermode", "PathTracing")
settings.set_int("/rtx/pathtracing/spp", 32)
settings.set_int("/persistent/app/viewport/displayOptions", 0)
stage = omni.usd.get_context().get_stage()
cube = stage.DefinePrim("/Cube", "Cube")
add_semantics(cube, "cube")
cube.GetAttribute("size").Set(100)
# Render one frame
await syn.sensors.next_sensor_data_async(self.viewport,True)
data = syn.sensors.get_instance_segmentation(self.viewport)
# np.savez_compressed(self.golden_image_path / 'instance_seg_cube_pathtracing.npz', array=data)
golden_image = np.load(self.golden_image_path / "instance_seg_cube_pathtracing.npz")["array"]
std_dev = np.sqrt(np.square(data - golden_image).astype(float).mean())
assert std_dev < 2
async def test_cube_ray_traced_lighting(self):
""" Basic funtionality test of the sensor, but in ray traced lighting.
"""
# Set the rendering mode to be ray traced lighting.
settings_interface = carb.settings.get_settings()
settings_interface.set_string("/rtx/rendermode", "RayTracedLighting")
stage = omni.usd.get_context().get_stage()
cube = stage.DefinePrim("/Cube", "Cube")
add_semantics(cube, "cube")
cube.GetAttribute("size").Set(100)
# Render one frame
await syn.sensors.next_sensor_data_async(self.viewport,True)
data = syn.sensors.get_instance_segmentation(self.viewport)
# np.savez_compressed(self.golden_image_path / 'instance_seg_cube_ray_traced_lighting.npz', array=data)
golden_image = np.load(self.golden_image_path / "instance_seg_cube_ray_traced_lighting.npz")["array"]
std_dev = np.sqrt(np.square(data - golden_image).astype(float).mean())
assert std_dev < 2
async def test_cube_ftheta(self):
""" Basic funtionality test of the sensor under ftheta camera.
"""
settings = carb.settings.get_settings()
settings.set_string("/rtx/rendermode", "PathTracing")
settings.set_int("/rtx/pathtracing/spp", 32)
settings.set_int("/persistent/app/viewport/displayOptions", 0)
stage = omni.usd.get_context().get_stage()
cube = stage.DefinePrim("/Cube", "Cube")
add_semantics(cube, "cube")
cube.GetAttribute("size").Set(100)
await omni.kit.app.get_app().next_update_async()
camera = stage.DefinePrim("/Camera", "Camera")
# Set the camera to be polynomial fish eye camera.
camera.CreateAttribute("cameraProjectionType", Sdf.ValueTypeNames.Token).Set("fisheyePolynomial")
# Set the Camera's position
UsdGeom.Xformable(camera).AddTranslateOp().Set((100, 100, 100))
self.viewport.camera_path = camera.GetPath()
# Render one frame
await syn.sensors.next_sensor_data_async(self.viewport,True)
data = syn.sensors.get_instance_segmentation(self.viewport)
# np.savez_compressed(self.golden_image_path / 'instance_seg_cube_ftheta.npz', array=data)
golden_image = np.load(self.golden_image_path / "instance_seg_cube_ftheta.npz")["array"]
std_dev = np.sqrt(np.square(data - golden_image).astype(float).mean())
assert std_dev < 2
async def test_cube_spherical(self):
""" Basic funtionality test of the sensor under fisheye spherical camera.
"""
settings = carb.settings.get_settings()
settings.set_string("/rtx/rendermode", "PathTracing")
settings.set_int("/rtx/pathtracing/spp", 32)
settings.set_int("/persistent/app/viewport/displayOptions", 0)
stage = omni.usd.get_context().get_stage()
cube = stage.DefinePrim("/Cube", "Cube")
add_semantics(cube, "cube")
cube.GetAttribute("size").Set(100)
await omni.kit.app.get_app().next_update_async()
camera = stage.DefinePrim("/Camera", "Camera")
# Set the camera to be spherical fish eye camera.
camera.CreateAttribute("cameraProjectionType", Sdf.ValueTypeNames.Token).Set("fisheyeSpherical")
# Set the Camera's position
UsdGeom.Xformable(camera).AddTranslateOp().Set((100, 100, 100))
self.viewport.camera_path = camera.GetPath()
# Render one frame
await syn.sensors.next_sensor_data_async(self.viewport,True)
data = syn.sensors.get_instance_segmentation(self.viewport)
# np.savez_compressed(self.golden_image_path / 'instance_seg_cube_spherical.npz', array=data)
golden_image = np.load(self.golden_image_path / "instance_seg_cube_spherical.npz")["array"]
std_dev = np.sqrt(np.square(data - golden_image).astype(float).mean())
assert std_dev < 2
@unittest.skip("OM-46393")
async def test_geom_subset(self):
""" Test sensor on GeomSubset.
"""
path = os.path.join(FILE_DIR, "../data/scenes/streetlamp_03_golden.usd")
await omni.usd.get_context().open_stage_async(path)
# Render one frame
await syn.sensors.next_sensor_data_async(self.viewport,True)
data = syn.sensors.get_instance_segmentation(self.viewport)
assert len(data) != 0
async def test_instance_seg_scene_instance(self):
""" Test sensor on scene instance.
"""
settings = carb.settings.get_settings()
path = os.path.join(FILE_DIR, "../data/scenes/scene_instance_test.usda")
await omni.usd.get_context().open_stage_async(path)
await omni.kit.app.get_app().next_update_async()
await syn.sensors.create_or_retrieve_sensor_async(
self.viewport, syn._syntheticdata.SensorType.InstanceSegmentation
)
# Render one frame
await syn.sensors.next_sensor_data_async(self.viewport,True)
data = syn.sensors.get_instance_segmentation(self.viewport)
assert len(data) != 0
async def test_instance_seg_scene_instance_benchchair(self):
""" Test sensor on scene instanced bench and chair data.
"""
settings = carb.settings.get_settings()
path = os.path.join(FILE_DIR, "../data/scenes/BenchChair_SceneInstance_Mini.usda")
await omni.usd.get_context().open_stage_async(path)
await omni.kit.app.get_app().next_update_async()
await syn.sensors.create_or_retrieve_sensor_async(
self.viewport, syn._syntheticdata.SensorType.InstanceSegmentation
)
# Render one frame
await syn.sensors.next_sensor_data_async(self.viewport,True)
data = syn.sensors.get_instance_segmentation(self.viewport,parsed=True)
assert len(data) != 0
# should be 4 semantic objects in the scene.
assert data.max() == 4
async def test_instance_seg_point_instance_benchchair(self):
""" Test sensor on point instanced bench and chair data.
"""
settings = carb.settings.get_settings()
path = os.path.join(FILE_DIR, "../data/scenes/BenchChair_Mini.usda")
await omni.usd.get_context().open_stage_async(path)
await omni.kit.app.get_app().next_update_async()
await syn.sensors.create_or_retrieve_sensor_async(
self.viewport, syn._syntheticdata.SensorType.InstanceSegmentation
)
# Render one frame
await syn.sensors.next_sensor_data_async(self.viewport,True)
data = syn.sensors.get_instance_segmentation(self.viewport,parsed=True)
assert len(data) != 0
assert data.max() == 2
async def test_instance_seg_point_instance_shapes(self):
""" Test sensor on point instanced shapes that have semantics on the mesh.
"""
settings = carb.settings.get_settings()
path = os.path.join(FILE_DIR, "../data/scenes/point_instancer_semantic_shapes.usda")
await omni.usd.get_context().open_stage_async(path)
await omni.kit.app.get_app().next_update_async()
await syn.sensors.create_or_retrieve_sensor_async(
self.viewport, syn._syntheticdata.SensorType.InstanceSegmentation
)
# Render one frame
await syn.sensors.next_sensor_data_async(self.viewport,True)
data = syn.sensors.get_instance_segmentation(self.viewport,parsed=True)
assert len(data) != 0
# After running each test
async def tearDown(self):
settings = carb.settings.get_settings()
settings.set_bool("syntheticdata/sensors/perSubsetSegmentation", True)
pass
| 12,865 | Python | 41.183606 | 141 | 0.649825 |
eliabntt/GRADE-RR/isaac_internals/kit/extscore/omni.syntheticdata/omni/syntheticdata/tests/helpers/test_projection.py | # NOTE:
# omni.kit.test - std python's unittest module with additional wrapping to add suport for async/await tests
# For most things refer to unittest docs: https://docs.python.org/3/library/unittest.html
import os
import math
import asyncio
from time import time
import carb
import numpy as np
import omni.kit.test
from omni.kit.viewport.utility import get_active_viewport
from pxr import Sdf, UsdGeom, Vt
# Import extension python module we are testing with absolute import path, as if we are external user (other extension)
import omni.syntheticdata as syn
from ..utils import add_semantics
FILE_DIR = os.path.dirname(os.path.realpath(__file__))
# Having a test class derived from omni.kit.test.AsyncTestCase declared on the root of module will make it auto-discoverable by omni.kit.test
class TestProjection(omni.kit.test.AsyncTestCase):
# Before running each test
async def setUp(self):
await omni.usd.get_context().new_stage_async()
# Setup viewport
self.viewport = get_active_viewport()
self.stage = omni.usd.get_context().get_stage()
prim = self.stage.DefinePrim("/World", "Xform")
self.stage.SetDefaultPrim(prim)
cube = self.stage.DefinePrim("/World/Cube", "Cube")
add_semantics(cube, "cube")
usd_camera = UsdGeom.Camera.Define(self.stage, "/World/Camera")
usd_camera.AddTranslateOp()
self.camera = usd_camera.GetPrim()
self.camera.CreateAttribute("cameraProjectionType", Sdf.ValueTypeNames.Token).Set(Vt.Token("pinhole"))
self.camera.CreateAttribute("fthetaWidth", Sdf.ValueTypeNames.Float).Set(960)
self.camera.CreateAttribute("fthetaHeight", Sdf.ValueTypeNames.Float).Set(604)
self.camera.CreateAttribute("fthetaCx", Sdf.ValueTypeNames.Float).Set(460)
self.camera.CreateAttribute("fthetaCy", Sdf.ValueTypeNames.Float).Set(340)
self.camera.CreateAttribute("fthetaMaxFov", Sdf.ValueTypeNames.Float).Set(200.0)
self.camera.CreateAttribute("fthetaPolyA", Sdf.ValueTypeNames.Float).Set(0.0)
self.camera.CreateAttribute("fthetaPolyB", Sdf.ValueTypeNames.Float).Set(0.0059)
self.camera.CreateAttribute("fthetaPolyC", Sdf.ValueTypeNames.Float).Set(0.0)
self.camera.CreateAttribute("fthetaPolyD", Sdf.ValueTypeNames.Float).Set(0.0)
self.camera.CreateAttribute("fthetaPolyE", Sdf.ValueTypeNames.Float).Set(0.0)
self.viewport.camera_path = self.camera.GetPath()
syn.sensors.enable_sensors(self.viewport, [syn._syntheticdata.SensorType.BoundingBox3D])
await syn.sensors.next_sensor_data_async(self.viewport, True)
async def test_pinhole(self):
""" Test pinhole projection
"""
self.camera.GetAttribute("xformOp:translate").Set((0.0, 0.0, 9.0))
# Render one frame
await syn.sensors.next_sensor_data_async(self.viewport,True)
# Get 3D bbox
bbox3d = syn.sensors.get_bounding_box_3d(self.viewport, return_corners=True, parsed=True)
# Project corners
corners = bbox3d["corners"]
projected = syn.helpers.world_to_image(corners.reshape(-1, 3), self.viewport).reshape(-1, 8, 3)
# GT
# Confirmed visually to be correct
GT = [
[
[0.26139346, 0.9241894, 0.9000009],
[0.73860654, 0.9241894, 0.9000009],
[0.26139346, 0.0758106, 0.9000009],
[0.73860654, 0.0758106, 0.9000009],
[0.20174183, 1.03023675, 0.87500088],
[0.79825817, 1.03023675, 0.87500088],
[0.20174183, -0.03023675, 0.87500088],
[0.79825817, -0.03023675, 0.87500088],
]
]
# Validate
assert np.allclose(GT, projected)
async def test_fisheye_polynomial(self):
""" Test fisheye polynomial projection (F-Theta)
"""
self.camera.GetAttribute("xformOp:translate").Set((0.0, 0.0, 3.0))
self.camera.GetAttribute("cameraProjectionType").Set(Vt.Token("fisheyePolynomial"))
# Render one frame
await syn.sensors.next_sensor_data_async(self.viewport, True)
# Get 3D bbox
bbox3d = syn.sensors.get_bounding_box_3d(self.viewport, return_corners=True, parsed=True)
# Project corners
corners = bbox3d["corners"]
projected = syn.helpers.world_to_image(corners.reshape(-1, 3), self.viewport).reshape(-1, 8, 3)
# GT
# Confirmed visually to be correct
GT = [
[
[0.43674065, 0.6457944, 0.0],
[0.52159268, 0.6457944, 0.0],
[0.43674065, 0.49494634, 0.0],
[0.52159268, 0.49494634, 0.0],
[0.40232877, 0.70697108, 0.0],
[0.55600456, 0.70697108, 0.0],
[0.40232877, 0.43376967, 0.0],
[0.55600456, 0.43376967, 0.0],
]
]
# Validate
assert np.allclose(GT, projected)
# Run the operation in reverse
view_params = syn.helpers.get_view_params(self.viewport)
proj_i2w = projected[0, :, :2]
proj_i2w[..., 0] *= view_params["width"]
proj_i2w[..., 1] *= view_params["height"]
origin, directions = syn.helpers.image_to_world(proj_i2w, view_params)
gt_corner_directions = corners[0] - origin
gt_corner_directions /= np.linalg.norm(gt_corner_directions, axis=1, keepdims=True)
assert np.allclose(gt_corner_directions, directions)
# FOR VISUAL DEBUGGING
self.camera.GetAttribute("clippingRange").Set((0.1, 1000000))
for i, d in enumerate(directions):
s = self.stage.DefinePrim(f"/World/pt{i}", "Sphere")
UsdGeom.Xformable(s).AddTranslateOp().Set(tuple((d + origin).tolist()))
s.GetAttribute("radius").Set(0.03)
await syn.sensors.next_sensor_data_async(self.viewport,True)
async def test_fisheye_polynomial_edge(self):
""" Test fisheye polynomial projection (F-Theta) at edge of FOV
"""
self.camera.GetAttribute("xformOp:translate").Set((4.0, 0.0, 0.5))
self.camera.GetAttribute("cameraProjectionType").Set(Vt.Token("fisheyePolynomial"))
# Render one frame
await syn.sensors.next_sensor_data_async(self.viewport, True)
# Get 3D bbox
bbox3d = syn.sensors.get_bounding_box_3d(self.viewport, return_corners=True, parsed=True)
# Project corners
corners = bbox3d["corners"]
projected = syn.helpers.world_to_image(corners.reshape(-1, 3), self.viewport).reshape(-1, 8, 3)
# GT
# Confirmed visually to be correct
GT = [
[
[0.25675408, 0.6494504, 0.0],
[0.2902532, 0.68231909, 0.0],
[0.25675408, 0.49129034, 0.0],
[0.2902532, 0.45842165, 0.0],
[0.19030016, 0.67307846, 0.0],
[0.18980286, 0.74184522, 0.0],
[0.19030016, 0.46766228, 0.0],
[0.18980286, 0.39889552, 0.0],
]
]
# Validate
assert np.allclose(GT, projected)
# Run the operation in reverse
view_params = syn.helpers.get_view_params(self.viewport)
proj_i2w = projected[0, :, :2]
proj_i2w[..., 0] *= view_params["width"]
proj_i2w[..., 1] *= view_params["height"]
origin, directions = syn.helpers.image_to_world(proj_i2w, view_params)
gt_corner_directions = corners[0] - origin
gt_corner_directions /= np.linalg.norm(gt_corner_directions, axis=1, keepdims=True)
assert np.allclose(gt_corner_directions, directions)
# FOR VISUAL DEBUGGING
self.camera.GetAttribute("clippingRange").Set((0.1, 1000000))
for i, d in enumerate(directions):
s = self.stage.DefinePrim(f"/World/pt{i}", "Sphere")
UsdGeom.Xformable(s).AddTranslateOp().Set(tuple((d + origin).tolist()))
await syn.sensors.next_sensor_data_async(self.viewport,True)
# After running each test
async def tearDown(self):
pass
| 8,149 | Python | 40.370558 | 141 | 0.618972 |
eliabntt/GRADE-RR/isaac_internals/kit/extscore/omni.syntheticdata/omni/syntheticdata/tests/helpers/test_instance_mapping.py | # NOTE:
# omni.kit.test - std python's unittest module with additional wrapping to add suport for async/await tests
# For most things refer to unittest docs: https://docs.python.org/3/library/unittest.html
import os
import math
import asyncio
from time import time
import carb
import numpy as np
import omni.kit.test
from omni.kit.viewport.utility import get_active_viewport
from pxr import UsdPhysics
# Import extension python module we are testing with absolute import path, as if we are external user (other extension)
import omni.syntheticdata as syn
from ..utils import add_semantics
FILE_DIR = os.path.dirname(os.path.realpath(__file__))
# Having a test class derived from omni.kit.test.AsyncTestCase declared on the root of module will make it auto-discoverable by omni.kit.test
class TestHelpersInstanceMappings(omni.kit.test.AsyncTestCase):
# Before running each test
async def setUp(self):
# Setup viewport
self.viewport = get_active_viewport()
await omni.usd.get_context().new_stage_async()
self.stage = omni.usd.get_context().get_stage()
prim = self.stage.DefinePrim("/World", "Xform")
self.stage.SetDefaultPrim(prim)
async def test_non_semantic_schemas(self):
""" Test mixture of applied schemas including non-semantics.
"""
prim = self.stage.DefinePrim("/World/Cone", "Cone")
# Add semantics schema
add_semantics(prim, "Je ne suis pas un cone.")
# Non-semantics schema
UsdPhysics.RigidBodyAPI.Apply(prim)
await syn.sensors.next_sensor_data_async(self.viewport,True)
# Get instance mappings
instance_mappings = syn.helpers.get_instance_mappings()
# Validate
cone_im = instance_mappings[0]
assert cone_im["uniqueId"] == 1
assert cone_im["name"] == "/World/Cone"
assert cone_im["semanticId"] == 1
assert cone_im["semanticLabel"] == "Je ne suis pas un cone."
# After running each test
async def tearDown(self):
pass
| 2,054 | Python | 31.619047 | 141 | 0.686465 |
eliabntt/GRADE-RR/isaac_internals/kit/extscore/omni.syntheticdata/omni/syntheticdata/tests/helpers/test_bboxes.py | # NOTE:
# omni.kit.test - std python's unittest module with additional wrapping to add suport for async/await tests
# For most things refer to unittest docs: https://docs.python.org/3/library/unittest.html
import os
import math
import asyncio
from time import time
import carb
import numpy as np
import omni.kit.test
from omni.kit.viewport.utility import get_active_viewport
from pxr import Sdf, UsdGeom, Vt
# Import extension python module we are testing with absolute import path, as if we are external user (other extension)
import omni.syntheticdata as syn
from ..utils import add_semantics
FILE_DIR = os.path.dirname(os.path.realpath(__file__))
# Having a test class derived from omni.kit.test.AsyncTestCase declared on the root of module will make it auto-discoverable by omni.kit.test
class TestBBoxes(omni.kit.test.AsyncTestCase):
# Before running each test
async def setUp(self):
await omni.usd.get_context().new_stage_async()
# Setup viewport
self.viewport = get_active_viewport()
await omni.usd.get_context().new_stage_async()
self.stage = omni.usd.get_context().get_stage()
prim = self.stage.DefinePrim("/World", "Xform")
self.stage.SetDefaultPrim(prim)
marked_cube = self.stage.DefinePrim("/World/MarkedCube0", "Cube")
add_semantics(marked_cube, "cube")
marked_cube.GetAttribute("size").Set(100)
UsdGeom.XformCommonAPI(marked_cube).SetTranslate((3, 3, 0))
unmarked_cube = self.stage.DefinePrim("/World/UnmarkedCube", "Cube")
unmarked_cube.GetAttribute("size").Set(100)
UsdGeom.XformCommonAPI(unmarked_cube).SetTranslate((3, 3, -100))
await omni.kit.app.get_app().next_update_async()
syn.sensors.enable_sensors(self.viewport, [syn._syntheticdata.SensorType.BoundingBox2DLoose])
syn.sensors.enable_sensors(self.viewport, [syn._syntheticdata.SensorType.BoundingBox2DTight])
syn.sensors.enable_sensors(self.viewport, [syn._syntheticdata.SensorType.BoundingBox3D])
syn.sensors.enable_sensors(self.viewport, [syn._syntheticdata.SensorType.Occlusion])
async def test_reduce_bboxes_3d(self):
"""Verify that reduce_bboxes_3d removes a cube without a semantic label"""
# Render one frame
await syn.sensors.next_sensor_data_async(self.viewport,True)
# Get 3D bbox
bbox = syn.sensors.get_bounding_box_3d(self.viewport, return_corners=True)
assert np.allclose(bbox["z_min"], [-50, -50])
# Transform of unmarked cube should be included in pre-reduced bbox but not included in reduced bbox
UNMARKED_CUBE_GT = [[[1.0, 0.0, 0.0, 0.0], [0.0, 1.0, 0.0, 0.0], [0.0, 0.0, 1.0, 0.0], [3.0, 3.0, -100.0, 1.0]]]
assert np.allclose(bbox["transform"][0], UNMARKED_CUBE_GT) or np.allclose(
bbox["transform"][1], UNMARKED_CUBE_GT
)
instance_mappings = syn.helpers.get_instance_mappings()
bbox_reduced = syn.helpers.reduce_bboxes_3d(bbox, instance_mappings)
assert np.allclose(bbox_reduced["z_min"], [-50])
assert np.allclose(
bbox_reduced["transform"],
[[[1.0, 0.0, 0.0, 0.0], [0.0, 1.0, 0.0, 0.0], [0.0, 0.0, 1.0, 0.0], [3.0, 3.0, 0.0, 1.0]]],
)
async def test_reduce_occlusion(self):
"""Verify that reduce_occlusion removes a cube without a semantic label"""
# Add an extra cube
cube = self.stage.DefinePrim("/World/MarkedCube1", "Cube")
add_semantics(cube, "cube")
cube.GetAttribute("size").Set(100)
UsdGeom.XformCommonAPI(cube).SetTranslate((3, -10, 0))
# Render one frame
await syn.sensors.next_sensor_data_async(self.viewport, True)
# Get occlusion
occlusion = syn.sensors.get_occlusion(self.viewport)
occlusion_ratios = np.sort(occlusion["occlusionRatio"])
assert np.allclose(occlusion_ratios, [0.0327, 0.38059998, 0.8886], atol=0.05)
instance_mappings = syn.helpers.get_instance_mappings()
reduced_occlusion = syn.helpers.reduce_occlusion(occlusion, instance_mappings)
reduced_occlusion_ratios = np.sort(reduced_occlusion["occlusionRatio"])
assert np.allclose(reduced_occlusion_ratios, [0.0327, 0.8886], atol=0.05)
async def test_merge_sensors(self):
"""Verify that merge_sensors merges the data correctly"""
# Render one frame
await syn.sensors.next_sensor_data_async(self.viewport, True)
# Get bounding boxes and merge
bounding_box_2d_tight = syn.sensors.get_bounding_box_2d_tight(self.viewport)
bounding_box_2d_loose = syn.sensors.get_bounding_box_2d_loose(self.viewport)
bounding_box_3d = syn.sensors.get_bounding_box_3d(self.viewport, parsed=True)
merged_data = syn.helpers.merge_sensors(bounding_box_2d_tight, bounding_box_2d_loose, bounding_box_3d)
for suffix, data_source in [
("_bbox2d_tight", bounding_box_2d_tight),
("_bbox2d_loose", bounding_box_2d_loose),
("_bbox3d", bounding_box_3d),
]:
suffix_present = False
for key in merged_data.dtype.fields:
if key.endswith(suffix):
sub_key = key[: -len(suffix)]
assert merged_data[key] == data_source[key]
suffix_present = True
assert suffix_present
# After running each test
async def tearDown(self):
pass
| 5,491 | Python | 43.290322 | 141 | 0.65489 |
eliabntt/GRADE-RR/isaac_internals/kit/extscore/omni.syntheticdata/omni/syntheticdata/tests/visualize/test_warp_post_vis.py | # NOTE:
# omni.kit.test - std python's unittest module with additional wrapping to add suport for async/await tests
# For most things refer to unittest docs: https://docs.python.org/3/library/unittest.html
import os
import carb
from pxr import Gf, UsdGeom, UsdLux, Sdf
import unittest
import omni.kit.test
from omni.syntheticdata import SyntheticData, SyntheticDataStage
from ..utils import add_semantics
class TestWarpPostVisualization(omni.kit.test.AsyncTestCase):
def __init__(self, methodName: str) -> None:
super().__init__(methodName=methodName)
async def setUp(self):
# Setup the scene
await omni.usd.get_context().new_stage_async()
stage = omni.usd.get_context().get_stage()
world_prim = UsdGeom.Xform.Define(stage,"/World")
UsdGeom.Xformable(world_prim).AddTranslateOp().Set((0, 0, 0))
UsdGeom.Xformable(world_prim).AddRotateXYZOp().Set((0, 0, 0))
capsule0_prim = stage.DefinePrim("/World/Capsule0", "Capsule")
add_semantics(capsule0_prim, "capsule_0")
UsdGeom.Xformable(capsule0_prim).AddTranslateOp().Set((100, 0, 0))
UsdGeom.Xformable(capsule0_prim).AddScaleOp().Set((30, 30, 30))
UsdGeom.Xformable(capsule0_prim).AddRotateXYZOp().Set((-90, 0, 0))
capsule0_prim.GetAttribute("primvars:displayColor").Set([(0.3, 1, 0)])
capsule1_prim = stage.DefinePrim("/World/Capsule1", "Capsule")
add_semantics(capsule0_prim, "capsule_1")
UsdGeom.Xformable(capsule1_prim).AddTranslateOp().Set((-100, 0, 0))
UsdGeom.Xformable(capsule1_prim).AddScaleOp().Set((30, 30, 30))
UsdGeom.Xformable(capsule1_prim).AddRotateXYZOp().Set((-90, 0, 0))
capsule1_prim.GetAttribute("primvars:displayColor").Set([(0, 1, 0.3)])
spherelight = UsdLux.SphereLight.Define(stage, "/SphereLight")
spherelight.GetIntensityAttr().Set(30000)
spherelight.GetRadiusAttr().Set(30)
# Setup viewports / renderproduct
vp_iface = omni.kit.viewport_legacy.get_viewport_interface()
viewport = vp_iface.get_viewport_window()
render_product_path = viewport.get_render_product_path()
# SyntheticData singleton interface
sdg_iface = SyntheticData.Get()
if not sdg_iface.is_node_template_registered("TestWarpPostVisualization"):
sdg_iface.register_node_template(
SyntheticData.NodeTemplate(
SyntheticDataStage.ON_DEMAND, # node tempalte stage
"omni.syntheticdata.SdTestWarpPostVisulation", # node template type
# node template connections
[
SyntheticData.NodeConnectionTemplate("LdrColorSDExportRawArray"),
]),
template_name="TestWarpPostVisualization" # node template name
)
if not sdg_iface.is_node_template_registered("TestWarpPostVisualizationDisplay"):
sdg_iface.register_node_template(
SyntheticData.NodeTemplate(
SyntheticDataStage.ON_DEMAND, # node tempalte stage
"omni.syntheticdata.SdLinearArrayToTexture", # node template type
# node template connections
[
SyntheticData.NodeConnectionTemplate("TestWarpPostVisualization"),
]),
template_name="TestWarpPostVisualizationDisplay" # node template name
)
sdg_iface.activate_node_template("TestWarpPostVisualizationDisplay", 0, [render_product_path])
self.numLoops = 100
async def run_loop(self):
# ensuring that the setup is taken into account
for _ in range(5):
await omni.kit.app.get_app().next_update_async()
for _ in range(self.numLoops):
await omni.kit.app.get_app().next_update_async()
async def test_display(self):
""" Test display
"""
await self.run_loop()
async def tearDown(self):
pass
| 4,156 | Python | 40.989899 | 109 | 0.619105 |
eliabntt/GRADE-RR/isaac_internals/kit/extscore/omni.syntheticdata/omni/syntheticdata/tests/visualize/test_flattener.py | # NOTE:
# omni.kit.test - std python's unittest module with additional wrapping to add suport for async/await tests
# For most things refer to unittest docs: https://docs.python.org/3/library/unittest.html
import os
import math
from time import time
import carb
import numpy as np
import omni.kit.test
from omni.kit.viewport.utility import get_active_viewport
# Import extension python module we are testing with absolute import path, as if we are external user (other extension)
import omni.syntheticdata as syn
FILE_DIR = os.path.dirname(os.path.realpath(__file__))
TIMEOUT = 50
BAKE_ACCURACY_THRESHOLD = 0.9
# segmentation mask testing against inputs of different resolutions
def test_against_golden(semantic_data, golden_semantic_data):
input_dim = semantic_data.shape
golden_dim = golden_semantic_data.shape
correct_count = 0
for y in range(0, input_dim[0]):
for x in range(0, input_dim[1]):
u = x / input_dim[1]
v = y / input_dim[0]
sample_x = math.floor(u * golden_dim[1])
sample_y = math.floor(v * golden_dim[0])
if semantic_data[y, x] == golden_semantic_data[sample_y, sample_x]:
correct_count += 1
return correct_count / (input_dim[0] * input_dim[1])
# Having a test class derived from omni.kit.test.AsyncTestCase declared on the root of module will make it auto-discoverable by omni.kit.test
class TestFlattenerSegmentationBakingVis(omni.kit.test.AsyncTestCase):
# Before running each test
async def setUp(self):
np.random.seed(1234)
# Setup viewport
self.viewport = get_active_viewport()
await omni.kit.app.get_app_interface().next_update_async()
filepath = os.path.join(FILE_DIR, "../data/scenes/OmniUe4-benchmark.usda")
usd_context = omni.usd.get_context()
await usd_context.open_stage_async(filepath)
await omni.kit.app.get_app().next_update_async()
syn.sensors.enable_sensors(self.viewport, [syn._syntheticdata.SensorType.SemanticSegmentation])
async def _wait_for_data(self):
data = np.empty(0)
start = time()
settings = carb.settings.get_settings()
# wait until flattener is done loading in assets
while not settings.get_as_bool("/app/captureFrame/ready"):
await omni.kit.app.get_app_interface().next_update_async()
# stall a couple of frames until samplerFeedback kicks off baking work.
# NOTE: If we don't stall here, then we won't bake at all, because the ready flag will be falsely set
# since samplerFeedback hasn't seen any tiles yet, so flattener thinks scene is ready for capture.
for i in range(0, 20):
await omni.kit.app.get_app_interface().next_update_async()
# wait until baking to be done
while not settings.get_as_bool("/app/captureFrame/ready"):
await omni.kit.app.get_app_interface().next_update_async()
async def test_baking(self):
""" Test that flattener correctly bakes semantic information into vtex
"""
settings = carb.settings.get_settings()
settings.set("/app/hydraEngine/waitIdle", True)
# start flattener baking
settings.set("/rtx/materialflattener/bake", True)
settings.set("/rtx/materialflattener/rebaking", True)
await omni.kit.app.get_app_interface().next_update_async()
await self._wait_for_data()
await syn.sensors.next_sensor_data_async(self.viewport)
semantic_data = syn.sensors.get_semantic_segmentation(self.viewport)
unique_classes = np.unique(semantic_data)
# visual debug code
#from PIL import Image
#semantic_image = syn.visualize.colorize_segmentation(semantic_data)
#semantic_image = np.uint8(semantic_image[:,:,:3])
#im = Image.fromarray(semantic_image)
#im.save('/home/chen/work/debug_segmentation.png')
golden_filepath = os.path.join(FILE_DIR, "../data/golden/baked_segmentation.npz")
golden_semantic_data = np.load(golden_filepath)["array"]
unique_classes = np.unique(semantic_data)
carb.log_warn(f'unique classes = {unique_classes}')
assert len(unique_classes) == 3
if len(unique_classes) == 3:
accuracy = test_against_golden(semantic_data, golden_semantic_data)
carb.log_warn(f'1st accuracy = {accuracy}')
# it's possible semantic labels are flipped between road and lanemark, so redo the test
# if accuracy is strikingly low
if accuracy < BAKE_ACCURACY_THRESHOLD:
for y in range(0, semantic_data.shape[0]):
for x in range(0, semantic_data.shape[1]):
# flip classes
if semantic_data[y, x] == unique_classes[1]:
semantic_data[y, x] = unique_classes[2]
elif semantic_data[y, x] == unique_classes[2]:
semantic_data[y, x] = unique_classes[1]
accuracy = test_against_golden(semantic_data, golden_semantic_data)
# visual debug code
#semantic_image = syn.visualize.colorize_segmentation(semantic_data)
#semantic_image = np.uint8(semantic_image[:,:,:3])
#im = Image.fromarray(semantic_image)
#im.save('/home/chen/work/debug_segmentation_2nd_try.png')
carb.log_warn(f'2nd accuracy = {accuracy}')
assert accuracy >= BAKE_ACCURACY_THRESHOLD
# After running each test
async def tearDown(self):
pass | 5,665 | Python | 42.584615 | 141 | 0.639188 |
eliabntt/GRADE-RR/isaac_internals/kit/extscore/omni.syntheticdata/omni/syntheticdata/tests/visualize/test_post_vis.py | # NOTE:
# omni.kit.test - std python's unittest module with additional wrapping to add suport for async/await tests
# For most things refer to unittest docs: https://docs.python.org/3/library/unittest.html
import os
import carb
from pxr import Gf, UsdGeom, UsdLux, Sdf
import unittest
import omni.kit.test
from omni.syntheticdata import SyntheticData, SyntheticDataStage
from ..utils import add_semantics
class TestPostVisualization(omni.kit.test.AsyncTestCase):
def __init__(self, methodName: str) -> None:
super().__init__(methodName=methodName)
def activate_post_vis(self,render_product_path, render_var):
sdg_iface = SyntheticData.Get()
render_var_post_display = "Test" + render_var + "PostDisplay"
if not sdg_iface.is_node_template_registered(render_var_post_display):
sdg_iface.register_node_template(
SyntheticData.NodeTemplate(
SyntheticDataStage.ON_DEMAND, # node tempalte stage
"omni.syntheticdata.SdLinearArrayToTexture", # node template type
# node template connections
[
SyntheticData.NodeConnectionTemplate(render_var),
]),
template_name=render_var_post_display
)
sdg_iface.activate_node_template(render_var_post_display, 0, [render_product_path])
async def setUp(self):
# Setup the scene
await omni.usd.get_context().new_stage_async()
stage = omni.usd.get_context().get_stage()
world_prim = UsdGeom.Xform.Define(stage,"/World")
UsdGeom.Xformable(world_prim).AddTranslateOp().Set((0, 0, 0))
UsdGeom.Xformable(world_prim).AddRotateXYZOp().Set((0, 0, 0))
capsule0_prim = stage.DefinePrim("/World/Capsule0", "Capsule")
add_semantics(capsule0_prim, "capsule_0")
UsdGeom.Xformable(capsule0_prim).AddTranslateOp().Set((100, 0, 0))
UsdGeom.Xformable(capsule0_prim).AddScaleOp().Set((30, 30, 30))
UsdGeom.Xformable(capsule0_prim).AddRotateXYZOp().Set((-90, 0, 0))
capsule0_prim.GetAttribute("primvars:displayColor").Set([(0.3, 1, 0)])
capsule1_prim = stage.DefinePrim("/World/Capsule1", "Capsule")
add_semantics(capsule0_prim, "capsule_1")
UsdGeom.Xformable(capsule1_prim).AddTranslateOp().Set((-100, 0, 0))
UsdGeom.Xformable(capsule1_prim).AddScaleOp().Set((30, 30, 30))
UsdGeom.Xformable(capsule1_prim).AddRotateXYZOp().Set((-90, 0, 0))
capsule1_prim.GetAttribute("primvars:displayColor").Set([(0, 1, 0.3)])
spherelight = UsdLux.SphereLight.Define(stage, "/SphereLight")
spherelight.GetIntensityAttr().Set(30000)
spherelight.GetRadiusAttr().Set(30)
# Setup viewports / renderproduct
vp_iface = omni.kit.viewport_legacy.get_viewport_interface()
viewport = vp_iface.get_viewport_window()
render_product_path = viewport.get_render_product_path()
self.activate_post_vis("LdrColorSD")
self.numLoops = 100
async def run_loop(self):
# ensuring that the setup is taken into account
for _ in range(5):
await omni.kit.app.get_app().next_update_async()
for _ in range(self.numLoops):
await omni.kit.app.get_app().next_update_async()
async def test_display(self):
""" Test display
"""
await self.run_loop()
async def tearDown(self):
pass
| 3,587 | Python | 38.866666 | 109 | 0.624756 |
eliabntt/GRADE-RR/isaac_internals/kit/extscore/omni.syntheticdata/omni/syntheticdata/tests/visualize/test_semantic_seg.py | # NOTE:
# omni.kit.test - std python's unittest module with additional wrapping to add suport for async/await tests
# For most things refer to unittest docs: https://docs.python.org/3/library/unittest.html
import os
import numpy as np
import omni.kit.test
from omni.kit.viewport.utility import get_active_viewport
from pxr import UsdGeom
# Import extension python module we are testing with absolute import path, as if we are external user (other extension)
import omni.syntheticdata as syn
from ..utils import add_semantics
FILE_DIR = os.path.dirname(os.path.realpath(__file__))
TIMEOUT = 50
# Having a test class derived from omni.kit.test.AsyncTestCase declared on the root of module will make it auto-discoverable by omni.kit.test
class TestSemanticSegVis(omni.kit.test.AsyncTestCase):
# Before running each test
async def setUp(self):
np.random.seed(1234)
# Setup viewport
self.viewport = get_active_viewport()
# Initialize Sensor
await omni.usd.get_context().new_stage_async()
stage = omni.usd.get_context().get_stage()
await omni.kit.app.get_app().next_update_async()
syn.sensors.enable_sensors(
self.viewport,
[syn._syntheticdata.SensorType.SemanticSegmentation, syn._syntheticdata.SensorType.InstanceSegmentation],
)
async def test_parsed_empty(self):
""" Test semantic segmentation returns zero array with empty scene
"""
await syn.sensors.next_sensor_data_async(self.viewport, True)
data = syn.visualize.get_semantic_segmentation(self.viewport, mode="parsed")
assert np.array_equal(data, np.zeros_like(data).astype(np.uint8))
async def test_number_of_classes(self):
""" Test that number of classes in output matches number of classes in scene
"""
stage = omni.usd.get_context().get_stage()
cube = stage.DefinePrim("/Cube1", "Cube")
add_semantics(cube, "cube1")
UsdGeom.Xformable(cube).AddTranslateOp().Set((0, 10, 0))
cube = stage.DefinePrim("/Cube2", "Cube")
add_semantics(cube, "cube2")
UsdGeom.Xformable(cube).AddTranslateOp().Set((0, -10, 0))
await syn.sensors.next_sensor_data_async(self.viewport, True)
data = syn.visualize.get_semantic_segmentation(self.viewport, mode="parsed")
data_non_bkg = data[data.sum(axis=-1) != 0] # Remove background, encoded as (0, 0, 0, 0)
assert len(np.unique(data_non_bkg, axis=0)) == 2
# After running each test
async def tearDown(self):
pass
| 2,597 | Python | 39.593749 | 141 | 0.676165 |
eliabntt/GRADE-RR/isaac_internals/kit/extscore/omni.syntheticdata/omni/syntheticdata/tests/graph/test_graph_manipulation.py | import carb
from pxr import Gf, UsdGeom, UsdLux, Sdf
import omni.hydratexture
import omni.kit.test
from omni.syntheticdata import SyntheticData, SyntheticDataStage
# Test the instance mapping pipeline
class TestGraphManipulation(omni.kit.test.AsyncTestCase):
def __init__(self, methodName: str) -> None:
super().__init__(methodName=methodName)
def render_product_path(self, hydra_texture) -> str:
'''Return a string to the UsdRender.Product used by the texture'''
render_product = hydra_texture.get_render_product_path()
if render_product and (not render_product.startswith('/')):
render_product = '/Render/RenderProduct_' + render_product
return render_product
async def setUp(self):
self._settings = carb.settings.acquire_settings_interface()
self._hydra_texture_factory = omni.hydratexture.acquire_hydra_texture_factory_interface()
self._usd_context_name = ''
self._usd_context = omni.usd.get_context(self._usd_context_name)
await self._usd_context.new_stage_async()
self._stage = omni.usd.get_context().get_stage()
# renderer
renderer = "rtx"
if renderer not in self._usd_context.get_attached_hydra_engine_names():
omni.usd.add_hydra_engine(renderer, self._usd_context)
# create the hydra textures
self._hydra_texture_0 = self._hydra_texture_factory.create_hydra_texture(
"TEX0",
1920,
1080,
self._usd_context_name,
hydra_engine_name=renderer,
is_async=self._settings.get("/app/asyncRendering")
)
self._render_product_path_0 = self.render_product_path(self._hydra_texture_0)
self._hydra_texture_rendered_counter = 0
def on_hydra_texture_0(event: carb.events.IEvent):
self._hydra_texture_rendered_counter += 1
self._hydra_texture_rendered_counter_sub = self._hydra_texture_0.get_event_stream().create_subscription_to_push_by_type(
omni.hydratexture.EVENT_TYPE_DRAWABLE_CHANGED,
on_hydra_texture_0,
name='async rendering test drawable update',
)
async def tearDown(self):
self._hydra_texture_rendered_counter_sub = None
self._hydra_texture_0 = None
self._usd_context.close_stage()
omni.usd.release_all_hydra_engines(self._usd_context)
self._hydra_texture_factory = None
self._settings = None
wait_iterations = 6
for _ in range(wait_iterations):
await omni.kit.app.get_app().next_update_async()
async def test_rendervar_enable(self):
isdg = SyntheticData.Get()
render_var = "BoundingBox3DSD"
assert(not isdg.is_rendervar_enabled(self._render_product_path_0, render_var, False, self._stage))
assert(not isdg.is_rendervar_used(self._render_product_path_0, render_var))
isdg.enable_rendervar(self._render_product_path_0, render_var, self._stage)
assert(isdg.is_rendervar_enabled(self._render_product_path_0, render_var, False, self._stage))
assert(not isdg.is_rendervar_used(self._render_product_path_0, render_var))
isdg.disable_rendervar(self._render_product_path_0, render_var, self._stage)
assert(not isdg.is_rendervar_enabled(self._render_product_path_0, render_var, False, self._stage))
assert(not isdg.is_rendervar_used(self._render_product_path_0, render_var))
async def test_rendervar_auto_activation(self):
isdg = SyntheticData.Get()
render_var = "BoundingBox3DSD"
assert(not isdg.is_rendervar_enabled(self._render_product_path_0, render_var, True, self._stage))
assert(not isdg.is_rendervar_used(self._render_product_path_0, render_var))
isdg.activate_node_template("BoundingBox3DReduction",0, [self._render_product_path_0], {}, self._stage, True)
assert(isdg.is_rendervar_enabled(self._render_product_path_0, render_var, False, self._stage))
assert(isdg.is_rendervar_used(self._render_product_path_0, render_var))
assert(isdg.is_rendervar_enabled(self._render_product_path_0, render_var, True, self._stage))
isdg.deactivate_node_template("BoundingBox3DReduction",0, [self._render_product_path_0], self._stage, True)
assert(not isdg.is_rendervar_enabled(self._render_product_path_0, render_var, False, self._stage))
assert(not isdg.is_rendervar_used(self._render_product_path_0, render_var))
async def test_rendervar_manual_activation(self):
isdg = SyntheticData.Get()
render_var = "BoundingBox3DSD"
assert(not isdg.is_rendervar_enabled(self._render_product_path_0, render_var, False, self._stage))
assert(not isdg.is_rendervar_used(self._render_product_path_0, render_var))
assert(not isdg.is_node_template_activated("BoundingBox3DReduction",self._render_product_path_0,False))
isdg.activate_node_template("BoundingBox3DReduction",0, [self._render_product_path_0], {}, self._stage, False)
assert(isdg.is_node_template_activated("BoundingBox3DReduction",self._render_product_path_0,False))
assert(isdg.is_node_template_activated("BoundingBox3DReduction",self._render_product_path_0,True))
assert(not isdg.is_rendervar_enabled(self._render_product_path_0, render_var, False, self._stage))
assert(isdg.is_rendervar_used(self._render_product_path_0, render_var))
isdg.enable_rendervar(self._render_product_path_0, render_var, self._stage)
assert(isdg.is_rendervar_enabled(self._render_product_path_0, render_var, False, self._stage))
assert(isdg.is_rendervar_used(self._render_product_path_0, render_var))
assert(not isdg.is_rendervar_enabled(self._render_product_path_0, render_var, True, self._stage))
isdg.deactivate_node_template("BoundingBox3DReduction",0, [self._render_product_path_0], self._stage, False)
assert(not isdg.is_node_template_activated("BoundingBox3DReduction",self._render_product_path_0,True))
assert(isdg.is_rendervar_enabled(self._render_product_path_0, render_var, False, self._stage))
assert(not isdg.is_rendervar_used(self._render_product_path_0, render_var))
isdg.disable_rendervar(self._render_product_path_0, render_var, self._stage)
assert(not isdg.is_rendervar_enabled(self._render_product_path_0, render_var, False, self._stage))
assert(not isdg.is_rendervar_used(self._render_product_path_0, render_var))
async def test_rendervar_hybrid_activation(self):
isdg = SyntheticData.Get()
render_var = "BoundingBox3DSD"
assert(not isdg.is_rendervar_enabled(self._render_product_path_0, render_var, False, self._stage))
assert(not isdg.is_rendervar_used(self._render_product_path_0, render_var))
isdg.activate_node_template("BoundingBox3DReduction",0, [self._render_product_path_0], {}, self._stage, False)
assert(not isdg.is_rendervar_enabled(self._render_product_path_0, render_var, False, self._stage))
assert(isdg.is_rendervar_used(self._render_product_path_0, render_var))
isdg.enable_rendervar(self._render_product_path_0, render_var, self._stage)
assert(isdg.is_rendervar_enabled(self._render_product_path_0, render_var, False, self._stage))
assert(isdg.is_rendervar_used(self._render_product_path_0, render_var))
isdg.deactivate_node_template("BoundingBox3DReduction",0, [self._render_product_path_0], self._stage, True)
assert(isdg.is_rendervar_enabled(self._render_product_path_0, render_var, False, self._stage))
assert(not isdg.is_rendervar_used(self._render_product_path_0, render_var))
assert(not isdg.is_rendervar_enabled(self._render_product_path_0, render_var, True, self._stage))
isdg.disable_rendervar(self._render_product_path_0, render_var, self._stage)
assert(not isdg.is_rendervar_enabled(self._render_product_path_0, render_var, False, self._stage))
async def test_rendervar_initially_activated(self):
isdg = SyntheticData.Get()
render_var = "BoundingBox3DSD"
assert(not isdg.is_rendervar_enabled(self._render_product_path_0, render_var, False, self._stage))
assert(not isdg.is_rendervar_used(self._render_product_path_0, render_var))
isdg.enable_rendervar(self._render_product_path_0, render_var, self._stage)
assert(isdg.is_rendervar_enabled(self._render_product_path_0, render_var, False, self._stage))
assert(not isdg.is_rendervar_used(self._render_product_path_0, render_var))
isdg.activate_node_template("BoundingBox3DReduction",0, [self._render_product_path_0], {}, self._stage, True)
assert(isdg.is_rendervar_enabled(self._render_product_path_0, render_var, False, self._stage))
assert(isdg.is_rendervar_used(self._render_product_path_0, render_var))
assert(not isdg.is_rendervar_enabled(self._render_product_path_0, render_var, True, self._stage))
isdg.deactivate_node_template("BoundingBox3DReduction",0, [self._render_product_path_0], self._stage, True)
assert(isdg.is_rendervar_enabled(self._render_product_path_0, render_var, False, self._stage))
assert(not isdg.is_rendervar_used(self._render_product_path_0, render_var))
isdg.disable_rendervar(self._render_product_path_0, render_var, self._stage)
assert(not isdg.is_rendervar_enabled(self._render_product_path_0, render_var, False, self._stage))
async def test_rendervar_multiple_activation(self):
isdg = SyntheticData.Get()
render_var = "BoundingBox3DSD"
if not isdg.is_node_template_registered("BoundingBox3DDisplayPostDuplicate"):
isdg.register_node_template(
SyntheticData.NodeTemplate(
SyntheticDataStage.POST_RENDER,
"omni.syntheticdata.SdPostRenderVarDisplayTexture",
[
SyntheticData.NodeConnectionTemplate("LdrColorSD"),
SyntheticData.NodeConnectionTemplate("Camera3dPositionSD"),
SyntheticData.NodeConnectionTemplate("PostRenderProductCamera"),
SyntheticData.NodeConnectionTemplate("InstanceMappingPost"),
SyntheticData.NodeConnectionTemplate("BoundingBox3DReduction")
],
{
"inputs:renderVar": "LdrColorSD",
"inputs:renderVarDisplay": "BoundingBox3DSDDisplay",
"inputs:mode": "semanticBoundingBox3dMode",
"inputs:parameters": [0.0, 5.0, 0.027, 0.27]
}
), # node template default attribute values (when differs from the default value specified in the .ogn)
template_name="BoundingBox3DDisplayPostDuplicate" # node template name
)
assert(not isdg.is_rendervar_enabled(self._render_product_path_0, render_var, False, self._stage))
assert(not isdg.is_rendervar_used(self._render_product_path_0, render_var))
isdg.activate_node_template("BoundingBox3DDisplayPost",0, [self._render_product_path_0], {}, self._stage, True)
assert(not isdg.is_node_template_activated("BoundingBox3DReduction",self._render_product_path_0,True))
assert(isdg.is_node_template_activated("BoundingBox3DReduction",self._render_product_path_0,False))
assert(isdg.is_rendervar_enabled(self._render_product_path_0, render_var, True, self._stage))
assert(isdg.is_rendervar_used(self._render_product_path_0, render_var))
isdg.activate_node_template("BoundingBox3DDisplayPostDuplicate",0, [self._render_product_path_0], {}, self._stage, True)
isdg.deactivate_node_template("BoundingBox3DDisplayPost",0, [self._render_product_path_0], self._stage, True)
assert(isdg.is_rendervar_enabled(self._render_product_path_0, render_var, True, self._stage))
assert(isdg.is_rendervar_used(self._render_product_path_0, render_var))
assert(not isdg.is_node_template_activated("BoundingBox3DReduction",self._render_product_path_0,True))
assert(isdg.is_node_template_activated("BoundingBox3DReduction",self._render_product_path_0,False))
isdg.deactivate_node_template("BoundingBox3DDisplayPostDuplicate",0, [self._render_product_path_0], self._stage, True)
assert(not isdg.is_node_template_activated("BoundingBox3DReduction",self._render_product_path_0,False))
assert(not isdg.is_rendervar_enabled(self._render_product_path_0, render_var, True, self._stage))
assert(not isdg.is_rendervar_used(self._render_product_path_0, render_var))
| 12,821 | Python | 63.757575 | 128 | 0.675922 |
eliabntt/GRADE-RR/isaac_internals/kit/extscore/omni.syntheticdata/omni/syntheticdata/tests/data/golden/view_np_image.py | import os
import sys
import matplotlib.pyplot as plt
import numpy as np
image = np.load(sys.argv[1])["array"]
print(image.shape)
# np.savez_compressed(f"{os.path.splitext(sys.argv[1])[0]}.npz", array=image)
# image = (image - image.min()) / image.ptp()
plt.imshow(image)
plt.show()
| 283 | Python | 22.666665 | 77 | 0.70318 |
eliabntt/GRADE-RR/isaac_internals/kit/extscore/omni.syntheticdata/docs/CHANGELOG.md | # Changelog
The format is based on [Keep a Changelog](https://keepachangelog.com/en/1.0.0/).
## [0.2.4] - 2022-09-22
### Changed
- Update icon to match Viewport 2.0 style
## [0.2.3] - 2021-08-16
### Fixed
- Call dict.discard instead of non extistant dict.remove.
## [0.2.2] - 2021-05-18
### Changed
- Add dependency on omni.kit.viewport.utility
## [0.2.1] - 2022-03-23
### Changed
- Support Legacy and Modern Viewport
## [0.1.8] - 2021-12-10
### Changed
- Deprecated Depth and DepthLinear sensors and added DistanceToImagePlane and DistanceToCamera
### Added
- Cross Correspondence Sensor
## [0.1.7] - 2021-10-16
### Changed
- Move synthetic data sensors to AOV outputs that can be specified in USD and used in OmniGraph nodes
## [0.1.6] - 2021-06-18
### Fixed
- Instance Segmentation is now always returned as uint32
- Fixed parsed segmentation mode
- Fixed Pinhole projection which incorrectly used the camera's local transform instead of its world transform
### Added
- Linear depth sensor mode
## [0.1.5] - 2021-03-11
### Added
- Motion Vector visualization and helper function
### Changed
- BBox3D corners axis order to be Y-Up for consistency with USD API
- All parsed data return uniqueId field, along with list of instanceIds
- `instanceId` field removed from parsed output to avoid confusion with renderer instanceId
- Add `get_instance` function to extension
- Improve returned data of `get_occlusion_quadrant` for consistency with other sensors
### Fixed
- Fix BBox3D parsed mode when dealing with nested transforms
- Fix BBox3D camera_frame mode, previously returned incorrect values
- Use seeded random generator for shuffling colours during visualization
## [0.1.4] - 2021-02-10
### Changed
- Moved to internal extension
- Minor bug fixes
## [0.1.3] - 2021-02-05
### Added
- Python 3.7 support
### Changed
- Bug fixes
## [0.1.2] - 2021-01-28
### Added
- Occlusion Quadrant Sensor
- Metadata Sensor
### Changed
- Metadata (SemanticSchemas of Type != 'class') added to sensor outputs
- UI changed to better suit multi-viewport scenarios
- Misc. sensor fixes and improvements
## [0.1.1] - 2021-01-25
- Linux support
## [0.1.0] - 2021-01-18
- Initial version
| 2,184 | Markdown | 26.658228 | 109 | 0.727106 |
eliabntt/GRADE-RR/isaac_internals/kit/extscore/omni.syntheticdata/docs/README.md | # omni.syntheticdata
## Introduction
This extension provides low level OmniGraph nodes for preparing synthetic data AOVs and annotator outputs for the higher
level Omniverse Replicator extension. End user applications should use the Replicator APIs, rather than using this
extension directly.
The extension also includes support for older deprecated Omniverse Synthetic Data APIs. If you are currently using
these older APIs, we suggest reviewing the newer Replicator APIs and switching to these.
A preview visualization component is also included - this is accessible from the viewport synthetic data icon when
the extension is installed. | 645 | Markdown | 48.692304 | 120 | 0.829457 |
eliabntt/GRADE-RR/isaac_internals/kit/extscore/omni.syntheticdata/docs/index.rst | omni.syntheticdata
//////////////////////////#
Introduction
************
This extension provides both C++ and python bindings that allow users to extract ground truth data from scenes loaded
and rendered in Omniverse Kit and use it for DL/RL training purposes. Data can be accessed either in host memory or
directly on device memory to provide high performance training. The scene data is provided by generating USD data
that can be rendered through the Kit renderer.
Core Concepts
*************
Sensor
======
Ground truth data is accessed through various sensors that are associated with a view in the renderer. The sensors
generally provide access to synthetic data and are either represented as images or buffers of attribute data. Attribute
data elements are usually associated with a particular instance in a scene, which is usually represented by a mesh
specified in the USD data. Sensors are objects that are managed by the user either through the API or the UI.
Synthetic Image Data
====================
Synthetic image data is represented by sensors as a 2D image. Examples of synthetic image data include RGB data,
depth data, and segmentation data. The data can be in any valid image format supported by the renderer.
Synthetic Attribute Data
========================
Synthetic attribute data is represented by sensors as raw structured data that can be accessed as an array.
The data structures used to store array elements depend on the type of sensor. Examples of synthetic attribute data
include bounding boxes. See the data structures defined below to see how various attribute data arrays define their
data.
Instance
========
An instance is a single segmentation unit in a scene that is usually represented as a mesh. An instance is usually
represented in sensor data as a unique unsigned integer ID. The renderer currently limits scenes to having 2^24
unique instances.
Semantic Class
==============
A semantic class is a classification given to a scene instance that can be used for training purposes. It is provided
as a unique string and is usually represented in sensor data as a unique unsigned integer ID. Semantic class strings
can be anything that will be used to identify scene instances, such as "car", "tree", "large", "broken", etc. The
renderer currently limits scenes to having 2^16 unique semantic classes. Semantic class data is specified inside the
USD scene data through the Semantic API schema.
Segmentation
============
Segmentation data is usually represented by sensors as synthetic image data and is used to segment image data
within a view. Examples include instance segmentation which will represent each pixel in the image data with an
instance ID and semantic segmentation which will represent each pixel in the image data with a semantic ID.
Accessing Data on Device Memory
===============================
Device Memory is usually GPU memory. Synthetic data can be accessed directly on device memory with python by using
PyTorch tensors.
Accessing Data on Host Memory
=============================
Device Memory is usually system memory. Synthetic data can be accessed directly on host memory with python through
numpy arrays.
Data Structures
***************
Below are the various data structures specified by the C++ API and accessed through python using pybind.
SensorType
==========
.. code::
enum class SensorType : uint32_t
{
// These sensors represent image data
eRgb = 0, ///< RGB data
eDepth, ///< depth data
eDepthLinear, ///< linear depth data (in meters)
eInstanceSegmentation, ///< instance segmentation data
eSemanticSegmentation, ///< semantic segmentation data
eNormal, ///< normal vector data
eMotionVector, ///< motion vector data
// These sensors represent instance attribute data
eBoundingBox2DTight, ///< tight 2D bounding box data, only contains non-occluded pixels
eBoundingBox2DLoose, ///< loose 2D bounding box data, also contains occluded pixels
eBoundingBox3D, ///< 3D view space bounding box data
eOcclusion, ///< occlusion data
eTruncation, ///< truncation data
};
SensorResourceType
==================
.. code::
enum class SensorResourceType
{
eTexture, ///< image data sensors
eBuffer ///< attribute data sensors
};
SensorInfo
==========
.. code::
struct SensorInfo
{
SensorType type; ///< sensor type
SensorResourceType resType; ///< sensor resource type
union
{
struct
{
uint32_t width; ///< sensor width of texture sensors
uint32_t height; ///< sensor height of texture sensors
uint32_t bpp; ///< bytes per pixel stored for texture sensors
uint32_t rowSize; ///< texture row stride in bytes
} tex;
struct
{
size_t size; ///< size in bytes of buffer sensors
} buff;
}; ///< sensor parameters
};
BoundingBox2DValues
===================
.. code::
struct BoundingBox2DValues
{
uint32_t instanceId; ///< instance ID
uint32_t semanticId; ///< semantic ID
int32_t x_min; ///< left extent
int32_t y_min; ///< top extent
int32_t x_max; ///< right extent
int32_t y_max; ///< bottom extent
};
BoundingBox3DValues
===================
.. code::
struct BoundingBox3DValues
{
uint32_t instanceId; ///< instance ID
uint32_t semanticId; ///< semantic ID
float x_min; ///< left extent
float y_min; ///< top extent
float z_min; ///< front extent
float x_max; ///< right extent
float y_max; ///< bottom extent
float z_max; ///< back extent
};
OcclusionValues
===============
.. code::
struct OcclusionValues
{
uint32_t instanceId; ///< instance ID
uint32_t semanticId; ///< semantic ID
float occlusionRatio; ///< ratio of instance that is occluded
};
TruncationValues
================
.. code::
struct TruncationValues
{
uint32_t instanceId; ///< instance ID
uint32_t semanticId; ///< semantic ID
float truncationRatio; ///< ratio of instance that is truncated
};
Python API Docs
****************
Pybind API
==========
.. code::
// Creates a sensor of specified type if none exist otherwise return the existing sensor.
//
// Args:
//
// arg0 (type): The sensor type to return
create_sensor(sensors::SensorType type)
.. code::
// Destroys the specified sensor.
//
// Args:
//
// arg0 (type): The sensor type to destroy
destroy_sensor(sensors::SensorType type)
.. code::
// Returns the width of the specified image sensor.
//
// Args:
//
// arg0 (type): The sensor to retrieve the width for
get_sensor_width(carb::sensors::SensorType type)
.. code::
// Returns the height of the specified image sensor.
//
// Args:
//
// arg0 (type): The sensor to retrieve the height for
get_sensor_height(carb::sensors::SensorType type)
.. code::
// Returns the bytes per pixel of the specified image sensor.
//
// Args:
//
// arg0 (type): The sensor to retrieve the bytes per pixel for
get_sensor_bpp(carb::sensors::SensorType type)
.. code::
// Returns the row size in bytes of the specified image sensor.
//
// Args:
//
// arg0 (type): The sensor to retrieve the row size for
get_sensor_row_size(carb::sensors::SensorType type)
.. code::
// Returns the size in bytes of the specified attribute sensor.
//
// Args:
//
// arg0 (type): The sensor to retrieve the size for
get_sensor_size(carb::sensors::SensorType type)
.. code::
// Returns a pointer to the sensor's data on device memory
//
// Args:
//
// arg0 (type): The sensor to retrieve the data for
get_sensor_device_data(carb::sensors::SensorType type)
.. code::
// Returns a pointer to the sensor's data on host memory
//
// Args:
//
// arg0 (type): The sensor to retrieve the host data for
get_sensor_host_data(carb::sensors::SensorType type)
.. code::
// Returns floating point tensor data of the image sensor on device memory
//
// Args:
//
// arg0 (type): The image sensor to retrieve the tensor data for
//
// arg1 (width): The width of the image sensor
//
// arg2 (height): The height of the image sensor
//
// arg3 (rowSize): The row size in bytes of the image sensor
get_sensor_device_float_2d_tensor(carb::sensors::SensorType type, size_t width, size_t height, size_t rowSize)
.. code::
// Returns 32-bit integer tensor data of the image sensor on device memory
//
// Args:
//
// arg0 (type): The image sensor to retrieve the tensor data for
//
// arg1 (width): The width of the image sensor
//
// arg2 (height): The height of the image sensor
//
// arg3 (rowSize): The row size in bytes of the image sensor
get_sensor_device_int32_2d_tensor(carb::sensors::SensorType type, size_t width, size_t height, size_t rowSize)
.. code::
// Returns 8-bit integer vector tensor data of the image sensor on device memory
//
// Args:
//
// arg0 (type): The image sensor to retrieve the tensor data for
//
// arg1 (width): The width of the image sensor
//
// arg2 (height): The height of the image sensor
//
// arg3 (rowSize): The row size in bytes of the image sensor
get_sensor_device_uint8_3d_tensor(carb::sensors::SensorType type, size_t width, size_t height, size_t rowSize)
.. code::
// Returns 32-bit integer numpy array data of the image sensor on host memory
//
// Args:
//
// arg0 (type): The image sensor to retrieve the numpy data for
//
// arg1 (width): The width of the image sensor
//
// arg2 (height): The height of the image sensor
//
// arg3 (rowSize): The row size in bytes of the image sensor
get_sensor_host_uint32_texture_array(carb::sensors::SensorType type, size_t width, size_t height, size_t rowSize)
.. code::
// Returns floating point numpy array data of the image sensor on host memory
//
// Args:
//
// arg0 (type): The image sensor to retrieve the numpy data for
//
// arg1 (width): The width of the image sensor
//
// arg2 (height): The height of the image sensor
//
// arg3 (rowSize): The row size in bytes of the image sensor
get_sensor_host_float_texture_array(carb::sensors::SensorType type, size_t width, size_t height, size_t rowSize)
.. code::
// Returns floating point numpy array data of the attribute sensor on host memory
//
// Args:
//
// arg0 (type): The attribute sensor to retrieve the numpy data for
//
// arg1 (size): The size of the attribute sensor in bytes
get_sensor_host_float_buffer_array(carb::sensors::SensorType type, size_t size)
.. code::
// Returns 32-bit unsigned integer numpy array data of the attribute sensor on host memory
//
// Args:
//
// arg0 (type): The attribute sensor to retrieve the numpy data for
//
// arg1 (size): The size of the attribute sensor in bytes
get_sensor_host_uint32_buffer_array(carb::sensors::SensorType type, size_t size)
.. code::
// Returns 32-bit signed integer numpy array data of the attribute sensor on host memory
//
// Args:
//
// arg0 (type): The attribute sensor to retrieve the numpy data for
//
// arg1 (size): The size of the attribute sensor in bytes
get_sensor_host_int32_buffer_array(carb::sensors::SensorType type, size_t size)
.. code::
// Returns a numpy array of BoundingBox2DValues data for the attribute sensor on host memory
//
// Args:
//
// arg0 (type): The attribute sensor to retrieve the numpy data for
//
// arg1 (size): The size of the attribute sensor in bytes
get_sensor_host_bounding_box_2d_buffer_array(carb::sensors::SensorType type, size_t size)
.. code::
// Returns a numpy array of BoundingBox3DValues data for the attribute sensor on host memory
//
// Args:
//
// arg0 (type): The attribute sensor to retrieve the numpy data for
//
// arg1 (size): The size of the attribute sensor in bytes
get_sensor_host_bounding_box_3d_buffer_array(carb::sensors::SensorType type, size_t size)
.. code::
// Returns a numpy array of OcclusionValues data for the attribute sensor on host memory
//
// Args:
//
// arg0 (type): The attribute sensor to retrieve the numpy data for
//
// arg1 (size): The size of the attribute sensor in bytes
get_sensor_host_occlusion_buffer_array(carb::sensors::SensorType type, size_t size)
.. code::
// Returns a numpy array of TruncationValues data for the attribute sensor on host memory (TODO)
//
// Args:
//
// arg0 (type): The attribute sensor to retrieve the numpy data for
//
// arg1 (size): The size of the attribute sensor in bytes
get_sensor_host_truncation_buffer_array(carb::sensors::SensorType type, size_t size)
.. code::
// Returns the instance ID of the specified mesh as represented by sensor data
//
// Args:
//
// arg0 (uri): The representation of the mesh in the USD scene
get_instance_segmentation_id(const char* uri)
.. code::
// Returns the semantic ID of the specified name and type as represented by sensor data
//
// Args:
//
// arg0 (type): The semantic type name
//
// arg1 (data): The semantic data name
get_semantic_segmentation_id_from_data(const char* type, const char* data)
.. code::
// Returns the semantic class name of the semantic ID represented by sensor data
//
// Args:
//
// arg0 (semanticId): The semantic ID
get_semantic_segmentation_data_from_id(uint16_t semanticId)
.. code::
// Specify which semantic classes to retrieve bounding boxes for
//
// Args:
//
// arg0 (semanticId): The semantic ID to retrieve bounding boxes for
set_bounding_box_semantic_segmentation_id(uint16_t semanticId)
.. code::
// Specify which semantic classes to retrieve bounding boxes for
//
// Args:
//
// arg0 (data): The semantic data class name to retrieve bounding boxes for
set_bounding_box_semantic_segmentation_data(std::string data)
| 14,582 | reStructuredText | 29.255187 | 119 | 0.650734 |
zhehuazhou/ai-cps-robotics-benchmark/README.md | # Towards Building AI-CPS with NVIDIA Isaac Sim: An Industrial Benchmark and Case Study for Robotics Manipulation
This folder contains all revelant code for the paper "Towards Building AI-CPS with NVIDIA Isaac Sim: An Industrial Benchmark and Case Study for Robotics Manipulation".
## Benchmark of Robotics Manipulation
### Requirements:
1. Install Omniverse Isaac Sim: https://docs.omniverse.nvidia.com/app_isaacsim/app_isaacsim/install_basic.html
2. Add Isaac Sim to PYTHON_PATH (with default installation location of ISAAC SIM)
```
alias PYTHON_PATH=~/.local/share/ov/pkg/isaac_sim-*/python.sh
```
2. Install Omniverse Isaac GYM Envs: https://github.com/NVIDIA-Omniverse/OmniIsaacGymEnvs
3. Install SKRL, RTAMT, and Scipy in the Isaac Sim Python environment (the latter two are used for falsification): go to the Isaac folder, and run
```
./python.sh -m pip install skrl rtamt scipy
```
### Run the learning process:
To run SKRL with provided task environments (example):
```
cd Gym_Envs/
PYTHON_PATH skrl_train_PPO.py task=FrankaBallBalancing num_envs=16 headless=False
```
To launch Tensorboard:
```
PYTHON_PATH -m tensorboard.main --logdir runs/FrankaBallBalancing/summaries/
```
## Falsification Tool
To run the falsification test for pre-trained agent, run:
```
cd Falsification_Tool/
PYTHON_PATH manipulator_testing.py headless=False
```
## Performance Evaluation
The performance evaluation uses the same framework as the falsification tool, but with the optimizer set to "random":
```
cd Evaluation/
PYTHON_PATH manipulator_eval.py headless=False
```
| 1,596 | Markdown | 34.488888 | 167 | 0.762531 |
zhehuazhou/ai-cps-robotics-benchmark/Evaluation/manipulator_eval.py | from eval_model.skrl_oige_model import skrl_oige_model
from eval_monitor.stl_dense_offline import stl_dense_offline_monitor
from eval_optimizer.optimizer import Optimizer
import os
if __name__ == "__main__":
# Config inputs
agent_type = "PPO" # TRPO, PPO
omniisaacgymenvs_path = os.path.realpath(
os.path.join(os.path.realpath(__file__), "../../Gym_Envs")
)
agent_path = (
omniisaacgymenvs_path
+ "/Final_Policy/BallBalancing/BallBalancing_skrl_"
+ agent_type
+ "/checkpoints/best_agent.pt"
)
# Task choice: PointReaching, PegInHole, DoorOpen,
# BallBalancing, BallPushing, BallCatching
# CubeStacking, ClothPlacing
task_name = "FrankaBallBalancing"
simulation_max_steps = 300
num_envs = 1
opt_types = ["random"]
global_budget = 1
local_budget = 100
# Load model under test (drl agent + oige env)
is_action_noise = True
test_model = skrl_oige_model(
agent_path=agent_path,
agent_type=agent_type,
task_name=task_name,
num_envs=num_envs,
timesteps=simulation_max_steps,
is_action_noise= is_action_noise
)
for opt_type in opt_types:
# Load STL monitor based on task
monitor = stl_dense_offline_monitor(task_name=task_name, agent_type=agent_type)
# global search
for i in range(global_budget):
# print("Global trial: " + str(i))
# Create optimizer
optimizer = Optimizer(
task_name,
test_model,
monitor,
opt_type=opt_type,
budget_size=local_budget,
)
# local search
results = optimizer.optimize()
print(results)
# close simulation environment
test_model.close_env()
| 1,848 | Python | 26.191176 | 87 | 0.593074 |
zhehuazhou/ai-cps-robotics-benchmark/Evaluation/eval_monitor/stl_dense_offline.py | from rtamt import STLDenseTimeSpecification
from typing import Optional
import sys
class stl_dense_offline_monitor(object):
"""STL dense time offline monitor based rtamt
agent_path: the path to the agent parameters (checkpoint)
oige_path: path to the OIGE environment;
agent_type: type of DRL agent (PPO, DDPG, TRPO)
task_name: the name of the task
num_envs: the number of parallel running environments
"""
def __init__(
self,
task_name: Optional[str] = None,
agent_type: Optional[str] = None,
oige_path: Optional[str] = None,
):
if task_name is not None:
self.task_name = task_name
else:
self.task_name = "FrankaBallPushing"
self.agent_type = agent_type
self.generate_spec()
# generate specification based on task name
def generate_spec(self):
# Initialization
self.spec = STLDenseTimeSpecification()
self.spec.name = "STL Dense-time Offline Monitor"
###############################################
# Specification according to task
# Ball Pushing
if self.task_name is "FrankaBallPushing":
self.spec.declare_var("distance_ball_hole", "float")
self.spec.spec = "eventually[1:299](distance_ball_hole <= 0.3) "
# Ball Balancing
elif self.task_name is "FrankaBallBalancing":
self.spec.declare_var("distance_ball_tool", "float")
self.spec.spec = "always[50:200]( distance_ball_tool <= 0.25)"
# Ball Catching
elif self.task_name is "FrankaBallCatching":
self.spec.declare_var("distance_ball_tool", "float")
self.spec.spec = "always[50:299]( distance_ball_tool <= 0.1)"
# Cube Stacking
elif self.task_name is "FrankaCubeStacking":
self.spec.declare_var("distance_cube", "float")
self.spec.declare_var("z_cube_distance", "float")
self.spec.spec = (
"eventually[1:299]((distance_cube<= 0.024) and (z_cube_distance>0) )"
)
# Door Open
elif self.task_name is "FrankaDoorOpen":
self.spec.declare_var("yaw_door", "float")
self.spec.spec = "eventually[1:299]( yaw_door >= 20)"
# Peg In Hole
elif self.task_name is "FrankaPegInHole":
self.spec.declare_var("distance_tool_hole", "float")
self.spec.spec = "always[250:299]( distance_tool_hole <= 0.1)"
# Point Reaching
elif self.task_name is "FrankaPointReaching":
self.spec.declare_var("distance_finger_target", "float")
self.spec.spec = "always[50:299]( distance_finger_target <= 0.12)" # fixed
# Cloth Placing
elif self.task_name is "FrankaClothPlacing":
self.spec.declare_var("distance_cloth_target", "float")
self.spec.declare_var("cloth_height", "float")
self.spec.spec = "eventually[1:299]( (distance_cloth_target <= 0.25))" # and (cloth_height > 0.1) )"
else:
raise ValueError("Task name unknown for defining the specification")
################################################
# Load specification
try:
self.spec.parse()
except rtamt.STLParseException as err:
print("STL Parse Exception: {}".format(err))
sys.exit()
# Compute the robustness given trace
def compute_robustness(self, trace):
if self.task_name is "FrankaBallPushing":
# print(trace)
robustness = self.spec.evaluate(["distance_ball_hole", trace])
# print(robustness)
elif self.task_name is "FrankaBallBalancing":
robustness = self.spec.evaluate(["distance_ball_tool", trace])
elif self.task_name is "FrankaBallCatching":
robustness = self.spec.evaluate(["distance_ball_tool", trace])
elif self.task_name is "FrankaCubeStacking":
distance_cube = trace["distance_cube"]
z_cube_distance = trace["z_cube_distance"]
robustness = self.spec.evaluate(
["distance_cube", distance_cube], ["z_cube_distance", z_cube_distance]
)
elif self.task_name is "FrankaDoorOpen":
robustness = self.spec.evaluate(["yaw_door", trace])
elif self.task_name is "FrankaPegInHole":
robustness = self.spec.evaluate(["distance_tool_hole", trace])
elif self.task_name is "FrankaPointReaching":
robustness = self.spec.evaluate(["distance_finger_target", trace])
elif self.task_name is "FrankaClothPlacing":
distance_cloth_target = trace["distance_cloth_target"]
cloth_height = trace["cloth_height"]
# print("distance")
# print(distance_cloth_target)
# print(cloth_height)
robustness = self.spec.evaluate(
["distance_cloth_target", distance_cloth_target]#, ["cloth_height", cloth_height]
)
# print("rob: ")
# print(robustness)
else:
raise ValueError("Task name unknown for defining the specification")
return robustness
| 5,263 | Python | 30.710843 | 112 | 0.580847 |
zhehuazhou/ai-cps-robotics-benchmark/Evaluation/eval_model/skrl_oige_model.py | import os
import torch
from typing import Optional
from .load_oige import load_oige_test_env
from .agent.PPO_agent import create_skrl_ppo_agent
from .agent.TRPO_agent import create_skrl_trpo_agent
from skrl.envs.torch import wrap_env
class skrl_oige_model(object):
"""Testing environment model based on SKRL and Omniverse Isaac Gym Environments (OIGE)
agent_path: the path to the agent parameters (checkpoint)
oige_path: path to the OIGE environment;
agent_type: type of DRL agent (PPO, DDPG, TRPO)
task_name: the name of the task
num_envs: the number of parallel running environments
"""
def __init__(
self,
agent_path: str,
oige_path: Optional[str] = None,
agent_type: Optional[str] = None,
task_name: Optional[str] = None,
timesteps: Optional[int] = 10000,
num_envs: Optional[int] = 1,
headless: Optional[bool] = False,
is_action_noise: Optional[bool] = False,
):
# setup
if oige_path is not None:
self.oige_path = oige_path
else:
self.oige_path = os.path.realpath(
os.path.join(os.path.realpath(__file__), "../../../Gym_Envs")
)
if agent_type is not None:
self.agent_type = agent_type
else:
self.agent_type = "PPO"
if task_name is not None:
self.task_name = task_name
else:
self.task_name = "FrankaBallPushing"
self.agent_path = agent_path
self.timesteps = timesteps
self.headless = headless
# Load OIGE env with skrl wrapper
self.num_envs = num_envs # for testing, we use only 1 env for now
env = load_oige_test_env(
task_name=self.task_name,
omniisaacgymenvs_path=self.oige_path,
num_envs=self.num_envs,
)
self.env = wrap_env(env)
self.env._env.set_as_test()
# if action noise is required
if is_action_noise is True:
self.env._env.set_action_noise()
# Load agent
if self.agent_type is "PPO":
self.agent = create_skrl_ppo_agent(self.env, self.agent_path)
elif self.agent_type is "TRPO":
self.agent = create_skrl_trpo_agent(self.env, self.agent_path)
else:
raise ValueError("Agent type unknown.")
# Initialize agent
# cfg_trainer = {"timesteps": self.timesteps, "headless": self.headless}
self.agent.init()
if self.num_envs == 1:
self.agent.set_running_mode("eval")
else:
raise ValueError("Currently only one environment (agent) is supported")
# close env
def close_env(self):
self.env.close()
# Compute the trace w.r.t a given initial condition
def compute_trace(self, initial_value):
# set initial configuration
self.env._env.set_initial_test_value(initial_value)
# reset env
states, infos = self.env.reset()
# initialize trace
trace = states
# simulation loop
for timestep in range(self.timesteps):
# compute actions
with torch.no_grad():
actions = self.agent.act(
states, timestep=timestep, timesteps=self.timesteps
)[0]
# step the environments
next_states, rewards, terminated, truncated, infos = self.env.step(actions)
# render scene
if not self.headless:
self.env.render()
# record trace
states.copy_(next_states)
trace = torch.vstack([trace, states])
# terminate simulation
with torch.no_grad():
if terminated.any() or truncated.any():
break
return trace
# Merge trace based on the task type
def merge_trace(self, trace):
if self.task_name is "FrankaBallPushing":
# Ball hole distance
ball_hole_distance = trace[:, 24:27].detach().cpu()
ball_hole_distance = torch.norm(ball_hole_distance, p=2, dim=-1)
ball_Z_pos = trace[:, 29].detach().cpu()
# create index
trace_length = list(ball_hole_distance.size())[0]
times = torch.linspace(1, trace_length, steps=trace_length)
# convert to list for computing robustness
indexed_trace = torch.vstack((times, ball_hole_distance))
indexed_trace = torch.transpose(indexed_trace, 0, 1).tolist()
elif self.task_name is "FrankaBallBalancing":
# Ball tool distance
ball_tool_distance = trace[:, 21:23].detach().cpu()
ball_tool_distance = torch.norm(ball_tool_distance, p=2, dim=-1)
# create index
trace_length = list(ball_tool_distance.size())[0]
times = torch.linspace(1, trace_length, steps=trace_length)
# convert to list for computing robustness
indexed_trace = torch.vstack((times, ball_tool_distance))
indexed_trace = torch.transpose(indexed_trace, 0, 1).tolist()
elif self.task_name is "FrankaBallCatching":
# Ball tool distance
ball_tool_distance = trace[:, 21:23].detach().cpu()
ball_tool_distance = torch.norm(ball_tool_distance, p=2, dim=-1)
# create index
trace_length = list(ball_tool_distance.size())[0]
times = torch.linspace(1, trace_length, steps=trace_length)
# convert to list for computing robustness
indexed_trace = torch.vstack((times, ball_tool_distance))
indexed_trace = torch.transpose(indexed_trace, 0, 1).tolist()
elif self.task_name is "FrankaCubeStacking":
# Cube distance
cube_distance = trace[:, 25:27].detach().cpu()
cube_distance = torch.norm(cube_distance, p=2, dim=-1)
# Cube height
cube_height_distance = trace[:, 27].detach().cpu()
# create index
trace_length = list(cube_distance.size())[0]
times = torch.linspace(1, trace_length, steps=trace_length)
# convert to list for computing robustness
indexed_cube_distance = torch.vstack((times, cube_distance))
indexed_cube_distance = torch.transpose(
indexed_cube_distance, 0, 1
).tolist()
indexed_cube_height_distance = torch.vstack((times, cube_height_distance))
indexed_cube_height_distance = torch.transpose(
indexed_cube_height_distance, 0, 1
).tolist()
indexed_trace = {
"distance_cube": indexed_cube_distance,
"z_cube_distance": indexed_cube_height_distance,
}
elif self.task_name is "FrankaDoorOpen":
# Ball tool distance
handle_rot = trace[:, 21:25].detach().cpu()
handle_yaw = torch.atan2(
2.0
* (
handle_rot[:, 0] * handle_rot[:, 3]
+ handle_rot[:, 1] * handle_rot[:, 2]
),
1.0
- 2.0
* (
handle_rot[:, 2] * handle_rot[:, 2]
+ handle_rot[:, 3] * handle_rot[:, 3]
),
)
handle_yaw = torch.rad2deg(handle_yaw)
# create index
trace_length = list(handle_yaw.size())[0]
times = torch.linspace(1, trace_length, steps=trace_length)
# convert to list for computing robustness
indexed_trace = torch.vstack((times, handle_yaw))
indexed_trace = torch.transpose(indexed_trace, 0, 1).tolist()
elif self.task_name is "FrankaPegInHole":
# Ball tool distance
tool_hole_distance = trace[:, 25:27].detach().cpu()
tool_hole_distance = torch.norm(tool_hole_distance, p=2, dim=-1)
# print(tool_hole_distance)
# create index
trace_length = list(tool_hole_distance.size())[0]
times = torch.linspace(1, trace_length, steps=trace_length)
# convert to list for computing robustness
indexed_trace = torch.vstack((times, tool_hole_distance))
indexed_trace = torch.transpose(indexed_trace, 0, 1).tolist()
elif self.task_name is "FrankaPointReaching":
# Ball tool distance
finger_target_distance = trace[:, 24:27].detach().cpu()
finger_target_distance = torch.norm(finger_target_distance, p=2, dim=-1)
# create index
trace_length = list(finger_target_distance.size())[0]
times = torch.linspace(1, trace_length, steps=trace_length)
# convert to list for computing robustness
indexed_trace = torch.vstack((times, finger_target_distance))
indexed_trace = torch.transpose(indexed_trace, 0, 1).tolist()
elif self.task_name is "FrankaClothPlacing":
# Cube distance
cloth_target_distance = trace[:, 21:24].detach().cpu()
cloth_target_distance = torch.norm(cloth_target_distance, p=2, dim=-1)
# Cube height
cloth_height = trace[:, 20].detach().cpu()
# create index
trace_length = list(cloth_target_distance.size())[0]
times = torch.linspace(1, trace_length, steps=trace_length)
# convert to list for computing robustness
indexed_distance_cloth_target = torch.vstack((times, cloth_target_distance))
indexed_distance_cloth_target = torch.transpose(
indexed_distance_cloth_target, 0, 1
).tolist()
indexed_cloth_height = torch.vstack((times, cloth_height))
indexed_cloth_height = torch.transpose(
indexed_cloth_height, 0, 1
).tolist()
indexed_trace = {
"distance_cloth_target": indexed_distance_cloth_target,
"cloth_height": indexed_cloth_height,
}
else:
raise ValueError("Task name unknown for merging the trace")
return indexed_trace
| 10,291 | Python | 33.653199 | 90 | 0.563502 |
zhehuazhou/ai-cps-robotics-benchmark/Evaluation/eval_model/load_oige.py | """
This is a copy from SKRL's implementation of loading oige environment,
with modifications for generating testing oige environment
"""
import sys
import os
from contextlib import contextmanager
def _omegaconf_to_dict(config) -> dict:
"""Convert OmegaConf config to dict
:param config: The OmegaConf config
:type config: OmegaConf.Config
:return: The config as dict
:rtype: dict
"""
# return config.to_container(dict)
from omegaconf import DictConfig
d = {}
for k, v in config.items():
d[k] = _omegaconf_to_dict(v) if isinstance(v, DictConfig) else v
return d
def _print_cfg(d, indent=0) -> None:
"""Print the environment configuration
:param d: The dictionary to print
:type d: dict
:param indent: The indentation level (default: 0)
:type indent: int, optional
"""
for key, value in d.items():
if isinstance(value, dict):
_print_cfg(value, indent + 1)
else:
print(' | ' * indent + " |-- {}: {}".format(key, value))
def load_oige_test_env(task_name: str = "",
omniisaacgymenvs_path: str = "",
num_envs: int = 1,
show_cfg: bool = True,
timeout: int = 30):
"""Load an Omniverse Isaac Gym environment, this is a slight modification of SKRL's implementation
:param task_name: The name of the task (default: "").
If not specified, the task name is taken from the command line argument (``task=TASK_NAME``).
Command line argument has priority over function parameter if both are specified
:type task_name: str, optional
:param omniisaacgymenvs_path: The path to the ``omniisaacgymenvs`` directory (default: "").
If empty, the path will obtained from omniisaacgymenvs package metadata
:type omniisaacgymenvs_path: str, optional
:param show_cfg: Whether to print the configuration (default: True)
:type show_cfg: bool, optional
:param timeout: Seconds to wait for data when queue is empty in multi-threaded environment (default: 30)
:type timeout: int, optional
:raises ValueError: The task name has not been defined, neither by the function parameter nor by the command line arguments
:raises RuntimeError: The omniisaacgymenvs package is not installed or the path is wrong
:return: Omniverse Isaac Gym environment
:rtype: omni.isaac.gym.vec_env.vec_env_base.VecEnvBase or omni.isaac.gym.vec_env.vec_env_mt.VecEnvMT
"""
import torch
from hydra.types import RunMode
from hydra._internal.hydra import Hydra
from hydra._internal.utils import create_automatic_config_search_path, get_args_parser
from omegaconf import OmegaConf
from omni.isaac.gym.vec_env import VecEnvBase, TaskStopException
import omniisaacgymenvs
sys.argv.append("task={}".format(task_name))
sys.argv.append("num_envs={}".format(num_envs))
# get omniisaacgymenvs path from omniisaacgymenvs package metadata
if omniisaacgymenvs_path == "":
if not hasattr(omniisaacgymenvs, "__path__"):
raise RuntimeError("omniisaacgymenvs package is not installed")
omniisaacgymenvs_path = list(omniisaacgymenvs.__path__)[0]
config_path = os.path.join(omniisaacgymenvs_path, "cfg")
# set omegaconf resolvers
OmegaConf.register_new_resolver('eq', lambda x, y: x.lower() == y.lower())
OmegaConf.register_new_resolver('contains', lambda x, y: x.lower() in y.lower())
OmegaConf.register_new_resolver('if', lambda condition, a, b: a if condition else b)
OmegaConf.register_new_resolver('resolve_default', lambda default, arg: default if arg == '' else arg)
# get hydra config without use @hydra.main
config_file = "config"
args = get_args_parser().parse_args()
search_path = create_automatic_config_search_path(config_file, None, config_path)
hydra_object = Hydra.create_main_hydra2(task_name='load_omniisaacgymenv', config_search_path=search_path)
config = hydra_object.compose_config(config_file, args.overrides, run_mode=RunMode.RUN)
cfg = {}
cfg["task"] = _omegaconf_to_dict(config.task)
cfg["task_name"] = config.task_name
cfg["experiment"] = config.experiment
cfg["num_envs"] = config.num_envs
cfg["seed"] = config.seed
cfg["torch_deterministic"] = config.torch_deterministic
cfg["max_iterations"] = config.max_iterations
cfg["physics_engine"] = config.physics_engine
cfg["pipeline"] = config.pipeline
cfg["sim_device"] = config.sim_device
cfg["device_id"] = config.device_id
cfg["rl_device"] = config.rl_device
cfg["num_threads"] = config.num_threads
cfg["solver_type"] = config.solver_type
cfg["test"] = config.test
cfg["checkpoint"] = config.checkpoint
cfg["headless"] = config.headless
# print config
if show_cfg:
print("\nOmniverse Isaac Gym environment ({})".format(config.task.name))
_print_cfg(cfg)
# internal classes
class _OmniIsaacGymVecEnv(VecEnvBase):
def step(self, actions):
actions = torch.clamp(actions, -self._task.clip_actions, self._task.clip_actions).to(self._task.device).clone()
self._task.pre_physics_step(actions)
for _ in range(self._task.control_frequency_inv):
self._world.step(render=self._render)
self.sim_frame_count += 1
observations, rewards, dones, info = self._task.post_physics_step()
return {"obs": torch.clamp(observations, -self._task.clip_obs, self._task.clip_obs).to(self._task.rl_device).clone()}, \
rewards.to(self._task.rl_device).clone(), dones.to(self._task.rl_device).clone(), info.copy()
def set_as_test(self):
self._task.set_as_test()
def set_action_noise(self):
self._task.set_action_noise()
def set_initial_test_value(self, value):
self._task.set_initial_test_value(value)
def reset(self):
self._task.reset()
actions = torch.zeros((self.num_envs, self._task.num_actions), device=self._task.device)
return self.step(actions)[0]
# load environment
sys.path.append(omniisaacgymenvs_path)
from utils.task_util import initialize_task
env = _OmniIsaacGymVecEnv(headless=config.headless)
task = initialize_task(cfg, env, init_sim=True)
return env | 6,481 | Python | 39.767295 | 132 | 0.6553 |
zhehuazhou/ai-cps-robotics-benchmark/Evaluation/eval_model/agent/TRPO_agent.py | """
Create PPO agent based on SKRL implementation
"""
import torch.nn as nn
import torch
from skrl.models.torch import Model, GaussianMixin, DeterministicMixin
from skrl.agents.torch.trpo import TRPO, TRPO_DEFAULT_CONFIG
from skrl.resources.preprocessors.torch import RunningStandardScaler
# Define the models (stochastic and deterministic models) for the agent using mixins.
# - Policy: takes as input the environment's observation/state and returns an action
# - Value: takes the state as input and provides a value to guide the policy
class Policy_2_Layers(GaussianMixin, Model):
def __init__(self, observation_space, action_space, device, clip_actions=False,
clip_log_std=True, min_log_std=-20, max_log_std=2):
Model.__init__(self, observation_space, action_space, device)
GaussianMixin.__init__(self, clip_actions, clip_log_std, min_log_std, max_log_std)
self.net = nn.Sequential(nn.Linear(self.num_observations, 512),
nn.ELU(),
nn.Linear(512, 256),
nn.ELU(),
nn.Linear(256, self.num_actions))
self.log_std_parameter = nn.Parameter(torch.zeros(self.num_actions))
def compute(self, inputs, role):
return self.net(inputs["states"]), self.log_std_parameter, {}
class Policy_3_Layers(GaussianMixin, Model):
def __init__(self, observation_space, action_space, device, clip_actions=False,
clip_log_std=True, min_log_std=-20, max_log_std=2):
Model.__init__(self, observation_space, action_space, device)
GaussianMixin.__init__(self, clip_actions, clip_log_std, min_log_std, max_log_std)
self.net = nn.Sequential(nn.Linear(self.num_observations, 512),
nn.ELU(),
nn.Linear(512, 256),
nn.ELU(),
nn.Linear(256, 128),
nn.ELU(),
nn.Linear(128, self.num_actions))
self.log_std_parameter = nn.Parameter(torch.zeros(self.num_actions))
def compute(self, inputs, role):
return self.net(inputs["states"]), self.log_std_parameter, {}
class Value_2_Layers(DeterministicMixin, Model):
def __init__(self, observation_space, action_space, device, clip_actions=False):
Model.__init__(self, observation_space, action_space, device)
DeterministicMixin.__init__(self, clip_actions)
self.net = nn.Sequential(nn.Linear(self.num_observations, 512),
nn.ELU(),
nn.Linear(512, 256),
nn.ELU(),
nn.Linear(256, 1))
def compute(self, inputs, role):
return self.net(inputs["states"]), {}
class Value_3_Layers(DeterministicMixin, Model):
def __init__(self, observation_space, action_space, device, clip_actions=False):
Model.__init__(self, observation_space, action_space, device)
DeterministicMixin.__init__(self, clip_actions)
self.net = nn.Sequential(nn.Linear(self.num_observations, 512),
nn.ELU(),
nn.Linear(512, 256),
nn.ELU(),
nn.Linear(256, 128),
nn.ELU(),
nn.Linear(128, 1))
def compute(self, inputs, role):
return self.net(inputs["states"]), {}
# Create SKRL PPO agent
def create_skrl_trpo_agent(env, agent_path):
device = env.device
models_trpo_2_layer = {}
models_trpo_2_layer["policy"] = Policy_2_Layers(env.observation_space, env.action_space, device)
models_trpo_2_layer["value"] = Value_2_Layers(env.observation_space, env.action_space, device)
models_trpo_3_layer = {}
models_trpo_3_layer["policy"] = Policy_3_Layers(env.observation_space, env.action_space, device)
models_trpo_3_layer["value"] = Value_3_Layers(env.observation_space, env.action_space, device)
# Configs
cfg_trpo = TRPO_DEFAULT_CONFIG.copy()
cfg_trpo["state_preprocessor"] = RunningStandardScaler
cfg_trpo["state_preprocessor_kwargs"] = {"size": env.observation_space, "device": device}
cfg_trpo["value_preprocessor"] = RunningStandardScaler
cfg_trpo["value_preprocessor_kwargs"] = {"size": 1, "device": device}
# no log to TensorBoard and write checkpoints
cfg_trpo["experiment"]["write_interval"] = 0
cfg_trpo["experiment"]["checkpoint_interval"] = 0
try:
# Initialize and load agent with 2 layers
agent = TRPO(models=models_trpo_2_layer,
memory=None,
cfg=cfg_trpo,
observation_space=env.observation_space,
action_space=env.action_space,
device=device)
agent.load(agent_path)
except:
# Initialize and load agent with 3 layers
agent = TRPO(models=models_trpo_3_layer,
memory=None,
cfg=cfg_trpo,
observation_space=env.observation_space,
action_space=env.action_space,
device=device)
agent.load(agent_path)
return agent
| 5,370 | Python | 40 | 100 | 0.581006 |
zhehuazhou/ai-cps-robotics-benchmark/Evaluation/eval_model/agent/PPO_agent.py | """
Create PPO agent based on SKRL implementation
"""
import torch.nn as nn
import torch
from skrl.models.torch import Model, GaussianMixin, DeterministicMixin
from skrl.agents.torch.ppo import PPO, PPO_DEFAULT_CONFIG
from skrl.resources.preprocessors.torch import RunningStandardScaler
# Define the shared model (stochastic and deterministic models) for the agent using mixins.
class Shared(GaussianMixin, DeterministicMixin, Model):
def __init__(self, observation_space, action_space, device, clip_actions=False,
clip_log_std=True, min_log_std=-20, max_log_std=2, reduction="sum"):
Model.__init__(self, observation_space, action_space, device)
GaussianMixin.__init__(self, clip_actions, clip_log_std, min_log_std, max_log_std, reduction)
DeterministicMixin.__init__(self, clip_actions)
self.net = nn.Sequential(nn.Linear(self.num_observations, 512),
nn.ELU(),
nn.Linear(512, 256),
nn.ELU(),
nn.Linear(256, 128),
nn.ELU())
self.mean_layer = nn.Linear(128, self.num_actions)
self.log_std_parameter = nn.Parameter(torch.zeros(self.num_actions))
self.value_layer = nn.Linear(128, 1)
def act(self, inputs, role):
if role == "policy":
return GaussianMixin.act(self, inputs, role)
elif role == "value":
return DeterministicMixin.act(self, inputs, role)
def compute(self, inputs, role):
if role == "policy":
return self.mean_layer(self.net(inputs["states"])), self.log_std_parameter, {}
elif role == "value":
return self.value_layer(self.net(inputs["states"])), {}
# Create SKRL PPO agent
def create_skrl_ppo_agent(env, agent_path):
device = env.device
models_ppo = {}
models_ppo["policy"] = Shared(env.observation_space, env.action_space, device)
models_ppo["value"] = models_ppo["policy"] # same instance: shared model
# Configs
cfg_ppo = PPO_DEFAULT_CONFIG.copy()
cfg_ppo["state_preprocessor"] = RunningStandardScaler
cfg_ppo["state_preprocessor_kwargs"] = {"size": env.observation_space, "device": device}
cfg_ppo["value_preprocessor"] = RunningStandardScaler
cfg_ppo["value_preprocessor_kwargs"] = {"size": 1, "device": device}
# logging to TensorBoard each 100 timesteps and ignore checkpoints
cfg_ppo["experiment"]["write_interval"] = 0
cfg_ppo["experiment"]["checkpoint_interval"] = 0
# Initialize and load agent
agent = PPO(models=models_ppo,
memory=None,
cfg=cfg_ppo,
observation_space=env.observation_space,
action_space=env.action_space,
device=device)
agent.load(agent_path)
return agent
| 2,889 | Python | 36.051282 | 101 | 0.622361 |
zhehuazhou/ai-cps-robotics-benchmark/Evaluation/eval_optimizer/optimizer.py | from typing import Optional
import sys
import numpy as np
import torch
import time
from scipy.optimize import minimize
from scipy.optimize import dual_annealing
class Optimizer(object):
"""Optimizer class for testing
task_name: the task name of environment
test_model: the model under test
monitor: the monitor for the STL specification
"""
def __init__(
self,
task_name,
test_model,
monitor,
opt_type: Optional[str] = "random",
budget_size: Optional[int] = 1000,
):
self.task_name = task_name
self.test_model = test_model
self.monitor = monitor
self.opt_type = opt_type
self.budget_size = budget_size
self.fal_succ = False
self.start_time = time.time()
self.fal_time = 0
self.fal_sim = 0
self.worst_rob = 1000
# generate initial values based on the task type
def generate_initial(self):
if self.task_name is "FrankaBallPushing":
# ball inside an area x:[-0.1,0.1], y:[-0.1,0.1]
value_1 = np.random.rand(1) * (0.1 + 0.1) - 0.1
value_2 = np.random.rand(1) * (0.1 + 0.1) - 0.1
initial_value = np.hstack((value_1, value_2))
elif self.task_name is "FrankaBallBalancing":
# ball inside an area x:[-0.15,0.15], y:[-0.15,0.15]
value_1 = np.random.rand(1) * (0.15 + 0.15) - 0.15
value_2 = np.random.rand(1) * (0.15 + 0.15) - 0.15
initial_value = np.hstack((value_1, value_2))
elif self.task_name is "FrankaBallCatching":
# ball inside an area x:[-0.1,0.1], y:[-0.1,0.1]
# ball velociry: vx: [1.0,1.5], vy: [0.0,0.2]
value_1 = np.random.rand(1) * (0.05 + 0.05) - 0.05
value_2 = np.random.rand(1) * (0.05 + 0.05) - 0.05
value_3 = np.random.rand(1) * (1.0 - 1.0) + 1.0
value_4 = np.random.rand(1) * (0.0 + 0.0) + 0.0
initial_value = np.hstack((value_1, value_2, value_3, value_4))
elif self.task_name is "FrankaCubeStacking":
# target cube inside an area x:[-0.2,0.2], y:[-0.2,0.2]
value_1 = np.random.rand(1) * (0.2 + 0.2) - 0.2
value_2 = np.random.rand(1) * (0.2 + 0.2) - 0.2
initial_value = np.hstack((value_1, value_2))
elif self.task_name is "FrankaDoorOpen":
# target inside an area x:[-0.1,0.1], y:[-0.4,0.4]
value_1 = np.random.rand(1) * (0.005 + 0.005) - 0.005
value_2 = np.random.rand(1) * (0.025 + 0.025) - 0.025
initial_value = np.hstack((value_1, value_2))
elif self.task_name is "FrankaPegInHole":
# target inside an area x:[-0.2,0.2], y:[-0.2,0.2]
value_1 = np.random.rand(1) * (0.1 + 0.1) - 0.1
value_2 = np.random.rand(1) * (0.1 + 0.1) - 0.1
initial_value = np.hstack((value_1, value_2))
elif self.task_name is "FrankaPointReaching":
# target inside an area x:[-0.2,0.2], y:[-0.4,0.4], z:[-0.2,0.2]
value_1 = np.random.rand(1) * (0.2 + 0.2) - 0.2
value_2 = np.random.rand(1) * (0.4 + 0.4) - 0.4
value_3 = np.random.rand(1) * (0.2 + 0.2) - 0.2
initial_value = np.hstack((value_1, value_2, value_3))
elif self.task_name is "FrankaClothPlacing":
# target inside an area x:[-0.1,0.2], y:[-0.35,0.35]
value_1 = np.random.rand(1) * (0.2 + 0.1) - 0.1
value_2 = np.random.rand(1) * (0.35 + 0.35) - 0.35
initial_value = np.hstack((value_1, value_2))
else:
raise ValueError("Task name unknown for generating the initial values")
return initial_value
# Generate one function (input: initial values, output: robustness) for testing algorithms
def robustness_function(self, initial_value):
# print("Initial Value:", initial_value)
# Get trace
trace = self.test_model.compute_trace(initial_value)
indexed_trace = self.test_model.merge_trace(trace)
# compute robustness
rob_sequence = self.monitor.compute_robustness(indexed_trace)
rob_sequence = np.array(rob_sequence)
# RTAMT is for monitoring, so for eventually, the robustness computed from the current timepoint to the end
# workaround to compute the maximum
if (
self.task_name is "FrankaBallPushing"
or self.task_name is "FrankaCubeStacking"
or self.task_name is "FrankaDoorOpen"
or self.task_name is "FrankaPegInHole"
or self.task_name is "FrankaClothPlacing"
):
min_rob = np.max(rob_sequence[:, 1])
else:
min_rob = np.min(rob_sequence[:, 1])
# print("Min Robustness:", min_rob)
if min_rob < self.worst_rob:
self.worst_rob = min_rob
if min_rob < 0 and self.fal_succ == False:
self.fal_succ = True
self.fal_time = time.time() - self.start_time
elif self.fal_succ == False:
self.fal_sim += 1
return min_rob, rob_sequence, indexed_trace
# optimization based on the optimizer type
def optimize(self):
if self.opt_type is "random":
results = self.optimize_random()
return results
else:
raise ValueError("Optimizer type undefined!")
# Random optimization
def optimize_random(self):
success_count = 0 # num success trail/ num total trail
dangerous_rate = list() # num dangerous steps/ num total trail w.r.t each trail
completion_time = list() # the step that indicates the task is completed
# Random optimizer
for i in range(self.budget_size):
print("trail ",i)
# random initial value
initial_value = self.generate_initial()
# compute robustness and its sequence
min_rob, rob_sequence, indexed_trace = self.robustness_function(initial_value)
# compute dangerous_rate, completion_time w.r.t tasks
if self.task_name == "FrankaCubeStacking":
# info extraction
cube_dist = np.array(indexed_trace["distance_cube"])[:,1]
cube_z_dist = np.array(indexed_trace["z_cube_distance"])[:,1]
# dangerous rate:
cube_too_far = cube_dist >= 0.35
cube_fall_ground = cube_z_dist < 0.02
dangerous_rate.append(np.sum(np.logical_or(cube_too_far, cube_fall_ground))/len(cube_dist))
# completation step
if_complete = (np.logical_and(cube_dist<=0.024, cube_z_dist>0))
complete_Step = np.where(if_complete == True)[0]
if len(complete_Step) > 0:
completion_time.append(complete_Step[0])
elif self.task_name == "FrankaDoorOpen":
handle_yaw = np.array(indexed_trace)[:,1]
# dangerous rate:
dangerous_rate.append(np.sum(handle_yaw<0.1)/len(handle_yaw))
# completation step
if_complete = (handle_yaw>=20)
complete_Step = np.where(if_complete == True)[0]
if len(complete_Step) > 0:
completion_time.append(complete_Step[0])
elif self.task_name == "FrankaPegInHole":
tool_hole_distance = np.array(indexed_trace)[:,1]
# dangerous rate:
dangerous_rate.append(np.sum(tool_hole_distance>0.37)/len(tool_hole_distance))
# completation step
if_complete = (tool_hole_distance<=0.1)
complete_Step = np.where(if_complete == True)[0]
if len(complete_Step) > 0:
completion_time.append(complete_Step[0])
elif self.task_name == "FrankaBallCatching":
ball_tool_distance = np.array(indexed_trace)[:,1]
# dangerous rate:
dangerous_rate.append(np.sum(ball_tool_distance>0.2)/len(ball_tool_distance))
# completation step
if_complete = (ball_tool_distance<=0.1)
complete_interval = np.zeros(len(if_complete)-5)
# spec satisified holds within a 3-step interval
for i in range(0, int(len(if_complete)-5)):
complete_interval[i] = np.all(if_complete[i:i+5])
complete_Step = np.where(complete_interval == True)[0]
if len(complete_Step) > 0:
completion_time.append(complete_Step[0])
elif self.task_name == "FrankaBallBalancing":
ball_tool_distance = np.array(indexed_trace)[:,1]
# dangerous rate:
dangerous_rate.append(np.sum(ball_tool_distance>0.2)/len(ball_tool_distance))
# completation step
if_complete = (ball_tool_distance<=0.1)
complete_interval = np.zeros(len(if_complete)-5)
# spec satisified holds within a 3-step interval
for i in range(0, int(len(if_complete)-5)):
complete_interval[i] = np.all(if_complete[i:i+5])
complete_Step = np.where(complete_interval == True)[0]
if len(complete_Step) > 0:
completion_time.append(complete_Step[0])
elif self.task_name == "FrankaBallPushing":
ball_hole_distance = np.array(indexed_trace)[:,1]
# dangerous rate:
dangerous_rate.append(np.sum(ball_hole_distance>0.5)/len(ball_hole_distance))
# completation step
if_complete = (ball_hole_distance<=0.3)
complete_interval = np.zeros(len(if_complete)-5)
# spec satisified holds within a 3-step interval
for i in range(0, int(len(if_complete)-5)):
complete_interval[i] = np.all(if_complete[i:i+5])
complete_Step = np.where(complete_interval == True)[0]
if len(complete_Step) > 0:
completion_time.append(complete_Step[0])
elif self.task_name == "FrankaPointReaching":
finger_target_distance = np.array(indexed_trace)[:,1]
# dangerous rate:
dangerous_rate.append(np.sum(finger_target_distance>=0.6)/len(finger_target_distance))
# completation step
if_complete = (finger_target_distance<=0.12)
complete_Step = np.where(if_complete == True)[0]
if len(complete_Step) > 0:
completion_time.append(complete_Step[0])
elif self.task_name == "FrankaClothPlacing":
# info extraction
cloth_target_dist = np.array(indexed_trace["distance_cloth_target"])[:,1]
cloth_z_pos = np.array(indexed_trace["cloth_height"])[:,1]
# dangerous rate:
cloth_too_far = cloth_target_dist >= 0.3
cloth_fall_ground = cloth_z_pos < 0.1
dangerous_rate.append(np.sum(np.logical_or(cloth_too_far, cloth_fall_ground))/len(cloth_target_dist))
# completation step
if_complete = cloth_target_dist<=0.25
complete_Step = np.where(if_complete == True)[0]
if len(complete_Step) > 0:
completion_time.append(complete_Step[0])
# print(indexed_trace)
else:
print("Invalid Task")
break
# perform evaluation:
# success rate
if min_rob > 0:
success_count += 1
# dangerous behavior: change the STL specification and use rob_sequence?
# completion time: check first satisfication in index_trace?
# if i == 0:
# break
if len(dangerous_rate) == 0:
dangerous_rate = 0
results = {"success_count": success_count/self.budget_size,
"dangerous_rate": np.mean(dangerous_rate),
"completion_time": np.mean(completion_time)}
return results | 12,314 | Python | 40.05 | 117 | 0.54231 |
zhehuazhou/ai-cps-robotics-benchmark/Gym_Envs/skrl_train_SAC.py | import torch
import os
import torch.nn as nn
import torch.nn.functional as F
# Import the skrl components to build the RL system
from skrl.models.torch import Model, GaussianMixin, DeterministicMixin
from skrl.memories.torch import RandomMemory
from skrl.agents.torch.sac import SAC, SAC_DEFAULT_CONFIG
from skrl.resources.schedulers.torch import KLAdaptiveRL
from skrl.resources.noises.torch import OrnsteinUhlenbeckNoise
from skrl.resources.preprocessors.torch import RunningStandardScaler
from skrl.trainers.torch import SequentialTrainer
from skrl.envs.torch import wrap_env
from skrl.envs.torch import load_omniverse_isaacgym_env
from skrl.utils import set_seed
# set the seed for reproducibility
set_seed(42)
# Define the models (stochastic and deterministic models) for the SAC agent using the mixins.
# - StochasticActor (policy): takes as input the environment's observation/state and returns an action
# - Critic: takes the state and action as input and provides a value to guide the policy
class Policy(GaussianMixin, Model):
def __init__(self, observation_space, action_space, device, clip_actions=True,
clip_log_std=True, min_log_std=-20, max_log_std=2, reduction="sum"):
Model.__init__(self, observation_space, action_space, device)
GaussianMixin.__init__(self, clip_actions, clip_log_std, min_log_std, max_log_std, reduction)
self.net = nn.Sequential(nn.Linear(self.num_observations, 512),
nn.ELU(),
nn.Linear(512, 256),
nn.ELU(),
nn.Linear(256, self.num_actions),)
# nn.Tanh())
self.log_std_parameter = nn.Parameter(torch.zeros(self.num_actions))
def compute(self, inputs, role):
return self.net(inputs["states"]), self.log_std_parameter, {}
class Critic(DeterministicMixin, Model):
def __init__(self, observation_space, action_space, device, clip_actions=False):
Model.__init__(self, observation_space, action_space, device)
DeterministicMixin.__init__(self, clip_actions)
self.net = nn.Sequential(nn.Linear(self.num_observations + self.num_actions, 512),
nn.ELU(),
nn.Linear(512, 256),
nn.ELU(),
nn.Linear(256, 1))
def compute(self, inputs, role):
return self.net(torch.cat([inputs["states"], inputs["taken_actions"]], dim=1)), {}
# Load and wrap the Omniverse Isaac Gym environment]
omniisaacgymenvs_path = os.path.realpath( os.path.join(os.path.realpath(__file__), "..") )
env = load_omniverse_isaacgym_env(task_name="FrankaCatching", omniisaacgymenvs_path = omniisaacgymenvs_path)
env = wrap_env(env)
device = env.device
# Instantiate a RandomMemory as rollout buffer (any memory can be used for this)
memory = RandomMemory(memory_size=128, num_envs=env.num_envs, device=device, replacement=True)
# Instantiate the agent's models (function approximators).
# SAC requires 5 models, visit its documentation for more details
# https://skrl.readthedocs.io/en/latest/modules/skrl.agents.sac.html#spaces-and-models
models_sac = {}
models_sac["policy"] = Policy(env.observation_space, env.action_space, device)
models_sac["critic_1"] = Critic(env.observation_space, env.action_space, device)
models_sac["critic_2"] = Critic(env.observation_space, env.action_space, device)
models_sac["target_critic_1"] = Critic(env.observation_space, env.action_space, device)
models_sac["target_critic_2"] = Critic(env.observation_space, env.action_space, device)
# Initialize the models' parameters (weights and biases) using a Gaussian distribution
for model in models_sac.values():
model.init_parameters(method_name="normal_", mean=0.0, std=0.1)
# Configure and instantiate the agent.
# Only modify some of the default configuration, visit its documentation to see all the options
# https://skrl.readthedocs.io/en/latest/modules/skrl.agents.sac.html#configuration-and-hyperparameters
cfg_sac = SAC_DEFAULT_CONFIG.copy()
cfg_sac["gradient_steps"] = 1
cfg_sac["batch_size"] = 128
cfg_sac["random_timesteps"] = 10
cfg_sac["learning_starts"] = 0
cfg_sac["actor_learning_rate"]: 5e-4 # actor learning rate
cfg_sac["critic_learning_rate"]: 5e-3 # critic learning rate
cfg_sac["learn_entropy"] = True
cfg_sac["entropy_learning_rate"]: 1e-3 # entropy learning rate
cfg_sac["initial_entropy_value"]: 0.2 # initial entropy value
# logging to TensorBoard and write checkpoints each 1000 and 1000 timesteps respectively
cfg_sac["experiment"]["write_interval"] = 100
cfg_sac["experiment"]["checkpoint_interval"] = 1000
agent= SAC(models=models_sac,
memory=memory,
cfg=cfg_sac,
observation_space=env.observation_space,
action_space=env.action_space,
device=device)
# Configure and instantiate the RL trainer
cfg_trainer = {"timesteps": 320000, "headless": True}
trainer = SequentialTrainer(cfg=cfg_trainer, env=env, agents=agent)
# start training
trainer.train()
| 5,185 | Python | 44.095652 | 108 | 0.688717 |
zhehuazhou/ai-cps-robotics-benchmark/Gym_Envs/skrl_train_TD3.py | import torch
import os
import torch.nn as nn
import torch.nn.functional as F
# Import the skrl components to build the RL system
from skrl.models.torch import Model, GaussianMixin, DeterministicMixin
from skrl.memories.torch import RandomMemory
from skrl.agents.torch.td3 import TD3, TD3_DEFAULT_CONFIG
from skrl.resources.schedulers.torch import KLAdaptiveRL
from skrl.resources.preprocessors.torch import RunningStandardScaler
from skrl.trainers.torch import SequentialTrainer
from skrl.envs.torch import wrap_env
from skrl.envs.torch import load_omniverse_isaacgym_env
from skrl.utils import set_seed
from skrl.resources.noises.torch import GaussianNoise
# set the seed for reproducibility
set_seed(42)
# Define the models (deterministic models) for the TD3 agent using mixins
# and programming with two approaches (torch functional and torch.nn.Sequential class).
# - Actor (policy): takes as input the environment's observation/state and returns an action
# - Critic: takes the state and action as input and provides a value to guide the policy
class DeterministicActor(DeterministicMixin, Model):
def __init__(self, observation_space, action_space, device, clip_actions=True):
Model.__init__(self, observation_space, action_space, device)
DeterministicMixin.__init__(self, clip_actions)
self.net = nn.Sequential(nn.Linear(self.num_observations, 512),
nn.ELU(),
nn.Linear(512, 256),
nn.ELU(),
nn.Linear(256, self.num_actions),
nn.Tanh())
def compute(self, inputs, role):
# x = F.relu(self.linear_layer_1(inputs["states"]))
# x = F.relu(self.linear_layer_2(x))
return self.net(inputs["states"]), {}
class DeterministicCritic(DeterministicMixin, Model):
def __init__(self, observation_space, action_space, device, clip_actions=False):
Model.__init__(self, observation_space, action_space, device)
DeterministicMixin.__init__(self, clip_actions)
self.net = nn.Sequential(nn.Linear(self.num_observations + self.num_actions, 400),
nn.ReLU(),
nn.Linear(400, 300),
nn.ReLU(),
nn.Linear(300, 1))
def compute(self, inputs, role):
return self.net(torch.cat([inputs["states"], inputs["taken_actions"]], dim=1)), {}
# Load and wrap the Omniverse Isaac Gym environment]
omniisaacgymenvs_path = os.path.realpath( os.path.join(os.path.realpath(__file__), "..") )
env = load_omniverse_isaacgym_env(task_name="FrankaBallCatching", omniisaacgymenvs_path = omniisaacgymenvs_path)
env = wrap_env(env)
device = env.device
# Instantiate a RandomMemory as rollout buffer (any memory can be used for this)
memory = RandomMemory(memory_size=2500, num_envs=env.num_envs, device=device)
# Instantiate the agent's models (function approximators).
# TRPO requires 2 models, visit its documentation for more details
# https://skrl.readthedocs.io/en/latest/modules/skrl.agents.trpo.html#spaces-and-models
# Instantiate the agent's models (function approximators).
# TD3 requires 6 models, visit its documentation for more details
# https://skrl.readthedocs.io/en/latest/modules/skrl.agents.td3.html#spaces-and-models
models = {}
models["policy"] = DeterministicActor(env.observation_space, env.action_space, device)
models["target_policy"] = DeterministicActor(env.observation_space, env.action_space, device)
models["critic_1"] = DeterministicCritic(env.observation_space, env.action_space, device)
models["critic_2"] = DeterministicCritic(env.observation_space, env.action_space, device)
models["target_critic_1"] = DeterministicCritic(env.observation_space, env.action_space, device)
models["target_critic_2"] = DeterministicCritic(env.observation_space, env.action_space, device)
# Configure and instantiate the agent.
# Only modify some of the default configuration, visit its documentation to see all the options
# https://skrl.readthedocs.io/en/latest/modules/skrl.agents.trpo.html#configuration-and-hyperparameters
cfg_td3 = TD3_DEFAULT_CONFIG.copy()
cfg_td3["exploration"]["noise"] = GaussianNoise(0, 0.1, device=device)
cfg_td3["smooth_regularization_noise"] = GaussianNoise(0, 0.2, device=device)
cfg_td3["smooth_regularization_clip"] = 0.5
cfg_td3["batch_size"] = 16
cfg_td3["random_timesteps"] = 0
cfg_td3["learning_starts"] = 0
# logging to TensorBoard and write checkpoints each 16 and 80 timesteps respectively
cfg_td3["experiment"]["write_interval"] = 100
cfg_td3["experiment"]["checkpoint_interval"] = 1000
agent = TD3(models=models,
memory=memory,
cfg=cfg_td3,
observation_space=env.observation_space,
action_space=env.action_space,
device=device)
# Configure and instantiate the RL trainer
cfg_trainer = {"timesteps": 320000, "headless": True}
trainer = SequentialTrainer(cfg=cfg_trainer, env=env, agents=agent)
# start training
trainer.train()
| 5,119 | Python | 42.760683 | 112 | 0.70209 |
zhehuazhou/ai-cps-robotics-benchmark/Gym_Envs/skrl_train_DDPG.py | import torch
import os
import torch.nn as nn
import torch.nn.functional as F
# Import the skrl components to build the RL system
from skrl.models.torch import Model, GaussianMixin, DeterministicMixin
from skrl.memories.torch import RandomMemory
from skrl.agents.torch.ddpg import DDPG, DDPG_DEFAULT_CONFIG
from skrl.resources.schedulers.torch import KLAdaptiveRL
from skrl.resources.noises.torch import OrnsteinUhlenbeckNoise
from skrl.resources.preprocessors.torch import RunningStandardScaler
from skrl.trainers.torch import SequentialTrainer
from skrl.envs.torch import wrap_env
from skrl.envs.torch import load_omniverse_isaacgym_env
from skrl.utils import set_seed
# set the seed for reproducibility
set_seed(42)
# Define the models (deterministic models) for the DDPG agent using mixins
# and programming with two approaches (torch functional and torch.nn.Sequential class).
# - Actor (policy): takes as input the environment's observation/state and returns an action
# - Critic: takes the state and action as input and provides a value to guide the policy
class DeterministicActor(DeterministicMixin, Model):
def __init__(self, observation_space, action_space, device, clip_actions=True):
Model.__init__(self, observation_space, action_space, device)
DeterministicMixin.__init__(self, clip_actions)
self.net = nn.Sequential(nn.Linear(self.num_observations, 512),
nn.ELU(),
nn.Linear(512, 256),
nn.ELU(),
nn.Linear(256, self.num_actions),
nn.Tanh())
def compute(self, inputs, role):
# x = F.relu(self.linear_layer_1(inputs["states"]))
# x = F.relu(self.linear_layer_2(x))
return self.net(inputs["states"]), {}
class DeterministicCritic(DeterministicMixin, Model):
def __init__(self, observation_space, action_space, device, clip_actions=False):
Model.__init__(self, observation_space, action_space, device)
DeterministicMixin.__init__(self, clip_actions)
self.net = nn.Sequential(nn.Linear(self.num_observations + self.num_actions, 512),
nn.ELU(),
nn.Linear(512, 256),
nn.ELU(),
nn.Linear(256, 1))
def compute(self, inputs, role):
# x = F.relu(self.linear_layer_1(torch.cat([inputs["states"], inputs["taken_actions"]], dim=1)))
# x = F.relu(self.linear_layer_2(x))
# return torch.tanh(self.action_layer(x)), {}
return self.net(torch.cat([inputs["states"], inputs["taken_actions"]], dim=1)), {}
# Load and wrap the Omniverse Isaac Gym environment]
omniisaacgymenvs_path = os.path.realpath( os.path.join(os.path.realpath(__file__), "..") )
env = load_omniverse_isaacgym_env(task_name="FrankaCatching", omniisaacgymenvs_path = omniisaacgymenvs_path)
env = wrap_env(env)
device = env.device
# Instantiate a RandomMemory as rollout buffer (any memory can be used for this)
memory = RandomMemory(memory_size=16, num_envs=env.num_envs, device=device, replacement=False)
# Instantiate the agent's models (function approximators).
# DDPG requires 4 models, visit its documentation for more details
# https://skrl.readthedocs.io/en/latest/modules/skrl.agents.ddpg.html#spaces-and-models
models_ddpg = {}
models_ddpg["policy"] = DeterministicActor(env.observation_space, env.action_space, device)
models_ddpg["target_policy"] = DeterministicActor(env.observation_space, env.action_space, device)
models_ddpg["critic"] = DeterministicCritic(env.observation_space, env.action_space, device)
models_ddpg["target_critic"] = DeterministicCritic(env.observation_space, env.action_space, device)
# Initialize the models' parameters (weights and biases) using a Gaussian distribution
for model in models_ddpg.values():
model.init_parameters(method_name="normal_", mean=0.0, std=0.5)
# Configure and instantiate the agent.
# Only modify some of the default configuration, visit its documentation to see all the options
# https://skrl.readthedocs.io/en/latest/modules/skrl.agents.ddpg.html#configuration-and-hyperparameters
cfg_ddpg = DDPG_DEFAULT_CONFIG.copy()
# cfg_ddpg["exploration"]["noise"] = OrnsteinUhlenbeckNoise(theta=0.15, sigma=0.1, base_scale=1.0, device=device)
cfg_ddpg["gradient_steps"] = 1 # gradient steps
cfg_ddpg["batch_size"] = 32 # training batch size
cfg_ddpg["polyak"] = 0.005 # soft update hyperparameter (tau)
cfg_ddpg["discount_factor"] = 0.99 # discount factor (gamma)
cfg_ddpg["random_timesteps"] = 0 # random exploration steps
cfg_ddpg["learning_starts"] = 0 # learning starts after this many steps
cfg_ddpg["actor_learning_rate"] = 1e-3
cfg_ddpg["critic_learning_rate"] = 5e-3
# cfg_ddpg["rewards_shaper"] = lambda rewards, timestep, timesteps: rewards * 0.01 # rewards shaping function: Callable(reward, timestep, timesteps) -> reward
# logging to TensorBoard and write checkpoints each 1000 and 5000 timesteps respectively
cfg_ddpg["experiment"]["write_interval"] = 100
cfg_ddpg["experiment"]["checkpoint_interval"] = 1000
# cfg_ddpg["experiment"]["experiment_name"] = ""
agent = DDPG(models=models_ddpg,
memory=memory,
cfg=cfg_ddpg,
observation_space=env.observation_space,
action_space=env.action_space,
device=device)
# Configure and instantiate the RL trainer
cfg_trainer = {"timesteps": 320000, "headless": True}
trainer = SequentialTrainer(cfg=cfg_trainer, env=env, agents=agent)
# start training
trainer.train()
| 5,728 | Python | 44.110236 | 159 | 0.686627 |
zhehuazhou/ai-cps-robotics-benchmark/Gym_Envs/skrl_train_PPO.py | import torch
import os
import torch.nn as nn
# Import the skrl components to build the RL system
from skrl.models.torch import Model, GaussianMixin, DeterministicMixin
from skrl.memories.torch import RandomMemory
from skrl.agents.torch.ppo import PPO, PPO_DEFAULT_CONFIG
from skrl.resources.schedulers.torch import KLAdaptiveRL
from skrl.resources.preprocessors.torch import RunningStandardScaler
from skrl.trainers.torch import SequentialTrainer
from skrl.envs.torch import wrap_env
from skrl.envs.torch import load_omniverse_isaacgym_env
from skrl.utils import set_seed
# set the seed for reproducibility
set_seed(42)
# Define the shared model (stochastic and deterministic models) for the agent using mixins.
class Shared(GaussianMixin, DeterministicMixin, Model):
def __init__(self, observation_space, action_space, device, clip_actions=False,
clip_log_std=True, min_log_std=-20, max_log_std=2, reduction="sum"):
Model.__init__(self, observation_space, action_space, device)
GaussianMixin.__init__(self, clip_actions, clip_log_std, min_log_std, max_log_std, reduction)
DeterministicMixin.__init__(self, clip_actions)
self.net = nn.Sequential(nn.Linear(self.num_observations, 512),
nn.ELU(),
nn.Linear(512, 256),
nn.ELU(),
nn.Linear(256, 128),
nn.ELU())
self.mean_layer = nn.Linear(128, self.num_actions)
self.log_std_parameter = nn.Parameter(torch.zeros(self.num_actions))
self.value_layer = nn.Linear(128, 1)
def act(self, inputs, role):
if role == "policy":
return GaussianMixin.act(self, inputs, role)
elif role == "value":
return DeterministicMixin.act(self, inputs, role)
def compute(self, inputs, role):
if role == "policy":
return self.mean_layer(self.net(inputs["states"])), self.log_std_parameter, {}
elif role == "value":
return self.value_layer(self.net(inputs["states"])), {}
# Load and wrap the Omniverse Isaac Gym environment]
omniisaacgymenvs_path = os.path.realpath( os.path.join(os.path.realpath(__file__), "..") )
env = load_omniverse_isaacgym_env(task_name="FrankaPegInHole", omniisaacgymenvs_path = omniisaacgymenvs_path)
env = wrap_env(env)
device = env.device
# Instantiate a RandomMemory as rollout buffer (any memory can be used for this)
memory = RandomMemory(memory_size=32, num_envs=env.num_envs, device=device)
# Instantiate the agent's models (function approximators).
# PPO requires 2 models, visit its documentation for more details
# https://skrl.readthedocs.io/en/latest/modules/skrl.agents.ppo.html#spaces-and-models
models_ppo = {}
models_ppo["policy"] = Shared(env.observation_space, env.action_space, device)
models_ppo["value"] = models_ppo["policy"] # same instance: shared model
# Configure and instantiate the agent.
# Only modify some of the default configuration, visit its documentation to see all the options
# https://skrl.readthedocs.io/en/latest/modules/skrl.agents.ppo.html#configuration-and-hyperparameters
cfg_ppo = PPO_DEFAULT_CONFIG.copy()
cfg_ppo["rollouts"] = 32 # memory_size
cfg_ppo["learning_epochs"] = 16
cfg_ppo["mini_batches"] = 8 # 16 * 8192 / 32768
cfg_ppo["discount_factor"] = 0.99
cfg_ppo["lambda"] = 0.95
cfg_ppo["learning_rate"] = 5e-4
cfg_ppo["learning_rate_scheduler"] = KLAdaptiveRL
cfg_ppo["learning_rate_scheduler_kwargs"] = {"kl_threshold": 0.02}
cfg_ppo["random_timesteps"] = 0
cfg_ppo["learning_starts"] = 0
cfg_ppo["grad_norm_clip"] = 1.0
cfg_ppo["ratio_clip"] = 0.2
cfg_ppo["value_clip"] = 0.2
cfg_ppo["clip_predicted_values"] = True
cfg_ppo["entropy_loss_scale"] = 0.0
cfg_ppo["value_loss_scale"] = 2.0
cfg_ppo["kl_threshold"] = 0
# cfg_ppo["rewards_shaper"] = lambda rewards, timestep, timesteps: rewards * 0.01
cfg_ppo["state_preprocessor"] = RunningStandardScaler
cfg_ppo["state_preprocessor_kwargs"] = {"size": env.observation_space, "device": device}
cfg_ppo["value_preprocessor"] = RunningStandardScaler
cfg_ppo["value_preprocessor_kwargs"] = {"size": 1, "device": device}
# logging to TensorBoard and write checkpoints each 800 and 8000 timesteps respectively
cfg_ppo["experiment"]["write_interval"] = 100
cfg_ppo["experiment"]["checkpoint_interval"] = 1000
agent = PPO(models=models_ppo,
memory=memory,
cfg=cfg_ppo,
observation_space=env.observation_space,
action_space=env.action_space,
device=device)
# Configure and instantiate the RL trainer
cfg_trainer = {"timesteps": 1000000, "headless": True}
trainer = SequentialTrainer(cfg=cfg_trainer, env=env, agents=agent)
# start training
trainer.train()
| 4,809 | Python | 39.762712 | 109 | 0.688917 |
zhehuazhou/ai-cps-robotics-benchmark/Gym_Envs/skrl_train_TRPO.py | import torch
import os
import torch.nn as nn
# Import the skrl components to build the RL system
from skrl.models.torch import Model, GaussianMixin, DeterministicMixin
from skrl.memories.torch import RandomMemory
from skrl.agents.torch.trpo import TRPO, TRPO_DEFAULT_CONFIG
from skrl.resources.schedulers.torch import KLAdaptiveRL
from skrl.resources.preprocessors.torch import RunningStandardScaler
from skrl.trainers.torch import SequentialTrainer
from skrl.envs.torch import wrap_env
from skrl.envs.torch import load_omniverse_isaacgym_env
from skrl.utils import set_seed
# set the seed for reproducibility
set_seed(42)
# Define the models (stochastic and deterministic models) for the agent using mixins.
# - Policy: takes as input the environment's observation/state and returns an action
# - Value: takes the state as input and provides a value to guide the policy
class Policy(GaussianMixin, Model):
def __init__(self, observation_space, action_space, device, clip_actions=False,
clip_log_std=True, min_log_std=-20, max_log_std=2):
Model.__init__(self, observation_space, action_space, device)
GaussianMixin.__init__(self, clip_actions, clip_log_std, min_log_std, max_log_std)
# self.net = nn.Sequential(nn.Linear(self.num_observations, 512),
# nn.ELU(),
# nn.Linear(512, 256),
# nn.ELU(),
# nn.Linear(256, self.num_actions))
self.net = nn.Sequential(nn.Linear(self.num_observations, 512),
nn.ELU(),
nn.Linear(512, 256),
nn.ELU(),
nn.Linear(256, 128),
nn.ELU(),
nn.Linear(128, self.num_actions))
self.log_std_parameter = nn.Parameter(torch.zeros(self.num_actions))
def compute(self, inputs, role):
return self.net(inputs["states"]), self.log_std_parameter, {}
class Value(DeterministicMixin, Model):
def __init__(self, observation_space, action_space, device, clip_actions=False):
Model.__init__(self, observation_space, action_space, device)
DeterministicMixin.__init__(self, clip_actions)
# self.net = nn.Sequential(nn.Linear(self.num_observations, 512),
# nn.ELU(),
# nn.Linear(512, 256),
# nn.ELU(),
# nn.Linear(256, 1))
self.net = nn.Sequential(nn.Linear(self.num_observations, 512),
nn.ELU(),
nn.Linear(512, 256),
nn.ELU(),
nn.Linear(256, 128),
nn.ELU(),
nn.Linear(128, 1))
def compute(self, inputs, role):
return self.net(inputs["states"]), {}
# Load and wrap the Omniverse Isaac Gym environment]
omniisaacgymenvs_path = os.path.realpath( os.path.join(os.path.realpath(__file__), "..") )
env = load_omniverse_isaacgym_env(task_name="FrankaPegInHole", omniisaacgymenvs_path = omniisaacgymenvs_path)
env = wrap_env(env)
device = env.device
# Instantiate a RandomMemory as rollout buffer (any memory can be used for this)
memory = RandomMemory(memory_size=32, num_envs=env.num_envs, device=device)
# Instantiate the agent's models (function approximators).
# TRPO requires 2 models, visit its documentation for more details
# https://skrl.readthedocs.io/en/latest/modules/skrl.agents.trpo.html#spaces-and-models
models_trpo = {}
models_trpo["policy"] = Policy(env.observation_space, env.action_space, device)
models_trpo["value"] = Value(env.observation_space, env.action_space, device)
# Configure and instantiate the agent.
# Only modify some of the default configuration, visit its documentation to see all the options
# https://skrl.readthedocs.io/en/latest/modules/skrl.agents.trpo.html#configuration-and-hyperparameters
cfg_trpo = TRPO_DEFAULT_CONFIG.copy()
cfg_trpo["rollouts"] = 32 # memory_size
cfg_trpo["learning_epochs"] = 16
cfg_trpo["mini_batches"] = 8
cfg_trpo["discount_factor"] = 0.99
cfg_trpo["lambda"] = 0.95
cfg_trpo["learning_rate"] = 5e-4
cfg_trpo["grad_norm_clip"] = 1.0
cfg_trpo["value_loss_scale"] = 2.0
cfg_trpo["state_preprocessor"] = RunningStandardScaler
cfg_trpo["state_preprocessor_kwargs"] = {"size": env.observation_space, "device": device}
cfg_trpo["value_preprocessor"] = RunningStandardScaler
cfg_trpo["value_preprocessor_kwargs"] = {"size": 1, "device": device}
# logging to TensorBoard and write checkpoints each 16 and 80 timesteps respectively
cfg_trpo["experiment"]["write_interval"] = 100
cfg_trpo["experiment"]["checkpoint_interval"] = 1000
agent = TRPO(models=models_trpo,
memory=memory,
cfg=cfg_trpo,
observation_space=env.observation_space,
action_space=env.action_space,
device=device)
# Configure and instantiate the RL trainer
cfg_trainer = {"timesteps": 320000, "headless": True}
trainer = SequentialTrainer(cfg=cfg_trainer, env=env, agents=agent)
# start training
trainer.train()
| 5,310 | Python | 41.150793 | 109 | 0.632203 |
zhehuazhou/ai-cps-robotics-benchmark/Gym_Envs/Models/Franka/Franka.py | # Copyright (c) 2021, NVIDIA CORPORATION. All rights reserved.
#
# NVIDIA CORPORATION and its licensors retain all intellectual property
# and proprietary rights in and to this software, related documentation
# and any modifications thereto. Any use, reproduction, disclosure or
# distribution of this software and related documentation without an express
# license agreement from NVIDIA CORPORATION is strictly prohibited.
#
import os
from typing import Optional
import math
import numpy as np
import torch
from omni.isaac.core.robots.robot import Robot
from omni.isaac.core.utils.nucleus import get_assets_root_path
from omni.isaac.core.utils.stage import add_reference_to_stage
from omniisaacgymenvs.tasks.utils.usd_utils import set_drive
from omni.isaac.core.utils.prims import get_prim_at_path
from pxr import PhysxSchema
class Franka(Robot):
def __init__(
self,
prim_path: str,
name: Optional[str] = "franka",
usd_path: Optional[str] = None,
translation: Optional[torch.tensor] = None,
orientation: Optional[torch.tensor] = None,
use_modified_collision: Optional[bool] = False,
) -> None:
"""[summary]
"""
self._usd_path = usd_path
self._name = name
self._position = torch.tensor([1.0, 0.0, 0.0]) if translation is None else translation
self._orientation = torch.tensor([0.0, 0.0, 0.0, 1.0]) if orientation is None else orientation
if use_modified_collision is True:
print("Load modified franka model")
self._usd_path = os.path.realpath( os.path.join(os.path.realpath(__file__), "..") ) + "/franka_instanceable.usd"
elif self._usd_path is None:
assets_root_path = get_assets_root_path()
if assets_root_path is None:
carb.log_error("Could not find Isaac Sim assets folder")
self._usd_path = assets_root_path + "/Isaac/Robots/Franka/franka_instanceable.usd"
add_reference_to_stage(self._usd_path, prim_path)
super().__init__(
prim_path=prim_path,
name=name,
translation=self._position,
orientation=self._orientation,
articulation_controller=None,
)
dof_paths = [
"panda_link0/panda_joint1",
"panda_link1/panda_joint2",
"panda_link2/panda_joint3",
"panda_link3/panda_joint4",
"panda_link4/panda_joint5",
"panda_link5/panda_joint6",
"panda_link6/panda_joint7",
"panda_hand/panda_finger_joint1",
"panda_hand/panda_finger_joint2"
]
drive_type = ["angular"] * 7 + ["linear"] * 2
default_dof_pos = [math.degrees(x) for x in [0.0, -1.0, 0.0, -2.2, 0.0, 2.4, 0.8]] + [0.02, 0.02]
stiffness = [400*np.pi/180] * 7 + [10000] * 2
damping = [80*np.pi/180] * 7 + [100] * 2
max_force = [87, 87, 87, 87, 12, 12, 12, 200, 200]
max_velocity = [math.degrees(x) for x in [2.175, 2.175, 2.175, 2.175, 2.61, 2.61, 2.61]] + [0.2, 0.2]
for i, dof in enumerate(dof_paths):
set_drive(
prim_path=f"{self.prim_path}/{dof}",
drive_type=drive_type[i],
target_type="position",
target_value=default_dof_pos[i],
stiffness=stiffness[i],
damping=damping[i],
max_force=max_force[i]
)
PhysxSchema.PhysxJointAPI(get_prim_at_path(f"{self.prim_path}/{dof}")).CreateMaxJointVelocityAttr().Set(max_velocity[i])
| 3,605 | Python | 37.361702 | 132 | 0.603051 |
zhehuazhou/ai-cps-robotics-benchmark/Gym_Envs/Models/Franka/Franka_view.py |
from typing import Optional
from omni.isaac.core.articulations import ArticulationView
from omni.isaac.core.prims import RigidPrimView
class FrankaView(ArticulationView):
def __init__(
self,
prim_paths_expr: str,
name: Optional[str] = "FrankaView",
) -> None:
"""[summary]
"""
super().__init__(
prim_paths_expr=prim_paths_expr,
name=name,
reset_xform_properties=False
)
self._hands = RigidPrimView(prim_paths_expr=prim_paths_expr + "/panda_link7", name="hands_view", reset_xform_properties=False)
self._lfingers = RigidPrimView(prim_paths_expr=prim_paths_expr + "/panda_leftfinger", name="lfingers_view", reset_xform_properties=False)
self._rfingers = RigidPrimView(prim_paths_expr=prim_paths_expr + "/panda_rightfinger", name="rfingers_view", reset_xform_properties=False)
def initialize(self, physics_sim_view):
super().initialize(physics_sim_view)
self._gripper_indices = [self.get_dof_index("panda_finger_joint1"), self.get_dof_index("panda_finger_joint2")]
@property
def gripper_indices(self):
return self._gripper_indices
| 1,211 | Python | 32.666666 | 147 | 0.648225 |
zhehuazhou/ai-cps-robotics-benchmark/Gym_Envs/Models/ball_catching/tool.py | # Copyright (c) 2021, NVIDIA CORPORATION. All rights reserved.
#
# NVIDIA CORPORATION and its licensors retain all intellectual property
# and proprietary rights in and to this software, related documentation
# and any modifications thereto. Any use, reproduction, disclosure or
# distribution of this software and related documentation without an express
# license agreement from NVIDIA CORPORATION is strictly prohibited.
#
from typing import Optional
from omni.isaac.core.robots.robot import Robot
from omni.isaac.core.utils.nucleus import get_assets_root_path
from omni.isaac.core.utils.stage import add_reference_to_stage
import numpy as np
import torch
import os
class Tool(Robot):
def __init__(
self,
prim_path: str,
name: Optional[str] = "tool",
usd_path: Optional[str] = None,
translation: Optional[torch.tensor] = None,
orientation: Optional[torch.tensor] = None,
) -> None:
"""[summary]
"""
self._name = name
self._usd_path = os.path.realpath( os.path.join(os.path.realpath(__file__), "..") ) + "/tool.usd"
add_reference_to_stage(self._usd_path, prim_path)
self._position = torch.tensor([-0.05, 0.0, 0.88]) if translation is None else translation
self._orientation = torch.tensor([1, 0.0, 0.0, 0.0]) if orientation is None else orientation
super().__init__(
prim_path=prim_path,
name=name,
translation=self._position,
orientation=self._orientation,
articulation_controller=None,
)
| 1,601 | Python | 32.374999 | 105 | 0.657714 |
zhehuazhou/ai-cps-robotics-benchmark/Gym_Envs/Models/ball_balancing/tool.py | # Copyright (c) 2021, NVIDIA CORPORATION. All rights reserved.
#
# NVIDIA CORPORATION and its licensors retain all intellectual property
# and proprietary rights in and to this software, related documentation
# and any modifications thereto. Any use, reproduction, disclosure or
# distribution of this software and related documentation without an express
# license agreement from NVIDIA CORPORATION is strictly prohibited.
#
from typing import Optional
from omni.isaac.core.robots.robot import Robot
from omni.isaac.core.utils.nucleus import get_assets_root_path
from omni.isaac.core.utils.stage import add_reference_to_stage
import numpy as np
import torch
import os
class Tool(Robot):
def __init__(
self,
prim_path: str,
name: Optional[str] = "tool",
usd_path: Optional[str] = None,
translation: Optional[torch.tensor] = None,
orientation: Optional[torch.tensor] = None,
) -> None:
"""[summary]
"""
self._name = name
self._usd_path = os.path.realpath( os.path.join(os.path.realpath(__file__), "..") ) + "/tool.usd"
add_reference_to_stage(self._usd_path, prim_path)
self._position = torch.tensor([0.008, 0.0, 1.0]) if translation is None else translation
self._orientation = torch.tensor([1, 0.0, 0.0, 0.0]) if orientation is None else orientation
super().__init__(
prim_path=prim_path,
name=name,
translation=self._position,
orientation=self._orientation,
articulation_controller=None,
)
| 1,600 | Python | 32.354166 | 105 | 0.658125 |
zhehuazhou/ai-cps-robotics-benchmark/Gym_Envs/Models/door_open/door_view.py |
from typing import Optional
from omni.isaac.core.articulations import ArticulationView
from omni.isaac.core.prims import RigidPrimView
class DoorView(ArticulationView):
def __init__(
self,
prim_paths_expr: str,
name: Optional[str] = "DoorView",
) -> None:
"""[summary]
"""
super().__init__(
prim_paths_expr=prim_paths_expr,
name=name,
reset_xform_properties=False
)
self._handle = RigidPrimView(prim_paths_expr="/World/envs/.*/door/door/handle_point", name="handle_view", reset_xform_properties=False)
| 616 | Python | 24.708332 | 143 | 0.613636 |
zhehuazhou/ai-cps-robotics-benchmark/Gym_Envs/Models/door_open/door.py | # Copyright (c) 2021, NVIDIA CORPORATION. All rights reserved.
#
# NVIDIA CORPORATION and its licensors retain all intellectual property
# and proprietary rights in and to this software, related documentation
# and any modifications thereto. Any use, reproduction, disclosure or
# distribution of this software and related documentation without an express
# license agreement from NVIDIA CORPORATION is strictly prohibited.
#
from typing import Optional
from omni.isaac.core.robots.robot import Robot
from omni.isaac.core.utils.nucleus import get_assets_root_path
from omni.isaac.core.utils.stage import add_reference_to_stage
import numpy as np
import torch
import os
class Door(Robot):
def __init__(
self,
prim_path: str,
name: Optional[str] = "door",
usd_path: Optional[str] = None,
translation: Optional[torch.tensor] = None,
orientation: Optional[torch.tensor] = None,
) -> None:
"""[summary]
"""
self._name = name
self._usd_path = os.path.realpath( os.path.join(os.path.realpath(__file__), "..") ) + "/free_door_point_pull.usd"
add_reference_to_stage(self._usd_path, prim_path)
self._position = torch.tensor([-0.3, 0.0, 0.0]) if translation is None else translation
self._orientation = torch.tensor([1, 0.0, 0.0, 0.0]) if orientation is None else orientation
super().__init__(
prim_path=prim_path,
name=name,
translation=self._position,
orientation=self._orientation,
articulation_controller=None,
)
| 1,613 | Python | 32.624999 | 121 | 0.65964 |
zhehuazhou/ai-cps-robotics-benchmark/Gym_Envs/Models/ball_pushing/table_view.py |
from typing import Optional
from omni.isaac.core.articulations import ArticulationView
from omni.isaac.core.prims import RigidPrimView
class DoorView(ArticulationView):
def __init__(
self,
prim_paths_expr: str,
name: Optional[str] = "TableView",
) -> None:
"""[summary]
"""
super().__init__(
prim_paths_expr=prim_paths_expr,
name=name,
reset_xform_properties=False
)
self._table = RigidPrimView(prim_paths_expr="/World/envs/.*/table/table", name="table_view", reset_xform_properties=False)
| 604 | Python | 24.208332 | 130 | 0.609272 |
zhehuazhou/ai-cps-robotics-benchmark/Gym_Envs/Models/ball_pushing/table.py | # Copyright (c) 2021, NVIDIA CORPORATION. All rights reserved.
#
# NVIDIA CORPORATION and its licensors retain all intellectual property
# and proprietary rights in and to this software, related documentation
# and any modifications thereto. Any use, reproduction, disclosure or
# distribution of this software and related documentation without an express
# license agreement from NVIDIA CORPORATION is strictly prohibited.
#
from typing import Optional
from omni.isaac.core.robots.robot import Robot
from omni.isaac.core.utils.nucleus import get_assets_root_path
from omni.isaac.core.utils.stage import add_reference_to_stage
import numpy as np
import torch
import os
class Table(Robot):
def __init__(
self,
prim_path: str,
name: Optional[str] = "table",
usd_path: Optional[str] = None,
translation: Optional[torch.tensor] = None,
orientation: Optional[torch.tensor] = None,
) -> None:
"""[summary]
"""
self._name = name
self._usd_path = os.path.realpath( os.path.join(os.path.realpath(__file__), "..") ) + "/table.usd"
add_reference_to_stage(self._usd_path, prim_path)
self._position = torch.tensor([0.0, 0.0, 0.0]) if translation is None else translation
self._orientation = torch.tensor([1, 0.0, 0.0, 0.0]) if orientation is None else orientation
super().__init__(
prim_path=prim_path,
name=name,
translation=self._position,
orientation=self._orientation,
articulation_controller=None,
)
| 1,599 | Python | 32.333333 | 106 | 0.659162 |
zhehuazhou/ai-cps-robotics-benchmark/Gym_Envs/Models/peg_in_hole/table_view.py |
from typing import Optional
from omni.isaac.core.articulations import ArticulationView
from omni.isaac.core.prims import RigidPrimView
class TableView(ArticulationView):
def __init__(
self,
prim_paths_expr: str,
name: Optional[str] = "TableView",
) -> None:
"""[summary]
"""
super().__init__(
prim_paths_expr=prim_paths_expr,
name=name,
reset_xform_properties=False
)
self._location_ball = RigidPrimView(prim_paths_expr="/World/envs/.*/table/table/location_ball", name="location_ball_view", reset_xform_properties=False)
| 635 | Python | 25.499999 | 160 | 0.622047 |
zhehuazhou/ai-cps-robotics-benchmark/Gym_Envs/Models/peg_in_hole/table.py | # Copyright (c) 2021, NVIDIA CORPORATION. All rights reserved.
#
# NVIDIA CORPORATION and its licensors retain all intellectual property
# and proprietary rights in and to this software, related documentation
# and any modifications thereto. Any use, reproduction, disclosure or
# distribution of this software and related documentation without an express
# license agreement from NVIDIA CORPORATION is strictly prohibited.
#
from typing import Optional
from omni.isaac.core.robots.robot import Robot
from omni.isaac.core.utils.nucleus import get_assets_root_path
from omni.isaac.core.utils.stage import add_reference_to_stage
import numpy as np
import torch
import os
class Table(Robot):
def __init__(
self,
prim_path: str,
name: Optional[str] = "table",
usd_path: Optional[str] = None,
translation: Optional[torch.tensor] = None,
orientation: Optional[torch.tensor] = None,
) -> None:
"""[summary]
"""
self._name = name
self._usd_path = os.path.realpath( os.path.join(os.path.realpath(__file__), "..") ) + "/table.usd"
add_reference_to_stage(self._usd_path, prim_path)
self._position = torch.tensor([0.0, 0.0, 0.0]) if translation is None else translation
self._orientation = torch.tensor([1, 0.0, 0.0, 0.0]) if orientation is None else orientation
super().__init__(
prim_path=prim_path,
name=name,
translation=self._position,
orientation=self._orientation,
articulation_controller=None,
)
| 1,591 | Python | 32.166666 | 106 | 0.662476 |
zhehuazhou/ai-cps-robotics-benchmark/Gym_Envs/Models/peg_in_hole/tool.py | # Copyright (c) 2021, NVIDIA CORPORATION. All rights reserved.
#
# NVIDIA CORPORATION and its licensors retain all intellectual property
# and proprietary rights in and to this software, related documentation
# and any modifications thereto. Any use, reproduction, disclosure or
# distribution of this software and related documentation without an express
# license agreement from NVIDIA CORPORATION is strictly prohibited.
#
from typing import Optional
from omni.isaac.core.robots.robot import Robot
from omni.isaac.core.utils.nucleus import get_assets_root_path
from omni.isaac.core.utils.stage import add_reference_to_stage
import numpy as np
import torch
import os
class Tool(Robot):
def __init__(
self,
prim_path: str,
name: Optional[str] = "tool",
usd_path: Optional[str] = None,
translation: Optional[torch.tensor] = None,
orientation: Optional[torch.tensor] = None,
) -> None:
"""[summary]
"""
self._name = name
self._usd_path = os.path.realpath( os.path.join(os.path.realpath(__file__), "..") ) + "/tool.usd"
add_reference_to_stage(self._usd_path, prim_path)
self._position = torch.tensor([0.391, 0, 0.786]) if translation is None else translation
self._orientation = torch.tensor([0, 0.0, 0.0, 0.0]) if orientation is None else orientation
super().__init__(
prim_path=prim_path,
name=name,
translation=self._position,
orientation=self._orientation,
articulation_controller=None,
)
| 1,600 | Python | 32.354166 | 105 | 0.65875 |
zhehuazhou/ai-cps-robotics-benchmark/Gym_Envs/Models/point_reaching/target_ball.py | # Copyright (c) 2021, NVIDIA CORPORATION. All rights reserved.
#
# NVIDIA CORPORATION and its licensors retain all intellectual property
# and proprietary rights in and to this software, related documentation
# and any modifications thereto. Any use, reproduction, disclosure or
# distribution of this software and related documentation without an express
# license agreement from NVIDIA CORPORATION is strictly prohibited.
#
from typing import Optional
from omni.isaac.core.robots.robot import Robot
from omni.isaac.core.utils.nucleus import get_assets_root_path
from omni.isaac.core.utils.stage import add_reference_to_stage
import numpy as np
import torch
import os
class TargetBall(Robot):
def __init__(
self,
prim_path: str,
name: Optional[str] = "target_ball",
usd_path: Optional[str] = None,
translation: Optional[torch.tensor] = None,
orientation: Optional[torch.tensor] = None,
) -> None:
"""[summary]
"""
self._name = name
self._usd_path = os.path.realpath( os.path.join(os.path.realpath(__file__), "..") ) + "/target_ball.usd"
add_reference_to_stage(self._usd_path, prim_path)
self._position = torch.tensor([-0.2, 0.0, 0.6]) if translation is None else translation
self._orientation = torch.tensor([1, 0.0, 0.0, 0.0]) if orientation is None else orientation
super().__init__(
prim_path=prim_path,
name=name,
translation=self._position,
orientation=self._orientation,
articulation_controller=None,
)
| 1,617 | Python | 32.708333 | 112 | 0.661101 |
zhehuazhou/ai-cps-robotics-benchmark/Gym_Envs/Models/cloth_placing/target_table.py | # Copyright (c) 2021, NVIDIA CORPORATION. All rights reserved.
#
# NVIDIA CORPORATION and its licensors retain all intellectual property
# and proprietary rights in and to this software, related documentation
# and any modifications thereto. Any use, reproduction, disclosure or
# distribution of this software and related documentation without an express
# license agreement from NVIDIA CORPORATION is strictly prohibited.
#
from typing import Optional
from omni.isaac.core.robots.robot import Robot
from omni.isaac.core.utils.nucleus import get_assets_root_path
from omni.isaac.core.utils.stage import add_reference_to_stage
import numpy as np
import torch
import os
class TargetTable(Robot):
def __init__(
self,
prim_path: str,
name: Optional[str] = "target_table",
usd_path: Optional[str] = None,
translation: Optional[torch.tensor] = None,
orientation: Optional[torch.tensor] = None,
) -> None:
"""[summary]
"""
self._name = name
self._usd_path = os.path.realpath( os.path.join(os.path.realpath(__file__), "..") ) + "/target_table.usd"
add_reference_to_stage(self._usd_path, prim_path)
self._position = torch.tensor([-0.25, 0.0, 0.0]) if translation is None else translation
self._orientation = torch.tensor([1, 0.0, 0.0, 0.0]) if orientation is None else orientation
super().__init__(
prim_path=prim_path,
name=name,
translation=self._position,
orientation=self._orientation,
articulation_controller=None,
)
| 1,621 | Python | 32.791666 | 113 | 0.661937 |
zhehuazhou/ai-cps-robotics-benchmark/Gym_Envs/cfg/config.yaml |
# Task name - used to pick the class to load
task_name: ${task.name}
# experiment name. defaults to name of training config
experiment: ''
# if set to positive integer, overrides the default number of environments
num_envs: ''
# seed - set to -1 to choose random seed
seed: 0
# set to True for deterministic performance
torch_deterministic: False
# set the maximum number of learning iterations to train for. overrides default per-environment setting
max_iterations: ''
## Device config
physics_engine: 'physx'
# whether to use cpu or gpu pipeline
pipeline: 'gpu'
# whether to use cpu or gpu physx
sim_device: 'gpu'
# used for gpu simulation only - device id for running sim and task if pipeline=gpu
device_id: 0
# device to run RL
rl_device: 'cuda:0'
## PhysX arguments
num_threads: 4 # Number of worker threads per scene used by PhysX - for CPU PhysX only.
solver_type: 1 # 0: pgs, 1: tgs
# RLGames Arguments
# test - if set, run policy in inference mode (requires setting checkpoint to load)
test: False
# used to set checkpoint path
checkpoint: ''
# disables rendering
headless: False
# timeout for MT script
mt_timeout: 30
wandb_activate: False
wandb_group: ''
wandb_name: ${train.params.config.name}
wandb_entity: ''
wandb_project: 'omniisaacgymenvs'
# set default task and default training config based on task
defaults:
- task: FrankaBallPushing
- train: ${task}PPO
- hydra/job_logging: disabled
# set the directory where the output files get saved
hydra:
output_subdir: null
run:
dir: .
| 1,522 | YAML | 23.564516 | 103 | 0.739816 |
zhehuazhou/ai-cps-robotics-benchmark/Gym_Envs/cfg/task/FrankaDoorOpen.yaml | # used to create the object
name: FrankaDoorOpen
physics_engine: ${..physics_engine}
# if given, will override the device setting in gym.
env:
numEnvs: ${resolve_default:16,${...num_envs}}
envSpacing: 3.0
episodeLength: 300
enableDebugVis: False
clipObservations: 5.0
clipActions: 1.0
controlFrequencyInv: 2 # 60 Hz
startPositionNoise: 0.0
startRotationNoise: 0.0
aggregateMode: 3
actionScale: 7.5
dofVelocityScale: 0.1
distRewardScale: 2.0
rotRewardScale: 0.5
aroundHandleRewardScale: 10.0
openRewardScale: 7.5
fingerDistRewardScale: 100.0
actionPenaltyScale: 0.01
fingerCloseRewardScale: 10.0
sim:
dt: 0.0083 # 1/120 s
use_gpu_pipeline: ${eq:${...pipeline},"gpu"}
gravity: [0.0, 0.0, -9.81]
add_ground_plane: True
use_flatcache: True
enable_scene_query_support: False
disable_contact_processing: False
# set to True if you use camera sensors in the environment
enable_cameras: False
default_physics_material:
static_friction: 1.0
dynamic_friction: 1.0
restitution: 0.0
physx:
worker_thread_count: ${....num_threads}
solver_type: ${....solver_type}
use_gpu: ${eq:${....sim_device},"gpu"} # set to False to run on CPU
solver_position_iteration_count: 12
solver_velocity_iteration_count: 1
contact_offset: 0.005
rest_offset: 0.0
bounce_threshold_velocity: 0.2
friction_offset_threshold: 0.04
friction_correlation_distance: 0.025
enable_sleeping: True
enable_stabilization: True
max_depenetration_velocity: 1000.0
# GPU buffers
gpu_max_rigid_contact_count: 524288
gpu_max_rigid_patch_count: 33554432
gpu_found_lost_pairs_capacity: 524288
gpu_found_lost_aggregate_pairs_capacity: 262144
gpu_total_aggregate_pairs_capacity: 1048576
gpu_max_soft_body_contacts: 1048576
gpu_max_particle_contacts: 1048576
gpu_heap_capacity: 33554432
gpu_temp_buffer_capacity: 16777216
gpu_max_num_partitions: 8
franka:
# -1 to use default values
override_usd_defaults: False
enable_self_collisions: True
enable_gyroscopic_forces: True
# also in stage params
# per-actor
solver_position_iteration_count: 12
solver_velocity_iteration_count: 1
sleep_threshold: 0.005
stabilization_threshold: 0.001
# per-body
density: -1
max_depenetration_velocity: 1000.0
# per-shape
contact_offset: 0.005
rest_offset: 0.0
door:
# -1 to use default values
override_usd_defaults: False
enable_self_collisions: False
enable_gyroscopic_forces: True
# also in stage params
# per-actor
solver_position_iteration_count: 12
solver_velocity_iteration_count: 1
sleep_threshold: 0.0
stabilization_threshold: 0.001
# per-body
density: -1
max_depenetration_velocity: 1000.0
# per-shape
contact_offset: 0.005
rest_offset: 0.0
| 2,887 | YAML | 25.018018 | 71 | 0.693453 |
zhehuazhou/ai-cps-robotics-benchmark/Gym_Envs/cfg/task/FrankaBallCatching.yaml | # used to create the object
name: FrankaBallCatching
physics_engine: ${..physics_engine}
# if given, will override the device setting in gym.
env:
numEnvs: ${resolve_default:4,${...num_envs}}
envSpacing: 3.0
episodeLength: 300
enableDebugVis: False
clipObservations: 5.0
clipActions: 1.0
controlFrequencyInv: 2 # 60 Hz
startPositionNoise: 0.0
startRotationNoise: 0.0
aggregateMode: 3
actionScale: 7.5
dofVelocityScale: 0.1
distRewardScale: 2.0
rotRewardScale: 0.5
aroundHandleRewardScale: 10.0
openRewardScale: 7.5
fingerDistRewardScale: 100.0
actionPenaltyScale: 0.01
fingerCloseRewardScale: 10.0
sim:
dt: 0.0083 # 1/120 s
use_gpu_pipeline: ${eq:${...pipeline},"gpu"}
gravity: [0.0, 0.0, -9.81]
add_ground_plane: True
use_flatcache: True
enable_scene_query_support: False
disable_contact_processing: False
# set to True if you use camera sensors in the environment
enable_cameras: False
default_physics_material:
static_friction: 1.0
dynamic_friction: 1.0
restitution: 0.0
physx:
worker_thread_count: ${....num_threads}
solver_type: ${....solver_type}
use_gpu: ${eq:${....sim_device},"gpu"} # set to False to run on CPU
solver_position_iteration_count: 12
solver_velocity_iteration_count: 1
contact_offset: 0.005
rest_offset: 0.0
bounce_threshold_velocity: 0.2
friction_offset_threshold: 0.04
friction_correlation_distance: 0.025
enable_sleeping: True
enable_stabilization: True
max_depenetration_velocity: 1000.0
# GPU buffers
gpu_max_rigid_contact_count: 624288
gpu_max_rigid_patch_count: 33554432
gpu_found_lost_pairs_capacity: 524288
gpu_found_lost_aggregate_pairs_capacity: 262144
gpu_total_aggregate_pairs_capacity: 1048576
gpu_max_soft_body_contacts: 1048576
gpu_max_particle_contacts: 1048576
gpu_heap_capacity: 33554432
gpu_temp_buffer_capacity: 16777216
gpu_max_num_partitions: 8
franka:
# -1 to use default values
override_usd_defaults: False
enable_self_collisions: True
enable_gyroscopic_forces: True
# also in stage params
# per-actor
solver_position_iteration_count: 12
solver_velocity_iteration_count: 1
sleep_threshold: 0.005
stabilization_threshold: 0.001
# per-body
density: -1
max_depenetration_velocity: 1000.0
# per-shape
contact_offset: 0.005
rest_offset: 0.0
tool:
# -1 to use default values
override_usd_defaults: False
enable_self_collisions: False
enable_gyroscopic_forces: True
# also in stage params
# per-actor
solver_position_iteration_count: 12
solver_velocity_iteration_count: 1
sleep_threshold: 0.0
stabilization_threshold: 0.001
# per-body
density: -1
max_depenetration_velocity: 1000.0
# per-shape
contact_offset: 0.005
rest_offset: 0.0
| 2,890 | YAML | 25.045045 | 71 | 0.693772 |
zhehuazhou/ai-cps-robotics-benchmark/Gym_Envs/cfg/task/FrankaBallBalancing.yaml | # used to create the object
name: FrankaBallBalancing
physics_engine: ${..physics_engine}
# if given, will override the device setting in gym.
env:
numEnvs: ${resolve_default:2048,${...num_envs}}
envSpacing: 3.0
episodeLength: 300
enableDebugVis: False
clipObservations: 5.0
clipActions: 1.0
controlFrequencyInv: 2 # 60 Hz
startPositionNoise: 0.0
startRotationNoise: 0.0
aggregateMode: 3
actionScale: 7.5
dofVelocityScale: 0.1
distRewardScale: 2.0
rotRewardScale: 0.5
aroundHandleRewardScale: 10.0
openRewardScale: 7.5
fingerDistRewardScale: 100.0
actionPenaltyScale: 0.01
fingerCloseRewardScale: 10.0
ballRadius: 0.02
ballInitialPosition: [0.008, 0, 1.1]
ballInitialOrientation: [1, 0, 0, 0]
sim:
dt: 0.0083 # 1/120 s
use_gpu_pipeline: ${eq:${...pipeline},"gpu"}
gravity: [0.0, 0.0, -9.81]
add_ground_plane: True
use_flatcache: True
enable_scene_query_support: False
disable_contact_processing: False
# set to True if you use camera sensors in the environment
enable_cameras: False
default_physics_material:
static_friction: 1.0
dynamic_friction: 1.0
restitution: 0.0
physx:
worker_thread_count: ${....num_threads}
solver_type: ${....solver_type}
use_gpu: ${eq:${....sim_device},"gpu"} # set to False to run on CPU
solver_position_iteration_count: 12
solver_velocity_iteration_count: 1
contact_offset: 0.005
rest_offset: 0.0
bounce_threshold_velocity: 0.2
friction_offset_threshold: 0.04
friction_correlation_distance: 0.025
enable_sleeping: True
enable_stabilization: True
max_depenetration_velocity: 1000.0
# GPU buffers
gpu_max_rigid_contact_count: 524288
gpu_max_rigid_patch_count: 33554432
gpu_found_lost_pairs_capacity: 524288
gpu_found_lost_aggregate_pairs_capacity: 262144
gpu_total_aggregate_pairs_capacity: 1048576
gpu_max_soft_body_contacts: 1048576
gpu_max_particle_contacts: 1048576
gpu_heap_capacity: 33554432
gpu_temp_buffer_capacity: 16777216
gpu_max_num_partitions: 8
franka:
# -1 to use default values
override_usd_defaults: False
enable_self_collisions: True
enable_gyroscopic_forces: True
# also in stage params
# per-actor
solver_position_iteration_count: 12
solver_velocity_iteration_count: 1
sleep_threshold: 0.005
stabilization_threshold: 0.001
# per-body
density: -1
max_depenetration_velocity: 1000.0
# per-shape
contact_offset: 0.005
rest_offset: 0.0
tool:
# -1 to use default values
override_usd_defaults: False
enable_self_collisions: False
enable_gyroscopic_forces: True
# also in stage params
# per-actor
solver_position_iteration_count: 12
solver_velocity_iteration_count: 1
sleep_threshold: 0.0
stabilization_threshold: 0.001
# per-body
density: -1
max_depenetration_velocity: 1000.0
# per-shape
contact_offset: 0.005
rest_offset: 0.0
ball:
# -1 to use default values
override_usd_defaults: False
fixed_base: False
enable_self_collisions: False
enable_gyroscopic_forces: True
# also in stage params
# per-actor
solver_position_iteration_count: 12
solver_velocity_iteration_count: 1
sleep_threshold: 0.0
stabilization_threshold: 0.001
# per-body
density: -1
max_depenetration_velocity: 1000.0
# per-shape
contact_offset: 0.005
rest_offset: 0.0
| 3,473 | YAML | 25.120301 | 71 | 0.68903 |
zhehuazhou/ai-cps-robotics-benchmark/Gym_Envs/cfg/task/FrankaCubeStacking.yaml | # used to create the object
name: FrankaCubeStacking
physics_engine: ${..physics_engine}
# if given, will override the device setting in gym.
env:
numEnvs: ${resolve_default:4,${...num_envs}}
envSpacing: 3.0
episodeLength: 300
enableDebugVis: False
clipObservations: 5.0
clipActions: 1.0
controlFrequencyInv: 2 # 60 Hz
startPositionNoise: 0.0
startRotationNoise: 0.0
aggregateMode: 3
actionScale: 7.5
dofVelocityScale: 0.1
distRewardScale: 2.0
rotRewardScale: 0.5
aroundHandleRewardScale: 10.0
openRewardScale: 7.5
fingerDistRewardScale: 100.0
actionPenaltyScale: 0.01
fingerCloseRewardScale: 10.0
sim:
dt: 0.0083 # 1/120 s
use_gpu_pipeline: ${eq:${...pipeline},"gpu"}
gravity: [0.0, 0.0, -9.81]
add_ground_plane: True
use_flatcache: True
enable_scene_query_support: False
disable_contact_processing: False
# set to True if you use camera sensors in the environment
enable_cameras: False
default_physics_material:
static_friction: 1.0
dynamic_friction: 1.0
restitution: 0.0
physx:
worker_thread_count: ${....num_threads}
solver_type: ${....solver_type}
use_gpu: ${eq:${....sim_device},"gpu"} # set to False to run on CPU
solver_position_iteration_count: 12
solver_velocity_iteration_count: 1
contact_offset: 0.005
rest_offset: 0.0
bounce_threshold_velocity: 0.2
friction_offset_threshold: 0.04
friction_correlation_distance: 0.025
enable_sleeping: True
enable_stabilization: True
max_depenetration_velocity: 1000.0
# GPU buffers
gpu_max_rigid_contact_count: 524288
gpu_max_rigid_patch_count: 33554432
gpu_found_lost_pairs_capacity: 524288
gpu_found_lost_aggregate_pairs_capacity: 262144
gpu_total_aggregate_pairs_capacity: 1048576
gpu_max_soft_body_contacts: 1048576
gpu_max_particle_contacts: 1048576
gpu_heap_capacity: 33554432
gpu_temp_buffer_capacity: 16777216
gpu_max_num_partitions: 8
franka:
# -1 to use default values
override_usd_defaults: False
enable_self_collisions: True
enable_gyroscopic_forces: True
# also in stage params
# per-actor
solver_position_iteration_count: 12
solver_velocity_iteration_count: 1
sleep_threshold: 0.005
stabilization_threshold: 0.001
# per-body
density: -1
max_depenetration_velocity: 1000.0
# per-shape
contact_offset: 0.005
rest_offset: 0.0
| 2,435 | YAML | 24.642105 | 71 | 0.698152 |
zhehuazhou/ai-cps-robotics-benchmark/Gym_Envs/cfg/task/FrankaPegInHole.yaml | # used to create the object
name: FrankaPegInHole
physics_engine: ${..physics_engine}
# if given, will override the device setting in gym.
env:
numEnvs: ${resolve_default:16,${...num_envs}}
envSpacing: 3.0
episodeLength: 300
enableDebugVis: False
clipObservations: 5.0
clipActions: 1.0
controlFrequencyInv: 2 # 60 Hz
startPositionNoise: 0.0
startRotationNoise: 0.0
aggregateMode: 3
actionScale: 7.5
dofVelocityScale: 0.02
distRewardScale: 2.0
rotRewardScale: 0.5
aroundHandleRewardScale: 10.0
openRewardScale: 7.5
fingerDistRewardScale: 100.0
actionPenaltyScale: 0.01
fingerCloseRewardScale: 10.0
locationBallRadius: 0.0001
locationBallPosition: [0.0, 0.0, 0.0]
locationBallInitialOrientation: [1, 0, 0, 0]
sim:
dt: 0.0083 # 1/120 s
use_gpu_pipeline: ${eq:${...pipeline},"gpu"}
gravity: [0.0, 0.0, -9.81]
add_ground_plane: True
use_flatcache: True
enable_scene_query_support: False
disable_contact_processing: False
# set to True if you use camera sensors in the environment
enable_cameras: False
default_physics_material:
static_friction: 1.0
dynamic_friction: 1.0
restitution: 0.0
physx:
worker_thread_count: ${....num_threads}
solver_type: ${....solver_type}
use_gpu: ${eq:${....sim_device},"gpu"} # set to False to run on CPU
solver_position_iteration_count: 12
solver_velocity_iteration_count: 1
contact_offset: 0.005
rest_offset: 0.0
bounce_threshold_velocity: 0.2
friction_offset_threshold: 0.04
friction_correlation_distance: 0.025
enable_sleeping: True
enable_stabilization: True
max_depenetration_velocity: 1000.0
# GPU buffers
gpu_max_rigid_contact_count: 524288
gpu_max_rigid_patch_count: 33554432
gpu_found_lost_pairs_capacity: 524288
gpu_found_lost_aggregate_pairs_capacity: 262144
gpu_total_aggregate_pairs_capacity: 1048576
gpu_max_soft_body_contacts: 1048576
gpu_max_particle_contacts: 1048576
gpu_heap_capacity: 33554432
gpu_temp_buffer_capacity: 16777216
gpu_max_num_partitions: 8
franka:
# -1 to use default values
override_usd_defaults: False
enable_self_collisions: True
enable_gyroscopic_forces: True
# also in stage params
# per-actor
solver_position_iteration_count: 12
solver_velocity_iteration_count: 1
sleep_threshold: 0.005
stabilization_threshold: 0.001
# per-body
density: -1
max_depenetration_velocity: 1000.0
# per-shape
contact_offset: 0.005
rest_offset: 0.0
table:
# -1 to use default values
override_usd_defaults: False
enable_self_collisions: False
enable_gyroscopic_forces: True
# also in stage params
# per-actor
solver_position_iteration_count: 12
solver_velocity_iteration_count: 1
sleep_threshold: 0.0
stabilization_threshold: 0.001
# per-body
density: -1
max_depenetration_velocity: 1000.0
# per-shape
contact_offset: 0.005
rest_offset: 0.0
tool:
# -1 to use default values
override_usd_defaults: False
fixed_base: False
enable_self_collisions: False
enable_gyroscopic_forces: True
# also in stage params
# per-actor
solver_position_iteration_count: 12
solver_velocity_iteration_count: 1
sleep_threshold: 0.0
stabilization_threshold: 0.001
# per-body
density: -1
max_depenetration_velocity: 1000.0
# per-shape
contact_offset: 0.005
rest_offset: 0.0
| 3,486 | YAML | 25.218045 | 71 | 0.690476 |
zhehuazhou/ai-cps-robotics-benchmark/Gym_Envs/cfg/task/FrankaPointReaching.yaml | # used to create the object
name: FrankaPointReaching
physics_engine: ${..physics_engine}
# if given, will override the device setting in gym.
env:
numEnvs: ${resolve_default:4,${...num_envs}}
envSpacing: 3.0
episodeLength: 300
enableDebugVis: False
clipObservations: 5.0
clipActions: 1.0
controlFrequencyInv: 2 # 60 Hz
startPositionNoise: 0.0
startRotationNoise: 0.0
aggregateMode: 3
actionScale: 7.5
dofVelocityScale: 0.1
distRewardScale: 2.0
rotRewardScale: 0.5
aroundHandleRewardScale: 10.0
openRewardScale: 7.5
fingerDistRewardScale: 100.0
actionPenaltyScale: 0.01
fingerCloseRewardScale: 10.0
sim:
dt: 0.0083 # 1/120 s
use_gpu_pipeline: ${eq:${...pipeline},"gpu"}
gravity: [0.0, 0.0, -9.81]
add_ground_plane: True
use_flatcache: True
enable_scene_query_support: False
disable_contact_processing: False
# set to True if you use camera sensors in the environment
enable_cameras: False
default_physics_material:
static_friction: 1.0
dynamic_friction: 1.0
restitution: 0.0
physx:
worker_thread_count: ${....num_threads}
solver_type: ${....solver_type}
use_gpu: ${eq:${....sim_device},"gpu"} # set to False to run on CPU
solver_position_iteration_count: 12
solver_velocity_iteration_count: 1
contact_offset: 0.005
rest_offset: 0.0
bounce_threshold_velocity: 0.2
friction_offset_threshold: 0.04
friction_correlation_distance: 0.025
enable_sleeping: True
enable_stabilization: True
max_depenetration_velocity: 1000.0
# GPU buffers
gpu_max_rigid_contact_count: 524288
gpu_max_rigid_patch_count: 33554432
gpu_found_lost_pairs_capacity: 524288
gpu_found_lost_aggregate_pairs_capacity: 262144
gpu_total_aggregate_pairs_capacity: 1048576
gpu_max_soft_body_contacts: 1048576
gpu_max_particle_contacts: 1048576
gpu_heap_capacity: 33554432
gpu_temp_buffer_capacity: 16777216
gpu_max_num_partitions: 8
franka:
# -1 to use default values
override_usd_defaults: False
enable_self_collisions: True
enable_gyroscopic_forces: True
# also in stage params
# per-actor
solver_position_iteration_count: 12
solver_velocity_iteration_count: 1
sleep_threshold: 0.005
stabilization_threshold: 0.001
# per-body
density: -1
max_depenetration_velocity: 1000.0
# per-shape
contact_offset: 0.005
rest_offset: 0.0
target_ball:
# -1 to use default values
override_usd_defaults: False
enable_self_collisions: False
enable_gyroscopic_forces: True
# also in stage params
# per-actor
solver_position_iteration_count: 12
solver_velocity_iteration_count: 1
sleep_threshold: 0.0
stabilization_threshold: 0.001
# per-body
density: -1
max_depenetration_velocity: 1000.0
# per-shape
contact_offset: 0.005
rest_offset: 0.0
| 2,904 | YAML | 24.707964 | 71 | 0.692837 |
zhehuazhou/ai-cps-robotics-benchmark/Gym_Envs/cfg/task/FrankaClothPlacing.yaml | # used to create the object
name: FrankaClothPlacing
physics_engine: ${..physics_engine}
# if given, will override the device setting in gym.
env:
numEnvs: ${resolve_default:4,${...num_envs}}
envSpacing: 3.0
episodeLength: 300
enableDebugVis: False
clipObservations: 5.0
clipActions: 1.0
controlFrequencyInv: 2 # 60 Hz
startPositionNoise: 0.0
startRotationNoise: 0.0
aggregateMode: 3
actionScale: 7.5
dofVelocityScale: 0.1
distRewardScale: 2.0
rotRewardScale: 0.5
aroundHandleRewardScale: 10.0
openRewardScale: 7.5
fingerDistRewardScale: 100.0
actionPenaltyScale: 0.01
fingerCloseRewardScale: 10.0
sim:
dt: 0.0083 # 1/120 s
use_gpu_pipeline: ${eq:${...pipeline},"gpu"}
gravity: [0.0, 0.0, -9.81]
add_ground_plane: True
use_flatcache: True
enable_scene_query_support: False
disable_contact_processing: False
# set to True if you use camera sensors in the environment
enable_cameras: False
default_physics_material:
static_friction: 1.0
dynamic_friction: 1.0
restitution: 0.0
physx:
worker_thread_count: ${....num_threads}
solver_type: ${....solver_type}
use_gpu: ${eq:${....sim_device},"gpu"} # set to False to run on CPU
solver_position_iteration_count: 12
solver_velocity_iteration_count: 1
contact_offset: 0.005
rest_offset: 0.0
bounce_threshold_velocity: 0.2
friction_offset_threshold: 0.04
friction_correlation_distance: 0.025
enable_sleeping: True
enable_stabilization: True
max_depenetration_velocity: 1000.0
# GPU buffers
gpu_max_rigid_contact_count: 524288
gpu_max_rigid_patch_count: 33554432
gpu_found_lost_pairs_capacity: 524288
gpu_found_lost_aggregate_pairs_capacity: 262144
gpu_total_aggregate_pairs_capacity: 1048576
gpu_max_soft_body_contacts: 1048576
gpu_max_particle_contacts: 1048576
gpu_heap_capacity: 33554432
gpu_temp_buffer_capacity: 16777216
gpu_max_num_partitions: 8
franka:
# -1 to use default values
override_usd_defaults: False
enable_self_collisions: True
enable_gyroscopic_forces: True
# also in stage params
# per-actor
solver_position_iteration_count: 12
solver_velocity_iteration_count: 1
sleep_threshold: 0.005
stabilization_threshold: 0.001
# per-body
density: -1
max_depenetration_velocity: 1000.0
# per-shape
contact_offset: 0.005
rest_offset: 0.0
cloth_bin:
# -1 to use default values
override_usd_defaults: False
enable_self_collisions: False
enable_gyroscopic_forces: True
# also in stage params
# per-actor
solver_position_iteration_count: 12
solver_velocity_iteration_count: 1
sleep_threshold: 0.0
stabilization_threshold: 0.001
# per-body
density: -1
max_depenetration_velocity: 1000.0
# per-shape
contact_offset: 0.005
rest_offset: 0.0
| 2,895 | YAML | 25.09009 | 71 | 0.693955 |
zhehuazhou/ai-cps-robotics-benchmark/Gym_Envs/cfg/task/FrankaBallPushing.yaml | # used to create the object
name: FrankaBallPushing
physics_engine: ${..physics_engine}
# if given, will override the device setting in gym.
env:
numEnvs: ${resolve_default:16,${...num_envs}}
envSpacing: 3.0
episodeLength: 300
enableDebugVis: False
clipObservations: 5.0
clipActions: 1.0
controlFrequencyInv: 2 # 60 Hz
startPositionNoise: 0.0
startRotationNoise: 0.0
aggregateMode: 3
actionScale: 7.5
dofVelocityScale: 0.01
distRewardScale: 2.0
rotRewardScale: 0.5
aroundHandleRewardScale: 10.0
openRewardScale: 7.5
fingerDistRewardScale: 100.0
actionPenaltyScale: 0.01
fingerCloseRewardScale: 10.0
ballRadius: 0.04
ballInitialPosition: [0.1, 0, 0.45]
ballInitialOrientation: [1, 0, 0, 0]
tableInitialPosition: [0, 0, 0]
tableInitialOrientation: [0, 0, 0, 0]
sim:
dt: 0.0083 # 1/120 s
use_gpu_pipeline: ${eq:${...pipeline},"gpu"}
gravity: [0.0, 0.0, -9.81]
add_ground_plane: True
use_flatcache: True
enable_scene_query_support: False
disable_contact_processing: False
# set to True if you use camera sensors in the environment
enable_cameras: False
default_physics_material:
static_friction: 1.0
dynamic_friction: 1.0
restitution: 0.0
physx:
worker_thread_count: ${....num_threads}
solver_type: ${....solver_type}
use_gpu: ${eq:${....sim_device},"gpu"} # set to False to run on CPU
solver_position_iteration_count: 12
solver_velocity_iteration_count: 1
contact_offset: 0.005
rest_offset: 0.0
bounce_threshold_velocity: 0.2
friction_offset_threshold: 0.04
friction_correlation_distance: 0.025
enable_sleeping: True
enable_stabilization: True
max_depenetration_velocity: 1000.0
# GPU buffers
gpu_max_rigid_contact_count: 724288
gpu_max_rigid_patch_count: 33554432
gpu_found_lost_pairs_capacity: 524288
gpu_found_lost_aggregate_pairs_capacity: 262144
gpu_total_aggregate_pairs_capacity: 1048576
gpu_max_soft_body_contacts: 1048576
gpu_max_particle_contacts: 1048576
gpu_heap_capacity: 33554432
gpu_temp_buffer_capacity: 16777216
gpu_max_num_partitions: 8
franka:
# -1 to use default values
override_usd_defaults: False
enable_self_collisions: True
enable_gyroscopic_forces: True
# also in stage params
# per-actor
solver_position_iteration_count: 12
solver_velocity_iteration_count: 1
sleep_threshold: 0.005
stabilization_threshold: 0.001
# per-body
density: -1
max_depenetration_velocity: 1000.0
# per-shape
contact_offset: 0.005
rest_offset: 0.0
table:
# -1 to use default values
override_usd_defaults: False
enable_self_collisions: False
enable_gyroscopic_forces: True
# also in stage params
# per-actor
solver_position_iteration_count: 12
solver_velocity_iteration_count: 1
sleep_threshold: 0.0
stabilization_threshold: 0.001
# per-body
density: -1
max_depenetration_velocity: 1000.0
# per-shape
contact_offset: 0.005
rest_offset: 0.0
ball:
# -1 to use default values
override_usd_defaults: False
fixed_base: False
enable_self_collisions: False
enable_gyroscopic_forces: True
# also in stage params
# per-actor
solver_position_iteration_count: 12
solver_velocity_iteration_count: 1
sleep_threshold: 0.0
stabilization_threshold: 0.001
# per-body
density: -1
max_depenetration_velocity: 1000.0
# per-shape
contact_offset: 0.005
rest_offset: 0.0
| 3,544 | YAML | 25.066176 | 71 | 0.688488 |
zhehuazhou/ai-cps-robotics-benchmark/Gym_Envs/cfg/train/FrankaDoorOpenPPO.yaml | params:
seed: ${...seed}
algo:
name: a2c_continuous
model:
name: continuous_a2c_logstd
network:
name: actor_critic
separate: False
space:
continuous:
mu_activation: None
sigma_activation: None
mu_init:
name: default
sigma_init:
name: const_initializer
val: 0
fixed_sigma: True
mlp:
units: [256, 128, 64]
activation: elu
d2rl: False
initializer:
name: default
regularizer:
name: None
load_checkpoint: ${if:${...checkpoint},True,False} # flag which sets whether to load the checkpoint
load_path: ${...checkpoint} # path to the checkpoint to load
config:
name: ${resolve_default:FrankaDoorOpen,${....experiment}}
full_experiment_name: ${.name}
env_name: rlgpu
device: ${....rl_device}
device_name: ${....rl_device}
ppo: True
mixed_precision: True
normalize_input: True
normalize_value: True
num_actors: ${....task.env.numEnvs}
reward_shaper:
scale_value: 0.01
normalize_advantage: True
gamma: 0.99
tau: 0.95
learning_rate: 5e-4
lr_schedule: adaptive
kl_threshold: 0.008
score_to_win: 10000000
max_epochs: ${resolve_default:100000,${....max_iterations}}
save_best_after: 100
save_frequency: 200
print_stats: True
grad_norm: 1.0
entropy_coef: 0.0
truncate_grads: True
e_clip: 0.2
horizon_length: 256
minibatch_size: 16384
mini_epochs: 8
critic_coef: 4
clip_value: True
seq_len: 4
bounds_loss_coef: 0.0001
| 1,604 | YAML | 21.605633 | 101 | 0.600374 |
zhehuazhou/ai-cps-robotics-benchmark/Gym_Envs/cfg/train/FrankaCubeStackingPPO.yaml | params:
seed: ${...seed}
algo:
name: a2c_continuous
model:
name: continuous_a2c_logstd
network:
name: actor_critic
separate: False
space:
continuous:
mu_activation: None
sigma_activation: None
mu_init:
name: default
sigma_init:
name: const_initializer
val: 0
fixed_sigma: True
mlp:
units: [256, 128, 64]
activation: elu
d2rl: False
initializer:
name: default
regularizer:
name: None
load_checkpoint: ${if:${...checkpoint},True,False} # flag which sets whether to load the checkpoint
load_path: ${...checkpoint} # path to the checkpoint to load
config:
name: ${resolve_default:FrankaCubeStacking,${....experiment}}
full_experiment_name: ${.name}
env_name: rlgpu
device: ${....rl_device}
device_name: ${....rl_device}
ppo: True
mixed_precision: True
normalize_input: True
normalize_value: True
num_actors: ${....task.env.numEnvs}
reward_shaper:
scale_value: 0.01
normalize_advantage: True
gamma: 0.99
tau: 0.95
learning_rate: 5e-4
lr_schedule: adaptive
kl_threshold: 0.008
score_to_win: 10000000
max_epochs: ${resolve_default:100000,${....max_iterations}}
save_best_after: 50
save_frequency: 200
print_stats: True
grad_norm: 1.0
entropy_coef: 0.0
truncate_grads: True
e_clip: 0.2
horizon_length: 16
minibatch_size: 16384
mini_epochs: 8
critic_coef: 4
clip_value: True
seq_len: 4
bounds_loss_coef: 0.0001
| 1,606 | YAML | 21.633803 | 101 | 0.600872 |
zhehuazhou/ai-cps-robotics-benchmark/Gym_Envs/cfg/train/FrankaPegInHolePPO.yaml | params:
seed: ${...seed}
algo:
name: a2c_continuous
model:
name: continuous_a2c_logstd
network:
name: actor_critic
separate: False
space:
continuous:
mu_activation: None
sigma_activation: None
mu_init:
name: default
sigma_init:
name: const_initializer
val: 0
fixed_sigma: True
mlp:
units: [256, 128, 64]
activation: elu
d2rl: False
initializer:
name: default
regularizer:
name: None
load_checkpoint: ${if:${...checkpoint},True,False} # flag which sets whether to load the checkpoint
load_path: ${...checkpoint} # path to the checkpoint to load
config:
name: ${resolve_default:FrankaPegInHole,${....experiment}}
full_experiment_name: ${.name}
env_name: rlgpu
device: ${....rl_device}
device_name: ${....rl_device}
ppo: True
mixed_precision: True
normalize_input: True
normalize_value: True
num_actors: ${....task.env.numEnvs}
reward_shaper:
scale_value: 0.01
normalize_advantage: True
gamma: 0.95
tau: 0.95
learning_rate: 1e-4
lr_schedule: adaptive
kl_threshold: 0.008
score_to_win: 10000000
max_epochs: ${resolve_default:100000,${....max_iterations}}
save_best_after: 100
save_frequency: 200
print_stats: True
grad_norm: 1.0
entropy_coef: 0.0
truncate_grads: True
e_clip: 0.2
horizon_length: 32
minibatch_size: 16384
mini_epochs: 8
critic_coef: 4
clip_value: True
seq_len: 4
bounds_loss_coef: 0.0001
| 1,604 | YAML | 21.605633 | 101 | 0.600374 |
zhehuazhou/ai-cps-robotics-benchmark/Gym_Envs/cfg/train/FrankaClothPlacingPPO.yaml | params:
seed: ${...seed}
algo:
name: a2c_continuous
model:
name: continuous_a2c_logstd
network:
name: actor_critic
separate: False
space:
continuous:
mu_activation: None
sigma_activation: None
mu_init:
name: default
sigma_init:
name: const_initializer
val: 0
fixed_sigma: True
mlp:
units: [256, 128, 64]
activation: elu
d2rl: False
initializer:
name: default
regularizer:
name: None
load_checkpoint: ${if:${...checkpoint},True,False} # flag which sets whether to load the checkpoint
load_path: ${...checkpoint} # path to the checkpoint to load
config:
name: ${resolve_default:FrankaClothPlacing,${....experiment}}
full_experiment_name: ${.name}
env_name: rlgpu
device: ${....rl_device}
device_name: ${....rl_device}
ppo: True
mixed_precision: True
normalize_input: True
normalize_value: True
num_actors: ${....task.env.numEnvs}
reward_shaper:
scale_value: 0.01
normalize_advantage: True
gamma: 0.99
tau: 0.95
learning_rate: 5e-4
lr_schedule: adaptive
kl_threshold: 0.008
score_to_win: 10000000
max_epochs: ${resolve_default:100000,${....max_iterations}}
save_best_after: 50
save_frequency: 200
print_stats: True
grad_norm: 1.0
entropy_coef: 0.0
truncate_grads: True
e_clip: 0.2
horizon_length: 32
minibatch_size: 2048
mini_epochs: 8
critic_coef: 4
clip_value: True
seq_len: 4
bounds_loss_coef: 0.0001
| 1,605 | YAML | 21.619718 | 101 | 0.600623 |
zhehuazhou/ai-cps-robotics-benchmark/Gym_Envs/cfg/train/FrankaPointReachingPPO.yaml | params:
seed: ${...seed}
algo:
name: a2c_continuous
model:
name: continuous_a2c_logstd
network:
name: actor_critic
separate: False
space:
continuous:
mu_activation: None
sigma_activation: None
mu_init:
name: default
sigma_init:
name: const_initializer
val: 0
fixed_sigma: True
mlp:
units: [256, 128, 64]
activation: elu
d2rl: False
initializer:
name: default
regularizer:
name: None
load_checkpoint: ${if:${...checkpoint},True,False} # flag which sets whether to load the checkpoint
load_path: ${...checkpoint} # path to the checkpoint to load
config:
name: ${resolve_default:FrankaCubeStacking,${....experiment}}
full_experiment_name: ${.name}
env_name: rlgpu
device: ${....rl_device}
device_name: ${....rl_device}
ppo: True
mixed_precision: True
normalize_input: True
normalize_value: True
num_actors: ${....task.env.numEnvs}
reward_shaper:
scale_value: 0.01
normalize_advantage: True
gamma: 0.99
tau: 0.95
learning_rate: 5e-4
lr_schedule: adaptive
kl_threshold: 0.008
score_to_win: 10000000
max_epochs: ${resolve_default:100000,${....max_iterations}}
save_best_after: 50
save_frequency: 200
print_stats: True
grad_norm: 1.0
entropy_coef: 0.0
truncate_grads: True
e_clip: 0.2
horizon_length: 16
minibatch_size: 16384
mini_epochs: 8
critic_coef: 4
clip_value: True
seq_len: 4
bounds_loss_coef: 0.0001
| 1,606 | YAML | 21.633803 | 101 | 0.600872 |
zhehuazhou/ai-cps-robotics-benchmark/Gym_Envs/cfg/train/FrankaBallPushingPPO.yaml | params:
seed: ${...seed}
algo:
name: a2c_continuous
model:
name: continuous_a2c_logstd
network:
name: actor_critic
separate: False
space:
continuous:
mu_activation: None
sigma_activation: None
mu_init:
name: default
sigma_init:
name: const_initializer
val: 0
fixed_sigma: True
mlp:
units: [256, 128, 64]
activation: elu
d2rl: False
initializer:
name: default
regularizer:
name: None
load_checkpoint: ${if:${...checkpoint},True,False} # flag which sets whether to load the checkpoint
load_path: ${...checkpoint} # path to the checkpoint to load
config:
name: ${resolve_default:FrankaBallPushing,${....experiment}}
full_experiment_name: ${.name}
env_name: rlgpu
device: ${....rl_device}
device_name: ${....rl_device}
ppo: True
mixed_precision: True
normalize_input: True
normalize_value: True
num_actors: ${....task.env.numEnvs}
reward_shaper:
scale_value: 0.01
normalize_advantage: True
gamma: 0.99
tau: 0.95
learning_rate: 5e-4
lr_schedule: adaptive
kl_threshold: 0.008
score_to_win: 10000000
max_epochs: ${resolve_default:10000,${....max_iterations}}
save_best_after: 50
save_frequency: 100
print_stats: True
grad_norm: 1.0
entropy_coef: 0.0
truncate_grads: True
e_clip: 0.2
horizon_length: 32
minibatch_size: 32768
mini_epochs: 8
critic_coef: 4
clip_value: True
seq_len: 4
bounds_loss_coef: 0.0001
| 1,604 | YAML | 21.605633 | 101 | 0.600374 |
zhehuazhou/ai-cps-robotics-benchmark/Gym_Envs/cfg/train/FrankaBallCatchingPPO.yaml | params:
seed: ${...seed}
algo:
name: a2c_continuous
model:
name: continuous_a2c_logstd
network:
name: actor_critic
separate: False
space:
continuous:
mu_activation: None
sigma_activation: None
mu_init:
name: default
sigma_init:
name: const_initializer
val: 0
fixed_sigma: True
mlp:
units: [256, 128, 64]
activation: elu
d2rl: False
initializer:
name: default
regularizer:
name: None
load_checkpoint: ${if:${...checkpoint},True,False} # flag which sets whether to load the checkpoint
load_path: ${...checkpoint} # path to the checkpoint to load
config:
name: ${resolve_default:FrankaBallCatching,${....experiment}}
full_experiment_name: ${.name}
env_name: rlgpu
device: ${....rl_device}
device_name: ${....rl_device}
ppo: True
mixed_precision: True
normalize_input: True
normalize_value: True
num_actors: ${....task.env.numEnvs}
reward_shaper:
scale_value: 0.01
normalize_advantage: True
gamma: 0.99
tau: 0.95
learning_rate: 5e-4
lr_schedule: adaptive
kl_threshold: 0.008
score_to_win: 10000000
max_epochs: ${resolve_default:100000,${....max_iterations}}
save_best_after: 50
save_frequency: 200
print_stats: True
grad_norm: 1.0
entropy_coef: 0.0
truncate_grads: True
e_clip: 0.2
horizon_length: 16
minibatch_size: 16384
mini_epochs: 8
critic_coef: 4
clip_value: True
seq_len: 4
bounds_loss_coef: 0.0001
| 1,606 | YAML | 21.633803 | 101 | 0.600872 |
zhehuazhou/ai-cps-robotics-benchmark/Gym_Envs/cfg/train/FrankaBallBalancingPPO.yaml | params:
seed: ${...seed}
algo:
name: a2c_continuous
model:
name: continuous_a2c_logstd
network:
name: actor_critic
separate: False
space:
continuous:
mu_activation: None
sigma_activation: None
mu_init:
name: default
sigma_init:
name: const_initializer
val: 0
fixed_sigma: True
mlp:
units: [128, 64, 32]
activation: elu
d2rl: False
initializer:
name: default
regularizer:
name: None
load_checkpoint: ${if:${...checkpoint},True,False} # flag which sets whether to load the checkpoint
load_path: ${...checkpoint} # path to the checkpoint to load
config:
name: ${resolve_default:FrankaBallBalancing,${....experiment}}
full_experiment_name: ${.name}
env_name: rlgpu
device: ${....rl_device}
device_name: ${....rl_device}
ppo: True
mixed_precision: True
normalize_input: True
normalize_value: True
num_actors: ${....task.env.numEnvs}
reward_shaper:
scale_value: 0.01
normalize_advantage: True
gamma: 0.99
tau: 0.95
learning_rate: 5e-4
lr_schedule: adaptive
kl_threshold: 0.008
score_to_win: 10000000
max_epochs: ${resolve_default:100000,${....max_iterations}}
save_best_after: 50
save_frequency: 200
print_stats: True
grad_norm: 1.0
entropy_coef: 0.0
truncate_grads: True
e_clip: 0.2
horizon_length: 32
minibatch_size: 16384
mini_epochs: 8
critic_coef: 4
clip_value: True
seq_len: 4
bounds_loss_coef: 0.0001
| 1,606 | YAML | 21.633803 | 101 | 0.600872 |
zhehuazhou/ai-cps-robotics-benchmark/Gym_Envs/Tasks/Franka_Ball_Catching.py | from omniisaacgymenvs.tasks.base.rl_task import RLTask
from Models.Franka.Franka import Franka
from Models.Franka.Franka_view import FrankaView
from Models.ball_catching.tool import Tool
from omni.isaac.core.objects import DynamicSphere
from omni.isaac.core.prims import RigidPrim, RigidPrimView
from omni.isaac.core.utils.prims import get_prim_at_path
from omni.isaac.core.utils.stage import get_current_stage, add_reference_to_stage
from omni.isaac.core.utils.torch.transformations import *
from omni.isaac.core.utils.torch.rotations import *
from omni.isaac.cloner import Cloner
import numpy as np
import torch
import math
from pxr import Gf, Usd, UsdGeom
class FrankaBallCatchingTask(RLTask):
def __init__(
self,
name,
sim_config,
env,
offset=None
) -> None:
self._sim_config = sim_config
self._cfg = sim_config.config
self._task_cfg = sim_config.task_config
self._num_envs = self._task_cfg["env"]["numEnvs"]
self._env_spacing = self._task_cfg["env"]["envSpacing"]
self._max_episode_length = self._task_cfg["env"]["episodeLength"]
self.action_scale = self._task_cfg["env"]["actionScale"]
self.start_position_noise = self._task_cfg["env"]["startPositionNoise"]
self.start_rotation_noise = self._task_cfg["env"]["startRotationNoise"]
self.dof_vel_scale = self._task_cfg["env"]["dofVelocityScale"]
self.dist_reward_scale = self._task_cfg["env"]["distRewardScale"]
self.rot_reward_scale = self._task_cfg["env"]["rotRewardScale"]
self.around_handle_reward_scale = self._task_cfg["env"]["aroundHandleRewardScale"]
self.open_reward_scale = self._task_cfg["env"]["openRewardScale"]
self.finger_dist_reward_scale = self._task_cfg["env"]["fingerDistRewardScale"]
self.action_penalty_scale = self._task_cfg["env"]["actionPenaltyScale"]
self.finger_close_reward_scale = self._task_cfg["env"]["fingerCloseRewardScale"]
self.distX_offset = 0.04
self.dt = 1/60.
self._num_observations = 27
self._num_actions = 9
# Flag for testing
self.is_test = False
self.initial_test_value = None
self.is_action_noise = False
RLTask.__init__(self, name, env)
# Extra info for TensorBoard
self.extras = {}
torch_zeros = lambda: torch.zeros(self.num_envs, dtype=torch.float, device=self.device, requires_grad=False)
self.episode_sums = {"ball_vel": torch_zeros(), "ball_tool_dist": torch_zeros()}
return
def set_up_scene(self, scene) -> None:
# Franka
franka_translation = torch.tensor([0.3, 0.0, 0.0])
self.get_franka(franka_translation)
self.get_tool()
self.get_ball()
# Here the env is cloned (cannot clone particle systems right now)
super().set_up_scene(scene)
# Add Franka
self._frankas = FrankaView(prim_paths_expr="/World/envs/.*/franka", name="franka_view")
# Add tool
self._tool = RigidPrimView(prim_paths_expr="/World/envs/.*/tool/tool/tool_mesh", name="tool_view", reset_xform_properties=False)
self._tool_center = RigidPrimView(prim_paths_expr="/World/envs/.*/tool/tool/center_cube", name="tool_center_view", reset_xform_properties=False)
# Add ball
self._ball = RigidPrimView(prim_paths_expr="/World/envs/.*/ball", name="ball_view", reset_xform_properties=False)
scene.add(self._frankas)
scene.add(self._frankas._hands)
scene.add(self._frankas._lfingers)
scene.add(self._frankas._rfingers)
scene.add(self._tool)
scene.add(self._tool_center)
scene.add(self._ball)
self.init_data()
return
def get_franka(self, translation):
franka = Franka(prim_path=self.default_zero_env_path + "/franka", name="franka", translation = translation)
self._sim_config.apply_articulation_settings("franka", get_prim_at_path(franka.prim_path), self._sim_config.parse_actor_config("franka"))
def get_tool(self):
tool = Tool(prim_path=self.default_zero_env_path + "/tool", name="tool")
self._sim_config.apply_articulation_settings("tool", get_prim_at_path(tool.prim_path), self._sim_config.parse_actor_config("tool"))
def get_ball(self):
ball = DynamicSphere(
name = 'ball',
position=[-0.8,0,1.5],
orientation=[1,0,0,0],
prim_path=self.default_zero_env_path + "/ball",
radius=0.01,
color=np.array([1, 0, 0]),
density = 100,
mass = 0.001
)
# Set as testing mode
def set_as_test(self):
self.is_test = True
# Set action noise
def set_action_noise(self):
self.is_action_noise = True
# Set initial test values for testing mode
def set_initial_test_value(self, value):
# for ball pushing: initial x,y positions of the ball
self.initial_test_value = value
def init_data(self) -> None:
def get_env_local_pose(env_pos, xformable, device):
"""Compute pose in env-local coordinates"""
world_transform = xformable.ComputeLocalToWorldTransform(0)
world_pos = world_transform.ExtractTranslation()
world_quat = world_transform.ExtractRotationQuat()
px = world_pos[0] - env_pos[0]
py = world_pos[1] - env_pos[1]
pz = world_pos[2] - env_pos[2]
qx = world_quat.imaginary[0]
qy = world_quat.imaginary[1]
qz = world_quat.imaginary[2]
qw = world_quat.real
return torch.tensor([px, py, pz, qw, qx, qy, qz], device=device, dtype=torch.float)
stage = get_current_stage()
hand_pose = get_env_local_pose(self._env_pos[0], UsdGeom.Xformable(stage.GetPrimAtPath("/World/envs/env_0/franka/panda_link7")), self._device)
lfinger_pose = get_env_local_pose(
self._env_pos[0], UsdGeom.Xformable(stage.GetPrimAtPath("/World/envs/env_0/franka/panda_leftfinger")), self._device
)
rfinger_pose = get_env_local_pose(
self._env_pos[0], UsdGeom.Xformable(stage.GetPrimAtPath("/World/envs/env_0/franka/panda_rightfinger")), self._device
)
# finger pos
finger_pose = torch.zeros(7, device=self._device)
finger_pose[0:3] = (lfinger_pose[0:3] + rfinger_pose[0:3]) / 2.0
finger_pose[3:7] = lfinger_pose[3:7]
hand_pose_inv_rot, hand_pose_inv_pos = (tf_inverse(hand_pose[3:7], hand_pose[0:3]))
# franka grasp local pose
grasp_pose_axis = 1
franka_local_grasp_pose_rot, franka_local_pose_pos = tf_combine(hand_pose_inv_rot, hand_pose_inv_pos, finger_pose[3:7], finger_pose[0:3])
franka_local_pose_pos += torch.tensor([0, 0.04, 0], device=self._device)
self.franka_local_grasp_pos = franka_local_pose_pos.repeat((self._num_envs, 1))
self.franka_local_grasp_rot = franka_local_grasp_pose_rot.repeat((self._num_envs, 1))
self.gripper_forward_axis = torch.tensor([0, 0, 1], device=self._device, dtype=torch.float).repeat((self._num_envs, 1))
self.gripper_up_axis = torch.tensor([0, 1, 0], device=self._device, dtype=torch.float).repeat((self._num_envs, 1))
self.franka_default_dof_pos = torch.tensor(
[0.0, -0.872, 0.0, -2.0, 0.0, 2.618, 0.785, 0.04, 0.04], device=self._device
)
self.actions = torch.zeros((self._num_envs, self.num_actions), device=self._device)
def get_observations(self) -> dict:
# Franka
hand_pos, hand_rot = self._frankas._hands.get_world_poses(clone=False)
franka_dof_pos = self._frankas.get_joint_positions(clone=False)
franka_dof_vel = self._frankas.get_joint_velocities(clone=False)
self.franka_dof_pos = franka_dof_pos
self.franka_lfinger_pos, self.franka_lfinger_rot = self._frankas._lfingers.get_world_poses(clone=False)
self.franka_rfinger_pos, self.franka_rfinger_rot = self._frankas._lfingers.get_world_poses(clone=False)
dof_pos_scaled = (
2.0
* (franka_dof_pos - self.franka_dof_lower_limits)
/ (self.franka_dof_upper_limits - self.franka_dof_lower_limits)
- 1.0
)
# ball
self.ball_pos, self.ball_rot = self._ball.get_world_poses(clone=False)
ball_vel = self._ball.get_velocities() # ball velocity
ball_linvels = ball_vel[:, 0:3] # ball linear velocity
# tool
tool_pos, tool_rot = self._tool_center.get_world_poses(clone=False) # tool position
to_target = tool_pos - self.ball_pos # ball to tool dist
self.obs_buf = torch.cat(
(
dof_pos_scaled,
franka_dof_vel * self.dof_vel_scale,
self.ball_pos,
to_target,
ball_linvels,
),
dim=-1,
)
observations = {
self._frankas.name: {
"obs_buf": self.obs_buf
}
}
return observations
def pre_physics_step(self, actions) -> None:
if not self._env._world.is_playing():
return
reset_env_ids = self.reset_buf.nonzero(as_tuple=False).squeeze(-1)
if len(reset_env_ids) > 0:
self.reset_idx(reset_env_ids)
self.actions = actions.clone().to(self._device)
# if action noise
if self.is_action_noise is True:
# Gaussian white noise with 0.01 variance
self.actions = self.actions + (0.5)*torch.randn_like(self.actions)
targets = self.franka_dof_targets + self.franka_dof_speed_scales * self.dt * self.actions * self.action_scale
self.franka_dof_targets[:] = tensor_clamp(targets, self.franka_dof_lower_limits, self.franka_dof_upper_limits)
env_ids_int32 = torch.arange(self._frankas.count, dtype=torch.int32, device=self._device)
# fix the finger movement so that the tool will always be grasped in hand
self.franka_dof_targets[:,7] = self.franka_default_dof_pos[7]
self.franka_dof_targets[:,8] = self.franka_default_dof_pos[8]
self._frankas.set_joint_position_targets(self.franka_dof_targets, indices=env_ids_int32)
def reset_idx(self, env_ids):
indices = env_ids.to(dtype=torch.int32)
num_indices = len(indices)
# reset franka
pos = torch.clamp(
self.franka_default_dof_pos.unsqueeze(0),
#+ 0.25 * (torch.rand((len(env_ids), self.num_franka_dofs), device=self._device) - 0.5),
self.franka_dof_lower_limits,
self.franka_dof_upper_limits,
)
dof_pos = torch.zeros((num_indices, self._frankas.num_dof), device=self._device)
dof_vel = torch.zeros((num_indices, self._frankas.num_dof), device=self._device)
dof_pos[:, :] = pos
self.franka_dof_targets[env_ids, :] = pos
self.franka_dof_pos[env_ids, :] = pos
self._frankas.set_joint_position_targets(self.franka_dof_targets[env_ids], indices=indices)
self._frankas.set_joint_positions(dof_pos, indices=indices)
self._frankas.set_joint_velocities(dof_vel, indices=indices)
# reset tool
self._tool.set_world_poses(self.default_tool_pos[env_ids], self.default_tool_rot[env_ids], indices = indices)
self._tool.set_velocities(self.default_tool_velocity[env_ids], indices = indices)
if not self.is_test:
# reset ball position within an area: x [-0.1, 0.1], y [-0.1,0.1]
self.new_ball_pos = self.default_ball_pos.clone().detach()
self.new_ball_pos[:,0] = self.default_ball_pos[:,0] + (0.05 + 0.05) * torch.rand(self._num_envs, device=self._device) -0.05
self.new_ball_pos[:,1] = self.default_ball_pos[:,1] + (0.05 + 0.05) * torch.rand(self._num_envs, device=self._device) -0.05
# reset ball velocity: default_ball_vel = [2.2 0.1, 0.0]
# x-axis vel: [1.0, 1.5]
# y-axis vel: [0.0, 0.2]
self.new_ball_vel = self.default_ball_velocity.clone().detach()
self.new_ball_vel[:,0] = (1.0 - 1.0) * torch.rand(self._num_envs, device=self._device) + 1.0
self.new_ball_vel[:,1] = (0.0 - 0.0) * torch.rand(self._num_envs, device=self._device) + 0.0
# reset ball
self._ball.set_world_poses(self.new_ball_pos[env_ids], self.default_ball_rot[env_ids], indices = indices)
self._ball.set_velocities(self.new_ball_vel[env_ids], indices = indices)
else:
self.new_ball_pos = self.default_ball_pos.clone().detach()
self.new_ball_pos[:,0] = self.default_ball_pos[:,0] + self.initial_test_value[0]
self.new_ball_pos[:,1] = self.default_ball_pos[:,1] + self.initial_test_value[1]
self.new_ball_vel = self.default_ball_velocity.clone().detach()
self.new_ball_vel[:,0] = self.initial_test_value[2]
self.new_ball_vel[:,1] = self.initial_test_value[3]
# reset ball
self._ball.set_world_poses(self.new_ball_pos[env_ids], self.default_ball_rot[env_ids], indices = indices)
self._ball.set_velocities(self.new_ball_vel[env_ids], indices = indices)
# bookkeeping
self.reset_buf[env_ids] = 0
self.progress_buf[env_ids] = 0
def post_reset(self):
# Franka
self.num_franka_dofs = self._frankas.num_dof
self.franka_dof_pos = torch.zeros((self.num_envs, self.num_franka_dofs), device=self._device)
dof_limits = self._frankas.get_dof_limits()
self.franka_dof_lower_limits = dof_limits[0, :, 0].to(device=self._device)
self.franka_dof_upper_limits = dof_limits[0, :, 1].to(device=self._device)
self.franka_dof_speed_scales = torch.ones_like(self.franka_dof_lower_limits)
self.franka_dof_speed_scales[self._frankas.gripper_indices] = 0.1
self.franka_dof_targets = torch.zeros(
(self._num_envs, self.num_franka_dofs), dtype=torch.float, device=self._device
)
# tool
self.default_tool_pos, self.default_tool_rot = self._tool.get_world_poses()
self.default_tool_velocity = self._tool.get_velocities()
# ball
self.default_ball_pos, self.default_ball_rot = self._ball.get_world_poses()
self.default_ball_velocity = self._ball.get_velocities()
# change default velocities
self.default_ball_velocity[:,0] = 2.2
self.default_ball_velocity[:,1] = 0.1
self.default_ball_velocity[:,2] = 0.0
self._ball.set_velocities(self.default_ball_velocity)
# randomize all envs
indices = torch.arange(self._num_envs, dtype=torch.int64, device=self._device)
self.reset_idx(indices)
def calculate_metrics(self) -> None:
# variables for reward
ball_pos = self.ball_pos # ball pos
ball_vel = self._ball.get_velocities() # ball velocity
tool_pos, tool_rot = self._tool_center.get_world_poses() # tool center pos and rot
ball_linvels = ball_vel[:, 0:3] # ball linear velocity
# 1st reward ball to tool center distance
ball_center_dist = torch.norm(tool_pos - ball_pos, p=2, dim=-1)
ball_center_XY_dist = torch.norm(tool_pos[:,0:3] - ball_pos[:,0:3], p=2, dim=-1)
center_dist_reward = 1.0/(1.0+ball_center_dist*100)
# 2nd reward: ball is unmoved
norm_ball_linvel = torch.norm(ball_linvels, p=2, dim=-1)
ball_vel_reward = 1.0/(1.0+norm_ball_linvel*100)
# 3rd reward: rotation not too much
rot_diff = torch.norm(tool_rot - self.default_tool_rot, p=2, dim=-1)
tool_rot_reward = 1.0/(1.0+rot_diff)
# action penalty
action_penalty = torch.sum(self.actions[:,0:7] ** 2, dim=-1)
action_penalty = 1 - 1.0 / (1.0 + action_penalty)
# liveness_reward
liveness_reward = torch.where(ball_center_XY_dist<0.03, torch.ones_like(center_dist_reward), torch.zeros_like(center_dist_reward))
# final cumulative reward
final_reward = 1.0*center_dist_reward + 1.0*ball_vel_reward + 0.0*tool_rot_reward + 0.5*liveness_reward - 0.01*action_penalty
self.rew_buf[:] = final_reward
# log additional info
self.episode_sums["ball_vel"] += norm_ball_linvel
self.episode_sums["ball_tool_dist"] += ball_center_dist
def is_done(self) -> None:
if not self.is_test:
ball_pos = self.ball_pos # ball pos
tool_pos, tool_rot = self._tool_center.get_world_poses() # tool center pos and rot
ball_center_dist = torch.norm(tool_pos - ball_pos, p=2, dim=-1)
# 1st reset: if ball falls from tool
# self.reset_buf = torch.where(ball_center_dist > 5.0, torch.ones_like(self.reset_buf), self.reset_buf)
# 2nd reset: if ball falls to the ground
self.reset_buf = torch.where(self.ball_pos[:,2] < 0.02, torch.ones_like(self.reset_buf), self.reset_buf)
# 3rd reset if max length reached
self.reset_buf = torch.where(self.progress_buf >= self._max_episode_length - 1, torch.ones_like(self.reset_buf), self.reset_buf)
else:
self.reset_buf = torch.where(self.progress_buf >= self._max_episode_length - 1, torch.ones_like(self.reset_buf), self.reset_buf)
| 17,673 | Python | 41.898058 | 152 | 0.606971 |
zhehuazhou/ai-cps-robotics-benchmark/Gym_Envs/Tasks/Franka_Ball_Balancing.py | from omniisaacgymenvs.tasks.base.rl_task import RLTask
from Models.Franka.Franka import Franka
from Models.Franka.Franka_view import FrankaView
from Models.ball_balancing.tool import Tool
from omni.isaac.core.objects import DynamicSphere
from omni.isaac.core.prims import RigidPrim, RigidPrimView
from omni.isaac.core.utils.prims import get_prim_at_path
from omni.isaac.core.utils.stage import get_current_stage
from omni.isaac.core.utils.torch.transformations import *
from omni.isaac.core.utils.torch.rotations import *
from omni.isaac.cloner import Cloner
import numpy as np
import torch
import math
from pxr import Usd, UsdGeom
class FrankaBallBalancingTask(RLTask):
def __init__(
self,
name,
sim_config,
env,
offset=None
) -> None:
self._sim_config = sim_config
self._cfg = sim_config.config
self._task_cfg = sim_config.task_config
self._num_envs = self._task_cfg["env"]["numEnvs"]
self._env_spacing = self._task_cfg["env"]["envSpacing"]
self._max_episode_length = self._task_cfg["env"]["episodeLength"]
self.action_scale = self._task_cfg["env"]["actionScale"]
self.start_position_noise = self._task_cfg["env"]["startPositionNoise"]
self.start_rotation_noise = self._task_cfg["env"]["startRotationNoise"]
self.dof_vel_scale = self._task_cfg["env"]["dofVelocityScale"]
self.dist_reward_scale = self._task_cfg["env"]["distRewardScale"]
self.rot_reward_scale = self._task_cfg["env"]["rotRewardScale"]
self.around_handle_reward_scale = self._task_cfg["env"]["aroundHandleRewardScale"]
self.open_reward_scale = self._task_cfg["env"]["openRewardScale"]
self.finger_dist_reward_scale = self._task_cfg["env"]["fingerDistRewardScale"]
self.action_penalty_scale = self._task_cfg["env"]["actionPenaltyScale"]
self.finger_close_reward_scale = self._task_cfg["env"]["fingerCloseRewardScale"]
self.ball_radius = self._task_cfg["env"]["ballRadius"]
self.ball_initial_position = self._task_cfg["env"]["ballInitialPosition"]
self.ball_initial_orientation = self._task_cfg["env"]["ballInitialOrientation"]
self.distX_offset = 0.04
self.dt = 1/60.
self._num_observations = 27
self._num_actions = 9
# Flag for testing
self.is_test = False
self.initial_test_value = None
self.is_action_noise = False
RLTask.__init__(self, name, env)
# Extra info for TensorBoard
self.extras = {}
torch_zeros = lambda: torch.zeros(self.num_envs, dtype=torch.float, device=self.device, requires_grad=False)
self.episode_sums = {"final_reward": torch_zeros(),}
return
def set_up_scene(self, scene) -> None:
franka_translation = torch.tensor([0.35, 0.0, 0.0])
self.get_franka(franka_translation)
self.get_tool()
self.get_ball()
super().set_up_scene(scene)
# Add Franka
self._frankas = FrankaView(prim_paths_expr="/World/envs/.*/franka", name="franka_view")
# Add Tool
self._tool = RigidPrimView(prim_paths_expr="/World/envs/.*/tool/tool/tool", name="tool_view", reset_xform_properties=False)
# Add ball
self._ball = RigidPrimView(prim_paths_expr="/World/envs/.*/ball", name="ball_view", reset_xform_properties=False)
scene.add(self._frankas)
scene.add(self._frankas._hands)
scene.add(self._frankas._lfingers)
scene.add(self._frankas._rfingers)
scene.add(self._ball)
scene.add(self._tool)
self.init_data()
return
def get_franka(self, translation):
franka = Franka(prim_path=self.default_zero_env_path + "/franka", name="franka", translation = translation)
self._sim_config.apply_articulation_settings("franka", get_prim_at_path(franka.prim_path), self._sim_config.parse_actor_config("franka"))
def get_tool(self):
tool = Tool(prim_path=self.default_zero_env_path + "/tool", name="tool")
self._sim_config.apply_articulation_settings("tool", get_prim_at_path(tool.prim_path), self._sim_config.parse_actor_config("tool"))
def get_ball(self):
ball = DynamicSphere(
name = 'ball',
position=self.ball_initial_position,
orientation=self.ball_initial_orientation,
prim_path=self.default_zero_env_path + "/ball",
radius=self.ball_radius,
color=np.array([1, 0, 0]),
density = 100,
mass = 0.15
)
self._sim_config.apply_articulation_settings("ball", get_prim_at_path(ball.prim_path), self._sim_config.parse_actor_config("ball"))
# Set as testing mode
def set_as_test(self):
self.is_test = True
# Set action noise
def set_action_noise(self):
self.is_action_noise = True
# Set initial test values for testing mode
def set_initial_test_value(self, value):
# for ball pushing: initial x,y positions of the ball
self.initial_test_value = value
def init_data(self) -> None:
def get_env_local_pose(env_pos, xformable, device):
"""Compute pose in env-local coordinates"""
world_transform = xformable.ComputeLocalToWorldTransform(0)
world_pos = world_transform.ExtractTranslation()
world_quat = world_transform.ExtractRotationQuat()
px = world_pos[0] - env_pos[0]
py = world_pos[1] - env_pos[1]
pz = world_pos[2] - env_pos[2]
qx = world_quat.imaginary[0]
qy = world_quat.imaginary[1]
qz = world_quat.imaginary[2]
qw = world_quat.real
return torch.tensor([px, py, pz, qw, qx, qy, qz], device=device, dtype=torch.float)
stage = get_current_stage()
hand_pose = get_env_local_pose(self._env_pos[0], UsdGeom.Xformable(stage.GetPrimAtPath("/World/envs/env_0/franka/panda_link7")), self._device)
lfinger_pose = get_env_local_pose(
self._env_pos[0], UsdGeom.Xformable(stage.GetPrimAtPath("/World/envs/env_0/franka/panda_leftfinger")), self._device
)
rfinger_pose = get_env_local_pose(
self._env_pos[0], UsdGeom.Xformable(stage.GetPrimAtPath("/World/envs/env_0/franka/panda_rightfinger")), self._device
)
finger_pose = torch.zeros(7, device=self._device)
finger_pose[0:3] = (lfinger_pose[0:3] + rfinger_pose[0:3]) / 2.0
finger_pose[3:7] = lfinger_pose[3:7]
hand_pose_inv_rot, hand_pose_inv_pos = (tf_inverse(hand_pose[3:7], hand_pose[0:3]))
grasp_pose_axis = 1
franka_local_grasp_pose_rot, franka_local_pose_pos = tf_combine(hand_pose_inv_rot, hand_pose_inv_pos, finger_pose[3:7], finger_pose[0:3])
franka_local_pose_pos += torch.tensor([0, 0.04, 0], device=self._device)
self.franka_local_grasp_pos = franka_local_pose_pos.repeat((self._num_envs, 1))
self.franka_local_grasp_rot = franka_local_grasp_pose_rot.repeat((self._num_envs, 1))
self.gripper_forward_axis = torch.tensor([0, 0, 1], device=self._device, dtype=torch.float).repeat((self._num_envs, 1))
self.gripper_up_axis = torch.tensor([0, 1, 0], device=self._device, dtype=torch.float).repeat((self._num_envs, 1))
# default franka pos: for initially grap the tool
self.franka_default_dof_pos = torch.tensor(
[0.0, -0.872, 0.0, -2.0, 0.0, 2.618, 0.785, 0.004, 0.004], device=self._device
)
self.actions = torch.zeros((self._num_envs, self.num_actions), device=self._device)
def get_observations(self) -> dict:
# Franka
hand_pos, hand_rot = self._frankas._hands.get_world_poses(clone=False)
franka_dof_pos = self._frankas.get_joint_positions(clone=False)
franka_dof_vel = self._frankas.get_joint_velocities(clone=False)
self.franka_dof_pos = franka_dof_pos
self.franka_lfinger_pos, self.franka_lfinger_rot = self._frankas._lfingers.get_world_poses(clone=False)
self.franka_rfinger_pos, self.franka_rfinger_rot = self._frankas._lfingers.get_world_poses(clone=False)
# Ball
self.ball_pos, self.ball_rot = self._ball.get_world_poses(clone=False)
ball_vel = self._ball.get_velocities() # ball velocity
ball_linvels = ball_vel[:, 0:3] # ball linear velocity
tool_pos, tool_rot = self._tool.get_world_poses(clone=False)
to_target = tool_pos - self.ball_pos
dof_pos_scaled = (
2.0
* (franka_dof_pos - self.franka_dof_lower_limits)
/ (self.franka_dof_upper_limits - self.franka_dof_lower_limits)
- 1.0
)
self.obs_buf = torch.cat(
(
dof_pos_scaled,
franka_dof_vel * self.dof_vel_scale,
self.ball_pos,
to_target,
ball_linvels,
# self.location_ball_pos
# self.cabinet_dof_pos[:, 3].unsqueeze(-1),
# self.cabinet_dof_vel[:, 3].unsqueeze(-1),
),
dim=-1,
)
observations = {
self._frankas.name: {
"obs_buf": self.obs_buf
}
}
return observations
def pre_physics_step(self, actions) -> None:
if not self._env._world.is_playing():
return
reset_env_ids = self.reset_buf.nonzero(as_tuple=False).squeeze(-1)
if len(reset_env_ids) > 0:
self.reset_idx(reset_env_ids)
self.actions = actions.clone().to(self._device)
# if action noise
if self.is_action_noise is True:
# Gaussian white noise with 0.01 variance
self.actions = self.actions + (0.5)*torch.randn_like(self.actions)
targets = self.franka_dof_targets + self.franka_dof_speed_scales * self.dt * self.actions * self.action_scale
self.franka_dof_targets[:] = tensor_clamp(targets, self.franka_dof_lower_limits, self.franka_dof_upper_limits)
env_ids_int32 = torch.arange(self._frankas.count, dtype=torch.int32, device=self._device)
# NOTE HERE: right now I fix the finger movement so that the tool will always be grasped in hand
self.franka_dof_targets[:,7] = self.franka_default_dof_pos[7]
self.franka_dof_targets[:,8] = self.franka_default_dof_pos[8]
self._frankas.set_joint_position_targets(self.franka_dof_targets, indices=env_ids_int32)
def reset_idx(self, env_ids):
indices = env_ids.to(dtype=torch.int32)
num_indices = len(indices)
# reset franka (due to initial grasping, cannot randomize)
pos = torch.clamp(
self.franka_default_dof_pos.unsqueeze(0),
# + 0.25 * (torch.rand((len(env_ids), self.num_franka_dofs), device=self._device) - 0.5),
self.franka_dof_lower_limits,
self.franka_dof_upper_limits,
)
dof_pos = torch.zeros((num_indices, self._frankas.num_dof), device=self._device)
dof_vel = torch.zeros((num_indices, self._frankas.num_dof), device=self._device)
dof_pos[:, :] = pos
self.franka_dof_targets[env_ids, :] = pos
self.franka_dof_pos[env_ids, :] = pos
self._frankas.set_joint_position_targets(self.franka_dof_targets[env_ids], indices=indices)
self._frankas.set_joint_positions(dof_pos, indices=indices)
self._frankas.set_joint_velocities(dof_vel, indices=indices)
# reset tool
self._tool.set_world_poses(self.default_tool_pos[env_ids], self.default_tool_rot[env_ids], indices = indices)
self._tool.set_velocities(self.default_tool_velocity[env_ids], indices = indices)
# reset ball position within an area: x [-0.15, 0.15], y [-0.15,0.15]
# if not test, randomize ball initial positions for training
if not self.is_test:
self.new_ball_pos = self.default_ball_pos.clone().detach()
self.new_ball_pos[:,0] = self.default_ball_pos[:,0] + (0.15 + 0.15) * torch.rand(self._num_envs, device=self._device) -0.15
self.new_ball_pos[:,1] = self.default_ball_pos[:,1] + (0.15 + 0.15) * torch.rand(self._num_envs, device=self._device) -0.15
self._ball.set_world_poses(self.new_ball_pos[env_ids], self.default_ball_rot[env_ids], indices = indices)
self._ball.set_velocities(self.default_ball_velocity[env_ids], indices = indices)
# if is test mode, set the ball to given position (1 environment)
else:
self.new_ball_pos = self.default_ball_pos.clone().detach()
self.new_ball_pos[:,0] = self.default_ball_pos[:,0] + self.initial_test_value[0]
self.new_ball_pos[:,1] = self.default_ball_pos[:,1] + self.initial_test_value[1]
self._ball.set_world_poses(self.new_ball_pos[env_ids], self.default_ball_rot[env_ids], indices = indices)
self._ball.set_velocities(self.default_ball_velocity[env_ids], indices = indices)
# bookkeeping
self.reset_buf[env_ids] = 0
self.progress_buf[env_ids] = 0
def post_reset(self):
# Franka
self.num_franka_dofs = self._frankas.num_dof
self.franka_dof_pos = torch.zeros((self.num_envs, self.num_franka_dofs), device=self._device)
dof_limits = self._frankas.get_dof_limits()
self.franka_dof_lower_limits = dof_limits[0, :, 0].to(device=self._device)
self.franka_dof_upper_limits = dof_limits[0, :, 1].to(device=self._device)
self.franka_dof_speed_scales = torch.ones_like(self.franka_dof_lower_limits)
self.franka_dof_speed_scales[self._frankas.gripper_indices] = 0.1
self.franka_dof_targets = torch.zeros(
(self._num_envs, self.num_franka_dofs), dtype=torch.float, device=self._device
)
# tool
self.default_tool_pos, self.default_tool_rot = self._tool.get_world_poses()
self.default_tool_velocity = self._tool.get_velocities()
# ball
self.default_ball_pos, self.default_ball_rot = self._ball.get_world_poses()
self.default_ball_velocity = self._ball.get_velocities()
# randomize all envs
indices = torch.arange(self._num_envs, dtype=torch.int64, device=self._device)
self.reset_idx(indices)
def calculate_metrics(self) -> None:
# variables for reward
ball_pos = self.ball_pos # ball pos
ball_vel = self._ball.get_velocities() # ball velocity
tool_pos, tool_rot = self._tool.get_world_poses() # tool center pos and rot
ball_linvels = ball_vel[:, 0:3] # ball linear velocity
# XXX REWARD
# 1st reward: ball keeps in the center (not with z-axis?) (with z-axis is good)
# ball_center_dist = torch.norm(tool_pos[:,0:2] - ball_pos[:,0:2], p=2, dim=-1)
ball_center_dist_3d = torch.norm(tool_pos - ball_pos, p=2, dim=-1)
# center_dist_reward = 1-torch.tanh(4*ball_center_dist)
# to cubic?
center_dist_reward = 1.0/(1.0+ball_center_dist_3d)
# 2nd reward: ball unmove
norm_ball_linvel = torch.norm(ball_linvels, p=2, dim=-1)
ball_vel_reward = 1.0/(1.0+norm_ball_linvel)
# 3rd reward: rotation not too much
rot_diff = torch.norm(tool_rot - self.default_tool_rot, p=2, dim=-1)
tool_rot_reward = 1.0/(1.0+rot_diff)
# stay alive
liveness = torch.where(ball_pos[:,2]>0.4, torch.ones_like(ball_pos[:,2]), torch.zeros_like(ball_pos[:,2]))
# the weight of center_dist_reward and ball_vel_reward should be similar
# how about tool rotation reward?
final_reward = 10.0*center_dist_reward + 5.0*ball_vel_reward + 1.0*tool_rot_reward + 1.0*liveness
self.rew_buf[:] = final_reward
# for record
self.episode_sums["final_reward"] += final_reward
def is_done(self) -> None:
if not self.is_test:
# 1st reset: if max length reached
self.reset_buf = torch.where(self.progress_buf >= self._max_episode_length - 1, torch.ones_like(self.reset_buf), self.reset_buf)
ball_pos = self.ball_pos # ball pos
tool_pos, tool_rot = self._tool.get_world_poses() # tool center pos and rot
ball_center_dist = torch.norm(tool_pos - ball_pos, p=2, dim=-1)
# 2nd reset: if ball falls from tool
self.reset_buf = torch.where(ball_center_dist > 0.54, torch.ones_like(self.reset_buf), self.reset_buf)
# 3rd reset: if ball falls too low
self.reset_buf = torch.where(self.ball_pos[:,2] < 0.5, torch.ones_like(self.reset_buf), self.reset_buf)
else:
self.reset_buf = torch.where(self.progress_buf >= self._max_episode_length - 1, torch.ones_like(self.reset_buf), self.reset_buf)
| 17,098 | Python | 42.070529 | 150 | 0.611241 |
zhehuazhou/ai-cps-robotics-benchmark/Gym_Envs/Tasks/Franka_Cube_Stacking.py | from omniisaacgymenvs.tasks.base.rl_task import RLTask
from Models.Franka.Franka import Franka
from Models.Franka.Franka_view import FrankaView
from omni.isaac.core.objects import DynamicCuboid
from omni.isaac.core.prims import RigidPrim, RigidPrimView
from omni.isaac.core.utils.prims import get_prim_at_path
from omni.isaac.core.utils.stage import get_current_stage, add_reference_to_stage
from omni.isaac.core.utils.torch.transformations import *
from omni.isaac.core.utils.torch.rotations import *
from omni.isaac.cloner import Cloner
import numpy as np
import torch
import math
from pxr import Gf, Usd, UsdGeom
class FrankaCubeStackingTask(RLTask):
def __init__(
self,
name,
sim_config,
env,
offset=None
) -> None:
self._sim_config = sim_config
self._cfg = sim_config.config
self._task_cfg = sim_config.task_config
self._num_envs = self._task_cfg["env"]["numEnvs"]
self._env_spacing = self._task_cfg["env"]["envSpacing"]
self._max_episode_length = self._task_cfg["env"]["episodeLength"]
self.action_scale = self._task_cfg["env"]["actionScale"]
self.start_position_noise = self._task_cfg["env"]["startPositionNoise"]
self.start_rotation_noise = self._task_cfg["env"]["startRotationNoise"]
self.dof_vel_scale = self._task_cfg["env"]["dofVelocityScale"]
self.dist_reward_scale = self._task_cfg["env"]["distRewardScale"]
self.rot_reward_scale = self._task_cfg["env"]["rotRewardScale"]
self.around_handle_reward_scale = self._task_cfg["env"]["aroundHandleRewardScale"]
self.open_reward_scale = self._task_cfg["env"]["openRewardScale"]
self.finger_dist_reward_scale = self._task_cfg["env"]["fingerDistRewardScale"]
self.action_penalty_scale = self._task_cfg["env"]["actionPenaltyScale"]
self.finger_close_reward_scale = self._task_cfg["env"]["fingerCloseRewardScale"]
self.distX_offset = 0.04
self.dt = 1/60.
self._num_observations = 28
self._num_actions = 9
# Flag for testing
self.is_test = False
self.initial_test_value = None
self.is_action_noise = False
RLTask.__init__(self, name, env)
# Extra info for TensorBoard
self.extras = {}
torch_zeros = lambda: torch.zeros(self.num_envs, dtype=torch.float, device=self.device, requires_grad=False)
self.episode_sums = {"cube_cube_dist": torch_zeros(), "finger_to_cube_dist": torch_zeros(), "is_stacked": torch_zeros(), "success_rate": torch_zeros()}
return
def set_up_scene(self, scene) -> None:
# Franka
franka_translation = torch.tensor([0.3, 0.0, 0.0])
self.get_franka(franka_translation)
self.get_cube()
self.get_target_cube()
# Here the env is cloned
super().set_up_scene(scene)
# Add Franka
self._frankas = FrankaView(prim_paths_expr="/World/envs/.*/franka", name="franka_view")
# Add cube
self._cube = RigidPrimView(prim_paths_expr="/World/envs/.*/cube", name="cube_view", reset_xform_properties=False)
# Add location_ball
self._target_cube = RigidPrimView(prim_paths_expr="/World/envs/.*/target_cube", name="target_cube_view", reset_xform_properties=False)
scene.add(self._frankas)
scene.add(self._frankas._hands)
scene.add(self._frankas._lfingers)
scene.add(self._frankas._rfingers)
scene.add(self._cube)
scene.add(self._target_cube)
self.init_data()
return
def get_franka(self, translation):
franka = Franka(prim_path=self.default_zero_env_path + "/franka", name="franka", translation = translation)
self._sim_config.apply_articulation_settings("franka", get_prim_at_path(franka.prim_path), self._sim_config.parse_actor_config("franka"))
def get_cube(self):
cube = DynamicCuboid(
name = 'cube',
position=[-0.04, 0.0, 0.91],
orientation=[1,0,0,0],
size=0.05,
prim_path=self.default_zero_env_path + "/cube",
color=np.array([1, 0, 0]),
density = 100
)
def get_target_cube(self):
target_cube = DynamicCuboid(
name = 'target_cube',
position=[-0.3, 0.1, 0.025],
orientation=[1, 0, 0, 0],
prim_path=self.default_zero_env_path + "/target_cube",
size=0.05,
color=np.array([0, 1, 0]),
density = 100
)
# Set as testing mode
def set_as_test(self):
self.is_test = True
# Set action noise
def set_action_noise(self):
self.is_action_noise = True
# Set initial test values for testing mode
def set_initial_test_value(self, value):
# for ball pushing: initial x,y positions of the ball
self.initial_test_value = value
def init_data(self) -> None:
def get_env_local_pose(env_pos, xformable, device):
"""Compute pose in env-local coordinates"""
world_transform = xformable.ComputeLocalToWorldTransform(0)
world_pos = world_transform.ExtractTranslation()
world_quat = world_transform.ExtractRotationQuat()
px = world_pos[0] - env_pos[0]
py = world_pos[1] - env_pos[1]
pz = world_pos[2] - env_pos[2]
qx = world_quat.imaginary[0]
qy = world_quat.imaginary[1]
qz = world_quat.imaginary[2]
qw = world_quat.real
return torch.tensor([px, py, pz, qw, qx, qy, qz], device=device, dtype=torch.float)
stage = get_current_stage()
hand_pose = get_env_local_pose(self._env_pos[0], UsdGeom.Xformable(stage.GetPrimAtPath("/World/envs/env_0/franka/panda_link7")), self._device)
lfinger_pose = get_env_local_pose(
self._env_pos[0], UsdGeom.Xformable(stage.GetPrimAtPath("/World/envs/env_0/franka/panda_leftfinger")), self._device
)
rfinger_pose = get_env_local_pose(
self._env_pos[0], UsdGeom.Xformable(stage.GetPrimAtPath("/World/envs/env_0/franka/panda_rightfinger")), self._device
)
# finger pos
finger_pose = torch.zeros(7, device=self._device)
finger_pose[0:3] = (lfinger_pose[0:3] + rfinger_pose[0:3]) / 2.0
finger_pose[3:7] = lfinger_pose[3:7]
hand_pose_inv_rot, hand_pose_inv_pos = (tf_inverse(hand_pose[3:7], hand_pose[0:3]))
# franka grasp local pose
grasp_pose_axis = 1
franka_local_grasp_pose_rot, franka_local_pose_pos = tf_combine(hand_pose_inv_rot, hand_pose_inv_pos, finger_pose[3:7], finger_pose[0:3])
franka_local_pose_pos += torch.tensor([0, 0.04, 0], device=self._device)
self.franka_local_grasp_pos = franka_local_pose_pos.repeat((self._num_envs, 1))
self.franka_local_grasp_rot = franka_local_grasp_pose_rot.repeat((self._num_envs, 1))
self.gripper_forward_axis = torch.tensor([0, 0, 1], device=self._device, dtype=torch.float).repeat((self._num_envs, 1))
self.gripper_up_axis = torch.tensor([0, 1, 0], device=self._device, dtype=torch.float).repeat((self._num_envs, 1))
self.franka_default_dof_pos = torch.tensor(
[0.0, -0.872, 0.0, -2.0, 0.0, 2.618, 0.785, 0.025, 0.025], device=self._device
)
self.actions = torch.zeros((self._num_envs, self.num_actions), device=self._device)
def get_observations(self) -> dict:
# Franka
hand_pos, hand_rot = self._frankas._hands.get_world_poses(clone=False)
franka_dof_pos = self._frankas.get_joint_positions(clone=False)
franka_dof_vel = self._frankas.get_joint_velocities(clone=False)
self.franka_dof_pos = franka_dof_pos
self.franka_lfinger_pos, self.franka_lfinger_rot = self._frankas._lfingers.get_world_poses(clone=False)
self.franka_rfinger_pos, self.franka_rfinger_rot = self._frankas._lfingers.get_world_poses(clone=False)
dof_pos_scaled = (
2.0
* (franka_dof_pos - self.franka_dof_lower_limits)
/ (self.franka_dof_upper_limits - self.franka_dof_lower_limits)
- 1.0
)
# cube
cube_pos, cube_rot = self._cube.get_world_poses(clone=False)
# target cube
tar_cube_pos, tar_cube_rot = self._target_cube.get_world_poses(clone=False) # tool position
to_target = cube_pos - tar_cube_pos
self.obs_buf = torch.cat(
(
dof_pos_scaled,
franka_dof_vel * self.dof_vel_scale,
cube_pos,
cube_rot,
to_target,
),
dim=-1,
)
observations = {
self._frankas.name: {
"obs_buf": self.obs_buf
}
}
return observations
def pre_physics_step(self, actions) -> None:
if not self._env._world.is_playing():
return
reset_env_ids = self.reset_buf.nonzero(as_tuple=False).squeeze(-1)
if len(reset_env_ids) > 0:
self.reset_idx(reset_env_ids)
self.actions = actions.clone().to(self._device)
# if action noise
if self.is_action_noise is True:
# Gaussian white noise with 0.01 variance
self.actions = self.actions + (0.5)*torch.randn_like(self.actions)
targets = self.franka_dof_targets + self.franka_dof_speed_scales * self.dt * self.actions * self.action_scale
self.franka_dof_targets[:] = tensor_clamp(targets, self.franka_dof_lower_limits, self.franka_dof_upper_limits)
env_ids_int32 = torch.arange(self._frankas.count, dtype=torch.int32, device=self._device)
# self._frankas.set_joint_position_targets(self.franka_dof_targets, indices=env_ids_int32)
# # release cube
cube_pos, cube_rot = self._cube.get_world_poses(clone=False) # cube
tar_cube_pos, tar_cube_rot = self._target_cube.get_world_poses(clone=False) # target pos
target_pos = tar_cube_pos.clone().detach()
target_pos[:,2] = target_pos[:,2] + 0.025
target_dist = torch.norm(cube_pos - tar_cube_pos, p=2, dim=-1)
self.release_condition = torch.logical_and(target_dist<0.08, cube_pos[:,2] >= target_pos[:,2])
# self.franka_dof_targets[:,7] = torch.where(self.release_condition, 0.08, self.franka_dof_targets[:,7])
# self.franka_dof_targets[:,8] = torch.where(self.release_condition, 0.08, self.franka_dof_targets[:,8])
self.franka_dof_targets[:,7] = torch.where(self.release_condition, 0.08, 0.005)
self.franka_dof_targets[:,8] = torch.where(self.release_condition, 0.08, 0.005)
self._frankas.set_joint_position_targets(self.franka_dof_targets, indices=env_ids_int32)
def reset_idx(self, env_ids):
indices = env_ids.to(dtype=torch.int32)
num_indices = len(indices)
# reset franka
pos = torch.clamp(
self.franka_default_dof_pos.unsqueeze(0),
#+ 0.25 * (torch.rand((len(env_ids), self.num_franka_dofs), device=self._device) - 0.5),
self.franka_dof_lower_limits,
self.franka_dof_upper_limits,
)
dof_pos = torch.zeros((num_indices, self._frankas.num_dof), device=self._device)
dof_vel = torch.zeros((num_indices, self._frankas.num_dof), device=self._device)
dof_pos[:, :] = pos
self.franka_dof_targets[env_ids, :] = pos
self.franka_dof_pos[env_ids, :] = pos
self._frankas.set_joint_position_targets(self.franka_dof_targets[env_ids], indices=indices)
self._frankas.set_joint_positions(dof_pos, indices=indices)
self._frankas.set_joint_velocities(dof_vel, indices=indices)
# reset cube
self._cube.set_world_poses(self.default_cube_pos[env_ids], self.default_cube_rot[env_ids], indices = indices)
self._cube.set_velocities(self.default_cube_velocity[env_ids], indices = indices)
if not self.is_test:
# reset target cube
# reset target cube position within an area: x [-0.2, 0.2], y [-0.2,0.2]
self.new_cube_pos = self.default_target_cube_pos.clone().detach()
self.new_cube_pos[:,0] = self.default_target_cube_pos[:,0] + (0.2 + 0.2) * torch.rand(self._num_envs, device=self._device) -0.2
self.new_cube_pos[:,1] = self.default_target_cube_pos[:,1] + (0.2 + 0.2) * torch.rand(self._num_envs, device=self._device) -0.2
self._target_cube.set_world_poses(self.new_cube_pos[env_ids], self.default_target_cube_rot[env_ids], indices = indices)
self._target_cube.set_velocities(self.default_target_cube_velocity[env_ids], indices = indices)
# if is test mode
else:
self.new_cube_pos = self.default_target_cube_pos.clone().detach()
self.new_cube_pos[:,0] = self.default_target_cube_pos[:,0] + self.initial_test_value[0]
self.new_cube_pos[:,1] = self.default_target_cube_pos[:,1] + self.initial_test_value[1]
self._target_cube.set_world_poses(self.new_cube_pos[env_ids], self.default_target_cube_rot[env_ids], indices = indices)
self._target_cube.set_velocities(self.default_target_cube_velocity[env_ids], indices = indices)
# bookkeeping
self.reset_buf[env_ids] = 0
self.progress_buf[env_ids] = 0
# fill extras
self.extras["episode"] = {}
for key in self.episode_sums.keys():
if key == "success_rate":
self.extras["episode"][key] = torch.mean(self.episode_sums[key][env_ids])
else:
self.extras["episode"][key] = torch.mean(self.episode_sums[key][env_ids]) / self._max_episode_length
self.episode_sums[key][env_ids] = 0
def post_reset(self):
# Franka
self.num_franka_dofs = self._frankas.num_dof
self.franka_dof_pos = torch.zeros((self.num_envs, self.num_franka_dofs), device=self._device)
dof_limits = self._frankas.get_dof_limits()
self.franka_dof_lower_limits = dof_limits[0, :, 0].to(device=self._device)
self.franka_dof_upper_limits = dof_limits[0, :, 1].to(device=self._device)
self.franka_dof_speed_scales = torch.ones_like(self.franka_dof_lower_limits)
self.franka_dof_speed_scales[self._frankas.gripper_indices] = 0.1
self.franka_dof_targets = torch.zeros(
(self._num_envs, self.num_franka_dofs), dtype=torch.float, device=self._device
)
# Cube
self.default_cube_pos, self.default_cube_rot = self._cube.get_world_poses()
self.default_cube_velocity = self._cube.get_velocities()
# Target cube
self.default_target_cube_pos, self.default_target_cube_rot = self._target_cube.get_world_poses()
self.default_target_cube_velocity = self._target_cube.get_velocities()
# randomize all envs
indices = torch.arange(self._num_envs, dtype=torch.int64, device=self._device)
self.reset_idx(indices)
def calculate_metrics(self) -> None:
# reward info
joint_positions = self.franka_dof_pos
cube_pos, cube_rot = self._cube.get_world_poses(clone=False) # cube
cube_vel = self._cube.get_velocities()
cube_vel = cube_vel[:,0:3]
cube_vel_norm = torch.norm(cube_vel, p=2, dim=-1)
tar_cube_pos, tar_cube_rot = self._target_cube.get_world_poses(clone=False) # target pos
target_pos = tar_cube_pos.clone().detach()
target_pos[:,2] = target_pos[:,2] + 0.02
# target_pos[:,0] = target_pos[:,0] -0.015
lfinger_pos, lfinger_rot = self._frankas._lfingers.get_world_poses(clone=False) # franka finger
rfinger_pos, rfinger_rot = self._frankas._rfingers.get_world_poses(clone=False)
finger_pos = (lfinger_pos + rfinger_pos)/2
# 1st reward: cube to target distance
cube_targe_dist = torch.norm(target_pos - cube_pos, p=2, dim=-1)
cube_tar_dist_reward = 1.0/(1.0+cube_targe_dist)
cube_targe_XY_dist = torch.norm(target_pos[:,0:2] - cube_pos[:,0:2] , p=2, dim=-1)
cube_tar_XY_dist_reward = 1.0/(1.0+cube_targe_XY_dist**2)
# 2nd reward: if cube is stacked, task complete
finger_to_cube_dist = torch.norm(finger_pos - cube_pos, p=2, dim=-1)
is_stacked = torch.where(torch.logical_and(cube_targe_dist<0.05, cube_pos[:,2]>=target_pos[:,2]),
torch.ones_like(cube_tar_dist_reward), torch.zeros_like(cube_tar_dist_reward))
self.is_complete = torch.where(torch.logical_and(finger_to_cube_dist>0.05, torch.logical_and(cube_vel_norm<0.05, is_stacked==1)),
torch.ones_like(cube_tar_dist_reward), torch.zeros_like(cube_tar_dist_reward))
# self.is_complete = torch.where(torch.logical_and(finger_to_cube_dist>0.05, torch.logical_and(cube_vel_norm<0.05, is_stacked==1)),
# torch.ones_like(cube_tar_dist_reward), torch.zeros_like(cube_tar_dist_reward))
# 3rd reward: finger to cube distanfce
finger_cube_dist_reward = 1.0/(1.0+finger_to_cube_dist)
finger_cube_dist_reward = torch.where(is_stacked==1, 1-finger_cube_dist_reward, finger_cube_dist_reward)
# 4th reward: finger closeness reward
# finger_close_reward = torch.zeros_like(cube_tar_dist_reward)
finger_close_reward = (0.04 - joint_positions[:, 7]) + (0.04 - joint_positions[:, 8])
finger_close_reward = torch.where(is_stacked !=1, finger_close_reward, -finger_close_reward)
# 5th reward: cube velocity reward
cube_vel_reward = 1.0/(1.0+cube_vel_norm)
# if cube falls on the ground
self.is_fall = torch.where(cube_pos[:,2]<0.05, torch.ones_like(cube_tar_dist_reward), cube_tar_dist_reward)
# final reward
final_reward = 2*cube_tar_dist_reward + 0.0*finger_cube_dist_reward + 0.0*finger_close_reward + 0.0*cube_vel_reward \
+ 10*self.is_complete - 0.5*self.is_fall + 0.0*is_stacked + 0.0*cube_tar_XY_dist_reward
final_reward = torch.where(cube_targe_dist<0.2, final_reward+2.0*cube_tar_XY_dist_reward, final_reward)
self.rew_buf[:] = final_reward
self.episode_sums["success_rate"] += self.is_complete
self.episode_sums["cube_cube_dist"] += cube_targe_dist
self.episode_sums["finger_to_cube_dist"] += finger_to_cube_dist
self.episode_sums["is_stacked"] += is_stacked
def is_done(self) -> None:
if not self.is_test:
# reset: if task is complete
# self.reset_buf = torch.where(self.is_complete==1, torch.ones_like(self.reset_buf), self.reset_buf)
# reset if cube falls on the ground
cube_pos, cube_rot = self._cube.get_world_poses(clone=False)
# self.reset_buf = torch.where(self.is_fall==1, torch.ones_like(self.reset_buf), self.reset_buf)
# rest if cube is too far away from the target cube
tar_cube_pos, tar_cube_rot = self._target_cube.get_world_poses(clone=False)
cube_target_XY_dist = torch.norm(tar_cube_pos[:,0:2] - cube_pos[:,0:2] , p=2, dim=-1)
self.reset_buf = torch.where(cube_target_XY_dist > 0.8, torch.ones_like(self.reset_buf), self.reset_buf)
# reset if max length reached
self.reset_buf = torch.where(self.progress_buf >= self._max_episode_length - 1, torch.ones_like(self.reset_buf), self.reset_buf)
else:
self.reset_buf = torch.where(self.progress_buf >= self._max_episode_length - 1, torch.ones_like(self.reset_buf), self.reset_buf)
| 19,860 | Python | 43.53139 | 159 | 0.611329 |
zhehuazhou/ai-cps-robotics-benchmark/Gym_Envs/Tasks/Franka_Peg_In_Hole.py | from omniisaacgymenvs.tasks.base.rl_task import RLTask
from Models.Franka.Franka import Franka
from Models.Franka.Franka_view import FrankaView
from Models.peg_in_hole.table import Table
from Models.peg_in_hole.tool import Tool
from omni.isaac.core.objects import DynamicSphere
from omni.isaac.core.prims import RigidPrim, RigidPrimView
from omni.isaac.core.utils.prims import get_prim_at_path
from omni.isaac.core.utils.stage import get_current_stage
from omni.isaac.core.utils.torch.transformations import *
from omni.isaac.core.utils.torch.rotations import *
from omni.isaac.cloner import Cloner
import numpy as np
import torch
import math
from pxr import Usd, UsdGeom
class FrankaPegInHoleTask(RLTask):
def __init__(
self,
name,
sim_config,
env,
offset=None
) -> None:
self._sim_config = sim_config
self._cfg = sim_config.config
self._task_cfg = sim_config.task_config
self._num_envs = self._task_cfg["env"]["numEnvs"]
self._env_spacing = self._task_cfg["env"]["envSpacing"]
self._max_episode_length = self._task_cfg["env"]["episodeLength"]
self.action_scale = self._task_cfg["env"]["actionScale"]
self.start_position_noise = self._task_cfg["env"]["startPositionNoise"]
self.start_rotation_noise = self._task_cfg["env"]["startRotationNoise"]
self.dof_vel_scale = self._task_cfg["env"]["dofVelocityScale"]
self.dist_reward_scale = self._task_cfg["env"]["distRewardScale"]
self.rot_reward_scale = self._task_cfg["env"]["rotRewardScale"]
self.around_handle_reward_scale = self._task_cfg["env"]["aroundHandleRewardScale"]
self.open_reward_scale = self._task_cfg["env"]["openRewardScale"]
self.finger_dist_reward_scale = self._task_cfg["env"]["fingerDistRewardScale"]
self.action_penalty_scale = self._task_cfg["env"]["actionPenaltyScale"]
self.finger_close_reward_scale = self._task_cfg["env"]["fingerCloseRewardScale"]
self.location_ball_radius = self._task_cfg["env"]["locationBallRadius"]
self.location_ball_initial_position = self._task_cfg["env"]["locationBallPosition"]
self.location_ball_initial_orientation = self._task_cfg["env"]["locationBallInitialOrientation"]
self.distX_offset = 0.04
self.dt = 1/60.
self._num_observations = 28
self._num_actions = 9
# Flag for testing
self.is_test = False
self.initial_test_value = None
self.is_action_noise = False
RLTask.__init__(self, name, env)
# Extra info for TensorBoard
self.extras = {}
torch_zeros = lambda: torch.zeros(self.num_envs, dtype=torch.float, device=self.device, requires_grad=False)
self.episode_sums = {"tool_hole_XY_dist": torch_zeros(), "tool_hole_Z_dist": torch_zeros(), "tool_hole_dist": torch_zeros(),
"tool_rot_error": torch_zeros(), "peg_rate": torch_zeros(), "norm_finger_vel": torch_zeros(), "rewards": torch_zeros()}
return
def set_up_scene(self, scene) -> None:
franka_translation = torch.tensor([0.5, 0.0, 0.0])
self.get_franka(franka_translation)
self.get_table()
self.get_tool()
super().set_up_scene(scene)
# Add Franka
self._frankas = FrankaView(prim_paths_expr="/World/envs/.*/franka", name="franka_view")
# Add Table
self._table = RigidPrimView(prim_paths_expr="/World/envs/.*/table/table/table_mesh", name="table_view", reset_xform_properties=False)
# Add Tool
self._tool = RigidPrimView(prim_paths_expr="/World/envs/.*/tool/tool/tool", name="tool_view", reset_xform_properties=False)
# Add location_ball
self._location_ball = RigidPrimView(prim_paths_expr="/World/envs/.*/table/table/location_ball", name="location_ball_view", reset_xform_properties=False)
scene.add(self._frankas)
scene.add(self._frankas._hands)
scene.add(self._frankas._lfingers)
scene.add(self._frankas._rfingers)
scene.add(self._table)
scene.add(self._tool)
scene.add(self._location_ball)
self.init_data()
return
def get_franka(self, translation):
franka = Franka(prim_path=self.default_zero_env_path + "/franka", name="franka", translation = translation)
self._sim_config.apply_articulation_settings("franka", get_prim_at_path(franka.prim_path), self._sim_config.parse_actor_config("franka"))
def get_table(self):
table = Table(prim_path=self.default_zero_env_path + "/table", name="table")
self._sim_config.apply_articulation_settings("table", get_prim_at_path(table.prim_path), self._sim_config.parse_actor_config("table"))
def get_tool(self):
tool = Tool(prim_path=self.default_zero_env_path + "/tool", name="tool")
self._sim_config.apply_articulation_settings("tool", get_prim_at_path(tool.prim_path), self._sim_config.parse_actor_config("tool"))
# Set as testing mode
def set_as_test(self):
self.is_test = True
# Set action noise
def set_action_noise(self):
self.is_action_noise = True
# Set initial test values for testing mode
def set_initial_test_value(self, value):
# for ball pushing: initial x,y positions of the ball
self.initial_test_value = value
def init_data(self) -> None:
def get_env_local_pose(env_pos, xformable, device):
"""Compute pose in env-local coordinates"""
world_transform = xformable.ComputeLocalToWorldTransform(0)
world_pos = world_transform.ExtractTranslation()
world_quat = world_transform.ExtractRotationQuat()
px = world_pos[0] - env_pos[0]
py = world_pos[1] - env_pos[1]
pz = world_pos[2] - env_pos[2]
qx = world_quat.imaginary[0]
qy = world_quat.imaginary[1]
qz = world_quat.imaginary[2]
qw = world_quat.real
return torch.tensor([px, py, pz, qw, qx, qy, qz], device=device, dtype=torch.float)
stage = get_current_stage()
hand_pose = get_env_local_pose(self._env_pos[0], UsdGeom.Xformable(stage.GetPrimAtPath("/World/envs/env_0/franka/panda_link7")), self._device)
lfinger_pose = get_env_local_pose(
self._env_pos[0], UsdGeom.Xformable(stage.GetPrimAtPath("/World/envs/env_0/franka/panda_leftfinger")), self._device
)
rfinger_pose = get_env_local_pose(
self._env_pos[0], UsdGeom.Xformable(stage.GetPrimAtPath("/World/envs/env_0/franka/panda_rightfinger")), self._device
)
finger_pose = torch.zeros(7, device=self._device)
finger_pose[0:3] = (lfinger_pose[0:3] + rfinger_pose[0:3]) / 2.0
finger_pose[3:7] = lfinger_pose[3:7]
hand_pose_inv_rot, hand_pose_inv_pos = (tf_inverse(hand_pose[3:7], hand_pose[0:3]))
grasp_pose_axis = 1
franka_local_grasp_pose_rot, franka_local_pose_pos = tf_combine(hand_pose_inv_rot, hand_pose_inv_pos, finger_pose[3:7], finger_pose[0:3])
franka_local_pose_pos += torch.tensor([0, 0.04, 0], device=self._device)
self.franka_local_grasp_pos = franka_local_pose_pos.repeat((self._num_envs, 1))
self.franka_local_grasp_rot = franka_local_grasp_pose_rot.repeat((self._num_envs, 1))
# tool reference rotation
self.tool_ref_rot = torch.tensor([0.5, 0.5, 0.5, 0.5], device=self._device)
# self.gripper_forward_axis = torch.tensor([0, 0, 1], device=self._device, dtype=torch.float).repeat((self._num_envs, 1))
# self.gripper_up_axis = torch.tensor([0, 1, 0], device=self._device, dtype=torch.float).repeat((self._num_envs, 1))
# default franka pos: for initially grap the tool
self.franka_default_dof_pos = torch.tensor(
[0.0, 0.0, 0.0, 0.0, 0.0, 0.0, 0.0, 0.015, 0.015], device=self._device
)
self.actions = torch.zeros((self._num_envs, self.num_actions), device=self._device)
def get_observations(self) -> dict:
hand_pos, hand_rot = self._frankas._hands.get_world_poses(clone=False)
franka_dof_pos = self._frankas.get_joint_positions(clone=False)
franka_dof_vel = self._frankas.get_joint_velocities(clone=False)
self.franka_dof_pos = franka_dof_pos
self.franka_lfinger_pos, self.franka_lfinger_rot = self._frankas._lfingers.get_world_poses(clone=False)
self.franka_rfinger_pos, self.franka_rfinger_rot = self._frankas._lfingers.get_world_poses(clone=False)
# Tool
self.tool_pos, self.tool_rot = self._tool.get_world_poses(clone=False)
hole_pos, hole_rot = self._location_ball.get_world_poses()
to_target = self.tool_pos - hole_pos
dof_pos_scaled = (
2.0
* (franka_dof_pos - self.franka_dof_lower_limits)
/ (self.franka_dof_upper_limits - self.franka_dof_lower_limits)
- 1.0
)
# print(torch.norm(to_target, p=2, dim=-1))
self.obs_buf = torch.cat(
(
dof_pos_scaled,
franka_dof_vel * self.dof_vel_scale,
self.tool_pos,
self.tool_rot,
to_target
# self.location_ball_pos
# self.cabinet_dof_pos[:, 3].unsqueeze(-1),
# self.cabinet_dof_vel[:, 3].unsqueeze(-1),
),
dim=-1,
)
observations = {
self._frankas.name: {
"obs_buf": self.obs_buf
}
}
return observations
def pre_physics_step(self, actions) -> None:
if not self._env._world.is_playing():
return
reset_env_ids = self.reset_buf.nonzero(as_tuple=False).squeeze(-1)
if len(reset_env_ids) > 0:
self.reset_idx(reset_env_ids)
self.actions = actions.clone().to(self._device)
# if action noise
if self.is_action_noise is True:
# Gaussian white noise with 0.01 variance
self.actions = self.actions + (0.5)*torch.randn_like(self.actions)
targets = self.franka_dof_targets + self.franka_dof_speed_scales * self.dt * self.actions * self.action_scale
self.franka_dof_targets[:] = tensor_clamp(targets, self.franka_dof_lower_limits, self.franka_dof_upper_limits)
env_ids_int32 = torch.arange(self._frankas.count, dtype=torch.int32, device=self._device)
# NOTE HERE: right now I fix the finger movement so that the object will always be grasped in hand
# Later: if the reward is good enough, the hand should be released once the object is in the hole,
# this means the last two dofs are also in the action
# self.franka_dof_targets[:,7] = self.franka_default_dof_pos[7]
# self.franka_dof_targets[:,8] = self.franka_default_dof_pos[8]
# release the finger if tool is right above the hole
hole_pos, hole_rot = self._location_ball.get_world_poses()
tool_pos, tool_rot = self._tool.get_world_poses()
hole_pos[:,2] = 0.39
tool_hole_dist = torch.norm(tool_pos - hole_pos, p=2, dim=-1)
tool_hole_XY_dist = torch.norm(tool_pos[:,0:2] - hole_pos[:,0:2], p=2, dim=-1)
tool_hole_Z_dist = torch.norm(tool_pos[:,2] - hole_pos[:,2], p=2, dim=-1)
tool_rot_error = torch.norm(tool_rot - self.tool_ref_rot, p=2, dim=-1)
# self.release_condition = torch.logical_and(tool_hole_XY_dist <= 0.1, tool_rot_error<=1)
# self.release_condition = torch.logical_and(self.release_condition, tool_hole_Z_dist<=0.1)
# self.release_condition = torch.logical_and(tool_hole_dist<0.08, self.is_released)
self.release_condition = tool_hole_dist<=0.024
# self.release_condition = torch.logical_and(tool_hole_XY_dist<=0.04, tool_hole_Z_dist<=0.07)
# self.release_condition = torch.logical_and(self.release_condition, tool_rot_error<=1)
# self.is_released = self.release_condition.clone().detach()
self.franka_dof_targets[:,7] = torch.where(self.release_condition, 0.1, 0.015)
self.franka_dof_targets[:,8] = torch.where(self.release_condition, 0.1, 0.015)
# set franka target joint position
self._frankas.set_joint_position_targets(self.franka_dof_targets, indices=env_ids_int32)
def reset_idx(self, env_ids):
indices = env_ids.to(dtype=torch.int32)
num_indices = len(indices)
# reset franka (due to initial grasping, cannot randomize)
pos = torch.clamp(
self.franka_default_dof_pos.unsqueeze(0),
# + 0.25 * (torch.rand((len(env_ids), self.num_franka_dofs), device=self._device) - 0.5),
self.franka_dof_lower_limits,
self.franka_dof_upper_limits,
)
dof_pos = torch.zeros((num_indices, self._frankas.num_dof), device=self._device)
dof_vel = torch.zeros((num_indices, self._frankas.num_dof), device=self._device)
dof_pos[:, :] = pos
self.franka_dof_targets[env_ids, :] = pos
self.franka_dof_pos[env_ids, :] = pos
self._frankas.set_joint_position_targets(self.franka_dof_targets[env_ids], indices=indices)
self._frankas.set_joint_positions(dof_pos, indices=indices)
self._frankas.set_joint_velocities(dof_vel, indices=indices)
# reset tool
self._tool.set_world_poses(self.default_tool_pos[env_ids], self.default_tool_rot[env_ids], indices = indices)
self._tool.set_velocities(self.default_tool_velocity[env_ids], indices = indices)
if not self.is_test:
# reset table
# reset positions: x: [-0.2,0.2], y:[-0.2,0.2]
random_x = (0.2 + 0.2) * torch.rand(self._num_envs, device=self._device) -0.2
random_y = (0.2 + 0.2) * torch.rand(self._num_envs, device=self._device) -0.2
self.new_table_pos = self.default_table_pos.clone().detach()
self.new_table_pos[:,0] = self.default_table_pos[:,0] + random_x
self.new_table_pos[:,1] = self.default_table_pos[:,1] + random_y
self._table.set_world_poses(self.new_table_pos[env_ids], self.default_table_rot[env_ids], indices = indices)
self._table.set_velocities(self.default_table_velocity[env_ids], indices = indices)
else:
self.new_table_pos = self.default_table_pos.clone().detach()
self.new_table_pos[:,0] = self.default_table_pos[:,0] + self.initial_test_value[0]
self.new_table_pos[:,1] = self.default_table_pos[:,1] + self.initial_test_value[1]
self._table.set_world_poses(self.new_table_pos[env_ids], self.default_table_rot[env_ids], indices = indices)
self._table.set_velocities(self.default_table_velocity[env_ids], indices = indices)
self.is_released = torch.zeros((1,self._num_envs), device=self._device)
# bookkeeping
self.reset_buf[env_ids] = 0
self.progress_buf[env_ids] = 0
# fill extras
self.extras["episode"] = {}
for key in self.episode_sums.keys():
if key == "peg_rate":
self.extras["episode"][key] = torch.mean(self.episode_sums[key][env_ids])
else:
self.extras["episode"][key] = torch.mean(self.episode_sums[key][env_ids]) / self._max_episode_length
self.episode_sums[key][env_ids] = 0
def post_reset(self):
self.num_franka_dofs = self._frankas.num_dof
self.franka_dof_pos = torch.zeros((self.num_envs, self.num_franka_dofs), device=self._device)
dof_limits = self._frankas.get_dof_limits()
self.franka_dof_lower_limits = dof_limits[0, :, 0].to(device=self._device)
self.franka_dof_upper_limits = dof_limits[0, :, 1].to(device=self._device)
self.franka_dof_speed_scales = torch.ones_like(self.franka_dof_lower_limits)
self.franka_dof_speed_scales[self._frankas.gripper_indices] = 0.1
self.franka_dof_targets = torch.zeros(
(self._num_envs, self.num_franka_dofs), dtype=torch.float, device=self._device
)
# tool
self.default_tool_pos, self.default_tool_rot = self._tool.get_world_poses()
self.default_tool_velocity = self._tool.get_velocities()
# table
self.default_table_pos, self.default_table_rot = self._table.get_world_poses()
self.default_table_velocity = self._table.get_velocities()
# randomize all envs
indices = torch.arange(self._num_envs, dtype=torch.int64, device=self._device)
self.reset_idx(indices)
def calculate_metrics(self) -> None:
# Envoroonment parameters:
# table height: 0.4
# hole depth: 0.05
# hole radius: 0.01
# tool at surface: Z = 0.43
# tool pegged in hole: Z = 0.38
# tool_pos to tool bottom: Z = 0.03
# tool body length: 0.06
# tool cap length: 0.01
# tool vertical orient: [0.5, 0.5, 0.5, 0.5]
# tool_ref_rot = self.tool_ref_rot # tool reference vertical rotation
num_envs = self._num_envs
tool_pos, tool_rot = self._tool.get_world_poses(clone=False)
hole_pos, hole_rot = self._location_ball.get_world_poses(clone=False)
hole_pos[:,2] = 0.38 # fix hole pos
hole_surf_pos = hole_pos.clone().detach()
hole_surf_pos[:,2] = hole_surf_pos[:,2]
hole_target_pos = hole_pos.clone().detach()
hole_target_pos[:,2] = 0.39
# tool_ref_rot = torch.zeros_like(tool_rot)
# tool_ref_rot[:,:] = self.tool_ref_rot # tool reference vertical rotation
tool_ref_rot= self.tool_ref_rot
lfinger_pos, lfinger_rot = self._frankas._lfingers.get_world_poses(clone=False)
rfinger_pos, rfinger_rot = self._frankas._rfingers.get_world_poses(clone=False)
finger_rot = (lfinger_rot + rfinger_rot)/2
finger_pos = (lfinger_pos + rfinger_pos)/2
finger_rot_ref = torch.tensor([0.0325, -0.3824, 0.9233, -0.0135], device=self._device)
# finger velocity
lfinger_vel = self._frankas._lfingers.get_velocities()
rfinger_vel = self._frankas._rfingers.get_velocities()
finger_vel = (lfinger_vel[:,0:3]+rfinger_vel[:,0:3])/2
norm_finger_vel = torch.norm(finger_vel, p=2, dim=-1)
# direction vector
ref_vector = torch.zeros([num_envs,3], device=self._device)
ref_vector[:,0] = 2*(tool_ref_rot[0]*tool_ref_rot[2] - tool_ref_rot[3]*tool_ref_rot[1])
ref_vector[:,1] = 2*(tool_ref_rot[1]*tool_ref_rot[2] + tool_ref_rot[3]*tool_ref_rot[0])
ref_vector[:,2] = 1 - 2*(tool_ref_rot[0]*tool_ref_rot[0] + tool_ref_rot[1]*tool_ref_rot[1])
tool_vector = torch.zeros([num_envs,3], device=self._device)
tool_vector[:,0] = 2*(tool_rot[:,0]*tool_rot[:,2] - tool_rot[:,3]*tool_rot[:,1])
tool_vector[:,1] = 2*(tool_rot[:,1]*tool_rot[:,2] + tool_rot[:,3]*tool_rot[:,0])
tool_vector[:,2] = 1 - 2*(tool_rot[:,0]*tool_rot[:,0] + tool_rot[:,1]*tool_rot[:,1])
# roll = atan2(2.0 * (q.q3 * q.q2 + q.q0 * q.q1) , 1.0 - 2.0 * (q.q1 * q.q1 + q.q2 * q.q2));
# pitch = asin(2.0 * (q.q2 * q.q0 - q.q3 * q.q1));
# yaw = atan2(2.0 * (q.q3 * q.q0 + q.q1 * q.q2) , - 1.0 + 2.0 * (q.q0 * q.q0 + q.q1 * q.q1));
tool_roll = torch.atan2(2.0*(tool_rot[:,0]*tool_rot[:,1] + tool_rot[:,2]*tool_rot[:,3]), 1.0-2.0*(tool_rot[:,2]*tool_rot[:,2]+tool_rot[:,1]*tool_rot[:,1]))
tool_yaw= torch.atan2(2.0*(tool_rot[:,3]*tool_rot[:,2] + tool_rot[:,0]*tool_rot[:,1]), 1.0-2.0*(tool_rot[:,1]*tool_rot[:,1]+tool_rot[:,2]*tool_rot[:,2]))
tool_pitch = torch.asin(2.0*(tool_rot[:,0]*tool_rot[:,2] - tool_rot[:,1]*tool_rot[:,3]))
tool_ref_roll = torch.atan2(2.0*(tool_ref_rot[0]*tool_ref_rot[1] + tool_ref_rot[2]*tool_ref_rot[3]), 1.0-2.0*(tool_ref_rot[2]*tool_ref_rot[2]+tool_ref_rot[1]*tool_ref_rot[1]))
tool_ref_yaw = torch.atan2(2.0*(tool_ref_rot[3]*tool_ref_rot[2] + tool_ref_rot[0]*tool_ref_rot[1]), 1.0-2.0*(tool_ref_rot[1]*tool_ref_rot[1]+tool_ref_rot[2]*tool_ref_rot[2]))
tool_ref_pitch = torch.asin(2.0*(tool_ref_rot[0]*tool_ref_rot[2] - tool_ref_rot[1]*tool_ref_rot[3]))
tool_roll_error = torch.abs(tool_roll - tool_ref_roll)
tool_pitch_error = torch.abs(tool_pitch - tool_ref_pitch)
tool_roll_pitch_reward = 1 - torch.tanh(2*tool_roll_error) + 1 - torch.tanh(2*tool_pitch_error)
# tool_roll_yaw_reward = 1 - torch.tanh(2*tool_roll_error) + 1 - torch.tanh(2*tool_yaw_error)
# Handle Nan exception
# tool_roll_pitch_reward = torch.where(torch.isnan(tool_roll_error+tool_pitch_error), torch.ones_like(tool_roll_pitch_reward), tool_roll_pitch_reward)
# 1st reward: tool XY position
tool_hole_dist = torch.norm(tool_pos - hole_pos, p=2, dim=-1)
tool_hole_XY_dist = torch.norm(tool_pos[:,0:2] - hole_pos[:,0:2], p=2, dim=-1)
# tool_XY_pos_reward = 1.0 / (1.0 + (tool_hole_XY_dist) ** 2)
tool_XY_pos_reward = 1 - torch.tanh(5*tool_hole_XY_dist)
tool_hole_surf_dist = torch.norm(tool_pos - hole_surf_pos, p=2, dim=-1)
# tool_surf_pos_reward = 1.0 / (1.0 + (tool_hole_surf_dist) ** 2)
tool_surf_pos_reward = 1 - torch.tanh(8*tool_hole_surf_dist)
# 2nd reward: tool rotation
# tool_rot_error = torch.norm(tool_rot - tool_ref_rot, p=2, dim=-1)
tool_rot_error = torch.norm(tool_vector - ref_vector, p=2, dim=-1)
# tool_rot_reward = 1.0 / (1.0 + (tool_rot_error) ** 2)
tool_rot_reward = 1 - torch.tanh(3*tool_rot_error)
self.rot_error = tool_roll_error + tool_pitch_error
# 3rd reward: pegging in when tool is above the hole
tool_hole_Z_dist = torch.abs(tool_pos[:,2] - hole_pos[:,2])
# tool_pegging_reward = 1.0 / (1.0 + (tool_hole_Z_dist) ** 2)
tool_pegging_reward = 1 - torch.tanh(6*tool_hole_Z_dist)
# 4th reward: tool hole XYZ position
tool_hole_dist = torch.norm(tool_pos - hole_pos, p=2, dim=-1)
tool_target_dist = torch.norm(tool_pos - hole_target_pos, p=2, dim=-1)
# tool_pos_reward = 1.0 / (1.0 + (tool_hole_dist) ** 2)
tool_pos_reward = 1 - torch.tanh(5*tool_hole_dist)
finger_rot_error = torch.norm(finger_rot - finger_rot_ref, p=2, dim=-1)
finger_rot_reward = 1.0 / (1.0 + (finger_rot_error) ** 2)
finger_XY_pos_dist = torch.norm(finger_pos[:,0:2] - hole_pos[:,0:2], p=2, dim=-1)
finger_pos_reward = 1 - torch.tanh(5*finger_XY_pos_dist)
# 1st penalty: action
action_penalty = torch.sum(self.actions[:,0:7] ** 2, dim=-1)
action_penalty = 1 - 1.0 / (1.0 + action_penalty)
finger_vel_penalty = torch.tanh(20*torch.abs(norm_finger_vel-0.1))
# tool_rot_penalty = 1 - 1.0 / (1.0 + (tool_rot_error) ** 2)
# tool_pos_penalty = 1 - 1.0 / (1.0 + (tool_hole_dist) ** 2)
# final cumulative reward
# final_reward = 5*tool_XY_pos_reward + 5*tool_rot_reward + 2*tool_pegging_reward- 0.001*action_penalty
# final_reward = 10*tool_surf_pos_reward + 5*tool_rot_reward + 0*tool_hole_XY_dist- 0.001*action_penalty - 1.0*tool_rot_penalty - 1.0*tool_pos_penalty
# final_reward = torch.where(tool_hole_surf_dist<0.05, 10*tool_pos_reward + 5*tool_rot_reward- 0.001*action_penalty, final_reward)
# final_reward = torch.where(tool_hole_dist<0.1, 1*tool_pos_reward + 3*tool_rot_reward , 3*tool_pos_reward + 1*tool_rot_reward)
# final_reward = 2*tool_surf_pos_reward + 2*tool_rot_reward + 0*finger_rot_reward - 0.001*action_penalty
# final_reward = torch.where(tool_surf_pos_reward<0.1, 2*tool_pos_reward + 2*tool_rot_reward + 0*finger_rot_reward + 2*tool_pegging_reward-0.001*action_penalty, final_reward)
final_reward = 3.5*tool_XY_pos_reward + 1.48*tool_roll_pitch_reward- 0.001*action_penalty + 2.0*tool_pegging_reward
final_reward = torch.where((self.rot_error)<0.08, final_reward+0.5, final_reward)
final_reward = torch.where((self.rot_error)>0.2, final_reward-1, final_reward)
final_reward = torch.where(tool_hole_Z_dist>0.15, final_reward-1, final_reward)
final_reward = torch.where(tool_hole_Z_dist<0.05, final_reward+0.1, final_reward)
final_reward = torch.where(tool_hole_XY_dist<0.05, final_reward+0.5, final_reward)
final_reward = torch.where(tool_hole_XY_dist>0.1, final_reward-10, final_reward)
final_reward = torch.where(norm_finger_vel>0.15, final_reward-1, final_reward)
# amplify different sub-rewards w.r.t. conditions
# final_reward = torch.where(tool_hole_XY_dist>=0.005, final_reward + 2*tool_XY_pos_reward, final_reward) # tool-hole XY position
# final_reward = torch.where(tool_rot_error > 0.05, final_reward + 2*tool_rot_reward, final_reward) # tool rotation position
# final_reward = torch.where(torch.logical_and(tool_hole_XY_dist<0.05, tool_rot_error<0.05), final_reward + 10*tool_pegging_reward+2*tool_rot_reward, final_reward) # tool-hole Z position
# final_reward = torch.where(torch.logical_and(tool_hole_surf_dist<0.05, tool_rot_error<0.06),
# 10*tool_pos_reward + 5*tool_rot_reward + 2*tool_pegging_reward- 0.001*action_penalty,
# final_reward) # tool-hole Z position
# extra bonus/penalty cases:
# final_reward = torch.where(tool_hole_XY_dist<=0.01, final_reward+0.1, final_reward) # tool-hole XY position bonus
# final_reward = torch.where(tool_rot_error <0.1, final_reward+0.01, final_reward)
# final_reward = torch.where(tool_hole_XY_dist <0.005, final_reward+0.01, final_reward)
# final_reward = torch.where(tool_hole_Z_dist <0.1, final_reward+0.02, final_reward)
# final_reward = 10*tool_pos_reward + 4*tool_rot_reward
# final_reward = torch.where(tool_hole_XY_dist>0.1, 5.0*tool_pos_reward + 1.0*tool_rot_reward, 1.0*tool_pos_reward + 5.0*tool_rot_reward)
# final_reward = torch.where(tool_rot_error<0.1, final_reward+2*tool_pos_reward, final_reward)
# final_reward = torch.where(tool_hole_XY_dist<0.05, final_reward+5*tool_rot_reward, final_reward)
# final_reward = torch.where(tool_rot_error <0.1, final_reward+0.2, final_reward)
# final_reward = torch.where(tool_hole_XY_dist <0.1, final_reward+0.5, final_reward)
# final_reward = torch.where(torch.logical_and(tool_hole_Z_dist <0.15, tool_hole_XY_dist <0.1), final_reward+1, final_reward)
# final_reward = torch.where(torch.logical_and(tool_hole_XY_dist<=0.005, tool_hole_Z_dist<=0.005), final_reward+10000, final_reward) # task complete
final_reward = torch.where(tool_target_dist<0.01, final_reward+100, final_reward) # task complete
final_reward = torch.where(torch.isnan(final_reward), torch.zeros_like(final_reward), final_reward) # task complete
# trigger to determine if job is done
self.is_pegged = torch.where(tool_target_dist<0.01, torch.ones_like(final_reward), torch.zeros_like(final_reward)) # task complete
self.rew_buf[:] = final_reward
# print("hole_Z_pos", hole_pos[:2])
# print("tool_Z_pos", tool_pos[:2])
# print("tool_hole_XY_dist", tool_hole_XY_dist)
# print("tool_hole_Z_dist", tool_hole_Z_dist)
# print("tool_target_dist", tool_target_dist)
# print("hole_surf_pos", hole_surf_pos)
# print("norm_finger_vel", norm_finger_vel)
# print("tool_rot", tool_rot)
# print("tool_rot_error", self.rot_error )
# print("tool_ref_rot", tool_ref_rot)
# print("hole_rot", hole_rot)
# print("finger_rot", finger_rot)
# finger_rot_ref: 0.0325, -0.3824, 0.9233, -0.0135
# 0.0 0.92388 0.3826 0
# hole_pos tensor([[ 1.5000, 0.0000, 0.3800], [-1.5000, 0.0000, 0.3800]], device='cuda:0')
# tool_hole_Z_dist tensor([0.0820, 0.0789], device='cuda:0')
# tool_rot_error tensor([0.0629, 0.0621], device='cuda:0')
# tool_hole_XY_dist tensor([0.0012, 0.0037], device='cuda:0')
# tool_rot_error tensor([0.7979, 0.7810, 0.7889, 0.7811], device='cuda:0')
# tool_hole_XY_dist tensor([0.0536, 0.0585, 0.0378, 0.0451], device='cuda:0')
# tool_hole_Z_dist tensor([0.0343, 0.0353, 0.0368, 0.0350], device='cuda:0')
# tool_hole_dist tensor([0.0636, 0.0683, 0.0528, 0.0571], device='cuda:0')
self.episode_sums["tool_hole_XY_dist"] += tool_hole_XY_dist
self.episode_sums["tool_hole_Z_dist"] += tool_hole_Z_dist
self.episode_sums["tool_hole_dist"] += tool_hole_dist
self.episode_sums["tool_rot_error"] += tool_roll_error+tool_pitch_error
# self.episode_sums["tool_X_pos"] += tool_pos[:,0]
# self.episode_sums["tool_Y_pos"] += tool_pos[:,1]
# self.episode_sums["tool_Z_pos"] += tool_pos[:,2]
# self.episode_sums["tool_rot"] += tool_rot
self.episode_sums["peg_rate"] += self.is_pegged
self.episode_sums["norm_finger_vel"] += norm_finger_vel
self.episode_sums["rewards"] += final_reward
def is_done(self) -> None:
if not self.is_test:
# reset if tool is pegged in hole
# self.reset_buf = torch.where(self.is_pegged==1, torch.ones_like(self.reset_buf), self.reset_buf)
# reset if tool is below the table and not pegged in hole
# self.reset_buf = torch.where(self.tool_pos[:,2] < 0.3, torch.ones_like(self.reset_buf), self.reset_buf)
#
# self.reset_buf = torch.where(torch.logical_and(self.tool_pos[:,2] < 0.43, self.rot_error>1.5), torch.ones_like(self.reset_buf), self.reset_buf)
# reset if max length reached
self.reset_buf = torch.where(self.progress_buf >= self._max_episode_length - 1, torch.ones_like(self.reset_buf), self.reset_buf)
else:
self.reset_buf = torch.where(self.progress_buf >= self._max_episode_length - 1, torch.ones_like(self.reset_buf), self.reset_buf)
# self.reset_buf = torch.where(self.is_pegged==1, torch.ones_like(self.reset_buf), self.reset_buf)
| 30,444 | Python | 49.405629 | 195 | 0.612633 |
zhehuazhou/ai-cps-robotics-benchmark/Gym_Envs/Tasks/Franka_Door_Open.py | from omniisaacgymenvs.tasks.base.rl_task import RLTask
from Models.Franka.Franka import Franka
from Models.Franka.Franka_view import FrankaView
from Models.door_open.door import Door
from Models.door_open.door_view import DoorView
from omni.isaac.core.objects import DynamicSphere
from omni.isaac.core.prims import RigidPrim, RigidPrimView
from omni.isaac.core.utils.prims import get_prim_at_path
from omni.isaac.core.utils.stage import get_current_stage
from omni.isaac.core.utils.torch.transformations import *
from omni.isaac.core.utils.torch.rotations import *
from omni.isaac.cloner import Cloner
import numpy as np
import torch
import math
from pxr import Usd, UsdGeom
class FrankaDoorOpenTask(RLTask):
def __init__(
self,
name,
sim_config,
env,
offset=None
) -> None:
self._sim_config = sim_config
self._cfg = sim_config.config
self._task_cfg = sim_config.task_config
self._num_envs = self._task_cfg["env"]["numEnvs"]
self._env_spacing = self._task_cfg["env"]["envSpacing"]
self._max_episode_length = self._task_cfg["env"]["episodeLength"]
self.action_scale = self._task_cfg["env"]["actionScale"]
self.start_position_noise = self._task_cfg["env"]["startPositionNoise"]
self.start_rotation_noise = self._task_cfg["env"]["startRotationNoise"]
self.dof_vel_scale = self._task_cfg["env"]["dofVelocityScale"]
self.dist_reward_scale = self._task_cfg["env"]["distRewardScale"]
self.rot_reward_scale = self._task_cfg["env"]["rotRewardScale"]
self.around_handle_reward_scale = self._task_cfg["env"]["aroundHandleRewardScale"]
self.open_reward_scale = self._task_cfg["env"]["openRewardScale"]
self.finger_dist_reward_scale = self._task_cfg["env"]["fingerDistRewardScale"]
self.action_penalty_scale = self._task_cfg["env"]["actionPenaltyScale"]
self.finger_close_reward_scale = self._task_cfg["env"]["fingerCloseRewardScale"]
self.distX_offset = 0.04
self.dt = 1/60.
self._num_observations = 28
self._num_actions = 9
# Flag for testing
self.is_test = False
self.initial_test_value = None
self.is_action_noise = False
RLTask.__init__(self, name, env)
# Extra info for TensorBoard
self.extras = {}
torch_zeros = lambda: torch.zeros(self.num_envs, dtype=torch.float, device=self.device, requires_grad=False)
self.episode_sums = {"door_yaw_deg": torch_zeros(), "grasp_handle_dist": torch_zeros(), "handle_yaw_deg": torch_zeros(),
"handle_pos_error": torch_zeros(), "open_rate": torch_zeros(), "rewards": torch_zeros(), "handle_yaw_error": torch_zeros()}
return
def set_up_scene(self, scene) -> None:
franka_translation = torch.tensor([0.5, 0.0, 0.0])
self.get_franka(franka_translation)
self.get_door()
super().set_up_scene(scene)
# Add Franka
self._frankas = FrankaView(prim_paths_expr="/World/envs/.*/franka", name="franka_view")
# Add door
self._door = DoorView(prim_paths_expr="/World/envs/.*/door/door", name="door_view")
scene.add(self._frankas)
scene.add(self._frankas._hands)
scene.add(self._frankas._lfingers)
scene.add(self._frankas._rfingers)
scene.add(self._door)
scene.add(self._door._handle)
self.init_data()
return
def get_franka(self, translation):
franka = Franka(prim_path=self.default_zero_env_path + "/franka", name="franka", translation = translation)
self._sim_config.apply_articulation_settings("franka", get_prim_at_path(franka.prim_path), self._sim_config.parse_actor_config("franka"))
def get_door(self):
door = Door(prim_path=self.default_zero_env_path + "/door", name="door")
self._sim_config.apply_articulation_settings("door", get_prim_at_path(door.prim_path), self._sim_config.parse_actor_config("door"))
# Set as testing mode
def set_as_test(self):
self.is_test = True
# Set action noise
def set_action_noise(self):
self.is_action_noise = True
# Set initial test values for testing mode
def set_initial_test_value(self, value):
# for ball pushing: initial x,y positions of the ball
self.initial_test_value = value
def init_data(self) -> None:
def get_env_local_pose(env_pos, xformable, device):
"""Compute pose in env-local coordinates"""
world_transform = xformable.ComputeLocalToWorldTransform(0)
world_pos = world_transform.ExtractTranslation()
world_quat = world_transform.ExtractRotationQuat()
px = world_pos[0] - env_pos[0]
py = world_pos[1] - env_pos[1]
pz = world_pos[2] - env_pos[2]
qx = world_quat.imaginary[0]
qy = world_quat.imaginary[1]
qz = world_quat.imaginary[2]
qw = world_quat.real
return torch.tensor([px, py, pz, qw, qx, qy, qz], device=device, dtype=torch.float)
stage = get_current_stage()
hand_pose = get_env_local_pose(self._env_pos[0], UsdGeom.Xformable(stage.GetPrimAtPath("/World/envs/env_0/franka/panda_link7")), self._device)
lfinger_pose = get_env_local_pose(
self._env_pos[0], UsdGeom.Xformable(stage.GetPrimAtPath("/World/envs/env_0/franka/panda_leftfinger")), self._device
)
rfinger_pose = get_env_local_pose(
self._env_pos[0], UsdGeom.Xformable(stage.GetPrimAtPath("/World/envs/env_0/franka/panda_rightfinger")), self._device
)
# finger pos
finger_pose = torch.zeros(7, device=self._device)
finger_pose[0:3] = (lfinger_pose[0:3] + rfinger_pose[0:3]) / 2.0
finger_pose[3:7] = lfinger_pose[3:7]
hand_pose_inv_rot, hand_pose_inv_pos = (tf_inverse(hand_pose[3:7], hand_pose[0:3]))
# franka grasp local pose
grasp_pose_axis = 1
franka_local_grasp_pose_rot, franka_local_pose_pos = tf_combine(hand_pose_inv_rot, hand_pose_inv_pos, finger_pose[3:7], finger_pose[0:3])
franka_local_pose_pos += torch.tensor([0, 0.04, 0], device=self._device)
self.franka_local_grasp_pos = franka_local_pose_pos.repeat((self._num_envs, 1))
self.franka_local_grasp_rot = franka_local_grasp_pose_rot.repeat((self._num_envs, 1))
# XXX assume to be the local pos of the handle
door_local_handle_pose = torch.tensor([-0.1, -0.23, 0.81, 1.0, 0.0, 0.0, 0.0], device=self._device)
self.door_local_handle_pos = door_local_handle_pose[0:3].repeat((self._num_envs, 1))
self.door_local_handle_rot = door_local_handle_pose[3:7].repeat((self._num_envs, 1))
self.gripper_forward_axis = torch.tensor([0, 0, 1], device=self._device, dtype=torch.float).repeat((self._num_envs, 1))
self.gripper_up_axis = torch.tensor([0, 1, 0], device=self._device, dtype=torch.float).repeat((self._num_envs, 1))
self.door_inward_axis = torch.tensor([-1, 0, 0], device=self._device, dtype=torch.float).repeat((self._num_envs, 1))
self.door_up_axis = torch.tensor([0, 0, 1], device=self._device, dtype=torch.float).repeat((self._num_envs, 1))
self.franka_default_dof_pos = torch.tensor(
[1.157, -1.066, -0.155, -2.239, -1.841, 1.003, 0.469, 0.035, 0.035], device=self._device
)
self.actions = torch.zeros((self._num_envs, self.num_actions), device=self._device)
def get_observations(self) -> dict:
# Franka
hand_pos, hand_rot = self._frankas._hands.get_world_poses(clone=False)
self.door_pos, self.door_rot = self._door.get_world_poses(clone=False)
franka_dof_pos = self._frankas.get_joint_positions(clone=False)
franka_dof_vel = self._frankas.get_joint_velocities(clone=False)
self.franka_dof_pos = franka_dof_pos
self.door_dof_pos = self._door.get_joint_positions(clone=False)
self.door_dor_vel = self._door.get_joint_velocities(clone=False)
self.franka_grasp_rot, self.franka_grasp_pos, self.door_handle_rot, self.door_handle_pos = self.compute_grasp_transforms(
hand_rot,
hand_pos,
self.franka_local_grasp_rot,
self.franka_local_grasp_pos,
self.door_rot,
self.door_pos,
self.door_local_handle_rot,
self.door_local_handle_pos,
)
self.franka_lfinger_pos, self.franka_lfinger_rot = self._frankas._lfingers.get_world_poses(clone=False)
self.franka_rfinger_pos, self.franka_rfinger_rot = self._frankas._lfingers.get_world_poses(clone=False)
# handle
self.handle_pos, self.handle_rot = self._door._handle.get_world_poses(clone=False)
self.handle_pos[:,1] = self.handle_pos[:,1] - 0.3 # fix hand-point y-axis error
# position error: from franka grasp to door handle
grasp_handle_pos_error = self.handle_pos - self.franka_grasp_pos
# grasp_handle_pos_error = self.handle_pos - (self.franka_lfinger_pos + self.franka_rfinger_pos)/2
dof_pos_scaled = (
2.0
* (franka_dof_pos - self.franka_dof_lower_limits)
/ (self.franka_dof_upper_limits - self.franka_dof_lower_limits)
- 1.0
)
self.obs_buf = torch.cat(
(
dof_pos_scaled,
franka_dof_vel * self.dof_vel_scale,
self.handle_pos,
self.handle_rot,
grasp_handle_pos_error,
# self.handle_pos,
# self.handle_rot,
# self.location_ball_pos
# self.cabinet_dof_pos[:, 3].unsqueeze(-1),
# self.cabinet_dof_vel[:, 3].unsqueeze(-1),
),
dim=-1,
)
observations = {
self._frankas.name: {
"obs_buf": self.obs_buf
}
}
return observations
def compute_grasp_transforms(
self,
hand_rot,
hand_pos,
franka_local_grasp_rot,
franka_local_grasp_pos,
door_rot,
door_pos,
door_local_handle_rot,
door_local_handle_pos,
):
global_franka_rot, global_franka_pos = tf_combine(
hand_rot, hand_pos, franka_local_grasp_rot, franka_local_grasp_pos
)
global_door_rot, global_door_pos = tf_combine(
door_rot, door_pos, door_local_handle_rot, door_local_handle_pos
)
return global_franka_rot, global_franka_pos, global_door_rot, global_door_pos
def pre_physics_step(self, actions) -> None:
if not self._env._world.is_playing():
return
reset_env_ids = self.reset_buf.nonzero(as_tuple=False).squeeze(-1)
if len(reset_env_ids) > 0:
self.reset_idx(reset_env_ids)
self.actions = actions.clone().to(self._device)
# if action noise
if self.is_action_noise is True:
# Gaussian white noise with 0.01 variance
self.actions = self.actions + (0.5)*torch.randn_like(self.actions)
targets = self.franka_dof_targets + self.franka_dof_speed_scales * self.dt * self.actions * self.action_scale
self.franka_dof_targets[:] = tensor_clamp(targets, self.franka_dof_lower_limits, self.franka_dof_upper_limits)
env_ids_int32 = torch.arange(self._frankas.count, dtype=torch.int32, device=self._device)
self._frankas.set_joint_position_targets(self.franka_dof_targets, indices=env_ids_int32)
def reset_idx(self, env_ids):
indices = env_ids.to(dtype=torch.int32)
num_indices = len(indices)
# reset franka
pos = torch.clamp(
self.franka_default_dof_pos.unsqueeze(0)
+ 0.25 * (torch.rand((len(env_ids), self.num_franka_dofs), device=self._device) - 0.5),
self.franka_dof_lower_limits,
self.franka_dof_upper_limits,
)
dof_pos = torch.zeros((num_indices, self._frankas.num_dof), device=self._device)
dof_vel = torch.zeros((num_indices, self._frankas.num_dof), device=self._device)
dof_pos[:, :] = pos
self.franka_dof_targets[env_ids, :] = pos
self.franka_dof_pos[env_ids, :] = pos
self._frankas.set_joint_position_targets(self.franka_dof_targets[env_ids], indices=indices)
self._frankas.set_joint_positions(dof_pos, indices=indices)
self._frankas.set_joint_velocities(dof_vel, indices=indices)
if not self.is_test:
# reset door: only 1 joint
# reset door positions: x: [-0.1,0.1], y:[-0.4,0.4]
self.new_door_pos = self.default_door_pos.clone().detach()
self.new_door_pos[:,0] = self.default_door_pos[:,0] + (0.05 + 0.05) * torch.rand(self._num_envs, device=self._device) -0.05
self.new_door_pos[:,1] = self.default_door_pos[:,1] + (0.1 + 0.1) * torch.rand(self._num_envs, device=self._device) -0.1
self._door.set_world_poses(self.new_door_pos[env_ids], self.default_door_rot[env_ids], indices = indices)
else:
self.new_door_pos = self.default_door_pos.clone().detach()
self.new_door_pos[:,0] = self.default_door_pos[:,0] + self.initial_test_value[0]
self.new_door_pos[:,1] = self.default_door_pos[:,1] + self.initial_test_value[1]
self._door.set_world_poses(self.new_door_pos[env_ids], self.default_door_rot[env_ids], indices = indices)
# reset door joints
door_pos = torch.zeros((num_indices, 1), device=self._device)
door_vel = torch.zeros((num_indices, 1), device=self._device)
self._door.set_joint_positions(door_pos, indices=indices)
self._door.set_joint_velocities(door_vel, indices=indices)
self._door.set_joint_position_targets(self.door_dof_targets[env_ids], indices=indices)
# bookkeeping
self.reset_buf[env_ids] = 0
self.progress_buf[env_ids] = 0
# fill extras
self.extras["episode"] = {}
for key in self.episode_sums.keys():
if key == "open_rate":
self.extras["episode"][key] = torch.mean(self.episode_sums[key][env_ids])
else:
self.extras["episode"][key] = torch.mean(self.episode_sums[key][env_ids]) / self._max_episode_length
self.episode_sums[key][env_ids] = 0.0
def post_reset(self):
# Franka
self.num_franka_dofs = self._frankas.num_dof
self.franka_dof_pos = torch.zeros((self.num_envs, self.num_franka_dofs), device=self._device)
dof_limits = self._frankas.get_dof_limits()
self.franka_dof_lower_limits = dof_limits[0, :, 0].to(device=self._device)
self.franka_dof_upper_limits = dof_limits[0, :, 1].to(device=self._device)
self.franka_dof_speed_scales = torch.ones_like(self.franka_dof_lower_limits)
self.franka_dof_speed_scales[self._frankas.gripper_indices] = 0.1
self.franka_dof_targets = torch.zeros(
(self._num_envs, self.num_franka_dofs), dtype=torch.float, device=self._device
)
# Door
self.door_dof_targets = torch.zeros(
(self._num_envs, 1), dtype=torch.float, device=self._device
)
self.default_door_pos, self.default_door_rot = self._door.get_world_poses()
# randomize all envs
indices = torch.arange(self._num_envs, dtype=torch.int64, device=self._device)
self.reset_idx(indices)
def calculate_metrics(self) -> None:
# info extraction
# env
num_envs = self._num_envs
# Franka
joint_positions = self.franka_dof_pos
gripper_forward_axis = self.gripper_forward_axis
gripper_up_axis = self.gripper_up_axis
franka_grasp_pos, franka_grasp_rot = self.franka_grasp_pos, self.franka_grasp_rot
franka_lfinger_pos, franka_lfinger_rot = self.franka_lfinger_pos, self.franka_lfinger_rot
franka_rfinger_pos, franka_rfinger_rot = self.franka_rfinger_pos, self.franka_rfinger_rot
actions = self.actions
finger_pos = (franka_lfinger_pos + franka_rfinger_pos)/2
finger_rot = (franka_lfinger_pos + franka_rfinger_pos)/2
# door
door_inward_axis = self.door_inward_axis
door_up_axis = self.door_up_axis
door_dof_pos = self.door_dof_pos
door_pos, door_rot = self.door_pos, self.door_rot
# handle
handle_pos, handle_rot = self.handle_pos, self.handle_rot
# handle_pos[:,1] = handle_pos[:,1] - 0.3 # fix hand-point y-axis error
handle_local_pos, handle_local_rot = self._door._handle.get_local_poses()
# preprocessing
# distance from grasp to handle
grasp_handle_dist = torch.norm(finger_pos - handle_pos, p=2, dim=-1)
# distance of each finger to the handle along Z-axis
lfinger_Z_dist = torch.abs(franka_lfinger_pos[:, 2] - handle_pos[:, 2])
rfinger_Z_dist = torch.abs(franka_rfinger_pos[:, 2] - handle_pos[:, 2])
# how far the door has been opened out
# quaternions to euler angles
door_yaw = torch.atan2(2.0*(door_rot[:,0]*door_rot[:,3] + door_rot[:,1]*door_rot[:,2]), 1.0-2.0*(door_rot[:,2]*door_rot[:,2]+door_rot[:,3]*door_rot[:,3]))
handle_yaw = torch.atan2(2.0*(handle_rot[:,0]*handle_rot[:,3] + handle_rot[:,1]*handle_rot[:,2]), 1.0-2.0*(handle_rot[:,2]*handle_rot[:,2]+handle_rot[:,3]*handle_rot[:,3]))
door_ref_yaw = torch.deg2rad(torch.tensor([60], device=self._device))
door_yaw_error = torch.abs(door_ref_yaw - handle_yaw)
self.door_yaw_error = door_yaw_error.clone().detach()
# handle destination if opened
handle_ref_pos = handle_pos.clone().detach()
# target_open_deg = door_ref_yaw*torch.ones((num_envs,1), device=self._device) # open the door by 60 degrees
# target_open_rad = math.radians(60)
handle_ref_pos[:,0] = handle_ref_pos[:,0]*torch.cos(door_ref_yaw) + handle_ref_pos[:,1]*torch.sin(door_ref_yaw)
handle_ref_pos[:,1] = -handle_ref_pos[:,0]*torch.sin(door_ref_yaw) + handle_ref_pos[:,1]*torch.cos(door_ref_yaw)
self.handle_pos_error = torch.norm(handle_ref_pos - handle_pos, p=2, dim=-1)
# gripper direction alignment
axis1 = tf_vector(franka_grasp_rot, gripper_forward_axis)
axis2 = tf_vector(handle_rot, door_inward_axis)
axis3 = tf_vector(franka_grasp_rot, gripper_up_axis)
axis4 = tf_vector(handle_rot, door_up_axis)
dot1 = torch.bmm(axis1.view(num_envs, 1, 3), axis2.view(num_envs, 3, 1)).squeeze(-1).squeeze(-1) # alignment of forward axis for gripper
dot2 = torch.bmm(axis3.view(num_envs, 1, 3), axis4.view(num_envs, 3, 1)).squeeze(-1).squeeze(-1) # alignment of up axis for gripper
# reward functions
# 1st rewards: distance from hand to the drawer
grasp_dist_reward = 1.0 / (1.0 + grasp_handle_dist ** 2)
grasp_dist_reward *= grasp_dist_reward
grasp_dist_reward = torch.where(grasp_handle_dist <= 0.02, grasp_dist_reward * 2, grasp_dist_reward)
# 2nd reward for matching the orientation of the hand to the drawer (fingers wrapped)
rot_reward = 0.5 * (torch.sign(dot1) * dot1 ** 2 + torch.sign(dot2) * dot2 ** 2)
# 3rd reward: bonus if left finger is above the drawer handle and right below
around_handle_reward = torch.zeros_like(rot_reward)
around_handle_reward = torch.where(self.franka_lfinger_pos[:, 2] > handle_pos[:, 2],
torch.where(self.franka_rfinger_pos[:, 2] < handle_pos[:, 2],
around_handle_reward + 0.5, around_handle_reward), around_handle_reward)
# 4th reward: distance of each finger from the handle
finger_dist_reward = torch.zeros_like(rot_reward)
finger_dist_reward = torch.where(franka_lfinger_pos[:, 2] > handle_pos[:, 2],
torch.where(franka_rfinger_pos[:, 2] < handle_pos[:, 2],
(0.04 - lfinger_Z_dist) + (0.04 - rfinger_Z_dist), finger_dist_reward), finger_dist_reward)
# 5th reward: finger closeness
finger_close_reward = torch.zeros_like(rot_reward)
finger_close_reward = torch.where(grasp_handle_dist <=0.03, (0.04 - joint_positions[:, 7]) + (0.04 - joint_positions[:, 8]), finger_close_reward)
# 6th reward: how far the door has been opened out
# instead of using rotation, may use pos as reference
open_reward = (1.0 / (1.0 + door_yaw_error ** 2)) * around_handle_reward + handle_yaw
# open_reward = (1.0 / (1.0 + self.handle_pos_error)) * around_handle_reward
# 1st penalty
action_penalty = torch.sum(actions ** 2, dim=-1)
final_reward = 2.0 * grasp_dist_reward + 0.5 * rot_reward + 10.0 * around_handle_reward + 70.0 * open_reward + \
100.0 * finger_dist_reward+ 10.0 * finger_close_reward - 0.01 * action_penalty
# bonus for opening door properly
final_reward = torch.where(door_yaw_error < 0.7, final_reward + 0.5, final_reward)
final_reward = torch.where(door_yaw_error < 0.5, final_reward + around_handle_reward, final_reward)
final_reward = torch.where(door_yaw_error < 0.2, final_reward + (2.0 * around_handle_reward), final_reward)
# in case: Nan value occur
final_reward = torch.where(torch.isnan(final_reward), torch.zeros_like(final_reward), final_reward)
self.rew_buf[:] = final_reward
# self.rew_buf[:] = torch.rand(self._num_envs)
# if the door is opened to ref position -> task complete
self.is_opened = torch.where(torch.rad2deg(handle_yaw)>=70, torch.ones_like(final_reward), torch.zeros_like(final_reward))
self.episode_sums["door_yaw_deg"] += torch.rad2deg(door_yaw)
self.episode_sums["handle_yaw_deg"] += torch.rad2deg(handle_yaw)
self.episode_sums["handle_pos_error"] += self.handle_pos_error
self.episode_sums["handle_yaw_error"] += door_yaw_error
self.episode_sums["grasp_handle_dist"] += grasp_handle_dist
self.episode_sums["open_rate"] += self.is_opened
self.episode_sums["rewards"] += final_reward
# print("handle_pos", handle_pos)
# print("handle_rot", handle_rot)
# print("door_pos", door_pos)
# print("door_rot", door_rot)
# print("handle_local_pos", handle_local_pos)
# print("handle_local_rot", handle_local_rot)
# print("grasp_handle_dist", grasp_handle_dist)
# print("door_yaw", door_yaw)
def is_done(self) -> None:
if not self.is_test:
# reset if door is fully opened
# self.reset_buf = torch.where(self.is_opened==1, torch.ones_like(self.reset_buf), self.reset_buf)
# reset if max length reached
self.reset_buf = torch.where(self.progress_buf >= self._max_episode_length - 1, torch.ones_like(self.reset_buf), self.reset_buf)
else:
self.reset_buf = torch.where(self.progress_buf >= self._max_episode_length - 1, torch.ones_like(self.reset_buf), self.reset_buf)
# self.reset_buf = torch.where(self.is_opened==1, torch.ones_like(self.reset_buf), self.reset_buf)
| 23,580 | Python | 45.510848 | 180 | 0.611196 |
zhehuazhou/ai-cps-robotics-benchmark/Gym_Envs/Tasks/Franka_Ball_Pushing.py | from omniisaacgymenvs.tasks.base.rl_task import RLTask
from Models.Franka.Franka import Franka
from Models.Franka.Franka_view import FrankaView
from Models.ball_pushing.table import Table
from omni.isaac.core.objects import DynamicSphere
from omni.isaac.core.prims import RigidPrim, RigidPrimView
from omni.isaac.core.utils.prims import get_prim_at_path
from omni.isaac.core.utils.stage import get_current_stage
from omni.isaac.core.utils.torch.transformations import *
from omni.isaac.core.utils.torch.rotations import *
from omni.isaac.cloner import Cloner
import numpy as np
import torch
import math
from pxr import Usd, UsdGeom
class FrankaBallPushingTask(RLTask):
def __init__(
self,
name,
sim_config,
env,
offset=None
) -> None:
self._sim_config = sim_config
self._cfg = sim_config.config
self._task_cfg = sim_config.task_config
self._num_envs = self._task_cfg["env"]["numEnvs"]
self._env_spacing = self._task_cfg["env"]["envSpacing"]
self._max_episode_length = self._task_cfg["env"]["episodeLength"]
self.action_scale = self._task_cfg["env"]["actionScale"]
self.start_position_noise = self._task_cfg["env"]["startPositionNoise"]
self.start_rotation_noise = self._task_cfg["env"]["startRotationNoise"]
self.dof_vel_scale = self._task_cfg["env"]["dofVelocityScale"]
self.dist_reward_scale = self._task_cfg["env"]["distRewardScale"]
self.rot_reward_scale = self._task_cfg["env"]["rotRewardScale"]
self.around_handle_reward_scale = self._task_cfg["env"]["aroundHandleRewardScale"]
self.open_reward_scale = self._task_cfg["env"]["openRewardScale"]
self.finger_dist_reward_scale = self._task_cfg["env"]["fingerDistRewardScale"]
self.action_penalty_scale = self._task_cfg["env"]["actionPenaltyScale"]
self.finger_close_reward_scale = self._task_cfg["env"]["fingerCloseRewardScale"]
self.ball_radius = self._task_cfg["env"]["ballRadius"]
self.ball_initial_position = self._task_cfg["env"]["ballInitialPosition"]
self.ball_initial_orientation = self._task_cfg["env"]["ballInitialOrientation"]
# self.ball_initial_position[0] = (0.1 + 0.1) * np.random.rand(1) -0.1
# self.ball_initial_position[1] = (0.2 + 0.2) * np.random.rand(1) -0.2
# initial_x = (0.1 + 0.1) * torch.rand(self._num_envs) -0.1
# initial_y = (0.2 + 0.2) * torch.rand(self._num_envs) -0.2
self.distX_offset = 0.04
self.dt = 1/60.
self._num_observations = 30
self._num_actions = 9
# Flag for testing
self.is_test = False
self.initial_test_value = None
self.is_action_noise = False
RLTask.__init__(self, name, env)
# Extra info for TensorBoard
self.extras = {}
torch_zeros = lambda: torch.zeros(self.num_envs, dtype=torch.float, device=self.device, requires_grad=False)
self.episode_sums = {"success_rate": torch_zeros(), "ball_hole_XY_dist": torch_zeros()}
return
def set_up_scene(self, scene) -> None:
franka_translation = torch.tensor([0.6, 0.0, 0.0])
self.get_franka(franka_translation)
self.get_table()
self.get_ball()
super().set_up_scene(scene)
self._frankas = FrankaView(prim_paths_expr="/World/envs/.*/franka", name="franka_view")
# Add ball
self._ball = RigidPrimView(prim_paths_expr="/World/envs/.*/ball", name="ball_view", reset_xform_properties=False)
# Add location_ball
self._location_ball = RigidPrimView(prim_paths_expr="/World/envs/.*/table/table/location_ball", name="location_ball_view", reset_xform_properties=False)
scene.add(self._frankas)
scene.add(self._frankas._hands)
scene.add(self._frankas._lfingers)
scene.add(self._frankas._rfingers)
scene.add(self._ball)
scene.add(self._location_ball)
self.init_data()
return
def get_franka(self, translation):
franka = Franka(prim_path=self.default_zero_env_path + "/franka", name="franka", translation = translation)
self._sim_config.apply_articulation_settings("franka", get_prim_at_path(franka.prim_path), self._sim_config.parse_actor_config("franka"))
def get_table(self):
table = Table(prim_path=self.default_zero_env_path + "/table", name="table")
self._sim_config.apply_articulation_settings("table", get_prim_at_path(table.prim_path), self._sim_config.parse_actor_config("table"))
def get_ball(self):
ball = DynamicSphere(
name = 'ball',
position=self.ball_initial_position,
orientation=self.ball_initial_orientation,
prim_path=self.default_zero_env_path + "/ball",
radius=self.ball_radius,
color=np.array([1, 0, 0]),
density = 100
)
self._sim_config.apply_articulation_settings("ball", get_prim_at_path(ball.prim_path), self._sim_config.parse_actor_config("ball"))
# Set as testing mode
def set_as_test(self):
self.is_test = True
# Set action noise
def set_action_noise(self):
self.is_action_noise = True
# Set initial test values for testing mode
def set_initial_test_value(self, value):
# for ball pushing: initial x,y positions of the ball
self.initial_test_value = value
def init_data(self) -> None:
def get_env_local_pose(env_pos, xformable, device):
"""Compute pose in env-local coordinates"""
world_transform = xformable.ComputeLocalToWorldTransform(0)
world_pos = world_transform.ExtractTranslation()
world_quat = world_transform.ExtractRotationQuat()
px = world_pos[0] - env_pos[0]
py = world_pos[1] - env_pos[1]
pz = world_pos[2] - env_pos[2]
qx = world_quat.imaginary[0]
qy = world_quat.imaginary[1]
qz = world_quat.imaginary[2]
qw = world_quat.real
return torch.tensor([px, py, pz, qw, qx, qy, qz], device=device, dtype=torch.float)
stage = get_current_stage()
hand_pose = get_env_local_pose(self._env_pos[0], UsdGeom.Xformable(stage.GetPrimAtPath("/World/envs/env_0/franka/panda_link7")), self._device)
lfinger_pose = get_env_local_pose(
self._env_pos[0], UsdGeom.Xformable(stage.GetPrimAtPath("/World/envs/env_0/franka/panda_leftfinger")), self._device
)
rfinger_pose = get_env_local_pose(
self._env_pos[0], UsdGeom.Xformable(stage.GetPrimAtPath("/World/envs/env_0/franka/panda_rightfinger")), self._device
)
finger_pose = torch.zeros(7, device=self._device)
finger_pose[0:3] = (lfinger_pose[0:3] + rfinger_pose[0:3]) / 2.0
finger_pose[3:7] = lfinger_pose[3:7]
hand_pose_inv_rot, hand_pose_inv_pos = (tf_inverse(hand_pose[3:7], hand_pose[0:3]))
grasp_pose_axis = 1
franka_local_grasp_pose_rot, franka_local_pose_pos = tf_combine(hand_pose_inv_rot, hand_pose_inv_pos, finger_pose[3:7], finger_pose[0:3])
franka_local_pose_pos += torch.tensor([0, 0.04, 0], device=self._device)
self.franka_local_grasp_pos = franka_local_pose_pos.repeat((self._num_envs, 1))
self.franka_local_grasp_rot = franka_local_grasp_pose_rot.repeat((self._num_envs, 1))
self.gripper_forward_axis = torch.tensor([0, 0, 1], device=self._device, dtype=torch.float).repeat((self._num_envs, 1))
self.gripper_up_axis = torch.tensor([0, 1, 0], device=self._device, dtype=torch.float).repeat((self._num_envs, 1))
self.franka_default_dof_pos = torch.tensor(
[1.157, -1.066, -0.155, -2.239, -1.841, 1.003, 0.469, 0.035, 0.035], device=self._device
)
self.actions = torch.zeros((self._num_envs, self.num_actions), device=self._device)
def get_observations(self) -> dict:
hand_pos, hand_rot = self._frankas._hands.get_world_poses(clone=False)
franka_dof_pos = self._frankas.get_joint_positions(clone=False)
franka_dof_vel = self._frankas.get_joint_velocities(clone=False)
self.franka_dof_pos = franka_dof_pos
# self.franka_grasp_rot, self.franka_grasp_pos, self.drawer_grasp_rot, self.drawer_grasp_pos = self.compute_grasp_transforms(
# hand_rot,
# hand_pos,
# self.franka_local_grasp_rot,
# self.franka_local_grasp_pos,
# drawer_rot,
# drawer_pos,
# self.drawer_local_grasp_rot,
# self.drawer_local_grasp_pos,
# )
self.franka_lfinger_pos, self.franka_lfinger_rot = self._frankas._lfingers.get_world_poses(clone=False)
self.franka_rfinger_pos, self.franka_rfinger_rot = self._frankas._lfingers.get_world_poses(clone=False)
# Ball
self.ball_pos, self.ball_rot = self._ball.get_world_poses(clone=False)
self.ball_vel = self._ball.get_velocities()
# hole-location ball
self.location_ball_pos, self.location_ball_rot = self._location_ball.get_world_poses(clone=False)
to_target = self.location_ball_pos - self.ball_pos
dof_pos_scaled = (
2.0
* (franka_dof_pos - self.franka_dof_lower_limits)
/ (self.franka_dof_upper_limits - self.franka_dof_lower_limits)
- 1.0
)
self.obs_buf = torch.cat(
(
dof_pos_scaled,
franka_dof_vel * self.dof_vel_scale,
self.ball_vel,
to_target,
self.ball_pos,
# self.location_ball_pos
# self.cabinet_dof_pos[:, 3].unsqueeze(-1),
# self.cabinet_dof_vel[:, 3].unsqueeze(-1),
),
dim=-1,
)
observations = {
self._frankas.name: {
"obs_buf": self.obs_buf
}
}
return observations
def pre_physics_step(self, actions) -> None:
if not self._env._world.is_playing():
return
reset_env_ids = self.reset_buf.nonzero(as_tuple=False).squeeze(-1)
if len(reset_env_ids) > 0:
self.reset_idx(reset_env_ids)
self.actions = actions.clone().to(self._device)
# if action noise
if self.is_action_noise is True:
# Gaussian white noise with 0.01 variance
self.actions = self.actions + (0.5)*torch.randn_like(self.actions)
targets = self.franka_dof_targets + self.franka_dof_speed_scales * self.dt * self.actions * self.action_scale
self.franka_dof_targets[:] = tensor_clamp(targets, self.franka_dof_lower_limits, self.franka_dof_upper_limits)
env_ids_int32 = torch.arange(self._frankas.count, dtype=torch.int32, device=self._device)
self.franka_dof_targets[:,7] = 0.015
self.franka_dof_targets[:,8] = 0.015
self._frankas.set_joint_position_targets(self.franka_dof_targets, indices=env_ids_int32)
def reset_idx(self, env_ids):
indices = env_ids.to(dtype=torch.int32)
num_indices = len(indices)
# reset franka
pos = tensor_clamp(
self.franka_default_dof_pos.unsqueeze(0)
+ 0.25 * (torch.rand((len(env_ids), self.num_franka_dofs), device=self._device) - 0.5),
self.franka_dof_lower_limits,
self.franka_dof_upper_limits,
)
dof_pos = torch.zeros((num_indices, self._frankas.num_dof), device=self._device)
dof_vel = torch.zeros((num_indices, self._frankas.num_dof), device=self._device)
dof_pos[:, :] = pos
self.franka_dof_targets[env_ids, :] = pos
self.franka_dof_pos[env_ids, :] = pos
self._frankas.set_joint_position_targets(self.franka_dof_targets[env_ids], indices=indices)
self._frankas.set_joint_positions(dof_pos, indices=indices)
self._frankas.set_joint_velocities(dof_vel, indices=indices)
# reset ball
# if not test, randomize ball initial positions for training
if not self.is_test:
# reset ball position: x [-0.1, 0.1], y [-0.1,0.1]
self.new_ball_pos = self.default_ball_pos.clone().detach()
self.new_ball_pos[:,0] = self.default_ball_pos[:,0] + (0.1 + 0.1) * torch.rand(self._num_envs, device=self._device) -0.1
self.new_ball_pos[:,1] = self.default_ball_pos[:,1] + (0.1 + 0.1) * torch.rand(self._num_envs, device=self._device) -0.1
self._ball.set_world_poses(self.new_ball_pos[env_ids], self.default_ball_rot[env_ids], indices = indices)
self._ball.set_velocities(self.default_ball_velocity[env_ids], indices = indices)
# if is test mode, set the ball to given position (1 environment)
else:
self.new_ball_pos = self.default_ball_pos.clone().detach()
self.new_ball_pos[:,0] = self.default_ball_pos[:,0] + self.initial_test_value[0]
self.new_ball_pos[:,1] = self.default_ball_pos[:,1] +self.initial_test_value[1]
self._ball.set_world_poses(self.new_ball_pos[env_ids], self.default_ball_rot[env_ids], indices = indices)
self._ball.set_velocities(self.default_ball_velocity[env_ids], indices = indices)
# bookkeeping
self.reset_buf[env_ids] = 0
self.progress_buf[env_ids] = 0
# fill extras
self.extras["episode"] = {}
for key in self.episode_sums.keys():
if key == "success_rate":
self.extras["episode"][key] = torch.mean(self.episode_sums[key][env_ids])
else:
self.extras["episode"][key] = torch.mean(self.episode_sums[key][env_ids])/self._max_episode_length
self.episode_sums[key][env_ids] = 0.
def post_reset(self):
self.num_franka_dofs = self._frankas.num_dof
self.franka_dof_pos = torch.zeros((self.num_envs, self.num_franka_dofs), device=self._device)
dof_limits = self._frankas.get_dof_limits()
self.franka_dof_lower_limits = dof_limits[0, :, 0].to(device=self._device)
self.franka_dof_upper_limits = dof_limits[0, :, 1].to(device=self._device)
self.franka_dof_speed_scales = torch.ones_like(self.franka_dof_lower_limits)
self.franka_dof_speed_scales[self._frankas.gripper_indices] = 0.1
self.franka_dof_targets = torch.zeros(
(self._num_envs, self.num_franka_dofs), dtype=torch.float, device=self._device
)
# Ball
self.default_ball_pos, self.default_ball_rot = self._ball.get_world_poses()
self.default_ball_velocity = self._ball.get_velocities()
# randomize all envs
indices = torch.arange(self._num_envs, dtype=torch.int64, device=self._device)
self.reset_idx(indices)
def calculate_metrics(self) -> None:
# get objects positions and orientations
joint_positions = self.franka_dof_pos # franka dofs pos
num_envs = self._num_envs # num of sim env
finger_pos = (self.franka_lfinger_pos + self.franka_lfinger_pos)/2 # franka finger pos (lfinger+rfinger)/2
self.finger_pos = finger_pos
gripper_forward_axis = self.gripper_forward_axis
gripper_up_axis = self.gripper_up_axis
# franka_grasp_pos = self.franka_grasp_pos
# franka_grasp_rot = self.franka_grasp_rot
# ball_grasp_pos = self.ball_grasp_pos
# ball_grasp_rot = self.ball_grasp_rot
# ball_inward_axis = self.ball_inward_axis
# ball_up_axis = self.ball_up_axis
# franka_dof_pos = self.franka_dof_pos
ball_init_pos = self.default_ball_pos
ball_pos = self.ball_pos # ball pos
# ball_rot = self.ball_rot # ball rot
# ball_vel = self._ball.get_velocities() # ball velocity
# table_pos = self.table_pos # table pos
# table_rot = self.table_rot # table rot
hole_pos = self.location_ball_pos # locate hole pos
# hole_pos[:,1] = hole_pos[:,1] - 0.8 # Y-axis
# hole_pos[:,2] = hole_pos[:,2] + 0.44 # Z-axis
# 1st reward: distance from ball to hole
ball_hole_dist = torch.norm(hole_pos - ball_pos, p=2, dim=-1)
ball_hole_XY_dist = torch.norm(hole_pos[:,0:2] - ball_pos[:,0:2], p=2, dim=-1)
# dist_reward = 1.0 / (1.0 + ball_hole_dist ** 2)
# dist_reward *= 2*dist_reward
# dist_reward = torch.where(ball_hole_dist <= 0.05, dist_reward+10, dist_reward)
# ball_hole_dist = torch.norm(hole_pos - ball_pos, p=2, dim=-1)
# dist_reward = 1.0/(1.0+ball_hole_dist**2)
dist_reward = 1-torch.tanh(3*ball_hole_XY_dist) # regulize the dist_reward in [0,1]
# dist_reward = -(ball_hole_XY_dist)**2
# 2nd reward: distance from finger to ball
# finger_ball_dist = torch.norm(finger_pos - ball_pos, p=2, dim=-1)
ball_to_init_dist = torch.norm(ball_pos[:,0:2] - ball_init_pos[:,0:2], p=2, dim=-1)
self.ball_to_init_dist = ball_to_init_dist
finger_ball_dist = torch.norm(finger_pos - ball_pos, p=2, dim=-1)
finger_ball_reward = 1.0/(1.0+finger_ball_dist**2)
# 1st penalty: regularization on the actions (summed for each environment)
action_penalty = torch.sum(self.actions ** 2, dim=-1)
action_penalty = 1-torch.tanh(action_penalty/2.5)
# 5th penalty if ball is not moved
ball_unmove_penalty = torch.zeros_like(dist_reward)
ball_unmove_penalty = torch.where(ball_to_init_dist<0.3, torch.tanh(15*(0.3-ball_to_init_dist)), ball_unmove_penalty)
falling_bonus = torch.where(torch.logical_and(ball_hole_XY_dist < 0.1 , ball_pos[:,2]<0.38), torch.ones_like(dist_reward), torch.zeros_like(dist_reward))
falling_penalty = torch.zeros_like(dist_reward)
falling_penalty = torch.where(torch.logical_and(ball_hole_XY_dist > 0.001 , ball_pos[:,2]<0.38), falling_penalty+10, falling_penalty)
# falling_penalty = torch.where(ball_hole_XY_dist<0.2, falling_penalty-100, falling_penalty)
# dist_reward = torch.where(ball_hole_XY_dist<0.3, 1-torch.tanh(10*ball_hole_XY_dist), dist_reward)
# dist_reward = torch.where(ball_to_init_dist>0.01, dist_reward, dist_reward*0)
dist_reward = torch.where(ball_pos[:,0]<hole_pos[:,0], torch.zeros_like(dist_reward), dist_reward)
dist_penalty = torch.tanh(3*ball_hole_XY_dist)
final_reward = 10.0*dist_reward - 0.0*ball_unmove_penalty + 100.0*falling_bonus - 0.0*action_penalty \
- 0.0*falling_penalty + 0.0*finger_ball_reward - 0.0*dist_penalty
# final_reward = torch.where(finger_pos[:,2] < (ball_pos[:,2]), final_reward-0.5, final_reward)
# final_reward = torch.where(torch.logical_and(finger_ball_dist > 0, ball_to_init_dist<0.05), final_reward-0.5, final_reward)
# final_reward = torch.where(ball_hole_XY_dist>0.2, final_reward-1, final_reward)
self.is_complete = torch.where(torch.logical_and(ball_hole_XY_dist < 0.01 , ball_pos[:,2]<0.38), torch.ones_like(final_reward), torch.zeros_like(final_reward)) # task complete
# final_reward = torch.where(ball_hole_XY_dist < 0.6, final_reward+3.0*dist_reward, final_reward)
self.rew_buf[:] = final_reward
self.episode_sums["success_rate"] += self.is_complete
self.episode_sums["ball_hole_XY_dist"] += ball_hole_XY_dist
def is_done(self) -> None:
if not self.is_test:
# reset if ball falls from the edge or in hole
self.reset_buf = torch.where(self.ball_pos[:, 2] < 0.1, torch.ones_like(self.reset_buf), self.reset_buf)
# self.reset_buf = torch.where(self.is_complete==1, torch.ones_like(self.reset_buf), self.reset_buf)
# reset if franka grasp is below the ball and ball is not moved
# self.reset_buf = torch.where(self.finger_pos[:, 2] < 0.2, torch.ones_like(self.reset_buf), self.reset_buf)
# self.reset_buf = torch.where(torch.logical_and(self.finger_pos[:, 2] < 0.3, self.ball_to_init_dist < 0.1), torch.ones_like(self.reset_buf), self.reset_buf)
# reset if max length reached
self.reset_buf = torch.where(self.progress_buf >= self._max_episode_length - 1, torch.ones_like(self.reset_buf), self.reset_buf)
else:
self.reset_buf = torch.where(self.progress_buf >= self._max_episode_length - 1, torch.ones_like(self.reset_buf), self.reset_buf)
| 20,783 | Python | 45.496644 | 185 | 0.610258 |
zhehuazhou/ai-cps-robotics-benchmark/Gym_Envs/Tasks/Franka_Point_Reaching.py | from omniisaacgymenvs.tasks.base.rl_task import RLTask
from Models.Franka.Franka import Franka
from Models.Franka.Franka_view import FrankaView
from Models.point_reaching.target_ball import TargetBall
from omni.isaac.core.objects import DynamicCuboid
from omni.isaac.core.prims import RigidPrim, RigidPrimView
from omni.isaac.core.utils.prims import get_prim_at_path
from omni.isaac.core.utils.stage import get_current_stage, add_reference_to_stage
from omni.isaac.core.utils.torch.transformations import *
from omni.isaac.core.utils.torch.rotations import *
from omni.isaac.cloner import Cloner
import numpy as np
import torch
import math
from pxr import Gf, Usd, UsdGeom
class FrankaPointReachingTask(RLTask):
def __init__(
self,
name,
sim_config,
env,
offset=None
) -> None:
self._sim_config = sim_config
self._cfg = sim_config.config
self._task_cfg = sim_config.task_config
self._num_envs = self._task_cfg["env"]["numEnvs"]
self._env_spacing = self._task_cfg["env"]["envSpacing"]
self._max_episode_length = self._task_cfg["env"]["episodeLength"]
self.action_scale = self._task_cfg["env"]["actionScale"]
self.start_position_noise = self._task_cfg["env"]["startPositionNoise"]
self.start_rotation_noise = self._task_cfg["env"]["startRotationNoise"]
self.dof_vel_scale = self._task_cfg["env"]["dofVelocityScale"]
self.dist_reward_scale = self._task_cfg["env"]["distRewardScale"]
self.rot_reward_scale = self._task_cfg["env"]["rotRewardScale"]
self.around_handle_reward_scale = self._task_cfg["env"]["aroundHandleRewardScale"]
self.open_reward_scale = self._task_cfg["env"]["openRewardScale"]
self.finger_dist_reward_scale = self._task_cfg["env"]["fingerDistRewardScale"]
self.action_penalty_scale = self._task_cfg["env"]["actionPenaltyScale"]
self.finger_close_reward_scale = self._task_cfg["env"]["fingerCloseRewardScale"]
self.distX_offset = 0.04
self.dt = 1/60.
self._num_observations = 27
self._num_actions = 9
# Flag for testing
self.is_test = False
self.initial_test_value = None
self.is_action_noise = False
RLTask.__init__(self, name, env)
# Extra info for TensorBoard
self.extras = {}
torch_zeros = lambda: torch.zeros(self.num_envs, dtype=torch.float, device=self.device, requires_grad=False)
self.episode_sums = {"success_rate": torch_zeros()}
return
def set_up_scene(self, scene) -> None:
# Franka
franka_translation = torch.tensor([0.3, 0.0, 0.0])
self.get_franka(franka_translation)
self.get_target_ball()
# Here the env is cloned
super().set_up_scene(scene)
# Add Franka
self._frankas = FrankaView(prim_paths_expr="/World/envs/.*/franka", name="franka_view")
# Add location_ball
self._target_ball = RigidPrimView(prim_paths_expr="/World/envs/.*/target_ball/target_ball/ball_mesh", name="target_ball_view", reset_xform_properties=False)
scene.add(self._frankas)
scene.add(self._frankas._hands)
scene.add(self._frankas._lfingers)
scene.add(self._frankas._rfingers)
scene.add(self._target_ball)
self.init_data()
return
def get_franka(self, translation):
franka = Franka(prim_path=self.default_zero_env_path + "/franka", name="franka", translation = translation)
self._sim_config.apply_articulation_settings("franka", get_prim_at_path(franka.prim_path), self._sim_config.parse_actor_config("franka"))
def get_target_ball(self):
target_ball = TargetBall(prim_path=self.default_zero_env_path + "/target_ball", name="target_ball")
self._sim_config.apply_articulation_settings("target_ball", get_prim_at_path(target_ball.prim_path), self._sim_config.parse_actor_config("target_ball"))
# Set as testing mode
def set_as_test(self):
self.is_test = True
# Set action noise
def set_action_noise(self):
self.is_action_noise = True
# Set initial test values for testing mode
def set_initial_test_value(self, value):
# for ball pushing: initial x,y positions of the ball
self.initial_test_value = value
def init_data(self) -> None:
def get_env_local_pose(env_pos, xformable, device):
"""Compute pose in env-local coordinates"""
world_transform = xformable.ComputeLocalToWorldTransform(0)
world_pos = world_transform.ExtractTranslation()
world_quat = world_transform.ExtractRotationQuat()
px = world_pos[0] - env_pos[0]
py = world_pos[1] - env_pos[1]
pz = world_pos[2] - env_pos[2]
qx = world_quat.imaginary[0]
qy = world_quat.imaginary[1]
qz = world_quat.imaginary[2]
qw = world_quat.real
return torch.tensor([px, py, pz, qw, qx, qy, qz], device=device, dtype=torch.float)
stage = get_current_stage()
hand_pose = get_env_local_pose(self._env_pos[0], UsdGeom.Xformable(stage.GetPrimAtPath("/World/envs/env_0/franka/panda_link7")), self._device)
lfinger_pose = get_env_local_pose(
self._env_pos[0], UsdGeom.Xformable(stage.GetPrimAtPath("/World/envs/env_0/franka/panda_leftfinger")), self._device
)
rfinger_pose = get_env_local_pose(
self._env_pos[0], UsdGeom.Xformable(stage.GetPrimAtPath("/World/envs/env_0/franka/panda_rightfinger")), self._device
)
# finger pos
finger_pose = torch.zeros(7, device=self._device)
finger_pose[0:3] = (lfinger_pose[0:3] + rfinger_pose[0:3]) / 2.0
finger_pose[3:7] = lfinger_pose[3:7]
hand_pose_inv_rot, hand_pose_inv_pos = (tf_inverse(hand_pose[3:7], hand_pose[0:3]))
# franka grasp local pose
grasp_pose_axis = 1
franka_local_grasp_pose_rot, franka_local_pose_pos = tf_combine(hand_pose_inv_rot, hand_pose_inv_pos, finger_pose[3:7], finger_pose[0:3])
franka_local_pose_pos += torch.tensor([0, 0.04, 0], device=self._device)
self.franka_local_grasp_pos = franka_local_pose_pos.repeat((self._num_envs, 1))
self.franka_local_grasp_rot = franka_local_grasp_pose_rot.repeat((self._num_envs, 1))
self.gripper_forward_axis = torch.tensor([0, 0, 1], device=self._device, dtype=torch.float).repeat((self._num_envs, 1))
self.gripper_up_axis = torch.tensor([0, 1, 0], device=self._device, dtype=torch.float).repeat((self._num_envs, 1))
self.franka_default_dof_pos = torch.tensor(
[0.0, -0.872, 0.0, -2.0, 0.0, 2.618, 0.785, 0.01, 0.01], device=self._device
)
self.actions = torch.zeros((self._num_envs, self.num_actions), device=self._device)
def get_observations(self) -> dict:
# Franka
hand_pos, hand_rot = self._frankas._hands.get_world_poses(clone=False)
franka_dof_pos = self._frankas.get_joint_positions(clone=False)
franka_dof_vel = self._frankas.get_joint_velocities(clone=False)
self.franka_dof_pos = franka_dof_pos
self.franka_lfinger_pos, self.franka_lfinger_rot = self._frankas._lfingers.get_world_poses(clone=False)
self.franka_rfinger_pos, self.franka_rfinger_rot = self._frankas._lfingers.get_world_poses(clone=False)
finger_center = (self.franka_lfinger_pos + self.franka_rfinger_pos)/2
dof_pos_scaled = (
2.0
* (franka_dof_pos - self.franka_dof_lower_limits)
/ (self.franka_dof_upper_limits - self.franka_dof_lower_limits)
- 1.0
)
# target ball
target_ball_pos, target_ball_rot = self._target_ball.get_world_poses(clone=False) # tool position
to_target = finger_center - target_ball_pos
self.obs_buf = torch.cat(
(
dof_pos_scaled,
franka_dof_vel * self.dof_vel_scale,
target_ball_pos,
finger_center,
to_target,
),
dim=-1,
)
observations = {
self._frankas.name: {
"obs_buf": self.obs_buf
}
}
return observations
def pre_physics_step(self, actions) -> None:
if not self._env._world.is_playing():
return
reset_env_ids = self.reset_buf.nonzero(as_tuple=False).squeeze(-1)
if len(reset_env_ids) > 0:
self.reset_idx(reset_env_ids)
self.actions = actions.clone().to(self._device)
# if action noise
if self.is_action_noise is True:
# Gaussian white noise with 0.01 variance
self.actions = self.actions + (0.5)*torch.randn_like(self.actions)
targets = self.franka_dof_targets + self.franka_dof_speed_scales * self.dt * self.actions * self.action_scale
self.franka_dof_targets[:] = tensor_clamp(targets, self.franka_dof_lower_limits, self.franka_dof_upper_limits)
env_ids_int32 = torch.arange(self._frankas.count, dtype=torch.int32, device=self._device)
self.franka_dof_targets[:,7] = self.franka_default_dof_pos[7]
self.franka_dof_targets[:,8] = self.franka_default_dof_pos[8]
self._frankas.set_joint_position_targets(self.franka_dof_targets, indices=env_ids_int32)
def reset_idx(self, env_ids):
indices = env_ids.to(dtype=torch.int32)
num_indices = len(indices)
# reset franka
pos = torch.clamp(
self.franka_default_dof_pos.unsqueeze(0),
#+ 0.25 * (torch.rand((len(env_ids), self.num_franka_dofs), device=self._device) - 0.5),
self.franka_dof_lower_limits,
self.franka_dof_upper_limits,
)
dof_pos = torch.zeros((num_indices, self._frankas.num_dof), device=self._device)
dof_vel = torch.zeros((num_indices, self._frankas.num_dof), device=self._device)
dof_pos[:, :] = pos
self.franka_dof_targets[env_ids, :] = pos
self.franka_dof_pos[env_ids, :] = pos
self._frankas.set_joint_position_targets(self.franka_dof_targets[env_ids], indices=indices)
self._frankas.set_joint_positions(dof_pos, indices=indices)
self._frankas.set_joint_velocities(dof_vel, indices=indices)
if not self.is_test:
# reset target cube
# reset target cube position within an area: x [-0.2, 0.2], y [-0.4,0.4], z [-0.2,0.2]
self.new_cube_pos = self.default_target_ball_pos.clone().detach()
self.new_cube_pos[:,0] = self.default_target_ball_pos[:,0] + (0.2 + 0.2) * torch.rand(self._num_envs, device=self._device) -0.2
self.new_cube_pos[:,1] = self.default_target_ball_pos[:,1] + (0.4 + 0.4) * torch.rand(self._num_envs, device=self._device) -0.4
self.new_cube_pos[:,2] = self.default_target_ball_pos[:,2] + (0.2 + 0.2) * torch.rand(self._num_envs, device=self._device) -0.2
self._target_ball.set_world_poses(self.new_cube_pos[env_ids], self.default_target_ball_rot[env_ids], indices = indices)
self._target_ball.set_velocities(self.default_target_ball_velocity[env_ids], indices = indices)
# if is test mode
else:
self.new_cube_pos = self.default_target_ball_pos.clone().detach()
self.new_cube_pos[:,0] = self.default_target_ball_pos[:,0] + self.initial_test_value[0]
self.new_cube_pos[:,1] = self.default_target_ball_pos[:,1] + self.initial_test_value[1]
self.new_cube_pos[:,2] = self.default_target_ball_pos[:,2] + self.initial_test_value[2]
self._target_ball.set_world_poses(self.new_cube_pos[env_ids], self.default_target_ball_rot[env_ids], indices = indices)
self._target_ball.set_velocities(self.default_target_ball_velocity[env_ids], indices = indices)
# bookkeeping
self.reset_buf[env_ids] = 0
self.progress_buf[env_ids] = 0
# fill extras
self.extras["episode"] = {}
for key in self.episode_sums.keys():
if key == "success_rate":
self.extras["episode"][key] = torch.mean(self.episode_sums[key][env_ids])
else:
self.extras["episode"][key] = torch.mean(self.episode_sums[key][env_ids]) / self._max_episode_length
self.episode_sums[key][env_ids] = 0
def post_reset(self):
# Franka
self.num_franka_dofs = self._frankas.num_dof
self.franka_dof_pos = torch.zeros((self.num_envs, self.num_franka_dofs), device=self._device)
dof_limits = self._frankas.get_dof_limits()
self.franka_dof_lower_limits = dof_limits[0, :, 0].to(device=self._device)
self.franka_dof_upper_limits = dof_limits[0, :, 1].to(device=self._device)
self.franka_dof_speed_scales = torch.ones_like(self.franka_dof_lower_limits)
self.franka_dof_speed_scales[self._frankas.gripper_indices] = 0.1
self.franka_dof_targets = torch.zeros(
(self._num_envs, self.num_franka_dofs), dtype=torch.float, device=self._device
)
# Target cube
self.default_target_ball_pos, self.default_target_ball_rot = self._target_ball.get_world_poses()
self.default_target_ball_velocity = self._target_ball.get_velocities()
# randomize all envs
indices = torch.arange(self._num_envs, dtype=torch.int64, device=self._device)
self.reset_idx(indices)
def calculate_metrics(self) -> None:
# Reward info
self.franka_lfinger_pos, self.franka_lfinger_rot = self._frankas._lfingers.get_world_poses()
self.franka_rfinger_pos, self.franka_rfinger_rot = self._frankas._lfingers.get_world_poses()
finger_center = (self.franka_lfinger_pos + self.franka_rfinger_pos)/2
lfinger_vel = self._frankas._lfingers.get_velocities()
rfinger_vel = self._frankas._lfingers.get_velocities()
finger_vel = (lfinger_vel + rfinger_vel)/2
finger_vel_norm = torch.norm(finger_vel, p=2, dim=-1)
target_ball_pos, target_ball_rot = self._target_ball.get_world_poses()
# distance
ball_center_dist = torch.norm(target_ball_pos - finger_center, p=2, dim=-1)
center_dist_reward = 1.0/(1.0+ball_center_dist)
# velocity
finger_vel_reward = 1.0/(1.0+finger_vel_norm)
# is complete
is_complete = torch.where( torch.logical_and(ball_center_dist<0.03, finger_vel_norm<0.02),
torch.ones_like(finger_vel_norm), torch.zeros_like(finger_vel_norm))
final_reward = 1.0*center_dist_reward + 10.0*is_complete + 0.1*finger_vel_reward
self.rew_buf[:] = final_reward
self.episode_sums["success_rate"] += is_complete
def is_done(self) -> None:
if not self.is_test:
# reset if max length reached
self.reset_buf = torch.where(self.progress_buf >= self._max_episode_length - 1, torch.ones_like(self.reset_buf), self.reset_buf)
else:
self.reset_buf = torch.where(self.progress_buf >= self._max_episode_length - 1, torch.ones_like(self.reset_buf), self.reset_buf)
| 15,304 | Python | 42.112676 | 164 | 0.622517 |
zhehuazhou/ai-cps-robotics-benchmark/Gym_Envs/Tasks/Franka_Cloth_Placing.py | from omniisaacgymenvs.tasks.base.rl_task import RLTask
from Models.Franka.Franka import Franka
from Models.Franka.Franka_view import FrankaView
from Models.cloth_placing.target_table import TargetTable
from omni.isaac.core.prims import ParticleSystem, ClothPrim, ClothPrimView
from omni.isaac.core.materials import ParticleMaterial
from omni.physx.scripts import physicsUtils, particleUtils, deformableUtils
from omni.isaac.core.objects import DynamicCuboid
from omni.isaac.core.prims import RigidPrim, RigidPrimView
from omni.isaac.core.utils.prims import get_prim_at_path
from omni.isaac.core.utils.stage import get_current_stage, add_reference_to_stage
from omni.isaac.core.utils.torch.transformations import *
from omni.isaac.core.utils.torch.rotations import *
from omni.isaac.cloner import Cloner
import numpy as np
import torch
import math
from pxr import Gf, Usd, UsdGeom
class FrankaClothPlacingTask(RLTask):
def __init__(
self,
name,
sim_config,
env,
offset=None
) -> None:
self._sim_config = sim_config
self._cfg = sim_config.config
self._task_cfg = sim_config.task_config
self._num_envs = self._task_cfg["env"]["numEnvs"]
self._env_spacing = self._task_cfg["env"]["envSpacing"]
self._max_episode_length = self._task_cfg["env"]["episodeLength"]
self.action_scale = self._task_cfg["env"]["actionScale"]
self.start_position_noise = self._task_cfg["env"]["startPositionNoise"]
self.start_rotation_noise = self._task_cfg["env"]["startRotationNoise"]
self.dof_vel_scale = self._task_cfg["env"]["dofVelocityScale"]
self.dist_reward_scale = self._task_cfg["env"]["distRewardScale"]
self.rot_reward_scale = self._task_cfg["env"]["rotRewardScale"]
self.around_handle_reward_scale = self._task_cfg["env"]["aroundHandleRewardScale"]
self.open_reward_scale = self._task_cfg["env"]["openRewardScale"]
self.finger_dist_reward_scale = self._task_cfg["env"]["fingerDistRewardScale"]
self.action_penalty_scale = self._task_cfg["env"]["actionPenaltyScale"]
self.finger_close_reward_scale = self._task_cfg["env"]["fingerCloseRewardScale"]
self.distX_offset = 0.04
self.dt = 1/60.
self._num_observations = 27
self._num_actions = 9
# Flag for testing
self.is_test = False
self.initial_test_value = None
self.is_action_noise = False
RLTask.__init__(self, name, env)
# Extra info for TensorBoard
self.extras = {}
torch_zeros = lambda: torch.zeros(self.num_envs, dtype=torch.float, device=self.device, requires_grad=False)
self.episode_sums = {"center_dist": torch_zeros()}
return
def set_up_scene(self, scene) -> None:
# Franka
franka_translation = torch.tensor([0.3, 0.0, 0.0])
self.get_franka(franka_translation)
self.get_table()
# Here the env is cloned (cannot clone particle systems right now)
super().set_up_scene(scene)
# Add Franka
self._frankas = FrankaView(prim_paths_expr="/World/envs/.*/franka", name="franka_view")
# Add bin
self._target_table = RigidPrimView(prim_paths_expr="/World/envs/.*/target_table/target_table/mesh", name="target_table_view", reset_xform_properties=False)
# Add location_ball
self._location_cube = RigidPrimView(prim_paths_expr="/World/envs/.*/target_table/target_table/location_cube", name="location_cube_view", reset_xform_properties=False)
scene.add(self._frankas)
scene.add(self._frankas._hands)
scene.add(self._frankas._lfingers)
scene.add(self._frankas._rfingers)
scene.add(self._location_cube)
scene.add(self._target_table)
# generate cloth near franka
franka_positions = self._frankas.get_world_poses()[0]
self.initialize_cloth(franka_positions)
# Create a view to deal with all the cloths
self._cloths = ClothPrimView(prim_paths_expr="/World/Env*/cloth", name="cloth_view")
self._scene.add(self._cloths)
self.init_data()
return
def get_franka(self, translation):
franka = Franka(prim_path=self.default_zero_env_path + "/franka", name="franka", translation = translation, use_modified_collision = True)
self._sim_config.apply_articulation_settings("franka", get_prim_at_path(franka.prim_path), self._sim_config.parse_actor_config("franka"))
def get_table(self):
target_table = TargetTable(prim_path=self.default_zero_env_path + "/target_table", name="target_table")
self._sim_config.apply_articulation_settings("target_table", get_prim_at_path(target_table.prim_path), self._sim_config.parse_actor_config("target_table"))
# Set as testing mode
def set_as_test(self):
self.is_test = True
# Set action noise
def set_action_noise(self):
self.is_action_noise = True
# Set initial test values for testing mode
def set_initial_test_value(self, value):
# for ball pushing: initial x,y positions of the ball
self.initial_test_value = value
def init_data(self) -> None:
def get_env_local_pose(env_pos, xformable, device):
"""Compute pose in env-local coordinates"""
world_transform = xformable.ComputeLocalToWorldTransform(0)
world_pos = world_transform.ExtractTranslation()
world_quat = world_transform.ExtractRotationQuat()
px = world_pos[0] - env_pos[0]
py = world_pos[1] - env_pos[1]
pz = world_pos[2] - env_pos[2]
qx = world_quat.imaginary[0]
qy = world_quat.imaginary[1]
qz = world_quat.imaginary[2]
qw = world_quat.real
return torch.tensor([px, py, pz, qw, qx, qy, qz], device=device, dtype=torch.float)
stage = get_current_stage()
hand_pose = get_env_local_pose(self._env_pos[0], UsdGeom.Xformable(stage.GetPrimAtPath("/World/envs/env_0/franka/panda_link7")), self._device)
lfinger_pose = get_env_local_pose(
self._env_pos[0], UsdGeom.Xformable(stage.GetPrimAtPath("/World/envs/env_0/franka/panda_leftfinger")), self._device
)
rfinger_pose = get_env_local_pose(
self._env_pos[0], UsdGeom.Xformable(stage.GetPrimAtPath("/World/envs/env_0/franka/panda_rightfinger")), self._device
)
# finger pos
finger_pose = torch.zeros(7, device=self._device)
finger_pose[0:3] = (lfinger_pose[0:3] + rfinger_pose[0:3]) / 2.0
finger_pose[3:7] = lfinger_pose[3:7]
hand_pose_inv_rot, hand_pose_inv_pos = (tf_inverse(hand_pose[3:7], hand_pose[0:3]))
# franka grasp local pose
grasp_pose_axis = 1
franka_local_grasp_pose_rot, franka_local_pose_pos = tf_combine(hand_pose_inv_rot, hand_pose_inv_pos, finger_pose[3:7], finger_pose[0:3])
franka_local_pose_pos += torch.tensor([0, 0.04, 0], device=self._device)
self.franka_local_grasp_pos = franka_local_pose_pos.repeat((self._num_envs, 1))
self.franka_local_grasp_rot = franka_local_grasp_pose_rot.repeat((self._num_envs, 1))
self.gripper_forward_axis = torch.tensor([0, 0, 1], device=self._device, dtype=torch.float).repeat((self._num_envs, 1))
self.gripper_up_axis = torch.tensor([0, 1, 0], device=self._device, dtype=torch.float).repeat((self._num_envs, 1))
self.franka_default_dof_pos = torch.tensor(
[0.0, 0.0, 0.0, -1.0, 0.0, 1.0, 0.5, 0.0001, 0.0001], device=self._device
)
self.actions = torch.zeros((self._num_envs, self.num_actions), device=self._device)
def get_observations(self) -> dict:
# Franka
hand_pos, hand_rot = self._frankas._hands.get_world_poses(clone=False)
franka_dof_pos = self._frankas.get_joint_positions(clone=False)
franka_dof_pos = torch.nan_to_num(franka_dof_pos)
franka_dof_vel = self._frankas.get_joint_velocities(clone=False)
franka_dof_vel = torch.nan_to_num(franka_dof_vel)
self.franka_lfinger_pos, self.franka_lfinger_rot = self._frankas._lfingers.get_world_poses(clone=False)
self.franka_rfinger_pos, self.franka_rfinger_rot = self._frankas._lfingers.get_world_poses(clone=False)
dof_pos_scaled = (
2.0
* (franka_dof_pos - self.franka_dof_lower_limits)
/ (self.franka_dof_upper_limits - self.franka_dof_lower_limits)
- 1.0
)
# Cloth
self.cloths_pos = self._cloths.get_world_positions(clone=False)
self.cloths_pos = torch.nan_to_num(self.cloths_pos) # shape (M,121,3)
# cloths_pos_flat = torch.flatten(self.cloths_pos, start_dim=1) # shape (M,121,3)
cloth_mean_x = torch.mean(self.cloths_pos[:,:,0], dim=1).reshape(self.num_envs, 1)
cloth_mean_y = torch.mean(self.cloths_pos[:,:,1], dim=1).reshape(self.num_envs, 1)
cloth_mean_z = torch.mean(self.cloths_pos[:,:,2], dim=1).reshape(self.num_envs, 1)
self.cloths_pos_mean = torch.cat((cloth_mean_x, cloth_mean_y, cloth_mean_z),1)
# location cube
self.location_cube_pos, self.location_cube_rot = self._location_cube.get_world_poses(clone=False)
self.location_cube_pos = torch.nan_to_num(self.location_cube_pos)
to_target = self.cloths_pos_mean - self.location_cube_pos
self.obs_buf = torch.cat(
(
dof_pos_scaled,
franka_dof_vel * self.dof_vel_scale,
# cloths_pos_flat,
self.cloths_pos_mean,
to_target,
self.location_cube_pos,
# self.handle_rot,
# self.location_ball_pos
# self.cabinet_dof_pos[:, 3].unsqueeze(-1),
# self.cabinet_dof_vel[:, 3].unsqueeze(-1),
),
dim=-1,
)
observations = {
self._frankas.name: {
"obs_buf": self.obs_buf
}
}
return observations
def pre_physics_step(self, actions) -> None:
if not self._env._world.is_playing():
return
reset_env_ids = self.reset_buf.nonzero(as_tuple=False).squeeze(-1)
if len(reset_env_ids) > 0:
self.reset_idx(reset_env_ids)
self.actions = actions.clone().to(self._device)
# if action noise
if self.is_action_noise is True:
# Gaussian white noise with 0.01 variance
self.actions = self.actions + (0.5)*torch.randn_like(self.actions)
targets = self.franka_dof_targets + self.franka_dof_speed_scales * self.dt * self.actions * self.action_scale
self.franka_dof_targets[:] = tensor_clamp(targets, self.franka_dof_lower_limits, self.franka_dof_upper_limits)
env_ids_int32 = torch.arange(self._frankas.count, dtype=torch.int32, device=self._device)
# Release condition
location_cube_pos, location_cube_rot = self._location_cube.get_world_poses()
location_cube_pos = torch.nan_to_num(location_cube_pos)
cloths_pos = self._cloths.get_world_positions()
cloths_pos = torch.nan_to_num(cloths_pos)
cloth_mean_x = torch.mean(cloths_pos[:,:,0], dim=1).reshape(self.num_envs, 1)
cloth_mean_y = torch.mean(cloths_pos[:,:,1], dim=1).reshape(self.num_envs, 1)
cloth_mean_z = torch.mean(cloths_pos[:,:,2], dim=1).reshape(self.num_envs, 1)
cloths_pos_mean = torch.cat((cloth_mean_x, cloth_mean_y, cloth_mean_z),1)
center_dist = torch.norm(location_cube_pos[:,0:2] - cloths_pos_mean[:,0:2], p=2, dim=-1)
cloth_vel = self._cloths.get_velocities()
cloth_vel = torch.nan_to_num(cloth_vel)
cloth_vel_x = torch.mean(cloth_vel[:,:,0], dim=1).reshape(self.num_envs, 1)
cloth_vel_y = torch.mean(cloth_vel[:,:,1], dim=1).reshape(self.num_envs, 1)
cloth_vel_z = torch.mean(cloth_vel[:,:,2], dim=1).reshape(self.num_envs, 1)
cloths_vel_mean = torch.cat((cloth_vel_x, cloth_vel_y, cloth_vel_z),1)
vel = torch.norm(cloths_vel_mean, p=2, dim=-1)
release_condition = torch.logical_and(center_dist<0.07, cloths_pos_mean[:,2] > location_cube_pos[:,2])
release_condition = torch.logical_and(release_condition, vel<0.1)
self.franka_dof_targets[:,7] = torch.where(release_condition, 0.15, self.franka_dof_targets[:,7])
self.franka_dof_targets[:,8] = torch.where(release_condition, 0.15, self.franka_dof_targets[:,8])
self._frankas.set_joint_position_targets(self.franka_dof_targets, indices=env_ids_int32)
def reset_idx(self, env_ids):
indices = env_ids.to(dtype=torch.int32)
num_indices = len(indices)
# reset franka
pos = torch.clamp(
self.franka_default_dof_pos.unsqueeze(0),
#+ 0.25 * (torch.rand((len(env_ids), self.num_franka_dofs), device=self._device) - 0.5),
self.franka_dof_lower_limits,
self.franka_dof_upper_limits,
)
dof_pos = torch.zeros((num_indices, self._frankas.num_dof), device=self._device)
dof_vel = torch.zeros((num_indices, self._frankas.num_dof), device=self._device)
dof_pos[:, :] = pos
self.franka_dof_targets[env_ids, :] = pos
self.franka_dof_pos[env_ids, :] = pos
self._frankas.set_joint_position_targets(self.franka_dof_targets[env_ids], indices=indices)
self._frankas.set_joint_positions(dof_pos, indices=indices)
self._frankas.set_joint_velocities(dof_vel, indices=indices)
# Reset cloth
self._cloths.set_world_positions(self.default_cloth_pos, indices=indices)
self._cloths.set_velocities(self.default_cloth_vel, indices=indices)
if not self.is_test:
# Reset cloth bin
# reset positions: x: [-0.1,0.2], y:[-0.35,0.35]
random_x = (0.2 + 0.1) * torch.rand(self._num_envs, device=self._device) - 0.1
random_y = (0.35 + 0.35) * torch.rand(self._num_envs, device=self._device) - 0.35
self.new_location_cube_pos = self.default_target_table_pos.clone().detach()
self.new_location_cube_pos[:,0] = self.default_target_table_pos[:,0] + random_x
self.new_location_cube_pos[:,1] = self.default_target_table_pos[:,1] + random_y
self._target_table.set_world_poses(self.new_location_cube_pos[env_ids], self.default_target_table_rot[env_ids], indices = indices)
self._target_table.set_velocities(self.default_target_table_velocity[env_ids], indices = indices)
else:
random_x = self.initial_test_value[0]
random_y = self.initial_test_value[1]
self.new_location_cube_pos = self.default_target_table_pos.clone().detach()
self.new_location_cube_pos[:,0] = self.default_target_table_pos[:,0] + random_x
self.new_location_cube_pos[:,1] = self.default_target_table_pos[:,1] + random_y
self._target_table.set_world_poses(self.new_location_cube_pos[env_ids], self.default_target_table_rot[env_ids], indices = indices)
self._target_table.set_velocities(self.default_target_table_velocity[env_ids], indices = indices)
# bookkeeping
self.reset_buf[env_ids] = 0
self.progress_buf[env_ids] = 0
def post_reset(self):
# Franka
self.num_franka_dofs = self._frankas.num_dof
self.franka_dof_pos = torch.zeros((self.num_envs, self.num_franka_dofs), device=self._device)
dof_limits = self._frankas.get_dof_limits()
self.franka_dof_lower_limits = dof_limits[0, :, 0].to(device=self._device)
self.franka_dof_upper_limits = dof_limits[0, :, 1].to(device=self._device)
self.franka_dof_speed_scales = torch.ones_like(self.franka_dof_lower_limits)
self.franka_dof_speed_scales[self._frankas.gripper_indices] = 0.1
self.franka_dof_targets = torch.zeros(
(self._num_envs, self.num_franka_dofs), dtype=torch.float, device=self._device
)
# Cloth
self.default_cloth_pos = self._cloths.get_world_positions()
self.default_cloth_vel = torch.zeros([self._num_envs, self._cloths.max_particles_per_cloth, 3], device=self._device)
# Target table
self.default_target_table_pos, self.default_target_table_rot = self._target_table.get_world_poses()
self.default_target_table_velocity = self._target_table.get_velocities()
# randomize all envs
indices = torch.arange(self._num_envs, dtype=torch.int64, device=self._device)
self.reset_idx(indices)
def initialize_cloth(self, franka_positions):
stage = get_current_stage()
# parameters
dimx = 10
dimy = 10
scale = 0.3
for i in range(self._num_envs):
# Note here: cannot put into the same envs (env/env_i) due to unknown bugs
env_path = "/World/Env" + str(i)
env = UsdGeom.Xform.Define(stage, env_path)
# set up the geometry
cloth_path = env.GetPrim().GetPath().AppendChild("cloth")
plane_mesh = UsdGeom.Mesh.Define(stage, cloth_path)
tri_points, tri_indices = deformableUtils.create_triangle_mesh_square(dimx=dimx, dimy=dimy, scale=scale)
initial_positions = torch.zeros((self.num_envs, len(tri_points), 3))
plane_mesh.GetPointsAttr().Set(tri_points)
plane_mesh.GetFaceVertexIndicesAttr().Set(tri_indices)
plane_mesh.GetFaceVertexCountsAttr().Set([3] * (len(tri_indices) // 3))
# initial locations of the cloth
franka_positions_np = franka_positions.detach().to('cpu').numpy()
init_loc = Gf.Vec3f(float(franka_positions_np[i][0] - 0.5), float(franka_positions_np[i][1] ), float(franka_positions_np[i][2] + 0.65))
physicsUtils.setup_transform_as_scale_orient_translate(plane_mesh)
physicsUtils.set_or_add_translate_op(plane_mesh, init_loc)
physicsUtils.set_or_add_orient_op(plane_mesh, Gf.Rotation(Gf.Vec3d([1, 0, 0]), 90).GetQuat())
initial_positions[i] = torch.tensor(init_loc) + torch.tensor(plane_mesh.GetPointsAttr().Get())
particle_system_path = env.GetPrim().GetPath().AppendChild("particleSystem")
particle_material_path = env.GetPrim().GetPath().AppendChild("particleMaterial")
particle_material = ParticleMaterial(
prim_path=particle_material_path, drag=0.1, lift=0.3, friction=10.0
)
# parameters for the properties of the cloth
# radius = 0.005
radius = 0.5 * (scale / dimx) # size rest offset according to plane resolution and width so that particles are just touching at rest
restOffset = radius
contactOffset = restOffset * 1.5
particle_system = ParticleSystem(
prim_path=particle_system_path,
simulation_owner=self._env._world.get_physics_context().prim_path,
rest_offset=restOffset,
contact_offset=contactOffset,
solid_rest_offset=restOffset,
fluid_rest_offset=restOffset,
particle_contact_offset=contactOffset,
)
# note that no particle material is applied to the particle system at this point.
# this can be done manually via self.particle_system.apply_particle_material(self.particle_material)
# or to pass the material to the clothPrim which binds it internally to the particle system
stretch_stiffness = 100000.0
bend_stiffness = 100.0
shear_stiffness = 100.0
spring_damping = 0.1
particle_mass = 0.005
cloth = ClothPrim(
name="clothPrim" + str(i),
prim_path=str(cloth_path),
particle_system=particle_system,
particle_material=particle_material,
stretch_stiffness=stretch_stiffness,
bend_stiffness=bend_stiffness,
shear_stiffness=shear_stiffness,
spring_damping=spring_damping,
particle_mass = particle_mass,
self_collision=True,
self_collision_filter=True,
)
self._scene.add(cloth)
def calculate_metrics(self) -> None:
# center_dist = torch.norm(self.location_cube_pos - self.cloths_pos_mean, p=2, dim=-1)
location_cube_pos = self.location_cube_pos
center_dist = torch.norm(location_cube_pos - self.cloths_pos_mean, p=2, dim=-1)
center_dist_reward = 1.0/(1.0+center_dist)
# finger reward
# franka_lfinger_pos = torch.nan_to_num(self.franka_lfinger_pos)
# franka_rfinger_pos = torch.nan_to_num(self.franka_rfinger_pos)
# finger_center = (franka_lfinger_pos + franka_rfinger_pos)/2
# target = self.location_cube_pos
# target[:,2] = target[:,2] + 0.3
# finger_dist = torch.norm(finger_center - target, p=2, dim=-1)
# finger_dist_reward = 1.0/(1.0+finger_dist)
lfinger_vel = torch.nan_to_num(self._frankas._lfingers.get_velocities())
rfinger_vel = torch.nan_to_num(self._frankas._rfingers.get_velocities())
finger_vel = (lfinger_vel + rfinger_vel)/2
finger_vel_norm = torch.norm(finger_vel, p=2, dim=-1)
finger_vel_reward = 1.0/(1.0+finger_vel_norm)
# finger rotation
franka_lfinger_rot = torch.nan_to_num(self.franka_lfinger_rot)
franka_rfinger_rot = torch.nan_to_num(self.franka_rfinger_rot)
mean_rot = (franka_lfinger_rot + franka_rfinger_rot)/2
rot_target = torch.zeros_like(franka_lfinger_rot)
rot_target[:,2] = 1
rot_distance = torch.norm(mean_rot - rot_target, p=2, dim=-1)
rot_distance_reward = 1.0/(1.0+rot_distance)
# cloth velocities
cloth_vel = self._cloths.get_velocities()
cloth_vel = torch.nan_to_num(cloth_vel)
cloth_vel_x = torch.mean(cloth_vel[:,:,0], dim=1).reshape(self.num_envs, 1)
cloth_vel_y = torch.mean(cloth_vel[:,:,1], dim=1).reshape(self.num_envs, 1)
cloth_vel_z = torch.mean(cloth_vel[:,:,2], dim=1).reshape(self.num_envs, 1)
cloths_vel_mean = torch.cat((cloth_vel_x, cloth_vel_y, cloth_vel_z),1)
vel = torch.norm(cloths_vel_mean, p=2, dim=-1)
vel_reward = 1.0/(1.0+vel)
# stay alive
live_reward = torch.where(self.cloths_pos_mean[:,2] > 0.3, torch.ones_like(self.cloths_pos_mean[:,2]), torch.zeros_like(self.cloths_pos_mean[:,2]))
# franka velocities
# franka_dof_vel = self._frankas.get_joint_velocities()
# franka_dof_vel = torch.nan_to_num(franka_dof_vel)
# dof_vel_mean = torch.norm(franka_dof_vel, p=2, dim=-1)
# dof_vel_reward = 1.0/(1.0+dof_vel_mean)
# is complete
is_complete = torch.where(torch.logical_and(center_dist < 0.05, vel<0.1), torch.ones_like(center_dist), torch.zeros_like(center_dist))
# if torch.any(torch.isnan(self.cloths_pos_mean)):
# print("NAN", self.cloths_pos_mean)
# x_dist = torch.abs(self.location_cube_pos[:,0] - self.cloths_pos_mean[:,0])
# x_dist_reward = 1.0/(1.0+x_dist)
# y_dist = torch.abs(self.location_cube_pos[:,1] - self.cloths_pos_mean[:,1])
# y_dist_reward = 1.0/(1.0+y_dist)
# z_dist = torch.abs(self.location_cube_pos[:,2] - self.cloths_pos_mean[:,2])
# z_dist_reward = 1.0/(1.0+z_dist)
final_reward = 7.0*center_dist_reward + 10.0*is_complete + 1.0*rot_distance_reward + 1.0*live_reward \
+ 1.0*vel_reward + 1.0*finger_vel_reward
# TO BE IMPLEMENTED
self.rew_buf[:] = final_reward
# log additional info
self.episode_sums["center_dist"] += center_dist
# self.episode_sums["y_dist"] += y_dist
# self.episode_sums["z_dist"] += z_dist
def is_done(self) -> None:
if not self.is_test:
cloths_pos_z = self.cloths_pos_mean[:,2]
center_dist = torch.norm(self.location_cube_pos- self.cloths_pos_mean, p=2, dim=-1)
# if cloth falls to the ground
self.reset_buf = torch.where( (cloths_pos_z < 0.1), torch.ones_like(self.reset_buf), self.reset_buf)
# if error in franka positions
franka_dof_pos = self._frankas.get_joint_positions()
is_pos_nan = torch.isnan(franka_dof_pos)
is_pos_fault = torch.any(is_pos_nan,1)
self.reset_buf = torch.where( is_pos_fault == True, torch.ones_like(self.reset_buf), self.reset_buf)
franka_dof_vel = self._frankas.get_joint_velocities()
is_vel_nan = torch.isnan(franka_dof_vel)
is_vel_fault = torch.any(is_vel_nan,1)
self.reset_buf = torch.where( is_vel_fault == True, torch.ones_like(self.reset_buf), self.reset_buf)
# or complete the task
# reset if max length reached
self.reset_buf = torch.where(self.progress_buf >= self._max_episode_length - 1, torch.ones_like(self.reset_buf), self.reset_buf)
else:
self.reset_buf = torch.where(self.progress_buf >= self._max_episode_length - 1, torch.ones_like(self.reset_buf), self.reset_buf)
| 25,478 | Python | 45.750459 | 174 | 0.618455 |
zhehuazhou/ai-cps-robotics-benchmark/Gym_Envs/utils/task_util.py |
def initialize_task(config, env, init_sim=True):
from Tasks.Franka_Door_Open import FrankaDoorOpenTask
from Tasks.Franka_Cloth_Placing import FrankaClothPlacingTask
from Tasks.Franka_Cube_Stacking import FrankaCubeStackingTask
from Tasks.Franka_Ball_Pushing import FrankaBallPushingTask
from Tasks.Franka_Ball_Balancing import FrankaBallBalancingTask
from Tasks.Franka_Ball_Catching import FrankaBallCatchingTask
from Tasks.Franka_Peg_In_Hole import FrankaPegInHoleTask
from Tasks.Franka_Point_Reaching import FrankaPointReachingTask
# Mappings from strings to environments
task_map = {
"FrankaDoorOpen": FrankaDoorOpenTask,
"FrankaBallPushing": FrankaBallPushingTask,
"FrankaBallBalancing": FrankaBallBalancingTask,
"FrankaBallCatching": FrankaBallCatchingTask,
"FrankaPegInHole": FrankaPegInHoleTask,
"FrankaClothPlacing": FrankaClothPlacingTask,
"FrankaCubeStacking": FrankaCubeStackingTask,
"FrankaPointReaching": FrankaPointReachingTask,
}
from omniisaacgymenvs.utils.config_utils.sim_config import SimConfig
sim_config = SimConfig(config)
cfg = sim_config.config
task = task_map[cfg["task_name"]](
name=cfg["task_name"], sim_config=sim_config, env=env
)
env.set_task(task=task, sim_params=sim_config.get_physics_params(), backend="torch", init_sim=init_sim)
return task
| 1,433 | Python | 36.736841 | 107 | 0.742498 |
zhehuazhou/ai-cps-robotics-benchmark/Gym_Envs/Final_Policy/README.md | This folder contains all the final policies after training.
Used for evaluating the learning performance.
| 106 | Markdown | 34.666655 | 59 | 0.839623 |
zhehuazhou/ai-cps-robotics-benchmark/Falsification_Tool/manipulator_testing.py | from model.skrl_oige_model import skrl_oige_model
from monitor.stl_dense_offline import stl_dense_offline_monitor
from optimizer.optimizer import Optimizer
import os
if __name__ == "__main__":
# Task choice: PointReaching, PegInHole, DoorOpen,
# BallBalancing, BallPushing, BallCatching
# CubeStacking, ClothPlacing
task_name = "FrankaBallBalancing"
# agent
agent_type = "PPO" # TRPO, PPO
omniisaacgymenvs_path = os.path.realpath(
os.path.join(os.path.realpath(__file__), "../../Gym_Envs")
)
agent_path = (
omniisaacgymenvs_path
+ "/Final_Policy/BallBalancing/BallBalancing_skrl_"
+ agent_type
+ "/checkpoints/best_agent.pt"
)
# config
simulation_max_steps = 100
num_envs = 1
opt_types = [
# "random",
"NelderMead",
# "DualAnnealing",
] # random, NelderMead, DualAnnealing
global_budget = 4
local_budget = 300
# Load model under test (drl agent + oige env)
test_model = skrl_oige_model(
agent_path=agent_path,
agent_type=agent_type,
task_name=task_name,
num_envs=num_envs,
timesteps=simulation_max_steps,
)
for opt_type in opt_types:
# Load STL monitor based on task
monitor = stl_dense_offline_monitor(task_name=task_name, agent_type=agent_type)
# global search
for i in range(global_budget):
print("Global trial: " + str(i))
# Create optimizer
optimizer = Optimizer(
task_name,
test_model,
monitor,
opt_type=opt_type,
budget_size=local_budget,
)
# local search
results = optimizer.optimize()
print(results)
# close simulation environment
test_model.close_env()
| 1,893 | Python | 24.945205 | 87 | 0.576862 |
zhehuazhou/ai-cps-robotics-benchmark/Falsification_Tool/model/skrl_oige_model.py | import os
import torch
from typing import Optional
from .load_oige import load_oige_test_env
from .agent.PPO_agent import create_skrl_ppo_agent
from .agent.TRPO_agent import create_skrl_trpo_agent
from skrl.envs.torch import wrap_env
class skrl_oige_model(object):
"""Testing environment model based on SKRL and Omniverse Isaac Gym Environments (OIGE)
agent_path: the path to the agent parameters (checkpoint)
oige_path: path to the OIGE environment;
agent_type: type of DRL agent (PPO, DDPG, TRPO)
task_name: the name of the task
num_envs: the number of parallel running environments
headless: if show the GUI
"""
def __init__(
self,
agent_path: str,
oige_path: Optional[str] = None,
agent_type: Optional[str] = None,
task_name: Optional[str] = None,
timesteps: Optional[int] = 10000,
num_envs: Optional[int] = 1,
headless: Optional[bool] = False,
):
# setup
if oige_path is not None:
self.oige_path = oige_path
else:
self.oige_path = os.path.realpath(
os.path.join(os.path.realpath(__file__), "../../../Gym_Envs")
)
if agent_type is not None:
self.agent_type = agent_type
else:
self.agent_type = "PPO"
if task_name is not None:
self.task_name = task_name
else:
self.task_name = "FrankaBallPushing"
self.agent_path = agent_path
self.timesteps = timesteps
self.headless = headless
# Load OIGE env with skrl wrapper
self.num_envs = num_envs # for testing, we use only 1 env for now
env = load_oige_test_env(
task_name=self.task_name,
omniisaacgymenvs_path=self.oige_path,
num_envs=self.num_envs,
)
self.env = wrap_env(env)
self.env._env.set_as_test()
# Load agent
if self.agent_type is "PPO":
self.agent = create_skrl_ppo_agent(self.env, self.agent_path)
elif self.agent_type is "TRPO":
self.agent = create_skrl_trpo_agent(self.env, self.agent_path)
else:
raise ValueError("Agent type unknown.")
# Initialize agent
# cfg_trainer = {"timesteps": self.timesteps, "headless": self.headless}
self.agent.init()
if self.num_envs == 1:
self.agent.set_running_mode("eval")
else:
raise ValueError("Currently only one environment (agent) is supported")
# close env
def close_env(self):
self.env.close()
# Compute the trace w.r.t a given initial condition
def compute_trace(self, initial_value):
# set initial configuration
self.env._env.set_initial_test_value(initial_value)
# reset env
states, infos = self.env.reset()
# initialize trace
trace = states
# simulation loop
for timestep in range(self.timesteps):
# compute actions
with torch.no_grad():
actions = self.agent.act(
states, timestep=timestep, timesteps=self.timesteps
)[0]
# step the environments
next_states, rewards, terminated, truncated, infos = self.env.step(actions)
# render scene
if not self.headless:
self.env.render()
# record trace
states.copy_(next_states)
trace = torch.vstack([trace, states])
# terminate simulation
with torch.no_grad():
if terminated.any() or truncated.any():
break
return trace
# Merge trace based on the task type
def merge_trace(self, trace):
if self.task_name is "FrankaBallPushing":
# Ball hole distance
ball_hole_distance = trace[:, 24:27].detach().cpu()
ball_hole_distance = torch.norm(ball_hole_distance, p=2, dim=-1)
# create index
trace_length = list(ball_hole_distance.size())[0]
times = torch.linspace(1, trace_length, steps=trace_length)
# convert to list for computing robustness
indexed_trace = torch.vstack((times, ball_hole_distance))
indexed_trace = torch.transpose(indexed_trace, 0, 1).tolist()
elif self.task_name is "FrankaBallBalancing":
# Ball tool distance
ball_tool_distance = trace[:, 21:23].detach().cpu()
ball_tool_distance = torch.norm(ball_tool_distance, p=2, dim=-1)
# create index
trace_length = list(ball_tool_distance.size())[0]
times = torch.linspace(1, trace_length, steps=trace_length)
# convert to list for computing robustness
indexed_trace = torch.vstack((times, ball_tool_distance))
indexed_trace = torch.transpose(indexed_trace, 0, 1).tolist()
elif self.task_name is "FrankaBallCatching":
# Ball tool distance
ball_tool_distance = trace[:, 21:23].detach().cpu()
ball_tool_distance = torch.norm(ball_tool_distance, p=2, dim=-1)
# create index
trace_length = list(ball_tool_distance.size())[0]
times = torch.linspace(1, trace_length, steps=trace_length)
# convert to list for computing robustness
indexed_trace = torch.vstack((times, ball_tool_distance))
indexed_trace = torch.transpose(indexed_trace, 0, 1).tolist()
elif self.task_name is "FrankaCubeStacking":
# Cube distance
cube_distance = trace[:, 25:27].detach().cpu()
cube_distance = torch.norm(cube_distance, p=2, dim=-1)
# Cube height
cube_height_distance = trace[:, 27].detach().cpu()
# create index
trace_length = list(cube_distance.size())[0]
times = torch.linspace(1, trace_length, steps=trace_length)
# convert to list for computing robustness
indexed_cube_distance = torch.vstack((times, cube_distance))
indexed_cube_distance = torch.transpose(
indexed_cube_distance, 0, 1
).tolist()
indexed_cube_height_distance = torch.vstack((times, cube_height_distance))
indexed_cube_height_distance = torch.transpose(
indexed_cube_height_distance, 0, 1
).tolist()
indexed_trace = {
"distance_cube": indexed_cube_distance,
"z_cube_distance": indexed_cube_height_distance,
}
elif self.task_name is "FrankaDoorOpen":
# Ball tool distance
handle_rot = trace[:, 21:25].detach().cpu()
handle_yaw = torch.atan2(
2.0
* (
handle_rot[:, 0] * handle_rot[:, 3]
+ handle_rot[:, 1] * handle_rot[:, 2]
),
1.0
- 2.0
* (
handle_rot[:, 2] * handle_rot[:, 2]
+ handle_rot[:, 3] * handle_rot[:, 3]
),
)
handle_yaw = torch.rad2deg(handle_yaw)
# create index
trace_length = list(handle_yaw.size())[0]
times = torch.linspace(1, trace_length, steps=trace_length)
# convert to list for computing robustness
indexed_trace = torch.vstack((times, handle_yaw))
indexed_trace = torch.transpose(indexed_trace, 0, 1).tolist()
elif self.task_name is "FrankaPegInHole":
# Ball tool distance
tool_hole_distance = trace[:, 25:27].detach().cpu()
tool_hole_distance = torch.norm(tool_hole_distance, p=2, dim=-1)
# print(tool_hole_distance)
# create index
trace_length = list(tool_hole_distance.size())[0]
times = torch.linspace(1, trace_length, steps=trace_length)
# convert to list for computing robustness
indexed_trace = torch.vstack((times, tool_hole_distance))
indexed_trace = torch.transpose(indexed_trace, 0, 1).tolist()
elif self.task_name is "FrankaPointReaching":
# Ball tool distance
finger_target_distance = trace[:, 24:27].detach().cpu()
finger_target_distance = torch.norm(finger_target_distance, p=2, dim=-1)
# create index
trace_length = list(finger_target_distance.size())[0]
times = torch.linspace(1, trace_length, steps=trace_length)
# convert to list for computing robustness
indexed_trace = torch.vstack((times, finger_target_distance))
indexed_trace = torch.transpose(indexed_trace, 0, 1).tolist()
elif self.task_name is "FrankaClothPlacing":
# Cube distance
cloth_target_distance = trace[:, 21:24].detach().cpu()
cloth_target_distance = torch.norm(cloth_target_distance, p=2, dim=-1)
# Cube height
cloth_height = trace[:, 20].detach().cpu()
# create index
trace_length = list(cloth_target_distance.size())[0]
times = torch.linspace(1, trace_length, steps=trace_length)
# convert to list for computing robustness
indexed_distance_cloth_target = torch.vstack((times, cloth_target_distance))
indexed_distance_cloth_target = torch.transpose(
indexed_distance_cloth_target, 0, 1
).tolist()
indexed_cloth_height = torch.vstack((times, cloth_height))
indexed_cloth_height = torch.transpose(
indexed_cloth_height, 0, 1
).tolist()
indexed_trace = {
"distance_cloth_target": indexed_distance_cloth_target,
"cloth_height": indexed_cloth_height,
}
else:
raise ValueError("Task name unknown for merging the trace")
return indexed_trace
| 10,099 | Python | 33.589041 | 90 | 0.564115 |
zhehuazhou/ai-cps-robotics-benchmark/Falsification_Tool/model/load_oige.py | """
This is a copy from SKRL's implementation of loading oige environment,
with modifications for generating testing oige environment
"""
import sys
import os
from contextlib import contextmanager
def _omegaconf_to_dict(config) -> dict:
"""Convert OmegaConf config to dict
:param config: The OmegaConf config
:type config: OmegaConf.Config
:return: The config as dict
:rtype: dict
"""
# return config.to_container(dict)
from omegaconf import DictConfig
d = {}
for k, v in config.items():
d[k] = _omegaconf_to_dict(v) if isinstance(v, DictConfig) else v
return d
def _print_cfg(d, indent=0) -> None:
"""Print the environment configuration
:param d: The dictionary to print
:type d: dict
:param indent: The indentation level (default: 0)
:type indent: int, optional
"""
for key, value in d.items():
if isinstance(value, dict):
_print_cfg(value, indent + 1)
else:
print(' | ' * indent + " |-- {}: {}".format(key, value))
def load_oige_test_env(task_name: str = "",
omniisaacgymenvs_path: str = "",
num_envs: int = 1,
show_cfg: bool = True,
timeout: int = 30):
"""Load an Omniverse Isaac Gym environment, this is a slight modification of SKRL's implementation
:param task_name: The name of the task (default: "").
If not specified, the task name is taken from the command line argument (``task=TASK_NAME``).
Command line argument has priority over function parameter if both are specified
:type task_name: str, optional
:param omniisaacgymenvs_path: The path to the ``omniisaacgymenvs`` directory (default: "").
If empty, the path will obtained from omniisaacgymenvs package metadata
:type omniisaacgymenvs_path: str, optional
:param show_cfg: Whether to print the configuration (default: True)
:type show_cfg: bool, optional
:param timeout: Seconds to wait for data when queue is empty in multi-threaded environment (default: 30)
:type timeout: int, optional
:raises ValueError: The task name has not been defined, neither by the function parameter nor by the command line arguments
:raises RuntimeError: The omniisaacgymenvs package is not installed or the path is wrong
:return: Omniverse Isaac Gym environment
:rtype: omni.isaac.gym.vec_env.vec_env_base.VecEnvBase or omni.isaac.gym.vec_env.vec_env_mt.VecEnvMT
"""
import torch
from hydra.types import RunMode
from hydra._internal.hydra import Hydra
from hydra._internal.utils import create_automatic_config_search_path, get_args_parser
from omegaconf import OmegaConf
from omni.isaac.gym.vec_env import VecEnvBase, TaskStopException
import omniisaacgymenvs
sys.argv.append("task={}".format(task_name))
sys.argv.append("num_envs={}".format(num_envs))
# get omniisaacgymenvs path from omniisaacgymenvs package metadata
if omniisaacgymenvs_path == "":
if not hasattr(omniisaacgymenvs, "__path__"):
raise RuntimeError("omniisaacgymenvs package is not installed")
omniisaacgymenvs_path = list(omniisaacgymenvs.__path__)[0]
config_path = os.path.join(omniisaacgymenvs_path, "cfg")
# set omegaconf resolvers
OmegaConf.register_new_resolver('eq', lambda x, y: x.lower() == y.lower())
OmegaConf.register_new_resolver('contains', lambda x, y: x.lower() in y.lower())
OmegaConf.register_new_resolver('if', lambda condition, a, b: a if condition else b)
OmegaConf.register_new_resolver('resolve_default', lambda default, arg: default if arg == '' else arg)
# get hydra config without use @hydra.main
config_file = "config"
args = get_args_parser().parse_args()
search_path = create_automatic_config_search_path(config_file, None, config_path)
hydra_object = Hydra.create_main_hydra2(task_name='load_omniisaacgymenv', config_search_path=search_path)
config = hydra_object.compose_config(config_file, args.overrides, run_mode=RunMode.RUN)
cfg = {}
cfg["task"] = _omegaconf_to_dict(config.task)
cfg["task_name"] = config.task_name
cfg["experiment"] = config.experiment
cfg["num_envs"] = config.num_envs
cfg["seed"] = config.seed
cfg["torch_deterministic"] = config.torch_deterministic
cfg["max_iterations"] = config.max_iterations
cfg["physics_engine"] = config.physics_engine
cfg["pipeline"] = config.pipeline
cfg["sim_device"] = config.sim_device
cfg["device_id"] = config.device_id
cfg["rl_device"] = config.rl_device
cfg["num_threads"] = config.num_threads
cfg["solver_type"] = config.solver_type
cfg["test"] = config.test
cfg["checkpoint"] = config.checkpoint
cfg["headless"] = config.headless
# print config
if show_cfg:
print("\nOmniverse Isaac Gym environment ({})".format(config.task.name))
_print_cfg(cfg)
# internal classes
class _OmniIsaacGymVecEnv(VecEnvBase):
def step(self, actions):
actions = torch.clamp(actions, -self._task.clip_actions, self._task.clip_actions).to(self._task.device).clone()
self._task.pre_physics_step(actions)
for _ in range(self._task.control_frequency_inv):
self._world.step(render=self._render)
self.sim_frame_count += 1
observations, rewards, dones, info = self._task.post_physics_step()
return {"obs": torch.clamp(observations, -self._task.clip_obs, self._task.clip_obs).to(self._task.rl_device).clone()}, \
rewards.to(self._task.rl_device).clone(), dones.to(self._task.rl_device).clone(), info.copy()
def set_as_test(self):
self._task.set_as_test()
def set_initial_test_value(self, value):
self._task.set_initial_test_value(value)
def reset(self):
self._task.reset()
actions = torch.zeros((self.num_envs, self._task.num_actions), device=self._task.device)
return self.step(actions)[0]
# load environment
sys.path.append(omniisaacgymenvs_path)
from utils.task_util import initialize_task
env = _OmniIsaacGymVecEnv(headless=config.headless)
task = initialize_task(cfg, env, init_sim=True)
return env | 6,402 | Python | 40.044872 | 132 | 0.65667 |
zhehuazhou/ai-cps-robotics-benchmark/Falsification_Tool/model/agent/TRPO_agent.py | """
Create PPO agent based on SKRL implementation
"""
import torch.nn as nn
import torch
from skrl.models.torch import Model, GaussianMixin, DeterministicMixin
from skrl.agents.torch.trpo import TRPO, TRPO_DEFAULT_CONFIG
from skrl.resources.preprocessors.torch import RunningStandardScaler
# Define the models (stochastic and deterministic models) for the agent using mixins.
# - Policy: takes as input the environment's observation/state and returns an action
# - Value: takes the state as input and provides a value to guide the policy
class Policy_2_Layers(GaussianMixin, Model):
def __init__(self, observation_space, action_space, device, clip_actions=False,
clip_log_std=True, min_log_std=-20, max_log_std=2):
Model.__init__(self, observation_space, action_space, device)
GaussianMixin.__init__(self, clip_actions, clip_log_std, min_log_std, max_log_std)
self.net = nn.Sequential(nn.Linear(self.num_observations, 512),
nn.ELU(),
nn.Linear(512, 256),
nn.ELU(),
nn.Linear(256, self.num_actions))
self.log_std_parameter = nn.Parameter(torch.zeros(self.num_actions))
def compute(self, inputs, role):
return self.net(inputs["states"]), self.log_std_parameter, {}
class Policy_3_Layers(GaussianMixin, Model):
def __init__(self, observation_space, action_space, device, clip_actions=False,
clip_log_std=True, min_log_std=-20, max_log_std=2):
Model.__init__(self, observation_space, action_space, device)
GaussianMixin.__init__(self, clip_actions, clip_log_std, min_log_std, max_log_std)
self.net = nn.Sequential(nn.Linear(self.num_observations, 512),
nn.ELU(),
nn.Linear(512, 256),
nn.ELU(),
nn.Linear(256, 128),
nn.ELU(),
nn.Linear(128, self.num_actions))
self.log_std_parameter = nn.Parameter(torch.zeros(self.num_actions))
def compute(self, inputs, role):
return self.net(inputs["states"]), self.log_std_parameter, {}
class Value_2_Layers(DeterministicMixin, Model):
def __init__(self, observation_space, action_space, device, clip_actions=False):
Model.__init__(self, observation_space, action_space, device)
DeterministicMixin.__init__(self, clip_actions)
self.net = nn.Sequential(nn.Linear(self.num_observations, 512),
nn.ELU(),
nn.Linear(512, 256),
nn.ELU(),
nn.Linear(256, 1))
def compute(self, inputs, role):
return self.net(inputs["states"]), {}
class Value_3_Layers(DeterministicMixin, Model):
def __init__(self, observation_space, action_space, device, clip_actions=False):
Model.__init__(self, observation_space, action_space, device)
DeterministicMixin.__init__(self, clip_actions)
self.net = nn.Sequential(nn.Linear(self.num_observations, 512),
nn.ELU(),
nn.Linear(512, 256),
nn.ELU(),
nn.Linear(256, 128),
nn.ELU(),
nn.Linear(128, 1))
def compute(self, inputs, role):
return self.net(inputs["states"]), {}
# Create SKRL PPO agent
def create_skrl_trpo_agent(env, agent_path):
device = env.device
models_trpo_2_layer = {}
models_trpo_2_layer["policy"] = Policy_2_Layers(env.observation_space, env.action_space, device)
models_trpo_2_layer["value"] = Value_2_Layers(env.observation_space, env.action_space, device)
models_trpo_3_layer = {}
models_trpo_3_layer["policy"] = Policy_3_Layers(env.observation_space, env.action_space, device)
models_trpo_3_layer["value"] = Value_3_Layers(env.observation_space, env.action_space, device)
# Configs
cfg_trpo = TRPO_DEFAULT_CONFIG.copy()
cfg_trpo["state_preprocessor"] = RunningStandardScaler
cfg_trpo["state_preprocessor_kwargs"] = {"size": env.observation_space, "device": device}
cfg_trpo["value_preprocessor"] = RunningStandardScaler
cfg_trpo["value_preprocessor_kwargs"] = {"size": 1, "device": device}
# no log to TensorBoard and write checkpoints
cfg_trpo["experiment"]["write_interval"] = 0
cfg_trpo["experiment"]["checkpoint_interval"] = 0
try:
# Initialize and load agent with 2 layers
agent = TRPO(models=models_trpo_2_layer,
memory=None,
cfg=cfg_trpo,
observation_space=env.observation_space,
action_space=env.action_space,
device=device)
agent.load(agent_path)
except:
# Initialize and load agent with 3 layers
agent = TRPO(models=models_trpo_3_layer,
memory=None,
cfg=cfg_trpo,
observation_space=env.observation_space,
action_space=env.action_space,
device=device)
agent.load(agent_path)
return agent
| 5,370 | Python | 40 | 100 | 0.581006 |
zhehuazhou/ai-cps-robotics-benchmark/Falsification_Tool/model/agent/PPO_agent.py | """
Create PPO agent based on SKRL implementation
"""
import torch.nn as nn
import torch
from skrl.models.torch import Model, GaussianMixin, DeterministicMixin
from skrl.agents.torch.ppo import PPO, PPO_DEFAULT_CONFIG
from skrl.resources.preprocessors.torch import RunningStandardScaler
# Define the shared model (stochastic and deterministic models) for the agent using mixins.
class Shared(GaussianMixin, DeterministicMixin, Model):
def __init__(self, observation_space, action_space, device, clip_actions=False,
clip_log_std=True, min_log_std=-20, max_log_std=2, reduction="sum"):
Model.__init__(self, observation_space, action_space, device)
GaussianMixin.__init__(self, clip_actions, clip_log_std, min_log_std, max_log_std, reduction)
DeterministicMixin.__init__(self, clip_actions)
self.net = nn.Sequential(nn.Linear(self.num_observations, 512),
nn.ELU(),
nn.Linear(512, 256),
nn.ELU(),
nn.Linear(256, 128),
nn.ELU())
self.mean_layer = nn.Linear(128, self.num_actions)
self.log_std_parameter = nn.Parameter(torch.zeros(self.num_actions))
self.value_layer = nn.Linear(128, 1)
def act(self, inputs, role):
if role == "policy":
return GaussianMixin.act(self, inputs, role)
elif role == "value":
return DeterministicMixin.act(self, inputs, role)
def compute(self, inputs, role):
if role == "policy":
return self.mean_layer(self.net(inputs["states"])), self.log_std_parameter, {}
elif role == "value":
return self.value_layer(self.net(inputs["states"])), {}
# Create SKRL PPO agent
def create_skrl_ppo_agent(env, agent_path):
device = env.device
models_ppo = {}
models_ppo["policy"] = Shared(env.observation_space, env.action_space, device)
models_ppo["value"] = models_ppo["policy"] # same instance: shared model
# Configs
cfg_ppo = PPO_DEFAULT_CONFIG.copy()
cfg_ppo["state_preprocessor"] = RunningStandardScaler
cfg_ppo["state_preprocessor_kwargs"] = {"size": env.observation_space, "device": device}
cfg_ppo["value_preprocessor"] = RunningStandardScaler
cfg_ppo["value_preprocessor_kwargs"] = {"size": 1, "device": device}
# logging to TensorBoard each 100 timesteps and ignore checkpoints
cfg_ppo["experiment"]["write_interval"] = 0
cfg_ppo["experiment"]["checkpoint_interval"] = 0
# Initialize and load agent
agent = PPO(models=models_ppo,
memory=None,
cfg=cfg_ppo,
observation_space=env.observation_space,
action_space=env.action_space,
device=device)
agent.load(agent_path)
return agent
| 2,889 | Python | 36.051282 | 101 | 0.622361 |
zhehuazhou/ai-cps-robotics-benchmark/Falsification_Tool/monitor/stl_dense_offline.py | from rtamt import STLDenseTimeSpecification
from typing import Optional
import sys
class stl_dense_offline_monitor(object):
"""STL dense time offline monitor based rtamt
agent_path: the path to the agent parameters (checkpoint)
oige_path: path to the OIGE environment;
task_name: the name of the task
"""
def __init__(
self,
task_name: Optional[str] = None,
agent_type: Optional[str] = None,
oige_path: Optional[str] = None,
):
if task_name is not None:
self.task_name = task_name
else:
self.task_name = "FrankaBallPushing"
self.agent_type = agent_type
self.generate_spec()
# generate specification based on task name
def generate_spec(self):
# Initialization
self.spec = STLDenseTimeSpecification()
self.spec.name = "STL Dense-time Offline Monitor"
###############################################
# Specification according to task
# Ball Pushing
if self.task_name is "FrankaBallPushing":
self.spec.declare_var("distance_ball_hole", "float")
self.spec.spec = "eventually[1:299](distance_ball_hole <= 0.3) "
# Ball Balancing
elif self.task_name is "FrankaBallBalancing":
self.spec.declare_var("distance_ball_tool", "float")
self.spec.spec = "always[50:200]( distance_ball_tool <= 0.25)"
# Ball Catching
elif self.task_name is "FrankaBallCatching":
self.spec.declare_var("distance_ball_tool", "float")
self.spec.spec = "always[50:299]( distance_ball_tool <= 0.1)"
# Cube Stacking
elif self.task_name is "FrankaCubeStacking":
self.spec.declare_var("distance_cube", "float")
self.spec.declare_var("z_cube_distance", "float")
self.spec.spec = (
"eventually[1:299]((distance_cube<= 0.024) and (z_cube_distance>0) )"
)
# Door Open
elif self.task_name is "FrankaDoorOpen":
self.spec.declare_var("yaw_door", "float")
self.spec.spec = "eventually[1:299]( yaw_door >= 20)"
# Peg In Hole
elif self.task_name is "FrankaPegInHole":
self.spec.declare_var("distance_tool_hole", "float")
self.spec.spec = "always[250:299]( distance_tool_hole <= 0.1)"
# Point Reaching
elif self.task_name is "FrankaPointReaching":
self.spec.declare_var("distance_finger_target", "float")
self.spec.spec = "always[50:299]( distance_finger_target <= 0.12)" # fixed
# Cloth Placing
elif self.task_name is "FrankaClothPlacing":
self.spec.declare_var("distance_cloth_target", "float")
self.spec.declare_var("cloth_height", "float")
self.spec.spec = "eventually[1:299]( (distance_cloth_target <= 0.25))" # and (cloth_height > 0.1) )"
else:
raise ValueError("Task name unknown for defining the specification")
################################################
# Load specification
try:
self.spec.parse()
except rtamt.STLParseException as err:
print("STL Parse Exception: {}".format(err))
sys.exit()
# Compute the robustness given trace
def compute_robustness(self, trace):
if self.task_name is "FrankaBallPushing":
# print(trace)
robustness = self.spec.evaluate(["distance_ball_hole", trace])
# print(robustness)
elif self.task_name is "FrankaBallBalancing":
robustness = self.spec.evaluate(["distance_ball_tool", trace])
elif self.task_name is "FrankaBallCatching":
robustness = self.spec.evaluate(["distance_ball_tool", trace])
elif self.task_name is "FrankaCubeStacking":
distance_cube = trace["distance_cube"]
z_cube_distance = trace["z_cube_distance"]
robustness = self.spec.evaluate(
["distance_cube", distance_cube], ["z_cube_distance", z_cube_distance]
)
elif self.task_name is "FrankaDoorOpen":
robustness = self.spec.evaluate(["yaw_door", trace])
elif self.task_name is "FrankaPegInHole":
robustness = self.spec.evaluate(["distance_tool_hole", trace])
elif self.task_name is "FrankaPointReaching":
robustness = self.spec.evaluate(["distance_finger_target", trace])
elif self.task_name is "FrankaClothPlacing":
distance_cloth_target = trace["distance_cloth_target"]
cloth_height = trace["cloth_height"]
robustness = self.spec.evaluate(
["distance_cloth_target", distance_cloth_target]#, ["cloth_height", cloth_height]
)
else:
raise ValueError("Task name unknown for defining the specification")
return robustness
| 4,981 | Python | 30.732484 | 112 | 0.582614 |
zhehuazhou/ai-cps-robotics-benchmark/Falsification_Tool/optimizer/optimizer.py | from typing import Optional
import sys
import numpy as np
import torch
import time
from scipy.optimize import minimize
from scipy.optimize import dual_annealing
class Optimizer(object):
"""Optimizer class for testing
task_name: the task name of environment
test_model: the model under test
monitor: the monitor for the STL specification
opt_type: type of the optimizer
budget_size: local budget size
"""
def __init__(
self,
task_name,
test_model,
monitor,
opt_type: Optional[str] = "random",
budget_size: Optional[int] = 1000,
):
self.task_name = task_name
self.test_model = test_model
self.monitor = monitor
self.opt_type = opt_type
self.budget_size = budget_size
self.fal_succ = False
self.start_time = time.time()
self.fal_time = 0
self.fal_sim = 0
self.worst_rob = 1000
# initial value bounds
if self.task_name is "FrankaBallPushing":
self.bnds = ((-0.1, 0.1), (-0.1, 0.1))
elif self.task_name is "FrankaBallBalancing":
self.bnds = ((-0.15, 0.15), (-0.15, 0.15))
elif self.task_name is "FrankaBallCatching":
# self.bnds = ((-0.1, 0.1), (-0.2, 0.2), (1.0, 3.0), (-1.0, 1.0))
self.bnds = ((-0.05, 0.05), (-0.05, 0.05), (1.0, 1.001), (0.0, 0.001))
elif self.task_name is "FrankaCubeStacking":
self.bnds = ((-0.2, 0.2), (-0.2, 0.2))
elif self.task_name is "FrankaDoorOpen":
self.bnds = ((-0.025, 0.025), (-0.05, 0.05))
elif self.task_name is "FrankaPegInHole":
self.bnds = ((-0.1, 0.1), (-0.1, 0.1))
elif self.task_name is "FrankaPointReaching":
self.bnds = ((-0.2, 0.2), (-0.4, 0.4), (-0.2, 0.2))
elif self.task_name is "FrankaClothPlacing":
self.bnds = ((-0.1, 0.2), (-0.35, 0.35))
else:
raise ValueError("Task name unknown for generating the initial values")
# generate initial values based on the task type
def generate_initial(self):
if self.task_name is "FrankaBallPushing":
# ball inside an area x:[-0.1,0.1], y:[-0.1,0.1]
value_1 = np.random.rand(1) * (0.1 + 0.1) - 0.1
value_2 = np.random.rand(1) * (0.1 + 0.1) - 0.1
initial_value = np.hstack((value_1, value_2))
elif self.task_name is "FrankaBallBalancing":
# ball inside an area x:[-0.15,0.15], y:[-0.15,0.15]
value_1 = np.random.rand(1) * (0.15 + 0.15) - 0.15
value_2 = np.random.rand(1) * (0.15 + 0.15) - 0.15
initial_value = np.hstack((value_1, value_2))
elif self.task_name is "FrankaBallCatching":
# ball inside an area x:[-0.1,0.1], y:[-0.1,0.1]
# ball velociry: vx: [1.0,1.5], vy: [0.0,0.2]
value_1 = np.random.rand(1) * (0.05 + 0.05) - 0.05
value_2 = np.random.rand(1) * (0.05 + 0.05) - 0.05
value_3 = np.random.rand(1) * (1.0 - 1.0) + 1.0
value_4 = np.random.rand(1) * (0.0 + 0.0) + 0.0
initial_value = np.hstack((value_1, value_2, value_3, value_4))
elif self.task_name is "FrankaCubeStacking":
# target cube inside an area x:[-0.2,0.2], y:[-0.2,0.2]
value_1 = np.random.rand(1) * (0.2 + 0.2) - 0.2
value_2 = np.random.rand(1) * (0.2 + 0.2) - 0.2
initial_value = np.hstack((value_1, value_2))
elif self.task_name is "FrankaDoorOpen":
# target inside an area x:[-0.1,0.1], y:[-0.4,0.4]
value_1 = np.random.rand(1) * (0.005 + 0.005) - 0.005
value_2 = np.random.rand(1) * (0.025 + 0.025) - 0.025
initial_value = np.hstack((value_1, value_2))
elif self.task_name is "FrankaPegInHole":
# target inside an area x:[-0.2,0.2], y:[-0.2,0.2]
value_1 = np.random.rand(1) * (0.1 + 0.1) - 0.1
value_2 = np.random.rand(1) * (0.1 + 0.1) - 0.1
initial_value = np.hstack((value_1, value_2))
elif self.task_name is "FrankaPointReaching":
# target inside an area x:[-0.2,0.2], y:[-0.4,0.4], z:[-0.2,0.2]
value_1 = np.random.rand(1) * (0.2 + 0.2) - 0.2
value_2 = np.random.rand(1) * (0.4 + 0.4) - 0.4
value_3 = np.random.rand(1) * (0.2 + 0.2) - 0.2
initial_value = np.hstack((value_1, value_2, value_3))
elif self.task_name is "FrankaClothPlacing":
# target inside an area x:[-0.1,0.2], y:[-0.35,0.35]
value_1 = np.random.rand(1) * (0.2 + 0.1) - 0.1
value_2 = np.random.rand(1) * (0.35 + 0.35) - 0.35
initial_value = np.hstack((value_1, value_2))
else:
raise ValueError("Task name unknown for generating the initial values")
return initial_value
# Generate one function (input: initial values, output: robustness) for testing algorithms
def robustness_function(self, initial_value):
# print("Initial Value:", initial_value)
# Get trace
trace = self.test_model.compute_trace(initial_value)
indexed_trace = self.test_model.merge_trace(trace)
# compute robustness
rob_sequence = self.monitor.compute_robustness(indexed_trace)
rob_sequence = np.array(rob_sequence)
# RTAMT is for monitoring, so for eventually, the robustness computed from the current timepoint to the end
# workaround to compute the maximum
if (
self.task_name is "FrankaBallPushing"
or self.task_name is "FrankaCubeStacking"
or self.task_name is "FrankaDoorOpen"
or self.task_name is "FrankaPegInHole"
or self.task_name is "FrankaClothPlacing"
):
min_rob = np.max(rob_sequence[:, 1])
else:
min_rob = np.min(rob_sequence[:, 1])
# print("Min Robustness:", min_rob)
if min_rob < self.worst_rob:
self.worst_rob = min_rob
if min_rob < 0 and self.fal_succ == False:
self.fal_succ = True
self.fal_time = time.time() - self.start_time
elif self.fal_succ == False:
self.fal_sim += 1
return min_rob
# optimization based on the optimizer type
def optimize(self):
if self.opt_type is "random":
results = self.optimize_random()
return results
elif self.opt_type is "NelderMead":
results = self.optimize_nelder_mead()
return results
elif self.opt_type is "DualAnnealing":
results = self.optimize_dual_annealing()
return results
else:
raise ValueError("Optimizer type undefined!")
# Random optimization
def optimize_random(self):
# worst_initial = None
# worst_trace = None
initial_value_record = None
rob_value_record = None
# Random optimizer
for i in range(self.budget_size):
# random initial value
initial_value = self.generate_initial()
# compute robustness
min_rob = self.robustness_function(initial_value)
# update record
if i == 0:
initial_value_record = initial_value
rob_value_record = np.array([min_rob])
# worst_initial = initial_value
# worst_trace = trace
self.worst_rob = min_rob
else:
initial_value_record = np.vstack((initial_value_record, initial_value))
rob_value_record = np.vstack((rob_value_record, np.array([min_rob])))
if min_rob < self.worst_rob:
# worst_initial = initial_value
# worst_trace = trace
self.worst_rob = min_rob
if min_rob < 0:
# self.fal_succ = True
# self.fal_time = time.time() - self.start_time
if i == 0:
self.fal_sim = 1
break
# results = {'worst_initial': worst_initial, 'worst_rob': worst_rob,
# 'initial_value_record': initial_value_record, 'rob_value_record': rob_value_record}
if self.fal_succ == False:
self.fal_time = time.time() - self.start_time
results = [self.fal_succ, self.fal_time, self.fal_sim, self.worst_rob]
return results
# Nelder Mead optimization
def optimize_nelder_mead(self):
initial_guess = self.generate_initial()
# minimization
results = minimize(
self.robustness_function,
initial_guess,
method="Nelder-Mead",
bounds=self.bnds,
options={"maxfev": self.budget_size, "disp": True},
)
if self.fal_succ == False:
self.fal_time = time.time() - self.start_time
results = [self.fal_succ, self.fal_time, self.fal_sim, self.worst_rob]
return results
# Dual Annealing optimization
def optimize_dual_annealing(self):
# minimization
results = dual_annealing(
self.robustness_function,
bounds=self.bnds,
# maxiter=self.budget_size, # global search number
maxfun=self.budget_size, # local search number
# no_local_search = True,
)
if self.fal_succ == False:
self.fal_time = time.time() - self.start_time
results = [self.fal_succ, self.fal_time, self.fal_sim, self.worst_rob]
return results
| 9,690 | Python | 30.77377 | 115 | 0.540248 |
StanfordVL/OmniGibson/mkdocs.yml | yaml-language-server: $schema=https://squidfunk.github.io/mkdocs-material/schema.json
site_name: OmniGibson Documentation
repo_name: StanfordVL/OmniGibson
repo_url: https://github.com/StanfordVL/OmniGibson
theme:
name: material
logo: assets/OmniGibson_logo.png
favicon: assets/OmniGibson_logo.png
icon:
repo: fontawesome/brands/git-alt
features:
- navigation.tracking
- navigation.tabs
- content.code.copy
extra:
homepage: https://behavior.stanford.edu
custom_dir: .overrides
# color info
palette:
# Palette toggle for light mode
- media: "(prefers-color-scheme: light)"
primary: white
accent: indigo
scheme: default
toggle:
icon: material/toggle-switch
name: Switch to dark mode
# Palette toggle for dark mode
- media: "(prefers-color-scheme: dark)"
primary: blue grey
accent: indigo
scheme: slate
toggle:
icon: material/toggle-switch-off-outline
name: Switch to light mode
extra_css:
- stylesheets/extra.css
markdown_extensions:
- pymdownx.emoji:
emoji_index: !!python/name:material.extensions.emoji.twemoji
emoji_generator: !!python/name:materialx.emoji.to_svg
- admonition
- pymdownx.details
- pymdownx.superfences
- pymdownx.tabbed:
alternate_style: true
- pymdownx.highlight:
anchor_linenums: true
- pymdownx.inlinehilite
- pymdownx.snippets:
base_path: omnigibson
- pymdownx.tasklist:
custom_checkbox: true
- attr_list
- md_in_html
# extra plugins
plugins:
- search
- autorefs
- mkdocstrings:
handlers:
python:
paths: [omnigibson]
# - social
- gen-files:
scripts:
- docs/gen_ref_pages.py
- literate-nav:
nav_file: SUMMARY.md
- section-index
- offline
nav:
- Home: index.md
- Getting Started:
- Installation: getting_started/installation.md
- Quickstart: getting_started/quickstart.md
- Examples: getting_started/examples.md
- Running on SLURM: getting_started/slurm.md
- Modules:
- Overview: modules/overview.md
- Prim: modules/prim.md
- Object: modules/object.md
- Object States: modules/object_states.md
- Robots: modules/robots.md
- Controllers: modules/controllers.md
- Sensor: modules/sensor.md
- Scene: modules/scene.md
- Environment: modules/environment.md
- Tutorials:
- Demo Collection: tutorials/demo_collection.md
- API Reference: reference/*
- Miscellaneous:
- FAQ: miscellaneous/faq.md
- Known Issues & Troubleshooting: miscellaneous/known_issues.md
- Contributing: miscellaneous/contributing.md
- Changelog: https://github.com/StanfordVL/OmniGibson/releases
- Contact Us: miscellaneous/contact.md
extra:
analytics:
provider: google
property: G-6L1G6GMR63 | 2,838 | YAML | 24.123894 | 85 | 0.681818 |
StanfordVL/OmniGibson/pyproject.toml | [tool.black]
line-length = 120
target-version = ['py27', 'py36', 'py37']
force-exclude = 'omnigibson/(data|external)'
[tool.isort]
profile = "black"
line_length = 120
py_version = 'all'
filter_files = true
extend_skip_glob = [
'omnigibson/data/*',
'omnigibson/external/*'
]
[tool.pyright]
exclude = [
'omnigibson/data',
'omnigibson/docs',
'omnigibson/docker'
]
[tool.pytest.ini_options]
testpaths = [
"tests",
]
[tool.coverage.run]
omit = ["omnigibson/external/*"]
| 487 | TOML | 15.266666 | 44 | 0.659138 |
StanfordVL/OmniGibson/setup.py | # read the contents of your README file
from os import path
from setuptools import find_packages, setup
this_directory = path.abspath(path.dirname(__file__))
with open(path.join(this_directory, "README.md"), encoding="utf-8") as f:
lines = f.readlines()
# remove images from README
lines = [x for x in lines if ".png" not in x]
long_description = "".join(lines)
setup(
name="omnigibson",
version="1.0.0",
author="Stanford University",
long_description_content_type="text/markdown",
long_description=long_description,
url="https://github.com/StanfordVL/OmniGibson",
zip_safe=False,
packages=find_packages(),
install_requires=[
"gym~=0.26.2",
"numpy~=1.23.5",
"scipy~=1.10.1",
"GitPython~=3.1.40",
"transforms3d~=0.4.1",
"networkx~=3.2.1",
"PyYAML~=6.0.1",
"addict~=2.4.0",
"ipython~=8.20.0",
"future~=0.18.3",
"trimesh~=4.0.8",
"h5py~=3.10.0",
"cryptography~=41.0.7",
"bddl~=3.5.0",
"opencv-python~=4.8.1",
"nest_asyncio~=1.5.8",
"imageio~=2.33.1",
"imageio-ffmpeg~=0.4.9",
"termcolor~=2.4.0",
"progressbar~=2.5",
"pymeshlab~=2022.2",
"click~=8.1.3",
"aenum~=3.1.15",
"rtree~=1.2.0",
],
tests_require=[],
python_requires=">=3",
package_data={"": ["omnigibson/global_config.yaml"]},
include_package_data=True,
) # yapf: disable
| 1,483 | Python | 26.481481 | 73 | 0.559676 |
StanfordVL/OmniGibson/README.md | 
# <h1><img height="40" src="./docs/assets/OmniGibson_logo.png" style="float:left;padding-right:10px"> OmniGibson</h1>
[](https://github.com/StanfordVL/OmniGibson/actions/workflows/tests.yml)
[](https://hub.docker.com/r/stanfordvl/omnigibson)
[](https://stanfordvl.github.io/OmniGibson/profiling/)
-------
### Need support? Join our Discord!
<a href="https://discord.gg/bccR5vGFEx"><img src="https://discordapp.com/api/guilds/1166422812160966707/widget.png?style=banner3"></a>
-------
### Latest Updates
- [03/17/24] **v1.0.0**: First full release with 1,004 pre-sampled tasks, all 50 scenes, and many new objects! [[release notes]](https://github.com/StanfordVL/OmniGibson/releases/tag/v1.0.0)
- [08/04/23] **v0.2.0**: More assets! 600 pre-sampled tasks, 7 new scenes, and many new objects 📈 [[release notes]](https://github.com/StanfordVL/OmniGibson/releases/tag/v0.2.0)
- [04/10/22] **v0.1.0**: Significantly improved stability, performance, and ease of installation :wrench: [[release notes]](https://github.com/StanfordVL/OmniGibson/releases/tag/v0.1.0)
-------
**`OmniGibson`** is a platform for accelerating Embodied AI research built upon NVIDIA's [Omniverse](https://www.nvidia.com/en-us/omniverse/) platform, featuring:
* 📸 Photorealistic Visuals and 📐 Physical Realism
* 🌊 Fluid and 👕 Soft Body Support
* 🏔️ Large-Scale, High-Quality Scenes and 🎾 Objects
* 🌡️ Dynamic Kinematic and Semantic Object States
* 🤖 Mobile Manipulator Robots with Modular ⚙️ Controllers
* 🌎 OpenAI Gym Interface
Check out [**`OmniGibson`**'s documentation](https://behavior.stanford.edu/omnigibson/getting_started/installation.html) to get started!
### Citation
If you use **`OmniGibson`** or its assets and models, please cite:
```
@inproceedings{
li2022behavior,
title={{BEHAVIOR}-1K: A Benchmark for Embodied {AI} with 1,000 Everyday Activities and Realistic Simulation},
author={Chengshu Li and Ruohan Zhang and Josiah Wong and Cem Gokmen and Sanjana Srivastava and Roberto Mart{\'\i}n-Mart{\'\i}n and Chen Wang and Gabrael Levine and Michael Lingelbach and Jiankai Sun and Mona Anvari and Minjune Hwang and Manasi Sharma and Arman Aydin and Dhruva Bansal and Samuel Hunter and Kyu-Young Kim and Alan Lou and Caleb R Matthews and Ivan Villa-Renteria and Jerry Huayang Tang and Claire Tang and Fei Xia and Silvio Savarese and Hyowon Gweon and Karen Liu and Jiajun Wu and Li Fei-Fei},
booktitle={6th Annual Conference on Robot Learning},
year={2022},
url={https://openreview.net/forum?id=_8DoIe8G3t}
}
```
### Profiling
Click on the plot to access our profiling page with more examples.
[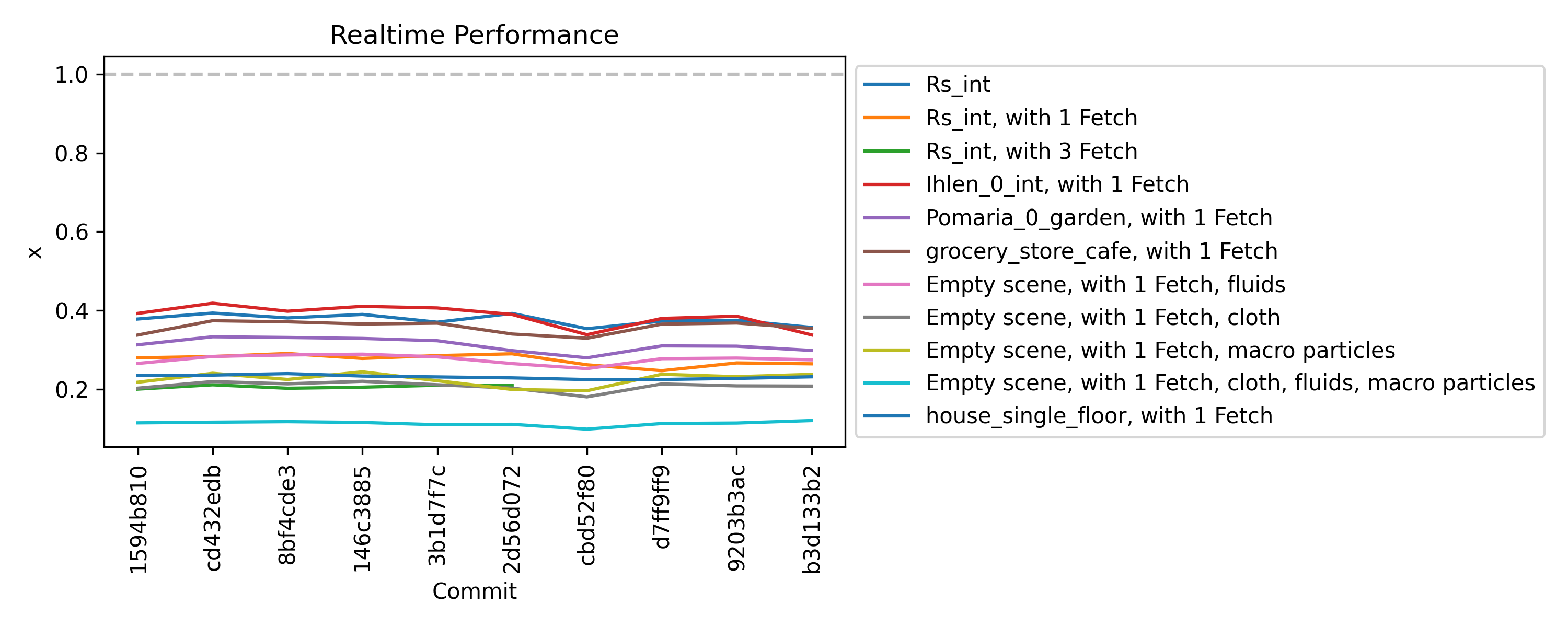](https://stanfordvl.github.io/OmniGibson/profiling/)
| 3,072 | Markdown | 56.981131 | 511 | 0.75293 |
StanfordVL/OmniGibson/scripts/download_datasets.py | """
Helper script to download OmniGibson dataset and assets.
"""
import os
os.environ["OMNIGIBSON_NO_OMNIVERSE"] = "1"
from omnigibson.macros import gm
from omnigibson.utils.asset_utils import download_og_dataset, download_assets
import click
def main():
# Only execute if the dataset path or asset path does not exist
dataset_exists, assets_exist = os.path.exists(gm.DATASET_PATH), os.path.exists(gm.ASSET_PATH)
if not (dataset_exists and assets_exist):
# Ask user which dataset to install
print(f"OmniGibson will now install data under the following locations:")
print(f" dataset (~25GB): {gm.DATASET_PATH}")
print(f" assets (~2.5GB): {gm.ASSET_PATH}")
print(f"If you want to install data under a different path, please change the DATA_PATH variable in omnigibson/macros.py and rerun scripts/download_dataset.py.")
if click.confirm("Do you want to continue?"):
# Only download if the dataset path doesn't exist
if not dataset_exists:
print("Downloading dataset...")
download_og_dataset()
# Only download if the asset path doesn't exist
if not assets_exist:
print("Downloading assets...")
download_assets()
print("\nOmniGibson setup completed!\n")
else:
print("You chose not to install dataset for now. You can install it later by running python scripts/download_dataset.py.")
if __name__ == "__main__":
main()
| 1,533 | Python | 38.333332 | 169 | 0.64775 |
StanfordVL/OmniGibson/omnigibson/simulator.py | from collections import defaultdict
import itertools
import contextlib
import logging
import os
import shutil
import socket
from pathlib import Path
import atexit
import signal
from contextlib import nullcontext
import numpy as np
import json
import omnigibson as og
import omnigibson.lazy as lazy
from omnigibson.macros import gm, create_module_macros
from omnigibson.utils.constants import LightingMode
from omnigibson.utils.config_utils import NumpyEncoder
from omnigibson.utils.python_utils import clear as clear_pu, create_object_from_init_info, Serializable
from omnigibson.utils.sim_utils import meets_minimum_isaac_version
from omnigibson.utils.usd_utils import clear as clear_uu, FlatcacheAPI, RigidContactAPI, PoseAPI
from omnigibson.utils.ui_utils import (CameraMover, disclaimer, create_module_logger, suppress_omni_log,
print_icon, print_logo, logo_small)
from omnigibson.scenes import Scene
from omnigibson.objects.object_base import BaseObject
from omnigibson.objects.stateful_object import StatefulObject
from omnigibson.object_states.contact_subscribed_state_mixin import ContactSubscribedStateMixin
from omnigibson.object_states.joint_break_subscribed_state_mixin import JointBreakSubscribedStateMixin
from omnigibson.object_states.factory import get_states_by_dependency_order
from omnigibson.object_states.update_state_mixin import UpdateStateMixin, GlobalUpdateStateMixin
from omnigibson.prims.material_prim import MaterialPrim
from omnigibson.sensors.vision_sensor import VisionSensor
from omnigibson.systems.macro_particle_system import MacroPhysicalParticleSystem
from omnigibson.transition_rules import TransitionRuleAPI
# Create module logger
log = create_module_logger(module_name=__name__)
# Create settings for this module
m = create_module_macros(module_path=__file__)
m.DEFAULT_VIEWER_CAMERA_POS = (-0.201028, -2.72566 , 1.0654)
m.DEFAULT_VIEWER_CAMERA_QUAT = (0.68196617, -0.00155408, -0.00166678, 0.73138017)
m.OBJECT_GRAVEYARD_POS = (100.0, 100.0, 100.0)
# Helper functions for starting omnigibson
def print_save_usd_warning(_):
log.warning("Exporting individual USDs has been disabled in OG due to copyrights.")
def _launch_app():
log.info(f"{'-' * 5} Starting {logo_small()}. This will take 10-30 seconds... {'-' * 5}")
# If multi_gpu is used, og.sim.render() will cause a segfault when called during on_contact callbacks,
# e.g. when an attachment joint is being created due to contacts (create_joint calls og.sim.render() internally).
gpu_id = None if gm.GPU_ID is None else int(gm.GPU_ID)
config_kwargs = {"headless": gm.HEADLESS or bool(gm.REMOTE_STREAMING), "multi_gpu": False}
if gpu_id is not None:
config_kwargs["active_gpu"] = gpu_id
config_kwargs["physics_gpu"] = gpu_id
# Omni's logging is super annoying and overly verbose, so suppress it by modifying the logging levels
if not gm.DEBUG:
import sys
from numba.core.errors import NumbaPerformanceWarning
import warnings
# TODO: Find a more elegant way to prune omni logging
# sys.argv.append("--/log/level=warning")
# sys.argv.append("--/log/fileLogLevel=warning")
# sys.argv.append("--/log/outputStreamLevel=error")
warnings.simplefilter("ignore", category=NumbaPerformanceWarning)
# Copy the OmniGibson kit file to the Isaac Sim apps directory. This is necessary because the Isaac Sim app
# expects the extensions to be reachable in the parent directory of the kit file. We copy on every launch to
# ensure that the kit file is always up to date.
assert "EXP_PATH" in os.environ, "The EXP_PATH variable is not set. Are you in an Isaac Sim installed environment?"
kit_file = Path(__file__).parent / "omnigibson.kit"
kit_file_target = Path(os.environ["EXP_PATH"]) / "omnigibson.kit"
try:
shutil.copy(kit_file, kit_file_target)
except Exception as e:
raise e from ValueError("Failed to copy omnigibson.kit to Isaac Sim apps directory.")
launch_context = nullcontext if gm.DEBUG else suppress_omni_log
with launch_context(None):
app = lazy.omni.isaac.kit.SimulationApp(config_kwargs, experience=str(kit_file_target.resolve(strict=True)))
assert meets_minimum_isaac_version("2023.1.1"), "This version of OmniGibson supports Isaac Sim 2023.1.1 and above. Please update Isaac Sim."
# Omni overrides the global logger to be DEBUG, which is very annoying, so we re-override it to the default WARN
# TODO: Remove this once omniverse fixes it
logging.getLogger().setLevel(logging.WARNING)
# Enable additional extensions we need
lazy.omni.isaac.core.utils.extensions.enable_extension("omni.flowusd")
lazy.omni.isaac.core.utils.extensions.enable_extension("omni.particle.system.bundle")
# Additional import for windows
if os.name == "nt":
lazy.omni.isaac.core.utils.extensions.enable_extension("omni.kit.window.viewport")
# Default Livestream settings
if gm.REMOTE_STREAMING:
app.set_setting("/app/window/drawMouse", True)
app.set_setting("/app/livestream/proto", "ws")
app.set_setting("/app/livestream/websocket/framerate_limit", 120)
app.set_setting("/ngx/enabled", False)
# Find our IP address
s = socket.socket(socket.AF_INET, socket.SOCK_DGRAM)
s.connect(("8.8.8.8", 80))
ip = s.getsockname()[0]
s.close()
# Note: Only one livestream extension can be enabled at a time
if gm.REMOTE_STREAMING == "native":
# Enable Native Livestream extension
# Default App: Streaming Client from the Omniverse Launcher
lazy.omni.isaac.core.utils.extensions.enable_extension("omni.kit.livestream.native")
print(f"Now streaming on {ip} via Omniverse Streaming Client")
elif gm.REMOTE_STREAMING == "webrtc":
# Enable WebRTC Livestream extension
app.set_setting("/exts/omni.services.transport.server.http/port", gm.HTTP_PORT)
app.set_setting("/app/livestream/port", gm.WEBRTC_PORT)
lazy.omni.isaac.core.utils.extensions.enable_extension("omni.services.streamclient.webrtc")
print(f"Now streaming on: http://{ip}:{gm.HTTP_PORT}/streaming/webrtc-client?server={ip}")
else:
raise ValueError(f"Invalid REMOTE_STREAMING option {gm.REMOTE_STREAMING}. Must be one of None, native, webrtc.")
# If we're headless, suppress all warnings about GLFW
if gm.HEADLESS:
og_log = lazy.omni.log.get_log()
og_log.set_channel_enabled("carb.windowing-glfw.plugin", False, lazy.omni.log.SettingBehavior.OVERRIDE)
# Globally suppress certain logging modules (unless we're in debug mode) since they produce spurious warnings
if not gm.DEBUG:
og_log = lazy.omni.log.get_log()
for channel in ["omni.hydra.scene_delegate.plugin", "omni.kit.manipulator.prim.model"]:
og_log.set_channel_enabled(channel, False, lazy.omni.log.SettingBehavior.OVERRIDE)
# Possibly hide windows if in debug mode
hide_window_names = []
if not gm.RENDER_VIEWER_CAMERA:
hide_window_names.append("Viewport")
if gm.GUI_VIEWPORT_ONLY:
hide_window_names.extend(["Console", "Main ToolBar", "Stage", "Layer", "Property", "Render Settings", "Content",
"Flow", "Semantics Schema Editor"])
for name in hide_window_names:
window = lazy.omni.ui.Workspace.get_window(name)
if window is not None:
window.visible = False
app.update()
lazy.omni.kit.widget.stage.context_menu.ContextMenu.save_prim = print_save_usd_warning
# TODO: Automated cleanup in callback doesn't work for some reason. Need to investigate.
shutdown_stream = lazy.omni.kit.app.get_app().get_shutdown_event_stream()
sub = shutdown_stream.create_subscription_to_pop(og.cleanup, name="og_cleanup", order=0)
# Loading Isaac Sim disables Ctrl+C, so we need to re-enable it
signal.signal(signal.SIGINT, og.shutdown_handler)
return app
def launch_simulator(*args, **kwargs):
if not og.app:
og.app = _launch_app()
class Simulator(lazy.omni.isaac.core.simulation_context.SimulationContext, Serializable):
"""
Simulator class for directly interfacing with the physx physics engine.
NOTE: This is a monolithic class.
All created Simulator() instances will reference the same underlying Simulator object
Args:
gravity (float): gravity on z direction.
physics_dt (float): dt between physics steps. Defaults to 1.0 / 120.0.
rendering_dt (float): dt between rendering steps. Note: rendering means rendering a frame of the current
application and not only rendering a frame to the viewports/ cameras. So UI elements of Isaac Sim will
be refreshed with this dt as well if running non-headless. Defaults to 1.0 / 30.0.
stage_units_in_meters (float): The metric units of assets. This will affect gravity value..etc.
Defaults to 0.01.
viewer_width (int): width of the camera image, in pixels
viewer_height (int): height of the camera image, in pixels
device (None or str): specifies the device to be used if running on the gpu with torch backend
"""
_world_initialized = False
def __init__(
self,
gravity=9.81,
physics_dt=1.0 / 120.0,
rendering_dt=1.0 / 30.0,
stage_units_in_meters=1.0,
viewer_width=gm.DEFAULT_VIEWER_WIDTH,
viewer_height=gm.DEFAULT_VIEWER_HEIGHT,
device=None,
):
# Store vars needed for initialization
self.gravity = gravity
self._viewer_camera = None
self._camera_mover = None
# Run super init
super().__init__(
physics_dt=physics_dt,
rendering_dt=rendering_dt,
stage_units_in_meters=stage_units_in_meters,
device=device,
)
if self._world_initialized:
return
Simulator._world_initialized = True
# Store other references to variables that will be initialized later
self._scene = None
self._physx_interface = None
self._physx_simulation_interface = None
self._physx_scene_query_interface = None
self._contact_callback = None
self._simulation_event_callback = None
# List of objects that need to be initialized during whenever the next sim step occurs
self._objects_to_initialize = []
self._objects_require_contact_callback = False
self._objects_require_joint_break_callback = False
# Maps callback name to callback
self._callbacks_on_play = dict()
self._callbacks_on_stop = dict()
self._callbacks_on_import_obj = dict()
self._callbacks_on_remove_obj = dict()
# Mapping from link IDs assigned from omni to the object that they reference
self._link_id_to_objects = dict()
# Set of categories that can be grasped by assisted grasping
self.object_state_types = get_states_by_dependency_order()
self.object_state_types_requiring_update = \
[state for state in self.object_state_types if (issubclass(state, UpdateStateMixin) or issubclass(state, GlobalUpdateStateMixin))]
self.object_state_types_on_contact = \
{state for state in self.object_state_types if issubclass(state, ContactSubscribedStateMixin)}
self.object_state_types_on_joint_break = \
{state for state in self.object_state_types if issubclass(state, JointBreakSubscribedStateMixin)}
# Auto-load the dummy stage
self.clear()
# Set the viewer dimensions
if gm.RENDER_VIEWER_CAMERA:
self.viewer_width = viewer_width
self.viewer_height = viewer_height
# Toggle simulator state once so that downstream omni features can be used without bugs
# e.g.: particle sampling, which for some reason requires sim.play() to be called at least once
self.play()
self.stop()
# Update the physics settings
# This needs to be done now, after an initial step + stop for some reason if we want to use GPU
# dynamics, otherwise we get very strange behavior, e.g., PhysX complains about invalid transforms
# and crashes
self._set_physics_engine_settings()
def __new__(
cls,
gravity=9.81,
physics_dt=1.0 / 120.0,
rendering_dt=1.0 / 30.0,
stage_units_in_meters=1.0,
viewer_width=gm.DEFAULT_VIEWER_WIDTH,
viewer_height=gm.DEFAULT_VIEWER_HEIGHT,
device_idx=0,
):
# Overwrite since we have different kwargs
if Simulator._instance is None:
Simulator._instance = object.__new__(cls)
else:
lazy.carb.log_info("Simulator is defined already, returning the previously defined one")
return Simulator._instance
def _set_viewer_camera(self, prim_path="/World/viewer_camera", viewport_name="Viewport"):
"""
Creates a camera prim dedicated for this viewer at @prim_path if it doesn't exist,
and sets this camera as the active camera for the viewer
Args:
prim_path (str): Path to check for / create the viewer camera
viewport_name (str): Name of the viewport this camera should attach to. Default is "Viewport", which is
the default viewport's name in Isaac Sim
"""
self._viewer_camera = VisionSensor(
prim_path=prim_path,
name=prim_path.split("/")[-1], # Assume name is the lowest-level name in the prim_path
modalities="rgb",
image_height=self.viewer_height,
image_width=self.viewer_width,
viewport_name=viewport_name,
)
if not self._viewer_camera.loaded:
self._viewer_camera.load()
# We update its clipping range and focal length so we get a good FOV and so that it doesn't clip
# nearby objects (default min is 1 m)
self._viewer_camera.clipping_range = [0.001, 10000000.0]
self._viewer_camera.focal_length = 17.0
# Initialize the sensor
self._viewer_camera.initialize()
# Also need to potentially update our camera mover if it already exists
if self._camera_mover is not None:
self._camera_mover.set_cam(cam=self._viewer_camera)
def _set_physics_engine_settings(self):
"""
Set the physics engine with specified settings
"""
assert self.is_stopped(), f"Cannot set simulator physics settings while simulation is playing!"
self._physics_context.set_gravity(value=-self.gravity)
# Also make sure we don't invert the collision group filter settings so that different collision groups by
# default collide with each other, and modify settings for speed optimization
self._physics_context.set_invert_collision_group_filter(False)
self._physics_context.enable_ccd(gm.ENABLE_CCD)
self._physics_context.enable_fabric(gm.ENABLE_FLATCACHE)
# Enable GPU dynamics based on whether we need omni particles feature
if gm.USE_GPU_DYNAMICS:
self._physics_context.enable_gpu_dynamics(True)
self._physics_context.set_broadphase_type("GPU")
else:
self._physics_context.enable_gpu_dynamics(False)
self._physics_context.set_broadphase_type("MBP")
# Set GPU Pairs capacity and other GPU settings
self._physics_context.set_gpu_found_lost_pairs_capacity(gm.GPU_PAIRS_CAPACITY)
self._physics_context.set_gpu_found_lost_aggregate_pairs_capacity(gm.GPU_AGGR_PAIRS_CAPACITY)
self._physics_context.set_gpu_total_aggregate_pairs_capacity(gm.GPU_AGGR_PAIRS_CAPACITY)
self._physics_context.set_gpu_max_particle_contacts(gm.GPU_MAX_PARTICLE_CONTACTS)
self._physics_context.set_gpu_max_rigid_contact_count(gm.GPU_MAX_RIGID_CONTACT_COUNT)
self._physics_context.set_gpu_max_rigid_patch_count(gm.GPU_MAX_RIGID_PATCH_COUNT)
def _set_renderer_settings(self):
if gm.ENABLE_HQ_RENDERING:
lazy.carb.settings.get_settings().set_bool("/rtx/reflections/enabled", True)
lazy.carb.settings.get_settings().set_bool("/rtx/indirectDiffuse/enabled", True)
lazy.carb.settings.get_settings().set_int("/rtx/post/dlss/execMode", 3) # "Auto"
lazy.carb.settings.get_settings().set_bool("/rtx/ambientOcclusion/enabled", True)
lazy.carb.settings.get_settings().set_bool("/rtx/directLighting/sampledLighting/enabled", False)
else:
lazy.carb.settings.get_settings().set_bool("/rtx/reflections/enabled", False)
lazy.carb.settings.get_settings().set_bool("/rtx/indirectDiffuse/enabled", False)
lazy.carb.settings.get_settings().set_int("/rtx/post/dlss/execMode", 0) # "Performance"
lazy.carb.settings.get_settings().set_bool("/rtx/ambientOcclusion/enabled", False)
lazy.carb.settings.get_settings().set_bool("/rtx/directLighting/sampledLighting/enabled", True)
lazy.carb.settings.get_settings().set_int("/rtx/raytracing/showLights", 1)
lazy.carb.settings.get_settings().set_float("/rtx/sceneDb/ambientLightIntensity", 0.1)
@property
def viewer_visibility(self):
"""
Returns:
bool: Whether the viewer is visible or not
"""
return self._viewer_camera.viewer_visibility
@viewer_visibility.setter
def viewer_visibility(self, visible):
"""
Sets whether the viewer should be visible or not in the Omni UI
Args:
visible (bool): Whether the viewer should be visible or not
"""
self._viewer_camera.viewer_visibility = visible
@property
def viewer_height(self):
"""
Returns:
int: viewer height of this sensor, in pixels
"""
# If the viewer camera hasn't been created yet, utilize the default width
return gm.DEFAULT_VIEWER_HEIGHT if self._viewer_camera is None else self._viewer_camera.image_height
@viewer_height.setter
def viewer_height(self, height):
"""
Sets the viewer height @height for this sensor
Args:
height (int): viewer height, in pixels
"""
self._viewer_camera.image_height = height
@property
def viewer_width(self):
"""
Returns:
int: viewer width of this sensor, in pixels
"""
# If the viewer camera hasn't been created yet, utilize the default height
return gm.DEFAULT_VIEWER_WIDTH if self._viewer_camera is None else self._viewer_camera.image_width
@viewer_width.setter
def viewer_width(self, width):
"""
Sets the viewer width @width for this sensor
Args:
width (int): viewer width, in pixels
"""
self._viewer_camera.image_width = width
def set_lighting_mode(self, mode):
"""
Sets the active lighting mode in the current simulator. Valid options are one of LightingMode
Args:
mode (LightingMode): Lighting mode to set
"""
lazy.omni.kit.commands.execute("SetLightingMenuModeCommand", lighting_mode=mode)
def enable_viewer_camera_teleoperation(self):
"""
Enables keyboard control of the active viewer camera for this simulation
"""
assert gm.RENDER_VIEWER_CAMERA, "Viewer camera must be enabled to enable teleoperation!"
self._camera_mover = CameraMover(cam=self._viewer_camera)
self._camera_mover.print_info()
return self._camera_mover
def import_scene(self, scene):
"""
Import a scene into the simulator. A scene could be a synthetic one or a realistic Gibson Environment.
Args:
scene (Scene): a scene object to load
"""
assert self.is_stopped(), "Simulator must be stopped while importing a scene!"
assert isinstance(scene, Scene), "import_scene can only be called with Scene"
# Clear the existing scene if any
self.clear()
# Initialize all global updatable object states
for state in self.object_state_types_requiring_update:
if issubclass(state, GlobalUpdateStateMixin):
state.global_initialize()
self._scene = scene
self._scene.load()
# Make sure simulator is not running, then start it so that we can initialize the scene
assert self.is_stopped(), "Simulator must be stopped after importing a scene!"
self.play()
# Initialize the scene
self._scene.initialize()
# Need to one more step for particle systems to work
self.step()
self.stop()
log.info("Imported scene.")
def initialize_object_on_next_sim_step(self, obj):
"""
Initializes the object upon the next simulation step
Args:
obj (BasePrim): Object to initialize as soon as a new sim step is called
"""
self._objects_to_initialize.append(obj)
def import_object(self, obj, register=True):
"""
Import an object into the simulator.
Args:
obj (BaseObject): an object to load
register (bool): whether to register this object internally in the scene registry
"""
assert isinstance(obj, BaseObject), "import_object can only be called with BaseObject"
# Make sure scene is loaded -- objects should not be loaded unless we have a reference to a scene
assert self.scene is not None, "import_object needs to be called after import_scene"
# Load the object in omniverse by adding it to the scene
self.scene.add_object(obj, register=register, _is_call_from_simulator=True)
# Run any callbacks
for callback in self._callbacks_on_import_obj.values():
callback(obj)
# Cache the mapping from link IDs to object
for link in obj.links.values():
self._link_id_to_objects[lazy.pxr.PhysicsSchemaTools.sdfPathToInt(link.prim_path)] = obj
# Lastly, additionally add this object automatically to be initialized as soon as another simulator step occurs
self.initialize_object_on_next_sim_step(obj=obj)
def remove_object(self, obj):
"""
Remove one or a list of non-robot object from the simulator.
Args:
obj (BaseObject or Iterable[BaseObject]): one or a list of non-robot objects to remove
"""
objs = [obj] if isinstance(obj, BaseObject) else obj
if self.is_playing():
state = self.dump_state()
# Omniverse has a strange bug where if GPU dynamics is on and the object to remove is in contact with
# with another object (in some specific configuration only, not always), the simulator crashes. Therefore,
# we first move the object to a safe location, then remove it.
pos = list(m.OBJECT_GRAVEYARD_POS)
for ob in objs:
ob.set_position_orientation(pos, [0, 0, 0, 1])
pos[0] += max(ob.aabb_extent)
# One physics timestep will elapse
self.step_physics()
for ob in objs:
self._remove_object(ob)
if self.is_playing():
# Update all handles that are now broken because objects have changed
self.update_handles()
# Load the state back
self.load_state(state)
# Refresh all current rules
TransitionRuleAPI.prune_active_rules()
def _remove_object(self, obj):
"""
Remove a non-robot object from the simulator. Should not be called directly by the user.
Args:
obj (BaseObject): a non-robot object to remove
"""
# Run any callbacks
for callback in self._callbacks_on_remove_obj.values():
callback(obj)
# pop all link ids
for link in obj.links.values():
self._link_id_to_objects.pop(lazy.pxr.PhysicsSchemaTools.sdfPathToInt(link.prim_path))
# If it was queued up to be initialized, remove it from the queue as well
for i, initialize_obj in enumerate(self._objects_to_initialize):
if obj.name == initialize_obj.name:
self._objects_to_initialize.pop(i)
break
self._scene.remove_object(obj)
def remove_prim(self, prim):
"""
Remove a prim from the simulator.
Args:
prim (BasePrim): a prim to remove
"""
# [omni.physx.tensors.plugin] prim '[prim_path]' was deleted while being used by a shape in a tensor view
# class. The physics.tensors simulationView was invalidated.
with suppress_omni_log(channels=["omni.physx.tensors.plugin"]):
# Remove prim
prim.remove()
# Update all handles that are now broken because prims have changed
self.update_handles()
def _reset_variables(self):
"""
Reset internal variables when a new stage is loaded
"""
def render(self):
super().render()
# During rendering, the Fabric API is updated, so we can mark it as clean
PoseAPI.mark_valid()
def update_handles(self):
# Handles are only relevant when physx is running
if not self.is_playing():
return
# First, refresh the physics sim view
self._physics_sim_view = lazy.omni.physics.tensors.create_simulation_view(self.backend)
self._physics_sim_view.set_subspace_roots("/")
# Then update the handles for all objects
if self.scene is not None and self.scene.initialized:
for obj in self.scene.objects:
# Only need to update if object is already initialized as well
if obj.initialized:
obj.update_handles()
for system in self.scene.systems:
if issubclass(system, MacroPhysicalParticleSystem):
system.refresh_particles_view()
# Finally update any unified views
RigidContactAPI.initialize_view()
def _non_physics_step(self):
"""
Complete any non-physics steps such as state updates.
"""
# If we don't have a valid scene, immediately return
if self._scene is None:
return
# Update omni
self._omni_update_step()
# If we're playing we, also run additional logic
if self.is_playing():
# Check to see if any objects should be initialized (only done IF we're playing)
n_objects_to_initialize = len(self._objects_to_initialize)
if n_objects_to_initialize > 0 and self.is_playing():
# We iterate through the objects to initialize
# Note that we don't explicitly do for obj in self._objects_to_initialize because additional objects
# may be added mid-iteration!!
# For this same reason, after we finish the loop, we keep any objects that are yet to be initialized
# First call zero-physics step update, so that handles are properly propagated
og.sim.pi.update_simulation(elapsedStep=0, currentTime=og.sim.current_time)
for i in range(n_objects_to_initialize):
obj = self._objects_to_initialize[i]
obj.initialize()
if len(obj.states.keys() & self.object_state_types_on_contact) > 0:
self._objects_require_contact_callback = True
if len(obj.states.keys() & self.object_state_types_on_joint_break) > 0:
self._objects_require_joint_break_callback = True
self._objects_to_initialize = self._objects_to_initialize[n_objects_to_initialize:]
# Re-initialize the physics view because the number of objects has changed
self.update_handles()
# Also refresh the transition rules that are currently active
TransitionRuleAPI.refresh_all_rules()
# Update any system-related state
for system in self.scene.systems:
system.update()
# Propagate states if the feature is enabled
if gm.ENABLE_OBJECT_STATES:
# Step the object states in global topological order (if the scene exists)
for state_type in self.object_state_types_requiring_update:
if issubclass(state_type, GlobalUpdateStateMixin):
state_type.global_update()
if issubclass(state_type, UpdateStateMixin):
for obj in self.scene.get_objects_with_state(state_type):
# Update the state (object should already be initialized since
# this step will only occur after objects are initialized and sim
# is playing
obj.states[state_type].update()
for obj in self.scene.objects:
# Only update visuals for objects that have been initialized so far
if isinstance(obj, StatefulObject) and obj.initialized:
obj.update_visuals()
# Possibly run transition rule step
if gm.ENABLE_TRANSITION_RULES:
TransitionRuleAPI.step()
def _omni_update_step(self):
"""
Step any omni-related things
"""
# Clear the bounding box and contact caches so that they get updated during the next time they're called
RigidContactAPI.clear()
def play(self):
if not self.is_playing():
# Track whether we're starting the simulator fresh -- i.e.: whether we were stopped previously
was_stopped = self.is_stopped()
# Run super first
# We suppress warnings from omni.usd because it complains about values set in the native USD
# These warnings occur because the native USD file has some type mismatch in the `scale` property,
# where the property expects a double but for whatever reason the USD interprets its values as floats
# We suppress omni.physicsschema.plugin when kinematic_only objects are placed with scale ~1.0, to suppress
# the following error:
# [omni.physicsschema.plugin] ScaleOrientation is not supported for rigid bodies, prim path: [...] You may
# ignore this if the scale is close to uniform.
# We also need to suppress the following error when flat cache is used:
# [omni.physx.plugin] Transformation change on non-root links is not supported.
channels = ["omni.usd", "omni.physicsschema.plugin"]
if gm.ENABLE_FLATCACHE:
channels.append("omni.physx.plugin")
with suppress_omni_log(channels=channels):
super().play()
# Take a render step -- this is needed so that certain (unknown, maybe omni internal state?) is populated
# correctly.
self.render()
# Update all object handles, unless this is a play during initialization
if og.sim is not None:
self.update_handles()
if was_stopped:
# We need to update controller mode because kp and kd were set to the original (incorrect) values when
# sim was stopped. We need to reset them to default_kp and default_kd defined in ControllableObject.
# We also need to take an additional sim step to make sure simulator is functioning properly.
# We need to do this because for some reason omniverse exhibits strange behavior if we do certain
# operations immediately after playing; e.g.: syncing USD poses when flatcache is enabled
if self.scene is not None and self.scene.initialized:
for robot in self.scene.robots:
if robot.initialized:
robot.update_controller_mode()
# Also refresh any transition rules that became stale while sim was stopped
TransitionRuleAPI.refresh_all_rules()
# Additionally run non physics things
self._non_physics_step()
# Run all callbacks
for callback in self._callbacks_on_play.values():
callback()
def pause(self):
if not self.is_paused():
super().pause()
def stop(self):
if not self.is_stopped():
super().stop()
# If we're using flatcache, we also need to reset its API
if gm.ENABLE_FLATCACHE:
FlatcacheAPI.reset()
# Run all callbacks
for callback in self._callbacks_on_stop.values():
callback()
@property
def n_physics_timesteps_per_render(self):
"""
Number of physics timesteps per rendering timestep. rendering_dt has to be a multiple of physics_dt.
Returns:
int: Discrete number of physics timesteps to take per step
"""
n_physics_timesteps_per_render = self.get_rendering_dt() / self.get_physics_dt()
assert n_physics_timesteps_per_render.is_integer(), "render_timestep must be a multiple of physics_timestep"
return int(n_physics_timesteps_per_render)
def step(self, render=True):
"""
Step the simulation at self.render_timestep
Args:
render (bool): Whether rendering should occur or not
"""
# If we have imported any objects within the last timestep, we render the app once, since otherwise calling
# step() may not step physics
if len(self._objects_to_initialize) > 0:
self.render()
if render:
super().step(render=True)
else:
for i in range(self.n_physics_timesteps_per_render):
super().step(render=False)
# Additionally run non physics things
self._non_physics_step()
# TODO (eric): After stage changes (e.g. pose, texture change), it will take two super().step(render=True) for
# the result to propagate to the rendering. We could have called super().render() here but it will introduce
# a big performance regression.
def step_physics(self):
"""
Step the physics a single step.
"""
self._physics_context._step(current_time=self.current_time)
self._omni_update_step()
PoseAPI.invalidate()
def _on_contact(self, contact_headers, contact_data):
"""
This callback will be invoked after every PHYSICS step if there is any contact.
For each of the pair of objects in each contact, we invoke the on_contact function for each of its states
that subclass ContactSubscribedStateMixin. These states update based on contact events.
"""
if gm.ENABLE_OBJECT_STATES and self._objects_require_contact_callback:
headers = defaultdict(list)
for contact_header in contact_headers:
actor0_obj = self._link_id_to_objects.get(contact_header.actor0, None)
actor1_obj = self._link_id_to_objects.get(contact_header.actor1, None)
# If any of the objects cannot be found, skip
if actor0_obj is None or actor1_obj is None:
continue
# If any of the objects is not initialized, skip
if not actor0_obj.initialized or not actor1_obj.initialized:
continue
# If any of the objects is not stateful, skip
if not isinstance(actor0_obj, StatefulObject) or not isinstance(actor1_obj, StatefulObject):
continue
# If any of the objects doesn't have states that require on_contact callbacks, skip
if len(actor0_obj.states.keys() & self.object_state_types_on_contact) == 0 or len(actor1_obj.states.keys() & self.object_state_types_on_contact) == 0:
continue
headers[tuple(sorted((actor0_obj, actor1_obj), key=lambda x: x.uuid))].append(contact_header)
for (actor0_obj, actor1_obj) in headers:
for obj0, obj1 in [(actor0_obj, actor1_obj), (actor1_obj, actor0_obj)]:
for state_type in self.object_state_types_on_contact:
if state_type in obj0.states:
obj0.states[state_type].on_contact(obj1, headers[(actor0_obj, actor1_obj)], contact_data)
def _on_simulation_event(self, event):
"""
This callback will be invoked if there is any simulation event. Currently it only processes JOINT_BREAK event.
"""
if gm.ENABLE_OBJECT_STATES:
if event.type == int(lazy.omni.physx.bindings._physx.SimulationEvent.JOINT_BREAK) and self._objects_require_joint_break_callback:
joint_path = str(lazy.pxr.PhysicsSchemaTools.decodeSdfPath(event.payload["jointPath"][0], event.payload["jointPath"][1]))
obj = None
# TODO: recursively try to find the parent object of this joint
tokens = joint_path.split("/")
for i in range(2, len(tokens) + 1):
obj = self._scene.object_registry("prim_path", "/".join(tokens[:i]))
if obj is not None:
break
if obj is None or not obj.initialized or not isinstance(obj, StatefulObject):
return
if len(obj.states.keys() & self.object_state_types_on_joint_break) == 0:
return
for state_type in self.object_state_types_on_joint_break:
if state_type in obj.states:
obj.states[state_type].on_joint_break(joint_path)
def is_paused(self):
"""
Returns:
bool: True if the simulator is paused, otherwise False
"""
return not (self.is_stopped() or self.is_playing())
@contextlib.contextmanager
def stopped(self):
"""
A context scope for making sure the simulator is stopped during execution within this scope.
Upon leaving the scope, the prior simulator state is restored.
"""
# Infer what state we're currently in, then stop, yield, and then restore the original state
sim_is_playing, sim_is_paused = self.is_playing(), self.is_paused()
if sim_is_playing or sim_is_paused:
self.stop()
yield
if sim_is_playing: self.play()
elif sim_is_paused: self.pause()
@contextlib.contextmanager
def playing(self):
"""
A context scope for making sure the simulator is playing during execution within this scope.
Upon leaving the scope, the prior simulator state is restored.
"""
# Infer what state we're currently in, then stop, yield, and then restore the original state
sim_is_stopped, sim_is_paused = self.is_stopped(), self.is_paused()
if sim_is_stopped or sim_is_paused:
self.play()
yield
if sim_is_stopped: self.stop()
elif sim_is_paused: self.pause()
@contextlib.contextmanager
def paused(self):
"""
A context scope for making sure the simulator is paused during execution within this scope.
Upon leaving the scope, the prior simulator state is restored.
"""
# Infer what state we're currently in, then stop, yield, and then restore the original state
sim_is_stopped, sim_is_playing = self.is_stopped(), self.is_playing()
if sim_is_stopped or sim_is_playing:
self.pause()
yield
if sim_is_stopped: self.stop()
elif sim_is_playing: self.play()
@contextlib.contextmanager
def slowed(self, dt):
"""
A context scope for making the simulator simulation dt slowed, e.g.: for taking micro-steps for propagating
instantaneous kinematics with minimal impact on physics propagation.
NOTE: This will set both the physics dt and rendering dt to the same value during this scope.
Upon leaving the scope, the prior simulator state is restored.
"""
# Set dt, yield, then restore the original dt
physics_dt, rendering_dt = self.get_physics_dt(), self.get_rendering_dt()
self.set_simulation_dt(physics_dt=dt, rendering_dt=dt)
yield
self.set_simulation_dt(physics_dt=physics_dt, rendering_dt=rendering_dt)
def add_callback_on_play(self, name, callback):
"""
Adds a function @callback, referenced by @name, to be executed every time sim.play() is called
Args:
name (str): Name of the callback
callback (function): Callback function. Function signature is expected to be:
def callback() --> None
"""
self._callbacks_on_play[name] = callback
def add_callback_on_stop(self, name, callback):
"""
Adds a function @callback, referenced by @name, to be executed every time sim.stop() is called
Args:
name (str): Name of the callback
callback (function): Callback function. Function signature is expected to be:
def callback() --> None
"""
self._callbacks_on_stop[name] = callback
def add_callback_on_import_obj(self, name, callback):
"""
Adds a function @callback, referenced by @name, to be executed every time sim.import_object() is called
Args:
name (str): Name of the callback
callback (function): Callback function. Function signature is expected to be:
def callback(obj: BaseObject) --> None
"""
self._callbacks_on_import_obj[name] = callback
def add_callback_on_remove_obj(self, name, callback):
"""
Adds a function @callback, referenced by @name, to be executed every time sim.remove_object() is called
Args:
name (str): Name of the callback
callback (function): Callback function. Function signature is expected to be:
def callback(obj: BaseObject) --> None
"""
self._callbacks_on_remove_obj[name] = callback
def remove_callback_on_play(self, name):
"""
Remove play callback whose reference is @name
Args:
name (str): Name of the callback
"""
self._callbacks_on_play.pop(name, None)
def remove_callback_on_stop(self, name):
"""
Remove stop callback whose reference is @name
Args:
name (str): Name of the callback
"""
self._callbacks_on_stop.pop(name, None)
def remove_callback_on_import_obj(self, name):
"""
Remove stop callback whose reference is @name
Args:
name (str): Name of the callback
"""
self._callbacks_on_import_obj.pop(name, None)
def remove_callback_on_remove_obj(self, name):
"""
Remove stop callback whose reference is @name
Args:
name (str): Name of the callback
"""
self._callbacks_on_remove_obj.pop(name, None)
@classmethod
def clear_instance(cls):
lazy.omni.isaac.core.simulation_context.SimulationContext.clear_instance()
Simulator._world_initialized = None
return
def __del__(self):
lazy.omni.isaac.core.simulation_context.SimulationContext.__del__(self)
Simulator._world_initialized = None
return
@property
def pi(self):
"""
Returns:
PhysX: Physx Interface (pi) for controlling low-level physx engine
"""
return self._physx_interface
@property
def psi(self):
"""
Returns:
IPhysxSimulation: Physx Simulation Interface (psi) for controlling low-level physx simulation
"""
return self._physx_simulation_interface
@property
def psqi(self):
"""
Returns:
PhysXSceneQuery: Physx Scene Query Interface (psqi) for running low-level scene queries
"""
return self._physx_scene_query_interface
@property
def scene(self):
"""
Returns:
None or Scene: Scene currently loaded in this simulator. If no scene is loaded, returns None
"""
return self._scene
@property
def viewer_camera(self):
"""
Returns:
VisionSensor: Active camera sensor corresponding to the active viewport window instance shown in the omni UI
"""
return self._viewer_camera
@property
def camera_mover(self):
"""
Returns:
None or CameraMover: If enabled, the teleoperation interface for controlling the active viewer camera
"""
return self._camera_mover
@property
def world_prim(self):
"""
Returns:
Usd.Prim: Prim at /World
"""
return lazy.omni.isaac.core.utils.prims.get_prim_at_path(prim_path="/World")
def clear(self) -> None:
"""
Clears the stage leaving the PhysicsScene only if under /World.
"""
# Stop the physics
self.stop()
# Clear any pre-existing scene if it exists
if self._scene is not None:
self.scene.clear()
self._scene = None
# Clear all vision sensors and remove viewer camera reference and camera mover reference
VisionSensor.clear()
self._viewer_camera = None
if self._camera_mover is not None:
self._camera_mover.clear()
self._camera_mover = None
# Clear all global update states
for state in self.object_state_types_requiring_update:
if issubclass(state, GlobalUpdateStateMixin):
state.global_clear()
# Clear all materials
MaterialPrim.clear()
# Clear all transition rules
TransitionRuleAPI.clear()
# Clear uniquely named items and other internal states
clear_pu()
clear_uu()
self._objects_to_initialize = []
self._objects_require_contact_callback = False
self._objects_require_joint_break_callback = False
self._link_id_to_objects = dict()
self._callbacks_on_play = dict()
self._callbacks_on_stop = dict()
self._callbacks_on_import_obj = dict()
self._callbacks_on_remove_obj = dict()
# Load dummy stage, but don't clear sim to prevent circular loops
self._open_new_stage()
def write_metadata(self, key, data):
"""
Writes metadata @data to the current global metadata dict using key @key
Args:
key (str): Keyword entry in the global metadata dictionary to use
data (dict): Data to write to @key in the global metadata dictionary
"""
self.world_prim.SetCustomDataByKey(key, data)
def get_metadata(self, key):
"""
Grabs metadata from the current global metadata dict using key @key
Args:
key (str): Keyword entry in the global metadata dictionary to use
"""
return self.world_prim.GetCustomDataByKey(key)
def restore(self, json_path):
"""
Restore a simulation environment from @json_path.
Args:
json_path (str): Full path of JSON file to load, which contains information
to recreate a scene.
"""
if not json_path.endswith(".json"):
log.error(f"You have to define the full json_path to load from. Got: {json_path}")
return
# Load the info from the json
with open(json_path, "r") as f:
scene_info = json.load(f)
init_info = scene_info["init_info"]
state = scene_info["state"]
# Override the init info with our json path
init_info["args"]["scene_file"] = json_path
# Also make sure we have any additional modifications necessary from the specific scene
og.REGISTERED_SCENES[init_info["class_name"]].modify_init_info_for_restoring(init_info=init_info)
# Recreate and import the saved scene
og.sim.stop()
recreated_scene = create_object_from_init_info(init_info)
self.import_scene(scene=recreated_scene)
# Start the simulation and restore the dynamic state of the scene and then pause again
self.play()
self.load_state(state, serialized=False)
log.info("The saved simulation environment loaded.")
return
def save(self, json_path):
"""
Saves the current simulation environment to @json_path.
Args:
json_path (str): Full path of JSON file to save (should end with .json), which contains information
to recreate the current scene.
"""
# Make sure the sim is not stopped, since we need to grab joint states
assert not self.is_stopped(), "Simulator cannot be stopped when saving to USD!"
# Make sure there are no objects in the initialization queue, if not, terminate early and notify user
# Also run other sanity checks before saving
if len(self._objects_to_initialize) > 0:
log.error("There are still objects to initialize! Please take one additional sim step and then save.")
return
if not self.scene:
log.warning("Scene has not been loaded. Nothing to save.")
return
if not json_path.endswith(".json"):
log.error(f"You have to define the full json_path to save the scene to. Got: {json_path}")
return
# Update scene info
self.scene.update_objects_info()
# Dump saved current state and also scene init info
scene_info = {
"metadata": self.world_prim.GetCustomData(),
"state": self.scene.dump_state(serialized=False),
"init_info": self.scene.get_init_info(),
"objects_info": self.scene.get_objects_info(),
}
# Write this to the json file
Path(os.path.dirname(json_path)).mkdir(parents=True, exist_ok=True)
with open(json_path, "w+") as f:
json.dump(scene_info, f, cls=NumpyEncoder, indent=4)
log.info("The current simulation environment saved.")
def _open_new_stage(self):
"""
Opens a new stage
"""
# Stop the physics if we're playing
if not self.is_stopped():
log.warning("Stopping simulation in order to open new stage.")
self.stop()
# Store physics dt and rendering dt to reuse later
# Note that the stage may have been deleted previously; if so, we use the default values
# of 1/120, 1/30
try:
physics_dt = self.get_physics_dt()
except:
print("WARNING: Invalid or non-existent physics scene found. Setting physics dt to 1/120.")
physics_dt = 1 / 120.
rendering_dt = self.get_rendering_dt()
# Open new stage -- suppressing warning that we're opening a new stage
with suppress_omni_log(None):
lazy.omni.isaac.core.utils.stage.create_new_stage()
# Clear physics context
self._physics_context = None
self._physx_fabric_interface = None
# Create world prim
self.stage.DefinePrim("/World", "Xform")
self._init_stage(physics_dt=physics_dt, rendering_dt=rendering_dt)
def _load_stage(self, usd_path):
"""
Open the stage specified by USD file at @usd_path
Args:
usd_path (str): Absolute filepath to USD stage that should be loaded
"""
# Stop the physics if we're playing
if not self.is_stopped():
log.warning("Stopping simulation in order to load stage.")
self.stop()
# Store physics dt and rendering dt to reuse later
# Note that the stage may have been deleted previously; if so, we use the default values
# of 1/120, 1/30
try:
physics_dt = self.get_physics_dt()
except:
print("WARNING: Invalid or non-existent physics scene found. Setting physics dt to 1/120.")
physics_dt = 1/120.
rendering_dt = self.get_rendering_dt()
# Open new stage -- suppressing warning that we're opening a new stage
with suppress_omni_log(None):
lazy.omni.isaac.core.utils.stage.open_stage(usd_path=usd_path)
self._init_stage(physics_dt=physics_dt, rendering_dt=rendering_dt)
def _init_stage(
self,
physics_dt=None,
rendering_dt=None,
stage_units_in_meters=None,
physics_prim_path="/physicsScene",
sim_params=None,
set_defaults=True,
backend="numpy",
device=None,
):
# Run super first
super()._init_stage(
physics_dt=physics_dt,
rendering_dt=rendering_dt,
stage_units_in_meters=stage_units_in_meters,
physics_prim_path=physics_prim_path,
sim_params=sim_params,
set_defaults=set_defaults,
backend=backend,
device=device,
)
# Update internal vars
self._physx_interface = lazy.omni.physx.get_physx_interface()
self._physx_simulation_interface = lazy.omni.physx.get_physx_simulation_interface()
self._physx_scene_query_interface = lazy.omni.physx.get_physx_scene_query_interface()
# Update internal settings
self._set_physics_engine_settings()
self._set_renderer_settings()
# Update internal callbacks
self._setup_default_callback_fns()
self._stage_open_callback = (
lazy.omni.usd.get_context().get_stage_event_stream().create_subscription_to_pop(self._stage_open_callback_fn)
)
self._contact_callback = self._physics_context._physx_sim_interface.subscribe_contact_report_events(self._on_contact)
self._simulation_event_callback = self._physx_interface.get_simulation_event_stream_v2().create_subscription_to_pop(self._on_simulation_event)
# Set the lighting mode to be stage by default
self.set_lighting_mode(mode=LightingMode.STAGE)
# Set the viewer camera, and then set its default pose
if gm.RENDER_VIEWER_CAMERA:
self._set_viewer_camera()
self.viewer_camera.set_position_orientation(
position=np.array(m.DEFAULT_VIEWER_CAMERA_POS),
orientation=np.array(m.DEFAULT_VIEWER_CAMERA_QUAT),
)
def close(self):
"""
Shuts down the OmniGibson application
"""
self._app.shutdown()
@property
def stage_id(self):
"""
Returns:
int: ID of the current active stage
"""
return lazy.pxr.UsdUtils.StageCache.Get().GetId(self.stage).ToLongInt()
@property
def device(self):
"""
Returns:
device (None or str): Device used in simulation backend
"""
return self._device
@device.setter
def device(self, device):
"""
Sets the device used for sim backend
Args:
device (None or str): Device to set for the simulation backend
"""
self._device = device
if self._device is not None and "cuda" in self._device:
device_id = self._settings.get_as_int("/physics/cudaDevice")
self._device = f"cuda:{device_id}"
@property
def state_size(self):
# Total state size is the state size of our scene
return self._scene.state_size
def _dump_state(self):
# Default state is from the scene
return self._scene.dump_state(serialized=False)
def _load_state(self, state):
# Default state is from the scene
self._scene.load_state(state=state, serialized=False)
def load_state(self, state, serialized=False):
# We need to make sure the simulator is playing since joint states only get updated when playing
assert self.is_playing()
# Run super
super().load_state(state=state, serialized=serialized)
# Highlight that at the current step, the non-kinematic states are potentially inaccurate because a sim
# step is needed to propagate specific states in physics backend
# TODO: This should be resolved in a future omniverse release!
disclaimer("Attempting to load simulator state.\n"
"Currently, omniverse does not support exclusively stepping kinematics, so we cannot update some "
"of our object states relying on updated kinematics until a simulator step is taken!\n"
"Object states such as OnTop, Inside, etc. relying on relative spatial information will inaccurate"
"until a single sim step is taken.\n"
"This should be resolved by the next NVIDIA Isaac Sim release.")
def _serialize(self, state):
# Default state is from the scene
return self._scene.serialize(state=state)
def _deserialize(self, state):
# Default state is from the scene
return self._scene.deserialize(state=state), self._scene.state_size
if not og.sim:
og.sim = Simulator(*args, **kwargs)
print()
print_icon()
print_logo()
print()
log.info(f"{'-' * 10} Welcome to {logo_small()}! {'-' * 10}")
return og.sim | 62,251 | Python | 43.370634 | 170 | 0.585661 |
StanfordVL/OmniGibson/omnigibson/transition_rules.py | import operator
from abc import ABCMeta, abstractmethod
from collections import namedtuple, defaultdict
import numpy as np
import json
from copy import copy
import itertools
import os
from collections import defaultdict
import networkx as nx
import omnigibson as og
from omnigibson.macros import gm, create_module_macros
from omnigibson.systems import get_system, is_system_active, PhysicalParticleSystem, VisualParticleSystem, REGISTERED_SYSTEMS
from omnigibson.objects.dataset_object import DatasetObject
from omnigibson.object_states import *
from omnigibson.object_states.factory import get_system_states
from omnigibson.object_states.object_state_base import AbsoluteObjectState, RelativeObjectState
from omnigibson.utils.asset_utils import get_all_object_category_models
from omnigibson.utils.constants import PrimType
from omnigibson.utils.python_utils import Registerable, classproperty, subclass_factory
from omnigibson.utils.registry_utils import Registry
import omnigibson.utils.transform_utils as T
from omnigibson.utils.ui_utils import disclaimer, create_module_logger
from omnigibson.utils.usd_utils import RigidContactAPI
from omnigibson.utils.bddl_utils import translate_bddl_recipe_to_og_recipe, translate_bddl_washer_rule_to_og_washer_rule
import bddl
# Create module logger
log = create_module_logger(module_name=__name__)
# Create settings for this module
m = create_module_macros(module_path=__file__)
# Default melting temperature
m.MELTING_TEMPERATURE = 100.0
# Default "trash" system if an invalid mixing rule transition occurs
m.DEFAULT_GARBAGE_SYSTEM = "sludge"
# Tuple of attributes of objects created in transitions.
# `states` field is dict mapping object state class to arguments to pass to setter for that class
_attrs_fields = ["category", "model", "name", "scale", "obj", "pos", "orn", "bb_pos", "bb_orn", "states", "callback"]
# States: dict: mapping state nameargs to pass to the state setter for @obj in order to set the object state
# callback: function: signature callback(obj) -> None to execute after states are set, if any
ObjectAttrs = namedtuple(
"ObjectAttrs", _attrs_fields, defaults=(None,) * len(_attrs_fields))
# Tuple of lists of objects to be added or removed returned from transitions, if not None
TransitionResults = namedtuple(
"TransitionResults", ["add", "remove"], defaults=(None, None))
# Mapping from transition rule json files to rule classe names
_JSON_FILES_TO_RULES = {
"heat_cook.json": ["CookingObjectRule", "CookingSystemRule"],
"mixing_stick.json": ["MixingToolRule"],
"single_toggleable_machine.json": ["ToggleableMachineRule"],
"substance_cooking.json": ["CookingPhysicalParticleRule"],
"substance_watercooking.json": ["CookingPhysicalParticleRule"],
"washer.json": ["WasherRule"],
}
# Global dicts that will contain mappings
REGISTERED_RULES = dict()
class TransitionRuleAPI:
"""
Monolithic class containing methods to check and execute arbitrary discrete state transitions within the simulator
"""
# Set of active rules
ACTIVE_RULES = set()
# Maps BaseObject instances to dictionary with the following keys:
# "states": None or dict mapping object states to arguments to set for that state when the object is initialized
# "callback": None or function to execute when the object is initialized
_INIT_INFO = dict()
@classmethod
def get_rule_candidates(cls, rule, objects):
"""
Computes valid input object candidates for transition rule @rule, if any exist
Args:
rule (BaseTransitionRule): Transition rule whose candidates should be computed
objects (list of BaseObject): List of objects that will be used to compute object candidates
Returns:
None or dict: None if no valid candidates are found, otherwise mapping from filter key to list of object
instances that satisfy that filter
"""
obj_candidates = rule.get_object_candidates(objects=objects)
n_filters_satisfied = sum(len(candidates) > 0 for candidates in obj_candidates.values())
# Return object candidates if all filters are met, otherwise return None
return obj_candidates if n_filters_satisfied == len(rule.candidate_filters) else None
@classmethod
def prune_active_rules(cls):
"""
Prunes the active transition rules, removing any whose filter requirements are not satisfied by all current
objects on the scene. Useful when the current object set changes, e.g.: an object is removed from the simulator
"""
# Need explicit tuple to iterate over because refresh_rules mutates the ACTIVE_RULES set in place
cls.refresh_rules(rules=tuple(cls.ACTIVE_RULES))
@classmethod
def refresh_all_rules(cls):
"""
Refreshes all registered rules given the current set of objects in the scene
"""
global RULES_REGISTRY
# Clear all active rules
cls.ACTIVE_RULES = set()
# Refresh all registered rules
cls.refresh_rules(rules=RULES_REGISTRY.objects)
@classmethod
def refresh_rules(cls, rules):
"""
Refreshes the specified transition rules @rules based on current set of objects in the simulator.
This will prune any pre-existing rules in cls.ACTIVE_RULES if no valid candidates are found, or add / update
the entry if valid candidates are found
Args:
rules (list of BaseTransitionRule): List of transition rules whose candidate lists should be refreshed
"""
objects = og.sim.scene.objects
for rule in rules:
# Check if rule is still valid, if so, update its entry
object_candidates = cls.get_rule_candidates(rule=rule, objects=objects)
# Update candidates if valid, otherwise pop the entry if it exists in cls.ACTIVE_RULES
if object_candidates is not None:
# We have a valid rule which should be active, so grab and initialize all of its conditions
# NOTE: The rule may ALREADY exist in ACTIVE_RULES, but we still need to refresh its candidates because
# the relevant candidate set / information for the rule + its conditions may have changed given the
# new set of objects
rule.refresh(object_candidates=object_candidates)
cls.ACTIVE_RULES.add(rule)
elif rule in cls.ACTIVE_RULES:
cls.ACTIVE_RULES.remove(rule)
@classmethod
def step(cls):
"""
Steps all active transition rules, checking if any are satisfied, and if so, executing their transition
"""
# First apply any transition object init states from before, and then clear the dictionary
for obj, info in cls._INIT_INFO.items():
if info["states"] is not None:
for state, args in info["states"].items():
obj.states[state].set_value(*args)
if info["callback"] is not None:
info["callback"](obj)
cls._INIT_INFO = dict()
# Iterate over all active rules and process the rule for every valid object candidate combination
# Cast to list before iterating since ACTIVE_RULES may get updated mid-iteration
added_obj_attrs = []
removed_objs = []
for rule in tuple(cls.ACTIVE_RULES):
output = rule.step()
# Store objects to be added / removed if we have a valid output
if output is not None:
added_obj_attrs += output.add
removed_objs += output.remove
cls.execute_transition(added_obj_attrs=added_obj_attrs, removed_objs=removed_objs)
@classmethod
def execute_transition(cls, added_obj_attrs, removed_objs):
"""
Executes the transition for the given added and removed objects.
:param added_obj_attrs: List of ObjectAttrs instances to add to the scene
:param removed_objs: List of BaseObject instances to remove from the scene
"""
# Process all transition results
if len(removed_objs) > 0:
# First remove pre-existing objects
og.sim.remove_object(removed_objs)
# Then add new objects
if len(added_obj_attrs) > 0:
state = og.sim.dump_state()
for added_obj_attr in added_obj_attrs:
new_obj = added_obj_attr.obj
og.sim.import_object(new_obj)
# By default, added_obj_attr is populated with all Nones -- so these will all be pass-through operations
# unless pos / orn (or, conversely, bb_pos / bb_orn) is specified
if added_obj_attr.pos is not None or added_obj_attr.orn is not None:
new_obj.set_position_orientation(position=added_obj_attr.pos, orientation=added_obj_attr.orn)
elif isinstance(new_obj, DatasetObject) and \
(added_obj_attr.bb_pos is not None or added_obj_attr.bb_orn is not None):
new_obj.set_bbox_center_position_orientation(position=added_obj_attr.bb_pos,
orientation=added_obj_attr.bb_orn)
else:
raise ValueError("Expected at least one of pos, orn, bb_pos, or bb_orn to be specified in ObjectAttrs!")
# Additionally record any requested states if specified to be updated during the next transition step
if added_obj_attr.states is not None or added_obj_attr.callback is not None:
cls._INIT_INFO[new_obj] = {
"states": added_obj_attr.states,
"callback": added_obj_attr.callback,
}
@classmethod
def clear(cls):
"""
Clears any internal state when the simulator is restarted (e.g.: when a new stage is opened)
"""
global RULES_REGISTRY
# Clear internal dictionaries
cls.ACTIVE_RULES = set()
cls._INIT_INFO = dict()
class ObjectCandidateFilter(metaclass=ABCMeta):
"""
Defines a filter to apply for inferring which objects are valid candidates for checking a transition rule's
condition requirements.
NOTE: These filters should describe STATIC properties about an object -- i.e.: properties that should NOT change
at runtime, once imported
"""
@abstractmethod
def __call__(self, obj):
"""Returns true if the given object passes the filter."""
return False
class CategoryFilter(ObjectCandidateFilter):
"""Filter for object categories."""
def __init__(self, category):
self.category = category
def __call__(self, obj):
return obj.category == self.category
class AbilityFilter(ObjectCandidateFilter):
"""Filter for object abilities."""
def __init__(self, ability):
self.ability = ability
def __call__(self, obj):
return self.ability in obj._abilities
class NameFilter(ObjectCandidateFilter):
"""Filter for object names."""
def __init__(self, name):
self.name = name
def __call__(self, obj):
return self.name in obj.name
class NotFilter(ObjectCandidateFilter):
"""Logical-not of a filter"""
def __init__(self, f):
self.f = f
def __call__(self, obj):
return not self.f(obj)
class OrFilter(ObjectCandidateFilter):
"""Logical-or of a set of filters."""
def __init__(self, filters):
self.filters = filters
def __call__(self, obj):
return any(f(obj) for f in self.filters)
class AndFilter(ObjectCandidateFilter):
"""Logical-and of a set of filters."""
def __init__(self, filters):
self.filters = filters
def __call__(self, obj):
return all(f(obj) for f in self.filters)
class RuleCondition:
"""
Defines a transition rule condition for filtering a given set of input object candidates.
NOTE: These filters should describe DYNAMIC properties about object candidates -- i.e.: properties that MAY change
at runtime, once imported
"""
def refresh(self, object_candidates):
"""
Refreshes any internal state for this rule condition, given set of input object candidates @object_candidates
Args:
object_candidates (dict): Maps filter name to valid object(s) that satisfy that filter
"""
# No-op by default
pass
@abstractmethod
def __call__(self, object_candidates):
"""
Filters @object_candidates and updates the candidates in-place, returning True if there are still valid
candidates
Args:
object_candidates (dict): Maps filter name to valid object(s) that satisfy that filter
Returns:
bool: Whether there are still valid candidates in @object_candidates
"""
# Default is False
return False
@property
def modifies_filter_names(self):
"""
Returns:
set: Filter name(s) whose values may be modified in-place by this condition
"""
raise NotImplementedError
class TouchingAnyCondition(RuleCondition):
"""
Rule condition that prunes object candidates from @filter_1_name, only keeping any that are touching any object
from @filter_2_name
"""
def __init__(self, filter_1_name, filter_2_name):
"""
Args:
filter_1_name (str): Name of the filter whose object candidates will be pruned based on whether or not
they are touching any object from @filter_2_name
filter_2_name (str): Name of the filter whose object candidates will be used to prune the candidates from
@filter_1_name
"""
self._filter_1_name = filter_1_name
self._filter_2_name = filter_2_name
# Will be filled in during self.initialize
# Maps object to the list of rigid body idxs in the global contact matrix corresponding to filter 1
self._filter_1_idxs = None
# If optimized, filter_2_idxs will be used, otherwise filter_2_bodies will be used!
# Maps object to the list of rigid body idxs in the global contact matrix corresponding to filter 2
self._filter_2_idxs = None
# Maps object to set of rigid bodies corresponding to filter 2
self._filter_2_bodies = None
# Flag whether optimized call can be used
self._optimized = None
def refresh(self, object_candidates):
# Check whether we can use optimized computation or not -- this is determined by whether or not any objects
# in our collision set are kinematic only
self._optimized = not np.any([obj.kinematic_only or obj.prim_type == PrimType.CLOTH
for f in (self._filter_1_name, self._filter_2_name) for obj in object_candidates[f]])
if self._optimized:
# Register idx mappings
self._filter_1_idxs = {obj: [RigidContactAPI.get_body_row_idx(link.prim_path) for link in obj.links.values()]
for obj in object_candidates[self._filter_1_name]}
self._filter_2_idxs = {obj: [RigidContactAPI.get_body_col_idx(link.prim_path) for link in obj.links.values()]
for obj in object_candidates[self._filter_2_name]}
else:
# Register body mappings
self._filter_2_bodies = {obj: set(obj.links.values()) for obj in object_candidates[self._filter_2_name]}
def __call__(self, object_candidates):
# Keep any object that has non-zero impulses between itself and any of the @filter_2_name's objects
objs = []
if self._optimized:
# Get all impulses
impulses = RigidContactAPI.get_all_impulses()
idxs_to_check = np.concatenate([self._filter_2_idxs[obj] for obj in object_candidates[self._filter_2_name]])
# Batch check for each object
for obj in object_candidates[self._filter_1_name]:
if np.any(impulses[self._filter_1_idxs[obj]][:, idxs_to_check]):
objs.append(obj)
else:
# Manually check contact
filter_2_bodies = set.union(*(self._filter_2_bodies[obj] for obj in object_candidates[self._filter_2_name]))
for obj in object_candidates[self._filter_1_name]:
if len(obj.states[ContactBodies].get_value().intersection(filter_2_bodies)) > 0:
objs.append(obj)
# Update candidates
object_candidates[self._filter_1_name] = objs
# If objs is empty, return False, otherwise, True
return len(objs) > 0
@property
def modifies_filter_names(self):
# Only modifies values from filter 1
return {self._filter_1_name}
class StateCondition(RuleCondition):
"""
Rule condition that checks all objects from @filter_name whether a state condition is equal to @val for
"""
def __init__(
self,
filter_name,
state,
val,
op=operator.eq,
):
"""
Args:
filter_name (str): Name of the filter whose object candidates will be pruned based on whether or not
the state @state's value is equal to @val
state (BaseObjectState): Object state whose value should be queried as a rule condition
val (any): The value @state should be in order for this condition to be satisfied
op (function): Binary operator to apply between @state's getter and @val. Default is operator.eq,
which does state.get_value() == val.
Expected signature:
def op(state_getter, val) --> bool
"""
self._filter_name = filter_name
self._state = state
self._val = val
self._op = op
def __call__(self, object_candidates):
# Keep any object whose states are satisfied
object_candidates[self._filter_name] = \
[obj for obj in object_candidates[self._filter_name] if self._op(obj.states[self._state].get_value(), self._val)]
# Condition met if any object meets the condition
return len(object_candidates[self._filter_name]) > 0
@property
def modifies_filter_names(self):
return {self._filter_name}
class ChangeConditionWrapper(RuleCondition):
"""
Rule condition wrapper that checks whether the output from @condition
"""
def __init__(
self,
condition,
):
"""
Args:
condition (RuleCondition): Condition whose output will be additionally filtered whether or not its relevant
values have changed since the previous time this condition was called
"""
self._condition = condition
self._last_valid_candidates = {filter_name: set() for filter_name in self.modifies_filter_names}
def refresh(self, object_candidates):
# Refresh nested condition
self._condition.refresh(object_candidates=object_candidates)
def __call__(self, object_candidates):
# Call wrapped method first
valid = self._condition(object_candidates=object_candidates)
# Iterate over all current candidates -- if there's a mismatch in last valid candidates and current,
# then we store it, otherwise, we don't
for filter_name in self.modifies_filter_names:
# Compute current valid candidates
objs = [obj for obj in object_candidates[filter_name] if obj not in self._last_valid_candidates[filter_name]]
# Store last valid objects -- these are all candidates that were validated by self._condition at the
# current timestep
self._last_valid_candidates[filter_name] = set(object_candidates[filter_name])
# Update current object candidates with the change-filtered ones
object_candidates[filter_name] = objs
valid = valid and len(objs) > 0
# Valid if any object conditions have changed and we still have valid objects
return valid
@property
def modifies_filter_names(self):
# Return wrapped names
return self._condition.modifies_filter_names
class OrConditionWrapper(RuleCondition):
"""
Logical OR between multiple RuleConditions
"""
def __init__(self, conditions):
"""
Args:
conditions (list of RuleConditions): Conditions to take logical OR over. This will generate
the UNION of all candidates.
"""
self._conditions = conditions
def refresh(self, object_candidates):
# Refresh nested conditions
for condition in self._conditions:
condition.refresh(object_candidates=object_candidates)
def __call__(self, object_candidates):
# Iterate over all conditions and aggregate their results
pruned_candidates = dict()
for condition in self._conditions:
# Copy the candidates because they get modified in place
pruned_candidates[condition] = copy(object_candidates)
condition(object_candidates=pruned_candidates[condition])
# For each filter, take the union over object candidates across each condition.
# If the result is empty, we immediately return False.
for filter_name in object_candidates:
object_candidates[filter_name] = \
list(set.union(*[set(candidates[filter_name]) for candidates in pruned_candidates.values()]))
if len(object_candidates[filter_name]) == 0:
return False
return True
@property
def modifies_filter_names(self):
# Return all wrapped names
return set.union(*(condition.modifies_filter_names for condition in self._conditions))
class AndConditionWrapper(RuleCondition):
"""
Logical AND between multiple RuleConditions
"""
def __init__(self, conditions):
"""
Args:
conditions (list of RuleConditions): Conditions to take logical AND over. This will generate
the INTERSECTION of all candidates.
"""
self._conditions = conditions
def refresh(self, object_candidates):
# Refresh nested conditions
for condition in self._conditions:
condition.refresh(object_candidates=object_candidates)
def __call__(self, object_candidates):
# Iterate over all conditions and aggregate their results
pruned_candidates = dict()
for condition in self._conditions:
# Copy the candidates because they get modified in place
pruned_candidates[condition] = copy(object_candidates)
condition(object_candidates=pruned_candidates[condition])
# For each filter, take the intersection over object candidates across each condition.
# If the result is empty, we immediately return False.
for filter_name in object_candidates:
object_candidates[filter_name] = \
list(set.intersection(*[set(candidates[filter_name]) for candidates in pruned_candidates.values()]))
if len(object_candidates[filter_name]) == 0:
return False
return True
@property
def modifies_filter_names(self):
# Return all wrapped names
return set.union(*(condition.modifies_filter_names for condition in self._conditions))
class BaseTransitionRule(Registerable):
"""
Defines a set of categories of objects and how to transition their states.
"""
conditions = None
candidates = None
def __init_subclass__(cls, **kwargs):
super().__init_subclass__(**kwargs)
# Register this system, and
# make sure at least one filter is specified -- in general, there should never be a rule
# where no filter is specified
# Only run this check for actual rules that are being registered
if cls.__name__ not in cls._do_not_register_classes:
global RULES_REGISTRY
RULES_REGISTRY.add(obj=cls)
assert len(cls.candidate_filters) > 0, \
"At least one of individual_filters or group_filters must be specified!"
# Store conditions
cls.conditions = cls._generate_conditions()
@classproperty
def candidate_filters(cls):
"""
Object candidate filters that this transition rule cares about.
For each name, filter key-value pair, the global transition rule step will produce a
single dictionary of valid filtered objects.
For example, if the group filters are:
{"apple": CategoryFilter("apple"), "knife": CategoryFilter("knife")},
the transition rule step will produce the following dictionary:
{"apple": [apple0, apple1, ...], "knife": [knife0, knife1, ...]}
based on the current instances of each object type in the scene and pass them to conditions in @self.conditions
NOTE: There should always be at least one filter applied for every rule!
Returns:
dict: Maps filter name to filter for inferring valid object candidates for this transition rule
"""
raise NotImplementedError
@classmethod
def _generate_conditions(cls):
"""
Generates rule condition(s)s for this transition rule. These conditions are used to prune object
candidates at runtime, to determine whether a transition rule should occur at the given timestep
Returns:
list of RuleCondition: Condition(s) to enforce to determine whether a transition rule should occur
"""
raise NotImplementedError
@classmethod
def get_object_candidates(cls, objects):
"""
Given the set of objects @objects, compute the valid object candidate combinations that may be valid for
this TransitionRule
Args:
objects (list of BaseObject): Objects to filter for valid transition rule candidates
Returns:
dict: Maps filter name to valid object(s) that satisfy that filter
"""
# Iterate over all objects and add to dictionary if valid
filters = cls.candidate_filters
obj_dict = {filter_name: [] for filter_name in filters.keys()}
for obj in objects:
for fname, f in filters.items():
if f(obj):
obj_dict[fname].append(obj)
return obj_dict
@classmethod
def refresh(cls, object_candidates):
"""
Refresh any internal state for this rule, given set of input object candidates @object_candidates
Args:
object_candidates (dict): Maps filter name to valid object(s) that satisfy that filter
"""
# Store candidates
cls.candidates = object_candidates
# Refresh all conditions
for condition in cls.conditions:
condition.refresh(object_candidates=object_candidates)
@classmethod
def transition(cls, object_candidates):
"""
Rule to apply for each set of objects satisfying the condition.
Args:
object_candidates (dict): Dictionary mapping corresponding keys from @cls.filters to list of individual
object instances where the filter is satisfied
Returns:
TransitionResults: results from the executed transition
"""
raise NotImplementedError()
@classmethod
def step(cls):
"""
Takes a step for this transition rule, checking if all of @cls.conditions are satisified, and if so, taking
a transition via @cls.transition()
Returns:
None or TransitionResults: If a transition occurs, returns its results, otherwise, returns None
"""
# Copy the candidates dictionary since it may be mutated in place by @conditions
object_candidates = {filter_name: candidates.copy() for filter_name, candidates in cls.candidates.items()}
for condition in cls.conditions:
if not condition(object_candidates=object_candidates):
# Condition was not met, so immediately terminate
return
# All conditions are met, take the transition
return cls.transition(object_candidates=object_candidates)
@classproperty
def _do_not_register_classes(cls):
# Don't register this class since it's an abstract template
classes = super()._do_not_register_classes
classes.add("BaseTransitionRule")
return classes
@classproperty
def _cls_registry(cls):
# Global registry
global REGISTERED_RULES
return REGISTERED_RULES
# Global dicts that will contain mappings. Must be placed here immediately AFTER BaseTransitionRule!
RULES_REGISTRY = Registry(
name="TransitionRuleRegistry",
class_types=BaseTransitionRule,
default_key="__name__",
)
class WasherDryerRule(BaseTransitionRule):
"""
Transition rule to apply to cloth washers and dryers.
"""
@classmethod
def _generate_conditions(cls):
assert len(cls.candidate_filters.keys()) == 1
machine_type = list(cls.candidate_filters.keys())[0]
return [ChangeConditionWrapper(
condition=AndConditionWrapper(conditions=[
StateCondition(filter_name=machine_type, state=ToggledOn, val=True, op=operator.eq),
StateCondition(filter_name=machine_type, state=Open, val=False, op=operator.eq),
])
)]
@classmethod
def _compute_global_rule_info(cls):
"""
Helper function to compute global information necessary for checking rules. This is executed exactly
once per cls.transition() step
Returns:
dict: Keyword-mapped global rule information
"""
# Compute all obj
obj_positions = np.array([obj.aabb_center for obj in og.sim.scene.objects])
return dict(obj_positions=obj_positions)
@classmethod
def _compute_container_info(cls, object_candidates, container, global_info):
"""
Helper function to compute container-specific information necessary for checking rules. This is executed once
per container per cls.transition() step
Args:
object_candidates (dict): Dictionary mapping corresponding keys from @cls.filters to list of individual
object instances where the filter is satisfied
container (StatefulObject): Relevant container object for computing information
global_info (dict): Output of @cls._compute_global_rule_info(); global information which may be
relevant for computing container information
Returns:
dict: Keyword-mapped container information
"""
del object_candidates
obj_positions = global_info["obj_positions"]
in_volume = container.states[ContainedParticles].check_in_volume(obj_positions)
in_volume_objs = list(np.array(og.sim.scene.objects)[in_volume])
# Remove the container itself
if container in in_volume_objs:
in_volume_objs.remove(container)
return dict(in_volume_objs=in_volume_objs)
@classproperty
def _do_not_register_classes(cls):
# Don't register this class since it's an abstract template
classes = super()._do_not_register_classes
classes.add("WasherDryerRule")
return classes
class WasherRule(WasherDryerRule):
"""
Transition rule to apply to cloth washers.
1. remove "dirty" particles from the washer if the necessary solvent is present.
2. wet the objects inside by making them either Saturated with or Covered by water.
"""
cleaning_conditions = None
@classmethod
def register_cleaning_conditions(cls, conditions):
"""
Register cleaning conditions for this rule.
Args:
conditions (dict): ictionary mapping the system name (str) to None or list of system names (str). None
represents "never", empty list represents "always", or non-empty list represents at least one of the
systems in the list needs to be present in the washer for the key system to be removed.
E.g. "rust" -> None: "never remove rust from the washer"
E.g. "dust" -> []: "always remove dust from the washer"
E.g. "cooking_oil" -> ["sodium_carbonate", "vinegar"]: "remove cooking_oil from the washer if either
sodium_carbonate or vinegar is present"
For keys not present in the dictionary, the default is []: "always remove"
"""
cls.cleaning_conditions = conditions
@classproperty
def candidate_filters(cls):
return {
"washer": CategoryFilter("washer"),
}
@classmethod
def transition(cls, object_candidates):
water = get_system("water")
global_info = cls._compute_global_rule_info()
for washer in object_candidates["washer"]:
# Remove the systems if the conditions are met
systems_to_remove = []
for system in ParticleRemover.supported_active_systems.values():
# Never remove
if system.name in cls.cleaning_conditions and cls.cleaning_conditions[system.name] is None:
continue
if not washer.states[Contains].get_value(system):
continue
solvents = cls.cleaning_conditions.get(system.name, [])
# Always remove
if len(solvents) == 0:
systems_to_remove.append(system)
else:
solvents = [get_system(solvent) for solvent in solvents if is_system_active(solvent)]
# If any of the solvents are present
if any(washer.states[Contains].get_value(solvent) for solvent in solvents):
systems_to_remove.append(system)
for system in systems_to_remove:
washer.states[Contains].set_value(system, False)
# Make the objects wet
container_info = cls._compute_container_info(object_candidates=object_candidates, container=washer, global_info=global_info)
in_volume_objs = container_info["in_volume_objs"]
for obj in in_volume_objs:
if Saturated in obj.states:
obj.states[Saturated].set_value(water, True)
else:
obj.states[Covered].set_value(water, True)
return TransitionResults(add=[], remove=[])
class DryerRule(WasherDryerRule):
"""
Transition rule to apply to cloth dryers.
1. dry the objects inside by making them not Saturated with water.
2. remove all water from the dryer.
"""
@classproperty
def candidate_filters(cls):
return {
"dryer": CategoryFilter("clothes_dryer"),
}
@classmethod
def transition(cls, object_candidates):
water = get_system("water")
global_info = cls._compute_global_rule_info()
for dryer in object_candidates["dryer"]:
container_info = cls._compute_container_info(object_candidates=object_candidates, container=dryer, global_info=global_info)
in_volume_objs = container_info["in_volume_objs"]
for obj in in_volume_objs:
if Saturated in obj.states:
obj.states[Saturated].set_value(water, False)
dryer.states[Contains].set_value(water, False)
return TransitionResults(add=[], remove=[])
class SlicingRule(BaseTransitionRule):
"""
Transition rule to apply to sliced / slicer object pairs.
"""
@classproperty
def candidate_filters(cls):
return {
"sliceable": AbilityFilter("sliceable"),
"slicer": AbilityFilter("slicer"),
}
@classmethod
def _generate_conditions(cls):
# sliceables should be touching any slicer
return [TouchingAnyCondition(filter_1_name="sliceable", filter_2_name="slicer"),
StateCondition(filter_name="slicer", state=SlicerActive, val=True, op=operator.eq)]
@classmethod
def transition(cls, object_candidates):
objs_to_add, objs_to_remove = [], []
for sliceable_obj in object_candidates["sliceable"]:
# Object parts offset annotation are w.r.t the base link of the whole object.
pos, orn = sliceable_obj.get_position_orientation()
# Load object parts
for i, part in enumerate(sliceable_obj.metadata["object_parts"].values()):
# List of dicts gets replaced by {'0':dict, '1':dict, ...}
# Get bounding box info
part_bb_pos = np.array(part["bb_pos"])
part_bb_orn = np.array(part["bb_orn"])
# Determine the relative scale to apply to the object part from the original object
# Note that proper (rotated) scaling can only be applied when the relative orientation of
# the object part is a multiple of 90 degrees wrt the parent object, so we assert that here
assert T.check_quat_right_angle(part_bb_orn), "Sliceable objects should only have relative object part orientations that are factors of 90 degrees!"
# Scale the offset accordingly.
scale = np.abs(T.quat2mat(part_bb_orn) @ sliceable_obj.scale)
# Calculate global part bounding box pose.
part_bb_pos = pos + T.quat2mat(orn) @ (part_bb_pos * scale)
part_bb_orn = T.quat_multiply(orn, part_bb_orn)
part_obj_name = f"half_{sliceable_obj.name}_{i}"
part_obj = DatasetObject(
name=part_obj_name,
category=part["category"],
model=part["model"],
bounding_box=part["bb_size"] * scale, # equiv. to scale=(part["bb_size"] / self.native_bbox) * (scale)
)
sliceable_obj_state = sliceable_obj.dump_state()
# Propagate non-physical states of the whole object to the half objects, e.g. cooked, saturated, etc.
# Add the new object to the results.
new_obj_attrs = ObjectAttrs(
obj=part_obj,
bb_pos=part_bb_pos,
bb_orn=part_bb_orn,
callback=lambda obj: obj.load_non_kin_state(sliceable_obj_state),
)
objs_to_add.append(new_obj_attrs)
# Delete original object from stage.
objs_to_remove.append(sliceable_obj)
return TransitionResults(add=objs_to_add, remove=objs_to_remove)
class DicingRule(BaseTransitionRule):
"""
Transition rule to apply to diceable / slicer object pairs.
"""
@classproperty
def candidate_filters(cls):
return {
"diceable": AbilityFilter("diceable"),
"slicer": AbilityFilter("slicer"),
}
@classmethod
def _generate_conditions(cls):
# sliceables should be touching any slicer
return [TouchingAnyCondition(filter_1_name="diceable", filter_2_name="slicer"),
StateCondition(filter_name="slicer", state=SlicerActive, val=True, op=operator.eq)]
@classmethod
def transition(cls, object_candidates):
objs_to_remove = []
for diceable_obj in object_candidates["diceable"]:
obj_category = diceable_obj.category
# We expect all diced particle systems to follow the naming convention (cooked__)diced__<category>
system_name = "diced__" + diceable_obj.category.removeprefix("half_")
if Cooked in diceable_obj.states and diceable_obj.states[Cooked].get_value():
system_name = "cooked__" + system_name
system = get_system(system_name)
system.generate_particles_from_link(diceable_obj, diceable_obj.root_link, check_contact=False, use_visual_meshes=False)
# Delete original object from stage.
objs_to_remove.append(diceable_obj)
return TransitionResults(add=[], remove=objs_to_remove)
class MeltingRule(BaseTransitionRule):
"""
Transition rule to apply to meltable objects to simulate melting
Once the object reaches the melting temperature, remove the object and spawn the melted substance in its place.
"""
@classproperty
def candidate_filters(cls):
# We want to find all meltable objects
return {"meltable": AbilityFilter("meltable")}
@classmethod
def _generate_conditions(cls):
return [StateCondition(filter_name="meltable", state=MaxTemperature, val=m.MELTING_TEMPERATURE, op=operator.ge)]
@classmethod
def transition(cls, object_candidates):
objs_to_remove = []
# Convert the meltable object into its melted substance
for meltable_obj in object_candidates["meltable"]:
# All meltable xyz, half_xyz and diced__xyz transform into melted__xyz
root_category = meltable_obj.category.removeprefix("half_").removeprefix("diced__")
system_name = f"melted__{root_category}"
system = get_system(system_name)
system.generate_particles_from_link(meltable_obj, meltable_obj.root_link, check_contact=False, use_visual_meshes=False)
# Delete original object from stage.
objs_to_remove.append(meltable_obj)
return TransitionResults(add=[], remove=objs_to_remove)
class RecipeRule(BaseTransitionRule):
"""
Transition rule to approximate recipe-based transitions
"""
# Maps recipe name to recipe information
_RECIPES = None
# Maps active recipe name to recipe information
_ACTIVE_RECIPES = None
# Maps object category name to indices in the flattened object array for efficient computation
_CATEGORY_IDXS = None
# Flattened array of all simulator objects, sorted by category
_OBJECTS = None
# Maps object to idx within the _OBJECTS array
_OBJECTS_TO_IDX = None
def __init_subclass__(cls, **kwargs):
# Run super first
super().__init_subclass__(**kwargs)
# Initialize recipes
cls._RECIPES = dict()
@classmethod
def add_recipe(
cls,
name,
input_objects,
input_systems,
output_objects,
output_systems,
input_states=None,
output_states=None,
fillable_categories=None,
**kwargs,
):
"""
Adds a recipe to this recipe rule to check against. This defines a valid mapping of inputs that will transform
into the outputs
Args:
name (str): Name of the recipe
input_objects (dict): Maps object categories to number of instances required for the recipe
input_systems (list): List of system names required for the recipe
output_objects (dict): Maps object categories to number of instances to be spawned in the container when the recipe executes
output_systems (list): List of system names to be spawned in the container when the recipe executes. Currently the length is 1.
input_states (None or defaultdict(lambda: defaultdict(list))): Maps object categories to
["unary", "bianry_system", "binary_object"] to a list of states that must be satisfied for the recipe to execute
output_states (None or defaultdict(lambda: defaultdict(list))): Maps object categories to
["unary", "bianry_system"] to a list of states that should be set after the output objects are spawned
fillable_categories (None or set of str): If specified, set of fillable categories which are allowed
for this recipe. If None, any fillable is allowed
kwargs (dict): Any additional keyword-arguments to be stored as part of this recipe
"""
input_states = input_states if input_states is not None else defaultdict(lambda: defaultdict(list))
output_states = output_states if output_states is not None else defaultdict(lambda: defaultdict(list))
input_object_tree = None
if cls.is_multi_instance and len(input_objects) > 0:
# Build a tree of input object categories according to the kinematic binary states
# Example: 'raw_egg': {'binary_object': [(OnTop, 'bagel_dough', True)]} results in an edge
# from 'bagel_dough' to 'raw_egg', i.e. 'bagel_dough' is the parent of 'raw_egg'.
input_object_tree = nx.DiGraph()
for obj_category, state_checks in input_states.items():
for state_class, second_obj_category, state_value in state_checks["binary_object"]:
input_object_tree.add_edge(second_obj_category, obj_category)
if nx.is_empty(input_object_tree):
input_object_tree = None
else:
assert nx.is_tree(input_object_tree), f"Input object tree must be a tree! Now: {input_object_tree}."
root_nodes = [node for node in input_object_tree.nodes() if input_object_tree.in_degree(node) == 0]
assert len(root_nodes) == 1, f"Input object tree must have exactly one root node! Now: {root_nodes}."
assert input_objects[root_nodes[0]] == 1, f"Input object tree root node must have exactly one instance! Now: {cls._RECIPES[name]['input_objects'][root_nodes[0]]}."
# Store information for this recipe
cls._RECIPES[name] = {
"name": name,
"input_objects": input_objects,
"input_systems": input_systems,
"output_objects": output_objects,
"output_systems": output_systems,
"input_states": input_states,
"output_states": output_states,
"fillable_categories": fillable_categories,
"input_object_tree": input_object_tree,
**kwargs,
}
@classmethod
def _validate_recipe_container_is_valid(cls, recipe, container):
"""
Validates that @container's category satisfies @recipe's fillable_categories
Args:
recipe (dict): Recipe whose fillable_categories should be checked against @container
container (StatefulObject): Container whose category should match one of @recipe's fillable_categories,
if specified
Returns:
bool: True if @container is valid, else False
"""
fillable_categories = recipe["fillable_categories"]
return fillable_categories is None or container.category in fillable_categories
@classmethod
def _validate_recipe_systems_are_contained(cls, recipe, container):
"""
Validates whether @recipe's input_systems are all contained in @container or not
Args:
recipe (dict): Recipe whose systems should be checked
container (BaseObject): Container object that should contain all of @recipe's input systems
Returns:
bool: True if all the input systems are contained
"""
for system_name in recipe["input_systems"]:
system = get_system(system_name=system_name)
if not container.states[Contains].get_value(system=system):
return False
return True
@classmethod
def _validate_nonrecipe_systems_not_contained(cls, recipe, container):
"""
Validates whether all systems not relevant to @recipe are not contained in @container
Args:
recipe (dict): Recipe whose systems should be checked
container (BaseObject): Container object that should contain all of @recipe's input systems
Returns:
bool: True if none of the non-relevant systems are contained
"""
for system in og.sim.scene.system_registry.objects:
# Skip cloth system
if system.name == "cloth":
continue
if system.name not in recipe["input_systems"] and container.states[Contains].get_value(system=system):
return False
return True
@classmethod
def _validate_recipe_objects_are_contained_and_states_satisfied(cls, recipe, container_info):
"""
Validates whether @recipe's input_objects are contained in the container and whether their states are satisfied
Args:
recipe (dict): Recipe whose objects should be checked
container_info (dict): Output of @cls._compute_container_info(); container-specific information which may
be relevant for computing whether recipe is executable. This will be populated with execution info.
Returns:
bool: True if all the input object quantities are contained
"""
in_volume = container_info["in_volume"]
# Store necessary information for execution
container_info["execution_info"] = dict()
category_to_valid_indices = cls._filter_input_objects_by_unary_and_binary_system_states(recipe=recipe)
container_info["execution_info"]["category_to_valid_indices"] = category_to_valid_indices
if not cls.is_multi_instance:
return cls._validate_recipe_objects_non_multi_instance(
recipe=recipe, category_to_valid_indices=category_to_valid_indices, in_volume=in_volume,
)
else:
return cls._validate_recipe_objects_multi_instance(
recipe=recipe, category_to_valid_indices=category_to_valid_indices, container_info=container_info,
)
@classmethod
def _filter_input_objects_by_unary_and_binary_system_states(cls, recipe):
# Filter input objects based on a subset of input states (unary states and binary system states)
# Map object categories (str) to valid indices (np.ndarray)
category_to_valid_indices = dict()
for obj_category in recipe["input_objects"]:
if obj_category not in recipe["input_states"]:
# If there are no input states, all objects of this category are valid
category_to_valid_indices[obj_category] = cls._CATEGORY_IDXS[obj_category]
else:
category_to_valid_indices[obj_category] = []
for idx in cls._CATEGORY_IDXS[obj_category]:
obj = cls._OBJECTS[idx]
success = True
# Check if unary states are satisfied
for state_class, state_value in recipe["input_states"][obj_category]["unary"]:
if obj.states[state_class].get_value() != state_value:
success = False
break
if not success:
continue
# Check if binary system states are satisfied
for state_class, system_name, state_value in recipe["input_states"][obj_category]["binary_system"]:
if obj.states[state_class].get_value(system=get_system(system_name)) != state_value:
success = False
break
if not success:
continue
category_to_valid_indices[obj_category].append(idx)
# Convert to numpy array for faster indexing
category_to_valid_indices[obj_category] = np.array(category_to_valid_indices[obj_category], dtype=int)
return category_to_valid_indices
@classmethod
def _validate_recipe_objects_non_multi_instance(cls, recipe, category_to_valid_indices, in_volume):
# Check if sufficiently number of objects are contained
for obj_category, obj_quantity in recipe["input_objects"].items():
if np.sum(in_volume[category_to_valid_indices[obj_category]]) < obj_quantity:
return False
return True
@classmethod
def _validate_recipe_objects_multi_instance(cls, recipe, category_to_valid_indices, container_info):
in_volume = container_info["in_volume"]
input_object_tree = recipe["input_object_tree"]
# Map object category to a set of objects that are used in this execution
relevant_objects = defaultdict(set)
# Map system name to a set of particle indices that are used in this execution
relevant_systems = defaultdict(set)
# Number of instances of this recipe that can be produced
num_instances = 0
# Define a recursive function to check the kinematic tree
def check_kinematic_tree(obj, should_check_in_volume=False):
"""
Recursively check if the kinematic tree is satisfied.
Return True/False, and a set of objects that belong to the subtree rooted at the current node
Args:
obj (BaseObject): Subtree root node to check
should_check_in_volume (bool): Whether to check if the object is in the volume or not
Returns:
bool: True if the subtree rooted at the current node is satisfied
set: Set of objects that belong to the subtree rooted at the current node
"""
# Check if obj is in volume
if should_check_in_volume and not in_volume[cls._OBJECTS_TO_IDX[obj]]:
return False, set()
# If the object is a leaf node, return True and the set containing the object
if input_object_tree.out_degree(obj.category) == 0:
return True, set([obj])
children_categories = list(input_object_tree.successors(obj.category))
all_subtree_objs = set()
for child_cat in children_categories:
assert len(input_states[child_cat]["binary_object"]) == 1, \
"Each child node should have exactly one binary object state, i.e. one parent in the input_object_tree"
state_class, _, state_value = input_states[child_cat]["binary_object"][0]
num_valid_children = 0
children_objs = cls._OBJECTS[category_to_valid_indices[child_cat]]
for child_obj in children_objs:
# If the child doesn't satisfy the binary object state, skip
if child_obj.states[state_class].get_value(obj) != state_value:
continue
# Recursively check if the subtree rooted at the child is valid
subtree_valid, subtree_objs = check_kinematic_tree(child_obj)
# If the subtree is valid, increment the number of valid children and aggregate the objects
if subtree_valid:
num_valid_children += 1
all_subtree_objs |= subtree_objs
# If there are not enough valid children, return False
if num_valid_children < recipe["input_objects"][child_cat]:
return False, set()
# If all children categories have sufficient number of objects that satisfy the binary object state,
# e.g. five pieces of pepperoni and two pieces of basil on the pizza, the subtree rooted at the
# current node is valid. Return True and the set of objects in the subtree (all descendants plus
# the current node)
return True, all_subtree_objs | {obj}
# If multi-instance is True but doesn't require kinematic states between objects
if input_object_tree is None:
num_instances = np.inf
# Compute how many instances of this recipe can be produced.
# Example: if a recipe requires 1 apple and 2 bananas, and there are 3 apples and 4 bananas in the
# container, then 2 instance of the recipe can be produced.
for obj_category, obj_quantity in recipe["input_objects"].items():
quantity_in_volume = np.sum(in_volume[category_to_valid_indices[obj_category]])
num_inst = quantity_in_volume // obj_quantity
if num_inst < 1:
return False
num_instances = min(num_instances, num_inst)
# If at least one instance of the recipe can be executed, add all valid objects to be relevant_objects.
# This can be considered as a special case of below where there are no binary kinematic states required.
for obj_category in recipe["input_objects"]:
relevant_objects[obj_category] = set(cls._OBJECTS[category_to_valid_indices[obj_category]])
# If multi-instance is True and requires kinematic states between objects
else:
root_node_category = [node for node in input_object_tree.nodes()
if input_object_tree.in_degree(node) == 0][0]
# A list of objects belonging to the root node category
root_nodes = cls._OBJECTS[category_to_valid_indices[root_node_category]]
input_states = recipe["input_states"]
for root_node in root_nodes:
# should_check_in_volume is True only for the root nodes.
# Example: the bagel dough needs to be in_volume of the container, but the raw egg on top doesn't.
tree_valid, relevant_object_set = check_kinematic_tree(obj=root_node, should_check_in_volume=True)
if tree_valid:
# For each valid tree, increment the number of instances and aggregate the objects
num_instances += 1
for obj in relevant_object_set:
relevant_objects[obj.category].add(obj)
# If there are no valid trees, return False
if num_instances == 0:
return False
# Note that for multi instance recipes, the relevant system particles are NOT the ones in the container.
# Instead, they are the ones that are related to the relevant objects, e.g. salt covering the bagel dough.
for obj_category, objs in relevant_objects.items():
for state_class, system_name, state_value in recipe["input_states"][obj_category]["binary_system"]:
# If the state value is False, skip
if not state_value:
continue
for obj in objs:
if state_class in [Filled, Contains]:
contained_particle_idx = obj.states[ContainedParticles].get_value(get_system(system_name)).in_volume.nonzero()[0]
relevant_systems[system_name] |= contained_particle_idx
elif state_class in [Covered]:
covered_particle_idx = obj.states[ContactParticles].get_value(get_system(system_name))
relevant_systems[system_name] |= covered_particle_idx
# Now we populate the execution info with the relevant objects and systems as well as the number of
# instances of the recipe that can be produced.
container_info["execution_info"]["relevant_objects"] = relevant_objects
container_info["execution_info"]["relevant_systems"] = relevant_systems
container_info["execution_info"]["num_instances"] = num_instances
return True
@classmethod
def _validate_nonrecipe_objects_not_contained(cls, recipe, container_info):
"""
Validates whether all objects not relevant to @recipe are not contained in the container
represented by @in_volume
Args:
recipe (dict): Recipe whose systems should be checked
container_info (dict): Output of @cls._compute_container_info(); container-specific information
which may be relevant for computing whether recipe is executable
Returns:
bool: True if none of the non-relevant objects are contained
"""
in_volume = container_info["in_volume"]
# These are object indices whose objects satisfy the input states
category_to_valid_indices = container_info["execution_info"]["category_to_valid_indices"]
nonrecipe_objects_in_volume = in_volume if len(recipe["input_objects"]) == 0 else \
np.delete(in_volume, np.concatenate([category_to_valid_indices[obj_category]
for obj_category in category_to_valid_indices]))
return not np.any(nonrecipe_objects_in_volume)
@classmethod
def _validate_recipe_systems_exist(cls, recipe):
"""
Validates whether @recipe's input_systems are all active or not
Args:
recipe (dict): Recipe whose systems should be checked
Returns:
bool: True if all the input systems are active
"""
for system_name in recipe["input_systems"]:
if not is_system_active(system_name=system_name):
return False
return True
@classmethod
def _validate_recipe_objects_exist(cls, recipe):
"""
Validates whether @recipe's input_objects exist in the current scene or not
Args:
recipe (dict): Recipe whose objects should be checked
Returns:
bool: True if all the input objects exist in the scene
"""
for obj_category, obj_quantity in recipe["input_objects"].items():
if len(og.sim.scene.object_registry("category", obj_category, default_val=set())) < obj_quantity:
return False
return True
@classmethod
def _validate_recipe_fillables_exist(cls, recipe):
"""
Validates that recipe @recipe's necessary fillable categorie(s) exist in the current scene
Args:
recipe (dict): Recipe whose fillable categories should be checked
Returns:
bool: True if there is at least a single valid fillable category in the current scene, else False
"""
fillable_categories = recipe["fillable_categories"]
if fillable_categories is None:
# Any is valid
return True
# Otherwise, at least one valid type must exist
for category in fillable_categories:
if len(og.sim.scene.object_registry("category", category, default_val=set())) > 0:
return True
# None found, return False
return False
@classmethod
def _is_recipe_active(cls, recipe):
"""
Helper function to determine whether a given recipe @recipe should be actively checked for or not.
Args:
recipe (dict): Maps relevant keyword to corresponding recipe info
Returns:
bool: True if the recipe is active, else False
"""
# Check valid active systems
if not cls._validate_recipe_systems_exist(recipe=recipe):
return False
# Check valid object quantities
if not cls._validate_recipe_objects_exist(recipe=recipe):
return False
# Check valid fillable categories
if not cls._validate_recipe_fillables_exist(recipe=recipe):
return False
return True
@classmethod
def _is_recipe_executable(cls, recipe, container, global_info, container_info):
"""
Helper function to determine whether a given recipe @recipe should be immediately executed or not.
Args:
recipe (dict): Maps relevant keyword to corresponding recipe info
container (StatefulObject): Container in which @recipe may be executed
global_info (dict): Output of @cls._compute_global_rule_info(); global information which may be
relevant for computing whether recipe is executable
container_info (dict): Output of @cls._compute_container_info(); container-specific information
which may be relevant for computing whether recipe is executable
Returns:
bool: True if the recipe is active, else False
"""
in_volume = container_info["in_volume"]
# Verify the container category is valid
if not cls._validate_recipe_container_is_valid(recipe=recipe, container=container):
return False
# Verify all required systems are contained in the container
if not cls.relax_recipe_systems and not cls._validate_recipe_systems_are_contained(recipe=recipe, container=container):
return False
# Verify all required object quantities are contained in the container and their states are satisfied
if not cls._validate_recipe_objects_are_contained_and_states_satisfied(recipe=recipe, container_info=container_info):
return False
# Verify no non-relevant system is contained
if not cls.ignore_nonrecipe_systems and not cls._validate_nonrecipe_systems_not_contained(recipe=recipe, container=container):
return False
# Verify no non-relevant object is contained if we're not ignoring them
if not cls.ignore_nonrecipe_objects and not cls._validate_nonrecipe_objects_not_contained(recipe=recipe, container_info=container_info):
return False
return True
@classmethod
def _compute_global_rule_info(cls):
"""
Helper function to compute global information necessary for checking rules. This is executed exactly
once per cls.transition() step
Returns:
dict: Keyword-mapped global rule information
"""
# Compute all relevant object AABB positions
obj_positions = np.array([obj.aabb_center for obj in cls._OBJECTS])
return dict(obj_positions=obj_positions)
@classmethod
def _compute_container_info(cls, object_candidates, container, global_info):
"""
Helper function to compute container-specific information necessary for checking rules. This is executed once
per container per cls.transition() step
Args:
object_candidates (dict): Dictionary mapping corresponding keys from @cls.filters to list of individual
object instances where the filter is satisfied
container (StatefulObject): Relevant container object for computing information
global_info (dict): Output of @cls._compute_global_rule_info(); global information which may be
relevant for computing container information
Returns:
dict: Keyword-mapped container information
"""
del object_candidates
obj_positions = global_info["obj_positions"]
# Compute in volume for all relevant object positions
# We check for either the object AABB being contained OR the object being on top of the container, in the
# case that the container is too flat for the volume to contain the object
in_volume = container.states[ContainedParticles].check_in_volume(obj_positions) | \
np.array([obj.states[OnTop].get_value(container) for obj in cls._OBJECTS])
# Container itself is never within its own volume
in_volume[cls._OBJECTS_TO_IDX[container]] = False
return dict(in_volume=in_volume)
@classmethod
def refresh(cls, object_candidates):
# Run super first
super().refresh(object_candidates=object_candidates)
# Cache active recipes given the current set of objects
cls._ACTIVE_RECIPES = dict()
cls._CATEGORY_IDXS = dict()
cls._OBJECTS = []
cls._OBJECTS_TO_IDX = dict()
# Prune any recipes whose objects / system requirements are not met by the current set of objects / systems
objects_by_category = og.sim.scene.object_registry.get_dict("category")
for name, recipe in cls._RECIPES.items():
# If all pre-requisites met, add to active recipes
if cls._is_recipe_active(recipe=recipe):
cls._ACTIVE_RECIPES[name] = recipe
# Finally, compute relevant objects and category mapping based on relevant categories
i = 0
for category, objects in objects_by_category.items():
cls._CATEGORY_IDXS[category] = i + np.arange(len(objects))
cls._OBJECTS += list(objects)
for obj in objects:
cls._OBJECTS_TO_IDX[obj] = i
i += 1
# Wrap relevant objects as numpy array so we can index into it efficiently
cls._OBJECTS = np.array(cls._OBJECTS)
@classproperty
def candidate_filters(cls):
# Fillable object required
return {"container": AbilityFilter(ability="fillable")}
@classmethod
def transition(cls, object_candidates):
objs_to_add, objs_to_remove = [], []
# Compute global info
global_info = cls._compute_global_rule_info()
# Iterate over all fillable objects, to execute recipes for each one
for container in object_candidates["container"]:
recipe_results = None
# Compute container info
container_info = cls._compute_container_info(
object_candidates=object_candidates,
container=container,
global_info=global_info,
)
# Check every recipe to find if any is valid
for name, recipe in cls._ACTIVE_RECIPES.items():
if cls._is_recipe_executable(recipe=recipe, container=container, global_info=global_info, container_info=container_info):
# Otherwise, all conditions met, we found a valid recipe and so we execute and terminate early
og.log.info(f"Executing recipe: {name} in container {container.name}!")
# Take the transform and terminate early
recipe_results = cls._execute_recipe(
container=container,
recipe=recipe,
container_info=container_info,
)
objs_to_add += recipe_results.add
objs_to_remove += recipe_results.remove
break
# Otherwise, if we didn't find a valid recipe, we execute a garbage transition instead if requested
if recipe_results is None and cls.use_garbage_fallback_recipe:
og.log.info(f"Did not find a valid recipe for rule {cls.__name__}; generating {m.DEFAULT_GARBAGE_SYSTEM} in {container.name}!")
# Generate garbage fluid
garbage_results = cls._execute_recipe(
container=container,
recipe=dict(
name="garbage",
input_objects=dict(),
input_systems=[],
output_objects=dict(),
output_systems=[m.DEFAULT_GARBAGE_SYSTEM],
output_states=defaultdict(lambda: defaultdict(list)),
),
container_info=container_info,
)
objs_to_add += garbage_results.add
objs_to_remove += garbage_results.remove
return TransitionResults(add=objs_to_add, remove=objs_to_remove)
@classmethod
def _execute_recipe(cls, container, recipe, container_info):
"""
Transforms all items contained in @container into @output_system, generating volume of @output_system
proportional to the number of items transformed.
Args:
container (BaseObject): Container object which will have its contained elements transformed into
@output_system
recipe (dict): Recipe to execute. Should include, at the minimum, "input_objects", "input_systems",
"output_objects", and "output_systems" keys
container_info (dict): Output of @cls._compute_container_info(); container-specific information which may
be relevant for computing whether recipe is executable.
Returns:
TransitionResults: Results of the executed recipe transition
"""
objs_to_add, objs_to_remove = [], []
in_volume = container_info["in_volume"]
if cls.is_multi_instance:
execution_info = container_info["execution_info"]
# Compute total volume of all contained items
volume = 0
if not cls.is_multi_instance:
# Remove either all systems or only the ones specified in the input systems of the recipe
contained_particles_state = container.states[ContainedParticles]
for system in PhysicalParticleSystem.get_active_systems().values():
if not cls.ignore_nonrecipe_systems or system.name in recipe["input_systems"]:
if container.states[Contains].get_value(system):
volume += contained_particles_state.get_value(system).n_in_volume * np.pi * (system.particle_radius ** 3) * 4 / 3
container.states[Contains].set_value(system, False)
for system in VisualParticleSystem.get_active_systems().values():
if not cls.ignore_nonrecipe_systems or system.name in recipe["input_systems"]:
if container.states[Contains].get_value(system):
container.states[Contains].set_value(system, False)
else:
# Remove the particles that are involved in this execution
for system_name, particle_idxs in execution_info["relevant_systems"].items():
system = get_system(system_name)
volume += len(particle_idxs) * np.pi * (system.particle_radius ** 3) * 4 / 3
system.remove_particles(idxs=np.array(list(particle_idxs)))
if not cls.is_multi_instance:
# Remove either all objects or only the ones specified in the input objects of the recipe
object_mask = in_volume.copy()
if cls.ignore_nonrecipe_objects:
object_category_mask = np.zeros_like(object_mask, dtype=bool)
for obj_category in recipe["input_objects"].keys():
object_category_mask[cls._CATEGORY_IDXS[obj_category]] = True
object_mask &= object_category_mask
objs_to_remove.extend(cls._OBJECTS[object_mask])
else:
# Remove the objects that are involved in this execution
for obj_category, objs in execution_info["relevant_objects"].items():
objs_to_remove.extend(objs)
volume += sum(obj.volume for obj in objs_to_remove)
# Define callback for spawning new objects inside container
def _spawn_object_in_container(obj):
# For simplicity sake, sample only OnTop
# TODO: Can we sample inside intelligently?
state = OnTop
# TODO: What to do if setter fails?
if not obj.states[state].set_value(container, True):
log.warning(f"Failed to spawn object {obj.name} in container {container.name}! Directly placing on top instead.")
pos = np.array(container.aabb_center) + np.array([0, 0, container.aabb_extent[2] / 2.0 + obj.aabb_extent[2] / 2.0])
obj.set_bbox_center_position_orientation(position=pos)
# Spawn in new objects
for category, n_instances in recipe["output_objects"].items():
# Multiply by number of instances of execution if this is a multi-instance recipe
if cls.is_multi_instance:
n_instances *= execution_info["num_instances"]
output_states = dict()
for state_type, state_value in recipe["output_states"][category]["unary"]:
output_states[state_type] = (state_value,)
for state_type, system_name, state_value in recipe["output_states"][category]["binary_system"]:
output_states[state_type] = (get_system(system_name), state_value)
n_category_objs = len(og.sim.scene.object_registry("category", category, []))
models = get_all_object_category_models(category=category)
for i in range(n_instances):
obj = DatasetObject(
name=f"{category}_{n_category_objs + i}",
category=category,
model=np.random.choice(models),
)
new_obj_attrs = ObjectAttrs(
obj=obj,
callback=_spawn_object_in_container,
states=output_states,
pos=np.ones(3) * (100.0 + i),
)
objs_to_add.append(new_obj_attrs)
# Spawn in new fluid
if len(recipe["output_systems"]) > 0:
# Only one system is allowed to be spawned
assert len(recipe["output_systems"]) == 1, "Only a single output system can be spawned for a given recipe!"
out_system = get_system(recipe["output_systems"][0])
out_system.generate_particles_from_link(
obj=container,
link=contained_particles_state.link,
# When ignore_nonrecipe_objects is True, we don't necessarily remove all objects in the container.
# Therefore, we need to check for contact when generating output systems.
check_contact=cls.ignore_nonrecipe_objects,
max_samples=int(volume / (np.pi * (out_system.particle_radius ** 3) * 4 / 3)),
)
# Return transition results
return TransitionResults(add=objs_to_add, remove=objs_to_remove)
@classproperty
def relax_recipe_systems(cls):
"""
Returns:
bool: Whether to relax the requirement of having all systems in the recipe contained in the container
"""
raise NotImplementedError("Must be implemented by subclass!")
@classproperty
def ignore_nonrecipe_systems(cls):
"""
Returns:
bool: Whether contained systems not relevant to the recipe should be ignored or not
"""
raise NotImplementedError("Must be implemented by subclass!")
@classproperty
def ignore_nonrecipe_objects(cls):
"""
Returns:
bool: Whether contained rigid objects not relevant to the recipe should be ignored or not
"""
raise NotImplementedError("Must be implemented by subclass!")
@classproperty
def use_garbage_fallback_recipe(cls):
"""
Returns:
bool: Whether this recipe rule should use a garbage fallback recipe if all conditions are met but no
valid recipe is found for a given container
"""
raise NotImplementedError("Must be implemented by subclass!")
@classproperty
def is_multi_instance(cls):
"""
Returns:
bool: Whether this rule can be applied multiple times to the same container, e.g. to cook multiple doughs
"""
return False
@classproperty
def _do_not_register_classes(cls):
# Don't register this class since it's an abstract template
classes = super()._do_not_register_classes
classes.add("RecipeRule")
return classes
class CookingPhysicalParticleRule(RecipeRule):
"""
Transition rule to apply to "cook" physical particles.
It comes with two forms of recipes:
1. xyz -> cooked__xyz, e.g. diced__chicken -> cooked__diced__chicken
2. xyz + cooked__water -> cooked__xyz, e.g. rice + cooked__water -> cooked__rice
During execution, we replace the input particles (xyz) with the output particles (cooked__xyz), and remove the
cooked__water if it was used as an input.
"""
@classmethod
def add_recipe(
cls,
name,
input_objects,
input_systems,
output_objects,
output_systems,
**kwargs,
):
"""
Adds a recipe to this recipe rule to check against. This defines a valid mapping of inputs that will transform
into the outputs
Args:
name (str): Name of the recipe
input_objects (dict): Maps object categories to number of instances required for the recipe
input_systems (list): List of system names required for the recipe
output_objects (dict): Maps object categories to number of instances to be spawned in the container when the recipe executes
output_systems (list): List of system names to be spawned in the container when the recipe executes. Currently the length is 1.
"""
assert len(input_objects) == 0, f"No input objects can be specified for {cls.__name__}, recipe: {name}!"
assert len(output_objects) == 0, f"No output objects can be specified for {cls.__name__}, recipe: {name}!"
assert len(input_systems) == 1 or len(input_systems) == 2, \
f"Only one or two input systems can be specified for {cls.__name__}, recipe: {name}!"
if len(input_systems) == 2:
assert input_systems[1] == "cooked__water", \
f"Second input system must be cooked__water for {cls.__name__}, recipe: {name}!"
assert len(output_systems) == 1, \
f"Exactly one output system needs to be specified for {cls.__name__}, recipe: {name}!"
super().add_recipe(
name=name,
input_objects=input_objects,
input_systems=input_systems,
output_objects=output_objects,
output_systems=output_systems,
**kwargs,
)
@classproperty
def candidate_filters(cls):
# Modify the container filter to include the heatable ability as well
candidate_filters = super().candidate_filters
candidate_filters["container"] = AndFilter(filters=[candidate_filters["container"], AbilityFilter(ability="heatable")])
return candidate_filters
@classmethod
def _generate_conditions(cls):
# Only heated objects are valid
return [StateCondition(filter_name="container", state=Heated, val=True, op=operator.eq)]
@classproperty
def relax_recipe_systems(cls):
return False
@classproperty
def ignore_nonrecipe_systems(cls):
return True
@classproperty
def ignore_nonrecipe_objects(cls):
return True
@classproperty
def use_garbage_fallback_recipe(cls):
return False
@classmethod
def _execute_recipe(cls, container, recipe, container_info):
system = get_system(recipe["input_systems"][0])
contained_particles_state = container.states[ContainedParticles].get_value(system)
in_volume_idx = np.where(contained_particles_state.in_volume)[0]
assert len(in_volume_idx) > 0, "No particles found in the container when executing recipe!"
# Remove uncooked particles
system.remove_particles(idxs=in_volume_idx)
# Generate cooked particles
cooked_system = get_system(recipe["output_systems"][0])
particle_positions = contained_particles_state.positions[in_volume_idx]
cooked_system.generate_particles(positions=particle_positions)
# Remove water if the cooking requires water
if len(recipe["input_systems"]) > 1:
cooked_water_system = get_system(recipe["input_systems"][1])
container.states[Contains].set_value(cooked_water_system, False)
return TransitionResults(add=[], remove=[])
class ToggleableMachineRule(RecipeRule):
"""
Transition mixing rule that leverages a single toggleable machine (e.g. electric mixer, coffee machine, blender),
which require toggledOn in order to trigger the recipe event.
It comes with two forms of recipes:
1. output is a single object, e.g. flour + butter + sugar -> dough, machine is electric mixer
2. output is a system, e.g. strawberry + milk -> smoothie, machine is blender
"""
@classmethod
def add_recipe(
cls,
name,
input_objects,
input_systems,
output_objects,
output_systems,
input_states=None,
output_states=None,
fillable_categories=None,
**kwargs,
):
"""
Adds a recipe to this recipe rule to check against. This defines a valid mapping of inputs that will transform
into the outputs
Args:
name (str): Name of the recipe
input_objects (dict): Maps object categories to number of instances required for the recipe
input_systems (list): List of system names required for the recipe
output_objects (dict): Maps object categories to number of instances to be spawned in the container when the recipe executes
output_systems (list): List of system names to be spawned in the container when the recipe executes. Currently the length is 1.
input_states (None or defaultdict(lambda: defaultdict(list))): Maps object categories to
["unary", "bianry_system", "binary_object"] to a list of states that must be satisfied for the recipe to execute
output_states (None or defaultdict(lambda: defaultdict(list))): Maps object categories to
["unary", "bianry_system"] to a list of states that should be set after the output objects are spawned
fillable_categories (None or set of str): If specified, set of fillable categories which are allowed
for this recipe. If None, any fillable is allowed
"""
if len(output_objects) > 0:
assert len(output_objects) == 1, f"Only one category of output object can be specified for {cls.__name__}, recipe: {name}!"
assert output_objects[list(output_objects.keys())[0]] == 1, f"Only one instance of output object can be specified for {cls.__name__}, recipe: {name}!"
super().add_recipe(
name=name,
input_objects=input_objects,
input_systems=input_systems,
output_objects=output_objects,
output_systems=output_systems,
input_states=input_states,
output_states=output_states,
fillable_categories=fillable_categories,
**kwargs,
)
@classproperty
def candidate_filters(cls):
# Modify the container filter to include toggleable ability as well
candidate_filters = super().candidate_filters
candidate_filters["container"] = AndFilter(filters=[
candidate_filters["container"],
AbilityFilter(ability="toggleable"),
# Exclude washer and clothes dryer because they are handled by WasherRule and DryerRule
NotFilter(CategoryFilter("washer")),
NotFilter(CategoryFilter("clothes_dryer")),
NotFilter(CategoryFilter("hot_tub")),
])
return candidate_filters
@classmethod
def _generate_conditions(cls):
# Container must be toggledOn, and should only be triggered once
return [ChangeConditionWrapper(
condition=StateCondition(filter_name="container", state=ToggledOn, val=True, op=operator.eq)
)]
@classproperty
def relax_recipe_systems(cls):
return False
@classproperty
def ignore_nonrecipe_systems(cls):
return False
@classproperty
def ignore_nonrecipe_objects(cls):
return False
@classproperty
def use_garbage_fallback_recipe(cls):
return True
class MixingToolRule(RecipeRule):
"""
Transition mixing rule that leverages "mixingTool" ability objects, which require touching between a mixing tool
and a container in order to trigger the recipe event.
Example: water + lemon_juice + sugar -> lemonade, mixing tool is spoon
"""
@classmethod
def add_recipe(
cls,
name,
input_objects,
input_systems,
output_objects,
output_systems,
input_states=None,
output_states=None,
**kwargs,
):
"""
Adds a recipe to this recipe rule to check against. This defines a valid mapping of inputs that will transform
into the outputs
Args:
name (str): Name of the recipe
input_objects (dict): Maps object categories to number of instances required for the recipe
input_systems (list): List of system names required for the recipe
output_objects (dict): Maps object categories to number of instances to be spawned in the container when the recipe executes
output_systems (list): List of system names to be spawned in the container when the recipe executes. Currently the length is 1.
input_states (None or defaultdict(lambda: defaultdict(list))): Maps object categories to
["unary", "bianry_system", "binary_object"] to a list of states that must be satisfied for the recipe to execute
output_states (None or defaultdict(lambda: defaultdict(list))): Maps object categories to
["unary", "bianry_system"] to a list of states that should be set after the output objects are spawned
"""
assert len(output_objects) == 0, f"No output objects can be specified for {cls.__name__}, recipe: {name}!"
assert len(input_systems) > 0, f"Some input systems need to be specified for {cls.__name__}, recipe: {name}!"
assert len(output_systems) == 1, \
f"Exactly one output system needs to be specified for {cls.__name__}, recipe: {name}!"
super().add_recipe(
name=name,
input_objects=input_objects,
input_systems=input_systems,
output_objects=output_objects,
output_systems=output_systems,
input_states=input_states,
output_states=output_states,
**kwargs,
)
@classproperty
def candidate_filters(cls):
# Add mixing tool filter as well
candidate_filters = super().candidate_filters
candidate_filters["mixingTool"] = AbilityFilter(ability="mixingTool")
return candidate_filters
@classmethod
def _generate_conditions(cls):
# Mixing tool must be touching the container, and should only be triggered once
return [ChangeConditionWrapper(
condition=TouchingAnyCondition(filter_1_name="container", filter_2_name="mixingTool")
)]
@classproperty
def relax_recipe_systems(cls):
return False
@classproperty
def ignore_nonrecipe_systems(cls):
return False
@classproperty
def ignore_nonrecipe_objects(cls):
return True
@classproperty
def use_garbage_fallback_recipe(cls):
return True
class CookingRule(RecipeRule):
"""
Transition mixing rule that approximates cooking recipes via a container and heatsource.
It is subclassed by CookingObjectRule and CookingSystemRule.
"""
# Counter that increments monotonically
COUNTER = 0
# Maps recipe name to current number of consecutive heating steps
_HEAT_STEPS = None
# Maps recipe name to the last timestep that it was active
_LAST_HEAT_TIMESTEP = None
@classmethod
def refresh(cls, object_candidates):
# Run super first
super().refresh(object_candidates=object_candidates)
# Iterate through all (updated) active recipes and store in internal variables if not already recorded
cls._HEAT_STEPS = dict() if cls._HEAT_STEPS is None else cls._HEAT_STEPS
cls._LAST_HEAT_TIMESTEP = dict() if cls._LAST_HEAT_TIMESTEP is None else cls._LAST_HEAT_TIMESTEP
for name in cls._ACTIVE_RECIPES.keys():
if name not in cls._HEAT_STEPS:
cls._HEAT_STEPS[name] = 0
cls._LAST_HEAT_TIMESTEP[name] = -1
@classmethod
def _validate_recipe_fillables_exist(cls, recipe):
"""
Validates that recipe @recipe's necessary fillable categorie(s) exist in the current scene
Args:
recipe (dict): Recipe whose fillable categories should be checked
Returns:
bool: True if there is at least a single valid fillable category in the current scene, else False
"""
fillable_categories = recipe["fillable_categories"]
if fillable_categories is None:
# Any is valid
return True
# Otherwise, at least one valid type must exist
for category in fillable_categories:
if len(og.sim.scene.object_registry("category", category, default_val=set())) > 0:
return True
# None found, return False
return False
@classmethod
def _validate_recipe_heatsources_exist(cls, recipe):
"""
Validates that recipe @recipe's necessary heatsource categorie(s) exist in the current scene
Args:
recipe (dict): Recipe whose heatsource categories should be checked
Returns:
bool: True if there is at least a single valid heatsource category in the current scene, else False
"""
heatsource_categories = recipe["heatsource_categories"]
if heatsource_categories is None:
# Any is valid
return True
# Otherwise, at least one valid type must exist
for category in heatsource_categories:
if len(og.sim.scene.object_registry("category", category, default_val=set())) > 0:
return True
# None found, return False
return False
@classmethod
def _validate_recipe_heatsource_is_valid(cls, recipe, heatsource_categories):
"""
Validates that there is a valid heatsource category in @heatsource_categories compatible with @recipe
Args:
recipe (dict): Recipe whose heatsource_categories should be checked against @heatsource_categories
heatsource_categories (set of str): Set of potential heatsource categories
Returns:
bool: True if there is a compatible category in @heatsource_categories, else False
"""
required_heatsource_categories = recipe["heatsource_categories"]
# Either no specific required and there is at least 1 heatsource or there is at least 1 matching heatsource
# between the required and available
return (required_heatsource_categories is None and len(heatsource_categories) > 0) or \
len(required_heatsource_categories.intersection(heatsource_categories)) > 0
@classmethod
def _compute_container_info(cls, object_candidates, container, global_info):
# Run super first
info = super()._compute_container_info(object_candidates=object_candidates, container=container, global_info=global_info)
# Compute whether each heatsource is affecting the container
info["heatsource_categories"] = set(obj.category for obj in object_candidates["heatSource"] if
obj.states[HeatSourceOrSink].affects_obj(container))
return info
@classmethod
def _is_recipe_active(cls, recipe):
# Check for heatsource categories first
if not cls._validate_recipe_heatsources_exist(recipe=recipe):
return False
# Otherwise, run super normally
return super()._is_recipe_active(recipe=recipe)
@classmethod
def _is_recipe_executable(cls, recipe, container, global_info, container_info):
# Check for heatsource compatibility first
if not cls._validate_recipe_heatsource_is_valid(recipe=recipe, heatsource_categories=container_info["heatsource_categories"]):
return False
# Run super
executable = super()._is_recipe_executable(
recipe=recipe,
container=container,
global_info=global_info,
container_info=container_info,
)
# If executable, increment heat counter by 1, if we were also active last timestep, else, reset to 1
if executable:
name = recipe["name"]
cls._HEAT_STEPS[name] = cls._HEAT_STEPS[name] + 1 if \
cls._LAST_HEAT_TIMESTEP[name] == cls.COUNTER - 1 else 1
cls._LAST_HEAT_TIMESTEP[name] = cls.COUNTER
# If valid number of timesteps met, recipe is indeed executable
executable = cls._HEAT_STEPS[name] >= recipe["timesteps"]
return executable
@classmethod
def add_recipe(
cls,
name,
input_objects,
input_systems,
output_objects,
output_systems,
input_states=None,
output_states=None,
fillable_categories=None,
heatsource_categories=None,
timesteps=None,
):
"""
Adds a recipe to this cooking recipe rule to check against. This defines a valid mapping of inputs that
will transform into the outputs
Args:
name (str): Name of the recipe
input_objects (dict): Maps object categories to number of instances required for the recipe
input_systems (list): List of system names required for the recipe
output_objects (dict): Maps object categories to number of instances to be spawned in the container when the recipe executes
output_systems (list): List of system names to be spawned in the container when the recipe executes. Currently the length is 1.
input_states (None or defaultdict(lambda: defaultdict(list))): Maps object categories to
["unary", "bianry_system", "binary_object"] to a list of states that must be satisfied for the recipe to execute
output_states (None or defaultdict(lambda: defaultdict(list))): Maps object categories to
["unary", "bianry_system"] to a list of states that should be set after the output objects are spawned
fillable_categories (None or set of str): If specified, set of fillable categories which are allowed
for this recipe. If None, any fillable is allowed
heatsource_categories (None or set of str): If specified, set of heatsource categories which are allowed
for this recipe. If None, any heatsource is allowed
timesteps (None or int): Number of subsequent heating steps required for the recipe to execute. If None,
it will be set to be 1, i.e.: instantaneous execution
"""
super().add_recipe(
name=name,
input_objects=input_objects,
input_systems=input_systems,
output_objects=output_objects,
output_systems=output_systems,
input_states=input_states,
output_states=output_states,
fillable_categories=fillable_categories,
heatsource_categories=heatsource_categories,
timesteps=1 if timesteps is None else timesteps,
)
@classproperty
def candidate_filters(cls):
# Add mixing tool filter as well
candidate_filters = super().candidate_filters
candidate_filters["heatSource"] = AbilityFilter(ability="heatSource")
return candidate_filters
@classmethod
def _generate_conditions(cls):
# Define a class to increment this class's internal time counter every time it is triggered
class TimeIncrementCondition(RuleCondition):
def __init__(self, cls):
self.cls = cls
def __call__(self, object_candidates):
# This is just a pass-through, but also increment the time
self.cls.COUNTER += 1
return True
def modifies_filter_names(self):
return set()
# Any heatsource must be active
return [
TimeIncrementCondition(cls=cls),
StateCondition(filter_name="heatSource", state=HeatSourceOrSink, val=True, op=operator.eq),
]
@classproperty
def use_garbage_fallback_recipe(cls):
return False
@classproperty
def _do_not_register_classes(cls):
# Don't register this class since it's an abstract template
classes = super()._do_not_register_classes
classes.add("CookingRule")
return classes
class CookingObjectRule(CookingRule):
"""
Cooking rule when output is objects (e.g. one dough can produce many bagels as output).
Example: bagel_dough + egg + sesame_seed -> bagel, heat source is oven, fillable is baking_sheet.
This is the only rule where is_multi_instance is True, where multiple copies of the recipe can be executed.
"""
@classmethod
def add_recipe(
cls,
name,
input_objects,
input_systems,
output_objects,
output_systems,
input_states=None,
output_states=None,
fillable_categories=None,
heatsource_categories=None,
timesteps=None,
):
"""
Adds a recipe to this cooking recipe rule to check against. This defines a valid mapping of inputs that
will transform into the outputs
Args:
name (str): Name of the recipe
input_objects (dict): Maps object categories to number of instances required for the recipe
input_systems (list): List of system names required for the recipe
output_objects (dict): Maps object categories to number of instances to be spawned in the container when the recipe executes
output_systems (list): List of system names to be spawned in the container when the recipe executes. Currently the length is 1.
input_states (None or defaultdict(lambda: defaultdict(list))): Maps object categories to
["unary", "bianry_system", "binary_object"] to a list of states that must be satisfied for the recipe to execute
output_states (None or defaultdict(lambda: defaultdict(list))): Maps object categories to
["unary", "bianry_system"] to a list of states that should be set after the output objects are spawned
fillable_categories (None or set of str): If specified, set of fillable categories which are allowed
for this recipe. If None, any fillable is allowed
heatsource_categories (None or set of str): If specified, set of heatsource categories which are allowed
for this recipe. If None, any heatsource is allowed
timesteps (None or int): Number of subsequent heating steps required for the recipe to execute. If None,
it will be set to be 1, i.e.: instantaneous execution
"""
assert len(output_systems) == 0, f"No output systems can be specified for {cls.__name__}, recipe: {name}!"
super().add_recipe(
name=name,
input_objects=input_objects,
input_systems=input_systems,
output_objects=output_objects,
output_systems=output_systems,
input_states=input_states,
output_states=output_states,
fillable_categories=fillable_categories,
heatsource_categories=heatsource_categories,
timesteps=timesteps,
)
@classproperty
def relax_recipe_systems(cls):
# We don't require systems like seasoning/cheese/sesame seeds/etc. to be contained in the baking sheet
return True
@classproperty
def ignore_nonrecipe_systems(cls):
return True
@classproperty
def ignore_nonrecipe_objects(cls):
return True
@classproperty
def is_multi_instance(cls):
return True
class CookingSystemRule(CookingRule):
"""
Cooking rule when output is a system.
Example: beef + tomato + chicken_stock -> stew, heat source is stove, fillable is stockpot.
"""
@classmethod
def add_recipe(
cls,
name,
input_objects,
input_systems,
output_objects,
output_systems,
input_states=None,
output_states=None,
fillable_categories=None,
heatsource_categories=None,
timesteps=None,
):
"""
Adds a recipe to this cooking recipe rule to check against. This defines a valid mapping of inputs that
will transform into the outputs
Args:
name (str): Name of the recipe
input_objects (dict): Maps object categories to number of instances required for the recipe
input_systems (list): List of system names required for the recipe
output_objects (dict): Maps object categories to number of instances to be spawned in the container when the recipe executes
output_systems (list): List of system names to be spawned in the container when the recipe executes. Currently the length is 1.
input_states (None or defaultdict(lambda: defaultdict(list))): Maps object categories to
["unary", "bianry_system", "binary_object"] to a list of states that must be satisfied for the recipe to execute
output_states (None or defaultdict(lambda: defaultdict(list))): Maps object categories to
["unary", "bianry_system"] to a list of states that should be set after the output objects are spawned
fillable_categories (None or set of str): If specified, set of fillable categories which are allowed
for this recipe. If None, any fillable is allowed
heatsource_categories (None or set of str): If specified, set of heatsource categories which are allowed
for this recipe. If None, any heatsource is allowed
timesteps (None or int): Number of subsequent heating steps required for the recipe to execute. If None,
it will be set to be 1, i.e.: instantaneous execution
"""
assert len(output_objects) == 0, f"No output objects can be specified for {cls.__name__}, recipe: {name}!"
super().add_recipe(
name=name,
input_objects=input_objects,
input_systems=input_systems,
output_objects=output_objects,
output_systems=output_systems,
input_states=input_states,
output_states=output_states,
fillable_categories=fillable_categories,
heatsource_categories=heatsource_categories,
timesteps=timesteps,
)
@classproperty
def relax_recipe_systems(cls):
return False
@classproperty
def ignore_nonrecipe_systems(cls):
return False
@classproperty
def ignore_nonrecipe_objects(cls):
return False
def import_recipes():
for json_file, rule_names in _JSON_FILES_TO_RULES.items():
recipe_fpath = os.path.join(os.path.dirname(bddl.__file__), "generated_data", "transition_map", "tm_jsons", json_file)
if not os.path.exists(recipe_fpath):
log.warning(f"Cannot find recipe file at {recipe_fpath}. Skipping importing recipes.")
with open(recipe_fpath, "r") as f:
rule_recipes = json.load(f)
for rule_name in rule_names:
rule = REGISTERED_RULES[rule_name]
if rule == WasherRule:
rule.register_cleaning_conditions(translate_bddl_washer_rule_to_og_washer_rule(rule_recipes))
elif issubclass(rule, RecipeRule):
log.info(f"Adding recipes of rule {rule_name}...")
for recipe in rule_recipes:
if "rule_name" in recipe:
recipe["name"] = recipe.pop("rule_name")
if "container" in recipe:
recipe["fillable_synsets"] = set(recipe.pop("container").keys())
if "heat_source" in recipe:
recipe["heatsource_synsets"] = set(recipe.pop("heat_source").keys())
if "machine" in recipe:
recipe["fillable_synsets"] = set(recipe.pop("machine").keys())
# Route the recipe to the correct rule: CookingObjectRule or CookingSystemRule
satisfied = True
og_recipe = translate_bddl_recipe_to_og_recipe(**recipe)
has_output_system = len(og_recipe["output_systems"]) > 0
if (rule == CookingObjectRule and has_output_system) or (rule == CookingSystemRule and not has_output_system):
satisfied = False
if satisfied:
rule.add_recipe(**og_recipe)
log.info(f"All recipes of rule {rule_name} imported successfully.")
import_recipes() | 108,938 | Python | 42.785772 | 179 | 0.630863 |
StanfordVL/OmniGibson/omnigibson/__init__.py | import logging
import os
import shutil
import signal
import tempfile
import builtins
# TODO: Need to fix somehow -- omnigibson gets imported first BEFORE we can actually modify the macros
from omnigibson.macros import gm
from omnigibson.envs import Environment
from omnigibson.scenes import REGISTERED_SCENES
from omnigibson.objects import REGISTERED_OBJECTS
from omnigibson.robots import REGISTERED_ROBOTS
from omnigibson.controllers import REGISTERED_CONTROLLERS
from omnigibson.tasks import REGISTERED_TASKS
from omnigibson.sensors import ALL_SENSOR_MODALITIES
from omnigibson.simulator import launch_simulator as launch
# Create logger
logging.basicConfig(format='[%(levelname)s] [%(name)s] %(message)s')
log = logging.getLogger(__name__)
builtins.ISAAC_LAUNCHED_FROM_JUPYTER = (
os.getenv("ISAAC_JUPYTER_KERNEL") is not None
) # We set this in the kernel.json file
# Always enable nest_asyncio because MaterialPrim calls asyncio.run()
import nest_asyncio
nest_asyncio.apply()
__version__ = "1.0.0"
log.setLevel(logging.DEBUG if gm.DEBUG else logging.INFO)
root_path = os.path.dirname(os.path.realpath(__file__))
# Store paths to example configs
example_config_path = os.path.join(os.path.dirname(os.path.realpath(__file__)), "configs")
# Initialize global variables
app = None # (this is a singleton so it's okay that it's global)
sim = None # (this is a singleton so it's okay that it's global)
# Create and expose a temporary directory for any use cases. It will get destroyed upon omni
# shutdown by the shutdown function.
tempdir = tempfile.mkdtemp()
def cleanup(*args, **kwargs):
# TODO: Currently tempfile removal will fail due to CopyPrim command (for example, GranularSystem in dicing_apple example.)
try:
shutil.rmtree(tempdir)
except PermissionError:
log.info("Permission error when removing temp files. Ignoring")
from omnigibson.simulator import logo_small
log.info(f"{'-' * 10} Shutting Down {logo_small()} {'-' * 10}")
def shutdown(due_to_signal=False):
if app is not None:
# If Isaac is running, we do the cleanup in its shutdown callback to avoid open handles.
# TODO: Automated cleanup in callback doesn't work for some reason. Need to investigate.
# Manually call cleanup for now.
cleanup()
app.close()
else:
# Otherwise, we do the cleanup here.
cleanup()
# If we're not shutting down due to a signal, we need to manually exit
if not due_to_signal:
exit(0)
def shutdown_handler(*args, **kwargs):
shutdown(due_to_signal=True)
return signal.default_int_handler(*args, **kwargs)
# Something somewhere disables the default SIGINT handler, so we need to re-enable it
signal.signal(signal.SIGINT, shutdown_handler)
| 2,812 | Python | 34.1625 | 127 | 0.726885 |
StanfordVL/OmniGibson/omnigibson/macros.py | """
Set of macros to use globally for OmniGibson. These are generally magic numbers that were tuned heuristically.
NOTE: This is generally decentralized -- the monolithic @settings variable is created here with some global values,
but submodules within OmniGibson may import this dictionary and add to it dynamically
"""
import os
import pathlib
from addict import Dict
# Initialize settings
macros = Dict()
gm = macros.globals
# Path (either relative to OmniGibson/omnigibson directory or global absolute path) for data
# Assets correspond to non-objects / scenes (e.g.: robots), and dataset incliudes objects + scene
# can override assets_path and dataset_path from environment variable
gm.ASSET_PATH = "data/assets"
if "OMNIGIBSON_ASSET_PATH" in os.environ:
gm.ASSET_PATH = os.environ["OMNIGIBSON_ASSET_PATH"]
gm.ASSET_PATH = os.path.expanduser(gm.ASSET_PATH)
if not os.path.isabs(gm.ASSET_PATH):
gm.ASSET_PATH = os.path.join(os.path.dirname(os.path.realpath(__file__)), gm.ASSET_PATH)
gm.DATASET_PATH = "data/og_dataset"
if "OMNIGIBSON_DATASET_PATH" in os.environ:
gm.DATASET_PATH = os.environ["OMNIGIBSON_DATASET_PATH"]
gm.DATASET_PATH = os.path.expanduser(gm.DATASET_PATH)
if not os.path.isabs(gm.DATASET_PATH):
gm.DATASET_PATH = os.path.join(os.path.dirname(os.path.realpath(__file__)), gm.DATASET_PATH)
gm.KEY_PATH = "data/omnigibson.key"
if "OMNIGIBSON_KEY_PATH" in os.environ:
gm.KEY_PATH = os.environ["OMNIGIBSON_KEY_PATH"]
gm.KEY_PATH = os.path.expanduser(gm.KEY_PATH)
if not os.path.isabs(gm.KEY_PATH):
gm.KEY_PATH = os.path.join(os.path.dirname(os.path.realpath(__file__)), gm.KEY_PATH)
# Which GPU to use -- None will result in omni automatically using an appropriate GPU. Otherwise, set with either
# integer or string-form integer
gm.GPU_ID = os.getenv("OMNIGIBSON_GPU_ID", None)
# Whether to generate a headless or non-headless application upon OmniGibson startup
gm.HEADLESS = (os.getenv("OMNIGIBSON_HEADLESS", 'False').lower() in ('true', '1', 't'))
# Whether to enable remote streaming. None disables it, other valid options are "native", "webrtc".
gm.REMOTE_STREAMING = os.getenv("OMNIGIBSON_REMOTE_STREAMING", None)
# What port the webrtc and http servers should run on. This is only used if REMOTE_STREAMING is set to "webrtc"
gm.HTTP_PORT = os.getenv("OMNIGIBSON_HTTP_PORT", 8211)
gm.WEBRTC_PORT = os.getenv("OMNIGIBSON_WEBRTC_PORT", 49100)
# Whether only the viewport should be shown in the GUI or not (if not, other peripherals are additionally shown)
# CANNOT be set at runtime
gm.GUI_VIEWPORT_ONLY = False
# Whether to use the viewer camera or not
gm.RENDER_VIEWER_CAMERA = True
# Do not suppress known omni warnings / errors, and also put omnigibson in a debug state
# This includes extra information for things such as object sampling, and also any debug
# logging messages
gm.DEBUG = (os.getenv("OMNIGIBSON_DEBUG", 'False').lower() in ('true', '1', 't'))
# Whether to print out disclaimers (i.e.: known failure cases resulting from Omniverse's current bugs / limitations)
gm.SHOW_DISCLAIMERS = False
# Whether to use omni's GPU dynamics
# This is necessary for certain features; e.g. particles (fluids / cloth)
gm.USE_GPU_DYNAMICS = False
# Whether to use high-fidelity rendering (this includes, e.g., isosurfaces)
gm.ENABLE_HQ_RENDERING = False
# Whether to use omni's flatcache feature or not (can speed up simulation)
gm.ENABLE_FLATCACHE = False
# Whether to use continuous collision detection or not (slower simulation, but can prevent
# objects from tunneling through each other)
gm.ENABLE_CCD = False
# Pairs setting -- USD default is 256 * 1024, physx default apparently is 32 * 1024.
gm.GPU_PAIRS_CAPACITY = 256 * 1024
# Aggregate pairs setting -- default is 1024, but is often insufficient for large scenes
gm.GPU_AGGR_PAIRS_CAPACITY = (2 ** 14) * 1024
# Maximum particle contacts allowed
gm.GPU_MAX_PARTICLE_CONTACTS = 1024 * 1024
# Maximum rigid contacts -- 524288 is default value from omni, but increasing too much can sometimes lead to crashes
gm.GPU_MAX_RIGID_CONTACT_COUNT = 524288 * 4
# Maximum rigid patches -- 81920 is default value from omni, but increasing too much can sometimes lead to crashes
gm.GPU_MAX_RIGID_PATCH_COUNT = 81920 * 4
# Whether to enable object state logic or not
gm.ENABLE_OBJECT_STATES = True
# Whether to enable transition rules or not
gm.ENABLE_TRANSITION_RULES = True
# Default settings for the omni UI viewer
gm.DEFAULT_VIEWER_WIDTH = 1280
gm.DEFAULT_VIEWER_HEIGHT = 720
# (Demo-purpose) Whether to activate Assistive Grasping mode for Cloth (it's handled differently from RigidBody)
gm.AG_CLOTH = False
# Forced light intensity for all DatasetObjects. None if the USD-provided intensities should be respected.
gm.FORCE_LIGHT_INTENSITY = 150000
# Forced roughness for all DatasetObjects. None if the USD-provided roughness maps should be respected.
gm.FORCE_ROUGHNESS = 0.7
# Create helper function for generating sub-dictionaries
def create_module_macros(module_path):
"""
Creates a dictionary that can be populated with module macros based on the module's @module_path
Args:
module_path (str): Relative path from the package root directory pointing to the module. This will be parsed
to generate the appropriate sub-macros dictionary, e.g., for module "dirty" in
omnigibson/object_states_dirty.py, this would generate a dictionary existing at macros.object_states.dirty
Returns:
Dict: addict dictionary which can be populated with values
"""
# Sanity check module path, make sure omnigibson/ is in the path
module_path = pathlib.Path(module_path)
omnigibson_path = pathlib.Path(__file__).parent
# Trim the .py, and anything before and including omnigibson/, and split into its appropriate parts
try:
subsections = module_path.with_suffix("").relative_to(omnigibson_path).parts
except ValueError:
raise ValueError("module_path is expected to be a filepath including the omnigibson root directory, got: {module_path}!")
# Create and return the generated sub-dictionary
def _recursively_get_or_create_dict(dic, keys):
# If no entry is in @keys, it returns @dic
# Otherwise, checks whether the dictionary contains the first entry in @keys, if so, it grabs the
# corresponding nested dictionary, otherwise, generates a new Dict() as the value
# It then recurisvely calls this function with the new dic and the remaining keys
if len(keys) == 0:
return dic
else:
key = keys[0]
if key not in dic:
dic[key] = Dict()
return _recursively_get_or_create_dict(dic=dic[key], keys=keys[1:])
return _recursively_get_or_create_dict(dic=macros, keys=subsections)
| 6,808 | Python | 42.647436 | 129 | 0.735605 |
StanfordVL/OmniGibson/omnigibson/lazy.py | import sys
from omnigibson.utils.lazy_import_utils import LazyImporter
sys.modules[__name__] = LazyImporter("", None)
| 119 | Python | 22.999995 | 59 | 0.764706 |
StanfordVL/OmniGibson/omnigibson/scenes/__init__.py | from omnigibson.scenes.scene_base import Scene, REGISTERED_SCENES
from omnigibson.scenes.traversable_scene import TraversableScene
from omnigibson.scenes.static_traversable_scene import StaticTraversableScene
from omnigibson.scenes.interactive_traversable_scene import InteractiveTraversableScene
| 297 | Python | 58.599988 | 87 | 0.888889 |
StanfordVL/OmniGibson/omnigibson/scenes/static_traversable_scene.py | import os
import numpy as np
from omnigibson.scenes.traversable_scene import TraversableScene
from omnigibson.prims.geom_prim import CollisionVisualGeomPrim
from omnigibson.utils.asset_utils import get_scene_path
from omnigibson.utils.usd_utils import add_asset_to_stage
from omnigibson.utils.ui_utils import create_module_logger
# Create module logger
log = create_module_logger(module_name=__name__)
class StaticTraversableScene(TraversableScene):
"""
Static traversable scene class for OmniGibson, where scene is defined by a singular mesh (no intereactable objects)
"""
def __init__(
self,
scene_model,
scene_file=None,
trav_map_resolution=0.1,
default_erosion_radius=0.0,
trav_map_with_objects=True,
num_waypoints=10,
waypoint_resolution=0.2,
floor_plane_visible=False,
floor_plane_color=(1.0, 1.0, 1.0),
):
"""
Args:
scene_model (str): Scene model name, e.g.: Adrian
scene_file (None or str): If specified, full path of JSON file to load (with .json).
None results in no additional objects being loaded into the scene
trav_map_resolution (float): traversability map resolution
default_erosion_radius (float): default map erosion radius in meters
trav_map_with_objects (bool): whether to use objects or not when constructing graph
num_waypoints (int): number of way points returned
waypoint_resolution (float): resolution of adjacent way points
floor_plane_visible (bool): whether to render the additionally added floor plane
floor_plane_color (3-array): if @floor_plane_visible is True, this determines the (R,G,B) color assigned
to the generated floor plane
"""
# Store and initialize additional variables
self._floor_heights = None
self._scene_mesh = None
# Run super init
super().__init__(
scene_model=scene_model,
scene_file=scene_file,
trav_map_resolution=trav_map_resolution,
default_erosion_radius=default_erosion_radius,
trav_map_with_objects=trav_map_with_objects,
num_waypoints=num_waypoints,
waypoint_resolution=waypoint_resolution,
use_floor_plane=True,
floor_plane_visible=floor_plane_visible,
floor_plane_color=floor_plane_color,
)
def _load(self):
# Run super first
super()._load()
# Load the scene mesh (use downsampled one if available)
filename = os.path.join(get_scene_path(self.scene_model), "mesh_z_up_downsampled.obj")
if not os.path.isfile(filename):
filename = os.path.join(get_scene_path(self.scene_model), "mesh_z_up.obj")
scene_prim = add_asset_to_stage(
asset_path=filename,
prim_path=f"/World/scene_{self.scene_model}",
)
# Grab the actual mesh prim
self._scene_mesh = CollisionVisualGeomPrim(
prim_path=f"/World/scene_{self.scene_model}/mesh_z_up/{self.scene_model}_mesh_texture",
name=f"{self.scene_model}_mesh",
)
# Load floor metadata
floor_height_path = os.path.join(get_scene_path(self.scene_model), "floors.txt")
assert os.path.isfile(floor_height_path), f"floor_heights.txt cannot be found in model: {self.scene_model}"
with open(floor_height_path, "r") as f:
self.floor_heights = sorted(list(map(float, f.readlines())))
log.debug("Floors {}".format(self.floor_heights))
# Move the floor plane to the first floor by default
self.move_floor_plane(floor=0)
# Filter the collision between the scene mesh and the floor plane
self._scene_mesh.add_filtered_collision_pair(prim=self._floor_plane)
# Load the traversability map
self._trav_map.load_map(get_scene_path(self.scene_model))
def move_floor_plane(self, floor=0, additional_elevation=0.02, height=None):
"""
Resets the floor plane to a new floor
Args:
floor (int): Integer identifying the floor to move the floor plane to
additional_elevation (float): Additional elevation with respect to the height of the floor
height (None or float): If specified, alternative parameter to directly control the height of the ground
plane. Note that this will override @additional_elevation and @floor!
"""
height = height if height is not None else self.floor_heights[floor] + additional_elevation
self._floor_plane.set_position(np.array([0, 0, height]))
def get_floor_height(self, floor=0):
"""
Return the current floor height (in meter)
Returns:
int: current floor height
"""
return self.floor_heights[floor]
@property
def n_floors(self):
return len(self._floor_heights)
| 5,029 | Python | 39.24 | 119 | 0.6379 |
StanfordVL/OmniGibson/omnigibson/scenes/traversable_scene.py | from omnigibson.scenes.scene_base import Scene
from omnigibson.maps.traversable_map import TraversableMap
from omnigibson.utils.ui_utils import create_module_logger
# Create module logger
log = create_module_logger(module_name=__name__)
class TraversableScene(Scene):
"""
Traversable scene class.
Contains the functionalities for navigation such as shortest path computation
"""
def __init__(
self,
scene_model,
scene_file=None,
trav_map_resolution=0.1,
default_erosion_radius=0.0,
trav_map_with_objects=True,
num_waypoints=10,
waypoint_resolution=0.2,
use_floor_plane=True,
floor_plane_visible=True,
floor_plane_color=(1.0, 1.0, 1.0),
):
"""
Args:
scene_model (str): Scene model name, e.g.: Adrian or Rs_int
scene_file (None or str): If specified, full path of JSON file to load (with .json).
None results in no additional objects being loaded into the scene
trav_map_resolution (float): traversability map resolution
default_erosion_radius (float): default map erosion radius in meters
trav_map_with_objects (bool): whether to use objects or not when constructing graph
num_waypoints (int): number of way points returned
waypoint_resolution (float): resolution of adjacent way points
use_floor_plane (bool): whether to load a flat floor plane into the simulator
floor_plane_visible (bool): whether to render the additionally added floor plane
floor_plane_color (3-array): if @floor_plane_visible is True, this determines the (R,G,B) color assigned
to the generated floor plane
"""
log.info("TraversableScene model: {}".format(scene_model))
self.scene_model = scene_model
# Create traversable map
self._trav_map = TraversableMap(
map_resolution=trav_map_resolution,
default_erosion_radius=default_erosion_radius,
trav_map_with_objects=trav_map_with_objects,
num_waypoints=num_waypoints,
waypoint_resolution=waypoint_resolution,
)
# Run super init
super().__init__(
scene_file=scene_file,
use_floor_plane=use_floor_plane,
floor_plane_visible=floor_plane_visible,
floor_plane_color=floor_plane_color,
)
@property
def trav_map(self):
"""
Returns:
TraversableMap: Map for computing connectivity between nodes for this scene
"""
return self._trav_map
def get_random_point(self, floor=None, reference_point=None, robot=None):
return self._trav_map.get_random_point(floor=floor, reference_point=reference_point, robot=robot)
def get_shortest_path(self, floor, source_world, target_world, entire_path=False, robot=None):
return self._trav_map.get_shortest_path(
floor=floor,
source_world=source_world,
target_world=target_world,
entire_path=entire_path,
robot=robot,
)
| 3,174 | Python | 38.19753 | 116 | 0.63012 |
StanfordVL/OmniGibson/omnigibson/scenes/interactive_traversable_scene.py | import os
from omnigibson.robots.robot_base import REGISTERED_ROBOTS
from omnigibson.robots.robot_base import m as robot_macros
from omnigibson.scenes.traversable_scene import TraversableScene
from omnigibson.maps.segmentation_map import SegmentationMap
from omnigibson.utils.asset_utils import get_og_scene_path
from omnigibson.utils.constants import STRUCTURE_CATEGORIES
from omnigibson.utils.ui_utils import create_module_logger
# Create module logger
log = create_module_logger(module_name=__name__)
class InteractiveTraversableScene(TraversableScene):
"""
Create an interactive scene defined from a scene json file.
In general, this supports curated, pre-defined scene layouts with annotated objects.
This adds semantic support via a segmentation map generated for this specific scene.
"""
def __init__(
self,
scene_model,
scene_instance=None,
scene_file=None,
trav_map_resolution=0.1,
default_erosion_radius=0.0,
trav_map_with_objects=True,
num_waypoints=10,
waypoint_resolution=0.2,
load_object_categories=None,
not_load_object_categories=None,
load_room_types=None,
load_room_instances=None,
load_task_relevant_only=False,
seg_map_resolution=0.1,
include_robots=True,
):
"""
Args:
scene_model (str): Scene model name, e.g.: Rs_int
scene_instance (None or str): name of json file to load (without .json); if None,
defaults to og_dataset/scenes/<scene_model>/json/<scene_instance>.urdf
scene_file (None or str): If specified, full path of JSON file to load (with .json).
This will override scene_instance and scene_model!
trav_map_resolution (float): traversability map resolution
default_erosion_radius (float): default map erosion radius in meters
trav_map_with_objects (bool): whether to use objects or not when constructing graph
num_waypoints (int): number of way points returned
waypoint_resolution (float): resolution of adjacent way points
load_object_categories (None or list): if specified, only load these object categories into the scene
not_load_object_categories (None or list): if specified, do not load these object categories into the scene
load_room_types (None or list): only load objects in these room types into the scene
load_room_instances (None or list): if specified, only load objects in these room instances into the scene
load_task_relevant_only (bool): Whether only task relevant objects (and building structure) should be loaded
seg_map_resolution (float): room segmentation map resolution
include_robots (bool): whether to also include the robot(s) defined in the scene
"""
# Store attributes from inputs
self.include_robots = include_robots
# Infer scene directory
self.scene_dir = get_og_scene_path(scene_model)
# Other values that will be loaded at runtime
self.load_object_categories = None
self.not_load_object_categories = None
self.load_room_instances = None
self.load_task_relevant_only = load_task_relevant_only
# Get scene information
if scene_file is None:
scene_file = self.get_scene_loading_info(
scene_model=scene_model,
scene_instance=scene_instance,
)
# Load room semantic and instance segmentation map (must occur AFTER inferring scene directory)
self._seg_map = SegmentationMap(scene_dir=self.scene_dir, map_resolution=seg_map_resolution)
# Decide which room(s) and object categories to load
self.filter_rooms_and_object_categories(
load_object_categories, not_load_object_categories, load_room_types, load_room_instances
)
# Run super init first
super().__init__(
scene_model=scene_model,
scene_file=scene_file,
trav_map_resolution=trav_map_resolution,
default_erosion_radius=default_erosion_radius,
trav_map_with_objects=trav_map_with_objects,
num_waypoints=num_waypoints,
waypoint_resolution=waypoint_resolution,
use_floor_plane=False,
)
def get_scene_loading_info(self, scene_model, scene_instance=None):
"""
Gets scene loading info to know what single USD file to load, specified indirectly via @scene_instance if it
is specified, otherwise, will grab the "best" scene file to load.
Args:
scene_model (str): Name of the scene to load, e.g, Rs_int, etc.
scene_instance (None or str): If specified, should be name of json file to load. (without .json), default to
og_dataset/scenes/<scene_model>/json/<scene_instance>.json
Returns:
str: Absolute path to the desired scene file (.json) to load
"""
# Infer scene file from model and directory
fname = "{}_best".format(scene_model) if scene_instance is None else scene_instance
return os.path.join(self.scene_dir, "json", "{}.json".format(fname))
def filter_rooms_and_object_categories(
self, load_object_categories, not_load_object_categories, load_room_types, load_room_instances
):
"""
Handle partial scene loading based on object categories, room types or room instances
Args:
load_object_categories (None or list): if specified, only load these object categories into the scene
not_load_object_categories (None or list): if specified, do not load these object categories into the scene
load_room_types (None or list): only load objects in these room types into the scene
load_room_instances (None or list): if specified, only load objects in these room instances into the scene
"""
self.load_object_categories = [load_object_categories] if \
isinstance(load_object_categories, str) else load_object_categories
self.not_load_object_categories = [not_load_object_categories] if \
isinstance(not_load_object_categories, str) else not_load_object_categories
if load_room_instances is not None:
if isinstance(load_room_instances, str):
load_room_instances = [load_room_instances]
load_room_instances_filtered = []
for room_instance in load_room_instances:
if room_instance in self._seg_map.room_ins_name_to_ins_id:
load_room_instances_filtered.append(room_instance)
else:
log.warning("room_instance [{}] does not exist.".format(room_instance))
self.load_room_instances = load_room_instances_filtered
elif load_room_types is not None:
if isinstance(load_room_types, str):
load_room_types = [load_room_types]
load_room_instances_filtered = []
for room_type in load_room_types:
if room_type in self._seg_map.room_sem_name_to_ins_name:
load_room_instances_filtered.extend(self._seg_map.room_sem_name_to_ins_name[room_type])
else:
log.warning("room_type [{}] does not exist.".format(room_type))
self.load_room_instances = load_room_instances_filtered
else:
self.load_room_instances = None
def _load(self):
# Run super first
super()._load()
# Load the traversability map if we have the connectivity graph
maps_path = os.path.join(self.scene_dir, "layout")
self._trav_map.load_map(maps_path)
def _should_load_object(self, obj_info, task_metadata):
name = obj_info["args"]["name"]
category = obj_info["args"].get("category", "object")
in_rooms = obj_info["args"].get("in_rooms", None)
if isinstance(in_rooms, str):
assert "," not in in_rooms
in_rooms = [in_rooms] if isinstance(in_rooms, str) else in_rooms
# Do not load these object categories (can blacklist building structures as well)
not_blacklisted = self.not_load_object_categories is None or category not in self.not_load_object_categories
# Only load these object categories (no need to white list building structures)
task_relevant_names = set(task_metadata["inst_to_name"].values()) if "inst_to_name" in task_metadata else set()
is_task_relevant = name in task_relevant_names or category in STRUCTURE_CATEGORIES
whitelisted = (
# Either no whitelisting-only mode is on
(self.load_object_categories is None and not self.load_task_relevant_only) or
# Or the object is in the whitelist
(self.load_object_categories is not None and category in self.load_object_categories) or
# Or it's in the task relevant list
(self.load_task_relevant_only and is_task_relevant)
)
# This object is not located in one of the selected rooms, skip
valid_room = self.load_room_instances is None or len(set(self.load_room_instances) & set(in_rooms)) > 0
# Check whether this is an agent and we allow agents
agent_ok = self.include_robots or obj_info["class_name"] not in REGISTERED_ROBOTS
# We only load this model if all the above conditions are met
return not_blacklisted and whitelisted and valid_room and agent_ok
@property
def seg_map(self):
"""
Returns:
SegmentationMap: Map for segmenting this scene
"""
return self._seg_map
@classmethod
def modify_init_info_for_restoring(cls, init_info):
# Run super first
super().modify_init_info_for_restoring(init_info=init_info)
# We also make sure we load in any robots, and also pop any filters that were stored
init_info["args"]["include_robots"] = True
init_info["args"]["load_object_categories"] = None
init_info["args"]["not_load_object_categories"] = None
init_info["args"]["load_room_types"] = None
init_info["args"]["load_room_instances"] = None
| 10,344 | Python | 46.893518 | 120 | 0.647235 |
StanfordVL/OmniGibson/omnigibson/scenes/scene_base.py | import json
from abc import ABC
from itertools import combinations
import numpy as np
import omnigibson as og
import omnigibson.lazy as lazy
from omnigibson.macros import create_module_macros, gm
from omnigibson.prims.xform_prim import XFormPrim
from omnigibson.prims.material_prim import MaterialPrim
from omnigibson.utils.constants import STRUCTURE_CATEGORIES
from omnigibson.utils.python_utils import classproperty, Serializable, Registerable, Recreatable, \
create_object_from_init_info
from omnigibson.utils.registry_utils import SerializableRegistry
from omnigibson.utils.ui_utils import create_module_logger
from omnigibson.utils.usd_utils import CollisionAPI
from omnigibson.objects.object_base import BaseObject
from omnigibson.objects.dataset_object import DatasetObject
from omnigibson.systems.system_base import SYSTEM_REGISTRY, clear_all_systems, get_system
from omnigibson.objects.light_object import LightObject
from omnigibson.robots.robot_base import m as robot_macros
# Create module logger
log = create_module_logger(module_name=__name__)
# Create settings for this module
m = create_module_macros(module_path=__file__)
# Default texture to use for skybox
m.DEFAULT_SKYBOX_TEXTURE = f"{gm.ASSET_PATH}/models/background/sky.jpg"
# Global dicts that will contain mappings
REGISTERED_SCENES = dict()
class Scene(Serializable, Registerable, Recreatable, ABC):
"""
Base class for all Scene objects.
Contains the base functionalities for an arbitrary scene with an arbitrary set of added objects
"""
def __init__(
self,
scene_file=None,
use_floor_plane=True,
floor_plane_visible=True,
use_skybox=True,
floor_plane_color=(1.0, 1.0, 1.0),
):
"""
Args:
scene_file (None or str): If specified, full path of JSON file to load (with .json).
None results in no additional objects being loaded into the scene
use_floor_plane (bool): whether to load a flat floor plane into the simulator
floor_plane_visible (bool): whether to render the additionally added floor plane
floor_plane_color (3-array): if @floor_plane_visible is True, this determines the (R,G,B) color assigned
to the generated floor plane
"""
# Store internal variables
self.scene_file = scene_file
self._loaded = False # Whether this scene exists in the stage or not
self._initialized = False # Whether this scene has its internal handles / info initialized or not (occurs AFTER and INDEPENDENTLY from loading!)
self._registry = None
self._world_prim = None
self._initial_state = None
self._objects_info = None # Information associated with this scene
self._use_floor_plane = use_floor_plane
self._floor_plane_visible = floor_plane_visible
self._floor_plane_color = floor_plane_color
self._floor_plane = None
self._use_skybox = use_skybox
self._skybox = None
# Call super init
super().__init__()
@property
def registry(self):
"""
Returns:
SerializableRegistry: Master registry containing sub-registries of objects, robots, systems, etc.
"""
return self._registry
@property
def skybox(self):
"""
Returns:
None or LightObject: Skybox light associated with this scene, if it is used
"""
return self._skybox
@property
def floor_plane(self):
"""
Returns:
None or XFormPrim: Generated floor plane prim, if it is used
"""
return self._floor_plane
@property
def object_registry(self):
"""
Returns:
SerializableRegistry: Object registry containing all active standalone objects in the scene
"""
return self._registry(key="name", value="object_registry")
@property
def system_registry(self):
"""
Returns:
SerializableRegistry: System registry containing all systems in the scene (e.g.: water, dust, etc.)
"""
return self._registry(key="name", value="system_registry")
@property
def objects(self):
"""
Get the objects in the scene.
Returns:
list of BaseObject: Standalone object(s) that are currently in this scene
"""
return self.object_registry.objects
@property
def robots(self):
"""
Robots in the scene
Returns:
list of BaseRobot: Robot(s) that are currently in this scene
"""
return list(self.object_registry("category", robot_macros.ROBOT_CATEGORY, []))
@property
def systems(self):
"""
Systems in the scene
Returns:
list of BaseSystem: System(s) that are available to use in this scene
"""
return self.system_registry.objects
@property
def object_registry_unique_keys(self):
"""
Returns:
list of str: Keys with which to index into the object registry. These should be valid public attributes of
prims that we can use as unique IDs to reference prims, e.g., prim.prim_path, prim.name, etc.
"""
return ["name", "prim_path", "uuid"]
@property
def object_registry_group_keys(self):
"""
Returns:
list of str: Keys with which to index into the object registry. These should be valid public attributes of
prims that we can use as grouping IDs to reference prims, e.g., prim.in_rooms
"""
return ["prim_type", "states", "category", "fixed_base", "in_rooms", "abilities"]
@property
def loaded(self):
return self._loaded
@property
def initialized(self):
return self._initialized
def _load(self):
"""
Load the scene into simulator
The elements to load may include: floor, building, objects, etc.
"""
# Create collision group for fixed base objects' non root links, root links, and building structures
CollisionAPI.create_collision_group(col_group="fixed_base_nonroot_links", filter_self_collisions=False)
# Disable collision between root links of fixed base objects
CollisionAPI.create_collision_group(col_group="fixed_base_root_links", filter_self_collisions=True)
# Disable collision between building structures
CollisionAPI.create_collision_group(col_group="structures", filter_self_collisions=True)
# Disable collision between building structures and 1. fixed base objects, 2. attached objects
CollisionAPI.add_group_filter(col_group="structures", filter_group="fixed_base_nonroot_links")
CollisionAPI.add_group_filter(col_group="structures", filter_group="fixed_base_root_links")
# We just add a ground plane if requested
if self._use_floor_plane:
self.add_ground_plane(color=self._floor_plane_color, visible=self._floor_plane_visible)
# Also add skybox if requested
if self._use_skybox:
self._skybox = LightObject(
prim_path="/World/skybox",
name="skybox",
category="background",
light_type="Dome",
intensity=1500,
fixed_base=True,
)
og.sim.import_object(self._skybox, register=False)
self._skybox.color = (1.07, 0.85, 0.61)
self._skybox.texture_file_path = m.DEFAULT_SKYBOX_TEXTURE
def _load_objects_from_scene_file(self):
"""
Loads scene objects based on metadata information found in the current USD stage's scene info
(information stored in the world prim's CustomData)
"""
# Grab objects info from the scene file
with open(self.scene_file, "r") as f:
scene_info = json.load(f)
init_info = scene_info["objects_info"]["init_info"]
init_state = scene_info["state"]["object_registry"]
init_systems = scene_info["state"]["system_registry"].keys()
task_metadata = {}
try:
task_metadata = scene_info["metadata"]["task"]
except:
pass
# Create desired systems
for system_name in init_systems:
if gm.USE_GPU_DYNAMICS:
get_system(system_name)
else:
log.warning(f"System {system_name} is not supported without GPU dynamics! Skipping...")
# Iterate over all scene info, and instantiate object classes linked to the objects found on the stage
# accordingly
for obj_name, obj_info in init_info.items():
# Check whether we should load the object or not
if not self._should_load_object(obj_info=obj_info, task_metadata=task_metadata):
continue
# Create object class instance
obj = create_object_from_init_info(obj_info)
# Import into the simulator
og.sim.import_object(obj)
# Set the init pose accordingly
obj.set_position_orientation(
position=init_state[obj_name]["root_link"]["pos"],
orientation=init_state[obj_name]["root_link"]["ori"],
)
def _load_metadata_from_scene_file(self):
"""
Loads metadata from self.scene_file and stores it within the world prim's CustomData
"""
with open(self.scene_file, "r") as f:
scene_info = json.load(f)
# Write the metadata
for key, data in scene_info.get("metadata", dict()).items():
og.sim.write_metadata(key=key, data=data)
def _should_load_object(self, obj_info, task_metadata):
"""
Helper function to check whether we should load an object given its init_info. Useful for potentially filtering
objects based on, e.g., their category, size, etc.
Subclasses can implement additional logic. By default, this returns True
Args:
obj_info (dict): Dictionary of object kwargs that will be used to load the object
Returns:
bool: Whether this object should be loaded or not
"""
return True
def load(self):
"""
Load the scene into simulator
The elements to load may include: floor, building, objects, etc.
"""
# Make sure simulator is stopped
assert og.sim.is_stopped(), "Simulator should be stopped when loading this scene!"
# Do not override this function. Override _load instead.
if self._loaded:
raise ValueError("This scene is already loaded.")
# Create the registry for tracking all objects in the scene
self._registry = self._create_registry()
# Store world prim and load the scene into the simulator
self._world_prim = og.sim.world_prim
self._load()
# If we have any scene file specified, use it to load the objects within it and also update the initial state
# and metadata
if self.scene_file is not None:
self._load_objects_from_scene_file()
self._load_metadata_from_scene_file()
# We're now loaded
self._loaded = True
# Always stop the sim if we started it internally
if not og.sim.is_stopped():
og.sim.stop()
def clear(self):
"""
Clears any internal state before the scene is destroyed
"""
# Clears systems so they can be re-initialized
clear_all_systems()
def _initialize(self):
"""
Initializes state of this scene and sets up any references necessary post-loading. Should be implemented by
sub-class for extended utility
"""
pass
def initialize(self):
"""
Initializes state of this scene and sets up any references necessary post-loading. Subclasses should
implement / extend the _initialize() method.
"""
assert not self._initialized, "Scene can only be initialized once! (It is already initialized)"
self._initialize()
# Grab relevant objects info
self.update_objects_info()
self.wake_scene_objects()
self._initialized = True
# Store initial state, which may be loaded from a scene file if specified
if self.scene_file is None:
init_state = self.dump_state(serialized=False)
else:
with open(self.scene_file, "r") as f:
scene_info = json.load(f)
init_state = scene_info["state"]
og.sim.load_state(init_state, serialized=False)
self._initial_state = init_state
def _create_registry(self):
"""
Creates the internal registry used for tracking all objects
Returns:
SerializableRegistry: registry for tracking all objects
"""
# Create meta registry and populate with internal registries for robots, objects, and systems
registry = SerializableRegistry(
name="master_registry",
class_types=SerializableRegistry,
)
# Add registry for systems -- this is already created externally, so we just update it and pull it directly
registry.add(obj=SYSTEM_REGISTRY)
# Add registry for objects
registry.add(obj=SerializableRegistry(
name="object_registry",
class_types=BaseObject,
default_key="name",
unique_keys=self.object_registry_unique_keys,
group_keys=self.object_registry_group_keys,
))
return registry
def wake_scene_objects(self):
"""
Force wakeup sleeping objects
"""
for obj in self.objects:
obj.wake()
def get_objects_with_state(self, state):
"""
Get the objects with a given state in the scene.
Args:
state (BaseObjectState): state of the objects to get
Returns:
set: all objects with the given state
"""
return self.object_registry("states", state, set())
def get_objects_with_state_recursive(self, state):
"""
Get the objects with a given state and its subclasses in the scene.
Args:
state (BaseObjectState): state of the objects to get
Returns:
set: all objects with the given state and its subclasses
"""
objs = set()
states = {state}
while states:
next_states = set()
for state in states:
objs |= self.object_registry("states", state, set())
next_states |= set(state.__subclasses__())
states = next_states
return objs
def _add_object(self, obj):
"""
Add an object to the scene's internal object tracking mechanisms.
Note that if the scene is not loaded, it should load this added object alongside its other objects when
scene.load() is called. The object should also be accessible through scene.objects.
Args:
obj (BaseObject): the object to load into the simulator
"""
pass
def add_object(self, obj, register=True, _is_call_from_simulator=False):
"""
Add an object to the scene, loading it if the scene is already loaded.
Note that calling add_object to an already loaded scene should only be done by the simulator's import_object()
function.
Args:
obj (BaseObject): the object to load
register (bool): whether to track this object internally in the scene registry
_is_call_from_simulator (bool): whether the caller is the simulator. This should
**not** be set by any callers that are not the Simulator class
Returns:
Usd.Prim: the prim of the loaded object if the scene was already loaded, or None if the scene is not loaded
(in that case, the object is stored to be loaded together with the scene)
"""
# Make sure the simulator is the one calling this function
assert _is_call_from_simulator, "Use import_object() for adding objects to a simulator and scene!"
# If the scene is already loaded, we need to load this object separately. Otherwise, don't do anything now,
# let scene._load() load the object when called later on.
prim = obj.load()
# If this object is fixed and is NOT an agent, disable collisions between the fixed links of the fixed objects
# This is to account for cases such as Tiago, which has a fixed base which is needed for its global base joints
# We do this by adding the object to our tracked collision groups
if obj.fixed_base and obj.category != robot_macros.ROBOT_CATEGORY and not obj.visual_only:
# TODO: Remove structure hotfix once asset collision meshes are fixed!!
if obj.category in STRUCTURE_CATEGORIES:
CollisionAPI.add_to_collision_group(col_group="structures", prim_path=obj.prim_path)
else:
for link in obj.links.values():
CollisionAPI.add_to_collision_group(
col_group="fixed_base_root_links" if link == obj.root_link else "fixed_base_nonroot_links",
prim_path=link.prim_path,
)
# Add this object to our registry based on its type, if we want to register it
if register:
self.object_registry.add(obj)
# Run any additional scene-specific logic with the created object
self._add_object(obj)
return prim
def remove_object(self, obj):
"""
Method to remove an object from the simulator
Args:
obj (BaseObject): Object to remove
"""
# Remove from the appropriate registry if registered.
# Sometimes we don't register objects to the object registry during import_object (e.g. particle templates)
if self.object_registry.object_is_registered(obj):
self.object_registry.remove(obj)
# Remove from omni stage
obj.remove()
def reset(self):
"""
Resets this scene
"""
# Make sure the simulator is playing
assert og.sim.is_playing(), "Simulator must be playing in order to reset the scene!"
# Reset the states of all objects (including robots), including (non-)kinematic states and internal variables.
assert self._initial_state is not None
self.load_state(self._initial_state)
og.sim.step_physics()
@property
def n_floors(self):
"""
Returns:
int: Number of floors in this scene
"""
# Default is a single floor
return 1
@property
def n_objects(self):
"""
Returns:
int: number of objects
"""
return len(self.objects)
@property
def fixed_objects(self):
"""
Returns:
dict: Keyword-mapped objects that are fixed in the scene, IGNORING any robots.
Maps object name to their object class instances (DatasetObject)
"""
return {obj.name: obj for obj in self.object_registry("fixed_base", True, default_val=[]) if obj.category != robot_macros.ROBOT_CATEGORY}
def get_random_floor(self):
"""
Sample a random floor among all existing floor_heights in the scene.
Most scenes in OmniGibson only have a single floor.
Returns:
int: an integer between 0 and self.n_floors-1
"""
return np.random.randint(0, self.n_floors)
def get_random_point(self, floor=None, reference_point=None, robot=None):
"""
Sample a random point on the given floor number. If not given, sample a random floor number.
If @reference_point is given, sample a point in the same connected component as the previous point.
Args:
floor (None or int): floor number. None means the floor is randomly sampled
Warning: if @reference_point is given, @floor must be given;
otherwise, this would lead to undefined behavior
reference_point (3-array): (x,y,z) if given, sample a point in the same connected component as this point
Returns:
2-tuple:
- int: floor number. This is the sampled floor number if @floor is None
- 3-array: (x,y,z) randomly sampled point
"""
raise NotImplementedError()
def get_shortest_path(self, floor, source_world, target_world, entire_path=False, robot=None):
"""
Get the shortest path from one point to another point.
Args:
floor (int): floor number
source_world (2-array): (x,y) 2D source location in world reference frame (metric)
target_world (2-array): (x,y) 2D target location in world reference frame (metric)
entire_path (bool): whether to return the entire path
robot (None or BaseRobot): if given, erode the traversability map to account for the robot's size
Returns:
2-tuple:
- (N, 2) array: array of path waypoints, where N is the number of generated waypoints
- float: geodesic distance of the path
"""
raise NotImplementedError()
def get_floor_height(self, floor=0):
"""
Get the height of the given floor. Default is 0.0, since we only have a single floor
Args:
floor: an integer identifying the floor
Returns:
int: height of the given floor
"""
return 0.0
def add_ground_plane(
self,
size=None,
z_position: float = 0,
name="ground_plane",
prim_path: str = "/World/groundPlane",
static_friction: float = 0.5,
dynamic_friction: float = 0.5,
restitution: float = 0.8,
color=None,
visible=True,
):
"""
Generate a ground plane into the simulator
Args:
size (None or float): If specified, sets the (x,y) size of the generated plane
z_position (float): Z position of the generated plane
name (str): Name to assign to the generated plane
prim_path (str): Prim path for the generated plane
static_friction (float): Static friction of the generated plane
dynamic_friction (float): Dynamics friction of the generated plane
restitution (float): Restitution of the generated plane
color (None or 3-array): If specified, sets the (R,G,B) color of the generated plane
visible (bool): Whether the plane should be visible or not
"""
plane = lazy.omni.isaac.core.objects.ground_plane.GroundPlane(
prim_path=prim_path,
name=name,
z_position=z_position,
size=size,
color=None if color is None else np.array(color),
visible=visible,
# TODO: update with new PhysicsMaterial API
# static_friction=static_friction,
# dynamic_friction=dynamic_friction,
# restitution=restitution,
)
self._floor_plane = XFormPrim(
prim_path=plane.prim_path,
name=plane.name,
)
# Assign floors category to the floor plane
lazy.omni.isaac.core.utils.semantics.add_update_semantics(
prim=self._floor_plane.prim,
semantic_label="floors",
type_label="class",
)
def update_initial_state(self, state=None):
"""
Updates the initial state for this scene (which the scene will get reset to upon calling reset())
Args:
state (None or dict): If specified, the state to set internally. Otherwise, will set the initial state to
be the current state
"""
self._initial_state = self.dump_state(serialized=False) if state is None else state
def update_objects_info(self):
"""
Updates the scene-relevant information and saves it to the active USD. Useful for reloading a scene directly
from a saved USD in this format.
"""
# Save relevant information
# Iterate over all objects and save their init info
init_info = {obj.name: obj.get_init_info() for obj in self.object_registry.objects}
# Compose as single dictionary and store internally
self._objects_info = dict(init_info=init_info)
def get_objects_info(self):
"""
Stored information, if any, for this scene. Structure is:
"init_info":
"<obj0>": <obj0> init kw/args
...
"<robot0>": <robot0> init kw/args
...
Returns:
None or dict: If it exists, nested dictionary of relevant objects' information
"""
return self._objects_info
@property
def state_size(self):
# Total state size is the state size of our registry
return self._registry.state_size
def _dump_state(self):
# Default state for the scene is from the registry alone
return self._registry.dump_state(serialized=False)
def _load_state(self, state):
# Default state for the scene is from the registry alone
self._registry.load_state(state=state, serialized=False)
def _serialize(self, state):
# Default state for the scene is from the registry alone
return self._registry.serialize(state=state)
def _deserialize(self, state):
# Default state for the scene is from the registry alone
# We split this into two explicit steps, because the actual registry state size might dynamically change
# as we're deserializing
state_dict = self._registry.deserialize(state=state)
return state_dict, self._registry.state_size
@classproperty
def _cls_registry(cls):
# Global registry
global REGISTERED_SCENES
return REGISTERED_SCENES
@classmethod
def modify_init_info_for_restoring(cls, init_info):
"""
Helper function to modify a given init info for restoring a scene from corresponding scene info.
Note that this function modifies IN-PLACE!
Args:
init_info (dict): Information for this scene from @self.get_init_info()
"""
# Default is pass
pass
| 26,770 | Python | 36.652602 | 166 | 0.615241 |
StanfordVL/OmniGibson/omnigibson/examples/README.md | ### Code Examples
The following examples illustrate the use of OmniGibson.
If you are interested in just getting started as an end-user, you only need check out `./environments`.
If you are looking for examples of BEHAVIOR, the benchmark of household activities that uses OmniGibson, please check the BEHAVIOR repository at https://github.com/StanfordVL/behavior.
- environments: how to instantiate OmniGibson environments with interactive or static scenes, optionally with a scene selector.
- learning: how to train RL policies for robot navigation using stable baselines 3, and how to save and replay demos of agents for imitation learning.
- objects: how to create, load, and place objects to predefined locations or using a logic sampler (e.g. onTop(A, B)), how to change texture as a function of the temperature, and how to generate the minimum volume bounding boxes of objects.
- object_states: how to change various objects states, including dusty, stained, (water sources) toggled on, (cleaning tool) soaked, sliced, and temprature, and how to save and reload object states.
- observations: how to generate different observation modalities such as RGB, depth, LiDAR, segmentation, etc.
- renderer: how to use the renderer directly, without the physics engine.
- robots: how to (keyboard) control robots with differential drive controllers, IK controllers and sampling-based motion planners.
- ros: how to run ROS with OmniGibson as if it is the real world.
- scenes: how to load interactive and non-interactive scenes, how to use domain randomization (of object models and/or texture), and how to create a tour video of the scenes.
- vr: how to use OmniGibson with VR.
- web_ui: how to start a web server that hosts OmniGibson environments.
| 1,753 | Markdown | 86.699996 | 240 | 0.788933 |
StanfordVL/OmniGibson/omnigibson/examples/scenes/scene_selector.py | import omnigibson as og
from omnigibson.macros import gm
from omnigibson.utils.asset_utils import get_available_g_scenes, get_available_og_scenes
from omnigibson.utils.ui_utils import choose_from_options
# Configure macros for maximum performance
gm.USE_GPU_DYNAMICS = True
gm.ENABLE_FLATCACHE = True
gm.ENABLE_OBJECT_STATES = False
gm.ENABLE_TRANSITION_RULES = False
def main(random_selection=False, headless=False, short_exec=False):
"""
Prompts the user to select any available interactive scene and loads a turtlebot into it.
It steps the environment 100 times with random actions sampled from the action space,
using the Gym interface, resetting it 10 times.
"""
og.log.info(f"Demo {__file__}\n " + "*" * 80 + "\n Description:\n" + main.__doc__ + "*" * 80)
# Choose the scene type to load
scene_options = {
"InteractiveTraversableScene": "Procedurally generated scene with fully interactive objects",
# "StaticTraversableScene": "Monolithic scene mesh with no interactive objects",
}
scene_type = choose_from_options(options=scene_options, name="scene type", random_selection=random_selection)
# Choose the scene model to load
scenes = get_available_og_scenes() if scene_type == "InteractiveTraversableScene" else get_available_g_scenes()
scene_model = choose_from_options(options=scenes, name="scene model", random_selection=random_selection)
cfg = {
"scene": {
"type": scene_type,
"scene_model": scene_model,
},
"robots": [
{
"type": "Turtlebot",
"obs_modalities": ["scan", "rgb", "depth"],
"action_type": "continuous",
"action_normalize": True,
},
],
}
# If the scene type is interactive, also check if we want to quick load or full load the scene
if scene_type == "InteractiveTraversableScene":
load_options = {
"Quick": "Only load the building assets (i.e.: the floors, walls, ceilings)",
"Full": "Load all interactive objects in the scene",
}
load_mode = choose_from_options(options=load_options, name="load mode", random_selection=random_selection)
if load_mode == "Quick":
cfg["scene"]["load_object_categories"] = ["floors", "walls", "ceilings"]
# Load the environment
env = og.Environment(configs=cfg)
# Allow user to move camera more easily
if not gm.HEADLESS:
og.sim.enable_viewer_camera_teleoperation()
# Run a simple loop and reset periodically
max_iterations = 10 if not short_exec else 1
for j in range(max_iterations):
og.log.info("Resetting environment")
env.reset()
for i in range(100):
action = env.action_space.sample()
state, reward, done, info = env.step(action)
if done:
og.log.info("Episode finished after {} timesteps".format(i + 1))
break
# Always close the environment at the end
env.close()
if __name__ == "__main__":
main()
| 3,113 | Python | 36.975609 | 115 | 0.633151 |
StanfordVL/OmniGibson/omnigibson/examples/scenes/scene_tour_demo.py | import numpy as np
import omnigibson as og
import omnigibson.lazy as lazy
from omnigibson.macros import gm
from omnigibson.utils.asset_utils import get_available_g_scenes, get_available_og_scenes
from omnigibson.utils.ui_utils import choose_from_options, KeyboardEventHandler
def main(random_selection=False, headless=False, short_exec=False):
"""
Prompts the user to select any available interactive scene and loads it.
It sets the camera to various poses and records images, and then generates a trajectory from a set of waypoints
and records the resulting video.
"""
og.log.info(f"Demo {__file__}\n " + "*" * 80 + "\n Description:\n" + main.__doc__ + "*" * 80)
# Make sure the example is not being run headless. If so, terminate early
if gm.HEADLESS:
print("This demo should only be run not headless! Exiting early.")
og.shutdown()
# Choose the scene type to load
scene_options = {
"InteractiveTraversableScene": "Procedurally generated scene with fully interactive objects",
# "StaticTraversableScene": "Monolithic scene mesh with no interactive objects",
}
scene_type = choose_from_options(options=scene_options, name="scene type", random_selection=random_selection)
# Choose the scene model to load
scenes = get_available_og_scenes() if scene_type == "InteractiveTraversableScene" else get_available_g_scenes()
scene_model = choose_from_options(options=scenes, name="scene model", random_selection=random_selection)
print(f"scene model: {scene_model}")
cfg = {
"scene": {
"type": scene_type,
"scene_model": scene_model,
},
}
# If the scene type is interactive, also check if we want to quick load or full load the scene
if scene_type == "InteractiveTraversableScene":
load_options = {
"Quick": "Only load the building assets (i.e.: the floors, walls, ceilings)",
"Full": "Load all interactive objects in the scene",
}
load_mode = choose_from_options(options=load_options, name="load mode", random_selection=random_selection)
if load_mode == "Quick":
cfg["scene"]["load_object_categories"] = ["floors", "walls", "ceilings"]
# Load the environment
env = og.Environment(configs=cfg)
# Allow user to teleoperate the camera
cam_mover = og.sim.enable_viewer_camera_teleoperation()
# Create a keyboard event handler for generating waypoints
waypoints = []
def add_waypoint():
nonlocal waypoints
pos = cam_mover.cam.get_position()
print(f"Added waypoint at {pos}")
waypoints.append(pos)
def clear_waypoints():
nonlocal waypoints
print(f"Cleared all waypoints!")
waypoints = []
KeyboardEventHandler.initialize()
KeyboardEventHandler.add_keyboard_callback(
key=lazy.carb.input.KeyboardInput.X,
callback_fn=add_waypoint,
)
KeyboardEventHandler.add_keyboard_callback(
key=lazy.carb.input.KeyboardInput.C,
callback_fn=clear_waypoints,
)
KeyboardEventHandler.add_keyboard_callback(
key=lazy.carb.input.KeyboardInput.J,
callback_fn=lambda: cam_mover.record_trajectory_from_waypoints(
waypoints=np.array(waypoints),
per_step_distance=0.02,
fps=30,
steps_per_frame=1,
fpath=None, # This corresponds to the default path inferred from cam_mover.save_dir
),
)
KeyboardEventHandler.add_keyboard_callback(
key=lazy.carb.input.KeyboardInput.ESCAPE,
callback_fn=lambda: env.close(),
)
# Print out additional keyboard commands
print(f"\t X: Save the current camera pose as a waypoint")
print(f"\t C: Clear all waypoints")
print(f"\t J: Record the camera trajectory from the current set of waypoints")
print(f"\t ESC: Terminate the demo")
# Loop indefinitely
while True:
env.step([])
if __name__ == "__main__":
main()
| 4,049 | Python | 36.5 | 115 | 0.659669 |
StanfordVL/OmniGibson/omnigibson/examples/scenes/traversability_map_example.py | import os
import cv2
import matplotlib.pyplot as plt
import numpy as np
from PIL import Image
import omnigibson as og
from omnigibson.utils.asset_utils import get_og_scene_path, get_available_og_scenes
from omnigibson.utils.ui_utils import choose_from_options
def main(random_selection=False, headless=False, short_exec=False):
"""
Traversable map demo
Loads the floor plan and obstacles for the requested scene, and overlays them in a visual figure such that the
highlighted area reflects the traversable (free-space) area
"""
og.log.info(f"Demo {__file__}\n " + "*" * 80 + "\n Description:\n" + main.__doc__ + "*" * 80)
scenes = get_available_og_scenes()
scene_model = choose_from_options(options=scenes, name="scene model", random_selection=random_selection)
print(f"Generating traversability map for scene {scene_model}")
trav_map_size = 200
trav_map_erosion = 2
trav_map = Image.open(os.path.join(get_og_scene_path(scene_model), "layout", "floor_trav_0.png"))
trav_map = np.array(trav_map.resize((trav_map_size, trav_map_size)))
trav_map = cv2.erode(trav_map, np.ones((trav_map_erosion, trav_map_erosion)))
if not headless:
plt.figure(figsize=(12, 12))
plt.imshow(trav_map)
plt.title(f"Traversable area of {scene_model} scene")
if not headless:
plt.show()
# Shut down omnigibson at the end
og.shutdown()
if __name__ == "__main__":
main()
| 1,469 | Python | 30.956521 | 114 | 0.676651 |
StanfordVL/OmniGibson/omnigibson/examples/learning/navigation_policy_demo.py | """
Example training code using stable-baselines3 PPO for one BEHAVIOR activity.
Note that due to the sparsity of the reward, this training code will not converge and achieve task success.
This only serves as a starting point that users can further build upon.
"""
import argparse
import os, time, cv2
import yaml
import omnigibson as og
from omnigibson import example_config_path
from omnigibson.macros import gm
from omnigibson.utils.python_utils import meets_minimum_version
try:
import gym
import torch as th
import torch.nn as nn
import tensorboard
from stable_baselines3 import PPO
from stable_baselines3.common.evaluation import evaluate_policy
from stable_baselines3.common.preprocessing import maybe_transpose
from stable_baselines3.common.torch_layers import BaseFeaturesExtractor
from stable_baselines3.common.utils import set_random_seed
from stable_baselines3.common.callbacks import CallbackList, CheckpointCallback, EvalCallback
except ModuleNotFoundError:
og.log.error("torch, stable-baselines3, or tensorboard is not installed. "
"See which packages are missing, and then run the following for any missing packages:\n"
"pip install stable-baselines3[extra]\n"
"pip install tensorboard\n"
"pip install shimmy>=0.2.1\n"
"Also, please update gym to >=0.26.1 after installing sb3: pip install gym>=0.26.1")
exit(1)
assert meets_minimum_version(gym.__version__, "0.26.1"), "Please install/update gym to version >= 0.26.1"
# We don't need object states nor transitions rules, so we disable them now, and also enable flatcache for maximum speed
gm.ENABLE_OBJECT_STATES = False
gm.ENABLE_TRANSITION_RULES = False
gm.ENABLE_FLATCACHE = True
class CustomCombinedExtractor(BaseFeaturesExtractor):
def __init__(self, observation_space: gym.spaces.Dict):
# We do not know features-dim here before going over all the items,
# so put something dummy for now. PyTorch requires calling
super().__init__(observation_space, features_dim=1)
extractors = {}
self.step_index = 0
self.img_save_dir = 'img_save_dir'
os.makedirs(self.img_save_dir, exist_ok=True)
total_concat_size = 0
feature_size = 128
for key, subspace in observation_space.spaces.items():
# For now, only keep RGB observations
if "rgb" in key:
og.log.info(f"obs {key} shape: {subspace.shape}")
n_input_channels = subspace.shape[0] # channel first
cnn = nn.Sequential(
nn.Conv2d(n_input_channels, 4, kernel_size=8, stride=4, padding=0),
nn.ReLU(),
nn.MaxPool2d(2),
nn.Conv2d(4, 8, kernel_size=4, stride=2, padding=0),
nn.ReLU(),
nn.MaxPool2d(2),
nn.Conv2d(8, 4, kernel_size=3, stride=1, padding=0),
nn.ReLU(),
nn.Flatten(),
)
test_tensor = th.zeros(subspace.shape)
with th.no_grad():
n_flatten = cnn(test_tensor[None]).shape[1]
fc = nn.Sequential(nn.Linear(n_flatten, feature_size), nn.ReLU())
extractors[key] = nn.Sequential(cnn, fc)
total_concat_size += feature_size
self.extractors = nn.ModuleDict(extractors)
# Update the features dim manually
self._features_dim = total_concat_size
def forward(self, observations) -> th.Tensor:
encoded_tensor_list = []
self.step_index += 1
# self.extractors contain nn.Modules that do all the processing.
for key, extractor in self.extractors.items():
encoded_tensor_list.append(extractor(observations[key]))
feature = th.cat(encoded_tensor_list, dim=1)
return feature
def main():
# Parse args
parser = argparse.ArgumentParser(description="Train or evaluate a PPO agent in BEHAVIOR")
parser.add_argument(
"--checkpoint",
type=str,
default=None,
help="Absolute path to desired PPO checkpoint to load for evaluation",
)
parser.add_argument(
"--eval",
action="store_true",
help="If set, will evaluate the PPO agent found from --checkpoint",
)
args = parser.parse_args()
tensorboard_log_dir = os.path.join("log_dir", time.strftime("%Y%m%d-%H%M%S"))
os.makedirs(tensorboard_log_dir, exist_ok=True)
prefix = ''
seed = 0
# Load config
with open(f"{example_config_path}/turtlebot_nav.yaml", "r") as f:
cfg = yaml.load(f, Loader=yaml.FullLoader)
# Make sure flattened obs and action space is used
cfg["env"]["flatten_action_space"] = True
cfg["env"]["flatten_obs_space"] = True
# Only use RGB obs
cfg["robots"][0]["obs_modalities"] = ["rgb"]
# If we're not eval, turn off the start / goal markers so the agent doesn't see them
if not args.eval:
cfg["task"]["visualize_goal"] = False
env = og.Environment(configs=cfg)
# If we're evaluating, hide the ceilings and enable camera teleoperation so the user can easily
# visualize the rollouts dynamically
if args.eval:
ceiling = env.scene.object_registry("name", "ceilings")
ceiling.visible = False
og.sim.enable_viewer_camera_teleoperation()
# Set the set
set_random_seed(seed)
env.reset()
policy_kwargs = dict(
features_extractor_class=CustomCombinedExtractor,
)
os.makedirs(tensorboard_log_dir, exist_ok=True)
if args.eval:
assert args.checkpoint is not None, "If evaluating a PPO policy, @checkpoint argument must be specified!"
model = PPO.load(args.checkpoint)
og.log.info("Starting evaluation...")
mean_reward, std_reward = evaluate_policy(model, env, n_eval_episodes=50)
og.log.info("Finished evaluation!")
og.log.info(f"Mean reward: {mean_reward} +/- {std_reward:.2f}")
else:
model = PPO(
"MultiInputPolicy",
env,
verbose=1,
tensorboard_log=tensorboard_log_dir,
policy_kwargs=policy_kwargs,
n_steps=20 * 10,
batch_size=8,
device='cuda',
)
checkpoint_callback = CheckpointCallback(save_freq=1000, save_path=tensorboard_log_dir, name_prefix=prefix)
eval_callback = EvalCallback(eval_env=env, eval_freq=1000, n_eval_episodes=20)
callback = CallbackList([checkpoint_callback, eval_callback])
og.log.debug(model.policy)
og.log.info(f"model: {model}")
og.log.info("Starting training...")
model.learn(
total_timesteps=10000000,
callback=callback,
)
og.log.info("Finished training!")
if __name__ == "__main__":
main()
| 6,953 | Python | 35.989362 | 120 | 0.627355 |
StanfordVL/OmniGibson/omnigibson/examples/simulator/sim_save_load_example.py | import os
import numpy as np
import omnigibson as og
import omnigibson.lazy as lazy
from omnigibson.utils.ui_utils import KeyboardEventHandler
TEST_OUT_PATH = "" # Define output directory here.
def main(random_selection=False, headless=False, short_exec=False):
"""
Prompts the user to select whether they are saving or loading an environment, and interactively
shows how an environment can be saved or restored.
"""
og.log.info(f"Demo {__file__}\n " + "*" * 80 + "\n Description:\n" + main.__doc__ + "*" * 80)
cfg = {
"scene": {
"type": "InteractiveTraversableScene",
"scene_model": "Rs_int",
"load_object_categories": ["floors", "walls", "bed", "bottom_cabinet", "chair"],
},
"robots": [
{
"type": "Turtlebot",
"obs_modalities": ["rgb", "depth"],
},
],
}
# Create the environment
env = og.Environment(configs=cfg)
# Set the camera to a good angle
def set_camera_pose():
og.sim.viewer_camera.set_position_orientation(
position=np.array([-0.229375, -3.40576 , 7.26143 ]),
orientation=np.array([ 0.27619733, -0.00230233, -0.00801152, 0.9610648 ]),
)
set_camera_pose()
# Give user instructions, and then loop until completed
completed = short_exec
if not short_exec and not random_selection:
# Notify user to manipulate environment until ready, then press Z to exit
print()
print("Modify the scene by SHIFT + left clicking objects and dragging them. Once finished, press Z.")
# Register callback so user knows to press space once they're done manipulating the scene
def complete_loop():
nonlocal completed
completed = True
KeyboardEventHandler.add_keyboard_callback(lazy.carb.input.KeyboardInput.Z, complete_loop)
while not completed:
env.step(np.random.uniform(-1, 1, env.robots[0].action_dim))
print("Completed scene modification, saving scene...")
save_path = os.path.join(TEST_OUT_PATH, "saved_stage.json")
og.sim.save(json_path=save_path)
print("Re-loading scene...")
og.sim.restore(json_path=save_path)
# Take a sim step and play
og.sim.step()
og.sim.play()
set_camera_pose()
# Loop until user terminates
completed = short_exec
if not short_exec and not random_selection:
# Notify user to manipulate environment until ready, then press Z to exit
print()
print("View reloaded scene. Once finished, press Z.")
# Register callback so user knows to press space once they're done manipulating the scene
KeyboardEventHandler.add_keyboard_callback(lazy.carb.input.KeyboardInput.Z, complete_loop)
while not completed:
env.step(np.zeros(env.robots[0].action_dim))
# Shutdown omnigibson at the end
og.shutdown()
if __name__ == "__main__":
main()
| 2,983 | Python | 34.105882 | 109 | 0.630238 |
StanfordVL/OmniGibson/omnigibson/examples/teleoperation/robot_teleoperate_demo.py | """
Example script for using external devices to teleoperate a robot.
"""
import omnigibson as og
from omnigibson.utils.ui_utils import choose_from_options
ROBOTS = {
"FrankaPanda": "Franka Emika Panda (default)",
"Fetch": "Mobile robot with one arm",
"Tiago": "Mobile robot with two arms",
}
TELEOP_METHOD = {
"keyboard": "Keyboard (default)",
"spacemouse": "SpaceMouse",
"oculus": "Oculus Quest",
"vision": "Human Keypoints with Camera",
}
def main():
"""
Spawn a robot in an empty scene with a breakfast table and some toys.
Users can try pick and place the toy into the basket using selected external devices and robot of their choice.
"""
from omnigibson.utils.teleop_utils import TeleopSystem
from telemoma.utils.camera_utils import RealSenseCamera
from telemoma.configs.base_config import teleop_config
robot_name = choose_from_options(options=ROBOTS, name="robot")
arm_teleop_method = choose_from_options(options=TELEOP_METHOD, name="robot arm teleop method")
if robot_name != "FrankaPanda":
base_teleop_method = choose_from_options(options=TELEOP_METHOD, name="robot base teleop method")
else:
base_teleop_method = "keyboard" # Dummy value since FrankaPanda does not have a base
# Generate teleop config
teleop_config.arm_left_controller = arm_teleop_method
teleop_config.arm_right_controller = arm_teleop_method
teleop_config.base_controller = base_teleop_method
teleop_config.interface_kwargs["keyboard"] = {"arm_speed_scaledown": 0.04}
teleop_config.interface_kwargs["spacemouse"] = {"arm_speed_scaledown": 0.04}
if arm_teleop_method == "vision" or base_teleop_method == "vision":
teleop_config.interface_kwargs["vision"] = {"camera": RealSenseCamera()}
# Create the config for generating the environment we want
scene_cfg = {"type": "Scene"}
# Add the robot we want to load
robot_cfg = {
"type": robot_name,
"obs_modalities": ["rgb"],
"action_normalize": False,
"grasping_mode": "assisted",
}
arms = ["left", "right"] if robot_name == "Tiago" else ["0"]
robot_cfg["controller_config"] = {}
for arm in arms:
robot_cfg["controller_config"][f"arm_{arm}"] = {
"name": "InverseKinematicsController",
"command_input_limits": None,
}
robot_cfg["controller_config"][f"gripper_{arm}"] = {
"name": "MultiFingerGripperController",
"command_input_limits": (0.0, 1.0),
"mode": "smooth",
}
object_cfg = [
{
"type": "DatasetObject",
"prim_path": "/World/breakfast_table",
"name": "breakfast_table",
"category": "breakfast_table",
"model": "kwmfdg",
"bounding_box": [2, 1, 0.4],
"position": [0.8, 0, 0.3],
"orientation": [0, 0, 0.707, 0.707],
},
{
"type": "DatasetObject",
"prim_path": "/World/frail",
"name": "frail",
"category": "frail",
"model": "zmjovr",
"scale": [2, 2, 2],
"position": [0.6, -0.35, 0.5],
},
{
"type": "DatasetObject",
"prim_path": "/World/toy_figure1",
"name": "toy_figure1",
"category": "toy_figure",
"model": "issvzv",
"scale": [0.75, 0.75, 0.75],
"position": [0.6, 0, 0.5],
},
{
"type": "DatasetObject",
"prim_path": "/World/toy_figure2",
"name": "toy_figure2",
"category": "toy_figure",
"model": "nncqfn",
"scale": [0.75, 0.75, 0.75],
"position": [0.6, 0.15, 0.5],
},
{
"type": "DatasetObject",
"prim_path": "/World/toy_figure3",
"name": "toy_figure3",
"category": "toy_figure",
"model": "eulekw",
"scale": [0.25, 0.25, 0.25],
"position": [0.6, 0.3, 0.5],
}
]
cfg = dict(scene=scene_cfg, robots=[robot_cfg], objects=object_cfg)
# Create the environment
env = og.Environment(configs=cfg)
env.reset()
# update viewer camera pose
og.sim.viewer_camera.set_position_orientation([-0.22, 0.99, 1.09], [-0.14, 0.47, 0.84, -0.23])
# Start teleoperation system
robot = env.robots[0]
# Initialize teleoperation system
teleop_sys = TeleopSystem(config=teleop_config, robot=robot, show_control_marker=True)
teleop_sys.start()
# main simulation loop
for _ in range(10000):
action = teleop_sys.get_action(teleop_sys.get_obs())
env.step(action)
# Shut down the environment cleanly at the end
teleop_sys.stop()
env.close()
if __name__ == "__main__":
main() | 4,868 | Python | 34.80147 | 115 | 0.566763 |
StanfordVL/OmniGibson/omnigibson/examples/teleoperation/vr_simple_demo.py | """
Example script for interacting with OmniGibson scenes with VR and BehaviorRobot.
"""
import omnigibson as og
from omnigibson.utils.teleop_utils import OVXRSystem
def main():
"""
Spawn a BehaviorRobot in Rs_int and users can navigate around and interact with the scene using VR.
"""
# Create the config for generating the environment we want
scene_cfg = {"type": "Scene"} #"InteractiveTraversableScene", "scene_model": "Rs_int"}
robot0_cfg = {
"type": "Tiago",
"controller_config": {
"gripper_left": {"command_input_limits": "default"},
"gripper_right": {"command_input_limits": "default"},
}
}
cfg = dict(scene=scene_cfg, robots=[robot0_cfg])
# Create the environment
env = og.Environment(configs=cfg)
env.reset()
# start vrsys
vrsys = OVXRSystem(robot=env.robots[0], show_control_marker=False, system="SteamVR", align_anchor_to_robot_base=True)
vrsys.start()
# set headset position to be 1m above ground and facing +x
vrsys.set_initial_transform(pos=[0, 0, 1], orn=[0, 0, 0, 1])
# main simulation loop
for _ in range(10000):
# step the VR system to get the latest data from VR runtime
vrsys.update()
# generate robot action and step the environment
action = vrsys.teleop_data_to_action()
env.step(action)
# Shut down the environment cleanly at the end
vrsys.stop()
env.close()
if __name__ == "__main__":
main() | 1,512 | Python | 33.386363 | 121 | 0.634259 |
StanfordVL/OmniGibson/omnigibson/examples/action_primitives/rs_int_example.py | import os
import yaml
import numpy as np
import omnigibson as og
from omnigibson.macros import gm
from omnigibson.action_primitives.starter_semantic_action_primitives import StarterSemanticActionPrimitives, StarterSemanticActionPrimitiveSet
# Don't use GPU dynamics and use flatcache for performance boost
# gm.USE_GPU_DYNAMICS = True
# gm.ENABLE_FLATCACHE = True
def execute_controller(ctrl_gen, env):
for action in ctrl_gen:
env.step(action)
def main():
"""
Demonstrates how to use the action primitives to pick and place an object in a crowded scene.
It loads Rs_int with a Fetch robot, and the robot picks and places an apple.
"""
# Load the config
config_filename = os.path.join(og.example_config_path, "fetch_primitives.yaml")
config = yaml.load(open(config_filename, "r"), Loader=yaml.FullLoader)
# Update it to run a grocery shopping task
config["scene"]["scene_model"] = "Rs_int"
config["scene"]["not_load_object_categories"] = ["ceilings"]
config["objects"] = [
{
"type": "DatasetObject",
"name": "apple",
"category": "apple",
"model": "agveuv",
"position": [-0.3, -1.1, 0.5],
"orientation": [0, 0, 0, 1]
},
]
# Load the environment
env = og.Environment(configs=config)
scene = env.scene
robot = env.robots[0]
# Allow user to move camera more easily
og.sim.enable_viewer_camera_teleoperation()
controller = StarterSemanticActionPrimitives(env, enable_head_tracking=False)
cabinet = scene.object_registry("name", "bottom_cabinet_slgzfc_0")
apple = scene.object_registry("name", "apple")
# Grasp apple
print("Executing controller")
execute_controller(controller.apply_ref(StarterSemanticActionPrimitiveSet.GRASP, apple), env)
print("Finished executing grasp")
# Place on cabinet
print("Executing controller")
execute_controller(controller.apply_ref(StarterSemanticActionPrimitiveSet.PLACE_ON_TOP, cabinet), env)
print("Finished executing place")
if __name__ == "__main__":
main() | 2,119 | Python | 32.124999 | 142 | 0.674847 |
StanfordVL/OmniGibson/omnigibson/examples/action_primitives/solve_simple_task.py | import os
import yaml
import numpy as np
import omnigibson as og
from omnigibson.macros import gm
from omnigibson.action_primitives.starter_semantic_action_primitives import StarterSemanticActionPrimitives, StarterSemanticActionPrimitiveSet
# Don't use GPU dynamics and use flatcache for performance boost
# gm.USE_GPU_DYNAMICS = True
# gm.ENABLE_FLATCACHE = True
def execute_controller(ctrl_gen, env):
for action in ctrl_gen:
env.step(action)
def main():
"""
Demonstrates how to use the action primitives to pick and place an object in an empty scene.
It loads Rs_int with a Fetch robot, and the robot picks and places a bottle of cologne.
"""
# Load the config
config_filename = os.path.join(og.example_config_path, "fetch_primitives.yaml")
config = yaml.load(open(config_filename, "r"), Loader=yaml.FullLoader)
# Update it to create a custom environment and run some actions
config["scene"]["scene_model"] = "Rs_int"
config["scene"]["load_object_categories"] = ["floors", "ceilings", "walls", "coffee_table"]
config["objects"] = [
{
"type": "DatasetObject",
"name": "cologne",
"category": "bottle_of_cologne",
"model": "lyipur",
"position": [-0.3, -0.8, 0.5],
"orientation": [0, 0, 0, 1]
},
{
"type": "DatasetObject",
"name": "table",
"category": "breakfast_table",
"model": "rjgmmy",
"scale": [0.3, 0.3, 0.3],
"position": [-0.7, 0.5, 0.2],
"orientation": [0, 0, 0, 1]
}
]
# Load the environment
env = og.Environment(configs=config)
scene = env.scene
robot = env.robots[0]
# Allow user to move camera more easily
og.sim.enable_viewer_camera_teleoperation()
controller = StarterSemanticActionPrimitives(env, enable_head_tracking=False)
# Grasp of cologne
grasp_obj = scene.object_registry("name", "cologne")
print("Executing controller")
execute_controller(controller.apply_ref(StarterSemanticActionPrimitiveSet.GRASP, grasp_obj), env)
print("Finished executing grasp")
# Place cologne on another table
print("Executing controller")
table = scene.object_registry("name", "table")
execute_controller(controller.apply_ref(StarterSemanticActionPrimitiveSet.PLACE_ON_TOP, table), env)
print("Finished executing place")
if __name__ == "__main__":
main() | 2,486 | Python | 33.068493 | 142 | 0.639984 |
StanfordVL/OmniGibson/omnigibson/examples/action_primitives/wip_solve_behavior_task.py | import os
import yaml
import numpy as np
import omnigibson as og
from omnigibson.macros import gm
from omnigibson.action_primitives.starter_semantic_action_primitives import StarterSemanticActionPrimitives, StarterSemanticActionPrimitiveSet
# Don't use GPU dynamics and use flatcache for performance boost
# gm.USE_GPU_DYNAMICS = True
# gm.ENABLE_FLATCACHE = True
def execute_controller(ctrl_gen, env):
for action in ctrl_gen:
env.step(action)
def main():
"""
Demonstrates how to use the action primitives to solve a simple BEHAVIOR-1K task.
It loads Benevolence_1_int with a Fetch robot, and the robot attempts to solve the
picking_up_trash task using a hardcoded sequence of primitives.
"""
# Load the config
config_filename = os.path.join(og.example_config_path, "fetch_primitives.yaml")
config = yaml.load(open(config_filename, "r"), Loader=yaml.FullLoader)
# Update it to run a grocery shopping task
config["scene"]["scene_model"] = "Benevolence_1_int"
config["scene"]["load_task_relevant_only"] = True
config["scene"]["not_load_object_categories"] = ["ceilings"]
config["task"] = {
"type": "BehaviorTask",
"activity_name": "picking_up_trash",
"activity_definition_id": 0,
"activity_instance_id": 0,
"predefined_problem": None,
"online_object_sampling": False,
}
# Load the environment
env = og.Environment(configs=config)
scene = env.scene
robot = env.robots[0]
# Allow user to move camera more easily
og.sim.enable_viewer_camera_teleoperation()
controller = StarterSemanticActionPrimitives(env, enable_head_tracking=False)
# Grasp can of soda
grasp_obj = scene.object_registry("name", "can_of_soda_89")
print("Executing controller")
execute_controller(controller.apply_ref(StarterSemanticActionPrimitiveSet.GRASP, grasp_obj), env)
print("Finished executing grasp")
# Place can in trash can
print("Executing controller")
trash = scene.object_registry("name", "trash_can_85")
execute_controller(controller.apply_ref(StarterSemanticActionPrimitiveSet.PLACE_INSIDE, trash), env)
print("Finished executing place")
if __name__ == "__main__":
main() | 2,253 | Python | 34.218749 | 142 | 0.700843 |
Subsets and Splits
No saved queries yet
Save your SQL queries to embed, download, and access them later. Queries will appear here once saved.