repo_id
stringlengths 15
86
| file_path
stringlengths 28
180
| content
stringlengths 1
1.75M
| __index_level_0__
int64 0
0
|
---|---|---|---|
hf_public_repos/diffusers/tests/pipelines | hf_public_repos/diffusers/tests/pipelines/deepfloyd_if/test_if_superresolution.py | # coding=utf-8
# Copyright 2023 HuggingFace Inc.
#
# Licensed under the Apache License, Version 2.0 (the "License");
# you may not use this file except in compliance with the License.
# You may obtain a copy of the License at
#
# http://www.apache.org/licenses/LICENSE-2.0
#
# Unless required by applicable law or agreed to in writing, software
# distributed under the License is distributed on an "AS IS" BASIS,
# WITHOUT WARRANTIES OR CONDITIONS OF ANY KIND, either express or implied.
# See the License for the specific language governing permissions and
# limitations under the License.
import random
import unittest
import torch
from diffusers import IFSuperResolutionPipeline
from diffusers.utils import floats_tensor
from diffusers.utils.import_utils import is_xformers_available
from diffusers.utils.testing_utils import skip_mps, torch_device
from ..pipeline_params import TEXT_GUIDED_IMAGE_VARIATION_BATCH_PARAMS, TEXT_GUIDED_IMAGE_VARIATION_PARAMS
from ..test_pipelines_common import PipelineTesterMixin
from . import IFPipelineTesterMixin
@skip_mps
class IFSuperResolutionPipelineFastTests(PipelineTesterMixin, IFPipelineTesterMixin, unittest.TestCase):
pipeline_class = IFSuperResolutionPipeline
params = TEXT_GUIDED_IMAGE_VARIATION_PARAMS - {"width", "height"}
batch_params = TEXT_GUIDED_IMAGE_VARIATION_BATCH_PARAMS
required_optional_params = PipelineTesterMixin.required_optional_params - {"latents"}
def get_dummy_components(self):
return self._get_superresolution_dummy_components()
def get_dummy_inputs(self, device, seed=0):
if str(device).startswith("mps"):
generator = torch.manual_seed(seed)
else:
generator = torch.Generator(device=device).manual_seed(seed)
image = floats_tensor((1, 3, 32, 32), rng=random.Random(seed)).to(device)
inputs = {
"prompt": "A painting of a squirrel eating a burger",
"image": image,
"generator": generator,
"num_inference_steps": 2,
"output_type": "numpy",
}
return inputs
@unittest.skipIf(
torch_device != "cuda" or not is_xformers_available(),
reason="XFormers attention is only available with CUDA and `xformers` installed",
)
def test_xformers_attention_forwardGenerator_pass(self):
self._test_xformers_attention_forwardGenerator_pass(expected_max_diff=1e-3)
def test_save_load_optional_components(self):
self._test_save_load_optional_components()
@unittest.skipIf(torch_device != "cuda", reason="float16 requires CUDA")
def test_save_load_float16(self):
# Due to non-determinism in save load of the hf-internal-testing/tiny-random-t5 text encoder
super().test_save_load_float16(expected_max_diff=1e-1)
def test_attention_slicing_forward_pass(self):
self._test_attention_slicing_forward_pass(expected_max_diff=1e-2)
def test_save_load_local(self):
self._test_save_load_local()
def test_inference_batch_single_identical(self):
self._test_inference_batch_single_identical(
expected_max_diff=1e-2,
)
| 0 |
hf_public_repos/diffusers/tests/pipelines | hf_public_repos/diffusers/tests/pipelines/deepfloyd_if/test_if_img2img_superresolution.py | # coding=utf-8
# Copyright 2023 HuggingFace Inc.
#
# Licensed under the Apache License, Version 2.0 (the "License");
# you may not use this file except in compliance with the License.
# You may obtain a copy of the License at
#
# http://www.apache.org/licenses/LICENSE-2.0
#
# Unless required by applicable law or agreed to in writing, software
# distributed under the License is distributed on an "AS IS" BASIS,
# WITHOUT WARRANTIES OR CONDITIONS OF ANY KIND, either express or implied.
# See the License for the specific language governing permissions and
# limitations under the License.
import random
import unittest
import torch
from diffusers import IFImg2ImgSuperResolutionPipeline
from diffusers.utils import floats_tensor
from diffusers.utils.import_utils import is_xformers_available
from diffusers.utils.testing_utils import skip_mps, torch_device
from ..pipeline_params import TEXT_GUIDED_IMAGE_VARIATION_BATCH_PARAMS, TEXT_GUIDED_IMAGE_VARIATION_PARAMS
from ..test_pipelines_common import PipelineTesterMixin
from . import IFPipelineTesterMixin
@skip_mps
class IFImg2ImgSuperResolutionPipelineFastTests(PipelineTesterMixin, IFPipelineTesterMixin, unittest.TestCase):
pipeline_class = IFImg2ImgSuperResolutionPipeline
params = TEXT_GUIDED_IMAGE_VARIATION_PARAMS - {"width", "height"}
batch_params = TEXT_GUIDED_IMAGE_VARIATION_BATCH_PARAMS.union({"original_image"})
required_optional_params = PipelineTesterMixin.required_optional_params - {"latents"}
def get_dummy_components(self):
return self._get_superresolution_dummy_components()
def get_dummy_inputs(self, device, seed=0):
if str(device).startswith("mps"):
generator = torch.manual_seed(seed)
else:
generator = torch.Generator(device=device).manual_seed(seed)
original_image = floats_tensor((1, 3, 32, 32), rng=random.Random(seed)).to(device)
image = floats_tensor((1, 3, 16, 16), rng=random.Random(seed)).to(device)
inputs = {
"prompt": "A painting of a squirrel eating a burger",
"image": image,
"original_image": original_image,
"generator": generator,
"num_inference_steps": 2,
"output_type": "numpy",
}
return inputs
@unittest.skipIf(
torch_device != "cuda" or not is_xformers_available(),
reason="XFormers attention is only available with CUDA and `xformers` installed",
)
def test_xformers_attention_forwardGenerator_pass(self):
self._test_xformers_attention_forwardGenerator_pass(expected_max_diff=1e-3)
def test_save_load_optional_components(self):
self._test_save_load_optional_components()
@unittest.skipIf(torch_device != "cuda", reason="float16 requires CUDA")
def test_save_load_float16(self):
# Due to non-determinism in save load of the hf-internal-testing/tiny-random-t5 text encoder
super().test_save_load_float16(expected_max_diff=1e-1)
def test_attention_slicing_forward_pass(self):
self._test_attention_slicing_forward_pass(expected_max_diff=1e-2)
def test_save_load_local(self):
self._test_save_load_local()
def test_inference_batch_single_identical(self):
self._test_inference_batch_single_identical(
expected_max_diff=1e-2,
)
| 0 |
hf_public_repos/diffusers/tests/pipelines | hf_public_repos/diffusers/tests/pipelines/deepfloyd_if/test_if_img2img.py | # coding=utf-8
# Copyright 2023 HuggingFace Inc.
#
# Licensed under the Apache License, Version 2.0 (the "License");
# you may not use this file except in compliance with the License.
# You may obtain a copy of the License at
#
# http://www.apache.org/licenses/LICENSE-2.0
#
# Unless required by applicable law or agreed to in writing, software
# distributed under the License is distributed on an "AS IS" BASIS,
# WITHOUT WARRANTIES OR CONDITIONS OF ANY KIND, either express or implied.
# See the License for the specific language governing permissions and
# limitations under the License.
import random
import unittest
import torch
from diffusers import IFImg2ImgPipeline
from diffusers.utils import floats_tensor
from diffusers.utils.import_utils import is_xformers_available
from diffusers.utils.testing_utils import skip_mps, torch_device
from ..pipeline_params import (
TEXT_GUIDED_IMAGE_VARIATION_BATCH_PARAMS,
TEXT_GUIDED_IMAGE_VARIATION_PARAMS,
)
from ..test_pipelines_common import PipelineTesterMixin
from . import IFPipelineTesterMixin
@skip_mps
class IFImg2ImgPipelineFastTests(PipelineTesterMixin, IFPipelineTesterMixin, unittest.TestCase):
pipeline_class = IFImg2ImgPipeline
params = TEXT_GUIDED_IMAGE_VARIATION_PARAMS - {"width", "height"}
batch_params = TEXT_GUIDED_IMAGE_VARIATION_BATCH_PARAMS
required_optional_params = PipelineTesterMixin.required_optional_params - {"latents"}
def get_dummy_components(self):
return self._get_dummy_components()
def get_dummy_inputs(self, device, seed=0):
if str(device).startswith("mps"):
generator = torch.manual_seed(seed)
else:
generator = torch.Generator(device=device).manual_seed(seed)
image = floats_tensor((1, 3, 32, 32), rng=random.Random(seed)).to(device)
inputs = {
"prompt": "A painting of a squirrel eating a burger",
"image": image,
"generator": generator,
"num_inference_steps": 2,
"output_type": "numpy",
}
return inputs
def test_save_load_optional_components(self):
self._test_save_load_optional_components()
@unittest.skipIf(
torch_device != "cuda" or not is_xformers_available(),
reason="XFormers attention is only available with CUDA and `xformers` installed",
)
def test_xformers_attention_forwardGenerator_pass(self):
self._test_xformers_attention_forwardGenerator_pass(expected_max_diff=1e-3)
@unittest.skipIf(torch_device != "cuda", reason="float16 requires CUDA")
def test_save_load_float16(self):
# Due to non-determinism in save load of the hf-internal-testing/tiny-random-t5 text encoder
super().test_save_load_float16(expected_max_diff=1e-1)
@unittest.skipIf(torch_device != "cuda", reason="float16 requires CUDA")
def test_float16_inference(self):
super().test_float16_inference(expected_max_diff=1e-1)
def test_attention_slicing_forward_pass(self):
self._test_attention_slicing_forward_pass(expected_max_diff=1e-2)
def test_save_load_local(self):
self._test_save_load_local()
def test_inference_batch_single_identical(self):
self._test_inference_batch_single_identical(
expected_max_diff=1e-2,
)
| 0 |
hf_public_repos/diffusers/tests/pipelines | hf_public_repos/diffusers/tests/pipelines/deepfloyd_if/test_if_inpainting.py | # coding=utf-8
# Copyright 2023 HuggingFace Inc.
#
# Licensed under the Apache License, Version 2.0 (the "License");
# you may not use this file except in compliance with the License.
# You may obtain a copy of the License at
#
# http://www.apache.org/licenses/LICENSE-2.0
#
# Unless required by applicable law or agreed to in writing, software
# distributed under the License is distributed on an "AS IS" BASIS,
# WITHOUT WARRANTIES OR CONDITIONS OF ANY KIND, either express or implied.
# See the License for the specific language governing permissions and
# limitations under the License.
import random
import unittest
import torch
from diffusers import IFInpaintingPipeline
from diffusers.utils import floats_tensor
from diffusers.utils.import_utils import is_xformers_available
from diffusers.utils.testing_utils import skip_mps, torch_device
from ..pipeline_params import (
TEXT_GUIDED_IMAGE_INPAINTING_BATCH_PARAMS,
TEXT_GUIDED_IMAGE_INPAINTING_PARAMS,
)
from ..test_pipelines_common import PipelineTesterMixin
from . import IFPipelineTesterMixin
@skip_mps
class IFInpaintingPipelineFastTests(PipelineTesterMixin, IFPipelineTesterMixin, unittest.TestCase):
pipeline_class = IFInpaintingPipeline
params = TEXT_GUIDED_IMAGE_INPAINTING_PARAMS - {"width", "height"}
batch_params = TEXT_GUIDED_IMAGE_INPAINTING_BATCH_PARAMS
required_optional_params = PipelineTesterMixin.required_optional_params - {"latents"}
def get_dummy_components(self):
return self._get_dummy_components()
def get_dummy_inputs(self, device, seed=0):
if str(device).startswith("mps"):
generator = torch.manual_seed(seed)
else:
generator = torch.Generator(device=device).manual_seed(seed)
image = floats_tensor((1, 3, 32, 32), rng=random.Random(seed)).to(device)
mask_image = floats_tensor((1, 3, 32, 32), rng=random.Random(seed)).to(device)
inputs = {
"prompt": "A painting of a squirrel eating a burger",
"image": image,
"mask_image": mask_image,
"generator": generator,
"num_inference_steps": 2,
"output_type": "numpy",
}
return inputs
@unittest.skipIf(
torch_device != "cuda" or not is_xformers_available(),
reason="XFormers attention is only available with CUDA and `xformers` installed",
)
def test_xformers_attention_forwardGenerator_pass(self):
self._test_xformers_attention_forwardGenerator_pass(expected_max_diff=1e-3)
def test_save_load_optional_components(self):
self._test_save_load_optional_components()
@unittest.skipIf(torch_device != "cuda", reason="float16 requires CUDA")
def test_save_load_float16(self):
# Due to non-determinism in save load of the hf-internal-testing/tiny-random-t5 text encoder
super().test_save_load_float16(expected_max_diff=1e-1)
def test_attention_slicing_forward_pass(self):
self._test_attention_slicing_forward_pass(expected_max_diff=1e-2)
def test_save_load_local(self):
self._test_save_load_local()
def test_inference_batch_single_identical(self):
self._test_inference_batch_single_identical(
expected_max_diff=1e-2,
)
| 0 |
hf_public_repos/diffusers/tests/pipelines | hf_public_repos/diffusers/tests/pipelines/deepfloyd_if/test_if_inpainting_superresolution.py | # coding=utf-8
# Copyright 2023 HuggingFace Inc.
#
# Licensed under the Apache License, Version 2.0 (the "License");
# you may not use this file except in compliance with the License.
# You may obtain a copy of the License at
#
# http://www.apache.org/licenses/LICENSE-2.0
#
# Unless required by applicable law or agreed to in writing, software
# distributed under the License is distributed on an "AS IS" BASIS,
# WITHOUT WARRANTIES OR CONDITIONS OF ANY KIND, either express or implied.
# See the License for the specific language governing permissions and
# limitations under the License.
import random
import unittest
import torch
from diffusers import IFInpaintingSuperResolutionPipeline
from diffusers.utils import floats_tensor
from diffusers.utils.import_utils import is_xformers_available
from diffusers.utils.testing_utils import skip_mps, torch_device
from ..pipeline_params import (
TEXT_GUIDED_IMAGE_INPAINTING_BATCH_PARAMS,
TEXT_GUIDED_IMAGE_INPAINTING_PARAMS,
)
from ..test_pipelines_common import PipelineTesterMixin
from . import IFPipelineTesterMixin
@skip_mps
class IFInpaintingSuperResolutionPipelineFastTests(PipelineTesterMixin, IFPipelineTesterMixin, unittest.TestCase):
pipeline_class = IFInpaintingSuperResolutionPipeline
params = TEXT_GUIDED_IMAGE_INPAINTING_PARAMS - {"width", "height"}
batch_params = TEXT_GUIDED_IMAGE_INPAINTING_BATCH_PARAMS.union({"original_image"})
required_optional_params = PipelineTesterMixin.required_optional_params - {"latents"}
def get_dummy_components(self):
return self._get_superresolution_dummy_components()
def get_dummy_inputs(self, device, seed=0):
if str(device).startswith("mps"):
generator = torch.manual_seed(seed)
else:
generator = torch.Generator(device=device).manual_seed(seed)
image = floats_tensor((1, 3, 16, 16), rng=random.Random(seed)).to(device)
original_image = floats_tensor((1, 3, 32, 32), rng=random.Random(seed)).to(device)
mask_image = floats_tensor((1, 3, 32, 32), rng=random.Random(seed)).to(device)
inputs = {
"prompt": "A painting of a squirrel eating a burger",
"image": image,
"original_image": original_image,
"mask_image": mask_image,
"generator": generator,
"num_inference_steps": 2,
"output_type": "numpy",
}
return inputs
@unittest.skipIf(
torch_device != "cuda" or not is_xformers_available(),
reason="XFormers attention is only available with CUDA and `xformers` installed",
)
def test_xformers_attention_forwardGenerator_pass(self):
self._test_xformers_attention_forwardGenerator_pass(expected_max_diff=1e-3)
def test_save_load_optional_components(self):
self._test_save_load_optional_components()
@unittest.skipIf(torch_device != "cuda", reason="float16 requires CUDA")
def test_save_load_float16(self):
# Due to non-determinism in save load of the hf-internal-testing/tiny-random-t5 text encoder
super().test_save_load_float16(expected_max_diff=1e-1)
def test_attention_slicing_forward_pass(self):
self._test_attention_slicing_forward_pass(expected_max_diff=1e-2)
def test_save_load_local(self):
self._test_save_load_local()
def test_inference_batch_single_identical(self):
self._test_inference_batch_single_identical(
expected_max_diff=1e-2,
)
| 0 |
hf_public_repos/diffusers/tests/pipelines | hf_public_repos/diffusers/tests/pipelines/consistency_models/test_consistency_models.py | import gc
import unittest
import numpy as np
import torch
from torch.backends.cuda import sdp_kernel
from diffusers import (
CMStochasticIterativeScheduler,
ConsistencyModelPipeline,
UNet2DModel,
)
from diffusers.utils import randn_tensor, slow, torch_device
from diffusers.utils.testing_utils import enable_full_determinism, require_torch_2, require_torch_gpu
from ..pipeline_params import UNCONDITIONAL_IMAGE_GENERATION_BATCH_PARAMS, UNCONDITIONAL_IMAGE_GENERATION_PARAMS
from ..test_pipelines_common import PipelineTesterMixin
enable_full_determinism()
class ConsistencyModelPipelineFastTests(PipelineTesterMixin, unittest.TestCase):
pipeline_class = ConsistencyModelPipeline
params = UNCONDITIONAL_IMAGE_GENERATION_PARAMS
batch_params = UNCONDITIONAL_IMAGE_GENERATION_BATCH_PARAMS
# Override required_optional_params to remove num_images_per_prompt
required_optional_params = frozenset(
[
"num_inference_steps",
"generator",
"latents",
"output_type",
"return_dict",
"callback",
"callback_steps",
]
)
@property
def dummy_uncond_unet(self):
unet = UNet2DModel.from_pretrained(
"diffusers/consistency-models-test",
subfolder="test_unet",
)
return unet
@property
def dummy_cond_unet(self):
unet = UNet2DModel.from_pretrained(
"diffusers/consistency-models-test",
subfolder="test_unet_class_cond",
)
return unet
def get_dummy_components(self, class_cond=False):
if class_cond:
unet = self.dummy_cond_unet
else:
unet = self.dummy_uncond_unet
# Default to CM multistep sampler
scheduler = CMStochasticIterativeScheduler(
num_train_timesteps=40,
sigma_min=0.002,
sigma_max=80.0,
)
components = {
"unet": unet,
"scheduler": scheduler,
}
return components
def get_dummy_inputs(self, device, seed=0):
if str(device).startswith("mps"):
generator = torch.manual_seed(seed)
else:
generator = torch.Generator(device=device).manual_seed(seed)
inputs = {
"batch_size": 1,
"num_inference_steps": None,
"timesteps": [22, 0],
"generator": generator,
"output_type": "np",
}
return inputs
def test_consistency_model_pipeline_multistep(self):
device = "cpu" # ensure determinism for the device-dependent torch.Generator
components = self.get_dummy_components()
pipe = ConsistencyModelPipeline(**components)
pipe = pipe.to(device)
pipe.set_progress_bar_config(disable=None)
inputs = self.get_dummy_inputs(device)
image = pipe(**inputs).images
assert image.shape == (1, 32, 32, 3)
image_slice = image[0, -3:, -3:, -1]
expected_slice = np.array([0.3572, 0.6273, 0.4031, 0.3961, 0.4321, 0.5730, 0.5266, 0.4780, 0.5004])
assert np.abs(image_slice.flatten() - expected_slice).max() < 1e-3
def test_consistency_model_pipeline_multistep_class_cond(self):
device = "cpu" # ensure determinism for the device-dependent torch.Generator
components = self.get_dummy_components(class_cond=True)
pipe = ConsistencyModelPipeline(**components)
pipe = pipe.to(device)
pipe.set_progress_bar_config(disable=None)
inputs = self.get_dummy_inputs(device)
inputs["class_labels"] = 0
image = pipe(**inputs).images
assert image.shape == (1, 32, 32, 3)
image_slice = image[0, -3:, -3:, -1]
expected_slice = np.array([0.3572, 0.6273, 0.4031, 0.3961, 0.4321, 0.5730, 0.5266, 0.4780, 0.5004])
assert np.abs(image_slice.flatten() - expected_slice).max() < 1e-3
def test_consistency_model_pipeline_onestep(self):
device = "cpu" # ensure determinism for the device-dependent torch.Generator
components = self.get_dummy_components()
pipe = ConsistencyModelPipeline(**components)
pipe = pipe.to(device)
pipe.set_progress_bar_config(disable=None)
inputs = self.get_dummy_inputs(device)
inputs["num_inference_steps"] = 1
inputs["timesteps"] = None
image = pipe(**inputs).images
assert image.shape == (1, 32, 32, 3)
image_slice = image[0, -3:, -3:, -1]
expected_slice = np.array([0.5004, 0.5004, 0.4994, 0.5008, 0.4976, 0.5018, 0.4990, 0.4982, 0.4987])
assert np.abs(image_slice.flatten() - expected_slice).max() < 1e-3
def test_consistency_model_pipeline_onestep_class_cond(self):
device = "cpu" # ensure determinism for the device-dependent torch.Generator
components = self.get_dummy_components(class_cond=True)
pipe = ConsistencyModelPipeline(**components)
pipe = pipe.to(device)
pipe.set_progress_bar_config(disable=None)
inputs = self.get_dummy_inputs(device)
inputs["num_inference_steps"] = 1
inputs["timesteps"] = None
inputs["class_labels"] = 0
image = pipe(**inputs).images
assert image.shape == (1, 32, 32, 3)
image_slice = image[0, -3:, -3:, -1]
expected_slice = np.array([0.5004, 0.5004, 0.4994, 0.5008, 0.4976, 0.5018, 0.4990, 0.4982, 0.4987])
assert np.abs(image_slice.flatten() - expected_slice).max() < 1e-3
@slow
@require_torch_gpu
class ConsistencyModelPipelineSlowTests(unittest.TestCase):
def tearDown(self):
super().tearDown()
gc.collect()
torch.cuda.empty_cache()
def get_inputs(self, seed=0, get_fixed_latents=False, device="cpu", dtype=torch.float32, shape=(1, 3, 64, 64)):
generator = torch.manual_seed(seed)
inputs = {
"num_inference_steps": None,
"timesteps": [22, 0],
"class_labels": 0,
"generator": generator,
"output_type": "np",
}
if get_fixed_latents:
latents = self.get_fixed_latents(seed=seed, device=device, dtype=dtype, shape=shape)
inputs["latents"] = latents
return inputs
def get_fixed_latents(self, seed=0, device="cpu", dtype=torch.float32, shape=(1, 3, 64, 64)):
if type(device) == str:
device = torch.device(device)
generator = torch.Generator(device=device).manual_seed(seed)
latents = randn_tensor(shape, generator=generator, device=device, dtype=dtype)
return latents
def test_consistency_model_cd_multistep(self):
unet = UNet2DModel.from_pretrained("diffusers/consistency_models", subfolder="diffusers_cd_imagenet64_l2")
scheduler = CMStochasticIterativeScheduler(
num_train_timesteps=40,
sigma_min=0.002,
sigma_max=80.0,
)
pipe = ConsistencyModelPipeline(unet=unet, scheduler=scheduler)
pipe.to(torch_device=torch_device)
pipe.set_progress_bar_config(disable=None)
inputs = self.get_inputs()
image = pipe(**inputs).images
assert image.shape == (1, 64, 64, 3)
image_slice = image[0, -3:, -3:, -1]
expected_slice = np.array([0.0888, 0.0881, 0.0666, 0.0479, 0.0292, 0.0195, 0.0201, 0.0163, 0.0254])
assert np.abs(image_slice.flatten() - expected_slice).max() < 2e-2
def test_consistency_model_cd_onestep(self):
unet = UNet2DModel.from_pretrained("diffusers/consistency_models", subfolder="diffusers_cd_imagenet64_l2")
scheduler = CMStochasticIterativeScheduler(
num_train_timesteps=40,
sigma_min=0.002,
sigma_max=80.0,
)
pipe = ConsistencyModelPipeline(unet=unet, scheduler=scheduler)
pipe.to(torch_device=torch_device)
pipe.set_progress_bar_config(disable=None)
inputs = self.get_inputs()
inputs["num_inference_steps"] = 1
inputs["timesteps"] = None
image = pipe(**inputs).images
assert image.shape == (1, 64, 64, 3)
image_slice = image[0, -3:, -3:, -1]
expected_slice = np.array([0.0340, 0.0152, 0.0063, 0.0267, 0.0221, 0.0107, 0.0416, 0.0186, 0.0217])
assert np.abs(image_slice.flatten() - expected_slice).max() < 2e-2
@require_torch_2
def test_consistency_model_cd_multistep_flash_attn(self):
unet = UNet2DModel.from_pretrained("diffusers/consistency_models", subfolder="diffusers_cd_imagenet64_l2")
scheduler = CMStochasticIterativeScheduler(
num_train_timesteps=40,
sigma_min=0.002,
sigma_max=80.0,
)
pipe = ConsistencyModelPipeline(unet=unet, scheduler=scheduler)
pipe.to(torch_device=torch_device, torch_dtype=torch.float16)
pipe.set_progress_bar_config(disable=None)
inputs = self.get_inputs(get_fixed_latents=True, device=torch_device)
# Ensure usage of flash attention in torch 2.0
with sdp_kernel(enable_flash=True, enable_math=False, enable_mem_efficient=False):
image = pipe(**inputs).images
assert image.shape == (1, 64, 64, 3)
image_slice = image[0, -3:, -3:, -1]
expected_slice = np.array([0.1875, 0.1428, 0.1289, 0.2151, 0.2092, 0.1477, 0.1877, 0.1641, 0.1353])
assert np.abs(image_slice.flatten() - expected_slice).max() < 1e-3
@require_torch_2
def test_consistency_model_cd_onestep_flash_attn(self):
unet = UNet2DModel.from_pretrained("diffusers/consistency_models", subfolder="diffusers_cd_imagenet64_l2")
scheduler = CMStochasticIterativeScheduler(
num_train_timesteps=40,
sigma_min=0.002,
sigma_max=80.0,
)
pipe = ConsistencyModelPipeline(unet=unet, scheduler=scheduler)
pipe.to(torch_device=torch_device, torch_dtype=torch.float16)
pipe.set_progress_bar_config(disable=None)
inputs = self.get_inputs(get_fixed_latents=True, device=torch_device)
inputs["num_inference_steps"] = 1
inputs["timesteps"] = None
# Ensure usage of flash attention in torch 2.0
with sdp_kernel(enable_flash=True, enable_math=False, enable_mem_efficient=False):
image = pipe(**inputs).images
assert image.shape == (1, 64, 64, 3)
image_slice = image[0, -3:, -3:, -1]
expected_slice = np.array([0.1663, 0.1948, 0.2275, 0.1680, 0.1204, 0.1245, 0.1858, 0.1338, 0.2095])
assert np.abs(image_slice.flatten() - expected_slice).max() < 1e-3
| 0 |
hf_public_repos/diffusers/tests/pipelines | hf_public_repos/diffusers/tests/pipelines/ddpm/test_ddpm.py | # coding=utf-8
# Copyright 2023 HuggingFace Inc.
#
# Licensed under the Apache License, Version 2.0 (the "License");
# you may not use this file except in compliance with the License.
# You may obtain a copy of the License at
#
# http://www.apache.org/licenses/LICENSE-2.0
#
# Unless required by applicable law or agreed to in writing, software
# distributed under the License is distributed on an "AS IS" BASIS,
# WITHOUT WARRANTIES OR CONDITIONS OF ANY KIND, either express or implied.
# See the License for the specific language governing permissions and
# limitations under the License.
import unittest
import numpy as np
import torch
from diffusers import DDPMPipeline, DDPMScheduler, UNet2DModel
from diffusers.utils.testing_utils import enable_full_determinism, require_torch_gpu, slow, torch_device
enable_full_determinism()
class DDPMPipelineFastTests(unittest.TestCase):
@property
def dummy_uncond_unet(self):
torch.manual_seed(0)
model = UNet2DModel(
block_out_channels=(32, 64),
layers_per_block=2,
sample_size=32,
in_channels=3,
out_channels=3,
down_block_types=("DownBlock2D", "AttnDownBlock2D"),
up_block_types=("AttnUpBlock2D", "UpBlock2D"),
)
return model
def test_fast_inference(self):
device = "cpu"
unet = self.dummy_uncond_unet
scheduler = DDPMScheduler()
ddpm = DDPMPipeline(unet=unet, scheduler=scheduler)
ddpm.to(device)
ddpm.set_progress_bar_config(disable=None)
generator = torch.Generator(device=device).manual_seed(0)
image = ddpm(generator=generator, num_inference_steps=2, output_type="numpy").images
generator = torch.Generator(device=device).manual_seed(0)
image_from_tuple = ddpm(generator=generator, num_inference_steps=2, output_type="numpy", return_dict=False)[0]
image_slice = image[0, -3:, -3:, -1]
image_from_tuple_slice = image_from_tuple[0, -3:, -3:, -1]
assert image.shape == (1, 32, 32, 3)
expected_slice = np.array(
[9.956e-01, 5.785e-01, 4.675e-01, 9.930e-01, 0.0, 1.000, 1.199e-03, 2.648e-04, 5.101e-04]
)
assert np.abs(image_slice.flatten() - expected_slice).max() < 1e-2
assert np.abs(image_from_tuple_slice.flatten() - expected_slice).max() < 1e-2
def test_inference_predict_sample(self):
unet = self.dummy_uncond_unet
scheduler = DDPMScheduler(prediction_type="sample")
ddpm = DDPMPipeline(unet=unet, scheduler=scheduler)
ddpm.to(torch_device)
ddpm.set_progress_bar_config(disable=None)
generator = torch.manual_seed(0)
image = ddpm(generator=generator, num_inference_steps=2, output_type="numpy").images
generator = torch.manual_seed(0)
image_eps = ddpm(generator=generator, num_inference_steps=2, output_type="numpy")[0]
image_slice = image[0, -3:, -3:, -1]
image_eps_slice = image_eps[0, -3:, -3:, -1]
assert image.shape == (1, 32, 32, 3)
tolerance = 1e-2 if torch_device != "mps" else 3e-2
assert np.abs(image_slice.flatten() - image_eps_slice.flatten()).max() < tolerance
@slow
@require_torch_gpu
class DDPMPipelineIntegrationTests(unittest.TestCase):
def test_inference_cifar10(self):
model_id = "google/ddpm-cifar10-32"
unet = UNet2DModel.from_pretrained(model_id)
scheduler = DDPMScheduler.from_pretrained(model_id)
ddpm = DDPMPipeline(unet=unet, scheduler=scheduler)
ddpm.to(torch_device)
ddpm.set_progress_bar_config(disable=None)
generator = torch.manual_seed(0)
image = ddpm(generator=generator, output_type="numpy").images
image_slice = image[0, -3:, -3:, -1]
assert image.shape == (1, 32, 32, 3)
expected_slice = np.array([0.4200, 0.3588, 0.1939, 0.3847, 0.3382, 0.2647, 0.4155, 0.3582, 0.3385])
assert np.abs(image_slice.flatten() - expected_slice).max() < 1e-2
| 0 |
hf_public_repos/diffusers/tests/pipelines | hf_public_repos/diffusers/tests/pipelines/audio_diffusion/test_audio_diffusion.py | # coding=utf-8
# Copyright 2023 HuggingFace Inc.
#
# Licensed under the Apache License, Version 2.0 (the "License");
# you may not use this file except in compliance with the License.
# You may obtain a copy of the License at
#
# http://www.apache.org/licenses/LICENSE-2.0
#
# Unless required by applicable law or agreed to in writing, software
# distributed under the License is distributed on an "AS IS" BASIS,
# WITHOUT WARRANTIES OR CONDITIONS OF ANY KIND, either express or implied.
# See the License for the specific language governing permissions and
# limitations under the License.
import gc
import unittest
import numpy as np
import torch
from diffusers import (
AudioDiffusionPipeline,
AutoencoderKL,
DDIMScheduler,
DDPMScheduler,
DiffusionPipeline,
Mel,
UNet2DConditionModel,
UNet2DModel,
)
from diffusers.utils import slow, torch_device
from diffusers.utils.testing_utils import enable_full_determinism, require_torch_gpu
enable_full_determinism()
class PipelineFastTests(unittest.TestCase):
def tearDown(self):
# clean up the VRAM after each test
super().tearDown()
gc.collect()
torch.cuda.empty_cache()
@property
def dummy_unet(self):
torch.manual_seed(0)
model = UNet2DModel(
sample_size=(32, 64),
in_channels=1,
out_channels=1,
layers_per_block=2,
block_out_channels=(128, 128),
down_block_types=("AttnDownBlock2D", "DownBlock2D"),
up_block_types=("UpBlock2D", "AttnUpBlock2D"),
)
return model
@property
def dummy_unet_condition(self):
torch.manual_seed(0)
model = UNet2DConditionModel(
sample_size=(64, 32),
in_channels=1,
out_channels=1,
layers_per_block=2,
block_out_channels=(128, 128),
down_block_types=("CrossAttnDownBlock2D", "DownBlock2D"),
up_block_types=("UpBlock2D", "CrossAttnUpBlock2D"),
cross_attention_dim=10,
)
return model
@property
def dummy_vqvae_and_unet(self):
torch.manual_seed(0)
vqvae = AutoencoderKL(
sample_size=(128, 64),
in_channels=1,
out_channels=1,
latent_channels=1,
layers_per_block=2,
block_out_channels=(128, 128),
down_block_types=("DownEncoderBlock2D", "DownEncoderBlock2D"),
up_block_types=("UpDecoderBlock2D", "UpDecoderBlock2D"),
)
unet = UNet2DModel(
sample_size=(64, 32),
in_channels=1,
out_channels=1,
layers_per_block=2,
block_out_channels=(128, 128),
down_block_types=("AttnDownBlock2D", "DownBlock2D"),
up_block_types=("UpBlock2D", "AttnUpBlock2D"),
)
return vqvae, unet
@slow
def test_audio_diffusion(self):
device = "cpu" # ensure determinism for the device-dependent torch.Generator
mel = Mel(
x_res=self.dummy_unet.config.sample_size[1],
y_res=self.dummy_unet.config.sample_size[0],
)
scheduler = DDPMScheduler()
pipe = AudioDiffusionPipeline(vqvae=None, unet=self.dummy_unet, mel=mel, scheduler=scheduler)
pipe = pipe.to(device)
pipe.set_progress_bar_config(disable=None)
generator = torch.Generator(device=device).manual_seed(42)
output = pipe(generator=generator, steps=4)
audio = output.audios[0]
image = output.images[0]
generator = torch.Generator(device=device).manual_seed(42)
output = pipe(generator=generator, steps=4, return_dict=False)
image_from_tuple = output[0][0]
assert audio.shape == (1, (self.dummy_unet.config.sample_size[1] - 1) * mel.hop_length)
assert (
image.height == self.dummy_unet.config.sample_size[0]
and image.width == self.dummy_unet.config.sample_size[1]
)
image_slice = np.frombuffer(image.tobytes(), dtype="uint8")[:10]
image_from_tuple_slice = np.frombuffer(image_from_tuple.tobytes(), dtype="uint8")[:10]
expected_slice = np.array([69, 255, 255, 255, 0, 0, 77, 181, 12, 127])
assert np.abs(image_slice.flatten() - expected_slice).max() == 0
assert np.abs(image_from_tuple_slice.flatten() - expected_slice).max() == 0
mel = Mel(
x_res=self.dummy_vqvae_and_unet[0].config.sample_size[1],
y_res=self.dummy_vqvae_and_unet[0].config.sample_size[0],
)
scheduler = DDIMScheduler()
dummy_vqvae_and_unet = self.dummy_vqvae_and_unet
pipe = AudioDiffusionPipeline(
vqvae=self.dummy_vqvae_and_unet[0], unet=dummy_vqvae_and_unet[1], mel=mel, scheduler=scheduler
)
pipe = pipe.to(device)
pipe.set_progress_bar_config(disable=None)
np.random.seed(0)
raw_audio = np.random.uniform(-1, 1, ((dummy_vqvae_and_unet[0].config.sample_size[1] - 1) * mel.hop_length,))
generator = torch.Generator(device=device).manual_seed(42)
output = pipe(raw_audio=raw_audio, generator=generator, start_step=5, steps=10)
image = output.images[0]
assert (
image.height == self.dummy_vqvae_and_unet[0].config.sample_size[0]
and image.width == self.dummy_vqvae_and_unet[0].config.sample_size[1]
)
image_slice = np.frombuffer(image.tobytes(), dtype="uint8")[:10]
expected_slice = np.array([120, 117, 110, 109, 138, 167, 138, 148, 132, 121])
assert np.abs(image_slice.flatten() - expected_slice).max() == 0
dummy_unet_condition = self.dummy_unet_condition
pipe = AudioDiffusionPipeline(
vqvae=self.dummy_vqvae_and_unet[0], unet=dummy_unet_condition, mel=mel, scheduler=scheduler
)
pipe = pipe.to(device)
pipe.set_progress_bar_config(disable=None)
np.random.seed(0)
encoding = torch.rand((1, 1, 10))
output = pipe(generator=generator, encoding=encoding)
image = output.images[0]
image_slice = np.frombuffer(image.tobytes(), dtype="uint8")[:10]
expected_slice = np.array([107, 103, 120, 127, 142, 122, 113, 122, 97, 111])
assert np.abs(image_slice.flatten() - expected_slice).max() == 0
@slow
@require_torch_gpu
class PipelineIntegrationTests(unittest.TestCase):
def tearDown(self):
# clean up the VRAM after each test
super().tearDown()
gc.collect()
torch.cuda.empty_cache()
def test_audio_diffusion(self):
device = torch_device
pipe = DiffusionPipeline.from_pretrained("teticio/audio-diffusion-ddim-256")
pipe = pipe.to(device)
pipe.set_progress_bar_config(disable=None)
generator = torch.Generator(device=device).manual_seed(42)
output = pipe(generator=generator)
audio = output.audios[0]
image = output.images[0]
assert audio.shape == (1, (pipe.unet.config.sample_size[1] - 1) * pipe.mel.hop_length)
assert image.height == pipe.unet.config.sample_size[0] and image.width == pipe.unet.config.sample_size[1]
image_slice = np.frombuffer(image.tobytes(), dtype="uint8")[:10]
expected_slice = np.array([151, 167, 154, 144, 122, 134, 121, 105, 70, 26])
assert np.abs(image_slice.flatten() - expected_slice).max() == 0
| 0 |
hf_public_repos/diffusers/tests/pipelines | hf_public_repos/diffusers/tests/pipelines/karras_ve/test_karras_ve.py | # coding=utf-8
# Copyright 2023 HuggingFace Inc.
#
# Licensed under the Apache License, Version 2.0 (the "License");
# you may not use this file except in compliance with the License.
# You may obtain a copy of the License at
#
# http://www.apache.org/licenses/LICENSE-2.0
#
# Unless required by applicable law or agreed to in writing, software
# distributed under the License is distributed on an "AS IS" BASIS,
# WITHOUT WARRANTIES OR CONDITIONS OF ANY KIND, either express or implied.
# See the License for the specific language governing permissions and
# limitations under the License.
import unittest
import numpy as np
import torch
from diffusers import KarrasVePipeline, KarrasVeScheduler, UNet2DModel
from diffusers.utils.testing_utils import enable_full_determinism, require_torch, slow, torch_device
enable_full_determinism()
class KarrasVePipelineFastTests(unittest.TestCase):
@property
def dummy_uncond_unet(self):
torch.manual_seed(0)
model = UNet2DModel(
block_out_channels=(32, 64),
layers_per_block=2,
sample_size=32,
in_channels=3,
out_channels=3,
down_block_types=("DownBlock2D", "AttnDownBlock2D"),
up_block_types=("AttnUpBlock2D", "UpBlock2D"),
)
return model
def test_inference(self):
unet = self.dummy_uncond_unet
scheduler = KarrasVeScheduler()
pipe = KarrasVePipeline(unet=unet, scheduler=scheduler)
pipe.to(torch_device)
pipe.set_progress_bar_config(disable=None)
generator = torch.manual_seed(0)
image = pipe(num_inference_steps=2, generator=generator, output_type="numpy").images
generator = torch.manual_seed(0)
image_from_tuple = pipe(num_inference_steps=2, generator=generator, output_type="numpy", return_dict=False)[0]
image_slice = image[0, -3:, -3:, -1]
image_from_tuple_slice = image_from_tuple[0, -3:, -3:, -1]
assert image.shape == (1, 32, 32, 3)
expected_slice = np.array([0.0, 1.0, 0.0, 0.0, 0.0, 1.0, 0.0, 0.0, 0.0])
assert np.abs(image_slice.flatten() - expected_slice).max() < 1e-2
assert np.abs(image_from_tuple_slice.flatten() - expected_slice).max() < 1e-2
@slow
@require_torch
class KarrasVePipelineIntegrationTests(unittest.TestCase):
def test_inference(self):
model_id = "google/ncsnpp-celebahq-256"
model = UNet2DModel.from_pretrained(model_id)
scheduler = KarrasVeScheduler()
pipe = KarrasVePipeline(unet=model, scheduler=scheduler)
pipe.to(torch_device)
pipe.set_progress_bar_config(disable=None)
generator = torch.manual_seed(0)
image = pipe(num_inference_steps=20, generator=generator, output_type="numpy").images
image_slice = image[0, -3:, -3:, -1]
assert image.shape == (1, 256, 256, 3)
expected_slice = np.array([0.578, 0.5811, 0.5924, 0.5809, 0.587, 0.5886, 0.5861, 0.5802, 0.586])
assert np.abs(image_slice.flatten() - expected_slice).max() < 1e-2
| 0 |
hf_public_repos/diffusers/tests/pipelines | hf_public_repos/diffusers/tests/pipelines/unclip/test_unclip.py | # coding=utf-8
# Copyright 2023 HuggingFace Inc.
#
# Licensed under the Apache License, Version 2.0 (the "License");
# you may not use this file except in compliance with the License.
# You may obtain a copy of the License at
#
# http://www.apache.org/licenses/LICENSE-2.0
#
# Unless required by applicable law or agreed to in writing, software
# distributed under the License is distributed on an "AS IS" BASIS,
# WITHOUT WARRANTIES OR CONDITIONS OF ANY KIND, either express or implied.
# See the License for the specific language governing permissions and
# limitations under the License.
import gc
import unittest
import numpy as np
import torch
from transformers import CLIPTextConfig, CLIPTextModelWithProjection, CLIPTokenizer
from diffusers import PriorTransformer, UnCLIPPipeline, UnCLIPScheduler, UNet2DConditionModel, UNet2DModel
from diffusers.pipelines.unclip.text_proj import UnCLIPTextProjModel
from diffusers.utils import load_numpy, nightly, slow, torch_device
from diffusers.utils.testing_utils import enable_full_determinism, require_torch_gpu, skip_mps
from ..pipeline_params import TEXT_TO_IMAGE_BATCH_PARAMS, TEXT_TO_IMAGE_PARAMS
from ..test_pipelines_common import PipelineTesterMixin, assert_mean_pixel_difference
enable_full_determinism()
class UnCLIPPipelineFastTests(PipelineTesterMixin, unittest.TestCase):
pipeline_class = UnCLIPPipeline
params = TEXT_TO_IMAGE_PARAMS - {
"negative_prompt",
"height",
"width",
"negative_prompt_embeds",
"guidance_scale",
"prompt_embeds",
"cross_attention_kwargs",
}
batch_params = TEXT_TO_IMAGE_BATCH_PARAMS
required_optional_params = [
"generator",
"return_dict",
"prior_num_inference_steps",
"decoder_num_inference_steps",
"super_res_num_inference_steps",
]
test_xformers_attention = False
@property
def text_embedder_hidden_size(self):
return 32
@property
def time_input_dim(self):
return 32
@property
def block_out_channels_0(self):
return self.time_input_dim
@property
def time_embed_dim(self):
return self.time_input_dim * 4
@property
def cross_attention_dim(self):
return 100
@property
def dummy_tokenizer(self):
tokenizer = CLIPTokenizer.from_pretrained("hf-internal-testing/tiny-random-clip")
return tokenizer
@property
def dummy_text_encoder(self):
torch.manual_seed(0)
config = CLIPTextConfig(
bos_token_id=0,
eos_token_id=2,
hidden_size=self.text_embedder_hidden_size,
projection_dim=self.text_embedder_hidden_size,
intermediate_size=37,
layer_norm_eps=1e-05,
num_attention_heads=4,
num_hidden_layers=5,
pad_token_id=1,
vocab_size=1000,
)
return CLIPTextModelWithProjection(config)
@property
def dummy_prior(self):
torch.manual_seed(0)
model_kwargs = {
"num_attention_heads": 2,
"attention_head_dim": 12,
"embedding_dim": self.text_embedder_hidden_size,
"num_layers": 1,
}
model = PriorTransformer(**model_kwargs)
return model
@property
def dummy_text_proj(self):
torch.manual_seed(0)
model_kwargs = {
"clip_embeddings_dim": self.text_embedder_hidden_size,
"time_embed_dim": self.time_embed_dim,
"cross_attention_dim": self.cross_attention_dim,
}
model = UnCLIPTextProjModel(**model_kwargs)
return model
@property
def dummy_decoder(self):
torch.manual_seed(0)
model_kwargs = {
"sample_size": 32,
# RGB in channels
"in_channels": 3,
# Out channels is double in channels because predicts mean and variance
"out_channels": 6,
"down_block_types": ("ResnetDownsampleBlock2D", "SimpleCrossAttnDownBlock2D"),
"up_block_types": ("SimpleCrossAttnUpBlock2D", "ResnetUpsampleBlock2D"),
"mid_block_type": "UNetMidBlock2DSimpleCrossAttn",
"block_out_channels": (self.block_out_channels_0, self.block_out_channels_0 * 2),
"layers_per_block": 1,
"cross_attention_dim": self.cross_attention_dim,
"attention_head_dim": 4,
"resnet_time_scale_shift": "scale_shift",
"class_embed_type": "identity",
}
model = UNet2DConditionModel(**model_kwargs)
return model
@property
def dummy_super_res_kwargs(self):
return {
"sample_size": 64,
"layers_per_block": 1,
"down_block_types": ("ResnetDownsampleBlock2D", "ResnetDownsampleBlock2D"),
"up_block_types": ("ResnetUpsampleBlock2D", "ResnetUpsampleBlock2D"),
"block_out_channels": (self.block_out_channels_0, self.block_out_channels_0 * 2),
"in_channels": 6,
"out_channels": 3,
}
@property
def dummy_super_res_first(self):
torch.manual_seed(0)
model = UNet2DModel(**self.dummy_super_res_kwargs)
return model
@property
def dummy_super_res_last(self):
# seeded differently to get different unet than `self.dummy_super_res_first`
torch.manual_seed(1)
model = UNet2DModel(**self.dummy_super_res_kwargs)
return model
def get_dummy_components(self):
prior = self.dummy_prior
decoder = self.dummy_decoder
text_proj = self.dummy_text_proj
text_encoder = self.dummy_text_encoder
tokenizer = self.dummy_tokenizer
super_res_first = self.dummy_super_res_first
super_res_last = self.dummy_super_res_last
prior_scheduler = UnCLIPScheduler(
variance_type="fixed_small_log",
prediction_type="sample",
num_train_timesteps=1000,
clip_sample_range=5.0,
)
decoder_scheduler = UnCLIPScheduler(
variance_type="learned_range",
prediction_type="epsilon",
num_train_timesteps=1000,
)
super_res_scheduler = UnCLIPScheduler(
variance_type="fixed_small_log",
prediction_type="epsilon",
num_train_timesteps=1000,
)
components = {
"prior": prior,
"decoder": decoder,
"text_proj": text_proj,
"text_encoder": text_encoder,
"tokenizer": tokenizer,
"super_res_first": super_res_first,
"super_res_last": super_res_last,
"prior_scheduler": prior_scheduler,
"decoder_scheduler": decoder_scheduler,
"super_res_scheduler": super_res_scheduler,
}
return components
def get_dummy_inputs(self, device, seed=0):
if str(device).startswith("mps"):
generator = torch.manual_seed(seed)
else:
generator = torch.Generator(device=device).manual_seed(seed)
inputs = {
"prompt": "horse",
"generator": generator,
"prior_num_inference_steps": 2,
"decoder_num_inference_steps": 2,
"super_res_num_inference_steps": 2,
"output_type": "numpy",
}
return inputs
def test_unclip(self):
device = "cpu"
components = self.get_dummy_components()
pipe = self.pipeline_class(**components)
pipe = pipe.to(device)
pipe.set_progress_bar_config(disable=None)
output = pipe(**self.get_dummy_inputs(device))
image = output.images
image_from_tuple = pipe(
**self.get_dummy_inputs(device),
return_dict=False,
)[0]
image_slice = image[0, -3:, -3:, -1]
image_from_tuple_slice = image_from_tuple[0, -3:, -3:, -1]
assert image.shape == (1, 64, 64, 3)
expected_slice = np.array(
[
0.9997,
0.9988,
0.0028,
0.9997,
0.9984,
0.9965,
0.0029,
0.9986,
0.0025,
]
)
assert np.abs(image_slice.flatten() - expected_slice).max() < 1e-2
assert np.abs(image_from_tuple_slice.flatten() - expected_slice).max() < 1e-2
def test_unclip_passed_text_embed(self):
device = torch.device("cpu")
class DummyScheduler:
init_noise_sigma = 1
components = self.get_dummy_components()
pipe = self.pipeline_class(**components)
pipe = pipe.to(device)
prior = components["prior"]
decoder = components["decoder"]
super_res_first = components["super_res_first"]
tokenizer = components["tokenizer"]
text_encoder = components["text_encoder"]
generator = torch.Generator(device=device).manual_seed(0)
dtype = prior.dtype
batch_size = 1
shape = (batch_size, prior.config.embedding_dim)
prior_latents = pipe.prepare_latents(
shape, dtype=dtype, device=device, generator=generator, latents=None, scheduler=DummyScheduler()
)
shape = (batch_size, decoder.config.in_channels, decoder.config.sample_size, decoder.config.sample_size)
decoder_latents = pipe.prepare_latents(
shape, dtype=dtype, device=device, generator=generator, latents=None, scheduler=DummyScheduler()
)
shape = (
batch_size,
super_res_first.config.in_channels // 2,
super_res_first.config.sample_size,
super_res_first.config.sample_size,
)
super_res_latents = pipe.prepare_latents(
shape, dtype=dtype, device=device, generator=generator, latents=None, scheduler=DummyScheduler()
)
pipe.set_progress_bar_config(disable=None)
prompt = "this is a prompt example"
generator = torch.Generator(device=device).manual_seed(0)
output = pipe(
[prompt],
generator=generator,
prior_num_inference_steps=2,
decoder_num_inference_steps=2,
super_res_num_inference_steps=2,
prior_latents=prior_latents,
decoder_latents=decoder_latents,
super_res_latents=super_res_latents,
output_type="np",
)
image = output.images
text_inputs = tokenizer(
prompt,
padding="max_length",
max_length=tokenizer.model_max_length,
return_tensors="pt",
)
text_model_output = text_encoder(text_inputs.input_ids)
text_attention_mask = text_inputs.attention_mask
generator = torch.Generator(device=device).manual_seed(0)
image_from_text = pipe(
generator=generator,
prior_num_inference_steps=2,
decoder_num_inference_steps=2,
super_res_num_inference_steps=2,
prior_latents=prior_latents,
decoder_latents=decoder_latents,
super_res_latents=super_res_latents,
text_model_output=text_model_output,
text_attention_mask=text_attention_mask,
output_type="np",
)[0]
# make sure passing text embeddings manually is identical
assert np.abs(image - image_from_text).max() < 1e-4
# Overriding PipelineTesterMixin::test_attention_slicing_forward_pass
# because UnCLIP GPU undeterminism requires a looser check.
@skip_mps
def test_attention_slicing_forward_pass(self):
test_max_difference = torch_device == "cpu"
self._test_attention_slicing_forward_pass(test_max_difference=test_max_difference, expected_max_diff=0.01)
# Overriding PipelineTesterMixin::test_inference_batch_single_identical
# because UnCLIP undeterminism requires a looser check.
@skip_mps
def test_inference_batch_single_identical(self):
test_max_difference = torch_device == "cpu"
relax_max_difference = True
additional_params_copy_to_batched_inputs = [
"prior_num_inference_steps",
"decoder_num_inference_steps",
"super_res_num_inference_steps",
]
self._test_inference_batch_single_identical(
test_max_difference=test_max_difference,
relax_max_difference=relax_max_difference,
additional_params_copy_to_batched_inputs=additional_params_copy_to_batched_inputs,
)
def test_inference_batch_consistent(self):
additional_params_copy_to_batched_inputs = [
"prior_num_inference_steps",
"decoder_num_inference_steps",
"super_res_num_inference_steps",
]
if torch_device == "mps":
# TODO: MPS errors with larger batch sizes
batch_sizes = [2, 3]
self._test_inference_batch_consistent(
batch_sizes=batch_sizes,
additional_params_copy_to_batched_inputs=additional_params_copy_to_batched_inputs,
)
else:
self._test_inference_batch_consistent(
additional_params_copy_to_batched_inputs=additional_params_copy_to_batched_inputs
)
@skip_mps
def test_dict_tuple_outputs_equivalent(self):
return super().test_dict_tuple_outputs_equivalent()
@skip_mps
def test_save_load_local(self):
return super().test_save_load_local()
@skip_mps
def test_save_load_optional_components(self):
return super().test_save_load_optional_components()
@nightly
class UnCLIPPipelineCPUIntegrationTests(unittest.TestCase):
def tearDown(self):
# clean up the VRAM after each test
super().tearDown()
gc.collect()
torch.cuda.empty_cache()
def test_unclip_karlo_cpu_fp32(self):
expected_image = load_numpy(
"https://huggingface.co/datasets/hf-internal-testing/diffusers-images/resolve/main"
"/unclip/karlo_v1_alpha_horse_cpu.npy"
)
pipeline = UnCLIPPipeline.from_pretrained("kakaobrain/karlo-v1-alpha")
pipeline.set_progress_bar_config(disable=None)
generator = torch.manual_seed(0)
output = pipeline(
"horse",
num_images_per_prompt=1,
generator=generator,
output_type="np",
)
image = output.images[0]
assert image.shape == (256, 256, 3)
assert np.abs(expected_image - image).max() < 1e-1
@slow
@require_torch_gpu
class UnCLIPPipelineIntegrationTests(unittest.TestCase):
def tearDown(self):
# clean up the VRAM after each test
super().tearDown()
gc.collect()
torch.cuda.empty_cache()
def test_unclip_karlo(self):
expected_image = load_numpy(
"https://huggingface.co/datasets/hf-internal-testing/diffusers-images/resolve/main"
"/unclip/karlo_v1_alpha_horse_fp16.npy"
)
pipeline = UnCLIPPipeline.from_pretrained("kakaobrain/karlo-v1-alpha", torch_dtype=torch.float16)
pipeline = pipeline.to(torch_device)
pipeline.set_progress_bar_config(disable=None)
generator = torch.Generator(device="cpu").manual_seed(0)
output = pipeline(
"horse",
generator=generator,
output_type="np",
)
image = output.images[0]
assert image.shape == (256, 256, 3)
assert_mean_pixel_difference(image, expected_image)
def test_unclip_pipeline_with_sequential_cpu_offloading(self):
torch.cuda.empty_cache()
torch.cuda.reset_max_memory_allocated()
torch.cuda.reset_peak_memory_stats()
pipe = UnCLIPPipeline.from_pretrained("kakaobrain/karlo-v1-alpha", torch_dtype=torch.float16)
pipe = pipe.to(torch_device)
pipe.set_progress_bar_config(disable=None)
pipe.enable_attention_slicing()
pipe.enable_sequential_cpu_offload()
_ = pipe(
"horse",
num_images_per_prompt=1,
prior_num_inference_steps=2,
decoder_num_inference_steps=2,
super_res_num_inference_steps=2,
output_type="np",
)
mem_bytes = torch.cuda.max_memory_allocated()
# make sure that less than 7 GB is allocated
assert mem_bytes < 7 * 10**9
| 0 |
hf_public_repos/diffusers/tests/pipelines | hf_public_repos/diffusers/tests/pipelines/unclip/test_unclip_image_variation.py | # coding=utf-8
# Copyright 2023 HuggingFace Inc.
#
# Licensed under the Apache License, Version 2.0 (the "License");
# you may not use this file except in compliance with the License.
# You may obtain a copy of the License at
#
# http://www.apache.org/licenses/LICENSE-2.0
#
# Unless required by applicable law or agreed to in writing, software
# distributed under the License is distributed on an "AS IS" BASIS,
# WITHOUT WARRANTIES OR CONDITIONS OF ANY KIND, either express or implied.
# See the License for the specific language governing permissions and
# limitations under the License.
import gc
import random
import unittest
import numpy as np
import torch
from transformers import (
CLIPImageProcessor,
CLIPTextConfig,
CLIPTextModelWithProjection,
CLIPTokenizer,
CLIPVisionConfig,
CLIPVisionModelWithProjection,
)
from diffusers import (
DiffusionPipeline,
UnCLIPImageVariationPipeline,
UnCLIPScheduler,
UNet2DConditionModel,
UNet2DModel,
)
from diffusers.pipelines.unclip.text_proj import UnCLIPTextProjModel
from diffusers.utils import floats_tensor, load_numpy, slow, torch_device
from diffusers.utils.testing_utils import enable_full_determinism, load_image, require_torch_gpu, skip_mps
from ..pipeline_params import IMAGE_VARIATION_BATCH_PARAMS, IMAGE_VARIATION_PARAMS
from ..test_pipelines_common import PipelineTesterMixin, assert_mean_pixel_difference
enable_full_determinism()
class UnCLIPImageVariationPipelineFastTests(PipelineTesterMixin, unittest.TestCase):
pipeline_class = UnCLIPImageVariationPipeline
params = IMAGE_VARIATION_PARAMS - {"height", "width", "guidance_scale"}
batch_params = IMAGE_VARIATION_BATCH_PARAMS
required_optional_params = [
"generator",
"return_dict",
"decoder_num_inference_steps",
"super_res_num_inference_steps",
]
test_xformers_attention = False
@property
def text_embedder_hidden_size(self):
return 32
@property
def time_input_dim(self):
return 32
@property
def block_out_channels_0(self):
return self.time_input_dim
@property
def time_embed_dim(self):
return self.time_input_dim * 4
@property
def cross_attention_dim(self):
return 100
@property
def dummy_tokenizer(self):
tokenizer = CLIPTokenizer.from_pretrained("hf-internal-testing/tiny-random-clip")
return tokenizer
@property
def dummy_text_encoder(self):
torch.manual_seed(0)
config = CLIPTextConfig(
bos_token_id=0,
eos_token_id=2,
hidden_size=self.text_embedder_hidden_size,
projection_dim=self.text_embedder_hidden_size,
intermediate_size=37,
layer_norm_eps=1e-05,
num_attention_heads=4,
num_hidden_layers=5,
pad_token_id=1,
vocab_size=1000,
)
return CLIPTextModelWithProjection(config)
@property
def dummy_image_encoder(self):
torch.manual_seed(0)
config = CLIPVisionConfig(
hidden_size=self.text_embedder_hidden_size,
projection_dim=self.text_embedder_hidden_size,
num_hidden_layers=5,
num_attention_heads=4,
image_size=32,
intermediate_size=37,
patch_size=1,
)
return CLIPVisionModelWithProjection(config)
@property
def dummy_text_proj(self):
torch.manual_seed(0)
model_kwargs = {
"clip_embeddings_dim": self.text_embedder_hidden_size,
"time_embed_dim": self.time_embed_dim,
"cross_attention_dim": self.cross_attention_dim,
}
model = UnCLIPTextProjModel(**model_kwargs)
return model
@property
def dummy_decoder(self):
torch.manual_seed(0)
model_kwargs = {
"sample_size": 32,
# RGB in channels
"in_channels": 3,
# Out channels is double in channels because predicts mean and variance
"out_channels": 6,
"down_block_types": ("ResnetDownsampleBlock2D", "SimpleCrossAttnDownBlock2D"),
"up_block_types": ("SimpleCrossAttnUpBlock2D", "ResnetUpsampleBlock2D"),
"mid_block_type": "UNetMidBlock2DSimpleCrossAttn",
"block_out_channels": (self.block_out_channels_0, self.block_out_channels_0 * 2),
"layers_per_block": 1,
"cross_attention_dim": self.cross_attention_dim,
"attention_head_dim": 4,
"resnet_time_scale_shift": "scale_shift",
"class_embed_type": "identity",
}
model = UNet2DConditionModel(**model_kwargs)
return model
@property
def dummy_super_res_kwargs(self):
return {
"sample_size": 64,
"layers_per_block": 1,
"down_block_types": ("ResnetDownsampleBlock2D", "ResnetDownsampleBlock2D"),
"up_block_types": ("ResnetUpsampleBlock2D", "ResnetUpsampleBlock2D"),
"block_out_channels": (self.block_out_channels_0, self.block_out_channels_0 * 2),
"in_channels": 6,
"out_channels": 3,
}
@property
def dummy_super_res_first(self):
torch.manual_seed(0)
model = UNet2DModel(**self.dummy_super_res_kwargs)
return model
@property
def dummy_super_res_last(self):
# seeded differently to get different unet than `self.dummy_super_res_first`
torch.manual_seed(1)
model = UNet2DModel(**self.dummy_super_res_kwargs)
return model
def get_dummy_components(self):
decoder = self.dummy_decoder
text_proj = self.dummy_text_proj
text_encoder = self.dummy_text_encoder
tokenizer = self.dummy_tokenizer
super_res_first = self.dummy_super_res_first
super_res_last = self.dummy_super_res_last
decoder_scheduler = UnCLIPScheduler(
variance_type="learned_range",
prediction_type="epsilon",
num_train_timesteps=1000,
)
super_res_scheduler = UnCLIPScheduler(
variance_type="fixed_small_log",
prediction_type="epsilon",
num_train_timesteps=1000,
)
feature_extractor = CLIPImageProcessor(crop_size=32, size=32)
image_encoder = self.dummy_image_encoder
return {
"decoder": decoder,
"text_encoder": text_encoder,
"tokenizer": tokenizer,
"text_proj": text_proj,
"feature_extractor": feature_extractor,
"image_encoder": image_encoder,
"super_res_first": super_res_first,
"super_res_last": super_res_last,
"decoder_scheduler": decoder_scheduler,
"super_res_scheduler": super_res_scheduler,
}
def get_dummy_inputs(self, device, seed=0, pil_image=True):
input_image = floats_tensor((1, 3, 32, 32), rng=random.Random(seed)).to(device)
if str(device).startswith("mps"):
generator = torch.manual_seed(seed)
else:
generator = torch.Generator(device=device).manual_seed(seed)
if pil_image:
input_image = input_image * 0.5 + 0.5
input_image = input_image.clamp(0, 1)
input_image = input_image.cpu().permute(0, 2, 3, 1).float().numpy()
input_image = DiffusionPipeline.numpy_to_pil(input_image)[0]
return {
"image": input_image,
"generator": generator,
"decoder_num_inference_steps": 2,
"super_res_num_inference_steps": 2,
"output_type": "np",
}
def test_unclip_image_variation_input_tensor(self):
device = "cpu"
components = self.get_dummy_components()
pipe = self.pipeline_class(**components)
pipe = pipe.to(device)
pipe.set_progress_bar_config(disable=None)
pipeline_inputs = self.get_dummy_inputs(device, pil_image=False)
output = pipe(**pipeline_inputs)
image = output.images
tuple_pipeline_inputs = self.get_dummy_inputs(device, pil_image=False)
image_from_tuple = pipe(
**tuple_pipeline_inputs,
return_dict=False,
)[0]
image_slice = image[0, -3:, -3:, -1]
image_from_tuple_slice = image_from_tuple[0, -3:, -3:, -1]
assert image.shape == (1, 64, 64, 3)
expected_slice = np.array(
[
0.9997,
0.0002,
0.9997,
0.9997,
0.9969,
0.0023,
0.9997,
0.9969,
0.9970,
]
)
assert np.abs(image_slice.flatten() - expected_slice).max() < 1e-2
assert np.abs(image_from_tuple_slice.flatten() - expected_slice).max() < 1e-2
def test_unclip_image_variation_input_image(self):
device = "cpu"
components = self.get_dummy_components()
pipe = self.pipeline_class(**components)
pipe = pipe.to(device)
pipe.set_progress_bar_config(disable=None)
pipeline_inputs = self.get_dummy_inputs(device, pil_image=True)
output = pipe(**pipeline_inputs)
image = output.images
tuple_pipeline_inputs = self.get_dummy_inputs(device, pil_image=True)
image_from_tuple = pipe(
**tuple_pipeline_inputs,
return_dict=False,
)[0]
image_slice = image[0, -3:, -3:, -1]
image_from_tuple_slice = image_from_tuple[0, -3:, -3:, -1]
assert image.shape == (1, 64, 64, 3)
expected_slice = np.array([0.9997, 0.0003, 0.9997, 0.9997, 0.9970, 0.0024, 0.9997, 0.9971, 0.9971])
assert np.abs(image_slice.flatten() - expected_slice).max() < 1e-2
assert np.abs(image_from_tuple_slice.flatten() - expected_slice).max() < 1e-2
def test_unclip_image_variation_input_list_images(self):
device = "cpu"
components = self.get_dummy_components()
pipe = self.pipeline_class(**components)
pipe = pipe.to(device)
pipe.set_progress_bar_config(disable=None)
pipeline_inputs = self.get_dummy_inputs(device, pil_image=True)
pipeline_inputs["image"] = [
pipeline_inputs["image"],
pipeline_inputs["image"],
]
output = pipe(**pipeline_inputs)
image = output.images
tuple_pipeline_inputs = self.get_dummy_inputs(device, pil_image=True)
tuple_pipeline_inputs["image"] = [
tuple_pipeline_inputs["image"],
tuple_pipeline_inputs["image"],
]
image_from_tuple = pipe(
**tuple_pipeline_inputs,
return_dict=False,
)[0]
image_slice = image[0, -3:, -3:, -1]
image_from_tuple_slice = image_from_tuple[0, -3:, -3:, -1]
assert image.shape == (2, 64, 64, 3)
expected_slice = np.array(
[
0.9997,
0.9989,
0.0008,
0.0021,
0.9960,
0.0018,
0.0014,
0.0002,
0.9933,
]
)
assert np.abs(image_slice.flatten() - expected_slice).max() < 1e-2
assert np.abs(image_from_tuple_slice.flatten() - expected_slice).max() < 1e-2
def test_unclip_passed_image_embed(self):
device = torch.device("cpu")
class DummyScheduler:
init_noise_sigma = 1
components = self.get_dummy_components()
pipe = self.pipeline_class(**components)
pipe = pipe.to(device)
pipe.set_progress_bar_config(disable=None)
generator = torch.Generator(device=device).manual_seed(0)
dtype = pipe.decoder.dtype
batch_size = 1
shape = (
batch_size,
pipe.decoder.config.in_channels,
pipe.decoder.config.sample_size,
pipe.decoder.config.sample_size,
)
decoder_latents = pipe.prepare_latents(
shape, dtype=dtype, device=device, generator=generator, latents=None, scheduler=DummyScheduler()
)
shape = (
batch_size,
pipe.super_res_first.config.in_channels // 2,
pipe.super_res_first.config.sample_size,
pipe.super_res_first.config.sample_size,
)
super_res_latents = pipe.prepare_latents(
shape, dtype=dtype, device=device, generator=generator, latents=None, scheduler=DummyScheduler()
)
pipeline_inputs = self.get_dummy_inputs(device, pil_image=False)
img_out_1 = pipe(
**pipeline_inputs, decoder_latents=decoder_latents, super_res_latents=super_res_latents
).images
pipeline_inputs = self.get_dummy_inputs(device, pil_image=False)
# Don't pass image, instead pass embedding
image = pipeline_inputs.pop("image")
image_embeddings = pipe.image_encoder(image).image_embeds
img_out_2 = pipe(
**pipeline_inputs,
decoder_latents=decoder_latents,
super_res_latents=super_res_latents,
image_embeddings=image_embeddings,
).images
# make sure passing text embeddings manually is identical
assert np.abs(img_out_1 - img_out_2).max() < 1e-4
# Overriding PipelineTesterMixin::test_attention_slicing_forward_pass
# because UnCLIP GPU undeterminism requires a looser check.
@skip_mps
def test_attention_slicing_forward_pass(self):
test_max_difference = torch_device == "cpu"
# Check is relaxed because there is not a torch 2.0 sliced attention added kv processor
expected_max_diff = 1e-2
self._test_attention_slicing_forward_pass(
test_max_difference=test_max_difference, expected_max_diff=expected_max_diff
)
# Overriding PipelineTesterMixin::test_inference_batch_single_identical
# because UnCLIP undeterminism requires a looser check.
@skip_mps
def test_inference_batch_single_identical(self):
test_max_difference = torch_device == "cpu"
relax_max_difference = True
additional_params_copy_to_batched_inputs = [
"decoder_num_inference_steps",
"super_res_num_inference_steps",
]
self._test_inference_batch_single_identical(
test_max_difference=test_max_difference,
relax_max_difference=relax_max_difference,
additional_params_copy_to_batched_inputs=additional_params_copy_to_batched_inputs,
)
def test_inference_batch_consistent(self):
additional_params_copy_to_batched_inputs = [
"decoder_num_inference_steps",
"super_res_num_inference_steps",
]
if torch_device == "mps":
# TODO: MPS errors with larger batch sizes
batch_sizes = [2, 3]
self._test_inference_batch_consistent(
batch_sizes=batch_sizes,
additional_params_copy_to_batched_inputs=additional_params_copy_to_batched_inputs,
)
else:
self._test_inference_batch_consistent(
additional_params_copy_to_batched_inputs=additional_params_copy_to_batched_inputs
)
@skip_mps
def test_dict_tuple_outputs_equivalent(self):
return super().test_dict_tuple_outputs_equivalent()
@skip_mps
def test_save_load_local(self):
return super().test_save_load_local()
@skip_mps
def test_save_load_optional_components(self):
return super().test_save_load_optional_components()
@slow
@require_torch_gpu
class UnCLIPImageVariationPipelineIntegrationTests(unittest.TestCase):
def tearDown(self):
# clean up the VRAM after each test
super().tearDown()
gc.collect()
torch.cuda.empty_cache()
def test_unclip_image_variation_karlo(self):
input_image = load_image(
"https://huggingface.co/datasets/hf-internal-testing/diffusers-images/resolve/main/unclip/cat.png"
)
expected_image = load_numpy(
"https://huggingface.co/datasets/hf-internal-testing/diffusers-images/resolve/main"
"/unclip/karlo_v1_alpha_cat_variation_fp16.npy"
)
pipeline = UnCLIPImageVariationPipeline.from_pretrained(
"kakaobrain/karlo-v1-alpha-image-variations", torch_dtype=torch.float16
)
pipeline = pipeline.to(torch_device)
pipeline.set_progress_bar_config(disable=None)
generator = torch.Generator(device="cpu").manual_seed(0)
output = pipeline(
input_image,
generator=generator,
output_type="np",
)
image = output.images[0]
assert image.shape == (256, 256, 3)
assert_mean_pixel_difference(image, expected_image, 15)
| 0 |
hf_public_repos/diffusers/tests/pipelines | hf_public_repos/diffusers/tests/pipelines/stable_unclip/test_stable_unclip_img2img.py | import gc
import random
import unittest
import numpy as np
import torch
from transformers import (
CLIPImageProcessor,
CLIPTextConfig,
CLIPTextModel,
CLIPTokenizer,
CLIPVisionConfig,
CLIPVisionModelWithProjection,
)
from diffusers import AutoencoderKL, DDIMScheduler, DDPMScheduler, StableUnCLIPImg2ImgPipeline, UNet2DConditionModel
from diffusers.pipelines.pipeline_utils import DiffusionPipeline
from diffusers.pipelines.stable_diffusion.stable_unclip_image_normalizer import StableUnCLIPImageNormalizer
from diffusers.utils.import_utils import is_xformers_available
from diffusers.utils.testing_utils import (
enable_full_determinism,
floats_tensor,
load_image,
load_numpy,
require_torch_gpu,
skip_mps,
slow,
torch_device,
)
from ..pipeline_params import TEXT_GUIDED_IMAGE_VARIATION_BATCH_PARAMS, TEXT_GUIDED_IMAGE_VARIATION_PARAMS
from ..test_pipelines_common import (
PipelineKarrasSchedulerTesterMixin,
PipelineLatentTesterMixin,
PipelineTesterMixin,
assert_mean_pixel_difference,
)
enable_full_determinism()
class StableUnCLIPImg2ImgPipelineFastTests(
PipelineLatentTesterMixin, PipelineKarrasSchedulerTesterMixin, PipelineTesterMixin, unittest.TestCase
):
pipeline_class = StableUnCLIPImg2ImgPipeline
params = TEXT_GUIDED_IMAGE_VARIATION_PARAMS
batch_params = TEXT_GUIDED_IMAGE_VARIATION_BATCH_PARAMS
image_params = frozenset(
[]
) # TO-DO: update image_params once pipeline is refactored with VaeImageProcessor.preprocess
image_latents_params = frozenset([])
def get_dummy_components(self):
embedder_hidden_size = 32
embedder_projection_dim = embedder_hidden_size
# image encoding components
feature_extractor = CLIPImageProcessor(crop_size=32, size=32)
torch.manual_seed(0)
image_encoder = CLIPVisionModelWithProjection(
CLIPVisionConfig(
hidden_size=embedder_hidden_size,
projection_dim=embedder_projection_dim,
num_hidden_layers=5,
num_attention_heads=4,
image_size=32,
intermediate_size=37,
patch_size=1,
)
)
# regular denoising components
torch.manual_seed(0)
image_normalizer = StableUnCLIPImageNormalizer(embedding_dim=embedder_hidden_size)
image_noising_scheduler = DDPMScheduler(beta_schedule="squaredcos_cap_v2")
torch.manual_seed(0)
tokenizer = CLIPTokenizer.from_pretrained("hf-internal-testing/tiny-random-clip")
torch.manual_seed(0)
text_encoder = CLIPTextModel(
CLIPTextConfig(
bos_token_id=0,
eos_token_id=2,
hidden_size=embedder_hidden_size,
projection_dim=32,
intermediate_size=37,
layer_norm_eps=1e-05,
num_attention_heads=4,
num_hidden_layers=5,
pad_token_id=1,
vocab_size=1000,
)
)
torch.manual_seed(0)
unet = UNet2DConditionModel(
sample_size=32,
in_channels=4,
out_channels=4,
down_block_types=("CrossAttnDownBlock2D", "DownBlock2D"),
up_block_types=("UpBlock2D", "CrossAttnUpBlock2D"),
block_out_channels=(32, 64),
attention_head_dim=(2, 4),
class_embed_type="projection",
# The class embeddings are the noise augmented image embeddings.
# I.e. the image embeddings concated with the noised embeddings of the same dimension
projection_class_embeddings_input_dim=embedder_projection_dim * 2,
cross_attention_dim=embedder_hidden_size,
layers_per_block=1,
upcast_attention=True,
use_linear_projection=True,
)
torch.manual_seed(0)
scheduler = DDIMScheduler(
beta_schedule="scaled_linear",
beta_start=0.00085,
beta_end=0.012,
prediction_type="v_prediction",
set_alpha_to_one=False,
steps_offset=1,
)
torch.manual_seed(0)
vae = AutoencoderKL()
components = {
# image encoding components
"feature_extractor": feature_extractor,
"image_encoder": image_encoder.eval(),
# image noising components
"image_normalizer": image_normalizer.eval(),
"image_noising_scheduler": image_noising_scheduler,
# regular denoising components
"tokenizer": tokenizer,
"text_encoder": text_encoder.eval(),
"unet": unet.eval(),
"scheduler": scheduler,
"vae": vae.eval(),
}
return components
def get_dummy_inputs(self, device, seed=0, pil_image=True):
if str(device).startswith("mps"):
generator = torch.manual_seed(seed)
else:
generator = torch.Generator(device=device).manual_seed(seed)
input_image = floats_tensor((1, 3, 32, 32), rng=random.Random(seed)).to(device)
if pil_image:
input_image = input_image * 0.5 + 0.5
input_image = input_image.clamp(0, 1)
input_image = input_image.cpu().permute(0, 2, 3, 1).float().numpy()
input_image = DiffusionPipeline.numpy_to_pil(input_image)[0]
return {
"prompt": "An anime racoon running a marathon",
"image": input_image,
"generator": generator,
"num_inference_steps": 2,
"output_type": "np",
}
@skip_mps
def test_image_embeds_none(self):
device = "cpu" # ensure determinism for the device-dependent torch.Generator
components = self.get_dummy_components()
sd_pipe = StableUnCLIPImg2ImgPipeline(**components)
sd_pipe = sd_pipe.to(device)
sd_pipe.set_progress_bar_config(disable=None)
inputs = self.get_dummy_inputs(device)
inputs.update({"image_embeds": None})
image = sd_pipe(**inputs).images
image_slice = image[0, -3:, -3:, -1]
assert image.shape == (1, 32, 32, 3)
expected_slice = np.array([0.3872, 0.7224, 0.5601, 0.4741, 0.6872, 0.5814, 0.4636, 0.3867, 0.5078])
assert np.abs(image_slice.flatten() - expected_slice).max() < 1e-3
# Overriding PipelineTesterMixin::test_attention_slicing_forward_pass
# because GPU undeterminism requires a looser check.
def test_attention_slicing_forward_pass(self):
test_max_difference = torch_device in ["cpu", "mps"]
self._test_attention_slicing_forward_pass(test_max_difference=test_max_difference)
# Overriding PipelineTesterMixin::test_inference_batch_single_identical
# because undeterminism requires a looser check.
def test_inference_batch_single_identical(self):
test_max_difference = torch_device in ["cpu", "mps"]
self._test_inference_batch_single_identical(test_max_difference=test_max_difference)
@unittest.skipIf(
torch_device != "cuda" or not is_xformers_available(),
reason="XFormers attention is only available with CUDA and `xformers` installed",
)
def test_xformers_attention_forwardGenerator_pass(self):
self._test_xformers_attention_forwardGenerator_pass(test_max_difference=False)
@slow
@require_torch_gpu
class StableUnCLIPImg2ImgPipelineIntegrationTests(unittest.TestCase):
def tearDown(self):
# clean up the VRAM after each test
super().tearDown()
gc.collect()
torch.cuda.empty_cache()
def test_stable_unclip_l_img2img(self):
input_image = load_image(
"https://huggingface.co/datasets/hf-internal-testing/diffusers-images/resolve/main/stable_unclip/turtle.png"
)
expected_image = load_numpy(
"https://huggingface.co/datasets/hf-internal-testing/diffusers-images/resolve/main/stable_unclip/stable_unclip_2_1_l_img2img_anime_turtle_fp16.npy"
)
pipe = StableUnCLIPImg2ImgPipeline.from_pretrained(
"fusing/stable-unclip-2-1-l-img2img", torch_dtype=torch.float16
)
pipe.to(torch_device)
pipe.set_progress_bar_config(disable=None)
# stable unclip will oom when integration tests are run on a V100,
# so turn on memory savings
pipe.enable_attention_slicing()
pipe.enable_sequential_cpu_offload()
generator = torch.Generator(device="cpu").manual_seed(0)
output = pipe(input_image, "anime turle", generator=generator, output_type="np")
image = output.images[0]
assert image.shape == (768, 768, 3)
assert_mean_pixel_difference(image, expected_image)
def test_stable_unclip_h_img2img(self):
input_image = load_image(
"https://huggingface.co/datasets/hf-internal-testing/diffusers-images/resolve/main/stable_unclip/turtle.png"
)
expected_image = load_numpy(
"https://huggingface.co/datasets/hf-internal-testing/diffusers-images/resolve/main/stable_unclip/stable_unclip_2_1_h_img2img_anime_turtle_fp16.npy"
)
pipe = StableUnCLIPImg2ImgPipeline.from_pretrained(
"fusing/stable-unclip-2-1-h-img2img", torch_dtype=torch.float16
)
pipe.to(torch_device)
pipe.set_progress_bar_config(disable=None)
# stable unclip will oom when integration tests are run on a V100,
# so turn on memory savings
pipe.enable_attention_slicing()
pipe.enable_sequential_cpu_offload()
generator = torch.Generator(device="cpu").manual_seed(0)
output = pipe(input_image, "anime turle", generator=generator, output_type="np")
image = output.images[0]
assert image.shape == (768, 768, 3)
assert_mean_pixel_difference(image, expected_image)
def test_stable_unclip_img2img_pipeline_with_sequential_cpu_offloading(self):
input_image = load_image(
"https://huggingface.co/datasets/hf-internal-testing/diffusers-images/resolve/main/stable_unclip/turtle.png"
)
torch.cuda.empty_cache()
torch.cuda.reset_max_memory_allocated()
torch.cuda.reset_peak_memory_stats()
pipe = StableUnCLIPImg2ImgPipeline.from_pretrained(
"fusing/stable-unclip-2-1-h-img2img", torch_dtype=torch.float16
)
pipe = pipe.to(torch_device)
pipe.set_progress_bar_config(disable=None)
pipe.enable_attention_slicing()
pipe.enable_sequential_cpu_offload()
_ = pipe(
input_image,
"anime turtle",
num_inference_steps=2,
output_type="np",
)
mem_bytes = torch.cuda.max_memory_allocated()
# make sure that less than 7 GB is allocated
assert mem_bytes < 7 * 10**9
| 0 |
hf_public_repos/diffusers/tests/pipelines | hf_public_repos/diffusers/tests/pipelines/stable_unclip/test_stable_unclip.py | import gc
import unittest
import torch
from transformers import CLIPTextConfig, CLIPTextModel, CLIPTextModelWithProjection, CLIPTokenizer
from diffusers import (
AutoencoderKL,
DDIMScheduler,
DDPMScheduler,
PriorTransformer,
StableUnCLIPPipeline,
UNet2DConditionModel,
)
from diffusers.pipelines.stable_diffusion.stable_unclip_image_normalizer import StableUnCLIPImageNormalizer
from diffusers.utils.testing_utils import enable_full_determinism, load_numpy, require_torch_gpu, slow, torch_device
from ..pipeline_params import TEXT_TO_IMAGE_BATCH_PARAMS, TEXT_TO_IMAGE_IMAGE_PARAMS, TEXT_TO_IMAGE_PARAMS
from ..test_pipelines_common import (
PipelineKarrasSchedulerTesterMixin,
PipelineLatentTesterMixin,
PipelineTesterMixin,
assert_mean_pixel_difference,
)
enable_full_determinism()
class StableUnCLIPPipelineFastTests(
PipelineLatentTesterMixin, PipelineKarrasSchedulerTesterMixin, PipelineTesterMixin, unittest.TestCase
):
pipeline_class = StableUnCLIPPipeline
params = TEXT_TO_IMAGE_PARAMS
batch_params = TEXT_TO_IMAGE_BATCH_PARAMS
image_params = TEXT_TO_IMAGE_IMAGE_PARAMS
image_latents_params = TEXT_TO_IMAGE_IMAGE_PARAMS
# TODO(will) Expected attn_bias.stride(1) == 0 to be true, but got false
test_xformers_attention = False
def get_dummy_components(self):
embedder_hidden_size = 32
embedder_projection_dim = embedder_hidden_size
# prior components
torch.manual_seed(0)
prior_tokenizer = CLIPTokenizer.from_pretrained("hf-internal-testing/tiny-random-clip")
torch.manual_seed(0)
prior_text_encoder = CLIPTextModelWithProjection(
CLIPTextConfig(
bos_token_id=0,
eos_token_id=2,
hidden_size=embedder_hidden_size,
projection_dim=embedder_projection_dim,
intermediate_size=37,
layer_norm_eps=1e-05,
num_attention_heads=4,
num_hidden_layers=5,
pad_token_id=1,
vocab_size=1000,
)
)
torch.manual_seed(0)
prior = PriorTransformer(
num_attention_heads=2,
attention_head_dim=12,
embedding_dim=embedder_projection_dim,
num_layers=1,
)
torch.manual_seed(0)
prior_scheduler = DDPMScheduler(
variance_type="fixed_small_log",
prediction_type="sample",
num_train_timesteps=1000,
clip_sample=True,
clip_sample_range=5.0,
beta_schedule="squaredcos_cap_v2",
)
# regular denoising components
torch.manual_seed(0)
image_normalizer = StableUnCLIPImageNormalizer(embedding_dim=embedder_hidden_size)
image_noising_scheduler = DDPMScheduler(beta_schedule="squaredcos_cap_v2")
torch.manual_seed(0)
tokenizer = CLIPTokenizer.from_pretrained("hf-internal-testing/tiny-random-clip")
torch.manual_seed(0)
text_encoder = CLIPTextModel(
CLIPTextConfig(
bos_token_id=0,
eos_token_id=2,
hidden_size=embedder_hidden_size,
projection_dim=32,
intermediate_size=37,
layer_norm_eps=1e-05,
num_attention_heads=4,
num_hidden_layers=5,
pad_token_id=1,
vocab_size=1000,
)
)
torch.manual_seed(0)
unet = UNet2DConditionModel(
sample_size=32,
in_channels=4,
out_channels=4,
down_block_types=("CrossAttnDownBlock2D", "DownBlock2D"),
up_block_types=("UpBlock2D", "CrossAttnUpBlock2D"),
block_out_channels=(32, 64),
attention_head_dim=(2, 4),
class_embed_type="projection",
# The class embeddings are the noise augmented image embeddings.
# I.e. the image embeddings concated with the noised embeddings of the same dimension
projection_class_embeddings_input_dim=embedder_projection_dim * 2,
cross_attention_dim=embedder_hidden_size,
layers_per_block=1,
upcast_attention=True,
use_linear_projection=True,
)
torch.manual_seed(0)
scheduler = DDIMScheduler(
beta_schedule="scaled_linear",
beta_start=0.00085,
beta_end=0.012,
prediction_type="v_prediction",
set_alpha_to_one=False,
steps_offset=1,
)
torch.manual_seed(0)
vae = AutoencoderKL()
components = {
# prior components
"prior_tokenizer": prior_tokenizer,
"prior_text_encoder": prior_text_encoder,
"prior": prior,
"prior_scheduler": prior_scheduler,
# image noising components
"image_normalizer": image_normalizer,
"image_noising_scheduler": image_noising_scheduler,
# regular denoising components
"tokenizer": tokenizer,
"text_encoder": text_encoder,
"unet": unet,
"scheduler": scheduler,
"vae": vae,
}
return components
def get_dummy_inputs(self, device, seed=0):
if str(device).startswith("mps"):
generator = torch.manual_seed(seed)
else:
generator = torch.Generator(device=device).manual_seed(seed)
inputs = {
"prompt": "A painting of a squirrel eating a burger",
"generator": generator,
"num_inference_steps": 2,
"prior_num_inference_steps": 2,
"output_type": "numpy",
}
return inputs
# Overriding PipelineTesterMixin::test_attention_slicing_forward_pass
# because UnCLIP GPU undeterminism requires a looser check.
def test_attention_slicing_forward_pass(self):
test_max_difference = torch_device == "cpu"
self._test_attention_slicing_forward_pass(test_max_difference=test_max_difference)
# Overriding PipelineTesterMixin::test_inference_batch_single_identical
# because UnCLIP undeterminism requires a looser check.
def test_inference_batch_single_identical(self):
test_max_difference = torch_device in ["cpu", "mps"]
self._test_inference_batch_single_identical(test_max_difference=test_max_difference)
@slow
@require_torch_gpu
class StableUnCLIPPipelineIntegrationTests(unittest.TestCase):
def tearDown(self):
# clean up the VRAM after each test
super().tearDown()
gc.collect()
torch.cuda.empty_cache()
def test_stable_unclip(self):
expected_image = load_numpy(
"https://huggingface.co/datasets/hf-internal-testing/diffusers-images/resolve/main/stable_unclip/stable_unclip_2_1_l_anime_turtle_fp16.npy"
)
pipe = StableUnCLIPPipeline.from_pretrained("fusing/stable-unclip-2-1-l", torch_dtype=torch.float16)
pipe.to(torch_device)
pipe.set_progress_bar_config(disable=None)
# stable unclip will oom when integration tests are run on a V100,
# so turn on memory savings
pipe.enable_attention_slicing()
pipe.enable_sequential_cpu_offload()
generator = torch.Generator(device="cpu").manual_seed(0)
output = pipe("anime turle", generator=generator, output_type="np")
image = output.images[0]
assert image.shape == (768, 768, 3)
assert_mean_pixel_difference(image, expected_image)
def test_stable_unclip_pipeline_with_sequential_cpu_offloading(self):
torch.cuda.empty_cache()
torch.cuda.reset_max_memory_allocated()
torch.cuda.reset_peak_memory_stats()
pipe = StableUnCLIPPipeline.from_pretrained("fusing/stable-unclip-2-1-l", torch_dtype=torch.float16)
pipe = pipe.to(torch_device)
pipe.set_progress_bar_config(disable=None)
pipe.enable_attention_slicing()
pipe.enable_sequential_cpu_offload()
_ = pipe(
"anime turtle",
prior_num_inference_steps=2,
num_inference_steps=2,
output_type="np",
)
mem_bytes = torch.cuda.max_memory_allocated()
# make sure that less than 7 GB is allocated
assert mem_bytes < 7 * 10**9
| 0 |
hf_public_repos/diffusers/tests | hf_public_repos/diffusers/tests/others/test_check_copies.py | # Copyright 2023 The HuggingFace Team. All rights reserved.
#
# Licensed under the Apache License, Version 2.0 (the "License");
# you may not use this file except in compliance with the License.
# You may obtain a copy of the License at
#
# http://www.apache.org/licenses/LICENSE-2.0
#
# Unless required by applicable law or agreed to in writing, software
# distributed under the License is distributed on an "AS IS" BASIS,
# WITHOUT WARRANTIES OR CONDITIONS OF ANY KIND, either express or implied.
# See the License for the specific language governing permissions and
# limitations under the License.
import os
import re
import shutil
import sys
import tempfile
import unittest
import black
git_repo_path = os.path.abspath(os.path.dirname(os.path.dirname(os.path.dirname(__file__))))
sys.path.append(os.path.join(git_repo_path, "utils"))
import check_copies # noqa: E402
# This is the reference code that will be used in the tests.
# If DDPMSchedulerOutput is changed in scheduling_ddpm.py, this code needs to be manually updated.
REFERENCE_CODE = """ \"""
Output class for the scheduler's step function output.
Args:
prev_sample (`torch.FloatTensor` of shape `(batch_size, num_channels, height, width)` for images):
Computed sample (x_{t-1}) of previous timestep. `prev_sample` should be used as next model input in the
denoising loop.
pred_original_sample (`torch.FloatTensor` of shape `(batch_size, num_channels, height, width)` for images):
The predicted denoised sample (x_{0}) based on the model output from the current timestep.
`pred_original_sample` can be used to preview progress or for guidance.
\"""
prev_sample: torch.FloatTensor
pred_original_sample: Optional[torch.FloatTensor] = None
"""
class CopyCheckTester(unittest.TestCase):
def setUp(self):
self.diffusers_dir = tempfile.mkdtemp()
os.makedirs(os.path.join(self.diffusers_dir, "schedulers/"))
check_copies.DIFFUSERS_PATH = self.diffusers_dir
shutil.copy(
os.path.join(git_repo_path, "src/diffusers/schedulers/scheduling_ddpm.py"),
os.path.join(self.diffusers_dir, "schedulers/scheduling_ddpm.py"),
)
def tearDown(self):
check_copies.DIFFUSERS_PATH = "src/diffusers"
shutil.rmtree(self.diffusers_dir)
def check_copy_consistency(self, comment, class_name, class_code, overwrite_result=None):
code = comment + f"\nclass {class_name}(nn.Module):\n" + class_code
if overwrite_result is not None:
expected = comment + f"\nclass {class_name}(nn.Module):\n" + overwrite_result
mode = black.Mode(target_versions={black.TargetVersion.PY35}, line_length=119)
code = black.format_str(code, mode=mode)
fname = os.path.join(self.diffusers_dir, "new_code.py")
with open(fname, "w", newline="\n") as f:
f.write(code)
if overwrite_result is None:
self.assertTrue(len(check_copies.is_copy_consistent(fname)) == 0)
else:
check_copies.is_copy_consistent(f.name, overwrite=True)
with open(fname, "r") as f:
self.assertTrue(f.read(), expected)
def test_find_code_in_diffusers(self):
code = check_copies.find_code_in_diffusers("schedulers.scheduling_ddpm.DDPMSchedulerOutput")
self.assertEqual(code, REFERENCE_CODE)
def test_is_copy_consistent(self):
# Base copy consistency
self.check_copy_consistency(
"# Copied from diffusers.schedulers.scheduling_ddpm.DDPMSchedulerOutput",
"DDPMSchedulerOutput",
REFERENCE_CODE + "\n",
)
# With no empty line at the end
self.check_copy_consistency(
"# Copied from diffusers.schedulers.scheduling_ddpm.DDPMSchedulerOutput",
"DDPMSchedulerOutput",
REFERENCE_CODE,
)
# Copy consistency with rename
self.check_copy_consistency(
"# Copied from diffusers.schedulers.scheduling_ddpm.DDPMSchedulerOutput with DDPM->Test",
"TestSchedulerOutput",
re.sub("DDPM", "Test", REFERENCE_CODE),
)
# Copy consistency with a really long name
long_class_name = "TestClassWithAReallyLongNameBecauseSomePeopleLikeThatForSomeReason"
self.check_copy_consistency(
f"# Copied from diffusers.schedulers.scheduling_ddpm.DDPMSchedulerOutput with DDPM->{long_class_name}",
f"{long_class_name}SchedulerOutput",
re.sub("Bert", long_class_name, REFERENCE_CODE),
)
# Copy consistency with overwrite
self.check_copy_consistency(
"# Copied from diffusers.schedulers.scheduling_ddpm.DDPMSchedulerOutput with DDPM->Test",
"TestSchedulerOutput",
REFERENCE_CODE,
overwrite_result=re.sub("DDPM", "Test", REFERENCE_CODE),
)
| 0 |
hf_public_repos/diffusers/tests | hf_public_repos/diffusers/tests/others/test_training.py | # coding=utf-8
# Copyright 2023 HuggingFace Inc.
#
# Licensed under the Apache License, Version 2.0 (the "License");
# you may not use this file except in compliance with the License.
# You may obtain a copy of the License at
#
# http://www.apache.org/licenses/LICENSE-2.0
#
# Unless required by applicable law or agreed to in writing, software
# distributed under the License is distributed on an "AS IS" BASIS,
# WITHOUT WARRANTIES OR CONDITIONS OF ANY KIND, either express or implied.
# See the License for the specific language governing permissions and
# limitations under the License.
import unittest
import torch
from diffusers import DDIMScheduler, DDPMScheduler, UNet2DModel
from diffusers.training_utils import set_seed
from diffusers.utils.testing_utils import slow
torch.backends.cuda.matmul.allow_tf32 = False
class TrainingTests(unittest.TestCase):
def get_model_optimizer(self, resolution=32):
set_seed(0)
model = UNet2DModel(sample_size=resolution, in_channels=3, out_channels=3)
optimizer = torch.optim.SGD(model.parameters(), lr=0.0001)
return model, optimizer
@slow
def test_training_step_equality(self):
device = "cpu" # ensure full determinism without setting the CUBLAS_WORKSPACE_CONFIG env variable
ddpm_scheduler = DDPMScheduler(
num_train_timesteps=1000,
beta_start=0.0001,
beta_end=0.02,
beta_schedule="linear",
clip_sample=True,
)
ddim_scheduler = DDIMScheduler(
num_train_timesteps=1000,
beta_start=0.0001,
beta_end=0.02,
beta_schedule="linear",
clip_sample=True,
)
assert ddpm_scheduler.config.num_train_timesteps == ddim_scheduler.config.num_train_timesteps
# shared batches for DDPM and DDIM
set_seed(0)
clean_images = [torch.randn((4, 3, 32, 32)).clip(-1, 1).to(device) for _ in range(4)]
noise = [torch.randn((4, 3, 32, 32)).to(device) for _ in range(4)]
timesteps = [torch.randint(0, 1000, (4,)).long().to(device) for _ in range(4)]
# train with a DDPM scheduler
model, optimizer = self.get_model_optimizer(resolution=32)
model.train().to(device)
for i in range(4):
optimizer.zero_grad()
ddpm_noisy_images = ddpm_scheduler.add_noise(clean_images[i], noise[i], timesteps[i])
ddpm_noise_pred = model(ddpm_noisy_images, timesteps[i]).sample
loss = torch.nn.functional.mse_loss(ddpm_noise_pred, noise[i])
loss.backward()
optimizer.step()
del model, optimizer
# recreate the model and optimizer, and retry with DDIM
model, optimizer = self.get_model_optimizer(resolution=32)
model.train().to(device)
for i in range(4):
optimizer.zero_grad()
ddim_noisy_images = ddim_scheduler.add_noise(clean_images[i], noise[i], timesteps[i])
ddim_noise_pred = model(ddim_noisy_images, timesteps[i]).sample
loss = torch.nn.functional.mse_loss(ddim_noise_pred, noise[i])
loss.backward()
optimizer.step()
del model, optimizer
self.assertTrue(torch.allclose(ddpm_noisy_images, ddim_noisy_images, atol=1e-5))
self.assertTrue(torch.allclose(ddpm_noise_pred, ddim_noise_pred, atol=1e-5))
| 0 |
hf_public_repos/diffusers/tests | hf_public_repos/diffusers/tests/others/test_ema.py | # coding=utf-8
# Copyright 2023 HuggingFace Inc.
#
# Licensed under the Apache License, Version 2.0 (the "License");
# you may not use this file except in compliance with the License.
# You may obtain a copy of the License at
#
# http://www.apache.org/licenses/LICENSE-2.0
#
# Unless required by applicable law or agreed to in writing, software
# distributed under the License is distributed on an "AS IS" BASIS,
# WITHOUT WARRANTIES OR CONDITIONS OF ANY KIND, either express or implied.
# See the License for the specific language governing permissions and
# limitations under the License.
import tempfile
import unittest
import torch
from diffusers import UNet2DConditionModel
from diffusers.training_utils import EMAModel
from diffusers.utils.testing_utils import enable_full_determinism, skip_mps, torch_device
enable_full_determinism()
class EMAModelTests(unittest.TestCase):
model_id = "hf-internal-testing/tiny-stable-diffusion-pipe"
batch_size = 1
prompt_length = 77
text_encoder_hidden_dim = 32
num_in_channels = 4
latent_height = latent_width = 64
generator = torch.manual_seed(0)
def get_models(self, decay=0.9999):
unet = UNet2DConditionModel.from_pretrained(self.model_id, subfolder="unet")
unet = unet.to(torch_device)
ema_unet = EMAModel(unet.parameters(), decay=decay, model_cls=UNet2DConditionModel, model_config=unet.config)
return unet, ema_unet
def get_dummy_inputs(self):
noisy_latents = torch.randn(
self.batch_size, self.num_in_channels, self.latent_height, self.latent_width, generator=self.generator
).to(torch_device)
timesteps = torch.randint(0, 1000, size=(self.batch_size,), generator=self.generator).to(torch_device)
encoder_hidden_states = torch.randn(
self.batch_size, self.prompt_length, self.text_encoder_hidden_dim, generator=self.generator
).to(torch_device)
return noisy_latents, timesteps, encoder_hidden_states
def simulate_backprop(self, unet):
updated_state_dict = {}
for k, param in unet.state_dict().items():
updated_param = torch.randn_like(param) + (param * torch.randn_like(param))
updated_state_dict.update({k: updated_param})
unet.load_state_dict(updated_state_dict)
return unet
def test_optimization_steps_updated(self):
unet, ema_unet = self.get_models()
# Take the first (hypothetical) EMA step.
ema_unet.step(unet.parameters())
assert ema_unet.optimization_step == 1
# Take two more.
for _ in range(2):
ema_unet.step(unet.parameters())
assert ema_unet.optimization_step == 3
def test_shadow_params_not_updated(self):
unet, ema_unet = self.get_models()
# Since the `unet` is not being updated (i.e., backprop'd)
# there won't be any difference between the `params` of `unet`
# and `ema_unet` even if we call `ema_unet.step(unet.parameters())`.
ema_unet.step(unet.parameters())
orig_params = list(unet.parameters())
for s_param, param in zip(ema_unet.shadow_params, orig_params):
assert torch.allclose(s_param, param)
# The above holds true even if we call `ema.step()` multiple times since
# `unet` params are still not being updated.
for _ in range(4):
ema_unet.step(unet.parameters())
for s_param, param in zip(ema_unet.shadow_params, orig_params):
assert torch.allclose(s_param, param)
def test_shadow_params_updated(self):
unet, ema_unet = self.get_models()
# Here we simulate the parameter updates for `unet`. Since there might
# be some parameters which are initialized to zero we take extra care to
# initialize their values to something non-zero before the multiplication.
unet_pseudo_updated_step_one = self.simulate_backprop(unet)
# Take the EMA step.
ema_unet.step(unet_pseudo_updated_step_one.parameters())
# Now the EMA'd parameters won't be equal to the original model parameters.
orig_params = list(unet_pseudo_updated_step_one.parameters())
for s_param, param in zip(ema_unet.shadow_params, orig_params):
assert ~torch.allclose(s_param, param)
# Ensure this is the case when we take multiple EMA steps.
for _ in range(4):
ema_unet.step(unet.parameters())
for s_param, param in zip(ema_unet.shadow_params, orig_params):
assert ~torch.allclose(s_param, param)
def test_consecutive_shadow_params_updated(self):
# If we call EMA step after a backpropagation consecutively for two times,
# the shadow params from those two steps should be different.
unet, ema_unet = self.get_models()
# First backprop + EMA
unet_step_one = self.simulate_backprop(unet)
ema_unet.step(unet_step_one.parameters())
step_one_shadow_params = ema_unet.shadow_params
# Second backprop + EMA
unet_step_two = self.simulate_backprop(unet_step_one)
ema_unet.step(unet_step_two.parameters())
step_two_shadow_params = ema_unet.shadow_params
for step_one, step_two in zip(step_one_shadow_params, step_two_shadow_params):
assert ~torch.allclose(step_one, step_two)
def test_zero_decay(self):
# If there's no decay even if there are backprops, EMA steps
# won't take any effect i.e., the shadow params would remain the
# same.
unet, ema_unet = self.get_models(decay=0.0)
unet_step_one = self.simulate_backprop(unet)
ema_unet.step(unet_step_one.parameters())
step_one_shadow_params = ema_unet.shadow_params
unet_step_two = self.simulate_backprop(unet_step_one)
ema_unet.step(unet_step_two.parameters())
step_two_shadow_params = ema_unet.shadow_params
for step_one, step_two in zip(step_one_shadow_params, step_two_shadow_params):
assert torch.allclose(step_one, step_two)
@skip_mps
def test_serialization(self):
unet, ema_unet = self.get_models()
noisy_latents, timesteps, encoder_hidden_states = self.get_dummy_inputs()
with tempfile.TemporaryDirectory() as tmpdir:
ema_unet.save_pretrained(tmpdir)
loaded_unet = UNet2DConditionModel.from_pretrained(tmpdir, model_cls=UNet2DConditionModel)
loaded_unet = loaded_unet.to(unet.device)
# Since no EMA step has been performed the outputs should match.
output = unet(noisy_latents, timesteps, encoder_hidden_states).sample
output_loaded = loaded_unet(noisy_latents, timesteps, encoder_hidden_states).sample
assert torch.allclose(output, output_loaded, atol=1e-4)
| 0 |
hf_public_repos/diffusers/tests | hf_public_repos/diffusers/tests/others/test_dependencies.py | # Copyright 2023 The HuggingFace Team. All rights reserved.
#
# Licensed under the Apache License, Version 2.0 (the "License");
# you may not use this file except in compliance with the License.
# You may obtain a copy of the License at
#
# http://www.apache.org/licenses/LICENSE-2.0
#
# Unless required by applicable law or agreed to in writing, software
# distributed under the License is distributed on an "AS IS" BASIS,
# WITHOUT WARRANTIES OR CONDITIONS OF ANY KIND, either express or implied.
# See the License for the specific language governing permissions and
# limitations under the License.
import inspect
import unittest
class DependencyTester(unittest.TestCase):
def test_diffusers_import(self):
try:
import diffusers # noqa: F401
except ImportError:
assert False
def test_backend_registration(self):
import diffusers
from diffusers.dependency_versions_table import deps
all_classes = inspect.getmembers(diffusers, inspect.isclass)
for cls_name, cls_module in all_classes:
if "dummy_" in cls_module.__module__:
for backend in cls_module._backends:
if backend == "k_diffusion":
backend = "k-diffusion"
elif backend == "invisible_watermark":
backend = "invisible-watermark"
assert backend in deps, f"{backend} is not in the deps table!"
| 0 |
hf_public_repos/diffusers/tests | hf_public_repos/diffusers/tests/others/test_config.py | # coding=utf-8
# Copyright 2023 HuggingFace Inc.
#
# Licensed under the Apache License, Version 2.0 (the "License");
# you may not use this file except in compliance with the License.
# You may obtain a copy of the License at
#
# http://www.apache.org/licenses/LICENSE-2.0
#
# Unless required by applicable law or agreed to in writing, software
# distributed under the License is distributed on an "AS IS" BASIS,
# WITHOUT WARRANTIES OR CONDITIONS OF ANY KIND, either express or implied.
# See the License for the specific language governing permissions and
# limitations under the License.
import tempfile
import unittest
from diffusers import (
DDIMScheduler,
DDPMScheduler,
DPMSolverMultistepScheduler,
EulerAncestralDiscreteScheduler,
EulerDiscreteScheduler,
PNDMScheduler,
logging,
)
from diffusers.configuration_utils import ConfigMixin, register_to_config
from diffusers.utils.testing_utils import CaptureLogger
class SampleObject(ConfigMixin):
config_name = "config.json"
@register_to_config
def __init__(
self,
a=2,
b=5,
c=(2, 5),
d="for diffusion",
e=[1, 3],
):
pass
class SampleObject2(ConfigMixin):
config_name = "config.json"
@register_to_config
def __init__(
self,
a=2,
b=5,
c=(2, 5),
d="for diffusion",
f=[1, 3],
):
pass
class SampleObject3(ConfigMixin):
config_name = "config.json"
@register_to_config
def __init__(
self,
a=2,
b=5,
c=(2, 5),
d="for diffusion",
e=[1, 3],
f=[1, 3],
):
pass
class SampleObject4(ConfigMixin):
config_name = "config.json"
@register_to_config
def __init__(
self,
a=2,
b=5,
c=(2, 5),
d="for diffusion",
e=[1, 5],
f=[5, 4],
):
pass
class ConfigTester(unittest.TestCase):
def test_load_not_from_mixin(self):
with self.assertRaises(ValueError):
ConfigMixin.load_config("dummy_path")
def test_register_to_config(self):
obj = SampleObject()
config = obj.config
assert config["a"] == 2
assert config["b"] == 5
assert config["c"] == (2, 5)
assert config["d"] == "for diffusion"
assert config["e"] == [1, 3]
# init ignore private arguments
obj = SampleObject(_name_or_path="lalala")
config = obj.config
assert config["a"] == 2
assert config["b"] == 5
assert config["c"] == (2, 5)
assert config["d"] == "for diffusion"
assert config["e"] == [1, 3]
# can override default
obj = SampleObject(c=6)
config = obj.config
assert config["a"] == 2
assert config["b"] == 5
assert config["c"] == 6
assert config["d"] == "for diffusion"
assert config["e"] == [1, 3]
# can use positional arguments.
obj = SampleObject(1, c=6)
config = obj.config
assert config["a"] == 1
assert config["b"] == 5
assert config["c"] == 6
assert config["d"] == "for diffusion"
assert config["e"] == [1, 3]
def test_save_load(self):
obj = SampleObject()
config = obj.config
assert config["a"] == 2
assert config["b"] == 5
assert config["c"] == (2, 5)
assert config["d"] == "for diffusion"
assert config["e"] == [1, 3]
with tempfile.TemporaryDirectory() as tmpdirname:
obj.save_config(tmpdirname)
new_obj = SampleObject.from_config(SampleObject.load_config(tmpdirname))
new_config = new_obj.config
# unfreeze configs
config = dict(config)
new_config = dict(new_config)
assert config.pop("c") == (2, 5) # instantiated as tuple
assert new_config.pop("c") == [2, 5] # saved & loaded as list because of json
config.pop("_use_default_values")
assert config == new_config
def test_load_ddim_from_pndm(self):
logger = logging.get_logger("diffusers.configuration_utils")
# 30 for warning
logger.setLevel(30)
with CaptureLogger(logger) as cap_logger:
ddim = DDIMScheduler.from_pretrained(
"hf-internal-testing/tiny-stable-diffusion-torch", subfolder="scheduler"
)
assert ddim.__class__ == DDIMScheduler
# no warning should be thrown
assert cap_logger.out == ""
def test_load_euler_from_pndm(self):
logger = logging.get_logger("diffusers.configuration_utils")
# 30 for warning
logger.setLevel(30)
with CaptureLogger(logger) as cap_logger:
euler = EulerDiscreteScheduler.from_pretrained(
"hf-internal-testing/tiny-stable-diffusion-torch", subfolder="scheduler"
)
assert euler.__class__ == EulerDiscreteScheduler
# no warning should be thrown
assert cap_logger.out == ""
def test_load_euler_ancestral_from_pndm(self):
logger = logging.get_logger("diffusers.configuration_utils")
# 30 for warning
logger.setLevel(30)
with CaptureLogger(logger) as cap_logger:
euler = EulerAncestralDiscreteScheduler.from_pretrained(
"hf-internal-testing/tiny-stable-diffusion-torch", subfolder="scheduler"
)
assert euler.__class__ == EulerAncestralDiscreteScheduler
# no warning should be thrown
assert cap_logger.out == ""
def test_load_pndm(self):
logger = logging.get_logger("diffusers.configuration_utils")
# 30 for warning
logger.setLevel(30)
with CaptureLogger(logger) as cap_logger:
pndm = PNDMScheduler.from_pretrained(
"hf-internal-testing/tiny-stable-diffusion-torch", subfolder="scheduler"
)
assert pndm.__class__ == PNDMScheduler
# no warning should be thrown
assert cap_logger.out == ""
def test_overwrite_config_on_load(self):
logger = logging.get_logger("diffusers.configuration_utils")
# 30 for warning
logger.setLevel(30)
with CaptureLogger(logger) as cap_logger:
ddpm = DDPMScheduler.from_pretrained(
"hf-internal-testing/tiny-stable-diffusion-torch",
subfolder="scheduler",
prediction_type="sample",
beta_end=8,
)
with CaptureLogger(logger) as cap_logger_2:
ddpm_2 = DDPMScheduler.from_pretrained("google/ddpm-celebahq-256", beta_start=88)
assert ddpm.__class__ == DDPMScheduler
assert ddpm.config.prediction_type == "sample"
assert ddpm.config.beta_end == 8
assert ddpm_2.config.beta_start == 88
# no warning should be thrown
assert cap_logger.out == ""
assert cap_logger_2.out == ""
def test_load_dpmsolver(self):
logger = logging.get_logger("diffusers.configuration_utils")
# 30 for warning
logger.setLevel(30)
with CaptureLogger(logger) as cap_logger:
dpm = DPMSolverMultistepScheduler.from_pretrained(
"hf-internal-testing/tiny-stable-diffusion-torch", subfolder="scheduler"
)
assert dpm.__class__ == DPMSolverMultistepScheduler
# no warning should be thrown
assert cap_logger.out == ""
def test_use_default_values(self):
# let's first save a config that should be in the form
# a=2,
# b=5,
# c=(2, 5),
# d="for diffusion",
# e=[1, 3],
config = SampleObject()
config_dict = {k: v for k, v in config.config.items() if not k.startswith("_")}
# make sure that default config has all keys in `_use_default_values`
assert set(config_dict.keys()) == set(config.config._use_default_values)
with tempfile.TemporaryDirectory() as tmpdirname:
config.save_config(tmpdirname)
# now loading it with SampleObject2 should put f into `_use_default_values`
config = SampleObject2.from_config(tmpdirname)
assert "f" in config._use_default_values
assert config.f == [1, 3]
# now loading the config, should **NOT** use [1, 3] for `f`, but the default [1, 4] value
# **BECAUSE** it is part of `config._use_default_values`
new_config = SampleObject4.from_config(config.config)
assert new_config.f == [5, 4]
config.config._use_default_values.pop()
new_config_2 = SampleObject4.from_config(config.config)
assert new_config_2.f == [1, 3]
# Nevertheless "e" should still be correctly loaded to [1, 3] from SampleObject2 instead of defaulting to [1, 5]
assert new_config_2.e == [1, 3]
| 0 |
hf_public_repos/diffusers/tests | hf_public_repos/diffusers/tests/others/test_outputs.py | import unittest
from dataclasses import dataclass
from typing import List, Union
import numpy as np
import PIL.Image
from diffusers.utils.outputs import BaseOutput
@dataclass
class CustomOutput(BaseOutput):
images: Union[List[PIL.Image.Image], np.ndarray]
class ConfigTester(unittest.TestCase):
def test_outputs_single_attribute(self):
outputs = CustomOutput(images=np.random.rand(1, 3, 4, 4))
# check every way of getting the attribute
assert isinstance(outputs.images, np.ndarray)
assert outputs.images.shape == (1, 3, 4, 4)
assert isinstance(outputs["images"], np.ndarray)
assert outputs["images"].shape == (1, 3, 4, 4)
assert isinstance(outputs[0], np.ndarray)
assert outputs[0].shape == (1, 3, 4, 4)
# test with a non-tensor attribute
outputs = CustomOutput(images=[PIL.Image.new("RGB", (4, 4))])
# check every way of getting the attribute
assert isinstance(outputs.images, list)
assert isinstance(outputs.images[0], PIL.Image.Image)
assert isinstance(outputs["images"], list)
assert isinstance(outputs["images"][0], PIL.Image.Image)
assert isinstance(outputs[0], list)
assert isinstance(outputs[0][0], PIL.Image.Image)
def test_outputs_dict_init(self):
# test output reinitialization with a `dict` for compatibility with `accelerate`
outputs = CustomOutput({"images": np.random.rand(1, 3, 4, 4)})
# check every way of getting the attribute
assert isinstance(outputs.images, np.ndarray)
assert outputs.images.shape == (1, 3, 4, 4)
assert isinstance(outputs["images"], np.ndarray)
assert outputs["images"].shape == (1, 3, 4, 4)
assert isinstance(outputs[0], np.ndarray)
assert outputs[0].shape == (1, 3, 4, 4)
# test with a non-tensor attribute
outputs = CustomOutput({"images": [PIL.Image.new("RGB", (4, 4))]})
# check every way of getting the attribute
assert isinstance(outputs.images, list)
assert isinstance(outputs.images[0], PIL.Image.Image)
assert isinstance(outputs["images"], list)
assert isinstance(outputs["images"][0], PIL.Image.Image)
assert isinstance(outputs[0], list)
assert isinstance(outputs[0][0], PIL.Image.Image)
| 0 |
hf_public_repos/diffusers/tests | hf_public_repos/diffusers/tests/others/test_check_dummies.py | # Copyright 2023 The HuggingFace Team. All rights reserved.
#
# Licensed under the Apache License, Version 2.0 (the "License");
# you may not use this file except in compliance with the License.
# You may obtain a copy of the License at
#
# http://www.apache.org/licenses/LICENSE-2.0
#
# Unless required by applicable law or agreed to in writing, software
# distributed under the License is distributed on an "AS IS" BASIS,
# WITHOUT WARRANTIES OR CONDITIONS OF ANY KIND, either express or implied.
# See the License for the specific language governing permissions and
# limitations under the License.
import os
import sys
import unittest
git_repo_path = os.path.abspath(os.path.dirname(os.path.dirname(os.path.dirname(__file__))))
sys.path.append(os.path.join(git_repo_path, "utils"))
import check_dummies # noqa: E402
from check_dummies import create_dummy_files, create_dummy_object, find_backend, read_init # noqa: E402
# Align TRANSFORMERS_PATH in check_dummies with the current path
check_dummies.PATH_TO_DIFFUSERS = os.path.join(git_repo_path, "src", "diffusers")
class CheckDummiesTester(unittest.TestCase):
def test_find_backend(self):
simple_backend = find_backend(" if not is_torch_available():")
self.assertEqual(simple_backend, "torch")
# backend_with_underscore = find_backend(" if not is_tensorflow_text_available():")
# self.assertEqual(backend_with_underscore, "tensorflow_text")
double_backend = find_backend(" if not (is_torch_available() and is_transformers_available()):")
self.assertEqual(double_backend, "torch_and_transformers")
# double_backend_with_underscore = find_backend(
# " if not (is_sentencepiece_available() and is_tensorflow_text_available()):"
# )
# self.assertEqual(double_backend_with_underscore, "sentencepiece_and_tensorflow_text")
triple_backend = find_backend(
" if not (is_torch_available() and is_transformers_available() and is_onnx_available()):"
)
self.assertEqual(triple_backend, "torch_and_transformers_and_onnx")
def test_read_init(self):
objects = read_init()
# We don't assert on the exact list of keys to allow for smooth grow of backend-specific objects
self.assertIn("torch", objects)
self.assertIn("torch_and_transformers", objects)
self.assertIn("flax_and_transformers", objects)
self.assertIn("torch_and_transformers_and_onnx", objects)
# Likewise, we can't assert on the exact content of a key
self.assertIn("UNet2DModel", objects["torch"])
self.assertIn("FlaxUNet2DConditionModel", objects["flax"])
self.assertIn("StableDiffusionPipeline", objects["torch_and_transformers"])
self.assertIn("FlaxStableDiffusionPipeline", objects["flax_and_transformers"])
self.assertIn("LMSDiscreteScheduler", objects["torch_and_scipy"])
self.assertIn("OnnxStableDiffusionPipeline", objects["torch_and_transformers_and_onnx"])
def test_create_dummy_object(self):
dummy_constant = create_dummy_object("CONSTANT", "'torch'")
self.assertEqual(dummy_constant, "\nCONSTANT = None\n")
dummy_function = create_dummy_object("function", "'torch'")
self.assertEqual(
dummy_function, "\ndef function(*args, **kwargs):\n requires_backends(function, 'torch')\n"
)
expected_dummy_class = """
class FakeClass(metaclass=DummyObject):
_backends = 'torch'
def __init__(self, *args, **kwargs):
requires_backends(self, 'torch')
@classmethod
def from_config(cls, *args, **kwargs):
requires_backends(cls, 'torch')
@classmethod
def from_pretrained(cls, *args, **kwargs):
requires_backends(cls, 'torch')
"""
dummy_class = create_dummy_object("FakeClass", "'torch'")
self.assertEqual(dummy_class, expected_dummy_class)
def test_create_dummy_files(self):
expected_dummy_pytorch_file = """# This file is autogenerated by the command `make fix-copies`, do not edit.
from ..utils import DummyObject, requires_backends
CONSTANT = None
def function(*args, **kwargs):
requires_backends(function, ["torch"])
class FakeClass(metaclass=DummyObject):
_backends = ["torch"]
def __init__(self, *args, **kwargs):
requires_backends(self, ["torch"])
@classmethod
def from_config(cls, *args, **kwargs):
requires_backends(cls, ["torch"])
@classmethod
def from_pretrained(cls, *args, **kwargs):
requires_backends(cls, ["torch"])
"""
dummy_files = create_dummy_files({"torch": ["CONSTANT", "function", "FakeClass"]})
self.assertEqual(dummy_files["torch"], expected_dummy_pytorch_file)
| 0 |
hf_public_repos/diffusers/tests | hf_public_repos/diffusers/tests/others/test_utils.py | # coding=utf-8
# Copyright 2023 HuggingFace Inc.
#
# Licensed under the Apache License, Version 2.0 (the "License");
# you may not use this file except in compliance with the License.
# You may obtain a copy of the License at
#
# http://www.apache.org/licenses/LICENSE-2.0
#
# Unless required by applicable law or agreed to in writing, software
# distributed under the License is distributed on an "AS IS" BASIS,
# WITHOUT WARRANTIES OR CONDITIONS OF ANY KIND, either express or implied.
# See the License for the specific language governing permissions and
# limitations under the License.
import unittest
from diffusers import __version__
from diffusers.utils import deprecate
class DeprecateTester(unittest.TestCase):
higher_version = ".".join([str(int(__version__.split(".")[0]) + 1)] + __version__.split(".")[1:])
lower_version = "0.0.1"
def test_deprecate_function_arg(self):
kwargs = {"deprecated_arg": 4}
with self.assertWarns(FutureWarning) as warning:
output = deprecate("deprecated_arg", self.higher_version, "message", take_from=kwargs)
assert output == 4
assert (
str(warning.warning)
== f"The `deprecated_arg` argument is deprecated and will be removed in version {self.higher_version}."
" message"
)
def test_deprecate_function_arg_tuple(self):
kwargs = {"deprecated_arg": 4}
with self.assertWarns(FutureWarning) as warning:
output = deprecate(("deprecated_arg", self.higher_version, "message"), take_from=kwargs)
assert output == 4
assert (
str(warning.warning)
== f"The `deprecated_arg` argument is deprecated and will be removed in version {self.higher_version}."
" message"
)
def test_deprecate_function_args(self):
kwargs = {"deprecated_arg_1": 4, "deprecated_arg_2": 8}
with self.assertWarns(FutureWarning) as warning:
output_1, output_2 = deprecate(
("deprecated_arg_1", self.higher_version, "Hey"),
("deprecated_arg_2", self.higher_version, "Hey"),
take_from=kwargs,
)
assert output_1 == 4
assert output_2 == 8
assert (
str(warning.warnings[0].message)
== "The `deprecated_arg_1` argument is deprecated and will be removed in version"
f" {self.higher_version}. Hey"
)
assert (
str(warning.warnings[1].message)
== "The `deprecated_arg_2` argument is deprecated and will be removed in version"
f" {self.higher_version}. Hey"
)
def test_deprecate_function_incorrect_arg(self):
kwargs = {"deprecated_arg": 4}
with self.assertRaises(TypeError) as error:
deprecate(("wrong_arg", self.higher_version, "message"), take_from=kwargs)
assert "test_deprecate_function_incorrect_arg in" in str(error.exception)
assert "line" in str(error.exception)
assert "got an unexpected keyword argument `deprecated_arg`" in str(error.exception)
def test_deprecate_arg_no_kwarg(self):
with self.assertWarns(FutureWarning) as warning:
deprecate(("deprecated_arg", self.higher_version, "message"))
assert (
str(warning.warning)
== f"`deprecated_arg` is deprecated and will be removed in version {self.higher_version}. message"
)
def test_deprecate_args_no_kwarg(self):
with self.assertWarns(FutureWarning) as warning:
deprecate(
("deprecated_arg_1", self.higher_version, "Hey"),
("deprecated_arg_2", self.higher_version, "Hey"),
)
assert (
str(warning.warnings[0].message)
== f"`deprecated_arg_1` is deprecated and will be removed in version {self.higher_version}. Hey"
)
assert (
str(warning.warnings[1].message)
== f"`deprecated_arg_2` is deprecated and will be removed in version {self.higher_version}. Hey"
)
def test_deprecate_class_obj(self):
class Args:
arg = 5
with self.assertWarns(FutureWarning) as warning:
arg = deprecate(("arg", self.higher_version, "message"), take_from=Args())
assert arg == 5
assert (
str(warning.warning)
== f"The `arg` attribute is deprecated and will be removed in version {self.higher_version}. message"
)
def test_deprecate_class_objs(self):
class Args:
arg = 5
foo = 7
with self.assertWarns(FutureWarning) as warning:
arg_1, arg_2 = deprecate(
("arg", self.higher_version, "message"),
("foo", self.higher_version, "message"),
("does not exist", self.higher_version, "message"),
take_from=Args(),
)
assert arg_1 == 5
assert arg_2 == 7
assert (
str(warning.warning)
== f"The `arg` attribute is deprecated and will be removed in version {self.higher_version}. message"
)
assert (
str(warning.warnings[0].message)
== f"The `arg` attribute is deprecated and will be removed in version {self.higher_version}. message"
)
assert (
str(warning.warnings[1].message)
== f"The `foo` attribute is deprecated and will be removed in version {self.higher_version}. message"
)
def test_deprecate_incorrect_version(self):
kwargs = {"deprecated_arg": 4}
with self.assertRaises(ValueError) as error:
deprecate(("wrong_arg", self.lower_version, "message"), take_from=kwargs)
assert (
str(error.exception)
== "The deprecation tuple ('wrong_arg', '0.0.1', 'message') should be removed since diffusers' version"
f" {__version__} is >= {self.lower_version}"
)
def test_deprecate_incorrect_no_standard_warn(self):
with self.assertWarns(FutureWarning) as warning:
deprecate(("deprecated_arg", self.higher_version, "This message is better!!!"), standard_warn=False)
assert str(warning.warning) == "This message is better!!!"
def test_deprecate_stacklevel(self):
with self.assertWarns(FutureWarning) as warning:
deprecate(("deprecated_arg", self.higher_version, "This message is better!!!"), standard_warn=False)
assert str(warning.warning) == "This message is better!!!"
assert "diffusers/tests/others/test_utils.py" in warning.filename
| 0 |
hf_public_repos/diffusers/tests | hf_public_repos/diffusers/tests/others/test_image_processor.py | # coding=utf-8
# Copyright 2023 HuggingFace Inc.
#
# Licensed under the Apache License, Version 2.0 (the "License");
# you may not use this file except in compliance with the License.
# You may obtain a copy of the License at
#
# http://www.apache.org/licenses/LICENSE-2.0
#
# Unless required by applicable law or agreed to in writing, software
# distributed under the License is distributed on an "AS IS" BASIS,
# WITHOUT WARRANTIES OR CONDITIONS OF ANY KIND, either express or implied.
# See the License for the specific language governing permissions and
# limitations under the License.
import unittest
import numpy as np
import PIL
import torch
from diffusers.image_processor import VaeImageProcessor
class ImageProcessorTest(unittest.TestCase):
@property
def dummy_sample(self):
batch_size = 1
num_channels = 3
height = 8
width = 8
sample = torch.rand((batch_size, num_channels, height, width))
return sample
def to_np(self, image):
if isinstance(image[0], PIL.Image.Image):
return np.stack([np.array(i) for i in image], axis=0)
elif isinstance(image, torch.Tensor):
return image.cpu().numpy().transpose(0, 2, 3, 1)
return image
def test_vae_image_processor_pt(self):
image_processor = VaeImageProcessor(do_resize=False, do_normalize=True)
input_pt = self.dummy_sample
input_np = self.to_np(input_pt)
for output_type in ["pt", "np", "pil"]:
out = image_processor.postprocess(
image_processor.preprocess(input_pt),
output_type=output_type,
)
out_np = self.to_np(out)
in_np = (input_np * 255).round() if output_type == "pil" else input_np
assert (
np.abs(in_np - out_np).max() < 1e-6
), f"decoded output does not match input for output_type {output_type}"
def test_vae_image_processor_np(self):
image_processor = VaeImageProcessor(do_resize=False, do_normalize=True)
input_np = self.dummy_sample.cpu().numpy().transpose(0, 2, 3, 1)
for output_type in ["pt", "np", "pil"]:
out = image_processor.postprocess(image_processor.preprocess(input_np), output_type=output_type)
out_np = self.to_np(out)
in_np = (input_np * 255).round() if output_type == "pil" else input_np
assert (
np.abs(in_np - out_np).max() < 1e-6
), f"decoded output does not match input for output_type {output_type}"
def test_vae_image_processor_pil(self):
image_processor = VaeImageProcessor(do_resize=False, do_normalize=True)
input_np = self.dummy_sample.cpu().numpy().transpose(0, 2, 3, 1)
input_pil = image_processor.numpy_to_pil(input_np)
for output_type in ["pt", "np", "pil"]:
out = image_processor.postprocess(image_processor.preprocess(input_pil), output_type=output_type)
for i, o in zip(input_pil, out):
in_np = np.array(i)
out_np = self.to_np(out) if output_type == "pil" else (self.to_np(out) * 255).round()
assert (
np.abs(in_np - out_np).max() < 1e-6
), f"decoded output does not match input for output_type {output_type}"
def test_preprocess_input_3d(self):
image_processor = VaeImageProcessor(do_resize=False, do_normalize=False)
input_pt_4d = self.dummy_sample
input_pt_3d = input_pt_4d.squeeze(0)
out_pt_4d = image_processor.postprocess(
image_processor.preprocess(input_pt_4d),
output_type="np",
)
out_pt_3d = image_processor.postprocess(
image_processor.preprocess(input_pt_3d),
output_type="np",
)
input_np_4d = self.to_np(self.dummy_sample)
input_np_3d = input_np_4d.squeeze(0)
out_np_4d = image_processor.postprocess(
image_processor.preprocess(input_np_4d),
output_type="np",
)
out_np_3d = image_processor.postprocess(
image_processor.preprocess(input_np_3d),
output_type="np",
)
assert np.abs(out_pt_4d - out_pt_3d).max() < 1e-6
assert np.abs(out_np_4d - out_np_3d).max() < 1e-6
def test_preprocess_input_list(self):
image_processor = VaeImageProcessor(do_resize=False, do_normalize=False)
input_pt_4d = self.dummy_sample
input_pt_list = list(input_pt_4d)
out_pt_4d = image_processor.postprocess(
image_processor.preprocess(input_pt_4d),
output_type="np",
)
out_pt_list = image_processor.postprocess(
image_processor.preprocess(input_pt_list),
output_type="np",
)
input_np_4d = self.to_np(self.dummy_sample)
list(input_np_4d)
out_np_4d = image_processor.postprocess(
image_processor.preprocess(input_pt_4d),
output_type="np",
)
out_np_list = image_processor.postprocess(
image_processor.preprocess(input_pt_list),
output_type="np",
)
assert np.abs(out_pt_4d - out_pt_list).max() < 1e-6
assert np.abs(out_np_4d - out_np_list).max() < 1e-6
| 0 |
hf_public_repos/diffusers/tests | hf_public_repos/diffusers/tests/others/test_hub_utils.py | # coding=utf-8
# Copyright 2023 HuggingFace Inc.
#
# Licensed under the Apache License, Version 2.0 (the "License");
# you may not use this file except in compliance with the License.
# You may obtain a copy of the License at
#
# http://www.apache.org/licenses/LICENSE-2.0
#
# Unless required by applicable law or agreed to in writing, software
# distributed under the License is distributed on an "AS IS" BASIS,
# WITHOUT WARRANTIES OR CONDITIONS OF ANY KIND, either express or implied.
# See the License for the specific language governing permissions and
# limitations under the License.
import unittest
from pathlib import Path
from tempfile import TemporaryDirectory
from unittest.mock import Mock, patch
import diffusers.utils.hub_utils
class CreateModelCardTest(unittest.TestCase):
@patch("diffusers.utils.hub_utils.get_full_repo_name")
def test_create_model_card(self, repo_name_mock: Mock) -> None:
repo_name_mock.return_value = "full_repo_name"
with TemporaryDirectory() as tmpdir:
# Dummy args values
args = Mock()
args.output_dir = tmpdir
args.local_rank = 0
args.hub_token = "hub_token"
args.dataset_name = "dataset_name"
args.learning_rate = 0.01
args.train_batch_size = 100000
args.eval_batch_size = 10000
args.gradient_accumulation_steps = 0.01
args.adam_beta1 = 0.02
args.adam_beta2 = 0.03
args.adam_weight_decay = 0.0005
args.adam_epsilon = 0.000001
args.lr_scheduler = 1
args.lr_warmup_steps = 10
args.ema_inv_gamma = 0.001
args.ema_power = 0.1
args.ema_max_decay = 0.2
args.mixed_precision = True
# Model card mush be rendered and saved
diffusers.utils.hub_utils.create_model_card(args, model_name="model_name")
self.assertTrue((Path(tmpdir) / "README.md").is_file())
| 0 |
hf_public_repos/diffusers/tests/fixtures | hf_public_repos/diffusers/tests/fixtures/custom_pipeline/what_ever.py | # Copyright 2023 The HuggingFace Team. All rights reserved.
#
# Licensed under the Apache License, Version 2.0 (the "License");
# you may not use this file except in compliance with the License.
# You may obtain a copy of the License at
#
# http://www.apache.org/licenses/LICENSE-2.0
#
# Unless required by applicable law or agreed to in writing, software
# distributed under the License is distributed on an "AS IS" BASIS,
# WITHOUT WARRANTIES OR CONDITIONS OF ANY KIND, either express or implied.
# See the License for the specific language governing permissions and
# limitations under the License.
from typing import Optional, Tuple, Union
import torch
from diffusers.pipeline_utils import DiffusionPipeline, ImagePipelineOutput
class CustomLocalPipeline(DiffusionPipeline):
r"""
This model inherits from [`DiffusionPipeline`]. Check the superclass documentation for the generic methods the
library implements for all the pipelines (such as downloading or saving, running on a particular device, etc.)
Parameters:
unet ([`UNet2DModel`]): U-Net architecture to denoise the encoded image.
scheduler ([`SchedulerMixin`]):
A scheduler to be used in combination with `unet` to denoise the encoded image. Can be one of
[`DDPMScheduler`], or [`DDIMScheduler`].
"""
def __init__(self, unet, scheduler):
super().__init__()
self.register_modules(unet=unet, scheduler=scheduler)
@torch.no_grad()
def __call__(
self,
batch_size: int = 1,
generator: Optional[torch.Generator] = None,
num_inference_steps: int = 50,
output_type: Optional[str] = "pil",
return_dict: bool = True,
**kwargs,
) -> Union[ImagePipelineOutput, Tuple]:
r"""
Args:
batch_size (`int`, *optional*, defaults to 1):
The number of images to generate.
generator (`torch.Generator`, *optional*):
A [torch generator](https://pytorch.org/docs/stable/generated/torch.Generator.html) to make generation
deterministic.
eta (`float`, *optional*, defaults to 0.0):
The eta parameter which controls the scale of the variance (0 is DDIM and 1 is one type of DDPM).
num_inference_steps (`int`, *optional*, defaults to 50):
The number of denoising steps. More denoising steps usually lead to a higher quality image at the
expense of slower inference.
output_type (`str`, *optional*, defaults to `"pil"`):
The output format of the generate image. Choose between
[PIL](https://pillow.readthedocs.io/en/stable/): `PIL.Image.Image` or `np.array`.
return_dict (`bool`, *optional*, defaults to `True`):
Whether or not to return a [`~pipeline_utils.ImagePipelineOutput`] instead of a plain tuple.
Returns:
[`~pipeline_utils.ImagePipelineOutput`] or `tuple`: [`~pipelines.utils.ImagePipelineOutput`] if
`return_dict` is True, otherwise a `tuple. When returning a tuple, the first element is a list with the
generated images.
"""
# Sample gaussian noise to begin loop
image = torch.randn(
(batch_size, self.unet.config.in_channels, self.unet.config.sample_size, self.unet.config.sample_size),
generator=generator,
)
image = image.to(self.device)
# set step values
self.scheduler.set_timesteps(num_inference_steps)
for t in self.progress_bar(self.scheduler.timesteps):
# 1. predict noise model_output
model_output = self.unet(image, t).sample
# 2. predict previous mean of image x_t-1 and add variance depending on eta
# eta corresponds to η in paper and should be between [0, 1]
# do x_t -> x_t-1
image = self.scheduler.step(model_output, t, image).prev_sample
image = (image / 2 + 0.5).clamp(0, 1)
image = image.cpu().permute(0, 2, 3, 1).numpy()
if output_type == "pil":
image = self.numpy_to_pil(image)
if not return_dict:
return (image,), "This is a local test"
return ImagePipelineOutput(images=image), "This is a local test"
| 0 |
hf_public_repos/diffusers/tests/fixtures | hf_public_repos/diffusers/tests/fixtures/custom_pipeline/pipeline.py | # Copyright 2023 The HuggingFace Team. All rights reserved.
#
# Licensed under the Apache License, Version 2.0 (the "License");
# you may not use this file except in compliance with the License.
# You may obtain a copy of the License at
#
# http://www.apache.org/licenses/LICENSE-2.0
#
# Unless required by applicable law or agreed to in writing, software
# distributed under the License is distributed on an "AS IS" BASIS,
# WITHOUT WARRANTIES OR CONDITIONS OF ANY KIND, either express or implied.
# See the License for the specific language governing permissions and
# limitations under the License.
from typing import Optional, Tuple, Union
import torch
from diffusers import DiffusionPipeline, ImagePipelineOutput
class CustomLocalPipeline(DiffusionPipeline):
r"""
This model inherits from [`DiffusionPipeline`]. Check the superclass documentation for the generic methods the
library implements for all the pipelines (such as downloading or saving, running on a particular device, etc.)
Parameters:
unet ([`UNet2DModel`]): U-Net architecture to denoise the encoded image.
scheduler ([`SchedulerMixin`]):
A scheduler to be used in combination with `unet` to denoise the encoded image. Can be one of
[`DDPMScheduler`], or [`DDIMScheduler`].
"""
def __init__(self, unet, scheduler):
super().__init__()
self.register_modules(unet=unet, scheduler=scheduler)
@torch.no_grad()
def __call__(
self,
batch_size: int = 1,
generator: Optional[torch.Generator] = None,
num_inference_steps: int = 50,
output_type: Optional[str] = "pil",
return_dict: bool = True,
**kwargs,
) -> Union[ImagePipelineOutput, Tuple]:
r"""
Args:
batch_size (`int`, *optional*, defaults to 1):
The number of images to generate.
generator (`torch.Generator`, *optional*):
A [torch generator](https://pytorch.org/docs/stable/generated/torch.Generator.html) to make generation
deterministic.
eta (`float`, *optional*, defaults to 0.0):
The eta parameter which controls the scale of the variance (0 is DDIM and 1 is one type of DDPM).
num_inference_steps (`int`, *optional*, defaults to 50):
The number of denoising steps. More denoising steps usually lead to a higher quality image at the
expense of slower inference.
output_type (`str`, *optional*, defaults to `"pil"`):
The output format of the generate image. Choose between
[PIL](https://pillow.readthedocs.io/en/stable/): `PIL.Image.Image` or `np.array`.
return_dict (`bool`, *optional*, defaults to `True`):
Whether or not to return a [`~pipelines.ImagePipelineOutput`] instead of a plain tuple.
Returns:
[`~pipelines.ImagePipelineOutput`] or `tuple`: [`~pipelines.utils.ImagePipelineOutput`] if
`return_dict` is True, otherwise a `tuple. When returning a tuple, the first element is a list with the
generated images.
"""
# Sample gaussian noise to begin loop
image = torch.randn(
(batch_size, self.unet.config.in_channels, self.unet.config.sample_size, self.unet.config.sample_size),
generator=generator,
)
image = image.to(self.device)
# set step values
self.scheduler.set_timesteps(num_inference_steps)
for t in self.progress_bar(self.scheduler.timesteps):
# 1. predict noise model_output
model_output = self.unet(image, t).sample
# 2. predict previous mean of image x_t-1 and add variance depending on eta
# eta corresponds to η in paper and should be between [0, 1]
# do x_t -> x_t-1
image = self.scheduler.step(model_output, t, image).prev_sample
image = (image / 2 + 0.5).clamp(0, 1)
image = image.cpu().permute(0, 2, 3, 1).numpy()
if output_type == "pil":
image = self.numpy_to_pil(image)
if not return_dict:
return (image,), "This is a local test"
return ImagePipelineOutput(images=image), "This is a local test"
| 0 |
hf_public_repos/diffusers/tests | hf_public_repos/diffusers/tests/schedulers/test_scheduler_deis.py | import tempfile
import torch
from diffusers import (
DEISMultistepScheduler,
DPMSolverMultistepScheduler,
DPMSolverSinglestepScheduler,
UniPCMultistepScheduler,
)
from .test_schedulers import SchedulerCommonTest
class DEISMultistepSchedulerTest(SchedulerCommonTest):
scheduler_classes = (DEISMultistepScheduler,)
forward_default_kwargs = (("num_inference_steps", 25),)
def get_scheduler_config(self, **kwargs):
config = {
"num_train_timesteps": 1000,
"beta_start": 0.0001,
"beta_end": 0.02,
"beta_schedule": "linear",
"solver_order": 2,
}
config.update(**kwargs)
return config
def check_over_configs(self, time_step=0, **config):
kwargs = dict(self.forward_default_kwargs)
num_inference_steps = kwargs.pop("num_inference_steps", None)
sample = self.dummy_sample
residual = 0.1 * sample
dummy_past_residuals = [residual + 0.2, residual + 0.15, residual + 0.10]
for scheduler_class in self.scheduler_classes:
scheduler_config = self.get_scheduler_config(**config)
scheduler = scheduler_class(**scheduler_config)
scheduler.set_timesteps(num_inference_steps)
# copy over dummy past residuals
scheduler.model_outputs = dummy_past_residuals[: scheduler.config.solver_order]
with tempfile.TemporaryDirectory() as tmpdirname:
scheduler.save_config(tmpdirname)
new_scheduler = scheduler_class.from_pretrained(tmpdirname)
new_scheduler.set_timesteps(num_inference_steps)
# copy over dummy past residuals
new_scheduler.model_outputs = dummy_past_residuals[: new_scheduler.config.solver_order]
output, new_output = sample, sample
for t in range(time_step, time_step + scheduler.config.solver_order + 1):
output = scheduler.step(residual, t, output, **kwargs).prev_sample
new_output = new_scheduler.step(residual, t, new_output, **kwargs).prev_sample
assert torch.sum(torch.abs(output - new_output)) < 1e-5, "Scheduler outputs are not identical"
def test_from_save_pretrained(self):
pass
def check_over_forward(self, time_step=0, **forward_kwargs):
kwargs = dict(self.forward_default_kwargs)
num_inference_steps = kwargs.pop("num_inference_steps", None)
sample = self.dummy_sample
residual = 0.1 * sample
dummy_past_residuals = [residual + 0.2, residual + 0.15, residual + 0.10]
for scheduler_class in self.scheduler_classes:
scheduler_config = self.get_scheduler_config()
scheduler = scheduler_class(**scheduler_config)
scheduler.set_timesteps(num_inference_steps)
# copy over dummy past residuals (must be after setting timesteps)
scheduler.model_outputs = dummy_past_residuals[: scheduler.config.solver_order]
with tempfile.TemporaryDirectory() as tmpdirname:
scheduler.save_config(tmpdirname)
new_scheduler = scheduler_class.from_pretrained(tmpdirname)
# copy over dummy past residuals
new_scheduler.set_timesteps(num_inference_steps)
# copy over dummy past residual (must be after setting timesteps)
new_scheduler.model_outputs = dummy_past_residuals[: new_scheduler.config.solver_order]
output = scheduler.step(residual, time_step, sample, **kwargs).prev_sample
new_output = new_scheduler.step(residual, time_step, sample, **kwargs).prev_sample
assert torch.sum(torch.abs(output - new_output)) < 1e-5, "Scheduler outputs are not identical"
def full_loop(self, scheduler=None, **config):
if scheduler is None:
scheduler_class = self.scheduler_classes[0]
scheduler_config = self.get_scheduler_config(**config)
scheduler = scheduler_class(**scheduler_config)
scheduler_class = self.scheduler_classes[0]
scheduler_config = self.get_scheduler_config(**config)
scheduler = scheduler_class(**scheduler_config)
num_inference_steps = 10
model = self.dummy_model()
sample = self.dummy_sample_deter
scheduler.set_timesteps(num_inference_steps)
for i, t in enumerate(scheduler.timesteps):
residual = model(sample, t)
sample = scheduler.step(residual, t, sample).prev_sample
return sample
def test_step_shape(self):
kwargs = dict(self.forward_default_kwargs)
num_inference_steps = kwargs.pop("num_inference_steps", None)
for scheduler_class in self.scheduler_classes:
scheduler_config = self.get_scheduler_config()
scheduler = scheduler_class(**scheduler_config)
sample = self.dummy_sample
residual = 0.1 * sample
if num_inference_steps is not None and hasattr(scheduler, "set_timesteps"):
scheduler.set_timesteps(num_inference_steps)
elif num_inference_steps is not None and not hasattr(scheduler, "set_timesteps"):
kwargs["num_inference_steps"] = num_inference_steps
# copy over dummy past residuals (must be done after set_timesteps)
dummy_past_residuals = [residual + 0.2, residual + 0.15, residual + 0.10]
scheduler.model_outputs = dummy_past_residuals[: scheduler.config.solver_order]
time_step_0 = scheduler.timesteps[5]
time_step_1 = scheduler.timesteps[6]
output_0 = scheduler.step(residual, time_step_0, sample, **kwargs).prev_sample
output_1 = scheduler.step(residual, time_step_1, sample, **kwargs).prev_sample
self.assertEqual(output_0.shape, sample.shape)
self.assertEqual(output_0.shape, output_1.shape)
def test_switch(self):
# make sure that iterating over schedulers with same config names gives same results
# for defaults
scheduler = DEISMultistepScheduler(**self.get_scheduler_config())
sample = self.full_loop(scheduler=scheduler)
result_mean = torch.mean(torch.abs(sample))
assert abs(result_mean.item() - 0.23916) < 1e-3
scheduler = DPMSolverSinglestepScheduler.from_config(scheduler.config)
scheduler = DPMSolverMultistepScheduler.from_config(scheduler.config)
scheduler = UniPCMultistepScheduler.from_config(scheduler.config)
scheduler = DEISMultistepScheduler.from_config(scheduler.config)
sample = self.full_loop(scheduler=scheduler)
result_mean = torch.mean(torch.abs(sample))
assert abs(result_mean.item() - 0.23916) < 1e-3
def test_timesteps(self):
for timesteps in [25, 50, 100, 999, 1000]:
self.check_over_configs(num_train_timesteps=timesteps)
def test_thresholding(self):
self.check_over_configs(thresholding=False)
for order in [1, 2, 3]:
for solver_type in ["logrho"]:
for threshold in [0.5, 1.0, 2.0]:
for prediction_type in ["epsilon", "sample"]:
self.check_over_configs(
thresholding=True,
prediction_type=prediction_type,
sample_max_value=threshold,
algorithm_type="deis",
solver_order=order,
solver_type=solver_type,
)
def test_prediction_type(self):
for prediction_type in ["epsilon", "v_prediction"]:
self.check_over_configs(prediction_type=prediction_type)
def test_solver_order_and_type(self):
for algorithm_type in ["deis"]:
for solver_type in ["logrho"]:
for order in [1, 2, 3]:
for prediction_type in ["epsilon", "sample"]:
self.check_over_configs(
solver_order=order,
solver_type=solver_type,
prediction_type=prediction_type,
algorithm_type=algorithm_type,
)
sample = self.full_loop(
solver_order=order,
solver_type=solver_type,
prediction_type=prediction_type,
algorithm_type=algorithm_type,
)
assert not torch.isnan(sample).any(), "Samples have nan numbers"
def test_lower_order_final(self):
self.check_over_configs(lower_order_final=True)
self.check_over_configs(lower_order_final=False)
def test_inference_steps(self):
for num_inference_steps in [1, 2, 3, 5, 10, 50, 100, 999, 1000]:
self.check_over_forward(num_inference_steps=num_inference_steps, time_step=0)
def test_full_loop_no_noise(self):
sample = self.full_loop()
result_mean = torch.mean(torch.abs(sample))
assert abs(result_mean.item() - 0.23916) < 1e-3
def test_full_loop_with_v_prediction(self):
sample = self.full_loop(prediction_type="v_prediction")
result_mean = torch.mean(torch.abs(sample))
assert abs(result_mean.item() - 0.091) < 1e-3
def test_fp16_support(self):
scheduler_class = self.scheduler_classes[0]
scheduler_config = self.get_scheduler_config(thresholding=True, dynamic_thresholding_ratio=0)
scheduler = scheduler_class(**scheduler_config)
num_inference_steps = 10
model = self.dummy_model()
sample = self.dummy_sample_deter.half()
scheduler.set_timesteps(num_inference_steps)
for i, t in enumerate(scheduler.timesteps):
residual = model(sample, t)
sample = scheduler.step(residual, t, sample).prev_sample
assert sample.dtype == torch.float16
| 0 |
hf_public_repos/diffusers/tests | hf_public_repos/diffusers/tests/schedulers/test_scheduler_dpm_multi.py | import tempfile
import torch
from diffusers import (
DEISMultistepScheduler,
DPMSolverMultistepScheduler,
DPMSolverSinglestepScheduler,
UniPCMultistepScheduler,
)
from .test_schedulers import SchedulerCommonTest
class DPMSolverMultistepSchedulerTest(SchedulerCommonTest):
scheduler_classes = (DPMSolverMultistepScheduler,)
forward_default_kwargs = (("num_inference_steps", 25),)
def get_scheduler_config(self, **kwargs):
config = {
"num_train_timesteps": 1000,
"beta_start": 0.0001,
"beta_end": 0.02,
"beta_schedule": "linear",
"solver_order": 2,
"prediction_type": "epsilon",
"thresholding": False,
"sample_max_value": 1.0,
"algorithm_type": "dpmsolver++",
"solver_type": "midpoint",
"lower_order_final": False,
"lambda_min_clipped": -float("inf"),
"variance_type": None,
}
config.update(**kwargs)
return config
def check_over_configs(self, time_step=0, **config):
kwargs = dict(self.forward_default_kwargs)
num_inference_steps = kwargs.pop("num_inference_steps", None)
sample = self.dummy_sample
residual = 0.1 * sample
dummy_past_residuals = [residual + 0.2, residual + 0.15, residual + 0.10]
for scheduler_class in self.scheduler_classes:
scheduler_config = self.get_scheduler_config(**config)
scheduler = scheduler_class(**scheduler_config)
scheduler.set_timesteps(num_inference_steps)
# copy over dummy past residuals
scheduler.model_outputs = dummy_past_residuals[: scheduler.config.solver_order]
with tempfile.TemporaryDirectory() as tmpdirname:
scheduler.save_config(tmpdirname)
new_scheduler = scheduler_class.from_pretrained(tmpdirname)
new_scheduler.set_timesteps(num_inference_steps)
# copy over dummy past residuals
new_scheduler.model_outputs = dummy_past_residuals[: new_scheduler.config.solver_order]
output, new_output = sample, sample
for t in range(time_step, time_step + scheduler.config.solver_order + 1):
output = scheduler.step(residual, t, output, **kwargs).prev_sample
new_output = new_scheduler.step(residual, t, new_output, **kwargs).prev_sample
assert torch.sum(torch.abs(output - new_output)) < 1e-5, "Scheduler outputs are not identical"
def test_from_save_pretrained(self):
pass
def check_over_forward(self, time_step=0, **forward_kwargs):
kwargs = dict(self.forward_default_kwargs)
num_inference_steps = kwargs.pop("num_inference_steps", None)
sample = self.dummy_sample
residual = 0.1 * sample
dummy_past_residuals = [residual + 0.2, residual + 0.15, residual + 0.10]
for scheduler_class in self.scheduler_classes:
scheduler_config = self.get_scheduler_config()
scheduler = scheduler_class(**scheduler_config)
scheduler.set_timesteps(num_inference_steps)
# copy over dummy past residuals (must be after setting timesteps)
scheduler.model_outputs = dummy_past_residuals[: scheduler.config.solver_order]
with tempfile.TemporaryDirectory() as tmpdirname:
scheduler.save_config(tmpdirname)
new_scheduler = scheduler_class.from_pretrained(tmpdirname)
# copy over dummy past residuals
new_scheduler.set_timesteps(num_inference_steps)
# copy over dummy past residual (must be after setting timesteps)
new_scheduler.model_outputs = dummy_past_residuals[: new_scheduler.config.solver_order]
output = scheduler.step(residual, time_step, sample, **kwargs).prev_sample
new_output = new_scheduler.step(residual, time_step, sample, **kwargs).prev_sample
assert torch.sum(torch.abs(output - new_output)) < 1e-5, "Scheduler outputs are not identical"
def full_loop(self, scheduler=None, **config):
if scheduler is None:
scheduler_class = self.scheduler_classes[0]
scheduler_config = self.get_scheduler_config(**config)
scheduler = scheduler_class(**scheduler_config)
num_inference_steps = 10
model = self.dummy_model()
sample = self.dummy_sample_deter
scheduler.set_timesteps(num_inference_steps)
for i, t in enumerate(scheduler.timesteps):
residual = model(sample, t)
sample = scheduler.step(residual, t, sample).prev_sample
return sample
def test_step_shape(self):
kwargs = dict(self.forward_default_kwargs)
num_inference_steps = kwargs.pop("num_inference_steps", None)
for scheduler_class in self.scheduler_classes:
scheduler_config = self.get_scheduler_config()
scheduler = scheduler_class(**scheduler_config)
sample = self.dummy_sample
residual = 0.1 * sample
if num_inference_steps is not None and hasattr(scheduler, "set_timesteps"):
scheduler.set_timesteps(num_inference_steps)
elif num_inference_steps is not None and not hasattr(scheduler, "set_timesteps"):
kwargs["num_inference_steps"] = num_inference_steps
# copy over dummy past residuals (must be done after set_timesteps)
dummy_past_residuals = [residual + 0.2, residual + 0.15, residual + 0.10]
scheduler.model_outputs = dummy_past_residuals[: scheduler.config.solver_order]
time_step_0 = scheduler.timesteps[5]
time_step_1 = scheduler.timesteps[6]
output_0 = scheduler.step(residual, time_step_0, sample, **kwargs).prev_sample
output_1 = scheduler.step(residual, time_step_1, sample, **kwargs).prev_sample
self.assertEqual(output_0.shape, sample.shape)
self.assertEqual(output_0.shape, output_1.shape)
def test_timesteps(self):
for timesteps in [25, 50, 100, 999, 1000]:
self.check_over_configs(num_train_timesteps=timesteps)
def test_thresholding(self):
self.check_over_configs(thresholding=False)
for order in [1, 2, 3]:
for solver_type in ["midpoint", "heun"]:
for threshold in [0.5, 1.0, 2.0]:
for prediction_type in ["epsilon", "sample"]:
self.check_over_configs(
thresholding=True,
prediction_type=prediction_type,
sample_max_value=threshold,
algorithm_type="dpmsolver++",
solver_order=order,
solver_type=solver_type,
)
def test_prediction_type(self):
for prediction_type in ["epsilon", "v_prediction"]:
self.check_over_configs(prediction_type=prediction_type)
def test_solver_order_and_type(self):
for algorithm_type in ["dpmsolver", "dpmsolver++", "sde-dpmsolver", "sde-dpmsolver++"]:
for solver_type in ["midpoint", "heun"]:
for order in [1, 2, 3]:
for prediction_type in ["epsilon", "sample"]:
if algorithm_type in ["sde-dpmsolver", "sde-dpmsolver++"]:
if order == 3:
continue
else:
self.check_over_configs(
solver_order=order,
solver_type=solver_type,
prediction_type=prediction_type,
algorithm_type=algorithm_type,
)
sample = self.full_loop(
solver_order=order,
solver_type=solver_type,
prediction_type=prediction_type,
algorithm_type=algorithm_type,
)
assert not torch.isnan(sample).any(), "Samples have nan numbers"
def test_lower_order_final(self):
self.check_over_configs(lower_order_final=True)
self.check_over_configs(lower_order_final=False)
def test_lambda_min_clipped(self):
self.check_over_configs(lambda_min_clipped=-float("inf"))
self.check_over_configs(lambda_min_clipped=-5.1)
def test_variance_type(self):
self.check_over_configs(variance_type=None)
self.check_over_configs(variance_type="learned_range")
def test_inference_steps(self):
for num_inference_steps in [1, 2, 3, 5, 10, 50, 100, 999, 1000]:
self.check_over_forward(num_inference_steps=num_inference_steps, time_step=0)
def test_full_loop_no_noise(self):
sample = self.full_loop()
result_mean = torch.mean(torch.abs(sample))
assert abs(result_mean.item() - 0.3301) < 1e-3
def test_full_loop_no_noise_thres(self):
sample = self.full_loop(thresholding=True, dynamic_thresholding_ratio=0.87, sample_max_value=0.5)
result_mean = torch.mean(torch.abs(sample))
assert abs(result_mean.item() - 1.1364) < 1e-3
def test_full_loop_with_v_prediction(self):
sample = self.full_loop(prediction_type="v_prediction")
result_mean = torch.mean(torch.abs(sample))
assert abs(result_mean.item() - 0.2251) < 1e-3
def test_full_loop_with_karras_and_v_prediction(self):
sample = self.full_loop(prediction_type="v_prediction", use_karras_sigmas=True)
result_mean = torch.mean(torch.abs(sample))
assert abs(result_mean.item() - 0.2096) < 1e-3
def test_switch(self):
# make sure that iterating over schedulers with same config names gives same results
# for defaults
scheduler = DPMSolverMultistepScheduler(**self.get_scheduler_config())
sample = self.full_loop(scheduler=scheduler)
result_mean = torch.mean(torch.abs(sample))
assert abs(result_mean.item() - 0.3301) < 1e-3
scheduler = DPMSolverSinglestepScheduler.from_config(scheduler.config)
scheduler = UniPCMultistepScheduler.from_config(scheduler.config)
scheduler = DEISMultistepScheduler.from_config(scheduler.config)
scheduler = DPMSolverMultistepScheduler.from_config(scheduler.config)
sample = self.full_loop(scheduler=scheduler)
result_mean = torch.mean(torch.abs(sample))
assert abs(result_mean.item() - 0.3301) < 1e-3
def test_fp16_support(self):
scheduler_class = self.scheduler_classes[0]
scheduler_config = self.get_scheduler_config(thresholding=True, dynamic_thresholding_ratio=0)
scheduler = scheduler_class(**scheduler_config)
num_inference_steps = 10
model = self.dummy_model()
sample = self.dummy_sample_deter.half()
scheduler.set_timesteps(num_inference_steps)
for i, t in enumerate(scheduler.timesteps):
residual = model(sample, t)
sample = scheduler.step(residual, t, sample).prev_sample
assert sample.dtype == torch.float16
def test_unique_timesteps(self, **config):
for scheduler_class in self.scheduler_classes:
scheduler_config = self.get_scheduler_config(**config)
scheduler = scheduler_class(**scheduler_config)
scheduler.set_timesteps(scheduler.config.num_train_timesteps)
assert len(scheduler.timesteps.unique()) == scheduler.num_inference_steps
| 0 |
hf_public_repos/diffusers/tests | hf_public_repos/diffusers/tests/schedulers/test_scheduler_kdpm2_ancestral.py | import torch
from diffusers import KDPM2AncestralDiscreteScheduler
from diffusers.utils import torch_device
from .test_schedulers import SchedulerCommonTest
class KDPM2AncestralDiscreteSchedulerTest(SchedulerCommonTest):
scheduler_classes = (KDPM2AncestralDiscreteScheduler,)
num_inference_steps = 10
def get_scheduler_config(self, **kwargs):
config = {
"num_train_timesteps": 1100,
"beta_start": 0.0001,
"beta_end": 0.02,
"beta_schedule": "linear",
}
config.update(**kwargs)
return config
def test_timesteps(self):
for timesteps in [10, 50, 100, 1000]:
self.check_over_configs(num_train_timesteps=timesteps)
def test_betas(self):
for beta_start, beta_end in zip([0.00001, 0.0001, 0.001], [0.0002, 0.002, 0.02]):
self.check_over_configs(beta_start=beta_start, beta_end=beta_end)
def test_schedules(self):
for schedule in ["linear", "scaled_linear"]:
self.check_over_configs(beta_schedule=schedule)
def test_full_loop_no_noise(self):
if torch_device == "mps":
return
scheduler_class = self.scheduler_classes[0]
scheduler_config = self.get_scheduler_config()
scheduler = scheduler_class(**scheduler_config)
scheduler.set_timesteps(self.num_inference_steps)
generator = torch.manual_seed(0)
model = self.dummy_model()
sample = self.dummy_sample_deter * scheduler.init_noise_sigma
sample = sample.to(torch_device)
for i, t in enumerate(scheduler.timesteps):
sample = scheduler.scale_model_input(sample, t)
model_output = model(sample, t)
output = scheduler.step(model_output, t, sample, generator=generator)
sample = output.prev_sample
result_sum = torch.sum(torch.abs(sample))
result_mean = torch.mean(torch.abs(sample))
assert abs(result_sum.item() - 13849.3877) < 1e-2
assert abs(result_mean.item() - 18.0331) < 5e-3
def test_prediction_type(self):
for prediction_type in ["epsilon", "v_prediction"]:
self.check_over_configs(prediction_type=prediction_type)
def test_full_loop_with_v_prediction(self):
if torch_device == "mps":
return
scheduler_class = self.scheduler_classes[0]
scheduler_config = self.get_scheduler_config(prediction_type="v_prediction")
scheduler = scheduler_class(**scheduler_config)
scheduler.set_timesteps(self.num_inference_steps)
model = self.dummy_model()
sample = self.dummy_sample_deter * scheduler.init_noise_sigma
sample = sample.to(torch_device)
generator = torch.manual_seed(0)
for i, t in enumerate(scheduler.timesteps):
sample = scheduler.scale_model_input(sample, t)
model_output = model(sample, t)
output = scheduler.step(model_output, t, sample, generator=generator)
sample = output.prev_sample
result_sum = torch.sum(torch.abs(sample))
result_mean = torch.mean(torch.abs(sample))
assert abs(result_sum.item() - 328.9970) < 1e-2
assert abs(result_mean.item() - 0.4284) < 1e-3
def test_full_loop_device(self):
if torch_device == "mps":
return
scheduler_class = self.scheduler_classes[0]
scheduler_config = self.get_scheduler_config()
scheduler = scheduler_class(**scheduler_config)
scheduler.set_timesteps(self.num_inference_steps, device=torch_device)
generator = torch.manual_seed(0)
model = self.dummy_model()
sample = self.dummy_sample_deter.to(torch_device) * scheduler.init_noise_sigma
for t in scheduler.timesteps:
sample = scheduler.scale_model_input(sample, t)
model_output = model(sample, t)
output = scheduler.step(model_output, t, sample, generator=generator)
sample = output.prev_sample
result_sum = torch.sum(torch.abs(sample))
result_mean = torch.mean(torch.abs(sample))
assert abs(result_sum.item() - 13849.3818) < 1e-1
assert abs(result_mean.item() - 18.0331) < 1e-3
| 0 |
hf_public_repos/diffusers/tests | hf_public_repos/diffusers/tests/schedulers/test_scheduler_ddpm_parallel.py | # Copyright 2023 ParaDiGMS authors and The HuggingFace Team. All rights reserved.
#
# Licensed under the Apache License, Version 2.0 (the "License");
# you may not use this file except in compliance with the License.
# You may obtain a copy of the License at
#
# http://www.apache.org/licenses/LICENSE-2.0
#
# Unless required by applicable law or agreed to in writing, software
# distributed under the License is distributed on an "AS IS" BASIS,
# WITHOUT WARRANTIES OR CONDITIONS OF ANY KIND, either express or implied.
# See the License for the specific language governing permissions and
# limitations under the License.
import torch
from diffusers import DDPMParallelScheduler
from .test_schedulers import SchedulerCommonTest
class DDPMParallelSchedulerTest(SchedulerCommonTest):
scheduler_classes = (DDPMParallelScheduler,)
def get_scheduler_config(self, **kwargs):
config = {
"num_train_timesteps": 1000,
"beta_start": 0.0001,
"beta_end": 0.02,
"beta_schedule": "linear",
"variance_type": "fixed_small",
"clip_sample": True,
}
config.update(**kwargs)
return config
def test_timesteps(self):
for timesteps in [1, 5, 100, 1000]:
self.check_over_configs(num_train_timesteps=timesteps)
def test_betas(self):
for beta_start, beta_end in zip([0.0001, 0.001, 0.01, 0.1], [0.002, 0.02, 0.2, 2]):
self.check_over_configs(beta_start=beta_start, beta_end=beta_end)
def test_schedules(self):
for schedule in ["linear", "squaredcos_cap_v2"]:
self.check_over_configs(beta_schedule=schedule)
def test_variance_type(self):
for variance in ["fixed_small", "fixed_large", "other"]:
self.check_over_configs(variance_type=variance)
def test_clip_sample(self):
for clip_sample in [True, False]:
self.check_over_configs(clip_sample=clip_sample)
def test_thresholding(self):
self.check_over_configs(thresholding=False)
for threshold in [0.5, 1.0, 2.0]:
for prediction_type in ["epsilon", "sample", "v_prediction"]:
self.check_over_configs(
thresholding=True,
prediction_type=prediction_type,
sample_max_value=threshold,
)
def test_prediction_type(self):
for prediction_type in ["epsilon", "sample", "v_prediction"]:
self.check_over_configs(prediction_type=prediction_type)
def test_time_indices(self):
for t in [0, 500, 999]:
self.check_over_forward(time_step=t)
def test_variance(self):
scheduler_class = self.scheduler_classes[0]
scheduler_config = self.get_scheduler_config()
scheduler = scheduler_class(**scheduler_config)
assert torch.sum(torch.abs(scheduler._get_variance(0) - 0.0)) < 1e-5
assert torch.sum(torch.abs(scheduler._get_variance(487) - 0.00979)) < 1e-5
assert torch.sum(torch.abs(scheduler._get_variance(999) - 0.02)) < 1e-5
def test_batch_step_no_noise(self):
scheduler_class = self.scheduler_classes[0]
scheduler_config = self.get_scheduler_config()
scheduler = scheduler_class(**scheduler_config)
num_trained_timesteps = len(scheduler)
model = self.dummy_model()
sample1 = self.dummy_sample_deter
sample2 = self.dummy_sample_deter + 0.1
sample3 = self.dummy_sample_deter - 0.1
per_sample_batch = sample1.shape[0]
samples = torch.stack([sample1, sample2, sample3], dim=0)
timesteps = torch.arange(num_trained_timesteps)[0:3, None].repeat(1, per_sample_batch)
residual = model(samples.flatten(0, 1), timesteps.flatten(0, 1))
pred_prev_sample = scheduler.batch_step_no_noise(residual, timesteps.flatten(0, 1), samples.flatten(0, 1))
result_sum = torch.sum(torch.abs(pred_prev_sample))
result_mean = torch.mean(torch.abs(pred_prev_sample))
assert abs(result_sum.item() - 1153.1833) < 1e-2
assert abs(result_mean.item() - 0.5005) < 1e-3
def test_full_loop_no_noise(self):
scheduler_class = self.scheduler_classes[0]
scheduler_config = self.get_scheduler_config()
scheduler = scheduler_class(**scheduler_config)
num_trained_timesteps = len(scheduler)
model = self.dummy_model()
sample = self.dummy_sample_deter
generator = torch.manual_seed(0)
for t in reversed(range(num_trained_timesteps)):
# 1. predict noise residual
residual = model(sample, t)
# 2. predict previous mean of sample x_t-1
pred_prev_sample = scheduler.step(residual, t, sample, generator=generator).prev_sample
sample = pred_prev_sample
result_sum = torch.sum(torch.abs(sample))
result_mean = torch.mean(torch.abs(sample))
assert abs(result_sum.item() - 258.9606) < 1e-2
assert abs(result_mean.item() - 0.3372) < 1e-3
def test_full_loop_with_v_prediction(self):
scheduler_class = self.scheduler_classes[0]
scheduler_config = self.get_scheduler_config(prediction_type="v_prediction")
scheduler = scheduler_class(**scheduler_config)
num_trained_timesteps = len(scheduler)
model = self.dummy_model()
sample = self.dummy_sample_deter
generator = torch.manual_seed(0)
for t in reversed(range(num_trained_timesteps)):
# 1. predict noise residual
residual = model(sample, t)
# 2. predict previous mean of sample x_t-1
pred_prev_sample = scheduler.step(residual, t, sample, generator=generator).prev_sample
sample = pred_prev_sample
result_sum = torch.sum(torch.abs(sample))
result_mean = torch.mean(torch.abs(sample))
assert abs(result_sum.item() - 202.0296) < 1e-2
assert abs(result_mean.item() - 0.2631) < 1e-3
def test_custom_timesteps(self):
scheduler_class = self.scheduler_classes[0]
scheduler_config = self.get_scheduler_config()
scheduler = scheduler_class(**scheduler_config)
timesteps = [100, 87, 50, 1, 0]
scheduler.set_timesteps(timesteps=timesteps)
scheduler_timesteps = scheduler.timesteps
for i, timestep in enumerate(scheduler_timesteps):
if i == len(timesteps) - 1:
expected_prev_t = -1
else:
expected_prev_t = timesteps[i + 1]
prev_t = scheduler.previous_timestep(timestep)
prev_t = prev_t.item()
self.assertEqual(prev_t, expected_prev_t)
def test_custom_timesteps_increasing_order(self):
scheduler_class = self.scheduler_classes[0]
scheduler_config = self.get_scheduler_config()
scheduler = scheduler_class(**scheduler_config)
timesteps = [100, 87, 50, 51, 0]
with self.assertRaises(ValueError, msg="`custom_timesteps` must be in descending order."):
scheduler.set_timesteps(timesteps=timesteps)
def test_custom_timesteps_passing_both_num_inference_steps_and_timesteps(self):
scheduler_class = self.scheduler_classes[0]
scheduler_config = self.get_scheduler_config()
scheduler = scheduler_class(**scheduler_config)
timesteps = [100, 87, 50, 1, 0]
num_inference_steps = len(timesteps)
with self.assertRaises(ValueError, msg="Can only pass one of `num_inference_steps` or `custom_timesteps`."):
scheduler.set_timesteps(num_inference_steps=num_inference_steps, timesteps=timesteps)
def test_custom_timesteps_too_large(self):
scheduler_class = self.scheduler_classes[0]
scheduler_config = self.get_scheduler_config()
scheduler = scheduler_class(**scheduler_config)
timesteps = [scheduler.config.num_train_timesteps]
with self.assertRaises(
ValueError,
msg="`timesteps` must start before `self.config.train_timesteps`: {scheduler.config.num_train_timesteps}}",
):
scheduler.set_timesteps(timesteps=timesteps)
| 0 |
hf_public_repos/diffusers/tests | hf_public_repos/diffusers/tests/schedulers/test_schedulers.py | # coding=utf-8
# Copyright 2023 HuggingFace Inc.
#
# Licensed under the Apache License, Version 2.0 (the "License");
# you may not use this file except in compliance with the License.
# You may obtain a copy of the License at
#
# http://www.apache.org/licenses/LICENSE-2.0
#
# Unless required by applicable law or agreed to in writing, software
# distributed under the License is distributed on an "AS IS" BASIS,
# WITHOUT WARRANTIES OR CONDITIONS OF ANY KIND, either express or implied.
# See the License for the specific language governing permissions and
# limitations under the License.
import inspect
import json
import os
import tempfile
import unittest
from typing import Dict, List, Tuple
import numpy as np
import torch
import diffusers
from diffusers import (
CMStochasticIterativeScheduler,
DDIMScheduler,
DEISMultistepScheduler,
DiffusionPipeline,
EulerAncestralDiscreteScheduler,
EulerDiscreteScheduler,
IPNDMScheduler,
LMSDiscreteScheduler,
UniPCMultistepScheduler,
VQDiffusionScheduler,
logging,
)
from diffusers.configuration_utils import ConfigMixin, register_to_config
from diffusers.schedulers.scheduling_utils import SchedulerMixin
from diffusers.utils import torch_device
from diffusers.utils.testing_utils import CaptureLogger
torch.backends.cuda.matmul.allow_tf32 = False
class SchedulerObject(SchedulerMixin, ConfigMixin):
config_name = "config.json"
@register_to_config
def __init__(
self,
a=2,
b=5,
c=(2, 5),
d="for diffusion",
e=[1, 3],
):
pass
class SchedulerObject2(SchedulerMixin, ConfigMixin):
config_name = "config.json"
@register_to_config
def __init__(
self,
a=2,
b=5,
c=(2, 5),
d="for diffusion",
f=[1, 3],
):
pass
class SchedulerObject3(SchedulerMixin, ConfigMixin):
config_name = "config.json"
@register_to_config
def __init__(
self,
a=2,
b=5,
c=(2, 5),
d="for diffusion",
e=[1, 3],
f=[1, 3],
):
pass
class SchedulerBaseTests(unittest.TestCase):
def test_save_load_from_different_config(self):
obj = SchedulerObject()
# mock add obj class to `diffusers`
setattr(diffusers, "SchedulerObject", SchedulerObject)
logger = logging.get_logger("diffusers.configuration_utils")
with tempfile.TemporaryDirectory() as tmpdirname:
obj.save_config(tmpdirname)
with CaptureLogger(logger) as cap_logger_1:
config = SchedulerObject2.load_config(tmpdirname)
new_obj_1 = SchedulerObject2.from_config(config)
# now save a config parameter that is not expected
with open(os.path.join(tmpdirname, SchedulerObject.config_name), "r") as f:
data = json.load(f)
data["unexpected"] = True
with open(os.path.join(tmpdirname, SchedulerObject.config_name), "w") as f:
json.dump(data, f)
with CaptureLogger(logger) as cap_logger_2:
config = SchedulerObject.load_config(tmpdirname)
new_obj_2 = SchedulerObject.from_config(config)
with CaptureLogger(logger) as cap_logger_3:
config = SchedulerObject2.load_config(tmpdirname)
new_obj_3 = SchedulerObject2.from_config(config)
assert new_obj_1.__class__ == SchedulerObject2
assert new_obj_2.__class__ == SchedulerObject
assert new_obj_3.__class__ == SchedulerObject2
assert cap_logger_1.out == ""
assert (
cap_logger_2.out
== "The config attributes {'unexpected': True} were passed to SchedulerObject, but are not expected and"
" will"
" be ignored. Please verify your config.json configuration file.\n"
)
assert cap_logger_2.out.replace("SchedulerObject", "SchedulerObject2") == cap_logger_3.out
def test_save_load_compatible_schedulers(self):
SchedulerObject2._compatibles = ["SchedulerObject"]
SchedulerObject._compatibles = ["SchedulerObject2"]
obj = SchedulerObject()
# mock add obj class to `diffusers`
setattr(diffusers, "SchedulerObject", SchedulerObject)
setattr(diffusers, "SchedulerObject2", SchedulerObject2)
logger = logging.get_logger("diffusers.configuration_utils")
with tempfile.TemporaryDirectory() as tmpdirname:
obj.save_config(tmpdirname)
# now save a config parameter that is expected by another class, but not origin class
with open(os.path.join(tmpdirname, SchedulerObject.config_name), "r") as f:
data = json.load(f)
data["f"] = [0, 0]
data["unexpected"] = True
with open(os.path.join(tmpdirname, SchedulerObject.config_name), "w") as f:
json.dump(data, f)
with CaptureLogger(logger) as cap_logger:
config = SchedulerObject.load_config(tmpdirname)
new_obj = SchedulerObject.from_config(config)
assert new_obj.__class__ == SchedulerObject
assert (
cap_logger.out
== "The config attributes {'unexpected': True} were passed to SchedulerObject, but are not expected and"
" will"
" be ignored. Please verify your config.json configuration file.\n"
)
def test_save_load_from_different_config_comp_schedulers(self):
SchedulerObject3._compatibles = ["SchedulerObject", "SchedulerObject2"]
SchedulerObject2._compatibles = ["SchedulerObject", "SchedulerObject3"]
SchedulerObject._compatibles = ["SchedulerObject2", "SchedulerObject3"]
obj = SchedulerObject()
# mock add obj class to `diffusers`
setattr(diffusers, "SchedulerObject", SchedulerObject)
setattr(diffusers, "SchedulerObject2", SchedulerObject2)
setattr(diffusers, "SchedulerObject3", SchedulerObject3)
logger = logging.get_logger("diffusers.configuration_utils")
logger.setLevel(diffusers.logging.INFO)
with tempfile.TemporaryDirectory() as tmpdirname:
obj.save_config(tmpdirname)
with CaptureLogger(logger) as cap_logger_1:
config = SchedulerObject.load_config(tmpdirname)
new_obj_1 = SchedulerObject.from_config(config)
with CaptureLogger(logger) as cap_logger_2:
config = SchedulerObject2.load_config(tmpdirname)
new_obj_2 = SchedulerObject2.from_config(config)
with CaptureLogger(logger) as cap_logger_3:
config = SchedulerObject3.load_config(tmpdirname)
new_obj_3 = SchedulerObject3.from_config(config)
assert new_obj_1.__class__ == SchedulerObject
assert new_obj_2.__class__ == SchedulerObject2
assert new_obj_3.__class__ == SchedulerObject3
assert cap_logger_1.out == ""
assert cap_logger_2.out == "{'f'} was not found in config. Values will be initialized to default values.\n"
assert cap_logger_3.out == "{'f'} was not found in config. Values will be initialized to default values.\n"
def test_default_arguments_not_in_config(self):
pipe = DiffusionPipeline.from_pretrained(
"hf-internal-testing/tiny-stable-diffusion-pipe", torch_dtype=torch.float16
)
assert pipe.scheduler.__class__ == DDIMScheduler
# Default for DDIMScheduler
assert pipe.scheduler.config.timestep_spacing == "leading"
# Switch to a different one, verify we use the default for that class
pipe.scheduler = EulerDiscreteScheduler.from_config(pipe.scheduler.config)
assert pipe.scheduler.config.timestep_spacing == "linspace"
# Override with kwargs
pipe.scheduler = EulerDiscreteScheduler.from_config(pipe.scheduler.config, timestep_spacing="trailing")
assert pipe.scheduler.config.timestep_spacing == "trailing"
# Verify overridden kwargs stick
pipe.scheduler = LMSDiscreteScheduler.from_config(pipe.scheduler.config)
assert pipe.scheduler.config.timestep_spacing == "trailing"
# And stick
pipe.scheduler = LMSDiscreteScheduler.from_config(pipe.scheduler.config)
assert pipe.scheduler.config.timestep_spacing == "trailing"
def test_default_solver_type_after_switch(self):
pipe = DiffusionPipeline.from_pretrained(
"hf-internal-testing/tiny-stable-diffusion-pipe", torch_dtype=torch.float16
)
assert pipe.scheduler.__class__ == DDIMScheduler
pipe.scheduler = DEISMultistepScheduler.from_config(pipe.scheduler.config)
assert pipe.scheduler.config.solver_type == "logrho"
# Switch to UniPC, verify the solver is the default
pipe.scheduler = UniPCMultistepScheduler.from_config(pipe.scheduler.config)
assert pipe.scheduler.config.solver_type == "bh2"
class SchedulerCommonTest(unittest.TestCase):
scheduler_classes = ()
forward_default_kwargs = ()
@property
def dummy_sample(self):
batch_size = 4
num_channels = 3
height = 8
width = 8
sample = torch.rand((batch_size, num_channels, height, width))
return sample
@property
def dummy_sample_deter(self):
batch_size = 4
num_channels = 3
height = 8
width = 8
num_elems = batch_size * num_channels * height * width
sample = torch.arange(num_elems)
sample = sample.reshape(num_channels, height, width, batch_size)
sample = sample / num_elems
sample = sample.permute(3, 0, 1, 2)
return sample
def get_scheduler_config(self):
raise NotImplementedError
def dummy_model(self):
def model(sample, t, *args):
# if t is a tensor, match the number of dimensions of sample
if isinstance(t, torch.Tensor):
num_dims = len(sample.shape)
# pad t with 1s to match num_dims
t = t.reshape(-1, *(1,) * (num_dims - 1)).to(sample.device).to(sample.dtype)
return sample * t / (t + 1)
return model
def check_over_configs(self, time_step=0, **config):
kwargs = dict(self.forward_default_kwargs)
num_inference_steps = kwargs.pop("num_inference_steps", None)
for scheduler_class in self.scheduler_classes:
# TODO(Suraj) - delete the following two lines once DDPM, DDIM, and PNDM have timesteps casted to float by default
if scheduler_class in (EulerAncestralDiscreteScheduler, EulerDiscreteScheduler, LMSDiscreteScheduler):
time_step = float(time_step)
scheduler_config = self.get_scheduler_config(**config)
scheduler = scheduler_class(**scheduler_config)
if scheduler_class == CMStochasticIterativeScheduler:
# Get valid timestep based on sigma_max, which should always be in timestep schedule.
scaled_sigma_max = scheduler.sigma_to_t(scheduler.config.sigma_max)
time_step = scaled_sigma_max
if scheduler_class == VQDiffusionScheduler:
num_vec_classes = scheduler_config["num_vec_classes"]
sample = self.dummy_sample(num_vec_classes)
model = self.dummy_model(num_vec_classes)
residual = model(sample, time_step)
else:
sample = self.dummy_sample
residual = 0.1 * sample
with tempfile.TemporaryDirectory() as tmpdirname:
scheduler.save_config(tmpdirname)
new_scheduler = scheduler_class.from_pretrained(tmpdirname)
if num_inference_steps is not None and hasattr(scheduler, "set_timesteps"):
scheduler.set_timesteps(num_inference_steps)
new_scheduler.set_timesteps(num_inference_steps)
elif num_inference_steps is not None and not hasattr(scheduler, "set_timesteps"):
kwargs["num_inference_steps"] = num_inference_steps
# Make sure `scale_model_input` is invoked to prevent a warning
if scheduler_class == CMStochasticIterativeScheduler:
# Get valid timestep based on sigma_max, which should always be in timestep schedule.
_ = scheduler.scale_model_input(sample, scaled_sigma_max)
_ = new_scheduler.scale_model_input(sample, scaled_sigma_max)
elif scheduler_class != VQDiffusionScheduler:
_ = scheduler.scale_model_input(sample, 0)
_ = new_scheduler.scale_model_input(sample, 0)
# Set the seed before step() as some schedulers are stochastic like EulerAncestralDiscreteScheduler, EulerDiscreteScheduler
if "generator" in set(inspect.signature(scheduler.step).parameters.keys()):
kwargs["generator"] = torch.manual_seed(0)
output = scheduler.step(residual, time_step, sample, **kwargs).prev_sample
if "generator" in set(inspect.signature(scheduler.step).parameters.keys()):
kwargs["generator"] = torch.manual_seed(0)
new_output = new_scheduler.step(residual, time_step, sample, **kwargs).prev_sample
assert torch.sum(torch.abs(output - new_output)) < 1e-5, "Scheduler outputs are not identical"
def check_over_forward(self, time_step=0, **forward_kwargs):
kwargs = dict(self.forward_default_kwargs)
kwargs.update(forward_kwargs)
num_inference_steps = kwargs.pop("num_inference_steps", None)
for scheduler_class in self.scheduler_classes:
if scheduler_class in (EulerAncestralDiscreteScheduler, EulerDiscreteScheduler, LMSDiscreteScheduler):
time_step = float(time_step)
scheduler_config = self.get_scheduler_config()
scheduler = scheduler_class(**scheduler_config)
if scheduler_class == VQDiffusionScheduler:
num_vec_classes = scheduler_config["num_vec_classes"]
sample = self.dummy_sample(num_vec_classes)
model = self.dummy_model(num_vec_classes)
residual = model(sample, time_step)
else:
sample = self.dummy_sample
residual = 0.1 * sample
with tempfile.TemporaryDirectory() as tmpdirname:
scheduler.save_config(tmpdirname)
new_scheduler = scheduler_class.from_pretrained(tmpdirname)
if num_inference_steps is not None and hasattr(scheduler, "set_timesteps"):
scheduler.set_timesteps(num_inference_steps)
new_scheduler.set_timesteps(num_inference_steps)
elif num_inference_steps is not None and not hasattr(scheduler, "set_timesteps"):
kwargs["num_inference_steps"] = num_inference_steps
if "generator" in set(inspect.signature(scheduler.step).parameters.keys()):
kwargs["generator"] = torch.manual_seed(0)
output = scheduler.step(residual, time_step, sample, **kwargs).prev_sample
if "generator" in set(inspect.signature(scheduler.step).parameters.keys()):
kwargs["generator"] = torch.manual_seed(0)
new_output = new_scheduler.step(residual, time_step, sample, **kwargs).prev_sample
assert torch.sum(torch.abs(output - new_output)) < 1e-5, "Scheduler outputs are not identical"
def test_from_save_pretrained(self):
kwargs = dict(self.forward_default_kwargs)
num_inference_steps = kwargs.pop("num_inference_steps", None)
for scheduler_class in self.scheduler_classes:
timestep = 1
if scheduler_class in (EulerAncestralDiscreteScheduler, EulerDiscreteScheduler, LMSDiscreteScheduler):
timestep = float(timestep)
scheduler_config = self.get_scheduler_config()
scheduler = scheduler_class(**scheduler_config)
if scheduler_class == CMStochasticIterativeScheduler:
# Get valid timestep based on sigma_max, which should always be in timestep schedule.
timestep = scheduler.sigma_to_t(scheduler.config.sigma_max)
if scheduler_class == VQDiffusionScheduler:
num_vec_classes = scheduler_config["num_vec_classes"]
sample = self.dummy_sample(num_vec_classes)
model = self.dummy_model(num_vec_classes)
residual = model(sample, timestep)
else:
sample = self.dummy_sample
residual = 0.1 * sample
with tempfile.TemporaryDirectory() as tmpdirname:
scheduler.save_config(tmpdirname)
new_scheduler = scheduler_class.from_pretrained(tmpdirname)
if num_inference_steps is not None and hasattr(scheduler, "set_timesteps"):
scheduler.set_timesteps(num_inference_steps)
new_scheduler.set_timesteps(num_inference_steps)
elif num_inference_steps is not None and not hasattr(scheduler, "set_timesteps"):
kwargs["num_inference_steps"] = num_inference_steps
if "generator" in set(inspect.signature(scheduler.step).parameters.keys()):
kwargs["generator"] = torch.manual_seed(0)
output = scheduler.step(residual, timestep, sample, **kwargs).prev_sample
if "generator" in set(inspect.signature(scheduler.step).parameters.keys()):
kwargs["generator"] = torch.manual_seed(0)
new_output = new_scheduler.step(residual, timestep, sample, **kwargs).prev_sample
assert torch.sum(torch.abs(output - new_output)) < 1e-5, "Scheduler outputs are not identical"
def test_compatibles(self):
for scheduler_class in self.scheduler_classes:
scheduler_config = self.get_scheduler_config()
scheduler = scheduler_class(**scheduler_config)
assert all(c is not None for c in scheduler.compatibles)
for comp_scheduler_cls in scheduler.compatibles:
comp_scheduler = comp_scheduler_cls.from_config(scheduler.config)
assert comp_scheduler is not None
new_scheduler = scheduler_class.from_config(comp_scheduler.config)
new_scheduler_config = {k: v for k, v in new_scheduler.config.items() if k in scheduler.config}
scheduler_diff = {k: v for k, v in new_scheduler.config.items() if k not in scheduler.config}
# make sure that configs are essentially identical
assert new_scheduler_config == dict(scheduler.config)
# make sure that only differences are for configs that are not in init
init_keys = inspect.signature(scheduler_class.__init__).parameters.keys()
assert set(scheduler_diff.keys()).intersection(set(init_keys)) == set()
def test_from_pretrained(self):
for scheduler_class in self.scheduler_classes:
scheduler_config = self.get_scheduler_config()
scheduler = scheduler_class(**scheduler_config)
with tempfile.TemporaryDirectory() as tmpdirname:
scheduler.save_pretrained(tmpdirname)
new_scheduler = scheduler_class.from_pretrained(tmpdirname)
# `_use_default_values` should not exist for just saved & loaded scheduler
scheduler_config = dict(scheduler.config)
del scheduler_config["_use_default_values"]
assert scheduler_config == new_scheduler.config
def test_step_shape(self):
kwargs = dict(self.forward_default_kwargs)
num_inference_steps = kwargs.pop("num_inference_steps", None)
timestep_0 = 0
timestep_1 = 1
for scheduler_class in self.scheduler_classes:
if scheduler_class in (EulerAncestralDiscreteScheduler, EulerDiscreteScheduler, LMSDiscreteScheduler):
timestep_0 = float(timestep_0)
timestep_1 = float(timestep_1)
scheduler_config = self.get_scheduler_config()
scheduler = scheduler_class(**scheduler_config)
if scheduler_class == VQDiffusionScheduler:
num_vec_classes = scheduler_config["num_vec_classes"]
sample = self.dummy_sample(num_vec_classes)
model = self.dummy_model(num_vec_classes)
residual = model(sample, timestep_0)
else:
sample = self.dummy_sample
residual = 0.1 * sample
if num_inference_steps is not None and hasattr(scheduler, "set_timesteps"):
scheduler.set_timesteps(num_inference_steps)
elif num_inference_steps is not None and not hasattr(scheduler, "set_timesteps"):
kwargs["num_inference_steps"] = num_inference_steps
output_0 = scheduler.step(residual, timestep_0, sample, **kwargs).prev_sample
output_1 = scheduler.step(residual, timestep_1, sample, **kwargs).prev_sample
self.assertEqual(output_0.shape, sample.shape)
self.assertEqual(output_0.shape, output_1.shape)
def test_scheduler_outputs_equivalence(self):
def set_nan_tensor_to_zero(t):
t[t != t] = 0
return t
def recursive_check(tuple_object, dict_object):
if isinstance(tuple_object, (List, Tuple)):
for tuple_iterable_value, dict_iterable_value in zip(tuple_object, dict_object.values()):
recursive_check(tuple_iterable_value, dict_iterable_value)
elif isinstance(tuple_object, Dict):
for tuple_iterable_value, dict_iterable_value in zip(tuple_object.values(), dict_object.values()):
recursive_check(tuple_iterable_value, dict_iterable_value)
elif tuple_object is None:
return
else:
self.assertTrue(
torch.allclose(
set_nan_tensor_to_zero(tuple_object), set_nan_tensor_to_zero(dict_object), atol=1e-5
),
msg=(
"Tuple and dict output are not equal. Difference:"
f" {torch.max(torch.abs(tuple_object - dict_object))}. Tuple has `nan`:"
f" {torch.isnan(tuple_object).any()} and `inf`: {torch.isinf(tuple_object)}. Dict has"
f" `nan`: {torch.isnan(dict_object).any()} and `inf`: {torch.isinf(dict_object)}."
),
)
kwargs = dict(self.forward_default_kwargs)
num_inference_steps = kwargs.pop("num_inference_steps", 50)
timestep = 0
if len(self.scheduler_classes) > 0 and self.scheduler_classes[0] == IPNDMScheduler:
timestep = 1
for scheduler_class in self.scheduler_classes:
if scheduler_class in (EulerAncestralDiscreteScheduler, EulerDiscreteScheduler, LMSDiscreteScheduler):
timestep = float(timestep)
scheduler_config = self.get_scheduler_config()
scheduler = scheduler_class(**scheduler_config)
if scheduler_class == CMStochasticIterativeScheduler:
# Get valid timestep based on sigma_max, which should always be in timestep schedule.
timestep = scheduler.sigma_to_t(scheduler.config.sigma_max)
if scheduler_class == VQDiffusionScheduler:
num_vec_classes = scheduler_config["num_vec_classes"]
sample = self.dummy_sample(num_vec_classes)
model = self.dummy_model(num_vec_classes)
residual = model(sample, timestep)
else:
sample = self.dummy_sample
residual = 0.1 * sample
if num_inference_steps is not None and hasattr(scheduler, "set_timesteps"):
scheduler.set_timesteps(num_inference_steps)
elif num_inference_steps is not None and not hasattr(scheduler, "set_timesteps"):
kwargs["num_inference_steps"] = num_inference_steps
# Set the seed before state as some schedulers are stochastic like EulerAncestralDiscreteScheduler, EulerDiscreteScheduler
if "generator" in set(inspect.signature(scheduler.step).parameters.keys()):
kwargs["generator"] = torch.manual_seed(0)
outputs_dict = scheduler.step(residual, timestep, sample, **kwargs)
if num_inference_steps is not None and hasattr(scheduler, "set_timesteps"):
scheduler.set_timesteps(num_inference_steps)
elif num_inference_steps is not None and not hasattr(scheduler, "set_timesteps"):
kwargs["num_inference_steps"] = num_inference_steps
# Set the seed before state as some schedulers are stochastic like EulerAncestralDiscreteScheduler, EulerDiscreteScheduler
if "generator" in set(inspect.signature(scheduler.step).parameters.keys()):
kwargs["generator"] = torch.manual_seed(0)
outputs_tuple = scheduler.step(residual, timestep, sample, return_dict=False, **kwargs)
recursive_check(outputs_tuple, outputs_dict)
def test_scheduler_public_api(self):
for scheduler_class in self.scheduler_classes:
scheduler_config = self.get_scheduler_config()
scheduler = scheduler_class(**scheduler_config)
if scheduler_class != VQDiffusionScheduler:
self.assertTrue(
hasattr(scheduler, "init_noise_sigma"),
f"{scheduler_class} does not implement a required attribute `init_noise_sigma`",
)
self.assertTrue(
hasattr(scheduler, "scale_model_input"),
(
f"{scheduler_class} does not implement a required class method `scale_model_input(sample,"
" timestep)`"
),
)
self.assertTrue(
hasattr(scheduler, "step"),
f"{scheduler_class} does not implement a required class method `step(...)`",
)
if scheduler_class != VQDiffusionScheduler:
sample = self.dummy_sample
if scheduler_class == CMStochasticIterativeScheduler:
# Get valid timestep based on sigma_max, which should always be in timestep schedule.
scaled_sigma_max = scheduler.sigma_to_t(scheduler.config.sigma_max)
scaled_sample = scheduler.scale_model_input(sample, scaled_sigma_max)
else:
scaled_sample = scheduler.scale_model_input(sample, 0.0)
self.assertEqual(sample.shape, scaled_sample.shape)
def test_add_noise_device(self):
for scheduler_class in self.scheduler_classes:
if scheduler_class == IPNDMScheduler:
continue
scheduler_config = self.get_scheduler_config()
scheduler = scheduler_class(**scheduler_config)
scheduler.set_timesteps(100)
sample = self.dummy_sample.to(torch_device)
if scheduler_class == CMStochasticIterativeScheduler:
# Get valid timestep based on sigma_max, which should always be in timestep schedule.
scaled_sigma_max = scheduler.sigma_to_t(scheduler.config.sigma_max)
scaled_sample = scheduler.scale_model_input(sample, scaled_sigma_max)
else:
scaled_sample = scheduler.scale_model_input(sample, 0.0)
self.assertEqual(sample.shape, scaled_sample.shape)
noise = torch.randn_like(scaled_sample).to(torch_device)
t = scheduler.timesteps[5][None]
noised = scheduler.add_noise(scaled_sample, noise, t)
self.assertEqual(noised.shape, scaled_sample.shape)
def test_deprecated_kwargs(self):
for scheduler_class in self.scheduler_classes:
has_kwarg_in_model_class = "kwargs" in inspect.signature(scheduler_class.__init__).parameters
has_deprecated_kwarg = len(scheduler_class._deprecated_kwargs) > 0
if has_kwarg_in_model_class and not has_deprecated_kwarg:
raise ValueError(
f"{scheduler_class} has `**kwargs` in its __init__ method but has not defined any deprecated"
" kwargs under the `_deprecated_kwargs` class attribute. Make sure to either remove `**kwargs` if"
" there are no deprecated arguments or add the deprecated argument with `_deprecated_kwargs ="
" [<deprecated_argument>]`"
)
if not has_kwarg_in_model_class and has_deprecated_kwarg:
raise ValueError(
f"{scheduler_class} doesn't have `**kwargs` in its __init__ method but has defined deprecated"
" kwargs under the `_deprecated_kwargs` class attribute. Make sure to either add the `**kwargs`"
f" argument to {self.model_class}.__init__ if there are deprecated arguments or remove the"
" deprecated argument from `_deprecated_kwargs = [<deprecated_argument>]`"
)
def test_trained_betas(self):
for scheduler_class in self.scheduler_classes:
if scheduler_class in (VQDiffusionScheduler, CMStochasticIterativeScheduler):
continue
scheduler_config = self.get_scheduler_config()
scheduler = scheduler_class(**scheduler_config, trained_betas=np.array([0.1, 0.3]))
with tempfile.TemporaryDirectory() as tmpdirname:
scheduler.save_pretrained(tmpdirname)
new_scheduler = scheduler_class.from_pretrained(tmpdirname)
assert scheduler.betas.tolist() == new_scheduler.betas.tolist()
def test_getattr_is_correct(self):
for scheduler_class in self.scheduler_classes:
scheduler_config = self.get_scheduler_config()
scheduler = scheduler_class(**scheduler_config)
# save some things to test
scheduler.dummy_attribute = 5
scheduler.register_to_config(test_attribute=5)
logger = logging.get_logger("diffusers.configuration_utils")
# 30 for warning
logger.setLevel(30)
with CaptureLogger(logger) as cap_logger:
assert hasattr(scheduler, "dummy_attribute")
assert getattr(scheduler, "dummy_attribute") == 5
assert scheduler.dummy_attribute == 5
# no warning should be thrown
assert cap_logger.out == ""
logger = logging.get_logger("diffusers.schedulers.schedulering_utils")
# 30 for warning
logger.setLevel(30)
with CaptureLogger(logger) as cap_logger:
assert hasattr(scheduler, "save_pretrained")
fn = scheduler.save_pretrained
fn_1 = getattr(scheduler, "save_pretrained")
assert fn == fn_1
# no warning should be thrown
assert cap_logger.out == ""
# warning should be thrown
with self.assertWarns(FutureWarning):
assert scheduler.test_attribute == 5
with self.assertWarns(FutureWarning):
assert getattr(scheduler, "test_attribute") == 5
with self.assertRaises(AttributeError) as error:
scheduler.does_not_exist
assert str(error.exception) == f"'{type(scheduler).__name__}' object has no attribute 'does_not_exist'"
| 0 |
hf_public_repos/diffusers/tests | hf_public_repos/diffusers/tests/schedulers/test_scheduler_flax.py | # coding=utf-8
# Copyright 2023 HuggingFace Inc.
#
# Licensed under the Apache License, Version 2.0 (the "License");
# you may not use this file except in compliance with the License.
# You may obtain a copy of the License at
#
# http://www.apache.org/licenses/LICENSE-2.0
#
# Unless required by applicable law or agreed to in writing, software
# distributed under the License is distributed on an "AS IS" BASIS,
# WITHOUT WARRANTIES OR CONDITIONS OF ANY KIND, either express or implied.
# See the License for the specific language governing permissions and
# limitations under the License.
import inspect
import tempfile
import unittest
from typing import Dict, List, Tuple
from diffusers import FlaxDDIMScheduler, FlaxDDPMScheduler, FlaxPNDMScheduler
from diffusers.utils import is_flax_available
from diffusers.utils.testing_utils import require_flax
if is_flax_available():
import jax
import jax.numpy as jnp
from jax import random
jax_device = jax.default_backend()
@require_flax
class FlaxSchedulerCommonTest(unittest.TestCase):
scheduler_classes = ()
forward_default_kwargs = ()
@property
def dummy_sample(self):
batch_size = 4
num_channels = 3
height = 8
width = 8
key1, key2 = random.split(random.PRNGKey(0))
sample = random.uniform(key1, (batch_size, num_channels, height, width))
return sample, key2
@property
def dummy_sample_deter(self):
batch_size = 4
num_channels = 3
height = 8
width = 8
num_elems = batch_size * num_channels * height * width
sample = jnp.arange(num_elems)
sample = sample.reshape(num_channels, height, width, batch_size)
sample = sample / num_elems
return jnp.transpose(sample, (3, 0, 1, 2))
def get_scheduler_config(self):
raise NotImplementedError
def dummy_model(self):
def model(sample, t, *args):
return sample * t / (t + 1)
return model
def check_over_configs(self, time_step=0, **config):
kwargs = dict(self.forward_default_kwargs)
num_inference_steps = kwargs.pop("num_inference_steps", None)
for scheduler_class in self.scheduler_classes:
sample, key = self.dummy_sample
residual = 0.1 * sample
scheduler_config = self.get_scheduler_config(**config)
scheduler = scheduler_class(**scheduler_config)
state = scheduler.create_state()
with tempfile.TemporaryDirectory() as tmpdirname:
scheduler.save_config(tmpdirname)
new_scheduler, new_state = scheduler_class.from_pretrained(tmpdirname)
if num_inference_steps is not None and hasattr(scheduler, "set_timesteps"):
state = scheduler.set_timesteps(state, num_inference_steps)
new_state = new_scheduler.set_timesteps(new_state, num_inference_steps)
elif num_inference_steps is not None and not hasattr(scheduler, "set_timesteps"):
kwargs["num_inference_steps"] = num_inference_steps
output = scheduler.step(state, residual, time_step, sample, key, **kwargs).prev_sample
new_output = new_scheduler.step(new_state, residual, time_step, sample, key, **kwargs).prev_sample
assert jnp.sum(jnp.abs(output - new_output)) < 1e-5, "Scheduler outputs are not identical"
def check_over_forward(self, time_step=0, **forward_kwargs):
kwargs = dict(self.forward_default_kwargs)
kwargs.update(forward_kwargs)
num_inference_steps = kwargs.pop("num_inference_steps", None)
for scheduler_class in self.scheduler_classes:
sample, key = self.dummy_sample
residual = 0.1 * sample
scheduler_config = self.get_scheduler_config()
scheduler = scheduler_class(**scheduler_config)
state = scheduler.create_state()
with tempfile.TemporaryDirectory() as tmpdirname:
scheduler.save_config(tmpdirname)
new_scheduler, new_state = scheduler_class.from_pretrained(tmpdirname)
if num_inference_steps is not None and hasattr(scheduler, "set_timesteps"):
state = scheduler.set_timesteps(state, num_inference_steps)
new_state = new_scheduler.set_timesteps(new_state, num_inference_steps)
elif num_inference_steps is not None and not hasattr(scheduler, "set_timesteps"):
kwargs["num_inference_steps"] = num_inference_steps
output = scheduler.step(state, residual, time_step, sample, key, **kwargs).prev_sample
new_output = new_scheduler.step(new_state, residual, time_step, sample, key, **kwargs).prev_sample
assert jnp.sum(jnp.abs(output - new_output)) < 1e-5, "Scheduler outputs are not identical"
def test_from_save_pretrained(self):
kwargs = dict(self.forward_default_kwargs)
num_inference_steps = kwargs.pop("num_inference_steps", None)
for scheduler_class in self.scheduler_classes:
sample, key = self.dummy_sample
residual = 0.1 * sample
scheduler_config = self.get_scheduler_config()
scheduler = scheduler_class(**scheduler_config)
state = scheduler.create_state()
with tempfile.TemporaryDirectory() as tmpdirname:
scheduler.save_config(tmpdirname)
new_scheduler, new_state = scheduler_class.from_pretrained(tmpdirname)
if num_inference_steps is not None and hasattr(scheduler, "set_timesteps"):
state = scheduler.set_timesteps(state, num_inference_steps)
new_state = new_scheduler.set_timesteps(new_state, num_inference_steps)
elif num_inference_steps is not None and not hasattr(scheduler, "set_timesteps"):
kwargs["num_inference_steps"] = num_inference_steps
output = scheduler.step(state, residual, 1, sample, key, **kwargs).prev_sample
new_output = new_scheduler.step(new_state, residual, 1, sample, key, **kwargs).prev_sample
assert jnp.sum(jnp.abs(output - new_output)) < 1e-5, "Scheduler outputs are not identical"
def test_step_shape(self):
kwargs = dict(self.forward_default_kwargs)
num_inference_steps = kwargs.pop("num_inference_steps", None)
for scheduler_class in self.scheduler_classes:
scheduler_config = self.get_scheduler_config()
scheduler = scheduler_class(**scheduler_config)
state = scheduler.create_state()
sample, key = self.dummy_sample
residual = 0.1 * sample
if num_inference_steps is not None and hasattr(scheduler, "set_timesteps"):
state = scheduler.set_timesteps(state, num_inference_steps)
elif num_inference_steps is not None and not hasattr(scheduler, "set_timesteps"):
kwargs["num_inference_steps"] = num_inference_steps
output_0 = scheduler.step(state, residual, 0, sample, key, **kwargs).prev_sample
output_1 = scheduler.step(state, residual, 1, sample, key, **kwargs).prev_sample
self.assertEqual(output_0.shape, sample.shape)
self.assertEqual(output_0.shape, output_1.shape)
def test_scheduler_outputs_equivalence(self):
def set_nan_tensor_to_zero(t):
return t.at[t != t].set(0)
def recursive_check(tuple_object, dict_object):
if isinstance(tuple_object, (List, Tuple)):
for tuple_iterable_value, dict_iterable_value in zip(tuple_object, dict_object.values()):
recursive_check(tuple_iterable_value, dict_iterable_value)
elif isinstance(tuple_object, Dict):
for tuple_iterable_value, dict_iterable_value in zip(tuple_object.values(), dict_object.values()):
recursive_check(tuple_iterable_value, dict_iterable_value)
elif tuple_object is None:
return
else:
self.assertTrue(
jnp.allclose(set_nan_tensor_to_zero(tuple_object), set_nan_tensor_to_zero(dict_object), atol=1e-5),
msg=(
"Tuple and dict output are not equal. Difference:"
f" {jnp.max(jnp.abs(tuple_object - dict_object))}. Tuple has `nan`:"
f" {jnp.isnan(tuple_object).any()} and `inf`: {jnp.isinf(tuple_object)}. Dict has"
f" `nan`: {jnp.isnan(dict_object).any()} and `inf`: {jnp.isinf(dict_object)}."
),
)
kwargs = dict(self.forward_default_kwargs)
num_inference_steps = kwargs.pop("num_inference_steps", None)
for scheduler_class in self.scheduler_classes:
scheduler_config = self.get_scheduler_config()
scheduler = scheduler_class(**scheduler_config)
state = scheduler.create_state()
sample, key = self.dummy_sample
residual = 0.1 * sample
if num_inference_steps is not None and hasattr(scheduler, "set_timesteps"):
state = scheduler.set_timesteps(state, num_inference_steps)
elif num_inference_steps is not None and not hasattr(scheduler, "set_timesteps"):
kwargs["num_inference_steps"] = num_inference_steps
outputs_dict = scheduler.step(state, residual, 0, sample, key, **kwargs)
if num_inference_steps is not None and hasattr(scheduler, "set_timesteps"):
state = scheduler.set_timesteps(state, num_inference_steps)
elif num_inference_steps is not None and not hasattr(scheduler, "set_timesteps"):
kwargs["num_inference_steps"] = num_inference_steps
outputs_tuple = scheduler.step(state, residual, 0, sample, key, return_dict=False, **kwargs)
recursive_check(outputs_tuple[0], outputs_dict.prev_sample)
def test_deprecated_kwargs(self):
for scheduler_class in self.scheduler_classes:
has_kwarg_in_model_class = "kwargs" in inspect.signature(scheduler_class.__init__).parameters
has_deprecated_kwarg = len(scheduler_class._deprecated_kwargs) > 0
if has_kwarg_in_model_class and not has_deprecated_kwarg:
raise ValueError(
f"{scheduler_class} has `**kwargs` in its __init__ method but has not defined any deprecated"
" kwargs under the `_deprecated_kwargs` class attribute. Make sure to either remove `**kwargs` if"
" there are no deprecated arguments or add the deprecated argument with `_deprecated_kwargs ="
" [<deprecated_argument>]`"
)
if not has_kwarg_in_model_class and has_deprecated_kwarg:
raise ValueError(
f"{scheduler_class} doesn't have `**kwargs` in its __init__ method but has defined deprecated"
" kwargs under the `_deprecated_kwargs` class attribute. Make sure to either add the `**kwargs`"
f" argument to {self.model_class}.__init__ if there are deprecated arguments or remove the"
" deprecated argument from `_deprecated_kwargs = [<deprecated_argument>]`"
)
@require_flax
class FlaxDDPMSchedulerTest(FlaxSchedulerCommonTest):
scheduler_classes = (FlaxDDPMScheduler,)
def get_scheduler_config(self, **kwargs):
config = {
"num_train_timesteps": 1000,
"beta_start": 0.0001,
"beta_end": 0.02,
"beta_schedule": "linear",
"variance_type": "fixed_small",
"clip_sample": True,
}
config.update(**kwargs)
return config
def test_timesteps(self):
for timesteps in [1, 5, 100, 1000]:
self.check_over_configs(num_train_timesteps=timesteps)
def test_betas(self):
for beta_start, beta_end in zip([0.0001, 0.001, 0.01, 0.1], [0.002, 0.02, 0.2, 2]):
self.check_over_configs(beta_start=beta_start, beta_end=beta_end)
def test_schedules(self):
for schedule in ["linear", "squaredcos_cap_v2"]:
self.check_over_configs(beta_schedule=schedule)
def test_variance_type(self):
for variance in ["fixed_small", "fixed_large", "other"]:
self.check_over_configs(variance_type=variance)
def test_clip_sample(self):
for clip_sample in [True, False]:
self.check_over_configs(clip_sample=clip_sample)
def test_time_indices(self):
for t in [0, 500, 999]:
self.check_over_forward(time_step=t)
def test_variance(self):
scheduler_class = self.scheduler_classes[0]
scheduler_config = self.get_scheduler_config()
scheduler = scheduler_class(**scheduler_config)
state = scheduler.create_state()
assert jnp.sum(jnp.abs(scheduler._get_variance(state, 0) - 0.0)) < 1e-5
assert jnp.sum(jnp.abs(scheduler._get_variance(state, 487) - 0.00979)) < 1e-5
assert jnp.sum(jnp.abs(scheduler._get_variance(state, 999) - 0.02)) < 1e-5
def test_full_loop_no_noise(self):
scheduler_class = self.scheduler_classes[0]
scheduler_config = self.get_scheduler_config()
scheduler = scheduler_class(**scheduler_config)
state = scheduler.create_state()
num_trained_timesteps = len(scheduler)
model = self.dummy_model()
sample = self.dummy_sample_deter
key1, key2 = random.split(random.PRNGKey(0))
for t in reversed(range(num_trained_timesteps)):
# 1. predict noise residual
residual = model(sample, t)
# 2. predict previous mean of sample x_t-1
output = scheduler.step(state, residual, t, sample, key1)
pred_prev_sample = output.prev_sample
state = output.state
key1, key2 = random.split(key2)
# if t > 0:
# noise = self.dummy_sample_deter
# variance = scheduler.get_variance(t) ** (0.5) * noise
#
# sample = pred_prev_sample + variance
sample = pred_prev_sample
result_sum = jnp.sum(jnp.abs(sample))
result_mean = jnp.mean(jnp.abs(sample))
if jax_device == "tpu":
assert abs(result_sum - 255.0714) < 1e-2
assert abs(result_mean - 0.332124) < 1e-3
else:
assert abs(result_sum - 255.1113) < 1e-2
assert abs(result_mean - 0.332176) < 1e-3
@require_flax
class FlaxDDIMSchedulerTest(FlaxSchedulerCommonTest):
scheduler_classes = (FlaxDDIMScheduler,)
forward_default_kwargs = (("num_inference_steps", 50),)
def get_scheduler_config(self, **kwargs):
config = {
"num_train_timesteps": 1000,
"beta_start": 0.0001,
"beta_end": 0.02,
"beta_schedule": "linear",
}
config.update(**kwargs)
return config
def full_loop(self, **config):
scheduler_class = self.scheduler_classes[0]
scheduler_config = self.get_scheduler_config(**config)
scheduler = scheduler_class(**scheduler_config)
state = scheduler.create_state()
key1, key2 = random.split(random.PRNGKey(0))
num_inference_steps = 10
model = self.dummy_model()
sample = self.dummy_sample_deter
state = scheduler.set_timesteps(state, num_inference_steps)
for t in state.timesteps:
residual = model(sample, t)
output = scheduler.step(state, residual, t, sample)
sample = output.prev_sample
state = output.state
key1, key2 = random.split(key2)
return sample
def check_over_configs(self, time_step=0, **config):
kwargs = dict(self.forward_default_kwargs)
num_inference_steps = kwargs.pop("num_inference_steps", None)
for scheduler_class in self.scheduler_classes:
sample, _ = self.dummy_sample
residual = 0.1 * sample
scheduler_config = self.get_scheduler_config(**config)
scheduler = scheduler_class(**scheduler_config)
state = scheduler.create_state()
with tempfile.TemporaryDirectory() as tmpdirname:
scheduler.save_config(tmpdirname)
new_scheduler, new_state = scheduler_class.from_pretrained(tmpdirname)
if num_inference_steps is not None and hasattr(scheduler, "set_timesteps"):
state = scheduler.set_timesteps(state, num_inference_steps)
new_state = new_scheduler.set_timesteps(new_state, num_inference_steps)
elif num_inference_steps is not None and not hasattr(scheduler, "set_timesteps"):
kwargs["num_inference_steps"] = num_inference_steps
output = scheduler.step(state, residual, time_step, sample, **kwargs).prev_sample
new_output = new_scheduler.step(new_state, residual, time_step, sample, **kwargs).prev_sample
assert jnp.sum(jnp.abs(output - new_output)) < 1e-5, "Scheduler outputs are not identical"
def test_from_save_pretrained(self):
kwargs = dict(self.forward_default_kwargs)
num_inference_steps = kwargs.pop("num_inference_steps", None)
for scheduler_class in self.scheduler_classes:
sample, _ = self.dummy_sample
residual = 0.1 * sample
scheduler_config = self.get_scheduler_config()
scheduler = scheduler_class(**scheduler_config)
state = scheduler.create_state()
with tempfile.TemporaryDirectory() as tmpdirname:
scheduler.save_config(tmpdirname)
new_scheduler, new_state = scheduler_class.from_pretrained(tmpdirname)
if num_inference_steps is not None and hasattr(scheduler, "set_timesteps"):
state = scheduler.set_timesteps(state, num_inference_steps)
new_state = new_scheduler.set_timesteps(new_state, num_inference_steps)
elif num_inference_steps is not None and not hasattr(scheduler, "set_timesteps"):
kwargs["num_inference_steps"] = num_inference_steps
output = scheduler.step(state, residual, 1, sample, **kwargs).prev_sample
new_output = new_scheduler.step(new_state, residual, 1, sample, **kwargs).prev_sample
assert jnp.sum(jnp.abs(output - new_output)) < 1e-5, "Scheduler outputs are not identical"
def check_over_forward(self, time_step=0, **forward_kwargs):
kwargs = dict(self.forward_default_kwargs)
kwargs.update(forward_kwargs)
num_inference_steps = kwargs.pop("num_inference_steps", None)
for scheduler_class in self.scheduler_classes:
sample, _ = self.dummy_sample
residual = 0.1 * sample
scheduler_config = self.get_scheduler_config()
scheduler = scheduler_class(**scheduler_config)
state = scheduler.create_state()
with tempfile.TemporaryDirectory() as tmpdirname:
scheduler.save_config(tmpdirname)
new_scheduler, new_state = scheduler_class.from_pretrained(tmpdirname)
if num_inference_steps is not None and hasattr(scheduler, "set_timesteps"):
state = scheduler.set_timesteps(state, num_inference_steps)
new_state = new_scheduler.set_timesteps(new_state, num_inference_steps)
elif num_inference_steps is not None and not hasattr(scheduler, "set_timesteps"):
kwargs["num_inference_steps"] = num_inference_steps
output = scheduler.step(state, residual, time_step, sample, **kwargs).prev_sample
new_output = new_scheduler.step(new_state, residual, time_step, sample, **kwargs).prev_sample
assert jnp.sum(jnp.abs(output - new_output)) < 1e-5, "Scheduler outputs are not identical"
def test_scheduler_outputs_equivalence(self):
def set_nan_tensor_to_zero(t):
return t.at[t != t].set(0)
def recursive_check(tuple_object, dict_object):
if isinstance(tuple_object, (List, Tuple)):
for tuple_iterable_value, dict_iterable_value in zip(tuple_object, dict_object.values()):
recursive_check(tuple_iterable_value, dict_iterable_value)
elif isinstance(tuple_object, Dict):
for tuple_iterable_value, dict_iterable_value in zip(tuple_object.values(), dict_object.values()):
recursive_check(tuple_iterable_value, dict_iterable_value)
elif tuple_object is None:
return
else:
self.assertTrue(
jnp.allclose(set_nan_tensor_to_zero(tuple_object), set_nan_tensor_to_zero(dict_object), atol=1e-5),
msg=(
"Tuple and dict output are not equal. Difference:"
f" {jnp.max(jnp.abs(tuple_object - dict_object))}. Tuple has `nan`:"
f" {jnp.isnan(tuple_object).any()} and `inf`: {jnp.isinf(tuple_object)}. Dict has"
f" `nan`: {jnp.isnan(dict_object).any()} and `inf`: {jnp.isinf(dict_object)}."
),
)
kwargs = dict(self.forward_default_kwargs)
num_inference_steps = kwargs.pop("num_inference_steps", None)
for scheduler_class in self.scheduler_classes:
scheduler_config = self.get_scheduler_config()
scheduler = scheduler_class(**scheduler_config)
state = scheduler.create_state()
sample, _ = self.dummy_sample
residual = 0.1 * sample
if num_inference_steps is not None and hasattr(scheduler, "set_timesteps"):
state = scheduler.set_timesteps(state, num_inference_steps)
elif num_inference_steps is not None and not hasattr(scheduler, "set_timesteps"):
kwargs["num_inference_steps"] = num_inference_steps
outputs_dict = scheduler.step(state, residual, 0, sample, **kwargs)
if num_inference_steps is not None and hasattr(scheduler, "set_timesteps"):
state = scheduler.set_timesteps(state, num_inference_steps)
elif num_inference_steps is not None and not hasattr(scheduler, "set_timesteps"):
kwargs["num_inference_steps"] = num_inference_steps
outputs_tuple = scheduler.step(state, residual, 0, sample, return_dict=False, **kwargs)
recursive_check(outputs_tuple[0], outputs_dict.prev_sample)
def test_step_shape(self):
kwargs = dict(self.forward_default_kwargs)
num_inference_steps = kwargs.pop("num_inference_steps", None)
for scheduler_class in self.scheduler_classes:
scheduler_config = self.get_scheduler_config()
scheduler = scheduler_class(**scheduler_config)
state = scheduler.create_state()
sample, _ = self.dummy_sample
residual = 0.1 * sample
if num_inference_steps is not None and hasattr(scheduler, "set_timesteps"):
state = scheduler.set_timesteps(state, num_inference_steps)
elif num_inference_steps is not None and not hasattr(scheduler, "set_timesteps"):
kwargs["num_inference_steps"] = num_inference_steps
output_0 = scheduler.step(state, residual, 0, sample, **kwargs).prev_sample
output_1 = scheduler.step(state, residual, 1, sample, **kwargs).prev_sample
self.assertEqual(output_0.shape, sample.shape)
self.assertEqual(output_0.shape, output_1.shape)
def test_timesteps(self):
for timesteps in [100, 500, 1000]:
self.check_over_configs(num_train_timesteps=timesteps)
def test_steps_offset(self):
for steps_offset in [0, 1]:
self.check_over_configs(steps_offset=steps_offset)
scheduler_class = self.scheduler_classes[0]
scheduler_config = self.get_scheduler_config(steps_offset=1)
scheduler = scheduler_class(**scheduler_config)
state = scheduler.create_state()
state = scheduler.set_timesteps(state, 5)
assert jnp.equal(state.timesteps, jnp.array([801, 601, 401, 201, 1])).all()
def test_betas(self):
for beta_start, beta_end in zip([0.0001, 0.001, 0.01, 0.1], [0.002, 0.02, 0.2, 2]):
self.check_over_configs(beta_start=beta_start, beta_end=beta_end)
def test_schedules(self):
for schedule in ["linear", "squaredcos_cap_v2"]:
self.check_over_configs(beta_schedule=schedule)
def test_time_indices(self):
for t in [1, 10, 49]:
self.check_over_forward(time_step=t)
def test_inference_steps(self):
for t, num_inference_steps in zip([1, 10, 50], [10, 50, 500]):
self.check_over_forward(time_step=t, num_inference_steps=num_inference_steps)
def test_variance(self):
scheduler_class = self.scheduler_classes[0]
scheduler_config = self.get_scheduler_config()
scheduler = scheduler_class(**scheduler_config)
state = scheduler.create_state()
assert jnp.sum(jnp.abs(scheduler._get_variance(state, 0, 0) - 0.0)) < 1e-5
assert jnp.sum(jnp.abs(scheduler._get_variance(state, 420, 400) - 0.14771)) < 1e-5
assert jnp.sum(jnp.abs(scheduler._get_variance(state, 980, 960) - 0.32460)) < 1e-5
assert jnp.sum(jnp.abs(scheduler._get_variance(state, 0, 0) - 0.0)) < 1e-5
assert jnp.sum(jnp.abs(scheduler._get_variance(state, 487, 486) - 0.00979)) < 1e-5
assert jnp.sum(jnp.abs(scheduler._get_variance(state, 999, 998) - 0.02)) < 1e-5
def test_full_loop_no_noise(self):
sample = self.full_loop()
result_sum = jnp.sum(jnp.abs(sample))
result_mean = jnp.mean(jnp.abs(sample))
assert abs(result_sum - 172.0067) < 1e-2
assert abs(result_mean - 0.223967) < 1e-3
def test_full_loop_with_set_alpha_to_one(self):
# We specify different beta, so that the first alpha is 0.99
sample = self.full_loop(set_alpha_to_one=True, beta_start=0.01)
result_sum = jnp.sum(jnp.abs(sample))
result_mean = jnp.mean(jnp.abs(sample))
if jax_device == "tpu":
assert abs(result_sum - 149.8409) < 1e-2
assert abs(result_mean - 0.1951) < 1e-3
else:
assert abs(result_sum - 149.8295) < 1e-2
assert abs(result_mean - 0.1951) < 1e-3
def test_full_loop_with_no_set_alpha_to_one(self):
# We specify different beta, so that the first alpha is 0.99
sample = self.full_loop(set_alpha_to_one=False, beta_start=0.01)
result_sum = jnp.sum(jnp.abs(sample))
result_mean = jnp.mean(jnp.abs(sample))
if jax_device == "tpu":
pass
# FIXME: both result_sum and result_mean are nan on TPU
# assert jnp.isnan(result_sum)
# assert jnp.isnan(result_mean)
else:
assert abs(result_sum - 149.0784) < 1e-2
assert abs(result_mean - 0.1941) < 1e-3
def test_prediction_type(self):
for prediction_type in ["epsilon", "sample", "v_prediction"]:
self.check_over_configs(prediction_type=prediction_type)
@require_flax
class FlaxPNDMSchedulerTest(FlaxSchedulerCommonTest):
scheduler_classes = (FlaxPNDMScheduler,)
forward_default_kwargs = (("num_inference_steps", 50),)
def get_scheduler_config(self, **kwargs):
config = {
"num_train_timesteps": 1000,
"beta_start": 0.0001,
"beta_end": 0.02,
"beta_schedule": "linear",
}
config.update(**kwargs)
return config
def check_over_configs(self, time_step=0, **config):
kwargs = dict(self.forward_default_kwargs)
num_inference_steps = kwargs.pop("num_inference_steps", None)
sample, _ = self.dummy_sample
residual = 0.1 * sample
dummy_past_residuals = jnp.array([residual + 0.2, residual + 0.15, residual + 0.1, residual + 0.05])
for scheduler_class in self.scheduler_classes:
scheduler_config = self.get_scheduler_config(**config)
scheduler = scheduler_class(**scheduler_config)
state = scheduler.create_state()
state = scheduler.set_timesteps(state, num_inference_steps, shape=sample.shape)
# copy over dummy past residuals
state = state.replace(ets=dummy_past_residuals[:])
with tempfile.TemporaryDirectory() as tmpdirname:
scheduler.save_config(tmpdirname)
new_scheduler, new_state = scheduler_class.from_pretrained(tmpdirname)
new_state = new_scheduler.set_timesteps(new_state, num_inference_steps, shape=sample.shape)
# copy over dummy past residuals
new_state = new_state.replace(ets=dummy_past_residuals[:])
(prev_sample, state) = scheduler.step_prk(state, residual, time_step, sample, **kwargs)
(new_prev_sample, new_state) = new_scheduler.step_prk(new_state, residual, time_step, sample, **kwargs)
assert jnp.sum(jnp.abs(prev_sample - new_prev_sample)) < 1e-5, "Scheduler outputs are not identical"
output, _ = scheduler.step_plms(state, residual, time_step, sample, **kwargs)
new_output, _ = new_scheduler.step_plms(new_state, residual, time_step, sample, **kwargs)
assert jnp.sum(jnp.abs(output - new_output)) < 1e-5, "Scheduler outputs are not identical"
def test_from_save_pretrained(self):
pass
def test_scheduler_outputs_equivalence(self):
def set_nan_tensor_to_zero(t):
return t.at[t != t].set(0)
def recursive_check(tuple_object, dict_object):
if isinstance(tuple_object, (List, Tuple)):
for tuple_iterable_value, dict_iterable_value in zip(tuple_object, dict_object.values()):
recursive_check(tuple_iterable_value, dict_iterable_value)
elif isinstance(tuple_object, Dict):
for tuple_iterable_value, dict_iterable_value in zip(tuple_object.values(), dict_object.values()):
recursive_check(tuple_iterable_value, dict_iterable_value)
elif tuple_object is None:
return
else:
self.assertTrue(
jnp.allclose(set_nan_tensor_to_zero(tuple_object), set_nan_tensor_to_zero(dict_object), atol=1e-5),
msg=(
"Tuple and dict output are not equal. Difference:"
f" {jnp.max(jnp.abs(tuple_object - dict_object))}. Tuple has `nan`:"
f" {jnp.isnan(tuple_object).any()} and `inf`: {jnp.isinf(tuple_object)}. Dict has"
f" `nan`: {jnp.isnan(dict_object).any()} and `inf`: {jnp.isinf(dict_object)}."
),
)
kwargs = dict(self.forward_default_kwargs)
num_inference_steps = kwargs.pop("num_inference_steps", None)
for scheduler_class in self.scheduler_classes:
scheduler_config = self.get_scheduler_config()
scheduler = scheduler_class(**scheduler_config)
state = scheduler.create_state()
sample, _ = self.dummy_sample
residual = 0.1 * sample
if num_inference_steps is not None and hasattr(scheduler, "set_timesteps"):
state = scheduler.set_timesteps(state, num_inference_steps, shape=sample.shape)
elif num_inference_steps is not None and not hasattr(scheduler, "set_timesteps"):
kwargs["num_inference_steps"] = num_inference_steps
outputs_dict = scheduler.step(state, residual, 0, sample, **kwargs)
if num_inference_steps is not None and hasattr(scheduler, "set_timesteps"):
state = scheduler.set_timesteps(state, num_inference_steps, shape=sample.shape)
elif num_inference_steps is not None and not hasattr(scheduler, "set_timesteps"):
kwargs["num_inference_steps"] = num_inference_steps
outputs_tuple = scheduler.step(state, residual, 0, sample, return_dict=False, **kwargs)
recursive_check(outputs_tuple[0], outputs_dict.prev_sample)
def check_over_forward(self, time_step=0, **forward_kwargs):
kwargs = dict(self.forward_default_kwargs)
num_inference_steps = kwargs.pop("num_inference_steps", None)
sample, _ = self.dummy_sample
residual = 0.1 * sample
dummy_past_residuals = jnp.array([residual + 0.2, residual + 0.15, residual + 0.1, residual + 0.05])
for scheduler_class in self.scheduler_classes:
scheduler_config = self.get_scheduler_config()
scheduler = scheduler_class(**scheduler_config)
state = scheduler.create_state()
state = scheduler.set_timesteps(state, num_inference_steps, shape=sample.shape)
# copy over dummy past residuals (must be after setting timesteps)
scheduler.ets = dummy_past_residuals[:]
with tempfile.TemporaryDirectory() as tmpdirname:
scheduler.save_config(tmpdirname)
new_scheduler, new_state = scheduler_class.from_pretrained(tmpdirname)
# copy over dummy past residuals
new_state = new_scheduler.set_timesteps(new_state, num_inference_steps, shape=sample.shape)
# copy over dummy past residual (must be after setting timesteps)
new_state.replace(ets=dummy_past_residuals[:])
output, state = scheduler.step_prk(state, residual, time_step, sample, **kwargs)
new_output, new_state = new_scheduler.step_prk(new_state, residual, time_step, sample, **kwargs)
assert jnp.sum(jnp.abs(output - new_output)) < 1e-5, "Scheduler outputs are not identical"
output, _ = scheduler.step_plms(state, residual, time_step, sample, **kwargs)
new_output, _ = new_scheduler.step_plms(new_state, residual, time_step, sample, **kwargs)
assert jnp.sum(jnp.abs(output - new_output)) < 1e-5, "Scheduler outputs are not identical"
def full_loop(self, **config):
scheduler_class = self.scheduler_classes[0]
scheduler_config = self.get_scheduler_config(**config)
scheduler = scheduler_class(**scheduler_config)
state = scheduler.create_state()
num_inference_steps = 10
model = self.dummy_model()
sample = self.dummy_sample_deter
state = scheduler.set_timesteps(state, num_inference_steps, shape=sample.shape)
for i, t in enumerate(state.prk_timesteps):
residual = model(sample, t)
sample, state = scheduler.step_prk(state, residual, t, sample)
for i, t in enumerate(state.plms_timesteps):
residual = model(sample, t)
sample, state = scheduler.step_plms(state, residual, t, sample)
return sample
def test_step_shape(self):
kwargs = dict(self.forward_default_kwargs)
num_inference_steps = kwargs.pop("num_inference_steps", None)
for scheduler_class in self.scheduler_classes:
scheduler_config = self.get_scheduler_config()
scheduler = scheduler_class(**scheduler_config)
state = scheduler.create_state()
sample, _ = self.dummy_sample
residual = 0.1 * sample
if num_inference_steps is not None and hasattr(scheduler, "set_timesteps"):
state = scheduler.set_timesteps(state, num_inference_steps, shape=sample.shape)
elif num_inference_steps is not None and not hasattr(scheduler, "set_timesteps"):
kwargs["num_inference_steps"] = num_inference_steps
# copy over dummy past residuals (must be done after set_timesteps)
dummy_past_residuals = jnp.array([residual + 0.2, residual + 0.15, residual + 0.1, residual + 0.05])
state = state.replace(ets=dummy_past_residuals[:])
output_0, state = scheduler.step_prk(state, residual, 0, sample, **kwargs)
output_1, state = scheduler.step_prk(state, residual, 1, sample, **kwargs)
self.assertEqual(output_0.shape, sample.shape)
self.assertEqual(output_0.shape, output_1.shape)
output_0, state = scheduler.step_plms(state, residual, 0, sample, **kwargs)
output_1, state = scheduler.step_plms(state, residual, 1, sample, **kwargs)
self.assertEqual(output_0.shape, sample.shape)
self.assertEqual(output_0.shape, output_1.shape)
def test_timesteps(self):
for timesteps in [100, 1000]:
self.check_over_configs(num_train_timesteps=timesteps)
def test_steps_offset(self):
for steps_offset in [0, 1]:
self.check_over_configs(steps_offset=steps_offset)
scheduler_class = self.scheduler_classes[0]
scheduler_config = self.get_scheduler_config(steps_offset=1)
scheduler = scheduler_class(**scheduler_config)
state = scheduler.create_state()
state = scheduler.set_timesteps(state, 10, shape=())
assert jnp.equal(
state.timesteps,
jnp.array([901, 851, 851, 801, 801, 751, 751, 701, 701, 651, 651, 601, 601, 501, 401, 301, 201, 101, 1]),
).all()
def test_betas(self):
for beta_start, beta_end in zip([0.0001, 0.001], [0.002, 0.02]):
self.check_over_configs(beta_start=beta_start, beta_end=beta_end)
def test_schedules(self):
for schedule in ["linear", "squaredcos_cap_v2"]:
self.check_over_configs(beta_schedule=schedule)
def test_time_indices(self):
for t in [1, 5, 10]:
self.check_over_forward(time_step=t)
def test_inference_steps(self):
for t, num_inference_steps in zip([1, 5, 10], [10, 50, 100]):
self.check_over_forward(num_inference_steps=num_inference_steps)
def test_pow_of_3_inference_steps(self):
# earlier version of set_timesteps() caused an error indexing alpha's with inference steps as power of 3
num_inference_steps = 27
for scheduler_class in self.scheduler_classes:
sample, _ = self.dummy_sample
residual = 0.1 * sample
scheduler_config = self.get_scheduler_config()
scheduler = scheduler_class(**scheduler_config)
state = scheduler.create_state()
state = scheduler.set_timesteps(state, num_inference_steps, shape=sample.shape)
# before power of 3 fix, would error on first step, so we only need to do two
for i, t in enumerate(state.prk_timesteps[:2]):
sample, state = scheduler.step_prk(state, residual, t, sample)
def test_inference_plms_no_past_residuals(self):
with self.assertRaises(ValueError):
scheduler_class = self.scheduler_classes[0]
scheduler_config = self.get_scheduler_config()
scheduler = scheduler_class(**scheduler_config)
state = scheduler.create_state()
scheduler.step_plms(state, self.dummy_sample, 1, self.dummy_sample).prev_sample
def test_full_loop_no_noise(self):
sample = self.full_loop()
result_sum = jnp.sum(jnp.abs(sample))
result_mean = jnp.mean(jnp.abs(sample))
if jax_device == "tpu":
assert abs(result_sum - 198.1275) < 1e-2
assert abs(result_mean - 0.2580) < 1e-3
else:
assert abs(result_sum - 198.1318) < 1e-2
assert abs(result_mean - 0.2580) < 1e-3
def test_full_loop_with_set_alpha_to_one(self):
# We specify different beta, so that the first alpha is 0.99
sample = self.full_loop(set_alpha_to_one=True, beta_start=0.01)
result_sum = jnp.sum(jnp.abs(sample))
result_mean = jnp.mean(jnp.abs(sample))
if jax_device == "tpu":
assert abs(result_sum - 186.83226) < 1e-2
assert abs(result_mean - 0.24327) < 1e-3
else:
assert abs(result_sum - 186.9466) < 1e-2
assert abs(result_mean - 0.24342) < 1e-3
def test_full_loop_with_no_set_alpha_to_one(self):
# We specify different beta, so that the first alpha is 0.99
sample = self.full_loop(set_alpha_to_one=False, beta_start=0.01)
result_sum = jnp.sum(jnp.abs(sample))
result_mean = jnp.mean(jnp.abs(sample))
if jax_device == "tpu":
assert abs(result_sum - 186.83226) < 1e-2
assert abs(result_mean - 0.24327) < 1e-3
else:
assert abs(result_sum - 186.9482) < 1e-2
assert abs(result_mean - 0.2434) < 1e-3
| 0 |
hf_public_repos/diffusers/tests | hf_public_repos/diffusers/tests/schedulers/test_scheduler_ddim_parallel.py | # Copyright 2023 ParaDiGMS authors and The HuggingFace Team. All rights reserved.
#
# Licensed under the Apache License, Version 2.0 (the "License");
# you may not use this file except in compliance with the License.
# You may obtain a copy of the License at
#
# http://www.apache.org/licenses/LICENSE-2.0
#
# Unless required by applicable law or agreed to in writing, software
# distributed under the License is distributed on an "AS IS" BASIS,
# WITHOUT WARRANTIES OR CONDITIONS OF ANY KIND, either express or implied.
# See the License for the specific language governing permissions and
# limitations under the License.
import torch
from diffusers import DDIMParallelScheduler
from .test_schedulers import SchedulerCommonTest
class DDIMParallelSchedulerTest(SchedulerCommonTest):
scheduler_classes = (DDIMParallelScheduler,)
forward_default_kwargs = (("eta", 0.0), ("num_inference_steps", 50))
def get_scheduler_config(self, **kwargs):
config = {
"num_train_timesteps": 1000,
"beta_start": 0.0001,
"beta_end": 0.02,
"beta_schedule": "linear",
"clip_sample": True,
}
config.update(**kwargs)
return config
def full_loop(self, **config):
scheduler_class = self.scheduler_classes[0]
scheduler_config = self.get_scheduler_config(**config)
scheduler = scheduler_class(**scheduler_config)
num_inference_steps, eta = 10, 0.0
model = self.dummy_model()
sample = self.dummy_sample_deter
scheduler.set_timesteps(num_inference_steps)
for t in scheduler.timesteps:
residual = model(sample, t)
sample = scheduler.step(residual, t, sample, eta).prev_sample
return sample
def test_timesteps(self):
for timesteps in [100, 500, 1000]:
self.check_over_configs(num_train_timesteps=timesteps)
def test_steps_offset(self):
for steps_offset in [0, 1]:
self.check_over_configs(steps_offset=steps_offset)
scheduler_class = self.scheduler_classes[0]
scheduler_config = self.get_scheduler_config(steps_offset=1)
scheduler = scheduler_class(**scheduler_config)
scheduler.set_timesteps(5)
assert torch.equal(scheduler.timesteps, torch.LongTensor([801, 601, 401, 201, 1]))
def test_betas(self):
for beta_start, beta_end in zip([0.0001, 0.001, 0.01, 0.1], [0.002, 0.02, 0.2, 2]):
self.check_over_configs(beta_start=beta_start, beta_end=beta_end)
def test_schedules(self):
for schedule in ["linear", "squaredcos_cap_v2"]:
self.check_over_configs(beta_schedule=schedule)
def test_prediction_type(self):
for prediction_type in ["epsilon", "v_prediction"]:
self.check_over_configs(prediction_type=prediction_type)
def test_clip_sample(self):
for clip_sample in [True, False]:
self.check_over_configs(clip_sample=clip_sample)
def test_timestep_spacing(self):
for timestep_spacing in ["trailing", "leading"]:
self.check_over_configs(timestep_spacing=timestep_spacing)
def test_rescale_betas_zero_snr(self):
for rescale_betas_zero_snr in [True, False]:
self.check_over_configs(rescale_betas_zero_snr=rescale_betas_zero_snr)
def test_thresholding(self):
self.check_over_configs(thresholding=False)
for threshold in [0.5, 1.0, 2.0]:
for prediction_type in ["epsilon", "v_prediction"]:
self.check_over_configs(
thresholding=True,
prediction_type=prediction_type,
sample_max_value=threshold,
)
def test_time_indices(self):
for t in [1, 10, 49]:
self.check_over_forward(time_step=t)
def test_inference_steps(self):
for t, num_inference_steps in zip([1, 10, 50], [10, 50, 500]):
self.check_over_forward(time_step=t, num_inference_steps=num_inference_steps)
def test_eta(self):
for t, eta in zip([1, 10, 49], [0.0, 0.5, 1.0]):
self.check_over_forward(time_step=t, eta=eta)
def test_variance(self):
scheduler_class = self.scheduler_classes[0]
scheduler_config = self.get_scheduler_config()
scheduler = scheduler_class(**scheduler_config)
assert torch.sum(torch.abs(scheduler._get_variance(0, 0) - 0.0)) < 1e-5
assert torch.sum(torch.abs(scheduler._get_variance(420, 400) - 0.14771)) < 1e-5
assert torch.sum(torch.abs(scheduler._get_variance(980, 960) - 0.32460)) < 1e-5
assert torch.sum(torch.abs(scheduler._get_variance(0, 0) - 0.0)) < 1e-5
assert torch.sum(torch.abs(scheduler._get_variance(487, 486) - 0.00979)) < 1e-5
assert torch.sum(torch.abs(scheduler._get_variance(999, 998) - 0.02)) < 1e-5
def test_batch_step_no_noise(self):
scheduler_class = self.scheduler_classes[0]
scheduler_config = self.get_scheduler_config()
scheduler = scheduler_class(**scheduler_config)
num_inference_steps, eta = 10, 0.0
scheduler.set_timesteps(num_inference_steps)
model = self.dummy_model()
sample1 = self.dummy_sample_deter
sample2 = self.dummy_sample_deter + 0.1
sample3 = self.dummy_sample_deter - 0.1
per_sample_batch = sample1.shape[0]
samples = torch.stack([sample1, sample2, sample3], dim=0)
timesteps = torch.arange(num_inference_steps)[0:3, None].repeat(1, per_sample_batch)
residual = model(samples.flatten(0, 1), timesteps.flatten(0, 1))
pred_prev_sample = scheduler.batch_step_no_noise(residual, timesteps.flatten(0, 1), samples.flatten(0, 1), eta)
result_sum = torch.sum(torch.abs(pred_prev_sample))
result_mean = torch.mean(torch.abs(pred_prev_sample))
assert abs(result_sum.item() - 1147.7904) < 1e-2
assert abs(result_mean.item() - 0.4982) < 1e-3
def test_full_loop_no_noise(self):
sample = self.full_loop()
result_sum = torch.sum(torch.abs(sample))
result_mean = torch.mean(torch.abs(sample))
assert abs(result_sum.item() - 172.0067) < 1e-2
assert abs(result_mean.item() - 0.223967) < 1e-3
def test_full_loop_with_v_prediction(self):
sample = self.full_loop(prediction_type="v_prediction")
result_sum = torch.sum(torch.abs(sample))
result_mean = torch.mean(torch.abs(sample))
assert abs(result_sum.item() - 52.5302) < 1e-2
assert abs(result_mean.item() - 0.0684) < 1e-3
def test_full_loop_with_set_alpha_to_one(self):
# We specify different beta, so that the first alpha is 0.99
sample = self.full_loop(set_alpha_to_one=True, beta_start=0.01)
result_sum = torch.sum(torch.abs(sample))
result_mean = torch.mean(torch.abs(sample))
assert abs(result_sum.item() - 149.8295) < 1e-2
assert abs(result_mean.item() - 0.1951) < 1e-3
def test_full_loop_with_no_set_alpha_to_one(self):
# We specify different beta, so that the first alpha is 0.99
sample = self.full_loop(set_alpha_to_one=False, beta_start=0.01)
result_sum = torch.sum(torch.abs(sample))
result_mean = torch.mean(torch.abs(sample))
assert abs(result_sum.item() - 149.0784) < 1e-2
assert abs(result_mean.item() - 0.1941) < 1e-3
| 0 |
hf_public_repos/diffusers/tests | hf_public_repos/diffusers/tests/schedulers/test_scheduler_dpm_single.py | import tempfile
import torch
from diffusers import (
DEISMultistepScheduler,
DPMSolverMultistepScheduler,
DPMSolverSinglestepScheduler,
UniPCMultistepScheduler,
)
from .test_schedulers import SchedulerCommonTest
class DPMSolverSinglestepSchedulerTest(SchedulerCommonTest):
scheduler_classes = (DPMSolverSinglestepScheduler,)
forward_default_kwargs = (("num_inference_steps", 25),)
def get_scheduler_config(self, **kwargs):
config = {
"num_train_timesteps": 1000,
"beta_start": 0.0001,
"beta_end": 0.02,
"beta_schedule": "linear",
"solver_order": 2,
"prediction_type": "epsilon",
"thresholding": False,
"sample_max_value": 1.0,
"algorithm_type": "dpmsolver++",
"solver_type": "midpoint",
"lambda_min_clipped": -float("inf"),
"variance_type": None,
}
config.update(**kwargs)
return config
def check_over_configs(self, time_step=0, **config):
kwargs = dict(self.forward_default_kwargs)
num_inference_steps = kwargs.pop("num_inference_steps", None)
sample = self.dummy_sample
residual = 0.1 * sample
dummy_past_residuals = [residual + 0.2, residual + 0.15, residual + 0.10]
for scheduler_class in self.scheduler_classes:
scheduler_config = self.get_scheduler_config(**config)
scheduler = scheduler_class(**scheduler_config)
scheduler.set_timesteps(num_inference_steps)
# copy over dummy past residuals
scheduler.model_outputs = dummy_past_residuals[: scheduler.config.solver_order]
with tempfile.TemporaryDirectory() as tmpdirname:
scheduler.save_config(tmpdirname)
new_scheduler = scheduler_class.from_pretrained(tmpdirname)
new_scheduler.set_timesteps(num_inference_steps)
# copy over dummy past residuals
new_scheduler.model_outputs = dummy_past_residuals[: new_scheduler.config.solver_order]
output, new_output = sample, sample
for t in range(time_step, time_step + scheduler.config.solver_order + 1):
output = scheduler.step(residual, t, output, **kwargs).prev_sample
new_output = new_scheduler.step(residual, t, new_output, **kwargs).prev_sample
assert torch.sum(torch.abs(output - new_output)) < 1e-5, "Scheduler outputs are not identical"
def test_from_save_pretrained(self):
pass
def check_over_forward(self, time_step=0, **forward_kwargs):
kwargs = dict(self.forward_default_kwargs)
num_inference_steps = kwargs.pop("num_inference_steps", None)
sample = self.dummy_sample
residual = 0.1 * sample
dummy_past_residuals = [residual + 0.2, residual + 0.15, residual + 0.10]
for scheduler_class in self.scheduler_classes:
scheduler_config = self.get_scheduler_config()
scheduler = scheduler_class(**scheduler_config)
scheduler.set_timesteps(num_inference_steps)
# copy over dummy past residuals (must be after setting timesteps)
scheduler.model_outputs = dummy_past_residuals[: scheduler.config.solver_order]
with tempfile.TemporaryDirectory() as tmpdirname:
scheduler.save_config(tmpdirname)
new_scheduler = scheduler_class.from_pretrained(tmpdirname)
# copy over dummy past residuals
new_scheduler.set_timesteps(num_inference_steps)
# copy over dummy past residual (must be after setting timesteps)
new_scheduler.model_outputs = dummy_past_residuals[: new_scheduler.config.solver_order]
output = scheduler.step(residual, time_step, sample, **kwargs).prev_sample
new_output = new_scheduler.step(residual, time_step, sample, **kwargs).prev_sample
assert torch.sum(torch.abs(output - new_output)) < 1e-5, "Scheduler outputs are not identical"
def full_loop(self, scheduler=None, **config):
if scheduler is None:
scheduler_class = self.scheduler_classes[0]
scheduler_config = self.get_scheduler_config(**config)
scheduler = scheduler_class(**scheduler_config)
scheduler_class = self.scheduler_classes[0]
scheduler_config = self.get_scheduler_config(**config)
scheduler = scheduler_class(**scheduler_config)
num_inference_steps = 10
model = self.dummy_model()
sample = self.dummy_sample_deter
scheduler.set_timesteps(num_inference_steps)
for i, t in enumerate(scheduler.timesteps):
residual = model(sample, t)
sample = scheduler.step(residual, t, sample).prev_sample
return sample
def test_full_uneven_loop(self):
scheduler = DPMSolverSinglestepScheduler(**self.get_scheduler_config())
num_inference_steps = 50
model = self.dummy_model()
sample = self.dummy_sample_deter
scheduler.set_timesteps(num_inference_steps)
# make sure that the first t is uneven
for i, t in enumerate(scheduler.timesteps[3:]):
residual = model(sample, t)
sample = scheduler.step(residual, t, sample).prev_sample
result_mean = torch.mean(torch.abs(sample))
assert abs(result_mean.item() - 0.2574) < 1e-3
def test_timesteps(self):
for timesteps in [25, 50, 100, 999, 1000]:
self.check_over_configs(num_train_timesteps=timesteps)
def test_switch(self):
# make sure that iterating over schedulers with same config names gives same results
# for defaults
scheduler = DPMSolverSinglestepScheduler(**self.get_scheduler_config())
sample = self.full_loop(scheduler=scheduler)
result_mean = torch.mean(torch.abs(sample))
assert abs(result_mean.item() - 0.2791) < 1e-3
scheduler = DEISMultistepScheduler.from_config(scheduler.config)
scheduler = DPMSolverMultistepScheduler.from_config(scheduler.config)
scheduler = UniPCMultistepScheduler.from_config(scheduler.config)
scheduler = DPMSolverSinglestepScheduler.from_config(scheduler.config)
sample = self.full_loop(scheduler=scheduler)
result_mean = torch.mean(torch.abs(sample))
assert abs(result_mean.item() - 0.2791) < 1e-3
def test_thresholding(self):
self.check_over_configs(thresholding=False)
for order in [1, 2, 3]:
for solver_type in ["midpoint", "heun"]:
for threshold in [0.5, 1.0, 2.0]:
for prediction_type in ["epsilon", "sample"]:
self.check_over_configs(
thresholding=True,
prediction_type=prediction_type,
sample_max_value=threshold,
algorithm_type="dpmsolver++",
solver_order=order,
solver_type=solver_type,
)
def test_prediction_type(self):
for prediction_type in ["epsilon", "v_prediction"]:
self.check_over_configs(prediction_type=prediction_type)
def test_solver_order_and_type(self):
for algorithm_type in ["dpmsolver", "dpmsolver++"]:
for solver_type in ["midpoint", "heun"]:
for order in [1, 2, 3]:
for prediction_type in ["epsilon", "sample"]:
self.check_over_configs(
solver_order=order,
solver_type=solver_type,
prediction_type=prediction_type,
algorithm_type=algorithm_type,
)
sample = self.full_loop(
solver_order=order,
solver_type=solver_type,
prediction_type=prediction_type,
algorithm_type=algorithm_type,
)
assert not torch.isnan(sample).any(), "Samples have nan numbers"
def test_lower_order_final(self):
self.check_over_configs(lower_order_final=True)
self.check_over_configs(lower_order_final=False)
def test_lambda_min_clipped(self):
self.check_over_configs(lambda_min_clipped=-float("inf"))
self.check_over_configs(lambda_min_clipped=-5.1)
def test_variance_type(self):
self.check_over_configs(variance_type=None)
self.check_over_configs(variance_type="learned_range")
def test_inference_steps(self):
for num_inference_steps in [1, 2, 3, 5, 10, 50, 100, 999, 1000]:
self.check_over_forward(num_inference_steps=num_inference_steps, time_step=0)
def test_full_loop_no_noise(self):
sample = self.full_loop()
result_mean = torch.mean(torch.abs(sample))
assert abs(result_mean.item() - 0.2791) < 1e-3
def test_full_loop_with_karras(self):
sample = self.full_loop(use_karras_sigmas=True)
result_mean = torch.mean(torch.abs(sample))
assert abs(result_mean.item() - 0.2248) < 1e-3
def test_full_loop_with_v_prediction(self):
sample = self.full_loop(prediction_type="v_prediction")
result_mean = torch.mean(torch.abs(sample))
assert abs(result_mean.item() - 0.1453) < 1e-3
def test_full_loop_with_karras_and_v_prediction(self):
sample = self.full_loop(prediction_type="v_prediction", use_karras_sigmas=True)
result_mean = torch.mean(torch.abs(sample))
assert abs(result_mean.item() - 0.0649) < 1e-3
def test_fp16_support(self):
scheduler_class = self.scheduler_classes[0]
scheduler_config = self.get_scheduler_config(thresholding=True, dynamic_thresholding_ratio=0)
scheduler = scheduler_class(**scheduler_config)
num_inference_steps = 10
model = self.dummy_model()
sample = self.dummy_sample_deter.half()
scheduler.set_timesteps(num_inference_steps)
for i, t in enumerate(scheduler.timesteps):
residual = model(sample, t)
sample = scheduler.step(residual, t, sample).prev_sample
assert sample.dtype == torch.float16
| 0 |
hf_public_repos/diffusers/tests | hf_public_repos/diffusers/tests/schedulers/test_scheduler_ddim_inverse.py | import torch
from diffusers import DDIMInverseScheduler
from .test_schedulers import SchedulerCommonTest
class DDIMInverseSchedulerTest(SchedulerCommonTest):
scheduler_classes = (DDIMInverseScheduler,)
forward_default_kwargs = (("eta", 0.0), ("num_inference_steps", 50))
def get_scheduler_config(self, **kwargs):
config = {
"num_train_timesteps": 1000,
"beta_start": 0.0001,
"beta_end": 0.02,
"beta_schedule": "linear",
"clip_sample": True,
}
config.update(**kwargs)
return config
def full_loop(self, **config):
scheduler_class = self.scheduler_classes[0]
scheduler_config = self.get_scheduler_config(**config)
scheduler = scheduler_class(**scheduler_config)
num_inference_steps, eta = 10, 0.0
model = self.dummy_model()
sample = self.dummy_sample_deter
scheduler.set_timesteps(num_inference_steps)
for t in scheduler.timesteps:
residual = model(sample, t)
sample = scheduler.step(residual, t, sample, eta).prev_sample
return sample
def test_timesteps(self):
for timesteps in [100, 500, 1000]:
self.check_over_configs(num_train_timesteps=timesteps)
def test_steps_offset(self):
for steps_offset in [0, 1]:
self.check_over_configs(steps_offset=steps_offset)
scheduler_class = self.scheduler_classes[0]
scheduler_config = self.get_scheduler_config(steps_offset=1)
scheduler = scheduler_class(**scheduler_config)
scheduler.set_timesteps(5)
assert torch.equal(scheduler.timesteps, torch.LongTensor([-199, 1, 201, 401, 601]))
def test_betas(self):
for beta_start, beta_end in zip([0.0001, 0.001, 0.01, 0.1], [0.002, 0.02, 0.2, 2]):
self.check_over_configs(beta_start=beta_start, beta_end=beta_end)
def test_schedules(self):
for schedule in ["linear", "squaredcos_cap_v2"]:
self.check_over_configs(beta_schedule=schedule)
def test_prediction_type(self):
for prediction_type in ["epsilon", "v_prediction"]:
self.check_over_configs(prediction_type=prediction_type)
def test_clip_sample(self):
for clip_sample in [True, False]:
self.check_over_configs(clip_sample=clip_sample)
def test_timestep_spacing(self):
for timestep_spacing in ["trailing", "leading"]:
self.check_over_configs(timestep_spacing=timestep_spacing)
def test_rescale_betas_zero_snr(self):
for rescale_betas_zero_snr in [True, False]:
self.check_over_configs(rescale_betas_zero_snr=rescale_betas_zero_snr)
def test_thresholding(self):
self.check_over_configs(thresholding=False)
for threshold in [0.5, 1.0, 2.0]:
for prediction_type in ["epsilon", "v_prediction"]:
self.check_over_configs(
thresholding=True,
prediction_type=prediction_type,
sample_max_value=threshold,
)
def test_time_indices(self):
for t in [1, 10, 49]:
self.check_over_forward(time_step=t)
def test_inference_steps(self):
for t, num_inference_steps in zip([1, 10, 50], [10, 50, 500]):
self.check_over_forward(time_step=t, num_inference_steps=num_inference_steps)
def test_add_noise_device(self):
pass
def test_full_loop_no_noise(self):
sample = self.full_loop()
result_sum = torch.sum(torch.abs(sample))
result_mean = torch.mean(torch.abs(sample))
assert abs(result_sum.item() - 509.1079) < 1e-2
assert abs(result_mean.item() - 0.6629) < 1e-3
def test_full_loop_with_v_prediction(self):
sample = self.full_loop(prediction_type="v_prediction")
result_sum = torch.sum(torch.abs(sample))
result_mean = torch.mean(torch.abs(sample))
assert abs(result_sum.item() - 1029.129) < 1e-2
assert abs(result_mean.item() - 1.3400) < 1e-3
def test_full_loop_with_set_alpha_to_one(self):
# We specify different beta, so that the first alpha is 0.99
sample = self.full_loop(set_alpha_to_one=True, beta_start=0.01)
result_sum = torch.sum(torch.abs(sample))
result_mean = torch.mean(torch.abs(sample))
assert abs(result_sum.item() - 259.8116) < 1e-2
assert abs(result_mean.item() - 0.3383) < 1e-3
def test_full_loop_with_no_set_alpha_to_one(self):
# We specify different beta, so that the first alpha is 0.99
sample = self.full_loop(set_alpha_to_one=False, beta_start=0.01)
result_sum = torch.sum(torch.abs(sample))
result_mean = torch.mean(torch.abs(sample))
assert abs(result_sum.item() - 239.055) < 1e-2
assert abs(result_mean.item() - 0.3113) < 1e-3
| 0 |
hf_public_repos/diffusers/tests | hf_public_repos/diffusers/tests/schedulers/test_scheduler_euler_ancestral.py | import torch
from diffusers import EulerAncestralDiscreteScheduler
from diffusers.utils import torch_device
from .test_schedulers import SchedulerCommonTest
class EulerAncestralDiscreteSchedulerTest(SchedulerCommonTest):
scheduler_classes = (EulerAncestralDiscreteScheduler,)
num_inference_steps = 10
def get_scheduler_config(self, **kwargs):
config = {
"num_train_timesteps": 1100,
"beta_start": 0.0001,
"beta_end": 0.02,
"beta_schedule": "linear",
}
config.update(**kwargs)
return config
def test_timesteps(self):
for timesteps in [10, 50, 100, 1000]:
self.check_over_configs(num_train_timesteps=timesteps)
def test_betas(self):
for beta_start, beta_end in zip([0.00001, 0.0001, 0.001], [0.0002, 0.002, 0.02]):
self.check_over_configs(beta_start=beta_start, beta_end=beta_end)
def test_schedules(self):
for schedule in ["linear", "scaled_linear"]:
self.check_over_configs(beta_schedule=schedule)
def test_prediction_type(self):
for prediction_type in ["epsilon", "v_prediction"]:
self.check_over_configs(prediction_type=prediction_type)
def test_full_loop_no_noise(self):
scheduler_class = self.scheduler_classes[0]
scheduler_config = self.get_scheduler_config()
scheduler = scheduler_class(**scheduler_config)
scheduler.set_timesteps(self.num_inference_steps)
generator = torch.manual_seed(0)
model = self.dummy_model()
sample = self.dummy_sample_deter * scheduler.init_noise_sigma.cpu()
sample = sample.to(torch_device)
for i, t in enumerate(scheduler.timesteps):
sample = scheduler.scale_model_input(sample, t)
model_output = model(sample, t)
output = scheduler.step(model_output, t, sample, generator=generator)
sample = output.prev_sample
result_sum = torch.sum(torch.abs(sample))
result_mean = torch.mean(torch.abs(sample))
assert abs(result_sum.item() - 152.3192) < 1e-2
assert abs(result_mean.item() - 0.1983) < 1e-3
def test_full_loop_with_v_prediction(self):
scheduler_class = self.scheduler_classes[0]
scheduler_config = self.get_scheduler_config(prediction_type="v_prediction")
scheduler = scheduler_class(**scheduler_config)
scheduler.set_timesteps(self.num_inference_steps)
generator = torch.manual_seed(0)
model = self.dummy_model()
sample = self.dummy_sample_deter * scheduler.init_noise_sigma
sample = sample.to(torch_device)
for i, t in enumerate(scheduler.timesteps):
sample = scheduler.scale_model_input(sample, t)
model_output = model(sample, t)
output = scheduler.step(model_output, t, sample, generator=generator)
sample = output.prev_sample
result_sum = torch.sum(torch.abs(sample))
result_mean = torch.mean(torch.abs(sample))
assert abs(result_sum.item() - 108.4439) < 1e-2
assert abs(result_mean.item() - 0.1412) < 1e-3
def test_full_loop_device(self):
scheduler_class = self.scheduler_classes[0]
scheduler_config = self.get_scheduler_config()
scheduler = scheduler_class(**scheduler_config)
scheduler.set_timesteps(self.num_inference_steps, device=torch_device)
generator = torch.manual_seed(0)
model = self.dummy_model()
sample = self.dummy_sample_deter * scheduler.init_noise_sigma.cpu()
sample = sample.to(torch_device)
for t in scheduler.timesteps:
sample = scheduler.scale_model_input(sample, t)
model_output = model(sample, t)
output = scheduler.step(model_output, t, sample, generator=generator)
sample = output.prev_sample
result_sum = torch.sum(torch.abs(sample))
result_mean = torch.mean(torch.abs(sample))
assert abs(result_sum.item() - 152.3192) < 1e-2
assert abs(result_mean.item() - 0.1983) < 1e-3
| 0 |
hf_public_repos/diffusers/tests | hf_public_repos/diffusers/tests/schedulers/test_scheduler_euler.py | import torch
from diffusers import EulerDiscreteScheduler
from diffusers.utils import torch_device
from .test_schedulers import SchedulerCommonTest
class EulerDiscreteSchedulerTest(SchedulerCommonTest):
scheduler_classes = (EulerDiscreteScheduler,)
num_inference_steps = 10
def get_scheduler_config(self, **kwargs):
config = {
"num_train_timesteps": 1100,
"beta_start": 0.0001,
"beta_end": 0.02,
"beta_schedule": "linear",
}
config.update(**kwargs)
return config
def test_timesteps(self):
for timesteps in [10, 50, 100, 1000]:
self.check_over_configs(num_train_timesteps=timesteps)
def test_betas(self):
for beta_start, beta_end in zip([0.00001, 0.0001, 0.001], [0.0002, 0.002, 0.02]):
self.check_over_configs(beta_start=beta_start, beta_end=beta_end)
def test_schedules(self):
for schedule in ["linear", "scaled_linear"]:
self.check_over_configs(beta_schedule=schedule)
def test_prediction_type(self):
for prediction_type in ["epsilon", "v_prediction"]:
self.check_over_configs(prediction_type=prediction_type)
def test_full_loop_no_noise(self):
scheduler_class = self.scheduler_classes[0]
scheduler_config = self.get_scheduler_config()
scheduler = scheduler_class(**scheduler_config)
scheduler.set_timesteps(self.num_inference_steps)
generator = torch.manual_seed(0)
model = self.dummy_model()
sample = self.dummy_sample_deter * scheduler.init_noise_sigma
sample = sample.to(torch_device)
for i, t in enumerate(scheduler.timesteps):
sample = scheduler.scale_model_input(sample, t)
model_output = model(sample, t)
output = scheduler.step(model_output, t, sample, generator=generator)
sample = output.prev_sample
result_sum = torch.sum(torch.abs(sample))
result_mean = torch.mean(torch.abs(sample))
assert abs(result_sum.item() - 10.0807) < 1e-2
assert abs(result_mean.item() - 0.0131) < 1e-3
def test_full_loop_with_v_prediction(self):
scheduler_class = self.scheduler_classes[0]
scheduler_config = self.get_scheduler_config(prediction_type="v_prediction")
scheduler = scheduler_class(**scheduler_config)
scheduler.set_timesteps(self.num_inference_steps)
generator = torch.manual_seed(0)
model = self.dummy_model()
sample = self.dummy_sample_deter * scheduler.init_noise_sigma
sample = sample.to(torch_device)
for i, t in enumerate(scheduler.timesteps):
sample = scheduler.scale_model_input(sample, t)
model_output = model(sample, t)
output = scheduler.step(model_output, t, sample, generator=generator)
sample = output.prev_sample
result_sum = torch.sum(torch.abs(sample))
result_mean = torch.mean(torch.abs(sample))
assert abs(result_sum.item() - 0.0002) < 1e-2
assert abs(result_mean.item() - 2.2676e-06) < 1e-3
def test_full_loop_device(self):
scheduler_class = self.scheduler_classes[0]
scheduler_config = self.get_scheduler_config()
scheduler = scheduler_class(**scheduler_config)
scheduler.set_timesteps(self.num_inference_steps, device=torch_device)
generator = torch.manual_seed(0)
model = self.dummy_model()
sample = self.dummy_sample_deter * scheduler.init_noise_sigma.cpu()
sample = sample.to(torch_device)
for t in scheduler.timesteps:
sample = scheduler.scale_model_input(sample, t)
model_output = model(sample, t)
output = scheduler.step(model_output, t, sample, generator=generator)
sample = output.prev_sample
result_sum = torch.sum(torch.abs(sample))
result_mean = torch.mean(torch.abs(sample))
assert abs(result_sum.item() - 10.0807) < 1e-2
assert abs(result_mean.item() - 0.0131) < 1e-3
def test_full_loop_device_karras_sigmas(self):
scheduler_class = self.scheduler_classes[0]
scheduler_config = self.get_scheduler_config()
scheduler = scheduler_class(**scheduler_config, use_karras_sigmas=True)
scheduler.set_timesteps(self.num_inference_steps, device=torch_device)
generator = torch.manual_seed(0)
model = self.dummy_model()
sample = self.dummy_sample_deter * scheduler.init_noise_sigma.cpu()
sample = sample.to(torch_device)
for t in scheduler.timesteps:
sample = scheduler.scale_model_input(sample, t)
model_output = model(sample, t)
output = scheduler.step(model_output, t, sample, generator=generator)
sample = output.prev_sample
result_sum = torch.sum(torch.abs(sample))
result_mean = torch.mean(torch.abs(sample))
assert abs(result_sum.item() - 124.52299499511719) < 1e-2
assert abs(result_mean.item() - 0.16213932633399963) < 1e-3
| 0 |
hf_public_repos/diffusers/tests | hf_public_repos/diffusers/tests/schedulers/test_scheduler_pndm.py | import tempfile
import torch
from diffusers import PNDMScheduler
from .test_schedulers import SchedulerCommonTest
class PNDMSchedulerTest(SchedulerCommonTest):
scheduler_classes = (PNDMScheduler,)
forward_default_kwargs = (("num_inference_steps", 50),)
def get_scheduler_config(self, **kwargs):
config = {
"num_train_timesteps": 1000,
"beta_start": 0.0001,
"beta_end": 0.02,
"beta_schedule": "linear",
}
config.update(**kwargs)
return config
def check_over_configs(self, time_step=0, **config):
kwargs = dict(self.forward_default_kwargs)
num_inference_steps = kwargs.pop("num_inference_steps", None)
sample = self.dummy_sample
residual = 0.1 * sample
dummy_past_residuals = [residual + 0.2, residual + 0.15, residual + 0.1, residual + 0.05]
for scheduler_class in self.scheduler_classes:
scheduler_config = self.get_scheduler_config(**config)
scheduler = scheduler_class(**scheduler_config)
scheduler.set_timesteps(num_inference_steps)
# copy over dummy past residuals
scheduler.ets = dummy_past_residuals[:]
with tempfile.TemporaryDirectory() as tmpdirname:
scheduler.save_config(tmpdirname)
new_scheduler = scheduler_class.from_pretrained(tmpdirname)
new_scheduler.set_timesteps(num_inference_steps)
# copy over dummy past residuals
new_scheduler.ets = dummy_past_residuals[:]
output = scheduler.step_prk(residual, time_step, sample, **kwargs).prev_sample
new_output = new_scheduler.step_prk(residual, time_step, sample, **kwargs).prev_sample
assert torch.sum(torch.abs(output - new_output)) < 1e-5, "Scheduler outputs are not identical"
output = scheduler.step_plms(residual, time_step, sample, **kwargs).prev_sample
new_output = new_scheduler.step_plms(residual, time_step, sample, **kwargs).prev_sample
assert torch.sum(torch.abs(output - new_output)) < 1e-5, "Scheduler outputs are not identical"
def test_from_save_pretrained(self):
pass
def check_over_forward(self, time_step=0, **forward_kwargs):
kwargs = dict(self.forward_default_kwargs)
num_inference_steps = kwargs.pop("num_inference_steps", None)
sample = self.dummy_sample
residual = 0.1 * sample
dummy_past_residuals = [residual + 0.2, residual + 0.15, residual + 0.1, residual + 0.05]
for scheduler_class in self.scheduler_classes:
scheduler_config = self.get_scheduler_config()
scheduler = scheduler_class(**scheduler_config)
scheduler.set_timesteps(num_inference_steps)
# copy over dummy past residuals (must be after setting timesteps)
scheduler.ets = dummy_past_residuals[:]
with tempfile.TemporaryDirectory() as tmpdirname:
scheduler.save_config(tmpdirname)
new_scheduler = scheduler_class.from_pretrained(tmpdirname)
# copy over dummy past residuals
new_scheduler.set_timesteps(num_inference_steps)
# copy over dummy past residual (must be after setting timesteps)
new_scheduler.ets = dummy_past_residuals[:]
output = scheduler.step_prk(residual, time_step, sample, **kwargs).prev_sample
new_output = new_scheduler.step_prk(residual, time_step, sample, **kwargs).prev_sample
assert torch.sum(torch.abs(output - new_output)) < 1e-5, "Scheduler outputs are not identical"
output = scheduler.step_plms(residual, time_step, sample, **kwargs).prev_sample
new_output = new_scheduler.step_plms(residual, time_step, sample, **kwargs).prev_sample
assert torch.sum(torch.abs(output - new_output)) < 1e-5, "Scheduler outputs are not identical"
def full_loop(self, **config):
scheduler_class = self.scheduler_classes[0]
scheduler_config = self.get_scheduler_config(**config)
scheduler = scheduler_class(**scheduler_config)
num_inference_steps = 10
model = self.dummy_model()
sample = self.dummy_sample_deter
scheduler.set_timesteps(num_inference_steps)
for i, t in enumerate(scheduler.prk_timesteps):
residual = model(sample, t)
sample = scheduler.step_prk(residual, t, sample).prev_sample
for i, t in enumerate(scheduler.plms_timesteps):
residual = model(sample, t)
sample = scheduler.step_plms(residual, t, sample).prev_sample
return sample
def test_step_shape(self):
kwargs = dict(self.forward_default_kwargs)
num_inference_steps = kwargs.pop("num_inference_steps", None)
for scheduler_class in self.scheduler_classes:
scheduler_config = self.get_scheduler_config()
scheduler = scheduler_class(**scheduler_config)
sample = self.dummy_sample
residual = 0.1 * sample
if num_inference_steps is not None and hasattr(scheduler, "set_timesteps"):
scheduler.set_timesteps(num_inference_steps)
elif num_inference_steps is not None and not hasattr(scheduler, "set_timesteps"):
kwargs["num_inference_steps"] = num_inference_steps
# copy over dummy past residuals (must be done after set_timesteps)
dummy_past_residuals = [residual + 0.2, residual + 0.15, residual + 0.1, residual + 0.05]
scheduler.ets = dummy_past_residuals[:]
output_0 = scheduler.step_prk(residual, 0, sample, **kwargs).prev_sample
output_1 = scheduler.step_prk(residual, 1, sample, **kwargs).prev_sample
self.assertEqual(output_0.shape, sample.shape)
self.assertEqual(output_0.shape, output_1.shape)
output_0 = scheduler.step_plms(residual, 0, sample, **kwargs).prev_sample
output_1 = scheduler.step_plms(residual, 1, sample, **kwargs).prev_sample
self.assertEqual(output_0.shape, sample.shape)
self.assertEqual(output_0.shape, output_1.shape)
def test_timesteps(self):
for timesteps in [100, 1000]:
self.check_over_configs(num_train_timesteps=timesteps)
def test_steps_offset(self):
for steps_offset in [0, 1]:
self.check_over_configs(steps_offset=steps_offset)
scheduler_class = self.scheduler_classes[0]
scheduler_config = self.get_scheduler_config(steps_offset=1)
scheduler = scheduler_class(**scheduler_config)
scheduler.set_timesteps(10)
assert torch.equal(
scheduler.timesteps,
torch.LongTensor(
[901, 851, 851, 801, 801, 751, 751, 701, 701, 651, 651, 601, 601, 501, 401, 301, 201, 101, 1]
),
)
def test_betas(self):
for beta_start, beta_end in zip([0.0001, 0.001], [0.002, 0.02]):
self.check_over_configs(beta_start=beta_start, beta_end=beta_end)
def test_schedules(self):
for schedule in ["linear", "squaredcos_cap_v2"]:
self.check_over_configs(beta_schedule=schedule)
def test_prediction_type(self):
for prediction_type in ["epsilon", "v_prediction"]:
self.check_over_configs(prediction_type=prediction_type)
def test_time_indices(self):
for t in [1, 5, 10]:
self.check_over_forward(time_step=t)
def test_inference_steps(self):
for t, num_inference_steps in zip([1, 5, 10], [10, 50, 100]):
self.check_over_forward(num_inference_steps=num_inference_steps)
def test_pow_of_3_inference_steps(self):
# earlier version of set_timesteps() caused an error indexing alpha's with inference steps as power of 3
num_inference_steps = 27
for scheduler_class in self.scheduler_classes:
sample = self.dummy_sample
residual = 0.1 * sample
scheduler_config = self.get_scheduler_config()
scheduler = scheduler_class(**scheduler_config)
scheduler.set_timesteps(num_inference_steps)
# before power of 3 fix, would error on first step, so we only need to do two
for i, t in enumerate(scheduler.prk_timesteps[:2]):
sample = scheduler.step_prk(residual, t, sample).prev_sample
def test_inference_plms_no_past_residuals(self):
with self.assertRaises(ValueError):
scheduler_class = self.scheduler_classes[0]
scheduler_config = self.get_scheduler_config()
scheduler = scheduler_class(**scheduler_config)
scheduler.step_plms(self.dummy_sample, 1, self.dummy_sample).prev_sample
def test_full_loop_no_noise(self):
sample = self.full_loop()
result_sum = torch.sum(torch.abs(sample))
result_mean = torch.mean(torch.abs(sample))
assert abs(result_sum.item() - 198.1318) < 1e-2
assert abs(result_mean.item() - 0.2580) < 1e-3
def test_full_loop_with_v_prediction(self):
sample = self.full_loop(prediction_type="v_prediction")
result_sum = torch.sum(torch.abs(sample))
result_mean = torch.mean(torch.abs(sample))
assert abs(result_sum.item() - 67.3986) < 1e-2
assert abs(result_mean.item() - 0.0878) < 1e-3
def test_full_loop_with_set_alpha_to_one(self):
# We specify different beta, so that the first alpha is 0.99
sample = self.full_loop(set_alpha_to_one=True, beta_start=0.01)
result_sum = torch.sum(torch.abs(sample))
result_mean = torch.mean(torch.abs(sample))
assert abs(result_sum.item() - 230.0399) < 1e-2
assert abs(result_mean.item() - 0.2995) < 1e-3
def test_full_loop_with_no_set_alpha_to_one(self):
# We specify different beta, so that the first alpha is 0.99
sample = self.full_loop(set_alpha_to_one=False, beta_start=0.01)
result_sum = torch.sum(torch.abs(sample))
result_mean = torch.mean(torch.abs(sample))
assert abs(result_sum.item() - 186.9482) < 1e-2
assert abs(result_mean.item() - 0.2434) < 1e-3
| 0 |
hf_public_repos/diffusers/tests | hf_public_repos/diffusers/tests/schedulers/test_scheduler_consistency_model.py | import torch
from diffusers import CMStochasticIterativeScheduler
from .test_schedulers import SchedulerCommonTest
class CMStochasticIterativeSchedulerTest(SchedulerCommonTest):
scheduler_classes = (CMStochasticIterativeScheduler,)
num_inference_steps = 10
def get_scheduler_config(self, **kwargs):
config = {
"num_train_timesteps": 201,
"sigma_min": 0.002,
"sigma_max": 80.0,
}
config.update(**kwargs)
return config
# Override test_step_shape to add CMStochasticIterativeScheduler-specific logic regarding timesteps
# Problem is that we don't know two timesteps that will always be in the timestep schedule from only the scheduler
# config; scaled sigma_max is always in the timestep schedule, but sigma_min is in the sigma schedule while scaled
# sigma_min is not in the timestep schedule
def test_step_shape(self):
num_inference_steps = 10
scheduler_config = self.get_scheduler_config()
scheduler = self.scheduler_classes[0](**scheduler_config)
scheduler.set_timesteps(num_inference_steps)
timestep_0 = scheduler.timesteps[0]
timestep_1 = scheduler.timesteps[1]
sample = self.dummy_sample
residual = 0.1 * sample
output_0 = scheduler.step(residual, timestep_0, sample).prev_sample
output_1 = scheduler.step(residual, timestep_1, sample).prev_sample
self.assertEqual(output_0.shape, sample.shape)
self.assertEqual(output_0.shape, output_1.shape)
def test_timesteps(self):
for timesteps in [10, 50, 100, 1000]:
self.check_over_configs(num_train_timesteps=timesteps)
def test_clip_denoised(self):
for clip_denoised in [True, False]:
self.check_over_configs(clip_denoised=clip_denoised)
def test_full_loop_no_noise_onestep(self):
scheduler_class = self.scheduler_classes[0]
scheduler_config = self.get_scheduler_config()
scheduler = scheduler_class(**scheduler_config)
num_inference_steps = 1
scheduler.set_timesteps(num_inference_steps)
timesteps = scheduler.timesteps
generator = torch.manual_seed(0)
model = self.dummy_model()
sample = self.dummy_sample_deter * scheduler.init_noise_sigma
for i, t in enumerate(timesteps):
# 1. scale model input
scaled_sample = scheduler.scale_model_input(sample, t)
# 2. predict noise residual
residual = model(scaled_sample, t)
# 3. predict previous sample x_t-1
pred_prev_sample = scheduler.step(residual, t, sample, generator=generator).prev_sample
sample = pred_prev_sample
result_sum = torch.sum(torch.abs(sample))
result_mean = torch.mean(torch.abs(sample))
assert abs(result_sum.item() - 192.7614) < 1e-2
assert abs(result_mean.item() - 0.2510) < 1e-3
def test_full_loop_no_noise_multistep(self):
scheduler_class = self.scheduler_classes[0]
scheduler_config = self.get_scheduler_config()
scheduler = scheduler_class(**scheduler_config)
timesteps = [106, 0]
scheduler.set_timesteps(timesteps=timesteps)
timesteps = scheduler.timesteps
generator = torch.manual_seed(0)
model = self.dummy_model()
sample = self.dummy_sample_deter * scheduler.init_noise_sigma
for t in timesteps:
# 1. scale model input
scaled_sample = scheduler.scale_model_input(sample, t)
# 2. predict noise residual
residual = model(scaled_sample, t)
# 3. predict previous sample x_t-1
pred_prev_sample = scheduler.step(residual, t, sample, generator=generator).prev_sample
sample = pred_prev_sample
result_sum = torch.sum(torch.abs(sample))
result_mean = torch.mean(torch.abs(sample))
assert abs(result_sum.item() - 347.6357) < 1e-2
assert abs(result_mean.item() - 0.4527) < 1e-3
def test_custom_timesteps_increasing_order(self):
scheduler_class = self.scheduler_classes[0]
scheduler_config = self.get_scheduler_config()
scheduler = scheduler_class(**scheduler_config)
timesteps = [39, 30, 12, 15, 0]
with self.assertRaises(ValueError, msg="`timesteps` must be in descending order."):
scheduler.set_timesteps(timesteps=timesteps)
def test_custom_timesteps_passing_both_num_inference_steps_and_timesteps(self):
scheduler_class = self.scheduler_classes[0]
scheduler_config = self.get_scheduler_config()
scheduler = scheduler_class(**scheduler_config)
timesteps = [39, 30, 12, 1, 0]
num_inference_steps = len(timesteps)
with self.assertRaises(ValueError, msg="Can only pass one of `num_inference_steps` or `timesteps`."):
scheduler.set_timesteps(num_inference_steps=num_inference_steps, timesteps=timesteps)
def test_custom_timesteps_too_large(self):
scheduler_class = self.scheduler_classes[0]
scheduler_config = self.get_scheduler_config()
scheduler = scheduler_class(**scheduler_config)
timesteps = [scheduler.config.num_train_timesteps]
with self.assertRaises(
ValueError,
msg="`timesteps` must start before `self.config.train_timesteps`: {scheduler.config.num_train_timesteps}}",
):
scheduler.set_timesteps(timesteps=timesteps)
| 0 |
hf_public_repos/diffusers/tests | hf_public_repos/diffusers/tests/schedulers/test_scheduler_ddpm.py | import torch
from diffusers import DDPMScheduler
from .test_schedulers import SchedulerCommonTest
class DDPMSchedulerTest(SchedulerCommonTest):
scheduler_classes = (DDPMScheduler,)
def get_scheduler_config(self, **kwargs):
config = {
"num_train_timesteps": 1000,
"beta_start": 0.0001,
"beta_end": 0.02,
"beta_schedule": "linear",
"variance_type": "fixed_small",
"clip_sample": True,
}
config.update(**kwargs)
return config
def test_timesteps(self):
for timesteps in [1, 5, 100, 1000]:
self.check_over_configs(num_train_timesteps=timesteps)
def test_betas(self):
for beta_start, beta_end in zip([0.0001, 0.001, 0.01, 0.1], [0.002, 0.02, 0.2, 2]):
self.check_over_configs(beta_start=beta_start, beta_end=beta_end)
def test_schedules(self):
for schedule in ["linear", "squaredcos_cap_v2"]:
self.check_over_configs(beta_schedule=schedule)
def test_variance_type(self):
for variance in ["fixed_small", "fixed_large", "other"]:
self.check_over_configs(variance_type=variance)
def test_clip_sample(self):
for clip_sample in [True, False]:
self.check_over_configs(clip_sample=clip_sample)
def test_thresholding(self):
self.check_over_configs(thresholding=False)
for threshold in [0.5, 1.0, 2.0]:
for prediction_type in ["epsilon", "sample", "v_prediction"]:
self.check_over_configs(
thresholding=True,
prediction_type=prediction_type,
sample_max_value=threshold,
)
def test_prediction_type(self):
for prediction_type in ["epsilon", "sample", "v_prediction"]:
self.check_over_configs(prediction_type=prediction_type)
def test_time_indices(self):
for t in [0, 500, 999]:
self.check_over_forward(time_step=t)
def test_variance(self):
scheduler_class = self.scheduler_classes[0]
scheduler_config = self.get_scheduler_config()
scheduler = scheduler_class(**scheduler_config)
assert torch.sum(torch.abs(scheduler._get_variance(0) - 0.0)) < 1e-5
assert torch.sum(torch.abs(scheduler._get_variance(487) - 0.00979)) < 1e-5
assert torch.sum(torch.abs(scheduler._get_variance(999) - 0.02)) < 1e-5
def test_full_loop_no_noise(self):
scheduler_class = self.scheduler_classes[0]
scheduler_config = self.get_scheduler_config()
scheduler = scheduler_class(**scheduler_config)
num_trained_timesteps = len(scheduler)
model = self.dummy_model()
sample = self.dummy_sample_deter
generator = torch.manual_seed(0)
for t in reversed(range(num_trained_timesteps)):
# 1. predict noise residual
residual = model(sample, t)
# 2. predict previous mean of sample x_t-1
pred_prev_sample = scheduler.step(residual, t, sample, generator=generator).prev_sample
# if t > 0:
# noise = self.dummy_sample_deter
# variance = scheduler.get_variance(t) ** (0.5) * noise
#
# sample = pred_prev_sample + variance
sample = pred_prev_sample
result_sum = torch.sum(torch.abs(sample))
result_mean = torch.mean(torch.abs(sample))
assert abs(result_sum.item() - 258.9606) < 1e-2
assert abs(result_mean.item() - 0.3372) < 1e-3
def test_full_loop_with_v_prediction(self):
scheduler_class = self.scheduler_classes[0]
scheduler_config = self.get_scheduler_config(prediction_type="v_prediction")
scheduler = scheduler_class(**scheduler_config)
num_trained_timesteps = len(scheduler)
model = self.dummy_model()
sample = self.dummy_sample_deter
generator = torch.manual_seed(0)
for t in reversed(range(num_trained_timesteps)):
# 1. predict noise residual
residual = model(sample, t)
# 2. predict previous mean of sample x_t-1
pred_prev_sample = scheduler.step(residual, t, sample, generator=generator).prev_sample
# if t > 0:
# noise = self.dummy_sample_deter
# variance = scheduler.get_variance(t) ** (0.5) * noise
#
# sample = pred_prev_sample + variance
sample = pred_prev_sample
result_sum = torch.sum(torch.abs(sample))
result_mean = torch.mean(torch.abs(sample))
assert abs(result_sum.item() - 202.0296) < 1e-2
assert abs(result_mean.item() - 0.2631) < 1e-3
def test_custom_timesteps(self):
scheduler_class = self.scheduler_classes[0]
scheduler_config = self.get_scheduler_config()
scheduler = scheduler_class(**scheduler_config)
timesteps = [100, 87, 50, 1, 0]
scheduler.set_timesteps(timesteps=timesteps)
scheduler_timesteps = scheduler.timesteps
for i, timestep in enumerate(scheduler_timesteps):
if i == len(timesteps) - 1:
expected_prev_t = -1
else:
expected_prev_t = timesteps[i + 1]
prev_t = scheduler.previous_timestep(timestep)
prev_t = prev_t.item()
self.assertEqual(prev_t, expected_prev_t)
def test_custom_timesteps_increasing_order(self):
scheduler_class = self.scheduler_classes[0]
scheduler_config = self.get_scheduler_config()
scheduler = scheduler_class(**scheduler_config)
timesteps = [100, 87, 50, 51, 0]
with self.assertRaises(ValueError, msg="`custom_timesteps` must be in descending order."):
scheduler.set_timesteps(timesteps=timesteps)
def test_custom_timesteps_passing_both_num_inference_steps_and_timesteps(self):
scheduler_class = self.scheduler_classes[0]
scheduler_config = self.get_scheduler_config()
scheduler = scheduler_class(**scheduler_config)
timesteps = [100, 87, 50, 1, 0]
num_inference_steps = len(timesteps)
with self.assertRaises(ValueError, msg="Can only pass one of `num_inference_steps` or `custom_timesteps`."):
scheduler.set_timesteps(num_inference_steps=num_inference_steps, timesteps=timesteps)
def test_custom_timesteps_too_large(self):
scheduler_class = self.scheduler_classes[0]
scheduler_config = self.get_scheduler_config()
scheduler = scheduler_class(**scheduler_config)
timesteps = [scheduler.config.num_train_timesteps]
with self.assertRaises(
ValueError,
msg="`timesteps` must start before `self.config.train_timesteps`: {scheduler.config.num_train_timesteps}}",
):
scheduler.set_timesteps(timesteps=timesteps)
| 0 |
hf_public_repos/diffusers/tests | hf_public_repos/diffusers/tests/schedulers/test_scheduler_unclip.py | import torch
from diffusers import UnCLIPScheduler
from .test_schedulers import SchedulerCommonTest
# UnCLIPScheduler is a modified DDPMScheduler with a subset of the configuration.
class UnCLIPSchedulerTest(SchedulerCommonTest):
scheduler_classes = (UnCLIPScheduler,)
def get_scheduler_config(self, **kwargs):
config = {
"num_train_timesteps": 1000,
"variance_type": "fixed_small_log",
"clip_sample": True,
"clip_sample_range": 1.0,
"prediction_type": "epsilon",
}
config.update(**kwargs)
return config
def test_timesteps(self):
for timesteps in [1, 5, 100, 1000]:
self.check_over_configs(num_train_timesteps=timesteps)
def test_variance_type(self):
for variance in ["fixed_small_log", "learned_range"]:
self.check_over_configs(variance_type=variance)
def test_clip_sample(self):
for clip_sample in [True, False]:
self.check_over_configs(clip_sample=clip_sample)
def test_clip_sample_range(self):
for clip_sample_range in [1, 5, 10, 20]:
self.check_over_configs(clip_sample_range=clip_sample_range)
def test_prediction_type(self):
for prediction_type in ["epsilon", "sample"]:
self.check_over_configs(prediction_type=prediction_type)
def test_time_indices(self):
for time_step in [0, 500, 999]:
for prev_timestep in [None, 5, 100, 250, 500, 750]:
if prev_timestep is not None and prev_timestep >= time_step:
continue
self.check_over_forward(time_step=time_step, prev_timestep=prev_timestep)
def test_variance_fixed_small_log(self):
scheduler_class = self.scheduler_classes[0]
scheduler_config = self.get_scheduler_config(variance_type="fixed_small_log")
scheduler = scheduler_class(**scheduler_config)
assert torch.sum(torch.abs(scheduler._get_variance(0) - 1.0000e-10)) < 1e-5
assert torch.sum(torch.abs(scheduler._get_variance(487) - 0.0549625)) < 1e-5
assert torch.sum(torch.abs(scheduler._get_variance(999) - 0.9994987)) < 1e-5
def test_variance_learned_range(self):
scheduler_class = self.scheduler_classes[0]
scheduler_config = self.get_scheduler_config(variance_type="learned_range")
scheduler = scheduler_class(**scheduler_config)
predicted_variance = 0.5
assert scheduler._get_variance(1, predicted_variance=predicted_variance) - -10.1712790 < 1e-5
assert scheduler._get_variance(487, predicted_variance=predicted_variance) - -5.7998052 < 1e-5
assert scheduler._get_variance(999, predicted_variance=predicted_variance) - -0.0010011 < 1e-5
def test_full_loop(self):
scheduler_class = self.scheduler_classes[0]
scheduler_config = self.get_scheduler_config()
scheduler = scheduler_class(**scheduler_config)
timesteps = scheduler.timesteps
model = self.dummy_model()
sample = self.dummy_sample_deter
generator = torch.manual_seed(0)
for i, t in enumerate(timesteps):
# 1. predict noise residual
residual = model(sample, t)
# 2. predict previous mean of sample x_t-1
pred_prev_sample = scheduler.step(residual, t, sample, generator=generator).prev_sample
sample = pred_prev_sample
result_sum = torch.sum(torch.abs(sample))
result_mean = torch.mean(torch.abs(sample))
assert abs(result_sum.item() - 252.2682495) < 1e-2
assert abs(result_mean.item() - 0.3284743) < 1e-3
def test_full_loop_skip_timesteps(self):
scheduler_class = self.scheduler_classes[0]
scheduler_config = self.get_scheduler_config()
scheduler = scheduler_class(**scheduler_config)
scheduler.set_timesteps(25)
timesteps = scheduler.timesteps
model = self.dummy_model()
sample = self.dummy_sample_deter
generator = torch.manual_seed(0)
for i, t in enumerate(timesteps):
# 1. predict noise residual
residual = model(sample, t)
if i + 1 == timesteps.shape[0]:
prev_timestep = None
else:
prev_timestep = timesteps[i + 1]
# 2. predict previous mean of sample x_t-1
pred_prev_sample = scheduler.step(
residual, t, sample, prev_timestep=prev_timestep, generator=generator
).prev_sample
sample = pred_prev_sample
result_sum = torch.sum(torch.abs(sample))
result_mean = torch.mean(torch.abs(sample))
assert abs(result_sum.item() - 258.2044983) < 1e-2
assert abs(result_mean.item() - 0.3362038) < 1e-3
def test_trained_betas(self):
pass
def test_add_noise_device(self):
pass
| 0 |
hf_public_repos/diffusers/tests | hf_public_repos/diffusers/tests/schedulers/test_scheduler_ddim.py | import torch
from diffusers import DDIMScheduler
from .test_schedulers import SchedulerCommonTest
class DDIMSchedulerTest(SchedulerCommonTest):
scheduler_classes = (DDIMScheduler,)
forward_default_kwargs = (("eta", 0.0), ("num_inference_steps", 50))
def get_scheduler_config(self, **kwargs):
config = {
"num_train_timesteps": 1000,
"beta_start": 0.0001,
"beta_end": 0.02,
"beta_schedule": "linear",
"clip_sample": True,
}
config.update(**kwargs)
return config
def full_loop(self, **config):
scheduler_class = self.scheduler_classes[0]
scheduler_config = self.get_scheduler_config(**config)
scheduler = scheduler_class(**scheduler_config)
num_inference_steps, eta = 10, 0.0
model = self.dummy_model()
sample = self.dummy_sample_deter
scheduler.set_timesteps(num_inference_steps)
for t in scheduler.timesteps:
residual = model(sample, t)
sample = scheduler.step(residual, t, sample, eta).prev_sample
return sample
def test_timesteps(self):
for timesteps in [100, 500, 1000]:
self.check_over_configs(num_train_timesteps=timesteps)
def test_steps_offset(self):
for steps_offset in [0, 1]:
self.check_over_configs(steps_offset=steps_offset)
scheduler_class = self.scheduler_classes[0]
scheduler_config = self.get_scheduler_config(steps_offset=1)
scheduler = scheduler_class(**scheduler_config)
scheduler.set_timesteps(5)
assert torch.equal(scheduler.timesteps, torch.LongTensor([801, 601, 401, 201, 1]))
def test_betas(self):
for beta_start, beta_end in zip([0.0001, 0.001, 0.01, 0.1], [0.002, 0.02, 0.2, 2]):
self.check_over_configs(beta_start=beta_start, beta_end=beta_end)
def test_schedules(self):
for schedule in ["linear", "squaredcos_cap_v2"]:
self.check_over_configs(beta_schedule=schedule)
def test_prediction_type(self):
for prediction_type in ["epsilon", "v_prediction"]:
self.check_over_configs(prediction_type=prediction_type)
def test_clip_sample(self):
for clip_sample in [True, False]:
self.check_over_configs(clip_sample=clip_sample)
def test_timestep_spacing(self):
for timestep_spacing in ["trailing", "leading"]:
self.check_over_configs(timestep_spacing=timestep_spacing)
def test_rescale_betas_zero_snr(self):
for rescale_betas_zero_snr in [True, False]:
self.check_over_configs(rescale_betas_zero_snr=rescale_betas_zero_snr)
def test_thresholding(self):
self.check_over_configs(thresholding=False)
for threshold in [0.5, 1.0, 2.0]:
for prediction_type in ["epsilon", "v_prediction"]:
self.check_over_configs(
thresholding=True,
prediction_type=prediction_type,
sample_max_value=threshold,
)
def test_time_indices(self):
for t in [1, 10, 49]:
self.check_over_forward(time_step=t)
def test_inference_steps(self):
for t, num_inference_steps in zip([1, 10, 50], [10, 50, 500]):
self.check_over_forward(time_step=t, num_inference_steps=num_inference_steps)
def test_eta(self):
for t, eta in zip([1, 10, 49], [0.0, 0.5, 1.0]):
self.check_over_forward(time_step=t, eta=eta)
def test_variance(self):
scheduler_class = self.scheduler_classes[0]
scheduler_config = self.get_scheduler_config()
scheduler = scheduler_class(**scheduler_config)
assert torch.sum(torch.abs(scheduler._get_variance(0, 0) - 0.0)) < 1e-5
assert torch.sum(torch.abs(scheduler._get_variance(420, 400) - 0.14771)) < 1e-5
assert torch.sum(torch.abs(scheduler._get_variance(980, 960) - 0.32460)) < 1e-5
assert torch.sum(torch.abs(scheduler._get_variance(0, 0) - 0.0)) < 1e-5
assert torch.sum(torch.abs(scheduler._get_variance(487, 486) - 0.00979)) < 1e-5
assert torch.sum(torch.abs(scheduler._get_variance(999, 998) - 0.02)) < 1e-5
def test_full_loop_no_noise(self):
sample = self.full_loop()
result_sum = torch.sum(torch.abs(sample))
result_mean = torch.mean(torch.abs(sample))
assert abs(result_sum.item() - 172.0067) < 1e-2
assert abs(result_mean.item() - 0.223967) < 1e-3
def test_full_loop_with_v_prediction(self):
sample = self.full_loop(prediction_type="v_prediction")
result_sum = torch.sum(torch.abs(sample))
result_mean = torch.mean(torch.abs(sample))
assert abs(result_sum.item() - 52.5302) < 1e-2
assert abs(result_mean.item() - 0.0684) < 1e-3
def test_full_loop_with_set_alpha_to_one(self):
# We specify different beta, so that the first alpha is 0.99
sample = self.full_loop(set_alpha_to_one=True, beta_start=0.01)
result_sum = torch.sum(torch.abs(sample))
result_mean = torch.mean(torch.abs(sample))
assert abs(result_sum.item() - 149.8295) < 1e-2
assert abs(result_mean.item() - 0.1951) < 1e-3
def test_full_loop_with_no_set_alpha_to_one(self):
# We specify different beta, so that the first alpha is 0.99
sample = self.full_loop(set_alpha_to_one=False, beta_start=0.01)
result_sum = torch.sum(torch.abs(sample))
result_mean = torch.mean(torch.abs(sample))
assert abs(result_sum.item() - 149.0784) < 1e-2
assert abs(result_mean.item() - 0.1941) < 1e-3
| 0 |
hf_public_repos/diffusers/tests | hf_public_repos/diffusers/tests/schedulers/test_scheduler_unipc.py | import tempfile
import torch
from diffusers import (
DEISMultistepScheduler,
DPMSolverMultistepScheduler,
DPMSolverSinglestepScheduler,
UniPCMultistepScheduler,
)
from .test_schedulers import SchedulerCommonTest
class UniPCMultistepSchedulerTest(SchedulerCommonTest):
scheduler_classes = (UniPCMultistepScheduler,)
forward_default_kwargs = (("num_inference_steps", 25),)
def get_scheduler_config(self, **kwargs):
config = {
"num_train_timesteps": 1000,
"beta_start": 0.0001,
"beta_end": 0.02,
"beta_schedule": "linear",
"solver_order": 2,
"solver_type": "bh2",
}
config.update(**kwargs)
return config
def check_over_configs(self, time_step=0, **config):
kwargs = dict(self.forward_default_kwargs)
num_inference_steps = kwargs.pop("num_inference_steps", None)
sample = self.dummy_sample
residual = 0.1 * sample
dummy_past_residuals = [residual + 0.2, residual + 0.15, residual + 0.10]
for scheduler_class in self.scheduler_classes:
scheduler_config = self.get_scheduler_config(**config)
scheduler = scheduler_class(**scheduler_config)
scheduler.set_timesteps(num_inference_steps)
# copy over dummy past residuals
scheduler.model_outputs = dummy_past_residuals[: scheduler.config.solver_order]
with tempfile.TemporaryDirectory() as tmpdirname:
scheduler.save_config(tmpdirname)
new_scheduler = scheduler_class.from_pretrained(tmpdirname)
new_scheduler.set_timesteps(num_inference_steps)
# copy over dummy past residuals
new_scheduler.model_outputs = dummy_past_residuals[: new_scheduler.config.solver_order]
output, new_output = sample, sample
for t in range(time_step, time_step + scheduler.config.solver_order + 1):
output = scheduler.step(residual, t, output, **kwargs).prev_sample
new_output = new_scheduler.step(residual, t, new_output, **kwargs).prev_sample
assert torch.sum(torch.abs(output - new_output)) < 1e-5, "Scheduler outputs are not identical"
def check_over_forward(self, time_step=0, **forward_kwargs):
kwargs = dict(self.forward_default_kwargs)
num_inference_steps = kwargs.pop("num_inference_steps", None)
sample = self.dummy_sample
residual = 0.1 * sample
dummy_past_residuals = [residual + 0.2, residual + 0.15, residual + 0.10]
for scheduler_class in self.scheduler_classes:
scheduler_config = self.get_scheduler_config()
scheduler = scheduler_class(**scheduler_config)
scheduler.set_timesteps(num_inference_steps)
# copy over dummy past residuals (must be after setting timesteps)
scheduler.model_outputs = dummy_past_residuals[: scheduler.config.solver_order]
with tempfile.TemporaryDirectory() as tmpdirname:
scheduler.save_config(tmpdirname)
new_scheduler = scheduler_class.from_pretrained(tmpdirname)
# copy over dummy past residuals
new_scheduler.set_timesteps(num_inference_steps)
# copy over dummy past residual (must be after setting timesteps)
new_scheduler.model_outputs = dummy_past_residuals[: new_scheduler.config.solver_order]
output = scheduler.step(residual, time_step, sample, **kwargs).prev_sample
new_output = new_scheduler.step(residual, time_step, sample, **kwargs).prev_sample
assert torch.sum(torch.abs(output - new_output)) < 1e-5, "Scheduler outputs are not identical"
def full_loop(self, scheduler=None, **config):
if scheduler is None:
scheduler_class = self.scheduler_classes[0]
scheduler_config = self.get_scheduler_config(**config)
scheduler = scheduler_class(**scheduler_config)
scheduler_class = self.scheduler_classes[0]
scheduler_config = self.get_scheduler_config(**config)
scheduler = scheduler_class(**scheduler_config)
num_inference_steps = 10
model = self.dummy_model()
sample = self.dummy_sample_deter
scheduler.set_timesteps(num_inference_steps)
for i, t in enumerate(scheduler.timesteps):
residual = model(sample, t)
sample = scheduler.step(residual, t, sample).prev_sample
return sample
def test_step_shape(self):
kwargs = dict(self.forward_default_kwargs)
num_inference_steps = kwargs.pop("num_inference_steps", None)
for scheduler_class in self.scheduler_classes:
scheduler_config = self.get_scheduler_config()
scheduler = scheduler_class(**scheduler_config)
sample = self.dummy_sample
residual = 0.1 * sample
if num_inference_steps is not None and hasattr(scheduler, "set_timesteps"):
scheduler.set_timesteps(num_inference_steps)
elif num_inference_steps is not None and not hasattr(scheduler, "set_timesteps"):
kwargs["num_inference_steps"] = num_inference_steps
# copy over dummy past residuals (must be done after set_timesteps)
dummy_past_residuals = [residual + 0.2, residual + 0.15, residual + 0.10]
scheduler.model_outputs = dummy_past_residuals[: scheduler.config.solver_order]
time_step_0 = scheduler.timesteps[5]
time_step_1 = scheduler.timesteps[6]
output_0 = scheduler.step(residual, time_step_0, sample, **kwargs).prev_sample
output_1 = scheduler.step(residual, time_step_1, sample, **kwargs).prev_sample
self.assertEqual(output_0.shape, sample.shape)
self.assertEqual(output_0.shape, output_1.shape)
def test_switch(self):
# make sure that iterating over schedulers with same config names gives same results
# for defaults
scheduler = UniPCMultistepScheduler(**self.get_scheduler_config())
sample = self.full_loop(scheduler=scheduler)
result_mean = torch.mean(torch.abs(sample))
assert abs(result_mean.item() - 0.2464) < 1e-3
scheduler = DPMSolverSinglestepScheduler.from_config(scheduler.config)
scheduler = DEISMultistepScheduler.from_config(scheduler.config)
scheduler = DPMSolverMultistepScheduler.from_config(scheduler.config)
scheduler = UniPCMultistepScheduler.from_config(scheduler.config)
sample = self.full_loop(scheduler=scheduler)
result_mean = torch.mean(torch.abs(sample))
assert abs(result_mean.item() - 0.2464) < 1e-3
def test_timesteps(self):
for timesteps in [25, 50, 100, 999, 1000]:
self.check_over_configs(num_train_timesteps=timesteps)
def test_thresholding(self):
self.check_over_configs(thresholding=False)
for order in [1, 2, 3]:
for solver_type in ["bh1", "bh2"]:
for threshold in [0.5, 1.0, 2.0]:
for prediction_type in ["epsilon", "sample"]:
self.check_over_configs(
thresholding=True,
prediction_type=prediction_type,
sample_max_value=threshold,
solver_order=order,
solver_type=solver_type,
)
def test_prediction_type(self):
for prediction_type in ["epsilon", "v_prediction"]:
self.check_over_configs(prediction_type=prediction_type)
def test_solver_order_and_type(self):
for solver_type in ["bh1", "bh2"]:
for order in [1, 2, 3]:
for prediction_type in ["epsilon", "sample"]:
self.check_over_configs(
solver_order=order,
solver_type=solver_type,
prediction_type=prediction_type,
)
sample = self.full_loop(
solver_order=order,
solver_type=solver_type,
prediction_type=prediction_type,
)
assert not torch.isnan(sample).any(), "Samples have nan numbers"
def test_lower_order_final(self):
self.check_over_configs(lower_order_final=True)
self.check_over_configs(lower_order_final=False)
def test_inference_steps(self):
for num_inference_steps in [1, 2, 3, 5, 10, 50, 100, 999, 1000]:
self.check_over_forward(num_inference_steps=num_inference_steps, time_step=0)
def test_full_loop_no_noise(self):
sample = self.full_loop()
result_mean = torch.mean(torch.abs(sample))
assert abs(result_mean.item() - 0.2464) < 1e-3
def test_full_loop_with_v_prediction(self):
sample = self.full_loop(prediction_type="v_prediction")
result_mean = torch.mean(torch.abs(sample))
assert abs(result_mean.item() - 0.1014) < 1e-3
def test_fp16_support(self):
scheduler_class = self.scheduler_classes[0]
scheduler_config = self.get_scheduler_config(thresholding=True, dynamic_thresholding_ratio=0)
scheduler = scheduler_class(**scheduler_config)
num_inference_steps = 10
model = self.dummy_model()
sample = self.dummy_sample_deter.half()
scheduler.set_timesteps(num_inference_steps)
for i, t in enumerate(scheduler.timesteps):
residual = model(sample, t)
sample = scheduler.step(residual, t, sample).prev_sample
assert sample.dtype == torch.float16
def test_unique_timesteps(self, **config):
for scheduler_class in self.scheduler_classes:
scheduler_config = self.get_scheduler_config(**config)
scheduler = scheduler_class(**scheduler_config)
scheduler.set_timesteps(scheduler.config.num_train_timesteps)
assert len(scheduler.timesteps.unique()) == scheduler.num_inference_steps
| 0 |
hf_public_repos/diffusers/tests | hf_public_repos/diffusers/tests/schedulers/test_scheduler_score_sde_ve.py | import tempfile
import unittest
import numpy as np
import torch
from diffusers import ScoreSdeVeScheduler
class ScoreSdeVeSchedulerTest(unittest.TestCase):
# TODO adapt with class SchedulerCommonTest (scheduler needs Numpy Integration)
scheduler_classes = (ScoreSdeVeScheduler,)
forward_default_kwargs = ()
@property
def dummy_sample(self):
batch_size = 4
num_channels = 3
height = 8
width = 8
sample = torch.rand((batch_size, num_channels, height, width))
return sample
@property
def dummy_sample_deter(self):
batch_size = 4
num_channels = 3
height = 8
width = 8
num_elems = batch_size * num_channels * height * width
sample = torch.arange(num_elems)
sample = sample.reshape(num_channels, height, width, batch_size)
sample = sample / num_elems
sample = sample.permute(3, 0, 1, 2)
return sample
def dummy_model(self):
def model(sample, t, *args):
return sample * t / (t + 1)
return model
def get_scheduler_config(self, **kwargs):
config = {
"num_train_timesteps": 2000,
"snr": 0.15,
"sigma_min": 0.01,
"sigma_max": 1348,
"sampling_eps": 1e-5,
}
config.update(**kwargs)
return config
def check_over_configs(self, time_step=0, **config):
kwargs = dict(self.forward_default_kwargs)
for scheduler_class in self.scheduler_classes:
sample = self.dummy_sample
residual = 0.1 * sample
scheduler_config = self.get_scheduler_config(**config)
scheduler = scheduler_class(**scheduler_config)
with tempfile.TemporaryDirectory() as tmpdirname:
scheduler.save_config(tmpdirname)
new_scheduler = scheduler_class.from_pretrained(tmpdirname)
output = scheduler.step_pred(
residual, time_step, sample, generator=torch.manual_seed(0), **kwargs
).prev_sample
new_output = new_scheduler.step_pred(
residual, time_step, sample, generator=torch.manual_seed(0), **kwargs
).prev_sample
assert torch.sum(torch.abs(output - new_output)) < 1e-5, "Scheduler outputs are not identical"
output = scheduler.step_correct(residual, sample, generator=torch.manual_seed(0), **kwargs).prev_sample
new_output = new_scheduler.step_correct(
residual, sample, generator=torch.manual_seed(0), **kwargs
).prev_sample
assert torch.sum(torch.abs(output - new_output)) < 1e-5, "Scheduler correction are not identical"
def check_over_forward(self, time_step=0, **forward_kwargs):
kwargs = dict(self.forward_default_kwargs)
kwargs.update(forward_kwargs)
for scheduler_class in self.scheduler_classes:
sample = self.dummy_sample
residual = 0.1 * sample
scheduler_config = self.get_scheduler_config()
scheduler = scheduler_class(**scheduler_config)
with tempfile.TemporaryDirectory() as tmpdirname:
scheduler.save_config(tmpdirname)
new_scheduler = scheduler_class.from_pretrained(tmpdirname)
output = scheduler.step_pred(
residual, time_step, sample, generator=torch.manual_seed(0), **kwargs
).prev_sample
new_output = new_scheduler.step_pred(
residual, time_step, sample, generator=torch.manual_seed(0), **kwargs
).prev_sample
assert torch.sum(torch.abs(output - new_output)) < 1e-5, "Scheduler outputs are not identical"
output = scheduler.step_correct(residual, sample, generator=torch.manual_seed(0), **kwargs).prev_sample
new_output = new_scheduler.step_correct(
residual, sample, generator=torch.manual_seed(0), **kwargs
).prev_sample
assert torch.sum(torch.abs(output - new_output)) < 1e-5, "Scheduler correction are not identical"
def test_timesteps(self):
for timesteps in [10, 100, 1000]:
self.check_over_configs(num_train_timesteps=timesteps)
def test_sigmas(self):
for sigma_min, sigma_max in zip([0.0001, 0.001, 0.01], [1, 100, 1000]):
self.check_over_configs(sigma_min=sigma_min, sigma_max=sigma_max)
def test_time_indices(self):
for t in [0.1, 0.5, 0.75]:
self.check_over_forward(time_step=t)
def test_full_loop_no_noise(self):
kwargs = dict(self.forward_default_kwargs)
scheduler_class = self.scheduler_classes[0]
scheduler_config = self.get_scheduler_config()
scheduler = scheduler_class(**scheduler_config)
num_inference_steps = 3
model = self.dummy_model()
sample = self.dummy_sample_deter
scheduler.set_sigmas(num_inference_steps)
scheduler.set_timesteps(num_inference_steps)
generator = torch.manual_seed(0)
for i, t in enumerate(scheduler.timesteps):
sigma_t = scheduler.sigmas[i]
for _ in range(scheduler.config.correct_steps):
with torch.no_grad():
model_output = model(sample, sigma_t)
sample = scheduler.step_correct(model_output, sample, generator=generator, **kwargs).prev_sample
with torch.no_grad():
model_output = model(sample, sigma_t)
output = scheduler.step_pred(model_output, t, sample, generator=generator, **kwargs)
sample, _ = output.prev_sample, output.prev_sample_mean
result_sum = torch.sum(torch.abs(sample))
result_mean = torch.mean(torch.abs(sample))
assert np.isclose(result_sum.item(), 14372758528.0)
assert np.isclose(result_mean.item(), 18714530.0)
def test_step_shape(self):
kwargs = dict(self.forward_default_kwargs)
num_inference_steps = kwargs.pop("num_inference_steps", None)
for scheduler_class in self.scheduler_classes:
scheduler_config = self.get_scheduler_config()
scheduler = scheduler_class(**scheduler_config)
sample = self.dummy_sample
residual = 0.1 * sample
if num_inference_steps is not None and hasattr(scheduler, "set_timesteps"):
scheduler.set_timesteps(num_inference_steps)
elif num_inference_steps is not None and not hasattr(scheduler, "set_timesteps"):
kwargs["num_inference_steps"] = num_inference_steps
output_0 = scheduler.step_pred(residual, 0, sample, generator=torch.manual_seed(0), **kwargs).prev_sample
output_1 = scheduler.step_pred(residual, 1, sample, generator=torch.manual_seed(0), **kwargs).prev_sample
self.assertEqual(output_0.shape, sample.shape)
self.assertEqual(output_0.shape, output_1.shape)
| 0 |
hf_public_repos/diffusers/tests | hf_public_repos/diffusers/tests/schedulers/test_scheduler_dpm_multi_inverse.py | import tempfile
import torch
from diffusers import DPMSolverMultistepInverseScheduler, DPMSolverMultistepScheduler
from .test_schedulers import SchedulerCommonTest
class DPMSolverMultistepSchedulerTest(SchedulerCommonTest):
scheduler_classes = (DPMSolverMultistepInverseScheduler,)
forward_default_kwargs = (("num_inference_steps", 25),)
def get_scheduler_config(self, **kwargs):
config = {
"num_train_timesteps": 1000,
"beta_start": 0.0001,
"beta_end": 0.02,
"beta_schedule": "linear",
"solver_order": 2,
"prediction_type": "epsilon",
"thresholding": False,
"sample_max_value": 1.0,
"algorithm_type": "dpmsolver++",
"solver_type": "midpoint",
"lower_order_final": False,
"lambda_min_clipped": -float("inf"),
"variance_type": None,
}
config.update(**kwargs)
return config
def check_over_configs(self, time_step=0, **config):
kwargs = dict(self.forward_default_kwargs)
num_inference_steps = kwargs.pop("num_inference_steps", None)
sample = self.dummy_sample
residual = 0.1 * sample
dummy_past_residuals = [residual + 0.2, residual + 0.15, residual + 0.10]
for scheduler_class in self.scheduler_classes:
scheduler_config = self.get_scheduler_config(**config)
scheduler = scheduler_class(**scheduler_config)
scheduler.set_timesteps(num_inference_steps)
# copy over dummy past residuals
scheduler.model_outputs = dummy_past_residuals[: scheduler.config.solver_order]
with tempfile.TemporaryDirectory() as tmpdirname:
scheduler.save_config(tmpdirname)
new_scheduler = scheduler_class.from_pretrained(tmpdirname)
new_scheduler.set_timesteps(num_inference_steps)
# copy over dummy past residuals
new_scheduler.model_outputs = dummy_past_residuals[: new_scheduler.config.solver_order]
output, new_output = sample, sample
for t in range(time_step, time_step + scheduler.config.solver_order + 1):
output = scheduler.step(residual, t, output, **kwargs).prev_sample
new_output = new_scheduler.step(residual, t, new_output, **kwargs).prev_sample
assert torch.sum(torch.abs(output - new_output)) < 1e-5, "Scheduler outputs are not identical"
def test_from_save_pretrained(self):
pass
def check_over_forward(self, time_step=0, **forward_kwargs):
kwargs = dict(self.forward_default_kwargs)
num_inference_steps = kwargs.pop("num_inference_steps", None)
sample = self.dummy_sample
residual = 0.1 * sample
dummy_past_residuals = [residual + 0.2, residual + 0.15, residual + 0.10]
for scheduler_class in self.scheduler_classes:
scheduler_config = self.get_scheduler_config()
scheduler = scheduler_class(**scheduler_config)
scheduler.set_timesteps(num_inference_steps)
# copy over dummy past residuals (must be after setting timesteps)
scheduler.model_outputs = dummy_past_residuals[: scheduler.config.solver_order]
with tempfile.TemporaryDirectory() as tmpdirname:
scheduler.save_config(tmpdirname)
new_scheduler = scheduler_class.from_pretrained(tmpdirname)
# copy over dummy past residuals
new_scheduler.set_timesteps(num_inference_steps)
# copy over dummy past residual (must be after setting timesteps)
new_scheduler.model_outputs = dummy_past_residuals[: new_scheduler.config.solver_order]
output = scheduler.step(residual, time_step, sample, **kwargs).prev_sample
new_output = new_scheduler.step(residual, time_step, sample, **kwargs).prev_sample
assert torch.sum(torch.abs(output - new_output)) < 1e-5, "Scheduler outputs are not identical"
def full_loop(self, scheduler=None, **config):
if scheduler is None:
scheduler_class = self.scheduler_classes[0]
scheduler_config = self.get_scheduler_config(**config)
scheduler = scheduler_class(**scheduler_config)
num_inference_steps = 10
model = self.dummy_model()
sample = self.dummy_sample_deter
scheduler.set_timesteps(num_inference_steps)
for i, t in enumerate(scheduler.timesteps):
residual = model(sample, t)
sample = scheduler.step(residual, t, sample).prev_sample
return sample
def test_step_shape(self):
kwargs = dict(self.forward_default_kwargs)
num_inference_steps = kwargs.pop("num_inference_steps", None)
for scheduler_class in self.scheduler_classes:
scheduler_config = self.get_scheduler_config()
scheduler = scheduler_class(**scheduler_config)
sample = self.dummy_sample
residual = 0.1 * sample
if num_inference_steps is not None and hasattr(scheduler, "set_timesteps"):
scheduler.set_timesteps(num_inference_steps)
elif num_inference_steps is not None and not hasattr(scheduler, "set_timesteps"):
kwargs["num_inference_steps"] = num_inference_steps
# copy over dummy past residuals (must be done after set_timesteps)
dummy_past_residuals = [residual + 0.2, residual + 0.15, residual + 0.10]
scheduler.model_outputs = dummy_past_residuals[: scheduler.config.solver_order]
time_step_0 = scheduler.timesteps[5]
time_step_1 = scheduler.timesteps[6]
output_0 = scheduler.step(residual, time_step_0, sample, **kwargs).prev_sample
output_1 = scheduler.step(residual, time_step_1, sample, **kwargs).prev_sample
self.assertEqual(output_0.shape, sample.shape)
self.assertEqual(output_0.shape, output_1.shape)
def test_timesteps(self):
for timesteps in [25, 50, 100, 999, 1000]:
self.check_over_configs(num_train_timesteps=timesteps)
def test_thresholding(self):
self.check_over_configs(thresholding=False)
for order in [1, 2, 3]:
for solver_type in ["midpoint", "heun"]:
for threshold in [0.5, 1.0, 2.0]:
for prediction_type in ["epsilon", "sample"]:
self.check_over_configs(
thresholding=True,
prediction_type=prediction_type,
sample_max_value=threshold,
algorithm_type="dpmsolver++",
solver_order=order,
solver_type=solver_type,
)
def test_prediction_type(self):
for prediction_type in ["epsilon", "v_prediction"]:
self.check_over_configs(prediction_type=prediction_type)
def test_solver_order_and_type(self):
for algorithm_type in ["dpmsolver", "dpmsolver++"]:
for solver_type in ["midpoint", "heun"]:
for order in [1, 2, 3]:
for prediction_type in ["epsilon", "sample"]:
self.check_over_configs(
solver_order=order,
solver_type=solver_type,
prediction_type=prediction_type,
algorithm_type=algorithm_type,
)
sample = self.full_loop(
solver_order=order,
solver_type=solver_type,
prediction_type=prediction_type,
algorithm_type=algorithm_type,
)
assert not torch.isnan(sample).any(), "Samples have nan numbers"
def test_lower_order_final(self):
self.check_over_configs(lower_order_final=True)
self.check_over_configs(lower_order_final=False)
def test_lambda_min_clipped(self):
self.check_over_configs(lambda_min_clipped=-float("inf"))
self.check_over_configs(lambda_min_clipped=-5.1)
def test_variance_type(self):
self.check_over_configs(variance_type=None)
self.check_over_configs(variance_type="learned_range")
def test_timestep_spacing(self):
for timestep_spacing in ["trailing", "leading"]:
self.check_over_configs(timestep_spacing=timestep_spacing)
def test_inference_steps(self):
for num_inference_steps in [1, 2, 3, 5, 10, 50, 100, 999, 1000]:
self.check_over_forward(num_inference_steps=num_inference_steps, time_step=0)
def test_full_loop_no_noise(self):
sample = self.full_loop()
result_mean = torch.mean(torch.abs(sample))
assert abs(result_mean.item() - 0.7047) < 1e-3
def test_full_loop_no_noise_thres(self):
sample = self.full_loop(thresholding=True, dynamic_thresholding_ratio=0.87, sample_max_value=0.5)
result_mean = torch.mean(torch.abs(sample))
assert abs(result_mean.item() - 19.8933) < 1e-3
def test_full_loop_with_v_prediction(self):
sample = self.full_loop(prediction_type="v_prediction")
result_mean = torch.mean(torch.abs(sample))
assert abs(result_mean.item() - 1.5194) < 1e-3
def test_full_loop_with_karras_and_v_prediction(self):
sample = self.full_loop(prediction_type="v_prediction", use_karras_sigmas=True)
result_mean = torch.mean(torch.abs(sample))
assert abs(result_mean.item() - 1.7833) < 1e-3
def test_switch(self):
# make sure that iterating over schedulers with same config names gives same results
# for defaults
scheduler = DPMSolverMultistepInverseScheduler(**self.get_scheduler_config())
sample = self.full_loop(scheduler=scheduler)
result_mean = torch.mean(torch.abs(sample))
assert abs(result_mean.item() - 0.7047) < 1e-3
scheduler = DPMSolverMultistepScheduler.from_config(scheduler.config)
scheduler = DPMSolverMultistepInverseScheduler.from_config(scheduler.config)
sample = self.full_loop(scheduler=scheduler)
new_result_mean = torch.mean(torch.abs(sample))
assert abs(new_result_mean.item() - result_mean.item()) < 1e-3
def test_fp16_support(self):
scheduler_class = self.scheduler_classes[0]
scheduler_config = self.get_scheduler_config(thresholding=True, dynamic_thresholding_ratio=0)
scheduler = scheduler_class(**scheduler_config)
num_inference_steps = 10
model = self.dummy_model()
sample = self.dummy_sample_deter.half()
scheduler.set_timesteps(num_inference_steps)
for i, t in enumerate(scheduler.timesteps):
residual = model(sample, t)
sample = scheduler.step(residual, t, sample).prev_sample
assert sample.dtype == torch.float16
def test_unique_timesteps(self, **config):
for scheduler_class in self.scheduler_classes:
scheduler_config = self.get_scheduler_config(**config)
scheduler = scheduler_class(**scheduler_config)
scheduler.set_timesteps(scheduler.config.num_train_timesteps)
assert len(scheduler.timesteps.unique()) == scheduler.num_inference_steps
| 0 |
hf_public_repos/diffusers/tests | hf_public_repos/diffusers/tests/schedulers/test_scheduler_ipndm.py | import tempfile
import torch
from diffusers import IPNDMScheduler
from .test_schedulers import SchedulerCommonTest
class IPNDMSchedulerTest(SchedulerCommonTest):
scheduler_classes = (IPNDMScheduler,)
forward_default_kwargs = (("num_inference_steps", 50),)
def get_scheduler_config(self, **kwargs):
config = {"num_train_timesteps": 1000}
config.update(**kwargs)
return config
def check_over_configs(self, time_step=0, **config):
kwargs = dict(self.forward_default_kwargs)
num_inference_steps = kwargs.pop("num_inference_steps", None)
sample = self.dummy_sample
residual = 0.1 * sample
dummy_past_residuals = [residual + 0.2, residual + 0.15, residual + 0.1, residual + 0.05]
for scheduler_class in self.scheduler_classes:
scheduler_config = self.get_scheduler_config(**config)
scheduler = scheduler_class(**scheduler_config)
scheduler.set_timesteps(num_inference_steps)
# copy over dummy past residuals
scheduler.ets = dummy_past_residuals[:]
if time_step is None:
time_step = scheduler.timesteps[len(scheduler.timesteps) // 2]
with tempfile.TemporaryDirectory() as tmpdirname:
scheduler.save_config(tmpdirname)
new_scheduler = scheduler_class.from_pretrained(tmpdirname)
new_scheduler.set_timesteps(num_inference_steps)
# copy over dummy past residuals
new_scheduler.ets = dummy_past_residuals[:]
output = scheduler.step(residual, time_step, sample, **kwargs).prev_sample
new_output = new_scheduler.step(residual, time_step, sample, **kwargs).prev_sample
assert torch.sum(torch.abs(output - new_output)) < 1e-5, "Scheduler outputs are not identical"
output = scheduler.step(residual, time_step, sample, **kwargs).prev_sample
new_output = new_scheduler.step(residual, time_step, sample, **kwargs).prev_sample
assert torch.sum(torch.abs(output - new_output)) < 1e-5, "Scheduler outputs are not identical"
def test_from_save_pretrained(self):
pass
def check_over_forward(self, time_step=0, **forward_kwargs):
kwargs = dict(self.forward_default_kwargs)
num_inference_steps = kwargs.pop("num_inference_steps", None)
sample = self.dummy_sample
residual = 0.1 * sample
dummy_past_residuals = [residual + 0.2, residual + 0.15, residual + 0.1, residual + 0.05]
for scheduler_class in self.scheduler_classes:
scheduler_config = self.get_scheduler_config()
scheduler = scheduler_class(**scheduler_config)
scheduler.set_timesteps(num_inference_steps)
# copy over dummy past residuals (must be after setting timesteps)
scheduler.ets = dummy_past_residuals[:]
if time_step is None:
time_step = scheduler.timesteps[len(scheduler.timesteps) // 2]
with tempfile.TemporaryDirectory() as tmpdirname:
scheduler.save_config(tmpdirname)
new_scheduler = scheduler_class.from_pretrained(tmpdirname)
# copy over dummy past residuals
new_scheduler.set_timesteps(num_inference_steps)
# copy over dummy past residual (must be after setting timesteps)
new_scheduler.ets = dummy_past_residuals[:]
output = scheduler.step(residual, time_step, sample, **kwargs).prev_sample
new_output = new_scheduler.step(residual, time_step, sample, **kwargs).prev_sample
assert torch.sum(torch.abs(output - new_output)) < 1e-5, "Scheduler outputs are not identical"
output = scheduler.step(residual, time_step, sample, **kwargs).prev_sample
new_output = new_scheduler.step(residual, time_step, sample, **kwargs).prev_sample
assert torch.sum(torch.abs(output - new_output)) < 1e-5, "Scheduler outputs are not identical"
def full_loop(self, **config):
scheduler_class = self.scheduler_classes[0]
scheduler_config = self.get_scheduler_config(**config)
scheduler = scheduler_class(**scheduler_config)
num_inference_steps = 10
model = self.dummy_model()
sample = self.dummy_sample_deter
scheduler.set_timesteps(num_inference_steps)
for i, t in enumerate(scheduler.timesteps):
residual = model(sample, t)
sample = scheduler.step(residual, t, sample).prev_sample
for i, t in enumerate(scheduler.timesteps):
residual = model(sample, t)
sample = scheduler.step(residual, t, sample).prev_sample
return sample
def test_step_shape(self):
kwargs = dict(self.forward_default_kwargs)
num_inference_steps = kwargs.pop("num_inference_steps", None)
for scheduler_class in self.scheduler_classes:
scheduler_config = self.get_scheduler_config()
scheduler = scheduler_class(**scheduler_config)
sample = self.dummy_sample
residual = 0.1 * sample
if num_inference_steps is not None and hasattr(scheduler, "set_timesteps"):
scheduler.set_timesteps(num_inference_steps)
elif num_inference_steps is not None and not hasattr(scheduler, "set_timesteps"):
kwargs["num_inference_steps"] = num_inference_steps
# copy over dummy past residuals (must be done after set_timesteps)
dummy_past_residuals = [residual + 0.2, residual + 0.15, residual + 0.1, residual + 0.05]
scheduler.ets = dummy_past_residuals[:]
time_step_0 = scheduler.timesteps[5]
time_step_1 = scheduler.timesteps[6]
output_0 = scheduler.step(residual, time_step_0, sample, **kwargs).prev_sample
output_1 = scheduler.step(residual, time_step_1, sample, **kwargs).prev_sample
self.assertEqual(output_0.shape, sample.shape)
self.assertEqual(output_0.shape, output_1.shape)
output_0 = scheduler.step(residual, time_step_0, sample, **kwargs).prev_sample
output_1 = scheduler.step(residual, time_step_1, sample, **kwargs).prev_sample
self.assertEqual(output_0.shape, sample.shape)
self.assertEqual(output_0.shape, output_1.shape)
def test_timesteps(self):
for timesteps in [100, 1000]:
self.check_over_configs(num_train_timesteps=timesteps, time_step=None)
def test_inference_steps(self):
for t, num_inference_steps in zip([1, 5, 10], [10, 50, 100]):
self.check_over_forward(num_inference_steps=num_inference_steps, time_step=None)
def test_full_loop_no_noise(self):
sample = self.full_loop()
result_mean = torch.mean(torch.abs(sample))
assert abs(result_mean.item() - 2540529) < 10
| 0 |
hf_public_repos/diffusers/tests | hf_public_repos/diffusers/tests/schedulers/test_scheduler_heun.py | import torch
from diffusers import HeunDiscreteScheduler
from diffusers.utils import torch_device
from .test_schedulers import SchedulerCommonTest
class HeunDiscreteSchedulerTest(SchedulerCommonTest):
scheduler_classes = (HeunDiscreteScheduler,)
num_inference_steps = 10
def get_scheduler_config(self, **kwargs):
config = {
"num_train_timesteps": 1100,
"beta_start": 0.0001,
"beta_end": 0.02,
"beta_schedule": "linear",
}
config.update(**kwargs)
return config
def test_timesteps(self):
for timesteps in [10, 50, 100, 1000]:
self.check_over_configs(num_train_timesteps=timesteps)
def test_betas(self):
for beta_start, beta_end in zip([0.00001, 0.0001, 0.001], [0.0002, 0.002, 0.02]):
self.check_over_configs(beta_start=beta_start, beta_end=beta_end)
def test_schedules(self):
for schedule in ["linear", "scaled_linear", "exp"]:
self.check_over_configs(beta_schedule=schedule)
def test_clip_sample(self):
for clip_sample_range in [1.0, 2.0, 3.0]:
self.check_over_configs(clip_sample_range=clip_sample_range, clip_sample=True)
def test_prediction_type(self):
for prediction_type in ["epsilon", "v_prediction", "sample"]:
self.check_over_configs(prediction_type=prediction_type)
def test_full_loop_no_noise(self):
scheduler_class = self.scheduler_classes[0]
scheduler_config = self.get_scheduler_config()
scheduler = scheduler_class(**scheduler_config)
scheduler.set_timesteps(self.num_inference_steps)
model = self.dummy_model()
sample = self.dummy_sample_deter * scheduler.init_noise_sigma
sample = sample.to(torch_device)
for i, t in enumerate(scheduler.timesteps):
sample = scheduler.scale_model_input(sample, t)
model_output = model(sample, t)
output = scheduler.step(model_output, t, sample)
sample = output.prev_sample
result_sum = torch.sum(torch.abs(sample))
result_mean = torch.mean(torch.abs(sample))
if torch_device in ["cpu", "mps"]:
assert abs(result_sum.item() - 0.1233) < 1e-2
assert abs(result_mean.item() - 0.0002) < 1e-3
else:
# CUDA
assert abs(result_sum.item() - 0.1233) < 1e-2
assert abs(result_mean.item() - 0.0002) < 1e-3
def test_full_loop_with_v_prediction(self):
scheduler_class = self.scheduler_classes[0]
scheduler_config = self.get_scheduler_config(prediction_type="v_prediction")
scheduler = scheduler_class(**scheduler_config)
scheduler.set_timesteps(self.num_inference_steps)
model = self.dummy_model()
sample = self.dummy_sample_deter * scheduler.init_noise_sigma
sample = sample.to(torch_device)
for i, t in enumerate(scheduler.timesteps):
sample = scheduler.scale_model_input(sample, t)
model_output = model(sample, t)
output = scheduler.step(model_output, t, sample)
sample = output.prev_sample
result_sum = torch.sum(torch.abs(sample))
result_mean = torch.mean(torch.abs(sample))
if torch_device in ["cpu", "mps"]:
assert abs(result_sum.item() - 4.6934e-07) < 1e-2
assert abs(result_mean.item() - 6.1112e-10) < 1e-3
else:
# CUDA
assert abs(result_sum.item() - 4.693428650170972e-07) < 1e-2
assert abs(result_mean.item() - 0.0002) < 1e-3
def test_full_loop_device(self):
scheduler_class = self.scheduler_classes[0]
scheduler_config = self.get_scheduler_config()
scheduler = scheduler_class(**scheduler_config)
scheduler.set_timesteps(self.num_inference_steps, device=torch_device)
model = self.dummy_model()
sample = self.dummy_sample_deter.to(torch_device) * scheduler.init_noise_sigma
for t in scheduler.timesteps:
sample = scheduler.scale_model_input(sample, t)
model_output = model(sample, t)
output = scheduler.step(model_output, t, sample)
sample = output.prev_sample
result_sum = torch.sum(torch.abs(sample))
result_mean = torch.mean(torch.abs(sample))
if str(torch_device).startswith("cpu"):
# The following sum varies between 148 and 156 on mps. Why?
assert abs(result_sum.item() - 0.1233) < 1e-2
assert abs(result_mean.item() - 0.0002) < 1e-3
elif str(torch_device).startswith("mps"):
# Larger tolerance on mps
assert abs(result_mean.item() - 0.0002) < 1e-2
else:
# CUDA
assert abs(result_sum.item() - 0.1233) < 1e-2
assert abs(result_mean.item() - 0.0002) < 1e-3
def test_full_loop_device_karras_sigmas(self):
scheduler_class = self.scheduler_classes[0]
scheduler_config = self.get_scheduler_config()
scheduler = scheduler_class(**scheduler_config, use_karras_sigmas=True)
scheduler.set_timesteps(self.num_inference_steps, device=torch_device)
model = self.dummy_model()
sample = self.dummy_sample_deter.to(torch_device) * scheduler.init_noise_sigma
sample = sample.to(torch_device)
for t in scheduler.timesteps:
sample = scheduler.scale_model_input(sample, t)
model_output = model(sample, t)
output = scheduler.step(model_output, t, sample)
sample = output.prev_sample
result_sum = torch.sum(torch.abs(sample))
result_mean = torch.mean(torch.abs(sample))
assert abs(result_sum.item() - 0.00015) < 1e-2
assert abs(result_mean.item() - 1.9869554535034695e-07) < 1e-2
| 0 |
hf_public_repos/diffusers/tests | hf_public_repos/diffusers/tests/schedulers/test_scheduler_dpm_sde.py | import torch
from diffusers import DPMSolverSDEScheduler
from diffusers.utils import torch_device
from diffusers.utils.testing_utils import require_torchsde
from .test_schedulers import SchedulerCommonTest
@require_torchsde
class DPMSolverSDESchedulerTest(SchedulerCommonTest):
scheduler_classes = (DPMSolverSDEScheduler,)
num_inference_steps = 10
def get_scheduler_config(self, **kwargs):
config = {
"num_train_timesteps": 1100,
"beta_start": 0.0001,
"beta_end": 0.02,
"beta_schedule": "linear",
"noise_sampler_seed": 0,
}
config.update(**kwargs)
return config
def test_timesteps(self):
for timesteps in [10, 50, 100, 1000]:
self.check_over_configs(num_train_timesteps=timesteps)
def test_betas(self):
for beta_start, beta_end in zip([0.00001, 0.0001, 0.001], [0.0002, 0.002, 0.02]):
self.check_over_configs(beta_start=beta_start, beta_end=beta_end)
def test_schedules(self):
for schedule in ["linear", "scaled_linear"]:
self.check_over_configs(beta_schedule=schedule)
def test_prediction_type(self):
for prediction_type in ["epsilon", "v_prediction"]:
self.check_over_configs(prediction_type=prediction_type)
def test_full_loop_no_noise(self):
scheduler_class = self.scheduler_classes[0]
scheduler_config = self.get_scheduler_config()
scheduler = scheduler_class(**scheduler_config)
scheduler.set_timesteps(self.num_inference_steps)
model = self.dummy_model()
sample = self.dummy_sample_deter * scheduler.init_noise_sigma
sample = sample.to(torch_device)
for i, t in enumerate(scheduler.timesteps):
sample = scheduler.scale_model_input(sample, t)
model_output = model(sample, t)
output = scheduler.step(model_output, t, sample)
sample = output.prev_sample
result_sum = torch.sum(torch.abs(sample))
result_mean = torch.mean(torch.abs(sample))
if torch_device in ["mps"]:
assert abs(result_sum.item() - 167.47821044921875) < 1e-2
assert abs(result_mean.item() - 0.2178705964565277) < 1e-3
elif torch_device in ["cuda"]:
assert abs(result_sum.item() - 171.59352111816406) < 1e-2
assert abs(result_mean.item() - 0.22342906892299652) < 1e-3
else:
assert abs(result_sum.item() - 162.52383422851562) < 1e-2
assert abs(result_mean.item() - 0.211619570851326) < 1e-3
def test_full_loop_with_v_prediction(self):
scheduler_class = self.scheduler_classes[0]
scheduler_config = self.get_scheduler_config(prediction_type="v_prediction")
scheduler = scheduler_class(**scheduler_config)
scheduler.set_timesteps(self.num_inference_steps)
model = self.dummy_model()
sample = self.dummy_sample_deter * scheduler.init_noise_sigma
sample = sample.to(torch_device)
for i, t in enumerate(scheduler.timesteps):
sample = scheduler.scale_model_input(sample, t)
model_output = model(sample, t)
output = scheduler.step(model_output, t, sample)
sample = output.prev_sample
result_sum = torch.sum(torch.abs(sample))
result_mean = torch.mean(torch.abs(sample))
if torch_device in ["mps"]:
assert abs(result_sum.item() - 124.77149200439453) < 1e-2
assert abs(result_mean.item() - 0.16226289014816284) < 1e-3
elif torch_device in ["cuda"]:
assert abs(result_sum.item() - 128.1663360595703) < 1e-2
assert abs(result_mean.item() - 0.16688326001167297) < 1e-3
else:
assert abs(result_sum.item() - 119.8487548828125) < 1e-2
assert abs(result_mean.item() - 0.1560530662536621) < 1e-3
def test_full_loop_device(self):
scheduler_class = self.scheduler_classes[0]
scheduler_config = self.get_scheduler_config()
scheduler = scheduler_class(**scheduler_config)
scheduler.set_timesteps(self.num_inference_steps, device=torch_device)
model = self.dummy_model()
sample = self.dummy_sample_deter.to(torch_device) * scheduler.init_noise_sigma
for t in scheduler.timesteps:
sample = scheduler.scale_model_input(sample, t)
model_output = model(sample, t)
output = scheduler.step(model_output, t, sample)
sample = output.prev_sample
result_sum = torch.sum(torch.abs(sample))
result_mean = torch.mean(torch.abs(sample))
if torch_device in ["mps"]:
assert abs(result_sum.item() - 167.46957397460938) < 1e-2
assert abs(result_mean.item() - 0.21805934607982635) < 1e-3
elif torch_device in ["cuda"]:
assert abs(result_sum.item() - 171.59353637695312) < 1e-2
assert abs(result_mean.item() - 0.22342908382415771) < 1e-3
else:
assert abs(result_sum.item() - 162.52383422851562) < 1e-2
assert abs(result_mean.item() - 0.211619570851326) < 1e-3
def test_full_loop_device_karras_sigmas(self):
scheduler_class = self.scheduler_classes[0]
scheduler_config = self.get_scheduler_config()
scheduler = scheduler_class(**scheduler_config, use_karras_sigmas=True)
scheduler.set_timesteps(self.num_inference_steps, device=torch_device)
model = self.dummy_model()
sample = self.dummy_sample_deter.to(torch_device) * scheduler.init_noise_sigma
sample = sample.to(torch_device)
for t in scheduler.timesteps:
sample = scheduler.scale_model_input(sample, t)
model_output = model(sample, t)
output = scheduler.step(model_output, t, sample)
sample = output.prev_sample
result_sum = torch.sum(torch.abs(sample))
result_mean = torch.mean(torch.abs(sample))
if torch_device in ["mps"]:
assert abs(result_sum.item() - 176.66974135742188) < 1e-2
assert abs(result_mean.item() - 0.23003872730981811) < 1e-2
elif torch_device in ["cuda"]:
assert abs(result_sum.item() - 177.63653564453125) < 1e-2
assert abs(result_mean.item() - 0.23003872730981811) < 1e-2
else:
assert abs(result_sum.item() - 170.3135223388672) < 1e-2
assert abs(result_mean.item() - 0.23003872730981811) < 1e-2
| 0 |
hf_public_repos/diffusers/tests | hf_public_repos/diffusers/tests/schedulers/test_scheduler_kdpm2_discrete.py | import torch
from diffusers import KDPM2DiscreteScheduler
from diffusers.utils import torch_device
from .test_schedulers import SchedulerCommonTest
class KDPM2DiscreteSchedulerTest(SchedulerCommonTest):
scheduler_classes = (KDPM2DiscreteScheduler,)
num_inference_steps = 10
def get_scheduler_config(self, **kwargs):
config = {
"num_train_timesteps": 1100,
"beta_start": 0.0001,
"beta_end": 0.02,
"beta_schedule": "linear",
}
config.update(**kwargs)
return config
def test_timesteps(self):
for timesteps in [10, 50, 100, 1000]:
self.check_over_configs(num_train_timesteps=timesteps)
def test_betas(self):
for beta_start, beta_end in zip([0.00001, 0.0001, 0.001], [0.0002, 0.002, 0.02]):
self.check_over_configs(beta_start=beta_start, beta_end=beta_end)
def test_schedules(self):
for schedule in ["linear", "scaled_linear"]:
self.check_over_configs(beta_schedule=schedule)
def test_prediction_type(self):
for prediction_type in ["epsilon", "v_prediction"]:
self.check_over_configs(prediction_type=prediction_type)
def test_full_loop_with_v_prediction(self):
scheduler_class = self.scheduler_classes[0]
scheduler_config = self.get_scheduler_config(prediction_type="v_prediction")
scheduler = scheduler_class(**scheduler_config)
scheduler.set_timesteps(self.num_inference_steps)
model = self.dummy_model()
sample = self.dummy_sample_deter * scheduler.init_noise_sigma
sample = sample.to(torch_device)
for i, t in enumerate(scheduler.timesteps):
sample = scheduler.scale_model_input(sample, t)
model_output = model(sample, t)
output = scheduler.step(model_output, t, sample)
sample = output.prev_sample
result_sum = torch.sum(torch.abs(sample))
result_mean = torch.mean(torch.abs(sample))
if torch_device in ["cpu", "mps"]:
assert abs(result_sum.item() - 4.6934e-07) < 1e-2
assert abs(result_mean.item() - 6.1112e-10) < 1e-3
else:
# CUDA
assert abs(result_sum.item() - 4.693428650170972e-07) < 1e-2
assert abs(result_mean.item() - 0.0002) < 1e-3
def test_full_loop_no_noise(self):
if torch_device == "mps":
return
scheduler_class = self.scheduler_classes[0]
scheduler_config = self.get_scheduler_config()
scheduler = scheduler_class(**scheduler_config)
scheduler.set_timesteps(self.num_inference_steps)
model = self.dummy_model()
sample = self.dummy_sample_deter * scheduler.init_noise_sigma
sample = sample.to(torch_device)
for i, t in enumerate(scheduler.timesteps):
sample = scheduler.scale_model_input(sample, t)
model_output = model(sample, t)
output = scheduler.step(model_output, t, sample)
sample = output.prev_sample
result_sum = torch.sum(torch.abs(sample))
result_mean = torch.mean(torch.abs(sample))
if torch_device in ["cpu", "mps"]:
assert abs(result_sum.item() - 20.4125) < 1e-2
assert abs(result_mean.item() - 0.0266) < 1e-3
else:
# CUDA
assert abs(result_sum.item() - 20.4125) < 1e-2
assert abs(result_mean.item() - 0.0266) < 1e-3
def test_full_loop_device(self):
if torch_device == "mps":
return
scheduler_class = self.scheduler_classes[0]
scheduler_config = self.get_scheduler_config()
scheduler = scheduler_class(**scheduler_config)
scheduler.set_timesteps(self.num_inference_steps, device=torch_device)
model = self.dummy_model()
sample = self.dummy_sample_deter.to(torch_device) * scheduler.init_noise_sigma
for t in scheduler.timesteps:
sample = scheduler.scale_model_input(sample, t)
model_output = model(sample, t)
output = scheduler.step(model_output, t, sample)
sample = output.prev_sample
result_sum = torch.sum(torch.abs(sample))
result_mean = torch.mean(torch.abs(sample))
if str(torch_device).startswith("cpu"):
# The following sum varies between 148 and 156 on mps. Why?
assert abs(result_sum.item() - 20.4125) < 1e-2
assert abs(result_mean.item() - 0.0266) < 1e-3
else:
# CUDA
assert abs(result_sum.item() - 20.4125) < 1e-2
assert abs(result_mean.item() - 0.0266) < 1e-3
| 0 |
hf_public_repos/diffusers/tests | hf_public_repos/diffusers/tests/schedulers/test_scheduler_vq_diffusion.py | import torch
import torch.nn.functional as F
from diffusers import VQDiffusionScheduler
from .test_schedulers import SchedulerCommonTest
class VQDiffusionSchedulerTest(SchedulerCommonTest):
scheduler_classes = (VQDiffusionScheduler,)
def get_scheduler_config(self, **kwargs):
config = {
"num_vec_classes": 4097,
"num_train_timesteps": 100,
}
config.update(**kwargs)
return config
def dummy_sample(self, num_vec_classes):
batch_size = 4
height = 8
width = 8
sample = torch.randint(0, num_vec_classes, (batch_size, height * width))
return sample
@property
def dummy_sample_deter(self):
assert False
def dummy_model(self, num_vec_classes):
def model(sample, t, *args):
batch_size, num_latent_pixels = sample.shape
logits = torch.rand((batch_size, num_vec_classes - 1, num_latent_pixels))
return_value = F.log_softmax(logits.double(), dim=1).float()
return return_value
return model
def test_timesteps(self):
for timesteps in [2, 5, 100, 1000]:
self.check_over_configs(num_train_timesteps=timesteps)
def test_num_vec_classes(self):
for num_vec_classes in [5, 100, 1000, 4000]:
self.check_over_configs(num_vec_classes=num_vec_classes)
def test_time_indices(self):
for t in [0, 50, 99]:
self.check_over_forward(time_step=t)
def test_add_noise_device(self):
pass
| 0 |
hf_public_repos/diffusers/tests | hf_public_repos/diffusers/tests/schedulers/test_scheduler_lms.py | import torch
from diffusers import LMSDiscreteScheduler
from diffusers.utils import torch_device
from .test_schedulers import SchedulerCommonTest
class LMSDiscreteSchedulerTest(SchedulerCommonTest):
scheduler_classes = (LMSDiscreteScheduler,)
num_inference_steps = 10
def get_scheduler_config(self, **kwargs):
config = {
"num_train_timesteps": 1100,
"beta_start": 0.0001,
"beta_end": 0.02,
"beta_schedule": "linear",
}
config.update(**kwargs)
return config
def test_timesteps(self):
for timesteps in [10, 50, 100, 1000]:
self.check_over_configs(num_train_timesteps=timesteps)
def test_betas(self):
for beta_start, beta_end in zip([0.00001, 0.0001, 0.001], [0.0002, 0.002, 0.02]):
self.check_over_configs(beta_start=beta_start, beta_end=beta_end)
def test_schedules(self):
for schedule in ["linear", "scaled_linear"]:
self.check_over_configs(beta_schedule=schedule)
def test_prediction_type(self):
for prediction_type in ["epsilon", "v_prediction"]:
self.check_over_configs(prediction_type=prediction_type)
def test_time_indices(self):
for t in [0, 500, 800]:
self.check_over_forward(time_step=t)
def test_full_loop_no_noise(self):
scheduler_class = self.scheduler_classes[0]
scheduler_config = self.get_scheduler_config()
scheduler = scheduler_class(**scheduler_config)
scheduler.set_timesteps(self.num_inference_steps)
model = self.dummy_model()
sample = self.dummy_sample_deter * scheduler.init_noise_sigma
for i, t in enumerate(scheduler.timesteps):
sample = scheduler.scale_model_input(sample, t)
model_output = model(sample, t)
output = scheduler.step(model_output, t, sample)
sample = output.prev_sample
result_sum = torch.sum(torch.abs(sample))
result_mean = torch.mean(torch.abs(sample))
assert abs(result_sum.item() - 1006.388) < 1e-2
assert abs(result_mean.item() - 1.31) < 1e-3
def test_full_loop_with_v_prediction(self):
scheduler_class = self.scheduler_classes[0]
scheduler_config = self.get_scheduler_config(prediction_type="v_prediction")
scheduler = scheduler_class(**scheduler_config)
scheduler.set_timesteps(self.num_inference_steps)
model = self.dummy_model()
sample = self.dummy_sample_deter * scheduler.init_noise_sigma
for i, t in enumerate(scheduler.timesteps):
sample = scheduler.scale_model_input(sample, t)
model_output = model(sample, t)
output = scheduler.step(model_output, t, sample)
sample = output.prev_sample
result_sum = torch.sum(torch.abs(sample))
result_mean = torch.mean(torch.abs(sample))
assert abs(result_sum.item() - 0.0017) < 1e-2
assert abs(result_mean.item() - 2.2676e-06) < 1e-3
def test_full_loop_device(self):
scheduler_class = self.scheduler_classes[0]
scheduler_config = self.get_scheduler_config()
scheduler = scheduler_class(**scheduler_config)
scheduler.set_timesteps(self.num_inference_steps, device=torch_device)
model = self.dummy_model()
sample = self.dummy_sample_deter * scheduler.init_noise_sigma.cpu()
sample = sample.to(torch_device)
for i, t in enumerate(scheduler.timesteps):
sample = scheduler.scale_model_input(sample, t)
model_output = model(sample, t)
output = scheduler.step(model_output, t, sample)
sample = output.prev_sample
result_sum = torch.sum(torch.abs(sample))
result_mean = torch.mean(torch.abs(sample))
assert abs(result_sum.item() - 1006.388) < 1e-2
assert abs(result_mean.item() - 1.31) < 1e-3
def test_full_loop_device_karras_sigmas(self):
scheduler_class = self.scheduler_classes[0]
scheduler_config = self.get_scheduler_config()
scheduler = scheduler_class(**scheduler_config, use_karras_sigmas=True)
scheduler.set_timesteps(self.num_inference_steps, device=torch_device)
model = self.dummy_model()
sample = self.dummy_sample_deter.to(torch_device) * scheduler.init_noise_sigma
sample = sample.to(torch_device)
for t in scheduler.timesteps:
sample = scheduler.scale_model_input(sample, t)
model_output = model(sample, t)
output = scheduler.step(model_output, t, sample)
sample = output.prev_sample
result_sum = torch.sum(torch.abs(sample))
result_mean = torch.mean(torch.abs(sample))
assert abs(result_sum.item() - 3812.9927) < 2e-2
assert abs(result_mean.item() - 4.9648) < 1e-3
| 0 |
hf_public_repos/diffusers | hf_public_repos/diffusers/docs/README.md | <!---
Copyright 2023- The HuggingFace Team. All rights reserved.
Licensed under the Apache License, Version 2.0 (the "License");
you may not use this file except in compliance with the License.
You may obtain a copy of the License at
http://www.apache.org/licenses/LICENSE-2.0
Unless required by applicable law or agreed to in writing, software
distributed under the License is distributed on an "AS IS" BASIS,
WITHOUT WARRANTIES OR CONDITIONS OF ANY KIND, either express or implied.
See the License for the specific language governing permissions and
limitations under the License.
-->
# Generating the documentation
To generate the documentation, you first have to build it. Several packages are necessary to build the doc,
you can install them with the following command, at the root of the code repository:
```bash
pip install -e ".[docs]"
```
Then you need to install our open source documentation builder tool:
```bash
pip install git+https://github.com/huggingface/doc-builder
```
---
**NOTE**
You only need to generate the documentation to inspect it locally (if you're planning changes and want to
check how they look before committing for instance). You don't have to commit the built documentation.
---
## Previewing the documentation
To preview the docs, first install the `watchdog` module with:
```bash
pip install watchdog
```
Then run the following command:
```bash
doc-builder preview {package_name} {path_to_docs}
```
For example:
```bash
doc-builder preview diffusers docs/source/en
```
The docs will be viewable at [http://localhost:3000](http://localhost:3000). You can also preview the docs once you have opened a PR. You will see a bot add a comment to a link where the documentation with your changes lives.
---
**NOTE**
The `preview` command only works with existing doc files. When you add a completely new file, you need to update `_toctree.yml` & restart `preview` command (`ctrl-c` to stop it & call `doc-builder preview ...` again).
---
## Adding a new element to the navigation bar
Accepted files are Markdown (.md).
Create a file with its extension and put it in the source directory. You can then link it to the toc-tree by putting
the filename without the extension in the [`_toctree.yml`](https://github.com/huggingface/diffusers/blob/main/docs/source/_toctree.yml) file.
## Renaming section headers and moving sections
It helps to keep the old links working when renaming the section header and/or moving sections from one document to another. This is because the old links are likely to be used in Issues, Forums, and Social media and it'd make for a much more superior user experience if users reading those months later could still easily navigate to the originally intended information.
Therefore, we simply keep a little map of moved sections at the end of the document where the original section was. The key is to preserve the original anchor.
So if you renamed a section from: "Section A" to "Section B", then you can add at the end of the file:
```
Sections that were moved:
[ <a href="#section-b">Section A</a><a id="section-a"></a> ]
```
and of course, if you moved it to another file, then:
```
Sections that were moved:
[ <a href="../new-file#section-b">Section A</a><a id="section-a"></a> ]
```
Use the relative style to link to the new file so that the versioned docs continue to work.
For an example of a rich moved section set please see the very end of [the transformers Trainer doc](https://github.com/huggingface/transformers/blob/main/docs/source/en/main_classes/trainer.md).
## Writing Documentation - Specification
The `huggingface/diffusers` documentation follows the
[Google documentation](https://sphinxcontrib-napoleon.readthedocs.io/en/latest/example_google.html) style for docstrings,
although we can write them directly in Markdown.
### Adding a new tutorial
Adding a new tutorial or section is done in two steps:
- Add a new file under `docs/source`. This file can either be ReStructuredText (.rst) or Markdown (.md).
- Link that file in `docs/source/_toctree.yml` on the correct toc-tree.
Make sure to put your new file under the proper section. It's unlikely to go in the first section (*Get Started*), so
depending on the intended targets (beginners, more advanced users, or researchers) it should go in sections two, three, or four.
### Adding a new pipeline/scheduler
When adding a new pipeline:
- create a file `xxx.md` under `docs/source/api/pipelines` (don't hesitate to copy an existing file as template).
- Link that file in (*Diffusers Summary*) section in `docs/source/api/pipelines/overview.md`, along with the link to the paper, and a colab notebook (if available).
- Write a short overview of the diffusion model:
- Overview with paper & authors
- Paper abstract
- Tips and tricks and how to use it best
- Possible an end-to-end example of how to use it
- Add all the pipeline classes that should be linked in the diffusion model. These classes should be added using our Markdown syntax. By default as follows:
```
## XXXPipeline
[[autodoc]] XXXPipeline
- all
- __call__
```
This will include every public method of the pipeline that is documented, as well as the `__call__` method that is not documented by default. If you just want to add additional methods that are not documented, you can put the list of all methods to add in a list that contains `all`.
```
[[autodoc]] XXXPipeline
- all
- __call__
- enable_attention_slicing
- disable_attention_slicing
- enable_xformers_memory_efficient_attention
- disable_xformers_memory_efficient_attention
```
You can follow the same process to create a new scheduler under the `docs/source/api/schedulers` folder
### Writing source documentation
Values that should be put in `code` should either be surrounded by backticks: \`like so\`. Note that argument names
and objects like True, None, or any strings should usually be put in `code`.
When mentioning a class, function, or method, it is recommended to use our syntax for internal links so that our tool
adds a link to its documentation with this syntax: \[\`XXXClass\`\] or \[\`function\`\]. This requires the class or
function to be in the main package.
If you want to create a link to some internal class or function, you need to
provide its path. For instance: \[\`pipelines.ImagePipelineOutput\`\]. This will be converted into a link with
`pipelines.ImagePipelineOutput` in the description. To get rid of the path and only keep the name of the object you are
linking to in the description, add a ~: \[\`~pipelines.ImagePipelineOutput\`\] will generate a link with `ImagePipelineOutput` in the description.
The same works for methods so you can either use \[\`XXXClass.method\`\] or \[~\`XXXClass.method\`\].
#### Defining arguments in a method
Arguments should be defined with the `Args:` (or `Arguments:` or `Parameters:`) prefix, followed by a line return and
an indentation. The argument should be followed by its type, with its shape if it is a tensor, a colon, and its
description:
```
Args:
n_layers (`int`): The number of layers of the model.
```
If the description is too long to fit in one line, another indentation is necessary before writing the description
after the argument.
Here's an example showcasing everything so far:
```
Args:
input_ids (`torch.LongTensor` of shape `(batch_size, sequence_length)`):
Indices of input sequence tokens in the vocabulary.
Indices can be obtained using [`AlbertTokenizer`]. See [`~PreTrainedTokenizer.encode`] and
[`~PreTrainedTokenizer.__call__`] for details.
[What are input IDs?](../glossary#input-ids)
```
For optional arguments or arguments with defaults we follow the following syntax: imagine we have a function with the
following signature:
```
def my_function(x: str = None, a: float = 1):
```
then its documentation should look like this:
```
Args:
x (`str`, *optional*):
This argument controls ...
a (`float`, *optional*, defaults to 1):
This argument is used to ...
```
Note that we always omit the "defaults to \`None\`" when None is the default for any argument. Also note that even
if the first line describing your argument type and its default gets long, you can't break it on several lines. You can
however write as many lines as you want in the indented description (see the example above with `input_ids`).
#### Writing a multi-line code block
Multi-line code blocks can be useful for displaying examples. They are done between two lines of three backticks as usual in Markdown:
````
```
# first line of code
# second line
# etc
```
````
#### Writing a return block
The return block should be introduced with the `Returns:` prefix, followed by a line return and an indentation.
The first line should be the type of the return, followed by a line return. No need to indent further for the elements
building the return.
Here's an example of a single value return:
```
Returns:
`List[int]`: A list of integers in the range [0, 1] --- 1 for a special token, 0 for a sequence token.
```
Here's an example of a tuple return, comprising several objects:
```
Returns:
`tuple(torch.FloatTensor)` comprising various elements depending on the configuration ([`BertConfig`]) and inputs:
- ** loss** (*optional*, returned when `masked_lm_labels` is provided) `torch.FloatTensor` of shape `(1,)` --
Total loss is the sum of the masked language modeling loss and the next sequence prediction (classification) loss.
- **prediction_scores** (`torch.FloatTensor` of shape `(batch_size, sequence_length, config.vocab_size)`) --
Prediction scores of the language modeling head (scores for each vocabulary token before SoftMax).
```
#### Adding an image
Due to the rapidly growing repository, it is important to make sure that no files that would significantly weigh down the repository are added. This includes images, videos, and other non-text files. We prefer to leverage a hf.co hosted `dataset` like
the ones hosted on [`hf-internal-testing`](https://huggingface.co/hf-internal-testing) in which to place these files and reference
them by URL. We recommend putting them in the following dataset: [huggingface/documentation-images](https://huggingface.co/datasets/huggingface/documentation-images).
If an external contribution, feel free to add the images to your PR and ask a Hugging Face member to migrate your images
to this dataset.
## Styling the docstring
We have an automatic script running with the `make style` command that will make sure that:
- the docstrings fully take advantage of the line width
- all code examples are formatted using black, like the code of the Transformers library
This script may have some weird failures if you made a syntax mistake or if you uncover a bug. Therefore, it's
recommended to commit your changes before running `make style`, so you can revert the changes done by that script
easily.
| 0 |
hf_public_repos/diffusers | hf_public_repos/diffusers/docs/TRANSLATING.md | ### Translating the Diffusers documentation into your language
As part of our mission to democratize machine learning, we'd love to make the Diffusers library available in many more languages! Follow the steps below if you want to help translate the documentation into your language 🙏.
**🗞️ Open an issue**
To get started, navigate to the [Issues](https://github.com/huggingface/diffusers/issues) page of this repo and check if anyone else has opened an issue for your language. If not, open a new issue by selecting the "Translation template" from the "New issue" button.
Once an issue exists, post a comment to indicate which chapters you'd like to work on, and we'll add your name to the list.
**🍴 Fork the repository**
First, you'll need to [fork the Diffusers repo](https://docs.github.com/en/get-started/quickstart/fork-a-repo). You can do this by clicking on the **Fork** button on the top-right corner of this repo's page.
Once you've forked the repo, you'll want to get the files on your local machine for editing. You can do that by cloning the fork with Git as follows:
```bash
git clone https://github.com/YOUR-USERNAME/diffusers.git
```
**📋 Copy-paste the English version with a new language code**
The documentation files are in one leading directory:
- [`docs/source`](https://github.com/huggingface/diffusers/tree/main/docs/source): All the documentation materials are organized here by language.
You'll only need to copy the files in the [`docs/source/en`](https://github.com/huggingface/diffusers/tree/main/docs/source/en) directory, so first navigate to your fork of the repo and run the following:
```bash
cd ~/path/to/diffusers/docs
cp -r source/en source/LANG-ID
```
Here, `LANG-ID` should be one of the ISO 639-1 or ISO 639-2 language codes -- see [here](https://www.loc.gov/standards/iso639-2/php/code_list.php) for a handy table.
**✍️ Start translating**
The fun part comes - translating the text!
The first thing we recommend is translating the part of the `_toctree.yml` file that corresponds to your doc chapter. This file is used to render the table of contents on the website.
> 🙋 If the `_toctree.yml` file doesn't yet exist for your language, you can create one by copy-pasting from the English version and deleting the sections unrelated to your chapter. Just make sure it exists in the `docs/source/LANG-ID/` directory!
The fields you should add are `local` (with the name of the file containing the translation; e.g. `autoclass_tutorial`), and `title` (with the title of the doc in your language; e.g. `Load pretrained instances with an AutoClass`) -- as a reference, here is the `_toctree.yml` for [English](https://github.com/huggingface/diffusers/blob/main/docs/source/en/_toctree.yml):
```yaml
- sections:
- local: pipeline_tutorial # Do not change this! Use the same name for your .md file
title: Pipelines for inference # Translate this!
...
title: Tutorials # Translate this!
```
Once you have translated the `_toctree.yml` file, you can start translating the [MDX](https://mdxjs.com/) files associated with your docs chapter.
> 🙋 If you'd like others to help you with the translation, you should [open an issue](https://github.com/huggingface/diffusers/issues) and tag @patrickvonplaten.
| 0 |
hf_public_repos/diffusers/docs | hf_public_repos/diffusers/docs/source/_config.py | # docstyle-ignore
INSTALL_CONTENT = """
# Diffusers installation
! pip install diffusers transformers datasets accelerate
# To install from source instead of the last release, comment the command above and uncomment the following one.
# ! pip install git+https://github.com/huggingface/diffusers.git
"""
notebook_first_cells = [{"type": "code", "content": INSTALL_CONTENT}]
| 0 |
hf_public_repos/diffusers/docs/source | hf_public_repos/diffusers/docs/source/ko/quicktour.md | <!--Copyright 2023 The HuggingFace Team. All rights reserved.
Licensed under the Apache License, Version 2.0 (the "License"); you may not use this file except in compliance with
the License. You may obtain a copy of the License at
http://www.apache.org/licenses/LICENSE-2.0
Unless required by applicable law or agreed to in writing, software distributed under the License is distributed on
an "AS IS" BASIS, WITHOUT WARRANTIES OR CONDITIONS OF ANY KIND, either express or implied. See the License for the
specific language governing permissions and limitations under the License.
-->
# 훑어보기
🧨 Diffusers로 빠르게 시작하고 실행하세요!
이 훑어보기는 여러분이 개발자, 일반사용자 상관없이 시작하는 데 도움을 주며, 추론을 위해 [`DiffusionPipeline`] 사용하는 방법을 보여줍니다.
시작하기에 앞서서, 필요한 모든 라이브러리가 설치되어 있는지 확인하세요:
```bash
pip install --upgrade diffusers accelerate transformers
```
- [`accelerate`](https://huggingface.co/docs/accelerate/index)은 추론 및 학습을 위한 모델 불러오기 속도를 높입니다.
- [`transformers`](https://huggingface.co/docs/transformers/index)는 [Stable Diffusion](https://huggingface.co/docs/diffusers/api/pipelines/stable_diffusion/overview)과 같이 가장 널리 사용되는 확산 모델을 실행하기 위해 필요합니다.
## DiffusionPipeline
[`DiffusionPipeline`]은 추론을 위해 사전학습된 확산 시스템을 사용하는 가장 쉬운 방법입니다. 다양한 양식의 많은 작업에 [`DiffusionPipeline`]을 바로 사용할 수 있습니다. 지원되는 작업은 아래의 표를 참고하세요:
| **Task** | **Description** | **Pipeline**
|------------------------------|--------------------------------------------------------------------------------------------------------------|-----------------|
| Unconditional Image Generation | 가우시안 노이즈에서 이미지 생성 | [unconditional_image_generation](./using-diffusers/unconditional_image_generation`) |
| Text-Guided Image Generation | 텍스트 프롬프트로 이미지 생성 | [conditional_image_generation](./using-diffusers/conditional_image_generation) |
| Text-Guided Image-to-Image Translation | 텍스트 프롬프트에 따라 이미지 조정 | [img2img](./using-diffusers/img2img) |
| Text-Guided Image-Inpainting | 마스크 및 텍스트 프롬프트가 주어진 이미지의 마스킹된 부분을 채우기 | [inpaint](./using-diffusers/inpaint) |
| Text-Guided Depth-to-Image Translation | 깊이 추정을 통해 구조를 유지하면서 텍스트 프롬프트에 따라 이미지의 일부를 조정 | [depth2image](./using-diffusers/depth2image) |
확산 파이프라인이 다양한 작업에 대해 어떻게 작동하는지는 [**Using Diffusers**](./using-diffusers/overview)를 참고하세요.
예를들어, [`DiffusionPipeline`] 인스턴스를 생성하여 시작하고, 다운로드하려는 파이프라인 체크포인트를 지정합니다.
모든 [Diffusers' checkpoint](https://huggingface.co/models?library=diffusers&sort=downloads)에 대해 [`DiffusionPipeline`]을 사용할 수 있습니다.
하지만, 이 가이드에서는 [Stable Diffusion](https://huggingface.co/CompVis/stable-diffusion)을 사용하여 text-to-image를 하는데 [`DiffusionPipeline`]을 사용합니다.
[Stable Diffusion](https://huggingface.co/CompVis/stable-diffusion) 기반 모델을 실행하기 전에 [license](https://huggingface.co/spaces/CompVis/stable-diffusion-license)를 주의 깊게 읽으세요.
이는 모델의 향상된 이미지 생성 기능과 이것으로 생성될 수 있는 유해한 콘텐츠 때문입니다. 선택한 Stable Diffusion 모델(*예*: [`runwayml/stable-diffusion-v1-5`](https://huggingface.co/runwayml/stable-diffusion-v1-5))로 이동하여 라이센스를 읽으세요.
다음과 같이 모델을 로드할 수 있습니다:
```python
>>> from diffusers import DiffusionPipeline
>>> pipeline = DiffusionPipeline.from_pretrained("runwayml/stable-diffusion-v1-5")
```
[`DiffusionPipeline`]은 모든 모델링, 토큰화 및 스케줄링 구성요소를 다운로드하고 캐시합니다.
모델은 약 14억개의 매개변수로 구성되어 있으므로 GPU에서 실행하는 것이 좋습니다.
PyTorch에서와 마찬가지로 생성기 객체를 GPU로 옮길 수 있습니다.
```python
>>> pipeline.to("cuda")
```
이제 `pipeline`을 사용할 수 있습니다:
```python
>>> image = pipeline("An image of a squirrel in Picasso style").images[0]
```
출력은 기본적으로 [PIL Image object](https://pillow.readthedocs.io/en/stable/reference/Image.html?highlight=image#the-image-class)로 래핑됩니다.
다음과 같이 함수를 호출하여 이미지를 저장할 수 있습니다:
```python
>>> image.save("image_of_squirrel_painting.png")
```
**참고**: 다음을 통해 가중치를 다운로드하여 로컬에서 파이프라인을 사용할 수도 있습니다:
```
git lfs install
git clone https://huggingface.co/runwayml/stable-diffusion-v1-5
```
그리고 저장된 가중치를 파이프라인에 불러옵니다.
```python
>>> pipeline = DiffusionPipeline.from_pretrained("./stable-diffusion-v1-5")
```
파이프라인 실행은 동일한 모델 아키텍처이므로 위의 코드와 동일합니다.
```python
>>> generator.to("cuda")
>>> image = generator("An image of a squirrel in Picasso style").images[0]
>>> image.save("image_of_squirrel_painting.png")
```
확산 시스템은 각각 장점이 있는 여러 다른 [schedulers](./api/schedulers/overview)와 함께 사용할 수 있습니다. 기본적으로 Stable Diffusion은 `PNDMScheduler`로 실행되지만 다른 스케줄러를 사용하는 방법은 매우 간단합니다. *예* [`EulerDiscreteScheduler`] 스케줄러를 사용하려는 경우, 다음과 같이 사용할 수 있습니다:
```python
>>> from diffusers import EulerDiscreteScheduler
>>> pipeline = StableDiffusionPipeline.from_pretrained("runwayml/stable-diffusion-v1-5")
>>> # change scheduler to Euler
>>> pipeline.scheduler = EulerDiscreteScheduler.from_config(pipeline.scheduler.config)
```
스케줄러 변경 방법에 대한 자세한 내용은 [Using Schedulers](./using-diffusers/schedulers) 가이드를 참고하세요.
[Stability AI's](https://stability.ai/)의 Stable Diffusion 모델은 인상적인 이미지 생성 모델이며 텍스트에서 이미지를 생성하는 것보다 훨씬 더 많은 작업을 수행할 수 있습니다. 우리는 Stable Diffusion만을 위한 전체 문서 페이지를 제공합니다 [link](./conceptual/stable_diffusion).
만약 더 적은 메모리, 더 높은 추론 속도, Mac과 같은 특정 하드웨어 또는 ONNX 런타임에서 실행되도록 Stable Diffusion을 최적화하는 방법을 알고 싶다면 최적화 페이지를 살펴보세요:
- [Optimized PyTorch on GPU](./optimization/fp16)
- [Mac OS with PyTorch](./optimization/mps)
- [ONNX](./optimization/onnx)
- [OpenVINO](./optimization/open_vino)
확산 모델을 미세조정하거나 학습시키려면, [**training section**](./training/overview)을 살펴보세요.
마지막으로, 생성된 이미지를 공개적으로 배포할 때 신중을 기해 주세요 🤗. | 0 |
hf_public_repos/diffusers/docs/source | hf_public_repos/diffusers/docs/source/ko/index.md | <!--Copyright 2023 The HuggingFace Team. All rights reserved.
Licensed under the Apache License, Version 2.0 (the "License"); you may not use this file except in compliance with
the License. You may obtain a copy of the License at
http://www.apache.org/licenses/LICENSE-2.0
Unless required by applicable law or agreed to in writing, software distributed under the License is distributed on
an "AS IS" BASIS, WITHOUT WARRANTIES OR CONDITIONS OF ANY KIND, either express or implied. See the License for the
specific language governing permissions and limitations under the License.
-->
<p align="center">
<br>
<img src="https://raw.githubusercontent.com/huggingface/diffusers/77aadfee6a891ab9fcfb780f87c693f7a5beeb8e/docs/source/imgs/diffusers_library.jpg" width="400"/>
<br>
</p>
# 🧨 Diffusers
🤗 Diffusers는 사전학습된 비전 및 오디오 확산 모델을 제공하고, 추론 및 학습을 위한 모듈식 도구 상자 역할을 합니다.
보다 정확하게, 🤗 Diffusers는 다음을 제공합니다:
- 단 몇 줄의 코드로 추론을 실행할 수 있는 최신 확산 파이프라인을 제공합니다. ([**Using Diffusers**](./using-diffusers/conditional_image_generation)를 살펴보세요) 지원되는 모든 파이프라인과 해당 논문에 대한 개요를 보려면 [**Pipelines**](#pipelines)을 살펴보세요.
- 추론에서 속도 vs 품질의 절충을 위해 상호교환적으로 사용할 수 있는 다양한 노이즈 스케줄러를 제공합니다. 자세한 내용은 [**Schedulers**](./api/schedulers/overview)를 참고하세요.
- UNet과 같은 여러 유형의 모델을 end-to-end 확산 시스템의 구성 요소로 사용할 수 있습니다. 자세한 내용은 [**Models**](./api/models)을 참고하세요.
- 가장 인기있는 확산 모델 테스크를 학습하는 방법을 보여주는 예제들을 제공합니다. 자세한 내용은 [**Training**](./training/overview)를 참고하세요.
## 🧨 Diffusers 파이프라인
다음 표에는 공시적으로 지원되는 모든 파이프라인, 관련 논문, 직접 사용해 볼 수 있는 Colab 노트북(사용 가능한 경우)이 요약되어 있습니다.
| Pipeline | Paper | Tasks | Colab
|---|---|:---:|:---:|
| [alt_diffusion](./api/pipelines/alt_diffusion) | [**AltDiffusion**](https://arxiv.org/abs/2211.06679) | Image-to-Image Text-Guided Generation |
| [audio_diffusion](./api/pipelines/audio_diffusion) | [**Audio Diffusion**](https://github.com/teticio/audio-diffusion.git) | Unconditional Audio Generation | [](https://colab.research.google.com/github/teticio/audio-diffusion/blob/master/notebooks/audio_diffusion_pipeline.ipynb)
| [cycle_diffusion](./api/pipelines/cycle_diffusion) | [**Cycle Diffusion**](https://arxiv.org/abs/2210.05559) | Image-to-Image Text-Guided Generation |
| [dance_diffusion](./api/pipelines/dance_diffusion) | [**Dance Diffusion**](https://github.com/williamberman/diffusers.git) | Unconditional Audio Generation |
| [ddpm](./api/pipelines/ddpm) | [**Denoising Diffusion Probabilistic Models**](https://arxiv.org/abs/2006.11239) | Unconditional Image Generation |
| [ddim](./api/pipelines/ddim) | [**Denoising Diffusion Implicit Models**](https://arxiv.org/abs/2010.02502) | Unconditional Image Generation |
| [latent_diffusion](./api/pipelines/latent_diffusion) | [**High-Resolution Image Synthesis with Latent Diffusion Models**](https://arxiv.org/abs/2112.10752)| Text-to-Image Generation |
| [latent_diffusion](./api/pipelines/latent_diffusion) | [**High-Resolution Image Synthesis with Latent Diffusion Models**](https://arxiv.org/abs/2112.10752)| Super Resolution Image-to-Image |
| [latent_diffusion_uncond](./api/pipelines/latent_diffusion_uncond) | [**High-Resolution Image Synthesis with Latent Diffusion Models**](https://arxiv.org/abs/2112.10752) | Unconditional Image Generation |
| [paint_by_example](./api/pipelines/paint_by_example) | [**Paint by Example: Exemplar-based Image Editing with Diffusion Models**](https://arxiv.org/abs/2211.13227) | Image-Guided Image Inpainting |
| [pndm](./api/pipelines/pndm) | [**Pseudo Numerical Methods for Diffusion Models on Manifolds**](https://arxiv.org/abs/2202.09778) | Unconditional Image Generation |
| [score_sde_ve](./api/pipelines/score_sde_ve) | [**Score-Based Generative Modeling through Stochastic Differential Equations**](https://openreview.net/forum?id=PxTIG12RRHS) | Unconditional Image Generation |
| [score_sde_vp](./api/pipelines/score_sde_vp) | [**Score-Based Generative Modeling through Stochastic Differential Equations**](https://openreview.net/forum?id=PxTIG12RRHS) | Unconditional Image Generation |
| [stable_diffusion](./api/pipelines/stable_diffusion/text2img) | [**Stable Diffusion**](https://stability.ai/blog/stable-diffusion-public-release) | Text-to-Image Generation | [](https://colab.research.google.com/github/huggingface/notebooks/blob/main/diffusers/training_example.ipynb)
| [stable_diffusion](./api/pipelines/stable_diffusion/img2img) | [**Stable Diffusion**](https://stability.ai/blog/stable-diffusion-public-release) | Image-to-Image Text-Guided Generation | [](https://colab.research.google.com/github/huggingface/notebooks/blob/main/diffusers/image_2_image_using_diffusers.ipynb)
| [stable_diffusion](./api/pipelines/stable_diffusion/inpaint) | [**Stable Diffusion**](https://stability.ai/blog/stable-diffusion-public-release) | Text-Guided Image Inpainting | [](https://colab.research.google.com/github/huggingface/notebooks/blob/main/diffusers/in_painting_with_stable_diffusion_using_diffusers.ipynb)
| [stable_diffusion_2](./api/pipelines/stable_diffusion_2) | [**Stable Diffusion 2**](https://stability.ai/blog/stable-diffusion-v2-release) | Text-to-Image Generation |
| [stable_diffusion_2](./api/pipelines/stable_diffusion_2) | [**Stable Diffusion 2**](https://stability.ai/blog/stable-diffusion-v2-release) | Text-Guided Image Inpainting |
| [stable_diffusion_2](./api/pipelines/stable_diffusion_2) | [**Stable Diffusion 2**](https://stability.ai/blog/stable-diffusion-v2-release) | Text-Guided Super Resolution Image-to-Image |
| [stable_diffusion_safe](./api/pipelines/stable_diffusion_safe) | [**Safe Stable Diffusion**](https://arxiv.org/abs/2211.05105) | Text-Guided Generation | [](https://colab.research.google.com/github/ml-research/safe-latent-diffusion/blob/main/examples/Safe%20Latent%20Diffusion.ipynb)
| [stochastic_karras_ve](./api/pipelines/stochastic_karras_ve) | [**Elucidating the Design Space of Diffusion-Based Generative Models**](https://arxiv.org/abs/2206.00364) | Unconditional Image Generation |
| [unclip](./api/pipelines/unclip) | [Hierarchical Text-Conditional Image Generation with CLIP Latents](https://arxiv.org/abs/2204.06125) | Text-to-Image Generation |
| [versatile_diffusion](./api/pipelines/versatile_diffusion) | [Versatile Diffusion: Text, Images and Variations All in One Diffusion Model](https://arxiv.org/abs/2211.08332) | Text-to-Image Generation |
| [versatile_diffusion](./api/pipelines/versatile_diffusion) | [Versatile Diffusion: Text, Images and Variations All in One Diffusion Model](https://arxiv.org/abs/2211.08332) | Image Variations Generation |
| [versatile_diffusion](./api/pipelines/versatile_diffusion) | [Versatile Diffusion: Text, Images and Variations All in One Diffusion Model](https://arxiv.org/abs/2211.08332) | Dual Image and Text Guided Generation |
| [vq_diffusion](./api/pipelines/vq_diffusion) | [Vector Quantized Diffusion Model for Text-to-Image Synthesis](https://arxiv.org/abs/2111.14822) | Text-to-Image Generation |
**참고**: 파이프라인은 해당 문서에 설명된 대로 확산 시스템을 사용한 방법에 대한 간단한 예입니다.
| 0 |
hf_public_repos/diffusers/docs/source | hf_public_repos/diffusers/docs/source/ko/installation.md | <!--Copyright 2023 The HuggingFace Team. All rights reserved.
Licensed under the Apache License, Version 2.0 (the "License"); you may not use this file except in compliance with
the License. You may obtain a copy of the License at
http://www.apache.org/licenses/LICENSE-2.0
Unless required by applicable law or agreed to in writing, software distributed under the License is distributed on
an "AS IS" BASIS, WITHOUT WARRANTIES OR CONDITIONS OF ANY KIND, either express or implied. See the License for the
specific language governing permissions and limitations under the License.
-->
# 설치
사용하시는 라이브러리에 맞는 🤗 Diffusers를 설치하세요.
🤗 Diffusers는 Python 3.7+, PyTorch 1.7.0+ 및 flax에서 테스트되었습니다. 사용중인 딥러닝 라이브러리에 대한 아래의 설치 안내를 따르세요.
- [PyTorch 설치 안내](https://pytorch.org/get-started/locally/)
- [Flax 설치 안내](https://flax.readthedocs.io/en/latest/)
## pip를 이용한 설치
[가상 환경](https://docs.python.org/3/library/venv.html)에 🤗 Diffusers를 설치해야 합니다.
Python 가상 환경에 익숙하지 않은 경우 [가상환경 pip 설치 가이드](https://packaging.python.org/guides/installing-using-pip-and-virtual-environments/)를 살펴보세요.
가상 환경을 사용하면 서로 다른 프로젝트를 더 쉽게 관리하고, 종속성간의 호환성 문제를 피할 수 있습니다.
프로젝트 디렉토리에 가상 환경을 생성하는 것으로 시작하세요:
```bash
python -m venv .env
```
그리고 가상 환경을 활성화합니다:
```bash
source .env/bin/activate
```
이제 다음의 명령어로 🤗 Diffusers를 설치할 준비가 되었습니다:
**PyTorch의 경우**
```bash
pip install diffusers["torch"]
```
**Flax의 경우**
```bash
pip install diffusers["flax"]
```
## 소스로부터 설치
소스에서 `diffusers`를 설치하기 전에, `torch` 및 `accelerate`이 설치되어 있는지 확인하세요.
`torch` 설치에 대해서는 [torch docs](https://pytorch.org/get-started/locally/#start-locally)를 참고하세요.
다음과 같이 `accelerate`을 설치하세요.
```bash
pip install accelerate
```
다음 명령어를 사용하여 소스에서 🤗 Diffusers를 설치하세요:
```bash
pip install git+https://github.com/huggingface/diffusers
```
이 명령어는 최신 `stable` 버전이 아닌 최첨단 `main` 버전을 설치합니다.
`main` 버전은 최신 개발 정보를 최신 상태로 유지하는 데 유용합니다.
예를 들어 마지막 공식 릴리즈 이후 버그가 수정되었지만, 새 릴리즈가 아직 출시되지 않은 경우입니다.
그러나 이는 `main` 버전이 항상 안정적이지 않을 수 있음을 의미합니다.
우리는 `main` 버전이 지속적으로 작동하도록 노력하고 있으며, 대부분의 문제는 보통 몇 시간 또는 하루 안에 해결됩니다.
문제가 발생하면 더 빨리 해결할 수 있도록 [Issue](https://github.com/huggingface/transformers/issues)를 열어주세요!
## 편집가능한 설치
다음을 수행하려면 편집가능한 설치가 필요합니다:
* 소스 코드의 `main` 버전을 사용
* 🤗 Diffusers에 기여 (코드의 변경 사항을 테스트하기 위해 필요)
저장소를 복제하고 다음 명령어를 사용하여 🤗 Diffusers를 설치합니다:
```bash
git clone https://github.com/huggingface/diffusers.git
cd diffusers
```
**PyTorch의 경우**
```
pip install -e ".[torch]"
```
**Flax의 경우**
```
pip install -e ".[flax]"
```
이러한 명령어들은 저장소를 복제한 폴더와 Python 라이브러리 경로를 연결합니다.
Python은 이제 일반 라이브러리 경로에 더하여 복제한 폴더 내부를 살펴봅니다.
예를들어 Python 패키지가 `~/anaconda3/envs/main/lib/python3.7/site-packages/`에 설치되어 있는 경우 Python은 복제한 폴더인 `~/diffusers/`도 검색합니다.
<Tip warning={true}>
라이브러리를 계속 사용하려면 `diffusers` 폴더를 유지해야 합니다.
</Tip>
이제 다음 명령어를 사용하여 최신 버전의 🤗 Diffusers로 쉽게 업데이트할 수 있습니다:
```bash
cd ~/diffusers/
git pull
```
이렇게 하면, 다음에 실행할 때 Python 환경이 🤗 Diffusers의 `main` 버전을 찾게 됩니다.
## 텔레메트리 로깅에 대한 알림
우리 라이브러리는 `from_pretrained()` 요청 중에 텔레메트리 정보를 원격으로 수집합니다.
이 데이터에는 Diffusers 및 PyTorch/Flax의 버전, 요청된 모델 또는 파이프라인 클래스, 그리고 허브에서 호스팅되는 경우 사전학습된 체크포인트에 대한 경로를 포함합니다.
이 사용 데이터는 문제를 디버깅하고 새로운 기능의 우선순위를 지정하는데 도움이 됩니다.
텔레메트리는 HuggingFace 허브에서 모델과 파이프라인을 불러올 때만 전송되며, 로컬 사용 중에는 수집되지 않습니다.
우리는 추가 정보를 공유하지 않기를 원하는 사람이 있다는 것을 이해하고 개인 정보를 존중하므로, 터미널에서 `DISABLE_TELEMETRY` 환경 변수를 설정하여 텔레메트리 수집을 비활성화할 수 있습니다.
Linux/MacOS에서:
```bash
export DISABLE_TELEMETRY=YES
```
Windows에서:
```bash
set DISABLE_TELEMETRY=YES
``` | 0 |
hf_public_repos/diffusers/docs/source | hf_public_repos/diffusers/docs/source/ko/_toctree.yml | - sections:
- local: index
title: "🧨 Diffusers"
- local: quicktour
title: "훑어보기"
- local: in_translation
title: Stable Diffusion
- local: installation
title: "설치"
title: "시작하기"
- sections:
- local: tutorials/tutorial_overview
title: 개요
- local: using-diffusers/write_own_pipeline
title: 모델과 스케줄러 이해하기
- local: tutorials/basic_training
title: Diffusion 모델 학습하기
title: Tutorials
- sections:
- sections:
- local: in_translation
title: 개요
- local: using-diffusers/loading
title: 파이프라인, 모델, 스케줄러 불러오기
- local: using-diffusers/schedulers
title: 다른 스케줄러들을 가져오고 비교하기
- local: using-diffusers/custom_pipeline_overview
title: 커뮤니티 파이프라인 불러오기
- local: using-diffusers/using_safetensors
title: 세이프텐서 불러오기
- local: using-diffusers/other-formats
title: 다른 형식의 Stable Diffusion 불러오기
title: 불러오기 & 허브
- sections:
- local: using-diffusers/pipeline_overview
title: 개요
- local: using-diffusers/unconditional_image_generation
title: Unconditional 이미지 생성
- local: in_translation
title: Text-to-image 생성
- local: using-diffusers/img2img
title: Text-guided image-to-image
- local: using-diffusers/inpaint
title: Text-guided 이미지 인페인팅
- local: using-diffusers/depth2img
title: Text-guided depth-to-image
- local: in_translation
title: Textual inversion
- local: in_translation
title: 여러 GPU를 사용한 분산 추론
- local: using-diffusers/reusing_seeds
title: Deterministic 생성으로 이미지 퀄리티 높이기
- local: in_translation
title: 재현 가능한 파이프라인 생성하기
- local: using-diffusers/custom_pipeline_examples
title: 커뮤니티 파이프라인들
- local: in_translation
title: 커뮤티니 파이프라인에 기여하는 방법
- local: in_translation
title: JAX/Flax에서의 Stable Diffusion
- local: in_translation
title: Weighting Prompts
title: 추론을 위한 파이프라인
- sections:
- local: training/overview
title: 개요
- local: in_translation
title: 학습을 위한 데이터셋 생성하기
- local: training/adapt_a_model
title: 새로운 태스크에 모델 적용하기
- local: training/unconditional_training
title: Unconditional 이미지 생성
- local: training/text_inversion
title: Textual Inversion
- local: training/dreambooth
title: DreamBooth
- local: training/text2image
title: Text-to-image
- local: training/lora
title: Low-Rank Adaptation of Large Language Models (LoRA)
- local: in_translation
title: ControlNet
- local: in_translation
title: InstructPix2Pix 학습
- local: in_translation
title: Custom Diffusion
title: Training
title: Diffusers 사용하기
- sections:
- local: optimization/opt_overview
title: 개요
- local: optimization/fp16
title: 메모리와 속도
- local: optimization/torch2.0
title: Torch2.0 지원
- local: optimization/xformers
title: xFormers
- local: optimization/onnx
title: ONNX
- local: optimization/open_vino
title: OpenVINO
- local: in_translation
title: Core ML
- local: optimization/mps
title: MPS
- local: optimization/habana
title: Habana Gaudi
- local: in_translation
title: Token Merging
title: 최적화/특수 하드웨어
| 0 |
hf_public_repos/diffusers/docs/source | hf_public_repos/diffusers/docs/source/ko/in_translation.md | <!--Copyright 2023 The HuggingFace Team. All rights reserved.
Licensed under the Apache License, Version 2.0 (the "License"); you may not use this file except in compliance with
the License. You may obtain a copy of the License at
http://www.apache.org/licenses/LICENSE-2.0
Unless required by applicable law or agreed to in writing, software distributed under the License is distributed on
an "AS IS" BASIS, WITHOUT WARRANTIES OR CONDITIONS OF ANY KIND, either express or implied. See the License for the
specific language governing permissions and limitations under the License.
-->
# 번역중
열심히 번역을 진행중입니다. 조금만 기다려주세요.
감사합니다! | 0 |
hf_public_repos/diffusers/docs/source/ko | hf_public_repos/diffusers/docs/source/ko/training/unconditional_training.md | <!--Copyright 2023 The HuggingFace Team. All rights reserved.
Licensed under the Apache License, Version 2.0 (the "License"); you may not use this file except in compliance with
the License. You may obtain a copy of the License at
http://www.apache.org/licenses/LICENSE-2.0
Unless required by applicable law or agreed to in writing, software distributed under the License is distributed on
an "AS IS" BASIS, WITHOUT WARRANTIES OR CONDITIONS OF ANY KIND, either express or implied. See the License for the
specific language governing permissions and limitations under the License.
-->
# Unconditional 이미지 생성
unconditional 이미지 생성은 text-to-image 또는 image-to-image 모델과 달리 텍스트나 이미지에 대한 조건이 없이 학습 데이터 분포와 유사한 이미지만을 생성합니다.
<iframe
src="https://stevhliu-ddpm-butterflies-128.hf.space"
frameborder="0"
width="850"
height="550"
></iframe>
이 가이드에서는 기존에 존재하던 데이터셋과 자신만의 커스텀 데이터셋에 대해 unconditional image generation 모델을 훈련하는 방법을 설명합니다. 훈련 세부 사항에 대해 더 자세히 알고 싶다면 unconditional image generation을 위한 모든 학습 스크립트를 [여기](https://github.com/huggingface/diffusers/tree/main/examples/unconditional_image_generation)에서 확인할 수 있습니다.
스크립트를 실행하기 전, 먼저 의존성 라이브러리들을 설치해야 합니다.
```bash
pip install diffusers[training] accelerate datasets
```
그 다음 🤗 [Accelerate](https://github.com/huggingface/accelerate/) 환경을 초기화합니다.
```bash
accelerate config
```
별도의 설정 없이 기본 설정으로 🤗 [Accelerate](https://github.com/huggingface/accelerate/) 환경을 초기화해봅시다.
```bash
accelerate config default
```
노트북과 같은 대화형 쉘을 지원하지 않는 환경의 경우, 다음과 같이 사용해볼 수도 있습니다.
```py
from accelerate.utils import write_basic_config
write_basic_config()
```
## 모델을 허브에 업로드하기
학습 스크립트에 다음 인자를 추가하여 허브에 모델을 업로드할 수 있습니다.
```bash
--push_to_hub
```
## 체크포인트 저장하고 불러오기
훈련 중 문제가 발생할 경우를 대비하여 체크포인트를 정기적으로 저장하는 것이 좋습니다. 체크포인트를 저장하려면 학습 스크립트에 다음 인자를 전달합니다:
```bash
--checkpointing_steps=500
```
전체 훈련 상태는 500스텝마다 `output_dir`의 하위 폴더에 저장되며, 학습 스크립트에 `--resume_from_checkpoint` 인자를 전달함으로써 체크포인트를 불러오고 훈련을 재개할 수 있습니다.
```bash
--resume_from_checkpoint="checkpoint-1500"
```
## 파인튜닝
이제 학습 스크립트를 시작할 준비가 되었습니다! `--dataset_name` 인자에 파인튜닝할 데이터셋 이름을 지정한 다음, `--output_dir` 인자에 지정된 경로로 저장합니다. 본인만의 데이터셋를 사용하려면, [학습용 데이터셋 만들기](create_dataset) 가이드를 참조하세요.
학습 스크립트는 `diffusion_pytorch_model.bin` 파일을 생성하고, 그것을 당신의 리포지토리에 저장합니다.
<Tip>
💡 전체 학습은 V100 GPU 4개를 사용할 경우, 2시간이 소요됩니다.
</Tip>
예를 들어, [Oxford Flowers](https://huggingface.co/datasets/huggan/flowers-102-categories) 데이터셋을 사용해 파인튜닝할 경우:
```bash
accelerate launch train_unconditional.py \
--dataset_name="huggan/flowers-102-categories" \
--resolution=64 \
--output_dir="ddpm-ema-flowers-64" \
--train_batch_size=16 \
--num_epochs=100 \
--gradient_accumulation_steps=1 \
--learning_rate=1e-4 \
--lr_warmup_steps=500 \
--mixed_precision=no \
--push_to_hub
```
<div class="flex justify-center">
<img src="https://user-images.githubusercontent.com/26864830/180248660-a0b143d0-b89a-42c5-8656-2ebf6ece7e52.png"/>
</div>
[Pokemon](https://huggingface.co/datasets/huggan/pokemon) 데이터셋을 사용할 경우:
```bash
accelerate launch train_unconditional.py \
--dataset_name="huggan/pokemon" \
--resolution=64 \
--output_dir="ddpm-ema-pokemon-64" \
--train_batch_size=16 \
--num_epochs=100 \
--gradient_accumulation_steps=1 \
--learning_rate=1e-4 \
--lr_warmup_steps=500 \
--mixed_precision=no \
--push_to_hub
```
<div class="flex justify-center">
<img src="https://user-images.githubusercontent.com/26864830/180248200-928953b4-db38-48db-b0c6-8b740fe6786f.png"/>
</div>
### 여러개의 GPU로 훈련하기
`accelerate`을 사용하면 원활한 다중 GPU 훈련이 가능합니다. `accelerate`을 사용하여 분산 훈련을 실행하려면 [여기](https://huggingface.co/docs/accelerate/basic_tutorials/launch) 지침을 따르세요. 다음은 명령어 예제입니다.
```bash
accelerate launch --mixed_precision="fp16" --multi_gpu train_unconditional.py \
--dataset_name="huggan/pokemon" \
--resolution=64 --center_crop --random_flip \
--output_dir="ddpm-ema-pokemon-64" \
--train_batch_size=16 \
--num_epochs=100 \
--gradient_accumulation_steps=1 \
--use_ema \
--learning_rate=1e-4 \
--lr_warmup_steps=500 \
--mixed_precision="fp16" \
--logger="wandb" \
--push_to_hub
```
| 0 |
hf_public_repos/diffusers/docs/source/ko | hf_public_repos/diffusers/docs/source/ko/training/text_inversion.md | <!--Copyright 2023 The HuggingFace Team. All rights reserved.
Licensed under the Apache License, Version 2.0 (the "License"); you may not use this file except in compliance with
the License. You may obtain a copy of the License at
http://www.apache.org/licenses/LICENSE-2.0
Unless required by applicable law or agreed to in writing, software distributed under the License is distributed on
an "AS IS" BASIS, WITHOUT WARRANTIES OR CONDITIONS OF ANY KIND, either express or implied. See the License for the
specific language governing permissions and limitations under the License.
-->
# Textual-Inversion
[[open-in-colab]]
[textual-inversion](https://arxiv.org/abs/2208.01618)은 소수의 예시 이미지에서 새로운 콘셉트를 포착하는 기법입니다. 이 기술은 원래 [Latent Diffusion](https://github.com/CompVis/latent-diffusion)에서 시연되었지만, 이후 [Stable Diffusion](https://huggingface.co/docs/diffusers/main/en/conceptual/stable_diffusion)과 같은 유사한 다른 모델에도 적용되었습니다. 학습된 콘셉트는 text-to-image 파이프라인에서 생성된 이미지를 더 잘 제어하는 데 사용할 수 있습니다. 이 모델은 텍스트 인코더의 임베딩 공간에서 새로운 '단어'를 학습하여 개인화된 이미지 생성을 위한 텍스트 프롬프트 내에서 사용됩니다.
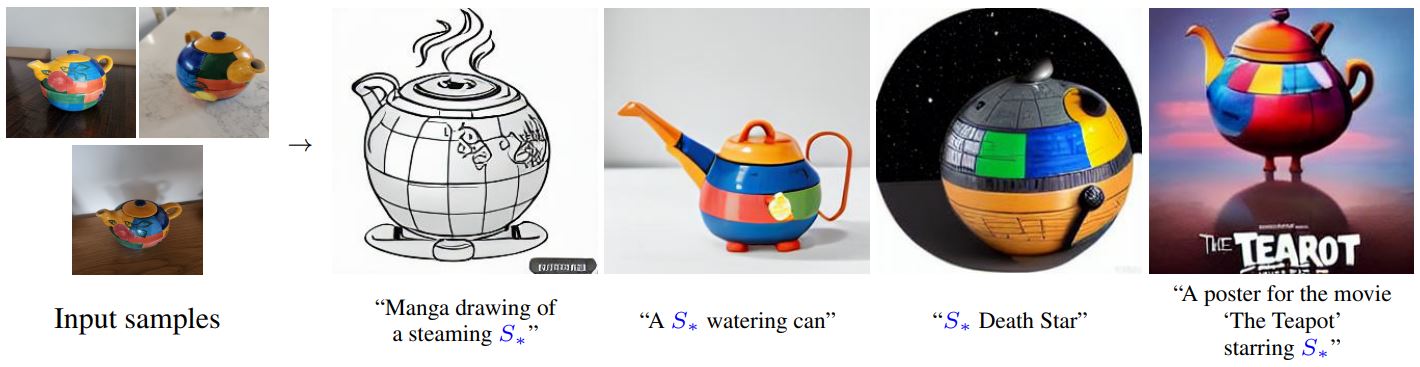
<small>By using just 3-5 images you can teach new concepts to a model such as Stable Diffusion for personalized image generation <a href="https://github.com/rinongal/textual_inversion">(image source)</a>.</small>
이 가이드에서는 textual-inversion으로 [`runwayml/stable-diffusion-v1-5`](https://huggingface.co/runwayml/stable-diffusion-v1-5) 모델을 학습하는 방법을 설명합니다. 이 가이드에서 사용된 모든 textual-inversion 학습 스크립트는 [여기](https://github.com/huggingface/diffusers/tree/main/examples/textual_inversion)에서 확인할 수 있습니다. 내부적으로 어떻게 작동하는지 자세히 살펴보고 싶으시다면 해당 링크를 참조해주시기 바랍니다.
<Tip>
[Stable Diffusion Textual Inversion Concepts Library](https://huggingface.co/sd-concepts-library)에는 커뮤니티에서 제작한 학습된 textual-inversion 모델들이 있습니다. 시간이 지남에 따라 더 많은 콘셉트들이 추가되어 유용한 리소스로 성장할 것입니다!
</Tip>
시작하기 전에 학습을 위한 의존성 라이브러리들을 설치해야 합니다:
```bash
pip install diffusers accelerate transformers
```
의존성 라이브러리들의 설치가 완료되면, [🤗Accelerate](https://github.com/huggingface/accelerate/) 환경을 초기화시킵니다.
```bash
accelerate config
```
별도의 설정없이, 기본 🤗Accelerate 환경을 설정하려면 다음과 같이 하세요:
```bash
accelerate config default
```
또는 사용 중인 환경이 노트북과 같은 대화형 셸을 지원하지 않는다면, 다음과 같이 사용할 수 있습니다:
```py
from accelerate.utils import write_basic_config
write_basic_config()
```
마지막으로, Memory-Efficient Attention을 통해 메모리 사용량을 줄이기 위해 [xFormers](https://huggingface.co/docs/diffusers/main/en/training/optimization/xformers)를 설치합니다. xFormers를 설치한 후, 학습 스크립트에 `--enable_xformers_memory_efficient_attention` 인자를 추가합니다. xFormers는 Flax에서 지원되지 않습니다.
## 허브에 모델 업로드하기
모델을 허브에 저장하려면, 학습 스크립트에 다음 인자를 추가해야 합니다.
```bash
--push_to_hub
```
## 체크포인트 저장 및 불러오기
학습중에 모델의 체크포인트를 정기적으로 저장하는 것이 좋습니다. 이렇게 하면 어떤 이유로든 학습이 중단된 경우 저장된 체크포인트에서 학습을 다시 시작할 수 있습니다. 학습 스크립트에 다음 인자를 전달하면 500단계마다 전체 학습 상태가 `output_dir`의 하위 폴더에 체크포인트로서 저장됩니다.
```bash
--checkpointing_steps=500
```
저장된 체크포인트에서 학습을 재개하려면, 학습 스크립트와 재개할 특정 체크포인트에 다음 인자를 전달하세요.
```bash
--resume_from_checkpoint="checkpoint-1500"
```
## 파인 튜닝
학습용 데이터셋으로 [고양이 장난감 데이터셋](https://huggingface.co/datasets/diffusers/cat_toy_example)을 다운로드하여 디렉토리에 저장하세요. 여러분만의 고유한 데이터셋을 사용하고자 한다면, [학습용 데이터셋 만들기](https://huggingface.co/docs/diffusers/training/create_dataset) 가이드를 살펴보시기 바랍니다.
```py
from huggingface_hub import snapshot_download
local_dir = "./cat"
snapshot_download(
"diffusers/cat_toy_example", local_dir=local_dir, repo_type="dataset", ignore_patterns=".gitattributes"
)
```
모델의 리포지토리 ID(또는 모델 가중치가 포함된 디렉터리 경로)를 `MODEL_NAME` 환경 변수에 할당하고, 해당 값을 [`pretrained_model_name_or_path`](https://huggingface.co/docs/diffusers/en/api/diffusion_pipeline#diffusers.DiffusionPipeline.from_pretrained.pretrained_model_name_or_path) 인자에 전달합니다. 그리고 이미지가 포함된 디렉터리 경로를 `DATA_DIR` 환경 변수에 할당합니다.
이제 [학습 스크립트](https://github.com/huggingface/diffusers/blob/main/examples/textual_inversion/textual_inversion.py)를 실행할 수 있습니다. 스크립트는 다음 파일을 생성하고 리포지토리에 저장합니다.
- `learned_embeds.bin`
- `token_identifier.txt`
- `type_of_concept.txt`.
<Tip>
💡V100 GPU 1개를 기준으로 전체 학습에는 최대 1시간이 걸립니다. 학습이 완료되기를 기다리는 동안 궁금한 점이 있으면 아래 섹션에서 [textual-inversion이 어떻게 작동하는지](https://huggingface.co/docs/diffusers/training/text_inversion#how-it-works) 자유롭게 확인하세요 !
</Tip>
<frameworkcontent>
<pt>
```bash
export MODEL_NAME="runwayml/stable-diffusion-v1-5"
export DATA_DIR="./cat"
accelerate launch textual_inversion.py \
--pretrained_model_name_or_path=$MODEL_NAME \
--train_data_dir=$DATA_DIR \
--learnable_property="object" \
--placeholder_token="<cat-toy>" --initializer_token="toy" \
--resolution=512 \
--train_batch_size=1 \
--gradient_accumulation_steps=4 \
--max_train_steps=3000 \
--learning_rate=5.0e-04 --scale_lr \
--lr_scheduler="constant" \
--lr_warmup_steps=0 \
--output_dir="textual_inversion_cat" \
--push_to_hub
```
<Tip>
💡학습 성능을 올리기 위해, 플레이스홀더 토큰(`<cat-toy>`)을 (단일한 임베딩 벡터가 아닌) 복수의 임베딩 벡터로 표현하는 것 역시 고려할 있습니다. 이러한 트릭이 모델이 보다 복잡한 이미지의 스타일(앞서 말한 콘셉트)을 더 잘 캡처하는 데 도움이 될 수 있습니다. 복수의 임베딩 벡터 학습을 활성화하려면 다음 옵션을 전달하십시오.
```bash
--num_vectors=5
```
</Tip>
</pt>
<jax>
TPU에 액세스할 수 있는 경우, [Flax 학습 스크립트](https://github.com/huggingface/diffusers/blob/main/examples/textual_inversion/textual_inversion_flax.py)를 사용하여 더 빠르게 모델을 학습시켜보세요. (물론 GPU에서도 작동합니다.) 동일한 설정에서 Flax 학습 스크립트는 PyTorch 학습 스크립트보다 최소 70% 더 빨라야 합니다! ⚡️
시작하기 앞서 Flax에 대한 의존성 라이브러리들을 설치해야 합니다.
```bash
pip install -U -r requirements_flax.txt
```
모델의 리포지토리 ID(또는 모델 가중치가 포함된 디렉터리 경로)를 `MODEL_NAME` 환경 변수에 할당하고, 해당 값을 [`pretrained_model_name_or_path`](https://huggingface.co/docs/diffusers/en/api/diffusion_pipeline#diffusers.DiffusionPipeline.from_pretrained.pretrained_model_name_or_path) 인자에 전달합니다.
그런 다음 [학습 스크립트](https://github.com/huggingface/diffusers/blob/main/examples/textual_inversion/textual_inversion_flax.py)를 시작할 수 있습니다.
```bash
export MODEL_NAME="duongna/stable-diffusion-v1-4-flax"
export DATA_DIR="./cat"
python textual_inversion_flax.py \
--pretrained_model_name_or_path=$MODEL_NAME \
--train_data_dir=$DATA_DIR \
--learnable_property="object" \
--placeholder_token="<cat-toy>" --initializer_token="toy" \
--resolution=512 \
--train_batch_size=1 \
--max_train_steps=3000 \
--learning_rate=5.0e-04 --scale_lr \
--output_dir="textual_inversion_cat" \
--push_to_hub
```
</jax>
</frameworkcontent>
### 중간 로깅
모델의 학습 진행 상황을 추적하는 데 관심이 있는 경우, 학습 과정에서 생성된 이미지를 저장할 수 있습니다. 학습 스크립트에 다음 인수를 추가하여 중간 로깅을 활성화합니다.
- `validation_prompt` : 샘플을 생성하는 데 사용되는 프롬프트(기본값은 `None`으로 설정되며, 이 때 중간 로깅은 비활성화됨)
- `num_validation_images` : 생성할 샘플 이미지 수
- `validation_steps` : `validation_prompt`로부터 샘플 이미지를 생성하기 전 스텝의 수
```bash
--validation_prompt="A <cat-toy> backpack"
--num_validation_images=4
--validation_steps=100
```
## 추론
모델을 학습한 후에는, 해당 모델을 [`StableDiffusionPipeline`]을 사용하여 추론에 사용할 수 있습니다.
textual-inversion 스크립트는 기본적으로 textual-inversion을 통해 얻어진 임베딩 벡터만을 저장합니다. 해당 임베딩 벡터들은 텍스트 인코더의 임베딩 행렬에 추가되어 있습습니다.
<frameworkcontent>
<pt>
<Tip>
💡 커뮤니티는 [sd-concepts-library](https://huggingface.co/sd-concepts-library) 라는 대규모의 textual-inversion 임베딩 벡터 라이브러리를 만들었습니다. textual-inversion 임베딩을 밑바닥부터 학습하는 대신, 해당 라이브러리에 본인이 찾는 textual-inversion 임베딩이 이미 추가되어 있지 않은지를 확인하는 것도 좋은 방법이 될 것 같습니다.
</Tip>
textual-inversion 임베딩 벡터을 불러오기 위해서는, 먼저 해당 임베딩 벡터를 학습할 때 사용한 모델을 불러와야 합니다. 여기서는 [`runwayml/stable-diffusion-v1-5`](https://huggingface.co/docs/diffusers/training/runwayml/stable-diffusion-v1-5) 모델이 사용되었다고 가정하고 불러오겠습니다.
```python
from diffusers import StableDiffusionPipeline
import torch
model_id = "runwayml/stable-diffusion-v1-5"
pipe = StableDiffusionPipeline.from_pretrained(model_id, torch_dtype=torch.float16).to("cuda")
```
다음으로 `TextualInversionLoaderMixin.load_textual_inversion` 함수를 통해, textual-inversion 임베딩 벡터를 불러와야 합니다. 여기서 우리는 이전의 `<cat-toy>` 예제의 임베딩을 불러올 것입니다.
```python
pipe.load_textual_inversion("sd-concepts-library/cat-toy")
```
이제 플레이스홀더 토큰(`<cat-toy>`)이 잘 동작하는지를 확인하는 파이프라인을 실행할 수 있습니다.
```python
prompt = "A <cat-toy> backpack"
image = pipe(prompt, num_inference_steps=50).images[0]
image.save("cat-backpack.png")
```
`TextualInversionLoaderMixin.load_textual_inversion`은 Diffusers 형식으로 저장된 텍스트 임베딩 벡터를 로드할 수 있을 뿐만 아니라, [Automatic1111](https://github.com/AUTOMATIC1111/stable-diffusion-webui) 형식으로 저장된 임베딩 벡터도 로드할 수 있습니다. 이렇게 하려면, 먼저 [civitAI](https://civitai.com/models/3036?modelVersionId=8387)에서 임베딩 벡터를 다운로드한 다음 로컬에서 불러와야 합니다.
```python
pipe.load_textual_inversion("./charturnerv2.pt")
```
</pt>
<jax>
현재 Flax에 대한 `load_textual_inversion` 함수는 없습니다. 따라서 학습 후 textual-inversion 임베딩 벡터가 모델의 일부로서 저장되었는지를 확인해야 합니다. 그런 다음은 다른 Flax 모델과 마찬가지로 실행할 수 있습니다.
```python
import jax
import numpy as np
from flax.jax_utils import replicate
from flax.training.common_utils import shard
from diffusers import FlaxStableDiffusionPipeline
model_path = "path-to-your-trained-model"
pipeline, params = FlaxStableDiffusionPipeline.from_pretrained(model_path, dtype=jax.numpy.bfloat16)
prompt = "A <cat-toy> backpack"
prng_seed = jax.random.PRNGKey(0)
num_inference_steps = 50
num_samples = jax.device_count()
prompt = num_samples * [prompt]
prompt_ids = pipeline.prepare_inputs(prompt)
# shard inputs and rng
params = replicate(params)
prng_seed = jax.random.split(prng_seed, jax.device_count())
prompt_ids = shard(prompt_ids)
images = pipeline(prompt_ids, params, prng_seed, num_inference_steps, jit=True).images
images = pipeline.numpy_to_pil(np.asarray(images.reshape((num_samples,) + images.shape[-3:])))
image.save("cat-backpack.png")
```
</jax>
</frameworkcontent>
## 작동 방식
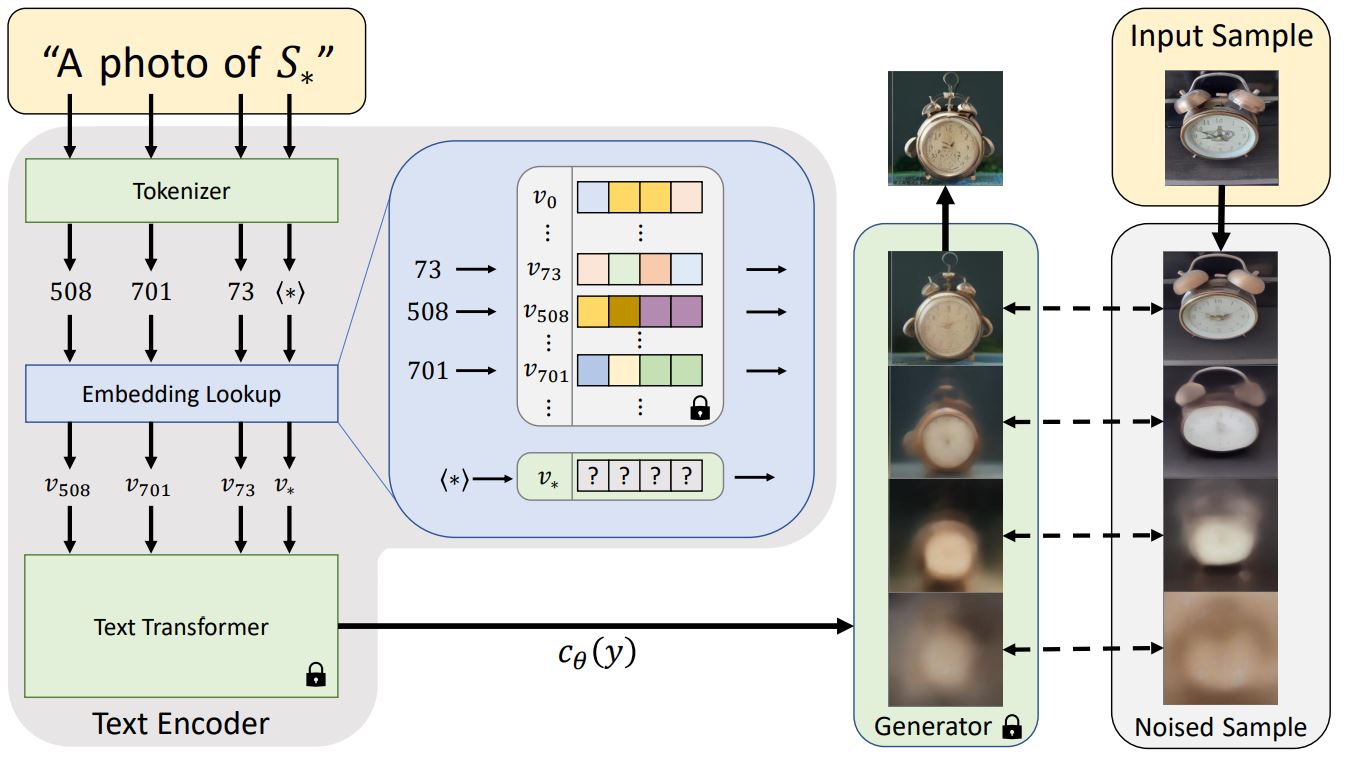
<small>Architecture overview from the Textual Inversion <a href="https://textual-inversion.github.io/">blog post.</a></small>
일반적으로 텍스트 프롬프트는 모델에 전달되기 전에 임베딩으로 토큰화됩니다. textual-inversion은 비슷한 작업을 수행하지만, 위 다이어그램의 특수 토큰 `S*`로부터 새로운 토큰 임베딩 `v*`를 학습합니다. 모델의 아웃풋은 디퓨전 모델을 조정하는 데 사용되며, 디퓨전 모델이 단 몇 개의 예제 이미지에서 신속하고 새로운 콘셉트를 이해하는 데 도움을 줍니다.
이를 위해 textual-inversion은 제너레이터 모델과 학습용 이미지의 노이즈 버전을 사용합니다. 제너레이터는 노이즈가 적은 버전의 이미지를 예측하려고 시도하며 토큰 임베딩 `v*`은 제너레이터의 성능에 따라 최적화됩니다. 토큰 임베딩이 새로운 콘셉트를 성공적으로 포착하면 디퓨전 모델에 더 유용한 정보를 제공하고 노이즈가 적은 더 선명한 이미지를 생성하는 데 도움이 됩니다. 이러한 최적화 프로세스는 일반적으로 다양한 프롬프트와 이미지에 수천 번에 노출됨으로써 이루어집니다.
| 0 |
hf_public_repos/diffusers/docs/source/ko | hf_public_repos/diffusers/docs/source/ko/training/overview.md | <!--Copyright 2023 The HuggingFace Team. All rights reserved.
Licensed under the Apache License, Version 2.0 (the "License"); you may not use this file except in compliance with
the License. You may obtain a copy of the License at
http://www.apache.org/licenses/LICENSE-2.0
Unless required by applicable law or agreed to in writing, software distributed under the License is distributed on
an "AS IS" BASIS, WITHOUT WARRANTIES OR CONDITIONS OF ANY KIND, either express or implied. See the License for the
specific language governing permissions and limitations under the License.
-->
# 🧨 Diffusers 학습 예시
이번 챕터에서는 다양한 유즈케이스들에 대한 예제 코드들을 통해 어떻게하면 효과적으로 `diffusers` 라이브러리를 사용할 수 있을까에 대해 알아보도록 하겠습니다.
**Note**: 혹시 오피셜한 예시코드를 찾고 있다면, [여기](https://github.com/huggingface/diffusers/tree/main/src/diffusers/pipelines)를 참고해보세요!
여기서 다룰 예시들은 다음을 지향합니다.
- **손쉬운 디펜던시 설치** (Self-contained) : 여기서 사용될 예시 코드들의 디펜던시 패키지들은 전부 `pip install` 명령어를 통해 설치 가능한 패키지들입니다. 또한 친절하게 `requirements.txt` 파일에 해당 패키지들이 명시되어 있어, `pip install -r requirements.txt`로 간편하게 해당 디펜던시들을 설치할 수 있습니다. 예시: [train_unconditional.py](https://github.com/huggingface/diffusers/blob/main/examples/unconditional_image_generation/train_unconditional.py), [requirements.txt](https://github.com/huggingface/diffusers/blob/main/examples/unconditional_image_generation/requirements.txt)
- **손쉬운 수정** (Easy-to-tweak) : 저희는 가능하면 많은 유즈 케이스들을 제공하고자 합니다. 하지만 예시는 결국 그저 예시라는 점들 기억해주세요. 여기서 제공되는 예시코드들을 그저 단순히 복사-붙혀넣기하는 식으로는 여러분이 마주한 문제들을 손쉽게 해결할 순 없을 것입니다. 다시 말해 어느 정도는 여러분의 상황과 니즈에 맞춰 코드를 일정 부분 고쳐나가야 할 것입니다. 따라서 대부분의 학습 예시들은 데이터의 전처리 과정과 학습 과정에 대한 코드들을 함께 제공함으로써, 사용자가 니즈에 맞게 손쉬운 수정할 수 있도록 돕고 있습니다.
- **입문자 친화적인** (Beginner-friendly) : 이번 챕터는 diffusion 모델과 `diffusers` 라이브러리에 대한 전반적인 이해를 돕기 위해 작성되었습니다. 따라서 diffusion 모델에 대한 최신 SOTA (state-of-the-art) 방법론들 가운데서도, 입문자에게는 많이 어려울 수 있다고 판단되면, 해당 방법론들은 여기서 다루지 않으려고 합니다.
- **하나의 태스크만 포함할 것**(One-purpose-only): 여기서 다룰 예시들은 하나의 태스크만 포함하고 있어야 합니다. 물론 이미지 초해상화(super-resolution)와 이미지 보정(modification)과 같은 유사한 모델링 프로세스를 갖는 태스크들이 존재하겠지만, 하나의 예제에 하나의 태스크만을 담는 것이 더 이해하기 용이하다고 판단했기 때문입니다.
저희는 diffusion 모델의 대표적인 태스크들을 다루는 공식 예제를 제공하고 있습니다. *공식* 예제는 현재 진행형으로 `diffusers` 관리자들(maintainers)에 의해 관리되고 있습니다. 또한 저희는 앞서 정의한 저희의 철학을 엄격하게 따르고자 노력하고 있습니다. 혹시 여러분께서 이러한 예시가 반드시 필요하다고 생각되신다면, 언제든지 [Feature Request](https://github.com/huggingface/diffusers/issues/new?assignees=&labels=&template=feature_request.md&title=) 혹은 직접 [Pull Request](https://github.com/huggingface/diffusers/compare)를 주시기 바랍니다. 저희는 언제나 환영입니다!
학습 예시들은 다양한 태스크들에 대해 diffusion 모델을 사전학습(pretrain)하거나 파인튜닝(fine-tuning)하는 법을 보여줍니다. 현재 다음과 같은 예제들을 지원하고 있습니다.
- [Unconditional Training](./unconditional_training)
- [Text-to-Image Training](./text2image)
- [Text Inversion](./text_inversion)
- [Dreambooth](./dreambooth)
memory-efficient attention 연산을 수행하기 위해, 가능하면 [xFormers](../optimization/xformers)를 설치해주시기 바랍니다. 이를 통해 학습 속도를 늘리고 메모리에 대한 부담을 줄일 수 있습니다.
| Task | 🤗 Accelerate | 🤗 Datasets | Colab
|---|---|:---:|:---:|
| [**Unconditional Image Generation**](./unconditional_training) | ✅ | ✅ | [](https://colab.research.google.com/github/huggingface/notebooks/blob/main/diffusers/training_example.ipynb)
| [**Text-to-Image fine-tuning**](./text2image) | ✅ | ✅ |
| [**Textual Inversion**](./text_inversion) | ✅ | - | [](https://colab.research.google.com/github/huggingface/notebooks/blob/main/diffusers/sd_textual_inversion_training.ipynb)
| [**Dreambooth**](./dreambooth) | ✅ | - | [](https://colab.research.google.com/github/huggingface/notebooks/blob/main/diffusers/sd_dreambooth_training.ipynb)
| [**Training with LoRA**](./lora) | ✅ | - | - |
| [**ControlNet**](./controlnet) | ✅ | ✅ | - |
| [**InstructPix2Pix**](./instructpix2pix) | ✅ | ✅ | - |
| [**Custom Diffusion**](./custom_diffusion) | ✅ | ✅ | - |
## 커뮤니티
공식 예제 외에도 **커뮤니티 예제** 역시 제공하고 있습니다. 해당 예제들은 우리의 커뮤니티에 의해 관리됩니다. 커뮤니티 예쩨는 학습 예시나 추론 파이프라인으로 구성될 수 있습니다. 이러한 커뮤니티 예시들의 경우, 앞서 정의했던 철학들을 좀 더 관대하게 적용하고 있습니다. 또한 이러한 커뮤니티 예시들의 경우, 모든 이슈들에 대한 유지보수를 보장할 수는 없습니다.
유용하긴 하지만, 아직은 대중적이지 못하거나 저희의 철학에 부합하지 않는 예제들은 [community examples](https://github.com/huggingface/diffusers/tree/main/examples/community) 폴더에 담기게 됩니다.
**Note**: 커뮤니티 예제는 `diffusers`에 기여(contribution)를 희망하는 분들에게 [아주 좋은 기여 수단](https://github.com/huggingface/diffusers/issues?q=is%3Aopen+is%3Aissue+label%3A%22good+first+issue%22)이 될 수 있습니다.
## 주목할 사항들
최신 버전의 예시 코드들의 성공적인 구동을 보장하기 위해서는, 반드시 **소스코드를 통해 `diffusers`를 설치해야 하며,** 해당 예시 코드들이 요구하는 디펜던시들 역시 설치해야 합니다. 이를 위해 새로운 가상 환경을 구축하고 다음의 명령어를 실행해야 합니다.
```bash
git clone https://github.com/huggingface/diffusers
cd diffusers
pip install .
```
그 다음 `cd` 명령어를 통해 해당 예제 디렉토리에 접근해서 다음 명령어를 실행하면 됩니다.
```bash
pip install -r requirements.txt
``` | 0 |
hf_public_repos/diffusers/docs/source/ko | hf_public_repos/diffusers/docs/source/ko/training/lora.md | <!--Copyright 2023 The HuggingFace Team. All rights reserved.
Licensed under the Apache License, Version 2.0 (the "License"); you may not use this file except in compliance with
the License. You may obtain a copy of the License at
http://www.apache.org/licenses/LICENSE-2.0
Unless required by applicable law or agreed to in writing, software distributed under the License is distributed on
an "AS IS" BASIS, WITHOUT WARRANTIES OR CONDITIONS OF ANY KIND, either express or implied. See the License for the
specific language governing permissions and limitations under the License.
-->
# Low-Rank Adaptation of Large Language Models (LoRA)
[[open-in-colab]]
<Tip warning={true}>
현재 LoRA는 [`UNet2DConditionalModel`]의 어텐션 레이어에서만 지원됩니다.
</Tip>
[LoRA(Low-Rank Adaptation of Large Language Models)](https://arxiv.org/abs/2106.09685)는 메모리를 적게 사용하면서 대규모 모델의 학습을 가속화하는 학습 방법입니다. 이는 rank-decomposition weight 행렬 쌍(**업데이트 행렬**이라고 함)을 추가하고 새로 추가된 가중치**만** 학습합니다. 여기에는 몇 가지 장점이 있습니다.
- 이전에 미리 학습된 가중치는 고정된 상태로 유지되므로 모델이 [치명적인 망각](https://www.pnas.org/doi/10.1073/pnas.1611835114) 경향이 없습니다.
- Rank-decomposition 행렬은 원래 모델보다 파라메터 수가 훨씬 적으므로 학습된 LoRA 가중치를 쉽게 끼워넣을 수 있습니다.
- LoRA 매트릭스는 일반적으로 원본 모델의 어텐션 레이어에 추가됩니다. 🧨 Diffusers는 [`~diffusers.loaders.UNet2DConditionLoadersMixin.load_attn_procs`] 메서드를 제공하여 LoRA 가중치를 모델의 어텐션 레이어로 불러옵니다. `scale` 매개변수를 통해 모델이 새로운 학습 이미지에 맞게 조정되는 범위를 제어할 수 있습니다.
- 메모리 효율성이 향상되어 Tesla T4, RTX 3080 또는 RTX 2080 Ti와 같은 소비자용 GPU에서 파인튜닝을 실행할 수 있습니다! T4와 같은 GPU는 무료이며 Kaggle 또는 Google Colab 노트북에서 쉽게 액세스할 수 있습니다.
<Tip>
💡 LoRA는 어텐션 레이어에만 한정되지는 않습니다. 저자는 언어 모델의 어텐션 레이어를 수정하는 것이 매우 효율적으로 죻은 성능을 얻기에 충분하다는 것을 발견했습니다. 이것이 LoRA 가중치를 모델의 어텐션 레이어에 추가하는 것이 일반적인 이유입니다. LoRA 작동 방식에 대한 자세한 내용은 [Using LoRA for effective Stable Diffusion fine-tuning](https://huggingface.co/blog/lora) 블로그를 확인하세요!
</Tip>
[cloneofsimo](https://github.com/cloneofsimo)는 인기 있는 [lora](https://github.com/cloneofsimo/lora) GitHub 리포지토리에서 Stable Diffusion을 위한 LoRA 학습을 최초로 시도했습니다. 🧨 Diffusers는 [text-to-image 생성](https://github.com/huggingface/diffusers/tree/main/examples/text_to_image#training-with-lora) 및 [DreamBooth](https://github.com/huggingface/diffusers/tree/main/examples/dreambooth#training-with-low-rank-adaptation-of-large-language-models-lora)을 지원합니다. 이 가이드는 두 가지를 모두 수행하는 방법을 보여줍니다.
모델을 저장하거나 커뮤니티와 공유하려면 Hugging Face 계정에 로그인하세요(아직 계정이 없는 경우 [생성](hf.co/join)하세요):
```bash
huggingface-cli login
```
## Text-to-image
수십억 개의 파라메터들이 있는 Stable Diffusion과 같은 모델을 파인튜닝하는 것은 느리고 어려울 수 있습니다. LoRA를 사용하면 diffusion 모델을 파인튜닝하는 것이 훨씬 쉽고 빠릅니다. 8비트 옵티마이저와 같은 트릭에 의존하지 않고도 11GB의 GPU RAM으로 하드웨어에서 실행할 수 있습니다.
### 학습 [[text-to-image 학습]]
[Pokémon BLIP 캡션](https://huggingface.co/datasets/lambdalabs/pokemon-blip-captions) 데이터셋으로 [`stable-diffusion-v1-5`](https://huggingface.co/runwayml/stable-diffusion-v1-5)를 파인튜닝해 나만의 포켓몬을 생성해 보겠습니다.
시작하려면 `MODEL_NAME` 및 `DATASET_NAME` 환경 변수가 설정되어 있는지 확인하십시오. `OUTPUT_DIR` 및 `HUB_MODEL_ID` 변수는 선택 사항이며 허브에서 모델을 저장할 위치를 지정합니다.
```bash
export MODEL_NAME="runwayml/stable-diffusion-v1-5"
export OUTPUT_DIR="/sddata/finetune/lora/pokemon"
export HUB_MODEL_ID="pokemon-lora"
export DATASET_NAME="lambdalabs/pokemon-blip-captions"
```
학습을 시작하기 전에 알아야 할 몇 가지 플래그가 있습니다.
* `--push_to_hub`를 명시하면 학습된 LoRA 임베딩을 허브에 저장합니다.
* `--report_to=wandb`는 학습 결과를 가중치 및 편향 대시보드에 보고하고 기록합니다(예를 들어, 이 [보고서](https://wandb.ai/pcuenq/text2image-fine-tune/run/b4k1w0tn?workspace=user-pcuenq)를 참조하세요).
* `--learning_rate=1e-04`, 일반적으로 LoRA에서 사용하는 것보다 더 높은 학습률을 사용할 수 있습니다.
이제 학습을 시작할 준비가 되었습니다 (전체 학습 스크립트는 [여기](https://github.com/huggingface/diffusers/blob/main/examples/text_to_image/train_text_to_image_lora.py)에서 찾을 수 있습니다).
```bash
accelerate launch train_dreambooth_lora.py \
--pretrained_model_name_or_path=$MODEL_NAME \
--instance_data_dir=$INSTANCE_DIR \
--output_dir=$OUTPUT_DIR \
--instance_prompt="a photo of sks dog" \
--resolution=512 \
--train_batch_size=1 \
--gradient_accumulation_steps=1 \
--checkpointing_steps=100 \
--learning_rate=1e-4 \
--report_to="wandb" \
--lr_scheduler="constant" \
--lr_warmup_steps=0 \
--max_train_steps=500 \
--validation_prompt="A photo of sks dog in a bucket" \
--validation_epochs=50 \
--seed="0" \
--push_to_hub
```
### 추론 [[dreambooth 추론]]
이제 [`StableDiffusionPipeline`]에서 기본 모델을 불러와 추론을 위해 모델을 사용할 수 있습니다:
```py
>>> import torch
>>> from diffusers import StableDiffusionPipeline
>>> model_base = "runwayml/stable-diffusion-v1-5"
>>> pipe = StableDiffusionPipeline.from_pretrained(model_base, torch_dtype=torch.float16)
```
*기본 모델의 가중치 위에* 파인튜닝된 DreamBooth 모델에서 LoRA 가중치를 불러온 다음, 더 빠른 추론을 위해 파이프라인을 GPU로 이동합니다. LoRA 가중치를 프리징된 사전 훈련된 모델 가중치와 병합할 때, 선택적으로 'scale' 매개변수로 어느 정도의 가중치를 병합할 지 조절할 수 있습니다:
<Tip>
💡 `0`의 `scale` 값은 LoRA 가중치를 사용하지 않아 원래 모델의 가중치만 사용한 것과 같고, `1`의 `scale` 값은 파인튜닝된 LoRA 가중치만 사용함을 의미합니다. 0과 1 사이의 값들은 두 결과들 사이로 보간됩니다.
</Tip>
```py
>>> pipe.unet.load_attn_procs(model_path)
>>> pipe.to("cuda")
# LoRA 파인튜닝된 모델의 가중치 절반과 기본 모델의 가중치 절반 사용
>>> image = pipe(
... "A picture of a sks dog in a bucket.",
... num_inference_steps=25,
... guidance_scale=7.5,
... cross_attention_kwargs={"scale": 0.5},
... ).images[0]
# 완전히 파인튜닝된 LoRA 모델의 가중치 사용
>>> image = pipe("A picture of a sks dog in a bucket.", num_inference_steps=25, guidance_scale=7.5).images[0]
>>> image.save("bucket-dog.png")
``` | 0 |
hf_public_repos/diffusers/docs/source/ko | hf_public_repos/diffusers/docs/source/ko/training/dreambooth.md | <!--Copyright 2023 The HuggingFace Team. All rights reserved.
Licensed under the Apache License, Version 2.0 (the "License"); you may not use this file except in compliance with
the License. You may obtain a copy of the License at
http://www.apache.org/licenses/LICENSE-2.0
Unless required by applicable law or agreed to in writing, software distributed under the License is distributed on
an "AS IS" BASIS, WITHOUT WARRANTIES OR CONDITIONS OF ANY KIND, either express or implied. See the License for the
specific language governing permissions and limitations under the License.
-->
# DreamBooth
[DreamBooth](https://arxiv.org/abs/2208.12242)는 한 주제에 대한 적은 이미지(3~5개)만으로도 stable diffusion과 같이 text-to-image 모델을 개인화할 수 있는 방법입니다. 이를 통해 모델은 다양한 장면, 포즈 및 장면(뷰)에서 피사체에 대해 맥락화(contextualized)된 이미지를 생성할 수 있습니다.
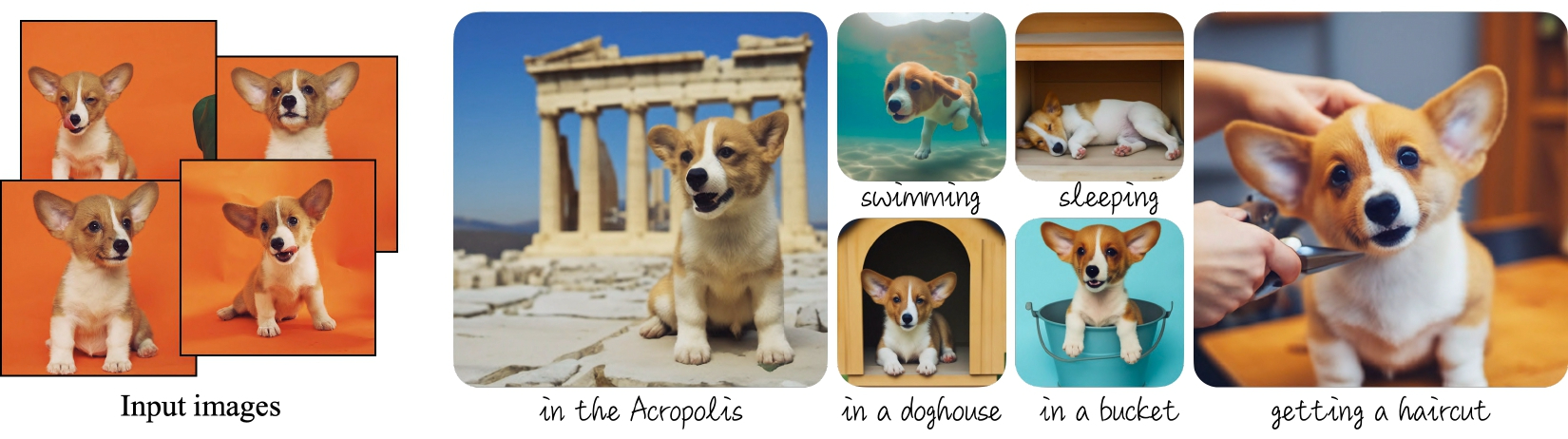
<a href="https://dreambooth.github.io">project's blog.</a></small>
<small><a href="https://dreambooth.github.io">프로젝트 블로그</a>에서의 Dreambooth 예시</small>
이 가이드는 다양한 GPU, Flax 사양에 대해 [`CompVis/stable-diffusion-v1-4`](https://huggingface.co/CompVis/stable-diffusion-v1-4) 모델로 DreamBooth를 파인튜닝하는 방법을 보여줍니다. 더 깊이 파고들어 작동 방식을 확인하는 데 관심이 있는 경우, 이 가이드에 사용된 DreamBooth의 모든 학습 스크립트를 [여기](https://github.com/huggingface/diffusers/tree/main/examples/dreambooth)에서 찾을 수 있습니다.
스크립트를 실행하기 전에 라이브러리의 학습에 필요한 dependencies를 설치해야 합니다. 또한 `main` GitHub 브랜치에서 🧨 Diffusers를 설치하는 것이 좋습니다.
```bash
pip install git+https://github.com/huggingface/diffusers
pip install -U -r diffusers/examples/dreambooth/requirements.txt
```
xFormers는 학습에 필요한 요구 사항은 아니지만, 가능하면 [설치](../optimization/xformers)하는 것이 좋습니다. 학습 속도를 높이고 메모리 사용량을 줄일 수 있기 때문입니다.
모든 dependencies을 설정한 후 다음을 사용하여 [🤗 Accelerate](https://github.com/huggingface/accelerate/) 환경을 다음과 같이 초기화합니다:
```bash
accelerate config
```
별도 설정 없이 기본 🤗 Accelerate 환경을 설치하려면 다음을 실행합니다:
```bash
accelerate config default
```
또는 현재 환경이 노트북과 같은 대화형 셸을 지원하지 않는 경우 다음을 사용할 수 있습니다:
```py
from accelerate.utils import write_basic_config
write_basic_config()
```
## 파인튜닝
<Tip warning={true}>
DreamBooth 파인튜닝은 하이퍼파라미터에 매우 민감하고 과적합되기 쉽습니다. 적절한 하이퍼파라미터를 선택하는 데 도움이 되도록 다양한 권장 설정이 포함된 [심층 분석](https://huggingface.co/blog/dreambooth)을 살펴보는 것이 좋습니다.
</Tip>
<frameworkcontent>
<pt>
[몇 장의 강아지 이미지들](https://drive.google.com/drive/folders/1BO_dyz-p65qhBRRMRA4TbZ8qW4rB99JZ)로 DreamBooth를 시도해봅시다.
이를 다운로드해 디렉터리에 저장한 다음 `INSTANCE_DIR` 환경 변수를 해당 경로로 설정합니다:
```bash
export MODEL_NAME="CompVis/stable-diffusion-v1-4"
export INSTANCE_DIR="path_to_training_images"
export OUTPUT_DIR="path_to_saved_model"
```
그런 다음, 다음 명령을 사용하여 학습 스크립트를 실행할 수 있습니다 (전체 학습 스크립트는 [여기](https://github.com/huggingface/diffusers/blob/main/examples/dreambooth/train_dreambooth.py)에서 찾을 수 있습니다):
```bash
accelerate launch train_dreambooth.py \
--pretrained_model_name_or_path=$MODEL_NAME \
--instance_data_dir=$INSTANCE_DIR \
--output_dir=$OUTPUT_DIR \
--instance_prompt="a photo of sks dog" \
--resolution=512 \
--train_batch_size=1 \
--gradient_accumulation_steps=1 \
--learning_rate=5e-6 \
--lr_scheduler="constant" \
--lr_warmup_steps=0 \
--max_train_steps=400
```
</pt>
<jax>
TPU에 액세스할 수 있거나 더 빠르게 훈련하고 싶다면 [Flax 학습 스크립트](https://github.com/huggingface/diffusers/blob/main/examples/dreambooth/train_dreambooth_flax.py)를 사용해 볼 수 있습니다. Flax 학습 스크립트는 gradient checkpointing 또는 gradient accumulation을 지원하지 않으므로, 메모리가 30GB 이상인 GPU가 필요합니다.
스크립트를 실행하기 전에 요구 사항이 설치되어 있는지 확인하십시오.
```bash
pip install -U -r requirements.txt
```
그러면 다음 명령어로 학습 스크립트를 실행시킬 수 있습니다:
```bash
export MODEL_NAME="duongna/stable-diffusion-v1-4-flax"
export INSTANCE_DIR="path-to-instance-images"
export OUTPUT_DIR="path-to-save-model"
python train_dreambooth_flax.py \
--pretrained_model_name_or_path=$MODEL_NAME \
--instance_data_dir=$INSTANCE_DIR \
--output_dir=$OUTPUT_DIR \
--instance_prompt="a photo of sks dog" \
--resolution=512 \
--train_batch_size=1 \
--learning_rate=5e-6 \
--max_train_steps=400
```
</jax>
</frameworkcontent>
### Prior-preserving(사전 보존) loss를 사용한 파인튜닝
과적합과 language drift를 방지하기 위해 사전 보존이 사용됩니다(관심이 있는 경우 [논문](https://arxiv.org/abs/2208.12242)을 참조하세요). 사전 보존을 위해 동일한 클래스의 다른 이미지를 학습 프로세스의 일부로 사용합니다. 좋은 점은 Stable Diffusion 모델 자체를 사용하여 이러한 이미지를 생성할 수 있다는 것입니다! 학습 스크립트는 생성된 이미지를 우리가 지정한 로컬 경로에 저장합니다.
저자들에 따르면 사전 보존을 위해 `num_epochs * num_samples`개의 이미지를 생성하는 것이 좋습니다. 200-300개에서 대부분 잘 작동합니다.
<frameworkcontent>
<pt>
```bash
export MODEL_NAME="CompVis/stable-diffusion-v1-4"
export INSTANCE_DIR="path_to_training_images"
export CLASS_DIR="path_to_class_images"
export OUTPUT_DIR="path_to_saved_model"
accelerate launch train_dreambooth.py \
--pretrained_model_name_or_path=$MODEL_NAME \
--instance_data_dir=$INSTANCE_DIR \
--class_data_dir=$CLASS_DIR \
--output_dir=$OUTPUT_DIR \
--with_prior_preservation --prior_loss_weight=1.0 \
--instance_prompt="a photo of sks dog" \
--class_prompt="a photo of dog" \
--resolution=512 \
--train_batch_size=1 \
--gradient_accumulation_steps=1 \
--learning_rate=5e-6 \
--lr_scheduler="constant" \
--lr_warmup_steps=0 \
--num_class_images=200 \
--max_train_steps=800
```
</pt>
<jax>
```bash
export MODEL_NAME="duongna/stable-diffusion-v1-4-flax"
export INSTANCE_DIR="path-to-instance-images"
export CLASS_DIR="path-to-class-images"
export OUTPUT_DIR="path-to-save-model"
python train_dreambooth_flax.py \
--pretrained_model_name_or_path=$MODEL_NAME \
--instance_data_dir=$INSTANCE_DIR \
--class_data_dir=$CLASS_DIR \
--output_dir=$OUTPUT_DIR \
--with_prior_preservation --prior_loss_weight=1.0 \
--instance_prompt="a photo of sks dog" \
--class_prompt="a photo of dog" \
--resolution=512 \
--train_batch_size=1 \
--learning_rate=5e-6 \
--num_class_images=200 \
--max_train_steps=800
```
</jax>
</frameworkcontent>
## 텍스트 인코더와 and UNet로 파인튜닝하기
해당 스크립트를 사용하면 `unet`과 함께 `text_encoder`를 파인튜닝할 수 있습니다. 실험에서(자세한 내용은 [🧨 Diffusers를 사용해 DreamBooth로 Stable Diffusion 학습하기](https://huggingface.co/blog/dreambooth) 게시물을 확인하세요), 특히 얼굴 이미지를 생성할 때 훨씬 더 나은 결과를 얻을 수 있습니다.
<Tip warning={true}>
텍스트 인코더를 학습시키려면 추가 메모리가 필요해 16GB GPU로는 동작하지 않습니다. 이 옵션을 사용하려면 최소 24GB VRAM이 필요합니다.
</Tip>
`--train_text_encoder` 인수를 학습 스크립트에 전달하여 `text_encoder` 및 `unet`을 파인튜닝할 수 있습니다:
<frameworkcontent>
<pt>
```bash
export MODEL_NAME="CompVis/stable-diffusion-v1-4"
export INSTANCE_DIR="path_to_training_images"
export CLASS_DIR="path_to_class_images"
export OUTPUT_DIR="path_to_saved_model"
accelerate launch train_dreambooth.py \
--pretrained_model_name_or_path=$MODEL_NAME \
--train_text_encoder \
--instance_data_dir=$INSTANCE_DIR \
--class_data_dir=$CLASS_DIR \
--output_dir=$OUTPUT_DIR \
--with_prior_preservation --prior_loss_weight=1.0 \
--instance_prompt="a photo of sks dog" \
--class_prompt="a photo of dog" \
--resolution=512 \
--train_batch_size=1 \
--use_8bit_adam
--gradient_checkpointing \
--learning_rate=2e-6 \
--lr_scheduler="constant" \
--lr_warmup_steps=0 \
--num_class_images=200 \
--max_train_steps=800
```
</pt>
<jax>
```bash
export MODEL_NAME="duongna/stable-diffusion-v1-4-flax"
export INSTANCE_DIR="path-to-instance-images"
export CLASS_DIR="path-to-class-images"
export OUTPUT_DIR="path-to-save-model"
python train_dreambooth_flax.py \
--pretrained_model_name_or_path=$MODEL_NAME \
--train_text_encoder \
--instance_data_dir=$INSTANCE_DIR \
--class_data_dir=$CLASS_DIR \
--output_dir=$OUTPUT_DIR \
--with_prior_preservation --prior_loss_weight=1.0 \
--instance_prompt="a photo of sks dog" \
--class_prompt="a photo of dog" \
--resolution=512 \
--train_batch_size=1 \
--learning_rate=2e-6 \
--num_class_images=200 \
--max_train_steps=800
```
</jax>
</frameworkcontent>
## LoRA로 파인튜닝하기
DreamBooth에서 대규모 모델의 학습을 가속화하기 위한 파인튜닝 기술인 LoRA(Low-Rank Adaptation of Large Language Models)를 사용할 수 있습니다. 자세한 내용은 [LoRA 학습](training/lora#dreambooth) 가이드를 참조하세요.
### 학습 중 체크포인트 저장하기
Dreambooth로 훈련하는 동안 과적합하기 쉬우므로, 때때로 학습 중에 정기적인 체크포인트를 저장하는 것이 유용합니다. 중간 체크포인트 중 하나가 최종 모델보다 더 잘 작동할 수 있습니다! 체크포인트 저장 기능을 활성화하려면 학습 스크립트에 다음 인수를 전달해야 합니다:
```bash
--checkpointing_steps=500
```
이렇게 하면 `output_dir`의 하위 폴더에 전체 학습 상태가 저장됩니다. 하위 폴더 이름은 접두사 `checkpoint-`로 시작하고 지금까지 수행된 step 수입니다. 예시로 `checkpoint-1500`은 1500 학습 step 후에 저장된 체크포인트입니다.
#### 저장된 체크포인트에서 훈련 재개하기
저장된 체크포인트에서 훈련을 재개하려면, `--resume_from_checkpoint` 인수를 전달한 다음 사용할 체크포인트의 이름을 지정하면 됩니다. 특수 문자열 `"latest"`를 사용하여 저장된 마지막 체크포인트(즉, step 수가 가장 많은 체크포인트)에서 재개할 수도 있습니다. 예를 들어 다음은 1500 step 후에 저장된 체크포인트에서부터 학습을 재개합니다:
```bash
--resume_from_checkpoint="checkpoint-1500"
```
원하는 경우 일부 하이퍼파라미터를 조정할 수 있습니다.
#### 저장된 체크포인트를 사용하여 추론 수행하기
저장된 체크포인트는 훈련 재개에 적합한 형식으로 저장됩니다. 여기에는 모델 가중치뿐만 아니라 옵티마이저, 데이터 로더 및 학습률의 상태도 포함됩니다.
**`"accelerate>=0.16.0"`**이 설치된 경우 다음 코드를 사용하여 중간 체크포인트에서 추론을 실행합니다.
```python
from diffusers import DiffusionPipeline, UNet2DConditionModel
from transformers import CLIPTextModel
import torch
# 학습에 사용된 것과 동일한 인수(model, revision)로 파이프라인을 불러옵니다.
model_id = "CompVis/stable-diffusion-v1-4"
unet = UNet2DConditionModel.from_pretrained("/sddata/dreambooth/daruma-v2-1/checkpoint-100/unet")
# `args.train_text_encoder`로 학습한 경우면 텍스트 인코더를 꼭 불러오세요
text_encoder = CLIPTextModel.from_pretrained("/sddata/dreambooth/daruma-v2-1/checkpoint-100/text_encoder")
pipeline = DiffusionPipeline.from_pretrained(model_id, unet=unet, text_encoder=text_encoder, dtype=torch.float16)
pipeline.to("cuda")
# 추론을 수행하거나 저장하거나, 허브에 푸시합니다.
pipeline.save_pretrained("dreambooth-pipeline")
```
If you have **`"accelerate<0.16.0"`** installed, you need to convert it to an inference pipeline first:
```python
from accelerate import Accelerator
from diffusers import DiffusionPipeline
# 학습에 사용된 것과 동일한 인수(model, revision)로 파이프라인을 불러옵니다.
model_id = "CompVis/stable-diffusion-v1-4"
pipeline = DiffusionPipeline.from_pretrained(model_id)
accelerator = Accelerator()
# 초기 학습에 `--train_text_encoder`가 사용된 경우 text_encoder를 사용합니다.
unet, text_encoder = accelerator.prepare(pipeline.unet, pipeline.text_encoder)
# 체크포인트 경로로부터 상태를 복원합니다. 여기서는 절대 경로를 사용해야 합니다.
accelerator.load_state("/sddata/dreambooth/daruma-v2-1/checkpoint-100")
# unwrapped 모델로 파이프라인을 다시 빌드합니다.(.unet and .text_encoder로의 할당도 작동해야 합니다)
pipeline = DiffusionPipeline.from_pretrained(
model_id,
unet=accelerator.unwrap_model(unet),
text_encoder=accelerator.unwrap_model(text_encoder),
)
# 추론을 수행하거나 저장하거나, 허브에 푸시합니다.
pipeline.save_pretrained("dreambooth-pipeline")
```
## 각 GPU 용량에서의 최적화
하드웨어에 따라 16GB에서 8GB까지 GPU에서 DreamBooth를 최적화하는 몇 가지 방법이 있습니다!
### xFormers
[xFormers](https://github.com/facebookresearch/xformers)는 Transformers를 최적화하기 위한 toolbox이며, 🧨 Diffusers에서 사용되는[memory-efficient attention](https://facebookresearch.github.io/xformers/components/ops.html#module-xformers.ops) 메커니즘을 포함하고 있습니다. [xFormers를 설치](./optimization/xformers)한 다음 학습 스크립트에 다음 인수를 추가합니다:
```bash
--enable_xformers_memory_efficient_attention
```
xFormers는 Flax에서 사용할 수 없습니다.
### 그래디언트 없음으로 설정
메모리 사용량을 줄일 수 있는 또 다른 방법은 [기울기 설정](https://pytorch.org/docs/stable/generated/torch.optim.Optimizer.zero_grad.html)을 0 대신 `None`으로 하는 것입니다. 그러나 이로 인해 특정 동작이 변경될 수 있으므로 문제가 발생하면 이 인수를 제거해 보십시오. 학습 스크립트에 다음 인수를 추가하여 그래디언트를 `None`으로 설정합니다.
```bash
--set_grads_to_none
```
### 16GB GPU
Gradient checkpointing과 [bitsandbytes](https://github.com/TimDettmers/bitsandbytes)의 8비트 옵티마이저의 도움으로, 16GB GPU에서 dreambooth를 훈련할 수 있습니다. bitsandbytes가 설치되어 있는지 확인하세요:
```bash
pip install bitsandbytes
```
그 다음, 학습 스크립트에 `--use_8bit_adam` 옵션을 명시합니다:
```bash
export MODEL_NAME="CompVis/stable-diffusion-v1-4"
export INSTANCE_DIR="path_to_training_images"
export CLASS_DIR="path_to_class_images"
export OUTPUT_DIR="path_to_saved_model"
accelerate launch train_dreambooth.py \
--pretrained_model_name_or_path=$MODEL_NAME \
--instance_data_dir=$INSTANCE_DIR \
--class_data_dir=$CLASS_DIR \
--output_dir=$OUTPUT_DIR \
--with_prior_preservation --prior_loss_weight=1.0 \
--instance_prompt="a photo of sks dog" \
--class_prompt="a photo of dog" \
--resolution=512 \
--train_batch_size=1 \
--gradient_accumulation_steps=2 --gradient_checkpointing \
--use_8bit_adam \
--learning_rate=5e-6 \
--lr_scheduler="constant" \
--lr_warmup_steps=0 \
--num_class_images=200 \
--max_train_steps=800
```
### 12GB GPU
12GB GPU에서 DreamBooth를 실행하려면 gradient checkpointing, 8비트 옵티마이저, xFormers를 활성화하고 그래디언트를 `None`으로 설정해야 합니다.
```bash
export MODEL_NAME="CompVis/stable-diffusion-v1-4"
export INSTANCE_DIR="path-to-instance-images"
export CLASS_DIR="path-to-class-images"
export OUTPUT_DIR="path-to-save-model"
accelerate launch train_dreambooth.py \
--pretrained_model_name_or_path=$MODEL_NAME \
--instance_data_dir=$INSTANCE_DIR \
--class_data_dir=$CLASS_DIR \
--output_dir=$OUTPUT_DIR \
--with_prior_preservation --prior_loss_weight=1.0 \
--instance_prompt="a photo of sks dog" \
--class_prompt="a photo of dog" \
--resolution=512 \
--train_batch_size=1 \
--gradient_accumulation_steps=1 --gradient_checkpointing \
--use_8bit_adam \
--enable_xformers_memory_efficient_attention \
--set_grads_to_none \
--learning_rate=2e-6 \
--lr_scheduler="constant" \
--lr_warmup_steps=0 \
--num_class_images=200 \
--max_train_steps=800
```
### 8GB GPU에서 학습하기
8GB GPU에 대해서는 [DeepSpeed](https://www.deepspeed.ai/)를 사용해 일부 텐서를 VRAM에서 CPU 또는 NVME로 오프로드하여 더 적은 GPU 메모리로 학습할 수도 있습니다.
🤗 Accelerate 환경을 구성하려면 다음 명령을 실행하세요:
```bash
accelerate config
```
환경 구성 중에 DeepSpeed를 사용할 것을 확인하세요.
그러면 DeepSpeed stage 2, fp16 혼합 정밀도를 결합하고 모델 매개변수와 옵티마이저 상태를 모두 CPU로 오프로드하면 8GB VRAM 미만에서 학습할 수 있습니다.
단점은 더 많은 시스템 RAM(약 25GB)이 필요하다는 것입니다. 추가 구성 옵션은 [DeepSpeed 문서](https://huggingface.co/docs/accelerate/usage_guides/deepspeed)를 참조하세요.
또한 기본 Adam 옵티마이저를 DeepSpeed의 최적화된 Adam 버전으로 변경해야 합니다.
이는 상당한 속도 향상을 위한 Adam인 [`deepspeed.ops.adam.DeepSpeedCPUAdam`](https://deepspeed.readthedocs.io/en/latest/optimizers.html#adam-cpu)입니다.
`DeepSpeedCPUAdam`을 활성화하려면 시스템의 CUDA toolchain 버전이 PyTorch와 함께 설치된 것과 동일해야 합니다.
8비트 옵티마이저는 현재 DeepSpeed와 호환되지 않는 것 같습니다.
다음 명령으로 학습을 시작합니다:
```bash
export MODEL_NAME="CompVis/stable-diffusion-v1-4"
export INSTANCE_DIR="path_to_training_images"
export CLASS_DIR="path_to_class_images"
export OUTPUT_DIR="path_to_saved_model"
accelerate launch train_dreambooth.py \
--pretrained_model_name_or_path=$MODEL_NAME \
--instance_data_dir=$INSTANCE_DIR \
--class_data_dir=$CLASS_DIR \
--output_dir=$OUTPUT_DIR \
--with_prior_preservation --prior_loss_weight=1.0 \
--instance_prompt="a photo of sks dog" \
--class_prompt="a photo of dog" \
--resolution=512 \
--train_batch_size=1 \
--sample_batch_size=1 \
--gradient_accumulation_steps=1 --gradient_checkpointing \
--learning_rate=5e-6 \
--lr_scheduler="constant" \
--lr_warmup_steps=0 \
--num_class_images=200 \
--max_train_steps=800 \
--mixed_precision=fp16
```
## 추론
모델을 학습한 후에는, 모델이 저장된 경로를 지정해 [`StableDiffusionPipeline`]로 추론을 수행할 수 있습니다. 프롬프트에 학습에 사용된 특수 `식별자`(이전 예시의 `sks`)가 포함되어 있는지 확인하세요.
**`"accelerate>=0.16.0"`**이 설치되어 있는 경우 다음 코드를 사용하여 중간 체크포인트에서 추론을 실행할 수 있습니다:
```python
from diffusers import StableDiffusionPipeline
import torch
model_id = "path_to_saved_model"
pipe = StableDiffusionPipeline.from_pretrained(model_id, torch_dtype=torch.float16).to("cuda")
prompt = "A photo of sks dog in a bucket"
image = pipe(prompt, num_inference_steps=50, guidance_scale=7.5).images[0]
image.save("dog-bucket.png")
```
[저장된 학습 체크포인트](#inference-from-a-saved-checkpoint)에서도 추론을 실행할 수도 있습니다. | 0 |
hf_public_repos/diffusers/docs/source/ko | hf_public_repos/diffusers/docs/source/ko/training/adapt_a_model.md | <!--Copyright 2023 The HuggingFace Team. All rights reserved.
Licensed under the Apache License, Version 2.0 (the "License"); you may not use this file except in compliance with
the License. You may obtain a copy of the License at
http://www.apache.org/licenses/LICENSE-2.0
Unless required by applicable law or agreed to in writing, software distributed under the License is distributed on
an "AS IS" BASIS, WITHOUT WARRANTIES OR CONDITIONS OF ANY KIND, either express or implied. See the License for the
specific language governing permissions and limitations under the License.
-->
# 새로운 작업에 대한 모델을 적용하기
많은 diffusion 시스템은 같은 구성 요소들을 공유하므로 한 작업에 대해 사전학습된 모델을 완전히 다른 작업에 적용할 수 있습니다.
이 인페인팅을 위한 가이드는 사전학습된 [`UNet2DConditionModel`]의 아키텍처를 초기화하고 수정하여 사전학습된 text-to-image 모델을 어떻게 인페인팅에 적용하는지를 알려줄 것입니다.
## UNet2DConditionModel 파라미터 구성
[`UNet2DConditionModel`]은 [input sample](https://huggingface.co/docs/diffusers/v0.16.0/en/api/models#diffusers.UNet2DConditionModel.in_channels)에서 4개의 채널을 기본적으로 허용합니다. 예를 들어, [`runwayml/stable-diffusion-v1-5`](https://huggingface.co/runwayml/stable-diffusion-v1-5)와 같은 사전학습된 text-to-image 모델을 불러오고 `in_channels`의 수를 확인합니다:
```py
from diffusers import StableDiffusionPipeline
pipeline = StableDiffusionPipeline.from_pretrained("runwayml/stable-diffusion-v1-5")
pipeline.unet.config["in_channels"]
4
```
인페인팅은 입력 샘플에 9개의 채널이 필요합니다. [`runwayml/stable-diffusion-inpainting`](https://huggingface.co/runwayml/stable-diffusion-inpainting)와 같은 사전학습된 인페인팅 모델에서 이 값을 확인할 수 있습니다:
```py
from diffusers import StableDiffusionPipeline
pipeline = StableDiffusionPipeline.from_pretrained("runwayml/stable-diffusion-inpainting")
pipeline.unet.config["in_channels"]
9
```
인페인팅에 대한 text-to-image 모델을 적용하기 위해, `in_channels` 수를 4에서 9로 수정해야 할 것입니다.
사전학습된 text-to-image 모델의 가중치와 [`UNet2DConditionModel`]을 초기화하고 `in_channels`를 9로 수정해 주세요. `in_channels`의 수를 수정하면 크기가 달라지기 때문에 크기가 안 맞는 오류를 피하기 위해 `ignore_mismatched_sizes=True` 및 `low_cpu_mem_usage=False`를 설정해야 합니다.
```py
from diffusers import UNet2DConditionModel
model_id = "runwayml/stable-diffusion-v1-5"
unet = UNet2DConditionModel.from_pretrained(
model_id, subfolder="unet", in_channels=9, low_cpu_mem_usage=False, ignore_mismatched_sizes=True
)
```
Text-to-image 모델로부터 다른 구성 요소의 사전학습된 가중치는 체크포인트로부터 초기화되지만 `unet`의 입력 채널 가중치 (`conv_in.weight`)는 랜덤하게 초기화됩니다. 그렇지 않으면 모델이 노이즈를 리턴하기 때문에 인페인팅의 모델을 파인튜닝 할 때 중요합니다.
| 0 |
hf_public_repos/diffusers/docs/source/ko | hf_public_repos/diffusers/docs/source/ko/training/text2image.md | <!--Copyright 2023 The HuggingFace Team. All rights reserved.
Licensed under the Apache License, Version 2.0 (the "License"); you may not use this file except in compliance with
the License. You may obtain a copy of the License at
http://www.apache.org/licenses/LICENSE-2.0
Unless required by applicable law or agreed to in writing, software distributed under the License is distributed on
an "AS IS" BASIS, WITHOUT WARRANTIES OR CONDITIONS OF ANY KIND, either express or implied. See the License for the
specific language governing permissions and limitations under the License.
-->
# Text-to-image
<Tip warning={true}>
text-to-image 파인튜닝 스크립트는 experimental 상태입니다. 과적합하기 쉽고 치명적인 망각과 같은 문제에 부딪히기 쉽습니다. 자체 데이터셋에서 최상의 결과를 얻으려면 다양한 하이퍼파라미터를 탐색하는 것이 좋습니다.
</Tip>
Stable Diffusion과 같은 text-to-image 모델은 텍스트 프롬프트에서 이미지를 생성합니다. 이 가이드는 PyTorch 및 Flax를 사용하여 자체 데이터셋에서 [`CompVis/stable-diffusion-v1-4`](https://huggingface.co/CompVis/stable-diffusion-v1-4) 모델로 파인튜닝하는 방법을 보여줍니다. 이 가이드에 사용된 text-to-image 파인튜닝을 위한 모든 학습 스크립트에 관심이 있는 경우 이 [리포지토리](https://github.com/huggingface/diffusers/tree/main/examples/text_to_image)에서 자세히 찾을 수 있습니다.
스크립트를 실행하기 전에, 라이브러리의 학습 dependency들을 설치해야 합니다:
```bash
pip install git+https://github.com/huggingface/diffusers.git
pip install -U -r requirements.txt
```
그리고 [🤗Accelerate](https://github.com/huggingface/accelerate/) 환경을 초기화합니다:
```bash
accelerate config
```
리포지토리를 이미 복제한 경우, 이 단계를 수행할 필요가 없습니다. 대신, 로컬 체크아웃 경로를 학습 스크립트에 명시할 수 있으며 거기에서 로드됩니다.
### 하드웨어 요구 사항
`gradient_checkpointing` 및 `mixed_precision`을 사용하면 단일 24GB GPU에서 모델을 파인튜닝할 수 있습니다. 더 높은 `batch_size`와 더 빠른 훈련을 위해서는 GPU 메모리가 30GB 이상인 GPU를 사용하는 것이 좋습니다. TPU 또는 GPU에서 파인튜닝을 위해 JAX나 Flax를 사용할 수도 있습니다. 자세한 내용은 [아래](#flax-jax-finetuning)를 참조하세요.
xFormers로 memory efficient attention을 활성화하여 메모리 사용량 훨씬 더 줄일 수 있습니다. [xFormers가 설치](./optimization/xformers)되어 있는지 확인하고 `--enable_xformers_memory_efficient_attention`를 학습 스크립트에 명시합니다.
xFormers는 Flax에 사용할 수 없습니다.
## Hub에 모델 업로드하기
학습 스크립트에 다음 인수를 추가하여 모델을 허브에 저장합니다:
```bash
--push_to_hub
```
## 체크포인트 저장 및 불러오기
학습 중 발생할 수 있는 일에 대비하여 정기적으로 체크포인트를 저장해 두는 것이 좋습니다. 체크포인트를 저장하려면 학습 스크립트에 다음 인수를 명시합니다.
```bash
--checkpointing_steps=500
```
500스텝마다 전체 학습 state가 'output_dir'의 하위 폴더에 저장됩니다. 체크포인트는 'checkpoint-'에 지금까지 학습된 step 수입니다. 예를 들어 'checkpoint-1500'은 1500 학습 step 후에 저장된 체크포인트입니다.
학습을 재개하기 위해 체크포인트를 불러오려면 '--resume_from_checkpoint' 인수를 학습 스크립트에 명시하고 재개할 체크포인트를 지정하십시오. 예를 들어 다음 인수는 1500개의 학습 step 후에 저장된 체크포인트에서부터 훈련을 재개합니다.
```bash
--resume_from_checkpoint="checkpoint-1500"
```
## 파인튜닝
<frameworkcontent>
<pt>
다음과 같이 [Pokémon BLIP 캡션](https://huggingface.co/datasets/lambdalabs/pokemon-blip-captions) 데이터셋에서 파인튜닝 실행을 위해 [PyTorch 학습 스크립트](https://github.com/huggingface/diffusers/blob/main/examples/text_to_image/train_text_to_image.py)를 실행합니다:
```bash
export MODEL_NAME="CompVis/stable-diffusion-v1-4"
export dataset_name="lambdalabs/pokemon-blip-captions"
accelerate launch train_text_to_image.py \
--pretrained_model_name_or_path=$MODEL_NAME \
--dataset_name=$dataset_name \
--use_ema \
--resolution=512 --center_crop --random_flip \
--train_batch_size=1 \
--gradient_accumulation_steps=4 \
--gradient_checkpointing \
--mixed_precision="fp16" \
--max_train_steps=15000 \
--learning_rate=1e-05 \
--max_grad_norm=1 \
--lr_scheduler="constant" --lr_warmup_steps=0 \
--output_dir="sd-pokemon-model"
```
자체 데이터셋으로 파인튜닝하려면 🤗 [Datasets](https://huggingface.co/docs/datasets/index)에서 요구하는 형식에 따라 데이터셋을 준비하세요. [데이터셋을 허브에 업로드](https://huggingface.co/docs/datasets/image_dataset#upload-dataset-to-the-hub)하거나 [파일들이 있는 로컬 폴더를 준비](https ://huggingface.co/docs/datasets/image_dataset#imagefolder)할 수 있습니다.
사용자 커스텀 loading logic을 사용하려면 스크립트를 수정하십시오. 도움이 되도록 코드의 적절한 위치에 포인터를 남겼습니다. 🤗 아래 예제 스크립트는 `TRAIN_DIR`의 로컬 데이터셋으로를 파인튜닝하는 방법과 `OUTPUT_DIR`에서 모델을 저장할 위치를 보여줍니다:
```bash
export MODEL_NAME="CompVis/stable-diffusion-v1-4"
export TRAIN_DIR="path_to_your_dataset"
export OUTPUT_DIR="path_to_save_model"
accelerate launch train_text_to_image.py \
--pretrained_model_name_or_path=$MODEL_NAME \
--train_data_dir=$TRAIN_DIR \
--use_ema \
--resolution=512 --center_crop --random_flip \
--train_batch_size=1 \
--gradient_accumulation_steps=4 \
--gradient_checkpointing \
--mixed_precision="fp16" \
--max_train_steps=15000 \
--learning_rate=1e-05 \
--max_grad_norm=1 \
--lr_scheduler="constant" --lr_warmup_steps=0 \
--output_dir=${OUTPUT_DIR}
```
</pt>
<jax>
[@duongna211](https://github.com/duongna21)의 기여로, Flax를 사용해 TPU 및 GPU에서 Stable Diffusion 모델을 더 빠르게 학습할 수 있습니다. 이는 TPU 하드웨어에서 매우 효율적이지만 GPU에서도 훌륭하게 작동합니다. Flax 학습 스크립트는 gradient checkpointing나 gradient accumulation과 같은 기능을 아직 지원하지 않으므로 메모리가 30GB 이상인 GPU 또는 TPU v3가 필요합니다.
스크립트를 실행하기 전에 요구 사항이 설치되어 있는지 확인하십시오:
```bash
pip install -U -r requirements_flax.txt
```
그러면 다음과 같이 [Flax 학습 스크립트](https://github.com/huggingface/diffusers/blob/main/examples/text_to_image/train_text_to_image_flax.py)를 실행할 수 있습니다.
```bash
export MODEL_NAME="runwayml/stable-diffusion-v1-5"
export dataset_name="lambdalabs/pokemon-blip-captions"
python train_text_to_image_flax.py \
--pretrained_model_name_or_path=$MODEL_NAME \
--dataset_name=$dataset_name \
--resolution=512 --center_crop --random_flip \
--train_batch_size=1 \
--max_train_steps=15000 \
--learning_rate=1e-05 \
--max_grad_norm=1 \
--output_dir="sd-pokemon-model"
```
자체 데이터셋으로 파인튜닝하려면 🤗 [Datasets](https://huggingface.co/docs/datasets/index)에서 요구하는 형식에 따라 데이터셋을 준비하세요. [데이터셋을 허브에 업로드](https://huggingface.co/docs/datasets/image_dataset#upload-dataset-to-the-hub)하거나 [파일들이 있는 로컬 폴더를 준비](https ://huggingface.co/docs/datasets/image_dataset#imagefolder)할 수 있습니다.
사용자 커스텀 loading logic을 사용하려면 스크립트를 수정하십시오. 도움이 되도록 코드의 적절한 위치에 포인터를 남겼습니다. 🤗 아래 예제 스크립트는 `TRAIN_DIR`의 로컬 데이터셋으로를 파인튜닝하는 방법을 보여줍니다:
```bash
export MODEL_NAME="duongna/stable-diffusion-v1-4-flax"
export TRAIN_DIR="path_to_your_dataset"
python train_text_to_image_flax.py \
--pretrained_model_name_or_path=$MODEL_NAME \
--train_data_dir=$TRAIN_DIR \
--resolution=512 --center_crop --random_flip \
--train_batch_size=1 \
--mixed_precision="fp16" \
--max_train_steps=15000 \
--learning_rate=1e-05 \
--max_grad_norm=1 \
--output_dir="sd-pokemon-model"
```
</jax>
</frameworkcontent>
## LoRA
Text-to-image 모델 파인튜닝을 위해, 대규모 모델 학습을 가속화하기 위한 파인튜닝 기술인 LoRA(Low-Rank Adaptation of Large Language Models)를 사용할 수 있습니다. 자세한 내용은 [LoRA 학습](lora#text-to-image) 가이드를 참조하세요.
## 추론
허브의 모델 경로 또는 모델 이름을 [`StableDiffusionPipeline`]에 전달하여 추론을 위해 파인 튜닝된 모델을 불러올 수 있습니다:
<frameworkcontent>
<pt>
```python
from diffusers import StableDiffusionPipeline
model_path = "path_to_saved_model"
pipe = StableDiffusionPipeline.from_pretrained(model_path, torch_dtype=torch.float16)
pipe.to("cuda")
image = pipe(prompt="yoda").images[0]
image.save("yoda-pokemon.png")
```
</pt>
<jax>
```python
import jax
import numpy as np
from flax.jax_utils import replicate
from flax.training.common_utils import shard
from diffusers import FlaxStableDiffusionPipeline
model_path = "path_to_saved_model"
pipe, params = FlaxStableDiffusionPipeline.from_pretrained(model_path, dtype=jax.numpy.bfloat16)
prompt = "yoda pokemon"
prng_seed = jax.random.PRNGKey(0)
num_inference_steps = 50
num_samples = jax.device_count()
prompt = num_samples * [prompt]
prompt_ids = pipeline.prepare_inputs(prompt)
# shard inputs and rng
params = replicate(params)
prng_seed = jax.random.split(prng_seed, jax.device_count())
prompt_ids = shard(prompt_ids)
images = pipeline(prompt_ids, params, prng_seed, num_inference_steps, jit=True).images
images = pipeline.numpy_to_pil(np.asarray(images.reshape((num_samples,) + images.shape[-3:])))
image.save("yoda-pokemon.png")
```
</jax>
</frameworkcontent> | 0 |
hf_public_repos/diffusers/docs/source/ko | hf_public_repos/diffusers/docs/source/ko/using-diffusers/pipeline_overview.md | <!--Copyright 2023 The HuggingFace Team. All rights reserved.
Licensed under the Apache License, Version 2.0 (the "License"); you may not use this file except in compliance with
the License. You may obtain a copy of the License at
http://www.apache.org/licenses/LICENSE-2.0
Unless required by applicable law or agreed to in writing, software distributed under the License is distributed on
an "AS IS" BASIS, WITHOUT WARRANTIES OR CONDITIONS OF ANY KIND, either express or implied. See the License for the
specific language governing permissions and limitations under the License.
-->
# Overview
파이프라인은 독립적으로 훈련된 모델과 스케줄러를 함께 모아서 추론을 위해 diffusion 시스템을 빠르고 쉽게 사용할 수 있는 방법을 제공하는 end-to-end 클래스입니다. 모델과 스케줄러의 특정 조합은 특수한 기능과 함께 [`StableDiffusionPipeline`] 또는 [`StableDiffusionControlNetPipeline`]과 같은 특정 파이프라인 유형을 정의합니다. 모든 파이프라인 유형은 기본 [`DiffusionPipeline`] 클래스에서 상속됩니다. 어느 체크포인트를 전달하면, 파이프라인 유형을 자동으로 감지하고 필요한 구성 요소들을 불러옵니다.
이 섹션에서는 unconditional 이미지 생성, text-to-image 생성의 다양한 테크닉과 변화를 파이프라인에서 지원하는 작업들을 소개합니다. 프롬프트에 있는 특정 단어가 출력에 영향을 미치는 것을 조정하기 위해 재현성을 위한 시드 설정과 프롬프트에 가중치를 부여하는 것으로 생성 프로세스를 더 잘 제어하는 방법에 대해 배울 수 있습니다. 마지막으로 음성에서부터 이미지 생성과 같은 커스텀 작업을 위한 커뮤니티 파이프라인을 만드는 방법을 알 수 있습니다.
| 0 |
hf_public_repos/diffusers/docs/source/ko | hf_public_repos/diffusers/docs/source/ko/using-diffusers/depth2img.md | <!--Copyright 2023 The HuggingFace Team. All rights reserved.
Licensed under the Apache License, Version 2.0 (the "License"); you may not use this file except in compliance with
the License. You may obtain a copy of the License at
http://www.apache.org/licenses/LICENSE-2.0
Unless required by applicable law or agreed to in writing, software distributed under the License is distributed on
an "AS IS" BASIS, WITHOUT WARRANTIES OR CONDITIONS OF ANY KIND, either express or implied. See the License for the
specific language governing permissions and limitations under the License.
-->
# Text-guided depth-to-image 생성
[[open-in-colab]]
[`StableDiffusionDepth2ImgPipeline`]을 사용하면 텍스트 프롬프트와 초기 이미지를 전달하여 새 이미지의 생성을 조절할 수 있습니다. 또한 이미지 구조를 보존하기 위해 `depth_map`을 전달할 수도 있습니다. `depth_map`이 제공되지 않으면 파이프라인은 통합된 [depth-estimation model](https://github.com/isl-org/MiDaS)을 통해 자동으로 깊이를 예측합니다.
먼저 [`StableDiffusionDepth2ImgPipeline`]의 인스턴스를 생성합니다:
```python
import torch
import requests
from PIL import Image
from diffusers import StableDiffusionDepth2ImgPipeline
pipe = StableDiffusionDepth2ImgPipeline.from_pretrained(
"stabilityai/stable-diffusion-2-depth",
torch_dtype=torch.float16,
).to("cuda")
```
이제 프롬프트를 파이프라인에 전달합니다. 특정 단어가 이미지 생성을 가이드 하는것을 방지하기 위해 `negative_prompt`를 전달할 수도 있습니다:
```python
url = "http://images.cocodataset.org/val2017/000000039769.jpg"
init_image = Image.open(requests.get(url, stream=True).raw)
prompt = "two tigers"
n_prompt = "bad, deformed, ugly, bad anatomy"
image = pipe(prompt=prompt, image=init_image, negative_prompt=n_prompt, strength=0.7).images[0]
image
```
| Input | Output |
|---------------------------------------------------------------------------------|---------------------------------------------------------------------------------------------------------------------------------------|
| <img src="https://huggingface.co/datasets/huggingface/documentation-images/resolve/main/diffusers/coco-cats.png" width="500"/> | <img src="https://huggingface.co/datasets/huggingface/documentation-images/resolve/main/diffusers/depth2img-tigers.png" width="500"/> |
아래의 Spaces를 가지고 놀며 depth map이 있는 이미지와 없는 이미지의 차이가 있는지 확인해 보세요!
<iframe
src="https://radames-stable-diffusion-depth2img.hf.space"
frameborder="0"
width="850"
height="500"
></iframe>
| 0 |
hf_public_repos/diffusers/docs/source/ko | hf_public_repos/diffusers/docs/source/ko/using-diffusers/reusing_seeds.md | <!--Copyright 2023 The HuggingFace Team. All rights reserved.
Licensed under the Apache License, Version 2.0 (the "License"); you may not use this file except in compliance with
the License. You may obtain a copy of the License at
http://www.apache.org/licenses/LICENSE-2.0
Unless required by applicable law or agreed to in writing, software distributed under the License is distributed on
an "AS IS" BASIS, WITHOUT WARRANTIES OR CONDITIONS OF ANY KIND, either express or implied. See the License for the
specific language governing permissions and limitations under the License.
-->
# Deterministic(결정적) 생성을 통한 이미지 품질 개선
생성된 이미지의 품질을 개선하는 일반적인 방법은 *결정적 batch(배치) 생성*을 사용하는 것입니다. 이 방법은 이미지 batch(배치)를 생성하고 두 번째 추론 라운드에서 더 자세한 프롬프트와 함께 개선할 이미지 하나를 선택하는 것입니다. 핵심은 일괄 이미지 생성을 위해 파이프라인에 [`torch.Generator`](https://pytorch.org/docs/stable/generated/torch.Generator.html#generator) 목록을 전달하고, 각 `Generator`를 시드에 연결하여 이미지에 재사용할 수 있도록 하는 것입니다.
예를 들어 [`runwayml/stable-diffusion-v1-5`](runwayml/stable-diffusion-v1-5)를 사용하여 다음 프롬프트의 여러 버전을 생성해 봅시다.
```py
prompt = "Labrador in the style of Vermeer"
```
(가능하다면) 파이프라인을 [`DiffusionPipeline.from_pretrained`]로 인스턴스화하여 GPU에 배치합니다.
```python
>>> from diffusers import DiffusionPipeline
>>> pipe = DiffusionPipeline.from_pretrained("runwayml/stable-diffusion-v1-5", torch_dtype=torch.float16)
>>> pipe = pipe.to("cuda")
```
이제 네 개의 서로 다른 `Generator`를 정의하고 각 `Generator`에 시드(`0` ~ `3`)를 할당하여 나중에 특정 이미지에 대해 `Generator`를 재사용할 수 있도록 합니다.
```python
>>> import torch
>>> generator = [torch.Generator(device="cuda").manual_seed(i) for i in range(4)]
```
이미지를 생성하고 살펴봅니다.
```python
>>> images = pipe(prompt, generator=generator, num_images_per_prompt=4).images
>>> images
```
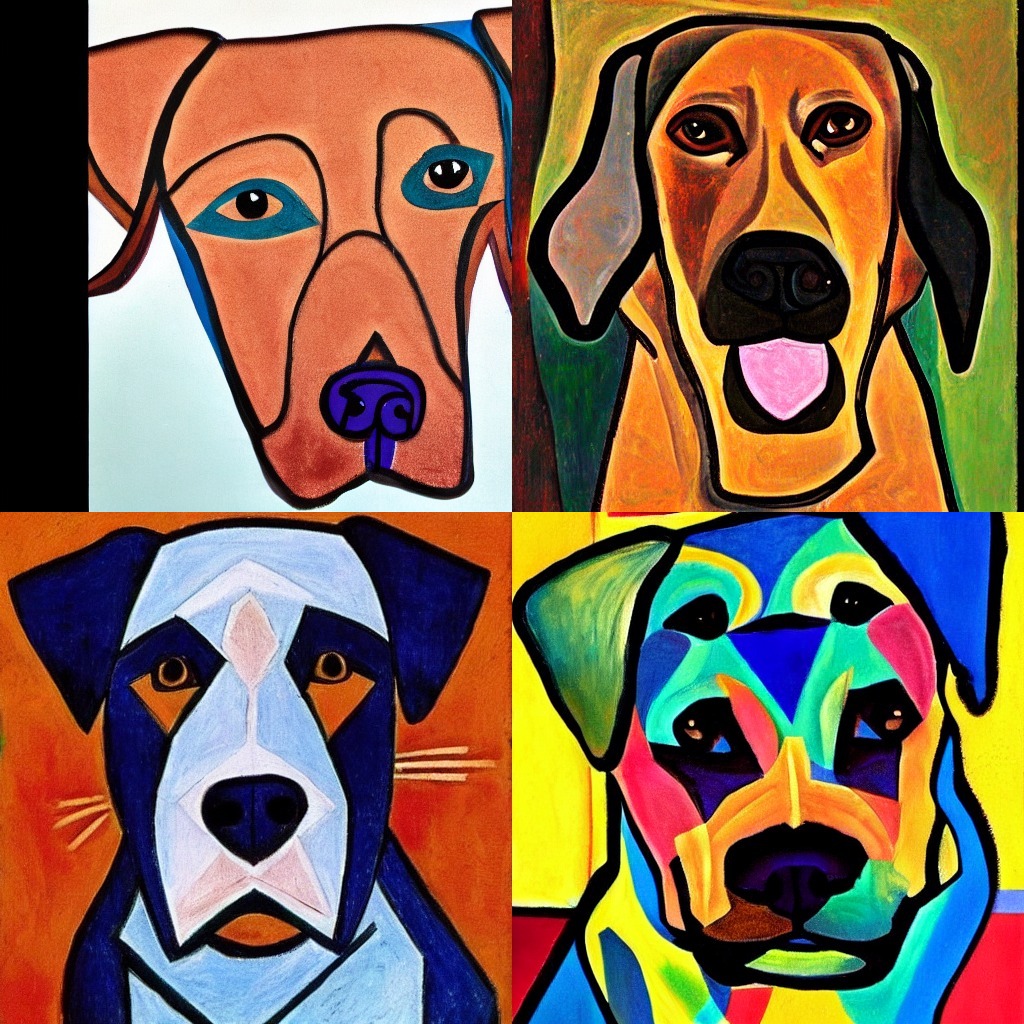
이 예제에서는 첫 번째 이미지를 개선했지만 실제로는 원하는 모든 이미지를 사용할 수 있습니다(심지어 두 개의 눈이 있는 이미지도!). 첫 번째 이미지에서는 시드가 '0'인 '생성기'를 사용했기 때문에 두 번째 추론 라운드에서는 이 '생성기'를 재사용할 것입니다. 이미지의 품질을 개선하려면 프롬프트에 몇 가지 텍스트를 추가합니다:
```python
prompt = [prompt + t for t in [", highly realistic", ", artsy", ", trending", ", colorful"]]
generator = [torch.Generator(device="cuda").manual_seed(0) for i in range(4)]
```
시드가 `0`인 제너레이터 4개를 생성하고, 이전 라운드의 첫 번째 이미지처럼 보이는 다른 이미지 batch(배치)를 생성합니다!
```python
>>> images = pipe(prompt, generator=generator).images
>>> images
```
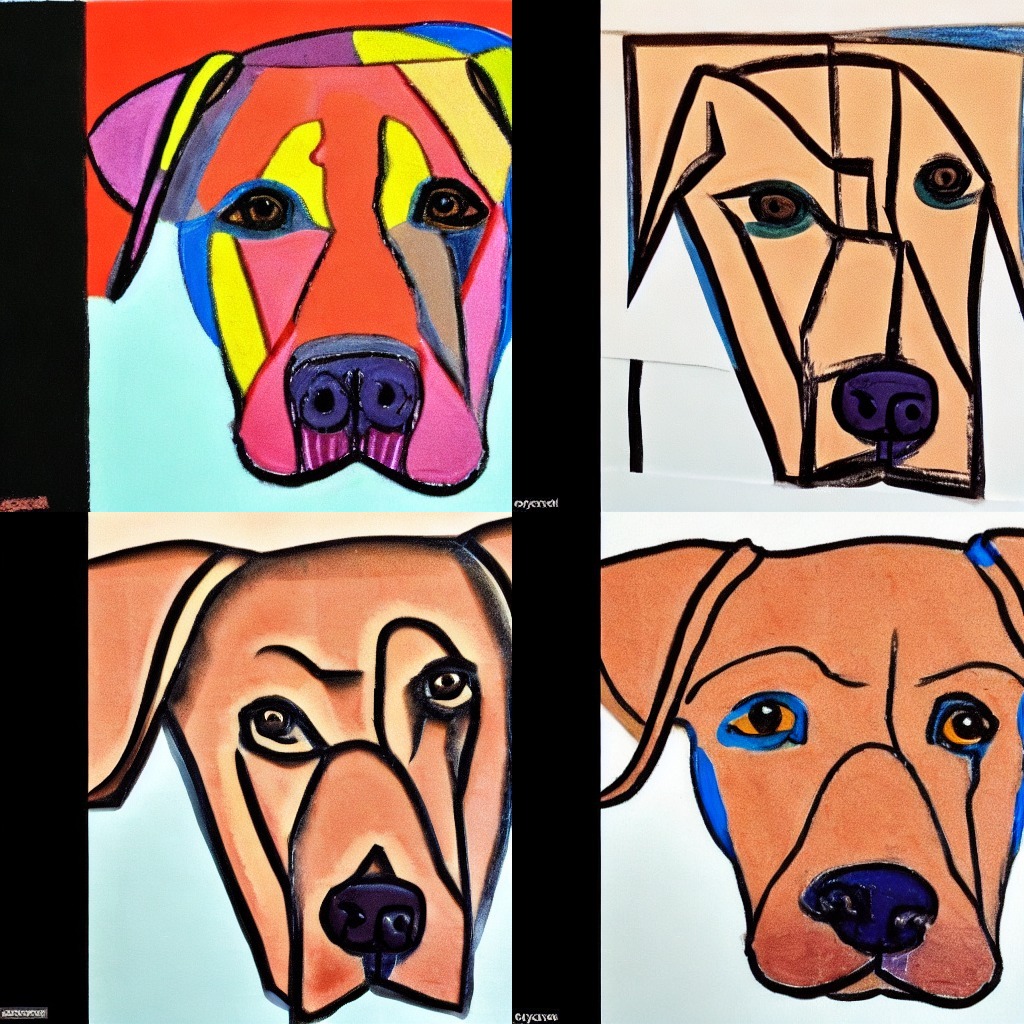
| 0 |
hf_public_repos/diffusers/docs/source/ko | hf_public_repos/diffusers/docs/source/ko/using-diffusers/unconditional_image_generation.md | <!--Copyright 2023 The HuggingFace Team. All rights reserved.
Licensed under the Apache License, Version 2.0 (the "License"); you may not use this file except in compliance with
the License. You may obtain a copy of the License at
http://www.apache.org/licenses/LICENSE-2.0
Unless required by applicable law or agreed to in writing, software distributed under the License is distributed on
an "AS IS" BASIS, WITHOUT WARRANTIES OR CONDITIONS OF ANY KIND, either express or implied. See the License for the
specific language governing permissions and limitations under the License.
-->
# Unconditional 이미지 생성
[[Colab에서 열기]]
Unconditional 이미지 생성은 비교적 간단한 작업입니다. 모델이 텍스트나 이미지와 같은 추가 조건 없이 이미 학습된 학습 데이터와 유사한 이미지만 생성합니다.
['DiffusionPipeline']은 추론을 위해 미리 학습된 diffusion 시스템을 사용하는 가장 쉬운 방법입니다.
먼저 ['DiffusionPipeline']의 인스턴스를 생성하고 다운로드할 파이프라인의 [체크포인트](https://huggingface.co/models?library=diffusers&sort=downloads)를 지정합니다. 허브의 🧨 diffusion 체크포인트 중 하나를 사용할 수 있습니다(사용할 체크포인트는 나비 이미지를 생성합니다).
<Tip>
💡 나만의 unconditional 이미지 생성 모델을 학습시키고 싶으신가요? 학습 가이드를 살펴보고 나만의 이미지를 생성하는 방법을 알아보세요.
</Tip>
이 가이드에서는 unconditional 이미지 생성에 ['DiffusionPipeline']과 [DDPM](https://arxiv.org/abs/2006.11239)을 사용합니다:
```python
>>> from diffusers import DiffusionPipeline
>>> generator = DiffusionPipeline.from_pretrained("anton-l/ddpm-butterflies-128")
```
[diffusion 파이프라인]은 모든 모델링, 토큰화, 스케줄링 구성 요소를 다운로드하고 캐시합니다. 이 모델은 약 14억 개의 파라미터로 구성되어 있기 때문에 GPU에서 실행할 것을 강력히 권장합니다. PyTorch에서와 마찬가지로 제너레이터 객체를 GPU로 옮길 수 있습니다:
```python
>>> generator.to("cuda")
```
이제 제너레이터를 사용하여 이미지를 생성할 수 있습니다:
```python
>>> image = generator().images[0]
```
출력은 기본적으로 [PIL.Image](https://pillow.readthedocs.io/en/stable/reference/Image.html?highlight=image#the-image-class) 객체로 감싸집니다.
다음을 호출하여 이미지를 저장할 수 있습니다:
```python
>>> image.save("generated_image.png")
```
아래 스페이스(데모 링크)를 이용해 보고, 추론 단계의 매개변수를 자유롭게 조절하여 이미지 품질에 어떤 영향을 미치는지 확인해 보세요!
<iframe src="https://stevhliu-ddpm-butterflies-128.hf.space" frameborder="0" width="850" height="500"></iframe> | 0 |
hf_public_repos/diffusers/docs/source/ko | hf_public_repos/diffusers/docs/source/ko/using-diffusers/custom_pipeline_examples.md | <!--Copyright 2023 The HuggingFace Team. All rights reserved.
Licensed under the Apache License, Version 2.0 (the "License"); you may not use this file except in compliance with
the License. You may obtain a copy of the License at
http://www.apache.org/licenses/LICENSE-2.0
Unless required by applicable law or agreed to in writing, software distributed under the License is distributed on
an "AS IS" BASIS, WITHOUT WARRANTIES OR CONDITIONS OF ANY KIND, either express or implied. See the License for the
specific language governing permissions and limitations under the License.
-->
# 커뮤니티 파이프라인
> **커뮤니티 파이프라인에 대한 자세한 내용은 [이 이슈](https://github.com/huggingface/diffusers/issues/841)를 참조하세요.
**커뮤니티** 예제는 커뮤니티에서 추가한 추론 및 훈련 예제로 구성되어 있습니다.
다음 표를 참조하여 모든 커뮤니티 예제에 대한 개요를 확인하시기 바랍니다. **코드 예제**를 클릭하면 복사하여 붙여넣기할 수 있는 코드 예제를 확인할 수 있습니다.
커뮤니티가 예상대로 작동하지 않는 경우 이슈를 개설하고 작성자에게 핑을 보내주세요.
| 예 | 설명 | 코드 예제 | 콜랩 |저자 |
|:---------------------------------------|:------------------------------------------------------------------------------------------------------------------------------------------------------------------------------------------------------------------------------------------------------------------------------------------------------------------------------------------------------------------------------------------------------------------------------------------------------------------------------------------------|:---------------------------------------------------------------------------------|:-------------------------------------------------------------------------------------------------------------------------------------------------------------------------------------------------------------------|-----------------------------------------------------------:|
| CLIP Guided Stable Diffusion | CLIP 가이드 기반의 Stable Diffusion으로 텍스트에서 이미지로 생성하기 | [CLIP Guided Stable Diffusion](#clip-guided-stable-diffusion) | [](https://colab.research.google.com/github/huggingface/notebooks/blob/main/diffusers/CLIP_Guided_Stable_diffusion_with_diffusers.ipynb) | [Suraj Patil](https://github.com/patil-suraj/) |
| One Step U-Net (Dummy) | 커뮤니티 파이프라인을 어떻게 사용해야 하는지에 대한 예시(참고 https://github.com/huggingface/diffusers/issues/841) | [One Step U-Net](#one-step-unet) | - | [Patrick von Platen](https://github.com/patrickvonplaten/) |
| Stable Diffusion Interpolation | 서로 다른 프롬프트/시드 간 Stable Diffusion의 latent space 보간 | [Stable Diffusion Interpolation](#stable-diffusion-interpolation) | - | [Nate Raw](https://github.com/nateraw/) |
| Stable Diffusion Mega | 모든 기능을 갖춘 **하나의** Stable Diffusion 파이프라인 [Text2Image](https://github.com/huggingface/diffusers/blob/main/src/diffusers/pipelines/stable_diffusion/pipeline_stable_diffusion.py), [Image2Image](https://github.com/huggingface/diffusers/blob/main/src/diffusers/pipelines/stable_diffusion/pipeline_stable_diffusion_img2img.py) and [Inpainting](https://github.com/huggingface/diffusers/blob/main/src/diffusers/pipelines/stable_diffusion/pipeline_stable_diffusion_inpaint.py) | [Stable Diffusion Mega](#stable-diffusion-mega) | - | [Patrick von Platen](https://github.com/patrickvonplaten/) |
| Long Prompt Weighting Stable Diffusion | 토큰 길이 제한이 없고 프롬프트에서 파싱 가중치 지원을 하는 **하나의** Stable Diffusion 파이프라인, | [Long Prompt Weighting Stable Diffusion](#long-prompt-weighting-stable-diffusion) |- | [SkyTNT](https://github.com/SkyTNT) |
| Speech to Image | 자동 음성 인식을 사용하여 텍스트를 작성하고 Stable Diffusion을 사용하여 이미지를 생성합니다. | [Speech to Image](#speech-to-image) | - | [Mikail Duzenli](https://github.com/MikailINTech) |
커스텀 파이프라인을 불러오려면 `diffusers/examples/community`에 있는 파일 중 하나로서 `custom_pipeline` 인수를 `DiffusionPipeline`에 전달하기만 하면 됩니다. 자신만의 파이프라인이 있는 PR을 보내주시면 빠르게 병합해드리겠습니다.
```py
pipe = DiffusionPipeline.from_pretrained(
"CompVis/stable-diffusion-v1-4", custom_pipeline="filename_in_the_community_folder"
)
```
## 사용 예시
### CLIP 가이드 기반의 Stable Diffusion
모든 노이즈 제거 단계에서 추가 CLIP 모델을 통해 Stable Diffusion을 가이드함으로써 CLIP 모델 기반의 Stable Diffusion은 보다 더 사실적인 이미지를 생성을 할 수 있습니다.
다음 코드는 약 12GB의 GPU RAM이 필요합니다.
```python
from diffusers import DiffusionPipeline
from transformers import CLIPImageProcessor, CLIPModel
import torch
feature_extractor = CLIPImageProcessor.from_pretrained("laion/CLIP-ViT-B-32-laion2B-s34B-b79K")
clip_model = CLIPModel.from_pretrained("laion/CLIP-ViT-B-32-laion2B-s34B-b79K", torch_dtype=torch.float16)
guided_pipeline = DiffusionPipeline.from_pretrained(
"CompVis/stable-diffusion-v1-4",
custom_pipeline="clip_guided_stable_diffusion",
clip_model=clip_model,
feature_extractor=feature_extractor,
torch_dtype=torch.float16,
)
guided_pipeline.enable_attention_slicing()
guided_pipeline = guided_pipeline.to("cuda")
prompt = "fantasy book cover, full moon, fantasy forest landscape, golden vector elements, fantasy magic, dark light night, intricate, elegant, sharp focus, illustration, highly detailed, digital painting, concept art, matte, art by WLOP and Artgerm and Albert Bierstadt, masterpiece"
generator = torch.Generator(device="cuda").manual_seed(0)
images = []
for i in range(4):
image = guided_pipeline(
prompt,
num_inference_steps=50,
guidance_scale=7.5,
clip_guidance_scale=100,
num_cutouts=4,
use_cutouts=False,
generator=generator,
).images[0]
images.append(image)
# 이미지 로컬에 저장하기
for i, img in enumerate(images):
img.save(f"./clip_guided_sd/image_{i}.png")
```
이미지` 목록에는 로컬에 저장하거나 구글 콜랩에 직접 표시할 수 있는 PIL 이미지 목록이 포함되어 있습니다. 생성된 이미지는 기본적으로 안정적인 확산을 사용하는 것보다 품질이 높은 경향이 있습니다. 예를 들어 위의 스크립트는 다음과 같은 이미지를 생성합니다:
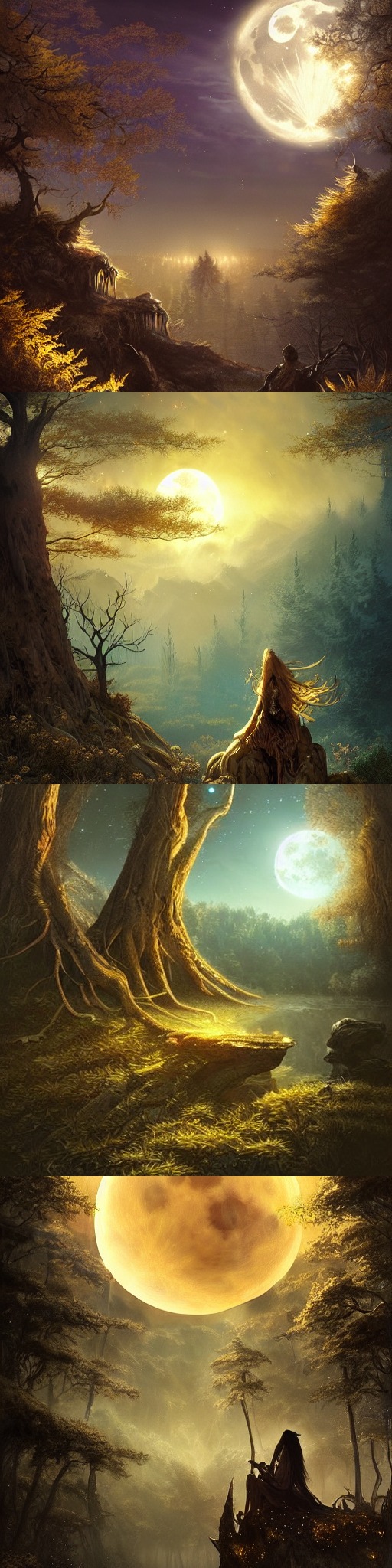.
### One Step Unet
예시 "one-step-unet"는 다음과 같이 실행할 수 있습니다.
```python
from diffusers import DiffusionPipeline
pipe = DiffusionPipeline.from_pretrained("google/ddpm-cifar10-32", custom_pipeline="one_step_unet")
pipe()
```
**참고**: 이 커뮤니티 파이프라인은 기능으로 유용하지 않으며 커뮤니티 파이프라인을 추가할 수 있는 방법의 예시일 뿐입니다(https://github.com/huggingface/diffusers/issues/841 참조).
### Stable Diffusion Interpolation
다음 코드는 최소 8GB VRAM의 GPU에서 실행할 수 있으며 약 5분 정도 소요됩니다.
```python
from diffusers import DiffusionPipeline
import torch
pipe = DiffusionPipeline.from_pretrained(
"CompVis/stable-diffusion-v1-4",
torch_dtype=torch.float16,
safety_checker=None, # Very important for videos...lots of false positives while interpolating
custom_pipeline="interpolate_stable_diffusion",
).to("cuda")
pipe.enable_attention_slicing()
frame_filepaths = pipe.walk(
prompts=["a dog", "a cat", "a horse"],
seeds=[42, 1337, 1234],
num_interpolation_steps=16,
output_dir="./dreams",
batch_size=4,
height=512,
width=512,
guidance_scale=8.5,
num_inference_steps=50,
)
```
walk(...)` 함수의 출력은 `output_dir`에 정의된 대로 폴더에 저장된 이미지 목록을 반환합니다. 이 이미지를 사용하여 안정적으로 확산되는 동영상을 만들 수 있습니다.
> 안정된 확산을 이용한 동영상 제작 방법과 더 많은 기능에 대한 자세한 내용은 https://github.com/nateraw/stable-diffusion-videos 에서 확인하시기 바랍니다.
### Stable Diffusion Mega
The Stable Diffusion Mega 파이프라인을 사용하면 Stable Diffusion 파이프라인의 주요 사용 사례를 단일 클래스에서 사용할 수 있습니다.
```python
#!/usr/bin/env python3
from diffusers import DiffusionPipeline
import PIL
import requests
from io import BytesIO
import torch
def download_image(url):
response = requests.get(url)
return PIL.Image.open(BytesIO(response.content)).convert("RGB")
pipe = DiffusionPipeline.from_pretrained(
"CompVis/stable-diffusion-v1-4",
custom_pipeline="stable_diffusion_mega",
torch_dtype=torch.float16,
)
pipe.to("cuda")
pipe.enable_attention_slicing()
### Text-to-Image
images = pipe.text2img("An astronaut riding a horse").images
### Image-to-Image
init_image = download_image(
"https://raw.githubusercontent.com/CompVis/stable-diffusion/main/assets/stable-samples/img2img/sketch-mountains-input.jpg"
)
prompt = "A fantasy landscape, trending on artstation"
images = pipe.img2img(prompt=prompt, image=init_image, strength=0.75, guidance_scale=7.5).images
### Inpainting
img_url = "https://raw.githubusercontent.com/CompVis/latent-diffusion/main/data/inpainting_examples/overture-creations-5sI6fQgYIuo.png"
mask_url = "https://raw.githubusercontent.com/CompVis/latent-diffusion/main/data/inpainting_examples/overture-creations-5sI6fQgYIuo_mask.png"
init_image = download_image(img_url).resize((512, 512))
mask_image = download_image(mask_url).resize((512, 512))
prompt = "a cat sitting on a bench"
images = pipe.inpaint(prompt=prompt, image=init_image, mask_image=mask_image, strength=0.75).images
```
위에 표시된 것처럼 하나의 파이프라인에서 '텍스트-이미지 변환', '이미지-이미지 변환', '인페인팅'을 모두 실행할 수 있습니다.
### Long Prompt Weighting Stable Diffusion
파이프라인을 사용하면 77개의 토큰 길이 제한 없이 프롬프트를 입력할 수 있습니다. 또한 "()"를 사용하여 단어 가중치를 높이거나 "[]"를 사용하여 단어 가중치를 낮출 수 있습니다.
또한 파이프라인을 사용하면 단일 클래스에서 Stable Diffusion 파이프라인의 주요 사용 사례를 사용할 수 있습니다.
#### pytorch
```python
from diffusers import DiffusionPipeline
import torch
pipe = DiffusionPipeline.from_pretrained(
"hakurei/waifu-diffusion", custom_pipeline="lpw_stable_diffusion", torch_dtype=torch.float16
)
pipe = pipe.to("cuda")
prompt = "best_quality (1girl:1.3) bow bride brown_hair closed_mouth frilled_bow frilled_hair_tubes frills (full_body:1.3) fox_ear hair_bow hair_tubes happy hood japanese_clothes kimono long_sleeves red_bow smile solo tabi uchikake white_kimono wide_sleeves cherry_blossoms"
neg_prompt = "lowres, bad_anatomy, error_body, error_hair, error_arm, error_hands, bad_hands, error_fingers, bad_fingers, missing_fingers, error_legs, bad_legs, multiple_legs, missing_legs, error_lighting, error_shadow, error_reflection, text, error, extra_digit, fewer_digits, cropped, worst_quality, low_quality, normal_quality, jpeg_artifacts, signature, watermark, username, blurry"
pipe.text2img(prompt, negative_prompt=neg_prompt, width=512, height=512, max_embeddings_multiples=3).images[0]
```
#### onnxruntime
```python
from diffusers import DiffusionPipeline
import torch
pipe = DiffusionPipeline.from_pretrained(
"CompVis/stable-diffusion-v1-4",
custom_pipeline="lpw_stable_diffusion_onnx",
revision="onnx",
provider="CUDAExecutionProvider",
)
prompt = "a photo of an astronaut riding a horse on mars, best quality"
neg_prompt = "lowres, bad anatomy, error body, error hair, error arm, error hands, bad hands, error fingers, bad fingers, missing fingers, error legs, bad legs, multiple legs, missing legs, error lighting, error shadow, error reflection, text, error, extra digit, fewer digits, cropped, worst quality, low quality, normal quality, jpeg artifacts, signature, watermark, username, blurry"
pipe.text2img(prompt, negative_prompt=neg_prompt, width=512, height=512, max_embeddings_multiples=3).images[0]
```
토큰 인덱스 시퀀스 길이가 이 모델에 지정된 최대 시퀀스 길이보다 길면(*** > 77). 이 시퀀스를 모델에서 실행하면 인덱싱 오류가 발생합니다`. 정상적인 현상이니 걱정하지 마세요.
### Speech to Image
다음 코드는 사전학습된 OpenAI whisper-small과 Stable Diffusion을 사용하여 오디오 샘플에서 이미지를 생성할 수 있습니다.
```Python
import torch
import matplotlib.pyplot as plt
from datasets import load_dataset
from diffusers import DiffusionPipeline
from transformers import (
WhisperForConditionalGeneration,
WhisperProcessor,
)
device = "cuda" if torch.cuda.is_available() else "cpu"
ds = load_dataset("hf-internal-testing/librispeech_asr_dummy", "clean", split="validation")
audio_sample = ds[3]
text = audio_sample["text"].lower()
speech_data = audio_sample["audio"]["array"]
model = WhisperForConditionalGeneration.from_pretrained("openai/whisper-small").to(device)
processor = WhisperProcessor.from_pretrained("openai/whisper-small")
diffuser_pipeline = DiffusionPipeline.from_pretrained(
"CompVis/stable-diffusion-v1-4",
custom_pipeline="speech_to_image_diffusion",
speech_model=model,
speech_processor=processor,
torch_dtype=torch.float16,
)
diffuser_pipeline.enable_attention_slicing()
diffuser_pipeline = diffuser_pipeline.to(device)
output = diffuser_pipeline(speech_data)
plt.imshow(output.images[0])
```
위 예시는 다음의 결과 이미지를 보입니다.
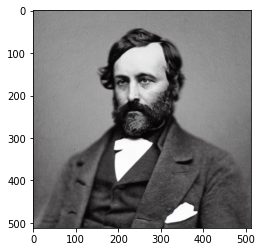 | 0 |
hf_public_repos/diffusers/docs/source/ko | hf_public_repos/diffusers/docs/source/ko/using-diffusers/using_safetensors.md | # 세이프센서란 무엇인가요?
[세이프텐서](https://github.com/huggingface/safetensors)는 피클을 사용하는 파이토치를 사용하는 기존의 '.bin'과는 다른 형식입니다.
피클은 악의적인 파일이 임의의 코드를 실행할 수 있는 안전하지 않은 것으로 악명이 높습니다.
허브 자체에서 문제를 방지하기 위해 노력하고 있지만 만병통치약은 아닙니다.
세이프텐서의 가장 중요한 목표는 컴퓨터를 탈취할 수 없다는 의미에서 머신 러닝 모델 로딩을 *안전하게* 만드는 것입니다.
# 왜 세이프센서를 사용하나요?
**잘 알려지지 않은 모델을 사용하려는 경우, 그리고 파일의 출처가 확실하지 않은 경우 "안전성"이 하나의 이유가 될 수 있습니다.
그리고 두 번째 이유는 **로딩 속도**입니다. 세이프센서는 일반 피클 파일보다 훨씬 빠르게 모델을 훨씬 빠르게 로드할 수 있습니다. 모델을 전환하는 데 많은 시간을 소비하는 경우, 이는 엄청난 시간 절약이 가능합니다. | 0 |
hf_public_repos/diffusers/docs/source/ko | hf_public_repos/diffusers/docs/source/ko/using-diffusers/schedulers.md | <!--Copyright 2023 The HuggingFace Team. All rights reserved.
Licensed under the Apache License, Version 2.0 (the "License"); you may not use this file except in compliance with
the License. You may obtain a copy of the License at
http://www.apache.org/licenses/LICENSE-2.0
Unless required by applicable law or agreed to in writing, software distributed under the License is distributed on
an "AS IS" BASIS, WITHOUT WARRANTIES OR CONDITIONS OF ANY KIND, either express or implied. See the License for the
specific language governing permissions and limitations under the License.
-->
# 스케줄러
diffusion 파이프라인은 diffusion 모델, 스케줄러 등의 컴포넌트들로 구성됩니다. 그리고 파이프라인 안의 일부 컴포넌트를 다른 컴포넌트로 교체하는 식의 커스터마이징 역시 가능합니다. 이와 같은 컴포넌트 커스터마이징의 가장 대표적인 예시가 바로 [스케줄러](../api/schedulers/overview.md)를 교체하는 것입니다.
스케쥴러는 다음과 같이 diffusion 시스템의 전반적인 디노이징 프로세스를 정의합니다.
- 디노이징 스텝을 얼마나 가져가야 할까?
- 확률적으로(stochastic) 혹은 확정적으로(deterministic)?
- 디노이징 된 샘플을 찾아내기 위해 어떤 알고리즘을 사용해야 할까?
이러한 프로세스는 다소 난해하고, 디노이징 속도와 디노이징 퀄리티 사이의 트레이드 오프를 정의해야 하는 문제가 될 수 있습니다. 주어진 파이프라인에 어떤 스케줄러가 가장 적합한지를 정량적으로 판단하는 것은 매우 어려운 일입니다. 이로 인해 일단 해당 스케줄러를 직접 사용하여, 생성되는 이미지를 직접 눈으로 보며, 정성적으로 성능을 판단해보는 것이 추천되곤 합니다.
## 파이프라인 불러오기
먼저 스테이블 diffusion 파이프라인을 불러오도록 해보겠습니다. 물론 스테이블 diffusion을 사용하기 위해서는, 허깅페이스 허브에 등록된 사용자여야 하며, 관련 [라이센스](https://huggingface.co/runwayml/stable-diffusion-v1-5)에 동의해야 한다는 점을 잊지 말아주세요.
*역자 주: 다만, 현재 신규로 생성한 허깅페이스 계정에 대해서는 라이센스 동의를 요구하지 않는 것으로 보입니다!*
```python
from huggingface_hub import login
from diffusers import DiffusionPipeline
import torch
# first we need to login with our access token
login()
# Now we can download the pipeline
pipeline = DiffusionPipeline.from_pretrained("runwayml/stable-diffusion-v1-5", torch_dtype=torch.float16)
```
다음으로, GPU로 이동합니다.
```python
pipeline.to("cuda")
```
## 스케줄러 액세스
스케줄러는 언제나 파이프라인의 컴포넌트로서 존재하며, 일반적으로 파이프라인 인스턴스 내에 `scheduler`라는 이름의 속성(property)으로 정의되어 있습니다.
```python
pipeline.scheduler
```
**Output**:
```
PNDMScheduler {
"_class_name": "PNDMScheduler",
"_diffusers_version": "0.8.0.dev0",
"beta_end": 0.012,
"beta_schedule": "scaled_linear",
"beta_start": 0.00085,
"clip_sample": false,
"num_train_timesteps": 1000,
"set_alpha_to_one": false,
"skip_prk_steps": true,
"steps_offset": 1,
"trained_betas": null
}
```
출력 결과를 통해, 우리는 해당 스케줄러가 [`PNDMScheduler`]의 인스턴스라는 것을 알 수 있습니다. 이제 [`PNDMScheduler`]와 다른 스케줄러들의 성능을 비교해보도록 하겠습니다. 먼저 테스트에 사용할 프롬프트를 다음과 같이 정의해보도록 하겠습니다.
```python
prompt = "A photograph of an astronaut riding a horse on Mars, high resolution, high definition."
```
다음으로 유사한 이미지 생성을 보장하기 위해서, 다음과 같이 랜덤시드를 고정해주도록 하겠습니다.
```python
generator = torch.Generator(device="cuda").manual_seed(8)
image = pipeline(prompt, generator=generator).images[0]
image
```
<p align="center">
<br>
<img src="https://huggingface.co/datasets/patrickvonplaten/images/resolve/main/diffusers_docs/astronaut_pndm.png" width="400"/>
<br>
</p>
## 스케줄러 교체하기
다음으로 파이프라인의 스케줄러를 다른 스케줄러로 교체하는 방법에 대해 알아보겠습니다. 모든 스케줄러는 [`SchedulerMixin.compatibles`]라는 속성(property)을 갖고 있습니다. 해당 속성은 **호환 가능한** 스케줄러들에 대한 정보를 담고 있습니다.
```python
pipeline.scheduler.compatibles
```
**Output**:
```
[diffusers.schedulers.scheduling_lms_discrete.LMSDiscreteScheduler,
diffusers.schedulers.scheduling_ddim.DDIMScheduler,
diffusers.schedulers.scheduling_dpmsolver_multistep.DPMSolverMultistepScheduler,
diffusers.schedulers.scheduling_euler_discrete.EulerDiscreteScheduler,
diffusers.schedulers.scheduling_pndm.PNDMScheduler,
diffusers.schedulers.scheduling_ddpm.DDPMScheduler,
diffusers.schedulers.scheduling_euler_ancestral_discrete.EulerAncestralDiscreteScheduler]
```
호환되는 스케줄러들을 살펴보면 아래와 같습니다.
- [`LMSDiscreteScheduler`],
- [`DDIMScheduler`],
- [`DPMSolverMultistepScheduler`],
- [`EulerDiscreteScheduler`],
- [`PNDMScheduler`],
- [`DDPMScheduler`],
- [`EulerAncestralDiscreteScheduler`].
앞서 정의했던 프롬프트를 사용해서 각각의 스케줄러들을 비교해보도록 하겠습니다.
먼저 파이프라인 안의 스케줄러를 바꾸기 위해 [`ConfigMixin.config`] 속성과 [`ConfigMixin.from_config`] 메서드를 활용해보려고 합니다.
```python
pipeline.scheduler.config
```
**Output**:
```
FrozenDict([('num_train_timesteps', 1000),
('beta_start', 0.00085),
('beta_end', 0.012),
('beta_schedule', 'scaled_linear'),
('trained_betas', None),
('skip_prk_steps', True),
('set_alpha_to_one', False),
('steps_offset', 1),
('_class_name', 'PNDMScheduler'),
('_diffusers_version', '0.8.0.dev0'),
('clip_sample', False)])
```
기존 스케줄러의 config를 호환 가능한 다른 스케줄러에 이식하는 것 역시 가능합니다.
다음 예시는 기존 스케줄러(`pipeline.scheduler`)를 다른 종류의 스케줄러(`DDIMScheduler`)로 바꾸는 코드입니다. 기존 스케줄러가 갖고 있던 config를 `.from_config` 메서드의 인자로 전달하는 것을 확인할 수 있습니다.
```python
from diffusers import DDIMScheduler
pipeline.scheduler = DDIMScheduler.from_config(pipeline.scheduler.config)
```
이제 파이프라인을 실행해서 두 스케줄러 사이의 생성된 이미지의 퀄리티를 비교해봅시다.
```python
generator = torch.Generator(device="cuda").manual_seed(8)
image = pipeline(prompt, generator=generator).images[0]
image
```
<p align="center">
<br>
<img src="https://huggingface.co/datasets/patrickvonplaten/images/resolve/main/diffusers_docs/astronaut_ddim.png" width="400"/>
<br>
</p>
## 스케줄러들 비교해보기
지금까지는 [`PNDMScheduler`]와 [`DDIMScheduler`] 스케줄러를 실행해보았습니다. 아직 비교해볼 스케줄러들이 더 많이 남아있으니 계속 비교해보도록 하겠습니다.
[`LMSDiscreteScheduler`]을 일반적으로 더 좋은 결과를 보여줍니다.
```python
from diffusers import LMSDiscreteScheduler
pipeline.scheduler = LMSDiscreteScheduler.from_config(pipeline.scheduler.config)
generator = torch.Generator(device="cuda").manual_seed(8)
image = pipeline(prompt, generator=generator).images[0]
image
```
<p align="center">
<br>
<img src="https://huggingface.co/datasets/patrickvonplaten/images/resolve/main/diffusers_docs/astronaut_lms.png" width="400"/>
<br>
</p>
[`EulerDiscreteScheduler`]와 [`EulerAncestralDiscreteScheduler`] 고작 30번의 inference step만으로도 높은 퀄리티의 이미지를 생성하는 것을 알 수 있습니다.
```python
from diffusers import EulerDiscreteScheduler
pipeline.scheduler = EulerDiscreteScheduler.from_config(pipeline.scheduler.config)
generator = torch.Generator(device="cuda").manual_seed(8)
image = pipeline(prompt, generator=generator, num_inference_steps=30).images[0]
image
```
<p align="center">
<br>
<img src="https://huggingface.co/datasets/patrickvonplaten/images/resolve/main/diffusers_docs/astronaut_euler_discrete.png" width="400"/>
<br>
</p>
```python
from diffusers import EulerAncestralDiscreteScheduler
pipeline.scheduler = EulerAncestralDiscreteScheduler.from_config(pipeline.scheduler.config)
generator = torch.Generator(device="cuda").manual_seed(8)
image = pipeline(prompt, generator=generator, num_inference_steps=30).images[0]
image
```
<p align="center">
<br>
<img src="https://huggingface.co/datasets/patrickvonplaten/images/resolve/main/diffusers_docs/astronaut_euler_ancestral.png" width="400"/>
<br>
</p>
지금 이 문서를 작성하는 현시점 기준에선, [`DPMSolverMultistepScheduler`]가 시간 대비 가장 좋은 품질의 이미지를 생성하는 것 같습니다. 20번 정도의 스텝만으로도 실행될 수 있습니다.
```python
from diffusers import DPMSolverMultistepScheduler
pipeline.scheduler = DPMSolverMultistepScheduler.from_config(pipeline.scheduler.config)
generator = torch.Generator(device="cuda").manual_seed(8)
image = pipeline(prompt, generator=generator, num_inference_steps=20).images[0]
image
```
<p align="center">
<br>
<img src="https://huggingface.co/datasets/patrickvonplaten/images/resolve/main/diffusers_docs/astronaut_dpm.png" width="400"/>
<br>
</p>
보시다시피 생성된 이미지들은 매우 비슷하고, 비슷한 퀄리티를 보이는 것 같습니다. 실제로 어떤 스케줄러를 선택할 것인가는 종종 특정 이용 사례에 기반해서 결정되곤 합니다. 결국 여러 종류의 스케줄러를 직접 실행시켜보고 눈으로 직접 비교해서 판단하는 게 좋은 선택일 것 같습니다.
## Flax에서 스케줄러 교체하기
JAX/Flax 사용자인 경우 기본 파이프라인 스케줄러를 변경할 수도 있습니다. 다음은 Flax Stable Diffusion 파이프라인과 초고속 [DDPM-Solver++ 스케줄러를](../api/schedulers/multistep_dpm_solver) 사용하여 추론을 실행하는 방법에 대한 예시입니다 .
```Python
import jax
import numpy as np
from flax.jax_utils import replicate
from flax.training.common_utils import shard
from diffusers import FlaxStableDiffusionPipeline, FlaxDPMSolverMultistepScheduler
model_id = "runwayml/stable-diffusion-v1-5"
scheduler, scheduler_state = FlaxDPMSolverMultistepScheduler.from_pretrained(
model_id,
subfolder="scheduler"
)
pipeline, params = FlaxStableDiffusionPipeline.from_pretrained(
model_id,
scheduler=scheduler,
revision="bf16",
dtype=jax.numpy.bfloat16,
)
params["scheduler"] = scheduler_state
# Generate 1 image per parallel device (8 on TPUv2-8 or TPUv3-8)
prompt = "a photo of an astronaut riding a horse on mars"
num_samples = jax.device_count()
prompt_ids = pipeline.prepare_inputs([prompt] * num_samples)
prng_seed = jax.random.PRNGKey(0)
num_inference_steps = 25
# shard inputs and rng
params = replicate(params)
prng_seed = jax.random.split(prng_seed, jax.device_count())
prompt_ids = shard(prompt_ids)
images = pipeline(prompt_ids, params, prng_seed, num_inference_steps, jit=True).images
images = pipeline.numpy_to_pil(np.asarray(images.reshape((num_samples,) + images.shape[-3:])))
```
<Tip warning={true}>
다음 Flax 스케줄러는 *아직* Flax Stable Diffusion 파이프라인과 호환되지 않습니다.
- `FlaxLMSDiscreteScheduler`
- `FlaxDDPMScheduler`
</Tip>
| 0 |
hf_public_repos/diffusers/docs/source/ko | hf_public_repos/diffusers/docs/source/ko/using-diffusers/write_own_pipeline.md | <!--Copyright 2023 The HuggingFace Team. All rights reserved.
Licensed under the Apache License, Version 2.0 (the "License"); you may not use this file except in compliance with
the License. You may obtain a copy of the License at
http://www.apache.org/licenses/LICENSE-2.0
Unless required by applicable law or agreed to in writing, software distributed under the License is distributed on
an "AS IS" BASIS, WITHOUT WARRANTIES OR CONDITIONS OF ANY KIND, either express or implied. See the License for the
specific language governing permissions and limitations under the License.
-->
# 파이프라인, 모델 및 스케줄러 이해하기
[[colab에서 열기]]
🧨 Diffusers는 사용자 친화적이며 유연한 도구 상자로, 사용사례에 맞게 diffusion 시스템을 구축 할 수 있도록 설계되었습니다. 이 도구 상자의 핵심은 모델과 스케줄러입니다. [`DiffusionPipeline`]은 편의를 위해 이러한 구성 요소를 번들로 제공하지만, 파이프라인을 분리하고 모델과 스케줄러를 개별적으로 사용해 새로운 diffusion 시스템을 만들 수도 있습니다.
이 튜토리얼에서는 기본 파이프라인부터 시작해 Stable Diffusion 파이프라인까지 진행하며 모델과 스케줄러를 사용해 추론을 위한 diffusion 시스템을 조립하는 방법을 배웁니다.
## 기본 파이프라인 해체하기
파이프라인은 추론을 위해 모델을 실행하는 빠르고 쉬운 방법으로, 이미지를 생성하는 데 코드가 4줄 이상 필요하지 않습니다:
```py
>>> from diffusers import DDPMPipeline
>>> ddpm = DDPMPipeline.from_pretrained("google/ddpm-cat-256").to("cuda")
>>> image = ddpm(num_inference_steps=25).images[0]
>>> image
```
<div class="flex justify-center">
<img src="https://huggingface.co/datasets/huggingface/documentation-images/resolve/main/diffusers/ddpm-cat.png" alt="Image of cat created from DDPMPipeline"/>
</div>
정말 쉽습니다. 그런데 파이프라인은 어떻게 이렇게 할 수 있었을까요? 파이프라인을 세분화하여 내부에서 어떤 일이 일어나고 있는지 살펴보겠습니다.
위 예시에서 파이프라인에는 [`UNet2DModel`] 모델과 [`DDPMScheduler`]가 포함되어 있습니다. 파이프라인은 원하는 출력 크기의 랜덤 노이즈를 받아 모델을 여러번 통과시켜 이미지의 노이즈를 제거합니다. 각 timestep에서 모델은 *noise residual*을 예측하고 스케줄러는 이를 사용하여 노이즈가 적은 이미지를 예측합니다. 파이프라인은 지정된 추론 스텝수에 도달할 때까지 이 과정을 반복합니다.
모델과 스케줄러를 별도로 사용하여 파이프라인을 다시 생성하기 위해 자체적인 노이즈 제거 프로세스를 작성해 보겠습니다.
1. 모델과 스케줄러를 불러옵니다:
```py
>>> from diffusers import DDPMScheduler, UNet2DModel
>>> scheduler = DDPMScheduler.from_pretrained("google/ddpm-cat-256")
>>> model = UNet2DModel.from_pretrained("google/ddpm-cat-256").to("cuda")
```
2. 노이즈 제거 프로세스를 실행할 timestep 수를 설정합니다:
```py
>>> scheduler.set_timesteps(50)
```
3. 스케줄러의 timestep을 설정하면 균등한 간격의 구성 요소를 가진 텐서가 생성됩니다.(이 예시에서는 50개) 각 요소는 모델이 이미지의 노이즈를 제거하는 시간 간격에 해당합니다. 나중에 노이즈 제거 루프를 만들 때 이 텐서를 반복하여 이미지의 노이즈를 제거합니다:
```py
>>> scheduler.timesteps
tensor([980, 960, 940, 920, 900, 880, 860, 840, 820, 800, 780, 760, 740, 720,
700, 680, 660, 640, 620, 600, 580, 560, 540, 520, 500, 480, 460, 440,
420, 400, 380, 360, 340, 320, 300, 280, 260, 240, 220, 200, 180, 160,
140, 120, 100, 80, 60, 40, 20, 0])
```
4. 원하는 출력과 같은 모양을 가진 랜덤 노이즈를 생성합니다:
```py
>>> import torch
>>> sample_size = model.config.sample_size
>>> noise = torch.randn((1, 3, sample_size, sample_size)).to("cuda")
```
5. 이제 timestep을 반복하는 루프를 작성합니다. 각 timestep에서 모델은 [`UNet2DModel.forward`]를 통해 noisy residual을 반환합니다. 스케줄러의 [`~DDPMScheduler.step`] 메서드는 noisy residual, timestep, 그리고 입력을 받아 이전 timestep에서 이미지를 예측합니다. 이 출력은 노이즈 제거 루프의 모델에 대한 다음 입력이 되며, `timesteps` 배열의 끝에 도달할 때까지 반복됩니다.
```py
>>> input = noise
>>> for t in scheduler.timesteps:
... with torch.no_grad():
... noisy_residual = model(input, t).sample
... previous_noisy_sample = scheduler.step(noisy_residual, t, input).prev_sample
... input = previous_noisy_sample
```
이것이 전체 노이즈 제거 프로세스이며, 동일한 패턴을 사용해 모든 diffusion 시스템을 작성할 수 있습니다.
6. 마지막 단계는 노이즈가 제거된 출력을 이미지로 변환하는 것입니다:
```py
>>> from PIL import Image
>>> import numpy as np
>>> image = (input / 2 + 0.5).clamp(0, 1)
>>> image = image.cpu().permute(0, 2, 3, 1).numpy()[0]
>>> image = Image.fromarray((image * 255).round().astype("uint8"))
>>> image
```
다음 섹션에서는 여러분의 기술을 시험해보고 좀 더 복잡한 Stable Diffusion 파이프라인을 분석해 보겠습니다. 방법은 거의 동일합니다. 필요한 구성요소들을 초기화하고 timestep수를 설정하여 `timestep` 배열을 생성합니다. 노이즈 제거 루프에서 `timestep` 배열이 사용되며, 이 배열의 각 요소에 대해 모델은 노이즈가 적은 이미지를 예측합니다. 노이즈 제거 루프는 `timestep`을 반복하고 각 timestep에서 noise residual을 출력하고 스케줄러는 이를 사용하여 이전 timestep에서 노이즈가 덜한 이미지를 예측합니다. 이 프로세스는 `timestep` 배열의 끝에 도달할 때까지 반복됩니다.
한번 사용해 봅시다!
## Stable Diffusion 파이프라인 해체하기
Stable Diffusion 은 text-to-image *latent diffusion* 모델입니다. latent diffusion 모델이라고 불리는 이유는 실제 픽셀 공간 대신 이미지의 저차원의 표현으로 작업하기 때문이고, 메모리 효율이 더 높습니다. 인코더는 이미지를 더 작은 표현으로 압축하고, 디코더는 압축된 표현을 다시 이미지로 변환합니다. text-to-image 모델의 경우 텍스트 임베딩을 생성하기 위해 tokenizer와 인코더가 필요합니다. 이전 예제에서 이미 UNet 모델과 스케줄러가 필요하다는 것은 알고 계셨을 것입니다.
보시다시피, 이것은 UNet 모델만 포함된 DDPM 파이프라인보다 더 복잡합니다. Stable Diffusion 모델에는 세 개의 개별 사전학습된 모델이 있습니다.
<Tip>
💡 VAE, UNet 및 텍스트 인코더 모델의 작동방식에 대한 자세한 내용은 [How does Stable Diffusion work?](https://huggingface.co/blog/stable_diffusion#how-does-stable-diffusion-work) 블로그를 참조하세요.
</Tip>
이제 Stable Diffusion 파이프라인에 필요한 구성요소들이 무엇인지 알았으니, [`~ModelMixin.from_pretrained`] 메서드를 사용해 모든 구성요소를 불러옵니다. 사전학습된 체크포인트 [`runwayml/stable-diffusion-v1-5`](https://huggingface.co/runwayml/stable-diffusion-v1-5)에서 찾을 수 있으며, 각 구성요소들은 별도의 하위 폴더에 저장되어 있습니다:
```py
>>> from PIL import Image
>>> import torch
>>> from transformers import CLIPTextModel, CLIPTokenizer
>>> from diffusers import AutoencoderKL, UNet2DConditionModel, PNDMScheduler
>>> vae = AutoencoderKL.from_pretrained("CompVis/stable-diffusion-v1-4", subfolder="vae")
>>> tokenizer = CLIPTokenizer.from_pretrained("CompVis/stable-diffusion-v1-4", subfolder="tokenizer")
>>> text_encoder = CLIPTextModel.from_pretrained("CompVis/stable-diffusion-v1-4", subfolder="text_encoder")
>>> unet = UNet2DConditionModel.from_pretrained("CompVis/stable-diffusion-v1-4", subfolder="unet")
```
기본 [`PNDMScheduler`] 대신, [`UniPCMultistepScheduler`]로 교체하여 다른 스케줄러를 얼마나 쉽게 연결할 수 있는지 확인합니다:
```py
>>> from diffusers import UniPCMultistepScheduler
>>> scheduler = UniPCMultistepScheduler.from_pretrained("CompVis/stable-diffusion-v1-4", subfolder="scheduler")
```
추론 속도를 높이려면 스케줄러와 달리 학습 가능한 가중치가 있으므로 모델을 GPU로 옮기세요:
```py
>>> torch_device = "cuda"
>>> vae.to(torch_device)
>>> text_encoder.to(torch_device)
>>> unet.to(torch_device)
```
### 텍스트 임베딩 생성하기
다음 단계는 임베딩을 생성하기 위해 텍스트를 토큰화하는 것입니다. 이 텍스트는 UNet 모델에서 condition으로 사용되고 입력 프롬프트와 유사한 방향으로 diffusion 프로세스를 조정하는 데 사용됩니다.
<Tip>
💡 `guidance_scale` 매개변수는 이미지를 생성할 때 프롬프트에 얼마나 많은 가중치를 부여할지 결정합니다.
</Tip>
다른 프롬프트를 생성하고 싶다면 원하는 프롬프트를 자유롭게 선택하세요!
```py
>>> prompt = ["a photograph of an astronaut riding a horse"]
>>> height = 512 # Stable Diffusion의 기본 높이
>>> width = 512 # Stable Diffusion의 기본 너비
>>> num_inference_steps = 25 # 노이즈 제거 스텝 수
>>> guidance_scale = 7.5 # classifier-free guidance를 위한 scale
>>> generator = torch.manual_seed(0) # 초기 잠재 노이즈를 생성하는 seed generator
>>> batch_size = len(prompt)
```
텍스트를 토큰화하고 프롬프트에서 임베딩을 생성합니다:
```py
>>> text_input = tokenizer(
... prompt, padding="max_length", max_length=tokenizer.model_max_length, truncation=True, return_tensors="pt"
... )
>>> with torch.no_grad():
... text_embeddings = text_encoder(text_input.input_ids.to(torch_device))[0]
```
또한 패딩 토큰의 임베딩인 *unconditional 텍스트 임베딩*을 생성해야 합니다. 이 임베딩은 조건부 `text_embeddings`과 동일한 shape(`batch_size` 그리고 `seq_length`)을 가져야 합니다:
```py
>>> max_length = text_input.input_ids.shape[-1]
>>> uncond_input = tokenizer([""] * batch_size, padding="max_length", max_length=max_length, return_tensors="pt")
>>> uncond_embeddings = text_encoder(uncond_input.input_ids.to(torch_device))[0]
```
두번의 forward pass를 피하기 위해 conditional 임베딩과 unconditional 임베딩을 배치(batch)로 연결하겠습니다:
```py
>>> text_embeddings = torch.cat([uncond_embeddings, text_embeddings])
```
### 랜덤 노이즈 생성
그다음 diffusion 프로세스의 시작점으로 초기 랜덤 노이즈를 생성합니다. 이것이 이미지의 잠재적 표현이며 점차적으로 노이즈가 제거됩니다. 이 시점에서 `latent` 이미지는 최종 이미지 크기보다 작지만 나중에 모델이 이를 512x512 이미지 크기로 변환하므로 괜찮습니다.
<Tip>
💡 `vae` 모델에는 3개의 다운 샘플링 레이어가 있기 때문에 높이와 너비가 8로 나뉩니다. 다음을 실행하여 확인할 수 있습니다:
```py
2 ** (len(vae.config.block_out_channels) - 1) == 8
```
</Tip>
```py
>>> latents = torch.randn(
... (batch_size, unet.in_channels, height // 8, width // 8),
... generator=generator,
... )
>>> latents = latents.to(torch_device)
```
### 이미지 노이즈 제거
먼저 [`UniPCMultistepScheduler`]와 같은 향상된 스케줄러에 필요한 노이즈 스케일 값인 초기 노이즈 분포 *sigma* 로 입력을 스케일링 하는 것부터 시작합니다:
```py
>>> latents = latents * scheduler.init_noise_sigma
```
마지막 단계는 `latent`의 순수한 노이즈를 점진적으로 프롬프트에 설명된 이미지로 변환하는 노이즈 제거 루프를 생성하는 것입니다. 노이즈 제거 루프는 세 가지 작업을 수행해야 한다는 점을 기억하세요:
1. 노이즈 제거 중에 사용할 스케줄러의 timesteps를 설정합니다.
2. timestep을 따라 반복합니다.
3. 각 timestep에서 UNet 모델을 호출하여 noise residual을 예측하고 스케줄러에 전달하여 이전 노이즈 샘플을 계산합니다.
```py
>>> from tqdm.auto import tqdm
>>> scheduler.set_timesteps(num_inference_steps)
>>> for t in tqdm(scheduler.timesteps):
... # classifier-free guidance를 수행하는 경우 두번의 forward pass를 수행하지 않도록 latent를 확장.
... latent_model_input = torch.cat([latents] * 2)
... latent_model_input = scheduler.scale_model_input(latent_model_input, timestep=t)
... # noise residual 예측
... with torch.no_grad():
... noise_pred = unet(latent_model_input, t, encoder_hidden_states=text_embeddings).sample
... # guidance 수행
... noise_pred_uncond, noise_pred_text = noise_pred.chunk(2)
... noise_pred = noise_pred_uncond + guidance_scale * (noise_pred_text - noise_pred_uncond)
... # 이전 노이즈 샘플을 계산 x_t -> x_t-1
... latents = scheduler.step(noise_pred, t, latents).prev_sample
```
### 이미지 디코딩
마지막 단계는 `vae`를 이용하여 잠재 표현을 이미지로 디코딩하고 `sample`과 함께 디코딩된 출력을 얻는 것입니다:
```py
# latent를 스케일링하고 vae로 이미지 디코딩
latents = 1 / 0.18215 * latents
with torch.no_grad():
image = vae.decode(latents).sample
```
마지막으로 이미지를 `PIL.Image`로 변환하면 생성된 이미지를 확인할 수 있습니다!
```py
>>> image = (image / 2 + 0.5).clamp(0, 1)
>>> image = image.detach().cpu().permute(0, 2, 3, 1).numpy()
>>> images = (image * 255).round().astype("uint8")
>>> pil_images = [Image.fromarray(image) for image in images]
>>> pil_images[0]
```
<div class="flex justify-center">
<img src="https://huggingface.co/blog/assets/98_stable_diffusion/stable_diffusion_k_lms.png"/>
</div>
## 다음 단계
기본 파이프라인부터 복잡한 파이프라인까지, 자신만의 diffusion 시스템을 작성하는 데 필요한 것은 노이즈 제거 루프뿐이라는 것을 알 수 있었습니다. 이 루프는 스케줄러의 timesteps를 설정하고, 이를 반복하며, UNet 모델을 호출하여 noise residual을 예측하고 스케줄러에 전달하여 이전 노이즈 샘플을 계산하는 과정을 번갈아 가며 수행해야 합니다.
이것이 바로 🧨 Diffusers가 설계된 목적입니다: 모델과 스케줄러를 사용해 자신만의 diffusion 시스템을 직관적이고 쉽게 작성할 수 있도록 하기 위해서입니다.
다음 단계를 자유롭게 진행하세요:
* 🧨 Diffusers에 [파이프라인 구축 및 기여](using-diffusers/#contribute_pipeline)하는 방법을 알아보세요. 여러분이 어떤 아이디어를 내놓을지 기대됩니다!
* 라이브러리에서 [기본 파이프라인](./api/pipelines/overview)을 살펴보고, 모델과 스케줄러를 별도로 사용하여 파이프라인을 처음부터 해체하고 빌드할 수 있는지 확인해 보세요.
| 0 |
hf_public_repos/diffusers/docs/source/ko | hf_public_repos/diffusers/docs/source/ko/using-diffusers/loading.md | <!--Copyright 2023 The HuggingFace Team. All rights reserved.
Licensed under the Apache License, Version 2.0 (the "License"); you may not use this file except in compliance with
the License. You may obtain a copy of the License at
http://www.apache.org/licenses/LICENSE-2.0
Unless required by applicable law or agreed to in writing, software distributed under the License is distributed on
an "AS IS" BASIS, WITHOUT WARRANTIES OR CONDITIONS OF ANY KIND, either express or implied. See the License for the
specific language governing permissions and limitations under the License.
-->
# 파이프라인, 모델, 스케줄러 불러오기
기본적으로 diffusion 모델은 다양한 컴포넌트들(모델, 토크나이저, 스케줄러) 간의 복잡한 상호작용을 기반으로 동작합니다. 디퓨저스(Diffusers)는 이러한 diffusion 모델을 보다 쉽고 간편한 API로 제공하는 것을 목표로 설계되었습니다. [`DiffusionPipeline`]은 diffusion 모델이 갖는 복잡성을 하나의 파이프라인 API로 통합하고, 동시에 이를 구성하는 각각의 컴포넌트들을 태스크에 맞춰 유연하게 커스터마이징할 수 있도록 지원하고 있습니다.
diffusion 모델의 훈련과 추론에 필요한 모든 것은 [`DiffusionPipeline.from_pretrained`] 메서드를 통해 접근할 수 있습니다. (이 말의 의미는 다음 단락에서 보다 자세하게 다뤄보도록 하겠습니다.)
이 문서에서는 설명할 내용은 다음과 같습니다.
* 허브를 통해 혹은 로컬로 파이프라인을 불러오는 법
* 파이프라인에 다른 컴포넌트들을 적용하는 법
* 오리지널 체크포인트가 아닌 variant를 불러오는 법 (variant란 기본으로 설정된 `fp32`가 아닌 다른 부동 소수점 타입(예: `fp16`)을 사용하거나 Non-EMA 가중치를 사용하는 체크포인트들을 의미합니다.)
* 모델과 스케줄러를 불러오는 법
## Diffusion 파이프라인
<Tip>
💡 [`DiffusionPipeline`] 클래스가 동작하는 방식에 보다 자세한 내용이 궁금하다면, [DiffusionPipeline explained](#diffusionpipeline에-대해-알아보기) 섹션을 확인해보세요.
</Tip>
[`DiffusionPipeline`] 클래스는 diffusion 모델을 [허브](https://huggingface.co/models?library=diffusers)로부터 불러오는 가장 심플하면서 보편적인 방식입니다. [`DiffusionPipeline.from_pretrained`] 메서드는 적합한 파이프라인 클래스를 자동으로 탐지하고, 필요한 구성요소(configuration)와 가중치(weight) 파일들을 다운로드하고 캐싱한 다음, 해당 파이프라인 인스턴스를 반환합니다.
```python
from diffusers import DiffusionPipeline
repo_id = "runwayml/stable-diffusion-v1-5"
pipe = DiffusionPipeline.from_pretrained(repo_id)
```
물론 [`DiffusionPipeline`] 클래스를 사용하지 않고, 명시적으로 직접 해당 파이프라인 클래스를 불러오는 것도 가능합니다. 아래 예시 코드는 위 예시와 동일한 인스턴스를 반환합니다.
```python
from diffusers import StableDiffusionPipeline
repo_id = "runwayml/stable-diffusion-v1-5"
pipe = StableDiffusionPipeline.from_pretrained(repo_id)
```
[CompVis/stable-diffusion-v1-4](https://huggingface.co/CompVis/stable-diffusion-v1-4)이나 [runwayml/stable-diffusion-v1-5](https://huggingface.co/runwayml/stable-diffusion-v1-5) 같은 체크포인트들의 경우, 하나 이상의 다양한 태스크에 활용될 수 있습니다. (예를 들어 위의 두 체크포인트의 경우, text-to-image와 image-to-image에 모두 활용될 수 있습니다.) 만약 이러한 체크포인트들을 기본 설정 태스크가 아닌 다른 태스크에 활용하고자 한다면, 해당 태스크에 대응되는 파이프라인(task-specific pipeline)을 사용해야 합니다.
```python
from diffusers import StableDiffusionImg2ImgPipeline
repo_id = "runwayml/stable-diffusion-v1-5"
pipe = StableDiffusionImg2ImgPipeline.from_pretrained(repo_id)
```
### 로컬 파이프라인
파이프라인을 로컬로 불러오고자 한다면, `git-lfs`를 사용하여 직접 체크포인트를 로컬 디스크에 다운로드 받아야 합니다. 아래의 명령어를 실행하면 `./stable-diffusion-v1-5`란 이름으로 폴더가 로컬디스크에 생성됩니다.
```bash
git lfs install
git clone https://huggingface.co/runwayml/stable-diffusion-v1-5
```
그런 다음 해당 로컬 경로를 [`~DiffusionPipeline.from_pretrained`] 메서드에 전달합니다.
```python
from diffusers import DiffusionPipeline
repo_id = "./stable-diffusion-v1-5"
stable_diffusion = DiffusionPipeline.from_pretrained(repo_id)
```
위의 예시코드처럼 만약 `repo_id`가 로컬 패스(local path)라면, [`~DiffusionPipeline.from_pretrained`] 메서드는 이를 자동으로 감지하여 허브에서 파일을 다운로드하지 않습니다. 만약 로컬 디스크에 저장된 파이프라인 체크포인트가 최신 버전이 아닐 경우에도, 최신 버전을 다운로드하지 않고 기존 로컬 디스크에 저장된 체크포인트를 사용한다는 것을 의미합니다.
### 파이프라인 내부의 컴포넌트 교체하기
파이프라인 내부의 컴포넌트들은 호환 가능한 다른 컴포넌트로 교체될 수 있습니다. 이와 같은 컴포넌트 교체가 중요한 이유는 다음과 같습니다.
- 어떤 스케줄러를 사용할 것인가는 생성속도와 생성품질 간의 트레이드오프를 정의하는 중요한 요소입니다.
- diffusion 모델 내부의 컴포넌트들은 일반적으로 각각 독립적으로 훈련되기 때문에, 더 좋은 성능을 보여주는 컴포넌트가 있다면 그걸로 교체하는 식으로 성능을 향상시킬 수 있습니다.
- 파인 튜닝 단계에서는 일반적으로 UNet 혹은 텍스트 인코더와 같은 일부 컴포넌트들만 훈련하게 됩니다.
어떤 스케줄러들이 호환가능한지는 `compatibles` 속성을 통해 확인할 수 있습니다.
```python
from diffusers import DiffusionPipeline
repo_id = "runwayml/stable-diffusion-v1-5"
stable_diffusion = DiffusionPipeline.from_pretrained(repo_id)
stable_diffusion.scheduler.compatibles
```
이번에는 [`SchedulerMixin.from_pretrained`] 메서드를 사용해서, 기존 기본 스케줄러였던 [`PNDMScheduler`]를 보다 우수한 성능의 [`EulerDiscreteScheduler`]로 바꿔봅시다. 스케줄러를 로드할 때는 `subfolder` 인자를 통해, 해당 파이프라인의 레포지토리에서 [스케줄러에 관한 하위폴더](https://huggingface.co/runwayml/stable-diffusion-v1-5/tree/main/scheduler)를 명시해주어야 합니다.
그 다음 새롭게 생성한 [`EulerDiscreteScheduler`] 인스턴스를 [`DiffusionPipeline`]의 `scheduler` 인자에 전달합니다.
```python
from diffusers import DiffusionPipeline, EulerDiscreteScheduler, DPMSolverMultistepScheduler
repo_id = "runwayml/stable-diffusion-v1-5"
scheduler = EulerDiscreteScheduler.from_pretrained(repo_id, subfolder="scheduler")
stable_diffusion = DiffusionPipeline.from_pretrained(repo_id, scheduler=scheduler)
```
### 세이프티 체커
스테이블 diffusion과 같은 diffusion 모델들은 유해한 이미지를 생성할 수도 있습니다. 이를 예방하기 위해 디퓨저스는 생성된 이미지의 유해성을 판단하는 [세이프티 체커(safety checker)](https://github.com/huggingface/diffusers/blob/main/src/diffusers/pipelines/stable_diffusion/safety_checker.py) 기능을 지원하고 있습니다. 만약 세이프티 체커의 사용을 원하지 않는다면, `safety_checker` 인자에 `None`을 전달해주시면 됩니다.
```python
from diffusers import DiffusionPipeline
repo_id = "runwayml/stable-diffusion-v1-5"
stable_diffusion = DiffusionPipeline.from_pretrained(repo_id, safety_checker=None)
```
### 컴포넌트 재사용
복수의 파이프라인에 동일한 모델이 반복적으로 사용한다면, 굳이 해당 모델의 동일한 가중치를 중복으로 RAM에 불러올 필요는 없을 것입니다. [`~DiffusionPipeline.components`] 속성을 통해 파이프라인 내부의 컴포넌트들을 참조할 수 있는데, 이번 단락에서는 이를 통해 동일한 모델 가중치를 RAM에 중복으로 불러오는 것을 방지하는 법에 대해 알아보겠습니다.
```python
from diffusers import StableDiffusionPipeline, StableDiffusionImg2ImgPipeline
model_id = "runwayml/stable-diffusion-v1-5"
stable_diffusion_txt2img = StableDiffusionPipeline.from_pretrained(model_id)
components = stable_diffusion_txt2img.components
```
그 다음 위 예시 코드에서 선언한 `components` 변수를 다른 파이프라인에 전달함으로써, 모델의 가중치를 중복으로 RAM에 로딩하지 않고, 동일한 컴포넌트를 재사용할 수 있습니다.
```python
stable_diffusion_img2img = StableDiffusionImg2ImgPipeline(**components)
```
물론 각각의 컴포넌트들을 따로 따로 파이프라인에 전달할 수도 있습니다. 예를 들어 `stable_diffusion_txt2img` 파이프라인 안의 컴포넌트들 가운데서 세이프티 체커(`safety_checker`)와 피쳐 익스트랙터(`feature_extractor`)를 제외한 컴포넌트들만 `stable_diffusion_img2img` 파이프라인에서 재사용하는 방식 역시 가능합니다.
```python
from diffusers import StableDiffusionPipeline, StableDiffusionImg2ImgPipeline
model_id = "runwayml/stable-diffusion-v1-5"
stable_diffusion_txt2img = StableDiffusionPipeline.from_pretrained(model_id)
stable_diffusion_img2img = StableDiffusionImg2ImgPipeline(
vae=stable_diffusion_txt2img.vae,
text_encoder=stable_diffusion_txt2img.text_encoder,
tokenizer=stable_diffusion_txt2img.tokenizer,
unet=stable_diffusion_txt2img.unet,
scheduler=stable_diffusion_txt2img.scheduler,
safety_checker=None,
feature_extractor=None,
requires_safety_checker=False,
)
```
## Checkpoint variants
Variant란 일반적으로 다음과 같은 체크포인트들을 의미합니다.
- `torch.float16`과 같이 정밀도는 더 낮지만, 용량 역시 더 작은 부동소수점 타입의 가중치를 사용하는 체크포인트. *(다만 이와 같은 variant의 경우, 추가적인 훈련과 CPU환경에서의 구동이 불가능합니다.)*
- Non-EMA 가중치를 사용하는 체크포인트. *(Non-EMA 가중치의 경우, 파인 튜닝 단계에서 사용하는 것이 권장되는데, 추론 단계에선 사용하지 않는 것이 권장됩니다.)*
<Tip>
💡 모델 구조는 동일하지만 서로 다른 학습 환경에서 서로 다른 데이터셋으로 학습된 체크포인트들이 있을 경우, 해당 체크포인트들은 variant 단계가 아닌 레포지토리 단계에서 분리되어 관리되어야 합니다. (즉, 해당 체크포인트들은 서로 다른 레포지토리에서 따로 관리되어야 합니다. 예시: [`stable-diffusion-v1-4`], [`stable-diffusion-v1-5`]).
</Tip>
| **checkpoint type** | **weight name** | **argument for loading weights** |
| ------------------- | ----------------------------------- | -------------------------------- |
| original | diffusion_pytorch_model.bin | |
| floating point | diffusion_pytorch_model.fp16.bin | `variant`, `torch_dtype` |
| non-EMA | diffusion_pytorch_model.non_ema.bin | `variant` |
variant를 로드할 때 2개의 중요한 argument가 있습니다.
* `torch_dtype`은 불러올 체크포인트의 부동소수점을 정의합니다. 예를 들어 `torch_dtype=torch.float16`을 명시함으로써 가중치의 부동소수점 타입을 `fl16`으로 변환할 수 있습니다. (만약 따로 설정하지 않을 경우, 기본값으로 `fp32` 타입의 가중치가 로딩됩니다.) 또한 `variant` 인자를 명시하지 않은 채로 체크포인트를 불러온 다음, 해당 체크포인트를 `torch_dtype=torch.float16` 인자를 통해 `fp16` 타입으로 변환하는 것 역시 가능합니다. 이 경우 기본으로 설정된 `fp32` 가중치가 먼저 다운로드되고, 해당 가중치들을 불러온 다음 `fp16` 타입으로 변환하게 됩니다.
* `variant` 인자는 레포지토리에서 어떤 variant를 불러올 것인가를 정의합니다. 가령 [`diffusers/stable-diffusion-variants`](https://huggingface.co/diffusers/stable-diffusion-variants/tree/main/unet) 레포지토리로부터 `non_ema` 체크포인트를 불러오고자 한다면, `variant="non_ema"` 인자를 전달해야 합니다.
```python
from diffusers import DiffusionPipeline
# load fp16 variant
stable_diffusion = DiffusionPipeline.from_pretrained(
"runwayml/stable-diffusion-v1-5", variant="fp16", torch_dtype=torch.float16
)
# load non_ema variant
stable_diffusion = DiffusionPipeline.from_pretrained("runwayml/stable-diffusion-v1-5", variant="non_ema")
```
다른 부동소수점 타입의 가중치 혹은 non-EMA 가중치를 사용하는 체크포인트를 저장하기 위해서는, [`DiffusionPipeline.save_pretrained`] 메서드를 사용해야 하며, 이 때 `variant` 인자를 명시해줘야 합니다. 원래의 체크포인트와 동일한 폴더에 variant를 저장해야 하며, 이렇게 하면 동일한 폴더에서 오리지널 체크포인트과 variant를 모두 불러올 수 있습니다.
```python
from diffusers import DiffusionPipeline
# save as fp16 variant
stable_diffusion.save_pretrained("runwayml/stable-diffusion-v1-5", variant="fp16")
# save as non-ema variant
stable_diffusion.save_pretrained("runwayml/stable-diffusion-v1-5", variant="non_ema")
```
만약 variant를 기존 폴더에 저장하지 않을 경우, `variant` 인자를 반드시 명시해야 합니다. 그렇게 하지 않을 경우 원래의 오리지널 체크포인트를 찾을 수 없게 되기 때문에 에러가 발생합니다.
```python
# 👎 this won't work
stable_diffusion = DiffusionPipeline.from_pretrained("./stable-diffusion-v1-5", torch_dtype=torch.float16)
# 👍 this works
stable_diffusion = DiffusionPipeline.from_pretrained(
"./stable-diffusion-v1-5", variant="fp16", torch_dtype=torch.float16
)
```
### 모델 불러오기
모델들은 [`ModelMixin.from_pretrained`] 메서드를 통해 불러올 수 있습니다. 해당 메서드는 최신 버전의 모델 가중치 파일과 설정 파일(configurations)을 다운로드하고 캐싱합니다. 만약 이러한 파일들이 최신 버전으로 로컬 캐시에 저장되어 있다면, [`ModelMixin.from_pretrained`]는 굳이 해당 파일들을 다시 다운로드하지 않으며, 그저 캐시에 있는 최신 파일들을 재사용합니다.
모델은 `subfolder` 인자에 명시된 하위 폴더로부터 로드됩니다. 예를 들어 `runwayml/stable-diffusion-v1-5`의 UNet 모델의 가중치는 [`unet`](https://huggingface.co/runwayml/stable-diffusion-v1-5/tree/main/unet) 폴더에 저장되어 있습니다.
```python
from diffusers import UNet2DConditionModel
repo_id = "runwayml/stable-diffusion-v1-5"
model = UNet2DConditionModel.from_pretrained(repo_id, subfolder="unet")
```
혹은 [해당 모델의 레포지토리](https://huggingface.co/google/ddpm-cifar10-32/tree/main)로부터 다이렉트로 가져오는 것 역시 가능합니다.
```python
from diffusers import UNet2DModel
repo_id = "google/ddpm-cifar10-32"
model = UNet2DModel.from_pretrained(repo_id)
```
또한 앞서 봤던 `variant` 인자를 명시함으로써, Non-EMA나 `fp16`의 가중치를 가져오는 것 역시 가능합니다.
```python
from diffusers import UNet2DConditionModel
model = UNet2DConditionModel.from_pretrained("runwayml/stable-diffusion-v1-5", subfolder="unet", variant="non-ema")
model.save_pretrained("./local-unet", variant="non-ema")
```
### 스케줄러
스케줄러들은 [`SchedulerMixin.from_pretrained`] 메서드를 통해 불러올 수 있습니다. 모델과 달리 스케줄러는 별도의 가중치를 갖지 않으며, 따라서 당연히 별도의 학습과정을 요구하지 않습니다. 이러한 스케줄러들은 (해당 스케줄러 하위폴더의) configration 파일을 통해 정의됩니다.
여러개의 스케줄러를 불러온다고 해서 많은 메모리를 소모하는 것은 아니며, 다양한 스케줄러들에 동일한 스케줄러 configration을 적용하는 것 역시 가능합니다. 다음 예시 코드에서 불러오는 스케줄러들은 모두 [`StableDiffusionPipeline`]과 호환되는데, 이는 곧 해당 스케줄러들에 동일한 스케줄러 configration 파일을 적용할 수 있음을 의미합니다.
```python
from diffusers import StableDiffusionPipeline
from diffusers import (
DDPMScheduler,
DDIMScheduler,
PNDMScheduler,
LMSDiscreteScheduler,
EulerDiscreteScheduler,
EulerAncestralDiscreteScheduler,
DPMSolverMultistepScheduler,
)
repo_id = "runwayml/stable-diffusion-v1-5"
ddpm = DDPMScheduler.from_pretrained(repo_id, subfolder="scheduler")
ddim = DDIMScheduler.from_pretrained(repo_id, subfolder="scheduler")
pndm = PNDMScheduler.from_pretrained(repo_id, subfolder="scheduler")
lms = LMSDiscreteScheduler.from_pretrained(repo_id, subfolder="scheduler")
euler_anc = EulerAncestralDiscreteScheduler.from_pretrained(repo_id, subfolder="scheduler")
euler = EulerDiscreteScheduler.from_pretrained(repo_id, subfolder="scheduler")
dpm = DPMSolverMultistepScheduler.from_pretrained(repo_id, subfolder="scheduler")
# replace `dpm` with any of `ddpm`, `ddim`, `pndm`, `lms`, `euler_anc`, `euler`
pipeline = StableDiffusionPipeline.from_pretrained(repo_id, scheduler=dpm)
```
### DiffusionPipeline에 대해 알아보기
클래스 메서드로서 [`DiffusionPipeline.from_pretrained`]은 2가지를 담당합니다.
- 첫째로, `from_pretrained` 메서드는 최신 버전의 파이프라인을 다운로드하고, 캐시에 저장합니다. 이미 로컬 캐시에 최신 버전의 파이프라인이 저장되어 있다면, [`DiffusionPipeline.from_pretrained`]은 해당 파일들을 다시 다운로드하지 않고, 로컬 캐시에 저장되어 있는 파이프라인을 불러옵니다.
- `model_index.json` 파일을 통해 체크포인트에 대응되는 적합한 파이프라인 클래스로 불러옵니다.
파이프라인의 폴더 구조는 해당 파이프라인 클래스의 구조와 직접적으로 일치합니다. 예를 들어 [`StableDiffusionPipeline`] 클래스는 [`runwayml/stable-diffusion-v1-5`](https://huggingface.co/runwayml/stable-diffusion-v1-5) 레포지토리와 대응되는 구조를 갖습니다.
```python
from diffusers import DiffusionPipeline
repo_id = "runwayml/stable-diffusion-v1-5"
pipeline = DiffusionPipeline.from_pretrained(repo_id)
print(pipeline)
```
위의 코드 출력 결과를 확인해보면, `pipeline`은 [`StableDiffusionPipeline`]의 인스턴스이며, 다음과 같이 총 7개의 컴포넌트로 구성된다는 것을 알 수 있습니다.
- `"feature_extractor"`: [`~transformers.CLIPFeatureExtractor`]의 인스턴스
- `"safety_checker"`: 유해한 컨텐츠를 스크리닝하기 위한 [컴포넌트](https://github.com/huggingface/diffusers/blob/e55687e1e15407f60f32242027b7bb8170e58266/src/diffusers/pipelines/stable_diffusion/safety_checker.py#L32)
- `"scheduler"`: [`PNDMScheduler`]의 인스턴스
- `"text_encoder"`: [`~transformers.CLIPTextModel`]의 인스턴스
- `"tokenizer"`: a [`~transformers.CLIPTokenizer`]의 인스턴스
- `"unet"`: [`UNet2DConditionModel`]의 인스턴스
- `"vae"` [`AutoencoderKL`]의 인스턴스
```json
StableDiffusionPipeline {
"feature_extractor": [
"transformers",
"CLIPImageProcessor"
],
"safety_checker": [
"stable_diffusion",
"StableDiffusionSafetyChecker"
],
"scheduler": [
"diffusers",
"PNDMScheduler"
],
"text_encoder": [
"transformers",
"CLIPTextModel"
],
"tokenizer": [
"transformers",
"CLIPTokenizer"
],
"unet": [
"diffusers",
"UNet2DConditionModel"
],
"vae": [
"diffusers",
"AutoencoderKL"
]
}
```
파이프라인 인스턴스의 컴포넌트들을 [`runwayml/stable-diffusion-v1-5`](https://huggingface.co/runwayml/stable-diffusion-v1-5)의 폴더 구조와 비교해볼 경우, 각각의 컴포넌트마다 별도의 폴더가 있음을 확인할 수 있습니다.
```
.
├── feature_extractor
│ └── preprocessor_config.json
├── model_index.json
├── safety_checker
│ ├── config.json
│ └── pytorch_model.bin
├── scheduler
│ └── scheduler_config.json
├── text_encoder
│ ├── config.json
│ └── pytorch_model.bin
├── tokenizer
│ ├── merges.txt
│ ├── special_tokens_map.json
│ ├── tokenizer_config.json
│ └── vocab.json
├── unet
│ ├── config.json
│ ├── diffusion_pytorch_model.bin
└── vae
├── config.json
├── diffusion_pytorch_model.bin
```
또한 각각의 컴포넌트들을 파이프라인 인스턴스의 속성으로써 참조할 수 있습니다.
```py
pipeline.tokenizer
```
```python
CLIPTokenizer(
name_or_path="/root/.cache/huggingface/hub/models--runwayml--stable-diffusion-v1-5/snapshots/39593d5650112b4cc580433f6b0435385882d819/tokenizer",
vocab_size=49408,
model_max_length=77,
is_fast=False,
padding_side="right",
truncation_side="right",
special_tokens={
"bos_token": AddedToken("<|startoftext|>", rstrip=False, lstrip=False, single_word=False, normalized=True),
"eos_token": AddedToken("<|endoftext|>", rstrip=False, lstrip=False, single_word=False, normalized=True),
"unk_token": AddedToken("<|endoftext|>", rstrip=False, lstrip=False, single_word=False, normalized=True),
"pad_token": "<|endoftext|>",
},
)
```
모든 파이프라인은 `model_index.json` 파일을 통해 [`DiffusionPipeline`]에 다음과 같은 정보를 전달합니다.
- `_class_name` 는 어떤 파이프라인 클래스를 사용해야 하는지에 대해 알려줍니다.
- `_diffusers_version`는 어떤 버전의 디퓨저스로 파이프라인 안의 모델들이 만들어졌는지를 알려줍니다.
- 그 다음은 각각의 컴포넌트들이 어떤 라이브러리의 어떤 클래스로 만들어졌는지에 대해 알려줍니다. (아래 예시에서 `"feature_extractor" : ["transformers", "CLIPImageProcessor"]`의 경우, `feature_extractor` 컴포넌트는 `transformers` 라이브러리의 `CLIPImageProcessor` 클래스를 통해 만들어졌다는 것을 의미합니다.)
```json
{
"_class_name": "StableDiffusionPipeline",
"_diffusers_version": "0.6.0",
"feature_extractor": [
"transformers",
"CLIPImageProcessor"
],
"safety_checker": [
"stable_diffusion",
"StableDiffusionSafetyChecker"
],
"scheduler": [
"diffusers",
"PNDMScheduler"
],
"text_encoder": [
"transformers",
"CLIPTextModel"
],
"tokenizer": [
"transformers",
"CLIPTokenizer"
],
"unet": [
"diffusers",
"UNet2DConditionModel"
],
"vae": [
"diffusers",
"AutoencoderKL"
]
}
```
| 0 |
hf_public_repos/diffusers/docs/source/ko | hf_public_repos/diffusers/docs/source/ko/using-diffusers/img2img.md | <!--Copyright 2023 The HuggingFace Team. All rights reserved.
Licensed under the Apache License, Version 2.0 (the "License"); you may not use this file except in compliance with
the License. You may obtain a copy of the License at
http://www.apache.org/licenses/LICENSE-2.0
Unless required by applicable law or agreed to in writing, software distributed under the License is distributed on
an "AS IS" BASIS, WITHOUT WARRANTIES OR CONDITIONS OF ANY KIND, either express or implied. See the License for the
specific language governing permissions and limitations under the License.
-->
# 텍스트 기반 image-to-image 생성
[[Colab에서 열기]]
[`StableDiffusionImg2ImgPipeline`]을 사용하면 텍스트 프롬프트와 시작 이미지를 전달하여 새 이미지 생성의 조건을 지정할 수 있습니다.
시작하기 전에 필요한 라이브러리가 모두 설치되어 있는지 확인하세요:
```bash
!pip install diffusers transformers ftfy accelerate
```
[`nitrosocke/Ghibli-Diffusion`](https://huggingface.co/nitrosocke/Ghibli-Diffusion)과 같은 사전학습된 stable diffusion 모델로 [`StableDiffusionImg2ImgPipeline`]을 생성하여 시작하세요.
```python
import torch
import requests
from PIL import Image
from io import BytesIO
from diffusers import StableDiffusionImg2ImgPipeline
device = "cuda"
pipe = StableDiffusionImg2ImgPipeline.from_pretrained("nitrosocke/Ghibli-Diffusion", torch_dtype=torch.float16).to(
device
)
```
초기 이미지를 다운로드하고 사전 처리하여 파이프라인에 전달할 수 있습니다:
```python
url = "https://raw.githubusercontent.com/CompVis/stable-diffusion/main/assets/stable-samples/img2img/sketch-mountains-input.jpg"
response = requests.get(url)
init_image = Image.open(BytesIO(response.content)).convert("RGB")
init_image.thumbnail((768, 768))
init_image
```
<div class="flex justify-center">
<img src="https://huggingface.co/datasets/YiYiXu/test-doc-assets/resolve/main/image_2_image_using_diffusers_cell_8_output_0.jpeg"/>
</div>
<Tip>
💡 `strength`는 입력 이미지에 추가되는 노이즈의 양을 제어하는 0.0에서 1.0 사이의 값입니다. 1.0에 가까운 값은 다양한 변형을 허용하지만 입력 이미지와 의미적으로 일치하지 않는 이미지를 생성합니다.
</Tip>
프롬프트를 정의하고(지브리 스타일(Ghibli-style)에 맞게 조정된 이 체크포인트의 경우 프롬프트 앞에 `ghibli style` 토큰을 붙여야 합니다) 파이프라인을 실행합니다:
```python
prompt = "ghibli style, a fantasy landscape with castles"
generator = torch.Generator(device=device).manual_seed(1024)
image = pipe(prompt=prompt, image=init_image, strength=0.75, guidance_scale=7.5, generator=generator).images[0]
image
```
<div class="flex justify-center">
<img src="https://huggingface.co/datasets/huggingface/documentation-images/resolve/main/diffusers/ghibli-castles.png"/>
</div>
다른 스케줄러로 실험하여 출력에 어떤 영향을 미치는지 확인할 수도 있습니다:
```python
from diffusers import LMSDiscreteScheduler
lms = LMSDiscreteScheduler.from_config(pipe.scheduler.config)
pipe.scheduler = lms
generator = torch.Generator(device=device).manual_seed(1024)
image = pipe(prompt=prompt, image=init_image, strength=0.75, guidance_scale=7.5, generator=generator).images[0]
image
```
<div class="flex justify-center">
<img src="https://huggingface.co/datasets/huggingface/documentation-images/resolve/main/diffusers/lms-ghibli.png"/>
</div>
아래 공백을 확인하고 `strength` 값을 다르게 설정하여 이미지를 생성해 보세요. `strength`를 낮게 설정하면 원본 이미지와 더 유사한 이미지가 생성되는 것을 확인할 수 있습니다.
자유롭게 스케줄러를 [`LMSDiscreteScheduler`]로 전환하여 출력에 어떤 영향을 미치는지 확인해 보세요.
<iframe
src="https://stevhliu-ghibli-img2img.hf.space"
frameborder="0"
width="850"
height="500"
></iframe> | 0 |
hf_public_repos/diffusers/docs/source/ko | hf_public_repos/diffusers/docs/source/ko/using-diffusers/other-formats.md | <!--Copyright 2023 The HuggingFace Team. All rights reserved.
Licensed under the Apache License, Version 2.0 (the "License"); you may not use this file except in compliance with
the License. You may obtain a copy of the License at
http://www.apache.org/licenses/LICENSE-2.0
Unless required by applicable law or agreed to in writing, software distributed under the License is distributed on
an "AS IS" BASIS, WITHOUT WARRANTIES OR CONDITIONS OF ANY KIND, either express or implied. See the License for the
specific language governing permissions and limitations under the License.
-->
# 다양한 Stable Diffusion 포맷 불러오기
Stable Diffusion 모델들은 학습 및 저장된 프레임워크와 다운로드 위치에 따라 다양한 형식으로 제공됩니다. 이러한 형식을 🤗 Diffusers에서 사용할 수 있도록 변환하면 추론을 위한 [다양한 스케줄러 사용](schedulers), 사용자 지정 파이프라인 구축, 추론 속도 최적화를 위한 다양한 기법과 방법 등 라이브러리에서 지원하는 모든 기능을 사용할 수 있습니다.
<Tip>
우리는 `.safetensors` 형식을 추천합니다. 왜냐하면 기존의 pickled 파일은 취약하고 머신에서 코드를 실행할 때 악용될 수 있는 것에 비해 훨씬 더 안전합니다. (safetensors 불러오기 가이드에서 자세히 알아보세요.)
</Tip>
이 가이드에서는 다른 Stable Diffusion 형식을 🤗 Diffusers와 호환되도록 변환하는 방법을 설명합니다.
## PyTorch .ckpt
체크포인트 또는 `.ckpt` 형식은 일반적으로 모델을 저장하는 데 사용됩니다. `.ckpt` 파일은 전체 모델을 포함하며 일반적으로 크기가 몇 GB입니다. `.ckpt` 파일을 [~StableDiffusionPipeline.from_ckpt] 메서드를 사용하여 직접 불러와서 사용할 수도 있지만, 일반적으로 두 가지 형식을 모두 사용할 수 있도록 `.ckpt` 파일을 🤗 Diffusers로 변환하는 것이 더 좋습니다.
`.ckpt` 파일을 변환하는 두 가지 옵션이 있습니다. Space를 사용하여 체크포인트를 변환하거나 스크립트를 사용하여 `.ckpt` 파일을 변환합니다.
### Space로 변환하기
`.ckpt` 파일을 변환하는 가장 쉽고 편리한 방법은 SD에서 Diffusers로 스페이스를 사용하는 것입니다. Space의 지침에 따라 .ckpt 파일을 변환 할 수 있습니다.
이 접근 방식은 기본 모델에서는 잘 작동하지만 더 많은 사용자 정의 모델에서는 어려움을 겪을 수 있습니다. 빈 pull request나 오류를 반환하면 Space가 실패한 것입니다.
이 경우 스크립트를 사용하여 `.ckpt` 파일을 변환해 볼 수 있습니다.
### 스크립트로 변환하기
🤗 Diffusers는 `.ckpt` 파일 변환을 위한 변환 스크립트를 제공합니다. 이 접근 방식은 위의 Space보다 더 안정적입니다.
시작하기 전에 스크립트를 실행할 🤗 Diffusers의 로컬 클론(clone)이 있는지 확인하고 Hugging Face 계정에 로그인하여 pull request를 열고 변환된 모델을 허브에 푸시할 수 있도록 하세요.
```bash
huggingface-cli login
```
스크립트를 사용하려면:
1. 변환하려는 `.ckpt` 파일이 포함된 리포지토리를 Git으로 클론(clone)합니다.
이 예제에서는 TemporalNet .ckpt 파일을 변환해 보겠습니다:
```bash
git lfs install
git clone https://huggingface.co/CiaraRowles/TemporalNet
```
2. 체크포인트를 변환할 리포지토리에서 pull request를 엽니다:
```bash
cd TemporalNet && git fetch origin refs/pr/13:pr/13
git checkout pr/13
```
3. 변환 스크립트에서 구성할 입력 인수는 여러 가지가 있지만 가장 중요한 인수는 다음과 같습니다:
- `checkpoint_path`: 변환할 `.ckpt` 파일의 경로를 입력합니다.
- `original_config_file`: 원래 아키텍처의 구성을 정의하는 YAML 파일입니다. 이 파일을 찾을 수 없는 경우 `.ckpt` 파일을 찾은 GitHub 리포지토리에서 YAML 파일을 검색해 보세요.
- `dump_path`: 변환된 모델의 경로
예를 들어, TemporalNet 모델은 Stable Diffusion v1.5 및 ControlNet 모델이기 때문에 ControlNet 리포지토리에서 cldm_v15.yaml 파일을 가져올 수 있습니다.
4. 이제 스크립트를 실행하여 .ckpt 파일을 변환할 수 있습니다:
```bash
python ../diffusers/scripts/convert_original_stable_diffusion_to_diffusers.py --checkpoint_path temporalnetv3.ckpt --original_config_file cldm_v15.yaml --dump_path ./ --controlnet
```
5. 변환이 완료되면 변환된 모델을 업로드하고 결과물을 pull request [pull request](https://huggingface.co/CiaraRowles/TemporalNet/discussions/13)를 테스트하세요!
```bash
git push origin pr/13:refs/pr/13
```
## **Keras .pb or .h5**
🧪 이 기능은 실험적인 기능입니다. 현재로서는 Stable Diffusion v1 체크포인트만 변환 KerasCV Space에서 지원됩니다.
[KerasCV](https://keras.io/keras_cv/)는 [Stable Diffusion](https://github.com/keras-team/keras-cv/blob/master/keras_cv/models/stable_diffusion) v1 및 v2에 대한 학습을 지원합니다. 그러나 추론 및 배포를 위한 Stable Diffusion 모델 실험을 제한적으로 지원하는 반면, 🤗 Diffusers는 다양한 [noise schedulers](https://huggingface.co/docs/diffusers/using-diffusers/schedulers), [flash attention](https://huggingface.co/docs/diffusers/optimization/xformers), and [other optimization techniques](https://huggingface.co/docs/diffusers/optimization/fp16) 등 이러한 목적을 위한 보다 완벽한 기능을 갖추고 있습니다.
[Convert KerasCV](https://huggingface.co/spaces/sayakpaul/convert-kerascv-sd-diffusers) Space 변환은 `.pb` 또는 `.h5`을 PyTorch로 변환한 다음, 추론할 수 있도록 [`StableDiffusionPipeline`] 으로 감싸서 준비합니다. 변환된 체크포인트는 Hugging Face Hub의 리포지토리에 저장됩니다.
예제로, textual-inversion으로 학습된 `[sayakpaul/textual-inversion-kerasio](https://huggingface.co/sayakpaul/textual-inversion-kerasio/tree/main)` 체크포인트를 변환해 보겠습니다. 이것은 특수 토큰 `<my-funny-cat>`을 사용하여 고양이로 이미지를 개인화합니다.
KerasCV Space 변환에서는 다음을 입력할 수 있습니다:
- Hugging Face 토큰.
- UNet 과 텍스트 인코더(text encoder) 가중치를 다운로드하는 경로입니다. 모델을 어떻게 학습할지 방식에 따라, UNet과 텍스트 인코더의 경로를 모두 제공할 필요는 없습니다. 예를 들어, textual-inversion에는 텍스트 인코더의 임베딩만 필요하고 텍스트-이미지(text-to-image) 모델 변환에는 UNet 가중치만 필요합니다.
- Placeholder 토큰은 textual-inversion 모델에만 적용됩니다.
- `output_repo_prefix`는 변환된 모델이 저장되는 리포지토리의 이름입니다.
**Submit** (제출) 버튼을 클릭하면 KerasCV 체크포인트가 자동으로 변환됩니다! 체크포인트가 성공적으로 변환되면, 변환된 체크포인트가 포함된 새 리포지토리로 연결되는 링크가 표시됩니다. 새 리포지토리로 연결되는 링크를 따라가면 변환된 모델을 사용해 볼 수 있는 추론 위젯이 포함된 모델 카드가 생성된 KerasCV Space 변환을 확인할 수 있습니다.
코드를 사용하여 추론을 실행하려면 모델 카드의 오른쪽 상단 모서리에 있는 **Use in Diffusers** 버튼을 클릭하여 예시 코드를 복사하여 붙여넣습니다:
```py
from diffusers import DiffusionPipeline
pipeline = DiffusionPipeline.from_pretrained("sayakpaul/textual-inversion-cat-kerascv_sd_diffusers_pipeline")
```
그러면 다음과 같은 이미지를 생성할 수 있습니다:
```py
from diffusers import DiffusionPipeline
pipeline = DiffusionPipeline.from_pretrained("sayakpaul/textual-inversion-cat-kerascv_sd_diffusers_pipeline")
pipeline.to("cuda")
placeholder_token = "<my-funny-cat-token>"
prompt = f"two {placeholder_token} getting married, photorealistic, high quality"
image = pipeline(prompt, num_inference_steps=50).images[0]
```
## **A1111 LoRA files**
[Automatic1111](https://github.com/AUTOMATIC1111/stable-diffusion-webui) (A1111)은 Stable Diffusion을 위해 널리 사용되는 웹 UI로, [Civitai](https://civitai.com/) 와 같은 모델 공유 플랫폼을 지원합니다. 특히 LoRA 기법으로 학습된 모델은 학습 속도가 빠르고 완전히 파인튜닝된 모델보다 파일 크기가 훨씬 작기 때문에 인기가 높습니다.
🤗 Diffusers는 [`~loaders.LoraLoaderMixin.load_lora_weights`]:를 사용하여 A1111 LoRA 체크포인트 불러오기를 지원합니다:
```py
from diffusers import DiffusionPipeline, UniPCMultistepScheduler
import torch
pipeline = DiffusionPipeline.from_pretrained(
"andite/anything-v4.0", torch_dtype=torch.float16, safety_checker=None
).to("cuda")
pipeline.scheduler = UniPCMultistepScheduler.from_config(pipeline.scheduler.config)
```
Civitai에서 LoRA 체크포인트를 다운로드하세요; 이 예제에서는 [Howls Moving Castle,Interior/Scenery LoRA (Ghibli Stlye)](https://civitai.com/models/14605?modelVersionId=19998) 체크포인트를 사용했지만, 어떤 LoRA 체크포인트든 자유롭게 사용해 보세요!
```bash
!wget https://civitai.com/api/download/models/19998 -O howls_moving_castle.safetensors
```
메서드를 사용하여 파이프라인에 LoRA 체크포인트를 불러옵니다:
```py
pipeline.load_lora_weights(".", weight_name="howls_moving_castle.safetensors")
```
이제 파이프라인을 사용하여 이미지를 생성할 수 있습니다:
```py
prompt = "masterpiece, illustration, ultra-detailed, cityscape, san francisco, golden gate bridge, california, bay area, in the snow, beautiful detailed starry sky"
negative_prompt = "lowres, cropped, worst quality, low quality, normal quality, artifacts, signature, watermark, username, blurry, more than one bridge, bad architecture"
images = pipeline(
prompt=prompt,
negative_prompt=negative_prompt,
width=512,
height=512,
num_inference_steps=25,
num_images_per_prompt=4,
generator=torch.manual_seed(0),
).images
```
마지막으로, 디스플레이에 이미지를 표시하는 헬퍼 함수를 만듭니다:
```py
from PIL import Image
def image_grid(imgs, rows=2, cols=2):
w, h = imgs[0].size
grid = Image.new("RGB", size=(cols * w, rows * h))
for i, img in enumerate(imgs):
grid.paste(img, box=(i % cols * w, i // cols * h))
return grid
image_grid(images)
```
<div class="flex justify-center">
<img src="https://huggingface.co/datasets/huggingface/documentation-images/resolve/main/diffusers/a1111-lora-sf.png" />
</div>
| 0 |
hf_public_repos/diffusers/docs/source/ko | hf_public_repos/diffusers/docs/source/ko/using-diffusers/inpaint.md | <!--Copyright 2023 The HuggingFace Team. All rights reserved.
Licensed under the Apache License, Version 2.0 (the "License"); you may not use this file except in compliance with
the License. You may obtain a copy of the License at
http://www.apache.org/licenses/LICENSE-2.0
Unless required by applicable law or agreed to in writing, software distributed under the License is distributed on
an "AS IS" BASIS, WITHOUT WARRANTIES OR CONDITIONS OF ANY KIND, either express or implied. See the License for the
specific language governing permissions and limitations under the License.
-->
# Text-guided 이미지 인페인팅(inpainting)
[[코랩에서 열기]]
[`StableDiffusionInpaintPipeline`]은 마스크와 텍스트 프롬프트를 제공하여 이미지의 특정 부분을 편집할 수 있도록 합니다. 이 기능은 인페인팅 작업을 위해 특별히 훈련된 [`runwayml/stable-diffusion-inpainting`](https://huggingface.co/runwayml/stable-diffusion-inpainting)과 같은 Stable Diffusion 버전을 사용합니다.
먼저 [`StableDiffusionInpaintPipeline`] 인스턴스를 불러옵니다:
```python
import PIL
import requests
import torch
from io import BytesIO
from diffusers import StableDiffusionInpaintPipeline
pipeline = StableDiffusionInpaintPipeline.from_pretrained(
"runwayml/stable-diffusion-inpainting",
torch_dtype=torch.float16,
)
pipeline = pipeline.to("cuda")
```
나중에 교체할 강아지 이미지와 마스크를 다운로드하세요:
```python
def download_image(url):
response = requests.get(url)
return PIL.Image.open(BytesIO(response.content)).convert("RGB")
img_url = "https://raw.githubusercontent.com/CompVis/latent-diffusion/main/data/inpainting_examples/overture-creations-5sI6fQgYIuo.png"
mask_url = "https://raw.githubusercontent.com/CompVis/latent-diffusion/main/data/inpainting_examples/overture-creations-5sI6fQgYIuo_mask.png"
init_image = download_image(img_url).resize((512, 512))
mask_image = download_image(mask_url).resize((512, 512))
```
이제 마스크를 다른 것으로 교체하라는 프롬프트를 만들 수 있습니다:
```python
prompt = "Face of a yellow cat, high resolution, sitting on a park bench"
image = pipe(prompt=prompt, image=init_image, mask_image=mask_image).images[0]
```
`image` | `mask_image` | `prompt` | output |
:-------------------------:|:-------------------------:|:-------------------------:|-------------------------:|
<img src="https://raw.githubusercontent.com/CompVis/latent-diffusion/main/data/inpainting_examples/overture-creations-5sI6fQgYIuo.png" alt="drawing" width="250"/> | <img src="https://raw.githubusercontent.com/CompVis/latent-diffusion/main/data/inpainting_examples/overture-creations-5sI6fQgYIuo_mask.png" alt="drawing" width="250"/> | ***Face of a yellow cat, high resolution, sitting on a park bench*** | <img src="https://huggingface.co/datasets/hf-internal-testing/diffusers-images/resolve/main/in_paint/yellow_cat_sitting_on_a_park_bench.png" alt="drawing" width="250"/> |
<Tip warning={true}>
이전의 실험적인 인페인팅 구현에서는 품질이 낮은 다른 프로세스를 사용했습니다. 이전 버전과의 호환성을 보장하기 위해 새 모델이 포함되지 않은 사전학습된 파이프라인을 불러오면 이전 인페인팅 방법이 계속 적용됩니다.
</Tip>
아래 Space에서 이미지 인페인팅을 직접 해보세요!
<iframe
src="https://runwayml-stable-diffusion-inpainting.hf.space"
frameborder="0"
width="850"
height="500"
></iframe>
| 0 |
hf_public_repos/diffusers/docs/source/ko | hf_public_repos/diffusers/docs/source/ko/using-diffusers/custom_pipeline_overview.md | <!--Copyright 2023 The HuggingFace Team. All rights reserved.
Licensed under the Apache License, Version 2.0 (the "License"); you may not use this file except in compliance with
the License. You may obtain a copy of the License at
http://www.apache.org/licenses/LICENSE-2.0
Unless required by applicable law or agreed to in writing, software distributed under the License is distributed on
an "AS IS" BASIS, WITHOUT WARRANTIES OR CONDITIONS OF ANY KIND, either express or implied. See the License for the
specific language governing permissions and limitations under the License.
-->
# 커스텀 파이프라인 불러오기
[[open-in-colab]]
커뮤니티 파이프라인은 논문에 명시된 원래의 구현체와 다른 형태로 구현된 모든 [`DiffusionPipeline`] 클래스를 의미합니다. (예를 들어, [`StableDiffusionControlNetPipeline`]는 ["Text-to-Image Generation with ControlNet Conditioning"](https://arxiv.org/abs/2302.05543) 해당) 이들은 추가 기능을 제공하거나 파이프라인의 원래 구현을 확장합니다.
[Speech to Image](https://github.com/huggingface/diffusers/tree/main/examples/community#speech-to-image) 또는 [Composable Stable Diffusion](https://github.com/huggingface/diffusers/tree/main/examples/community#composable-stable-diffusion) 과 같은 멋진 커뮤니티 파이프라인이 많이 있으며 [여기에서](https://github.com/huggingface/diffusers/tree/main/examples/community) 모든 공식 커뮤니티 파이프라인을 찾을 수 있습니다.
허브에서 커뮤니티 파이프라인을 로드하려면, 커뮤니티 파이프라인의 리포지토리 ID와 (파이프라인 가중치 및 구성 요소를 로드하려는) 모델의 리포지토리 ID를 인자로 전달해야 합니다. 예를 들어, 아래 예시에서는 `hf-internal-testing/diffusers-dummy-pipeline`에서 더미 파이프라인을 불러오고, `google/ddpm-cifar10-32`에서 파이프라인의 가중치와 컴포넌트들을 로드합니다.
<Tip warning={true}>
🔒 허깅 페이스 허브에서 커뮤니티 파이프라인을 불러오는 것은 곧 해당 코드가 안전하다고 신뢰하는 것입니다. 코드를 자동으로 불러오고 실행하기 앞서 반드시 온라인으로 해당 코드의 신뢰성을 검사하세요!
</Tip>
```py
from diffusers import DiffusionPipeline
pipeline = DiffusionPipeline.from_pretrained(
"google/ddpm-cifar10-32", custom_pipeline="hf-internal-testing/diffusers-dummy-pipeline"
)
```
공식 커뮤니티 파이프라인을 불러오는 것은 비슷하지만, 공식 리포지토리 ID에서 가중치를 불러오는 것과 더불어 해당 파이프라인 내의 컴포넌트를 직접 지정하는 것 역시 가능합니다. 아래 예제를 보면 커뮤니티 [CLIP Guided Stable Diffusion](https://github.com/huggingface/diffusers/tree/main/examples/community#clip-guided-stable-diffusion) 파이프라인을 로드할 때, 해당 파이프라인에서 사용할 `clip_model` 컴포넌트와 `feature_extractor` 컴포넌트를 직접 설정하는 것을 확인할 수 있습니다.
```py
from diffusers import DiffusionPipeline
from transformers import CLIPImageProcessor, CLIPModel
clip_model_id = "laion/CLIP-ViT-B-32-laion2B-s34B-b79K"
feature_extractor = CLIPImageProcessor.from_pretrained(clip_model_id)
clip_model = CLIPModel.from_pretrained(clip_model_id)
pipeline = DiffusionPipeline.from_pretrained(
"runwayml/stable-diffusion-v1-5",
custom_pipeline="clip_guided_stable_diffusion",
clip_model=clip_model,
feature_extractor=feature_extractor,
)
```
커뮤니티 파이프라인에 대한 자세한 내용은 [커뮤니티 파이프라인](https://github.com/huggingface/diffusers/blob/main/docs/source/en/using-diffusers/custom_pipeline_examples) 가이드를 살펴보세요. 커뮤니티 파이프라인 등록에 관심이 있는 경우 [커뮤니티 파이프라인에 기여하는 방법](https://github.com/huggingface/diffusers/blob/main/docs/source/en/using-diffusers/contribute_pipeline)에 대한 가이드를 확인하세요 ! | 0 |
hf_public_repos/diffusers/docs/source/ko | hf_public_repos/diffusers/docs/source/ko/tutorials/basic_training.md | <!--Copyright 2023 The HuggingFace Team. All rights reserved.
Licensed under the Apache License, Version 2.0 (the "License"); you may not use this file except in compliance with
the License. You may obtain a copy of the License at
http://www.apache.org/licenses/LICENSE-2.0
Unless required by applicable law or agreed to in writing, software distributed under the License is distributed on
an "AS IS" BASIS, WITHOUT WARRANTIES OR CONDITIONS OF ANY KIND, either express or implied. See the License for the
specific language governing permissions and limitations under the License.
-->
[[open-in-colab]]
# Diffusion 모델을 학습하기
Unconditional 이미지 생성은 학습에 사용된 데이터셋과 유사한 이미지를 생성하는 diffusion 모델에서 인기 있는 어플리케이션입니다. 일반적으로, 가장 좋은 결과는 특정 데이터셋에 사전 훈련된 모델을 파인튜닝하는 것으로 얻을 수 있습니다. 이 [허브](https://huggingface.co/search/full-text?q=unconditional-image-generation&type=model)에서 이러한 많은 체크포인트를 찾을 수 있지만, 만약 마음에 드는 체크포인트를 찾지 못했다면, 언제든지 스스로 학습할 수 있습니다!
이 튜토리얼은 나만의 🦋 나비 🦋를 생성하기 위해 [Smithsonian Butterflies](https://huggingface.co/datasets/huggan/smithsonian_butterflies_subset) 데이터셋의 하위 집합에서 [`UNet2DModel`] 모델을 학습하는 방법을 가르쳐줄 것입니다.
<Tip>
💡 이 학습 튜토리얼은 [Training with 🧨 Diffusers](https://colab.research.google.com/github/huggingface/notebooks/blob/main/diffusers/training_example.ipynb) 노트북 기반으로 합니다. Diffusion 모델의 작동 방식 및 자세한 내용은 노트북을 확인하세요!
</Tip>
시작 전에, 🤗 Datasets을 불러오고 전처리하기 위해 데이터셋이 설치되어 있는지 다수 GPU에서 학습을 간소화하기 위해 🤗 Accelerate 가 설치되어 있는지 확인하세요. 그 후 학습 메트릭을 시각화하기 위해 [TensorBoard](https://www.tensorflow.org/tensorboard)를 또한 설치하세요. (또한 학습 추적을 위해 [Weights & Biases](https://docs.wandb.ai/)를 사용할 수 있습니다.)
```bash
!pip install diffusers[training]
```
커뮤니티에 모델을 공유할 것을 권장하며, 이를 위해서 Hugging Face 계정에 로그인을 해야 합니다. (계정이 없다면 [여기](https://hf.co/join)에서 만들 수 있습니다.) 노트북에서 로그인할 수 있으며 메시지가 표시되면 토큰을 입력할 수 있습니다.
```py
>>> from huggingface_hub import notebook_login
>>> notebook_login()
```
또는 터미널로 로그인할 수 있습니다:
```bash
huggingface-cli login
```
모델 체크포인트가 상당히 크기 때문에 [Git-LFS](https://git-lfs.com/)에서 대용량 파일의 버전 관리를 할 수 있습니다.
```bash
!sudo apt -qq install git-lfs
!git config --global credential.helper store
```
## 학습 구성
편의를 위해 학습 파라미터들을 포함한 `TrainingConfig` 클래스를 생성합니다 (자유롭게 조정 가능):
```py
>>> from dataclasses import dataclass
>>> @dataclass
... class TrainingConfig:
... image_size = 128 # 생성되는 이미지 해상도
... train_batch_size = 16
... eval_batch_size = 16 # 평가 동안에 샘플링할 이미지 수
... num_epochs = 50
... gradient_accumulation_steps = 1
... learning_rate = 1e-4
... lr_warmup_steps = 500
... save_image_epochs = 10
... save_model_epochs = 30
... mixed_precision = "fp16" # `no`는 float32, 자동 혼합 정밀도를 위한 `fp16`
... output_dir = "ddpm-butterflies-128" # 로컬 및 HF Hub에 저장되는 모델명
... push_to_hub = True # 저장된 모델을 HF Hub에 업로드할지 여부
... hub_private_repo = False
... overwrite_output_dir = True # 노트북을 다시 실행할 때 이전 모델에 덮어씌울지
... seed = 0
>>> config = TrainingConfig()
```
## 데이터셋 불러오기
🤗 Datasets 라이브러리와 [Smithsonian Butterflies](https://huggingface.co/datasets/huggan/smithsonian_butterflies_subset) 데이터셋을 쉽게 불러올 수 있습니다.
```py
>>> from datasets import load_dataset
>>> config.dataset_name = "huggan/smithsonian_butterflies_subset"
>>> dataset = load_dataset(config.dataset_name, split="train")
```
💡[HugGan Community Event](https://huggingface.co/huggan) 에서 추가의 데이터셋을 찾거나 로컬의 [`ImageFolder`](https://huggingface.co/docs/datasets/image_dataset#imagefolder)를 만듦으로써 나만의 데이터셋을 사용할 수 있습니다. HugGan Community Event 에 가져온 데이터셋의 경우 레포지토리의 id로 `config.dataset_name` 을 설정하고, 나만의 이미지를 사용하는 경우 `imagefolder` 를 설정합니다.
🤗 Datasets은 [`~datasets.Image`] 기능을 사용해 자동으로 이미지 데이터를 디코딩하고 [`PIL.Image`](https://pillow.readthedocs.io/en/stable/reference/Image.html)로 불러옵니다. 이를 시각화 해보면:
```py
>>> import matplotlib.pyplot as plt
>>> fig, axs = plt.subplots(1, 4, figsize=(16, 4))
>>> for i, image in enumerate(dataset[:4]["image"]):
... axs[i].imshow(image)
... axs[i].set_axis_off()
>>> fig.show()
```

이미지는 모두 다른 사이즈이기 때문에, 우선 전처리가 필요합니다:
- `Resize` 는 `config.image_size` 에 정의된 이미지 사이즈로 변경합니다.
- `RandomHorizontalFlip` 은 랜덤적으로 이미지를 미러링하여 데이터셋을 보강합니다.
- `Normalize` 는 모델이 예상하는 [-1, 1] 범위로 픽셀 값을 재조정 하는데 중요합니다.
```py
>>> from torchvision import transforms
>>> preprocess = transforms.Compose(
... [
... transforms.Resize((config.image_size, config.image_size)),
... transforms.RandomHorizontalFlip(),
... transforms.ToTensor(),
... transforms.Normalize([0.5], [0.5]),
... ]
... )
```
학습 도중에 `preprocess` 함수를 적용하려면 🤗 Datasets의 [`~datasets.Dataset.set_transform`] 방법이 사용됩니다.
```py
>>> def transform(examples):
... images = [preprocess(image.convert("RGB")) for image in examples["image"]]
... return {"images": images}
>>> dataset.set_transform(transform)
```
이미지의 크기가 조정되었는지 확인하기 위해 이미지를 다시 시각화해보세요. 이제 [DataLoader](https://pytorch.org/docs/stable/data#torch.utils.data.DataLoader)에 데이터셋을 포함해 학습할 준비가 되었습니다!
```py
>>> import torch
>>> train_dataloader = torch.utils.data.DataLoader(dataset, batch_size=config.train_batch_size, shuffle=True)
```
## UNet2DModel 생성하기
🧨 Diffusers에 사전학습된 모델들은 모델 클래스에서 원하는 파라미터로 쉽게 생성할 수 있습니다. 예를 들어, [`UNet2DModel`]를 생성하려면:
```py
>>> from diffusers import UNet2DModel
>>> model = UNet2DModel(
... sample_size=config.image_size, # 타겟 이미지 해상도
... in_channels=3, # 입력 채널 수, RGB 이미지에서 3
... out_channels=3, # 출력 채널 수
... layers_per_block=2, # UNet 블럭당 몇 개의 ResNet 레이어가 사용되는지
... block_out_channels=(128, 128, 256, 256, 512, 512), # 각 UNet 블럭을 위한 출력 채널 수
... down_block_types=(
... "DownBlock2D", # 일반적인 ResNet 다운샘플링 블럭
... "DownBlock2D",
... "DownBlock2D",
... "DownBlock2D",
... "AttnDownBlock2D", # spatial self-attention이 포함된 일반적인 ResNet 다운샘플링 블럭
... "DownBlock2D",
... ),
... up_block_types=(
... "UpBlock2D", # 일반적인 ResNet 업샘플링 블럭
... "AttnUpBlock2D", # spatial self-attention이 포함된 일반적인 ResNet 업샘플링 블럭
... "UpBlock2D",
... "UpBlock2D",
... "UpBlock2D",
... "UpBlock2D",
... ),
... )
```
샘플의 이미지 크기와 모델 출력 크기가 맞는지 빠르게 확인하기 위한 좋은 아이디어가 있습니다:
```py
>>> sample_image = dataset[0]["images"].unsqueeze(0)
>>> print("Input shape:", sample_image.shape)
Input shape: torch.Size([1, 3, 128, 128])
>>> print("Output shape:", model(sample_image, timestep=0).sample.shape)
Output shape: torch.Size([1, 3, 128, 128])
```
훌륭해요! 다음, 이미지에 약간의 노이즈를 더하기 위해 스케줄러가 필요합니다.
## 스케줄러 생성하기
스케줄러는 모델을 학습 또는 추론에 사용하는지에 따라 다르게 작동합니다. 추론시에, 스케줄러는 노이즈로부터 이미지를 생성합니다. 학습시 스케줄러는 diffusion 과정에서의 특정 포인트로부터 모델의 출력 또는 샘플을 가져와 *노이즈 스케줄* 과 *업데이트 규칙*에 따라 이미지에 노이즈를 적용합니다.
`DDPMScheduler`를 보면 이전으로부터 `sample_image`에 랜덤한 노이즈를 더하는 `add_noise` 메서드를 사용합니다:
```py
>>> import torch
>>> from PIL import Image
>>> from diffusers import DDPMScheduler
>>> noise_scheduler = DDPMScheduler(num_train_timesteps=1000)
>>> noise = torch.randn(sample_image.shape)
>>> timesteps = torch.LongTensor([50])
>>> noisy_image = noise_scheduler.add_noise(sample_image, noise, timesteps)
>>> Image.fromarray(((noisy_image.permute(0, 2, 3, 1) + 1.0) * 127.5).type(torch.uint8).numpy()[0])
```
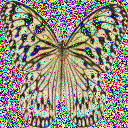
모델의 학습 목적은 이미지에 더해진 노이즈를 예측하는 것입니다. 이 단계에서 손실은 다음과 같이 계산될 수 있습니다:
```py
>>> import torch.nn.functional as F
>>> noise_pred = model(noisy_image, timesteps).sample
>>> loss = F.mse_loss(noise_pred, noise)
```
## 모델 학습하기
지금까지, 모델 학습을 시작하기 위해 많은 부분을 갖추었으며 이제 남은 것은 모든 것을 조합하는 것입니다.
우선 옵티마이저(optimizer)와 학습률 스케줄러(learning rate scheduler)가 필요할 것입니다:
```py
>>> from diffusers.optimization import get_cosine_schedule_with_warmup
>>> optimizer = torch.optim.AdamW(model.parameters(), lr=config.learning_rate)
>>> lr_scheduler = get_cosine_schedule_with_warmup(
... optimizer=optimizer,
... num_warmup_steps=config.lr_warmup_steps,
... num_training_steps=(len(train_dataloader) * config.num_epochs),
... )
```
그 후, 모델을 평가하는 방법이 필요합니다. 평가를 위해, `DDPMPipeline`을 사용해 배치의 이미지 샘플들을 생성하고 그리드 형태로 저장할 수 있습니다:
```py
>>> from diffusers import DDPMPipeline
>>> import math
>>> import os
>>> def make_grid(images, rows, cols):
... w, h = images[0].size
... grid = Image.new("RGB", size=(cols * w, rows * h))
... for i, image in enumerate(images):
... grid.paste(image, box=(i % cols * w, i // cols * h))
... return grid
>>> def evaluate(config, epoch, pipeline):
... # 랜덤한 노이즈로 부터 이미지를 추출합니다.(이는 역전파 diffusion 과정입니다.)
... # 기본 파이프라인 출력 형태는 `List[PIL.Image]` 입니다.
... images = pipeline(
... batch_size=config.eval_batch_size,
... generator=torch.manual_seed(config.seed),
... ).images
... # 이미지들을 그리드로 만들어줍니다.
... image_grid = make_grid(images, rows=4, cols=4)
... # 이미지들을 저장합니다.
... test_dir = os.path.join(config.output_dir, "samples")
... os.makedirs(test_dir, exist_ok=True)
... image_grid.save(f"{test_dir}/{epoch:04d}.png")
```
TensorBoard에 로깅, 그래디언트 누적 및 혼합 정밀도 학습을 쉽게 수행하기 위해 🤗 Accelerate를 학습 루프에 함께 앞서 말한 모든 구성 정보들을 묶어 진행할 수 있습니다. 허브에 모델을 업로드 하기 위해 레포지토리 이름 및 정보를 가져오기 위한 함수를 작성하고 허브에 업로드할 수 있습니다.
💡아래의 학습 루프는 어렵고 길어 보일 수 있지만, 나중에 한 줄의 코드로 학습을 한다면 그만한 가치가 있을 것입니다! 만약 기다리지 못하고 이미지를 생성하고 싶다면, 아래 코드를 자유롭게 붙여넣고 작동시키면 됩니다. 🤗
```py
>>> from accelerate import Accelerator
>>> from huggingface_hub import HfFolder, Repository, whoami
>>> from tqdm.auto import tqdm
>>> from pathlib import Path
>>> import os
>>> def get_full_repo_name(model_id: str, organization: str = None, token: str = None):
... if token is None:
... token = HfFolder.get_token()
... if organization is None:
... username = whoami(token)["name"]
... return f"{username}/{model_id}"
... else:
... return f"{organization}/{model_id}"
>>> def train_loop(config, model, noise_scheduler, optimizer, train_dataloader, lr_scheduler):
... # accelerator와 tensorboard 로깅 초기화
... accelerator = Accelerator(
... mixed_precision=config.mixed_precision,
... gradient_accumulation_steps=config.gradient_accumulation_steps,
... log_with="tensorboard",
... logging_dir=os.path.join(config.output_dir, "logs"),
... )
... if accelerator.is_main_process:
... if config.push_to_hub:
... repo_name = get_full_repo_name(Path(config.output_dir).name)
... repo = Repository(config.output_dir, clone_from=repo_name)
... elif config.output_dir is not None:
... os.makedirs(config.output_dir, exist_ok=True)
... accelerator.init_trackers("train_example")
... # 모든 것이 준비되었습니다.
... # 기억해야 할 특정한 순서는 없으며 준비한 방법에 제공한 것과 동일한 순서로 객체의 압축을 풀면 됩니다.
... model, optimizer, train_dataloader, lr_scheduler = accelerator.prepare(
... model, optimizer, train_dataloader, lr_scheduler
... )
... global_step = 0
... # 이제 모델을 학습합니다.
... for epoch in range(config.num_epochs):
... progress_bar = tqdm(total=len(train_dataloader), disable=not accelerator.is_local_main_process)
... progress_bar.set_description(f"Epoch {epoch}")
... for step, batch in enumerate(train_dataloader):
... clean_images = batch["images"]
... # 이미지에 더할 노이즈를 샘플링합니다.
... noise = torch.randn(clean_images.shape).to(clean_images.device)
... bs = clean_images.shape[0]
... # 각 이미지를 위한 랜덤한 타임스텝(timestep)을 샘플링합니다.
... timesteps = torch.randint(
... 0, noise_scheduler.config.num_train_timesteps, (bs,), device=clean_images.device
... ).long()
... # 각 타임스텝의 노이즈 크기에 따라 깨끗한 이미지에 노이즈를 추가합니다.
... # (이는 foward diffusion 과정입니다.)
... noisy_images = noise_scheduler.add_noise(clean_images, noise, timesteps)
... with accelerator.accumulate(model):
... # 노이즈를 반복적으로 예측합니다.
... noise_pred = model(noisy_images, timesteps, return_dict=False)[0]
... loss = F.mse_loss(noise_pred, noise)
... accelerator.backward(loss)
... accelerator.clip_grad_norm_(model.parameters(), 1.0)
... optimizer.step()
... lr_scheduler.step()
... optimizer.zero_grad()
... progress_bar.update(1)
... logs = {"loss": loss.detach().item(), "lr": lr_scheduler.get_last_lr()[0], "step": global_step}
... progress_bar.set_postfix(**logs)
... accelerator.log(logs, step=global_step)
... global_step += 1
... # 각 에포크가 끝난 후 evaluate()와 몇 가지 데모 이미지를 선택적으로 샘플링하고 모델을 저장합니다.
... if accelerator.is_main_process:
... pipeline = DDPMPipeline(unet=accelerator.unwrap_model(model), scheduler=noise_scheduler)
... if (epoch + 1) % config.save_image_epochs == 0 or epoch == config.num_epochs - 1:
... evaluate(config, epoch, pipeline)
... if (epoch + 1) % config.save_model_epochs == 0 or epoch == config.num_epochs - 1:
... if config.push_to_hub:
... repo.push_to_hub(commit_message=f"Epoch {epoch}", blocking=True)
... else:
... pipeline.save_pretrained(config.output_dir)
```
휴, 코드가 꽤 많았네요! 하지만 🤗 Accelerate의 [`~accelerate.notebook_launcher`] 함수와 학습을 시작할 준비가 되었습니다. 함수에 학습 루프, 모든 학습 인수, 학습에 사용할 프로세스 수(사용 가능한 GPU의 수를 변경할 수 있음)를 전달합니다:
```py
>>> from accelerate import notebook_launcher
>>> args = (config, model, noise_scheduler, optimizer, train_dataloader, lr_scheduler)
>>> notebook_launcher(train_loop, args, num_processes=1)
```
한번 학습이 완료되면, diffusion 모델로 생성된 최종 🦋이미지🦋를 확인해보길 바랍니다!
```py
>>> import glob
>>> sample_images = sorted(glob.glob(f"{config.output_dir}/samples/*.png"))
>>> Image.open(sample_images[-1])
```

## 다음 단계
Unconditional 이미지 생성은 학습될 수 있는 작업 중 하나의 예시입니다. 다른 작업과 학습 방법은 [🧨 Diffusers 학습 예시](../training/overview) 페이지에서 확인할 수 있습니다. 다음은 학습할 수 있는 몇 가지 예시입니다:
- [Textual Inversion](../training/text_inversion), 특정 시각적 개념을 학습시켜 생성된 이미지에 통합시키는 알고리즘입니다.
- [DreamBooth](../training/dreambooth), 주제에 대한 몇 가지 입력 이미지들이 주어지면 주제에 대한 개인화된 이미지를 생성하기 위한 기술입니다.
- [Guide](../training/text2image) 데이터셋에 Stable Diffusion 모델을 파인튜닝하는 방법입니다.
- [Guide](../training/lora) LoRA를 사용해 매우 큰 모델을 빠르게 파인튜닝하기 위한 메모리 효율적인 기술입니다.
| 0 |
hf_public_repos/diffusers/docs/source/ko | hf_public_repos/diffusers/docs/source/ko/tutorials/tutorial_overview.md | <!--Copyright 2023 The HuggingFace Team. All rights reserved.
Licensed under the Apache License, Version 2.0 (the "License"); you may not use this file except in compliance with
the License. You may obtain a copy of the License at
http://www.apache.org/licenses/LICENSE-2.0
Unless required by applicable law or agreed to in writing, software distributed under the License is distributed on
an "AS IS" BASIS, WITHOUT WARRANTIES OR CONDITIONS OF ANY KIND, either express or implied. See the License for the
specific language governing permissions and limitations under the License.
-->
# Overview
🧨 Diffusers에 오신 걸 환영합니다! 여러분이 diffusion 모델과 생성 AI를 처음 접하고, 더 많은 걸 배우고 싶으셨다면 제대로 찾아오셨습니다. 이 튜토리얼은 diffusion model을 여러분에게 젠틀하게 소개하고, 라이브러리의 기본 사항(핵심 구성요소와 🧨 Diffusers 사용법)을 이해하는 데 도움이 되도록 설계되었습니다.
여러분은 이 튜토리얼을 통해 빠르게 생성하기 위해선 추론 파이프라인을 어떻게 사용해야 하는지, 그리고 라이브러리를 modular toolbox처럼 이용해서 여러분만의 diffusion system을 구축할 수 있도록 파이프라인을 분해하는 법을 배울 수 있습니다. 다음 단원에서는 여러분이 원하는 것을 생성하기 위해 자신만의 diffusion model을 학습하는 방법을 배우게 됩니다.
튜토리얼을 완료한다면 여러분은 라이브러리를 직접 탐색하고, 자신의 프로젝트와 애플리케이션에 적용할 스킬들을 습득할 수 있을 겁니다.
[Discord](https://discord.com/invite/JfAtkvEtRb)나 [포럼](https://discuss.huggingface.co/c/discussion-related-to-httpsgithubcomhuggingfacediffusers/63) 커뮤니티에 자유롭게 참여해서 다른 사용자와 개발자들과 교류하고 협업해 보세요!
자 지금부터 diffusing을 시작해 보겠습니다! 🧨 | 0 |
hf_public_repos/diffusers/docs/source/ko | hf_public_repos/diffusers/docs/source/ko/optimization/xformers.md | <!--Copyright 2023 The HuggingFace Team. All rights reserved.
Licensed under the Apache License, Version 2.0 (the "License"); you may not use this file except in compliance with
the License. You may obtain a copy of the License at
http://www.apache.org/licenses/LICENSE-2.0
Unless required by applicable law or agreed to in writing, software distributed under the License is distributed on
an "AS IS" BASIS, WITHOUT WARRANTIES OR CONDITIONS OF ANY KIND, either express or implied. See the License for the
specific language governing permissions and limitations under the License.
-->
# xFormers 설치하기
추론과 학습 모두에 [xFormers](https://github.com/facebookresearch/xformers)를 사용하는 것이 좋습니다.
자체 테스트로 어텐션 블록에서 수행된 최적화가 더 빠른 속도와 적은 메모리 소비를 확인했습니다.
2023년 1월에 출시된 xFormers 버전 '0.0.16'부터 사전 빌드된 pip wheel을 사용하여 쉽게 설치할 수 있습니다:
```bash
pip install xformers
```
<Tip>
xFormers PIP 패키지에는 최신 버전의 PyTorch(xFormers 0.0.16에 1.13.1)가 필요합니다. 이전 버전의 PyTorch를 사용해야 하는 경우 [프로젝트 지침](https://github.com/facebookresearch/xformers#installing-xformers)의 소스를 사용해 xFormers를 설치하는 것이 좋습니다.
</Tip>
xFormers를 설치하면, [여기](fp16#memory-efficient-attention)서 설명한 것처럼 'enable_xformers_memory_efficient_attention()'을 사용하여 추론 속도를 높이고 메모리 소비를 줄일 수 있습니다.
<Tip warning={true}>
[이 이슈](https://github.com/huggingface/diffusers/issues/2234#issuecomment-1416931212)에 따르면 xFormers `v0.0.16`에서 GPU를 사용한 학습(파인 튜닝 또는 Dreambooth)을 할 수 없습니다. 해당 문제가 발견되면. 해당 코멘트를 참고해 development 버전을 설치하세요.
</Tip>
| 0 |
hf_public_repos/diffusers/docs/source/ko | hf_public_repos/diffusers/docs/source/ko/optimization/mps.md | <!--Copyright 2023 The HuggingFace Team. All rights reserved.
Licensed under the Apache License, Version 2.0 (the "License"); you may not use this file except in compliance with
the License. You may obtain a copy of the License at
http://www.apache.org/licenses/LICENSE-2.0
Unless required by applicable law or agreed to in writing, software distributed under the License is distributed on
an "AS IS" BASIS, WITHOUT WARRANTIES OR CONDITIONS OF ANY KIND, either express or implied. See the License for the
specific language governing permissions and limitations under the License.
-->
# Apple Silicon (M1/M2)에서 Stable Diffusion을 사용하는 방법
Diffusers는 Stable Diffusion 추론을 위해 PyTorch `mps`를 사용해 Apple 실리콘과 호환됩니다. 다음은 Stable Diffusion이 있는 M1 또는 M2 컴퓨터를 사용하기 위해 따라야 하는 단계입니다.
## 요구 사항
- Apple silicon (M1/M2) 하드웨어의 Mac 컴퓨터.
- macOS 12.6 또는 이후 (13.0 또는 이후 추천).
- Python arm64 버전
- PyTorch 2.0(추천) 또는 1.13(`mps`를 지원하는 최소 버전). Yhttps://pytorch.org/get-started/locally/의 지침에 따라 `pip` 또는 `conda`로 설치할 수 있습니다.
## 추론 파이프라인
아래 코도는 익숙한 `to()` 인터페이스를 사용하여 `mps` 백엔드로 Stable Diffusion 파이프라인을 M1 또는 M2 장치로 이동하는 방법을 보여줍니다.
<Tip warning={true}>
**PyTorch 1.13을 사용 중일 때 ** 추가 일회성 전달을 사용하여 파이프라인을 "프라이밍"하는 것을 추천합니다. 이것은 발견한 이상한 문제에 대한 임시 해결 방법입니다. 첫 번째 추론 전달은 후속 전달와 약간 다른 결과를 생성합니다. 이 전달은 한 번만 수행하면 되며 추론 단계를 한 번만 사용하고 결과를 폐기해도 됩니다.
</Tip>
이전 팁에서 설명한 것들을 포함한 여러 문제를 해결하므로 PyTorch 2 이상을 사용하는 것이 좋습니다.
```python
# `huggingface-cli login`에 로그인되어 있음을 확인
from diffusers import DiffusionPipeline
pipe = DiffusionPipeline.from_pretrained("runwayml/stable-diffusion-v1-5")
pipe = pipe.to("mps")
# 컴퓨터가 64GB 이하의 RAM 램일 때 추천
pipe.enable_attention_slicing()
prompt = "a photo of an astronaut riding a horse on mars"
# 처음 "워밍업" 전달 (위 설명을 보세요)
_ = pipe(prompt, num_inference_steps=1)
# 결과는 워밍업 전달 후의 CPU 장치의 결과와 일치합니다.
image = pipe(prompt).images[0]
```
## 성능 추천
M1/M2 성능은 메모리 압력에 매우 민감합니다. 시스템은 필요한 경우 자동으로 스왑되지만 스왑할 때 성능이 크게 저하됩니다.
특히 컴퓨터의 시스템 RAM이 64GB 미만이거나 512 × 512픽셀보다 큰 비표준 해상도에서 이미지를 생성하는 경우, 추론 중에 메모리 압력을 줄이고 스와핑을 방지하기 위해 *어텐션 슬라이싱*을 사용하는 것이 좋습니다. 어텐션 슬라이싱은 비용이 많이 드는 어텐션 작업을 한 번에 모두 수행하는 대신 여러 단계로 수행합니다. 일반적으로 범용 메모리가 없는 컴퓨터에서 ~20%의 성능 영향을 미치지만 64GB 이상이 아닌 경우 대부분의 Apple Silicon 컴퓨터에서 *더 나은 성능*이 관찰되었습니다.
```python
pipeline.enable_attention_slicing()
```
## Known Issues
- 여러 프롬프트를 배치로 생성하는 것은 [충돌이 발생하거나 안정적으로 작동하지 않습니다](https://github.com/huggingface/diffusers/issues/363). 우리는 이것이 [PyTorch의 `mps` 백엔드](https://github.com/pytorch/pytorch/issues/84039)와 관련이 있다고 생각합니다. 이 문제는 해결되고 있지만 지금은 배치 대신 반복 방법을 사용하는 것이 좋습니다. | 0 |
hf_public_repos/diffusers/docs/source/ko | hf_public_repos/diffusers/docs/source/ko/optimization/open_vino.md | <!--Copyright 2023 The HuggingFace Team. All rights reserved.
Licensed under the Apache License, Version 2.0 (the "License"); you may not use this file except in compliance with
the License. You may obtain a copy of the License at
http://www.apache.org/licenses/LICENSE-2.0
Unless required by applicable law or agreed to in writing, software distributed under the License is distributed on
an "AS IS" BASIS, WITHOUT WARRANTIES OR CONDITIONS OF ANY KIND, either express or implied. See the License for the
specific language governing permissions and limitations under the License.
-->
# 추론을 위한 OpenVINO 사용 방법
🤗 [Optimum](https://github.com/huggingface/optimum-intel)은 OpenVINO와 호환되는 Stable Diffusion 파이프라인을 제공합니다.
이제 다양한 Intel 프로세서에서 OpenVINO Runtime으로 쉽게 추론을 수행할 수 있습니다. ([여기](https://docs.openvino.ai/latest/openvino_docs_OV_UG_supported_plugins_Supported_Devices.html)서 지원되는 전 기기 목록을 확인하세요).
## 설치
다음 명령어로 🤗 Optimum을 설치합니다:
```
pip install optimum["openvino"]
```
## Stable Diffusion 추론
OpenVINO 모델을 불러오고 OpenVINO 런타임으로 추론을 실행하려면 `StableDiffusionPipeline`을 `OVStableDiffusionPipeline`으로 교체해야 합니다. PyTorch 모델을 불러오고 즉시 OpenVINO 형식으로 변환하려는 경우 `export=True`로 설정합니다.
```python
from optimum.intel.openvino import OVStableDiffusionPipeline
model_id = "runwayml/stable-diffusion-v1-5"
pipe = OVStableDiffusionPipeline.from_pretrained(model_id, export=True)
prompt = "a photo of an astronaut riding a horse on mars"
images = pipe(prompt).images[0]
```
[Optimum 문서](https://huggingface.co/docs/optimum/intel/inference#export-and-inference-of-stable-diffusion-models)에서 (정적 reshaping과 모델 컴파일 등의) 더 많은 예시들을 찾을 수 있습니다.
| 0 |
hf_public_repos/diffusers/docs/source/ko | hf_public_repos/diffusers/docs/source/ko/optimization/onnx.md | <!--Copyright 2023 The HuggingFace Team. All rights reserved.
Licensed under the Apache License, Version 2.0 (the "License"); you may not use this file except in compliance with
the License. You may obtain a copy of the License at
http://www.apache.org/licenses/LICENSE-2.0
Unless required by applicable law or agreed to in writing, software distributed under the License is distributed on
an "AS IS" BASIS, WITHOUT WARRANTIES OR CONDITIONS OF ANY KIND, either express or implied. See the License for the
specific language governing permissions and limitations under the License.
-->
# 추론을 위해 ONNX 런타임을 사용하는 방법
🤗 Diffusers는 ONNX Runtime과 호환되는 Stable Diffusion 파이프라인을 제공합니다. 이를 통해 ONNX(CPU 포함)를 지원하고 PyTorch의 가속 버전을 사용할 수 없는 모든 하드웨어에서 Stable Diffusion을 실행할 수 있습니다.
## 설치
다음 명령어로 ONNX Runtime를 지원하는 🤗 Optimum를 설치합니다:
```
pip install optimum["onnxruntime"]
```
## Stable Diffusion 추론
아래 코드는 ONNX 런타임을 사용하는 방법을 보여줍니다. `StableDiffusionPipeline` 대신 `OnnxStableDiffusionPipeline`을 사용해야 합니다.
PyTorch 모델을 불러오고 즉시 ONNX 형식으로 변환하려는 경우 `export=True`로 설정합니다.
```python
from optimum.onnxruntime import ORTStableDiffusionPipeline
model_id = "runwayml/stable-diffusion-v1-5"
pipe = ORTStableDiffusionPipeline.from_pretrained(model_id, export=True)
prompt = "a photo of an astronaut riding a horse on mars"
images = pipe(prompt).images[0]
pipe.save_pretrained("./onnx-stable-diffusion-v1-5")
```
파이프라인을 ONNX 형식으로 오프라인으로 내보내고 나중에 추론에 사용하려는 경우,
[`optimum-cli export`](https://huggingface.co/docs/optimum/main/en/exporters/onnx/usage_guides/export_a_model#exporting-a-model-to-onnx-using-the-cli) 명령어를 사용할 수 있습니다:
```bash
optimum-cli export onnx --model runwayml/stable-diffusion-v1-5 sd_v15_onnx/
```
그 다음 추론을 수행합니다:
```python
from optimum.onnxruntime import ORTStableDiffusionPipeline
model_id = "sd_v15_onnx"
pipe = ORTStableDiffusionPipeline.from_pretrained(model_id)
prompt = "a photo of an astronaut riding a horse on mars"
images = pipe(prompt).images[0]
```
Notice that we didn't have to specify `export=True` above.
[Optimum 문서](https://huggingface.co/docs/optimum/)에서 더 많은 예시를 찾을 수 있습니다.
## 알려진 이슈들
- 여러 프롬프트를 배치로 생성하면 너무 많은 메모리가 사용되는 것 같습니다. 이를 조사하는 동안, 배치 대신 반복 방법이 필요할 수도 있습니다.
| 0 |
hf_public_repos/diffusers/docs/source/ko | hf_public_repos/diffusers/docs/source/ko/optimization/torch2.0.md | <!--Copyright 2023 The HuggingFace Team. All rights reserved.
Licensed under the Apache License, Version 2.0 (the "License"); you may not use this file except in compliance with
the License. You may obtain a copy of the License at
http://www.apache.org/licenses/LICENSE-2.0
Unless required by applicable law or agreed to in writing, software distributed under the License is distributed on
an "AS IS" BASIS, WITHOUT WARRANTIES OR CONDITIONS OF ANY KIND, either express or implied. See the License for the
specific language governing permissions and limitations under the License.
-->
# Diffusers에서의 PyTorch 2.0 가속화 지원
`0.13.0` 버전부터 Diffusers는 [PyTorch 2.0](https://pytorch.org/get-started/pytorch-2.0/)에서의 최신 최적화를 지원합니다. 이는 다음을 포함됩니다.
1. momory-efficient attention을 사용한 가속화된 트랜스포머 지원 - `xformers`같은 추가적인 dependencies 필요 없음
2. 추가 성능 향상을 위한 개별 모델에 대한 컴파일 기능 [torch.compile](https://pytorch.org/tutorials/intermediate/torch_compile_tutorial.html) 지원
## 설치
가속화된 어텐션 구현과 및 `torch.compile()`을 사용하기 위해, pip에서 최신 버전의 PyTorch 2.0을 설치되어 있고 diffusers 0.13.0. 버전 이상인지 확인하세요. 아래 설명된 바와 같이, PyTorch 2.0이 활성화되어 있을 때 diffusers는 최적화된 어텐션 프로세서([`AttnProcessor2_0`](https://github.com/huggingface/diffusers/blob/1a5797c6d4491a879ea5285c4efc377664e0332d/src/diffusers/models/attention_processor.py#L798))를 사용합니다.
```bash
pip install --upgrade torch diffusers
```
## 가속화된 트랜스포머와 `torch.compile` 사용하기.
1. **가속화된 트랜스포머 구현**
PyTorch 2.0에는 [`torch.nn.functional.scaled_dot_product_attention`](https://pytorch.org/docs/master/generated/torch.nn.functional.scaled_dot_product_attention) 함수를 통해 최적화된 memory-efficient attention의 구현이 포함되어 있습니다. 이는 입력 및 GPU 유형에 따라 여러 최적화를 자동으로 활성화합니다. 이는 [xFormers](https://github.com/facebookresearch/xformers)의 `memory_efficient_attention`과 유사하지만 기본적으로 PyTorch에 내장되어 있습니다.
이러한 최적화는 PyTorch 2.0이 설치되어 있고 `torch.nn.functional.scaled_dot_product_attention`을 사용할 수 있는 경우 Diffusers에서 기본적으로 활성화됩니다. 이를 사용하려면 `torch 2.0`을 설치하고 파이프라인을 사용하기만 하면 됩니다. 예를 들어:
```Python
import torch
from diffusers import DiffusionPipeline
pipe = DiffusionPipeline.from_pretrained("runwayml/stable-diffusion-v1-5", torch_dtype=torch.float16)
pipe = pipe.to("cuda")
prompt = "a photo of an astronaut riding a horse on mars"
image = pipe(prompt).images[0]
```
이를 명시적으로 활성화하려면(필수는 아님) 아래와 같이 수행할 수 있습니다.
```diff
import torch
from diffusers import DiffusionPipeline
+ from diffusers.models.attention_processor import AttnProcessor2_0
pipe = DiffusionPipeline.from_pretrained("runwayml/stable-diffusion-v1-5", torch_dtype=torch.float16).to("cuda")
+ pipe.unet.set_attn_processor(AttnProcessor2_0())
prompt = "a photo of an astronaut riding a horse on mars"
image = pipe(prompt).images[0]
```
이 실행 과정은 `xFormers`만큼 빠르고 메모리적으로 효율적이어야 합니다. 자세한 내용은 [벤치마크](#benchmark)에서 확인하세요.
파이프라인을 보다 deterministic으로 만들거나 파인 튜닝된 모델을 [Core ML](https://huggingface.co/docs/diffusers/v0.16.0/en/optimization/coreml#how-to-run-stable-diffusion-with-core-ml)과 같은 다른 형식으로 변환해야 하는 경우 바닐라 어텐션 프로세서 ([`AttnProcessor`](https://github.com/huggingface/diffusers/blob/1a5797c6d4491a879ea5285c4efc377664e0332d/src/diffusers/models/attention_processor.py#L402))로 되돌릴 수 있습니다. 일반 어텐션 프로세서를 사용하려면 [`~diffusers.UNet2DConditionModel.set_default_attn_processor`] 함수를 사용할 수 있습니다:
```Python
import torch
from diffusers import DiffusionPipeline
from diffusers.models.attention_processor import AttnProcessor
pipe = DiffusionPipeline.from_pretrained("runwayml/stable-diffusion-v1-5", torch_dtype=torch.float16).to("cuda")
pipe.unet.set_default_attn_processor()
prompt = "a photo of an astronaut riding a horse on mars"
image = pipe(prompt).images[0]
```
2. **torch.compile**
추가적인 속도 향상을 위해 새로운 `torch.compile` 기능을 사용할 수 있습니다. 파이프라인의 UNet은 일반적으로 계산 비용이 가장 크기 때문에 나머지 하위 모델(텍스트 인코더와 VAE)은 그대로 두고 `unet`을 `torch.compile`로 래핑합니다. 자세한 내용과 다른 옵션은 [torch 컴파일 문서](https://pytorch.org/tutorials/intermediate/torch_compile_tutorial.html)를 참조하세요.
```python
pipe.unet = torch.compile(pipe.unet, mode="reduce-overhead", fullgraph=True)
images = pipe(prompt, num_inference_steps=steps, num_images_per_prompt=batch_size).images
```
GPU 유형에 따라 `compile()`은 가속화된 트랜스포머 최적화를 통해 **5% - 300%**의 _추가 성능 향상_을 얻을 수 있습니다. 그러나 컴파일은 Ampere(A100, 3090), Ada(4090) 및 Hopper(H100)와 같은 최신 GPU 아키텍처에서 더 많은 성능 향상을 가져올 수 있음을 참고하세요.
컴파일은 완료하는 데 약간의 시간이 걸리므로, 파이프라인을 한 번 준비한 다음 동일한 유형의 추론 작업을 여러 번 수행해야 하는 상황에 가장 적합합니다. 다른 이미지 크기에서 컴파일된 파이프라인을 호출하면 시간적 비용이 많이 들 수 있는 컴파일 작업이 다시 트리거됩니다.
## 벤치마크
PyTorch 2.0의 효율적인 어텐션 구현과 `torch.compile`을 사용하여 가장 많이 사용되는 5개의 파이프라인에 대해 다양한 GPU와 배치 크기에 걸쳐 포괄적인 벤치마크를 수행했습니다. 여기서는 [`torch.compile()`이 최적으로 활용되도록 하는](https://github.com/huggingface/diffusers/pull/3313) `diffusers 0.17.0.dev0`을 사용했습니다.
### 벤치마킹 코드
#### Stable Diffusion text-to-image
```python
from diffusers import DiffusionPipeline
import torch
path = "runwayml/stable-diffusion-v1-5"
run_compile = True # Set True / False
pipe = DiffusionPipeline.from_pretrained(path, torch_dtype=torch.float16)
pipe = pipe.to("cuda")
pipe.unet.to(memory_format=torch.channels_last)
if run_compile:
print("Run torch compile")
pipe.unet = torch.compile(pipe.unet, mode="reduce-overhead", fullgraph=True)
prompt = "ghibli style, a fantasy landscape with castles"
for _ in range(3):
images = pipe(prompt=prompt).images
```
#### Stable Diffusion image-to-image
```python
from diffusers import StableDiffusionImg2ImgPipeline
import requests
import torch
from PIL import Image
from io import BytesIO
url = "https://raw.githubusercontent.com/CompVis/stable-diffusion/main/assets/stable-samples/img2img/sketch-mountains-input.jpg"
response = requests.get(url)
init_image = Image.open(BytesIO(response.content)).convert("RGB")
init_image = init_image.resize((512, 512))
path = "runwayml/stable-diffusion-v1-5"
run_compile = True # Set True / False
pipe = StableDiffusionImg2ImgPipeline.from_pretrained(path, torch_dtype=torch.float16)
pipe = pipe.to("cuda")
pipe.unet.to(memory_format=torch.channels_last)
if run_compile:
print("Run torch compile")
pipe.unet = torch.compile(pipe.unet, mode="reduce-overhead", fullgraph=True)
prompt = "ghibli style, a fantasy landscape with castles"
for _ in range(3):
image = pipe(prompt=prompt, image=init_image).images[0]
```
#### Stable Diffusion - inpainting
```python
from diffusers import StableDiffusionInpaintPipeline
import requests
import torch
from PIL import Image
from io import BytesIO
url = "https://raw.githubusercontent.com/CompVis/stable-diffusion/main/assets/stable-samples/img2img/sketch-mountains-input.jpg"
def download_image(url):
response = requests.get(url)
return Image.open(BytesIO(response.content)).convert("RGB")
img_url = "https://raw.githubusercontent.com/CompVis/latent-diffusion/main/data/inpainting_examples/overture-creations-5sI6fQgYIuo.png"
mask_url = "https://raw.githubusercontent.com/CompVis/latent-diffusion/main/data/inpainting_examples/overture-creations-5sI6fQgYIuo_mask.png"
init_image = download_image(img_url).resize((512, 512))
mask_image = download_image(mask_url).resize((512, 512))
path = "runwayml/stable-diffusion-inpainting"
run_compile = True # Set True / False
pipe = StableDiffusionInpaintPipeline.from_pretrained(path, torch_dtype=torch.float16)
pipe = pipe.to("cuda")
pipe.unet.to(memory_format=torch.channels_last)
if run_compile:
print("Run torch compile")
pipe.unet = torch.compile(pipe.unet, mode="reduce-overhead", fullgraph=True)
prompt = "ghibli style, a fantasy landscape with castles"
for _ in range(3):
image = pipe(prompt=prompt, image=init_image, mask_image=mask_image).images[0]
```
#### ControlNet
```python
from diffusers import StableDiffusionControlNetPipeline, ControlNetModel
import requests
import torch
from PIL import Image
from io import BytesIO
url = "https://raw.githubusercontent.com/CompVis/stable-diffusion/main/assets/stable-samples/img2img/sketch-mountains-input.jpg"
response = requests.get(url)
init_image = Image.open(BytesIO(response.content)).convert("RGB")
init_image = init_image.resize((512, 512))
path = "runwayml/stable-diffusion-v1-5"
run_compile = True # Set True / False
controlnet = ControlNetModel.from_pretrained("lllyasviel/sd-controlnet-canny", torch_dtype=torch.float16)
pipe = StableDiffusionControlNetPipeline.from_pretrained(
path, controlnet=controlnet, torch_dtype=torch.float16
)
pipe = pipe.to("cuda")
pipe.unet.to(memory_format=torch.channels_last)
pipe.controlnet.to(memory_format=torch.channels_last)
if run_compile:
print("Run torch compile")
pipe.unet = torch.compile(pipe.unet, mode="reduce-overhead", fullgraph=True)
pipe.controlnet = torch.compile(pipe.controlnet, mode="reduce-overhead", fullgraph=True)
prompt = "ghibli style, a fantasy landscape with castles"
for _ in range(3):
image = pipe(prompt=prompt, image=init_image).images[0]
```
#### IF text-to-image + upscaling
```python
from diffusers import DiffusionPipeline
import torch
run_compile = True # Set True / False
pipe = DiffusionPipeline.from_pretrained("DeepFloyd/IF-I-M-v1.0", variant="fp16", text_encoder=None, torch_dtype=torch.float16)
pipe.to("cuda")
pipe_2 = DiffusionPipeline.from_pretrained("DeepFloyd/IF-II-M-v1.0", variant="fp16", text_encoder=None, torch_dtype=torch.float16)
pipe_2.to("cuda")
pipe_3 = DiffusionPipeline.from_pretrained("stabilityai/stable-diffusion-x4-upscaler", torch_dtype=torch.float16)
pipe_3.to("cuda")
pipe.unet.to(memory_format=torch.channels_last)
pipe_2.unet.to(memory_format=torch.channels_last)
pipe_3.unet.to(memory_format=torch.channels_last)
if run_compile:
pipe.unet = torch.compile(pipe.unet, mode="reduce-overhead", fullgraph=True)
pipe_2.unet = torch.compile(pipe_2.unet, mode="reduce-overhead", fullgraph=True)
pipe_3.unet = torch.compile(pipe_3.unet, mode="reduce-overhead", fullgraph=True)
prompt = "the blue hulk"
prompt_embeds = torch.randn((1, 2, 4096), dtype=torch.float16)
neg_prompt_embeds = torch.randn((1, 2, 4096), dtype=torch.float16)
for _ in range(3):
image = pipe(prompt_embeds=prompt_embeds, negative_prompt_embeds=neg_prompt_embeds, output_type="pt").images
image_2 = pipe_2(image=image, prompt_embeds=prompt_embeds, negative_prompt_embeds=neg_prompt_embeds, output_type="pt").images
image_3 = pipe_3(prompt=prompt, image=image, noise_level=100).images
```
PyTorch 2.0 및 `torch.compile()`로 얻을 수 있는 가능한 속도 향상에 대해, [Stable Diffusion text-to-image pipeline](StableDiffusionPipeline)에 대한 상대적인 속도 향상을 보여주는 차트를 5개의 서로 다른 GPU 제품군(배치 크기 4)에 대해 나타냅니다:
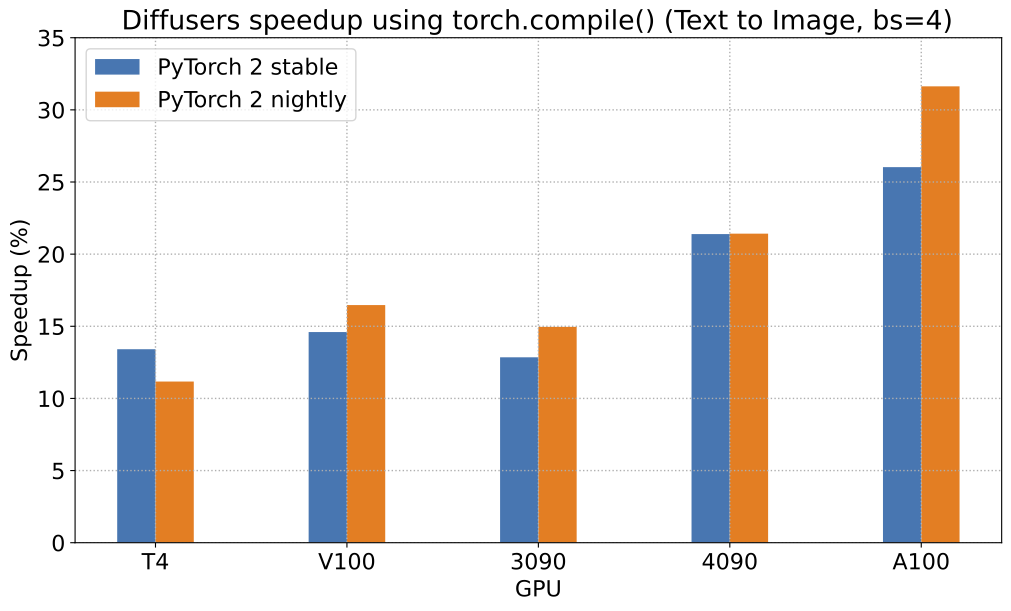
To give you an even better idea of how this speed-up holds for the other pipelines presented above, consider the following
plot that shows the benchmarking numbers from an A100 across three different batch sizes
(with PyTorch 2.0 nightly and `torch.compile()`):
이 속도 향상이 위에 제시된 다른 파이프라인에 대해서도 어떻게 유지되는지 더 잘 이해하기 위해, 세 가지의 다른 배치 크기에 걸쳐 A100의 벤치마킹(PyTorch 2.0 nightly 및 `torch.compile() 사용) 수치를 보여주는 차트를 보입니다:
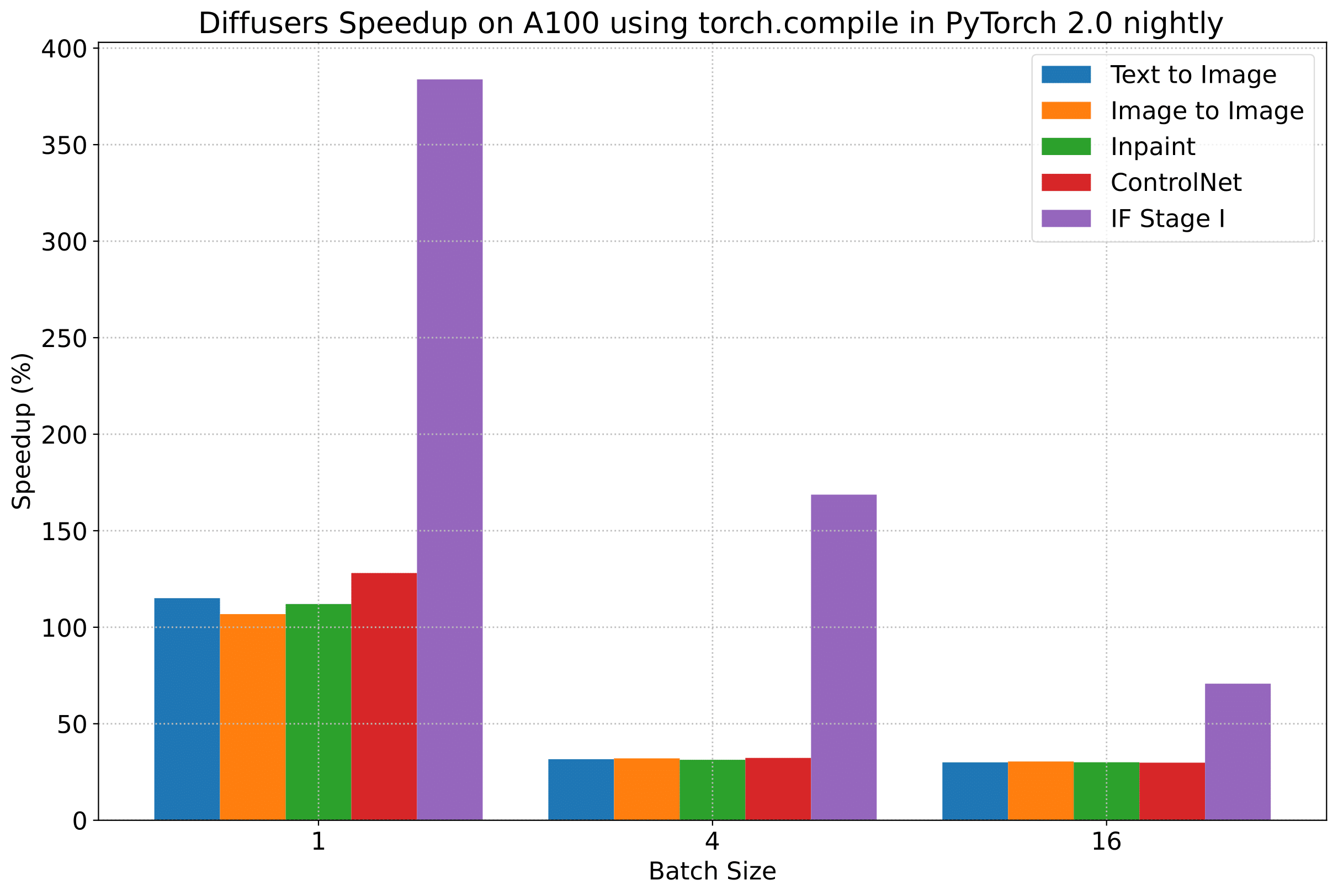
_(위 차트의 벤치마크 메트릭은 **초당 iteration 수(iterations/second)**입니다)_
그러나 투명성을 위해 모든 벤치마킹 수치를 공개합니다!
다음 표들에서는, **_초당 처리되는 iteration_** 수 측면에서의 결과를 보여줍니다.
### A100 (batch size: 1)
| **Pipeline** | **torch 2.0 - <br>no compile** | **torch nightly - <br>no compile** | **torch 2.0 - <br>compile** | **torch nightly - <br>compile** |
|:---:|:---:|:---:|:---:|:---:|
| SD - txt2img | 21.66 | 23.13 | 44.03 | 49.74 |
| SD - img2img | 21.81 | 22.40 | 43.92 | 46.32 |
| SD - inpaint | 22.24 | 23.23 | 43.76 | 49.25 |
| SD - controlnet | 15.02 | 15.82 | 32.13 | 36.08 |
| IF | 20.21 / <br>13.84 / <br>24.00 | 20.12 / <br>13.70 / <br>24.03 | ❌ | 97.34 / <br>27.23 / <br>111.66 |
### A100 (batch size: 4)
| **Pipeline** | **torch 2.0 - <br>no compile** | **torch nightly - <br>no compile** | **torch 2.0 - <br>compile** | **torch nightly - <br>compile** |
|:---:|:---:|:---:|:---:|:---:|
| SD - txt2img | 11.6 | 13.12 | 14.62 | 17.27 |
| SD - img2img | 11.47 | 13.06 | 14.66 | 17.25 |
| SD - inpaint | 11.67 | 13.31 | 14.88 | 17.48 |
| SD - controlnet | 8.28 | 9.38 | 10.51 | 12.41 |
| IF | 25.02 | 18.04 | ❌ | 48.47 |
### A100 (batch size: 16)
| **Pipeline** | **torch 2.0 - <br>no compile** | **torch nightly - <br>no compile** | **torch 2.0 - <br>compile** | **torch nightly - <br>compile** |
|:---:|:---:|:---:|:---:|:---:|
| SD - txt2img | 3.04 | 3.6 | 3.83 | 4.68 |
| SD - img2img | 2.98 | 3.58 | 3.83 | 4.67 |
| SD - inpaint | 3.04 | 3.66 | 3.9 | 4.76 |
| SD - controlnet | 2.15 | 2.58 | 2.74 | 3.35 |
| IF | 8.78 | 9.82 | ❌ | 16.77 |
### V100 (batch size: 1)
| **Pipeline** | **torch 2.0 - <br>no compile** | **torch nightly - <br>no compile** | **torch 2.0 - <br>compile** | **torch nightly - <br>compile** |
|:---:|:---:|:---:|:---:|:---:|
| SD - txt2img | 18.99 | 19.14 | 20.95 | 22.17 |
| SD - img2img | 18.56 | 19.18 | 20.95 | 22.11 |
| SD - inpaint | 19.14 | 19.06 | 21.08 | 22.20 |
| SD - controlnet | 13.48 | 13.93 | 15.18 | 15.88 |
| IF | 20.01 / <br>9.08 / <br>23.34 | 19.79 / <br>8.98 / <br>24.10 | ❌ | 55.75 / <br>11.57 / <br>57.67 |
### V100 (batch size: 4)
| **Pipeline** | **torch 2.0 - <br>no compile** | **torch nightly - <br>no compile** | **torch 2.0 - <br>compile** | **torch nightly - <br>compile** |
|:---:|:---:|:---:|:---:|:---:|
| SD - txt2img | 5.96 | 5.89 | 6.83 | 6.86 |
| SD - img2img | 5.90 | 5.91 | 6.81 | 6.82 |
| SD - inpaint | 5.99 | 6.03 | 6.93 | 6.95 |
| SD - controlnet | 4.26 | 4.29 | 4.92 | 4.93 |
| IF | 15.41 | 14.76 | ❌ | 22.95 |
### V100 (batch size: 16)
| **Pipeline** | **torch 2.0 - <br>no compile** | **torch nightly - <br>no compile** | **torch 2.0 - <br>compile** | **torch nightly - <br>compile** |
|:---:|:---:|:---:|:---:|:---:|
| SD - txt2img | 1.66 | 1.66 | 1.92 | 1.90 |
| SD - img2img | 1.65 | 1.65 | 1.91 | 1.89 |
| SD - inpaint | 1.69 | 1.69 | 1.95 | 1.93 |
| SD - controlnet | 1.19 | 1.19 | OOM after warmup | 1.36 |
| IF | 5.43 | 5.29 | ❌ | 7.06 |
### T4 (batch size: 1)
| **Pipeline** | **torch 2.0 - <br>no compile** | **torch nightly - <br>no compile** | **torch 2.0 - <br>compile** | **torch nightly - <br>compile** |
|:---:|:---:|:---:|:---:|:---:|
| SD - txt2img | 6.9 | 6.95 | 7.3 | 7.56 |
| SD - img2img | 6.84 | 6.99 | 7.04 | 7.55 |
| SD - inpaint | 6.91 | 6.7 | 7.01 | 7.37 |
| SD - controlnet | 4.89 | 4.86 | 5.35 | 5.48 |
| IF | 17.42 / <br>2.47 / <br>18.52 | 16.96 / <br>2.45 / <br>18.69 | ❌ | 24.63 / <br>2.47 / <br>23.39 |
### T4 (batch size: 4)
| **Pipeline** | **torch 2.0 - <br>no compile** | **torch nightly - <br>no compile** | **torch 2.0 - <br>compile** | **torch nightly - <br>compile** |
|:---:|:---:|:---:|:---:|:---:|
| SD - txt2img | 1.79 | 1.79 | 2.03 | 1.99 |
| SD - img2img | 1.77 | 1.77 | 2.05 | 2.04 |
| SD - inpaint | 1.81 | 1.82 | 2.09 | 2.09 |
| SD - controlnet | 1.34 | 1.27 | 1.47 | 1.46 |
| IF | 5.79 | 5.61 | ❌ | 7.39 |
### T4 (batch size: 16)
| **Pipeline** | **torch 2.0 - <br>no compile** | **torch nightly - <br>no compile** | **torch 2.0 - <br>compile** | **torch nightly - <br>compile** |
|:---:|:---:|:---:|:---:|:---:|
| SD - txt2img | 2.34s | 2.30s | OOM after 2nd iteration | 1.99s |
| SD - img2img | 2.35s | 2.31s | OOM after warmup | 2.00s |
| SD - inpaint | 2.30s | 2.26s | OOM after 2nd iteration | 1.95s |
| SD - controlnet | OOM after 2nd iteration | OOM after 2nd iteration | OOM after warmup | OOM after warmup |
| IF * | 1.44 | 1.44 | ❌ | 1.94 |
### RTX 3090 (batch size: 1)
| **Pipeline** | **torch 2.0 - <br>no compile** | **torch nightly - <br>no compile** | **torch 2.0 - <br>compile** | **torch nightly - <br>compile** |
|:---:|:---:|:---:|:---:|:---:|
| SD - txt2img | 22.56 | 22.84 | 23.84 | 25.69 |
| SD - img2img | 22.25 | 22.61 | 24.1 | 25.83 |
| SD - inpaint | 22.22 | 22.54 | 24.26 | 26.02 |
| SD - controlnet | 16.03 | 16.33 | 17.38 | 18.56 |
| IF | 27.08 / <br>9.07 / <br>31.23 | 26.75 / <br>8.92 / <br>31.47 | ❌ | 68.08 / <br>11.16 / <br>65.29 |
### RTX 3090 (batch size: 4)
| **Pipeline** | **torch 2.0 - <br>no compile** | **torch nightly - <br>no compile** | **torch 2.0 - <br>compile** | **torch nightly - <br>compile** |
|:---:|:---:|:---:|:---:|:---:|
| SD - txt2img | 6.46 | 6.35 | 7.29 | 7.3 |
| SD - img2img | 6.33 | 6.27 | 7.31 | 7.26 |
| SD - inpaint | 6.47 | 6.4 | 7.44 | 7.39 |
| SD - controlnet | 4.59 | 4.54 | 5.27 | 5.26 |
| IF | 16.81 | 16.62 | ❌ | 21.57 |
### RTX 3090 (batch size: 16)
| **Pipeline** | **torch 2.0 - <br>no compile** | **torch nightly - <br>no compile** | **torch 2.0 - <br>compile** | **torch nightly - <br>compile** |
|:---:|:---:|:---:|:---:|:---:|
| SD - txt2img | 1.7 | 1.69 | 1.93 | 1.91 |
| SD - img2img | 1.68 | 1.67 | 1.93 | 1.9 |
| SD - inpaint | 1.72 | 1.71 | 1.97 | 1.94 |
| SD - controlnet | 1.23 | 1.22 | 1.4 | 1.38 |
| IF | 5.01 | 5.00 | ❌ | 6.33 |
### RTX 4090 (batch size: 1)
| **Pipeline** | **torch 2.0 - <br>no compile** | **torch nightly - <br>no compile** | **torch 2.0 - <br>compile** | **torch nightly - <br>compile** |
|:---:|:---:|:---:|:---:|:---:|
| SD - txt2img | 40.5 | 41.89 | 44.65 | 49.81 |
| SD - img2img | 40.39 | 41.95 | 44.46 | 49.8 |
| SD - inpaint | 40.51 | 41.88 | 44.58 | 49.72 |
| SD - controlnet | 29.27 | 30.29 | 32.26 | 36.03 |
| IF | 69.71 / <br>18.78 / <br>85.49 | 69.13 / <br>18.80 / <br>85.56 | ❌ | 124.60 / <br>26.37 / <br>138.79 |
### RTX 4090 (batch size: 4)
| **Pipeline** | **torch 2.0 - <br>no compile** | **torch nightly - <br>no compile** | **torch 2.0 - <br>compile** | **torch nightly - <br>compile** |
|:---:|:---:|:---:|:---:|:---:|
| SD - txt2img | 12.62 | 12.84 | 15.32 | 15.59 |
| SD - img2img | 12.61 | 12,.79 | 15.35 | 15.66 |
| SD - inpaint | 12.65 | 12.81 | 15.3 | 15.58 |
| SD - controlnet | 9.1 | 9.25 | 11.03 | 11.22 |
| IF | 31.88 | 31.14 | ❌ | 43.92 |
### RTX 4090 (batch size: 16)
| **Pipeline** | **torch 2.0 - <br>no compile** | **torch nightly - <br>no compile** | **torch 2.0 - <br>compile** | **torch nightly - <br>compile** |
|:---:|:---:|:---:|:---:|:---:|
| SD - txt2img | 3.17 | 3.2 | 3.84 | 3.85 |
| SD - img2img | 3.16 | 3.2 | 3.84 | 3.85 |
| SD - inpaint | 3.17 | 3.2 | 3.85 | 3.85 |
| SD - controlnet | 2.23 | 2.3 | 2.7 | 2.75 |
| IF | 9.26 | 9.2 | ❌ | 13.31 |
## 참고
* Follow [this PR](https://github.com/huggingface/diffusers/pull/3313) for more details on the environment used for conducting the benchmarks.
* For the IF pipeline and batch sizes > 1, we only used a batch size of >1 in the first IF pipeline for text-to-image generation and NOT for upscaling. So, that means the two upscaling pipelines received a batch size of 1.
*Thanks to [Horace He](https://github.com/Chillee) from the PyTorch team for their support in improving our support of `torch.compile()` in Diffusers.*
* 벤치마크 수행에 사용된 환경에 대한 자세한 내용은 [이 PR](https://github.com/huggingface/diffusers/pull/3313)을 참조하세요.
* IF 파이프라인와 배치 크기 > 1의 경우 첫 번째 IF 파이프라인에서 text-to-image 생성을 위한 배치 크기 > 1만 사용했으며 업스케일링에는 사용하지 않았습니다. 즉, 두 개의 업스케일링 파이프라인이 배치 크기 1임을 의미합니다.
*Diffusers에서 `torch.compile()` 지원을 개선하는 데 도움을 준 PyTorch 팀의 [Horace He](https://github.com/Chillee)에게 감사드립니다.* | 0 |
hf_public_repos/diffusers/docs/source/ko | hf_public_repos/diffusers/docs/source/ko/optimization/opt_overview.md | <!--Copyright 2023 The HuggingFace Team. All rights reserved.
Licensed under the Apache License, Version 2.0 (the "License"); you may not use this file except in compliance with
the License. You may obtain a copy of the License at
http://www.apache.org/licenses/LICENSE-2.0
Unless required by applicable law or agreed to in writing, software distributed under the License is distributed on
an "AS IS" BASIS, WITHOUT WARRANTIES OR CONDITIONS OF ANY KIND, either express or implied. See the License for the
specific language governing permissions and limitations under the License.
-->
# 개요
노이즈가 많은 출력에서 적은 출력으로 만드는 과정으로 고품질 생성 모델의 출력을 만드는 각각의 반복되는 스텝은 많은 계산이 필요합니다. 🧨 Diffuser의 목표 중 하나는 모든 사람이 이 기술을 널리 이용할 수 있도록 하는 것이며, 여기에는 소비자 및 특수 하드웨어에서 빠른 추론을 가능하게 하는 것을 포함합니다.
이 섹션에서는 추론 속도를 최적화하고 메모리 소비를 줄이기 위한 반정밀(half-precision) 가중치 및 sliced attention과 같은 팁과 요령을 다룹니다. 또한 [`torch.compile`](https://pytorch.org/tutorials/intermediate/torch_compile_tutorial.html) 또는 [ONNX Runtime](https://onnxruntime.ai/docs/)을 사용하여 PyTorch 코드의 속도를 높이고, [xFormers](https://facebookresearch.github.io/xformers/)를 사용하여 memory-efficient attention을 활성화하는 방법을 배울 수 있습니다. Apple Silicon, Intel 또는 Habana 프로세서와 같은 특정 하드웨어에서 추론을 실행하기 위한 가이드도 있습니다. | 0 |
hf_public_repos/diffusers/docs/source/ko | hf_public_repos/diffusers/docs/source/ko/optimization/habana.md | <!--Copyright 2023 The HuggingFace Team. All rights reserved.
Licensed under the Apache License, Version 2.0 (the "License"); you may not use this file except in compliance with
the License. You may obtain a copy of the License at
http://www.apache.org/licenses/LICENSE-2.0
Unless required by applicable law or agreed to in writing, software distributed under the License is distributed on
an "AS IS" BASIS, WITHOUT WARRANTIES OR CONDITIONS OF ANY KIND, either express or implied. See the License for the
specific language governing permissions and limitations under the License.
-->
# Habana Gaudi에서 Stable Diffusion을 사용하는 방법
🤗 Diffusers는 🤗 [Optimum Habana](https://huggingface.co/docs/optimum/habana/usage_guides/stable_diffusion)를 통해서 Habana Gaudi와 호환됩니다.
## 요구 사항
- Optimum Habana 1.4 또는 이후, [여기](https://huggingface.co/docs/optimum/habana/installation)에 설치하는 방법이 있습니다.
- SynapseAI 1.8.
## 추론 파이프라인
Gaudi에서 Stable Diffusion 1 및 2로 이미지를 생성하려면 두 인스턴스를 인스턴스화해야 합니다:
- [`GaudiStableDiffusionPipeline`](https://huggingface.co/docs/optimum/habana/package_reference/stable_diffusion_pipeline)이 포함된 파이프라인. 이 파이프라인은 *텍스트-이미지 생성*을 지원합니다.
- [`GaudiDDIMScheduler`](https://huggingface.co/docs/optimum/habana/package_reference/stable_diffusion_pipeline#optimum.habana.diffusers.GaudiDDIMScheduler)이 포함된 스케줄러. 이 스케줄러는 Habana Gaudi에 최적화되어 있습니다.
파이프라인을 초기화할 때, HPU에 배포하기 위해 `use_habana=True`를 지정해야 합니다.
또한 가능한 가장 빠른 생성을 위해 `use_hpu_graphs=True`로 **HPU 그래프**를 활성화해야 합니다.
마지막으로, [Hugging Face Hub](https://huggingface.co/Habana)에서 다운로드할 수 있는 [Gaudi configuration](https://huggingface.co/docs/optimum/habana/package_reference/gaudi_config)을 지정해야 합니다.
```python
from optimum.habana import GaudiConfig
from optimum.habana.diffusers import GaudiDDIMScheduler, GaudiStableDiffusionPipeline
model_name = "stabilityai/stable-diffusion-2-base"
scheduler = GaudiDDIMScheduler.from_pretrained(model_name, subfolder="scheduler")
pipeline = GaudiStableDiffusionPipeline.from_pretrained(
model_name,
scheduler=scheduler,
use_habana=True,
use_hpu_graphs=True,
gaudi_config="Habana/stable-diffusion",
)
```
파이프라인을 호출하여 하나 이상의 프롬프트에서 배치별로 이미지를 생성할 수 있습니다.
```python
outputs = pipeline(
prompt=[
"High quality photo of an astronaut riding a horse in space",
"Face of a yellow cat, high resolution, sitting on a park bench",
],
num_images_per_prompt=10,
batch_size=4,
)
```
더 많은 정보를 얻기 위해, Optimum Habana의 [문서](https://huggingface.co/docs/optimum/habana/usage_guides/stable_diffusion)와 공식 Github 저장소에 제공된 [예시](https://github.com/huggingface/optimum-habana/tree/main/examples/stable-diffusion)를 확인하세요.
## 벤치마크
다음은 [Habana/stable-diffusion](https://huggingface.co/Habana/stable-diffusion) Gaudi 구성(혼합 정밀도 bf16/fp32)을 사용하는 Habana first-generation Gaudi 및 Gaudi2의 지연 시간입니다:
| | Latency (배치 크기 = 1) | Throughput (배치 크기 = 8) |
| ---------------------- |:------------------------:|:---------------------------:|
| first-generation Gaudi | 4.29s | 0.283 images/s |
| Gaudi2 | 1.54s | 0.904 images/s |
| 0 |
hf_public_repos/diffusers/docs/source/ko | hf_public_repos/diffusers/docs/source/ko/optimization/fp16.md | <!--Copyright 2023 The HuggingFace Team. All rights reserved.
Licensed under the Apache License, Version 2.0 (the "License"); you may not use this file except in compliance with
the License. You may obtain a copy of the License at
http://www.apache.org/licenses/LICENSE-2.0
Unless required by applicable law or agreed to in writing, software distributed under the License is distributed on
an "AS IS" BASIS, WITHOUT WARRANTIES OR CONDITIONS OF ANY KIND, either express or implied. See the License for the
specific language governing permissions and limitations under the License.
-->
# 메모리와 속도
메모리 또는 속도에 대해 🤗 Diffusers *추론*을 최적화하기 위한 몇 가지 기술과 아이디어를 제시합니다.
일반적으로, memory-efficient attention을 위해 [xFormers](https://github.com/facebookresearch/xformers) 사용을 추천하기 때문에, 추천하는 [설치 방법](xformers)을 보고 설치해 보세요.
다음 설정이 성능과 메모리에 미치는 영향에 대해 설명합니다.
| | 지연시간 | 속도 향상 |
| ---------------- | ------- | ------- |
| 별도 설정 없음 | 9.50s | x1 |
| cuDNN auto-tuner | 9.37s | x1.01 |
| fp16 | 3.61s | x2.63 |
| Channels Last 메모리 형식 | 3.30s | x2.88 |
| traced UNet | 3.21s | x2.96 |
| memory-efficient attention | 2.63s | x3.61 |
<em>
NVIDIA TITAN RTX에서 50 DDIM 스텝의 "a photo of an astronaut riding a horse on mars" 프롬프트로 512x512 크기의 단일 이미지를 생성하였습니다.
</em>
## cuDNN auto-tuner 활성화하기
[NVIDIA cuDNN](https://developer.nvidia.com/cudnn)은 컨볼루션을 계산하는 많은 알고리즘을 지원합니다. Autotuner는 짧은 벤치마크를 실행하고 주어진 입력 크기에 대해 주어진 하드웨어에서 최고의 성능을 가진 커널을 선택합니다.
**컨볼루션 네트워크**를 활용하고 있기 때문에 (다른 유형들은 현재 지원되지 않음), 다음 설정을 통해 추론 전에 cuDNN autotuner를 활성화할 수 있습니다:
```python
import torch
torch.backends.cudnn.benchmark = True
```
### fp32 대신 tf32 사용하기 (Ampere 및 이후 CUDA 장치들에서)
Ampere 및 이후 CUDA 장치에서 행렬곱 및 컨볼루션은 TensorFloat32(TF32) 모드를 사용하여 더 빠르지만 약간 덜 정확할 수 있습니다.
기본적으로 PyTorch는 컨볼루션에 대해 TF32 모드를 활성화하지만 행렬 곱셈은 활성화하지 않습니다.
네트워크에 완전한 float32 정밀도가 필요한 경우가 아니면 행렬 곱셈에 대해서도 이 설정을 활성화하는 것이 좋습니다.
이는 일반적으로 무시할 수 있는 수치의 정확도 손실이 있지만, 계산 속도를 크게 높일 수 있습니다.
그것에 대해 [여기](https://huggingface.co/docs/transformers/v4.18.0/en/performance#tf32)서 더 읽을 수 있습니다.
추론하기 전에 다음을 추가하기만 하면 됩니다:
```python
import torch
torch.backends.cuda.matmul.allow_tf32 = True
```
## 반정밀도 가중치
더 많은 GPU 메모리를 절약하고 더 빠른 속도를 얻기 위해 모델 가중치를 반정밀도(half precision)로 직접 불러오고 실행할 수 있습니다.
여기에는 `fp16`이라는 브랜치에 저장된 float16 버전의 가중치를 불러오고, 그 때 `float16` 유형을 사용하도록 PyTorch에 지시하는 작업이 포함됩니다.
```Python
pipe = StableDiffusionPipeline.from_pretrained(
"runwayml/stable-diffusion-v1-5",
torch_dtype=torch.float16,
)
pipe = pipe.to("cuda")
prompt = "a photo of an astronaut riding a horse on mars"
image = pipe(prompt).images[0]
```
<Tip warning={true}>
어떤 파이프라인에서도 [`torch.autocast`](https://pytorch.org/docs/stable/amp.html#torch.autocast) 를 사용하는 것은 검은색 이미지를 생성할 수 있고, 순수한 float16 정밀도를 사용하는 것보다 항상 느리기 때문에 사용하지 않는 것이 좋습니다.
</Tip>
## 추가 메모리 절약을 위한 슬라이스 어텐션
추가 메모리 절약을 위해, 한 번에 모두 계산하는 대신 단계적으로 계산을 수행하는 슬라이스 버전의 어텐션(attention)을 사용할 수 있습니다.
<Tip>
Attention slicing은 모델이 하나 이상의 어텐션 헤드를 사용하는 한, 배치 크기가 1인 경우에도 유용합니다.
하나 이상의 어텐션 헤드가 있는 경우 *QK^T* 어텐션 매트릭스는 상당한 양의 메모리를 절약할 수 있는 각 헤드에 대해 순차적으로 계산될 수 있습니다.
</Tip>
각 헤드에 대해 순차적으로 어텐션 계산을 수행하려면, 다음과 같이 추론 전에 파이프라인에서 [`~StableDiffusionPipeline.enable_attention_slicing`]를 호출하면 됩니다:
```Python
import torch
from diffusers import StableDiffusionPipeline
pipe = StableDiffusionPipeline.from_pretrained(
"runwayml/stable-diffusion-v1-5",
torch_dtype=torch.float16,
)
pipe = pipe.to("cuda")
prompt = "a photo of an astronaut riding a horse on mars"
pipe.enable_attention_slicing()
image = pipe(prompt).images[0]
```
추론 시간이 약 10% 느려지는 약간의 성능 저하가 있지만 이 방법을 사용하면 3.2GB 정도의 작은 VRAM으로도 Stable Diffusion을 사용할 수 있습니다!
## 더 큰 배치를 위한 sliced VAE 디코드
제한된 VRAM에서 대규모 이미지 배치를 디코딩하거나 32개 이상의 이미지가 포함된 배치를 활성화하기 위해, 배치의 latent 이미지를 한 번에 하나씩 디코딩하는 슬라이스 VAE 디코드를 사용할 수 있습니다.
이를 [`~StableDiffusionPipeline.enable_attention_slicing`] 또는 [`~StableDiffusionPipeline.enable_xformers_memory_efficient_attention`]과 결합하여 메모리 사용을 추가로 최소화할 수 있습니다.
VAE 디코드를 한 번에 하나씩 수행하려면 추론 전에 파이프라인에서 [`~StableDiffusionPipeline.enable_vae_slicing`]을 호출합니다. 예를 들어:
```Python
import torch
from diffusers import StableDiffusionPipeline
pipe = StableDiffusionPipeline.from_pretrained(
"runwayml/stable-diffusion-v1-5",
torch_dtype=torch.float16,
)
pipe = pipe.to("cuda")
prompt = "a photo of an astronaut riding a horse on mars"
pipe.enable_vae_slicing()
images = pipe([prompt] * 32).images
```
다중 이미지 배치에서 VAE 디코드가 약간의 성능 향상이 이루어집니다. 단일 이미지 배치에서는 성능 영향은 없습니다.
<a name="sequential_offloading"></a>
## 메모리 절약을 위해 가속 기능을 사용하여 CPU로 오프로딩
추가 메모리 절약을 위해 가중치를 CPU로 오프로드하고 순방향 전달을 수행할 때만 GPU로 로드할 수 있습니다.
CPU 오프로딩을 수행하려면 [`~StableDiffusionPipeline.enable_sequential_cpu_offload`]를 호출하기만 하면 됩니다:
```Python
import torch
from diffusers import StableDiffusionPipeline
pipe = StableDiffusionPipeline.from_pretrained(
"runwayml/stable-diffusion-v1-5",
torch_dtype=torch.float16,
)
prompt = "a photo of an astronaut riding a horse on mars"
pipe.enable_sequential_cpu_offload()
image = pipe(prompt).images[0]
```
그러면 메모리 소비를 3GB 미만으로 줄일 수 있습니다.
참고로 이 방법은 전체 모델이 아닌 서브모듈 수준에서 작동합니다. 이는 메모리 소비를 최소화하는 가장 좋은 방법이지만 프로세스의 반복적 특성으로 인해 추론 속도가 훨씬 느립니다. 파이프라인의 UNet 구성 요소는 여러 번 실행됩니다('num_inference_steps' 만큼). 매번 UNet의 서로 다른 서브모듈이 순차적으로 온로드된 다음 필요에 따라 오프로드되므로 메모리 이동 횟수가 많습니다.
<Tip>
또 다른 최적화 방법인 <a href="#model_offloading">모델 오프로딩</a>을 사용하는 것을 고려하십시오. 이는 훨씬 빠르지만 메모리 절약이 크지는 않습니다.
</Tip>
또한 ttention slicing과 연결해서 최소 메모리(< 2GB)로도 동작할 수 있습니다.
```Python
import torch
from diffusers import StableDiffusionPipeline
pipe = StableDiffusionPipeline.from_pretrained(
"runwayml/stable-diffusion-v1-5",
torch_dtype=torch.float16,
)
prompt = "a photo of an astronaut riding a horse on mars"
pipe.enable_sequential_cpu_offload()
pipe.enable_attention_slicing(1)
image = pipe(prompt).images[0]
```
**참고**: 'enable_sequential_cpu_offload()'를 사용할 때, 미리 파이프라인을 CUDA로 이동하지 **않는** 것이 중요합니다.그렇지 않으면 메모리 소비의 이득이 최소화됩니다. 더 많은 정보를 위해 [이 이슈](https://github.com/huggingface/diffusers/issues/1934)를 보세요.
<a name="model_offloading"></a>
## 빠른 추론과 메모리 메모리 절약을 위한 모델 오프로딩
[순차적 CPU 오프로딩](#sequential_offloading)은 이전 섹션에서 설명한 것처럼 많은 메모리를 보존하지만 필요에 따라 서브모듈을 GPU로 이동하고 새 모듈이 실행될 때 즉시 CPU로 반환되기 때문에 추론 속도가 느려집니다.
전체 모델 오프로딩은 각 모델의 구성 요소인 _modules_을 처리하는 대신, 전체 모델을 GPU로 이동하는 대안입니다. 이로 인해 추론 시간에 미치는 영향은 미미하지만(파이프라인을 'cuda'로 이동하는 것과 비교하여) 여전히 약간의 메모리를 절약할 수 있습니다.
이 시나리오에서는 파이프라인의 주요 구성 요소 중 하나만(일반적으로 텍스트 인코더, unet 및 vae) GPU에 있고, 나머지는 CPU에서 대기할 것입니다.
여러 반복을 위해 실행되는 UNet과 같은 구성 요소는 더 이상 필요하지 않을 때까지 GPU에 남아 있습니다.
이 기능은 아래와 같이 파이프라인에서 `enable_model_cpu_offload()`를 호출하여 활성화할 수 있습니다.
```Python
import torch
from diffusers import StableDiffusionPipeline
pipe = StableDiffusionPipeline.from_pretrained(
"runwayml/stable-diffusion-v1-5",
torch_dtype=torch.float16,
)
prompt = "a photo of an astronaut riding a horse on mars"
pipe.enable_model_cpu_offload()
image = pipe(prompt).images[0]
```
이는 추가적인 메모리 절약을 위한 attention slicing과도 호환됩니다.
```Python
import torch
from diffusers import StableDiffusionPipeline
pipe = StableDiffusionPipeline.from_pretrained(
"runwayml/stable-diffusion-v1-5",
torch_dtype=torch.float16,
)
prompt = "a photo of an astronaut riding a horse on mars"
pipe.enable_model_cpu_offload()
pipe.enable_attention_slicing(1)
image = pipe(prompt).images[0]
```
<Tip>
이 기능을 사용하려면 'accelerate' 버전 0.17.0 이상이 필요합니다.
</Tip>
## Channels Last 메모리 형식 사용하기
Channels Last 메모리 형식은 차원 순서를 보존하는 메모리에서 NCHW 텐서 배열을 대체하는 방법입니다.
Channels Last 텐서는 채널이 가장 조밀한 차원이 되는 방식으로 정렬됩니다(일명 픽셀당 이미지를 저장).
현재 모든 연산자 Channels Last 형식을 지원하는 것은 아니라 성능이 저하될 수 있으므로, 사용해보고 모델에 잘 작동하는지 확인하는 것이 좋습니다.
예를 들어 파이프라인의 UNet 모델이 channels Last 형식을 사용하도록 설정하려면 다음을 사용할 수 있습니다:
```python
print(pipe.unet.conv_out.state_dict()["weight"].stride()) # (2880, 9, 3, 1)
pipe.unet.to(memory_format=torch.channels_last) # in-place 연산
# 2번째 차원에서 스트라이드 1을 가지는 (2880, 1, 960, 320)로, 연산이 작동함을 증명합니다.
print(pipe.unet.conv_out.state_dict()["weight"].stride())
```
## 추적(tracing)
추적은 모델을 통해 예제 입력 텐서를 통해 실행되는데, 해당 입력이 모델의 레이어를 통과할 때 호출되는 작업을 캡처하여 실행 파일 또는 'ScriptFunction'이 반환되도록 하고, 이는 just-in-time 컴파일로 최적화됩니다.
UNet 모델을 추적하기 위해 다음을 사용할 수 있습니다:
```python
import time
import torch
from diffusers import StableDiffusionPipeline
import functools
# torch 기울기 비활성화
torch.set_grad_enabled(False)
# 변수 설정
n_experiments = 2
unet_runs_per_experiment = 50
# 입력 불러오기
def generate_inputs():
sample = torch.randn(2, 4, 64, 64).half().cuda()
timestep = torch.rand(1).half().cuda() * 999
encoder_hidden_states = torch.randn(2, 77, 768).half().cuda()
return sample, timestep, encoder_hidden_states
pipe = StableDiffusionPipeline.from_pretrained(
"runwayml/stable-diffusion-v1-5",
torch_dtype=torch.float16,
).to("cuda")
unet = pipe.unet
unet.eval()
unet.to(memory_format=torch.channels_last) # Channels Last 메모리 형식 사용
unet.forward = functools.partial(unet.forward, return_dict=False) # return_dict=False을 기본값으로 설정
# 워밍업
for _ in range(3):
with torch.inference_mode():
inputs = generate_inputs()
orig_output = unet(*inputs)
# 추적
print("tracing..")
unet_traced = torch.jit.trace(unet, inputs)
unet_traced.eval()
print("done tracing")
# 워밍업 및 그래프 최적화
for _ in range(5):
with torch.inference_mode():
inputs = generate_inputs()
orig_output = unet_traced(*inputs)
# 벤치마킹
with torch.inference_mode():
for _ in range(n_experiments):
torch.cuda.synchronize()
start_time = time.time()
for _ in range(unet_runs_per_experiment):
orig_output = unet_traced(*inputs)
torch.cuda.synchronize()
print(f"unet traced inference took {time.time() - start_time:.2f} seconds")
for _ in range(n_experiments):
torch.cuda.synchronize()
start_time = time.time()
for _ in range(unet_runs_per_experiment):
orig_output = unet(*inputs)
torch.cuda.synchronize()
print(f"unet inference took {time.time() - start_time:.2f} seconds")
# 모델 저장
unet_traced.save("unet_traced.pt")
```
그 다음, 파이프라인의 `unet` 특성을 다음과 같이 추적된 모델로 바꿀 수 있습니다.
```python
from diffusers import StableDiffusionPipeline
import torch
from dataclasses import dataclass
@dataclass
class UNet2DConditionOutput:
sample: torch.FloatTensor
pipe = StableDiffusionPipeline.from_pretrained(
"runwayml/stable-diffusion-v1-5",
torch_dtype=torch.float16,
).to("cuda")
# jitted unet 사용
unet_traced = torch.jit.load("unet_traced.pt")
# pipe.unet 삭제
class TracedUNet(torch.nn.Module):
def __init__(self):
super().__init__()
self.in_channels = pipe.unet.in_channels
self.device = pipe.unet.device
def forward(self, latent_model_input, t, encoder_hidden_states):
sample = unet_traced(latent_model_input, t, encoder_hidden_states)[0]
return UNet2DConditionOutput(sample=sample)
pipe.unet = TracedUNet()
with torch.inference_mode():
image = pipe([prompt] * 1, num_inference_steps=50).images[0]
```
## Memory-efficient attention
어텐션 블록의 대역폭을 최적화하는 최근 작업으로 GPU 메모리 사용량이 크게 향상되고 향상되었습니다.
@tridao의 가장 최근의 플래시 어텐션: [code](https://github.com/HazyResearch/flash-attention), [paper](https://arxiv.org/pdf/2205.14135.pdf).
배치 크기 1(프롬프트 1개)의 512x512 크기로 추론을 실행할 때 몇 가지 Nvidia GPU에서 얻은 속도 향상은 다음과 같습니다:
| GPU | 기준 어텐션 FP16 | 메모리 효율적인 어텐션 FP16 |
|------------------ |--------------------- |--------------------------------- |
| NVIDIA Tesla T4 | 3.5it/s | 5.5it/s |
| NVIDIA 3060 RTX | 4.6it/s | 7.8it/s |
| NVIDIA A10G | 8.88it/s | 15.6it/s |
| NVIDIA RTX A6000 | 11.7it/s | 21.09it/s |
| NVIDIA TITAN RTX | 12.51it/s | 18.22it/s |
| A100-SXM4-40GB | 18.6it/s | 29.it/s |
| A100-SXM-80GB | 18.7it/s | 29.5it/s |
이를 활용하려면 다음을 만족해야 합니다:
- PyTorch > 1.12
- Cuda 사용 가능
- [xformers 라이브러리를 설치함](xformers)
```python
from diffusers import StableDiffusionPipeline
import torch
pipe = StableDiffusionPipeline.from_pretrained(
"runwayml/stable-diffusion-v1-5",
torch_dtype=torch.float16,
).to("cuda")
pipe.enable_xformers_memory_efficient_attention()
with torch.inference_mode():
sample = pipe("a small cat")
# 선택: 이를 비활성화 하기 위해 다음을 사용할 수 있습니다.
# pipe.disable_xformers_memory_efficient_attention()
```
| 0 |
hf_public_repos/diffusers/docs/source | hf_public_repos/diffusers/docs/source/zh/quicktour.md | <!--Copyright 2023 The HuggingFace Team. All rights reserved.
Licensed under the Apache License, Version 2.0 (the "License"); you may not use this file except in compliance with
the License. You may obtain a copy of the License at
http://www.apache.org/licenses/LICENSE-2.0
Unless required by applicable law or agreed to in writing, software distributed under the License is distributed on
an "AS IS" BASIS, WITHOUT WARRANTIES OR CONDITIONS OF ANY KIND, either express or implied. See the License for the
specific language governing permissions and limitations under the License.
-->
[[open-in-colab]]
# 快速上手
训练扩散模型,是为了对随机高斯噪声进行逐步去噪,以生成令人感兴趣的样本,比如图像或者语音。
扩散模型的发展引起了人们对生成式人工智能的极大兴趣,你可能已经在网上见过扩散生成的图像了。🧨 Diffusers库的目的是让大家更易上手扩散模型。
无论你是开发人员还是普通用户,本文将向你介绍🧨 Diffusers 并帮助你快速开始生成内容!
🧨 Diffusers 库的三个主要组件:
无论你是开发者还是普通用户,这个快速指南将向你介绍🧨 Diffusers,并帮助你快速使用和生成!该库三个主要部分如下:
* [`DiffusionPipeline`]是一个高级的端到端类,旨在通过预训练的扩散模型快速生成样本进行推理。
* 作为创建扩散系统做组件的流行的预训练[模型](./api/models)框架和模块。
* 许多不同的[调度器](./api/schedulers/overview):控制如何在训练过程中添加噪声的算法,以及如何在推理过程中生成去噪图像的算法。
快速入门将告诉你如何使用[`DiffusionPipeline`]进行推理,然后指导你如何结合模型和调度器以复现[`DiffusionPipeline`]内部发生的事情。
<Tip>
快速入门是🧨[Diffusers入门](https://colab.research.google.com/github/huggingface/notebooks/blob/main/diffusers/diffusers_intro.ipynb)的简化版,可以帮助你快速上手。如果你想了解更多关于🧨 Diffusers的目标、设计理念以及关于它的核心API的更多细节,可以点击🧨[Diffusers入门](https://colab.research.google.com/github/huggingface/notebooks/blob/main/diffusers/diffusers_intro.ipynb)查看。
</Tip>
在开始之前,确认一下你已经安装好了所需要的库:
```bash
pip install --upgrade diffusers accelerate transformers
```
- [🤗 Accelerate](https://huggingface.co/docs/accelerate/index) 在推理和训练过程中加速模型加载。
- [🤗 Transformers](https://huggingface.co/docs/transformers/index) 是运行最流行的扩散模型所必须的库,比如[Stable Diffusion](https://huggingface.co/docs/diffusers/api/pipelines/stable_diffusion/overview).
## 扩散模型管道
[`DiffusionPipeline`]是用预训练的扩散系统进行推理的最简单方法。它是一个包含模型和调度器的端到端系统。你可以直接使用[`DiffusionPipeline`]完成许多任务。请查看下面的表格以了解一些支持的任务,要获取完整的支持任务列表,请查看[🧨 Diffusers 总结](./api/pipelines/overview#diffusers-summary) 。
| **任务** | **描述** | **管道**
|------------------------------|--------------------------------------------------------------------------------------------------------------|-----------------|
| Unconditional Image Generation | 从高斯噪声中生成图片 | [unconditional_image_generation](./using-diffusers/unconditional_image_generation) |
| Text-Guided Image Generation | 给定文本提示生成图像 | [conditional_image_generation](./using-diffusers/conditional_image_generation) |
| Text-Guided Image-to-Image Translation | 在文本提示的指导下调整图像 | [img2img](./using-diffusers/img2img) |
| Text-Guided Image-Inpainting | 给出图像、遮罩和文本提示,填充图像的遮罩部分 | [inpaint](./using-diffusers/inpaint) |
| Text-Guided Depth-to-Image Translation | 在文本提示的指导下调整图像的部分内容,同时通过深度估计保留其结构 | [depth2img](./using-diffusers/depth2img) |
首先创建一个[`DiffusionPipeline`]的实例,并指定要下载的pipeline检查点。
你可以使用存储在Hugging Face Hub上的任何[`DiffusionPipeline`][检查点](https://huggingface.co/models?library=diffusers&sort=downloads)。
在教程中,你将加载[`stable-diffusion-v1-5`](https://huggingface.co/runwayml/stable-diffusion-v1-5)检查点,用于文本到图像的生成。
首先创建一个[DiffusionPipeline]实例,并指定要下载的管道检查点。
您可以在Hugging Face Hub上使用[DiffusionPipeline]的任何检查点。
在本快速入门中,您将加载stable-diffusion-v1-5检查点,用于文本到图像生成。
<Tip warning={true}>。
对于[Stable Diffusion](https://huggingface.co/CompVis/stable-diffusion)模型,在运行该模型之前,请先仔细阅读[许可证](https://huggingface.co/spaces/CompVis/stable-diffusion-license)。🧨 Diffusers实现了一个[`safety_checker`](https://github.com/huggingface/diffusers/blob/main/src/diffusers/pipelines/stable_diffusion/safety_checker.py),以防止有攻击性的或有害的内容,但Stable Diffusion模型改进图像的生成能力仍有可能产生潜在的有害内容。
</Tip>
用[`~DiffusionPipeline.from_pretrained`]方法加载模型。
```python
>>> from diffusers import DiffusionPipeline
>>> pipeline = DiffusionPipeline.from_pretrained("runwayml/stable-diffusion-v1-5")
```
[`DiffusionPipeline`]会下载并缓存所有的建模、标记化和调度组件。你可以看到Stable Diffusion的pipeline是由[`UNet2DConditionModel`]和[`PNDMScheduler`]等组件组成的:
```py
>>> pipeline
StableDiffusionPipeline {
"_class_name": "StableDiffusionPipeline",
"_diffusers_version": "0.13.1",
...,
"scheduler": [
"diffusers",
"PNDMScheduler"
],
...,
"unet": [
"diffusers",
"UNet2DConditionModel"
],
"vae": [
"diffusers",
"AutoencoderKL"
]
}
```
我们强烈建议你在GPU上运行这个pipeline,因为该模型由大约14亿个参数组成。
你可以像在Pytorch里那样把生成器对象移到GPU上:
```python
>>> pipeline.to("cuda")
```
现在你可以向`pipeline`传递一个文本提示来生成图像,然后获得去噪的图像。默认情况下,图像输出被放在一个[`PIL.Image`](https://pillow.readthedocs.io/en/stable/reference/Image.html?highlight=image#the-image-class)对象中。
```python
>>> image = pipeline("An image of a squirrel in Picasso style").images[0]
>>> image
```
<div class="flex justify-center">
<img src="https://huggingface.co/datasets/huggingface/documentation-images/resolve/main/image_of_squirrel_painting.png"/>
</div>
调用`save`保存图像:
```python
>>> image.save("image_of_squirrel_painting.png")
```
### 本地管道
你也可以在本地使用管道。唯一的区别是你需提前下载权重:
```
git lfs install
git clone https://huggingface.co/runwayml/stable-diffusion-v1-5
```
将下载好的权重加载到管道中:
```python
>>> pipeline = DiffusionPipeline.from_pretrained("./stable-diffusion-v1-5")
```
现在你可以像上一节中那样运行管道了。
### 更换调度器
不同的调度器对去噪速度和质量的权衡是不同的。要想知道哪种调度器最适合你,最好的办法就是试用一下。🧨 Diffusers的主要特点之一是允许你轻松切换不同的调度器。例如,要用[`EulerDiscreteScheduler`]替换默认的[`PNDMScheduler`],用[`~diffusers.ConfigMixin.from_config`]方法加载即可:
```py
>>> from diffusers import EulerDiscreteScheduler
>>> pipeline = StableDiffusionPipeline.from_pretrained("runwayml/stable-diffusion-v1-5")
>>> pipeline.scheduler = EulerDiscreteScheduler.from_config(pipeline.scheduler.config)
```
试着用新的调度器生成一个图像,看看你能否发现不同之处。
在下一节中,你将仔细观察组成[`DiffusionPipeline`]的组件——模型和调度器,并学习如何使用这些组件来生成猫咪的图像。
## 模型
大多数模型取一个噪声样本,在每个时间点预测*噪声残差*(其他模型则直接学习预测前一个样本或速度或[`v-prediction`](https://github.com/huggingface/diffusers/blob/5e5ce13e2f89ac45a0066cb3f369462a3cf1d9ef/src/diffusers/schedulers/scheduling_ddim.py#L110)),即噪声较小的图像与输入图像的差异。你可以混搭模型创建其他扩散系统。
模型是用[`~ModelMixin.from_pretrained`]方法启动的,该方法还在本地缓存了模型权重,所以下次加载模型时更快。对于快速入门,你默认加载的是[`UNet2DModel`],这是一个基础的无条件图像生成模型,该模型有一个在猫咪图像上训练的检查点:
```py
>>> from diffusers import UNet2DModel
>>> repo_id = "google/ddpm-cat-256"
>>> model = UNet2DModel.from_pretrained(repo_id)
```
想知道模型的参数,调用 `model.config`:
```py
>>> model.config
```
模型配置是一个🧊冻结的🧊字典,意思是这些参数在模型创建后就不变了。这是特意设置的,确保在开始时用于定义模型架构的参数保持不变,其他参数仍然可以在推理过程中进行调整。
一些最重要的参数:
* `sample_size`:输入样本的高度和宽度尺寸。
* `in_channels`:输入样本的输入通道数。
* `down_block_types`和`up_block_types`:用于创建U-Net架构的下采样和上采样块的类型。
* `block_out_channels`:下采样块的输出通道数;也以相反的顺序用于上采样块的输入通道数。
* `layers_per_block`:每个U-Net块中存在的ResNet块的数量。
为了使用该模型进行推理,用随机高斯噪声生成图像形状。它应该有一个`batch`轴,因为模型可以接收多个随机噪声,一个`channel`轴,对应于输入通道的数量,以及一个`sample_size`轴,对应图像的高度和宽度。
```py
>>> import torch
>>> torch.manual_seed(0)
>>> noisy_sample = torch.randn(1, model.config.in_channels, model.config.sample_size, model.config.sample_size)
>>> noisy_sample.shape
torch.Size([1, 3, 256, 256])
```
对于推理,将噪声图像和一个`timestep`传递给模型。`timestep` 表示输入图像的噪声程度,开始时噪声更多,结束时噪声更少。这有助于模型确定其在扩散过程中的位置,是更接近开始还是结束。使用 `sample` 获得模型输出:
```py
>>> with torch.no_grad():
... noisy_residual = model(sample=noisy_sample, timestep=2).sample
```
想生成实际的样本,你需要一个调度器指导去噪过程。在下一节中,你将学习如何把模型与调度器结合起来。
## 调度器
调度器管理一个噪声样本到一个噪声较小的样本的处理过程,给出模型输出 —— 在这种情况下,它是`noisy_residual`。
<Tip>
🧨 Diffusers是一个用于构建扩散系统的工具箱。预定义好的扩散系统[`DiffusionPipeline`]能方便你快速试用,你也可以单独选择自己的模型和调度器组件来建立一个自定义的扩散系统。
</Tip>
在快速入门教程中,你将用它的[`~diffusers.ConfigMixin.from_config`]方法实例化[`DDPMScheduler`]:
```py
>>> from diffusers import DDPMScheduler
>>> scheduler = DDPMScheduler.from_config(repo_id)
>>> scheduler
DDPMScheduler {
"_class_name": "DDPMScheduler",
"_diffusers_version": "0.13.1",
"beta_end": 0.02,
"beta_schedule": "linear",
"beta_start": 0.0001,
"clip_sample": true,
"clip_sample_range": 1.0,
"num_train_timesteps": 1000,
"prediction_type": "epsilon",
"trained_betas": null,
"variance_type": "fixed_small"
}
```
<Tip>
💡 注意调度器是如何从配置中实例化的。与模型不同,调度器没有可训练的权重,而且是无参数的。
</Tip>
* `num_train_timesteps`:去噪过程的长度,或者换句话说,将随机高斯噪声处理成数据样本所需的时间步数。
* `beta_schedule`:用于推理和训练的噪声表。
* `beta_start`和`beta_end`:噪声表的开始和结束噪声值。
要预测一个噪音稍小的图像,请将 模型输出、`timestep`和当前`sample` 传递给调度器的[`~diffusers.DDPMScheduler.step`]方法:
```py
>>> less_noisy_sample = scheduler.step(model_output=noisy_residual, timestep=2, sample=noisy_sample).prev_sample
>>> less_noisy_sample.shape
```
这个 `less_noisy_sample` 去噪样本 可以被传递到下一个`timestep` ,处理后会将变得噪声更小。现在让我们把所有步骤合起来,可视化整个去噪过程。
首先,创建一个函数,对去噪后的图像进行后处理并显示为`PIL.Image`:
```py
>>> import PIL.Image
>>> import numpy as np
>>> def display_sample(sample, i):
... image_processed = sample.cpu().permute(0, 2, 3, 1)
... image_processed = (image_processed + 1.0) * 127.5
... image_processed = image_processed.numpy().astype(np.uint8)
... image_pil = PIL.Image.fromarray(image_processed[0])
... display(f"Image at step {i}")
... display(image_pil)
```
将输入和模型移到GPU上加速去噪过程:
```py
>>> model.to("cuda")
>>> noisy_sample = noisy_sample.to("cuda")
```
现在创建一个去噪循环,该循环预测噪声较少样本的残差,并使用调度程序计算噪声较少的样本:
```py
>>> import tqdm
>>> sample = noisy_sample
>>> for i, t in enumerate(tqdm.tqdm(scheduler.timesteps)):
... # 1. predict noise residual
... with torch.no_grad():
... residual = model(sample, t).sample
... # 2. compute less noisy image and set x_t -> x_t-1
... sample = scheduler.step(residual, t, sample).prev_sample
... # 3. optionally look at image
... if (i + 1) % 50 == 0:
... display_sample(sample, i + 1)
```
看!这样就从噪声中生成出一只猫了!😻
<div class="flex justify-center">
<img src="https://huggingface.co/datasets/huggingface/documentation-images/resolve/main/diffusers/diffusion-quicktour.png"/>
</div>
## 下一步
希望你在这次快速入门教程中用🧨Diffuser 生成了一些很酷的图像! 下一步你可以:
* 在[训练](./tutorials/basic_training)教程中训练或微调一个模型来生成你自己的图像。
* 查看官方和社区的[训练或微调脚本](https://github.com/huggingface/diffusers/tree/main/examples#-diffusers-examples)的例子,了解更多使用情况。
* 在[使用不同的调度器](./using-diffusers/schedulers)指南中了解更多关于加载、访问、更改和比较调度器的信息。
* 在[Stable Diffusion](./stable_diffusion)教程中探索提示工程、速度和内存优化,以及生成更高质量图像的技巧。
* 通过[在GPU上优化PyTorch](./optimization/fp16)指南,以及运行[Apple (M1/M2)上的Stable Diffusion](./optimization/mps)和[ONNX Runtime](./optimization/onnx)的教程,更深入地了解如何加速🧨Diffuser。 | 0 |
hf_public_repos/diffusers/docs/source | hf_public_repos/diffusers/docs/source/zh/index.md | <!--Copyright 2023 The HuggingFace Team. All rights reserved.
Licensed under the Apache License, Version 2.0 (the "License"); you may not use this file except in compliance with
the License. You may obtain a copy of the License at
http://www.apache.org/licenses/LICENSE-2.0
Unless required by applicable law or agreed to in writing, software distributed under the License is distributed on
an "AS IS" BASIS, WITHOUT WARRANTIES OR CONDITIONS OF ANY KIND, either express or implied. See the License for the
specific language governing permissions and limitations under the License.
-->
<p align="center">
<br>
<img src="https://raw.githubusercontent.com/huggingface/diffusers/77aadfee6a891ab9fcfb780f87c693f7a5beeb8e/docs/source/imgs/diffusers_library.jpg" width="400"/>
<br>
</p>
# 🧨 Diffusers
🤗 Diffusers 是一个值得首选用于生成图像、音频甚至 3D 分子结构的,最先进的预训练扩散模型库。
无论您是在寻找简单的推理解决方案,还是想训练自己的扩散模型,🤗 Diffusers 这一模块化工具箱都能对其提供支持。
本库的设计更偏重于[可用而非高性能](conceptual/philosophy#usability-over-performance)、[简明而非简单](conceptual/philosophy#simple-over-easy)以及[易用而非抽象](conceptual/philosophy#tweakable-contributorfriendly-over-abstraction)。
本库包含三个主要组件:
- 最先进的扩散管道 [diffusion pipelines](api/pipelines/overview),只需几行代码即可进行推理。
- 可交替使用的各种噪声调度器 [noise schedulers](api/schedulers/overview),用于平衡生成速度和质量。
- 预训练模型 [models](api/models),可作为构建模块,并与调度程序结合使用,来创建您自己的端到端扩散系统。
<div class="mt-10">
<div class="w-full flex flex-col space-y-4 md:space-y-0 md:grid md:grid-cols-2 md:gap-y-4 md:gap-x-5">
<a class="!no-underline border dark:border-gray-700 p-5 rounded-lg shadow hover:shadow-lg" href="./tutorials/tutorial_overview"
><div class="w-full text-center bg-gradient-to-br from-blue-400 to-blue-500 rounded-lg py-1.5 font-semibold mb-5 text-white text-lg leading-relaxed">Tutorials</div>
<p class="text-gray-700">Learn the fundamental skills you need to start generating outputs, build your own diffusion system, and train a diffusion model. We recommend starting here if you're using 🤗 Diffusers for the first time!</p>
</a>
<a class="!no-underline border dark:border-gray-700 p-5 rounded-lg shadow hover:shadow-lg" href="./using-diffusers/loading_overview"
><div class="w-full text-center bg-gradient-to-br from-indigo-400 to-indigo-500 rounded-lg py-1.5 font-semibold mb-5 text-white text-lg leading-relaxed">How-to guides</div>
<p class="text-gray-700">Practical guides for helping you load pipelines, models, and schedulers. You'll also learn how to use pipelines for specific tasks, control how outputs are generated, optimize for inference speed, and different training techniques.</p>
</a>
<a class="!no-underline border dark:border-gray-700 p-5 rounded-lg shadow hover:shadow-lg" href="./conceptual/philosophy"
><div class="w-full text-center bg-gradient-to-br from-pink-400 to-pink-500 rounded-lg py-1.5 font-semibold mb-5 text-white text-lg leading-relaxed">Conceptual guides</div>
<p class="text-gray-700">Understand why the library was designed the way it was, and learn more about the ethical guidelines and safety implementations for using the library.</p>
</a>
<a class="!no-underline border dark:border-gray-700 p-5 rounded-lg shadow hover:shadow-lg" href="./api/models"
><div class="w-full text-center bg-gradient-to-br from-purple-400 to-purple-500 rounded-lg py-1.5 font-semibold mb-5 text-white text-lg leading-relaxed">Reference</div>
<p class="text-gray-700">Technical descriptions of how 🤗 Diffusers classes and methods work.</p>
</a>
</div>
</div>
## 🧨 Diffusers pipelines
下表汇总了当前所有官方支持的pipelines及其对应的论文.
| 管道 | 论文/仓库 | 任务 |
|---|---|:---:|
| [alt_diffusion](./api/pipelines/alt_diffusion) | [AltCLIP: Altering the Language Encoder in CLIP for Extended Language Capabilities](https://arxiv.org/abs/2211.06679) | Image-to-Image Text-Guided Generation |
| [audio_diffusion](./api/pipelines/audio_diffusion) | [Audio Diffusion](https://github.com/teticio/audio-diffusion.git) | Unconditional Audio Generation |
| [controlnet](./api/pipelines/stable_diffusion/controlnet) | [Adding Conditional Control to Text-to-Image Diffusion Models](https://arxiv.org/abs/2302.05543) | Image-to-Image Text-Guided Generation |
| [cycle_diffusion](./api/pipelines/cycle_diffusion) | [Unifying Diffusion Models' Latent Space, with Applications to CycleDiffusion and Guidance](https://arxiv.org/abs/2210.05559) | Image-to-Image Text-Guided Generation |
| [dance_diffusion](./api/pipelines/dance_diffusion) | [Dance Diffusion](https://github.com/williamberman/diffusers.git) | Unconditional Audio Generation |
| [ddpm](./api/pipelines/ddpm) | [Denoising Diffusion Probabilistic Models](https://arxiv.org/abs/2006.11239) | Unconditional Image Generation |
| [ddim](./api/pipelines/ddim) | [Denoising Diffusion Implicit Models](https://arxiv.org/abs/2010.02502) | Unconditional Image Generation |
| [if](./if) | [**IF**](./api/pipelines/if) | Image Generation |
| [if_img2img](./if) | [**IF**](./api/pipelines/if) | Image-to-Image Generation |
| [if_inpainting](./if) | [**IF**](./api/pipelines/if) | Image-to-Image Generation |
| [latent_diffusion](./api/pipelines/latent_diffusion) | [High-Resolution Image Synthesis with Latent Diffusion Models](https://arxiv.org/abs/2112.10752)| Text-to-Image Generation |
| [latent_diffusion](./api/pipelines/latent_diffusion) | [High-Resolution Image Synthesis with Latent Diffusion Models](https://arxiv.org/abs/2112.10752)| Super Resolution Image-to-Image |
| [latent_diffusion_uncond](./api/pipelines/latent_diffusion_uncond) | [High-Resolution Image Synthesis with Latent Diffusion Models](https://arxiv.org/abs/2112.10752) | Unconditional Image Generation |
| [paint_by_example](./api/pipelines/paint_by_example) | [Paint by Example: Exemplar-based Image Editing with Diffusion Models](https://arxiv.org/abs/2211.13227) | Image-Guided Image Inpainting |
| [pndm](./api/pipelines/pndm) | [Pseudo Numerical Methods for Diffusion Models on Manifolds](https://arxiv.org/abs/2202.09778) | Unconditional Image Generation |
| [score_sde_ve](./api/pipelines/score_sde_ve) | [Score-Based Generative Modeling through Stochastic Differential Equations](https://openreview.net/forum?id=PxTIG12RRHS) | Unconditional Image Generation |
| [score_sde_vp](./api/pipelines/score_sde_vp) | [Score-Based Generative Modeling through Stochastic Differential Equations](https://openreview.net/forum?id=PxTIG12RRHS) | Unconditional Image Generation |
| [semantic_stable_diffusion](./api/pipelines/semantic_stable_diffusion) | [Semantic Guidance](https://arxiv.org/abs/2301.12247) | Text-Guided Generation |
| [stable_diffusion_text2img](./api/pipelines/stable_diffusion/text2img) | [Stable Diffusion](https://stability.ai/blog/stable-diffusion-public-release) | Text-to-Image Generation |
| [stable_diffusion_img2img](./api/pipelines/stable_diffusion/img2img) | [Stable Diffusion](https://stability.ai/blog/stable-diffusion-public-release) | Image-to-Image Text-Guided Generation |
| [stable_diffusion_inpaint](./api/pipelines/stable_diffusion/inpaint) | [Stable Diffusion](https://stability.ai/blog/stable-diffusion-public-release) | Text-Guided Image Inpainting |
| [stable_diffusion_panorama](./api/pipelines/stable_diffusion/panorama) | [MultiDiffusion](https://multidiffusion.github.io/) | Text-to-Panorama Generation |
| [stable_diffusion_pix2pix](./api/pipelines/stable_diffusion/pix2pix) | [InstructPix2Pix: Learning to Follow Image Editing Instructions](https://arxiv.org/abs/2211.09800) | Text-Guided Image Editing|
| [stable_diffusion_pix2pix_zero](./api/pipelines/stable_diffusion/pix2pix_zero) | [Zero-shot Image-to-Image Translation](https://pix2pixzero.github.io/) | Text-Guided Image Editing |
| [stable_diffusion_attend_and_excite](./api/pipelines/stable_diffusion/attend_and_excite) | [Attend-and-Excite: Attention-Based Semantic Guidance for Text-to-Image Diffusion Models](https://arxiv.org/abs/2301.13826) | Text-to-Image Generation |
| [stable_diffusion_self_attention_guidance](./api/pipelines/stable_diffusion/self_attention_guidance) | [Improving Sample Quality of Diffusion Models Using Self-Attention Guidance](https://arxiv.org/abs/2210.00939) | Text-to-Image Generation Unconditional Image Generation |
| [stable_diffusion_image_variation](./stable_diffusion/image_variation) | [Stable Diffusion Image Variations](https://github.com/LambdaLabsML/lambda-diffusers#stable-diffusion-image-variations) | Image-to-Image Generation |
| [stable_diffusion_latent_upscale](./stable_diffusion/latent_upscale) | [Stable Diffusion Latent Upscaler](https://twitter.com/StabilityAI/status/1590531958815064065) | Text-Guided Super Resolution Image-to-Image |
| [stable_diffusion_model_editing](./api/pipelines/stable_diffusion/model_editing) | [Editing Implicit Assumptions in Text-to-Image Diffusion Models](https://time-diffusion.github.io/) | Text-to-Image Model Editing |
| [stable_diffusion_2](./api/pipelines/stable_diffusion_2) | [Stable Diffusion 2](https://stability.ai/blog/stable-diffusion-v2-release) | Text-to-Image Generation |
| [stable_diffusion_2](./api/pipelines/stable_diffusion_2) | [Stable Diffusion 2](https://stability.ai/blog/stable-diffusion-v2-release) | Text-Guided Image Inpainting |
| [stable_diffusion_2](./api/pipelines/stable_diffusion_2) | [Depth-Conditional Stable Diffusion](https://github.com/Stability-AI/stablediffusion#depth-conditional-stable-diffusion) | Depth-to-Image Generation |
| [stable_diffusion_2](./api/pipelines/stable_diffusion_2) | [Stable Diffusion 2](https://stability.ai/blog/stable-diffusion-v2-release) | Text-Guided Super Resolution Image-to-Image |
| [stable_diffusion_safe](./api/pipelines/stable_diffusion_safe) | [Safe Stable Diffusion](https://arxiv.org/abs/2211.05105) | Text-Guided Generation |
| [stable_unclip](./stable_unclip) | Stable unCLIP | Text-to-Image Generation |
| [stable_unclip](./stable_unclip) | Stable unCLIP | Image-to-Image Text-Guided Generation |
| [stochastic_karras_ve](./api/pipelines/stochastic_karras_ve) | [Elucidating the Design Space of Diffusion-Based Generative Models](https://arxiv.org/abs/2206.00364) | Unconditional Image Generation |
| [text_to_video_sd](./api/pipelines/text_to_video) | [Modelscope's Text-to-video-synthesis Model in Open Domain](https://modelscope.cn/models/damo/text-to-video-synthesis/summary) | Text-to-Video Generation |
| [unclip](./api/pipelines/unclip) | [Hierarchical Text-Conditional Image Generation with CLIP Latents](https://arxiv.org/abs/2204.06125)(implementation by [kakaobrain](https://github.com/kakaobrain/karlo)) | Text-to-Image Generation |
| [versatile_diffusion](./api/pipelines/versatile_diffusion) | [Versatile Diffusion: Text, Images and Variations All in One Diffusion Model](https://arxiv.org/abs/2211.08332) | Text-to-Image Generation |
| [versatile_diffusion](./api/pipelines/versatile_diffusion) | [Versatile Diffusion: Text, Images and Variations All in One Diffusion Model](https://arxiv.org/abs/2211.08332) | Image Variations Generation |
| [versatile_diffusion](./api/pipelines/versatile_diffusion) | [Versatile Diffusion: Text, Images and Variations All in One Diffusion Model](https://arxiv.org/abs/2211.08332) | Dual Image and Text Guided Generation |
| [vq_diffusion](./api/pipelines/vq_diffusion) | [Vector Quantized Diffusion Model for Text-to-Image Synthesis](https://arxiv.org/abs/2111.14822) | Text-to-Image Generation |
| 0 |
hf_public_repos/diffusers/docs/source | hf_public_repos/diffusers/docs/source/zh/installation.md | <!--Copyright 2023 The HuggingFace Team. All rights reserved.
Licensed under the Apache License, Version 2.0 (the "License"); you may not use this file except in compliance with
the License. You may obtain a copy of the License at
http://www.apache.org/licenses/LICENSE-2.0
Unless required by applicable law or agreed to in writing, software distributed under the License is distributed on
an "AS IS" BASIS, WITHOUT WARRANTIES OR CONDITIONS OF ANY KIND, either express or implied. See the License for the
specific language governing permissions and limitations under the License.
-->
# 安装
在你正在使用的任意深度学习框架中安装 🤗 Diffusers 。
🤗 Diffusers已在Python 3.7+、PyTorch 1.7.0+和Flax上进行了测试。按照下面的安装说明,针对你正在使用的深度学习框架进行安装:
- [PyTorch](https://pytorch.org/get-started/locally/) installation instructions.
- [Flax](https://flax.readthedocs.io/en/latest/) installation instructions.
## 使用pip安装
你需要在[虚拟环境](https://docs.python.org/3/library/venv.html)中安装 🤗 Diffusers 。
如果你对 Python 虚拟环境不熟悉,可以看看这个[教程](https://packaging.python.org/guides/installing-using-pip-and-virtual-environments/).
在虚拟环境中,你可以轻松管理不同的项目,避免依赖项之间的兼容性问题。
首先,在你的项目目录下创建一个虚拟环境:
```bash
python -m venv .env
```
激活虚拟环境:
```bash
source .env/bin/activate
```
现在,你就可以安装 🤗 Diffusers了!使用下边这个命令:
**PyTorch**
```bash
pip install diffusers["torch"]
```
**Flax**
```bash
pip install diffusers["flax"]
```
## 从源代码安装
在从源代码安装 `diffusers` 之前,确保你已经安装了 `torch` 和 `accelerate`。
`torch`的安装教程可以看 `torch` [文档](https://pytorch.org/get-started/locally/#start-locally).
安装 `accelerate`
```bash
pip install accelerate
```
从源码安装 🤗 Diffusers 需要使用以下命令:
```bash
pip install git+https://github.com/huggingface/diffusers
```
这个命令安装的是最新的 `main`版本,而不是最近的`stable`版。
`main`是一直和最新进展保持一致的。比如,上次发布的正式版中有bug,在`main`中可以看到这个bug被修复了,但是新的正式版此时尚未推出。
但是这也意味着 `main`版本不保证是稳定的。
我们努力保持`main`版本正常运行,大多数问题都能在几个小时或一天之内解决
如果你遇到了问题,可以提 [Issue](https://github.com/huggingface/transformers/issues),这样我们就能更快修复问题了。
## 可修改安装
如果你想做以下两件事,那你可能需要一个可修改代码的安装方式:
* 使用 `main`版本的源代码。
* 为 🤗 Diffusers 贡献,需要测试代码中的变化。
使用以下命令克隆并安装 🤗 Diffusers:
```bash
git clone https://github.com/huggingface/diffusers.git
cd diffusers
```
**PyTorch**
```
pip install -e ".[torch]"
```
**Flax**
```
pip install -e ".[flax]"
```
这些命令将连接到你克隆的版本库和你的 Python 库路径。
现在,不只是在通常的库路径,Python 还会在你克隆的文件夹内寻找包。
例如,如果你的 Python 包通常安装在 `~/anaconda3/envs/main/lib/python3.7/Site-packages/`,Python 也会搜索你克隆到的文件夹。`~/diffusers/`。
<Tip warning={true}>
如果你想继续使用这个库,你必须保留 `diffusers` 文件夹。
</Tip>
现在你可以用下面的命令轻松地将你克隆的 🤗 Diffusers 库更新到最新版本。
```bash
cd ~/diffusers/
git pull
```
你的Python环境将在下次运行时找到`main`版本的 🤗 Diffusers。
## 注意 Telemetry 日志
我们的库会在使用`from_pretrained()`请求期间收集 telemetry 信息。这些数据包括Diffusers和PyTorch/Flax的版本,请求的模型或管道类,以及预训练检查点的路径(如果它被托管在Hub上的话)。
这些使用数据有助于我们调试问题并确定新功能的开发优先级。
Telemetry 数据仅在从 HuggingFace Hub 中加载模型和管道时发送,而不会在本地使用期间收集。
我们知道,并不是每个人都想分享这些的信息,我们尊重您的隐私,
因此您可以通过在终端中设置 `DISABLE_TELEMETRY` 环境变量从而禁用 Telemetry 数据收集:
Linux/MacOS :
```bash
export DISABLE_TELEMETRY=YES
```
Windows :
```bash
set DISABLE_TELEMETRY=YES
``` | 0 |
hf_public_repos/diffusers/docs/source | hf_public_repos/diffusers/docs/source/zh/_toctree.yml | - sections:
- local: index
title: 🧨 Diffusers
- local: quicktour
title: 快速入门
- local: installation
title: 安装
title: 开始
| 0 |
hf_public_repos/diffusers/docs/source | hf_public_repos/diffusers/docs/source/en/quicktour.md | <!--Copyright 2023 The HuggingFace Team. All rights reserved.
Licensed under the Apache License, Version 2.0 (the "License"); you may not use this file except in compliance with
the License. You may obtain a copy of the License at
http://www.apache.org/licenses/LICENSE-2.0
Unless required by applicable law or agreed to in writing, software distributed under the License is distributed on
an "AS IS" BASIS, WITHOUT WARRANTIES OR CONDITIONS OF ANY KIND, either express or implied. See the License for the
specific language governing permissions and limitations under the License.
-->
[[open-in-colab]]
# Quicktour
Diffusion models are trained to denoise random Gaussian noise step-by-step to generate a sample of interest, such as an image or audio. This has sparked a tremendous amount of interest in generative AI, and you have probably seen examples of diffusion generated images on the internet. 🧨 Diffusers is a library aimed at making diffusion models widely accessible to everyone.
Whether you're a developer or an everyday user, this quicktour will introduce you to 🧨 Diffusers and help you get up and generating quickly! There are three main components of the library to know about:
* The [`DiffusionPipeline`] is a high-level end-to-end class designed to rapidly generate samples from pretrained diffusion models for inference.
* Popular pretrained [model](./api/models) architectures and modules that can be used as building blocks for creating diffusion systems.
* Many different [schedulers](./api/schedulers/overview) - algorithms that control how noise is added for training, and how to generate denoised images during inference.
The quicktour will show you how to use the [`DiffusionPipeline`] for inference, and then walk you through how to combine a model and scheduler to replicate what's happening inside the [`DiffusionPipeline`].
<Tip>
The quicktour is a simplified version of the introductory 🧨 Diffusers [notebook](https://colab.research.google.com/github/huggingface/notebooks/blob/main/diffusers/diffusers_intro.ipynb) to help you get started quickly. If you want to learn more about 🧨 Diffusers goal, design philosophy, and additional details about it's core API, check out the notebook!
</Tip>
Before you begin, make sure you have all the necessary libraries installed:
```py
# uncomment to install the necessary libraries in Colab
#!pip install --upgrade diffusers accelerate transformers
```
- [🤗 Accelerate](https://huggingface.co/docs/accelerate/index) speeds up model loading for inference and training.
- [🤗 Transformers](https://huggingface.co/docs/transformers/index) is required to run the most popular diffusion models, such as [Stable Diffusion](https://huggingface.co/docs/diffusers/api/pipelines/stable_diffusion/overview).
## DiffusionPipeline
The [`DiffusionPipeline`] is the easiest way to use a pretrained diffusion system for inference. It is an end-to-end system containing the model and the scheduler. You can use the [`DiffusionPipeline`] out-of-the-box for many tasks. Take a look at the table below for some supported tasks, and for a complete list of supported tasks, check out the [🧨 Diffusers Summary](./api/pipelines/overview#diffusers-summary) table.
| **Task** | **Description** | **Pipeline**
|------------------------------|--------------------------------------------------------------------------------------------------------------|-----------------|
| Unconditional Image Generation | generate an image from Gaussian noise | [unconditional_image_generation](./using-diffusers/unconditional_image_generation) |
| Text-Guided Image Generation | generate an image given a text prompt | [conditional_image_generation](./using-diffusers/conditional_image_generation) |
| Text-Guided Image-to-Image Translation | adapt an image guided by a text prompt | [img2img](./using-diffusers/img2img) |
| Text-Guided Image-Inpainting | fill the masked part of an image given the image, the mask and a text prompt | [inpaint](./using-diffusers/inpaint) |
| Text-Guided Depth-to-Image Translation | adapt parts of an image guided by a text prompt while preserving structure via depth estimation | [depth2img](./using-diffusers/depth2img) |
Start by creating an instance of a [`DiffusionPipeline`] and specify which pipeline checkpoint you would like to download.
You can use the [`DiffusionPipeline`] for any [checkpoint](https://huggingface.co/models?library=diffusers&sort=downloads) stored on the Hugging Face Hub.
In this quicktour, you'll load the [`stable-diffusion-v1-5`](https://huggingface.co/runwayml/stable-diffusion-v1-5) checkpoint for text-to-image generation.
<Tip warning={true}>
For [Stable Diffusion](https://huggingface.co/CompVis/stable-diffusion) models, please carefully read the [license](https://huggingface.co/spaces/CompVis/stable-diffusion-license) first before running the model. 🧨 Diffusers implements a [`safety_checker`](https://github.com/huggingface/diffusers/blob/main/src/diffusers/pipelines/stable_diffusion/safety_checker.py) to prevent offensive or harmful content, but the model's improved image generation capabilities can still produce potentially harmful content.
</Tip>
Load the model with the [`~DiffusionPipeline.from_pretrained`] method:
```python
>>> from diffusers import DiffusionPipeline
>>> pipeline = DiffusionPipeline.from_pretrained("runwayml/stable-diffusion-v1-5")
```
The [`DiffusionPipeline`] downloads and caches all modeling, tokenization, and scheduling components. You'll see that the Stable Diffusion pipeline is composed of the [`UNet2DConditionModel`] and [`PNDMScheduler`] among other things:
```py
>>> pipeline
StableDiffusionPipeline {
"_class_name": "StableDiffusionPipeline",
"_diffusers_version": "0.13.1",
...,
"scheduler": [
"diffusers",
"PNDMScheduler"
],
...,
"unet": [
"diffusers",
"UNet2DConditionModel"
],
"vae": [
"diffusers",
"AutoencoderKL"
]
}
```
We strongly recommend running the pipeline on a GPU because the model consists of roughly 1.4 billion parameters.
You can move the generator object to a GPU, just like you would in PyTorch:
```python
>>> pipeline.to("cuda")
```
Now you can pass a text prompt to the `pipeline` to generate an image, and then access the denoised image. By default, the image output is wrapped in a [`PIL.Image`](https://pillow.readthedocs.io/en/stable/reference/Image.html?highlight=image#the-image-class) object.
```python
>>> image = pipeline("An image of a squirrel in Picasso style").images[0]
>>> image
```
<div class="flex justify-center">
<img src="https://huggingface.co/datasets/huggingface/documentation-images/resolve/main/image_of_squirrel_painting.png"/>
</div>
Save the image by calling `save`:
```python
>>> image.save("image_of_squirrel_painting.png")
```
### Local pipeline
You can also use the pipeline locally. The only difference is you need to download the weights first:
```bash
!git lfs install
!git clone https://huggingface.co/runwayml/stable-diffusion-v1-5
```
Then load the saved weights into the pipeline:
```python
>>> pipeline = DiffusionPipeline.from_pretrained("./stable-diffusion-v1-5")
```
Now you can run the pipeline as you would in the section above.
### Swapping schedulers
Different schedulers come with different denoising speeds and quality trade-offs. The best way to find out which one works best for you is to try them out! One of the main features of 🧨 Diffusers is to allow you to easily switch between schedulers. For example, to replace the default [`PNDMScheduler`] with the [`EulerDiscreteScheduler`], load it with the [`~diffusers.ConfigMixin.from_config`] method:
```py
>>> from diffusers import EulerDiscreteScheduler
>>> pipeline = DiffusionPipeline.from_pretrained("runwayml/stable-diffusion-v1-5")
>>> pipeline.scheduler = EulerDiscreteScheduler.from_config(pipeline.scheduler.config)
```
Try generating an image with the new scheduler and see if you notice a difference!
In the next section, you'll take a closer look at the components - the model and scheduler - that make up the [`DiffusionPipeline`] and learn how to use these components to generate an image of a cat.
## Models
Most models take a noisy sample, and at each timestep it predicts the *noise residual* (other models learn to predict the previous sample directly or the velocity or [`v-prediction`](https://github.com/huggingface/diffusers/blob/5e5ce13e2f89ac45a0066cb3f369462a3cf1d9ef/src/diffusers/schedulers/scheduling_ddim.py#L110)), the difference between a less noisy image and the input image. You can mix and match models to create other diffusion systems.
Models are initiated with the [`~ModelMixin.from_pretrained`] method which also locally caches the model weights so it is faster the next time you load the model. For the quicktour, you'll load the [`UNet2DModel`], a basic unconditional image generation model with a checkpoint trained on cat images:
```py
>>> from diffusers import UNet2DModel
>>> repo_id = "google/ddpm-cat-256"
>>> model = UNet2DModel.from_pretrained(repo_id)
```
To access the model parameters, call `model.config`:
```py
>>> model.config
```
The model configuration is a 🧊 frozen 🧊 dictionary, which means those parameters can't be changed after the model is created. This is intentional and ensures that the parameters used to define the model architecture at the start remain the same, while other parameters can still be adjusted during inference.
Some of the most important parameters are:
* `sample_size`: the height and width dimension of the input sample.
* `in_channels`: the number of input channels of the input sample.
* `down_block_types` and `up_block_types`: the type of down- and upsampling blocks used to create the UNet architecture.
* `block_out_channels`: the number of output channels of the downsampling blocks; also used in reverse order for the number of input channels of the upsampling blocks.
* `layers_per_block`: the number of ResNet blocks present in each UNet block.
To use the model for inference, create the image shape with random Gaussian noise. It should have a `batch` axis because the model can receive multiple random noises, a `channel` axis corresponding to the number of input channels, and a `sample_size` axis for the height and width of the image:
```py
>>> import torch
>>> torch.manual_seed(0)
>>> noisy_sample = torch.randn(1, model.config.in_channels, model.config.sample_size, model.config.sample_size)
>>> noisy_sample.shape
torch.Size([1, 3, 256, 256])
```
For inference, pass the noisy image to the model and a `timestep`. The `timestep` indicates how noisy the input image is, with more noise at the beginning and less at the end. This helps the model determine its position in the diffusion process, whether it is closer to the start or the end. Use the `sample` method to get the model output:
```py
>>> with torch.no_grad():
... noisy_residual = model(sample=noisy_sample, timestep=2).sample
```
To generate actual examples though, you'll need a scheduler to guide the denoising process. In the next section, you'll learn how to couple a model with a scheduler.
## Schedulers
Schedulers manage going from a noisy sample to a less noisy sample given the model output - in this case, it is the `noisy_residual`.
<Tip>
🧨 Diffusers is a toolbox for building diffusion systems. While the [`DiffusionPipeline`] is a convenient way to get started with a pre-built diffusion system, you can also choose your own model and scheduler components separately to build a custom diffusion system.
</Tip>
For the quicktour, you'll instantiate the [`DDPMScheduler`] with it's [`~diffusers.ConfigMixin.from_config`] method:
```py
>>> from diffusers import DDPMScheduler
>>> scheduler = DDPMScheduler.from_config(repo_id)
>>> scheduler
DDPMScheduler {
"_class_name": "DDPMScheduler",
"_diffusers_version": "0.13.1",
"beta_end": 0.02,
"beta_schedule": "linear",
"beta_start": 0.0001,
"clip_sample": true,
"clip_sample_range": 1.0,
"num_train_timesteps": 1000,
"prediction_type": "epsilon",
"trained_betas": null,
"variance_type": "fixed_small"
}
```
<Tip>
💡 Notice how the scheduler is instantiated from a configuration. Unlike a model, a scheduler does not have trainable weights and is parameter-free!
</Tip>
Some of the most important parameters are:
* `num_train_timesteps`: the length of the denoising process or in other words, the number of timesteps required to process random Gaussian noise into a data sample.
* `beta_schedule`: the type of noise schedule to use for inference and training.
* `beta_start` and `beta_end`: the start and end noise values for the noise schedule.
To predict a slightly less noisy image, pass the following to the scheduler's [`~diffusers.DDPMScheduler.step`] method: model output, `timestep`, and current `sample`.
```py
>>> less_noisy_sample = scheduler.step(model_output=noisy_residual, timestep=2, sample=noisy_sample).prev_sample
>>> less_noisy_sample.shape
```
The `less_noisy_sample` can be passed to the next `timestep` where it'll get even less noisier! Let's bring it all together now and visualize the entire denoising process.
First, create a function that postprocesses and displays the denoised image as a `PIL.Image`:
```py
>>> import PIL.Image
>>> import numpy as np
>>> def display_sample(sample, i):
... image_processed = sample.cpu().permute(0, 2, 3, 1)
... image_processed = (image_processed + 1.0) * 127.5
... image_processed = image_processed.numpy().astype(np.uint8)
... image_pil = PIL.Image.fromarray(image_processed[0])
... display(f"Image at step {i}")
... display(image_pil)
```
To speed up the denoising process, move the input and model to a GPU:
```py
>>> model.to("cuda")
>>> noisy_sample = noisy_sample.to("cuda")
```
Now create a denoising loop that predicts the residual of the less noisy sample, and computes the less noisy sample with the scheduler:
```py
>>> import tqdm
>>> sample = noisy_sample
>>> for i, t in enumerate(tqdm.tqdm(scheduler.timesteps)):
... # 1. predict noise residual
... with torch.no_grad():
... residual = model(sample, t).sample
... # 2. compute less noisy image and set x_t -> x_t-1
... sample = scheduler.step(residual, t, sample).prev_sample
... # 3. optionally look at image
... if (i + 1) % 50 == 0:
... display_sample(sample, i + 1)
```
Sit back and watch as a cat is generated from nothing but noise! 😻
<div class="flex justify-center">
<img src="https://huggingface.co/datasets/huggingface/documentation-images/resolve/main/diffusers/diffusion-quicktour.png"/>
</div>
## Next steps
Hopefully you generated some cool images with 🧨 Diffusers in this quicktour! For your next steps, you can:
* Train or finetune a model to generate your own images in the [training](./tutorials/basic_training) tutorial.
* See example official and community [training or finetuning scripts](https://github.com/huggingface/diffusers/tree/main/examples#-diffusers-examples) for a variety of use cases.
* Learn more about loading, accessing, changing and comparing schedulers in the [Using different Schedulers](./using-diffusers/schedulers) guide.
* Explore prompt engineering, speed and memory optimizations, and tips and tricks for generating higher quality images with the [Stable Diffusion](./stable_diffusion) guide.
* Dive deeper into speeding up 🧨 Diffusers with guides on [optimized PyTorch on a GPU](./optimization/fp16), and inference guides for running [Stable Diffusion on Apple Silicon (M1/M2)](./optimization/mps) and [ONNX Runtime](./optimization/onnx).
| 0 |
hf_public_repos/diffusers/docs/source | hf_public_repos/diffusers/docs/source/en/index.md | <!--Copyright 2023 The HuggingFace Team. All rights reserved.
Licensed under the Apache License, Version 2.0 (the "License"); you may not use this file except in compliance with
the License. You may obtain a copy of the License at
http://www.apache.org/licenses/LICENSE-2.0
Unless required by applicable law or agreed to in writing, software distributed under the License is distributed on
an "AS IS" BASIS, WITHOUT WARRANTIES OR CONDITIONS OF ANY KIND, either express or implied. See the License for the
specific language governing permissions and limitations under the License.
-->
<p align="center">
<br>
<img src="https://raw.githubusercontent.com/huggingface/diffusers/77aadfee6a891ab9fcfb780f87c693f7a5beeb8e/docs/source/imgs/diffusers_library.jpg" width="400"/>
<br>
</p>
# Diffusers
🤗 Diffusers is the go-to library for state-of-the-art pretrained diffusion models for generating images, audio, and even 3D structures of molecules. Whether you're looking for a simple inference solution or want to train your own diffusion model, 🤗 Diffusers is a modular toolbox that supports both. Our library is designed with a focus on [usability over performance](conceptual/philosophy#usability-over-performance), [simple over easy](conceptual/philosophy#simple-over-easy), and [customizability over abstractions](conceptual/philosophy#tweakable-contributorfriendly-over-abstraction).
The library has three main components:
- State-of-the-art [diffusion pipelines](api/pipelines/overview) for inference with just a few lines of code.
- Interchangeable [noise schedulers](api/schedulers/overview) for balancing trade-offs between generation speed and quality.
- Pretrained [models](api/models) that can be used as building blocks, and combined with schedulers, for creating your own end-to-end diffusion systems.
<div class="mt-10">
<div class="w-full flex flex-col space-y-4 md:space-y-0 md:grid md:grid-cols-2 md:gap-y-4 md:gap-x-5">
<a class="!no-underline border dark:border-gray-700 p-5 rounded-lg shadow hover:shadow-lg" href="./tutorials/tutorial_overview"
><div class="w-full text-center bg-gradient-to-br from-blue-400 to-blue-500 rounded-lg py-1.5 font-semibold mb-5 text-white text-lg leading-relaxed">Tutorials</div>
<p class="text-gray-700">Learn the fundamental skills you need to start generating outputs, build your own diffusion system, and train a diffusion model. We recommend starting here if you're using 🤗 Diffusers for the first time!</p>
</a>
<a class="!no-underline border dark:border-gray-700 p-5 rounded-lg shadow hover:shadow-lg" href="./using-diffusers/loading_overview"
><div class="w-full text-center bg-gradient-to-br from-indigo-400 to-indigo-500 rounded-lg py-1.5 font-semibold mb-5 text-white text-lg leading-relaxed">How-to guides</div>
<p class="text-gray-700">Practical guides for helping you load pipelines, models, and schedulers. You'll also learn how to use pipelines for specific tasks, control how outputs are generated, optimize for inference speed, and different training techniques.</p>
</a>
<a class="!no-underline border dark:border-gray-700 p-5 rounded-lg shadow hover:shadow-lg" href="./conceptual/philosophy"
><div class="w-full text-center bg-gradient-to-br from-pink-400 to-pink-500 rounded-lg py-1.5 font-semibold mb-5 text-white text-lg leading-relaxed">Conceptual guides</div>
<p class="text-gray-700">Understand why the library was designed the way it was, and learn more about the ethical guidelines and safety implementations for using the library.</p>
</a>
<a class="!no-underline border dark:border-gray-700 p-5 rounded-lg shadow hover:shadow-lg" href="./api/models/overview"
><div class="w-full text-center bg-gradient-to-br from-purple-400 to-purple-500 rounded-lg py-1.5 font-semibold mb-5 text-white text-lg leading-relaxed">Reference</div>
<p class="text-gray-700">Technical descriptions of how 🤗 Diffusers classes and methods work.</p>
</a>
</div>
</div>
## Supported pipelines
| Pipeline | Paper/Repository | Tasks |
|---|---|:---:|
| [alt_diffusion](./api/pipelines/alt_diffusion) | [AltCLIP: Altering the Language Encoder in CLIP for Extended Language Capabilities](https://arxiv.org/abs/2211.06679) | Image-to-Image Text-Guided Generation |
| [audio_diffusion](./api/pipelines/audio_diffusion) | [Audio Diffusion](https://github.com/teticio/audio-diffusion.git) | Unconditional Audio Generation |
| [controlnet](./api/pipelines/controlnet) | [Adding Conditional Control to Text-to-Image Diffusion Models](https://arxiv.org/abs/2302.05543) | Image-to-Image Text-Guided Generation |
| [cycle_diffusion](./api/pipelines/cycle_diffusion) | [Unifying Diffusion Models' Latent Space, with Applications to CycleDiffusion and Guidance](https://arxiv.org/abs/2210.05559) | Image-to-Image Text-Guided Generation |
| [dance_diffusion](./api/pipelines/dance_diffusion) | [Dance Diffusion](https://github.com/williamberman/diffusers.git) | Unconditional Audio Generation |
| [ddpm](./api/pipelines/ddpm) | [Denoising Diffusion Probabilistic Models](https://arxiv.org/abs/2006.11239) | Unconditional Image Generation |
| [ddim](./api/pipelines/ddim) | [Denoising Diffusion Implicit Models](https://arxiv.org/abs/2010.02502) | Unconditional Image Generation |
| [if](./if) | [**IF**](./api/pipelines/if) | Image Generation |
| [if_img2img](./if) | [**IF**](./api/pipelines/if) | Image-to-Image Generation |
| [if_inpainting](./if) | [**IF**](./api/pipelines/if) | Image-to-Image Generation |
| [latent_diffusion](./api/pipelines/latent_diffusion) | [High-Resolution Image Synthesis with Latent Diffusion Models](https://arxiv.org/abs/2112.10752)| Text-to-Image Generation |
| [latent_diffusion](./api/pipelines/latent_diffusion) | [High-Resolution Image Synthesis with Latent Diffusion Models](https://arxiv.org/abs/2112.10752)| Super Resolution Image-to-Image |
| [latent_diffusion_uncond](./api/pipelines/latent_diffusion_uncond) | [High-Resolution Image Synthesis with Latent Diffusion Models](https://arxiv.org/abs/2112.10752) | Unconditional Image Generation |
| [paint_by_example](./api/pipelines/paint_by_example) | [Paint by Example: Exemplar-based Image Editing with Diffusion Models](https://arxiv.org/abs/2211.13227) | Image-Guided Image Inpainting |
| [pndm](./api/pipelines/pndm) | [Pseudo Numerical Methods for Diffusion Models on Manifolds](https://arxiv.org/abs/2202.09778) | Unconditional Image Generation |
| [score_sde_ve](./api/pipelines/score_sde_ve) | [Score-Based Generative Modeling through Stochastic Differential Equations](https://openreview.net/forum?id=PxTIG12RRHS) | Unconditional Image Generation |
| [score_sde_vp](./api/pipelines/score_sde_vp) | [Score-Based Generative Modeling through Stochastic Differential Equations](https://openreview.net/forum?id=PxTIG12RRHS) | Unconditional Image Generation |
| [semantic_stable_diffusion](./api/pipelines/semantic_stable_diffusion) | [Semantic Guidance](https://arxiv.org/abs/2301.12247) | Text-Guided Generation |
| [stable_diffusion_adapter](./api/pipelines/stable_diffusion/adapter) | [**T2I-Adapter**](https://arxiv.org/abs/2302.08453) | Image-to-Image Text-Guided Generation | -
| [stable_diffusion_text2img](./api/pipelines/stable_diffusion/text2img) | [Stable Diffusion](https://stability.ai/blog/stable-diffusion-public-release) | Text-to-Image Generation |
| [stable_diffusion_img2img](./api/pipelines/stable_diffusion/img2img) | [Stable Diffusion](https://stability.ai/blog/stable-diffusion-public-release) | Image-to-Image Text-Guided Generation |
| [stable_diffusion_inpaint](./api/pipelines/stable_diffusion/inpaint) | [Stable Diffusion](https://stability.ai/blog/stable-diffusion-public-release) | Text-Guided Image Inpainting |
| [stable_diffusion_panorama](./api/pipelines/stable_diffusion/panorama) | [MultiDiffusion](https://multidiffusion.github.io/) | Text-to-Panorama Generation |
| [stable_diffusion_pix2pix](./api/pipelines/stable_diffusion/pix2pix) | [InstructPix2Pix: Learning to Follow Image Editing Instructions](https://arxiv.org/abs/2211.09800) | Text-Guided Image Editing|
| [stable_diffusion_pix2pix_zero](./api/pipelines/stable_diffusion/pix2pix_zero) | [Zero-shot Image-to-Image Translation](https://pix2pixzero.github.io/) | Text-Guided Image Editing |
| [stable_diffusion_attend_and_excite](./api/pipelines/stable_diffusion/attend_and_excite) | [Attend-and-Excite: Attention-Based Semantic Guidance for Text-to-Image Diffusion Models](https://arxiv.org/abs/2301.13826) | Text-to-Image Generation |
| [stable_diffusion_self_attention_guidance](./api/pipelines/stable_diffusion/self_attention_guidance) | [Improving Sample Quality of Diffusion Models Using Self-Attention Guidance](https://arxiv.org/abs/2210.00939) | Text-to-Image Generation Unconditional Image Generation |
| [stable_diffusion_image_variation](./stable_diffusion/image_variation) | [Stable Diffusion Image Variations](https://github.com/LambdaLabsML/lambda-diffusers#stable-diffusion-image-variations) | Image-to-Image Generation |
| [stable_diffusion_latent_upscale](./stable_diffusion/latent_upscale) | [Stable Diffusion Latent Upscaler](https://twitter.com/StabilityAI/status/1590531958815064065) | Text-Guided Super Resolution Image-to-Image |
| [stable_diffusion_model_editing](./api/pipelines/stable_diffusion/model_editing) | [Editing Implicit Assumptions in Text-to-Image Diffusion Models](https://time-diffusion.github.io/) | Text-to-Image Model Editing |
| [stable_diffusion_2](./api/pipelines/stable_diffusion_2) | [Stable Diffusion 2](https://stability.ai/blog/stable-diffusion-v2-release) | Text-to-Image Generation |
| [stable_diffusion_2](./api/pipelines/stable_diffusion_2) | [Stable Diffusion 2](https://stability.ai/blog/stable-diffusion-v2-release) | Text-Guided Image Inpainting |
| [stable_diffusion_2](./api/pipelines/stable_diffusion_2) | [Depth-Conditional Stable Diffusion](https://github.com/Stability-AI/stablediffusion#depth-conditional-stable-diffusion) | Depth-to-Image Generation |
| [stable_diffusion_2](./api/pipelines/stable_diffusion_2) | [Stable Diffusion 2](https://stability.ai/blog/stable-diffusion-v2-release) | Text-Guided Super Resolution Image-to-Image |
| [stable_diffusion_safe](./api/pipelines/stable_diffusion_safe) | [Safe Stable Diffusion](https://arxiv.org/abs/2211.05105) | Text-Guided Generation |
| [stable_unclip](./stable_unclip) | Stable unCLIP | Text-to-Image Generation |
| [stable_unclip](./stable_unclip) | Stable unCLIP | Image-to-Image Text-Guided Generation |
| [stochastic_karras_ve](./api/pipelines/stochastic_karras_ve) | [Elucidating the Design Space of Diffusion-Based Generative Models](https://arxiv.org/abs/2206.00364) | Unconditional Image Generation |
| [text_to_video_sd](./api/pipelines/text_to_video) | [Modelscope's Text-to-video-synthesis Model in Open Domain](https://modelscope.cn/models/damo/text-to-video-synthesis/summary) | Text-to-Video Generation |
| [unclip](./api/pipelines/unclip) | [Hierarchical Text-Conditional Image Generation with CLIP Latents](https://arxiv.org/abs/2204.06125)(implementation by [kakaobrain](https://github.com/kakaobrain/karlo)) | Text-to-Image Generation |
| [versatile_diffusion](./api/pipelines/versatile_diffusion) | [Versatile Diffusion: Text, Images and Variations All in One Diffusion Model](https://arxiv.org/abs/2211.08332) | Text-to-Image Generation |
| [versatile_diffusion](./api/pipelines/versatile_diffusion) | [Versatile Diffusion: Text, Images and Variations All in One Diffusion Model](https://arxiv.org/abs/2211.08332) | Image Variations Generation |
| [versatile_diffusion](./api/pipelines/versatile_diffusion) | [Versatile Diffusion: Text, Images and Variations All in One Diffusion Model](https://arxiv.org/abs/2211.08332) | Dual Image and Text Guided Generation |
| [vq_diffusion](./api/pipelines/vq_diffusion) | [Vector Quantized Diffusion Model for Text-to-Image Synthesis](https://arxiv.org/abs/2111.14822) | Text-to-Image Generation |
| [stable_diffusion_ldm3d](./api/pipelines/stable_diffusion/ldm3d_diffusion) | [LDM3D: Latent Diffusion Model for 3D](https://arxiv.org/abs/2305.10853) | Text to Image and Depth Generation |
| 0 |
hf_public_repos/diffusers/docs/source | hf_public_repos/diffusers/docs/source/en/installation.md | <!--Copyright 2023 The HuggingFace Team. All rights reserved.
Licensed under the Apache License, Version 2.0 (the "License"); you may not use this file except in compliance with
the License. You may obtain a copy of the License at
http://www.apache.org/licenses/LICENSE-2.0
Unless required by applicable law or agreed to in writing, software distributed under the License is distributed on
an "AS IS" BASIS, WITHOUT WARRANTIES OR CONDITIONS OF ANY KIND, either express or implied. See the License for the
specific language governing permissions and limitations under the License.
-->
# Installation
Install 🤗 Diffusers for whichever deep learning library you're working with.
🤗 Diffusers is tested on Python 3.7+, PyTorch 1.7.0+ and Flax. Follow the installation instructions below for the deep learning library you are using:
- [PyTorch](https://pytorch.org/get-started/locally/) installation instructions.
- [Flax](https://flax.readthedocs.io/en/latest/) installation instructions.
## Install with pip
You should install 🤗 Diffusers in a [virtual environment](https://docs.python.org/3/library/venv.html).
If you're unfamiliar with Python virtual environments, take a look at this [guide](https://packaging.python.org/guides/installing-using-pip-and-virtual-environments/).
A virtual environment makes it easier to manage different projects and avoid compatibility issues between dependencies.
Start by creating a virtual environment in your project directory:
```bash
python -m venv .env
```
Activate the virtual environment:
```bash
source .env/bin/activate
```
🤗 Diffusers also relies on the 🤗 Transformers library, and you can install both with the following command:
<frameworkcontent>
<pt>
```bash
pip install diffusers["torch"] transformers
```
</pt>
<jax>
```bash
pip install diffusers["flax"] transformers
```
</jax>
</frameworkcontent>
## Install from source
Before installing 🤗 Diffusers from source, make sure you have `torch` and 🤗 Accelerate installed.
For `torch` installation, refer to the `torch` [installation](https://pytorch.org/get-started/locally/#start-locally) guide.
To install 🤗 Accelerate:
```bash
pip install accelerate
```
Install 🤗 Diffusers from source with the following command:
```bash
pip install git+https://github.com/huggingface/diffusers
```
This command installs the bleeding edge `main` version rather than the latest `stable` version.
The `main` version is useful for staying up-to-date with the latest developments.
For instance, if a bug has been fixed since the last official release but a new release hasn't been rolled out yet.
However, this means the `main` version may not always be stable.
We strive to keep the `main` version operational, and most issues are usually resolved within a few hours or a day.
If you run into a problem, please open an [Issue](https://github.com/huggingface/diffusers/issues/new/choose), so we can fix it even sooner!
## Editable install
You will need an editable install if you'd like to:
* Use the `main` version of the source code.
* Contribute to 🤗 Diffusers and need to test changes in the code.
Clone the repository and install 🤗 Diffusers with the following commands:
```bash
git clone https://github.com/huggingface/diffusers.git
cd diffusers
```
<frameworkcontent>
<pt>
```bash
pip install -e ".[torch]"
```
</pt>
<jax>
```bash
pip install -e ".[flax]"
```
</jax>
</frameworkcontent>
These commands will link the folder you cloned the repository to and your Python library paths.
Python will now look inside the folder you cloned to in addition to the normal library paths.
For example, if your Python packages are typically installed in `~/anaconda3/envs/main/lib/python3.7/site-packages/`, Python will also search the `~/diffusers/` folder you cloned to.
<Tip warning={true}>
You must keep the `diffusers` folder if you want to keep using the library.
</Tip>
Now you can easily update your clone to the latest version of 🤗 Diffusers with the following command:
```bash
cd ~/diffusers/
git pull
```
Your Python environment will find the `main` version of 🤗 Diffusers on the next run.
## Notice on telemetry logging
Our library gathers telemetry information during `from_pretrained()` requests.
This data includes the version of Diffusers and PyTorch/Flax, the requested model or pipeline class,
and the path to a pre-trained checkpoint if it is hosted on the Hub.
This usage data helps us debug issues and prioritize new features.
Telemetry is only sent when loading models and pipelines from the HuggingFace Hub,
and is not collected during local usage.
We understand that not everyone wants to share additional information, and we respect your privacy,
so you can disable telemetry collection by setting the `DISABLE_TELEMETRY` environment variable from your terminal:
On Linux/MacOS:
```bash
export DISABLE_TELEMETRY=YES
```
On Windows:
```bash
set DISABLE_TELEMETRY=YES
```
| 0 |
hf_public_repos/diffusers/docs/source | hf_public_repos/diffusers/docs/source/en/_toctree.yml | - sections:
- local: index
title: 🧨 Diffusers
- local: quicktour
title: Quicktour
- local: stable_diffusion
title: Effective and efficient diffusion
- local: installation
title: Installation
title: Get started
- sections:
- local: tutorials/tutorial_overview
title: Overview
- local: using-diffusers/write_own_pipeline
title: Understanding models and schedulers
- local: tutorials/basic_training
title: Train a diffusion model
title: Tutorials
- sections:
- sections:
- local: using-diffusers/loading_overview
title: Overview
- local: using-diffusers/loading
title: Load pipelines, models, and schedulers
- local: using-diffusers/schedulers
title: Load and compare different schedulers
- local: using-diffusers/custom_pipeline_overview
title: Load community pipelines
- local: using-diffusers/using_safetensors
title: Load safetensors
- local: using-diffusers/other-formats
title: Load different Stable Diffusion formats
title: Loading & Hub
- sections:
- local: using-diffusers/pipeline_overview
title: Overview
- local: using-diffusers/unconditional_image_generation
title: Unconditional image generation
- local: using-diffusers/conditional_image_generation
title: Text-to-image generation
- local: using-diffusers/img2img
title: Text-guided image-to-image
- local: using-diffusers/inpaint
title: Text-guided image-inpainting
- local: using-diffusers/depth2img
title: Text-guided depth-to-image
- local: using-diffusers/textual_inversion_inference
title: Textual inversion
- local: training/distributed_inference
title: Distributed inference with multiple GPUs
- local: using-diffusers/reusing_seeds
title: Improve image quality with deterministic generation
- local: using-diffusers/control_brightness
title: Control image brightness
- local: using-diffusers/reproducibility
title: Create reproducible pipelines
- local: using-diffusers/custom_pipeline_examples
title: Community pipelines
- local: using-diffusers/contribute_pipeline
title: How to contribute a community pipeline
- local: using-diffusers/stable_diffusion_jax_how_to
title: Stable Diffusion in JAX/Flax
- local: using-diffusers/weighted_prompts
title: Weighting Prompts
title: Pipelines for Inference
- sections:
- local: training/overview
title: Overview
- local: training/create_dataset
title: Create a dataset for training
- local: training/adapt_a_model
title: Adapt a model to a new task
- local: training/unconditional_training
title: Unconditional image generation
- local: training/text_inversion
title: Textual Inversion
- local: training/dreambooth
title: DreamBooth
- local: training/text2image
title: Text-to-image
- local: training/lora
title: Low-Rank Adaptation of Large Language Models (LoRA)
- local: training/controlnet
title: ControlNet
- local: training/instructpix2pix
title: InstructPix2Pix Training
- local: training/custom_diffusion
title: Custom Diffusion
title: Training
- sections:
- local: using-diffusers/other-modalities
title: Other Modalities
title: Taking Diffusers Beyond Images
title: Using Diffusers
- sections:
- local: optimization/opt_overview
title: Overview
- local: optimization/fp16
title: Memory and Speed
- local: optimization/torch2.0
title: Torch2.0 support
- local: optimization/xformers
title: xFormers
- local: optimization/onnx
title: ONNX
- local: optimization/open_vino
title: OpenVINO
- local: optimization/coreml
title: Core ML
- local: optimization/mps
title: MPS
- local: optimization/habana
title: Habana Gaudi
- local: optimization/tome
title: Token Merging
title: Optimization/Special Hardware
- sections:
- local: conceptual/philosophy
title: Philosophy
- local: using-diffusers/controlling_generation
title: Controlled generation
- local: conceptual/contribution
title: How to contribute?
- local: conceptual/ethical_guidelines
title: Diffusers' Ethical Guidelines
- local: conceptual/evaluation
title: Evaluating Diffusion Models
title: Conceptual Guides
- sections:
- sections:
- local: api/attnprocessor
title: Attention Processor
- local: api/diffusion_pipeline
title: Diffusion Pipeline
- local: api/logging
title: Logging
- local: api/configuration
title: Configuration
- local: api/outputs
title: Outputs
- local: api/loaders
title: Loaders
- local: api/utilities
title: Utilities
- local: api/image_processor
title: VAE Image Processor
title: Main Classes
- sections:
- local: api/models/overview
title: Overview
- local: api/models/unet
title: UNet1DModel
- local: api/models/unet2d
title: UNet2DModel
- local: api/models/unet2d-cond
title: UNet2DConditionModel
- local: api/models/unet3d-cond
title: UNet3DConditionModel
- local: api/models/vq
title: VQModel
- local: api/models/autoencoderkl
title: AutoencoderKL
- local: api/models/asymmetricautoencoderkl
title: AsymmetricAutoencoderKL
- local: api/models/transformer2d
title: Transformer2D
- local: api/models/transformer_temporal
title: Transformer Temporal
- local: api/models/prior_transformer
title: Prior Transformer
- local: api/models/controlnet
title: ControlNet
title: Models
- sections:
- local: api/pipelines/overview
title: Overview
- local: api/pipelines/alt_diffusion
title: AltDiffusion
- local: api/pipelines/attend_and_excite
title: Attend-and-Excite
- local: api/pipelines/audio_diffusion
title: Audio Diffusion
- local: api/pipelines/audioldm
title: AudioLDM
- local: api/pipelines/auto_pipeline
title: AutoPipeline
- local: api/pipelines/consistency_models
title: Consistency Models
- local: api/pipelines/controlnet
title: ControlNet
- local: api/pipelines/cycle_diffusion
title: Cycle Diffusion
- local: api/pipelines/dance_diffusion
title: Dance Diffusion
- local: api/pipelines/ddim
title: DDIM
- local: api/pipelines/ddpm
title: DDPM
- local: api/pipelines/deepfloyd_if
title: DeepFloyd IF
- local: api/pipelines/diffedit
title: DiffEdit
- local: api/pipelines/dit
title: DiT
- local: api/pipelines/pix2pix
title: InstructPix2Pix
- local: api/pipelines/kandinsky
title: Kandinsky
- local: api/pipelines/kandinsky_v22
title: Kandinsky 2.2
- local: api/pipelines/latent_diffusion
title: Latent Diffusion
- local: api/pipelines/panorama
title: MultiDiffusion
- local: api/pipelines/paint_by_example
title: PaintByExample
- local: api/pipelines/paradigms
title: Parallel Sampling of Diffusion Models
- local: api/pipelines/pix2pix_zero
title: Pix2Pix Zero
- local: api/pipelines/pndm
title: PNDM
- local: api/pipelines/repaint
title: RePaint
- local: api/pipelines/score_sde_ve
title: Score SDE VE
- local: api/pipelines/self_attention_guidance
title: Self-Attention Guidance
- local: api/pipelines/semantic_stable_diffusion
title: Semantic Guidance
- local: api/pipelines/shap_e
title: Shap-E
- local: api/pipelines/spectrogram_diffusion
title: Spectrogram Diffusion
- sections:
- local: api/pipelines/stable_diffusion/overview
title: Overview
- local: api/pipelines/stable_diffusion/text2img
title: Text-to-image
- local: api/pipelines/stable_diffusion/img2img
title: Image-to-image
- local: api/pipelines/stable_diffusion/inpaint
title: Inpainting
- local: api/pipelines/stable_diffusion/depth2img
title: Depth-to-image
- local: api/pipelines/stable_diffusion/image_variation
title: Image variation
- local: api/pipelines/stable_diffusion/stable_diffusion_safe
title: Safe Stable Diffusion
- local: api/pipelines/stable_diffusion/stable_diffusion_2
title: Stable Diffusion 2
- local: api/pipelines/stable_diffusion/stable_diffusion_xl
title: Stable Diffusion XL
- local: api/pipelines/stable_diffusion/latent_upscale
title: Latent upscaler
- local: api/pipelines/stable_diffusion/upscale
title: Super-resolution
- local: api/pipelines/stable_diffusion/ldm3d_diffusion
title: LDM3D Text-to-(RGB, Depth)
- local: api/pipelines/stable_diffusion/adapter
title: Stable Diffusion T2I-adapter
title: Stable Diffusion
- local: api/pipelines/stable_unclip
title: Stable unCLIP
- local: api/pipelines/stochastic_karras_ve
title: Stochastic Karras VE
- local: api/pipelines/model_editing
title: Text-to-image model editing
- local: api/pipelines/text_to_video
title: Text-to-video
- local: api/pipelines/text_to_video_zero
title: Text2Video-Zero
- local: api/pipelines/unclip
title: UnCLIP
- local: api/pipelines/latent_diffusion_uncond
title: Unconditional Latent Diffusion
- local: api/pipelines/unidiffuser
title: UniDiffuser
- local: api/pipelines/value_guided_sampling
title: Value-guided sampling
- local: api/pipelines/versatile_diffusion
title: Versatile Diffusion
- local: api/pipelines/vq_diffusion
title: VQ Diffusion
title: Pipelines
- sections:
- local: api/schedulers/overview
title: Overview
- local: api/schedulers/cm_stochastic_iterative
title: Consistency Model Multistep Scheduler
- local: api/schedulers/ddim
title: DDIM
- local: api/schedulers/ddim_inverse
title: DDIMInverse
- local: api/schedulers/ddpm
title: DDPM
- local: api/schedulers/deis
title: DEIS
- local: api/schedulers/dpm_discrete
title: DPM Discrete Scheduler
- local: api/schedulers/dpm_discrete_ancestral
title: DPM Discrete Scheduler with ancestral sampling
- local: api/schedulers/dpm_sde
title: DPMSolverSDEScheduler
- local: api/schedulers/euler_ancestral
title: Euler Ancestral Scheduler
- local: api/schedulers/euler
title: Euler scheduler
- local: api/schedulers/heun
title: Heun Scheduler
- local: api/schedulers/multistep_dpm_solver_inverse
title: Inverse Multistep DPM-Solver
- local: api/schedulers/ipndm
title: IPNDM
- local: api/schedulers/lms_discrete
title: Linear Multistep
- local: api/schedulers/multistep_dpm_solver
title: Multistep DPM-Solver
- local: api/schedulers/pndm
title: PNDM
- local: api/schedulers/repaint
title: RePaint Scheduler
- local: api/schedulers/singlestep_dpm_solver
title: Singlestep DPM-Solver
- local: api/schedulers/stochastic_karras_ve
title: Stochastic Kerras VE
- local: api/schedulers/unipc
title: UniPCMultistepScheduler
- local: api/schedulers/score_sde_ve
title: VE-SDE
- local: api/schedulers/score_sde_vp
title: VP-SDE
- local: api/schedulers/vq_diffusion
title: VQDiffusionScheduler
title: Schedulers
title: API
| 0 |
hf_public_repos/diffusers/docs/source | hf_public_repos/diffusers/docs/source/en/stable_diffusion.md | <!--Copyright 2023 The HuggingFace Team. All rights reserved.
Licensed under the Apache License, Version 2.0 (the "License"); you may not use this file except in compliance with
the License. You may obtain a copy of the License at
http://www.apache.org/licenses/LICENSE-2.0
Unless required by applicable law or agreed to in writing, software distributed under the License is distributed on
an "AS IS" BASIS, WITHOUT WARRANTIES OR CONDITIONS OF ANY KIND, either express or implied. See the License for the
specific language governing permissions and limitations under the License.
-->
# Effective and efficient diffusion
[[open-in-colab]]
Getting the [`DiffusionPipeline`] to generate images in a certain style or include what you want can be tricky. Often times, you have to run the [`DiffusionPipeline`] several times before you end up with an image you're happy with. But generating something out of nothing is a computationally intensive process, especially if you're running inference over and over again.
This is why it's important to get the most *computational* (speed) and *memory* (GPU RAM) efficiency from the pipeline to reduce the time between inference cycles so you can iterate faster.
This tutorial walks you through how to generate faster and better with the [`DiffusionPipeline`].
Begin by loading the [`runwayml/stable-diffusion-v1-5`](https://huggingface.co/runwayml/stable-diffusion-v1-5) model:
```python
from diffusers import DiffusionPipeline
model_id = "runwayml/stable-diffusion-v1-5"
pipeline = DiffusionPipeline.from_pretrained(model_id)
```
The example prompt you'll use is a portrait of an old warrior chief, but feel free to use your own prompt:
```python
prompt = "portrait photo of a old warrior chief"
```
## Speed
<Tip>
💡 If you don't have access to a GPU, you can use one for free from a GPU provider like [Colab](https://colab.research.google.com/)!
</Tip>
One of the simplest ways to speed up inference is to place the pipeline on a GPU the same way you would with any PyTorch module:
```python
pipeline = pipeline.to("cuda")
```
To make sure you can use the same image and improve on it, use a [`Generator`](https://pytorch.org/docs/stable/generated/torch.Generator.html) and set a seed for [reproducibility](./using-diffusers/reproducibility):
```python
import torch
generator = torch.Generator("cuda").manual_seed(0)
```
Now you can generate an image:
```python
image = pipeline(prompt, generator=generator).images[0]
image
```
<div class="flex justify-center">
<img src="https://huggingface.co/datasets/diffusers/docs-images/resolve/main/stable_diffusion_101/sd_101_1.png">
</div>
This process took ~30 seconds on a T4 GPU (it might be faster if your allocated GPU is better than a T4). By default, the [`DiffusionPipeline`] runs inference with full `float32` precision for 50 inference steps. You can speed this up by switching to a lower precision like `float16` or running fewer inference steps.
Let's start by loading the model in `float16` and generate an image:
```python
import torch
pipeline = DiffusionPipeline.from_pretrained(model_id, torch_dtype=torch.float16)
pipeline = pipeline.to("cuda")
generator = torch.Generator("cuda").manual_seed(0)
image = pipeline(prompt, generator=generator).images[0]
image
```
<div class="flex justify-center">
<img src="https://huggingface.co/datasets/diffusers/docs-images/resolve/main/stable_diffusion_101/sd_101_2.png">
</div>
This time, it only took ~11 seconds to generate the image, which is almost 3x faster than before!
<Tip>
💡 We strongly suggest always running your pipelines in `float16`, and so far, we've rarely seen any degradation in output quality.
</Tip>
Another option is to reduce the number of inference steps. Choosing a more efficient scheduler could help decrease the number of steps without sacrificing output quality. You can find which schedulers are compatible with the current model in the [`DiffusionPipeline`] by calling the `compatibles` method:
```python
pipeline.scheduler.compatibles
[
diffusers.schedulers.scheduling_lms_discrete.LMSDiscreteScheduler,
diffusers.schedulers.scheduling_unipc_multistep.UniPCMultistepScheduler,
diffusers.schedulers.scheduling_k_dpm_2_discrete.KDPM2DiscreteScheduler,
diffusers.schedulers.scheduling_deis_multistep.DEISMultistepScheduler,
diffusers.schedulers.scheduling_euler_discrete.EulerDiscreteScheduler,
diffusers.schedulers.scheduling_dpmsolver_multistep.DPMSolverMultistepScheduler,
diffusers.schedulers.scheduling_ddpm.DDPMScheduler,
diffusers.schedulers.scheduling_dpmsolver_singlestep.DPMSolverSinglestepScheduler,
diffusers.schedulers.scheduling_k_dpm_2_ancestral_discrete.KDPM2AncestralDiscreteScheduler,
diffusers.schedulers.scheduling_heun_discrete.HeunDiscreteScheduler,
diffusers.schedulers.scheduling_pndm.PNDMScheduler,
diffusers.schedulers.scheduling_euler_ancestral_discrete.EulerAncestralDiscreteScheduler,
diffusers.schedulers.scheduling_ddim.DDIMScheduler,
]
```
The Stable Diffusion model uses the [`PNDMScheduler`] by default which usually requires ~50 inference steps, but more performant schedulers like [`DPMSolverMultistepScheduler`], require only ~20 or 25 inference steps. Use the [`ConfigMixin.from_config`] method to load a new scheduler:
```python
from diffusers import DPMSolverMultistepScheduler
pipeline.scheduler = DPMSolverMultistepScheduler.from_config(pipeline.scheduler.config)
```
Now set the `num_inference_steps` to 20:
```python
generator = torch.Generator("cuda").manual_seed(0)
image = pipeline(prompt, generator=generator, num_inference_steps=20).images[0]
image
```
<div class="flex justify-center">
<img src="https://huggingface.co/datasets/diffusers/docs-images/resolve/main/stable_diffusion_101/sd_101_3.png">
</div>
Great, you've managed to cut the inference time to just 4 seconds! ⚡️
## Memory
The other key to improving pipeline performance is consuming less memory, which indirectly implies more speed, since you're often trying to maximize the number of images generated per second. The easiest way to see how many images you can generate at once is to try out different batch sizes until you get an `OutOfMemoryError` (OOM).
Create a function that'll generate a batch of images from a list of prompts and `Generators`. Make sure to assign each `Generator` a seed so you can reuse it if it produces a good result.
```python
def get_inputs(batch_size=1):
generator = [torch.Generator("cuda").manual_seed(i) for i in range(batch_size)]
prompts = batch_size * [prompt]
num_inference_steps = 20
return {"prompt": prompts, "generator": generator, "num_inference_steps": num_inference_steps}
```
You'll also need a function that'll display each batch of images:
```python
from PIL import Image
def image_grid(imgs, rows=2, cols=2):
w, h = imgs[0].size
grid = Image.new("RGB", size=(cols * w, rows * h))
for i, img in enumerate(imgs):
grid.paste(img, box=(i % cols * w, i // cols * h))
return grid
```
Start with `batch_size=4` and see how much memory you've consumed:
```python
images = pipeline(**get_inputs(batch_size=4)).images
image_grid(images)
```
Unless you have a GPU with more RAM, the code above probably returned an `OOM` error! Most of the memory is taken up by the cross-attention layers. Instead of running this operation in a batch, you can run it sequentially to save a significant amount of memory. All you have to do is configure the pipeline to use the [`~DiffusionPipeline.enable_attention_slicing`] function:
```python
pipeline.enable_attention_slicing()
```
Now try increasing the `batch_size` to 8!
```python
images = pipeline(**get_inputs(batch_size=8)).images
image_grid(images, rows=2, cols=4)
```
<div class="flex justify-center">
<img src="https://huggingface.co/datasets/diffusers/docs-images/resolve/main/stable_diffusion_101/sd_101_5.png">
</div>
Whereas before you couldn't even generate a batch of 4 images, now you can generate a batch of 8 images at ~3.5 seconds per image! This is probably the fastest you can go on a T4 GPU without sacrificing quality.
## Quality
In the last two sections, you learned how to optimize the speed of your pipeline by using `fp16`, reducing the number of inference steps by using a more performant scheduler, and enabling attention slicing to reduce memory consumption. Now you're going to focus on how to improve the quality of generated images.
### Better checkpoints
The most obvious step is to use better checkpoints. The Stable Diffusion model is a good starting point, and since its official launch, several improved versions have also been released. However, using a newer version doesn't automatically mean you'll get better results. You'll still have to experiment with different checkpoints yourself, and do a little research (such as using [negative prompts](https://minimaxir.com/2022/11/stable-diffusion-negative-prompt/)) to get the best results.
As the field grows, there are more and more high-quality checkpoints finetuned to produce certain styles. Try exploring the [Hub](https://huggingface.co/models?library=diffusers&sort=downloads) and [Diffusers Gallery](https://huggingface.co/spaces/huggingface-projects/diffusers-gallery) to find one you're interested in!
### Better pipeline components
You can also try replacing the current pipeline components with a newer version. Let's try loading the latest [autodecoder](https://huggingface.co/stabilityai/stable-diffusion-2-1/tree/main/vae) from Stability AI into the pipeline, and generate some images:
```python
from diffusers import AutoencoderKL
vae = AutoencoderKL.from_pretrained("stabilityai/sd-vae-ft-mse", torch_dtype=torch.float16).to("cuda")
pipeline.vae = vae
images = pipeline(**get_inputs(batch_size=8)).images
image_grid(images, rows=2, cols=4)
```
<div class="flex justify-center">
<img src="https://huggingface.co/datasets/diffusers/docs-images/resolve/main/stable_diffusion_101/sd_101_6.png">
</div>
### Better prompt engineering
The text prompt you use to generate an image is super important, so much so that it is called *prompt engineering*. Some considerations to keep during prompt engineering are:
- How is the image or similar images of the one I want to generate stored on the internet?
- What additional detail can I give that steers the model towards the style I want?
With this in mind, let's improve the prompt to include color and higher quality details:
```python
prompt += ", tribal panther make up, blue on red, side profile, looking away, serious eyes"
prompt += " 50mm portrait photography, hard rim lighting photography--beta --ar 2:3 --beta --upbeta"
```
Generate a batch of images with the new prompt:
```python
images = pipeline(**get_inputs(batch_size=8)).images
image_grid(images, rows=2, cols=4)
```
<div class="flex justify-center">
<img src="https://huggingface.co/datasets/diffusers/docs-images/resolve/main/stable_diffusion_101/sd_101_7.png">
</div>
Pretty impressive! Let's tweak the second image - corresponding to the `Generator` with a seed of `1` - a bit more by adding some text about the age of the subject:
```python
prompts = [
"portrait photo of the oldest warrior chief, tribal panther make up, blue on red, side profile, looking away, serious eyes 50mm portrait photography, hard rim lighting photography--beta --ar 2:3 --beta --upbeta",
"portrait photo of a old warrior chief, tribal panther make up, blue on red, side profile, looking away, serious eyes 50mm portrait photography, hard rim lighting photography--beta --ar 2:3 --beta --upbeta",
"portrait photo of a warrior chief, tribal panther make up, blue on red, side profile, looking away, serious eyes 50mm portrait photography, hard rim lighting photography--beta --ar 2:3 --beta --upbeta",
"portrait photo of a young warrior chief, tribal panther make up, blue on red, side profile, looking away, serious eyes 50mm portrait photography, hard rim lighting photography--beta --ar 2:3 --beta --upbeta",
]
generator = [torch.Generator("cuda").manual_seed(1) for _ in range(len(prompts))]
images = pipeline(prompt=prompts, generator=generator, num_inference_steps=25).images
image_grid(images)
```
<div class="flex justify-center">
<img src="https://huggingface.co/datasets/diffusers/docs-images/resolve/main/stable_diffusion_101/sd_101_8.png">
</div>
## Next steps
In this tutorial, you learned how to optimize a [`DiffusionPipeline`] for computational and memory efficiency as well as improving the quality of generated outputs. If you're interested in making your pipeline even faster, take a look at the following resources:
- Learn how [PyTorch 2.0](./optimization/torch2.0) and [`torch.compile`](https://pytorch.org/docs/stable/generated/torch.compile.html) can yield 5 - 300% faster inference speed. On an A100 GPU, inference can be up to 50% faster!
- If you can't use PyTorch 2, we recommend you install [xFormers](./optimization/xformers). Its memory-efficient attention mechanism works great with PyTorch 1.13.1 for faster speed and reduced memory consumption.
- Other optimization techniques, such as model offloading, are covered in [this guide](./optimization/fp16).
| 0 |
hf_public_repos/diffusers/docs/source/en | hf_public_repos/diffusers/docs/source/en/training/unconditional_training.md | <!--Copyright 2023 The HuggingFace Team. All rights reserved.
Licensed under the Apache License, Version 2.0 (the "License"); you may not use this file except in compliance with
the License. You may obtain a copy of the License at
http://www.apache.org/licenses/LICENSE-2.0
Unless required by applicable law or agreed to in writing, software distributed under the License is distributed on
an "AS IS" BASIS, WITHOUT WARRANTIES OR CONDITIONS OF ANY KIND, either express or implied. See the License for the
specific language governing permissions and limitations under the License.
-->
# Unconditional image generation
Unconditional image generation is not conditioned on any text or images, unlike text- or image-to-image models. It only generates images that resemble its training data distribution.
<iframe
src="https://stevhliu-ddpm-butterflies-128.hf.space"
frameborder="0"
width="850"
height="550"
></iframe>
This guide will show you how to train an unconditional image generation model on existing datasets as well as your own custom dataset. All the training scripts for unconditional image generation can be found [here](https://github.com/huggingface/diffusers/tree/main/examples/unconditional_image_generation) if you're interested in learning more about the training details.
Before running the script, make sure you install the library's training dependencies:
```bash
pip install diffusers[training] accelerate datasets
```
Next, initialize an 🤗 [Accelerate](https://github.com/huggingface/accelerate/) environment with:
```bash
accelerate config
```
To setup a default 🤗 Accelerate environment without choosing any configurations:
```bash
accelerate config default
```
Or if your environment doesn't support an interactive shell like a notebook, you can use:
```bash
from accelerate.utils import write_basic_config
write_basic_config()
```
## Upload model to Hub
You can upload your model on the Hub by adding the following argument to the training script:
```bash
--push_to_hub
```
## Save and load checkpoints
It is a good idea to regularly save checkpoints in case anything happens during training. To save a checkpoint, pass the following argument to the training script:
```bash
--checkpointing_steps=500
```
The full training state is saved in a subfolder in the `output_dir` every 500 steps, which allows you to load a checkpoint and resume training if you pass the `--resume_from_checkpoint` argument to the training script:
```bash
--resume_from_checkpoint="checkpoint-1500"
```
## Finetuning
You're ready to launch the [training script](https://github.com/huggingface/diffusers/blob/main/examples/unconditional_image_generation/train_unconditional.py) now! Specify the dataset name to finetune on with the `--dataset_name` argument and then save it to the path in `--output_dir`. To use your own dataset, take a look at the [Create a dataset for training](create_dataset) guide.
The training script creates and saves a `diffusion_pytorch_model.bin` file in your repository.
<Tip>
💡 A full training run takes 2 hours on 4xV100 GPUs.
</Tip>
For example, to finetune on the [Oxford Flowers](https://huggingface.co/datasets/huggan/flowers-102-categories) dataset:
```bash
accelerate launch train_unconditional.py \
--dataset_name="huggan/flowers-102-categories" \
--resolution=64 \
--output_dir="ddpm-ema-flowers-64" \
--train_batch_size=16 \
--num_epochs=100 \
--gradient_accumulation_steps=1 \
--learning_rate=1e-4 \
--lr_warmup_steps=500 \
--mixed_precision=no \
--push_to_hub
```
<div class="flex justify-center">
<img src="https://user-images.githubusercontent.com/26864830/180248660-a0b143d0-b89a-42c5-8656-2ebf6ece7e52.png"/>
</div>
Or if you want to train your model on the [Pokemon](https://huggingface.co/datasets/huggan/pokemon) dataset:
```bash
accelerate launch train_unconditional.py \
--dataset_name="huggan/pokemon" \
--resolution=64 \
--output_dir="ddpm-ema-pokemon-64" \
--train_batch_size=16 \
--num_epochs=100 \
--gradient_accumulation_steps=1 \
--learning_rate=1e-4 \
--lr_warmup_steps=500 \
--mixed_precision=no \
--push_to_hub
```
<div class="flex justify-center">
<img src="https://user-images.githubusercontent.com/26864830/180248200-928953b4-db38-48db-b0c6-8b740fe6786f.png"/>
</div>
### Training with multiple GPUs
`accelerate` allows for seamless multi-GPU training. Follow the instructions [here](https://huggingface.co/docs/accelerate/basic_tutorials/launch)
for running distributed training with `accelerate`. Here is an example command:
```bash
accelerate launch --mixed_precision="fp16" --multi_gpu train_unconditional.py \
--dataset_name="huggan/pokemon" \
--resolution=64 --center_crop --random_flip \
--output_dir="ddpm-ema-pokemon-64" \
--train_batch_size=16 \
--num_epochs=100 \
--gradient_accumulation_steps=1 \
--use_ema \
--learning_rate=1e-4 \
--lr_warmup_steps=500 \
--mixed_precision="fp16" \
--logger="wandb" \
--push_to_hub
``` | 0 |
hf_public_repos/diffusers/docs/source/en | hf_public_repos/diffusers/docs/source/en/training/text_inversion.md | <!--Copyright 2023 The HuggingFace Team. All rights reserved.
Licensed under the Apache License, Version 2.0 (the "License"); you may not use this file except in compliance with
the License. You may obtain a copy of the License at
http://www.apache.org/licenses/LICENSE-2.0
Unless required by applicable law or agreed to in writing, software distributed under the License is distributed on
an "AS IS" BASIS, WITHOUT WARRANTIES OR CONDITIONS OF ANY KIND, either express or implied. See the License for the
specific language governing permissions and limitations under the License.
-->
# Textual Inversion
[Textual Inversion](https://arxiv.org/abs/2208.01618) is a technique for capturing novel concepts from a small number of example images. While the technique was originally demonstrated with a [latent diffusion model](https://github.com/CompVis/latent-diffusion), it has since been applied to other model variants like [Stable Diffusion](https://huggingface.co/docs/diffusers/main/en/conceptual/stable_diffusion). The learned concepts can be used to better control the images generated from text-to-image pipelines. It learns new "words" in the text encoder's embedding space, which are used within text prompts for personalized image generation.
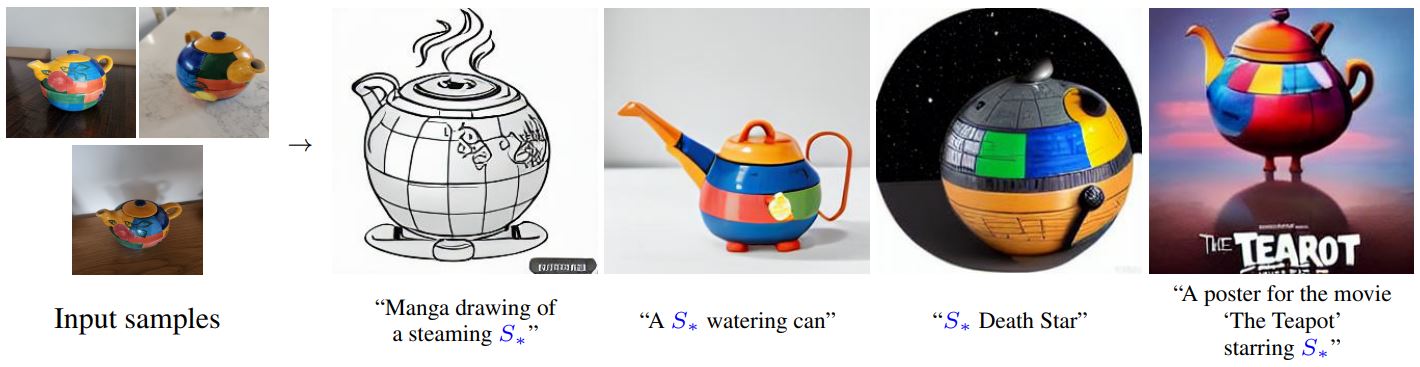
<small>By using just 3-5 images you can teach new concepts to a model such as Stable Diffusion for personalized image generation <a href="https://github.com/rinongal/textual_inversion">(image source)</a>.</small>
This guide will show you how to train a [`runwayml/stable-diffusion-v1-5`](https://huggingface.co/runwayml/stable-diffusion-v1-5) model with Textual Inversion. All the training scripts for Textual Inversion used in this guide can be found [here](https://github.com/huggingface/diffusers/tree/main/examples/textual_inversion) if you're interested in taking a closer look at how things work under the hood.
<Tip>
There is a community-created collection of trained Textual Inversion models in the [Stable Diffusion Textual Inversion Concepts Library](https://huggingface.co/sd-concepts-library) which are readily available for inference. Over time, this'll hopefully grow into a useful resource as more concepts are added!
</Tip>
Before you begin, make sure you install the library's training dependencies:
```bash
pip install diffusers accelerate transformers
```
After all the dependencies have been set up, initialize a [🤗Accelerate](https://github.com/huggingface/accelerate/) environment with:
```bash
accelerate config
```
To setup a default 🤗 Accelerate environment without choosing any configurations:
```bash
accelerate config default
```
Or if your environment doesn't support an interactive shell like a notebook, you can use:
```bash
from accelerate.utils import write_basic_config
write_basic_config()
```
Finally, you try and [install xFormers](https://huggingface.co/docs/diffusers/main/en/training/optimization/xformers) to reduce your memory footprint with xFormers memory-efficient attention. Once you have xFormers installed, add the `--enable_xformers_memory_efficient_attention` argument to the training script. xFormers is not supported for Flax.
## Upload model to Hub
If you want to store your model on the Hub, add the following argument to the training script:
```bash
--push_to_hub
```
## Save and load checkpoints
It is often a good idea to regularly save checkpoints of your model during training. This way, you can resume training from a saved checkpoint if your training is interrupted for any reason. To save a checkpoint, pass the following argument to the training script to save the full training state in a subfolder in `output_dir` every 500 steps:
```bash
--checkpointing_steps=500
```
To resume training from a saved checkpoint, pass the following argument to the training script and the specific checkpoint you'd like to resume from:
```bash
--resume_from_checkpoint="checkpoint-1500"
```
## Finetuning
For your training dataset, download these [images of a cat toy](https://huggingface.co/datasets/diffusers/cat_toy_example) and store them in a directory. To use your own dataset, take a look at the [Create a dataset for training](create_dataset) guide.
```py
from huggingface_hub import snapshot_download
local_dir = "./cat"
snapshot_download(
"diffusers/cat_toy_example", local_dir=local_dir, repo_type="dataset", ignore_patterns=".gitattributes"
)
```
Specify the `MODEL_NAME` environment variable (either a Hub model repository id or a path to the directory containing the model weights) and pass it to the [`pretrained_model_name_or_path`](https://huggingface.co/docs/diffusers/en/api/diffusion_pipeline#diffusers.DiffusionPipeline.from_pretrained.pretrained_model_name_or_path) argument, and the `DATA_DIR` environment variable to the path of the directory containing the images.
Now you can launch the [training script](https://github.com/huggingface/diffusers/blob/main/examples/textual_inversion/textual_inversion.py). The script creates and saves the following files to your repository: `learned_embeds.bin`, `token_identifier.txt`, and `type_of_concept.txt`.
<Tip>
💡 A full training run takes ~1 hour on one V100 GPU. While you're waiting for the training to complete, feel free to check out [how Textual Inversion works](#how-it-works) in the section below if you're curious!
</Tip>
<frameworkcontent>
<pt>
```bash
export MODEL_NAME="runwayml/stable-diffusion-v1-5"
export DATA_DIR="./cat"
accelerate launch textual_inversion.py \
--pretrained_model_name_or_path=$MODEL_NAME \
--train_data_dir=$DATA_DIR \
--learnable_property="object" \
--placeholder_token="<cat-toy>" --initializer_token="toy" \
--resolution=512 \
--train_batch_size=1 \
--gradient_accumulation_steps=4 \
--max_train_steps=3000 \
--learning_rate=5.0e-04 --scale_lr \
--lr_scheduler="constant" \
--lr_warmup_steps=0 \
--output_dir="textual_inversion_cat" \
--push_to_hub
```
<Tip>
💡 If you want to increase the trainable capacity, you can associate your placeholder token, *e.g.* `<cat-toy>` to
multiple embedding vectors. This can help the model to better capture the style of more (complex) images.
To enable training multiple embedding vectors, simply pass:
```bash
--num_vectors=5
```
</Tip>
</pt>
<jax>
If you have access to TPUs, try out the [Flax training script](https://github.com/huggingface/diffusers/blob/main/examples/textual_inversion/textual_inversion_flax.py) to train even faster (this'll also work for GPUs). With the same configuration settings, the Flax training script should be at least 70% faster than the PyTorch training script! ⚡️
Before you begin, make sure you install the Flax specific dependencies:
```bash
pip install -U -r requirements_flax.txt
```
Specify the `MODEL_NAME` environment variable (either a Hub model repository id or a path to the directory containing the model weights) and pass it to the [`pretrained_model_name_or_path`](https://huggingface.co/docs/diffusers/en/api/diffusion_pipeline#diffusers.DiffusionPipeline.from_pretrained.pretrained_model_name_or_path) argument.
Then you can launch the [training script](https://github.com/huggingface/diffusers/blob/main/examples/textual_inversion/textual_inversion_flax.py):
```bash
export MODEL_NAME="duongna/stable-diffusion-v1-4-flax"
export DATA_DIR="./cat"
python textual_inversion_flax.py \
--pretrained_model_name_or_path=$MODEL_NAME \
--train_data_dir=$DATA_DIR \
--learnable_property="object" \
--placeholder_token="<cat-toy>" --initializer_token="toy" \
--resolution=512 \
--train_batch_size=1 \
--max_train_steps=3000 \
--learning_rate=5.0e-04 --scale_lr \
--output_dir="textual_inversion_cat" \
--push_to_hub
```
</jax>
</frameworkcontent>
### Intermediate logging
If you're interested in following along with your model training progress, you can save the generated images from the training process. Add the following arguments to the training script to enable intermediate logging:
- `validation_prompt`, the prompt used to generate samples (this is set to `None` by default and intermediate logging is disabled)
- `num_validation_images`, the number of sample images to generate
- `validation_steps`, the number of steps before generating `num_validation_images` from the `validation_prompt`
```bash
--validation_prompt="A <cat-toy> backpack"
--num_validation_images=4
--validation_steps=100
```
## Inference
Once you have trained a model, you can use it for inference with the [`StableDiffusionPipeline`].
The textual inversion script will by default only save the textual inversion embedding vector(s) that have
been added to the text encoder embedding matrix and consequently been trained.
<frameworkcontent>
<pt>
<Tip>
💡 The community has created a large library of different textual inversion embedding vectors, called [sd-concepts-library](https://huggingface.co/sd-concepts-library).
Instead of training textual inversion embeddings from scratch you can also see whether a fitting textual inversion embedding has already been added to the libary.
</Tip>
To load the textual inversion embeddings you first need to load the base model that was used when training
your textual inversion embedding vectors. Here we assume that [`runwayml/stable-diffusion-v1-5`](runwayml/stable-diffusion-v1-5)
was used as a base model so we load it first:
```python
from diffusers import StableDiffusionPipeline
import torch
model_id = "runwayml/stable-diffusion-v1-5"
pipe = StableDiffusionPipeline.from_pretrained(model_id, torch_dtype=torch.float16).to("cuda")
```
Next, we need to load the textual inversion embedding vector which can be done via the [`TextualInversionLoaderMixin.load_textual_inversion`]
function. Here we'll load the embeddings of the "<cat-toy>" example from before.
```python
pipe.load_textual_inversion("sd-concepts-library/cat-toy")
```
Now we can run the pipeline making sure that the placeholder token `<cat-toy>` is used in our prompt.
```python
prompt = "A <cat-toy> backpack"
image = pipe(prompt, num_inference_steps=50).images[0]
image.save("cat-backpack.png")
```
The function [`TextualInversionLoaderMixin.load_textual_inversion`] can not only
load textual embedding vectors saved in Diffusers' format, but also embedding vectors
saved in [Automatic1111](https://github.com/AUTOMATIC1111/stable-diffusion-webui) format.
To do so, you can first download an embedding vector from [civitAI](https://civitai.com/models/3036?modelVersionId=8387)
and then load it locally:
```python
pipe.load_textual_inversion("./charturnerv2.pt")
```
</pt>
<jax>
Currently there is no `load_textual_inversion` function for Flax so one has to make sure the textual inversion
embedding vector is saved as part of the model after training.
The model can then be run just like any other Flax model:
```python
import jax
import numpy as np
from flax.jax_utils import replicate
from flax.training.common_utils import shard
from diffusers import FlaxStableDiffusionPipeline
model_path = "path-to-your-trained-model"
pipeline, params = FlaxStableDiffusionPipeline.from_pretrained(model_path, dtype=jax.numpy.bfloat16)
prompt = "A <cat-toy> backpack"
prng_seed = jax.random.PRNGKey(0)
num_inference_steps = 50
num_samples = jax.device_count()
prompt = num_samples * [prompt]
prompt_ids = pipeline.prepare_inputs(prompt)
# shard inputs and rng
params = replicate(params)
prng_seed = jax.random.split(prng_seed, jax.device_count())
prompt_ids = shard(prompt_ids)
images = pipeline(prompt_ids, params, prng_seed, num_inference_steps, jit=True).images
images = pipeline.numpy_to_pil(np.asarray(images.reshape((num_samples,) + images.shape[-3:])))
image.save("cat-backpack.png")
```
</jax>
</frameworkcontent>
## How it works
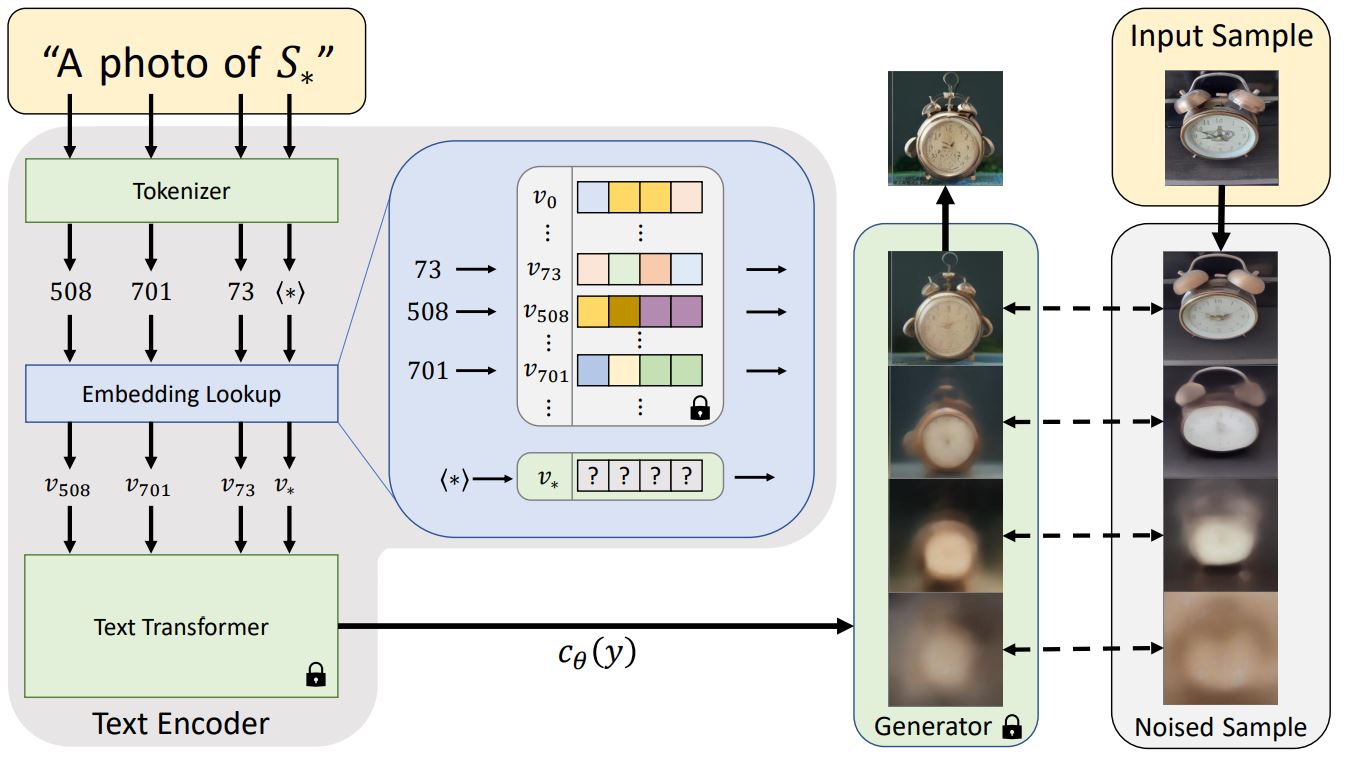
<small>Architecture overview from the Textual Inversion <a href="https://textual-inversion.github.io/">blog post.</a></small>
Usually, text prompts are tokenized into an embedding before being passed to a model, which is often a transformer. Textual Inversion does something similar, but it learns a new token embedding, `v*`, from a special token `S*` in the diagram above. The model output is used to condition the diffusion model, which helps the diffusion model understand the prompt and new concepts from just a few example images.
To do this, Textual Inversion uses a generator model and noisy versions of the training images. The generator tries to predict less noisy versions of the images, and the token embedding `v*` is optimized based on how well the generator does. If the token embedding successfully captures the new concept, it gives more useful information to the diffusion model and helps create clearer images with less noise. This optimization process typically occurs after several thousand steps of exposure to a variety of prompt and image variants.
| 0 |
hf_public_repos/diffusers/docs/source/en | hf_public_repos/diffusers/docs/source/en/training/create_dataset.md | # Create a dataset for training
There are many datasets on the [Hub](https://huggingface.co/datasets?task_categories=task_categories:text-to-image&sort=downloads) to train a model on, but if you can't find one you're interested in or want to use your own, you can create a dataset with the 🤗 [Datasets](hf.co/docs/datasets) library. The dataset structure depends on the task you want to train your model on. The most basic dataset structure is a directory of images for tasks like unconditional image generation. Another dataset structure may be a directory of images and a text file containing their corresponding text captions for tasks like text-to-image generation.
This guide will show you two ways to create a dataset to finetune on:
- provide a folder of images to the `--train_data_dir` argument
- upload a dataset to the Hub and pass the dataset repository id to the `--dataset_name` argument
<Tip>
💡 Learn more about how to create an image dataset for training in the [Create an image dataset](https://huggingface.co/docs/datasets/image_dataset) guide.
</Tip>
## Provide a dataset as a folder
For unconditional generation, you can provide your own dataset as a folder of images. The training script uses the [`ImageFolder`](https://huggingface.co/docs/datasets/en/image_dataset#imagefolder) builder from 🤗 Datasets to automatically build a dataset from the folder. Your directory structure should look like:
```bash
data_dir/xxx.png
data_dir/xxy.png
data_dir/[...]/xxz.png
```
Pass the path to the dataset directory to the `--train_data_dir` argument, and then you can start training:
```bash
accelerate launch train_unconditional.py \
--train_data_dir <path-to-train-directory> \
<other-arguments>
```
## Upload your data to the Hub
<Tip>
💡 For more details and context about creating and uploading a dataset to the Hub, take a look at the [Image search with 🤗 Datasets](https://huggingface.co/blog/image-search-datasets) post.
</Tip>
Start by creating a dataset with the [`ImageFolder`](https://huggingface.co/docs/datasets/image_load#imagefolder) feature, which creates an `image` column containing the PIL-encoded images.
You can use the `data_dir` or `data_files` parameters to specify the location of the dataset. The `data_files` parameter supports mapping specific files to dataset splits like `train` or `test`:
```python
from datasets import load_dataset
# example 1: local folder
dataset = load_dataset("imagefolder", data_dir="path_to_your_folder")
# example 2: local files (supported formats are tar, gzip, zip, xz, rar, zstd)
dataset = load_dataset("imagefolder", data_files="path_to_zip_file")
# example 3: remote files (supported formats are tar, gzip, zip, xz, rar, zstd)
dataset = load_dataset(
"imagefolder",
data_files="https://download.microsoft.com/download/3/E/1/3E1C3F21-ECDB-4869-8368-6DEBA77B919F/kagglecatsanddogs_3367a.zip",
)
# example 4: providing several splits
dataset = load_dataset(
"imagefolder", data_files={"train": ["path/to/file1", "path/to/file2"], "test": ["path/to/file3", "path/to/file4"]}
)
```
Then use the [`~datasets.Dataset.push_to_hub`] method to upload the dataset to the Hub:
```python
# assuming you have ran the huggingface-cli login command in a terminal
dataset.push_to_hub("name_of_your_dataset")
# if you want to push to a private repo, simply pass private=True:
dataset.push_to_hub("name_of_your_dataset", private=True)
```
Now the dataset is available for training by passing the dataset name to the `--dataset_name` argument:
```bash
accelerate launch --mixed_precision="fp16" train_text_to_image.py \
--pretrained_model_name_or_path="runwayml/stable-diffusion-v1-5" \
--dataset_name="name_of_your_dataset" \
<other-arguments>
```
## Next steps
Now that you've created a dataset, you can plug it into the `train_data_dir` (if your dataset is local) or `dataset_name` (if your dataset is on the Hub) arguments of a training script.
For your next steps, feel free to try and use your dataset to train a model for [unconditional generation](uncondtional_training) or [text-to-image generation](text2image)! | 0 |
Subsets and Splits