licenses
sequencelengths 1
3
| version
stringclasses 677
values | tree_hash
stringlengths 40
40
| path
stringclasses 1
value | type
stringclasses 2
values | size
stringlengths 2
8
| text
stringlengths 25
67.1M
| package_name
stringlengths 2
41
| repo
stringlengths 33
86
|
---|---|---|---|---|---|---|---|---|
[
"MIT"
] | 0.1.0 | 6eba29d96bef815c6fda407240c0c4ba358f0f6f | code | 755 | module OpenADMIXTURE
using Random, LoopVectorization
import LinearAlgebra: svd, norm, diag, mul!, dot
using SnpArrays
using Base.Threads
using SparseKmeansFeatureRanking
export AdmixData
using Requires, Adapt
using Polyester
using ProgressMeter, Suppressor, Formatting
function __init__()
@require CUDA="052768ef-5323-5732-b1bb-66c8b64840ba" begin
using .CUDA
CUDA.allowscalar(true)
include("cuda/structs.jl")
include("cuda/kernels.jl")
include("cuda/runners.jl")
include("cuda/transfer.jl")
end
end
include("structs.jl")
include("projections.jl")
include("qp.jl")
include("quasi_newton.jl")
include("loops.jl")
include("algorithms_inner.jl")
include("algorithms_outer.jl")
include("driver.jl")
end
| OpenADMIXTURE | https://github.com/OpenMendel/OpenADMIXTURE.jl.git |
|
[
"MIT"
] | 0.1.0 | 6eba29d96bef815c6fda407240c0c4ba358f0f6f | code | 11198 | """
loglikelihood(g, q, p, qp_small, K, skipmissing)
Compute loglikelihood.
# Input
- `g`: genotype matrix
- `q`: Q matrix
- `p`: P matrix
- `qp_small`: cache memory for storing partial QP matrix.
- `K`: number of clusters
- `skipmissing`: must be `true` in most cases
"""
function loglikelihood(g::AbstractArray{T}, q, p, qp_small::AbstractArray{T, 3}, K, skipmissing) where T
I = size(q, 2)
J = size(p, 2)
#K = 0 # dummy
# r = tiler_scalar_1d(loglikelihood_loop, typeof(qp_small), zero(T), (g, q, p, qp_small), 1:I, 1:J, K)
if !skipmissing
r = loglikelihood_loop(g, q, p, nothing, 1:I, 1:J, K)
else
r = loglikelihood_loop_skipmissing(g, q, p, nothing, 1:I, 1:J, K)
end
# r = tiler_scalar(loglikelihood_loop, typeof(qp_small), zero(T), (g, q, p, qp_small), 1:I, 1:J, K)
r
end
function loglikelihood(g::SnpLinAlg{T}, q, p, qp_small::AbstractArray{T, 3}, K, skipmissing) where T
I = size(q, 2)
J = size(p, 2)
#K = 0 # dummy
r = threader_scalar(skipmissing ? loglikelihood_loop_skipmissing : loglikelihood_loop,
typeof(qp_small), zero(Float64), (g, q, p, qp_small), 1:I, 1:J, K)
# r = loglikelihood_loop(g, q, p, nothing, 1:I, 1:J, K)
r
end
# function loglikelihood(g::AbstractArray{T}, q, p, qp_small, K, tmp) where T
# I = size(q, 2)
# J = size(p, 2)
# #K = 0 # dummy
# # r = tiler_scalar_1d(loglikelihood_loop, typeof(qp_small), zero(T), (g, q, p, qp_small), 1:I, 1:J, K)
# r = loglikelihood_loop(g, q, p, nothing, 1:I, 1:J, K, tmp)
# r
# end
# function loglikelihood(g::AbstractArray{T}, qf) where T
# I = size(qp, 1)
# J = size(qp, 2)
# K = 0 # dummy
# r = loglikelihood_loop(g, qp, 1:I, 1:J, K)
# r
# end
"""
em_q!(d, g, mode=:base, verbose=false)
FRAPPE EM algorithm for updaing Q.
Assumes qp to be pre-computed.
# Input
- `d`: an `AdmixData`.
- `g`: a genotype matrix.
- `mode`: selects which member of `d` is used..
- `:base` or `:fallback`: `q_next` is updated based on `q` and `p`.
- `:fallback2`: `q_next2` is updated based on `q_next` and `p_next`.
- `verbose`: whether to print timings (boolean)
"""
# function em_q!(q_new, g::AbstractArray{T}, q, p, qf) where T # summation over j, block I side.
function em_q!(d::AdmixData{T}, g::AbstractArray{T}; mode=:base, verbose=false) where T
I, J, K = d.I, d.J, d.K
qp_small = d.qp_small
if mode == :base || mode == :fallback
q_next, q, p = d.q_next, d.q, d.p
elseif mode == :fallback2
q_next, q, p = d.q_next2, d.q_next, d.p_next
end
fill!(q_next, zero(T))
if verbose
@time threader!(d.skipmissing ? em_q_loop_skipmissing! : em_q_loop!,
typeof(q_next), (q_next, g, q, p, qp_small),
1:I, 1:J, K, true; maxL=64)
else
threader!(d.skipmissing ? em_q_loop_skipmissing! : em_q_loop!,
typeof(q_next), (q_next, g, q, p, qp_small),
1:I, 1:J, K, true; maxL=64)
end
# @time tiler!(d.skipmissing ? em_q_loop_skipmissing! : em_q_loop!,
# typeof(q_next), (q_next, g, q, p, qp_small), 1:I, 1:J, K)
# @time em_q_loop!(q_next, g, q, p, qp, 1:I, 1:J, K)
q_next ./= 2J
# @tullio q_new[k, i] = (g[i, j] * q[k, i] * f[k, j] / qf[i, j] +
# (2 - g[i, j]) * q[k, i] * (1 - f[k, j]) / (1 - qf[i, j])) / 2J
end
"""
em_p!(d, g, mode=:base, verbose=false)
FRAPPE EM algorithm for updaing P.
Assumes qp to be pre-computed.
# Input
- `d`: an `AdmixData`.
- `g`: a genotype matrix.
- `mode`: selects which member of `d` is used..
- `:base`: `p_next` is updated based on `q` and `p`.
- `:fallback`: `p_next` is updated based on `q_next` and `p`.
- `:fallback2`: `p_next2` is updated based on `q_next` and `p_next`.
- `verbose`: whether to print timings (boolean)
"""
function em_p!(d::AdmixData{T}, g::AbstractArray{T}; mode=:base, verbose=false) where T
# summation over i: block J side.
I, J, K = d.I, d.J, d.K
p_tmp, qp_small = d.p_tmp, d.qp_small
if mode == :base
p_next, p, q = d.p_next, d.p, d.q
elseif mode == :fallback
p_next, p, q = d.p_next, d.p, d.q_next
elseif mode == :fallback2
p_next, p, q = d.p_next2, d.p_next, d.q_next2
end
fill!(p_tmp, zero(T))
fill!(p_next, zero(T))
if verbose
@time threader!(d.skipmissing ? em_p_loop_skipmissing! : em_p_loop!,
typeof(p_next), (p_next, g, q, p, p_tmp, qp_small),
1:I, 1:J, K, false; maxL=64)
else
threader!(d.skipmissing ? em_p_loop_skipmissing! : em_p_loop!,
typeof(p_next), (p_next, g, q, p, p_tmp, qp_small),
1:I, 1:J, K, false; maxL=64)
end
# @time tiler!(d.skipmissing ? em_f_loop_skipmissing! : em_f_loop!,
# typeof(p_next), (p_next, g, q, p, f_tmp, qp_small), 1:I, 1:J, K)
# @time em_f_loop!(p_next, g, q, p, f_tmp, qp_small, 1:I, 1:J, K)
@turbo for j in 1:J
for k in 1:K
p_next[k, j] = p_tmp[k, j] / (p_tmp[k, j] + p_next[k, j])
end
end
# @tullio f_tmp[k, j] = g[i, j] * q[k, i] * f[k, j] / qf[i, j]
# @tullio f_new[k, j] = (2 - g[i, j]) * q[k, i] * (1 - f[k, j]) / (1 - qf[i, j])
# @tullio f_new[k, j] = f_tmp[k, j] / (f_tmp[k, j] + f_new[k, j])
end
const tau_schedule = collect(0.7^i for i in 1:10)
function print_timed(timed_results, verbose::Bool)
if verbose
allocs = timed_results.gcstats.malloc + timed_results.gcstats.realloc +
timed_results.gcstats.poolalloc + timed_results.gcstats.bigalloc
allocd = timed_results.gcstats.allocd
seconds = timed_results.time
println("$seconds seconds ($allocs allocations: $allocd bytes)")
end
end
"""
update_q!(d, g, update2=false; d_cu=nothing, g_cu=nothing, verbose=false)
Update Q using sequential quadratic programming.
# Input
- `d`: an `AdmixData`.
- `g`: a genotype matrix.
- `update2`: if running the second update for quasi-Newton step
- `d_cu`: a `CuAdmixData` if using GPU, `nothing` otherwise.
- `g_cu`: a `CuMatrix{UInt8}` corresponding to the data part of
- `verbose`: whether to print timings (boolean)
"""
function update_q!(d::AdmixData{T}, g::AbstractArray{T}, update2=false;
d_cu=nothing, g_cu=nothing, verbose=false) where T
# function update_q!(q_next, g::AbstractArray{T}, q, p, qdiff, XtX, Xtz, qf) where T
I, J, K = d.I, d.J, d.K
qdiff, XtX, Xtz, qp_small = d.q_tmp, d.XtX_q, d.Xtz_q, d.qp_small
q_next = update2 ? d.q_next2 : d.q_next
q = update2 ? d.q_next : d.q
p = update2 ? d.p_next : d.p
qv = update2 ? d.q_nextv : d.qv
qdiffv = d.q_tmpv
XtXv = d.XtX_qv
Xtzv = d.Xtz_qv
# qf!(qp, q, f)
d.ll_prev = d.ll_new # loglikelihood(g, qf)
if verbose
println(d.ll_prev)
end
a = @timed if d_cu === nothing # CPU operation
fill!(XtX, zero(T))
fill!(Xtz, zero(T))
threader!(d.skipmissing ? update_q_loop_skipmissing! : update_q_loop!,
typeof(XtX), (XtX, Xtz, g, q, p, qp_small), 1:I, 1:J, K, true; maxL=16)
else # GPU operation
@assert d.skipmissing "`skipmissing`` must be true for GPU computation"
copyto_sync!([d_cu.q, d_cu.p], [q, p])
update_q_cuda!(d_cu, g_cu)
copyto_sync!([XtX, Xtz], [d_cu.XtX_q, d_cu.Xtz_q])
end
print_timed(a, verbose)
# Solve the quadratic programs
b = @timed begin
Xtz .*= -1
pmin = zeros(T, K)
pmax = ones(T, K)
@batch threadlocal=QPThreadLocal{T}(K) for i in 1:I
# even the views are allocating something, so we use preallocated views.
XtX_ = XtXv[i]
Xtz_ = Xtzv[i]
q_ = qv[i]
qdiff_ = qdiffv[i]
tableau_k2 = threadlocal.tableau_k2
tmp_k = threadlocal.tmp_k
tmp_k2 = threadlocal.tmp_k2
tmp_k2_ = threadlocal.tmp_k2_
swept = threadlocal.swept
create_tableau!(tableau_k2, XtX_, Xtz_, q_, d.v_kk, tmp_k, true)
quadratic_program!(qdiff_, tableau_k2, q_, pmin, pmax, K, 1,
tmp_k2, tmp_k2_, swept)
end
@turbo for i in 1:I
for k in 1:K
q_next[k, i] = q[k, i] + qdiff[k, i]
end
end
project_q!(q_next, d.idxv[1])
end
print_timed(b, verbose)
end
"""
update_p!(d, g, update2=false; d_cu=nothing, g_cu=nothing, verbose=false)
Update P with sequential quadratic programming.
# Input
- `d`: an `AdmixData`.
- `g`: a genotype matrix.
- `update2`: if running the second update for quasi-Newton step
- `d_cu`: a `CuAdmixData` if using GPU, `nothing` otherwise.
- `g_cu`: a `CuMatrix{UInt8}` corresponding to the data part of
- `verbose`: whether to print timings (boolean)
"""
function update_p!(d::AdmixData{T}, g::AbstractArray{T},
update2=false; d_cu=nothing, g_cu=nothing,
verbose=false) where T
# function update_p!(p_next, g::AbstractArray{T}, p, q, fdiff, XtX, Xtz, qf) where T
I, J, K = d.I, d.J, d.K
pdiff, XtX, Xtz, qp_small = d.p_tmp, d.XtX_p, d.Xtz_p, d.qp_small
p_next = update2 ? d.p_next2 : d.p_next
q = update2 ? d.q_next2 : d.q_next
p = update2 ? d.p_next : d.p
pv = update2 ? d.p_nextv : d.pv
pdiffv = d.p_tmpv
XtXv = d.XtX_pv
Xtzv = d.Xtz_pv
d.ll_prev = d.ll_new
a = @timed if d_cu === nothing # CPU operation
fill!(XtX, zero(T))
fill!(Xtz, zero(T))
threader!(d.skipmissing ? update_p_loop_skipmissing! : update_p_loop!,
typeof(XtX), (XtX, Xtz, g, q, p, qp_small), 1:I, 1:J, K, false; maxL=16)
else # GPU operation
@assert d.skipmissing "`skipmissing`` must be true for GPU computation"
copyto_sync!([d_cu.q, d_cu.p], [q, p])
update_p_cuda!(d_cu, g_cu)
copyto_sync!([XtX, Xtz], [d_cu.XtX_p, d_cu.Xtz_p])
end
print_timed(a, verbose)
# solve quadratic programming problems.
b = @timed begin
Xtz .*= -1
pmin = zeros(T, K)
pmax = ones(T, K)
@batch threadlocal=QPThreadLocal{T}(K) for j in 1:J
# even the views are allocating something, so we use preallocated views.
XtX_ = XtXv[j]
Xtz_ = Xtzv[j]
p_ = pv[j]
pdiff_ = pdiffv[j]
t = threadid()
tableau_k1 = threadlocal.tableau_k1
tmp_k = threadlocal.tmp_k
tmp_k1 = threadlocal.tmp_k1
tmp_k1_ = threadlocal.tmp_k1_
swept = threadlocal.swept
create_tableau!(tableau_k1, XtX_, Xtz_, p_, d.v_kk, tmp_k, false)
quadratic_program!(pdiff_, tableau_k1, p_, pmin, pmax, K, 0,
tmp_k1, tmp_k1_, swept)
end
@turbo for j in 1:J
for k in 1:K
p_next[k, j] = p[k, j] + pdiff[k, j]
end
end
# p_next .= f .+ fdiff
project_p!(p_next)
# println(maximum(abs.(fdiff)))
end
print_timed(b, verbose)
end
| OpenADMIXTURE | https://github.com/OpenMendel/OpenADMIXTURE.jl.git |
|
[
"MIT"
] | 0.1.0 | 6eba29d96bef815c6fda407240c0c4ba358f0f6f | code | 7421 | """
init_em!(d, g, iter; d_cu=nothing, g_cu=nothing)
Initialize P and Q with the FRAPPE EM algorithm
# Input
- `d`: an `AdmixData`.
- `g`: a genotype matrix.
- `iter`: number of iterations.
- `d_cu`: a `CuAdmixData` if using GPU, `nothing` otherwise.
- `g_cu`: a `CuMatrix{UInt8}` corresponding to the data part of
"""
function init_em!(d::AdmixData{T}, g::AbstractArray{T}, iter::Integer;
d_cu=nothing, g_cu=nothing, verbose=false) where T
# qf!(d.qf, d.q, d.f)
if d_cu !== nothing
copyto_sync!([d_cu.q, d_cu.p], [d.q, d.p])
end
d_ = (d_cu === nothing) ? d : d_cu
g_ = (g_cu === nothing) ? g : g_cu
println("Performing $iter EM steps for priming")
for i in 1:iter
if verbose
println("Initialization EM Iteration $(i)")
end
t = @timed begin
em_q!(d_, g_; verbose=verbose)
em_p!(d_, g_; verbose=verbose)
d_.p .= d_.p_next
d_.q .= d_.q_next
end
if d_cu !== nothing
copyto_sync!([d.p, d.q], [d_cu.p, d_cu.q])
ll = loglikelihood(d_cu, g_cu)
else
ll = loglikelihood(g, d.q, d.p, d.qp_small, d.K, d.skipmissing)
end
println("EM Iteration $(i) ($(t.time) sec): Loglikelihood = $ll")
end
if d_cu !== nothing
copyto_sync!([d.p, d.q], [d_cu.p, d_cu.q])
d.ll_new = loglikelihood(d_cu, g_cu)
else
d.ll_new = loglikelihood(g, d.q, d.p, d.qp_small, d.K, d.skipmissing)
end
end
"""
admixture_qn!(d, g, iter=1000, rtol=1e-7; d_cu=nothing, g_cu=nothing,
mode=:ZAL, iter_count_offset=0)
Initialize P and Q with the FRAPPE EM algorithm
# Input
- `d`: an `AdmixData`.
- `g`: a genotype matrix.
- `iter`: number of iterations.
- `rtol`: convergence tolerance in terms of relative change of loglikelihood.
- `d_cu`: a `CuAdmixData` if using GPU, `nothing` otherwise.
- `g_cu`: a `CuMatrix{UInt8}` corresponding to the data part of genotype matrix
- `mode`: `:ZAL` for Zhou-Alexander-Lange acceleration (2009), `:LBQN` for Agarwal-Xu (2020).
- `verbose`: Print verbose timing information like original script.
- `progress_bar`: Show progress bar while executing.
"""
function admixture_qn!(d::AdmixData{T}, g::AbstractArray{T}, iter::Int=1000,
rtol= 1e-7; d_cu=nothing, g_cu=nothing, mode=:ZAL, iter_count_offset=0, fix_p=false, fix_q=false,
verbose=false, progress_bar=false) where T
# qf!(d.qf, d.q, d.f)
# ll_prev = loglikelihood(g, d.q, d.f, d.qp_small, d.K, d.skipmissing)
# d.ll_new = ll_prev
bgpartial = ['▁' ,'▂' ,'▃' ,'▄' ,'▅' ,'▆', '▇']
p = Progress(iter, dt=0.5, desc="Running main algorithm",
barglyphs=BarGlyphs('|','█', bgpartial,' ','|'),
barlen=50, showspeed=true, enabled=progress_bar);
fspec = FormatSpec(".4e")
if isnan(d.ll_new)
if d_cu !== nothing
copyto_sync!([d_cu.p, d_cu.q], [d.p, d.q])
d.ll_new = loglikelihood(d_cu, g_cu)
else
d.ll_new = loglikelihood(g, d.q, d.p, d.qp_small, d.K, d.skipmissing)
end
end
println("initial ll: ", d.ll_new)
if !progress_bar
println("Starting main algorithm")
end
llhist = [d.ll_new]
converged = false
i = 0
loopstats = @timed for outer i = (iter_count_offset + 1):iter
iterinfo = @timed begin
# qf!(d.qf, d.q, d.f)
# ll_prev = loglikelihood(g, d.qf)
d.ll_prev = d.ll_new
if !fix_q
update_q!(d, g; d_cu=d_cu, g_cu=g_cu, verbose=verbose)
else
d.q_next .= d.q
end
if !fix_p
update_p!(d, g; d_cu=d_cu, g_cu=g_cu, verbose=verbose)
else
d.p_next .= d.p
end
if !fix_q
update_q!(d, g, true; d_cu=d_cu, g_cu=g_cu, verbose=verbose)
else
d.q_next2 .= d.q
end
if !fix_p
update_p!(d, g, true; d_cu=d_cu, g_cu=g_cu, verbose=verbose)
else
d.p_next2 .= d.p
end
# qf!(d.qf, d.q_next2, d.f_next2)
ll_basic = if d_cu !== nothing
copyto_sync!([d_cu.p, d_cu.q], [d.p_next2, d.q_next2])
loglikelihood(d_cu, g_cu)
else
loglikelihood(g, d.q_next2, d.p_next2, d.qp_small, d.K, d.skipmissing)
end
if mode == :ZAL
update_UV!(d.U, d.V, d.x_flat, d.x_next_flat, d.x_next2_flat, i, d.Q)
U_part = i < d.Q ? view(d.U, :, 1:i) : view(d.U, :, :)
V_part = i < d.Q ? view(d.V, :, 1:i) : view(d.V, :, :)
update_QN!(d.x_tmp_flat, d.x_next_flat, d.x_flat, U_part, V_part)
elseif mode == :LBQN
update_UV_LBQN!(d.U, d.V, d.x_flat, d.x_next_flat, d.x_next2_flat, i, d.Q)
U_part = i < d.Q ? view(d.U, :, 1:i) : view(d.U, :, :)
V_part = i < d.Q ? view(d.V, :, 1:i) : view(d.V, :, :)
update_QN_LBQN!(d.x_tmp_flat, d.x_flat, d.x_qq, d.x_rr, U_part, V_part)
else
@assert false "Invalid mode"
end
project_p!(d.p_tmp)
project_q!(d.q_tmp, d.idxv[1])
# qf!(d.qf, d.q_tmp, d.f_tmp)
ll_qn = if d_cu !== nothing # GPU mode
copyto_sync!([d_cu.p, d_cu.q], [d.p_tmp, d.q_tmp])
loglikelihood(d_cu, g_cu)
else # CPU mode
loglikelihood(g, d.q_tmp, d.p_tmp, d.qp_small, d.K, d.skipmissing)
end
if d.ll_prev < ll_qn
d.x .= d.x_tmp
d.ll_new = ll_qn
else
d.x .= d.x_next2
d.ll_new = ll_basic
end
(prev = d.ll_prev, basic = ll_basic, qn = ll_qn, new = d.ll_new,
reldiff = abs((d.ll_new - d.ll_prev) / d.ll_prev))
end
lls = iterinfo[1]
println("Iteration $i ($(iterinfo.time) sec): " *
"LogLikelihood = $(lls.new), reldiff = $(lls.reldiff)")
ll_other = "Previous = $(lls.prev) QN = $(lls.qn), Basic = $(lls.basic)"
println(" LogLikelihoods: $ll_other")
if verbose
println("\n")
end
last_vals = map((x) -> fmt(fspec, x),
llhist[end-min(length(llhist)-1, 5):end])
ProgressMeter.next!(p;
showvalues = [
(:INFO,"Percent is for max $iter iterations. " *
"Likely to converge early at LL ↗ of $rtol."),
(:Iteration,i),
(Symbol("Execution time"),iterinfo.time),
(Symbol("Initial LogLikelihood"),llhist[1]),
(Symbol("Current LogLikelihood"),lls.new),
(Symbol("Other LogLikelihoods"),ll_other),
(Symbol("LogLikelihood ↗"),lls.reldiff),
(Symbol("Past LogLikelihoods"),last_vals)])
push!(llhist, lls.new)
if lls.reldiff < rtol
converged = true
ProgressMeter.finish!(p)
break
end
end
if converged
println("Main algorithm converged in $i iterations over $(loopstats.time) sec.")
else
println("Main algorithm failed to converge after $i iterations over $(loopstats.time) sec.")
end
end
| OpenADMIXTURE | https://github.com/OpenMendel/OpenADMIXTURE.jl.git |
|
[
"MIT"
] | 0.1.0 | 6eba29d96bef815c6fda407240c0c4ba358f0f6f | code | 6961 | """
run_admixture(filename, K;
rng=Random.GLOBAL_RNG,
sparsity=nothing,
prefix=filename[1:end-4],
skfr_tries = 1,
skfr_max_inner_iter = 50,
admix_n_iter = 1000,
admix_rtol=1e-7,
admix_n_em_iter = 5,
T = Float64,
Q = 3,
use_gpu=false,
verbose=false,
progress_bar=true)
The main runner function for admixture.
# Input:
- `filename``: the PLINK BED file name to analyze, including the extension.
- `K`: number of clusters.
- `rng`: random number generator.
- `sparsity`: number of AIMs to be utilized. `nothing` to not run the SKFR step.
- `prefix`: prefix used for the output PLINK file if SKFR is used.
- `skfr_tries`: number of repeats of SKFR with different initializations.
- `skfr_max_inner_iter`: maximum number of iterations for each call for SKFR.
- `admix_n_iter`: number of Admixture iterations
- `admix_rtol`: relative tolerance for Admixture
- `admix_n_em_iters`: number of iterations for EM initialization
- `T`: Internal type for floating-point numbers
- `Q`: number of steps used for quasi-Newton acceleration
- `use_gpu`: whether to use GPU for computation
- `progress_bar`: whether to show a progress bar for main loop
"""
function run_admixture(filename, K;
rng=Random.GLOBAL_RNG,
sparsity=nothing,
prefix=filename[1:end-4],
skfr_tries = 1,
skfr_max_inner_iter=50,
skfr_mode=:global,
admix_n_iter=1000,
admix_rtol=1e-7,
admix_n_em_iters = 5,
T=Float64,
Q=3,
use_gpu=false,
verbose=false,
progress_bar=true,
fix_q=false,
fix_p=false,
init_q=nothing,
init_p=nothing)
@assert endswith(filename, ".bed") "filename should end with .bed"
println("Using $filename as input.")
if sparsity !== nothing
ftn = if skfr_mode == :global
SparseKmeansFeatureRanking.sparsekmeans1
elseif skfr_mode == :local
SparseKmeansFeatureRanking.sparsekmeans2
else
@assert false "skfr_mode can only be :global or :local"
end
admix_input, clusters, aims = _filter_SKFR(filename, K, sparsity; rng=rng, prefix=prefix,
tries=skfr_tries, max_inner_iter=skfr_max_inner_iter, ftn=ftn)
else
admix_input = filename
clusters, aims = nothing, nothing
end
d = _admixture_base(admix_input, K;
n_iter=admix_n_iter, rtol=admix_rtol, rng=rng, em_iters=admix_n_em_iters,
T=T, Q=Q, use_gpu=use_gpu, verbose=verbose, progress_bar=progress_bar,
fix_q=fix_q, fix_p=fix_p, init_q=init_q, init_p=init_p)
d, clusters, aims
end
"""
Run SKFR then filter the PLINK file to only keep AIMs.
"""
function _filter_SKFR(filename, K, sparsity::Integer;
rng=Random.GLOBAL_RNG,
prefix=filename[1:end-4],
tries = 10,
max_inner_iter = 50,
ftn = SparseKmeansFeatureRanking.sparsekmeans1)
@assert endswith(filename, ".bed") "filename should end with .bed"
g = SnpArray(filename)
ISM = SparseKmeansFeatureRanking.ImputedSnpMatrix{Float64}(g, K; rng=rng)
if tries == 1
(clusters, _, aims, _, _) = ftn(ISM, sparsity; max_iter = max_inner_iter, squares=false)
else
(clusters, _, aims, _, _, _) = SparseKmeansFeatureRanking.sparsekmeans_repeat(ISM, sparsity; iter = tries,
max_inner_iter=max_inner_iter, ftn=ftn)
end
I, J = size(g)
aims_sorted = sort(aims)
des = "$(prefix)_$(K)_$(sparsity)aims"
println(des)
SnpArrays.filter(filename[1:end-4], trues(I), aims_sorted; des=des)
des * ".bed", clusters, aims
end
"""
Run SKFR then filter the PLINK file to only keep AIMs. Run for multiple sparsities (in decreasing order)
"""
function _filter_SKFR(filename, K, sparsities::AbstractVector{<:Integer};
rng=Random.GLOBAL_RNG,
prefix=filename[1:end-4],
tries = 10,
max_inner_iter = 50)
@assert endswith(filename, ".bed") "filename should end with .bed"
g = SnpArray(filename)
ISM = SparseKmeansFeatureRanking.ImputedSnpMatrix{Float64}(g, K; rng=rng)
if typeof(sparsities) <: AbstractVector
@assert issorted(sparsities; rev=true) "sparsities should be decreasing"
(clusters, aims) = SparseKmeansFeatureRanking.sparsekmeans_path(ISM, sparsities; iter=tries, max_inner_iter=max_inner_iter)
end
I, J = size(g)
outputfiles = String[]
for (s, aimlist) in zip(sparsities, aims)
aimlist_sorted = sort(aimlist)
des = "$(prefix)_$(K)_$(s)aims"
SnpArrays.filter(filename[1:end-4], trues(I), aimlist_sorted; des=des)
push!(outputfiles, des)
end
outputfiles, clusters, aims
end
function _admixture_base(filename, K;
n_iter=1000,
rtol=1e-7,
rng=Random.GLOBAL_RNG,
em_iters = 5,
T=Float64,
Q=3,
use_gpu=false,
verbose=false,
progress_bar=true,
fix_q=false,
fix_p=false,
init_q=nothing,
init_p=nothing)
println("Loading genotype data...")
g = SnpArray(filename)
g_la = SnpLinAlg{T}(g)
I = size(g_la, 1)
J = size(g_la, 2)
println("Loaded $I samples and $J SNPs")
d = AdmixData{T}(I, J, K, Q; skipmissing=true, rng=rng)
if use_gpu
d_cu, g_cu = _cu_admixture_base(d, g_la, I, J)
else
d_cu = nothing
g_cu = nothing
end
if verbose
if init_q === nothing || init_p === nothing
@time init_em!(d, g_la, em_iters;
d_cu = d_cu, g_cu=g_cu, verbose=verbose)
end
if init_q !== nothing
d.q .= init_q
end
if init_p !== nothing
d.p .= init_p
end
@time if progress_bar
messages = @capture_out admixture_qn!(d, g_la, n_iter, rtol;
d_cu = d_cu, g_cu = g_cu, mode=:ZAL,
verbose=verbose, progress_bar=true,
fix_q=fix_q, fix_p=fix_p)
println(messages)
else
admixture_qn!(d, g_la, n_iter, rtol;
d_cu = d_cu, g_cu = g_cu, mode=:ZAL,
fix_q=fix_q, fix_p=fix_p,
verbose=verbose)
end
else
if init_q === nothing || init_p === nothing
init_em!(d, g_la, em_iters; d_cu = d_cu, g_cu=g_cu)
end
if init_q !== nothing
d.q .= init_q
end
if init_p !== nothing
d.p .= init_p
end
if progress_bar
messages = @capture_out admixture_qn!(d, g_la, n_iter, rtol;
d_cu = d_cu, g_cu = g_cu, mode=:ZAL, progress_bar=true,
fix_q=fix_q, fix_p=fix_p)
println(messages)
else
admixture_qn!(d, g_la, n_iter, rtol;
d_cu = d_cu, g_cu = g_cu, mode=:ZAL,
fix_q=fix_q, fix_p=fix_p,
verbose=verbose)
end
end
d
end
function _cu_admixture_base(d, g_la, I, J)
# dummy, main body defined inside CUDA portion.
end | OpenADMIXTURE | https://github.com/OpenMendel/OpenADMIXTURE.jl.git |
|
[
"MIT"
] | 0.1.0 | 6eba29d96bef815c6fda407240c0c4ba358f0f6f | code | 35904 | const TILE = Ref(512) # this is now a length, in bytes!
const nonmissing_map_Float64 = [1.0, 0.0, 1.0, 1.0]
const g_map_Float64 = [0.0, 0.0, 1.0, 2.0]
const nonmissing_map_Float32 = [1.0f0, 0.0f0, 1.0f0, 1.0f0]
const g_map_Float32 = [0.0f0, 0.0f0, 1.0f0, 2.0f0]
function tile_maxiter(::Type{<:AbstractArray{T}}) where {T}
isbitstype(T) || return TILE[] ÷ 8
max(TILE[] ÷ sizeof(T), 4)
end
tile_maxiter(::Type{AT}) where {AT} = TILE[] ÷ 8 # treat anything unkown like Float64
"""
Split the index space into two pieces.
"""
@inline function findcleft(r::UnitRange, step::Int)
if length(r) >= 2*step
minimum(r) - 1 + step * div(length(r), step * 2)
else
# minimum(r) - 1 + div(length(r), 2, RoundNearest) # not in Julia 1.3
minimum(r) - 1 + round(Int, length(r)/2)
end
end
@inline function cleave(range::UnitRange, step::Int=4)
cleft = findcleft(range, step)
first(range):cleft, cleft+1:last(range)
end
@inline maybe32divsize(::Type{<:AbstractArray{T}}) where T<:Number = max(1, 32 ÷ sizeof(T))
@inline maybe32divsize(::Type) = 4
"""
Split the index space into three.
"""
@inline function findthree(r::UnitRange)
d = div(length(r), 3)
i0 = first(r)
(i0 : i0+d-1), (i0+d : i0+2d-1), (i0+2d : i0+length(r)-1)
end
"""
threader!(ftn!, ::Type{T}, arrs, irange, jrange, K, split_i;
maxL=tile_maxiter(T), threads=nthreads())
Apply `ftn!` over the index space `irange` x `jrange` of `arrs` with multiple threads.
It splits the index space in one dimension over either i dimension or j dimension
in a single call.
It recursively calls `threader!()` without changing `split_i` or
calls `tiler!()` to eventually split in both directions.
# Input
- `ftn!`: The function to be applied
- `T`: element type of updated `arrs`
- `arrs`: a tuple of arrays to be updated
- `irange`: an index range
- `jrange`: another index range
- `K`: number of clusters
- `split_i`: if the dimension to split along is the "i" dimension. "j" dimension is split if `false`.
- `maxL`: maximum length of tile.
- `threads`: number of threads to be utilized
"""
function threader!(ftn!::F, ::Type{T}, arrs::Tuple, irange, jrange, K, split_i::Bool;
maxL = tile_maxiter(T), threads=nthreads()) where {F <: Function, T}
threads = min(threads, split_i ? length(irange) : length(jrange))
# println("starting threader: $irange $jrange on $(threadid()), $threads threads")
if threads == 1 # single-thread
# println("launch tiler: $irange $jrange on thread # $(threadid())")
tiler!(ftn!, T, arrs, irange, jrange, K; maxL=maxL)
return
elseif threads > 2 && threads % 3 == 0 # split into three pieces
if split_i
I1, I2, I3 = findthree(irange)
task1 = Threads.@spawn begin
# println("threader: $I1 $jrange on $(threadid())")
threader!(ftn!, T, arrs, I1, jrange, K, split_i; maxL=maxL, threads=threads÷3)
end
task2 = Threads.@spawn begin
# println("threader: $I2 $jrange on $(threadid())")
threader!(ftn!, T, arrs, I2, jrange, K, split_i; maxL=maxL, threads=threads÷3)
end
# println("threader: $I3 $jrange on $(threadid())")
threader!(ftn!, T, arrs, I3, jrange, K, split_i; maxL=maxL, threads=threads÷3)
wait(task1)
wait(task2)
else # split j.
J1, J2, J3 = findthree(jrange)
task1 = Threads.@spawn begin
# println("threader: $irange $J1 on $(threadid())")
threader!(ftn!, T, arrs, irange, J1, K, split_i; maxL=maxL, threads=threads÷3)
end
task2 = Threads.@spawn begin
# println("threader: $irange $J2 on $(threadid())")
threader!(ftn!, T, arrs, irange, J2, K, split_i; maxL=maxL, threads=threads÷3)
end
# println("threader: $irange $J3 on $(threadid())")
threader!(ftn!, T, arrs, irange, J3, K, split_i; maxL=maxL, threads=threads÷3)
wait(task1)
wait(task2)
end
else # split into two pieces.
if split_i
I1, I2 = cleave(irange, maybe32divsize(T))
task = Threads.@spawn begin
# println("threader: $I1 $jrange on $(threadid())")
threader!(ftn!, T, arrs, I1, jrange, K, split_i; maxL=maxL, threads=threads÷2)
end
# println("threader: $I2 $jrange on $(threadid())")
threader!(ftn!, T, arrs, I2, jrange, K, split_i; maxL=maxL, threads=threads÷2)
wait(task)
else # split j.
J1, J2 = cleave(jrange, maybe32divsize(T))
task = Threads.@spawn begin
# println("threader: $irange $J1 on $(threadid())")
threader!(ftn!, T, arrs, irange, J1, K, split_i; maxL=maxL, threads=threads÷2)
end
# println("threader: $irange $J2 on $(threadid())")
threader!(ftn!, T, arrs, irange, J2, K, split_i; maxL=maxL, threads=threads÷2)
wait(task)
end
end
end
"""
tiler!(ftn!, ::Type{T}, arrs, irange, jrange, K;
maxL=tile_maxiter(T), threads=nthreads())
Apply `ftn!` over the index space `irange` x `jrange` of `arrs` with a single thread.
It splits the index space in two dimensions in small tiles.
# Input
- `ftn!`: The function to be applied
- `T`: element type of updated `arrs`
- `arrs`: a tuple of arrays to be updated
- `irange`: an index range
- `jrange`: another index range
- `K`: number of clusters
- `maxL`: maximum length of tile.
"""
function tiler!(ftn!::F, ::Type{T}, arrs::Tuple, irange, jrange, K; maxL = tile_maxiter(T)) where {F <: Function, T}
ilen, jlen = length(irange), length(jrange)
maxL = tile_maxiter(T)
if ilen > maxL || jlen > maxL
if ilen > jlen # split i dimension
I1s, I2s = cleave(irange, maybe32divsize(T))
tiler!(ftn!, T, arrs, I1s, jrange, K; maxL=maxL)
tiler!(ftn!, T, arrs, I2s, jrange, K; maxL=maxL)
else # split j dimension
J1s, J2s = cleave(jrange, maybe32divsize(T))
tiler!(ftn!, T, arrs, irange, J1s, K; maxL=maxL)
tiler!(ftn!, T, arrs, irange, J2s, K; maxL=maxL)
end
else # base case.
ftn!(arrs..., irange, jrange, K)
end
end
"""
tiler_1d!(ftn!, ::Type{T}, arrs, irange, jrange, K;
maxL=tile_maxiter(T), threads=nthreads())
Apply `ftn!` over the index space `irange` x `jrange` of `arrs` with a single thread.
It splits the index space only in j dimension.
# Input
- `ftn!`: The function to be applied
- `T`: element type of updated `arrs`
- `arrs`: a tuple of arrays to be updated
- `irange`: an index range
- `jrange`: another index range
- `K`: number of clusters
- `maxL`: maximum length of tile.
"""
function tiler_1d!(ftn!::F, ::Type{T}, arrs::Tuple, irange, jrange, K; maxL = tile_maxiter(T)) where {F <: Function, T}
ilen, jlen = length(irange), length(jrange)
maxL = tile_maxiter(T)
if jlen > maxL
J1s, J2s = cleave(jrange)
tiler!(ftn!, T, arrs, irange, J1s, K)
tiler!(ftn!, T, arrs, irange, J2s, K)
else
ftn!(arrs..., irange, jrange, K)
end
end
"""
tiler_scalar(ftn, ::Type{T}, z::RT, arrs, irange, jrange, K;
maxL=tile_maxiter(T))
Apply a reduction `ftn` over the index space `irange` x `jrange` of `arrs` with a single thread.
It splits the index space both dimensions.
# Input
- `ftn`: The function to be applied
- `T`: element type of updated `arrs`
- `z`: the "zero" value for the return.
- `arrs`: a tuple of input arrays
- `irange`: an index range
- `jrange`: another index range
- `K`: number of clusters
- `maxL`: maximum length of tile.
"""
function tiler_scalar(ftn::F, ::Type{T}, z::RT, arrs::Tuple, irange, jrange, K; maxL=tile_maxiter(T)) where {F <: Function, T, RT}
ilen, jlen = length(irange), length(jrange)
maxL = tile_maxiter(T)
r = z
if ilen > maxL || jlen > maxL
if ilen > jlen
I1s, I2s = cleave(irange)
r += tiler_scalar(ftn, T, z, arrs, I1s, jrange, K)
r += tiler_scalar(ftn, T, z, arrs, I2s, jrange, K)
else
J1s, J2s = cleave(jrange)
r += tiler_scalar(ftn, T, z, arrs, irange, J1s, K)
r += tiler_scalar(ftn, T, z, arrs, irange, J2s, K)
end
return r
else
return ftn(arrs..., irange, jrange, K)::RT
end
end
function threader_scalar(ftn::F, ::Type{T}, z::RT, arrs::Tuple, irange, jrange, K; maxL=tile_maxiter(T)) where {F <: Function, T, RT}
ilen, jlen = length(irange), length(jrange)
maxL = tile_maxiter(T)
r = Threads.Atomic{RT}(z)
if ilen > maxL || jlen > maxL
if ilen > jlen
I1s, I2s = cleave(irange)
task1 = Threads.@spawn begin
# println("threader: $irange $J1 on $(threadid())")
r1 = threader_scalar(ftn, T, z, arrs, I1s, jrange, K)
Threads.atomic_add!(r, r1)
end
task2 = Threads.@spawn begin
r2 = threader_scalar(ftn, T, z, arrs, I2s, jrange, K)
Threads.atomic_add!(r, r2)
end
wait(task1)
wait(task2)
else
J1s, J2s = cleave(jrange)
task1 = Threads.@spawn begin
r1 = threader_scalar(ftn, T, z, arrs, irange, J1s, K)
Threads.atomic_add!(r, r1)
end
task2 = Threads.@spawn begin
r2 = threader_scalar(ftn, T, z, arrs, irange, J2s, K)
Threads.atomic_add!(r, r2)
end
wait(task1)
wait(task2)
end
return r[]
else
return ftn(arrs..., irange, jrange, K)::RT
end
end
"""
tiler_scalar_1d(ftn, ::Type{T}, z::RT, arrs, irange, jrange, K;
maxL=tile_maxiter(T))
Apply a reduction `ftn` over the index space `irange` x `jrange` of `arrs` with a single thread.
It splits the index space only in j dimensions.
# Input
- `ftn`: The function to be applied
- `T`: element type of updated `arrs`
- `z`: the "zero" value for the return.
- `arrs`: a tuple of input arrays
- `irange`: an index range
- `jrange`: another index range
- `K`: number of clusters
- `maxL`: maximum length of tile.
"""
function tiler_scalar_1d(ftn::F, ::Type{T}, z::RT, arrs::Tuple, irange, jrange, K; maxL=tile_maxiter(T)) where {F <: Function, T, RT}
ilen, jlen = length(irange), length(jrange)
maxL = tile_maxiter(T)
r = z
if jlen > maxL
J1s, J2s = cleave(jrange)
r += tiler_scalar_1d(ftn, T, z, arrs, irange, J1s, K)
r += tiler_scalar_1d(ftn, T, z, arrs, irange, J2s, K)
return r
else
return ftn(arrs..., irange, jrange, K)::RT
end
end
"""
qp_block!(qp_small, q, p, irange, jrange, K)
Compute a block of the matrix Q x P.
# Input
- `qp_small`: the output.
- `q`: Q matrix.
- `p`: P matrix.
- `irange`: i index range over which this function is applied
- `jrange`: j index range over which this function is applied
- `K`: number of clusters
"""
@inline function qp_block!(qp_small::AbstractArray{T}, q, p, irange, jrange, K) where T
tid = threadid()
fill!(view(qp_small, :, :, tid), zero(T))
firsti, firstj = first(irange), first(jrange)
@turbo for j in jrange
for i in irange
for k in 1:K
qp_small[i-firsti+1, j-firstj+1, tid] += q[k, i] * p[k, j]
end
end
end
end
"""
loglikelihood_loop(g, qp, irange, jrange, K)
Compute loglikelihood using `qp`. Always returns the double precision result.
# Input
- `g`: genotype matrix.
- `qp`: QP matrix.
- `irange`: i index range over which this function is applied
- `jrange`: j index range over which this function is applied
- `K`: number of clusters
"""
@inline function loglikelihood_loop(g::AbstractArray{T}, qp, irange, jrange, K) where T
oneT = one(T)
twoT = 2one(T)
r = zero(Float64)
@turbo for j in jrange
for i in irange
gij = g[i, j]
r += (gij * log(qp[i, j]) + (twoT - gij) * log(oneT - qp[i, j]))
end
end
r
end
"""
loglikelihood_loop_skipmissing(g, qp, irange, jrange, K)
Compute loglikelihood using `qp`. Always returns the double precision result.
# Input
- `g`: genotype matrix.
- `qp`: QP matrix.
- `irange`: i index range over which this function is applied
- `jrange`: j index range over which this function is applied
- `K`: number of clusters
"""
@inline function loglikelihood_loop_skipmissing(g::AbstractArray{T}, qp, irange, jrange, K) where T
oneT = one(T)
twoT = 2one(T)
r = zero(Float64)
@turbo for j in jrange
for i in irange
gij = g[i, j]
r += gij != gij ? zero(T) : (gij * log(qp[i, j]) + (twoT - gij) * log(oneT - qp[i, j]))
end
end
# @tullio r = g[i, j] * log(qf[i, j]) + (2 - g[i, j]) * log(1 - qf[i, j])
r
end
@inline function loglikelihood_loop(g::SnpLinAlg{T}, qp, irange, jrange, K) where T
oneT = one(T)
twoT = 2one(T)
rslt = zero(Float64)
gmat = g.s.data
g_map = T == Float64 ? g_map_Float64 : g_map_Float32
@turbo for j in jrange
for i in irange
blk = gmat[(i - 1) >> 2 + 1, j]
r = (i - 1) % 4
blk_shifted = blk >> (r << 1)
gij_pre = blk_shifted & 0x03
gij = g_map[gij_pre + 0x01] + (gij_pre == 0x01) * g.μ[j]
rslt += (gij * log(qp[i, j]) + (twoT - gij) * log(oneT - qp[i, j]))
end
end
# @tullio r = g[i, j] * log(qf[i, j]) + (2 - g[i, j]) * log(1 - qf[i, j])
rslt
end
@inline function loglikelihood_loop_skipmissing(g::SnpLinAlg{T}, qp, irange, jrange, K) where T
oneT = one(T)
twoT = 2one(T)
rslt = zero(Float64)
gmat = g.s.data
g_map = T == Float64 ? g_map_Float64 : g_map_Float32
nonmissing_map = T == Float64 ? nonmissing_map_Float64 : nonmissing_map_Float32
@turbo for j in jrange
for i in irange
blk = gmat[(i - 1) >> 2 + 1, j]
r = (i - 1) % 4
blk_shifted = blk >> (r << 1)
gij_pre = blk_shifted & 0x03
gij = g_map[gij_pre + 0x01]
nonmissing = nonmissing_map[gij_pre + 0x01]
rslt += nonmissing ? (gij * log(qf[i, j]) + (twoT - gij) * log(oneT - qf[i, j])) : zero(T)
end
end
# @tullio r = g[i, j] * log(qf[i, j]) + (2 - g[i, j]) * log(1 - qf[i, j])
rslt
end
"""
loglikelihood_loop(g, q, p, qp_small, irange, jrange, K)
Compute loglikelihood using `q` and `p`, compute local `qp` on-the-fly.
Always returns the double precision result.
# Input
- `g`: genotype matrix.
- `q`: Q matrix.
- `p`: P matrix.
- `qp_small`: dummy.
- `irange`: i index range over which this function is applied
- `jrange`: j index range over which this function is applied
- `K`: number of clusters
"""
@inline function loglikelihood_loop(g::AbstractArray{T}, q, p, qp_small::AbstractArray{T}, irange, jrange, K) where T
oneT = one(T)
twoT = 2one(T)
firsti, firstj = first(irange), first(jrange)
r = zero(Float64)
@turbo for j in jrange
for i in irange
gij = g[i, j]
qp_local = zero(T)
for k in 1:K
qp_local += q[k, i] * p[k, j]
end
r += gij * log(qp_local)
end
end
@turbo for j in jrange
for i in irange
gij = g[i, j]
qp_local = zero(T)
for k in 1:K
qp_local += q[k, i] * p[k, j]
end
r += (twoT - gij) * log(oneT - qp_local)
end
end
r
end
"""
loglikelihood_loop_skipmissing(g, q, p, qp_small, irange, jrange, K)
Compute loglikelihood using `q` and `p`, compute local `qp` on-the-fly.
Always returns the double precision result.
# Input
- `g`: genotype matrix.
- `q`: Q matrix.
- `p`: P matrix.
- `qp_small`: dummy.
- `irange`: i index range over which this function is applied
- `jrange`: j index range over which this function is applied
- `K`: number of clusters
"""
@inline function loglikelihood_loop_skipmissing(g::AbstractArray{T}, q, p, qp_small::AbstractArray{T}, irange, jrange, K) where T
oneT = one(T)
twoT = 2one(T)
# firsti, firstj = first(irange), first(jrange)
r = zero(Float64)
@turbo for j in jrange
for i in irange
gij = g[i, j]
nonmissing = (gij == gij)
qp_local = zero(T)
for k in 1:K
qp_local += q[k, i] * p[k, j]
end
r += nonmissing ? gij * log(qp_local) : zero(T)
end
end
@turbo for j in jrange
for i in irange
gij = g[i, j]
nonmissing = (gij == gij)
qp_small = zero(T)
for k in 1:K
qp_small += q[k, i] * p[k, j]
end
r += nonmissing ? (twoT - gij) * log(oneT - qp_small) : zero(T)
end
end
r
end
@inline function loglikelihood_loop(g::SnpLinAlg{T}, q, p, qp_small::AbstractArray{T}, irange, jrange, K) where T
oneT = one(T)
twoT = 2one(T)
firsti, firstj = first(irange), first(jrange)
tid = threadid()
qp_block!(qp_small, q, p, irange, jrange, K)
r = zero(Float64)
gmat = g.s.data
g_map = T == Float64 ? g_map_Float64 : g_map_Float32
@turbo for j in jrange
for i in irange
blk = gmat[(i - 1) >> 2 + 1, j]
re = (i - 1) % 4
blk_shifted = blk >> (re << 1)
gij_pre = blk_shifted & 0x03
gij = g_map[gij_pre + 0x01] + (gij_pre == 0x01) * g.μ[j]
r += (gij * log(qp_small[i-firsti+1, j-firstj+1, tid])) + ((twoT - gij) * log(oneT - qp_small[i-firsti+1, j-firstj+1, tid]))
end
end
r
end
@inline function loglikelihood_loop_skipmissing(g::SnpLinAlg{T}, q, p, qp_small::AbstractArray{T}, irange, jrange, K) where T
oneT = one(T)
twoT = 2one(T)
firsti, firstj = first(irange), first(jrange)
tid = threadid()
qp_block!(qp_small, q, p, irange, jrange, K)
r = zero(Float64)
gmat = g.s.data
g_map = T == Float64 ? g_map_Float64 : g_map_Float32
nonmissing_map = T == Float64 ? nonmissing_map_Float64 : nonmissing_map_Float32
@turbo for j in jrange
for i in irange
blk = gmat[(i - 1) >> 2 + 1, j]
re = (i - 1) % 4
blk_shifted = blk >> (re << 1)
gij_pre = blk_shifted & 0x03
gij = g_map[gij_pre + 0x01]
nonmissing = nonmissing_map[gij_pre + 0x01]
r += nonmissing * ((gij * log(qp_small[i-firsti+1, j-firstj+1, tid])) + ((twoT - gij) * log(oneT - qp_small[i-firsti+1, j-firstj+1, tid])))
end
end
r
end
@inline function em_q_loop!(q_next, g::AbstractArray{T}, q, p, qp_small::AbstractArray{T},
irange, jrange, K) where T
firsti, firstj = first(irange), first(jrange)
tid = threadid()
qp_block!(qp_small, q, p, irange, jrange, K)
@turbo for j in jrange
for i in irange
for k in 1:K
gij = g[i, j]
q_next[k, i] += (gij * q[k, i] * p[k, j] / qp_small[i-firsti+1, j-firstj+1, tid] +
(2 - gij) * q[k, i] * (1 - p[k, j]) / (1 - qp_small[i-firsti+1, j-firstj+1, tid]))
end
end
end
end
"""
em_q_loop_skipmissing!(q_next, g, q, p, qp_small, irange, jrange, K)
Compute EM update for `q`, compute local `qp` on-the-fly.
# Input
- `q_next`: result to be updated inplace.
- `g`: genotype matrix.
- `q`: Q matrix.
- `p`: P matrix.
- `qp_small`: dummy.
- `irange`: i index range over which this function is applied
- `jrange`: j index range over which this function is applied
- `K`: number of clusters
"""
@inline function em_q_loop_skipmissing!(q_next, g::AbstractArray{T}, q, p, qp_small::AbstractArray{T},
irange, jrange, K) where T
firsti, firstj = first(irange), first(jrange)
tid = threadid()
qp_block!(qp_small, q, p, irange, jrange, K)
@turbo for j in jrange
for i in irange
for k in 1:K
gij = g[i, j]
nonmissing = (gij == gij)
tmp = (gij * q[k, i] * p[k, j] / qp_small[i-firsti+1, j-firstj+1, tid] +
(2 - gij) * q[k, i] * (1 - p[k, j]) / (1 - qp_small[i-firsti+1, j-firstj+1, tid]))
q_next[k, i] += nonmissing ? tmp : zero(T)
end
end
end
end
@inline function em_q_loop!(q_next, g::SnpLinAlg{T}, q, p, qp_small, irange, jrange, K) where T
firsti, firstj = first(irange), first(jrange)
tid = threadid()
qp_block!(qp_small, q, p, irange, jrange, K)
gmat = g.s.data
g_map = T == Float64 ? g_map_Float64 : g_map_Float32
@turbo for j in jrange
for i in irange
for k in 1:K
blk = gmat[(i - 1) >> 2 + 1, j]
re = (i - 1) % 4
blk_shifted = blk >> (re << 1)
gij_pre = blk_shifted & 0x03
gij = g_map[gij_pre + 0x01] + (gij_pre == 0x01) * g.μ[j]
q_next[k, i] += (gij * q[k, i] * p[k, j] / qp_small[i-firsti+1, j-firstj+1, tid] +
(2 - gij) * q[k, i] * (1 - p[k, j]) / (1 - qp_small[i-firsti+1, j-firstj+1, tid]))
end
end
end
end
@inline function em_q_loop_skipmissing!(q_next, g::SnpLinAlg{T}, q, p, qp_small::AbstractArray{T}, irange, jrange, K) where T
firsti, firstj = first(irange), first(jrange)
tid = threadid()
qp_block!(qp_small, q, p, irange, jrange, K)
gmat = g.s.data
g_map = T == Float64 ? g_map_Float64 : g_map_Float32
nonmissing_map = T == Float64 ? nonmissing_map_Float64 : nonmissing_map_Float32
@turbo for j in jrange
for i in irange
for k in 1:K
blk = gmat[(i - 1) >> 2 + 1, j]
re = (i - 1) % 4
blk_shifted = blk >> (re << 1)
gij_pre = blk_shifted & 0x03
gij = g_map[gij_pre + 0x01]
nonmissing = nonmissing_map[gij_pre + 0x01]
tmp = (gij * q[k, i] * p[k, j] / qp_small[i-firsti+1, j-firstj+1, tid] +
(2 - gij) * q[k, i] * (1 - p[k, j]) / (1 - qp_small[i-firsti+1, j-firstj+1, tid]))
q_next[k, i] += nonmissing * tmp
end
end
end
end
@inline function em_p_loop!(p_next, g::AbstractArray{T}, q, p, p_tmp, qp_small::AbstractArray{T}, irange, jrange, K) where T
firsti, firstj = first(irange), first(jrange)
tid = threadid()
qp_block!(qp_small, q, p, irange, jrange, K)
@turbo for j in jrange
for i in irange
gij = g[i, j]
for k in 1:K
p_tmp[k, j] += gij * q[k, i] * p[k, j] / qp_small[i-firsti+1, j-firstj+1, tid]
p_next[k, j] += (2 - gij) * q[k, i] * (one(T) - p[k, j]) / (one(T) - qp_small[i-firsti+1, j-firstj+1, tid])
end
end
end
end
"""
em_p_loop_skipmissing!(p_next, g, q, p, qp_small, irange, jrange, K)
Compute EM update for `p`, compute local `qp` on-the-fly.
# Input
- `p_next`: result to be updated inplace
- `g`: genotype matrix.
- `q`: Q matrix.
- `p`: P matrix.
- `qp_small`: dummy.
- `irange`: i index range over which this function is applied
- `jrange`: j index range over which this function is applied
- `K`: number of clusters
"""
@inline function em_p_loop_skipmissing!(p_next, g::AbstractArray{T}, q, p, p_tmp, qp_small::AbstractArray{T}, irange, jrange, K) where T
firsti, firstj = first(irange), first(jrange)
tid = threadid()
qp_block!(qp_small, q, p, irange, jrange, K)
@turbo for j in jrange
for i in irange
gij = g[i, j]
nonmissing = (gij == gij)
for k in 1:K
p_tmp[k, j] += nonmissing ? (gij * q[k, i] * p[k, j] / qp_small[i-firsti+1, j-firstj+1, tid]) : zero(T)
p_next[k, j] += nonmissing ? ((2 - gij) * q[k, i] * (one(T) - p[k, j]) / (one(T) - qp_small[i-firsti+1, j-firstj+1, tid])) : zero(T)
end
end
end
end
@inline function em_p_loop!(p_next, g::SnpLinAlg{T}, q, p, p_tmp, qp_small::AbstractArray{T}, irange, jrange, K) where T
firsti, firstj = first(irange), first(jrange)
tid = threadid()
qp_block!(qp_small, q, p, irange, jrange, K)
gmat = g.s.data
g_map = T == Float64 ? g_map_Float64 : g_map_Float32
@turbo for j in jrange
for i in irange
blk = gmat[(i - 1) >> 2 + 1, j]
re = (i - 1) % 4
blk_shifted = blk >> (re << 1)
gij_pre = blk_shifted & 0x03
gij = g_map[gij_pre + 0x01] + (gij_pre == 0x01) * g.μ[j]
for k in 1:K
p_tmp[k, j] += gij * q[k, i] * p[k, j] / qp_small[i-firsti+1, j-firstj+1, tid]
p_next[k, j] += (2 - gij) * q[k, i] * (one(T) - p[k, j]) / (one(T) - qp_small[i-firsti+1, j-firstj+1, tid])
end
end
end
end
@inline function em_p_loop_skipmissing!(f_next, g::SnpLinAlg{T}, q, p, p_tmp, qp_small::AbstractArray{T}, irange, jrange, K) where T
firsti, firstj = first(irange), first(jrange)
tid = threadid()
qp_block!(qp_small, q, p, irange, jrange, K)
gmat = g.s.data
g_map = T == Float64 ? g_map_Float64 : g_map_Float32
nonmissing_map = T == Float64 ? nonmissing_map_Float64 : nonmissing_map_Float32
@turbo for j in jrange
for i in irange
blk = gmat[(i - 1) >> 2 + 1, j]
re = (i - 1) % 4
blk_shifted = blk >> (re << 1)
gij_pre = blk_shifted & 0x03
gij = g_map[gij_pre + 0x01]
nonmissing = nonmissing_map[gij_pre + 0x01]
for k in 1:K
p_tmp[k, j] += nonmissing * (gij * q[k, i] * p[k, j] / qp_small[i-firsti+1, j-firstj+1, tid])
f_next[k, j] += nonmissing * ((2 - gij) * q[k, i] * (one(T) - p[k, j]) / (one(T) - qp_small[i-firsti+1, j-firstj+1, tid]))
end
end
end
end
@inline function update_q_loop!(XtX, Xtz, g::AbstractArray{T}, q, p, qp_small::AbstractArray{T}, irange, jrange, K) where T
oneT = one(T)
twoT = 2one(T)
firsti, firstj = first(irange), first(jrange)
tid = threadid()
qp_block!(qp_small, q, p, irange, jrange, K)
@turbo for j in jrange
for i in irange
gij = g[i, j]
for k in 1:K
Xtz[k, i] += gij * p[k, j] / qp_small[i-firsti+1, j-firstj+1, tid] +
(twoT - gij) * (oneT - p[k, j]) / (oneT - qp_small[i-firsti+1, j-firstj+1, tid])
for k2 in 1:K
XtX[k2, k, i] += gij / (qp_small[i-firsti+1, j-firstj+1, tid]) ^ 2 * p[k, j] * p[k2, j] +
(twoT - gij) / (oneT - qp_small[i-firsti+1, j-firstj+1, tid]) ^ 2 * (oneT - p[k, j]) * (oneT - p[k2, j])
end
end
end
end
end
"""
update_q_loop_skipmissing!(XtX, Xtz, g, q, p, qp_small, irange, jrange, K)
Compute gradient and hessian of loglikelihood w.r.t. `q`, compute local `qp` on-the-fly.
# Arguments
- `XtX`: Hessian of loglikelihood computed inplace
- `Xtz`: Gradient of loglikelihood computed inplace
- `g`: genotype matrix.
- `q`: Q matrix.
- `p`: P matrix.
- `qp_small`: dummy.
- `irange`: i index range over which this function is applied
- `jrange`: j index range over which this function is applied
- `K`: number of clusters
"""
@inline function update_q_loop_skipmissing!(XtX, Xtz, g::AbstractArray{T}, q, p, qp_small::AbstractArray{T}, irange, jrange, K) where T
oneT = one(T)
twoT = 2one(T)
firsti, firstj = first(irange), first(jrange)
tid = threadid()
qp_block!(qp_small, q, p, irange, jrange, K)
@turbo for j in jrange
for i in irange
gij = g[i, j]
nonmissing = (gij == gij)
for k in 1:K
Xtz[k, i] += nonmissing ? (gij * p[k, j] / qp_small[i-firsti+1, j-firstj+1, tid] +
(twoT - gij) * (oneT - p[k, j]) / (oneT - qp_small[i-firsti+1, j-firstj+1, tid])) : zero(T)
for k2 in 1:K
XtX[k2, k, i] += nonmissing ? (gij / (qp_small[i-firsti+1, j-firstj+1, tid]) ^ 2 * p[k, j] * p[k2, j] +
(twoT - gij) / (oneT - qp_small[i-firsti+1, j-firstj+1, tid]) ^ 2 * (oneT - p[k, j]) * (oneT - p[k2, j])) : zero(T)
end
end
end
end
end
@inline function update_p_loop!(XtX, Xtz, g::AbstractArray{T}, q, p, qp_small::AbstractArray{T}, irange, jrange, K) where T
oneT = one(T)
twoT = 2one(T)
firsti, firstj = first(irange), first(jrange)
tid = threadid()
qp_block!(qp_small, q, p, irange, jrange, K)
@turbo for j in jrange
for i in irange
gij = g[i, j]
for k in 1:K
Xtz[k, j] += gij * q[k, i] / qp_small[i-firsti+1, j-firstj+1, tid] -
(twoT - gij) * q[k, i] / (oneT - qp_small[i-firsti+1, j-firstj+1, tid])
for k2 in 1:K
XtX[k2, k, j] += gij / (qp_small[i-firsti+1, j-firstj+1, tid]) ^ 2 * q[k, i] * q[k2, i] +
(twoT - gij) / (oneT - qp_small[i-firsti+1, j-firstj+1, tid]) ^ 2 * q[k, i] * q[k2, i]
end
end
end
end
end
"""
update_p_loop_skipmissing!(XtX, Xtz, g, q, p, qp_small, irange, jrange, K)
Compute gradient and hessian of loglikelihood w.r.t. `p`, compute local `qp` on-the-fly.
# Arguments
- `XtX`: Hessian of loglikelihood computed inplace
- `Xtz`: Gradient of loglikelihood computed inplace
- `g`: genotype matrix.
- `q`: Q matrix.
- `p`: P matrix.
- `qp_small`: dummy.
- `irange`: i index range over which this function is applied
- `jrange`: j index range over which this function is applied
- `K`: number of clusters
"""
@inline function update_p_loop_skipmissing!(XtX, Xtz, g::AbstractArray{T}, q, p, qp_small::AbstractArray{T}, irange, jrange, K) where T
oneT = one(T)
twoT = 2one(T)
firsti, firstj = first(irange), first(jrange)
tid = threadid()
qp_block!(qp_small, q, p, irange, jrange, K)
@turbo for j in jrange
for i in irange
gij = g[i, j]
nonmissing = (gij == gij)
for k in 1:K
Xtz[k, j] += nonmissing ? (gij * q[k, i] / qp_small[i-firsti+1, j-firstj+1, tid] -
(twoT - gij) * q[k, i] / (oneT - qp_small[i-firsti+1, j-firstj+1, tid])) : zero(T)
for k2 in 1:K
XtX[k2, k, j] += nonmissing ? (gij / (qp_small[i-firsti+1, j-firstj+1, tid]) ^ 2 * q[k, i] * q[k2, i] +
(twoT - gij) / (oneT - qp_small[i-firsti+1, j-firstj+1, tid]) ^ 2 * q[k, i] * q[k2, i]) : zero(T)
end
end
end
end
end
@inline function update_q_loop!(XtX, Xtz, g::SnpLinAlg{T}, q, p, qp_small::AbstractArray{T}, irange, jrange, K) where T
oneT = one(T)
twoT = 2one(T)
gmat = g.s.data
firsti, firstj = first(irange), first(jrange)
tid = threadid()
qp_block!(qp_small, q, p, irange, jrange, K)
g_map = T == Float64 ? g_map_Float64 : g_map_Float32
@turbo for j in jrange
for i in irange
blk = gmat[(i - 1) >> 2 + 1, j]
re = (i - 1) % 4
blk_shifted = blk >> (re << 1)
gij_pre = blk_shifted & 0x03
gij = g_map[gij_pre + 0x01] + (gij_pre == 0x01) * g.μ[j]
for k in 1:K
Xtz[k, i] += (gij * p[k, j] / qp_small[i-firsti+1, j-firstj+1, tid] +
(twoT - gij) * (oneT - p[k, j]) / (oneT - qp_small[i-firsti+1, j-firstj+1, tid]))
for k2 in 1:K
XtX[k2, k, i] += (gij / (qp_small[i-firsti+1, j-firstj+1, tid]) ^ 2 * p[k, j] * p[k2, j] +
(twoT - gij) / (oneT - qp_small[i-firsti+1, j-firstj+1, tid]) ^ 2 * (oneT - p[k, j]) * (oneT - p[k2, j]))
end
end
end
end
end
@inline function update_q_loop_skipmissing!(XtX, Xtz, g::SnpLinAlg{T}, q, p, qp_small::AbstractArray{T}, irange, jrange, K) where T
oneT = one(T)
twoT = 2one(T)
gmat = g.s.data
firsti, firstj = first(irange), first(jrange)
tid = threadid()
qp_block!(qp_small, q, p, irange, jrange, K)
g_map = T == Float64 ? g_map_Float64 : g_map_Float32
nonmissing_map = T == Float64 ? nonmissing_map_Float64 : nonmissing_map_Float32
@turbo for j in jrange
for i in irange
blk = gmat[(i - 1) >> 2 + 1, j]
re = (i - 1) % 4
blk_shifted = blk >> (re << 1)
gij_pre = blk_shifted & 0x03
gij = g_map[gij_pre + 0x01]
nonmissing = nonmissing_map[gij_pre + 0x01]
for k in 1:K
Xtz[k, i] += nonmissing * (gij * p[k, j] / qp_small[i-firsti+1, j-firstj+1, tid] +
(twoT - gij) * (oneT - p[k, j]) / (oneT - qp_small[i-firsti+1, j-firstj+1, tid]))
for k2 in 1:K
XtX[k2, k, i] += nonmissing * (gij / (qp_small[i-firsti+1, j-firstj+1, tid]) ^ 2 * p[k, j] * p[k2, j] +
(twoT - gij) / (oneT - qp_small[i-firsti+1, j-firstj+1, tid]) ^ 2 * (oneT - p[k, j]) * (oneT - p[k2, j]))
end
end
end
end
end
function update_p_loop!(XtX, Xtz, g::SnpLinAlg{T}, q, p, qp_small::AbstractArray{T}, irange, jrange, K) where T
oneT = one(T)
twoT = 2one(T)
gmat = g.s.data
firsti, firstj = first(irange), first(jrange)
tid = threadid()
qp_block!(qp_small, q, p, irange, jrange, K)
g_map = T == Float64 ? g_map_Float64 : g_map_Float32
@turbo for j in jrange
for i in irange
blk = gmat[(i - 1) >> 2 + 1, j]
re = (i - 1) % 4
blk_shifted = blk >> (re << 1)
gij_pre = blk_shifted & 0x03
gij = g_map[gij_pre + 0x01] + (gij_pre == 0x01) * g.μ[j]
for k in 1:K
Xtz[k, j] += (gij * q[k, i] / qp_small[i-firsti+1, j-firstj+1, tid] -
(twoT - gij) * q[k, i] / (oneT - qp_small[i-firsti+1, j-firstj+1, tid]))
for k2 in 1:K
XtX[k2, k, j] += (gij / (qp_small[i-firsti+1, j-firstj+1, tid]) ^ 2 * q[k, i] * q[k2, i] +
(twoT - gij) / (oneT - qp_small[i-firsti+1, j-firstj+1, tid]) ^ 2 * q[k, i] * q[k2, i])
end
end
end
end
end
function update_p_loop_skipmissing!(XtX, Xtz, g::SnpLinAlg{T}, q, p, qp_small::AbstractArray{T}, irange, jrange, K) where T
oneT = one(T)
twoT = 2one(T)
gmat = g.s.data
firsti, firstj = first(irange), first(jrange)
tid = threadid()
qp_block!(qp_small, q, p, irange, jrange, K)
g_map = T == Float64 ? g_map_Float64 : g_map_Float32
nonmissing_map = T == Float64 ? nonmissing_map_Float64 : nonmissing_map_Float32
@turbo for j in jrange
for i in irange
blk = gmat[(i - 1) >> 2 + 1, j]
re = (i - 1) % 4
blk_shifted = blk >> (re << 1)
gij_pre = blk_shifted & 0x03
gij = g_map[gij_pre + 0x01]
nonmissing = nonmissing_map[gij_pre + 0x01]
for k in 1:K
Xtz[k, j] += nonmissing * (gij * q[k, i] / qp_small[i-firsti+1, j-firstj+1, tid] -
(twoT - gij) * q[k, i] / (oneT - qp_small[i-firsti+1, j-firstj+1, tid]))
for k2 in 1:K
XtX[k2, k, j] += nonmissing * (gij / (qp_small[i-firsti+1, j-firstj+1, tid]) ^ 2 * q[k, i] * q[k2, i] +
(twoT - gij) / (oneT - qp_small[i-firsti+1, j-firstj+1, tid]) ^ 2 * q[k, i] * q[k2, i])
end
end
end
end
end
| OpenADMIXTURE | https://github.com/OpenMendel/OpenADMIXTURE.jl.git |
|
[
"MIT"
] | 0.1.0 | 6eba29d96bef815c6fda407240c0c4ba358f0f6f | code | 1848 | function project_q!(b::AbstractMatrix{T}, idx::AbstractVector{Int}; pseudocount=T(1e-5)) where T
I = size(b, 2)
@inbounds for i in 1:I
project_q!(@view(b[:, i]), idx; pseudocount=pseudocount)
end
b
end
function qsortperm!(idx, a,lo,hi)
i, j = lo, hi
while i < hi
pivot = idx[(lo+hi) ÷ 2]
while i <= j
while a[idx[i]] > a[pivot]; i = i+1; end
while a[idx[j]] < a[pivot]; j = j-1; end
if i <= j
idx[i], idx[j] = idx[j], idx[i]
i, j = i+1, j-1
end
end
if lo < j; qsortperm!(idx,a,lo,j); end
lo, j = i, hi
end
return idx
end
"""
project_q!(b, idx; pseudocount=1e-5)
Project the Q matrix onto the probability simplex.
"""
function project_q!(b::AbstractVector{T}, idx::AbstractVector{Int}; pseudocount=T(1e-5)) where T
n = length(b)
τ = one(T) - n * pseudocount
b .-= pseudocount
bget = false
@assert length(b) == length(idx)
@inbounds for i in 1:length(idx)
idx[i] = i
end
qsortperm!(idx, b, 1, length(idx))
# sortperm!(idx, b, rev=true) # this allocates something
tsum = zero(T)
@inbounds for i = 1:n-1
tsum += b[idx[i]]
tmax = (tsum - τ)/i
if tmax ≥ b[idx[i+1]]
bget = true
break
end
end
if !bget
tmax = (tsum + b[idx[n]] - τ) / n
end
@inbounds for i = 1:n
b[i] = max(b[i] - tmax, 0)
end
b.+= pseudocount
end
"""
project_p!(b; pseudocount=1e-5)
Project the P matrix onto [0, 1].
"""
function project_p!(b::AbstractArray{T}; pseudocount=T(1e-5)) where T
@turbo for i in 1:length(b)
b[i] = min(max(b[i], pseudocount), one(T) - pseudocount)
end
# b .= min.(max.(b, pseudocount), one(T) - pseudocount)
end
| OpenADMIXTURE | https://github.com/OpenMendel/OpenADMIXTURE.jl.git |
|
[
"MIT"
] | 0.1.0 | 6eba29d96bef815c6fda407240c0c4ba358f0f6f | code | 10432 | # This file is originally written by Dr. Kenneth Lange.
# Modified by Dr. Seyoon Ko for performance improvement.
"""
Calculates the tableau used in minimizing the quadratic
0.5 x' Q x + r' x, subject to Ax = b and parameter lower
and upper bounds.
"""
function create_tableau!(tableau::AbstractMatrix{T},
matrix_q::AbstractMatrix{T}, r::AbstractVector{T}, x::AbstractVector{T},
v::AbstractMatrix{T}, tmp_k::AbstractVector{T},
simplex::Bool, p_double::Bool=false;
tmp_4k_k::Union{Nothing, AbstractMatrix{T}}=nothing,
tmp_4k_k_2::Union{Nothing, AbstractMatrix{T}}=nothing,
verbose=false) where T
#matrix_a::AbstractMatrix{T}, b::AbstractVector{T}, x::AbstractVector{T}) where T
@assert !(simplex && p_double) "Only one of simplex and p_double can be true."
fill!(tableau, zero(T))
K = size(matrix_q, 1)
if p_double
K ÷= 4
end
if simplex
@assert size(tableau) == (K + 2, K + 2)
elseif p_double
@assert size(tableau) == (5K + 1, 5K + 1)
else
@assert size(tableau) == (K + 1, K + 1)
end
sz = size(tableau, 1)
#
# Create the tableau in the absence of constraints.
#
@turbo for j in 1:(p_double ? 4K : K)
for i in 1:(p_double ? 4K : K)
tableau[i, j] = matrix_q[i, j]
end
end
@turbo for i in 1:(p_double ? 4K : K)
tableau[sz, i] = -r[i]
tableau[i, sz] = -r[i]
end
tableau[sz, sz] = zero(T)
if !simplex && !p_double
return tableau
#tableau = [matrix_q (-r); -r' 0]
elseif simplex
#
# In the presence of constraints compute a constant mu via
# the Gerschgorin circle theorem so that Q + mu * A' * A
# is positive definite.
#
# (matrix_u, d, matrix_v) = svd(matrix_a, full = true)
# matrix_p = v * matrix_q * v'
mul!(tmp_k, matrix_q, @view(v[:, 1]))
mul!(tmp_k, v, tmp_k)
mu = (norm(tmp_k, 1) - 2tmp_k[1]) / K
mu = T(2mu)
#
# Now create the tableau.
#
# add mu * A' * A
@turbo for j in 1:K
for i in 1:K
tableau[i, j] += mu
end
end
# A
@inbounds for i in 1:K
tableau[K+1, i] = one(T)
tableau[i, K+1] = one(T)
end
# b - A * x
tableau[K+1, K+1] = zero(T)
tableau[K+2, K+1] = tableau[K+1, K+2] = one(T) - sum(x)
tableau[K+2, K+2] = zero(T)
else # p_double
# matrix_q: 4K x 4K.
# compute mu
#
# (matrix_u, d, matrix_v) = svd(matrix_a, full = true) (precomputed)
# matrix_p = v * matrix_q * v'
mul!(tmp_4k_k, matrix_q, transpose(@view(v[1:K, :])))
mul!(tmp_4k_k_2, v, tmp_4k_k)
mu = zero(T)
for i = 1:K
mu = max((norm(@view(tmp_4k_k_2[:, i]), 1) - 2.0 * tmp_4k_k_2[i, i]) / 4, mu)
end
mu = T(2.5 * mu)
if verbose
println("mu: $mu")
end
# fill the rest
# add mu * A' * A
for l2 in 1:4
for l in 1:4
for k in 1:K
tableau[(l-1) * K + k, (l2-1) * K + k] += mu
end
end
end
# A
for l in 1:4
for k in 1:K
tableau[4K + k, (l-1) * K + k] += 1
tableau[(l-1) * K + k, 4K + k] += 1
end
end
# b - A * x
tableau[4K+1:5K, 5K+1] .= one(T)
for l in 1:4
for k in 1:K
tableau[4K+k, 5K+1] -= x[(l-1)*K + k]
end
end
tableau[4K+1:5K, 5K+1] .= max.(zero(T), @view(tableau[4K+1:5K, 5K+1]))
for k in 1:K
tableau[5K+1, 4K+k] = tableau[4K+k, 5K+1]
end
end
if verbose
println(tableau)
end
return tableau
end # function create_tableau!
# function create_tableau(matrix_q::AbstractMatrix{T}, r::AbstractVector{T},
# matrix_a::AbstractMatrix{T}, b::AbstractVector{T}, x::AbstractVector{T}) where T
# m = size(matrix_a, 1)
# #
# # Create the tableau in the absence of constraints.
# #
# if m == 0
# tableau = [matrix_q (-r); -r' 0]
# else
# #
# # In the presence of constraints compute a constant mu via
# # the Gerschgorin circle theorem so that Q + mu * A' * A
# # is positive definite.
# #
# (matrix_u, d, matrix_v) = svd(matrix_a, full = true)
# matrix_p = matrix_v * matrix_q * matrix_v'
# mu = 0.0
# for i = 1:m
# mu = max((norm(matrix_p[:, i], 1) - 2matrix_p[i, i]) / d[i]^2, mu)
# end
# mu = T(2mu)
# #
# # Now create the tableau.
# #
# tableau = [matrix_q + mu * matrix_a' * matrix_a matrix_a' (-r);
# matrix_a zeros(T, m, m) b - matrix_a * x;
# -r' (b - matrix_a * x)' 0]
# end
# return tableau
# end # function create_tableau
"""
Solves the p-dimensional quadratic programming problem
min [df * delta + 0.5 * delta' * d^2 f * delta]
subject to: constraint * delta = 0 and pmin <= par + delta <= pmax.
See: Jennrich JI, Sampson PF (1978) "Some problems faced in making
a variance component algorithm into a general mixed model program."
Proceedings of the Eleventh Annual Symposium on the Interface.
Gallant AR, Gerig TM, editors. Institute of Statistics,
North Carolina State University.
"""
function quadratic_program!(delta::AbstractVector{T}, tableau::AbstractMatrix{T}, par::AbstractVector{T},
pmin::AbstractVector{T}, pmax::AbstractVector{T}, p::Int, c::Int, d::AbstractVector{T},
tmp::AbstractVector{T}, swept::AbstractVector{Bool}; verbose=false) where T
# delta = zeros(T, size(par))
fill!(delta, zero(T))
#
# See function create_tableau for the construction of the tableau.
# For checking tolerance, set diag to the diagonal elements of tableau.
# Begin by sweeping on those diagonal elements of tableau corresponding
# to the parameters. Then sweep on the diagonal elements corresponding
# to the constraints. If any parameter fails the tolerance test, then
# return and reset the approximate Hessian.
#
small = T(1e-5)
tol = T(1e-8)
# d = diag(tableau)
for i in 1:(p+c+1)
d[i] = tableau[i, i]
end
for i = 1:p
if d[i] <= zero(T) || tableau[i, i] < d[i] * tol
return 0
else
sweep!(tableau, i, tmp, false)
end
end
# swept = trues(p)
fill!(swept, true)
for i = p + 1:p + c
if tableau[i, i] >= zero(T)
return 0
else
sweep!(tableau, i, tmp, false)
end
end
#
# Take a step in the direction tableau(i, end) for the parameters i
# that are currently swept. If a boundary is encountered, determine
# the maximal fractional step possible.
#
cycle_main_loop = false
for iteration = 1:1000
if verbose
println(swept)
end
a = one(T)
for i = 1:p
if swept[i]
ui = tableau[i, end]
if ui > zero(T)
ai = pmax[i] - par[i] - delta[i]
else
ai = pmin[i] - par[i] - delta[i]
end
if abs(ui) > T(1e-10)
a = min(a, ai / ui)
end
end
end
#
# Take the fractional step for the currently swept parameters, and
# reset the transformed partial derivatives for these parameters.
#
for i = 1:p
if swept[i]
ui = tableau[i, end]
delta[i] = delta[i] + a * ui
tableau[i, end] = (one(T) - a) * ui
tableau[end, i] = tableau[i, end]
end
end
#
# Find a swept parameter that is critical, and inverse sweep it.
# Go back and try to take another step or fractional step.
#
cycle_main_loop = false
for i = 1:p
critical = pmin[i] >= par[i] + delta[i] - small
critical = critical || pmax[i]<= par[i] + delta[i] + small
if swept[i] && abs(tableau[i, i])>1e-10 && critical
if verbose
println("unsweeping: $i")
println("est: $(par[i]) + $(delta[i]) = $(par[i] + delta[i])")
println("diagonal: $(tableau[i, i])")
end
sweep!(tableau, i, tmp, true)
swept[i] = false
cycle_main_loop = true
break
end
end
if cycle_main_loop; continue; end
#
# Find an unswept parameter that violates the KKT condition
# and sweep it. Go back and try to take a step or fractional step.
# If no such parameter exists, then the problem is solved.
#
for i = 1:p
ui = tableau[i, end]
violation = ui > zero(T) && pmin[i] >= par[i] + delta[i] - small
violation = violation || (ui < zero(T) && pmax[i]<= par[i] + delta[i] + small)
if !swept[i] && violation
if verbose
println("sweeping: $i")
println("ui: $ui, est: $(par[i]) + $(delta[i]) = $(par[i] + delta[i]))")
println("diagonal: $(tableau[i, i])")
end
sweep!(tableau, i, tmp, false)
swept[i] = true
cycle_main_loop = true
break
end
end
if cycle_main_loop; continue; end
return iteration
end
return 0
end # function quadratic_program
"""
Sweeps or inverse sweeps the symmetric tableau A on its kth diagonal entry.
"""
function sweep!(matrix_a::AbstractMatrix{T}, k::Int, tmp::AbstractVector{T}, inverse::Bool = false) where T
p = one(T) / matrix_a[k, k]
# v = matrix_a[:, k]
@turbo for i in 1:size(matrix_a, 1)
tmp[i] = matrix_a[i, k]
matrix_a[i, k] = zero(T)
matrix_a[k, i] = zero(T)
end
if inverse
tmp[k] = one(T)
else
tmp[k] = -one(T)
end
@inbounds for i = 1:size(matrix_a, 1)
pv = p * tmp[i] # scalar
for j in 1:size(matrix_a, 1)
matrix_a[j, i] = matrix_a[j, i] - pv * tmp[j]
end
# matrix_a[:, i] .= matrix_a[:, i] .- pv * v
end
end # function sweep!
| OpenADMIXTURE | https://github.com/OpenMendel/OpenADMIXTURE.jl.git |
|
[
"MIT"
] | 0.1.0 | 6eba29d96bef815c6fda407240c0c4ba358f0f6f | code | 1586 | """
update_UV!(U, V, x, x_next, x_next2, iter, Q)
Update U and V matrices for quasi-Newton acceleration.
"""
function update_UV!(U, V, x, x_next, x_next2, iter, Q)
idx = (iter - 1) % Q + 1
V[:, idx] .= x_next2 .- x_next
U[:, idx] .= x_next .- x
end
"""
update_UV_LBQN!(U, V, x, x_next, x_next2, iter, Q)
Update U and V matrices for Limited-memory Broyden's quasi-Newton acceleration.
"""
function update_UV_LBQN!(U, V, x, x_next, x_next2, iter, Q)
@inbounds for i in min(iter, Q):-1:2
U[:, i] .= @view(U[:, i-1])
V[:, i] .= @view(V[:, i-1])
end
U[:, 1] .= x_next .- x
V[:, 1] .= x_next2 .- x_next
V[:, 1] .= @view(V[:, 1]) .- @view(U[:, 1]) # x2 - 2x1 + x0
end
"""
update_QN!(x_next, x_mapped, x, U, V)
Update one step of quasi-Newton algorithm.
"""
function update_QN!(x_next, x_mapped, x, U, V)
x_next .= x_mapped .- V * (inv(U' * (U .- V)) * (U' * (x .- x_mapped)))
end
"""
update_QN_LBQN!(x_next, x, x_q, x_r, U, V)
Update one step of limited-memory broyden's quasi-Newton acceleration.
"""
function update_QN_LBQN!(x_next, x, x_q, x_r, U, V)
Q = size(U, 2)
u = @view(U[:, 1])
v = @view(V[:, 1])
gamma_t = dot(u, v) / dot(v, v)
x_q .= u
alpha = zeros(Q)
@inbounds for i in 1:Q
rho = 1 / dot(@view(V[:, i]), @view(V[:, i]))
alpha[i] = rho * dot(@view(V[:, i]), x_q)
x_q .= x_q .- alpha[i] .* @view(V[:, i])
end
x_r .= gamma_t .* x_q
@inbounds for i in 1:Q
x_r .= x_r .+ alpha[i] .* @view(U[:, i])
end
x_next .= x .- x_r
end
| OpenADMIXTURE | https://github.com/OpenMendel/OpenADMIXTURE.jl.git |
|
[
"MIT"
] | 0.1.0 | 6eba29d96bef815c6fda407240c0c4ba358f0f6f | code | 8123 | const SAT{T} = Matrix{T}
# const SAT{T} = SubArray{T, 2, Matrix{T},
# Tuple{Base.Slice{Base.OneTo{Int}}, UnitRange{Int}}, true}
const TwoDSlice{T} = Vector{SubArray{T, 2, Array{T, 3},
Tuple{Base.Slice{Base.OneTo{Int64}}, Base.Slice{Base.OneTo{Int64}}, Int64}, true}}
const OneDSlice{T} = Vector{SubArray{T, 1, Matrix{T},
Tuple{Base.Slice{Base.OneTo{Int64}}, Int64}, true}}
mutable struct AdmixData{T}
I ::Int
J ::Int
K ::Int
Q ::Int
skipmissing ::Bool
x ::Matrix{T} # K x (I + J)
x_next ::Matrix{T}
x_next2 ::Matrix{T}
x_tmp ::Matrix{T}
x_flat ::Vector{T} # K(I + J)
x_next_flat ::Vector{T}
x_next2_flat::Vector{T}
x_tmp_flat ::Vector{T}
# intermediate vectors for LBQN
x_qq ::Vector{T}
x_rr ::Vector{T}
q ::SAT{T} # K x I
q_next ::SAT{T}
q_next2 ::SAT{T}
q_tmp ::SAT{T}
p ::SAT{T} # K x J
p_next ::SAT{T}
p_next2 ::SAT{T}
p_tmp ::SAT{T}
XtX_q ::Array{T, 3} # K x K x I
Xtz_q ::Matrix{T} # K x I
XtX_p ::Array{T, 3} # K x K x J
Xtz_p ::Matrix{T} # K x J
# views
qv :: OneDSlice{T}
q_nextv :: OneDSlice{T}
q_tmpv :: OneDSlice{T}
pv :: OneDSlice{T}
p_nextv :: OneDSlice{T}
p_tmpv :: OneDSlice{T}
XtX_qv :: TwoDSlice{T}
Xtz_qv :: OneDSlice{T}
XtX_pv :: TwoDSlice{T}
Xtz_pv :: OneDSlice{T}
qp_small :: Array{T, 3} # 64 x 64
qp_smallv :: TwoDSlice{T}
U ::Matrix{T} # K(I + J) x Q
V ::Matrix{T} # K(I + J) x Q
# for QP
v_kk ::Matrix{T} # K x K, a full svd of ones(1, K)
tmp_k ::Matrix{T}
tmp_k1 ::Matrix{T}
tmp_k1_ ::Matrix{T}
tmp_k2 ::Matrix{T}
tmp_k2_ ::Matrix{T}
tableau_k1 ::Array{T, 3} # (K + 1) x (K + 1)
tableau_k2 ::Array{T, 3} # (K + 2) x (K + 2)
swept ::Matrix{Bool}
tmp_kv ::OneDSlice{T}
tmp_k1v ::OneDSlice{T}
tmp_k1_v ::OneDSlice{T}
tmp_k2v ::OneDSlice{T}
tmp_k2_v ::OneDSlice{T}
tableau_k1v ::TwoDSlice{T} # (K + 1) x (K + 1)
tableau_k2v ::TwoDSlice{T} # (K + 2) x (K + 2)
sweptv ::OneDSlice{Bool}
idx ::Matrix{Int}
idxv ::OneDSlice{Int}
# loglikelihoods
ll_prev ::Float64
ll_new ::Float64
end
"""
AdmixData{T}(I, J, K, Q; skipmissing=true, rng=Random.GLOBAL_RNG)
Constructor for Admixture information.
# Arguments:
- I: Number of samples
- J: Number of variants
- K: Number of clusters
- Q: Number of steps used for quasi-Newton update
- skipmissing: skip computation of loglikelihood for missing values. Should be kept `true` in most cases
- rng: Random number generation.
"""
function AdmixData{T}(I, J, K, Q; skipmissing=true, rng=Random.GLOBAL_RNG) where T
NT = nthreads()
x = convert(Array{T}, rand(rng, K, I + J))
x_next = similar(x)
x_next2 = similar(x)
x_tmp = similar(x)
x_flat = reshape(x, :)
x_next_flat = reshape(x_next, :)
x_next2_flat = reshape(x_next2, :)
x_tmp_flat = reshape(x_tmp, :)
x_qq = similar(x_flat)
x_rr = similar(x_flat)
q = view(x , :, 1:I)#rand(T, K, J)
q = unsafe_wrap(Array, pointer(q), size(q))
q_next = view(x_next , :, 1:I)
q_next = unsafe_wrap(Array, pointer(q_next), size(q_next))
q_next2 = view(x_next2, :, 1:I)
q_next2 = unsafe_wrap(Array, pointer(q_next2), size(q_next2))
q_tmp = view(x_tmp, :, 1:I)
q_tmp = unsafe_wrap(Array, pointer(q_tmp), size(q_tmp))
q ./= sum(q, dims=1)
p = view(x , :, (I+1):(I+J))#rand(T, K, J)
p = unsafe_wrap(Array, pointer(p), size(p))
p_next = view(x_next , :, (I+1):(I+J))
p_next = unsafe_wrap(Array, pointer(p_next), size(p_next))
p_next2 = view(x_next2, :, (I+1):(I+J))
p_next2 = unsafe_wrap(Array, pointer(p_next2), size(p_next2))
p_tmp = view(x_tmp, :, (I+1):(I+J))
p_tmp = unsafe_wrap(Array, pointer(p_tmp), size(p_tmp))
XtX_q = convert(Array{T}, rand(rng, K, K, I))
Xtz_q = convert(Array{T}, rand(rng, K, I))
XtX_p = convert(Array{T}, rand(rng, K, K, J))
Xtz_p = convert(Array{T}, rand(rng, K, J))
qv = [view(q, :, i) for i in 1:I]
q_nextv = [view(q_next, :, i) for i in 1:I]
q_tmpv = [view(q_tmp, :, i) for i in 1:I]
pv = [view(p, :, j) for j in 1:J]
p_nextv = [view(p_next, :, j) for j in 1:J]
p_tmpv = [view(p_tmp, :, j) for j in 1:J]
XtX_qv = [view(XtX_q, :, :, i) for i in 1:I]
Xtz_qv = [view(Xtz_q, :, i) for i in 1:I]
XtX_pv = [view(XtX_p, :, :, j) for j in 1:J]
Xtz_pv = [view(Xtz_p, :, j) for j in 1:J]
# q,
# q_arr = unsafe_wrap(Array, pointer(q), size(q))
# q_next_arr = unsafe_wrap(Array, pointer(q_next), size(q_next))
# q_tmp_arr = unsafe_wrap(Array, pointer(q_tmp), size(q_tmp))
# f_arr = unsafe_wrap(Array, pointer(q), size(q))
# f_next_arr = unsafe_wrap(Array, pointer(q_next), size(q_next))
# f_tmp_arr = unsafe_wrap(Array, pointer(q_tmp), size(q_tmp))
# qf = rand(T, I, J);
maxL = tile_maxiter(typeof(Xtz_p))
qp_small = convert(Array{T}, rand(rng, maxL, maxL, NT))
qp_smallv = [view(qp_small, :, :, t) for t in 1:NT]
# qf_thin = rand(T, I, maxL)
# f_tmp = similar(f)
# q_tmp = similar(q);
V = convert(Array{T}, rand(rng, K * (I+J), Q))
# V_q = view(reshape(V, K, (I+J), Q), :, 1:I, :)
# V_f = view(reshape(V, K, (I+J), Q), :, (I+1):(I+J), :)
U = convert(Array{T}, rand(rng, K * (I+J), Q))
_, _, vt = svd(ones(T, 1, K), full=true)
tmp_k = Matrix{T}(undef, K, NT)
tmp_kv = [view(tmp_k, :, t) for t in 1:NT]
tmp_k1 = Matrix{T}(undef, K+1, NT)
tmp_k1v = [view(tmp_k1, :, t) for t in 1:NT]
tmp_k1_ = similar(tmp_k1)
tmp_k1_v = [view(tmp_k1_, :, t) for t in 1:NT]
tmp_k2 = Matrix{T}(undef, K+2, NT)
tmp_k2v = [view(tmp_k2, :, t) for t in 1:NT]
tmp_k2_ = similar(tmp_k2)
tmp_k2_v = [view(tmp_k2_, :, t) for t in 1:NT]
tableau_k1 = Array{T, 3}(undef, K+1, K+1, NT)
tableau_k1v = [view(tableau_k1, :, :, t) for t in 1:NT]
tableau_k2 = Array{T, 3}(undef, K+2, K+2, NT)
tableau_k2v = [view(tableau_k2, :, :, t) for t in 1:NT]
swept = convert(Matrix{Bool}, trues(K, NT))
sweptv = [view(swept, :, t) for t in 1:NT]
idx = Array{Int}(undef, K, NT)
idxv = [view(idx, :, t) for t in 1:NT]
# U_q = view(reshape(U, K, (I+J), Q), :, 1:I, :)
# U_f = view(reshape(U, K, (I+J), Q), :, (I+1):(I+J), :)
AdmixData{T}(I, J, K, Q, skipmissing, x, x_next, x_next2, x_tmp,
x_flat, x_next_flat, x_next2_flat, x_tmp_flat,
x_qq, x_rr,
q, q_next, q_next2, q_tmp, p, p_next, p_next2, p_tmp,
XtX_q, Xtz_q, XtX_p, Xtz_p,
qv, q_nextv, q_tmpv, pv, p_nextv, p_tmpv, XtX_qv, Xtz_qv, XtX_pv, Xtz_pv,
qp_small, qp_smallv, U, V, vt,
tmp_k, tmp_k1, tmp_k1_, tmp_k2, tmp_k2_,
tableau_k1, tableau_k2, swept,
tmp_kv, tmp_k1v, tmp_k1_v, tmp_k2v, tmp_k2_v,
tableau_k1v, tableau_k2v, sweptv,
idx, idxv,
NaN, NaN)
end
struct QPThreadLocal{T}
tmp_k :: Vector{T}
tmp_k1 :: Vector{T}
tmp_k1_ :: Vector{T}
tmp_k2 :: Vector{T}
tmp_k2_ :: Vector{T}
tableau_k1 :: Matrix{T}
tableau_k2 :: Matrix{T}
swept :: Vector{Bool}
idx :: Vector{Int}
end
function QPThreadLocal{T}(K::Int) where T
tmp_k = Vector{T}(undef, K)
tmp_k1 = Vector{T}(undef, K+1)
tmp_k1_ = Vector{T}(undef, K+1)
tmp_k2 = Vector{T}(undef, K+2)
tmp_k2_ = similar(tmp_k2)
tableau_k1 = Array{T, 2}(undef, K+1, K+1)
tableau_k2 = Array{T, 2}(undef, K+2, K+2)
swept = convert(Vector{Bool}, trues(K))
idx = Array{Int}(undef, K)
QPThreadLocal{T}(tmp_k, tmp_k1, tmp_k1_, tmp_k2, tmp_k2_,
tableau_k1, tableau_k2, swept, idx)
end | OpenADMIXTURE | https://github.com/OpenMendel/OpenADMIXTURE.jl.git |
|
[
"MIT"
] | 0.1.0 | 6eba29d96bef815c6fda407240c0c4ba358f0f6f | code | 6947 | using .CUDA
@inline function loglikelihood_kernel(out, g::AbstractArray{UInt8, 2},
q::AbstractArray{T, 2}, p::AbstractArray{T, 2}, irange, jrange, joffset=0) where T
K = size(q, 1)
firsti, firstj = first(irange), first(jrange)
lasti, lastj = last(irange), last(jrange)
xindex = (blockIdx().x - 1) * blockDim().x + threadIdx().x
xstride = blockDim().x * gridDim().x
yindex = (blockIdx().y - 1) * blockDim().y + threadIdx().y
ystride = blockDim().y * gridDim().y
oneT = one(T)
twoT = 2one(T)
acc = zero(Float64)
@inbounds for j = (firstj + yindex - 1):ystride:lastj
for i = (firsti + xindex - 1):xstride:lasti
qp_local = zero(eltype(out))
for k in 1:K
qp_local += q[k, i] * p[k, j]
end
blk = g[(i - 1) >> 2 + 1, j - joffset]
re = (i - 1) % 4
blk_shifted = blk >> (re << 1)
gij_pre = blk_shifted & 0x03
gij = T(gij_pre > 0x01) * T(gij_pre - 0x01)
nonmissing = T(gij_pre != 0x01)
@inbounds acc += nonmissing * (gij * log(qp_local) +
(twoT - gij) * log(oneT - qp_local))
end
end
CUDA.@atomic out[] += acc
return nothing
end
@inline function em_q_kernel!(q_next, g::AbstractArray{UInt8, 2},
q::AbstractArray{T, 2}, p::AbstractArray{T, 2}, irange, jrange, joffset=0) where T
K = size(q, 1)
firsti, firstj = first(irange), first(jrange)
lasti, lastj = last(irange), last(jrange)
xindex = (blockIdx().x - 1) * blockDim().x + threadIdx().x
xstride = blockDim().x * gridDim().x
yindex = (blockIdx().y - 1) * blockDim().y + threadIdx().y
ystride = blockDim().y * gridDim().y
oneT = one(T)
twoT = 2one(T)
@inbounds for j = (firstj + yindex - 1):ystride:lastj
for i = (firsti + xindex - 1):xstride:lasti
qp_local = zero(eltype(q_next))
for k in 1:K
qp_local += q[k, i] * p[k, j]
end
blk = g[(i - 1) >> 2 + 1, j - joffset]
re = (i - 1) % 4
blk_shifted = blk >> (re << 1)
gij_pre = blk_shifted & 0x03
gij = T(gij_pre > 0x01) * T(gij_pre - 0x01)
nonmissing = T(gij_pre != 0x01)
for k in 1:K
tmp = (gij * q[k, i] * p[k, j] / qp_local +
(2 - gij) * q[k, i] * (1 - p[k, j]) / (1 - qp_local))
CUDA.@atomic q_next[k, i] += nonmissing * tmp
end
end
end
return nothing
end
@inline function em_p_kernel!(p_next, p_tmp, g::AbstractArray{UInt8, 2},
q::AbstractArray{T, 2}, p::AbstractArray{T, 2}, irange, jrange, joffset=0) where T
K = size(q, 1)
firsti, firstj = first(irange), first(jrange)
lasti, lastj = last(irange), last(jrange)
xindex = (blockIdx().x - 1) * blockDim().x + threadIdx().x
xstride = blockDim().x * gridDim().x
yindex = (blockIdx().y - 1) * blockDim().y + threadIdx().y
ystride = blockDim().y * gridDim().y
oneT = one(T)
twoT = 2one(T)
@inbounds for j = (firstj + yindex - 1):ystride:lastj
for i = (firsti + xindex - 1):xstride:lasti
qp_local = zero(eltype(p_next))
for k in 1:K
qp_local += q[k, i] * p[k, j]
end
blk = g[(i - 1) >> 2 + 1, j - joffset]
re = (i - 1) % 4
blk_shifted = blk >> (re << 1)
gij_pre = blk_shifted & 0x03
gij = T(gij_pre > 0x01) * T(gij_pre - 0x01)
nonmissing = T(gij_pre != 0x01)
for k in 1:K
CUDA.@atomic p_tmp[k, j] += nonmissing * (gij * q[k, i] * p[k, j] / qp_local)
CUDA.@atomic p_next[k, j] += nonmissing * ((twoT - gij) * q[k, i] *
(oneT - p[k, j]) / (oneT - qp_local))
end
end
end
return nothing
end
@inline function update_q_kernel!(XtX, Xtz, g::AbstractArray{UInt8, 2},
q::AbstractArray{T, 2}, p::AbstractArray{T, 2}, irange, jrange, joffset=0) where T
K = size(q, 1)
firsti, firstj = first(irange), first(jrange)
lasti, lastj = last(irange), last(jrange)
xindex = (blockIdx().x - 1) * blockDim().x + threadIdx().x
xstride = blockDim().x * gridDim().x
yindex = (blockIdx().y - 1) * blockDim().y + threadIdx().y
ystride = blockDim().y * gridDim().y
oneT = one(T)
twoT = 2one(T)
@inbounds for j = (firstj + yindex - 1):ystride:lastj
for i = (firsti + xindex - 1):xstride:lasti
qp_local = zero(eltype(XtX))
for k in 1:K
qp_local += q[k, i] * p[k, j]
end
blk = g[(i - 1) >> 2 + 1, j - joffset]
re = (i - 1) % 4
blk_shifted = blk >> (re << 1)
gij_pre = blk_shifted & 0x03
gij = T(gij_pre > 0x01) * T(gij_pre - 0x01)
nonmissing = T(gij_pre != 0x01)
for k in 1:K
CUDA.@atomic Xtz[k, i] += nonmissing * (gij * p[k, j] / qp_local +
(twoT - gij) * (oneT - p[k, j]) / (oneT - qp_local))
for k2 in 1:K
CUDA.@atomic XtX[k2, k, i] += nonmissing * (gij / (qp_local) ^ 2 * p[k, j] * p[k2, j] +
(twoT - gij) / (oneT - qp_local) ^ 2 * (oneT - p[k, j]) * (oneT - p[k2, j]))
end
end
end
end
return nothing
end
@inline function update_p_kernel!(XtX, Xtz, g::AbstractArray{UInt8, 2},
q::AbstractArray{T, 2}, p::AbstractArray{T, 2}, irange, jrange, joffset=0) where T
K = size(q, 1)
firsti, firstj = first(irange), first(jrange)
lasti, lastj = last(irange), last(jrange)
xindex = (blockIdx().x - 1) * blockDim().x + threadIdx().x
xstride = blockDim().x * gridDim().x
yindex = (blockIdx().y - 1) * blockDim().y + threadIdx().y
ystride = blockDim().y * gridDim().y
oneT = one(T)
twoT = 2one(T)
@inbounds for j = (firstj + yindex - 1):ystride:lastj
for i = (firsti + xindex - 1):xstride:lasti
qp_local = zero(eltype(XtX))
for k in 1:K
qp_local += q[k, i] * p[k, j]
end
blk = g[(i - 1) >> 2 + 1, j - joffset]
re = (i - 1) % 4
blk_shifted = blk >> (re << 1)
gij_pre = blk_shifted & 0x03
gij = T(gij_pre > 0x01) * T(gij_pre - 0x01)
nonmissing = T(gij_pre != 0x01)
for k in 1:K
CUDA.@atomic Xtz[k, j] += nonmissing * (gij * q[k, i] / qp_local -
(twoT - gij) * q[k, i] / (oneT - qp_local))
for k2 in 1:K
CUDA.@atomic XtX[k2, k, j] += nonmissing * (gij / (qp_local) ^ 2 * q[k, i] * q[k2, i] +
(twoT - gij) / (oneT - qp_local) ^ 2 * q[k, i] * q[k2, i])
end
end
end
end
return nothing
end
| OpenADMIXTURE | https://github.com/OpenMendel/OpenADMIXTURE.jl.git |
|
[
"MIT"
] | 0.1.0 | 6eba29d96bef815c6fda407240c0c4ba358f0f6f | code | 4068 | using .CUDA
function loglikelihood(g::CuArray{UInt8, 2}, q::CuArray{T, 2}, p::CuArray{T, 2}, I, J) where T
out = CUDA.zeros(Float64)
kernel = @cuda launch=false loglikelihood_kernel(out, g, q, p, 1:I, 1:J)
config = launch_configuration(kernel.fun)
threads = config.threads
threads_1d = Int(floor(sqrt(threads)))
CUDA.@sync kernel(out, g, q, p, 1:I, 1:J; threads=(threads_1d, threads_1d),
blocks=(threads_1d, threads_1d))
out[]
end
function loglikelihood(d::CuAdmixData{T}, g_cu::CuMatrix{UInt8}) where T
I, J, K = size(d.q, 2), size(g_cu, 2), size(d.q, 1)
if J == size(d.p, 2)
return loglikelihood(g_cu, d.q, d.p, I, J)
else
@assert false "Not implemented"
end
end
function em_q!(q_next, g::CuArray{UInt8, 2},
q::CuArray{T, 2}, p::CuArray{T, 2}, I, J) where T
fill!(q_next, zero(eltype(q_next)))
kernel = @cuda launch=false em_q_kernel!(q_next, g, q, p, 1:I, 1:J)
config = launch_configuration(kernel.fun)
threads = config.threads
threads_1d = Int(floor(sqrt(threads)))
# println("threads: $threads")
CUDA.@sync kernel(q_next, g, q, p, 1:I, 1:J; threads=(threads_1d, threads_1d),
blocks=(cld(I, threads_1d), cld(I, threads_1d)))
q_next ./= 2J
q_next
end
function em_q!(d::CuAdmixData{T}, g_cu::CuMatrix{UInt8}; verbose=false) where T
I, J, K = size(d.q, 2), size(g_cu, 2), size(d.q, 1)
if J == size(d.p, 2)
em_q!(d.q_next, g_cu, d.q, d.p, I, J)
else
@assert false "Not implemented"
end
end
function em_p!(p_next, p_tmp, g::CuArray{UInt8, 2},
q::CuArray{T, 2}, p::CuArray{T, 2}, I, J) where T
fill!(p_next, zero(eltype(p_next)))
fill!(p_tmp, zero(eltype(p_tmp)))
kernel = @cuda launch=false em_p_kernel!(p_next, p_tmp, g, q, p, 1:I, 1:J)
config = launch_configuration(kernel.fun)
threads = config.threads
threads_1d = Int(floor(sqrt(threads)))
# println("threads: $threads")
CUDA.@sync kernel(p_next, p_tmp, g, q, p, 1:I, 1:J; threads=(threads_1d, threads_1d),
blocks=(2threads_1d, 2threads_1d))
p_next .= p_tmp ./ (p_tmp .+ p_next)
p_next
end
function em_p!(d::CuAdmixData{T}, g_cu::CuMatrix{UInt8}; verbose=false) where T
I, J, K = size(d.q, 2), size(g_cu, 2), size(d.q, 1)
if J == size(d.p, 2)
em_p!(d.p_next, d.p_tmp, g_cu, d.q, d.p, I, J)
else
@assert false "Not implemented"
end
end
function update_q_cuda!(XtX, Xtz, g, q, p, I, J)
fill!(XtX, zero(eltype(XtX)))
fill!(Xtz, zero(eltype(Xtz)))
kernel = @cuda launch=false update_q_kernel!(XtX, Xtz, g, q, p, 1:I, 1:J)
config = launch_configuration(kernel.fun)
threads = config.threads
threads_1d = Int(floor(sqrt(threads)))
# println("threads: $threads")
CUDA.@sync kernel(XtX, Xtz, g, q, p, 1:I, 1:J; threads=(threads_1d, threads_1d),
blocks=(threads_1d, threads_1d))#cld(I, threads_1d), cld(I, threads_1d)))
nothing
end
function update_q_cuda!(d::CuAdmixData{T}, g_cu::CuMatrix{UInt8}) where T
I, J, K = size(d.q, 2), size(g_cu, 2), size(d.q, 1)
if J == size(d.p, 2)
update_q_cuda!(d.XtX_q, d.Xtz_q, g_cu, d.q, d.p, I, J)
else
@assert false "Not implemented"
end
end
function update_p_cuda!(XtX, Xtz, g, q, p, I, J)
fill!(XtX, zero(eltype(XtX)))
fill!(Xtz, zero(eltype(Xtz)))
kernel = @cuda launch=false update_p_kernel!(XtX, Xtz, g, q, p, 1:I, 1:J)
config = launch_configuration(kernel.fun)
threads = config.threads
threads_1d = Int(floor(sqrt(threads)))
# println("threads: $threads")
CUDA.@sync kernel(XtX, Xtz, g, q, p, 1:I, 1:J; threads=(threads_1d, threads_1d),
blocks=(threads_1d, threads_1d))#cld(I, threads_1d), cld(I, threads_1d)))
nothing
end
function update_p_cuda!(d::CuAdmixData{T}, g_cu::CuMatrix{UInt8}) where T
I, J, K = size(d.q, 2), size(g_cu, 2), size(d.q, 1)
if J == size(d.p, 2)
update_p_cuda!(d.XtX_p, d.Xtz_p, g_cu, d.q, d.p, I, J)
else
@assert false "Not implemented"
end
end
| OpenADMIXTURE | https://github.com/OpenMendel/OpenADMIXTURE.jl.git |
|
[
"MIT"
] | 0.1.0 | 6eba29d96bef815c6fda407240c0c4ba358f0f6f | code | 1204 | struct CuAdmixData{T}
# g::CuArray{UInt8, 2}
q::CuArray{T, 2}
q_next::CuArray{T, 2}
p::CuArray{T, 2}
p_next::CuArray{T, 2}
p_tmp::CuArray{T, 2}
XtX_q::CuArray{T, 3}
Xtz_q::CuArray{T, 2}
XtX_p::CuArray{T, 3}
Xtz_p::CuArray{T, 2}
end
function CuAdmixData(d::AdmixData{T}, g::SnpLinAlg{T}, width=d.J) where T
I, J, K = d.I, d.J, d.K
@assert d.skipmissing "skipmissing must be true for CuAdmixData."
Ibytes = (I + 3) ÷ 4
# g_cu = CuArray{UInt8, 2}(undef, Ibytes, width)
# if size(g, 2) == J
# copyto!(g_cu, g.s.data)
# end
q = CuArray{T, 2}(undef, K, I)
q_next = similar(q)
p = CuArray{T, 2}(undef, K, J)
p_next = similar(p)
p_tmp = similar(p)
XtX_q = CuArray{T, 3}(undef, K, K, I)
Xtz_q = CuArray{T, 2}(undef, K, I)
XtX_p = CuArray{T, 3}(undef, K, K, J)
Xtz_p = CuArray{T, 2}(undef, K, J)
CuAdmixData{T}(q, q_next, p, p_next, p_tmp, XtX_q, Xtz_q, XtX_p, Xtz_p)
end
function _cu_admixture_base(d::AdmixData, g_la::SnpLinAlg, I::Int, J::Int)
d_cu = CuAdmixData(d, g_la)
Ibytes = (I + 3) ÷ 4
g_cu = CuArray{UInt8, 2}(undef, Ibytes, J)
copyto!(g_cu, g_la.s.data)
d_cu, g_cu
end | OpenADMIXTURE | https://github.com/OpenMendel/OpenADMIXTURE.jl.git |
|
[
"MIT"
] | 0.1.0 | 6eba29d96bef815c6fda407240c0c4ba358f0f6f | code | 223 | function copyto_sync!(As_d1::Vector{<:AbstractArray{T}}, As_d2::Vector{<:AbstractArray{T}}) where T
CUDA.@sync begin
for (A_d1, A_d2) in zip(As_d1, As_d2)
copyto!(A_d1, A_d2)
end
end
end
| OpenADMIXTURE | https://github.com/OpenMendel/OpenADMIXTURE.jl.git |
|
[
"MIT"
] | 0.1.0 | 6eba29d96bef815c6fda407240c0c4ba358f0f6f | code | 556 | using OpenADMIXTURE, SnpArrays, Random
using Test
@testset "OpenADMIXTURE.jl" begin
EUR = SnpArrays.datadir("EUR_subset.bed")
rng = MersenneTwister(7856)
d, _, _ = OpenADMIXTURE.run_admixture(EUR, 4; rng=rng)
@test d.ll_new ≈ -1.555219392972111e7
rng = MersenneTwister(7856)
d, _, _ = OpenADMIXTURE.run_admixture(EUR, 4; sparsity=1000, rng=rng, prefix="test")
@test d.ll_new ≈ -216519.78193892565
rm("test_4_1000aims.bed", force=true)
rm("test_4_1000aims.bim", force=true)
rm("test_4_1000aims.fam", force=true)
end
| OpenADMIXTURE | https://github.com/OpenMendel/OpenADMIXTURE.jl.git |
|
[
"MIT"
] | 0.1.0 | 6eba29d96bef815c6fda407240c0c4ba358f0f6f | docs | 2612 | # OpenADMIXTURE.jl
[](https://OpenMendel.github.io/OpenADMIXTURE.jl/dev)
This software package is an open-source Julia reimplementation of the [ADMIXTURE](https://dalexander.github.io/admixture/) package. It estimates ancestry with maximum-likelihood method for a large SNP genotype datasets, where individuals are assumed to be unrelated. The input is binary PLINK 1 BED-formatted file (`.bed`). Also, you will need an idea of $K$, the number of ancestral populations. If the number of SNPs is too large, you may choose to run on a subset of SNPs selected by their information content, using the [sparse K-means via feature ranking](https://github.com/kose-y/SKFR.jl) (SKFR) method.
With more efficient multi-threading scheme, it is 8 times faster than the original software on a 16-threaded machine. It also supports computation on an Nvidia CUDA GPU. By directly using the data format of the PLINK BED file, the memory usage is 16x-32x smaller than using `Float32` or `Float64` type.
## Installation
This package requires Julia v1.9 or later, which can be obtained from
<https://julialang.org/downloads/> or by building Julia from the sources in the
<https://github.com/JuliaLang/julia> repository.
The package can be installed by running the following code:
```julia
using Pkg
pkg"add https://github.com/kose-y/SKFR.jl"
pkg"add https://github.com/OpenMendel/OpenADMIXTURE.jl"
```
For running the examples in our documentation, the following are also necessary.
```julia
pkg"add SnpArrays CSV DelimitedFiles"
```
For GPU support, an Nvidia GPU is required. Also, the following package has to be installed:
```julia
pkg"add CUDA"
```
## Citation
The methods and applications of this software package are detailed in the following publication:
_Ko S, Chu BB, Peterson D, Okenwa C, Papp JC, Alexander DH, Sobel EM, Zhou H, Lange K. Unsupervised Discovery of Ancestry Informative Markers and Genetic Admixture Proportions in Biobank-Scale Data Sets. Am J Hum Genet. 110, pp. 314–325. [[DOI](https://doi.org/10.1016/j.ajhg.2022.12.008)]_
If you use OpenMendel analysis packages in your research, please cite the following reference in the resulting publications:
_Zhou H, Sinsheimer JS, Bates DM, Chu BB, German CA, Ji SS, Keys KL, Kim J, Ko S, Mosher GD, Papp JC, Sobel EM, Zhai J, Zhou JJ, Lange K. OPENMENDEL: a cooperative programming project for statistical genetics. Hum Genet. 2020 Jan;139(1):61-71. doi: 10.1007/s00439-019-02001-z. Epub 2019 Mar 26. PMID: 30915546; PMCID: [PMC6763373](https://www.ncbi.nlm.nih.gov/pmc/articles/PMC6763373/)._
| OpenADMIXTURE | https://github.com/OpenMendel/OpenADMIXTURE.jl.git |
|
[
"MIT"
] | 0.1.0 | 6eba29d96bef815c6fda407240c0c4ba358f0f6f | docs | 127740 | # OpenADMIXTURE.jl
This software package is an open-source Julia reimplementation of the [ADMIXTURE](https://dalexander.github.io/admixture/) package. With more efficient multi-threading scheme, it is 8 times faster than the original software. It also supports computation on an Nvidia CUDA GPU. By directly using the data format of the PLINK BED file, the memory usage is 16x-32x smaller than using `Float32` or `Float64` type.
It estimates ancestry with maximum-likelihood method for a large SNP genotype datasets, where individuals are assumed to be unrelated. The input is binary PLINK 1 BED-formatted file (`.bed`). Also, you will need an idea of $K$, the number of ancestral populations. If the number of SNPs is too large, you may choose to run on a subset of SNPs selected by their information content, using the [sparse $K$-means via feature ranking](https://github.com/kose-y/SparseKmeansFeatureRanking.jl) (SKFR) method.
## Installation
This package requires Julia v1.6 or later, which can be obtained from
<https://julialang.org/downloads/> or by building Julia from the sources in the
<https://github.com/JuliaLang/julia> repository.
The package can be installed by running the following code:
```julia
using Pkg
pkg"add https://github.com/kose-y/SparseKmeansFeatureRanking.jl"
pkg"add https://github.com/OpenMendel/OpenADMIXTURE.jl"
```
For running the examples below, the following are also necessary.
```julia
pkg"add SnpArrays CSV DelimitedFiles"
```
For GPU support, an Nvidia GPU is required. Also, the following package has to be installed:
```julia
pkg"add CUDA"
```
## Basic Usage
We first import necessary packages:
```julia
using LinearAlgebra, Random, SnpArrays
using OpenADMIXTURE
using CSV, DelimitedFiles
```
We will use the PLINK file included in the `SnpArrays` package, whose path is obtained by:
```julia
filename = SnpArrays.datadir("EUR_subset.bed");
```
This file contains information on 54051 single nucleotide polymorphisms (SNP)s of 379 samples. The main driver function for admixture proportion estimation is called `run_admixture()`.
```julia
d, clusters, aims = OpenADMIXTURE.run_admixture(filename, 4; T=Float64, use_gpu=false, rng=MersenneTwister(7856))
```
0.087914 seconds (4 allocations: 64 bytes)
0.092882 seconds (4 allocations: 64 bytes)
0.088550 seconds (4 allocations: 64 bytes)
0.092690 seconds (4 allocations: 64 bytes)
0.088199 seconds (4 allocations: 64 bytes)
0.092607 seconds (4 allocations: 64 bytes)
0.087901 seconds (4 allocations: 64 bytes)
0.092424 seconds (4 allocations: 64 bytes)
0.088549 seconds (4 allocations: 64 bytes)
0.093026 seconds (4 allocations: 64 bytes)
22.884278 seconds (34.59 M allocations: 1.742 GiB, 3.08% gc time, 95.16% compilation time)
initial ll: -1.5844784297993343e7
-1.5844784297993343e7
0.281655 seconds (4 allocations: 64 bytes)
2.816971 seconds (3.95 M allocations: 210.701 MiB, 9.53% gc time, 99.98% compilation time)
0.370153 seconds (4 allocations: 64 bytes)
0.097757 seconds (25.01 k allocations: 1.334 MiB, 64.46% compilation time)
-1.5844784297993343e7
0.277199 seconds (4 allocations: 64 bytes)
0.000504 seconds (2.66 k allocations: 125.109 KiB)
0.369947 seconds (4 allocations: 64 bytes)
0.033502 seconds (8 allocations: 768 bytes)
-1.5844784297993343e7
-1.5658797780233778e7
-1.5661966737375751e7
Iteration 1: ll = -1.5661966737375751e7, reldiff = 0.011538027730724302
4.664041 seconds (4.00 M allocations: 217.843 MiB, 5.76% gc time, 62.15% compilation time)
-1.5661966737375751e7
0.277490 seconds (4 allocations: 64 bytes)
0.000491 seconds (2.66 k allocations: 125.109 KiB)
0.369987 seconds (4 allocations: 64 bytes)
0.031646 seconds (8 allocations: 768 bytes)
-1.5661966737375751e7
0.280284 seconds (4 allocations: 64 bytes)
0.000493 seconds (2.66 k allocations: 125.109 KiB)
0.368922 seconds (4 allocations: 64 bytes)
0.030659 seconds (8 allocations: 768 bytes)
-1.5661966737375751e7
-1.5609040036582384e7
-1.5605199791986194e7
Iteration 2: ll = -1.5605199791986194e7, reldiff = 0.0036245093825980914
1.758175 seconds (8.49 k allocations: 7.039 MiB)
-1.5605199791986194e7
0.277546 seconds (4 allocations: 64 bytes)
0.000498 seconds (2.66 k allocations: 125.109 KiB)
0.369400 seconds (4 allocations: 64 bytes)
0.029828 seconds (8 allocations: 768 bytes)
-1.5605199791986194e7
0.277210 seconds (4 allocations: 64 bytes)
0.000967 seconds (2.66 k allocations: 125.109 KiB)
0.371205 seconds (4 allocations: 64 bytes)
0.028913 seconds (8 allocations: 768 bytes)
-1.5605199791986194e7
-1.558989311248903e7
-1.5586228372093504e7
Iteration 3: ll = -1.5586228372093504e7, reldiff = 0.0012157114388520478
1.755166 seconds (8.49 k allocations: 8.697 MiB)
-1.5586228372093504e7
0.277433 seconds (4 allocations: 64 bytes)
0.000543 seconds (2.66 k allocations: 125.109 KiB)
0.369406 seconds (4 allocations: 64 bytes)
0.028202 seconds (8 allocations: 768 bytes)
-1.5586228372093504e7
0.277489 seconds (4 allocations: 64 bytes)
0.000514 seconds (2.66 k allocations: 125.109 KiB)
0.369718 seconds (4 allocations: 64 bytes)
0.027662 seconds (8 allocations: 768 bytes)
-1.5586228372093504e7
-1.557593892598145e7
-1.557902813062439e7
Iteration 4: ll = -1.557902813062439e7, reldiff = 0.00046196175862575915
1.750993 seconds (8.49 k allocations: 8.697 MiB)
-1.557902813062439e7
0.278042 seconds (4 allocations: 64 bytes)
0.000524 seconds (2.66 k allocations: 125.109 KiB)
0.371190 seconds (4 allocations: 64 bytes)
0.032144 seconds (8 allocations: 768 bytes)
-1.557902813062439e7
0.402467 seconds (4 allocations: 64 bytes)
0.000528 seconds (2.66 k allocations: 125.109 KiB)
0.369614 seconds (4 allocations: 64 bytes)
0.027682 seconds (8 allocations: 768 bytes)
-1.557902813062439e7
-1.5566929427850641e7
-1.5567066087137524e7
Iteration 5: ll = -1.5567066087137524e7, reldiff = 0.0007678298919912029
1.966889 seconds (8.49 k allocations: 8.697 MiB)
-1.5567066087137524e7
0.346047 seconds (4 allocations: 64 bytes)
0.000535 seconds (2.66 k allocations: 125.109 KiB)
0.369873 seconds (4 allocations: 64 bytes)
0.027421 seconds (8 allocations: 768 bytes)
-1.5567066087137524e7
0.276677 seconds (4 allocations: 64 bytes)
0.000541 seconds (2.66 k allocations: 125.109 KiB)
0.368943 seconds (4 allocations: 64 bytes)
0.027093 seconds (8 allocations: 768 bytes)
-1.5567066087137524e7
-1.556264024120358e7
-1.5561476004841125e7
Iteration 6: ll = -1.5561476004841125e7, reldiff = 0.000359096715149059
1.821452 seconds (8.49 k allocations: 8.701 MiB)
-1.5561476004841125e7
0.279316 seconds (4 allocations: 64 bytes)
0.000554 seconds (2.66 k allocations: 125.109 KiB)
0.369430 seconds (4 allocations: 64 bytes)
0.027207 seconds (8 allocations: 768 bytes)
-1.5561476004841125e7
0.277530 seconds (4 allocations: 64 bytes)
0.000546 seconds (2.66 k allocations: 125.109 KiB)
0.369106 seconds (4 allocations: 64 bytes)
0.026839 seconds (8 allocations: 768 bytes)
-1.5561476004841125e7
-1.5558483191215409e7
-1.5558616674667932e7
Iteration 7: ll = -1.5558616674667932e7, reldiff = 0.00018374414948190118
1.751149 seconds (8.49 k allocations: 8.697 MiB)
-1.5558616674667932e7
0.277001 seconds (4 allocations: 64 bytes)
0.000557 seconds (2.66 k allocations: 125.109 KiB)
0.369540 seconds (4 allocations: 64 bytes)
0.026845 seconds (8 allocations: 768 bytes)
-1.5558616674667932e7
0.278763 seconds (4 allocations: 64 bytes)
0.000558 seconds (2.66 k allocations: 125.109 KiB)
0.369124 seconds (4 allocations: 64 bytes)
0.026629 seconds (8 allocations: 768 bytes)
-1.5558616674667932e7
-1.555656394773972e7
-1.5556607054798864e7
Iteration 8: ll = -1.5556607054798864e7, reldiff = 0.00012916443094457538
1.901857 seconds (8.49 k allocations: 8.697 MiB, 8.00% gc time)
-1.5556607054798864e7
0.280088 seconds (4 allocations: 64 bytes)
0.000560 seconds (2.66 k allocations: 125.109 KiB)
0.369409 seconds (4 allocations: 64 bytes)
0.028105 seconds (8 allocations: 768 bytes)
-1.5556607054798864e7
0.277509 seconds (4 allocations: 64 bytes)
0.000579 seconds (2.66 k allocations: 125.109 KiB)
0.370149 seconds (4 allocations: 64 bytes)
0.026658 seconds (8 allocations: 768 bytes)
-1.5556607054798864e7
-1.5555272556777712e7
-1.5555028877712639e7
Iteration 9: ll = -1.5555028877712639e7, reldiff = 0.00010144738378141057
1.752803 seconds (8.49 k allocations: 8.697 MiB)
-1.5555028877712639e7
0.275904 seconds (4 allocations: 64 bytes)
0.000563 seconds (2.66 k allocations: 125.109 KiB)
0.368746 seconds (4 allocations: 64 bytes)
0.026281 seconds (8 allocations: 768 bytes)
-1.5555028877712639e7
0.276656 seconds (4 allocations: 64 bytes)
0.000562 seconds (2.66 k allocations: 125.109 KiB)
0.369720 seconds (4 allocations: 64 bytes)
0.026309 seconds (8 allocations: 768 bytes)
-1.5555028877712639e7
-1.5554389948288705e7
-1.5572307162296195e7
Iteration 10: ll = -1.5554389948288705e7, reldiff = 4.107542512179528e-5
1.783213 seconds (8.49 k allocations: 8.697 MiB)
-1.5554389948288705e7
0.314982 seconds (4 allocations: 64 bytes)
0.000567 seconds (2.66 k allocations: 125.109 KiB)
0.367198 seconds (4 allocations: 64 bytes)
0.026479 seconds (8 allocations: 768 bytes)
-1.5554389948288705e7
0.277563 seconds (4 allocations: 64 bytes)
0.000566 seconds (2.66 k allocations: 125.109 KiB)
0.368985 seconds (4 allocations: 64 bytes)
0.026321 seconds (8 allocations: 768 bytes)
-1.5554389948288705e7
-1.5553867013950767e7
-1.5562818039977925e7
Iteration 11: ll = -1.5553867013950767e7, reldiff = 3.361972662873465e-5
1.782861 seconds (8.49 k allocations: 8.697 MiB)
-1.5553867013950767e7
0.277292 seconds (4 allocations: 64 bytes)
0.000597 seconds (2.66 k allocations: 125.109 KiB)
0.369716 seconds (4 allocations: 64 bytes)
0.026243 seconds (8 allocations: 768 bytes)
-1.5553867013950767e7
0.277867 seconds (4 allocations: 64 bytes)
0.000576 seconds (2.66 k allocations: 125.109 KiB)
0.369590 seconds (4 allocations: 64 bytes)
0.026170 seconds (8 allocations: 768 bytes)
-1.5553867013950767e7
-1.5553428107705034e7
-1.5559131012878079e7
Iteration 12: ll = -1.5553428107705034e7, reldiff = 2.8218464600411636e-5
1.748249 seconds (8.49 k allocations: 8.697 MiB)
-1.5553428107705034e7
0.277564 seconds (4 allocations: 64 bytes)
0.000570 seconds (2.66 k allocations: 125.109 KiB)
0.369791 seconds (4 allocations: 64 bytes)
0.026247 seconds (8 allocations: 768 bytes)
-1.5553428107705034e7
0.276816 seconds (4 allocations: 64 bytes)
0.000571 seconds (2.66 k allocations: 125.109 KiB)
0.370342 seconds (4 allocations: 64 bytes)
0.026179 seconds (8 allocations: 768 bytes)
-1.5553428107705034e7
-1.5553067050573548e7
-1.5554525394768834e7
Iteration 13: ll = -1.5553067050573548e7, reldiff = 2.3213990445434272e-5
1.748259 seconds (8.49 k allocations: 8.697 MiB)
-1.5553067050573548e7
0.277551 seconds (4 allocations: 64 bytes)
0.001085 seconds (2.66 k allocations: 125.109 KiB)
0.371064 seconds (4 allocations: 64 bytes)
0.026033 seconds (8 allocations: 768 bytes)
-1.5553067050573548e7
0.277290 seconds (4 allocations: 64 bytes)
0.000573 seconds (2.66 k allocations: 125.109 KiB)
0.376844 seconds (4 allocations: 64 bytes)
0.026263 seconds (8 allocations: 768 bytes)
-1.5553067050573548e7
-1.555278497410975e7
-1.5553105606121972e7
Iteration 14: ll = -1.555278497410975e7, reldiff = 1.8136388332978923e-5
1.756941 seconds (8.49 k allocations: 8.713 MiB)
-1.555278497410975e7
0.284532 seconds (4 allocations: 64 bytes)
0.000573 seconds (2.66 k allocations: 125.109 KiB)
0.367819 seconds (4 allocations: 64 bytes)
0.026059 seconds (8 allocations: 768 bytes)
-1.555278497410975e7
0.276429 seconds (4 allocations: 64 bytes)
0.000572 seconds (2.66 k allocations: 125.109 KiB)
0.369100 seconds (4 allocations: 64 bytes)
0.026108 seconds (8 allocations: 768 bytes)
-1.555278497410975e7
-1.5552595414835572e7
-1.5552639211087858e7
Iteration 15: ll = -1.5552639211087858e7, reldiff = 9.372149241089872e-6
1.750865 seconds (8.49 k allocations: 8.697 MiB)
-1.5552639211087858e7
0.277442 seconds (4 allocations: 64 bytes)
0.000793 seconds (2.66 k allocations: 125.109 KiB)
0.371618 seconds (4 allocations: 64 bytes)
0.025982 seconds (8 allocations: 768 bytes)
-1.5552639211087858e7
0.276378 seconds (4 allocations: 64 bytes)
0.000678 seconds (2.66 k allocations: 125.109 KiB)
0.372626 seconds (4 allocations: 64 bytes)
0.026436 seconds (8 allocations: 768 bytes)
-1.5552639211087858e7
-1.5552311626407882e7
-1.5552328125458859e7
Iteration 16: ll = -1.5552328125458859e7, reldiff = 2.000211184591851e-5
1.756623 seconds (8.60 k allocations: 8.754 MiB)
-1.5552328125458859e7
0.277391 seconds (4 allocations: 64 bytes)
0.000688 seconds (2.66 k allocations: 125.109 KiB)
0.367031 seconds (4 allocations: 64 bytes)
0.026239 seconds (8 allocations: 768 bytes)
-1.5552328125458859e7
0.279845 seconds (4 allocations: 64 bytes)
0.000681 seconds (2.66 k allocations: 125.109 KiB)
0.369247 seconds (4 allocations: 64 bytes)
0.026166 seconds (8 allocations: 768 bytes)
-1.5552328125458859e7
-1.5552266154130427e7
-1.5552259885576095e7
Iteration 17: ll = -1.5552259885576095e7, reldiff = 4.387759968346335e-6
1.772075 seconds (8.49 k allocations: 8.699 MiB, 0.94% gc time)
-1.5552259885576095e7
0.279333 seconds (4 allocations: 64 bytes)
0.000689 seconds (2.66 k allocations: 125.109 KiB)
0.367916 seconds (4 allocations: 64 bytes)
0.026056 seconds (8 allocations: 768 bytes)
-1.5552259885576095e7
0.276034 seconds (4 allocations: 64 bytes)
0.000681 seconds (2.66 k allocations: 125.109 KiB)
0.370069 seconds (4 allocations: 64 bytes)
0.026190 seconds (8 allocations: 768 bytes)
-1.5552259885576095e7
-1.5552235725528445e7
-1.5552230063723285e7
Iteration 18: ll = -1.5552230063723285e7, reldiff = 1.917525364808176e-6
1.743838 seconds (8.49 k allocations: 8.697 MiB)
-1.5552230063723285e7
0.280163 seconds (4 allocations: 64 bytes)
0.000682 seconds (2.66 k allocations: 125.109 KiB)
0.367957 seconds (4 allocations: 64 bytes)
0.026116 seconds (8 allocations: 768 bytes)
-1.5552230063723285e7
0.275350 seconds (4 allocations: 64 bytes)
0.000582 seconds (2.66 k allocations: 125.109 KiB)
0.371504 seconds (4 allocations: 64 bytes)
0.026176 seconds (8 allocations: 768 bytes)
-1.5552230063723285e7
-1.555222164931152e7
-1.5552216346354242e7
Iteration 19: ll = -1.5552216346354242e7, reldiff = 8.820194265473032e-7
1.840729 seconds (8.49 k allocations: 8.701 MiB)
-1.5552216346354242e7
0.280761 seconds (4 allocations: 64 bytes)
0.000586 seconds (2.66 k allocations: 125.109 KiB)
0.369551 seconds (4 allocations: 64 bytes)
0.026236 seconds (8 allocations: 768 bytes)
-1.5552216346354242e7
0.277313 seconds (4 allocations: 64 bytes)
0.000580 seconds (2.66 k allocations: 125.109 KiB)
0.368692 seconds (4 allocations: 64 bytes)
0.026159 seconds (8 allocations: 768 bytes)
-1.5552216346354242e7
-1.5552210406862404e7
-1.5552207512250777e7
Iteration 20: ll = -1.5552207512250777e7, reldiff = 5.680285863018589e-7
1.746993 seconds (8.49 k allocations: 8.697 MiB)
-1.5552207512250777e7
0.277832 seconds (4 allocations: 64 bytes)
0.000609 seconds (2.66 k allocations: 125.109 KiB)
0.382785 seconds (4 allocations: 64 bytes)
0.026582 seconds (8 allocations: 768 bytes)
-1.5552207512250777e7
0.296152 seconds (4 allocations: 64 bytes)
0.000590 seconds (2.66 k allocations: 125.109 KiB)
0.384742 seconds (4 allocations: 64 bytes)
0.026081 seconds (8 allocations: 768 bytes)
-1.5552207512250777e7
-1.5552204951377708e7
-1.5552200611477997e7
Iteration 21: ll = -1.5552200611477997e7, reldiff = 4.437166090244169e-7
1.793417 seconds (8.49 k allocations: 8.697 MiB)
-1.5552200611477997e7
0.278324 seconds (4 allocations: 64 bytes)
0.000580 seconds (2.66 k allocations: 125.109 KiB)
0.369446 seconds (4 allocations: 64 bytes)
0.025987 seconds (8 allocations: 768 bytes)
-1.5552200611477997e7
0.277572 seconds (4 allocations: 64 bytes)
0.000581 seconds (2.66 k allocations: 125.109 KiB)
0.399638 seconds (4 allocations: 64 bytes)
0.026015 seconds (8 allocations: 768 bytes)
-1.5552200611477997e7
-1.5552198775938418e7
-1.5552197336867832e7
Iteration 22: ll = -1.5552197336867832e7, reldiff = 2.1055606517124269e-7
1.777111 seconds (8.49 k allocations: 8.697 MiB)
-1.5552197336867832e7
0.278276 seconds (4 allocations: 64 bytes)
0.000583 seconds (2.66 k allocations: 125.109 KiB)
0.369680 seconds (4 allocations: 64 bytes)
0.026021 seconds (8 allocations: 768 bytes)
-1.5552197336867832e7
0.282055 seconds (4 allocations: 64 bytes)
0.000581 seconds (2.66 k allocations: 125.109 KiB)
0.369828 seconds (4 allocations: 64 bytes)
0.026026 seconds (8 allocations: 768 bytes)
-1.5552197336867832e7
-1.5552196340740334e7
-1.5552194868097581e7
Iteration 23: ll = -1.5552194868097581e7, reldiff = 1.587409288349003e-7
1.750541 seconds (8.49 k allocations: 8.705 MiB)
-1.5552194868097581e7
0.286798 seconds (4 allocations: 64 bytes)
0.000606 seconds (2.66 k allocations: 125.109 KiB)
0.369022 seconds (4 allocations: 64 bytes)
0.025984 seconds (8 allocations: 768 bytes)
-1.5552194868097581e7
0.276643 seconds (4 allocations: 64 bytes)
0.000578 seconds (2.66 k allocations: 125.109 KiB)
0.371678 seconds (4 allocations: 64 bytes)
0.026030 seconds (8 allocations: 768 bytes)
-1.5552194868097581e7
-1.5552194288014418e7
-1.5552193929721253e7
Iteration 24: ll = -1.5552193929721253e7, reldiff = 6.033722802055984e-8
55.143828 seconds (24.93 M allocations: 1.403 GiB, 1.45% gc time, 22.49% compilation time)
(AdmixData{Float64}(379, 54051, 4, 3, true, [0.07097194246589222 0.03448150055692116 … 0.99999 0.9903852642383892; 0.41754050336181514 0.7462395931384954 … 0.9939184541764243 0.9207298826668833; 1.0e-5 0.21926890630458337 … 0.9817535223439229 0.99999; 0.5114775541722926 1.0e-5 … 0.9692260172250666 0.99999], [0.07075723653349945 0.03486513012092069 … 0.99999 0.9902814082751056; 0.41762319843677725 0.7438524827975902 … 0.9939080061760321 0.9206403753110268; 1.0e-5 0.2212723870814891 … 0.981798541025298 0.99999; 0.5116095650297232 1.0e-5 … 0.9692097179053448 0.99999], [0.0707761786648089 0.034789786470223884 … 0.99999 0.9903072106563765; 0.41760673781774055 0.7444353552668401 … 0.9939107258213955 0.9206600600274841; 1.0e-5 0.22076485826293593 … 0.9817883444261288 0.99999; 0.5116070835174504 1.0e-5 … 0.9692129945070692 0.99999], [0.07097194246589222 0.03448150055692116 … 0.99999 0.9903852642383892; 0.41754050336181514 0.7462395931384954 … 0.9939184541764243 0.9207298826668833; 1.0e-5 0.21926890630458337 … 0.9817535223439229 0.99999; 0.5114775541722926 1.0e-5 … 0.9692260172250666 0.99999], [0.07097194246589222, 0.41754050336181514, 1.0e-5, 0.5114775541722926, 0.03448150055692116, 0.7462395931384954, 0.21926890630458337, 1.0e-5, 0.08018092101420532, 0.7144266384362248 … 0.9479365586807617, 0.9685993514983139, 0.99999, 0.9939184541764243, 0.9817535223439229, 0.9692260172250666, 0.9903852642383892, 0.9207298826668833, 0.99999, 0.99999], [0.07075723653349945, 0.41762319843677725, 1.0e-5, 0.5116095650297232, 0.03486513012092069, 0.7438524827975902, 0.2212723870814891, 1.0e-5, 0.08017037747345482, 0.7128118897135658 … 0.9479592824854917, 0.9686421564528299, 0.99999, 0.9939080061760321, 0.981798541025298, 0.9692097179053448, 0.9902814082751056, 0.9206403753110268, 0.99999, 0.99999], [0.0707761786648089, 0.41760673781774055, 1.0e-5, 0.5116070835174504, 0.034789786470223884, 0.7444353552668401, 0.22076485826293593, 1.0e-5, 0.08028380357696328, 0.7130294409704403 … 0.9479524409541661, 0.9686249917676314, 0.99999, 0.9939107258213955, 0.9817883444261288, 0.9692129945070692, 0.9903072106563765, 0.9206600600274841, 0.99999, 0.99999], [0.07097194246589222, 0.41754050336181514, 1.0e-5, 0.5114775541722926, 0.03448150055692116, 0.7462395931384954, 0.21926890630458337, 1.0e-5, 0.08018092101420532, 0.7144266384362248 … 0.9479365586807617, 0.9685993514983139, 0.99999, 0.9939184541764243, 0.9817535223439229, 0.9692260172250666, 0.9903852642383892, 0.9207298826668833, 0.99999, 0.99999], [0.0, 0.0, 0.0, 0.0, 0.0, 0.0, 0.0, 0.0, 0.0, 0.0 … 0.0, 0.0, 0.0, 0.0, 0.0, 0.0, 0.0, 0.0, 0.0, 0.0], [0.0, 0.0, 0.0, 0.0, 0.0, 0.0, 0.0, 0.0, 0.0, 0.0 … 0.0, 0.0, 0.0, 0.0, 0.0, 0.0, 0.0, 0.0, 0.0, 0.0], [0.07097194246589222 0.03448150055692116 … 1.0e-5 1.0e-5; 0.41754050336181514 0.7462395931384954 … 1.0e-5 1.0e-5; 1.0e-5 0.21926890630458337 … 1.0e-5 1.0e-5; 0.5114775541722926 1.0e-5 … 0.9999699999999999 0.9999699999999999], [0.07075723653349945 0.03486513012092069 … 1.0e-5 1.0e-5; 0.41762319843677725 0.7438524827975902 … 1.0e-5 1.0e-5; 1.0e-5 0.2212723870814891 … 1.0e-5 1.0e-5; 0.5116095650297232 1.0e-5 … 0.9999699999999999 0.9999699999999999], [0.0707761786648089 0.034789786470223884 … 1.0e-5 1.0e-5; 0.41760673781774055 0.7444353552668401 … 1.0e-5 1.0e-5; 1.0e-5 0.22076485826293593 … 1.0e-5 1.0e-5; 0.5116070835174504 1.0e-5 … 0.9999699999999999 0.9999699999999999], [0.07097194246589222 0.03448150055692116 … 1.0e-5 1.0e-5; 0.41754050336181514 0.7462395931384954 … 1.0e-5 1.0e-5; 1.0e-5 0.21926890630458337 … 1.0e-5 1.0e-5; 0.5114775541722926 1.0e-5 … 0.9999699999999999 0.9999699999999999], [0.8417036781784455 0.9719130016593888 … 0.99999 0.9903852642383892; 0.9235910956222408 0.9920924958274663 … 0.9939184541764243 0.9207298826668833; 0.91779284272664 0.9829380093686094 … 0.9817535223439229 0.99999; 0.9129060765064565 0.9997632898448726 … 0.9692260172250666 0.99999], [0.8417308884182984 0.9719333669762293 … 0.99999 0.9902814082751056; 0.9237319202056826 0.9921163128465725 … 0.9939080061760321 0.9206403753110268; 0.9176758088904347 0.9829477937654668 … 0.981798541025298 0.99999; 0.9128799686593018 0.9997248131649172 … 0.9692097179053448 0.99999], [0.841690966855962 0.9719287572433678 … 0.99999 0.9903072106563765; 0.9236789074293282 0.9921107074985339 … 0.9939107258213955 0.9206600600274841; 0.9177364888509721 0.9829464228879098 … 0.9817883444261288 0.99999; 0.9129052987772184 0.9997319203980494 … 0.9692129945070692 0.99999], [0.8417036781784455 0.9719130016593888 … 0.99999 0.9903852642383892; 0.9235910956222408 0.9920924958274663 … 0.9939184541764243 0.9207298826668833; 0.91779284272664 0.9829380093686094 … 0.9817535223439229 0.99999; 0.9129060765064565 0.9997632898448726 … 0.9692260172250666 0.99999], [112429.32359917002 108324.98954890843 108500.55874838612 107321.65837279192; 108324.98954890843 109873.97275634346 107801.78465825132 106624.68476500905; 108500.55874838612 107801.78465825132 111230.48889015679 108062.85088666201; 107321.65837279192 106624.68476500905 108062.85088666201 109415.85315484426;;; 113156.90784154511 107685.88282075372 108700.2019605354 108747.49005223403; 107685.88282075372 108436.25957312505 107047.39093836695 107098.21700861708; 108700.2019605354 107047.39093836695 111542.06205844568 109548.63620848597; 108747.49005223403 107098.21700861708 109548.63620848597 111208.83072976909;;; 112308.03142412317 107517.24558495541 108487.70293022148 108716.68538726468; 107517.24558495541 108471.14732477836 107059.13756936864 107220.56178693537; 108487.7029302215 107059.13756936864 111537.15613171147 109478.71640831263; 108716.68538726468 107220.56178693537 109478.71640831263 111355.75193228382;;; … ;;; 113611.68931612682 110080.65040250416 107257.70045606881 108707.17677049637; 110080.65040250416 112995.51987769718 107411.64877388586 108763.28793921293; 107257.70045606881 107411.64877388586 108267.21786953261 107555.8197428564; 108707.17677049637 108763.28793921293 107555.81974285639 110739.47079430547;;; 112904.84798858447 109852.2892163234 108807.81333412504 107518.5263835833; 109852.2892163234 111648.01373136841 108742.1737792436 107454.05957415157; 108807.81333412504 108742.1737792436 110905.85334016576 107791.91612136445; 107518.5263835833 107454.05957415157 107791.91612136445 108102.03082914864;;; 114151.93577995931 110844.7316121083 109990.83498763376 107612.39773994582; 110844.7316121083 112764.8322387724 109840.57607247417 107574.54134033063; 109990.83498763376 109840.57607247417 112402.00967340921 108064.02014068769; 107612.39773994582 107574.54134033063 108064.02014068769 108102.02109867314], [-108102.08881321369 -108101.08225339273 … -107518.61647729724 -107612.51924303739; -108101.98344736466 -108102.76593143288 … -107454.13837713147 -107574.64860548984; -107984.82624843404 -108099.5880300587 … -107791.96692228544 -108064.1005542907; -108102.00351894205 -107697.97033973054 … -108102.01541324455 -108102.01054763237], [924.5741271099636 227.25240605343262 160.09517945686386 115.71526330876449; 227.25240605343257 1732.512599932291 688.5541017787021 339.6747249587286; 160.09517945686386 688.5541017787021 1570.707478695452 267.6595248475699; 115.71526330876449 339.6747249587286 267.6595248475699 1178.0746757891968;;; 4768.153448329896 2586.3242978235135 628.6298851193931 2104.539504392266; 2586.3242978235135 14152.812368293296 2864.630742804071 5534.830341543266; 628.6298851193931 2864.630742804071 10107.367856338271 2885.235009219228; 2104.539504392266 5534.830341543266 2885.235009219228 10558.203131915776;;; 6279.959099555947 998.4064986577184 472.30159200491687 489.69489152367606; 998.4064986577184 2689.138852356804 1077.9498881189143 453.11301014643175; 472.30159200491687 1077.9498881189145 2767.0784548892957 589.8876790539748; 489.69489152367606 453.11301014643175 589.8876790539748 1678.7805484618664;;; … ;;; 1631.311460636115 336.15706054385527 302.53764718806946 281.01944344319566; 336.1570605438553 2240.600708663522 1062.3874895759602 1129.0304343233577; 302.53764718806946 1062.3874895759602 2359.20908542582 452.0190754402239; 281.01944344319566 1129.0304343233577 452.0190754402239 1585.943164837384;;; 9262.734784434671 192.32792457293561 640.2147875336885 4281.532621735132; 192.32792457293561 29718.58058305707 2938.956083808823 34.91871865568121; 640.2147875336884 2938.956083808823 8550.450794983517 1294.7924247800922; 4281.532621735132 34.91871865568121 1294.7924247800922 4353.246261607954;;; 5275.579066366831 1404.022476678692 144.8544174690879 84.65866849531672; 1404.022476678692 2893.8569447678096 1350.0814380376473 1137.6907137204062; 144.8544174690879 1350.0814380376473 2426.02875785844 645.1324357344375; 84.65866849531672 1137.6907137204062 645.1324357344374 1338.7204582833865], [0.03631207419205951 0.022381483177531747 … -16.52354541323669 -0.16577538547253035; 0.050532137525475626 0.05584343156612803 … -0.05289433670461863 -0.11545962378720276; -0.05919690085704499 0.012304994058437657 … 0.06854793421832994 -107.37909057514909; -0.02345570553259435 -0.03035829188345396 … -0.04397166759326865 -65.5267184035896], SubArray{Float64, 1, Matrix{Float64}, Tuple{Base.Slice{Base.OneTo{Int64}}, Int64}, true}[[0.07097194246589222, 0.41754050336181514, 1.0e-5, 0.5114775541722926], [0.03448150055692116, 0.7462395931384954, 0.21926890630458337, 1.0e-5], [0.08018092101420532, 0.7144266384362248, 0.20538244054956978, 1.0e-5], [0.11632077555134018, 0.36853675824615245, 1.0000000000055512e-5, 0.5151324662025073], [1.0e-5, 0.974944905318529, 0.025035094681470978, 1.0e-5], [0.005807777302670947, 0.856662436189591, 0.137519786507738, 1.0000000000055512e-5], [1.0e-5, 0.6329044385157624, 0.3670755614842376, 1.0e-5], [0.17770918465002392, 0.6115257239486562, 0.2107550914013197, 1.0e-5], [0.05705494573765796, 0.5054313809923692, 1.0e-5, 0.4375036732699729], [0.026642645032760592, 0.935823075121205, 0.03752427984603438, 1.0000000000055512e-5] … [1.0e-5, 1.0e-5, 1.0e-5, 0.9999699999999999], [1.0e-5, 1.0e-5, 0.3967512591287673, 0.6032287408712326], [0.02454209264096739, 0.2013724991853736, 0.7740754081736589, 1.0e-5], [1.0e-5, 1.0e-5, 1.0e-5, 0.9999699999999999], [1.0000000000055512e-5, 0.2084490281708426, 0.7915309718291573, 1.0000000000055512e-5], [0.04935553945217813, 1.0e-5, 0.5341517884758102, 0.4164826720720115], [1.0e-5, 0.19909881536078572, 0.4688920647547747, 0.33199911988443964], [0.09811802806306286, 0.07930050718129622, 0.8225714647556408, 1.0000000000083268e-5], [1.0e-5, 1.0e-5, 1.0e-5, 0.9999699999999999], [1.0e-5, 1.0e-5, 1.0e-5, 0.9999699999999999]], SubArray{Float64, 1, Matrix{Float64}, Tuple{Base.Slice{Base.OneTo{Int64}}, Int64}, true}[[0.07075723653349945, 0.41762319843677725, 1.0e-5, 0.5116095650297232], [0.03486513012092069, 0.7438524827975902, 0.2212723870814891, 1.0e-5], [0.08017037747345482, 0.7128118897135658, 0.20700773281297916, 1.0e-5], [0.11598873061362942, 0.3688546567006869, 1.0e-5, 0.5151466126856836], [1.0e-5, 0.9713631129429602, 0.028616887057039804, 1.0e-5], [0.006068636897630073, 0.854336837500445, 0.13958452560192494, 1.0e-5], [1.0e-5, 0.631190662255502, 0.3687893377444979, 1.0e-5], [0.17812156197868603, 0.6095970194611892, 0.21227141856012466, 1.0e-5], [0.05714253180678263, 0.5047449621694039, 1.0e-5, 0.4381025060238134], [0.02883022923620386, 0.9286854208184038, 0.04247434994539219, 1.0e-5] … [1.0e-5, 1.0e-5, 1.0e-5, 0.9999699999999999], [1.0e-5, 1.0e-5, 0.3966804462769348, 0.6032995537230652], [0.024850351560056876, 0.19994871739243403, 0.775190931047509, 1.0e-5], [1.0e-5, 1.0e-5, 1.0e-5, 0.9999699999999999], [1.0e-5, 0.20759714100354, 0.7923828589964599, 1.0e-5], [0.04937874968182977, 1.0e-5, 0.5341343821799872, 0.416476868138183], [1.0e-5, 0.19883802393724676, 0.4691310311769159, 0.3320209448858374], [0.0987833535268654, 0.07699509686296713, 0.8242115496101674, 1.0e-5], [1.0e-5, 1.0e-5, 1.0e-5, 0.9999699999999999], [1.0e-5, 1.0e-5, 1.0e-5, 0.9999699999999999]], SubArray{Float64, 1, Matrix{Float64}, Tuple{Base.Slice{Base.OneTo{Int64}}, Int64}, true}[[0.07097194246589222, 0.41754050336181514, 1.0e-5, 0.5114775541722926], [0.03448150055692116, 0.7462395931384954, 0.21926890630458337, 1.0e-5], [0.08018092101420532, 0.7144266384362248, 0.20538244054956978, 1.0e-5], [0.11632077555134018, 0.36853675824615245, 1.0000000000055512e-5, 0.5151324662025073], [1.0e-5, 0.974944905318529, 0.025035094681470978, 1.0e-5], [0.005807777302670947, 0.856662436189591, 0.137519786507738, 1.0000000000055512e-5], [1.0e-5, 0.6329044385157624, 0.3670755614842376, 1.0e-5], [0.17770918465002392, 0.6115257239486562, 0.2107550914013197, 1.0e-5], [0.05705494573765796, 0.5054313809923692, 1.0e-5, 0.4375036732699729], [0.026642645032760592, 0.935823075121205, 0.03752427984603438, 1.0000000000055512e-5] … [1.0e-5, 1.0e-5, 1.0e-5, 0.9999699999999999], [1.0e-5, 1.0e-5, 0.3967512591287673, 0.6032287408712326], [0.02454209264096739, 0.2013724991853736, 0.7740754081736589, 1.0e-5], [1.0e-5, 1.0e-5, 1.0e-5, 0.9999699999999999], [1.0000000000055512e-5, 0.2084490281708426, 0.7915309718291573, 1.0000000000055512e-5], [0.04935553945217813, 1.0e-5, 0.5341517884758102, 0.4164826720720115], [1.0e-5, 0.19909881536078572, 0.4688920647547747, 0.33199911988443964], [0.09811802806306286, 0.07930050718129622, 0.8225714647556408, 1.0000000000083268e-5], [1.0e-5, 1.0e-5, 1.0e-5, 0.9999699999999999], [1.0e-5, 1.0e-5, 1.0e-5, 0.9999699999999999]], SubArray{Float64, 1, Matrix{Float64}, Tuple{Base.Slice{Base.OneTo{Int64}}, Int64}, true}[[0.8417036781784455, 0.9235910956222408, 0.91779284272664, 0.9129060765064565], [0.9719130016593888, 0.9920924958274663, 0.9829380093686094, 0.9997632898448726], [0.9915180800008472, 0.9392051926830348, 0.9593727624537517, 0.935531397359741], [0.5460568839367185, 0.47168935139816404, 0.5656330896981703, 0.45669235846612516], [0.7261583819137465, 0.691390905524685, 0.666198216932041, 0.6239379823851668], [0.8565411071858399, 0.9205265143270079, 0.9164140274699304, 0.9364270052423508], [0.6595924061529945, 0.6528518334406135, 0.6365491200564127, 0.5391835503089367], [0.9579078014880861, 0.9373407852283834, 0.9590424848836159, 0.9348729848753671], [0.9592145462442412, 0.9279632971710615, 0.9562201778657994, 0.9385245880678953], [0.8327041355065217, 0.9048774985294098, 0.9010071125939634, 0.8956880541752188] … [0.6756704584235717, 0.7314549617306517, 0.8340600114648455, 0.7513361730841577], [0.746784293163343, 0.691617785276156, 0.9007057137392552, 0.8208805519516201], [0.8115350059973747, 0.8586501627604565, 0.9391172968658348, 0.8775937175036945], [0.840948246262924, 0.800505282567632, 0.8852047370256159, 0.9780599290375627], [0.9356703995236214, 0.866093814271617, 0.9314922044160869, 0.99999], [0.9251428631025986, 0.8555205017588279, 0.9367611058889435, 0.99999], [0.851459893740546, 0.7789118270663608, 0.8798011776415458, 0.9880406588401981], [0.9201564359347043, 0.9427045029667565, 0.9479365586807617, 0.9685993514983139], [0.99999, 0.9939184541764243, 0.9817535223439229, 0.9692260172250666], [0.9903852642383892, 0.9207298826668833, 0.99999, 0.99999]], SubArray{Float64, 1, Matrix{Float64}, Tuple{Base.Slice{Base.OneTo{Int64}}, Int64}, true}[[0.8417308884182984, 0.9237319202056826, 0.9176758088904347, 0.9128799686593018], [0.9719333669762293, 0.9921163128465725, 0.9829477937654668, 0.9997248131649172], [0.9915378028060944, 0.9391058490331118, 0.9593574051503967, 0.9356052120220393], [0.5460666204954089, 0.47155804092937803, 0.565508067879408, 0.4567741765774998], [0.7262142717138594, 0.6912287188179169, 0.6662600119917078, 0.624090119345088], [0.8565783393796349, 0.9205736021876723, 0.9163574980741109, 0.9364346903358332], [0.6596255930906725, 0.6527885747776728, 0.6365353109878313, 0.5393069104772885], [0.957884064224064, 0.937350345287009, 0.9590070022595227, 0.9348661885955184], [0.9591862111878382, 0.9279692192990981, 0.9561730294782625, 0.9385217770001347], [0.8327590788222605, 0.9049088448357865, 0.900922473418964, 0.8957438058281848] … [0.6756732049422645, 0.7313177183047539, 0.8340165589155497, 0.7513526598164667], [0.7468481276884283, 0.6912146548167456, 0.900595911237113, 0.8209839218356867], [0.8116160570015214, 0.8584870823670131, 0.9390497280468919, 0.8776512059643605], [0.8409705342939152, 0.8002549962754852, 0.8851973308760203, 0.9781811183941158], [0.9356595335105431, 0.8659892987794476, 0.9314625058198851, 0.99999], [0.9251380776944745, 0.8553966251733212, 0.9367172842854734, 0.99999], [0.8514854440000209, 0.7786587539071353, 0.8797496883906782, 0.9881643094013802], [0.9201776168035013, 0.9426370315872961, 0.9479592824854917, 0.9686421564528299], [0.99999, 0.9939080061760321, 0.981798541025298, 0.9692097179053448], [0.9902814082751056, 0.9206403753110268, 0.99999, 0.99999]], SubArray{Float64, 1, Matrix{Float64}, Tuple{Base.Slice{Base.OneTo{Int64}}, Int64}, true}[[0.8417036781784455, 0.9235910956222408, 0.91779284272664, 0.9129060765064565], [0.9719130016593888, 0.9920924958274663, 0.9829380093686094, 0.9997632898448726], [0.9915180800008472, 0.9392051926830348, 0.9593727624537517, 0.935531397359741], [0.5460568839367185, 0.47168935139816404, 0.5656330896981703, 0.45669235846612516], [0.7261583819137465, 0.691390905524685, 0.666198216932041, 0.6239379823851668], [0.8565411071858399, 0.9205265143270079, 0.9164140274699304, 0.9364270052423508], [0.6595924061529945, 0.6528518334406135, 0.6365491200564127, 0.5391835503089367], [0.9579078014880861, 0.9373407852283834, 0.9590424848836159, 0.9348729848753671], [0.9592145462442412, 0.9279632971710615, 0.9562201778657994, 0.9385245880678953], [0.8327041355065217, 0.9048774985294098, 0.9010071125939634, 0.8956880541752188] … [0.6756704584235717, 0.7314549617306517, 0.8340600114648455, 0.7513361730841577], [0.746784293163343, 0.691617785276156, 0.9007057137392552, 0.8208805519516201], [0.8115350059973747, 0.8586501627604565, 0.9391172968658348, 0.8775937175036945], [0.840948246262924, 0.800505282567632, 0.8852047370256159, 0.9780599290375627], [0.9356703995236214, 0.866093814271617, 0.9314922044160869, 0.99999], [0.9251428631025986, 0.8555205017588279, 0.9367611058889435, 0.99999], [0.851459893740546, 0.7789118270663608, 0.8798011776415458, 0.9880406588401981], [0.9201564359347043, 0.9427045029667565, 0.9479365586807617, 0.9685993514983139], [0.99999, 0.9939184541764243, 0.9817535223439229, 0.9692260172250666], [0.9903852642383892, 0.9207298826668833, 0.99999, 0.99999]], SubArray{Float64, 2, Array{Float64, 3}, Tuple{Base.Slice{Base.OneTo{Int64}}, Base.Slice{Base.OneTo{Int64}}, Int64}, true}[[112429.32359917002 108324.98954890843 108500.55874838612 107321.65837279192; 108324.98954890843 109873.97275634346 107801.78465825132 106624.68476500905; 108500.55874838612 107801.78465825132 111230.48889015679 108062.85088666201; 107321.65837279192 106624.68476500905 108062.85088666201 109415.85315484426], [113156.90784154511 107685.88282075372 108700.2019605354 108747.49005223403; 107685.88282075372 108436.25957312505 107047.39093836695 107098.21700861708; 108700.2019605354 107047.39093836695 111542.06205844568 109548.63620848597; 108747.49005223403 107098.21700861708 109548.63620848597 111208.83072976909], [112308.03142412317 107517.24558495541 108487.70293022148 108716.68538726468; 107517.24558495541 108471.14732477836 107059.13756936864 107220.56178693537; 108487.7029302215 107059.13756936864 111537.15613171147 109478.71640831263; 108716.68538726468 107220.56178693537 109478.71640831263 111355.75193228382], [112239.46671552368 108242.06907723553 108550.08009652735 107070.56746775005; 108242.06907723552 109953.22243309986 107856.87186511658 106744.47710962991; 108550.08009652735 107856.87186511658 111392.42406952858 108077.08567763619; 107070.56746775005 106744.47710962991 108077.08567763619 109306.49146875324], [113106.42060693474 107855.8479790398 109649.35517063465 109354.36295709205; 107855.8479790398 108106.9895734995 107938.04115721623 107606.17802904946; 109649.35517063465 107938.04115721623 113498.34008650856 111046.13742248678; 109354.36295709205 107606.17802904946 111046.13742248678 112166.95961757172], [112985.3688008684 107839.57677823436 109486.74542535232 109698.12871292105; 107839.57677823436 108224.64199896785 107366.56411450148 107620.12343758611; 109486.74542535232 107366.56411450148 112520.33482003838 110453.84835244845; 109698.12871292105 107620.12343758611 110453.84835244845 129833.56079129408], [112500.0252252583 108058.33153839948 108037.86922013585 108394.7487783574; 108058.33153839948 108839.43335672998 106842.22644847479 107300.72320930884; 108037.86922013585 106842.22644847479 110254.13841129624 108741.57273523886; 108394.74877835742 107300.72320930884 108741.57273523886 111051.82492157437], [111111.91314175083 107287.57887795389 107913.05041311138 108060.2623950258; 107287.57887795389 108685.30808919617 107113.60013253857 107257.86156625516; 107913.05041311138 107113.60013253857 111091.31909002071 109397.54174970483; 108060.2623950258 107257.86156625516 109397.54174970483 111435.56322715625], [112462.18985731964 108212.6137940057 108748.22052833323 107405.95187965578; 108212.6137940057 109337.1273311108 107638.3838486113 106665.49898055702; 108748.22052833323 107638.3838486113 112011.63179487186 108482.37134474129; 107405.95187965578 106665.49898055702 108482.37134474129 109846.7138936029], [113601.27010983805 107849.70267820818 109759.21993710937 109448.75429964192; 107849.70267820818 108124.03089502535 107804.19564437009 107531.91572238208; 109759.21993710937 107804.19564437009 113302.04721723696 110912.46870600317; 109448.75429964192 107531.91572238208 110912.46870600317 112260.76899609643] … [113797.6686591533 110344.82072796888 109018.26735377399 107574.18681414625; 110344.82072796891 112231.48951242777 108940.81615440211 107548.72133117607; 109018.26735377399 108940.81615440211 111115.8271952366 107879.25544822127; 107574.18681414625 107548.72133117607 107879.25544822127 108102.02607542684], [114360.35812794034 110622.28099352628 108531.98256567089 107785.83096222022; 110622.28099352628 113089.08907724683 108491.42747498136 107644.1849635769; 108531.98256567089 108491.42747498136 109748.56145933064 107019.43786092225; 107785.83096222022 107644.1849635769 107019.43786092225 108813.75819344888], [115432.31722747313 110158.06761393118 107337.47732338728 108982.55267993452; 110158.06761393118 111611.6726574322 107132.42520862664 108497.60455282792; 107337.47732338728 107132.42520862664 108376.16561354336 107610.7717238049; 108982.55267993452 108497.60455282792 107610.7717238049 110799.8458217023], [113526.55451490029 110564.99050444039 109162.4306444606 107765.61604330955; 110564.99050444039 112637.69868627921 109098.39611370387 107787.74314891658; 109162.4306444606 109098.39611370387 111039.20220254558 107752.45775518261; 107765.61604330955 107787.74314891658 107752.45775518261 108102.0200020675], [114003.4215749945 109706.9062625 107286.55401906556 108386.84415451944; 109706.9062625 111649.1057775992 107174.25220959245 108375.5199017655; 107286.55401906556 107174.25220959245 108344.67249786768 107626.67994724773; 108386.84415451944 108375.5199017655 107626.67994724773 112125.7300429555], [113388.8803411852 110005.83237210888 107775.07197910821 107893.75553967796; 110005.83237210888 112399.5501116194 107946.11110865443 107874.94765921326; 107775.07197910821 107946.11110865443 108966.50406561652 107032.11120618941; 107893.75553967796 107874.94765921326 107032.11120618941 109498.81766728371], [112377.10264117553 109046.560495385 107447.82002888172 107403.8019881487; 109046.560495385 111192.7959992327 107369.78644277585 107286.64214744967; 107447.82002888172 107369.78644277585 109067.07679814361 107176.3286535458; 107403.8019881487 107286.64214744967 107176.3286535458 109898.45881279373], [113611.68931612682 110080.65040250416 107257.70045606881 108707.17677049637; 110080.65040250416 112995.51987769718 107411.64877388586 108763.28793921293; 107257.70045606881 107411.64877388586 108267.21786953261 107555.8197428564; 108707.17677049637 108763.28793921293 107555.81974285639 110739.47079430547], [112904.84798858447 109852.2892163234 108807.81333412504 107518.5263835833; 109852.2892163234 111648.01373136841 108742.1737792436 107454.05957415157; 108807.81333412504 108742.1737792436 110905.85334016576 107791.91612136445; 107518.5263835833 107454.05957415157 107791.91612136445 108102.03082914864], [114151.93577995931 110844.7316121083 109990.83498763376 107612.39773994582; 110844.7316121083 112764.8322387724 109840.57607247417 107574.54134033063; 109990.83498763376 109840.57607247417 112402.00967340921 108064.02014068769; 107612.39773994582 107574.54134033063 108064.02014068769 108102.02109867314]], SubArray{Float64, 1, Matrix{Float64}, Tuple{Base.Slice{Base.OneTo{Int64}}, Int64}, true}[[-108102.08881321369, -108101.98344736466, -107984.82624843404, -108102.00351894205], [-108101.08225339273, -108102.76593143288, -108099.5880300587, -107697.97033973054], [-108102.22886330938, -108102.36321819141, -108100.67485946715, -107808.00340113461], [-108102.23014928652, -108101.75267960558, -108050.75396303699, -108102.1262611736], [-107907.24006265373, -108102.14727622095, -108097.20779778186, -107704.68204815735], [-108100.74256128413, -108102.52473834292, -108098.84823687006, -108028.5003793744], [-108050.83303470758, -108102.86154557625, -108100.53415157701, -107832.14160303895], [-108101.55272742211, -108102.6994268794, -108100.3783503928, -107855.02118003159], [-108102.05007924567, -108102.40571757284, -108071.59949596935, -108101.52672822447], [-108096.64318052726, -108102.53123413575, -108094.10819727638, -107730.81269694115] … [-107574.2911961091, -107548.81004080015, -107879.30981966468, -108102.01303628046], [-108081.90882303941, -107980.35373110719, -108102.0576732268, -108101.96442817365], [-108102.6268024637, -108103.24692015903, -108101.66189170057, -107822.21394027401], [-107765.71561458506, -107787.832527475, -107752.51818173948, -108102.00999963666], [-107789.09039660082, -108103.25635593763, -108101.6788311389, -107782.1895730179], [-108101.72608219048, -108018.22417317769, -108102.03255508128, -108101.99273594472], [-107751.14480537978, -108102.35722871934, -108101.80675975636, -108102.06967299628], [-108102.73658326604, -108105.24591822934, -108101.61261461335, -107762.55561840134], [-107518.61647729724, -107454.13837713147, -107791.96692228544, -108102.01541324455], [-107612.51924303739, -107574.64860548984, -108064.1005542907, -108102.01054763237]], SubArray{Float64, 2, Array{Float64, 3}, Tuple{Base.Slice{Base.OneTo{Int64}}, Base.Slice{Base.OneTo{Int64}}, Int64}, true}[[924.5741271099636 227.25240605343262 160.09517945686386 115.71526330876449; 227.25240605343257 1732.512599932291 688.5541017787021 339.6747249587286; 160.09517945686386 688.5541017787021 1570.707478695452 267.6595248475699; 115.71526330876449 339.6747249587286 267.6595248475699 1178.0746757891968], [4768.153448329896 2586.3242978235135 628.6298851193931 2104.539504392266; 2586.3242978235135 14152.812368293296 2864.630742804071 5534.830341543266; 628.6298851193931 2864.630742804071 10107.367856338271 2885.235009219228; 2104.539504392266 5534.830341543266 2885.235009219228 10558.203131915776], [6279.959099555947 998.4064986577184 472.30159200491687 489.69489152367606; 998.4064986577184 2689.138852356804 1077.9498881189143 453.11301014643175; 472.30159200491687 1077.9498881189145 2767.0784548892957 589.8876790539748; 489.69489152367606 453.11301014643175 589.8876790539748 1678.7805484618664], [459.24672772190337 87.9738666243315 61.31969423598148 40.5786154945309; 87.9738666243315 510.37971827723754 197.86987536704245 133.80417321596596; 61.31969423598147 197.86987536704245 499.0507518978236 91.38413729900438; 40.5786154945309 133.80417321596596 91.38413729900438 350.39540199131187], [563.8964748434147 104.30557882739748 68.25660110567912 48.726885144557805; 104.30557882739748 595.1445719744926 225.0158994126817 144.94274587164233; 68.25660110567912 225.01589941268165 566.4796694328207 95.85726948044041; 48.726885144557805 144.94274587164233 95.85726948044041 389.5202388295697], [988.6733270446975 257.9468709323089 162.3122866400809 140.37766939995745; 257.9468709323089 1681.3527758432103 644.8051156176427 452.2160648087685; 162.3122866400809 644.8051156176426 1692.8692326863206 215.35996627816206; 140.37766939995745 452.2160648087685 215.35996627816203 1456.7643702186033], [500.40731732570276 96.98430903972941 66.64213583856144 43.83260869026991; 96.98430903972941 551.9816380189432 214.95946998065668 138.53167043756687; 66.64213583856144 214.95946998065668 534.6180087409708 92.50271134576334; 43.83260869026991 138.53167043756687 92.50271134576334 361.9764682940456], [2706.3964013435575 412.7462271027425 455.3847498869713 165.36968429554145; 412.7462271027425 2378.8966025310274 1071.6650134015902 547.6619764912397; 455.3847498869713 1071.6650134015902 2584.4304306495605 441.67647336144046; 165.36968429554148 547.6619764912397 441.6764733614404 1604.6988082838138], [2763.2517256808783 377.0687765999483 434.9711310980846 168.69860851682614; 377.0687765999483 2176.0476486301586 1010.5430463344144 503.4598375150728; 434.97113109808447 1010.5430463344144 2380.333624817995 436.7798767018105; 168.69860851682614 503.4598375150728 436.7798767018105 1654.4798961255965], [853.6167961839351 226.3368418140697 133.664716768385 117.48816721948931; 226.3368418140697 1413.8321671371964 561.5618823115217 296.54305321283255; 133.664716768385 561.5618823115217 1371.349941152869 223.74901883228577; 117.48816721948933 296.54305321283255 223.74901883228577 978.1917507118118] … [533.414850451095 110.16386583991769 87.69546360602304 54.76479661808685; 110.16386583991769 685.6470763809817 274.57751720086856 175.04724269318038; 87.69546360602304 274.57751720086856 773.65496093058 143.55708465231322; 54.76479661808685 175.04724269318038 143.55708465231322 467.4432950329113], [605.7347588289492 117.85327287727469 110.16406149424112 78.0780989302318; 117.85327287727469 695.0211558109091 288.1757004863465 175.38239702134433; 110.16406149424114 288.1757004863465 1029.8981253137217 191.2204681992634; 78.07809893023179 175.38239702134433 191.2204681992634 561.8121870451746], [767.3530685170407 174.04777741050853 165.57819225140977 126.39581990771471; 174.04777741050853 1178.6275253066021 498.5903749760059 292.1509051903901; 165.57819225140977 498.5903749760059 1638.037383790167 251.36232843613382; 126.39581990771471 292.1509051903901 251.36232843613382 772.6129470958479], [902.815736573503 156.08648815443775 118.59443769914365 106.80855118015243; 156.08648815443775 1003.2306549851588 366.37254809346405 443.24026236556716; 118.59443769914365 366.3725480934641 1242.215338265734 365.5086925143014; 106.80855118015245 443.24026236556716 365.5086925143014 793.5666273692071], [1994.8401631250113 249.39907110162815 170.83696185361921 116.19562173224263; 249.39907110162815 1516.7401371056997 549.0348434709191 604.4022563202761; 170.83696185361921 549.0348434709191 2102.2800852077035 860.0868707985828; 116.19562173224263 604.4022563202761 860.0868707985828 1454.3495130897697], [1744.5498117256263 235.4850037719598 160.91234186045597 92.01781450941424; 235.4850037719598 1427.7981907112016 540.2217328711631 521.4745245422888; 160.91234186045597 540.2217328711631 2189.9423563819746 992.4328384842911; 92.01781450941424 521.4745245422888 992.4328384842911 1492.09435819795], [949.7819758486739 148.53155080672877 122.54568287977382 115.31526599245463; 148.53155080672875 944.8883686727771 371.08582798621876 402.7876862341025; 122.54568287977382 371.08582798621876 1156.545549635621 404.79439780397365; 115.31526599245463 402.7876862341025 404.79439780397365 829.8933758221423], [1631.311460636115 336.15706054385527 302.53764718806946 281.01944344319566; 336.1570605438553 2240.600708663522 1062.3874895759602 1129.0304343233577; 302.53764718806946 1062.3874895759602 2359.20908542582 452.0190754402239; 281.01944344319566 1129.0304343233577 452.0190754402239 1585.943164837384], [9262.734784434671 192.32792457293561 640.2147875336885 4281.532621735132; 192.32792457293561 29718.58058305707 2938.956083808823 34.91871865568121; 640.2147875336884 2938.956083808823 8550.450794983517 1294.7924247800922; 4281.532621735132 34.91871865568121 1294.7924247800922 4353.246261607954], [5275.579066366831 1404.022476678692 144.8544174690879 84.65866849531672; 1404.022476678692 2893.8569447678096 1350.0814380376473 1137.6907137204062; 144.8544174690879 1350.0814380376473 2426.02875785844 645.1324357344375; 84.65866849531672 1137.6907137204062 645.1324357344374 1338.7204582833865]], SubArray{Float64, 1, Matrix{Float64}, Tuple{Base.Slice{Base.OneTo{Int64}}, Int64}, true}[[0.03631207419205951, 0.050532137525475626, -0.05919690085704499, -0.02345570553259435], [0.022381483177531747, 0.05584343156612803, 0.012304994058437657, -0.03035829188345396], [-0.004033528899298977, -0.05562870078293125, -0.031594664668368466, 0.026117608307547613], [-0.013937882491945351, -0.017242489070684375, -0.013986217544460278, 0.004197377910870159], [-0.0017265747252316888, -0.013667339271916226, 0.00518976419300432, 0.015935291833042098], [0.00711914061187624, 0.01669532291499687, -0.011596001652783627, 0.008639245899799697], [0.00369567401511485, -0.0014594707768407211, -0.0071396009484230305, 0.009977322338360572], [0.019906146912113343, 0.0019155468612339632, -0.05082548297901113, -0.021610456307453063], [0.013599691528992874, -0.002958827927533658, -0.05496295022887665, -0.02151460623813506], [0.008257653265075826, 0.011207412338922307, -0.021224594148147702, 0.0175430712654574] … [-0.01065191181482672, -0.014391173681816749, -0.018888173899533456, -0.0024329966732121733], [-0.0094370113208333, -0.06217420815871755, -0.04465346246731983, -0.0037311328234324037], [0.004139827799419127, -0.04022837800429224, -0.03801383375258638, -0.0008775074656099946], [-0.0062726909318362445, -0.04447897952980595, -0.007127897734317212, 0.006276372401689656], [-0.018993632447294795, -0.04825147141959052, -0.029367722703220522, -12.671725877801748], [-0.0146557879589031, -0.05414866783823391, -0.03838786873811273, -15.83602821929777], [-0.005765388082796363, -0.04822872254642441, -0.015804994188296284, 0.004818167149197805], [0.0018797724409228486, -0.013467394009664346, 0.004969611107007665, 0.010171768617283306], [-16.52354541323669, -0.05289433670461863, 0.06854793421832994, -0.04397166759326865], [-0.16577538547253035, -0.11545962378720276, -107.37909057514909, -65.5267184035896]], [0.999984077247513 0.9742443230122627 … 0.0 0.0; 0.9934251433547399 0.9864386931523093 … 0.0 0.0; … ; 0.0 0.0 … 0.0 0.0; 0.0 0.0 … 0.0 0.0;;;], SubArray{Float64, 2, Array{Float64, 3}, Tuple{Base.Slice{Base.OneTo{Int64}}, Base.Slice{Base.OneTo{Int64}}, Int64}, true}[[0.999984077247513 0.9742443230122627 … 0.0 0.0; 0.9934251433547399 0.9864386931523093 … 0.0 0.0; … ; 0.0 0.0 … 0.0 0.0; 0.0 0.0 … 0.0 0.0]], [0.00021264759968354918 0.0001366312192898067 6.893326536645816e-5; -7.116216528202379e-5 -9.624603074709359e-5 -8.168702910338332e-5; … ; 0.0 0.0 0.0; 0.0 0.0 0.0], [0.00012193189761751377 9.136569716483578e-5 1.8942131309443444e-5; -9.172536249002494e-5 -3.223945158764563e-5 -1.6460619036706703e-5; … ; 0.0 0.0 0.0; 0.0 0.0 0.0], [-0.5 -0.5 -0.5 -0.5; -0.5 0.8333333333333334 -0.16666666666666666 -0.16666666666666666; -0.5 -0.16666666666666666 0.8333333333333334 -0.16666666666666666; -0.5 -0.16666666666666666 -0.16666666666666666 0.8333333333333334], [0.0; 0.0; 0.0; 0.0;;], [5275.579066366831; 2893.8569447678096; … ; 1338.7204582833865; 0.0;;], [-0.00012052768567257065; 0.0005243291188868516; … ; 1.0; 0.07759491792130875;;], [114151.93577995931; 112764.8322387724; … ; 0.0; 0.0;;], [0.5522037149772232; 0.5265175267226798; … ; -0.00868396722208467; -0.008645197003287726;;], [-0.00021765700313519876 0.00010560139303391282 … -0.1017151721365145 0.0; 0.00010560139303391282 -0.0003967945725390119 … 0.4424894271067903 0.0; … ; -0.1017151721365145 0.4424894271067903 … 843.9154171833779 65.48354752887124; 0.0 0.0 … 65.48354752887124 -0.0030486101809490407;;;], [7029.161398740817 3759.8136305049893 … -489.62335872731694 -489.3949460151179; 3759.8136305049893 5717.770656784291 … -527.4797583425097 -527.2632190631304; … ; -489.62335872731694 -527.4797583425097 … 108102.02109867314 108102.00248300146; -489.3949460151179 -527.2632190631304 … 108102.00248300146 -0.01612153787936449;;;], Bool[1; 1; 0; 0;;], SubArray{Float64, 1, Matrix{Float64}, Tuple{Base.Slice{Base.OneTo{Int64}}, Int64}, true}[[0.0, 0.0, 0.0, 0.0]], SubArray{Float64, 1, Matrix{Float64}, Tuple{Base.Slice{Base.OneTo{Int64}}, Int64}, true}[[5275.579066366831, 2893.8569447678096, 2426.02875785844, 1338.7204582833865, 0.0]], SubArray{Float64, 1, Matrix{Float64}, Tuple{Base.Slice{Base.OneTo{Int64}}, Int64}, true}[[-0.00012052768567257065, 0.0005243291188868516, 0.0740234914604904, 1.0, 0.07759491792130875]], SubArray{Float64, 1, Matrix{Float64}, Tuple{Base.Slice{Base.OneTo{Int64}}, Int64}, true}[[114151.93577995931, 112764.8322387724, 112402.00967340921, 108102.02109867314, 0.0, 0.0]], SubArray{Float64, 1, Matrix{Float64}, Tuple{Base.Slice{Base.OneTo{Int64}}, Int64}, true}[[0.5522037149772232, 0.5265175267226798, 1.0, 0.00022851969219851807, -0.00868396722208467, -0.008645197003287726]], SubArray{Float64, 2, Array{Float64, 3}, Tuple{Base.Slice{Base.OneTo{Int64}}, Base.Slice{Base.OneTo{Int64}}, Int64}, true}[[-0.00021765700313519876 0.00010560139303391282 … -0.1017151721365145 0.0; 0.00010560139303391282 -0.0003967945725390119 … 0.4424894271067903 0.0; … ; -0.1017151721365145 0.4424894271067903 … 843.9154171833779 65.48354752887124; 0.0 0.0 … 65.48354752887124 -0.0030486101809490407]], SubArray{Float64, 2, Array{Float64, 3}, Tuple{Base.Slice{Base.OneTo{Int64}}, Base.Slice{Base.OneTo{Int64}}, Int64}, true}[[7029.161398740817 3759.8136305049893 … -489.62335872731694 -489.3949460151179; 3759.8136305049893 5717.770656784291 … -527.4797583425097 -527.2632190631304; … ; -489.62335872731694 -527.4797583425097 … 108102.02109867314 108102.00248300146; -489.3949460151179 -527.2632190631304 … 108102.00248300146 -0.01612153787936449]], SubArray{Bool, 1, Matrix{Bool}, Tuple{Base.Slice{Base.OneTo{Int64}}, Int64}, true}[[1, 1, 0, 0]], [4; 1; 2; 3;;], SubArray{Int64, 1, Matrix{Int64}, Tuple{Base.Slice{Base.OneTo{Int64}}, Int64}, true}[[4, 1, 2, 3]], -1.5552194868097581e7, -1.5552193929721253e7), nothing, nothing)
The first argument is the path to the PLINK 1 `.bed`, and the second argument is the number of populations. The following keyword arguments are supported:
- `T`: Precision of the estimation. `Float64` or `Float32`. Default `Float64`.
- `use_gpu`: Whether to use GPU for estimation. Default `false`.
- `rng`: Random number generator. Default `Random.GLOBAL_RNG`.
- `prefix`: Prefix of the PLINK file only with the SNPs selected using SKFR. The output file is named `$(prefix)_$(K)_$(sparsity)aims.bed`.
- `sparsity`: Number of SNPs selected by SKFR. Default `nothing` and skip SKFR.
- `skfr_tries`: Runs SKFR this many times and choose the best clustering. Default 1.
- `skfr_max_inner_iter`: Runs each SKFR for up to this many iterations or until convergence. Default 50.
- `admix_n_iter`: Maximum number of iterations for ADMIXTURE. Default 1000.
- `admix_rtol`: Convergence criteria in terms of relative change in loglikelihood. Default 1e-7.
- `admix_n_em_iter`: Number of EM iterations to get a good initial guess for estimation. Default 5.
- `Q`: Number of steps to be used in quasi-Newton acceleration. Default 3.
The output are:
- `d`: the strucutre to store Admixture data. In particular, `d.p` stores the allele frequencies and `d.q` stores the admixture proportions.
- `clusters`: cluster labels of each samples, `nothing` if `sparsity == nothing`.
- `aims`: The index of the selected SNPs in the decreasing order of importance, `nothing` if `sparsity == nothing`.
To see the allele frequencies of the first allele listed in the `.bim` file accompanying the `.bed` file:
```julia
d.p
```
4×54051 Matrix{Float64}:
0.841704 0.971913 0.991518 0.546057 … 0.920156 0.99999 0.990385
0.923591 0.992092 0.939205 0.471689 0.942705 0.993918 0.92073
0.917793 0.982938 0.959373 0.565633 0.947937 0.981754 0.99999
0.912906 0.999763 0.935531 0.456692 0.968599 0.969226 0.99999
To see the admixture proportion of each sample:
```julia
d.q
```
4×379 Matrix{Float64}:
0.0709719 0.0344815 0.0801809 0.116321 … 0.098118 1.0e-5 1.0e-5
0.417541 0.74624 0.714427 0.368537 0.0793005 1.0e-5 1.0e-5
1.0e-5 0.219269 0.205382 1.0e-5 0.822571 1.0e-5 1.0e-5
0.511478 1.0e-5 1.0e-5 0.515132 1.0e-5 0.99997 0.99997
The following shows the final loglikelihood of the parameters.
```julia
d.ll_new
```
-1.5552193929721253e7
The following is an example with `sparsity` defined.
```julia
d, clusters, aims = OpenADMIXTURE.run_admixture(filename, 4; T=Float64, use_gpu=false, rng=MersenneTwister(7856), sparsity=10000, admix_rtol=1e-7, prefix="./EUR_subset")
```
cnt of sparse1:6
./EUR_subset_4_10000aims
0.016605 seconds (4 allocations: 64 bytes)
0.017555 seconds (4 allocations: 64 bytes)
0.016559 seconds (4 allocations: 64 bytes)
0.017248 seconds (4 allocations: 64 bytes)
0.016535 seconds (4 allocations: 64 bytes)
0.017239 seconds (4 allocations: 64 bytes)
0.016542 seconds (4 allocations: 64 bytes)
0.017329 seconds (4 allocations: 64 bytes)
0.016328 seconds (4 allocations: 64 bytes)
0.017016 seconds (4 allocations: 64 bytes)
0.207011 seconds (434 allocations: 19.938 KiB)
initial ll: -3.077259034240569e6
-3.077259034240569e6
0.051540 seconds (4 allocations: 64 bytes)
0.000510 seconds (2.66 k allocations: 125.109 KiB)
0.068163 seconds (4 allocations: 64 bytes)
0.006425 seconds (8 allocations: 768 bytes)
-3.077259034240569e6
0.051073 seconds (4 allocations: 64 bytes)
0.000492 seconds (2.66 k allocations: 125.109 KiB)
0.068530 seconds (4 allocations: 64 bytes)
0.006167 seconds (8 allocations: 768 bytes)
-3.077259034240569e6
-3.0280405173204164e6
-3.0300059018500494e6
Iteration 1: ll = -3.0300059018500494e6, reldiff = 0.015355591409346895
0.331178 seconds (8.49 k allocations: 1.342 MiB)
-3.0300059018500494e6
0.056997 seconds (4 allocations: 64 bytes)
0.000501 seconds (2.66 k allocations: 125.109 KiB)
0.070048 seconds (4 allocations: 64 bytes)
0.005810 seconds (8 allocations: 768 bytes)
-3.0300059018500494e6
0.052859 seconds (4 allocations: 64 bytes)
0.000508 seconds (2.66 k allocations: 125.109 KiB)
0.068050 seconds (4 allocations: 64 bytes)
0.005522 seconds (8 allocations: 768 bytes)
-3.0300059018500494e6
-3.0057198788971743e6
-3.003246863866929e6
Iteration 2: ll = -3.003246863866929e6, reldiff = 0.008831348469249512
0.335381 seconds (8.49 k allocations: 1.658 MiB)
-3.003246863866929e6
0.051635 seconds (4 allocations: 64 bytes)
0.000634 seconds (2.66 k allocations: 125.109 KiB)
0.068657 seconds (4 allocations: 64 bytes)
0.005354 seconds (8 allocations: 768 bytes)
-3.003246863866929e6
0.051419 seconds (4 allocations: 64 bytes)
0.000527 seconds (2.66 k allocations: 125.109 KiB)
0.068346 seconds (4 allocations: 64 bytes)
0.005266 seconds (8 allocations: 768 bytes)
-3.003246863866929e6
-2.9954683999207392e6
-2.9951395344067365e6
Iteration 3: ll = -2.9951395344067365e6, reldiff = 0.0026995214937987526
0.326860 seconds (8.49 k allocations: 1.977 MiB)
-2.9951395344067365e6
0.054100 seconds (4 allocations: 64 bytes)
0.001317 seconds (2.66 k allocations: 125.109 KiB)
0.068212 seconds (4 allocations: 64 bytes)
0.005262 seconds (8 allocations: 768 bytes)
-2.9951395344067365e6
0.052234 seconds (4 allocations: 64 bytes)
0.001201 seconds (2.66 k allocations: 125.109 KiB)
0.068150 seconds (4 allocations: 64 bytes)
0.005203 seconds (8 allocations: 768 bytes)
-2.9951395344067365e6
-2.992523970635955e6
-2.993074174142398e6
Iteration 4: ll = -2.993074174142398e6, reldiff = 0.0006895706328912819
0.331379 seconds (8.49 k allocations: 1.975 MiB)
-2.993074174142398e6
0.053118 seconds (4 allocations: 64 bytes)
0.001216 seconds (2.66 k allocations: 125.109 KiB)
0.067760 seconds (4 allocations: 64 bytes)
0.005182 seconds (8 allocations: 768 bytes)
-2.993074174142398e6
0.051179 seconds (4 allocations: 64 bytes)
0.001521 seconds (2.66 k allocations: 125.109 KiB)
0.068610 seconds (4 allocations: 64 bytes)
0.005191 seconds (8 allocations: 768 bytes)
-2.993074174142398e6
-2.9914347372060185e6
-2.9912617609358244e6
Iteration 5: ll = -2.9912617609358244e6, reldiff = 0.0006055356804155662
0.329000 seconds (8.49 k allocations: 1.975 MiB)
-2.9912617609358244e6
0.051459 seconds (4 allocations: 64 bytes)
0.001472 seconds (2.66 k allocations: 125.109 KiB)
0.068897 seconds (4 allocations: 64 bytes)
0.005212 seconds (8 allocations: 768 bytes)
-2.9912617609358244e6
0.051166 seconds (4 allocations: 64 bytes)
0.001426 seconds (2.66 k allocations: 125.109 KiB)
0.068332 seconds (4 allocations: 64 bytes)
0.005103 seconds (8 allocations: 768 bytes)
-2.9912617609358244e6
-2.9907587680529426e6
-2.9919207918732297e6
Iteration 6: ll = -2.9907587680529426e6, reldiff = 0.00016815408449055843
0.328581 seconds (8.49 k allocations: 1.975 MiB)
-2.9907587680529426e6
0.051610 seconds (4 allocations: 64 bytes)
0.001440 seconds (2.66 k allocations: 125.109 KiB)
0.068850 seconds (4 allocations: 64 bytes)
0.005143 seconds (8 allocations: 768 bytes)
-2.9907587680529426e6
0.051673 seconds (4 allocations: 64 bytes)
0.001431 seconds (2.66 k allocations: 125.109 KiB)
0.068392 seconds (4 allocations: 64 bytes)
0.006488 seconds (8 allocations: 768 bytes)
-2.9907587680529426e6
-2.9904190503580016e6
-2.992138931188849e6
Iteration 7: ll = -2.9904190503580016e6, reldiff = 0.000113589132821348
0.330491 seconds (8.49 k allocations: 1.984 MiB)
-2.9904190503580016e6
0.051826 seconds (4 allocations: 64 bytes)
0.001549 seconds (2.66 k allocations: 125.109 KiB)
0.068626 seconds (4 allocations: 64 bytes)
0.005074 seconds (8 allocations: 768 bytes)
-2.9904190503580016e6
0.051142 seconds (4 allocations: 64 bytes)
0.001537 seconds (2.66 k allocations: 125.109 KiB)
0.068163 seconds (4 allocations: 64 bytes)
0.005082 seconds (8 allocations: 768 bytes)
-2.9904190503580016e6
-2.9901733383521987e6
-2.99042389440459e6
Iteration 8: ll = -2.9901733383521987e6, reldiff = 8.216641268837562e-5
0.328440 seconds (8.49 k allocations: 1.975 MiB)
-2.9901733383521987e6
0.051310 seconds (4 allocations: 64 bytes)
0.001440 seconds (2.66 k allocations: 125.109 KiB)
0.068794 seconds (4 allocations: 64 bytes)
0.005056 seconds (8 allocations: 768 bytes)
-2.9901733383521987e6
0.051610 seconds (4 allocations: 64 bytes)
0.001434 seconds (2.66 k allocations: 125.109 KiB)
0.068217 seconds (4 allocations: 64 bytes)
0.005080 seconds (8 allocations: 768 bytes)
-2.9901733383521987e6
-2.990000767067695e6
-2.990061270031604e6
Iteration 9: ll = -2.990061270031604e6, reldiff = 3.747887092608675e-5
0.328438 seconds (8.49 k allocations: 1.975 MiB)
-2.990061270031604e6
0.051703 seconds (4 allocations: 64 bytes)
0.001236 seconds (2.66 k allocations: 125.109 KiB)
0.068567 seconds (4 allocations: 64 bytes)
0.005093 seconds (8 allocations: 768 bytes)
-2.990061270031604e6
0.051414 seconds (4 allocations: 64 bytes)
0.001220 seconds (2.66 k allocations: 125.109 KiB)
0.068460 seconds (4 allocations: 64 bytes)
0.005338 seconds (8 allocations: 768 bytes)
-2.990061270031604e6
-2.98974042373545e6
-2.989726746092522e6
Iteration 10: ll = -2.989726746092522e6, reldiff = 0.00011187862350338979
0.336451 seconds (8.49 k allocations: 1.975 MiB)
-2.989726746092522e6
0.051309 seconds (4 allocations: 64 bytes)
0.001235 seconds (2.66 k allocations: 125.109 KiB)
0.068019 seconds (4 allocations: 64 bytes)
0.005074 seconds (8 allocations: 768 bytes)
-2.989726746092522e6
0.051070 seconds (4 allocations: 64 bytes)
0.001263 seconds (2.66 k allocations: 125.109 KiB)
0.067901 seconds (4 allocations: 64 bytes)
0.005053 seconds (8 allocations: 768 bytes)
-2.989726746092522e6
-2.989633149826683e6
-2.989850885712909e6
Iteration 11: ll = -2.989633149826683e6, reldiff = 3.1305959971517296e-5
0.326089 seconds (8.49 k allocations: 1.975 MiB)
-2.989633149826683e6
0.051355 seconds (4 allocations: 64 bytes)
0.001224 seconds (2.66 k allocations: 125.109 KiB)
0.069572 seconds (4 allocations: 64 bytes)
0.005054 seconds (8 allocations: 768 bytes)
-2.989633149826683e6
0.051855 seconds (4 allocations: 64 bytes)
0.001561 seconds (2.66 k allocations: 125.109 KiB)
0.068535 seconds (4 allocations: 64 bytes)
0.005109 seconds (8 allocations: 768 bytes)
-2.989633149826683e6
-2.9895800598967243e6
-2.9910787931252318e6
Iteration 12: ll = -2.9895800598967243e6, reldiff = 1.7758008189694848e-5
0.329700 seconds (8.49 k allocations: 1.975 MiB)
-2.9895800598967243e6
0.053446 seconds (4 allocations: 64 bytes)
0.001343 seconds (2.66 k allocations: 125.109 KiB)
0.068643 seconds (4 allocations: 64 bytes)
0.005082 seconds (8 allocations: 768 bytes)
-2.9895800598967243e6
0.051077 seconds (4 allocations: 64 bytes)
0.001326 seconds (2.66 k allocations: 125.109 KiB)
0.068270 seconds (4 allocations: 64 bytes)
0.005109 seconds (8 allocations: 768 bytes)
-2.9895800598967243e6
-2.9895361151002464e6
-3.0470678619324937e6
Iteration 13: ll = -2.9895361151002464e6, reldiff = 1.4699320840207977e-5
0.330408 seconds (8.49 k allocations: 1.975 MiB)
-2.9895361151002464e6
0.051299 seconds (4 allocations: 64 bytes)
0.001231 seconds (2.66 k allocations: 125.109 KiB)
0.068383 seconds (4 allocations: 64 bytes)
0.005068 seconds (8 allocations: 768 bytes)
-2.9895361151002464e6
0.051155 seconds (4 allocations: 64 bytes)
0.001107 seconds (2.66 k allocations: 125.109 KiB)
0.068226 seconds (4 allocations: 64 bytes)
0.005089 seconds (8 allocations: 768 bytes)
-2.9895361151002464e6
-2.989495350413259e6
-4.870537010936856e6
Iteration 14: ll = -2.989495350413259e6, reldiff = 1.363579010849405e-5
0.326845 seconds (8.49 k allocations: 1.975 MiB)
-2.989495350413259e6
0.051376 seconds (4 allocations: 64 bytes)
0.001114 seconds (2.66 k allocations: 125.109 KiB)
0.068882 seconds (4 allocations: 64 bytes)
0.005072 seconds (8 allocations: 768 bytes)
-2.989495350413259e6
0.051083 seconds (4 allocations: 64 bytes)
0.001111 seconds (2.66 k allocations: 125.109 KiB)
0.068961 seconds (4 allocations: 64 bytes)
0.005080 seconds (8 allocations: 768 bytes)
-2.989495350413259e6
-2.989455754492594e6
-3.204234679307862e6
Iteration 15: ll = -2.989455754492594e6, reldiff = 1.3245018313781956e-5
0.328163 seconds (8.49 k allocations: 1.975 MiB)
-2.989455754492594e6
0.051956 seconds (4 allocations: 64 bytes)
0.001235 seconds (2.66 k allocations: 125.109 KiB)
0.068537 seconds (4 allocations: 64 bytes)
0.005054 seconds (8 allocations: 768 bytes)
-2.989455754492594e6
0.051440 seconds (4 allocations: 64 bytes)
0.001220 seconds (2.66 k allocations: 125.109 KiB)
0.068383 seconds (4 allocations: 64 bytes)
0.005095 seconds (8 allocations: 768 bytes)
-2.989455754492594e6
-2.9894188142380556e6
-3.005116706744177e6
Iteration 16: ll = -2.9894188142380556e6, reldiff = 1.2356849397354584e-5
0.329269 seconds (8.60 k allocations: 2.031 MiB)
-2.9894188142380556e6
0.060329 seconds (4 allocations: 64 bytes)
0.001310 seconds (2.66 k allocations: 125.109 KiB)
0.070944 seconds (4 allocations: 64 bytes)
0.005112 seconds (8 allocations: 768 bytes)
-2.9894188142380556e6
0.051192 seconds (4 allocations: 64 bytes)
0.001234 seconds (2.66 k allocations: 125.109 KiB)
0.069936 seconds (4 allocations: 64 bytes)
0.005086 seconds (8 allocations: 768 bytes)
-2.9894188142380556e6
-2.9893843473597807e6
-3.0024701291123424e6
Iteration 17: ll = -2.9893843473597807e6, reldiff = 1.152962512671757e-5
0.341146 seconds (8.49 k allocations: 1.976 MiB)
-2.9893843473597807e6
0.051523 seconds (4 allocations: 64 bytes)
0.001348 seconds (2.66 k allocations: 125.109 KiB)
0.068716 seconds (4 allocations: 64 bytes)
0.005079 seconds (8 allocations: 768 bytes)
-2.9893843473597807e6
0.051583 seconds (4 allocations: 64 bytes)
0.001335 seconds (2.66 k allocations: 125.109 KiB)
0.067773 seconds (4 allocations: 64 bytes)
0.005060 seconds (8 allocations: 768 bytes)
-2.9893843473597807e6
-2.9893519062841535e6
-3.2076681927966746e6
Iteration 18: ll = -2.9893519062841535e6, reldiff = 1.0852092557411792e-5
0.327834 seconds (8.49 k allocations: 1.977 MiB)
-2.9893519062841535e6
0.051513 seconds (4 allocations: 64 bytes)
0.001347 seconds (2.66 k allocations: 125.109 KiB)
0.068581 seconds (4 allocations: 64 bytes)
0.005065 seconds (8 allocations: 768 bytes)
-2.9893519062841535e6
0.051358 seconds (4 allocations: 64 bytes)
0.001324 seconds (2.66 k allocations: 125.109 KiB)
0.067730 seconds (4 allocations: 64 bytes)
0.005019 seconds (8 allocations: 768 bytes)
-2.9893519062841535e6
-2.989320160419182e6
-4.216603856297227e6
Iteration 19: ll = -2.989320160419182e6, reldiff = 1.0619647992866069e-5
0.327422 seconds (8.49 k allocations: 1.979 MiB)
-2.989320160419182e6
0.053565 seconds (4 allocations: 64 bytes)
0.001343 seconds (2.66 k allocations: 125.109 KiB)
0.068597 seconds (4 allocations: 64 bytes)
0.005200 seconds (8 allocations: 768 bytes)
-2.989320160419182e6
0.051766 seconds (4 allocations: 64 bytes)
0.001229 seconds (2.66 k allocations: 125.109 KiB)
0.068190 seconds (4 allocations: 64 bytes)
0.005048 seconds (8 allocations: 768 bytes)
-2.989320160419182e6
-2.9892881573209567e6
-3.0190224119707076e6
Iteration 20: ll = -2.9892881573209567e6, reldiff = 1.0705811524963374e-5
0.330434 seconds (8.49 k allocations: 1.975 MiB)
-2.9892881573209567e6
0.051287 seconds (4 allocations: 64 bytes)
0.001235 seconds (2.66 k allocations: 125.109 KiB)
0.068540 seconds (4 allocations: 64 bytes)
0.005040 seconds (8 allocations: 768 bytes)
-2.9892881573209567e6
0.051115 seconds (4 allocations: 64 bytes)
0.001226 seconds (2.66 k allocations: 125.109 KiB)
0.068497 seconds (4 allocations: 64 bytes)
0.005067 seconds (8 allocations: 768 bytes)
-2.9892881573209567e6
-2.989255848399305e6
-3.070369539860443e6
Iteration 21: ll = -2.989255848399305e6, reldiff = 1.0808232579648808e-5
0.327615 seconds (8.49 k allocations: 1.975 MiB)
-2.989255848399305e6
0.055029 seconds (4 allocations: 64 bytes)
0.001283 seconds (2.66 k allocations: 125.109 KiB)
0.068664 seconds (4 allocations: 64 bytes)
0.005064 seconds (8 allocations: 768 bytes)
-2.989255848399305e6
0.051607 seconds (4 allocations: 64 bytes)
0.001233 seconds (2.66 k allocations: 125.109 KiB)
0.068599 seconds (4 allocations: 64 bytes)
0.005057 seconds (8 allocations: 768 bytes)
-2.989255848399305e6
-2.989223200480792e6
-2.721573989958778e7
Iteration 22: ll = -2.989223200480792e6, reldiff = 1.0921754499555953e-5
0.332062 seconds (8.49 k allocations: 1.975 MiB)
-2.989223200480792e6
0.051913 seconds (4 allocations: 64 bytes)
0.001239 seconds (2.66 k allocations: 125.109 KiB)
0.068575 seconds (4 allocations: 64 bytes)
0.005057 seconds (8 allocations: 768 bytes)
-2.989223200480792e6
0.051357 seconds (4 allocations: 64 bytes)
0.001113 seconds (2.66 k allocations: 125.109 KiB)
0.067779 seconds (4 allocations: 64 bytes)
0.004986 seconds (8 allocations: 768 bytes)
-2.989223200480792e6
-2.98918862571243e6
-3.0027735217421465e6
Iteration 23: ll = -2.98918862571243e6, reldiff = 1.1566472639627613e-5
0.327517 seconds (8.49 k allocations: 1.983 MiB)
-2.98918862571243e6
0.051341 seconds (4 allocations: 64 bytes)
0.001012 seconds (2.66 k allocations: 125.109 KiB)
0.068575 seconds (4 allocations: 64 bytes)
0.005209 seconds (8 allocations: 768 bytes)
-2.98918862571243e6
0.051170 seconds (4 allocations: 64 bytes)
0.001009 seconds (2.66 k allocations: 125.109 KiB)
0.067795 seconds (4 allocations: 64 bytes)
0.005078 seconds (8 allocations: 768 bytes)
-2.98918862571243e6
-2.9891504427890005e6
-3.055894439483546e6
Iteration 24: ll = -2.9891504427890005e6, reldiff = 1.2773674802989472e-5
0.326734 seconds (8.49 k allocations: 1.975 MiB)
-2.9891504427890005e6
0.052237 seconds (4 allocations: 64 bytes)
0.001021 seconds (2.66 k allocations: 125.109 KiB)
0.068584 seconds (4 allocations: 64 bytes)
0.005008 seconds (8 allocations: 768 bytes)
-2.9891504427890005e6
0.051356 seconds (4 allocations: 64 bytes)
0.001006 seconds (2.66 k allocations: 125.109 KiB)
0.067855 seconds (4 allocations: 64 bytes)
0.005038 seconds (8 allocations: 768 bytes)
-2.9891504427890005e6
-2.9891102235109448e6
-2.9937728315902813e6
Iteration 25: ll = -2.9891102235109448e6, reldiff = 1.3455086595836446e-5
0.327716 seconds (8.49 k allocations: 1.975 MiB)
-2.9891102235109448e6
0.051503 seconds (4 allocations: 64 bytes)
0.000797 seconds (2.66 k allocations: 125.109 KiB)
0.072497 seconds (4 allocations: 64 bytes)
0.005026 seconds (8 allocations: 768 bytes)
-2.9891102235109448e6
0.051099 seconds (4 allocations: 64 bytes)
0.000790 seconds (2.66 k allocations: 125.109 KiB)
0.067850 seconds (4 allocations: 64 bytes)
0.005050 seconds (8 allocations: 768 bytes)
-2.9891102235109448e6
-2.989069852298082e6
-2.993139156894906e6
Iteration 26: ll = -2.989069852298082e6, reldiff = 1.3506097080447741e-5
0.330044 seconds (8.49 k allocations: 1.975 MiB)
-2.989069852298082e6
0.051596 seconds (4 allocations: 64 bytes)
0.000912 seconds (2.66 k allocations: 125.109 KiB)
0.068444 seconds (4 allocations: 64 bytes)
0.005081 seconds (8 allocations: 768 bytes)
-2.989069852298082e6
0.051489 seconds (4 allocations: 64 bytes)
0.000898 seconds (2.66 k allocations: 125.109 KiB)
0.068667 seconds (4 allocations: 64 bytes)
0.005089 seconds (8 allocations: 768 bytes)
-2.989069852298082e6
-2.9890318651039726e6
-2.991710920354535e6
Iteration 27: ll = -2.9890318651039726e6, reldiff = 1.2708700694979335e-5
0.327652 seconds (8.49 k allocations: 1.975 MiB)
-2.9890318651039726e6
0.051948 seconds (4 allocations: 64 bytes)
0.000903 seconds (2.66 k allocations: 125.109 KiB)
0.068620 seconds (4 allocations: 64 bytes)
0.005098 seconds (8 allocations: 768 bytes)
-2.9890318651039726e6
0.051546 seconds (4 allocations: 64 bytes)
0.000907 seconds (2.66 k allocations: 125.109 KiB)
0.068082 seconds (4 allocations: 64 bytes)
0.005061 seconds (8 allocations: 768 bytes)
-2.9890318651039726e6
-2.9889978041921854e6
-2.9892098593712384e6
Iteration 28: ll = -2.9889978041921854e6, reldiff = 1.1395298987895992e-5
0.327987 seconds (8.49 k allocations: 1.975 MiB)
-2.9889978041921854e6
0.051315 seconds (4 allocations: 64 bytes)
0.001014 seconds (2.66 k allocations: 125.109 KiB)
0.068676 seconds (4 allocations: 64 bytes)
0.005056 seconds (8 allocations: 768 bytes)
-2.9889978041921854e6
0.051113 seconds (4 allocations: 64 bytes)
0.000999 seconds (2.66 k allocations: 125.109 KiB)
0.068374 seconds (4 allocations: 64 bytes)
0.005035 seconds (8 allocations: 768 bytes)
-2.9889978041921854e6
-2.9889710379184415e6
-2.9892181158235935e6
Iteration 29: ll = -2.9889710379184415e6, reldiff = 8.954932555118614e-6
0.327091 seconds (8.49 k allocations: 1.975 MiB)
-2.9889710379184415e6
0.051287 seconds (4 allocations: 64 bytes)
0.001010 seconds (2.66 k allocations: 125.109 KiB)
0.067741 seconds (4 allocations: 64 bytes)
0.005077 seconds (8 allocations: 768 bytes)
-2.9889710379184415e6
0.051320 seconds (4 allocations: 64 bytes)
0.001005 seconds (2.66 k allocations: 125.109 KiB)
0.068327 seconds (4 allocations: 64 bytes)
0.005077 seconds (8 allocations: 768 bytes)
-2.9889710379184415e6
-2.9889486095322985e6
-2.9900355066040237e6
Iteration 30: ll = -2.9889486095322985e6, reldiff = 7.503714776226964e-6
0.326470 seconds (8.49 k allocations: 1.975 MiB)
-2.9889486095322985e6
0.051307 seconds (4 allocations: 64 bytes)
0.001011 seconds (2.66 k allocations: 125.109 KiB)
0.068545 seconds (4 allocations: 64 bytes)
0.005031 seconds (8 allocations: 768 bytes)
-2.9889486095322985e6
0.051066 seconds (4 allocations: 64 bytes)
0.000996 seconds (2.66 k allocations: 125.109 KiB)
0.068230 seconds (4 allocations: 64 bytes)
0.005030 seconds (8 allocations: 768 bytes)
-2.9889486095322985e6
-2.988929193837599e6
-2.9900550381234307e6
Iteration 31: ll = -2.988929193837599e6, reldiff = 6.495827542066974e-6
0.329288 seconds (8.49 k allocations: 1.975 MiB)
-2.988929193837599e6
0.051494 seconds (4 allocations: 64 bytes)
0.001013 seconds (2.66 k allocations: 125.109 KiB)
0.068373 seconds (4 allocations: 64 bytes)
0.005045 seconds (8 allocations: 768 bytes)
-2.988929193837599e6
0.051083 seconds (4 allocations: 64 bytes)
0.001001 seconds (2.66 k allocations: 125.109 KiB)
0.067739 seconds (4 allocations: 64 bytes)
0.005013 seconds (8 allocations: 768 bytes)
-2.988929193837599e6
-2.988913407219005e6
-2.9889200662938342e6
Iteration 32: ll = -2.9889200662938342e6, reldiff = 3.053783871318594e-6
0.326375 seconds (8.49 k allocations: 1.975 MiB)
-2.9889200662938342e6
0.051381 seconds (4 allocations: 64 bytes)
0.000797 seconds (2.66 k allocations: 125.109 KiB)
0.070106 seconds (4 allocations: 64 bytes)
0.005042 seconds (8 allocations: 768 bytes)
-2.9889200662938342e6
0.051473 seconds (4 allocations: 64 bytes)
0.001423 seconds (2.66 k allocations: 125.109 KiB)
0.069658 seconds (4 allocations: 64 bytes)
0.005092 seconds (8 allocations: 768 bytes)
-2.9889200662938342e6
-2.9888740258513074e6
-2.9888789198555816e6
Iteration 33: ll = -2.9888789198555816e6, reldiff = 1.3766322731964776e-5
0.332496 seconds (8.60 k allocations: 2.032 MiB)
-2.9888789198555816e6
0.051510 seconds (4 allocations: 64 bytes)
0.000801 seconds (2.66 k allocations: 125.109 KiB)
0.068655 seconds (4 allocations: 64 bytes)
0.005081 seconds (8 allocations: 768 bytes)
-2.9888789198555816e6
0.051496 seconds (4 allocations: 64 bytes)
0.000782 seconds (2.66 k allocations: 125.109 KiB)
0.068511 seconds (4 allocations: 64 bytes)
0.005005 seconds (8 allocations: 768 bytes)
-2.9888789198555816e6
-2.9888553511574874e6
-2.988873849177302e6
Iteration 34: ll = -2.988873849177302e6, reldiff = 1.696515120117397e-6
0.327016 seconds (8.49 k allocations: 1.976 MiB)
-2.988873849177302e6
0.051493 seconds (4 allocations: 64 bytes)
0.000796 seconds (2.66 k allocations: 125.109 KiB)
0.068231 seconds (4 allocations: 64 bytes)
0.005037 seconds (8 allocations: 768 bytes)
-2.988873849177302e6
0.051156 seconds (4 allocations: 64 bytes)
0.000784 seconds (2.66 k allocations: 125.109 KiB)
0.068227 seconds (4 allocations: 64 bytes)
0.004956 seconds (8 allocations: 768 bytes)
-2.988873849177302e6
-2.9888501509662215e6
-2.9888497143354164e6
Iteration 35: ll = -2.9888497143354164e6, reldiff = 8.074894794258681e-6
0.326043 seconds (8.49 k allocations: 1.977 MiB)
-2.9888497143354164e6
0.051530 seconds (4 allocations: 64 bytes)
0.000795 seconds (2.66 k allocations: 125.109 KiB)
0.068550 seconds (4 allocations: 64 bytes)
0.005018 seconds (8 allocations: 768 bytes)
-2.9888497143354164e6
0.051266 seconds (4 allocations: 64 bytes)
0.000788 seconds (2.66 k allocations: 125.109 KiB)
0.068305 seconds (4 allocations: 64 bytes)
0.005013 seconds (8 allocations: 768 bytes)
-2.9888497143354164e6
-2.98884770192568e6
-2.98884653275144e6
Iteration 36: ll = -2.98884653275144e6, reldiff = 1.0644844272742283e-6
0.328312 seconds (8.49 k allocations: 1.975 MiB)
-2.98884653275144e6
0.051698 seconds (4 allocations: 64 bytes)
0.000808 seconds (2.66 k allocations: 125.109 KiB)
0.068499 seconds (4 allocations: 64 bytes)
0.005027 seconds (8 allocations: 768 bytes)
-2.98884653275144e6
0.051365 seconds (4 allocations: 64 bytes)
0.000783 seconds (2.66 k allocations: 125.109 KiB)
0.068251 seconds (4 allocations: 64 bytes)
0.005036 seconds (8 allocations: 768 bytes)
-2.98884653275144e6
-2.9888461026714854e6
-2.9888459670782355e6
Iteration 37: ll = -2.9888459670782355e6, reldiff = 1.8926137505086115e-7
0.326842 seconds (8.49 k allocations: 1.979 MiB)
-2.9888459670782355e6
0.051509 seconds (4 allocations: 64 bytes)
0.000800 seconds (2.66 k allocations: 125.109 KiB)
0.068580 seconds (4 allocations: 64 bytes)
0.005017 seconds (8 allocations: 768 bytes)
-2.9888459670782355e6
0.051076 seconds (4 allocations: 64 bytes)
0.000784 seconds (2.66 k allocations: 125.109 KiB)
0.068157 seconds (4 allocations: 64 bytes)
0.005003 seconds (8 allocations: 768 bytes)
-2.9888459670782355e6
-2.9888457179705338e6
-2.9888456882726927e6
Iteration 38: ll = -2.9888456882726927e6, reldiff = 9.328200442854993e-8
12.511960 seconds (325.18 k allocations: 74.353 MiB)
(AdmixData{Float64}(379, 10000, 4, 3, true, [0.582214916426889 1.0000000000027756e-5 … 0.9327568172111603 0.99999; 0.14092902448354105 0.41258807922799495 … 0.8596468613040379 0.8877110943870553; 0.05402188629156628 0.1339463980931601 … 0.9599270223437033 0.99999; 0.2228341727980035 0.45345552267884487 … 0.8107491631733151 0.99999], [0.5822686983061394 1.0e-5 … 0.932690502090477 0.99999; 0.14157978700364626 0.4122387868461372 … 0.8596295407288184 0.8876821133904721; 0.05422353338551516 0.13369030486485345 … 0.9604830519644512 0.99999; 0.2219279813046991 0.45406090828900936 … 0.8106984216220864 0.99999], [0.582226360690002 1.0e-5 … 0.9326919414077695 0.99999; 0.14088115931089432 0.4123807383809744 … 0.8596604973569697 0.8876695309831731; 0.05418392365429152 0.13379645401479928 … 0.9604303962397303 0.99999; 0.2227085563448121 0.45381280760422626 … 0.8106865432804832 0.99999], [0.582214916426889 1.0000000000027756e-5 … 0.9327568172111603 0.99999; 0.14092902448354105 0.41258807922799495 … 0.8596468613040379 0.8877110943870553; 0.05402188629156628 0.1339463980931601 … 0.9599270223437033 0.99999; 0.2228341727980035 0.45345552267884487 … 0.8107491631733151 0.99999], [0.582214916426889, 0.14092902448354105, 0.05402188629156628, 0.2228341727980035, 1.0000000000027756e-5, 0.41258807922799495, 0.1339463980931601, 0.45345552267884487, 1.0e-5, 0.5069557466869863 … 0.9785293750103126, 0.7676496020277787, 0.9327568172111603, 0.8596468613040379, 0.9599270223437033, 0.8107491631733151, 0.99999, 0.8877110943870553, 0.99999, 0.99999], [0.5822686983061394, 0.14157978700364626, 0.05422353338551516, 0.2219279813046991, 1.0e-5, 0.4122387868461372, 0.13369030486485345, 0.45406090828900936, 1.0e-5, 0.5061059458488054 … 0.9782466651408858, 0.7677475795250771, 0.932690502090477, 0.8596295407288184, 0.9604830519644512, 0.8106984216220864, 0.99999, 0.8876821133904721, 0.99999, 0.99999], [0.582226360690002, 0.14088115931089432, 0.05418392365429152, 0.2227085563448121, 1.0e-5, 0.4123807383809744, 0.13379645401479928, 0.45381280760422626, 1.0e-5, 0.5063020337105326 … 0.9784626120269361, 0.767673260692788, 0.9326919414077695, 0.8596604973569697, 0.9604303962397303, 0.8106865432804832, 0.99999, 0.8876695309831731, 0.99999, 0.99999], [0.582214916426889, 0.14092902448354105, 0.05402188629156628, 0.2228341727980035, 1.0000000000027756e-5, 0.41258807922799495, 0.1339463980931601, 0.45345552267884487, 1.0e-5, 0.5069557466869863 … 0.9785293750103126, 0.7676496020277787, 0.9327568172111603, 0.8596468613040379, 0.9599270223437033, 0.8107491631733151, 0.99999, 0.8877110943870553, 0.99999, 0.99999], [0.0, 0.0, 0.0, 0.0, 0.0, 0.0, 0.0, 0.0, 0.0, 0.0 … 0.0, 0.0, 0.0, 0.0, 0.0, 0.0, 0.0, 0.0, 0.0, 0.0], [0.0, 0.0, 0.0, 0.0, 0.0, 0.0, 0.0, 0.0, 0.0, 0.0 … 0.0, 0.0, 0.0, 0.0, 0.0, 0.0, 0.0, 0.0, 0.0, 0.0], [0.582214916426889 1.0000000000027756e-5 … 0.9999699999999999 0.9999699999999999; 0.14092902448354105 0.41258807922799495 … 1.0e-5 1.0e-5; 0.05402188629156628 0.1339463980931601 … 1.0e-5 1.0e-5; 0.2228341727980035 0.45345552267884487 … 1.0e-5 1.0e-5], [0.5822686983061394 1.0e-5 … 0.9999699999999999 0.9999699999999999; 0.14157978700364626 0.4122387868461372 … 1.0e-5 1.0e-5; 0.05422353338551516 0.13369030486485345 … 1.0e-5 1.0e-5; 0.2219279813046991 0.45406090828900936 … 1.0e-5 1.0e-5], [0.582226360690002 1.0e-5 … 0.9999699999999999 0.9999699999999999; 0.14088115931089432 0.4123807383809744 … 1.0e-5 1.0e-5; 0.05418392365429152 0.13379645401479928 … 1.0e-5 1.0e-5; 0.2227085563448121 0.45381280760422626 … 1.0e-5 1.0e-5], [0.582214916426889 1.0000000000027756e-5 … 0.9999699999999999 0.9999699999999999; 0.14092902448354105 0.41258807922799495 … 1.0e-5 1.0e-5; 0.05402188629156628 0.1339463980931601 … 1.0e-5 1.0e-5; 0.2228341727980035 0.45345552267884487 … 1.0e-5 1.0e-5], [0.794600708769161 0.8699629358466604 … 0.9327568172111603 0.99999; 0.6295417302123216 0.9882835240192088 … 0.8596468613040379 0.8877110943870553; 0.795332666017797 0.9004058223965158 … 0.9599270223437033 0.99999; 0.7314117939132884 0.8910746807079742 … 0.8107491631733151 0.99999], [0.7946379362185242 0.8699568858567608 … 0.932690502090477 0.99999; 0.6294705873253528 0.9882931595924863 … 0.8596295407288184 0.8876821133904721; 0.7947761216806404 0.9014162794343112 … 0.9604830519644512 0.99999; 0.7315585615635503 0.8908533540490032 … 0.8106984216220864 0.99999], [0.7946218752583306 0.8699668115606753 … 0.9326919414077695 0.99999; 0.629492931909354 0.9882919440582303 … 0.8596604973569697 0.8876695309831731; 0.7949439576510982 0.9012387456154918 … 0.9604303962397303 0.99999; 0.7315050094973324 0.890896060077284 … 0.8106865432804832 0.99999], [0.794600708769161 0.8699629358466604 … 0.9327568172111603 0.99999; 0.6295417302123216 0.9882835240192088 … 0.8596468613040379 0.8877110943870553; 0.795332666017797 0.9004058223965158 … 0.9599270223437033 0.99999; 0.7314117939132884 0.8910746807079742 … 0.8107491631733151 0.99999], [20324.008613992417 19517.205560497827 19445.052396609426 19593.620284729197; 19517.205560497827 21129.69564684323 19991.760457049983 20546.476546981514; 19445.052396609426 19991.760457049983 27061.91936795712 19733.76140174633; 19593.620284729197 20546.476546981514 19733.76140174633 20783.794235985824;;; 20537.320580307394 19532.61527210971 19904.474838050613 19816.064775376904; 19532.61527210971 20429.751878926836 19208.639123757057 19842.885390646392; 19904.474838050613 19208.639123757057 24043.105805147836 19529.028777521504; 19816.064775376904 19842.885390646392 19529.028777521504 20281.005129401456;;; 20554.33202737424 19527.167907738505 20146.012553921053 19827.729637940454; 19527.167907738512 20277.465892718952 19338.0657146504 19815.571658413777; 20146.01255392105 19338.0657146504 24509.245681961882 19667.149225394885; 19827.729637940458 19815.571658413777 19667.149225394885 20326.462531857924;;; … ;;; 20161.223377180504 19799.481341587463 19499.054021029686 19738.89607701232; 19799.481341587463 20880.864584502502 19303.322514294978 20163.93967566195; 19499.054021029686 19303.322514294978 22942.205947459446 19089.16440119291; 19738.89607701232 20163.93967566195 19089.16440119291 20285.72352733147;;; 20000.02114719698 19652.53691666853 19632.312142147246 19657.796437926452; 19652.53691666853 21076.84228656145 19791.029925493247 20375.34137743504; 19632.312142147246 19791.029925493247 24012.84358997869 19676.25199063923; 19657.796437926452 20375.34137743504 19676.25199063923 20499.803807281765;;; 20000.014106027465 19707.89979655304 19747.929550133194 19838.811482171674; 19707.89979655304 22045.021897104154 20875.403318563087 21565.27026454133; 19747.929550133194 20875.403318563087 25203.810149595418 20909.98526841688; 19838.811482171674 21565.270264541326 20909.98526841688 21840.790994511528], [-20000.0278902452 -19711.042676807927 … -20000.010573017542 -20000.007052012574; -19999.657638990368 -20000.018840334767 … -19652.559772696888 -19707.95341651394; -19999.54125456821 -20000.44382380299 … -19632.357974038034 -19748.00700423404; -20000.25732032506 -19999.858582666187 … -19657.81221800508 -19838.859478292485], [566.628571738167 83.90099875125914 62.70760160459943 206.69526708612761; 83.90099875125914 493.3179302661993 50.12892125483464 184.51024479111086; 62.70760160459943 50.12892125483464 103.82896536324253 202.09259891424378; 206.69526708612761 184.51024479111086 202.09259891424378 1153.4061405968855;;; 958.3641448625666 96.0657103671523 99.84997857047428 452.8669952750855; 96.0657103671523 8422.223541256804 173.75693630488144 541.6772331313043; 99.84997857047429 173.75693630488135 205.75467061768856 477.66509054457424; 452.8669952750855 541.6772331313044 477.66509054457424 2396.37473968966;;; 4787.553491320607 279.1023137653173 161.93765801627018 1347.7563046867367; 279.1023137653173 411.5348370695136 213.93028528940792 958.6175587313586; 161.93765801627018 213.93028528940792 411.75403584058546 1352.7814161727115; 1347.7563046867367 958.6175587313586 1352.7814161727115 7467.536092617086;;; … ;;; 650.1821214406797 81.84322018117557 59.023027883089135 273.76127310783073; 81.84322018117557 520.8311086730197 52.169654366007286 197.2850117549217; 59.02302788308914 52.169654366007286 333.9347030552088 270.49525897176716; 273.76127310783073 197.2850117549217 270.49525897176716 1339.7021125851502;;; 944.8744923000977 210.87018222789402 197.4712494119201 430.93057398367193; 210.87018222789402 935.5813002844052 105.64660374792102 292.4439054220507; 197.47124941192013 105.64660374792102 185.08439855823875 292.02256227961254; 430.93057398367193 292.44390542205076 292.02256227961254 1682.286841236985;;; 1047.3154837338877 785.9251092419285 309.8161299481653 241.61464140247605; 785.9251092419285 1708.5153389919187 485.3986936556763 765.6967877385282; 309.8161299481654 485.39869365567637 266.94660041782066 305.25080391581776; 241.61464140247605 765.6967877385282 305.25080391581776 886.2985403373846], [0.007770233480390409 -0.011009070285414424 … 0.007628928298945681 -76.78147650807796; -0.008207984630832166 0.016998830640420515 … -0.020229304424980027 0.0055804901744127555; -0.006716724522825013 0.015349359734320167 … 0.00965981047948361 -24.821817094463924; 0.027045794349309205 -0.021374536238511155 … 0.02568611436415935 -257.2160433157398], SubArray{Float64, 1, Matrix{Float64}, Tuple{Base.Slice{Base.OneTo{Int64}}, Int64}, true}[[0.582214916426889, 0.14092902448354105, 0.05402188629156628, 0.2228341727980035], [1.0000000000027756e-5, 0.41258807922799495, 0.1339463980931601, 0.45345552267884487], [1.0e-5, 0.5069557466869863, 0.10389750859810855, 0.38913674471490506], [0.4475320713476874, 0.028059304873610785, 0.06263047002167084, 0.46177815375703096], [1.0000000000055512e-5, 0.3248282288404811, 0.11654224261353534, 0.5586195285459835], [1.0000000000027756e-5, 0.4937610338831034, 0.1284319653692813, 0.3777970007476152], [1.0e-5, 1.0e-5, 0.201860121489011, 0.798119878510989], [1.0e-5, 0.5683277274079043, 0.04197256837358268, 0.3896897042185129], [0.432818900266916, 0.12616848672266018, 1.0000000000027756e-5, 0.4410026130104237], [1.0e-5, 1.0e-5, 1.0e-5, 0.9999699999999999] … [0.9999699999999999, 1.0e-5, 1.0e-5, 1.0e-5], [0.20421694513583225, 1.0e-5, 0.7957630548641677, 1.0e-5], [1.0e-5, 0.21768156700974545, 0.22585943488504254, 0.556448998105212], [0.9999699999999999, 1.0e-5, 1.0e-5, 1.0e-5], [1.0e-5, 1.0e-5, 0.2670590285882958, 0.7329209714117042], [0.47701859813822794, 1.0e-5, 1.0e-5, 0.522961401861772], [0.3995928820841258, 1.0e-5, 0.14401354326622046, 0.4563835746496538], [1.0000000000055512e-5, 0.05193865569654182, 0.2338316743309882, 0.71421966997247], [0.9999699999999999, 1.0e-5, 1.0e-5, 1.0e-5], [0.9999699999999999, 1.0e-5, 1.0e-5, 1.0e-5]], SubArray{Float64, 1, Matrix{Float64}, Tuple{Base.Slice{Base.OneTo{Int64}}, Int64}, true}[[0.5822686983061394, 0.14157978700364626, 0.05422353338551516, 0.2219279813046991], [1.0e-5, 0.4122387868461372, 0.13369030486485345, 0.45406090828900936], [1.0e-5, 0.5061059458488054, 0.10326456862507746, 0.3906194855261171], [0.44755321977495693, 0.027942585975311938, 0.06239837383851021, 0.462105820411221], [1.0e-5, 0.325005603335161, 0.11657610868601087, 0.5584082879788281], [1.0e-5, 0.4936202131430932, 0.1285433768248627, 0.37782641003204415], [1.0e-5, 1.0e-5, 0.20177013123801546, 0.7982098687619844], [1.0e-5, 0.567854974015666, 0.041866892126303186, 0.3902681338580307], [0.4328984655887788, 0.12661866186872353, 1.0e-5, 0.4404728725424976], [1.0e-5, 1.0e-5, 1.0e-5, 0.9999699999999999] … [0.9999699999999999, 1.0e-5, 1.0e-5, 1.0e-5], [0.20403525394233787, 1.0e-5, 0.795944746057662, 1.0e-5], [1.0e-5, 0.2175467131256098, 0.22553880954161065, 0.5569044773327795], [0.9999699999999999, 1.0e-5, 1.0e-5, 1.0e-5], [1.0e-5, 1.0e-5, 0.2663651985736278, 0.7336148014263721], [0.477083438661804, 1.0e-5, 1.0e-5, 0.522896561338196], [0.3996465219669129, 1.0e-5, 0.14411265962056485, 0.4562308184125222], [1.0e-5, 0.05168193530267726, 0.23354006083142623, 0.7147680038658963], [0.9999699999999999, 1.0e-5, 1.0e-5, 1.0e-5], [0.9999699999999999, 1.0e-5, 1.0e-5, 1.0e-5]], SubArray{Float64, 1, Matrix{Float64}, Tuple{Base.Slice{Base.OneTo{Int64}}, Int64}, true}[[0.582214916426889, 0.14092902448354105, 0.05402188629156628, 0.2228341727980035], [1.0000000000027756e-5, 0.41258807922799495, 0.1339463980931601, 0.45345552267884487], [1.0e-5, 0.5069557466869863, 0.10389750859810855, 0.38913674471490506], [0.4475320713476874, 0.028059304873610785, 0.06263047002167084, 0.46177815375703096], [1.0000000000055512e-5, 0.3248282288404811, 0.11654224261353534, 0.5586195285459835], [1.0000000000027756e-5, 0.4937610338831034, 0.1284319653692813, 0.3777970007476152], [1.0e-5, 1.0e-5, 0.201860121489011, 0.798119878510989], [1.0e-5, 0.5683277274079043, 0.04197256837358268, 0.3896897042185129], [0.432818900266916, 0.12616848672266018, 1.0000000000027756e-5, 0.4410026130104237], [1.0e-5, 1.0e-5, 1.0e-5, 0.9999699999999999] … [0.9999699999999999, 1.0e-5, 1.0e-5, 1.0e-5], [0.20421694513583225, 1.0e-5, 0.7957630548641677, 1.0e-5], [1.0e-5, 0.21768156700974545, 0.22585943488504254, 0.556448998105212], [0.9999699999999999, 1.0e-5, 1.0e-5, 1.0e-5], [1.0e-5, 1.0e-5, 0.2670590285882958, 0.7329209714117042], [0.47701859813822794, 1.0e-5, 1.0e-5, 0.522961401861772], [0.3995928820841258, 1.0e-5, 0.14401354326622046, 0.4563835746496538], [1.0000000000055512e-5, 0.05193865569654182, 0.2338316743309882, 0.71421966997247], [0.9999699999999999, 1.0e-5, 1.0e-5, 1.0e-5], [0.9999699999999999, 1.0e-5, 1.0e-5, 1.0e-5]], SubArray{Float64, 1, Matrix{Float64}, Tuple{Base.Slice{Base.OneTo{Int64}}, Int64}, true}[[0.794600708769161, 0.6295417302123216, 0.795332666017797, 0.7314117939132884], [0.8699629358466604, 0.9882835240192088, 0.9004058223965158, 0.8910746807079742], [0.9737349382463225, 0.99999, 0.8579504459370331, 0.9841868447992824], [0.9896365156352461, 0.99999, 0.9166339405314132, 0.9979607677791603], [0.6468518890092232, 0.6478882446742358, 0.494559790425589, 0.5599804804012329], [0.6743182225242905, 0.6472794791502667, 0.7235288407693508, 0.6734354662135091], [0.7469737872889323, 0.7039895414260632, 0.8789128988015523, 0.6672052923426718], [0.8607860295663952, 0.855127530928302, 0.5702185709368484, 0.9129512026702861], [0.8519524721903828, 0.8376414128223882, 0.5892841242129675, 0.8840536068250283], [0.5328553708993604, 0.46118733987300753, 0.3879047896303323, 0.553649639999572] … [0.9620399261441169, 0.9861773938986236, 0.9256286539109566, 0.9839677062672065], [0.8741312762540471, 0.6799753331701319, 0.6678787641267006, 0.8799493479739465], [0.9302414910591702, 0.9094934930781865, 0.8561830373420675, 0.9697104426569796], [0.5921661766180285, 0.7758305587523612, 0.822145442576713, 0.6655953985078696], [0.9682851330624743, 0.973439956988747, 0.9503928900333226, 0.9432971849309506], [0.8418753575433897, 0.9795046963142533, 0.73576110676302, 0.8590486768118991], [0.934462853272321, 0.7354040200346937, 0.99999, 0.8470050399575564], [0.8322509557951396, 0.639827870913738, 0.9785293750103126, 0.7676496020277787], [0.9327568172111603, 0.8596468613040379, 0.9599270223437033, 0.8107491631733151], [0.99999, 0.8877110943870553, 0.99999, 0.99999]], SubArray{Float64, 1, Matrix{Float64}, Tuple{Base.Slice{Base.OneTo{Int64}}, Int64}, true}[[0.7946379362185242, 0.6294705873253528, 0.7947761216806404, 0.7315585615635503], [0.8699568858567608, 0.9882931595924863, 0.9014162794343112, 0.8908533540490032], [0.9737288831070886, 0.99999, 0.8583960664737883, 0.9840594249932237], [0.9896451023631544, 0.99999, 0.916643911983247, 0.9979374543131986], [0.6467656418485923, 0.6479694526127282, 0.49541475699629417, 0.559774837963584], [0.6743605643204243, 0.6472086404740693, 0.7228263739828681, 0.6736236500461458], [0.7469561522385367, 0.7040609955778488, 0.8800527339886484, 0.6669619900938552], [0.8607443290730644, 0.8551055746339635, 0.570778125418224, 0.9127684360300229], [0.8519045526131043, 0.8376074684093889, 0.5896231667925191, 0.8839468953184086], [0.5328993281224814, 0.4610079681118647, 0.38537291133768914, 0.5542425554896754] … [0.9620904238107759, 0.9861790045306884, 0.9253944281744522, 0.9839850914887557], [0.8741633166232774, 0.6799008864771244, 0.6682967903170592, 0.8798032701585558], [0.9302834980465161, 0.9094488829788975, 0.8556166814167883, 0.9698126899072685], [0.5921917731312764, 0.7759738840219016, 0.822008243916343, 0.6655876537132536], [0.9682908656598623, 0.9734716336131075, 0.9502424124591029, 0.9433182434551405], [0.841859229091953, 0.9796269382104448, 0.7356924340905762, 0.8590041696522682], [0.9344774585661986, 0.7354502518248635, 0.99999, 0.846984895742093], [0.8322734576267384, 0.6397921048838265, 0.9782466651408858, 0.7677475795250771], [0.932690502090477, 0.8596295407288184, 0.9604830519644512, 0.8106984216220864], [0.99999, 0.8876821133904721, 0.99999, 0.99999]], SubArray{Float64, 1, Matrix{Float64}, Tuple{Base.Slice{Base.OneTo{Int64}}, Int64}, true}[[0.794600708769161, 0.6295417302123216, 0.795332666017797, 0.7314117939132884], [0.8699629358466604, 0.9882835240192088, 0.9004058223965158, 0.8910746807079742], [0.9737349382463225, 0.99999, 0.8579504459370331, 0.9841868447992824], [0.9896365156352461, 0.99999, 0.9166339405314132, 0.9979607677791603], [0.6468518890092232, 0.6478882446742358, 0.494559790425589, 0.5599804804012329], [0.6743182225242905, 0.6472794791502667, 0.7235288407693508, 0.6734354662135091], [0.7469737872889323, 0.7039895414260632, 0.8789128988015523, 0.6672052923426718], [0.8607860295663952, 0.855127530928302, 0.5702185709368484, 0.9129512026702861], [0.8519524721903828, 0.8376414128223882, 0.5892841242129675, 0.8840536068250283], [0.5328553708993604, 0.46118733987300753, 0.3879047896303323, 0.553649639999572] … [0.9620399261441169, 0.9861773938986236, 0.9256286539109566, 0.9839677062672065], [0.8741312762540471, 0.6799753331701319, 0.6678787641267006, 0.8799493479739465], [0.9302414910591702, 0.9094934930781865, 0.8561830373420675, 0.9697104426569796], [0.5921661766180285, 0.7758305587523612, 0.822145442576713, 0.6655953985078696], [0.9682851330624743, 0.973439956988747, 0.9503928900333226, 0.9432971849309506], [0.8418753575433897, 0.9795046963142533, 0.73576110676302, 0.8590486768118991], [0.934462853272321, 0.7354040200346937, 0.99999, 0.8470050399575564], [0.8322509557951396, 0.639827870913738, 0.9785293750103126, 0.7676496020277787], [0.9327568172111603, 0.8596468613040379, 0.9599270223437033, 0.8107491631733151], [0.99999, 0.8877110943870553, 0.99999, 0.99999]], SubArray{Float64, 2, Array{Float64, 3}, Tuple{Base.Slice{Base.OneTo{Int64}}, Base.Slice{Base.OneTo{Int64}}, Int64}, true}[[20324.008613992417 19517.205560497827 19445.052396609426 19593.620284729197; 19517.205560497827 21129.69564684323 19991.760457049983 20546.476546981514; 19445.052396609426 19991.760457049983 27061.91936795712 19733.76140174633; 19593.620284729197 20546.476546981514 19733.76140174633 20783.794235985824], [20537.320580307394 19532.61527210971 19904.474838050613 19816.064775376904; 19532.61527210971 20429.751878926836 19208.639123757057 19842.885390646392; 19904.474838050613 19208.639123757057 24043.105805147836 19529.028777521504; 19816.064775376904 19842.885390646392 19529.028777521504 20281.005129401456], [20554.33202737424 19527.167907738505 20146.012553921053 19827.729637940454; 19527.167907738512 20277.465892718952 19338.0657146504 19815.571658413777; 20146.01255392105 19338.0657146504 24509.245681961882 19667.149225394885; 19827.729637940458 19815.571658413777 19667.149225394885 20326.462531857924], [20556.41070737937 19564.363129783676 19569.14953476913 19545.65979654395; 19564.363129783676 21160.217133344173 20156.301774785272 20330.734108958746; 19569.14953476913 20156.301774785272 25935.389042991374 19607.66575933755; 19545.65979654395 20330.734108958746 19607.66575933755 20472.803926885408], [20738.68452415396 19547.04190311882 19986.87333496084 19774.520712450467; 19547.04190311882 20607.449826191758 19367.81446189019 19778.27309676111; 19986.87333496084 19367.81446189019 24115.635312216564 19508.22326067277; 19774.520712450467 19778.27309676111 19508.22326067277 20231.933071780884], [29115.766672205205 19736.911593229335 20025.31836148489 19962.334757940967; 19736.911593229335 20298.33626249491 19343.40982603822 19833.675560129097; 20025.31836148489 19343.40982603822 23889.466523741874 19533.288493945907; 19962.33475794097 19833.675560129097 19533.288493945907 20376.452799022445], [20324.696601491036 19768.31715243907 19571.894649782054 19731.684938919876; 19768.317152439075 20806.688816268073 19453.77420120726 20082.307620287003; 19571.894649782054 19453.774201207263 23346.79055965274 19154.314509536325; 19731.684938919876 20082.307620287003 19154.314509536325 20213.70327499686], [21086.11788854685 19524.422635720646 21136.020827604458 19972.3878700753; 19524.422635720646 20188.699167270217 19567.87966176365 19771.92454600974; 21136.020827604458 19567.87966176365 26788.80122868821 19901.80305390232; 19972.3878700753 19771.924546009737 19901.80305390232 20342.087580709125], [20630.330394418503 19498.715545835796 19681.119243186386 19524.670512182012; 19498.715545835796 20923.8424877803 19733.139316755387 20226.49185506534; 19681.119243186386 19733.139316755387 23969.02414737194 19718.648704449308; 19524.670512182012 20226.49185506534 19718.648704449308 20402.191260865442], [20446.465987621606 19845.666905542515 20419.297927072817 19648.570545431245; 19845.666905542515 20846.783846009883 20066.018857225496 19968.892383880248; 20419.297927072817 20066.018857225496 25251.211991353313 19914.93021724633; 19648.570545431245 19968.892383880248 19914.93021724633 20000.00935169697] … [20000.012013136115 19694.044998495727 19993.029393624973 19712.228872456348; 19694.044998495727 21304.70736265766 20559.027111921965 20518.570359894573; 19993.02939362497 20559.027111921965 27496.19528042929 19992.066301000348; 19712.228872456348 20518.57035989457 19992.066301000348 20649.219381936982], [24467.79577912119 20911.8650341461 18855.309605645212 20991.870132511227; 20911.8650341461 22304.30536982939 19119.58529600222 21788.03937092589; 18855.309605645212 19119.58529600222 20293.313019723617 19083.54212225295; 20991.870132511227 21788.03937092589 19083.54212225295 22029.958866969235], [20265.720529504815 19626.26120331209 19467.630344483172 19816.551302234795; 19626.261203312093 20947.046472290487 19170.429288011834 19966.055578938172; 19467.630344483172 19170.429288011834 22583.40605282289 19278.364551434977; 19816.551302234795 19966.055578938172 19278.364551434977 20305.293737867087], [20000.016250475015 19583.30882916866 19929.17880271616 19674.979977249706; 19583.30882916866 20833.69645194361 19910.599207547042 20318.859656570818; 19929.17880271616 19910.599207547042 24830.29519993198 19966.31480264646; 19674.979977249706 20318.859656570818 19966.31480264646 20547.415910226428], [22603.95026169848 20053.11149815297 19581.497305905228 19880.160560723336; 20053.11149815297 20976.490812875414 19214.835893986507 20232.15791907455; 19581.497305905228 19214.835893986507 22625.62466062325 19048.012007653953; 19880.160560723336 20232.15791907455 19048.012007653953 20345.17598759747], [20530.08071498536 19605.211692440156 20045.941897415705 19516.404624214025; 19605.211692440156 20998.886009443206 20209.15875088167 20307.40599497684; 20045.941897415705 20209.15875088167 25722.11215343645 19941.747516334442; 19516.404624214025 20307.405994976838 19941.74751633444 20441.189198610202], [20666.64864225557 19526.766568006435 19345.291052039345 19622.92899416531; 19526.766568006435 21482.104669623564 19783.013056694053 20223.771547515877; 19345.291052039345 19783.013056694053 23415.675962667327 19493.86923556915; 19622.92899416531 20223.771547515877 19493.86923556915 20490.336207422664], [20161.223377180504 19799.481341587463 19499.054021029686 19738.89607701232; 19799.481341587463 20880.864584502502 19303.322514294978 20163.93967566195; 19499.054021029686 19303.322514294978 22942.205947459446 19089.16440119291; 19738.89607701232 20163.93967566195 19089.16440119291 20285.72352733147], [20000.02114719698 19652.53691666853 19632.312142147246 19657.796437926452; 19652.53691666853 21076.84228656145 19791.029925493247 20375.34137743504; 19632.312142147246 19791.029925493247 24012.84358997869 19676.25199063923; 19657.796437926452 20375.34137743504 19676.25199063923 20499.803807281765], [20000.014106027465 19707.89979655304 19747.929550133194 19838.811482171674; 19707.89979655304 22045.021897104154 20875.403318563087 21565.27026454133; 19747.929550133194 20875.403318563087 25203.810149595418 20909.98526841688; 19838.811482171674 21565.270264541326 20909.98526841688 21840.790994511528]], SubArray{Float64, 1, Matrix{Float64}, Tuple{Base.Slice{Base.OneTo{Int64}}, Int64}, true}[[-20000.0278902452, -19999.657638990368, -19999.54125456821, -20000.25732032506], [-19711.042676807927, -20000.018840334767, -20000.44382380299, -19999.858582666187], [-19708.488173234004, -20000.02674741278, -20000.619894388008, -19999.80893161833], [-20000.01296204302, -20000.035916413508, -20000.59765104925, -19999.904573253116], [-19725.353708817314, -19999.908197239423, -19999.708568893617, -20000.119190170106], [-19859.248986731913, -20000.02009442893, -19999.523299720917, -20000.13965449756], [-19699.45032774874, -19955.492453739073, -20000.23812277887, -19999.944130617892], [-19766.737416368596, -20000.046887559227, -20000.532546571743, -19999.88062366939], [-20000.024160044974, -19999.731277950286, -19704.279543948513, -20000.06021615895], [-19648.588202623083, -19968.900901804824, -19914.990134627566, -20000.004675347882] … [-20000.006005808373, -19694.078000194124, -19993.110075630073, -19712.249104150604], [-20000.496578457794, -19485.33207925158, -19999.885792975976, -19472.96482157121], [-19696.46359093068, -20000.018918953847, -20000.300608013516, -19999.876317809518], [-20000.00812466358, -19583.331961456952, -19929.22799944419, -19674.9980537541], [-19800.63603095345, -19961.184388713365, -20000.970515473484, -19999.650866162294], [-20000.018882731878, -19972.40665484161, -19991.517407708634, -19999.983461587013], [-20000.035817557484, -19881.709636629846, -19999.67537067944, -20000.07376033089], [-19686.018735665253, -19999.99951361597, -20000.076162936093, -19999.979542807254], [-20000.010573017542, -19652.559772696888, -19632.357974038034, -19657.81221800508], [-20000.007052012574, -19707.95341651394, -19748.00700423404, -19838.859478292485]], SubArray{Float64, 2, Array{Float64, 3}, Tuple{Base.Slice{Base.OneTo{Int64}}, Base.Slice{Base.OneTo{Int64}}, Int64}, true}[[566.628571738167 83.90099875125914 62.70760160459943 206.69526708612761; 83.90099875125914 493.3179302661993 50.12892125483464 184.51024479111086; 62.70760160459943 50.12892125483464 103.82896536324253 202.09259891424378; 206.69526708612761 184.51024479111086 202.09259891424378 1153.4061405968855], [958.3641448625666 96.0657103671523 99.84997857047428 452.8669952750855; 96.0657103671523 8422.223541256804 173.75693630488144 541.6772331313043; 99.84997857047429 173.75693630488135 205.75467061768856 477.66509054457424; 452.8669952750855 541.6772331313044 477.66509054457424 2396.37473968966], [4787.553491320607 279.1023137653173 161.93765801627018 1347.7563046867367; 279.1023137653173 411.5348370695136 213.93028528940792 958.6175587313586; 161.93765801627018 213.93028528940792 411.75403584058546 1352.7814161727115; 1347.7563046867367 958.6175587313586 1352.7814161727115 7467.536092617086], [7767.178149087687 3862.697527547379 920.8781344728436 4829.563439493999; 3862.6975275473797 9554.786331558318 1177.9110050688953 6700.0341545802585; 920.8781344728436 1177.9110050688953 783.4548895988855 3020.6659537625974; 4829.563439493999 6700.0341545802585 3020.6659537625974 19283.020349423085], [410.42566951077333 69.19386885522066 47.63981368130034 166.26081696105726; 69.19386885522066 470.73724653646144 46.57125357358593 162.3642840607006; 47.63981368130034 46.57125357358593 86.99245751330125 148.4481762818415; 166.26081696105726 162.3642840607006 148.4481762818415 906.7796047602883], [441.6608378423226 67.8182524868344 45.58456363198682 196.36088728498288; 67.81825248683438 488.19032217377 47.412403385162335 179.80911344251018; 45.58456363198682 47.412403385162335 116.21441409086555 162.32181701959905; 196.36088728498288 179.80911344251015 162.32181701959905 1002.402513083182], [503.9229298358099 80.39658980374581 58.874083051406146 199.85393717343624; 80.39658980374581 539.4246098890644 55.11026961290292 184.30301365371213; 58.874083051406146 55.11026961290292 108.41042355622672 187.17230190911135; 199.85393717343624 184.30301365371213 187.17230190911135 1065.047904070726], [849.430931507485 93.74875249803007 81.091245853374 294.374847145811; 93.74875249803007 884.2497375684054 82.15283012480859 344.76286746770626; 81.091245853374 82.15283012480859 124.57520279265023 274.5750308482612; 294.374847145811 344.76286746770626 274.57503084826124 2106.5862234020346], [803.0756671298689 94.30617516935874 77.65861720413116 250.87872248055902; 94.30617516935874 789.8248193423992 81.23373077083103 298.8790967047208; 77.65861720413116 81.23373077083103 119.16107309648585 255.85138132750058; 250.87872248055905 298.8790967047208 255.85138132750058 1813.8260832494495], [398.8832417694709 60.480381626956614 43.20059800292722 159.6623594136319; 60.480381626956614 443.60375678229354 44.797296067346906 159.3399382436201; 43.20059800292722 44.797296067346906 75.10094630562021 145.68448614315943; 159.6623594136319 159.3399382436201 145.68448614315943 899.0940015170539] … [3018.072020431244 202.71172670529344 359.66963498405073 1663.6436721776724; 202.71172670529344 6186.653880166012 674.968457907945 2243.8190247219572; 359.66963498405073 674.968457907945 514.3018209982837 1283.2142114964613; 1663.6436721776724 2243.8190247219572 1283.2142114964613 9783.241072487388], [787.5047787921353 94.28435314400008 67.33624711829918 374.1155690677025; 94.28435314400008 566.9581944937457 56.15164653798182 222.24141714800865; 67.33624711829918 56.151646537981826 124.34313686619643 273.9116929337594; 374.1155690677026 222.24141714800865 273.9116929337594 1633.1478321182278], [1627.669953546169 255.78823966607212 141.1003459592787 660.9850428023368; 255.7882396660721 1489.9176285904578 154.1583165859123 506.9057450087949; 141.1003459592787 154.1583165859123 280.6649939459262 691.7769382351324; 660.9850428023369 506.9057450087949 691.7769382351324 5511.4760199851025], [431.7817592172977 67.98943118540842 50.96925760895643 174.1181117861671; 67.98943118540842 583.5463251115096 53.69039099167622 209.8953893774308; 50.96925760895643 53.69039099167622 101.28300981373619 177.13140541099423; 174.1181117861671 209.8953893774308 177.13140541099423 1033.2935461177208], [2912.28067234033 290.6105762344447 137.25232229757876 1141.2183757896164; 290.6105762344447 3443.7686511850484 217.09733969883405 1248.0203305948573; 137.25232229757876 217.09733969883402 832.9418782781245 554.8820210009371; 1141.2183757896164 1248.0203305948573 554.8820210009371 4569.174432194636], [821.2137333477225 144.16699671408674 76.18016516850544 293.1195042935607; 144.16699671408674 3816.5909425829636 111.1201934991942 440.5113842872693; 76.18016516850544 111.1201934991942 145.79868310117928 287.76342276551594; 293.1195042935607 440.5113842872693 287.76342276551594 1856.0394038107715], [1306.5791208861426 112.15748161968828 112.6510737025306 445.515693993082; 112.15748161968827 649.906612484158 72.63588753838884 273.4773785992364; 112.65107370253061 72.63588753838884 118.85161378623019 353.3327017080312; 445.515693993082 273.4773785992364 353.3327017080312 1914.788970412585], [650.1821214406797 81.84322018117557 59.023027883089135 273.76127310783073; 81.84322018117557 520.8311086730197 52.169654366007286 197.2850117549217; 59.02302788308914 52.169654366007286 333.9347030552088 270.49525897176716; 273.76127310783073 197.2850117549217 270.49525897176716 1339.7021125851502], [944.8744923000977 210.87018222789402 197.4712494119201 430.93057398367193; 210.87018222789402 935.5813002844052 105.64660374792102 292.4439054220507; 197.47124941192013 105.64660374792102 185.08439855823875 292.02256227961254; 430.93057398367193 292.44390542205076 292.02256227961254 1682.286841236985], [1047.3154837338877 785.9251092419285 309.8161299481653 241.61464140247605; 785.9251092419285 1708.5153389919187 485.3986936556763 765.6967877385282; 309.8161299481654 485.39869365567637 266.94660041782066 305.25080391581776; 241.61464140247605 765.6967877385282 305.25080391581776 886.2985403373846]], SubArray{Float64, 1, Matrix{Float64}, Tuple{Base.Slice{Base.OneTo{Int64}}, Int64}, true}[[0.007770233480390409, -0.008207984630832166, -0.006716724522825013, 0.027045794349309205], [-0.011009070285414424, 0.016998830640420515, 0.015349359734320167, -0.021374536238511155], [-0.06747874196181058, -126.47533832619307, 0.002986716228887687, -0.12732992969222678], [-0.11511737242070552, -24.927226237445016, -0.021825917181970134, -0.12852949450422813], [-0.009264326037800918, 0.011992961001884073, 0.011363580100436366, -0.025655309241940927], [0.007299056543518034, -0.019566503671352375, -0.013120364383844674, 0.032159741088385374], [0.008690135363860207, 0.015595850445510706, 0.014869786322051048, -0.013667826322333099], [-0.019000450771105903, 0.012470765595396172, 0.01019042457955699, -0.10512913155584869], [-0.016007075414943728, 0.014075384475613184, 0.005652536637894556, -0.061913702990143094], [-0.001941844666859538, -0.027969343086239773, -0.01892587331633866, 0.042920050965896905] … [0.013627363339328014, -0.020993829347255133, -0.01117067362963109, -0.04375485546886715], [0.00911496751036367, -0.004003612009534585, -0.008856384349900992, 0.01764736165946701], [0.009770035240974195, -0.009433777044988378, -0.017536518527551337, 0.04064013568779501], [0.0014454487025630236, 0.021321909070572964, 0.002708061115555438, -0.01781383827045424], [0.01428882385254937, 0.028443838404892396, -0.012617229914278827, -0.01815425325777653], [-0.017106663099349717, 0.0962303645699476, 0.0009694209913120311, -0.03637112041325086], [0.013776619148526237, 0.005453621113234108, -9.138562932677196, 0.019422970935315753], [0.014351748040734691, -0.004095098949083109, -0.05212730104687857, 0.041335159944987154], [0.007628928298945681, -0.020229304424980027, 0.00965981047948361, 0.02568611436415935], [-76.78147650807796, 0.0055804901744127555, -24.821817094463924, -257.2160433157398]], [0.9749512513803503 0.9749512513803503 … 0.0 0.0; 0.981569903892765 0.981569903892765 … 0.0 0.0; … ; 0.0 0.0 … 0.0 0.0; 0.0 0.0 … 0.0 0.0;;;], SubArray{Float64, 2, Array{Float64, 3}, Tuple{Base.Slice{Base.OneTo{Int64}}, Base.Slice{Base.OneTo{Int64}}, Int64}, true}[[0.9749512513803503 0.9749512513803503 … 0.0 0.0; 0.981569903892765 0.981569903892765 … 0.0 0.0; … ; 0.0 0.0 … 0.0 0.0; 0.0 0.0 … 0.0 0.0]], [-0.00019912353358042534 -8.786143277184078e-5 0.0003996715928610284; -0.001010710455337599 -0.0008883743420693424 0.002671966296975248; … ; 0.0 0.0 0.0; 0.0 0.0 0.0], [-0.00011283827391972423 -4.233761613736675e-5 0.00019677106400273203; -0.0007188156708032578 -0.0006986276927519464 0.001696388905521179; … ; 0.0 0.0 0.0; 0.0 0.0 0.0], [-0.5 -0.5 -0.5 -0.5; -0.5 0.8333333333333334 -0.16666666666666666 -0.16666666666666666; -0.5 -0.16666666666666666 0.8333333333333334 -0.16666666666666666; -0.5 -0.16666666666666666 -0.16666666666666666 0.8333333333333334], [0.0; 0.0; 0.0; 0.0;;], [1047.3154837338877; 1708.5153389919187; … ; 886.2985403373846; 0.0;;], [0.6705595953304453; 0.0022016494740073954; … ; 0.6797167005044513; 0.1923469393993006;;], [20000.014106027465; 22045.021897104154; … ; 0.0; 0.0;;], [0.16076233081185012; 0.046115945456698504; … ; -0.17815723178570345; -0.017383501184341836;;], [685.7862289240434 0.4600047136279448 … -110.60949016702281 76.78131250930491; 0.4600047136279447 -0.0005853034954839992 … 0.44816500634423034 -0.0; … ; -110.60949016702281 0.44816500634423034 … 543.1400346027904 257.21271509888857; 76.78131250930491 -0.0 … 257.21271509888857 -0.006306245873800798;;;], [2163.1821361956468 144.60904435156553 … -2001.9795123398549 161.09418147682655; 144.60904435156553 755.2723625330268 … -275.52072997020105 -130.90032710809507; … ; -2001.9795123398549 -275.52072997020105 … 21840.790994511528 19838.90594006875; 161.09418147682655 -130.90032710809507 … 19838.90594006875 -0.0138550945177498;;;], Bool[0; 1; 0; 0;;], SubArray{Float64, 1, Matrix{Float64}, Tuple{Base.Slice{Base.OneTo{Int64}}, Int64}, true}[[0.0, 0.0, 0.0, 0.0]], SubArray{Float64, 1, Matrix{Float64}, Tuple{Base.Slice{Base.OneTo{Int64}}, Int64}, true}[[1047.3154837338877, 1708.5153389919187, 266.94660041782066, 886.2985403373846, 0.0]], SubArray{Float64, 1, Matrix{Float64}, Tuple{Base.Slice{Base.OneTo{Int64}}, Int64}, true}[[0.6705595953304453, 0.0022016494740073954, 1.0, 0.6797167005044513, 0.1923469393993006]], SubArray{Float64, 1, Matrix{Float64}, Tuple{Base.Slice{Base.OneTo{Int64}}, Int64}, true}[[20000.014106027465, 22045.021897104154, 25203.810149595418, 21840.790994511528, 0.0, 0.0]], SubArray{Float64, 1, Matrix{Float64}, Tuple{Base.Slice{Base.OneTo{Int64}}, Int64}, true}[[0.16076233081185012, 0.046115945456698504, 1.0, 0.00019140109132460083, -0.17815723178570345, -0.017383501184341836]], SubArray{Float64, 2, Array{Float64, 3}, Tuple{Base.Slice{Base.OneTo{Int64}}, Base.Slice{Base.OneTo{Int64}}, Int64}, true}[[685.7862289240434 0.4600047136279448 … -110.60949016702281 76.78131250930491; 0.4600047136279447 -0.0005853034954839992 … 0.44816500634423034 -0.0; … ; -110.60949016702281 0.44816500634423034 … 543.1400346027904 257.21271509888857; 76.78131250930491 -0.0 … 257.21271509888857 -0.006306245873800798]], SubArray{Float64, 2, Array{Float64, 3}, Tuple{Base.Slice{Base.OneTo{Int64}}, Base.Slice{Base.OneTo{Int64}}, Int64}, true}[[2163.1821361956468 144.60904435156553 … -2001.9795123398549 161.09418147682655; 144.60904435156553 755.2723625330268 … -275.52072997020105 -130.90032710809507; … ; -2001.9795123398549 -275.52072997020105 … 21840.790994511528 19838.90594006875; 161.09418147682655 -130.90032710809507 … 19838.90594006875 -0.0138550945177498]], SubArray{Bool, 1, Matrix{Bool}, Tuple{Base.Slice{Base.OneTo{Int64}}, Int64}, true}[[0, 1, 0, 0]], [1; 2; 3; 4;;], SubArray{Int64, 1, Matrix{Int64}, Tuple{Base.Slice{Base.OneTo{Int64}}, Int64}, true}[[1, 2, 3, 4]], -2.9888459670782355e6, -2.9888456882726927e6), [1, 2, 2, 2, 2, 2, 2, 2, 2, 2 … 1, 4, 2, 1, 2, 2, 2, 2, 1, 1], [5800, 5691, 5677, 5688, 5775, 5769, 5668, 5673, 5674, 5675 … 29537, 14293, 30551, 23892, 35746, 35747, 3783, 40709, 50996, 4707])
The following shows the clustering result of the samples:
```julia
clusters
```
379-element Vector{Int64}:
1
2
2
2
2
2
2
2
2
2
1
2
2
⋮
2
2
1
4
2
1
2
2
2
2
1
1
And the following shows the SNPs selected.
```julia
aims
```
10000-element Vector{Int64}:
5800
5691
5677
5688
5775
5769
5668
5673
5674
5675
5676
5679
5683
⋮
12387
21962
29537
14293
30551
23892
35746
35747
3783
40709
50996
4707
The allele frequencies can be shown as in:
```julia
d.p
```
4×10000 Matrix{Float64}:
0.794601 0.869963 0.973735 0.989637 … 0.832251 0.932757 0.99999
0.629542 0.988284 0.99999 0.99999 0.639828 0.859647 0.887711
0.795333 0.900406 0.85795 0.916634 0.978529 0.959927 0.99999
0.731412 0.891075 0.984187 0.997961 0.76765 0.810749 0.99999
!!! The order of alleles is in the order of index (as in `sort(aim)`). This can be verified by checking the `.bim` file generated along with the newly filtered `.bed` file.
The admixture proportions can be viewed by:
```julia
d.q
```
4×379 Matrix{Float64}:
0.582215 1.0e-5 1.0e-5 0.447532 … 1.0e-5 0.99997 0.99997
0.140929 0.412588 0.506956 0.0280593 0.0519387 1.0e-5 1.0e-5
0.0540219 0.133946 0.103898 0.0626305 0.233832 1.0e-5 1.0e-5
0.222834 0.453456 0.389137 0.461778 0.71422 1.0e-5 1.0e-5
```julia
d.ll_new
```
-2.9888456882726927e6
## Multi-threading
Multi-threading is supported by default if the julia session is launched with multiple threads. For example, one may launch Julia with 8 thread using the following command:
```bash
julia -t 8
```
## GPU support
GPU is enabled by setting the keyword argument `use_gpu` to `true`. The parts computing gradients and Hessians of the loglikelihood is moved to GPU.
| OpenADMIXTURE | https://github.com/OpenMendel/OpenADMIXTURE.jl.git |
|
[
"MIT"
] | 0.2.8 | 1bd1095d53392e953ecf4e9f84db2016774d380d | code | 9893 | module OceanographyCruises
using StaticArrays, Dates
using FieldMetadata, FieldDefaults
using PrettyTables
using Unitful
using Unitful: °
using Distances, TravelingSalesmanHeuristics
using RecipesBase
"""
Station
Type containing `(lat,lon)` information.
(And optionally a `name` and a `date`).
"""
@default_kw struct Station <: FieldVector{2, Float64}
lat::Float64 | NaN
lon::Float64 | NaN
name::String | ""
date::DateTime | Date(0)
end
pretty_data(st::Station) = [st.name (st.date == Date(0) ? "" : st.date) st.lat st.lon]
Base.show(io::IO, m::MIME"text/plain", st::Station) = println(io, string(st))
Base.show(io::IO, st::Station) = println(io, string(st))
function Base.string(st::Station)
name = st.name == "" ? "Unnamed station " : "Station $(st.name) "
date = st.date == Date(0) ? "" : "$(st.date) "
return string(name, date, "($(latstring(st.lat)), $(lonstring(st.lon)))")
end
latstring(lat) = string(round(abs(lat)*10)/10, lat < 0 ? "S" : "N")
lonstring(lon) = string(round(abs(lon)*10)/10, lon < 0 ? "W" : "E")
# shift longitude for cruises that cross 0 to (-180,180)
shiftlon(lon; baselon=0) = mod(lon - baselon, 360) + baselon
shiftlon(st::Station; baselon=0) = Station(st.lat, shiftlon(st.lon, baselon=baselon), st.name, st.date)
"""
CruiseTrack
Compact type containing cruise track information:
- cruise name
- stations
"""
@default_kw struct CruiseTrack
name::String | ""
stations::Vector{Station} | Station[]
end
Base.length(ct::CruiseTrack) = length(ct.stations)
Base.isempty(ct::CruiseTrack) = isempty(ct.stations)
pretty_data(ct::CruiseTrack) = reduce(vcat, [pretty_data(st) for st in ct.stations])
function Base.show(io::IO, ::MIME"text/plain", ct::CruiseTrack)
if isempty(ct)
println("Empty cruise $(ct.name)")
else
println("Cruise $(ct.name)")
pretty_table(pretty_data(ct); header=[:Station, :Date, :Lat, :Lon])
end
end
latitudes(ct::CruiseTrack) = [st.lat for st in ct.stations]
longitudes(ct::CruiseTrack) = [st.lon for st in ct.stations]
# shift longitude for cruises that cross 0 to (-180,180)
shiftlon(ct::CruiseTrack; baselon=0) = CruiseTrack(ct.name, shiftlon.(ct.stations, baselon=baselon))
function autoshift(ct::CruiseTrack)
if any([0 ≤ st.lon < 90 for st in ct.stations]) && any([270 ≤ st.lon < 360 for st in ct.stations])
shiftlon(ct, baselon=-180)
else
ct
end
end
"""
DepthProfile
A depth profile at a given station.
"""
@default_kw struct DepthProfile{V}
station::Station | Station()
depths::Vector{Float64} | Float64[]
values::Vector{V} | Float64[]
DepthProfile(st,d,v::Vector{V}) where {V} = (length(d) ≠ length(v)) ? error("`depths` and `values` must have same length") : new{V}(st,d,v)
end
Base.length(p::DepthProfile) = length(p.depths)
Base.isempty(p::DepthProfile) = isempty(p.depths)
pretty_data(p::DepthProfile) = [p.depths p.values]
pretty_data(p::DepthProfile{V}) where {V <: Quantity} = [p.depths ustrip.(p.values)]
function Base.show(io::IO, m::MIME"text/plain", p::DepthProfile)
if isempty(p)
println("Empty profile at ", string(p.station))
else
println("Depth profile at ", string(p.station))
pretty_table(pretty_data(p); header=[:Depth, :Value])
end
end
function Base.show(io::IO, m::MIME"text/plain", p::DepthProfile{V}) where {V <: Quantity}
if isempty(p)
println("Empty profile at ", string(p.station))
else
println("Depth profile at ", string(p.station))
pretty_table(pretty_data(p); header=[:Depth, Symbol("Value [$(unit(V))]")])
end
end
Base.:*(pro::DepthProfile, q::Quantity) = DepthProfile(pro.station, pro.depths, pro.values .* q)
Base.:-(pro1::DepthProfile, pro2::DepthProfile) = DepthProfile(pro1.station, pro1.depths, pro1.values .- pro2.values)
Unitful.uconvert(u, pro::DepthProfile) = DepthProfile(pro.station, pro.depths, uconvert.(u, pro.values))
# shift longitude for cruises that cross 0 to (-180,180)
shiftlon(pro::DepthProfile; baselon=0) = DepthProfile(shiftlon(pro.station, baselon=baselon), pro.depths, pro.values)
"""
Transect
A transect of depth profiles for a given tracer.
"""
@default_kw struct Transect{V}
tracer::String | ""
cruise::String | ""
profiles::Vector{DepthProfile{V}} | DepthProfile{Float64}[]
end
Base.length(t::Transect) = length(t.profiles)
function Base.show(io::IO, m::MIME"text/plain", t::Transect)
if isempty(t)
println("Empty transect")
else
println("Transect of $(t.tracer)")
show(io, m, CruiseTrack(t))
end
end
CruiseTrack(t::Transect) = CruiseTrack(stations=[p.station for p in t.profiles], name=t.cruise)
Base.isempty(t::Transect) = isempty(t.profiles)
Base.:*(t::Transect, q::Quantity) = Transect(t.tracer, t.cruise, t.profiles .* q)
Base.:-(t1::Transect, t2::Transect) = Transect("$(t1.tracer)˟", t1.cruise, t1.profiles .- t2.profiles)
Unitful.uconvert(u, t::Transect) = Transect(t.tracer, t.cruise, uconvert.(u, t.profiles))
# shift longitude for cruises that cross 0 to (-180,180)
shiftlon(t::Transect; baselon=0) = Transect(t.tracer, t.cruise, shiftlon.(t.profiles, baselon=baselon))
function autoshift(t::Transect)
if any([0 ≤ pro.station.lon < 90 for pro in t.profiles]) && any([270 ≤ pro.station.lon < 360 for pro in t.profiles])
shiftlon(t, baselon=-180)
else
t
end
end
latitudes(t::Transect) = [pro.station.lat for pro in t.profiles]
longitudes(t::Transect) = [pro.station.lon for pro in t.profiles]
"""
Transects
A collection of transects for a given tracer.
"""
@default_kw struct Transects{V}
tracer::String | ""
cruises::Vector{String} | String[]
transects::Vector{Transect{V}} | Transect{Float64}[]
end
function Base.show(io::IO, m::MIME"text/plain", ts::Transects)
println("Transects of $(ts.tracer)")
print("(Cruises ")
[print("$c, ") for c in ts.cruises[1:end-1]]
println("and $(last(ts.cruises)).)")
end
Base.:*(ts::Transects, q::Quantity) = Transects(ts.tracer, ts.cruises, ts.transects .* q)
Base.:*(q::Quantity, ts::Transects) = ts * q
Base.:-(ts1::Transects, ts2::Transects) = Transects("$(ts1.tracer)˟", ts1.cruises, ts1.transects .- ts2.transects)
Unitful.uconvert(u, ts::Transects) = Transects(ts.tracer, ts.cruises, uconvert.(u, ts.transects ))
# shift longitude for cruises that cross 0 to (-180,180)
shiftlon(ts::Transects; baselon=0) = Transects(ts.tracer, ts.cruises, shiftlon.(t.transects, baselon=baselon))
autoshift(ts::Transects) = Transects(ts.tracer, ts.cruises, autoshift.(ts.transects))
import Base: sort, sortperm, getindex
getindex(ts::Transects, i::Int) = ts.transects[i]
"""
sort(t::Transect)
Sorts the transect using a travelling salesman problem heuristic.
"""
sort(t::Transect; start=cruise_default_start(t)) = Transect(t.tracer, t.cruise, [t for t in t.profiles[sortperm(t; start=start)]])
sort(ct::CruiseTrack; start=cruise_default_start(ct)) = CruiseTrack(name=ct.name, stations=ct.stations[sortperm(ct; start=start)])
function sortperm(t::Union{CruiseTrack, Transect}; start=cruise_default_start(t))
t = autoshift(t)
n = length(t)
lats = latitudes(t)
lons = longitudes(t)
pts = [lons lats]
dist_mat = zeros(n+1, n+1)
dist_mat[1:n,1:n] .= pairwise(Haversine(1), pts, dims=1)
path, cost = solve_tsp(dist_mat)
i = findall(path .== n+1)
if length(i) == 1
path = vcat(path[i[1]+1:end-1], path[1:i[1]-1])
else
path = path[2:end-1]
end
start == :south && pts[path[1],2] > pts[path[end],2] && reverse!(path)
start == :west && pts[path[1],1] > pts[path[end],1] && reverse!(path)
return path
end
export sort, sortperm, getindex
function cruise_default_start(t)
t = autoshift(t)
extremalat = extrema(latitudes(t))
extremalon = extrema(longitudes(t))
Δlat = extremalat[2] - extremalat[1]
Δlon = extremalon[2] - extremalon[1]
Δlon > Δlat ? :west : :south
end
"""
diff(t)
Computes the distance in km of each segment of the transect
"""
function Base.diff(t::Union{CruiseTrack,Transect})
t = autoshift(t) # not sure this is needed since Haversine takes lat and lon?
n = length(t)
lats = latitudes(t)
lons = longitudes(t)
pts = [lons lats]
return [Haversine(6371.0)(pts[i,:], pts[i+1,:]) for i in 1:n-1] * u"km"
end
export diff
function Base.range(departure::Station, arrival::Station; length::Int64, westmostlon=-180)
lonstart = lonconvert(departure.lon, westmostlon)
lonend = lonconvert(arrival.lon, westmostlon)
lonstart - lonend > 180 && (lonend += 360)
lonstart - lonend < -180 && (lonstart += 360)
lats = range(departure.lat, arrival.lat, length=length)
lons = range(lonstart, lonend, length=length)
names = string.("$(departure.name) to $(arrival.name) ", 1:length)
return [Station(lat=x[1], lon=lonconvert(x[2], westmostlon), name=x[3]) for x in zip(lats, lons, names)]
end
lonconvert(lon, westmostlon=-180) = mod(lon - westmostlon, 360) + westmostlon
export CruiseTrack, Station, DepthProfile, Transect, Transects
export latitudes, longitudes
Unitful.unit(t::Transect) = unit(t.profiles[1].values[1])
# nested functions
# TODO: Fix this so that one can use the dataframes approach
# Note: maximum(transect/profile) is not obviously supposed to be the tracer's max
for f in (:maximum, :minimum)
@eval begin
import Base: $f
"""
$($f)(t)
Applies `$($f)` recursively.
"""
$f(ts::Transects) = $f($f(t) for t in ts.transects)
$f(t::Transect) = $f($f(pro) for pro in t.profiles)
$f(pro::DepthProfile) = $f(pro.values)
export $f
end
end
# recipe TODO split this file into a few different ones that make sens and include them all here
include("recipes.jl")
end # module
| OceanographyCruises | https://github.com/briochemc/OceanographyCruises.jl.git |
|
[
"MIT"
] | 0.2.8 | 1bd1095d53392e953ecf4e9f84db2016774d380d | code | 1973 |
convertdepth(depth::Real) = depth * u"m"
convertdepth(depth::Quantity{U, Unitful.𝐋, V}) where {U,V} = depth
convertdepth(x) = error("Not a valid depth")
"""
plotscattertransect(t)
Plots a scatter of the discrete obs of `t` in (lat,depth) space.
"""
@userplot PlotScatterTransect
@recipe function f(p::PlotScatterTransect)
transect, = p.args
x, y, v = scattertransect(transect)
@series begin
seriestype := :scatter
yflip := true
marker_z --> v
markershape --> :circle
clims --> (0, 1) .* maximum(transect)
label --> ""
yguide --> "Depth"
xguide --> "Distance"
x, y
end
end
function scattertransect(t::Transect)
depths = reduce(vcat, pro.depths for pro in t.profiles)
values = reduce(vcat, pro.values for pro in t.profiles)
distances = reduce(vcat, [fill(d, length(pro)) for (d,pro) in zip(vcat(0.0u"km", cumsum(diff(t))), t.profiles)])
return distances, convertdepth.(depths), values
end
export scattertransect
"""
plotcruisetrack(t)
Plots the cruise track of `t` in (lat,lon) space.
"""
@userplot PlotCruiseTrack
@recipe function f(p::PlotCruiseTrack, central_longitude=200°)
wlon = central_longitude - 180°
ct, = p.args
ctlon, ctlat = [s.lon for s in ct.stations]°, [s.lat for s in ct.stations]°
@series begin
label := ""
xguide --> ""
yguide --> ""
markershape --> :circle
markersize --> 4
color_palette --> :tab10
markercolor --> 4
markerstrokewidth --> 0
[mod(ctlon[1] - wlon, 360°) + wlon], [ctlat[1]]
end
@series begin
color_palette --> :tab10
seriescolor --> 4
xguide --> ""
yguide --> ""
title --> ct.name
markershape --> :circle
markersize --> 1
markercolor --> :black
titlefontsize --> 10
linewidth --> 2
mod.(ctlon .- wlon, 360°) .+ wlon, ctlat
end
end
| OceanographyCruises | https://github.com/briochemc/OceanographyCruises.jl.git |
|
[
"MIT"
] | 0.2.8 | 1bd1095d53392e953ecf4e9f84db2016774d380d | code | 1970 | using Test, OceanographyCruises, Unitful, Plots
st = Station(name="ALOHA", lat=22.75, lon=-158)
N = 10
stations = [Station(name=string(i), lat=i, lon=2i) for i in 1:N]
ct = CruiseTrack(stations=stations, name="TestCruiseTrack")
depths = [10, 50, 100, 200, 300, 400, 500, 700, 1000, 2000, 3000, 5000]
@testset "Station" begin
println("Station example:")
show(stdout, MIME("text/plain"), st)
@test st isa Station
@test st.name == "ALOHA"
@test st.lon == -158
@test st.lat == 22.75
end
@testset "CruiseTrack" begin
println("CruiseTrack example:")
show(stdout, MIME("text/plain"), ct)
@test ct isa CruiseTrack
@test ct.name == "TestCruiseTrack"
@test latitudes(ct) == collect(1:N)
@test longitudes(ct) == 2collect(1:N)
@testset "lon/lat" for i in 1:N
st = ct.stations[i]
@test st.lon == 2i
@test st.lat == i
end
@test plotcruisetrack(ct) isa Plots.Plot
end
@testset "DepthProfile" begin
values = rand(length(depths))
p = DepthProfile(station=st, depths=depths, values=values)
println("profile without units:")
show(stdout, MIME("text/plain"), p)
p = DepthProfile(station=st, depths=depths, values=values * u"nM")
println("profile with units:")
show(stdout, MIME("text/plain"), p)
@test p isa DepthProfile
@test p.station == st
@test p.values == values * u"nM"
@test p.depths == depths
end
@testset "Transect" begin
idepths = [rand(Bool, length(depths)) for i in 1:N]
profiles = [DepthProfile(station=stations[i], depths=depths[idepths[i]], values=rand(12)[idepths[i]]) for i in 1:N]
t = Transect(tracer="PO₄", cruise=ct.name, profiles=profiles)
println("Transect example:")
show(stdout, MIME("text/plain"), t)
@test t isa Transect
@test t.tracer == "PO₄"
@test t.cruise == "TestCruiseTrack"
@test t.profiles == profiles
@test plotscattertransect(t) isa Plots.Plot
@test sort(t) isa Transect
end
| OceanographyCruises | https://github.com/briochemc/OceanographyCruises.jl.git |
|
[
"MIT"
] | 0.2.8 | 1bd1095d53392e953ecf4e9f84db2016774d380d | docs | 3134 | # OceanographyCruises.jl
*An interface for dealing with oceanographic cruises data*
<p>
<a href="https://github.com/briochemc/OceanographyCruises.jl/actions">
<img src="https://img.shields.io/github/workflow/status/briochemc/OceanographyCruises.jl/Mac%20OS%20X?label=OSX&logo=Apple&logoColor=white&style=flat-square">
</a>
<a href="https://github.com/briochemc/OceanographyCruises.jl/actions">
<img src="https://img.shields.io/github/workflow/status/briochemc/OceanographyCruises.jl/Linux?label=Linux&logo=Linux&logoColor=white&style=flat-square">
</a>
<a href="https://github.com/briochemc/OceanographyCruises.jl/actions">
<img src="https://img.shields.io/github/workflow/status/briochemc/OceanographyCruises.jl/Windows?label=Windows&logo=Windows&logoColor=white&style=flat-square">
</a>
<a href="https://codecov.io/gh/briochemc/OceanographyCruises.jl">
<img src="https://img.shields.io/codecov/c/github/briochemc/OceanographyCruises.jl/master?label=Codecov&logo=codecov&logoColor=white&style=flat-square">
</a>
</p>
Create a `Station`,
```julia
julia> using OceanographyCruises
julia> st = Station(name="ALOHA", lat=22.75, lon=-158)
Station ALOHA (22.8N, 158.0W)
```
a `CruiseTrack` of stations,
```julia
julia> N = 10 ;
julia> stations = [Station(name=string(i), lat=i, lon=2i) for i in 1:N] ;
julia> ct = CruiseTrack(stations=stations, name="TestCruiseTrack")
Cruise TestCruiseTrack
┌─────────┬──────┬──────┬──────┐
│ Station │ Date │ Lat │ Lon │
├─────────┼──────┼──────┼──────┤
│ 1 │ │ 1.0 │ 2.0 │
│ 2 │ │ 2.0 │ 4.0 │
│ 3 │ │ 3.0 │ 6.0 │
│ 4 │ │ 4.0 │ 8.0 │
│ 5 │ │ 5.0 │ 10.0 │
│ 6 │ │ 6.0 │ 12.0 │
│ 7 │ │ 7.0 │ 14.0 │
│ 8 │ │ 8.0 │ 16.0 │
│ 9 │ │ 9.0 │ 18.0 │
│ 10 │ │ 10.0 │ 20.0 │
└─────────┴──────┴──────┴──────┘
```
And make a `Transect` of `DepthProfiles` along that `CruiseTrack`
```julia
julia> depths = [10, 50, 100, 200, 300, 400, 500, 700, 1000, 2000, 3000, 5000] ;
julia> idepths = [rand(Bool, length(depths)) for i in 1:N] ;
julia> profiles = [DepthProfile(station=stations[i], depths=depths[idepths[i]], values=rand(12)[idepths[i]]) for i in 1:N] ;
julia> t = Transect(tracer="PO₄", cruise=ct.name, profiles=profiles)
Transect of PO₄
Cruise TestCruiseTrack
┌─────────┬──────┬──────┬──────┐
│ Station │ Date │ Lat │ Lon │
├─────────┼──────┼──────┼──────┤
│ 1 │ │ 1.0 │ 2.0 │
│ 2 │ │ 2.0 │ 4.0 │
│ 3 │ │ 3.0 │ 6.0 │
│ 4 │ │ 4.0 │ 8.0 │
│ 5 │ │ 5.0 │ 10.0 │
│ 6 │ │ 6.0 │ 12.0 │
│ 7 │ │ 7.0 │ 14.0 │
│ 8 │ │ 8.0 │ 16.0 │
│ 9 │ │ 9.0 │ 18.0 │
│ 10 │ │ 10.0 │ 20.0 │
└─────────┴──────┴──────┴──────┘
julia> t.profiles[3]
Depth profile at Station 3 (3.0N, 6.0E)
┌────────┬────────────────────┐
│ Depth │ Value │
├────────┼────────────────────┤
│ 50.0 │ 0.519255214063679 │
│ 300.0 │ 0.6289521421572468 │
│ 500.0 │ 0.8564006614918445 │
│ 5000.0 │ 0.7610393670925686 │
└────────┴────────────────────┘
```
| OceanographyCruises | https://github.com/briochemc/OceanographyCruises.jl.git |
|
[
"MIT"
] | 0.1.3 | 3f9ccd1115b939d87118aa3a7687a00001f109a3 | code | 205 | using Documenter, Waveforms
makedocs(
modules=[Waveforms],
sitename="Waveforms.jl",
pages=[
"Home" => "index.md",
],
)
deploydocs(
repo="github.com/Paalon/Waveforms.jl.git",
)
| Waveforms | https://github.com/Paalon/Waveforms.jl.git |
|
[
"MIT"
] | 0.1.3 | 3f9ccd1115b939d87118aa3a7687a00001f109a3 | code | 2329 | module Waveforms
export
squarewave,
squarewave1,
sawtoothwave,
sawtoothwave1,
trianglewave,
trianglewave1
@doc raw"""
squarewave(x)
Compute ``2\pi``-periodic square wave of `x` with a peak amplitude ``1``.
"""
squarewave(x::Real) = ifelse(mod2pi(x) < π, 1.0, -1.0)
squarewave(::Missing) = missing
@doc raw"""
squarewave(x, θ)
Compute ``2\pi``-periodic square wave of `x` with a duty cycle `θ`
and a peak amplitude ``1``.
"""
function squarewave(x::Real, θ::Real)
0 ≤ θ ≤ 1 || throw(DomainError(θ, "squwarewave(x, θ) is only defined for 0 ≤ θ ≤ 1."))
ifelse(mod2pi(x) < 2π * θ, 1.0, -1.0)
end
squarewave(::Missing, ::Real) = missing
squarewave(::Real, ::Missing) = missing
squarewave(::Missing, ::Missing) = missing
@doc raw"""
squarewave1(x)
Compute ``1``-periodic square wave of `x` with a peak amplitude ``1``.
"""
squarewave1(x::Real) = ifelse(mod(x, 1) < 1/2, 1.0, -1.0)
squarewave1(::Missing) = missing
@doc raw"""
squarewave1(x, θ)
Compute ``1``-periodic square wave of `x` with a duty cycle `θ`
and a peak amplitude ``1``.
"""
function squarewave1(x::Real, θ::Real)
0 ≤ θ ≤ 1 || throw(DomainError(θ, "squwarewave1(x, θ) is only defined for 0 ≤ θ ≤ 1."))
ifelse(mod(x, 1) < θ, 1.0, -1.0)
end
squarewave1(::Missing, ::Real) = missing
squarewave1(::Real, ::Missing) = missing
squarewave1(::Missing, ::Missing) = missing
@doc raw"""
trianglewave(x)
Compute ``2\pi``-periodic triangle wave of `x` with a peak amplitude ``1``.
"""
function trianglewave(x::Real)
modx = mod2pi(x + π/2)
ifelse(modx < π, 2modx/π - 1, -2modx/π + 3)
end
trianglewave(::Missing) = missing
@doc raw"""
trianglewave1(x)
Compute ``1``-periodic triangle wave of `x` with a peak amplitude ``1``.
"""
function trianglewave1(x::Real)
modx = mod(x + 1/4, 1.0)
ifelse(modx < 1/2, 4modx - 1, -4modx + 3)
end
trianglewave1(::Missing) = missing
@doc raw"""
sawtoothwave(x)
Compute ``2\pi``-periodic sawtooth wave of `x` with a peak amplitude ``1``.
"""
sawtoothwave(x::Real) = rem2pi(x, RoundNearest) / π
sawtoothwave(::Missing) = missing
@doc raw"""
sawtoothwave1(x)
Compute ``1``-periodic sawtooth wave of `x` with a peak amplitude ``1``.
"""
sawtoothwave1(x::Real) = rem(x, 1.0, RoundNearest) * 2
sawtoothwave1(::Missing) = missing
end # module
| Waveforms | https://github.com/Paalon/Waveforms.jl.git |
|
[
"MIT"
] | 0.1.3 | 3f9ccd1115b939d87118aa3a7687a00001f109a3 | code | 2014 | using Test, Waveforms
const x = 0.4
const y = 3π/7
const θ = 0.05
@testset "square waves" begin
@testset "squarewave" begin
@test squarewave(x) == 1.0
@test squarewave(y) == 1.0
@test isequal(squarewave(missing), missing)
@test squarewave(x, θ) == -1.0
@test squarewave(y, θ) == -1.0
@test_throws DomainError squarewave(x, -1.0)
@test_throws DomainError squarewave(x, 2.0)
@test isequal(squarewave(missing, θ), missing)
@test isequal(squarewave(x, missing), missing)
@test isequal(squarewave(missing, missing), missing)
end
@testset "squarewave1" begin
@test squarewave1(x) == 1.0
@test squarewave1(y) == 1.0
@test isequal(squarewave1(missing), missing)
@test squarewave1(x, θ) == -1.0
@test squarewave1(y, θ) == -1.0
@test_throws DomainError squarewave1(x, -1.0)
@test_throws DomainError squarewave1(x, 2.0)
@test isequal(squarewave1(missing, θ), missing)
@test isequal(squarewave1(x, missing), missing)
@test isequal(squarewave1(missing, missing), missing)
end
end
@testset "triangle waves" begin
@testset "trianglewave" begin
@test trianglewave(x) == 0.2546479089470326
@test trianglewave(y) == 0.8571428571428572
@test isequal(trianglewave(missing), missing)
end
@testset "trianglewave1" begin
@test trianglewave1(x) == 0.3999999999999999
@test trianglewave1(y) == 0.6144125938460689
@test isequal(trianglewave1(missing), missing)
end
end
@testset "sawtooth waves" begin
@testset "sawtoothwave" begin
@test sawtoothwave(x) == 0.12732395447351627
@test sawtoothwave(y) == 0.42857142857142855
@test isequal(sawtoothwave(missing), missing)
end
@testset "sawtoothwave1" begin
@test sawtoothwave1(x) == 0.8
@test sawtoothwave1(y) == 0.6927937030769655
@test isequal(sawtoothwave1(missing), missing)
end
end
| Waveforms | https://github.com/Paalon/Waveforms.jl.git |
|
[
"MIT"
] | 0.1.3 | 3f9ccd1115b939d87118aa3a7687a00001f109a3 | docs | 354 | # Waveforms.jl
This package implements waveform functions:
* Square waves
* Triangle waves
* Sawtooth waves
```@meta
CurrentModule = Waveforms
```
Square Waves
------------
```@docs
squarewave
squarewave1
```
Triangle Waves
------------
```@docs
trianglewave
trianglewave1
```
Sawtooth Waves
------------
```@docs
sawtoothwave
sawtoothwave1
```
| Waveforms | https://github.com/Paalon/Waveforms.jl.git |
|
[
"MIT"
] | 0.4.0 | 1e1db73ffd713902290f2dcffc0259cd18a6ffc7 | code | 3425 | module Binscatters
using Statistics
using StatsBase
using DataFrames
using FixedEffectModels
using RecipesBase
include("utils.jl")
"""
binscatter(df::Union{DataFrame, GroupedDataFrame}, f::FormulaTerm, n::Integer = 20;
weights::Union{Symbol, Nothing} = nothing, seriestype::Symbol = :scatter,
kwargs...)
Outputs a binned scatterplot
### Arguments
* `df`: A Table, a DataFrame or a GroupedDataFrame
* `f`: A formula created using [`@formula`](@ref). The variable(s) in the left-hand side are plotted on the y-axis. The first variable in the right-hand side is plotted on the x-axis. Add other variables for controls.
* `n`: Number of bins (default to 20).
### Keyword arguments
* `weights`: A symbol for weights
* `seriestype`: `:scatter` (the default) plots bins, `:scatterpath` adds a line to connect the bins, `:linearfit` adds a regression line (requires Plots 1.12)
* `kwargs...`: Additional attributes for [`plot`](@ref).
### Examples
```julia
using DataFrames, Binscatters, RDatasets, Plots
df = dataset("plm", "Cigar")
binscatter(df, @formula(Sales ~ Price))
# Change the number of bins
binscatter(df, @formula(Sales ~ Price), 10)
# Residualize w.r.t. controls
binscatter(df, @formula(Sales ~ Price + NDI))
binscatter(df, @formula(Sales ~ Price + fe(Year)))
# Plot multiple variables on the y-axis
binscatter(df, @formula(Sales + NDI ~ Price))
# Plot binscatters within groups
df.Post70 = df.Year .>= 70
binscatter(groupby(df, :Post70), @formula(Sales ~ Price))
# Use keyword argument from [`plot'](@ref) to customize the plot:
binscatter(df, @formula(SepalLength ~ SepalWidth), msc = :auto)
binscatter(df, @formula(SepalLength ~ SepalWidth), seriestype = :scatterpath, linecolor = :blue, markercolor = :red)
```
"""
binscatter
mutable struct Binscatter
args
end
binscatter(args...; kwargs...) = RecipesBase.plot(Binscatter(args); kwargs...)
binscatter!(args...; kwargs...) = RecipesBase.plot!(Binscatter(args); kwargs...)
binscatter!(p::AbstractPlot, args...; kwargs...) = RecipesBase.plot!(p, Binscatter(args); kwargs...)
# User recipe
@recipe function f(bs::Binscatter; weights = nothing)
df = bin(bs.args...; weights = weights)
if df isa DataFrame
cols = names(df)
N = length(cols)
x = df[!, end]
Y = Matrix(df[!, 1:(end-1)])
@series begin
seriestype --> :scatter
xguide --> cols[end]
if size(Y, 2) == 1
yguide --> cols[1]
label --> ""
else
label --> reshape(cols[1:(end-1)], 1, N-1)
end
x, Y
end
elseif df isa GroupedDataFrame
for (k, out) in pairs(df)
cols = string.(valuecols(df))
N = length(cols)
x = out[!, end]
Y = Matrix(out[!, (end-(N-1)):(end-1)])
str = "(" * join((string(k) * " = " * string(v) for (k, v) in pairs(NamedTuple(k))), ", ") * ")"
@series begin
seriestype --> :scatter
xguide --> cols[end]
if size(Y, 2) == 1
yguide --> cols[1]
label --> str
else
label --> reshape(cols[1:(end-1)], 1, N-1) .* " " .* str
end
x, Y
end
end
end
end
export @formula, fe, binscatter, binscatter!, Binscatter
end
| Binscatters | https://github.com/matthieugomez/Binscatters.jl.git |
|
[
"MIT"
] | 0.4.0 | 1e1db73ffd713902290f2dcffc0259cd18a6ffc7 | code | 3120 | function bin(df::AbstractDataFrame, xvar::Symbol, n::Integer = 20; weights::Union{Symbol, Nothing} = nothing)
if weights === nothing
cols = propertynames(df)
esample = .!ismissing.(df[!, xvar])
df = df[esample, :]
df.__cut = cut(df[!, xvar], n)
df = groupby(df, :__cut, sort = true)
return combine(df, (col => mean ∘ skipmissing => col for col in cols)...; keepkeys = false)
else
cols = propertynames(df)
esample = .!ismissing.(df[!, xvar]) .& .!ismissing.(df[!, weights])
df = df[esample, :]
df.__cut = cut(df[!, xvar], n, Weights(df[!, weights]))
df = groupby(df, :__cut, sort = true)
cols = setdiff(cols, [weights])
return combine(df, ([col, weights] => ((x, w) -> mean(collect(skipmissing(x)), Weights(w))) => col for col in cols)...; keepkeys = false)
end
end
function bin(df::AbstractDataFrame, @nospecialize(f::FormulaTerm), n::Integer = 20;
weights::Union{Symbol, Nothing} = nothing)
f_shifted = _shift(f)
df2 = partial_out(df, f_shifted; weights = weights, align = true, add_mean = true)[1]
xvar = propertynames(df2)[end]
if weights !== nothing
df2[!, weights] = df[!, weights]
end
bin(df2, xvar, n; weights = weights)
end
function bin(df::GroupedDataFrame, @nospecialize(f::FormulaTerm), n::Integer = 20;
weights::Union{Symbol, Nothing} = nothing)
combine(d -> bin(d, f, n; weights = weights), df; ungroup = false)
end
function bin(df, @nospecialize(f::FormulaTerm), n::Integer = 20;
weights::Union{Symbol, Nothing} = nothing)
bin(DataFrame(df), f, n; weights = weights)
end
# simplified version of CategoricalArrays' cut which also includes weights but does not handle missings
function cut(x::AbstractArray, ngroups::Integer)
cut(x, Statistics.quantile(x, (1:ngroups-1)/ngroups))
end
function cut(x::AbstractArray, ngroups::Integer, weights::AbstractWeights)
cut(x, StatsBase.quantile(x, weights, (1:ngroups-1)/ngroups))
end
function cut(x::AbstractArray, breaks::AbstractVector)
min_x, max_x = extrema(x)
if first(breaks) > min_x
breaks = [min_x; breaks]
end
if last(breaks) < max_x
breaks = [breaks; max_x]
end
refs = Array{UInt32}(undef, size(x))
fill_refs!(refs, x, breaks)
end
function fill_refs!(refs::AbstractArray, X::AbstractArray, breaks::AbstractVector)
upper = last(breaks)
@inbounds for i in eachindex(X)
x = X[i]
if ismissing(x)
refs[i] = 0
elseif x == upper
refs[i] = length(breaks)-1
else
refs[i] = searchsortedlast(breaks, x)
end
end
return refs
end
#transform lhs ~ x + rhs to lhs + x ~ rhs
function _shift(@nospecialize(formula::FormulaTerm))
lhs = formula.lhs
rhs = formula.rhs
if !(lhs isa Tuple)
lhs = (lhs,)
end
if !(rhs isa Tuple)
rhs = (rhs,)
end
i = findfirst(x -> !(x isa ConstantTerm), rhs)
FormulaTerm(tuple(lhs..., rhs[i]), Tuple(term for term in rhs if term != rhs[i]))
end
| Binscatters | https://github.com/matthieugomez/Binscatters.jl.git |
|
[
"MIT"
] | 0.4.0 | 1e1db73ffd713902290f2dcffc0259cd18a6ffc7 | code | 2359 |
using CSV, DataFrames, Test, Plots, Binscatters
df = DataFrame(CSV.File(joinpath(dirname(pathof(Binscatters)), "../dataset/Cigar.csv")))
# bin
out = Binscatters.bin(df, @formula(Sales ~ Price))
@test issorted(out.Price)
@test minimum(out.Price) >= minimum(df.Price)
@test maximum(out.Price) <= maximum(df.Price)
out = Binscatters.bin(df, @formula(Sales ~ log(Price)))
@test issorted(out."log(Price)")
@test minimum(out."log(Price)") >= log(minimum(df.Price))
@test maximum(out."log(Price)") <= log(maximum(df.Price))
out = Binscatters.bin(df, @formula(log(Sales) ~ log(Price)))
@test minimum(out."log(Sales)") >= log(minimum(df.Sales))
@test maximum(out."log(Sales)") <= log(maximum(df.Sales))
out = Binscatters.bin(df, @formula(Sales ~ Price), weights = :Pop)
@test minimum(out.Price) >= minimum(df.Price)
@test maximum(out.Price) <= maximum(df.Price)
Binscatters.bin(df, @formula(Sales ~ Price), 10)
Binscatters.bin(df, @formula(Sales ~ Price + NDI))
Binscatters.bin(df, @formula(Sales ~ Price + fe(State)))
Binscatters.bin(df, @formula(Sales + NDI ~ Price))
df.Price_missing = ifelse.(df.Price .>= 30, df.Price, missing)
Binscatters.bin(df, @formula(Sales ~ Price_missing))
Binscatters.bin(groupby(df, :State), @formula(Sales ~ Price))
binscatter(df, @formula(Sales ~ Price))
binscatter(df, @formula(Sales ~ Price), seriestype = :scatterpath)
binscatter(df, @formula(Sales ~ Price), seriestype = :linearfit)
binscatter(df, @formula(log(Sales) ~ log(Price)))
binscatter(df, @formula(Sales + NDI ~ Price))
binscatter(df, @formula(Sales + NDI ~ Price), seriestype = :scatterpath)
binscatter(df, @formula(Sales + NDI ~ Price), seriestype = :linearfit)
binscatter(df, @formula(Sales ~ Price + fe(State)))
binscatter(df, @formula(Sales ~ Price), weights = :Pop)
binscatter(df, @formula(Sales + NDI~ Price))
binscatter(df, @formula(Sales ~ Price + NDI))
df.dummy = df.State .>= 25
binscatter(groupby(df, :dummy), @formula(Sales ~ Price))
binscatter(groupby(df, :dummy), @formula(Sales ~ Price), seriestype = :scatterpath)
binscatter(groupby(df, :dummy), @formula(Sales ~ Price), seriestype = :linearfit)
binscatter(groupby(df, :dummy), @formula(Sales + NDI ~ Price))
binscatter(groupby(df, :dummy), @formula(Sales + NDI ~ Price), seriestype = :scatterpath)
binscatter(groupby(df, :dummy), @formula(Sales + NDI ~ Price), seriestype = :linearfit)
| Binscatters | https://github.com/matthieugomez/Binscatters.jl.git |
|
[
"MIT"
] | 0.4.0 | 1e1db73ffd713902290f2dcffc0259cd18a6ffc7 | docs | 2763 | [](https://github.com/matthieugomez/Binscatters.jl/actions)
[](http://codecov.io/github/matthieugomez/Binscatters.jl/?branch=main)
This package defines a [`Plots`](https://github.com/JuliaPlots/Plots.jl) recipe to implement the Stata command [`binscatter`](https://github.com/michaelstepner/binscatter) in Julia.
## Syntax
```julia
using DataFrames, Plots, Binscatters
binscatter(df::Union{DataFrame, GroupedDataFrame}, f::FormulaTerm, n = 20;
weights::Union{Symbol, Nothing} = nothing, seriestype::Symbol = :scatter, kwargs...)
```
### Arguments
* `df`: A DataFrame or a GroupedDataFrame
* `f`: A formula created using [`@formula`](@ref). The variable(s) in the left-hand side are plotted on the y-axis. The first variable in the right-hand side is plotted on the x-axis. Add other variables for controls.
* `n`: Number of bins (default to 20).
### Keyword arguments
* `weights`: A symbol for weights
* `seriestype`:
- `:scatter` (default) only plots bins
- `:linearfit` plots bins with a regression line
- `:scatterpath` plots bins with a connecting line
* `kwargs...`: Additional attributes from [`Plots`](http://docs.juliaplots.org/latest/).
## Examples
```julia
using DataFrames, Plots, Binscatters, RDatasets
df = dataset("datasets", "iris")
```
#### Options
You can use the typical options in [`Plot`](http://docs.juliaplots.org/latest/) to customize the plot:
```julia
binscatter(df, @formula(SepalLength ~ SepalWidth), seriestype = :scatterpath, linecolor = :blue, markercolor = :blue)
```
#### Residualizing
Length seems to be a decreasing function of with in the `iris` dataset
```julia
binscatter(df, @formula(SepalLength ~ SepalWidth), seriestype = :linearfit)
```
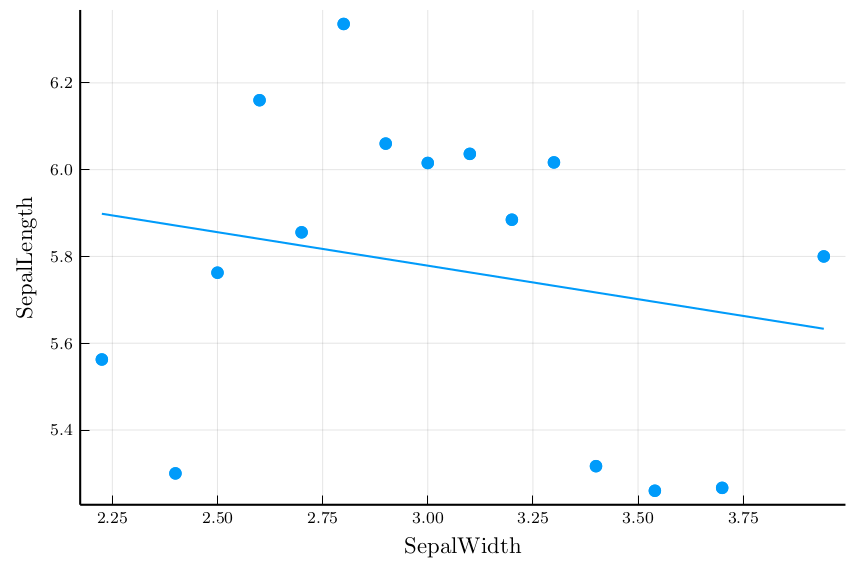
However, it is an increasing function within species. To show this, you can apply `binscatter` on a `GroupedDataFrame`
```julia
binscatter(groupby(df, :Species), @formula(SepalLength ~ SepalWidth), seriestype = :linearfit)
```
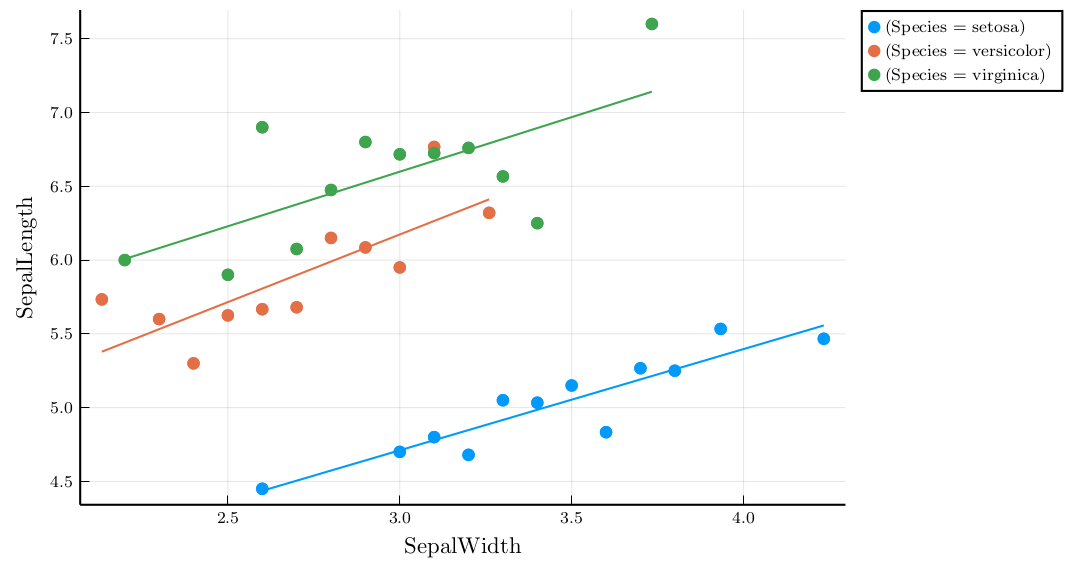
When there is a large number of groups, a better way to visualize this fact is to partial out the variables with respect to the group:
```julia
binscatter(df, @formula(SepalLength ~ SepalWidth + fe(Species)), seriestype = :linearfit)
```
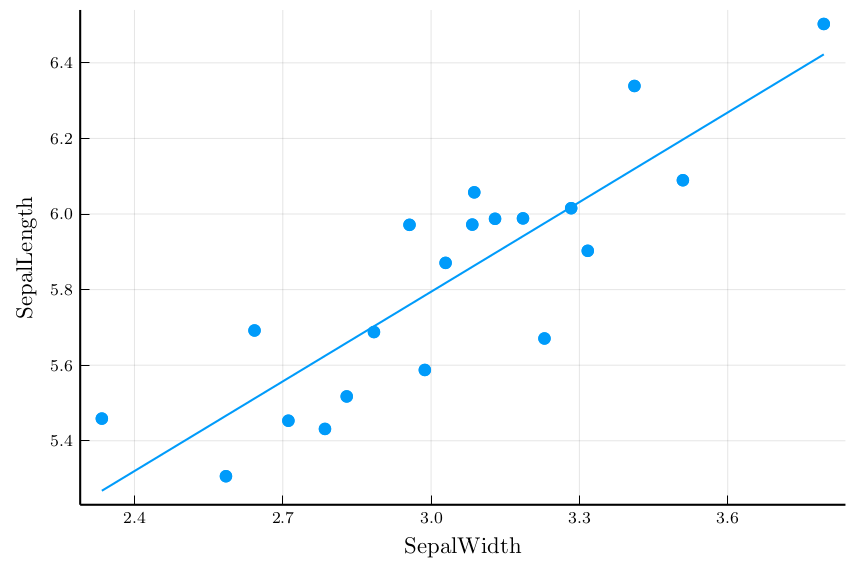
See more examples by typing `?binscatter` in the REPL.
## Installation
The package is registered in the [`General`](https://github.com/JuliaRegistries/General) registry and so can be installed at the REPL with `] add Binscatter`.
| Binscatters | https://github.com/matthieugomez/Binscatters.jl.git |
|
[
"MIT"
] | 0.5.5 | 359d601ae45b05ea9a53d303dfbf477fcaca4195 | code | 306 | #jacobigrowth2.jl
import Distributions
function jacobigrowth2(m1,m2,p,beta)
CJ=eye(p)
SJ=zeros(p, p)
for k=1:m2
Ju = sqrt(rand(Chisq(beta),1,p))
Ju *= sqrt( rand(Chisq(beta*p)) / rand(Chisq(beta*(k+m1-p))) ) / norm(Ju)
_, _, _, CJ, SJ=svd(CJ,[SJ; Ju])
end
diag(CJ)
end
| RandomMatrices | https://github.com/JuliaMath/RandomMatrices.jl.git |
|
[
"MIT"
] | 0.5.5 | 359d601ae45b05ea9a53d303dfbf477fcaca4195 | code | 1069 | #bellcurve2.jl
#Algorithm 2.1 of Random Eigenvalues by Alan Edelman
#Experiment: Generate random samples from the normal distribution
#Plot: Histogram of random samples
#Theory: The normal distribution curve
## Experiment
t = 10000 # Trials
dx = 0.25 # binsize
v = randn(t,2) # samples
x = -4:dx:4 # range
## Plot
using Winston
module Plot3D
using Winston
# Warning: the 3D interface is very new at the time
# of writing this and is likely to not be available
# and/or have changed
if Winston.output_surface == :gtk
include(Pkg.dir("Winston","src","canvas3d_gtk.jl"))
else
include(Pkg.dir("Winston","src","canvas3d.jl"))
end
end
edg1, edg2, count = hist2(v, x-dx/2)
#cntrs = ((first(x)+dx/2), (last(x)-dx/2), length(x)-1)
#x,y = meshgrid(cntrs, cntrs)
cntrs = (first(x)+dx/2):dx:(last(x)-dx/2)
x = Float64[x for x = cntrs, y = cntrs]
y = Float64[y for x = cntrs, y = cntrs]
Plot3D.plot3d(Plot3D.surf(x,count./(t*dx^2) *50,y))
Plot3D.plot3d(Plot3D.surf(x,exp(-(x.^2+y.^2)/2)/(2*pi) *50,y))
| RandomMatrices | https://github.com/JuliaMath/RandomMatrices.jl.git |
|
[
"MIT"
] | 0.5.5 | 359d601ae45b05ea9a53d303dfbf477fcaca4195 | code | 1239 | #haar.jl
#Algorithm 2.4 of Random Eigenvalues by Alan Edelman
#Experiment: Generate random orthogonal/unitary matrices
#Plot: Histogram eigenvalues
#Theory: Eigenvalues are on unit circle
## Parameters
t = 5000 # trials
dx = 0.05 # binsize
n = 10 # matrix size
function haar_experiment(t,dx,n)
v = Complex{Float64}[] # eigenvalue samples
for i = 1:t
# Sample random orthogonal matrix
# X = QR(randn(n,n))[:Q]
# If you have non-uniformly sampled eigenvalues, you may need this fix:
#X = X*diagm(sign(randn(n,1)))
#
# Sample random unitary matrix
X = QR(randn(n,n)+im*randn(n,n))[:Q]
# If you have non-uniformly sampled eigenvalues, you may need this fix:
#X = X*diagm(sign(randn(n)+im*randn(n)))
append!(v, eigvals(full(X)))
end
x = ((-1+dx/2):dx:(1+dx/2))*pi
x, v
end
x, v = haar_experiment(t,dx,n)
grid, count = hist(angle(v), x)
## Theory
h2 = t*n*dx/2*x.^0
## Plot
using Winston
p = FramedPlot()
h = Histogram(count, step(grid))
h.x0 = first(grid)
add(p, h)
add(p, Curve(x, h2, "color", "blue", "linewidth", 2))
if isinteractive()
Winston.display(p)
else
file(p, "haar.png")
end
| RandomMatrices | https://github.com/JuliaMath/RandomMatrices.jl.git |
|
[
"MIT"
] | 0.5.5 | 359d601ae45b05ea9a53d303dfbf477fcaca4195 | code | 109 | #hist2d.jl
#Algorithm 2.2 of Random Eigenvalues by Alan Edelman
#see Base.hist2 for a simple implementation
| RandomMatrices | https://github.com/JuliaMath/RandomMatrices.jl.git |
|
[
"MIT"
] | 0.5.5 | 359d601ae45b05ea9a53d303dfbf477fcaca4195 | code | 451 | #patiencesort.jl
#Algorithm 2.5 of Random Eigenvalues by Alan Edelman
function patiencesort(p)
piles = similar(p, 0)
for pi in p
idx = 1
for pp in piles
if pi > pp
idx += 1
end
end
if idx > length(piles)
d = length(piles)
resize!(piles, idx)
piles[d+1:end] = 0
end
piles[idx] = pi
end
return length(piles)
end
| RandomMatrices | https://github.com/JuliaMath/RandomMatrices.jl.git |
|
[
"MIT"
] | 0.5.5 | 359d601ae45b05ea9a53d303dfbf477fcaca4195 | code | 749 | #spherecomponent.jl
#Algorithm 2.3 of Random Eigenvalues by Alan Edelman
#Experiment: Random components of a vector on a sphere
#Plot: Histogram of a single component
#Theory: Beta distribution
## Parameters
t = 100000 # trials
n = 12 # dimensions of sphere
dx = 0.1 # bin size
## Experiment
v = randn(n, t)
v = (v[1,:] ./ sqrt(sum(v.^2,1)))'
grid, count = hist(v, -1:dx:1)
## Theory
x = grid
y = (gamma(n-1)/(2^(n-2)*gamma((n-1)/2)^2))*(1-x.^2).^((n-3)/2)
## Plot
using Winston
p = FramedPlot()
h = Histogram(count/(t*dx), step(grid))
h.x0 = first(grid)
add(p, h)
add(p, Curve(x, y, "color", "blue", "linewidth", 2))
if isinteractive()
Winston.display(p)
else
file(p, "spherecomponent.png")
end
| RandomMatrices | https://github.com/JuliaMath/RandomMatrices.jl.git |
|
[
"MIT"
] | 0.5.5 | 359d601ae45b05ea9a53d303dfbf477fcaca4195 | code | 1107 | #tracywidomlis.jl
#Algorithm 2.7 of Random Eigenvalues by Alan Edelman
#Experiment: Sample random permutations
#Plot: Histogram of lengths of longest increasing subsequences
#Theory: The Tracy-Widom law
## Parameters
t = 10000 # number of trials
n = 6^6 # length of permutations
dx = 1/6 # bin size
## Experiment
require("patiencesort.jl")
function tracywidomlis(t, n, dx)
## single processor: 1x speed
#v = [patiencesort(randperm(n)) for i = 1:t]
## simple parallelism: Nx speed * 130%
v = pmap((i)->patiencesort(randperm(n)), 1:t)
## maximum parallelism: Nx speed
#grouped = floor(t/(nprocs()-2))
#println(grouped)
#v = vcat(pmap((i)->[patiencesort(randperm(n)) for j = 1:grouped], 1:t/grouped)...)
w = (v-2sqrt(n))/n^(1/6)
return hist(w, -5:dx:2)
end
@time grid, count = tracywidomlis(t, n, dx)
## Plot
using Winston
p = FramedPlot()
h = Histogram(count/(t*dx), step(grid))
h.x0 = first(grid)
include("../1/tracywidom.jl")
add(p, h)
if isinteractive()
Winston.display(p)
else
file(p, "tracywidomlis.png")
end
| RandomMatrices | https://github.com/JuliaMath/RandomMatrices.jl.git |
|
[
"MIT"
] | 0.5.5 | 359d601ae45b05ea9a53d303dfbf477fcaca4195 | code | 773 | #unitarylis.jl
#Algorithm 2.5 of Random Eigenvalues by Alan Edelman
#Experiment: Generate random orthogonal/unitary matrices
#Theory: Counts longest increasing subsequence statistics
## Parameters
t = 200000 # Number of trials
n = 4 # permutation size
k = 2 # length of longest increasing subsequence
include("patiencesort.jl")
function unitarylis(t, n, k)
v = zeros(t) # samples
## Experiment
for i = 1:t
X = QR(complex(randn(k,k), randn(k,k)))[:Q]
X = X*diagm(sign(complex(randn(k),randn(k))))
v[i] = abs(trace(X)) ^ (2n)
end
z = mean(v)
c = 0
for i=1:factorial(n)
c = c + int(patiencesort(nthperm([1:n],i))<=k)
end
return (z, c)
end
println(unitarylis(t, n, k))
| RandomMatrices | https://github.com/JuliaMath/RandomMatrices.jl.git |
|
[
"MIT"
] | 0.5.5 | 359d601ae45b05ea9a53d303dfbf477fcaca4195 | code | 547 | #cauchymp.jl
#Algorithm 4.1 of Random Eigenvalues by Alan Edelman
#Experiment: Calculate Cauchy transform as trace of resolvent of Wishart matrix
#Theory: Marcenko-Pastur law
m = 2000 # larger dimension of matrix
n = 1000 # smaller dimension of matrix
r = n/m # aspect ratio
a = (1-sqrt(r))^2
b = (1+sqrt(r))^2
z = 3 # should be outside [a,b]
X = randn(m,n)
W = X'*X/m # Generate Wishart matrix
println(( trace(inv(z*eye(n)-W))/n,
(z-1+r-sqrt((z-a)*(z-b)))/(2*r*z) ))
| RandomMatrices | https://github.com/JuliaMath/RandomMatrices.jl.git |
|
[
"MIT"
] | 0.5.5 | 359d601ae45b05ea9a53d303dfbf477fcaca4195 | code | 1343 | #mpexperiment.jl
#Algorithm 4.2 of Random Eigenvalues by Alan Edelman
#Experiment: Marcenko-Pastur for applications
#Plot: Histogram eigenvalues of the covariance matrix
#Theory: Marcenko-Pastur as n->Infinity
## Parameters
t = 100 # trials
r = 1 # aspect ratio
n = 100 # matrix column size
dx = 0.1 # binsize
function mp_experiment(n,r,t,dx)
m = iround(n/r)
## Experiment
v = Float64[] # eigenvalue samples
for i = 1:t
A = randn(m,n) + 4*sqrt(n)*diagm(((1:n).<10))
A = A + sqrt(n) * diagm((1:n).>(n-1)) * 3 #3+0.1*randn(n,1) #3/sqrt(n)
append!(v, svd(A)[2]) # eigenvalues
end
v = v / sqrt(m) # normalized eigenvalues
## Theory
a = 1-sqrt(r)
b = 10
x = a-dx/2:dx:b
y = real(sqrt((x.^2-a^2).*(2^2-x.^2) + 0im)./(pi*x*r))
return (hist(v, x), (x, y))
end
((grid, count), (x,y)) = mp_experiment(n,r,t,dx)
## Plot
using Winston
p = FramedPlot()
h = Histogram(count/(t*n*dx), step(grid))
h.x0 = first(grid)
add(p, h)
add(p, Curve(x, y, "linewidth", 2, "color", "blue"))
last = length(count)-2
while count[last] == 0
last -= 1
end
setattr(p, "xrange", (0, grid[last+2]))
setattr(p, "yrange", (-.1, ceil(max(count/(t*n*dx)))))
if isinteractive()
Winston.display(p)
else
file(p, "mpexperiment.png")
end
| RandomMatrices | https://github.com/JuliaMath/RandomMatrices.jl.git |
|
[
"MIT"
] | 0.5.5 | 359d601ae45b05ea9a53d303dfbf477fcaca4195 | code | 947 | include("orthopoly_evaluate.jl")
function compute_hermite(n, x, all=true);
# Evaluate Orthonormalized Hermite polynomials at x
# Physicists Hermite:
# w(x)=exp(-x^2) with integral sqrt(pi)
# Equivalent Mathematica: HermiteH[n,x]/Sqrt[Sqrt[Pi]*n!*2^n]
# Probabilists Hermite:
# w(x)=exp(-x^2/2) with integral sqrt(2*pi)
# Equivalent Mathematica: HermiteH[n,x/Sqrt[2]]/Sqrt[Sqrt[Pi]*n!*2^(1/2+n)]
## Physicists Hermite Tridiagonal matrix (w=exp(-x^2))
T = diagm(sqrt((1:n)/2),1) # n+1 by n+1 matrix
T = T+T'
c = sqrt(pi) #Integral of weight function
## Probabilists Hermite Tridiagonal matrix (w=exp(-x^2/2))
# T = diagm(sqrt((1:n)),1) # n+1 by n+1 matrix
# T = T+T'
# c = sqrt(2*pi)
## Default to 0 through n computation
if all
phi = orthopoly_evaluate_all(T, x)
else
phi = orthopoly_evaluate_n(T, x)
end
phi/sqrt(c)
end
| RandomMatrices | https://github.com/JuliaMath/RandomMatrices.jl.git |
|
[
"MIT"
] | 0.5.5 | 359d601ae45b05ea9a53d303dfbf477fcaca4195 | code | 753 | ## This function computes the Generalized Laguerre polynomials using the Tridiagonal matrix
include("orthopoly_evaluate.jl")
function compute_laguerre(n, a, x, all=true)
# Evaluate Orthonormalized Laguerre polynomials at x
# Laguerre:
# w(x)=x^a exp(-x) with integral gamma(a+1) (a>-1)
# Equivalent Mathematica:LaguerreL[n,a,x]/Sqrt[Gamma[n+a+1]/Gamma[n+1]]
## Laguerre Bidiagonal matrix
B = diagm(sqrt((a + 1 +(0:n)))) # diagonal
B = B - diagm(sqrt((1:n)), 1) # superdiagonal
T = B'*B
c = gamma(a+1) #Integral of weight function
## Default to 0 through n computation
if all
phi = orthopoly_evaluate_all(T, x)
else
phi = orthopoly_evaluate_n(T, x)
end
phi/sqrt(c)
end
| RandomMatrices | https://github.com/JuliaMath/RandomMatrices.jl.git |
|
[
"MIT"
] | 0.5.5 | 359d601ae45b05ea9a53d303dfbf477fcaca4195 | code | 979 | #finitesemi.jl
#Algorithm 5.6 of Random Eigenvalues by Alan Edelman
#Experiment: Eigenvalues of GUE matrices
#Plot: Histogram of eigenvalues
#Theory: Semicircle and finite semicircle
## Parameters
n = 3 # size of matrix
s = 10000 # number of samples
d = 0.1 # bin size
## Experiment
function finitesemi_experiment(n,s,d)
e = Float64[]
for i = 1:s
a = randn(n,n)+im*randn(n,n)
a = (a+a')/(2*sqrt(4n))
v = eigvals(a)
append!(e, v)
end
return hist(e, -1.5:d:1.5)
end
grid, count = finitesemi_experiment(n,s,d)
## Theory
x = -1:0.01:1
y = sqrt(1-x.^2)
## Plot
using Winston
p = FramedPlot()
h = Histogram(count*pi/(2*d*n*s), step(grid))
h.x0 = first(grid)
add(p, h)
add(p, Curve(x, y, "color", "red", "linewidth", 2))
include("levels.jl")
add(p, Curve(levels(n)..., "color", "blue", "linewidth", 2))
if isinteractive()
Winston.display(p)
else
file(p, "spherecomponent.png")
end
| RandomMatrices | https://github.com/JuliaMath/RandomMatrices.jl.git |
|
[
"MIT"
] | 0.5.5 | 359d601ae45b05ea9a53d303dfbf477fcaca4195 | code | 494 | include("compute_hermite.jl")
function hermitekernel(n,x)
#Compute the Hermite kernel matrix at points x
#including degrees from 0 to n inclusive
# # Method 1: Direct Computation
phi=compute_hermite(n,x);
K=phi'*phi;
# # Method 2: Christoffel-Darboux
# phinp1 = compute_hermite(n+1,x,false)
# phin = compute_hermite(n,x,false)
# K = phinp1'*phin*sqrt((n+1)/2)
# x = x[:]*ones(1,length(x))
# K = (K-K')./(x-x')
K
end
| RandomMatrices | https://github.com/JuliaMath/RandomMatrices.jl.git |
|
[
"MIT"
] | 0.5.5 | 359d601ae45b05ea9a53d303dfbf477fcaca4195 | code | 940 | #levels.jl
#Algorithm 5.7 of Random Eigenvalues by Alan Edelman
function levels(n)
# Plot exact semicircle formula for GUE
# This is given in formula (5.2.16) in Mehta as the sum of phi_j^2
# but Christoffel-Darboux seems smarter
## Parameter
# n: Dimension of matrix for which to determine exact semicircle
x = (-1:0.001:1) * sqrt(2n) * 1.3
pold = zeros(size(x)) # -1st Hermite Polynomial
p = ones(size(x)) # 0th Hermite Polynomial
k = p
for j = 1:n # Compute the three-term recurrence
pnew = (sqrt(2) * x .* p - sqrt(j - 1) * pold) / sqrt(j)
pold = p
p = pnew
end
pnew = (sqrt(2) * x .* p - sqrt(n) * pold)/sqrt(n + 1)
k = n * p .^ 2 - sqrt(n * (n+1)) * pnew .* pold # Use p.420 of Mehta
k = k .* exp(-x .^ 2) / sqrt(pi) # Normalize
# Rescale so that "semicircle" is on [-1, 1] and area is pi/2
(x / sqrt(2n), k * pi / sqrt(2n))
end
| RandomMatrices | https://github.com/JuliaMath/RandomMatrices.jl.git |
|
[
"MIT"
] | 0.5.5 | 359d601ae45b05ea9a53d303dfbf477fcaca4195 | code | 804 | #levels2.jl
#Algorithm 5.8 of Random Eigenvalues by Alan Edelman
function levels2(n)
# Plot exact semicircle formula for GUE
xfull = (-1:0.001:1) * sqrt(2n) * 1.3
# Form the T matrix
T = diagm(sqrt(1:n-1),1)
T = T + T'
# Do the eigen-decomposition of T, T = UVU'
V, U = eig(T)
# Precompute U'*e_n
# tmp_en = U' * ((0:n-1) == n-1)'
tmp_en = U[end, :]'
y = Array(Float64, length(xfull))
for i = 1:length(xfull)
x = xfull[i]
# generate the v vector as in (2.5)
v = U * (tmp_en ./ (V - sqrt(2) * x))
# multiply the normalization term
y[i] = norm((sqrt(pi)) ^ (-1/2) * exp(-x^2/2) * v/v[1])^2
end
# Rescale so that "semicircle" is on [-1, 1] and area is pi/2
(xfull/sqrt(2n), y * pi / sqrt(2n))
end
| RandomMatrices | https://github.com/JuliaMath/RandomMatrices.jl.git |
|
[
"MIT"
] | 0.5.5 | 359d601ae45b05ea9a53d303dfbf477fcaca4195 | code | 1750 | function orthopoly_evaluate_all(T, x)
## Evaluate 0th through nth orthogonal polynomial at x
# Parameters
# T: Tridiagonal matrix encoding the three term recurrence
# size is (n+1)x(n+1)
# x: evaluation points
#
# Returns
# phi: Polynomials 0 through n at x
# Method: Spectrally solve (T-XI)u = constant * (last column of I)
# WARNING: potential for dividing by 0
# 1st row of phi is 1 -- for w not a probability measure
# divide by 1/sqrt(c), where c is the total weight
# of the real line
n = size(T,1)
Lambda, H = eig(T)
Hn = H[end, :]'
phi = Array(Float64, length(Lambda), length(x))
for m = 1:length(x)
xi = x[m]
# generate the u vector as in (2.5)
u = H * (Hn./(Lambda - xi))
phi[:,m] = u/u[1]
end
phi
end
function orthopoly_evaluate_n(T, x)
## Evaluate nth orthogonal polynomial at x
# Parameters
# T: Tridiagonal matrix encoding the three term recurrence
# size is (n+1)x(n+1)
# x: evaluation points
#
# Returns
# phi: nth at x
# normalized so that 0'th is 1 (w has unit weight)
# Method: characteristic polynomial of n x n T
# with leading coefficient taken from the
# product of the superdiagonal of the
# (n+1)x(n+1) T
n = size(T,1)-1
## compute the characteristic polynomial of T[1:n,1:n]
Lambda = eigvals(T[1:n, 1:n])
phi = ones(size(x))
for i = 1:n
phi = phi.*(x - Lambda[i])
end
## normalization
if n > 0
phi = phi / prod(diag(T,1))
end
phi
end
| RandomMatrices | https://github.com/JuliaMath/RandomMatrices.jl.git |
|
[
"MIT"
] | 0.5.5 | 359d601ae45b05ea9a53d303dfbf477fcaca4195 | code | 685 | #sinekernel.jl
n=200
x=(0:.025:1)*3*pi
include("hermitekernel.jl")
K=hermitekernel(n-1,x/sqrt(2*n))*pi/sqrt(2*n)
## Plot
using Winston
module Plot3D
using Winston
# Warning: the 3D interface is very new at the time
# of writing this and is likely to not be available
# and/or have changed
if Winston.output_surface == :gtk
include(Pkg.dir("Winston","src","canvas3d_gtk.jl"))
else
include(Pkg.dir("Winston","src","canvas3d.jl"))
end
end
xx = Float64[xx for xx = x, yy = x]
yy = Float64[yy for xx = x, yy = x]
Plot3D.plot3d(Plot3D.surf(xx,K,yy))
Plot3D.plot3d(Plot3D.surf(
(u,v)->u,
(u,v)->sin(u-v)./(u-v),
(u,v)->v,
x, x))
| RandomMatrices | https://github.com/JuliaMath/RandomMatrices.jl.git |
|
[
"MIT"
] | 0.5.5 | 359d601ae45b05ea9a53d303dfbf477fcaca4195 | code | 1272 | #centrallimit.jl
#Algorithm 7.2 of Random Eigenvalues by Alan Edelman
using Winston
function centrallimit(m, t)
# sums m random matrices and histograms their eigenvalues
# normalized by 1/sqrt(m)
# Input :
# m : number of matrices to sum
# t : number of trials to perform
n = 100 # matrix size
dx = .1 # bin size
v = Float64[]
# we choose a random diagonal {-1,1} matrx as our prototype
# A = diagm(sign(randn(1,n)))
# proto = kron([1 0; 0 -1], eye(n/2))
for i = 1:t # for each trial
B = zeros(n,n) # initialize the accumulator
for z = 1:m # sum up the random matrices
Q = full(QR(randn(n,n) + im*randn(n,n))[:Q]) # (piecewise) Haar orthogonal
A = diagm(sign(randn(n,1)))
B = B + Q'*A*Q # A and Q'AQ are asymptotically free
end
append!(v, real(eigvals(B)/sqrt(m))) # normalize eigenvalues
end
grid, count = hist(v, -2-dx/2:dx:2)
p = FramedPlot()
h = Histogram(count*pi/2/(dx*n*t), step(grid))
h.x0 = first(grid)
add(p, h)
if isinteractive()
Winston.display(p)
else
file(p, "centrallimit_m=$(m)_t=$(t).png")
end
end
| RandomMatrices | https://github.com/JuliaMath/RandomMatrices.jl.git |
|
[
"MIT"
] | 0.5.5 | 359d601ae45b05ea9a53d303dfbf477fcaca4195 | code | 1314 | #randomgrowth.jl
using Winston
function random_growth(M, N, q)
G = zeros(M, N)
T = 1000
G[1,1] = true
# update the possible sets
display(imagesc((0,N), (M,0), G))
for t = 1:T
sets = next_possible_squares(G)
## Highlights all the possible squares
for i = 1:length(sets)
idx = sets[i]::(Int,Int)
G[idx[1], idx[2]] = 0.25
end
display(imagesc((0,N), (M,0), G))
sleep(.01)
## Actual growth
for i = 1:length(sets)
ison = 0.5 * (rand() > (1-q))
if ison > 0
idx = sets[i]::(Int,Int)
G[idx[1], idx[2]] = ison
end
end
display(imagesc((0,N), (M,0), G))
G[G .== 0.5] = 1
G[G .== 0.25] = 0
sleep(.01)
end
return G
end
function next_possible_squares(G)
M, N = size(G)::(Int,Int)
sets = Array((Int,Int),0)
for ii = 1:M
for jj = 1:N
if G[ii, jj] == 0
if (ii == 1 && jj > 1 && G[ii, jj-1] == 1) ||
(jj == 1 && ii > 1 && G[ii-1, jj] == 1) ||
(ii > 1 && jj > 1 && G[ii-1, jj] == 1 && G[ii, jj-1] == 1)
push!(sets, (ii,jj))
end
end
end
end
sets
end
| RandomMatrices | https://github.com/JuliaMath/RandomMatrices.jl.git |
|
[
"MIT"
] | 0.5.5 | 359d601ae45b05ea9a53d303dfbf477fcaca4195 | code | 2316 | #randomgrowth2.jl
using PyPlot
using OrdinaryDiffEq
function randomgrowth2()
num_trials = 2000
g = 1
q = 0.7
Ns = 80
# Ns = [50, 100, 200]
clf()
# [-1.771086807411 08131947928329]
# Gap = c d^N
for jj = 1:length(Ns)
N = Ns[jj]
M = round(N*g)
B = zeros(num_trials)
@time begin
for ii = 1:num_trials
# G = G[M, N, q]
# B[ii] = G[M,N]
B[ii] = G(M, N, q)
end
end
C = (B - N*om(g,q)) / (sigma_growth(g,q)*N^(1/3))
d = 0.2
subplot(1,length(Ns),jj)
plt.hist(C - exp(-N*0.0025 - 3), normed = true)
xlim(-6, 4)
## Theory
t0 = 4
tn = -6
dx = 0.005
deq = function (t, y, dy)
dy[1] = y[2]
dy[2] = t*y[1]+2y[1]^3
dy[3] = y[4]
dy[4] = y[1]^2
end
y0 = big.([airy(t0); airy(1,t0); 0; airy(t0)^2]) # boundary conditions
prob = ODEProblem(deq,y0,(t0,tn))
sol = solve(prob,Vern8(), saveat=-dx, abstol=1e-12, reltol=1e-12) # solve
F2 = Float64[exp(-sol[i][3]) for i = 1:length(y)] # the distribution
f2 = gradient(F2, t) # the density
# add(p, Curve(t, f2, "color", "red", "linewidth", 3))
# Winston.display(p)
subplot(1,length(Ns),jj)
plot(sol.t, f2, "r", linewidth = 3)
ylim(0, 0.6)
println(mean(C))
end
end
function G(N, M, q)
# computes matrix G[N,M] for a given q
GG = floor(log(rand(N,M))/log(q))
#float(rand(N,M) < q)
# Compute the edges: the boundary
for ii = 2:N
GG[ii, 1] = GG[ii, 1] + GG[ii-1, 1]
GG[1, ii] = GG[1, ii] + GG[1, ii-1]
end
# Compute the inside
for ii = 2:N
for jj = 2:M
GG[ii, jj] = GG[ii, jj] + max(GG[ii, jj-1], GG[ii-1, jj])
end
end
## Plot
# imagesc((0,N),(M,0),GG)
# sleep(.01)
return GG[N, M]
end
# helper functions sigma.m and om, which describe mean and
# standard deviation of G[N, M]
# Computes G[qN,N]/N for N->inf
om(g,q) = ((1 + sqrt(g * q)) ^ 2) / (1 - q) - 1
sigma_growth(r, q) =
q^(1/6) * r^(1/6) / (1 - q) *
(sqrt(r) + sqrt(q))^(2/3) *
(1 + sqrt(q * r))^(2/3)
randomgrowth2()
| RandomMatrices | https://github.com/JuliaMath/RandomMatrices.jl.git |
|
[
"MIT"
] | 0.5.5 | 359d601ae45b05ea9a53d303dfbf477fcaca4195 | code | 2156 | #jacobian2by2.jl
# Code 8.1 of Random Eigenvalues by Alan Edelman
#Experiment: Compute the Jacobian of a 2x2 matrix function
#Comment: Symbolic tools are not perfect. The author
# exercised care in choosing the variables
warn("Julia doesn't currently have a good symbolic math package")
warn("This may use features of PyCall and/or SymPy which are not publicly available yet")
using PyCall
pyinitialize("/opt/local/bin/python2")
using SymPy
jacobian(Y,X) = Sym(Y[:reshape](1,length(Y))[:jacobian](X[:reshape](1,length(X))))
p,q,r,s, a,b,c,d, t, e1,e2 = Sym(:p,:q,:r,:s, :a,:b,:c,:d, :t, :e1,:e2)
X = SymMatrix([p q; r s])
A = SymMatrix([a b; c d])
Y = X^2; J = jacobian(Y,X); JAC_square = Sym(J[:det]()[:factor]()); println("square:\n", J, "\n\n", JAC_square, "\n\n")
Y = X^3; J = jacobian(Y,X); JAC_cube = Sym(J[:det]()[:factor]()); println("cube:\n", J, "\n\n", JAC_cube, "\n\n")
Y = X[:inv](); J = jacobian(Y,X); JAC_inv = Sym(J[:det]()[:factor]()); println("inv:\n", J, "\n\n", JAC_inv, "\n\n")
Y = A*X; J = jacobian(Y,X); JAC_linear = Sym(J[:det]()[:factor]()); println("linear:\n", J, "\n\n", JAC_linear, "\n\n")
Y = SymMatrix([p q; r/p X[:det]()/p]); J = jacobian(Y,X); JAC_lu = Sym(J[:det]()[:factor]()); println("lu:\n", J, "\n\n", JAC_lu, "\n\n")
x = SymMatrix([p, s, r])
y = SymMatrix([sqrt(p), sqrt(s), r/(sqrt(p)*sqrt(s))])
J = jacobian(y,x)
JAC_DMD = Sym(J[:det]()[:factor]())
println("DMD:\n", J, "\n\n", JAC_DMD, "\n\n")
x = SymMatrix([p, s])
y = SymMatrix([sqrt(p^2 + s^2), atan(s/p)])
J = jacobian(y,x)
JAC_notrace = Sym(J[:det]()[:factor]())
println("notrace:\n", J, "\n\n", JAC_notrace, "\n\n")
Q = SymMatrix([cos(t) -sin(t); sin(t) cos(t)])
D = SymMatrix([e1 0; 0 e2])
Y = Q*D*Q[:transpose]()
y = SymMatrix([Y[1,1], Y[2,2], Y[1,2]])
x = SymMatrix([t, e1, e2])
J = jacobian(Y,X)
JAC_symeig = Sym(J[:det]()[:factor]())
println("symeig:\n", J, "\n\n", JAC_symeig, "\n\n")
X = SymMatrix([p s; s r])
Y = Sym(A[:transpose]())*X*A
y = SymMatrix([Y[1,1], Y[2,2], Y[1,2]])
x = SymMatrix([p, r, s])
J = jacobian(Y,X)
JAC_symcong = Sym(J[:det]()[:factor]())
println("symcong:\n", J, "\n\n", JAC_symcong, "\n\n")
| RandomMatrices | https://github.com/JuliaMath/RandomMatrices.jl.git |
|
[
"MIT"
] | 0.5.5 | 359d601ae45b05ea9a53d303dfbf477fcaca4195 | code | 1946 | export hist_eig
#Uses the method of Sturm sequences to compute the histogram of eigenvalues of a matrix
#
# Reference
# Cy P. Chan, "Sturm Sequences and the Eigenvalue Distribution of
# the Beta-Hermite Random Matrix Ensemble", M.Sc. thesis (MIT), 2007
#
# For better numerical stability, this algorithm is modified so that only the sign
# of the determinant is stored.
#
#For general matrices, this is slower than hist(eigvals(M), bins) and is NOT recommended
function hist_eig(M::AbstractMatrix, bins::Vector{GridPoint}) where {GridPoint <: Number}
n = size(M)[1]
NumBins = length(bins)
histogram = zeros(NumBins)
SturmSequence = zeros(n)
for BinId=1:NumBins
K = M - bins[BinId]*eye(n)
#Progression of determinants of lower-right submatrices
#SturmSequence = [det(K[end-j+1:end,end-j+1:end]) for j=1:n]
#SturmRatioSequence = [SturmSequence[j+1]/SturmSequence[j] for j=1:n]
SturmSequenceSigns = [int(sign(det(K[end-j+1:end,end-j+1:end]))) for j=1:n]
SturmSequenceSigns = [1; SturmSequenceSigns]
SturmRatioSequence = [SturmSequenceSigns[j+1]/SturmSequenceSigns[j] for j=1:n]
histogram[BinId] = sum([r < 0 for r in SturmRatioSequence])
end
histogram = int(diff([histogram; n]))
end
#Uses the method of Sturm sequences to compute the histogram of eigenvalues of
#a symmetric tridiagonal mmatrix
#
# Reference
# Cy P. Chan, "Sturm Sequences and the Eigenvalue Distribution of
# the Beta-Hermite Random Matrix Ensemble", M.Sc. thesis (MIT), 2007
#
function hist_eig(M::SymTridiagonal, bins::Vector{GridPoint}) where {GridPoint <: Number}
n = size(M)[1]
NumBins = length(bins)
histogram = zeros(NumBins)
r = zeros(n)
for BinId=1:NumBins
a, b = M.dv - bins[BinId], M.ev
#Formula (2.3)
r[1] = a[1]
for i=2:n
r[i] = a[i] - b[i-1]^2/r[i-1]
end
histogram[BinId] = sum([SturmRatio < 0 for SturmRatio in r])
end
histogram = int(diff([histogram; n]))
end
| RandomMatrices | https://github.com/JuliaMath/RandomMatrices.jl.git |
|
[
"MIT"
] | 0.5.5 | 359d601ae45b05ea9a53d303dfbf477fcaca4195 | code | 8655 | # Excursions in formal power series (fps)
#
# Jiahao Chen <[email protected]> 2013
#
# The primary reference is
# [H] Peter Henrici, "Applied and Computational Complex Analysis", Volume I:
# Power Series---Integration---Conformal Mapping---Location of Zeros,
# Wiley-Interscience: New York, 1974
import Base: zero, one, inv, length, ==, +, -, *, ^
import Base.Broadcast: broadcasted
export FormalPowerSeries, fps, tovector, trim, isunit, isnonunit,
MatrixForm, reciprocal, derivative, isconstant, compose,
isalmostunit, FormalLaurentSeries
#############################
# Formal power series (fps) #
#############################
# Definition [H, p.9] - we limit this to finitely many coefficients
mutable struct FormalPowerSeries{Coefficients}
c :: Dict{BigInt, Coefficients}
FormalPowerSeries{Coefficients}(c::Dict{BigInt, Coefficients}) where Coefficients = new(c)
function FormalPowerSeries{Coefficients}(v::Vector{Coefficients}) where Coefficients
c=Dict{BigInt, Coefficients}()
for i in eachindex(v)
if v[i] != 0
c[i-1] = v[i] #Note this off by 1 - allows constant term c[0] to be set
end
end
FormalPowerSeries{Coefficients}(c)
end
end
FormalPowerSeries(v::Vector{T}) where T = FormalPowerSeries{T}(v)
#Convenient abbreviation for floats
fps = FormalPowerSeries{Float64}
zero(::Type{FormalPowerSeries{T}}) where {T} = FormalPowerSeries(T[])
one(::Type{FormalPowerSeries{T}}) where {T} = FormalPowerSeries(T[1])
zero(::FormalPowerSeries{T}) where {T} = zero(FormalPowerSeries{T})
one(::FormalPowerSeries{T}) where {T} = one(FormalPowerSeries{T})
#Return truncated vector with c[i] = P[n[i]]
function tovector(P::FormalPowerSeries{T}, n::AbstractVector{Index}) where {T,Index<:Integer}
nn = length(n)
c = zeros(nn)
for (k,v) in P.c, i in eachindex(n)
n[i]==k && (c[i]=v)
end
c
end
tovector(P::FormalPowerSeries, n::Integer)=tovector(P,1:n)
# Basic housekeeping and properties
# Remove extraneous zeros
function trim(P::FormalPowerSeries{T}) where {T}
for (k,v) in P.c
if v==0
delete!(P.c, k)
end
end
return P
end
length(P::FormalPowerSeries{T}) where {T} = maximum([k for (k,v) in P.c])
function ==(P::FormalPowerSeries{T}, Q::FormalPowerSeries{T}) where {T}
for (k,v) in P.c
if v==0 #ignore explicit zeros
continue
elseif !haskey(Q.c, k)
return false
elseif Q.c[k] != v
return false
end
end
for (k,v) in Q.c
if v==0 #ignore explicit zeros
continue
elseif !haskey(P.c, k)
return false
elseif P.c[k] != v
return false
end
end
return true
end
# Basic arithmetic [H, p.10]
function +(P::FormalPowerSeries{T}, Q::FormalPowerSeries{T}) where {T}
c = Dict{BigInt, T}()
for (k,v) in P.c
haskey(c,k) ? (c[k]+=v) : (c[k]=v)
end
for (k,v) in Q.c
haskey(c,k) ? (c[k]+=v) : (c[k]=v)
end
FormalPowerSeries{T}(c)
end
function -(P::FormalPowerSeries{T}, Q::FormalPowerSeries{T}) where {T}
c = Dict{BigInt, T}()
for (k,v) in P.c
c[k] = get(c,k,zero(T)) + v
end
for (k,v) in Q.c
c[k] = get(c,k,zero(T)) - v
end
FormalPowerSeries{T}(c)
end
#negation
function -(P::FormalPowerSeries{T}) where {T}
c = Dict{BigInt, T}()
for (k,v) in P.c
c[k] = -v
end
FormalPowerSeries{T}(c)
end
#multiplication by scalar
function *(P::FormalPowerSeries{T}, n::Number) where {T}
c = Dict{BigInt, T}()
for (k,v) in P.c
c[k] = n*v
end
FormalPowerSeries{T}(c)
end
*(n::Number, P::FormalPowerSeries{T}) where {T} = *(P, n)
#Cauchy product [H, p.10]
*(P::FormalPowerSeries{T}, Q::FormalPowerSeries{T}) where {T} = CauchyProduct(P, Q)
function CauchyProduct(P::FormalPowerSeries{T}, Q::FormalPowerSeries{T}) where {T}
c = Dict{BigInt, T}()
for (k1, v1) in P.c, (k2, v2) in Q.c
c[k1+k2]=get(c, k1+k2, zero(T))+v1*v2
end
FormalPowerSeries{T}(c)
end
#Hadamard product [H, p.10] - the elementwise product
broadcasted(::typeof(*), P::FormalPowerSeries{T}, Q::FormalPowerSeries{T}) where T =
HadamardProduct(P, Q)
function HadamardProduct(P::FormalPowerSeries{T}, Q::FormalPowerSeries{T}) where {T}
c = Dict{BigInt, T}()
for (k,v) in P.c
if v!=0 && haskey(Q.c,k) && Q.c[k] !=0
c[k] = v * Q.c[k]
end
end
FormalPowerSeries{T}(c)
end
#The identity element over the complex numbers
#replacement for previous function eye(P::FormalPowerSeries{T})
function FormalPowerSeries{T}(s::UniformScaling) where {T}
c = Dict{BigInt, T}()
c[0] = 1
return FormalPowerSeries{T}(c)
end
isunit(P::FormalPowerSeries{T}) where {T <: Number} = P==FormalPowerSeries{Float64}(I)
# [H, p.12]
isnonunit(P::FormalPowerSeries{T}) where {T} = (!haskey(P.c, 0) || P.c[0]==0) && !isunit(P)
#Constructs the top left m x m block of the (infinite) semicirculant matrix
#associated with the fps [H, Sec.1.3, p.14]
#[H] calls it the semicirculant, but in contemporary nomenclature this is an
#upper triangular Toeplitz matrix
#This constructs the dense matrix - Toeplitz matrices don't exist in Julia yet
function MatrixForm(P::FormalPowerSeries{T}, m :: Integer) where {T}
m<0 && error("Invalid matrix dimension $m requested")
M=zeros(T, m, m)
for (k,v) in P.c
if k < m
for i=1:m-k
M[i, i+k]=v
end
end
end
M
end
#Reciprocal of power series [H, Sec.1.3, p.15]
#
#This algorithm is NOT that in [H], since it uses determinantal inversion
#formulae for the inverse. It is much quicker to find the inverse of the
#associated infinite triangular Toeplitz matrix!
#
#Every fps is isomorphic to such a triangular Toeplitz matrix [H. Sec.1.3, p.15]
#
#As most reciprocals of finite series are actually infinite,
#allow the inverse to have a finite truncation
#
#TODO implement the FFT-based algorithm of one of the following
# doi:10.1109/TAC.1984.1103499
# https://cs.uwaterloo.ca/~glabahn/Papers/tamir-george-1.pdf
function reciprocal(P::FormalPowerSeries, n::Integer)
n<0 && error(sprintf("Invalid inverse truncation length %d requested", n))
a = tovector(P, 0:n-1) #Extract original coefficients in vector
a[1]==0 && error("Inverse does not exist")
inv_a1 = inv(a[1])
b = zeros(n) #Inverse
b[1] = inv_a1
for k=2:n
b[k] = -inv_a1 * sum([b[k+1-i] * a[i] for i=2:k])
end
FormalPowerSeries(b)
end
#Derivative of fps [H. Sec.1.4, p.18]
function derivative(P::FormalPowerSeries{T}) where T
c = Dict{BigInt, T}()
for (k,v) in P.c
if k != 0 && v != 0
c[k-1] = k*v
end
end
FormalPowerSeries{T}(c)
end
#[H, Sec.1.4, p.18]
function isconstant(P::FormalPowerSeries)
for (k,v) in P.c
if k!=0 && v!=0
return false
end
end
return true
end
# Power of fps [H, Sec.1.5, p.35]
function ^(P::FormalPowerSeries{T}, n::Integer) where T
if n == 0
return FormalPowerSeries{T}([one(T)])
elseif n > 1
#The straightforward recursive way
return P^(n-1) * P
#TODO implement the non-recursive formula
elseif n==1
return P
else
error("I don't know what to do for power n = $n")
end
end
# Composition of fps [H, Sec.1.5, p.35]
# This is the quick and dirty version
function compose(P::FormalPowerSeries{T}, Q::FormalPowerSeries{T}) where {T}
sum([v * Q^k for (k, v) in P.c])
end
#[H, p.45]
function isalmostunit(P::FormalPowerSeries{T}) where {T}
(haskey(P.c, 0) && P.c[0]!=0) ? (return false) :
(haskey(P.c, 1) && P.c[1]!=0) ? (return true) : (return false)
end
# Reversion of a series (inverse with respect to composition)
# P^[-1]
# [H. Sec.1.7, p.47, but more succinctly stated on p.55]
# Constructs the upper left nxn subblock of the matrix representation
# and inverts it
function reversion(P::FormalPowerSeries{T}, n :: Integer) where {T}
n>0 ? error("Need non-negative dimension") : nothing
Q = P
A = zeros(n, n)
#Construct the matrix representation (1.9-1), p.55
for i=1:n
Q = P
ai = tovector(Q, n) #Extract coefficients P[1]...P[n]
A[i,:] = ai
i<n && (Q *= P)
end
#TODO I just need the first row of the inverse
B = inv(A)
FormalPowerSeries{T}(B[1, :]'[:,1])
end
inv(P::FormalPowerSeries, n::Integer) = reversion(P, n)
###############################
# Formal Laurent series (fLs) #
# [H., Sec. 1.8, p. 51] #
###############################
mutable struct FormalLaurentSeries{Coefficients}
c :: Dict{BigInt, Coefficients}
FormalLaurentSeries{Coefficients}(c::Dict{BigInt, Coefficients}) where Coefficients = new(c)
function FormalLaurentSeries{Coefficients}(v::Vector{Coefficients}) where Coefficients
c = new(c)
for i=1:length(v)
c[i] = v[i]
end
c
end
end
| RandomMatrices | https://github.com/JuliaMath/RandomMatrices.jl.git |
|
[
"MIT"
] | 0.5.5 | 359d601ae45b05ea9a53d303dfbf477fcaca4195 | code | 10202 | # Generates samples of dense random matrices that are distributed according
# to the classical Gaussian random matrix ensembles
#
# The notation follows closely that in:
#
# Ioana Dumitriu and Alan Edelman, " Models for Beta s"
# Journal of Mathematical Physics, vol. 43 no. 11 (2002), pp. 5830--5547
# doi: 10.1063/1.1507823
# arXiv: math-ph/0206043
#
# References
#
# Alan Edelman, Per-Olof Persson and Brian D Sutton, "The fourfold way"
# http://www-math.mit.edu/~edelman/homepage/papers/ffw.pdf
export GaussianHermite, GaussianLaguerre, GaussianJacobi,
Wigner, Wishart, MANOVA #Synonyms for Hermite, Laguerre and Jacobi
#A convenience function to define a chi scalar random variable
χ(df::Real) = rand(Chi(df))
#####################
# Hermite ensemble #
#####################
"""
GaussianHermite{β} represents a Gaussian Hermite ensemble with parameter β.
Wigner{β} is a synonym.
Example of usage:
β = 2 #β = 1, 2, 4 generates real, complex and quaternionic matrices respectively.
d = Wigner{β} #same as GaussianHermite{β}
n = 20 #Generate square matrices of this size
S = rand(d, n) #Generate a 20x20 symmetric Wigner matrix
T = tridrand(d, n) #Generate the symmetric tridiagonal form
v = eigvalrand(d, n) #Generate a sample of eigenvalues
"""
struct GaussianHermite{β} <: ContinuousMatrixDistribution end
GaussianHermite(β) = GaussianHermite{β}()
"""
Synonym for GaussianHermite{β}
"""
const Wigner{β} = GaussianHermite{β}
rand(d::Type{Wigner{β}}, dims...) where {β} = rand(d(), dims...)
function rand(d::Wigner{1}, n::Int)
A = randn(n, n)
normalization = 1 / √(2n)
return Symmetric((A + A') / 2 * normalization)
end
function rand(d::Wigner{2}, n::Int)
A = randn(n, n) + im*randn(n, n)
normalization = √(4*n)
return Hermitian((A + A') / normalization)
end
function rand(d::Wigner{4}, n::Int)
#Employs 2x2 matrix representation of quaternions
X = randn(n, n) + im*randn(n, n)
Y = randn(n, n) + im*randn(n, n)
A = [X Y; -conj(Y) conj(X)]
normalization = √(8*n)
return Hermitian((A + A') / normalization)
end
rand(d::Wigner{β}, n::Int) where {β} =
throw(ArgumentError("Cannot sample random matrix of size $n x $n for β=$β"))
function rand(d::Wigner{β}, dims::Int...) where {β}
if length(dims)==2 && dims[1] == dims[2]
return rand(d, dims[1])
else
throw(ArgumentError("Cannot sample random matrix of size $dims for β=$β"))
end
end
"""
Generates a nxn symmetric tridiagonal Wigner matrix
Unlike for `rand(Wigner{β}, n)`, which is restricted to β=1,2 or 4,
`trirand(Wigner{β}, n)` will generate a
The β=∞ case is defined in Edelman, Persson and Sutton, 2012
"""
function tridrand(d::Wigner{β}, n::Int) where {β}
χ(df::Real) = rand(Distributions.Chi(df))
if β≤0
throw(ArgumentError("β = $β cannot be nonpositive"))
elseif isinf(β)
return tridrand(Wigner{Inf}, n)
else
normalization = 1 / √(2n)
Hd = rand(Distributions.Normal(0,2), n)./√2
He = [χ(β*i)/√2 for i=n-1:-1:1]
return normalization * SymTridiagonal(Hd, He)
end
end
function tridrand(d::Wigner{β}, dims...) where {β}
if length(dims)==2 && dims[1] == dims[2]
return rand(d, dims[1])
else
throw(ArgumentError("Cannot sample random matrix of size $dims for β=$β"))
end
end
"""
Return n eigenvalues distributed according to the Hermite ensemble
"""
eigvalrand(d::Wigner, n::Int) = eigvals(tridrand(d, n))
"""
Calculate Vandermonde determinant term
"""
function VandermondeDeterminant(λ::AbstractVector{Eigenvalue}, β::Real) where {Eigenvalue<:Number}
n = length(λ)
Vandermonde = one(Eigenvalue)^β
for j=1:n, i=1:j-1
Vandermonde *= abs(λ[i] - λ[j])^β
end
Vandermonde
end
"""
Calculate joint eigenvalue density
"""
function eigvaljpdf(d::Wigner{β}, λ::AbstractVector{Eigenvalue}) where {β,Eigenvalue<:Number}
n = length(λ)
#Calculate normalization constant
c = (2π)^(-n/2)
for j=1:n
c *= gamma(1 + β/2)/gamma(1 + β*j/2)
end
Energy = sum(λ.^2/2) #Calculate argument of exponential
VandermondeDeterminant(λ, β) * exp(-Energy)
end
#####################
# Laguerre ensemble #
#####################
mutable struct GaussianLaguerre <: ContinuousMatrixDistribution
beta::Real
a::Real
end
const Wishart = GaussianLaguerre
#Generates a NxN Hermitian Wishart matrix
#TODO Check - the eigenvalue distribution looks funky
#TODO The appropriate matrix size should be calculated from a and one matrix dimension
function rand(d::GaussianLaguerre, dims::Dim2)
n = 2.0*a/d.beta
if d.beta == 1 #real
A = randn(dims)
elseif d.beta == 2 #complex
A = randn(dims) + im*randn(dims)
elseif d.beta == 4 #quaternion
#Employs 2x2 matrix representation of quaternions
X = randn(dims) + im*randn(dims)
Y = randn(dims) + im*randn(dims)
A = [X Y; -conj(Y) conj(X)]
error("beta = $(d.beta) is not implemented")
end
return (A * A') / dims[1]
end
#Generates a NxN bidiagonal Wishart matrix
function bidrand(d::GaussianLaguerre, m::Integer)
if d.a <= d.beta*(m-1)/2.0
error("Given your choice of m and beta, a must be at least $(d.beta*(m-1)/2.0) (You said a = $(d.a))")
end
Bidiagonal([chi(2*d.a-i*d.beta) for i=0:m-1], [chi(d.beta*i) for i=m-1:-1:1], true)
end
#Generates a NxN tridiagonal Wishart matrix
function tridrand(d::GaussianLaguerre, m::Integer)
B = bidrand(d, m)
L = B * B'
SymTridiagonal(diag(L)/sqrt(m), diag(L,1)/sqrt(m))
end
#Return n eigenvalues distributed according to the Laguerre ensemble
eigvalrand(d::GaussianLaguerre, m::Integer) = eigvals(tridrand(d, m))
#TODO Check m and ns
function eigvaljpdf(d::GaussianLaguerre, lambda::Vector{Eigenvalue}) where {Eigenvalue<:Number}
m = length(lambda)
#Laguerre parameters
p = 0.5*d.beta*(m-1) + 1.0
#Calculate normalization constant
c = 2.0^-(m*d.a)
z = (d.a - d.beta*(m)*0.5)
for j=1:m
z += 0.5*d.beta
if z < 0 && (int(z) - z) < eps()
#Pole of gamma function, there is no density here no matter what
return 0.0
end
c *= gamma(1 + beta/2)/(gamma(1 + beta*j/2)*gamma(z))
end
Prod = prod(lambda.^(a-p)) #Calculate Laguerre product term
Energy = sum(lambda)/2 #Calculate argument of exponential
return c * VandermondeDeterminant(lambda, beta) * Prod * exp(-Energy)
end
###################
# Jacobi ensemble #
###################
#Generates a NxN self-dual MANOVA matrix
mutable struct GaussianJacobi <: ContinuousMatrixDistribution
beta::Real
a::Real
b::Real
end
const MANOVA = GaussianJacobi
function rand(d::GaussianJacobi, m::Integer)
w1 = Wishart(m, int(2.0*d.a/d.beta), d.beta)
w2 = Wishart(m, int(2.0*d.b/d.beta), d.beta)
return (w1 + w2) \ w1
end
# A helper function for Jacobi samples
function SampleCSValues(n::Integer, a::Real, b::Real, beta::Real)
if beta == Inf
c =sqrt((a+[1:n])./(a+b+2*[1:n]))
s =sqrt((b+[1:n])./(a+b+2*[1:n]))
cp=sqrt([1:n-1]./(a+b+1+2*[1:n-1]))
sp=sqrt((a+b+1+[1:n-1])./(a+b+1+2*[1:n-1]))
else
#Generate cosine-squared values
csq = [rand(Beta(beta*(a+i)/2,beta*(b+i)/2)) for i=1:n]
cpsq = [rand(Beta(beta*i/2,beta*(a+b+1+i)/2)) for i=1:n]
#Cosine-sine pairs
c , s = sqrt(csq) , sqrt(1-csq)
cp, sp = sqrt(cpsq), sqrt(1-cpsq)
end
return c, s, cp, sp
end
#Generates a 2Mx2M sparse MANOVA matrix
#Jacobi ensemble
#
# Reference:
# A. Edelman and B. D. Sutton, "The beta-Jacobi matrix model, the CS decomposition,
# and generalized singular value problems", Foundations of Computational Mathematics,
# vol. 8 iss. 2 (2008), pp 259-285.
#TODO check normalization
function sprand(d::GaussianJacobi, n::Integer, a::Real, b::Real)
CoordI = zeros(8n-4)
CoordJ = zeros(8n-4)
Values = zeros(8n-4)
c, s, cp, sp = SampleCSValues(n, a, b, d.beta)
#Diagonals of each block
for i=1:n
CoordI[i], CoordJ[i] = i, i
Values[i] = i==1 ? c[n] : c[n+1-i] * sp[n+1-i]
end
for i=1:n
CoordI[n+i], CoordJ[n+i] = i, n+i
Values[n+i] = i==n ? s[1] : s[n+1-i] * sp[n-i]
end
for i=1:n
CoordI[2n+i], CoordJ[2n+i] = n+i, i
Values[2n+i] = i==1 ? -s[n] : -s[n+1-i] * sp[n+1-i]
end
for i=1:n
CoordI[3n+i], CoordJ[3n+i] = n+i, n+i
Values[3n+i] = i==n ? c[1] : c[n+1-i] * sp[n-i]
end
#Off-diagonals of each block
for i=1:n+1
CoordI[4n+i], CoordJ[4n+i] = i,i+1
Values[4n+i] = -s[n+1-i]*cp[n-i]
end
for i=1:n+1
CoordI[5n-1+i], CoordJ[5n-1+i] = i+1,n+i
Values[5n-1+i] = c[n-i]*cp[n-i]
end
for i=1:n+1
CoordI[6n-2+i], CoordJ[6n-2+i] = n+i,i+1
Values[6n-2+i] = -c[n+1-i]*cp[n-i]
end
for i=1:n+1
CoordI[7n-3+i], CoordJ[7n-3+i] = n+i,i+1
Values[7n-3+i] = -s[n-i]*cp[n-i]
end
return sparse(CoordI, CoordJ, Values)
end
#Return n eigenvalues distributed according to the Jacobi ensemble
function eigvalrand(d::GaussianJacobi, n::Integer)
#Generate just the upper left quadrant of the matrix
c, s, cp, sp = SampleCSValues(n, d.a, d.b, d.beta)
dv = [i==1 ? c[n] : c[n+1-i] * sp[n+1-i] for i=1:n]
ev = [-s[n+1-i]*cp[n-i] for i=1:n-1]
##TODO: understand why dv and ev are returned as Array{Any,1}
M = Bidiagonal(convert(Array{Float64,1},dv), convert(Array{Float64,1},ev), false)
return svdvals(M)
end
#TODO Check m and ns
function eigvaljpdf(d::GaussianJacobi, lambda::Vector{Eigenvalue}) where {Eigenvalue<:Number}
m = length(lambda)
#Jacobi parameters
a1, a2 = d.a, d.b
p = 1.0 + d.beta*(m-1)/2.0
#Calculate normalization constant
c = 1.0
for j=1:m
z1 = (a1 - beta*(m-j)/2.0)
if z1 < 0 && (int(z1) - z1) < eps()
return 0.0 #Pole of gamma function, there is no density here no matter what
end
z2 = (a2 - beta*(m-j)/2.0)
if z2 < 0 && (int(z2) - z2) < eps()
return 0.0 #Pole of gamma function, there is no density here no matter what
end
c *= gamma(1 + beta/2)*gamma(a1+a2-beta*(m-j)/2)
c /= gamma(1 + beta*j/2)*gamma(z1)*gamma(z2)
end
Prod = prod(lambda.^(a1-p))*prod((1-lambda).^(a2-p)) #Calculate Laguerre product term
Energy = sum(lambda/2) #Calculate argument of exponential
return c * VandermondeDeterminant(lambda, beta) * Prod * exp(-Energy)
end
| RandomMatrices | https://github.com/JuliaMath/RandomMatrices.jl.git |
|
[
"MIT"
] | 0.5.5 | 359d601ae45b05ea9a53d303dfbf477fcaca4195 | code | 1157 | export rand, Ginibre
import Base.rand
#Samples a matrix from the Ginibre ensemble
#This ensemble lives in GL(N, F), the set of all invertible N x N matrices
#over the field F
#For beta=1,2,4, F=R, C, H respectively
struct Ginibre <: ContinuousMatrixDistribution
beta::Float64
N::Integer
end
function rand(W::Ginibre)
beta, n = W.beta, W.N
if beta==1
randn(n,n)
elseif beta==2
randn(n,n)+im*randn(n,n)
elseif beta==4
Q0=randn(n,n)
Q1=randn(n,n)
Q2=randn(n,n)
Q3=randn(n,n)
[Q0+im*Q1 Q2+im*Q3;-Q2+im*Q3 Q0-im*Q1]
else
error(string("beta = ", beta, " not implemented"))
end
end
function jpdf(Z::AbstractMatrix{z}) where {z<:Complex}
pi^(size(Z,1)^2)*exp(-trace(Z'*Z))
end
#Dyson Circular orthogonal, unitary and symplectic ensembles
struct CircularOrthogonal
N :: Int64
end
function rand(M::CircularOrthogonal)
U = rand(Ginibre(2, M.N))
U * U'
end
struct CircularUnitary
N :: Int64
end
rand(M::CircularUnitary) = rand(Ginibre(2, M.N))
struct CircularSymplectic
N :: Int64
end
rand(M::CircularSymplectic) = error("Not implemented")
| RandomMatrices | https://github.com/JuliaMath/RandomMatrices.jl.git |
|
[
"MIT"
] | 0.5.5 | 359d601ae45b05ea9a53d303dfbf477fcaca4195 | code | 11282 | export permutations_in_Sn, compose, cycle_structure, data, part, #Functions working with partitions and permutations
partition, Haar, expectation, WeingartenUnitary, Stewart
const partition = Vector{Int}
#Functions working with partitions and permutations
# TODO Migrate these functions to Catalan
#Iterate over partitions of n in lexicographic order
function part(n::Integer)
if n==0 produce([]) end
if n<=0 return end
for p in @task part(n-1)
p = [p; 1]
produce(p)
p = p[1:end-1]
if length(p) == 1 || (length(p)>1 && p[end]<p[end-1])
p[end] += 1
produce(p)
p[end] -= 1
end
end
end
function permutations_in_Sn(n::Integer)
P = permutation_calloc(n)
while true
produce(P)
try permutation_next(P) catch; break end
end
end
function compose(P::Ptr{gsl_permutation}, Q::Ptr{gsl_permutation})
#Compose the permutations
n=convert(Int64, permutation_size(P))
@assert n==permutation_size(Q)
x=permutation_alloc(n)
Pv = [x+1 for x in pointer_to_array(permutation_data(P), (n,))]
Qv = [x+1 for x in pointer_to_array(permutation_data(Q), (n,))]
Xv = [Qv[i] for i in Pv]
for i=1:n
unsafe_assign(permutation_data(x), Xv[i]-1, i)
end
permutation_valid(x)
x
end
#Compute cycle structure, i.e. lengths of each cycle in the cycle factorization of the permutatation
function cycle_structure(P::Ptr{gsl_permutation})
n=convert(Int64, permutation_size(P))
Pcanon = permutation_linear_to_canonical(P)
PCANON = data(Pcanon)
HaveNewCycleAtPos = [PCANON[i]>PCANON[i+1] for i=1:n-1]
cycleindices = Int[1]
for i=1:n-1 if HaveNewCycleAtPos[i] push!(cycleindices, i+1) end end
push!(cycleindices, n+1)
cyclestructure = Int[cycleindices[i+1]-cycleindices[i] for i=1:length(cycleindices)-1]
@assert sum(cyclestructure) == n
cyclestructure
end
#Returns a vector of indices (starting from 1 in the Julia convention)
data(P::Ptr{gsl_permutation}) = [convert(Int64, x)+1 for x in
pointer_to_array(permutation_data(P), (convert(Int64, permutation_size(P)) ,))]
mutable struct Haar <: ContinuousMatrixDistribution
beta::Real
end
# In random matrix theory one often encounters expressions of the form
#
#X = Q * A * Q' * B
#
#where A and B are deterministic matrices with fixed numerical matrix entries
#and Q is a random matrix that does not have explicitly defined matrix
#elements. Instead, one takes an expectation over of expressions of this form
#and this "integrates out" the Qs to produce a numeric result.
#
#expectation(X) #= some number
#
#Here is a function that symbolically calculates the expectation of a product
#of matrices over the symmetric group that Q is uniform Haar over.
#It takes an expression consisting of a product of matrices and replaces it
#with an evaluated symbolic expression which is the expectation.
function expectation(X::Expr)
if X.head != :call
error(string("Unexpected type of expression: ", X.head))
end
n = length(X.args) - 1
if n < 1 return eval(X) end #nothing to do Haar-wise
if X.args[1] != :*
error("Unexpected expression, only products supported")
end
# Parse expression involving products of matrices to extract the
# positions of Haar matrices and their ctransposes
Qidx=[] #Indices for Haar matrices
Qpidx=[] #Indices for ctranspose of Haar matrices
Others=[]
MyQ=Nothing
for i=1:n
thingy=X.args[i+1]
if isa(thingy, Symbol)
if isa(eval(thingy), Haar)
if MyQ==Nothing MyQ=thingy end
if MyQ == thingy
Qidx=[Qidx; i]
else
warning("only one instance of Haar supported, skipping the other guy ", thingy) end
else
Others = [Others; (thingy, i, i+1)]
end
println(i, ' ', thingy, "::", typeof(eval(thingy)))
elseif isa(thingy, Expr)
println(i, ' ', thingy, "::Expr")
if thingy.head==symbol('\'') && length(thingy.args)>=1 #Maybe this is a Q'
if isa(thingy.args[1], Symbol) && isa(eval(thingy.args[1]), Haar)
println("Here is a Qtranspose")
Qpidx=[Qpidx; i]
end
end
else
error(string("Unexpected token ", thingy ," of type ", typeof(thingy)))
end
end
if length(Qidx) == length(Qpidx) == 0 return eval(X) end #nothing to do Haar-wise
println(MyQ, " is in places ", Qidx)
println(MyQ, "' is in places ", Qpidx)
n = length(Qidx)
#If there are different Qs and Q's, the answer is a big fat 0
if n != length(Qpidx) return zeros(size(eval(X.args[2]),1)...) end
##################################
# Step 2. Enumerate permutations #
##################################
AllTerms = Any[]
for sigma in @task permutations_in_Sn(n)
for tau in @task permutations_in_Sn(n)
sigma_inv=permutation_inverse(sigma)
#Compose the permutations
perm=compose(sigma_inv, tau)
#Compute cycle structure, i.e. lengths of each cycle in the cycle
#factorization of the permutatation
cyclestruct = cycle_structure(perm)
Qr = Int64[n+1 for n in Qidx]
Qpr= Int64[Qpidx[n]+1 for n in data(tau)]
#Consolidate deltas
Deltas=Dict()
for i=1:n
Deltas[Qr[i]] = Qpidx[data(sigma)[i]]
Deltas[Qidx[i]] = Qpr[data(tau)[i]]
end
#Print deltas
print("V(", cyclestruct, ") ")
for k in keys(Deltas)
print("δ(",k,",",Deltas[k],") ")
end
for (Symb, col_idx, row_idx) in Others
print(Symb,"(",col_idx,",",row_idx,") ")
end
println()
print("= V(", cyclestruct, ") ")
#Evaluate deltas over the indices of the other matrices
ReindexedSymbols = []
for (Symb, col_idx, row_idx) in Others
new_col, new_row = col_idx, row_idx
if has(Deltas, col_idx) new_col = Deltas[col_idx] end
if has(Deltas, row_idx) new_row = Deltas[row_idx] end
print(Symb,"(",new_col,",",new_row,") ")
ReindexedSymbols = [ReindexedSymbols; (Symb, new_col, new_row)]
end
println()
#Parse coefficient
Coefficient= WeingartenUnitary(perm)
#Reconstruct expression
println("START PARSING")
println("The term is =", ReindexedSymbols[end])
Symb, left_idx, right_idx = pop!(ReindexedSymbols)
Expression=[Any[Symbol[Symb], left_idx, right_idx]]
while length(ReindexedSymbols) > 0
pop_idx = expr_idx = do_transpose = is_left = nothing
for expr_iter in enumerate(Expression)
expr_idx, expr_string = expr_iter
_, left_idx, right_idx = expr_string
for iter in enumerate(ReindexedSymbols)
idx, data = iter
Symb, col_idx, row_idx = data
if row_idx == left_idx
pop_idx = idx
do_transpose = false
is_left = true
println("Case A")
break
elseif col_idx == right_idx
pop_idx = idx
do_transpose = false
is_left = false
println("Case B")
break
elseif row_idx == right_idx
pop_idx = idx
do_transpose = true
is_left = false
println("Case C")
break
elseif col_idx == left_idx
pop_idx = idx
do_transpose = true
is_left = true
println("Case D")
break
end
end
if pop_idx != nothing break end
end
println("Terms left = ", ReindexedSymbols)
if pop_idx == nothing #Found nothing, start new expression blob
println("New word: The term is ", ReindexedSymbols[1])
Symb, left_idx, right_idx = delete!(ReindexedSymbols, 1)
push!(Expression, [Symbol[Symb], left_idx, right_idx])
else #Found something
println("The term is =", ReindexedSymbols[pop_idx])
Symb, col_idx, row_idx = delete!(ReindexedSymbols, pop_idx)
Term = do_transpose ? Expr(symbol("'"), Symb) : Symb
if is_left
insert!(Expression[expr_idx][1], 1, Term)
Expression[expr_idx][2] = do_transpose ? row_idx : col_idx
else
push!(Expression[expr_idx][1], Term)
Expression[expr_idx][3] = do_transpose ? col_idx : row_idx
end
end
end
println("DONE PARSING TREE")
# Evaluate closed cycles
NewExpression=Any[]
for ExprChunk in Expression
ExprBlob, start_idx, end_idx = ExprChunk
print(ExprChunk, " => ")
if start_idx == end_idx #Have a cycle; this is a trace
ex= length(ExprBlob)==1 ? :(trace($(ExprBlob[1]))) :
Expr(:call, :trace, Expr(:call, :*, ExprBlob...))
else #Not a cycle, regular chain of multiplications
ex= length(ExprBlob)==1 ? ExprBlob[1] :
Expr(:call, :*, ExprBlob...)
end
push!(NewExpression, ex)
println(ex)
end
ex = Expr(:call, :*, Coefficient, NewExpression...)
println("Final expression is: ", ex)
push!(AllTerms, ex)
end
end
X = length(AllTerms)==1 ? AllTerms[1] : Expr(:call, :+, AllTerms...)
println("THE ANSWER IS ", X)
eval(X)
end
#Computes the Weingarten function for permutations
function WeingartenUnitary(P::Ptr{gsl_permutation})
C = cycle_structure(P)
WeingartenUnitary(C)
end
#Computes the Weingarten function for partitions
function WeingartenUnitary(P::partition)
n = sum(P)
m = length(P)
thesum = 0.0
for irrep in @task part(n)
#Character of the partition
S = character(irrep, P)
#Character of the identity divided by n!
T = character_identity(irrep)
#Denominator f_r(N) of (2.10)
f = prod([factorial(BigInt(N + P[i] - i)) / factorial(BigInt(N - i))
for i=1:m])
thesum += S*T/(factorial(n)*f)
println(irrep, " ", thesum)
end
thesum
end
| RandomMatrices | https://github.com/JuliaMath/RandomMatrices.jl.git |
|
[
"MIT"
] | 0.5.5 | 359d601ae45b05ea9a53d303dfbf477fcaca4195 | code | 4951 | # Computes samples of real or complex Haar matrices of size nxn
#
# For beta=1,2,4, generates random orthogonal, unitary and symplectic matrices
# of uniform Haar measure.
# These matrices are distributed with uniform Haar measure over the
# classical orthogonal, unitary and symplectic groups O(N), U(N) and
# Sp(N)~USp(2N) respectively.
#
# The last parameter specifies whether or not the piecewise correction
# is applied to ensure that it truly of Haar measure
# This addresses an inconsistency in the Householder reflections as
# implemented in most versions of LAPACK
# Method 0: No correction
# Method 1: Multiply rows by uniform random phases
# Method 2: Multiply rows by phases of diag(R)
# References:
# Edelman and Rao, 2005
# Mezzadri, 2006, math-ph/0609050
#TODO implement O(n^2) method
#By default, always do piecewise correction
#For most applications where you use the HaarMatrix as a similarity transform
#it doesn't matter, but better safe than sorry... let the user choose else
function rand(W::Haar, n::Int, doCorrection::Int=1)
beta = W.beta
M=rand(Ginibre(beta,n))
q,r=qr(M)
if doCorrection==0
q
elseif doCorrection==1
if beta==1
L = sign.(rand(n).-0.5)
elseif beta==2
L = exp.(im*rand(n)*2pi)
elseif beta==4
L = exp.(im*rand(2n)*2pi)
else
error(string("beta = ",beta, " not implemented."))
end
q*Diagonal(L)
elseif doCorrection==2
if beta==1
L=sign.(diag(r))
elseif (beta==2 || beta==4)
L=diag(r)
L=L./abs.(L)
else
error(string("beta = ",beta, " not implemented."))
end
q*Diagonal(L)
end
end
#A utility method to check if the piecewise correction is needed
#This checks the R part of the QR factorization; if correctly done,
#the diagonals are all chi variables so are non-negative
function NeedPiecewiseCorrection()
n=20
R=qr(randn(Ginibre(2,n)))[2]
return any([x<0 for x in diag(R)])
end
#TODO maybe, someday
#Haar measure on U(N) in terms of local coordinates
#Zyczkowski and Kus, Random unitary matrices, J. Phys. A: Math. Gen. 27,
#4235–4245 (1994).
import LinearAlgebra.BLAS: @blasfunc
using LinearAlgebra: BlasInt
for (s, elty) in (("dlarfg_", Float64),
("zlarfg_", ComplexF64))
@eval begin
function larfg!(n::Int, α::Ptr{$elty}, x::Ptr{$elty}, incx::Int, τ::Ptr{$elty})
ccall((@blasfunc($s), LAPACK.liblapack), Nothing,
(Ptr{BlasInt}, Ptr{$elty}, Ptr{$elty}, Ptr{BlasInt}, Ptr{$elty}),
Ref(n), α, x, Ref(incx), τ)
end
end
end
import Base.size
import LinearAlgebra: lmul!, rmul!, QRPackedQ, Diagonal, Adjoint
struct StewartQ{T,S<:AbstractMatrix{T},C<:AbstractVector{T},D<:AbstractVector{T}} <: LinearAlgebra.AbstractQ{T}
factors::S
τ::C
signs::D
end
size(Q::StewartQ, dim::Integer) = size(Q.factors, dim == 2 ? 1 : dim)
size(Q::StewartQ) = (n = size(Q.factors, 1); (n, n))
lmul!(A::StewartQ, B::AbstractVecOrMat) = lmul!(QRPackedQ(A.factors,A.τ), lmul!(Diagonal(A.signs), B))
rmul!(A::AbstractVecOrMat, B::StewartQ) = rmul!(rmul!(A, QRPackedQ(B.factors,B.τ)), Diagonal(B.signs))
@static if VERSION ≥ v"1.10"
import LinearAlgebra.AdjointQ
lmul!(adjA::AdjointQ{<:Any,<:StewartQ}, B::AbstractVecOrMat) =
lmul!(Diagonal(adjA.Q.signs), lmul!(QRPackedQ(adjA.Q.factors, adjA.Q.τ)', B))
rmul!(A::AbstractVecOrMat, adjB::AdjointQ{<:Any,<:StewartQ}) =
rmul!(rmul!(A, Diagonal(adjB.Q.signs)), QRPackedQ(adjB.Q.factors, adjB.Q.τ)')
else
lmul!(adjA::Adjoint{<:Any,<:StewartQ}, B::AbstractVecOrMat) =
lmul!(Diagonal(adjA.parent.signs), lmul!(QRPackedQ(adjA.parent.factors, adjA.parent.τ)', B))
rmul!(A::AbstractVecOrMat, adjB::Adjoint{<:Any,<:StewartQ}) =
rmul!(rmul!(A, Diagonal(adjB.parent.signs)), QRPackedQ(adjB.parent.factors,adjB.parent.τ)')
end
StewartQ{T}(Q::StewartQ) where {T} = StewartQ(convert(AbstractMatrix{T}, Q.factors), convert(Vector{T}, Q.τ), convert(Vector{T}, Q.signs))
AbstractMatrix{T}(Q::StewartQ{T}) where {T} = Q
AbstractMatrix{T}(Q::StewartQ) where {T} = StewartQ{T}(Q)
"""
Stewart's algorithm for sampling orthogonal/unitary random matrices in time O(n^2)
"""
function Stewart(::Type{T}, n) where {T<:Union{Float64,ComplexF64}}
τ = Array{T}(undef, n)
signs = Vector{T}(undef, n)
H = randn(T, n, n)
pτ = pointer(τ)
pβ = pointer(H)
pH = pointer(H, 2)
incr = (T <: Real ? 1 : 2)
for i = 0:n-1
larfg!(n - i, pβ + (n + 1)*8*incr*i, pH + (n + 1)*8*incr*i, 1, pτ + 8*incr*i)
signs[i+1] = sign(real(H[i+1, i+1]))
end
return StewartQ(H, τ, signs)
end
export randfast
function randfast(W::Haar, n::Int)
if W.beta==1
Stewart(Float64, n)
elseif W.beta==2
Stewart(ComplexF64, n)
else
error("beta = $beta not implemented")
end
end
| RandomMatrices | https://github.com/JuliaMath/RandomMatrices.jl.git |
|
[
"MIT"
] | 0.5.5 | 359d601ae45b05ea9a53d303dfbf477fcaca4195 | code | 1444 | module RandomMatrices
using Combinatorics
using GSL
using SpecialFunctions, FastGaussQuadrature
using LinearAlgebra
import Base: isinf, rand, convert
import Distributions: ContinuousUnivariateDistribution,
ContinuousMatrixDistribution,
Beta, Chi,
cdf, pdf, entropy, insupport, mean, median, modes, kurtosis, skewness, std, var, moment
export bidrand, #Generate random bidiagonal matrix
tridrand, #Generate random tridiagonal matrix
sprand, #Generate random sparse matrix
eigvalrand, #Generate random set of eigenvalues
eigvaljpdf, #Eigenvalue joint probability density
cumulant, freecumulant, cf, mgf,
cdf, pdf, entropy, insupport, mean, median, modes, kurtosis, skewness, std, var, moment
const Dim2 = Tuple{Int, Int} #Dimensions of a rectangular matrix
#Classical Gaussian matrix ensembles
include("GaussianEnsembles.jl")
# Classical univariate distributions
include("densities/Semicircle.jl")
include("densities/TracyWidom.jl")
# Ginibre
include("Ginibre.jl")
# determinantal point processes
include("dpp.jl")
#Generating matrices of Haar measure
include("Haar.jl")
include("HaarMeasure.jl")
#Fast histogrammer for matrix eigenvalues - hist_eig
include("FastHistogram.jl")
#Formal power series
include("FormalPowerSeries.jl")
#Statistical tests based on random matrix theory
include("StatisticalTests.jl")
#Stochastic processes
include("StochasticProcess.jl")
end
| RandomMatrices | https://github.com/JuliaMath/RandomMatrices.jl.git |
|
[
"MIT"
] | 0.5.5 | 359d601ae45b05ea9a53d303dfbf477fcaca4195 | code | 1062 | import Distributions
#Compute Mauchly's statistic (valid under assumption of multinormality)
#Mauchly, 1940; Kendall and Stuart, 1968
#n is the number of data points (samples)
#This returns both the value of the test statistic and the expected distribution to test against
function MauchlySphericityTestStatistic(PopulationCovariance::AbstractMatrix{T}, SampleCovariance::AbstractMatrix{T}, n::Integer) where {T<:Real}
p = size(SampleCovariance)[1]
C = inv(PopulationCovariance) * SampleCovariance
l = (det(C)/(trace(C)/p)^p)
x = -n*log(l)
f = p*(p+1)/2-1
return x, Chisq(f)
end
#Johnstone's variant of the sphericity test
#n, p>= 10 recommended
#Johnstone (2001)
function JohnstoneSphericityTestStatistic(PopulationCovariance::AbstractMatrix{T}, SampleCovariance::AbstractMatrix{T}, n::Integer) where {T<:Real}
C = inv(PopulationCovariance) * SampleCovariance
v = max(eigvals(C))
mu=(sqrt(n-1)+sqrt(p))^2
sigma=sqrt(mu)*(1/sqrt(n-1)+1/sqrt(p))^(1/3)
(n*l-mu)/sigma #To be tested against Tracy-Widom with beta=1
end
| RandomMatrices | https://github.com/JuliaMath/RandomMatrices.jl.git |
|
[
"MIT"
] | 0.5.5 | 359d601ae45b05ea9a53d303dfbf477fcaca4195 | code | 1643 | #Matrices related to stochastic processes
import Base: iterate
export AiryProcess, BrownianProcess, WhiteNoiseProcess, next!
abstract type StochasticProcess{T<:Real} end
struct WhiteNoiseProcess{T<:Real} <: StochasticProcess{T}
dt::T
end
struct BrownianProcess{T<:Real} <: StochasticProcess{T}
dt::T
end
struct AiryProcess{S<:Real, T<:Real} <: StochasticProcess{T}
dt::T
beta::S
end
done(p::StochasticProcess{T}, x...) where {T} = false #Processes can always go on forever
#######################
# White noise process #
#######################
iterate(p::WhiteNoiseProcess, state=()) = (randn()*sqrt(p.dt), ())
####################
# Brownian process #
####################
function iterate(p::BrownianProcess{T}, state=zero(T)) where {T}
newx = state + randn()*sqrt(p.dt)
return newx, newx
end
################
# Airy process #
################
"""
Like next, but update only the state of the AiryProcess
Skip the eigenvalue computation, which gets expensive
"""
function next!(p::AiryProcess{T}, S::SymTridiagonal{T}=SymTridiagonal(T[-(2/p.dt^2)], T[])) where {T} # TODO maybe change this to iterate!
t = (size(S, 1)-1)*p.dt
#Discredited Airy operator plus diagonal noise
x = inv(p.dt^2)
push!(S.dv, -2x - t + 2/sqrt(p.dt*p.beta)*randn())
push!(S.ev, x)
return S
end
function iterate(p::AiryProcess{T}, S::SymTridiagonal{T}= SymTridiagonal(T[-(2/p.dt^2)], T[])) where {T}
t = (size(S, 1)-1)*p.dt
#Discredited Airy operator plus diagonal noise
x = inv(p.dt^2)
push!(S.dv, -2x - t + 2/sqrt(p.dt*p.beta)*randn())
push!(S.ev, x)
return (eigmax(S), S)
end
| RandomMatrices | https://github.com/JuliaMath/RandomMatrices.jl.git |
|
[
"MIT"
] | 0.5.5 | 359d601ae45b05ea9a53d303dfbf477fcaca4195 | code | 2422 | # Random samples from determinantal point processes
"""
Computes a random sample from the determinantal point process defined by the
spectral factorization object `L`.
Inputs:
`L`: `Eigen` factorization object of an N x N matrix
Output:
`Y`: A `Vector{Int}` with entries in [1:N].
References:
Algorithm 18 of \\cite{HKPV05}, as described in Algorithm 1 of \\cite{KT12}.
@article{HKPV05,
author = {Hough, J Ben and Krishnapur, Manjunath and Peres, Yuval and Vir\'{a}g, B\'{a}lint},
doi = {10.1214/154957806000000078},
journal = {Probability Surveys},
pages = {206--229},
title = {Determinantal Processes and Independence},
volume = {3},
year = {2005}
archivePrefix = {arXiv},
eprint = {0503110},
}
@article{KT12,
author = {Kulesza, Alex and Taskar, Ben},
doi = {10.1561/2200000044},
journal = {Foundations and Trends in Machine Learning},
number = {2-3},
pages = {123--286},
title = {Determinantal Point Processes for Machine Learning},
volume = {5},
year = {2012},
archivePrefix = {arXiv},
eprint = {1207.6083},
}
TODO Check loss of orthogonality - a tip from Zelda Mariet
"""
function rand(L::LinearAlgebra.Eigen{S,T}) where {S<:Real,T}
N = length(L.values)
J = Int[]
for n=1:N
λ = L.values[n]
rand() < λ/(λ+1) && push!(J, n)
end
V = L.vectors[:, J]
Y = Int[]
nV = size(V, 2)
while true
# Select i from 𝒴=[1:N] (ground set) with probabilities
# Pr(i) = 1/|V| Σ_{v∈V} (v⋅eᵢ)²
#Compute selection probabilities
Pr = zeros(N)
for i=1:N
for j=1:nV #TODO this loop is a bottleneck - why?
Pr[i] += (V[i,j])^2 #ith entry of jth eigenvector
end
Pr[i] /= nV
end
@assert abs(1-sum(Pr)) < N*eps() #Check normalization
#Simple discrete sampler
i, ρ = N, rand()
for j=1:N
if ρ < Pr[j]
i = j
break
else
ρ -= Pr[j]
end
end
push!(Y, i)
nV == 1 && break #Done
#V = V⊥ #an orthonormal basis for the subspace of V ⊥ eᵢ
V[i, :] = 0 #Project out eᵢ
V = full(qrfact!(V)[:Q])[:, 1:nV-1]
nV = size(V, 2)
end
return Y
end
| RandomMatrices | https://github.com/JuliaMath/RandomMatrices.jl.git |
|
[
"MIT"
] | 0.5.5 | 359d601ae45b05ea9a53d303dfbf477fcaca4195 | code | 4035 | export Semicircle
"""
Wigner semicircle distribution
By default, creates a standardized distribution
with mean 0 and variance 1 (radius 2)
"""
struct Semicircle{T<:Real} <: ContinuousUnivariateDistribution
mean::T
radius::T
Semicircle{T}(μ::T,r::T) where T = new(μ,r)
end
Semicircle(μ::T=0.0, r::T=2.0) where {T<:Real} = r > 0 ? Semicircle{T}(μ, r) :
throw(ArgumentError("radius r must be positive, got $r"))
Semicircle{T}(d::Semicircle) where T = Semicircle{T}(convert(T, d.mean), convert(T, d.radius))
convert(::Type{Semicircle{T}}, d::Semicircle{T}) where T = d
convert(::Type{Semicircle}, d::Semicircle) = d
convert(::Type{Semicircle{T}}, d::Semicircle) where T = Semicircle{T}(convert(T, d.mean), convert(T, d.radius))
# Distribution function methods
###############################
# cumulative distribution function
function cdf(d::Semicircle{T}, x::T) where {T<:Real}
a, r = d.mean, d.radius
if insupport(d, x)
return 0.5 + (x-a)/(π*r^2) * √(r^2 - (x-a)^2) + asin((x-a)/r)/π
elseif x ≥ a
return one(T)
else
return zero(T)
end
end
# probability density function
function pdf(d::Semicircle{T}, x::T) where {T<:Real}
a, r = d.mean, d.radius
if insupport(d, x)
return 2/(π*r^2) * √(r^2 - (x-a)^2)
else
return zero(T)
end
end
# predicate is x in the support of the distribution?
insupport(d::Semicircle{T}, x::T) where {T<:Real} = abs(x-d.mean) < d.radius
function cdf(X::Semicircle{T}, x::V) where {T<:Real,V<:Real}
TV = promote_type(T,V)
cdf(convert(Semicircle{TV},X), convert(TV,x))
end
# probability density function
function pdf(X::Semicircle{T}, x::V) where {T<:Real,V<:Real}
TV = promote_type(T,V)
pdf(convert(Semicircle{TV},X), convert(TV,x))
end
# predicate is x in the support of the distribution?
function insupport(X::Semicircle{T}, x::V) where {T<:Real,V<:Real}
TV = promote_type(T,V)
insupport(convert(Semicircle{TV},X), convert(TV,x))
end
#Entropy methods
################
# entropy of distribution in nats
entropy(X::Semicircle)=log(π*X.radius) - 0.5
#Measures of central tendency methods
#####################################
# mean of distribution
mean(X::Semicircle)=X.mean
# median of distribution
median(X::Semicircle)=X.mean
# mode(s) of distribution as vector
modes(X::Semicircle{T}) where {T} = T[X.mean]
# kurtosis of the distribution
kurtosis(X::Semicircle{T}) where {T} = T(2)
# skewness of the distribution
skewness(X::Semicircle{T}) where {T} = T(0)
# standard deviation of distribution
std(X::Semicircle)=X.radius/2
# variance of distribution
var(X::Semicircle)=std(X)^2
# moment of distribution
function moment(X::Semicircle{T}, order::Integer) where {T<:Real}
a, r = X.mean, X.radius
if X.mean != 0
return a^n*hypergeom([(1-n)/2, -n/2], 2, (r/a)^2)
elseif iseven(order)
return (0.5*r)^(2order) * T(catalannum(order÷2))
else
return zero(T)
end
end
# cumulant of distribution
function cumulant(d::Semicircle{T}, order::Integer) where {T<:Real}
if d.mean != 0
throw(ArgumentError("Non-central Semicircle not supported"))
end
if order==0
return one(T)
elseif iseven(order)
return (0.5*d.radius)^(2order) * T(lassallenum(order÷2))
else
return zero(T)
end
end
# free cumulant of distribution
function freecumulant(d::Semicircle{T}, order::Int) where {T<:Real}
if order == 0
return one(T)
elseif order == 1
return mean(X)
elseif order == 2
return var(X)
else
return zero(T)
end
end
#Generating function methods
############################
# characteristic function
function cf(d::Semicircle, t::Real)
r = t / d.mean
2 * besselj(1, r)/r
end
# moment generating function
function mgf(d::Semicircle, t::Real)
r = t * d.mean
2 * besseli(1, r)/r
end
#Sampling methods
#################
# random sampler
# Use relationship with beta distribution
function rand(X::Semicircle)
Y = rand(Beta(1.5, 1.5))
X.mean + 2 * X.radius * Y - X.radius
end
| RandomMatrices | https://github.com/JuliaMath/RandomMatrices.jl.git |
|
[
"MIT"
] | 0.5.5 | 359d601ae45b05ea9a53d303dfbf477fcaca4195 | code | 3769 | export TracyWidom
"""
Tracy-Widom distribution
The probability distribution of the normalized largest eigenvalue of a random
matrix with iid Gaussian matrix elements of variance 1/2 and mean 0.
The cdf of Tracy-Widom is given by
``
F_β (s) = lim_{n→∞} Pr(√2 n^{1/6} (λ_max - √(2n) ≤ s)
``
where β = 1, 2, or 4 for the orthogonal, unitary, or symplectic ensembles.
References:
1. doi:10.1016/0370-2693(93)91114-3
2. doi:10.1007/BF02100489
3. doi.org/10.1007/BF02099545
Numerical routines adapted from Alan Edelman's course notes for MIT 18.338,
Random Matrix Theory, 2016.
"""
struct TracyWidom{T} <: ContinuousUnivariateDistribution
β::T
end
"""
Cumulative density function of the Tracy-Widom distribution.
Computes the Tracy-Widom distribution by Bornemann's method of evaluating
a finite dimensional approximation to the Fredholm determinant using quadrature.
doi.org/10.1090/S0025-5718-09-02280-7
# Arguments
* `d::TracyWidom` or `Type{TracyWidom}`: an instance of `TracyWidom` or the type itself
* `s::Real`: The point at which to evaluate the cdf
* `num_points::Integer = 25`: The number of points in the quadrature
"""
function cdf(d::TracyWidom, s::T; num_points::Integer=25) where {T<:Real}
d.β ∈ (1,2,4) || throw(ArgumentError("β must be 1, 2, or 4"))
quad = gausslegendre(num_points)
_TWcdf(s, d.β, quad)
end
function cdf(d::Type{TracyWidom}, s::T; beta::Integer=2, num_points::Integer=25) where {T<: Real}
cdf(d(beta), s, num_points=num_points)
end
function cdf(d::TracyWidom, s_vals::AbstractArray{T}; num_points::Integer=25) where {T<:Real}
d.β ∈ (1,2,4) || throw(ArgumentError("β must be 1, 2, or 4"))
quad = gausslegendre(num_points)
[_TWcdf(s, d.β, quad) for s in s_vals]
end
function cdf(d::Type{TracyWidom}, s_vals::AbstractArray{T}; beta::Integer=2, num_points::Integer=25) where {T<:Real}
cdf(d(beta), s_vals, num_points=num_points)
end
function _TWcdf(s::T, beta::Integer, quad::Tuple{Array{Float64,1},Array{Float64,1}}) where {T<:Real}
if beta == 2
kernel = ((ξ,η) -> _K2tilde(ξ,η,s))
return _fredholm_det(kernel, quad)
elseif beta == 1
kernel = ((ξ,η) -> _K1tilde(ξ,η,s))
return _fredholm_det(kernel, quad)
elseif beta == 4
kernel2 = ((ξ,η) -> _K2tilde(ξ,η,s*sqrt(2)))
kernel1 = ((ξ,η) -> _K1tilde(ξ,η,s*sqrt(2)))
F2 = _fredholm_det(kernel2, quad)
F1 = _fredholm_det(kernel1, quad)
return (F1 + F2/F1) / 2
end
end
function _fredholm_det(kernel::Function, quad::Tuple{Array{T,1},Array{T,1}}) where {T<:Real}
nodes, weights = quad
N = length(nodes)
sqrt_weights = sqrt.(weights)
weights_matrix = kron(transpose(sqrt_weights),sqrt_weights)
K_matrix = [kernel(ξ,η) for ξ in nodes, η in nodes]
det(I - weights_matrix .* K_matrix)
end
_ϕ(ξ, s) = s + 10*tan(π*(ξ+1)/4)
_ϕprime(ξ) = (5π/2)*(sec(π*(ξ+1)/4))^2
# For beta = 2
function _airy_kernel(x, y)
if x==y
return (airyaiprime(x))^2 - x * (airyai(x))^2
else
return (airyai(x) * airyaiprime(y) - airyai(y) * airyaiprime(x)) / (x - y)
end
end
_K2tilde(ξ,η,s) = sqrt(_ϕprime(ξ) * _ϕprime(η)) * _airy_kernel(_ϕ(ξ,s), _ϕ(η,s))
# For beta = 1
_A_kernel(x,y) = airyai((x+y)/2) / 2
_K1tilde(ξ,η,s) = sqrt(_ϕprime(ξ) * _ϕprime(η)) * _A_kernel(_ϕ(ξ,s), _ϕ(η,s))
"""
Samples the largest eigenvalue of the n × n GUE matrix
"""
function rand(d::TracyWidom, n::Int)
n > 1 || error("Cannot construct $n × $n matrix")
if n < 100
k = n
else #Exploit the fact that the eigenvector is concentrated in the top left corner
k = round(Int, n-10*n^(1/3)-1)
end
a=randn(n-k+1)
b=[χ(i) for i=(n-1):-1:k]
v=eigmax(SymTridiagonal(a, b))
end
rand(d::Type{TracyWidom}, t::Integer) = rand(d(2), t)
| RandomMatrices | https://github.com/JuliaMath/RandomMatrices.jl.git |
|
[
"MIT"
] | 0.5.5 | 359d601ae45b05ea9a53d303dfbf477fcaca4195 | code | 2456 | using RandomMatrices
using LinearAlgebra: norm
using Test
@testset "FormalPowerSeries" begin
seed!(4)
# Excursions in formal power series (fps)
MaxSeriesSize=8
MaxRange = 20
MatrixSize=10
TT=Int64
(n1, n2, n3) = rand(1:MaxSeriesSize, 3)
X = FormalPowerSeries{TT}(rand(1:MaxRange, n1))
Y = FormalPowerSeries{TT}(rand(1:MaxRange, n2))
Z = FormalPowerSeries{TT}(rand(1:MaxRange, n3))
c = rand(1:MatrixSize) #Size of matrix representation to generate
nzeros = rand(1:MaxSeriesSize)
@test X == trim(X)
XX = deepcopy(X)
for i=1:nzeros
idx = rand(1:MaxRange)
if !haskey(XX.c, idx)
XX.c[idx] = convert(TT, 0)
end
end
@test trim(XX) == X
#Test addition, p.15, (1.3-4)
@test X+X == 2X
@test X+Y == Y+X
@test MatrixForm(X+Y,c) == MatrixForm(X,c)+MatrixForm(Y,c)
#Test subtraction, p.15, (1.3-4)
@test X-X == 0X
@test X-Y == -(Y-X)
@test MatrixForm(X-Y,c) == MatrixForm(X,c)-MatrixForm(Y,c)
#Test multiplication, p.15, (1.3-5)
@test X*Y == Y*X
@test MatrixForm(X*X,c) == MatrixForm(X,c)*MatrixForm(X,c)
@test MatrixForm(X*Y,c) == MatrixForm(X,c)*MatrixForm(Y,c)
@test MatrixForm(Y*X,c) == MatrixForm(Y,c)*MatrixForm(X,c)
@test MatrixForm(Y*Y,c) == MatrixForm(Y,c)*MatrixForm(Y,c)
@test X.*Y == Y.*X
#The reciprocal series has associated matrix that is the matrix inverse
#of the original series
#Force reciprocal to exist
X.c[0] = 1
discrepancy = (norm(inv(float(MatrixForm(X,c)))[1, :][:, 1] - tovector(reciprocal(X, c), 0:c-1)))
tol = c*√eps()
if discrepancy > tol
error(string("Error ", discrepancy, " exceeds tolerance ", tol))
end
#@test norm(inv(float(MatrixForm(X,c)))[1, :]'[:, 1] - tovector(reciprocal(X, c),c)) < c*sqrt(eps())
#Test differentiation
XX = derivative(X)
for (k, v) in XX.c
k==0 && continue
@test X.c[k+1] == v/(k+1)
end
#Test product rule [H, Sec.1.4, p.19]
@test derivative(X*Y) == derivative(X)*Y + X*derivative(Y)
#Test right distributive law of composition [H, Sec.1.6, p.38]
@test compose(X,Z)*compose(Y,Z) == compose(X*Y, Z)
#Test chain rule [H, Sec.1.6, p.40]
@test derivative(compose(X,Y)) == compose(derivative(X),Y)*derivative(Y)
# zero and one elements
x = FormalPowerSeries([1,2,3])
@test zero(x) == FormalPowerSeries{Int64}(Dict{BigInt, Int64}())
@test zero(typeof(x)) == FormalPowerSeries{Int64}(Dict{BigInt, Int64}())
@test one(x) == FormalPowerSeries{Int64}(Dict{BigInt, Int64}(0 => 1))
@test one(typeof(x)) == FormalPowerSeries{Int64}(Dict{BigInt, Int64}(0 => 1))
end # testset
| RandomMatrices | https://github.com/JuliaMath/RandomMatrices.jl.git |
|
[
"MIT"
] | 0.5.5 | 359d601ae45b05ea9a53d303dfbf477fcaca4195 | code | 602 | using RandomMatrices
using Test
@testset "GaussianEnsembles" begin
@test Wigner{3} == GaussianHermite{3}
n = 25
for (β, T, N) in [(1, Real, n), (2, Complex, n), (4, Complex, 2n)]
d = Wigner(β)
A = rand(d, n)
@test eltype(A) <: T
@test size(A) == (N, N)
At = tridrand(d, n)
@test eltype(At) <: Real
@test size(At) == (n, n)
vals = eigvalrand(d, n)
@test eltype(vals) <: Real
@test length(vals) == n
vd = RandomMatrices.VandermondeDeterminant(vals, β)
@test isa(vd, Real)
ed = eigvaljpdf(d, vals)
@test isa(ed, Real)
end
end # testset
| RandomMatrices | https://github.com/JuliaMath/RandomMatrices.jl.git |
|
[
"MIT"
] | 0.5.5 | 359d601ae45b05ea9a53d303dfbf477fcaca4195 | code | 789 | using RandomMatrices
using LinearAlgebra: I, tr, Diagonal, QRPackedQ
using Test
@testset "Haar" begin
N=5
A=randn(N,N)
B=randn(N,N)
Q=rand(Haar(1), N)
#Test case where there are no symbolic Haar matrices
@test_broken eval(expectation(:(A*B))) ≈ A*B
#Test case where there is one pair of symbolic Haar matrices
@test_broken tr(eval(expectation(:(A*Q*B*Q')))) ≈ tr(A)*tr(B)/N
println("Case 3")
@test_broken println("E(A*Q*B*Q'*A*Q*B*Q') = ", eval(expectation(N, :Q, :(A*Q*B*Q'*A*Q*B*Q'))))
for T in (Float64, ComplexF64)
A = Stewart(T, N)
@test A'A ≈ Matrix{T}(I, N, N)
A2 = Matrix(A)
@test A2 ≈ QRPackedQ(A.factors, A.τ)*Diagonal(A.signs)
C = randn(T, N, N)
@test A*C ≈ A2*C
@test C*A ≈ C*A2
@test A'*C ≈ A2'*C
@test C*A' ≈ C*A2'
end
end # testset
| RandomMatrices | https://github.com/JuliaMath/RandomMatrices.jl.git |
|
[
"MIT"
] | 0.5.5 | 359d601ae45b05ea9a53d303dfbf477fcaca4195 | code | 470 | using RandomMatrices
using Test
@testset "StochasticProcess" begin
seed!(1)
dx = 0.001
let
p = WhiteNoiseProcess(dx)
x, S = iterate(p)
@test typeof(x) == typeof(dx)
@test isa(S, Tuple{})
@test iterate(p, S) != nothing
end
let
p = BrownianProcess(dx)
x, S = iterate(p)
@test x == S
@test typeof(x) == typeof(dx)
@test iterate(p, S) != nothing
end
let
p = AiryProcess(dx, 2.0)
x, S = iterate(p)
@test S[1,1] == -2/dx^2
@test next!(p, S) != nothing
end
end # testset
| RandomMatrices | https://github.com/JuliaMath/RandomMatrices.jl.git |
|
[
"MIT"
] | 0.5.5 | 359d601ae45b05ea9a53d303dfbf477fcaca4195 | code | 328 | using RandomMatrices
using Random: seed!
using Test
@testset "RandomMatrices" begin
seed!(1)
include("GaussianEnsembles.jl")
include("FormalPowerSeries.jl")
include("Haar.jl")
include("StochasticProcess.jl")
@testset "densities" begin
include("densities/Semicircle.jl")
include("densities/TracyWidom.jl")
end
end
| RandomMatrices | https://github.com/JuliaMath/RandomMatrices.jl.git |
|
[
"MIT"
] | 0.5.5 | 359d601ae45b05ea9a53d303dfbf477fcaca4195 | code | 687 | using RandomMatrices
using Test
@testset "Semicircle" begin
let
d = Semicircle()
@test cdf(d, 2.0) == 1.0
@test cdf(d, -2.0) == 0.0
@test pdf(d, 2.0) == 0.0
@test pdf(d, -2.0) == 0.0
@test entropy(d) == log(2π) - 0.5
@test mean(d) == median(d) == skewness(d) == 0
@test modes(d) == [0]
@test std(d) == var(d) == 1
@test kurtosis(d) == 2
@test isfinite(rand(d))
@test moment(d, 4) == 2
@test cumulant(d, 4) == 1
@test moment(d, 5) == cumulant(d, 5) == freecumulant(d, 5) == 0
@test cf(d, 1.0) == 0.0
#XXX Throws AmosException - ???
#@show mgf(d, 1.0)
x = rand(d)
@test insupport(d, x)
end
end # testset
| RandomMatrices | https://github.com/JuliaMath/RandomMatrices.jl.git |
|
[
"MIT"
] | 0.5.5 | 359d601ae45b05ea9a53d303dfbf477fcaca4195 | code | 525 | using RandomMatrices
using Test
@testset "TracyWidom" begin
# CDF lies in correct range
@test all(i->(0<i<1), cdf(TracyWidom, randn(5)))
#Test far outside support
@test cdf(TracyWidom, -10.1) ≈ 0 atol=1e-14
@test cdf(TracyWidom, 10.1) ≈ 1 atol=1e-14
# Test exact values
# See https://arxiv.org/abs/0904.1581
@test cdf(TracyWidom,0,beta=1) ≈ 0.83190806620295 atol=1e-14
@test cdf(TracyWidom,0,beta=2) ≈ 0.96937282835526 atol=1e-14
@test isfinite(rand(TracyWidom, 10))
@test isfinite(rand(TracyWidom, 100))
end # testset
| RandomMatrices | https://github.com/JuliaMath/RandomMatrices.jl.git |
|
[
"MIT"
] | 0.5.5 | 359d601ae45b05ea9a53d303dfbf477fcaca4195 | docs | 9406 | RandomMatrices.jl
=================
Random matrix package for [Julia](http://julialang.org).
[](http://pkg.julialang.org/?pkg=RandomMatrices)
[](https://travis-ci.org/JuliaMath/RandomMatrices.jl)
[](https://coveralls.io/github/JuliaMath/RandomMatrices.jl?branch=master)
[](https://codecov.io/github/JuliaMath/RandomMatrices.jl?branch=master)
[](https://zenodo.org/badge/latestdoi/5087/jiahao/RandomMatrices.jl)
This extends the [Distributions](https://github.com/JuliaStats/Distributions.jl)
package to provide methods for working with matrix-valued random variables,
a.k.a. random matrices. State of the art methods for computing random matrix
samples and their associated distributions are provided.
The names of the various ensembles can vary widely across disciplines. Where possible,
synonyms are listed.
Additional functionality is provided when these optional packages are installed:
- Symbolic manipulation of Haar matrices with [GSL.jl](https://github.com/jiahao/GSL.jl)
# Gaussian matrix ensembles
Much of classical random matrix theory has focused on matrices with matrix elements comprised of
independently and identically distributed (iid) real, complex or quaternionic Gaussians.
(Traditionally, these are associated with a parameter `beta` tracking the number of independent
real random variables per matrix element, i.e. `beta=1,2,4` respectively. This is also referred
to as the Dyson 3-fold way.)
Methods are provided for calculating random variates (samples) and various properties of these
random matrices.
The hierarchy of dense matrices provided are
- Ginibre ensemble - all matrix elements are iid with no global symmetry
- Hermite ensemble - one global symmetry
- Gaussian orthogonal ensemble (GOE, `beta=1`) - real and symmetric
- Gaussian unitary ensemble (GUE, `beta=2`) - complex and Hermitian
- Gaussian symplectic ensemble (GSE, `beta=4`) - quaternionic and self-dual
- Circular ensemble - uniformly distributed with `|det|=1`
- Circular orthogonal ensemble (COE, `beta=1`)
- Circular unitary ensemble (CUE, `beta=2`)
- Circular symplectic ensemble (CSE, `beta=4`)
- Laguerre matrices = white Wishart matrices
- Jacobi matrices = MANOVA matrices
Unless otherwise specified, `beta=1,2,4` are supported. For the symplectic matrices `beta=4`,
the 2x2 outer block-diagonal complex representation `USp(2N)` is used.
## Joint probability density functions (jpdfs)
Given eigenvalues `lambda` and the `beta` parameter of the random matrix distribution:
- `VandermondeDeterminant(lambda, beta)` computes the Vandermonde determinant
- `HermiteJPDF(lambda, beta)` computes the jpdf for the Hermite ensemble
- `LaguerreJPDF(lambda, n, beta)` computes the jpdf for the Laguerre(n) ensemble
- `JacobiJPDF(lambda, n1, n2, beta)` computes the jpdf for the Jacobi(n1, n2) ensemble
## Matrix samples
Constructs samples of random matrices corresponding to the classical Gaussian
Hermite, Laguerre(m) and Jacobi(m1, m2) ensembles.
- `GaussianHermiteMatrix(n, beta)`, `GaussianLaguerreMatrix(n, m, beta)`,
`GaussianJacobiMatrix(n, m1, m2, beta)`
each construct a sample dense `n`x`n` matrix for the corresponding matrix
ensemble with `beta=1,2,4`
- `GaussianHermiteTridiagonalMatrix(n, beta)`,
`GaussianLaguerreTridiagonalMatrix(n, m, beta)`,
`GaussianJacobiSparseMatrix(n, m1, m2, beta)`
each construct a sparse `n`x`n` matrix for the corresponding matrix ensemble
for arbitrary positive finite `beta`.
`GaussianHermiteTridiagonalMatrix(n, Inf)` is also allowed.
These sampled matrices have the same eigenvalues as above but are much faster
to diagonalize oweing to their sparsity. They also extend Dyson's threefold
way to arbitrary `beta`.
- `GaussianHermiteSamples(n, beta)`,
`GaussianLaguerreSamples(n, m, beta)`,
`GaussianJacobiSamples(n, m1, m2, beta)`
return a set of `n` eigenvalues from the sparse random matrix samples
- `HaarMatrix(n, beta)`
Generates a random matrix from the `beta`-circular ensemble.
- `HaarMatrix(n, beta, correction)` provides fine-grained control of what kind of correction
is applied to the raw QR decomposition. By default, `correction=1` (Edelman's correction) is
used. Other valid values are `0` (no correction) and `2` (Mezzadri's correction).
- `NeedsPiecewiseCorrection()` implements a simple test to see if a correction is necessary.
The parameters `m`, `m1`, `m2` refer to the number to independent "data" degrees of freedom.
For the dense samples these must be `Integer`s but can be `Real`s for the rest.
# Formal power series
Allows for manipulations of formal power series (fps) and formal Laurent series
(fLs), which come in handy for the computation of free cumulants.
## Types
- `FormalPowerSeries`: power series with coefficients allowed only for
non-negative integer powers
- `FormalLaurentSeries`: power series with coefficients allowed for all
integer powers
## FormalPowerSeries methods
### Elementary operations
- basic arithmetic operations `==`, `+`, `-`, `^`
- `*` computes the Cauchy product (discrete convolution)
- `.*` computes the Hadamard product (elementwise multiplication)
- `compose(P,Q)` computes the series composition P.Q
- `derivative` computes the series derivative
- `reciprocal` computes the series reciprocal
### Utility methods
- `trim(P)` removes extraneous zeroes in the internal representation of `P`
- `isalmostunit(P)` determines if `P` is an almost unit series
- `isconstant(P)` determines if `P` is a constant series
- `isnonunit(P)` determines if `P` is a non-unit series
- `isunit(P)` determines if `P` is a unit series
- `MatrixForm(P)` returns a matrix representation of `P` as an upper triangular
Toeplitz matrix
- `tovector` returns the series coefficients
# Densities
Famous distributions in random matrix theory
- `Semicircle` provides the semicircle distribution
- `TracyWidom` computes the Tracy-Widom density distribution
by brute-force integration of the Painlevé II equation
# Utility functions
- `hist_eig` computes the histogram of eigenvalues of a matrix using the
method of Sturm sequences.
This is recommended for `SymTridiagonal` matrices as it is significantly
faster than `hist(eigvals())`
This is also implemented for dense matrices, but it is pretty slow and
not really practical.
# Stochastic processes
Julia iterators for stochastic operators.
All subtypes of `StochasticProcess` contain at least one field, `dt`,
representing the time interval being discretized over.
The available `StochasticProcess`es are
- `BrownianProcess(dt)`: Brownian random walk.
The state of the iterator is the cumulative displacement of the random walk.
- `WhiteNoiseProcess(dt)` : White noise.
The value of this iterator is `randn()*dt`.
The state associated with this iterator is `nothing`.
- `StochasticAiryProcess(dt, beta)`: stochastic Airy process with real positive `beta`.
The value of this iterator in the limit of an infinite number of iterations
is known to follow the `beta`-Tracy-Widom law.
The state associated with this iteratior is a `SymTridiagonal` matrix whose
largest eigenvalue is the value of this process.
# Invariant ensembles
`InvariantEnsemble(str,n)` supports n x n unitary invariant ensemble
with distribution. This has been moved to separate package [InvariantEnsembles.jl](https://github.com/dlfivefifty/InvariantEnsembles.jl)
# References
- James Albrecht, Cy Chan, and Alan Edelman,
"Sturm Sequences and Random Eigenvalue Distributions",
*Foundations of Computational Mathematics*,
vol. 9 iss. 4 (2009), pp 461-483.
[[pdf]](www-math.mit.edu/~edelman/homepage/papers/sturm.pdf)
[[doi]](http://dx.doi.org/10.1007/s10208-008-9037-x)
- Ioana Dumitriu and Alan Edelman,
"Matrix Models for Beta Ensembles",
*Journal of Mathematical Physics*,
vol. 43 no. 11 (2002), pp. 5830-5547
[[doi]](http://dx.doi.org/doi: 10.1063/1.1507823)
[arXiv:math-ph/0206043](http://arxiv.org/abs/math-ph/0206043)
- Alan Edelman, Per-Olof Persson and Brian D Sutton,
"The fourfold way",
*Journal of Mathematical Physics*,
submitted (2013).
[[pdf]](http://www-math.mit.edu/~edelman/homepage/papers/ffw.pdf)
- Alan Edelman and Brian D. Sutton,
"The beta-Jacobi matrix model, the CS decomposition,
and generalized singular value problems",
*Foundations of Computational Mathematics*,
vol. 8 iss. 2 (2008), pp 259-285.
[[pdf]](http://www-math.mit.edu/~edelman/homepage/papers/betajacobi.pdf)
[[doi]](http://dx.doi.org/10.1007/s10208-006-0215-9)
- Peter Henrici,
*Applied and Computational Complex Analysis,
Volume I: Power Series---Integration---Conformal Mapping---Location of Zeros*,
Wiley-Interscience: New York, 1974
[[worldcat]](http://www.worldcat.org/oclc/746035)
- Frank Mezzadri,
"How to generate random matrices from the classical compact groups",
Notices of the AMS, vol. 54 (2007), pp592-604
[[arXiv]](http://arxiv.org/abs/math-ph/0609050)
| RandomMatrices | https://github.com/JuliaMath/RandomMatrices.jl.git |
|
[
"MIT"
] | 0.5.5 | 359d601ae45b05ea9a53d303dfbf477fcaca4195 | docs | 97 | This folder contains code snippets from the upcoming book "Random Eigenvalues" by Alan Edelman.
| RandomMatrices | https://github.com/JuliaMath/RandomMatrices.jl.git |
|
[
"MIT"
] | 0.9.1 | ed1a12069bdb85038eb736b2a3bde5d59093eb0b | code | 621 | #!/usr/bin/env -S julia --startup-file=no
function cleanup()
rm(joinpath(@__DIR__, "build"), force=true, recursive=true)
for (root, _, files) in walkdir(joinpath(@__DIR__, "src"))
for file in files
if endswith(file, ".md")
rm(joinpath(root, file))
end
end
end
end
cleanup()
using Pkg
Pkg.activate(@__DIR__)
Pkg.develop(PackageSpec(; path=dirname(@__DIR__)))
Pkg.instantiate()
using LiveServer
Base.exit_on_sigint(false)
try
servedocs(doc_env=true, foldername=@__DIR__)
finally
Pkg.rm(PackageSpec(; path=dirname(@__DIR__)))
cleanup()
end
| DataToolkitBase | https://github.com/tecosaur/DataToolkitBase.jl.git |
|
[
"MIT"
] | 0.9.1 | ed1a12069bdb85038eb736b2a3bde5d59093eb0b | code | 1905 | #!/usr/bin/env -S julia --startup-file=no
using Documenter
using DataToolkitBase
using Org
orgfiles = filter(f -> endswith(f, ".org"),
readdir(joinpath(@__DIR__, "src"), join=true))
let orgconverted = 0
html2utf8entity_dirty(text) = # Remove as soon as unnecesary
replace(text,
"…" => "…",
"—" => "—",
"—" => "–",
"­" => "-")
for (root, _, files) in walkdir(joinpath(@__DIR__, "src"))
orgfiles = joinpath.(root, filter(f -> endswith(f, ".org"), files))
for orgfile in orgfiles
mdfile = replace(orgfile, r"\.org$" => ".md")
read(orgfile, String) |>
c -> Org.parse(OrgDoc, c) |>
o -> sprint(markdown, o) |>
html2utf8entity_dirty |>
s -> replace(s, r"\.org]" => ".md]") |>
m -> string("```@meta\nEditURL=\"$(basename(orgfile))\"\n```\n\n", m) |>
m -> write(mdfile, m)
end
orgconverted += length(orgfiles)
end
@info "Converted $orgconverted files from .org to .md"
end
makedocs(;
modules=[DataToolkitBase],
format=Documenter.HTML(),
pages=[
"Introduction" => "index.md",
"Usage" => "usage.md",
"Data.toml" => "datatoml.md",
"REPL" => "repl.md",
"Extensions" => Any[
"Transformer backends" => "newtransformer.md",
"Packages" => "packages.md",
"Data Advice" => "advising.md",
],
"Internals" => "libinternal.md",
"Errors" => "errors.md",
],
sitename="DataToolkitBase.jl",
authors = "tecosaur and contributors: https://github.com/tecosaur/DataToolkitBase.jl/graphs/contributors",
warnonly = [:missing_docs],
)
deploydocs(;
repo="github.com/tecosaur/DataToolkitBase.jl",
devbranch = "main"
)
| DataToolkitBase | https://github.com/tecosaur/DataToolkitBase.jl.git |
|
[
"MIT"
] | 0.9.1 | ed1a12069bdb85038eb736b2a3bde5d59093eb0b | code | 230 | module AbstractTreesExt
using DataToolkitBase
using AbstractTrees
AbstractTrees.children(dataset::DataSet) =
DataToolkitBase.referenced_datasets(dataset)
AbstractTrees.printnode(io::IO, d::DataSet) = print(io, d.name)
end
| DataToolkitBase | https://github.com/tecosaur/DataToolkitBase.jl.git |
|
[
"MIT"
] | 0.9.1 | ed1a12069bdb85038eb736b2a3bde5d59093eb0b | code | 1165 | module GraphExt
using DataToolkitBase: DataCollection, DataSet, referenced_datasets, Identifier
using MetaGraphsNext
using MetaGraphsNext.Graphs
import MetaGraphsNext.MetaGraph
function MetaGraph(datasets::Vector{DataSet})
graph = MetaGraphsNext.MetaGraph(DiGraph(), label_type=String, vertex_data_type=DataSet)
labels = Dict{DataSet, String}()
function getlabel(ds::DataSet)
get!(labels, ds) do
replace(sprint(io -> show(io, MIME("text/plain"), Identifier(ds); collection=ds.collection)),
"■:" => "")
end
end
seen = Set{DataSet}()
queue = copy(datasets)
while !isempty(queue)
ds = popfirst!(queue)
ds in seen && continue
push!(seen, ds)
haskey(labels, ds) || add_vertex!(graph, getlabel(ds), ds)
deps = referenced_datasets(ds)
for dep in deps
haskey(labels, dep) || add_vertex!(graph, getlabel(dep), dep)
add_edge!(graph, labels[dep], labels[ds])
push!(queue, dep)
end
end
graph
end
MetaGraph(ds::DataSet) = MetaGraph([ds])
MetaGraph(dc::DataCollection) = MetaGraph(dc.datasets)
end
| DataToolkitBase | https://github.com/tecosaur/DataToolkitBase.jl.git |
|
[
"MIT"
] | 0.9.1 | ed1a12069bdb85038eb736b2a3bde5d59093eb0b | code | 1872 | module DataToolkitBase
using UUIDs, TOML, Dates
using PrecompileTools
using Compat
# For general usage
export loadcollection!, dataset
# For extension packages
export AbstractDataTransformer, DataStorage, DataLoader, DataWriter,
DataSet, DataCollection, QualifiedType, Identifier, FilePath, SmallDict,
LintItem, LintReport
export load, storage, getstorage, putstorage, save, getlayer, resolve, refine,
parse_ident, supportedtypes, typeify, create, createpriority, lint
export IdentifierException, UnresolveableIdentifier, AmbiguousIdentifier,
PackageException, UnregisteredPackage, MissingPackage,
DataOperationException, CollectionVersionMismatch, EmptyStackError,
ReadonlyCollection, TransformerError, UnsatisfyableTransformer,
OrphanDataSet, InvalidParameterType
export STACK, DATA_CONFIG_RESERVED_ATTRIBUTES
export @import, @addpkg, @dataplugin, @advise, @getparam
# For plugin packages
export PLUGINS, PLUGINS_DOCUMENTATION, DEFAULT_PLUGINS, Plugin,
fromspec, tospec, Advice, AdviceAmalgamation
export ReplCmd, REPL_CMDS, help, completions, allcompletions,
prompt, prompt_char, confirm_yn, peelword
include("model/types.jl")
include("model/globals.jl")
include("model/utils.jl")
include("model/advice.jl")
include("model/errors.jl")
include("model/smalldict.jl")
include("model/qualifiedtype.jl")
include("model/identification.jl")
include("model/parameters.jl")
include("model/stack.jl")
include("model/parser.jl")
include("model/writer.jl")
include("model/usepkg.jl")
include("model/dataplugin.jl")
include("interaction/externals.jl")
include("interaction/display.jl")
include("interaction/manipulation.jl")
include("interaction/repl.jl")
include("interaction/lint.jl")
include("precompile.jl")
function add_datasets! end # For `ext/AbstractTreesExt.jl`
function __init__()
isinteractive() && init_repl()
end
end
| DataToolkitBase | https://github.com/tecosaur/DataToolkitBase.jl.git |
|
[
"MIT"
] | 0.9.1 | ed1a12069bdb85038eb736b2a3bde5d59093eb0b | code | 2000 | @setup_workload begin
datatoml = """
data_config_version = 0
uuid = "84068d44-24db-4e28-b693-58d2e1f59d05"
name = "precompile"
plugins = []
[[dataset]]
uuid = "d9826666-5049-4051-8d2e-fe306c20802c"
self = "$(DATASET_REFERENCE_WRAPPER[1])dataset$(DATASET_REFERENCE_WRAPPER[2])"
other = {a = [1, 2], b = [3, 4]}
[[dataset.storage]]
driver = "raw"
value = 3
type = "Int"
[[dataset.loader]]
driver = "passthrough"
type = "Int"
"""
# function getstorage(storage::DataStorage{:raw}, T::Type)
# get(storage, "value", nothing)::Union{T, Nothing}
# end
# function load(::DataLoader{:passthrough}, from::T, ::Type{T}) where {T <: Any}
# from
# end
if VERSION >= v"1.9"
Base.active_repl =
REPL.LineEditREPL(REPL.Terminals.TTYTerminal("", stdin, stdout, stderr), true)
end
@compile_workload begin
loadcollection!(IOBuffer(datatoml))
write(devnull, STACK[1])
dataset("dataset")
# read(dataset("dataset"))
sprint(show, STACK[1], context = :color => true)
sprint(show, dataset("dataset"), context = :color => true)
lint(STACK[1])
@advise STACK[1] sum(1:3)
# REPL
if VERSION >= v"1.9"
init_repl()
redirect_stdio(stdout=devnull, stderr=devnull) do
toplevel_execute_repl_cmd("?")
toplevel_execute_repl_cmd("?help")
toplevel_execute_repl_cmd("help help")
toplevel_execute_repl_cmd("help :")
end
end
complete_repl_cmd("help ")
# Other stuff
get(dataset("dataset"), "self")
get(dataset("dataset"), "other")
end
# Base.delete_method(first(methods(getstorage, Tuple{DataStorage{:raw}, Type}).ms))
# Base.delete_method(first(methods(load, Tuple{DataLoader{:passthrough}, Any, Any}).ms))
empty!(STACK)
end
| DataToolkitBase | https://github.com/tecosaur/DataToolkitBase.jl.git |
|
[
"MIT"
] | 0.9.1 | ed1a12069bdb85038eb736b2a3bde5d59093eb0b | code | 7742 | """
displaytable(rows::Vector{<:Vector};
spacing::Integer=2, maxwidth::Int=80)
Return a `vector` of strings, formed from each row in `rows`.
Each string is of the same `displaywidth`, and individual values
are separated by `spacing` spaces. Values are truncated if necessary
to ensure the no row is no wider than `maxwidth`.
"""
function displaytable(rows::Vector{<:Vector};
spacing::Integer=2, maxwidth::Int=80)
column_widths = min.(maxwidth,
maximum.(textwidth.(string.(getindex.(rows, i)))
for i in 1:length(rows[1])))
if sum(column_widths) > maxwidth
# Resize columns according to the square root of their width
rootwidths = sqrt.(column_widths)
table_width = sum(column_widths) + spacing * length(column_widths)
rootcorrection = sum(column_widths) / sum(sqrt, column_widths)
rootwidths = rootcorrection .* sqrt.(column_widths) .* maxwidth/table_width
# Look for any expanded columns, and redistribute their excess space
# proportionally.
overwides = column_widths .< rootwidths
if any(overwides)
gap = sum((rootwidths .- column_widths)[overwides])
rootwidths[overwides] = column_widths[overwides]
@. rootwidths[.!overwides] += gap * rootwidths[.!overwides]/sum(rootwidths[.!overwides])
end
column_widths = max.(1, floor.(Int, rootwidths))
end
makelen(content::String, len::Int) =
if length(content) <= len
rpad(content, len)
else
string(content[1:len-1], '…')
end
makelen(content::Any, len::Int) = makelen(string(content), len)
map(rows) do row
join([makelen(col, width) for (col, width) in zip(row, column_widths)],
' '^spacing)
end
end
"""
displaytable(headers::Vector, rows::Vector{<:Vector};
spacing::Integer=2, maxwidth::Int=80)
Prepend the `displaytable` for `rows` with a header row given by `headers`.
"""
function displaytable(headers::Vector, rows::Vector{<:Vector};
spacing::Integer=2, maxwidth::Int=80)
rows = displaytable(vcat([headers], rows); spacing, maxwidth)
rule = '─'^length(rows[1])
vcat("\e[1m" * rows[1] * "\e[0m", rule, rows[2:end])
end
function Base.show(io::IO, ::MIME"text/plain", dsi::Identifier;
collection::Union{DataCollection, Nothing}=nothing)
printstyled(io, if isnothing(dsi.collection)
'□'
elseif !isempty(STACK) && ((dsi.collection isa UUID && dsi.collection == first(STACK).uuid) ||
(dsi.collection isa AbstractString && dsi.collection == first(STACK).name))
'■'
else
dsi.collection
end,
':', color=:magenta)
if isnothing(collection)
print(io, dsi.dataset)
else
dname = string(dsi.dataset)
nameonly = Identifier(nothing, dsi.dataset, nothing, dsi.parameters)
namestr = @advise collection string(nameonly)
if startswith(namestr, dname)
print(io, dsi.dataset)
printstyled(io, namestr[nextind(namestr, length(dname)):end],
color=:cyan)
else
print(io, namestr)
end
end
if !isnothing(dsi.type)
printstyled(io, "::", string(dsi.type), color=:yellow)
end
end
function Base.show(io::IO, adt::AbstractDataTransformer)
adtt = typeof(adt)
get(io, :omittype, false) || print(io, nameof(adtt), '{')
printstyled(io, first(adtt.parameters), color=:green)
get(io, :omittype, false) || print(io, '}')
print(io, "(")
for qtype in adt.type
type = typeify(qtype, mod=adt.dataset.collection.mod)
if !isnothing(type)
printstyled(io, type, color=:yellow)
else
printstyled(io, qtype.name, color=:yellow)
if !isempty(qtype.parameters)
printstyled(io, '{', join(string.(qtype.parameters), ','), '}',
color=:yellow)
end
end
qtype === last(adt.type) || print(io, ", ")
end
print(io, ")")
end
function Base.show(io::IO, ::MIME"text/plain", ::Advice{F, C}) where {F, C}
print(io, "Advice{$F, $C}")
end
function Base.show(io::IO, p::Plugin)
print(io, "Plugin(")
show(io, p.name)
print(io, ", [")
context(::Advice{F, C}) where {F, C} = (F, C)
print(io, join(string.(context.(p.advisors)), ", "))
print(io, "])")
end
function Base.show(io::IO, dta::AdviceAmalgamation)
get(io, :omittype, false) || print(io, "AdviceAmalgamation(")
for plugin in dta.plugins_wanted
if plugin in dta.plugins_used
print(io, plugin, ' ')
printstyled(io, '✔', color = :green)
else
printstyled(io, plugin, ' ', color = :light_black)
printstyled(io, '✘', color = :red)
end
plugin === last(dta.plugins_wanted) || print(io, ", ")
end
get(io, :omittype, false) || print(io, ")")
end
function Base.show(io::IO, dataset::DataSet)
if get(io, :compact, false)
printstyled(io, dataset.name, color=:blue)
print(io, " (")
qtypes = vcat(getfield.(dataset.loaders, :type)...) |> unique
for qtype in qtypes
type = typeify(qtype, mod=dataset.collection.mod)
if !isnothing(type)
printstyled(io, type, color=:yellow)
else
printstyled(io, qtype.name, color=:yellow)
if !isempty(qtype.parameters)
printstyled(io, '{', join(string.(qtype.parameters), ','), '}',
color=:yellow)
end
end
qtype === last(qtypes) || print(io, ", ")
end
print(io, ')')
return
end
print(io, "DataSet ")
if !isnothing(dataset.collection.name)
color = if length(STACK) > 0 && dataset.collection === first(STACK)
:light_black
else
:magenta
end
printstyled(io, dataset.collection.name; color)
printstyled(io, ':', color=:light_black)
end
printstyled(io, dataset.name, bold=true, color=:blue)
for (label, field) in [("Storage", :storage),
("Loaders", :loaders),
("Writers", :writers)]
entries = getfield(dataset, field)
if !isempty(entries)
print(io, "\n ", label, ": ")
for entry in entries
show(IOContext(io, :compact => true, :omittype => true), entry)
entry === last(entries) || print(io, ", ")
end
end
end
end
function Base.show(io::IO, datacollection::DataCollection)
if get(io, :compact, false)
printstyled(io, datacollection.name, color=:magenta)
return
end
print(io, "DataCollection:")
if !isnothing(datacollection.name)
printstyled(io, ' ', datacollection.name, color=:magenta)
end
if iswritable(datacollection)
printstyled(io, " (writable)", color=:light_black)
elseif get(datacollection, "locked", false) === true
printstyled(io, " (locked)", color=:light_black)
end
if !isempty(datacollection.plugins)
print(io, "\n Plugins: ")
show(IOContext(io, :compact => true, :omittype => true),
datacollection.advise)
end
print(io, "\n Data sets:")
for dataset in sort(datacollection.datasets, by = d -> natkeygen(d.name))
print(io, "\n ")
show(IOContext(io, :compact => true), dataset)
end
end
| DataToolkitBase | https://github.com/tecosaur/DataToolkitBase.jl.git |
|
[
"MIT"
] | 0.9.1 | ed1a12069bdb85038eb736b2a3bde5d59093eb0b | code | 17683 | """
loadcollection!(source::Union{<:AbstractString, <:IO}, mod::Module=Base.Main;
soft::Bool=false, index::Int=1)
Load a data collection from `source` and add it to the data stack at `index`.
`source` must be accepted by `read(source, DataCollection)`.
`mod` should be set to the Module within which `loadcollection!` is being
invoked. This is important when code is run by the collection. As such,
it is usually appropriate to call:
```julia
loadcollection!(source, @__MODULE__; soft)
```
When `soft` is set, should an data collection already exist with the same UUID,
nothing will be done and `nothing` will be returned.
"""
function loadcollection!(source::Union{<:AbstractString, <:IO}, mod::Module=Base.Main;
soft::Bool=false, index::Int=1)
uuid = UUID(get(if source isa AbstractString
open(source, "r") do io TOML.parse(io) end
else
mark(source)
t = TOML.parse(source)
reset(source)
t
end, "uuid", uuid4()))
existingpos = findfirst(c -> c.uuid == uuid, STACK)
if !isnothing(existingpos)
if soft
return nothing
else
@warn "Data collection already existed on stack, replacing."
deleteat!(STACK, existingpos)
end
end
collection = read(source, DataCollection; mod)
nameconflicts = filter(c -> c.name == collection.name, STACK)
if !isempty(nameconflicts)
printstyled(stderr, "!", color=:yellow, bold=true)
print(stderr, " the data collection ")
printstyled(stderr, collection.name, color=:green)
print(stderr, " (UUID: ")
printstyled(stderr, collection.uuid, color=:yellow)
print(stderr, ")\n conflicts with datasets already loaded with the exact same name:\n")
for conflict in nameconflicts
print(stderr, " • ")
printstyled(stderr, conflict.uuid, '\n', color=:yellow)
end
println(stderr, " You must now refer to these datasets by their UUID to be unambiguous.")
end
insert!(STACK, clamp(index, 1, length(STACK)+1), collection)
collection
end
"""
dataset([collection::DataCollection], identstr::AbstractString, [parameters::Dict{String, Any}])
dataset([collection::DataCollection], identstr::AbstractString, [parameters::Pair{String, Any}...])
Return the data set identified by `identstr`, optionally specifying the `collection`
the data set should be found in and any `parameters` that apply.
"""
dataset(identstr::AbstractString) = resolve(identstr; resolvetype=false)::DataSet
dataset(identstr::AbstractString, parameters::SmallDict{String, Any}) =
resolve(identstr, parameters; resolvetype=false)::DataSet
dataset(identstr::AbstractString, parameters::Dict{String, Any}) =
dataset(identstr, smallify(parameters))
function dataset(identstr::AbstractString, kv::Pair{<:AbstractString, <:Any}, kvs::Pair{<:AbstractString, <:Any}...)
parameters = SmallDict{String, Any}()
parameters[String(first(kv))] = last(kv)
for (key, value) in kvs
parameters[String(key)] = value
end
dataset(identstr, parameters)
end
dataset(collection::DataCollection, identstr::AbstractString) =
resolve(collection, @advise collection parse_ident(identstr);
resolvetype=false)::DataSet
function dataset(collection::DataCollection, identstr::AbstractString, parameters::Dict{String, Any})
ident = @advise collection parse_ident(identstr)
resolve(collection, Identifier(ident, parameters); resolvetype=false)::DataSet
end
"""
read(filename::AbstractString, DataCollection; writer::Union{Function, Nothing})
Read the entire contents of a file as a `DataCollection`.
The default value of writer is `self -> write(filename, self)`.
"""
Base.read(f::AbstractString, ::Type{DataCollection}; mod::Module=Base.Main) =
open(f, "r") do io read(io, DataCollection; path=abspath(f), mod) end
"""
read(io::IO, DataCollection; path::Union{String, Nothing}=nothing, mod::Module=Base.Main)
Read the entirety of `io`, as a `DataCollection`.
"""
Base.read(io::IO, ::Type{DataCollection};
path::Union{String, Nothing}=nothing, mod::Module=Base.Main) =
DataCollection(TOML.parse(io); path, mod)
"""
read(dataset::DataSet, as::Type)
read(dataset::DataSet) # as default type
Obtain information from `dataset` in the form of `as`, with the appropriate
loader and storage provider automatically determined.
This executes this component of the overall data flow:
```
╭────loader─────╮
╵ ▼
Storage ◀────▶ Data Information
```
The loader and storage provider are selected by identifying the highest priority
loader that can be satisfied by a storage provider. What this looks like in practice
is illustrated in the diagram below.
```
read(dataset, Matrix) ⟶ ::Matrix ◀╮
╭───╯ ╰────────────▷┬───╯
╔═════╸dataset╺══════════════════╗ │
║ STORAGE LOADERS ║ │
║ (⟶ File)─┬─╮ (File ⟶ String) ║ │
║ (⟶ IO) ┊ ╰─(File ⟶ Matrix)─┬─╫──╯
║ (⟶ File)┄╯ (IO ⟶ String) ┊ ║
║ (IO ⟶ Matrix)╌╌╌╯ ║
╚════════════════════════════════╝
─ the load path used
┄ an option not taken
TODO explain further
```
"""
function Base.read(dataset::DataSet, as::Type)
@advise _read(dataset, as)
end
function Base.read(dataset::DataSet)
as = nothing
for qtype in getproperty.(dataset.loaders, :type) |> Iterators.flatten
as = typeify(qtype, mod=dataset.collection.mod)
isnothing(as) || break
end
if isnothing(as)
possiblepkgs = getproperty.(getproperty.(dataset.loaders, :type) |> Iterators.flatten, :root)
helpfulextra = if isempty(possiblepkgs)
"There are no known types (from any packages) that this data set can be loaded as."
else
"You may have better luck with one of the following packages loaded: $(join(sort(unique(possiblepkgs)), ", "))"
end
throw(TransformerError(
"Data set $(sprint(show, dataset.name)) could not be loaded in any form.\n $helpfulextra"))
end
@advise _read(dataset, as)
end
"""
_read(dataset::DataSet, as::Type)
The advisible implementation of `read(dataset::DataSet, as::Type)`
This is essentially an excersise in useful indirection.
"""
function _read(dataset::DataSet, as::Type)
all_load_fn_sigs = map(fn -> Base.unwrap_unionall(fn.sig),
methods(load, Tuple{DataLoader, Any, Any}))
qtype = QualifiedType(as)
# Filter to loaders which are declared in `dataset` as supporting `as`.
# These will have already been ordered by priority during parsing.
potential_loaders =
filter(loader -> any(st -> ⊆(st, qtype, mod=dataset.collection.mod), loader.type),
dataset.loaders)
# If no matching loaders could be found, be a bit generous and /just try/
# filtering to the specified `as` type. If this works, it's probably what's
# wanted, and incompatibility should be caught by later stages.
if isempty(potential_loaders)
# Here I use `!isempty(methods(...))` which may seem strange, given
# `hasmethod` exists. While in theory it would be reasonable to expect
# that `hasmethod(f, Tuple{A, Union{}, B})` would return true if a method
# with the signature `Tuple{A, <:Any, B}` existed, this is unfortunately
# not the case in practice, and so we must resort to `methods`.
potential_loaders =
filter(loader -> !isempty(methods(load, Tuple{typeof(loader), <:Any, Type{as}})),
dataset.loaders)
end
for loader in potential_loaders
load_fn_sigs = filter(fnsig -> loader isa fnsig.types[2], all_load_fn_sigs)
# Find the highest priority load function that can be satisfied,
# by going through each of the storage backends one at a time:
# looking for the first that is (a) compatible with a load function,
# and (b) available (checked via `!isnothing`).
for storage in dataset.storage
for load_fn_sig in load_fn_sigs
supported_storage_types = Vector{Type}(
filter(!isnothing, typeify.(storage.type)))
valid_storage_types =
filter(stype -> let accept = load_fn_sig.types[3]
if accept isa TypeVar
# We can't really handle complex `TypeVar` situations,
# but we'll give the very most basic a shot, and cross
# our fingers with the rest.
if load_fn_sig.types[4] == Type{load_fn_sig.types[3]}
stype <: as
else
accept.lb <: stype <: accept.ub
end
else # must be a Type
stype <: accept
end
end,
supported_storage_types)
for storage_type in valid_storage_types
datahandle = open(dataset, storage_type; write = false)
if !isnothing(datahandle)
result = @advise dataset load(loader, datahandle, as)
if !isnothing(result)
return result
end
end
end
end
end
# Check for a "null storage" option. This is to enable loaders
# like DataToolkitCommon's `:julia` which can construct information
# without an explicit storage backend.
for load_fn_sig in load_fn_sigs
if load_fn_sig.types[3] == Nothing
return @advise dataset load(loader, nothing, as)
end
end
end
if length(potential_loaders) == 0
throw(UnsatisfyableTransformer(dataset, DataLoader, [qtype]))
else
loadertypes = map(
f -> QualifiedType( # Repeat the logic from `valid_storage_types`
if f.types[3] isa TypeVar
if f.types[4] == Type{f.types[3]}
as
else
f.types[3].ub
end
else
f.types[3]
end),
filter(f -> any(l -> l isa f.types[2], potential_loaders),
all_load_fn_sigs))
throw(UnsatisfyableTransformer(dataset, DataStorage, loadertypes))
end
end
function Base.read(ident::Identifier, as::Type)
dataset = resolve(ident, resolvetype=false)
read(dataset, as)
end
function Base.read(ident::Identifier)
isnothing(ident.type) &&
throw(ArgumentError("Cannot read from DataSet Identifier without type information."))
mod = getlayer(ident.collection).mod
read(ident, typeify(ident.type; mod))
end
"""
load(loader::DataLoader{driver}, source::Any, as::Type)
Using a certain `loader`, obtain information in the form of
`as` from the data given by `source`.
This fulfils this component of the overall data flow:
```
╭────loader─────╮
╵ ▼
Data Information
```
When the loader produces `nothing` this is taken to indicate that it was unable
to load the data for some reason, and that another loader should be tried if
possible. This can be considered a soft failure. Any other value is considered
valid information.
"""
function load end
load((loader, source, as)::Tuple{DataLoader, Any, Type}) =
load(loader, source, as)
"""
open(dataset::DataSet, as::Type; write::Bool=false)
Obtain the data of `dataset` in the form of `as`, with the appropriate storage
provider automatically selected.
A `write` flag is also provided, to help the driver pick a more appropriate form
of `as`.
This executes this component of the overall data flow:
```
╭────loader─────╮
╵ ▼
Storage ◀────▶ Data Information
```
"""
function Base.open(data::DataSet, as::Type; write::Bool=false)
for storage_provider in data.storage
if any(t -> ⊆(as, t, mod=data.collection.mod), storage_provider.type)
result = @advise data storage(storage_provider, as; write)
if !isnothing(result)
return result
end
end
end
end
# Base.open(data::DataSet, qas::QualifiedType; write::Bool) =
# open(typeify(qas, mod=data.collection.mod), data; write)
"""
storage(storer::DataStorage, as::Type; write::Bool=false)
Fetch a `storer` in form `as`, appropiate for reading from or writing to
(depending on `write`).
By default, this just calls `getstorage` or `putstorage` (when `write=true`).
This executes this component of the overall data flow:
```
Storage ◀────▶ Data
```
"""
function storage(storer::DataStorage, as::Type; write::Bool=false)
if write
putstorage(storer, as)
else
getstorage(storer, as)
end
end
getstorage(::DataStorage, ::Any) = nothing
putstorage(::DataStorage, ::Any) = nothing
"""
write(dataset::DataSet, info::Any)
TODO write docstring
"""
function Base.write(dataset::DataSet, info::T) where {T}
all_write_fn_sigs = map(fn -> Base.unwrap_unionall(fn.sig),
methods(save, Tuple{DataWriter, Any, Any}))
qtype = QualifiedType(T)
# Filter to loaders which are declared in `dataset` as supporting `as`.
# These will have already been orderd by priority during parsing.
potential_writers =
filter(writer -> any(st -> ⊆(qtype, st, mod=dataset.collection.mod), writer.type),
dataset.writers)
for writer in potential_writers
write_fn_sigs = filter(fnsig -> writer isa fnsig.types[2], all_write_fn_sigs)
# Find the highest priority save function that can be satisfied,
# by going through each of the storage backends one at a time:
# looking for the first that is (a) compatible with a save function,
# and (b) available (checked via `!isnothing`).
for storage in dataset.storage
for write_fn_sig in write_fn_sigs
supported_storage_types = Vector{Type}(
filter(!isnothing, typeify.(storage.type)))
valid_storage_types =
filter(stype -> stype <: write_fn_sig.types[3],
supported_storage_types)
for storage_type in valid_storage_types
datahandle = open(dataset, storage_type; write = true)
if !isnothing(datahandle)
res = @advise dataset save(writer, datahandle, info)
if res isa IO && isopen(res)
close(res)
end
return nothing
end
end
end
end
end
if length(potential_writers) == 0
throw(TransformerError("There are no writers for $(sprint(show, dataset.name)) that can work with $T"))
else
TransformerError("There are no available storage backends for $(sprint(show, dataset.name)) that can be used by a writer for $T.")
end
end
"""
save(writer::Datasaveer{driver}, destination::Any, information::Any)
Using a certain `writer`, save the `information` to the `destination`.
This fulfils this component of the overall data flow:
```
Data Information
▲ ╷
╰────writer─────╯
```
"""
function save end
save((writer, dest, info)::Tuple{DataWriter, Any, Any}) =
save(writer, dest, info)
# For use during parsing, see `fromspec` in `model/parser.jl`.
function extracttypes(T::Type)
splitunions(T::Type) = if T isa Union Base.uniontypes(T) else (T,) end
if T == Type || T == Any
(Any,)
elseif T isa UnionAll
first(Base.unwrap_unionall(T).parameters).ub |> splitunions
elseif T isa Union
first.(getproperty.(Base.uniontypes(T), :parameters))
else
T1 = first(T.parameters)
if T1 isa TypeVar T1.ub else T1 end |> splitunions
end
end
const genericstore = first(methods(storage, Tuple{DataStorage{Any}, Any}))
const genericstoreget = first(methods(getstorage, Tuple{DataStorage{Any}, Any}))
const genericstoreput = first(methods(putstorage, Tuple{DataStorage{Any}, Any}))
supportedtypes(L::Type{<:DataLoader}, T::Type=Any)::Vector{QualifiedType} =
map(fn -> extracttypes(Base.unwrap_unionall(fn.sig).types[4]),
methods(load, Tuple{L, T, Any})) |>
Iterators.flatten .|> QualifiedType |> unique |> reverse
supportedtypes(W::Type{<:DataWriter}, T::Type=Any)::Vector{QualifiedType} =
map(fn -> QualifiedType(Base.unwrap_unionall(fn.sig).types[4]),
methods(save, Tuple{W, T, Any})) |> unique |> reverse
supportedtypes(S::Type{<:DataStorage})::Vector{QualifiedType} =
map(fn -> extracttypes(Base.unwrap_unionall(fn.sig).types[3]),
let ms = filter(m -> m != genericstore, methods(storage, Tuple{S, Any}))
if isempty(ms)
vcat(filter(m -> m != genericstoreget,
methods(getstorage, Tuple{S, Any})),
filter(m -> m != genericstoreput,
methods(putstorage, Tuple{S, Any})))
else ms end
end) |> Iterators.flatten .|> QualifiedType |> unique |> reverse
| DataToolkitBase | https://github.com/tecosaur/DataToolkitBase.jl.git |
|
[
"MIT"
] | 0.9.1 | ed1a12069bdb85038eb736b2a3bde5d59093eb0b | code | 10676 | """
Representation of a lint item.
# Constructors
```julia
LintItem(source, severity::Union{Int, Symbol}, id::Symbol, message::String,
fixer::Union{Function, Nothing}=nothing, autoapply::Bool=false)
```
`source` is the object that the lint applies to.
`severity` should be one of the following values:
- `0` or `:debug`, for messages that may assist with debugging problems that may
be associated with particular configuration.
- `1` or `:info`, for informational messages understandable to end-users.
- `2` or `:warning`, for potentially harmful situations.
- `3` or `:error`, for severe issues that will prevent normal functioning.
`id` is a symbol representing the type of lint (e.g. `:unknown_driver`)
`message` is a message, intelligible to the end-user, describing the particular
nature of the issue with respect to `source`. It should be as specific as possible.
`fixer` can be set to a function which modifies `source` to resolve the issue.
If `autoapply` is set to `true` then `fixer` will be called spontaneously.
The function should return `true` or `false` to indicate whether it was able
to successfully fix the issue.
As a general rule, fixers that do or might require user input should *not* be
run automatically, and fixers that can run without any user input and
always "do the right thing" should be run automatically.
# Examples
TODO
# Structure
```julia
struct LintItem{S}
source ::S
severity ::UInt8
id ::Symbol
message ::String
fixer ::Union{Function, Nothing}
autoapply ::Bool
end
```
"""
struct LintItem{S}
source::S
severity::UInt8
id::Symbol
message::String
fixer::Union{Function, Nothing}
autoapply::Bool
end
LintItem(source, severity::Symbol, id::Symbol, message::String,
fixer::Union{Function, Nothing}=nothing, autoapply::Bool=false) =
LintItem(source, LINT_SEVERITY_MAPPING[severity], id,
message, fixer, autoapply)
"""
lint(obj::T)
Call all of the relevant linter functions on `obj`. More specifically,
the method table is searched for `lint(obj::T, ::Val{:linter_id})` methods
(where `:linter_id` is a stand-in for the actual IDs used), and each specific
lint function is invoked and the results combined.
!!! note
Each specific linter function should return a vector of relevant
`LintItem`s, i.e.
```julia
lint(obj::T, ::Val{:linter_id}) -> Union{Vector{LintItem{T}}, LintItem{T}, Nothing}
```
See the documentation on `LintItem` for more information on how it should be
constructed.
"""
function lint(obj::T) where {T <: Union{DataCollection, DataSet, <:AbstractDataTransformer}}
linters = methods(lint, Tuple{T, Val}).ms
@advise lint(obj, linters)
end
function lint(obj::T, linters::Vector{Method}) where {T}
lintiter(l::Vector{LintItem}) = l
lintiter(l::LintItem) = (l,)
lintiter(::Nothing) = ()
issues = Iterators.map(linters) do linter
func = first(linter.sig.parameters).instance
val = last(linter.sig.parameters)
invoke(func, Tuple{T, val}, obj, val()) |> lintiter
end |> Iterators.flatten |> collect
sort(issues, by=i -> i.severity)
end
struct LintReport
collection::DataCollection
results::Vector{LintItem}
partial::Bool
end
function LintReport(collection::DataCollection)
results = Vector{Vector{LintItem}}()
push!(results, lint(collection))
for dataset in collection.datasets
push!(results, lint(dataset))
for adtfield in (:storage, :loaders, :writers)
for adt in getfield(dataset, adtfield)
push!(results, lint(adt))
end
end
end
LintReport(collection, Vector{LintItem}(Iterators.flatten(results) |> collect), false)
end
function LintReport(dataset::DataSet)
results = Vector{Vector{LintItem}}()
push!(results, lint(dataset))
for adtfield in (:storage, :loaders, :writers)
for adt in getfield(dataset, adtfield)
push!(results, lint(adt))
end
end
LintReport(dataset.collection,
Vector{LintItem}(Iterators.flatten(results) |> collect),
true)
end
function Base.show(io::IO, report::LintReport)
printstyled(io, ifelse(report.partial, "Partial lint results for '", "Lint results for '"),
report.collection.name, "' collection",
color=:blue, bold=true)
printstyled(io, " ", report.collection.uuid, color=:light_black)
if isempty(report.results)
printstyled("\n ✓ No issues found", color=:green)
end
lastsource::Any = nothing
objinfo(::DataCollection) = nothing
function objinfo(d::DataSet)
printstyled(io, "\n• ", d.name, color=:blue, bold=true)
printstyled(io, " ", d.uuid, color=:light_black)
end
function objinfo(a::A) where {A <: AbstractDataTransformer}
if lastsource isa DataSet
lastsource == a.dataset
elseif lastsource isa AbstractDataTransformer
lastsource.dataset == a.dataset
else
false
end || objinfo(a.dataset)
printstyled("\n ‣ ", first(A.parameters), ' ',
join(lowercase.(split(string(nameof(A)), r"(?=[A-Z])")), ' '),
color=:blue, bold=true)
end
indentlevel(::DataCollection) = 0
indentlevel(::DataSet) = 2
indentlevel(::AbstractDataTransformer) = 4
for (i, lintitem) in enumerate(report.results)
if lintitem.source !== lastsource
objinfo(lintitem.source)
lastsource = lintitem.source
end
let (color, label) = LINT_SEVERITY_MESSAGES[lintitem.severity]
printstyled(io, '\n', ' '^indentlevel(lintitem.source), label,
'[', i, ']', ':'; color)
end
first, rest... = split(lintitem.message, '\n')
print(io, ' ', first)
for line in rest
print(io, '\n', ' '^indentlevel(lintitem.source), line)
end
end
if length(report.results) > 12
print("\n\n")
printstyled(length(report.results), color=:light_white),
print(" issues identified:")
for category in (:error, :warning, :suggestion, :info, :debug)
catcode = LINT_SEVERITY_MAPPING[category]
ncat = sum(r -> r.severity == catcode, report.results)
if ncat > 0
printstyled("\n • ", color=:blue)
printstyled(ncat, color=:light_white)
printstyled(category, ifelse(ncat == 1, "", "s"),
color=LINT_SEVERITY_MESSAGES[catcode])
end
end
end
end
"""
lintfix(report::LintReport)
Attempt to fix as many issues raised in `report` as possible.
"""
function lintfix(report::LintReport, manualfix::Bool=false)
autofixed = Vector{Tuple{Int, LintItem, Bool}}()
fixprompt = Vector{Tuple{Int, LintItem}}()
# Auto-apply fixes
for (i, lintitem) in enumerate(report.results)
isnothing(lintitem.fixer) && continue
if lintitem.autoapply
push!(autofixed, (i, lintitem, lintitem.fixer(lintitem)))
else
push!(fixprompt, (i, lintitem))
end
end
if !isempty(autofixed)
print("Automatically fixed ")
printstyled(sum(last.(autofixed)), color=:light_white)
print(ifelse(sum(last.(autofixed)) == 1, " issue: ", " issues: "))
for fixresult in filter(last, autofixed)
i, lintitem, _ = fixresult
printstyled(i, color=first(LINT_SEVERITY_MESSAGES[lintitem.severity]))
fixresult === last(autofixed) || print(", ")
end
print(".\n")
if !all(last, autofixed)
print("Failed to automatically fix ", sum(.!last.(autofixed)), " issues: ")
printstyled(sum(.!last.(autofixed)), color=:light_white)
print(ifelse(sum(.!last.(autofixed)) == 1, "issue: ", " issues: "))
for fixresult in filter(last, autofixed)
i, lintitem, _ = fixresult
printstyled(i, color=first(LINT_SEVERITY_MESSAGES[lintitem.severity]))
fixresult === last(autofixed) || print(", ")
end
print('\n')
end
end
# Manual fixes
if !isempty(fixprompt) && (isinteractive() || manualfix)
printstyled(length(fixprompt), color=:light_white)
print(ifelse(length(fixprompt) == 1, " issue (", " issues ("))
for fixitem in fixprompt
i, lintitem = fixitem
printstyled(i, color=first(LINT_SEVERITY_MESSAGES[lintitem.severity]))
fixitem === last(fixprompt) || print(", ")
end
print(") can be manually fixed.\n")
if manualfix || isinteractive() &&
confirm_yn("Would you like to try?", true)
lastsource::Any = nothing
objinfo(c::DataCollection) =
printstyled("• ", c.name, '\n', color=:blue, bold=true)
function objinfo(d::DataSet)
printstyled("• ", d.name, color=:blue, bold=true)
printstyled(" ", d.uuid, "\n", color=:light_black)
end
objinfo(a::A) where {A <: AbstractDataTransformer} =
printstyled("• ", first(A.parameters), ' ',
join(lowercase.(split(string(nameof(A)), r"(?=[A-Z])")), ' '),
" for ", a.dataset.name, '\n', color=:blue, bold=true)
objinfo(::DataLoader{driver}) where {driver} =
printstyled("• ", driver, " loader\n", color=:blue, bold=true)
objinfo(::DataWriter{driver}) where {driver} =
printstyled("• ", driver, " writer\n", color=:blue, bold=true)
for (i, lintitem) in fixprompt
if lintitem.source !== lastsource
objinfo(lintitem.source)
lastsource = lintitem.source
end
printstyled(" [", i, "]: ", bold=true,
color=first(LINT_SEVERITY_MESSAGES[lintitem.severity]))
print(first(split(lintitem.message, '\n')), '\n')
try
lintitem.fixer(lintitem)
catch e
if e isa InterruptException
printstyled("!", color=:red, bold=true)
print(" Aborted\n")
else
rethrow()
end
end
end
write(report.collection)
end
end
if !isempty(autofixed)
write(report.collection)
end
nothing
end
| DataToolkitBase | https://github.com/tecosaur/DataToolkitBase.jl.git |
|
[
"MIT"
] | 0.9.1 | ed1a12069bdb85038eb736b2a3bde5d59093eb0b | code | 23757 | # ------------------
# Initialisation
# ------------------
"""
init(name::Union{AbstractString, Missing},
path::Union{AbstractString, Nothing};
uuid::UUID=uuid4(), plugins::Vector{String}=DEFAULT_PLUGINS,
write::Bool=true, addtostack::Bool=true, quiet::Bool=false)
Create a new data collection.
This can be an in-memory data collection, when `path` is set to `nothing`, or a
collection which corresponds to a Data TOML file, in which case `path` should be
set to either a path to a .toml file or a directory in which a Data.toml file
should be placed.
When `path` is a string and `write` is set, the data collection file will be
immediately written, overwriting any existing file at the path.
When `addtostack` is set, the data collection will also be added to the top of
the data collection stack.
Unless `quiet` is set, a message will be send to stderr reporting successful
creating of the data collection file.
### Example
```julia-repl
julia> init("test", "/tmp/test/Data.toml")
```
"""
function init(name::Union{AbstractString, Missing},
path::Union{AbstractString, Nothing};
uuid::UUID=uuid4(), plugins::Vector{String}=DEFAULT_PLUGINS,
write::Bool=true, addtostack::Bool=true, quiet::Bool=false)
if !endswith(path, ".toml")
path = joinpath(path, "Data.toml")
end
if ismissing(name)
name = if !isnothing(Base.active_project(false))
Base.active_project(false) |> dirname |> basename
else
something(path, string(gensym("unnamed"))[3:end]) |>
dirname |> basename
end
end
newcollection = DataCollection(
LATEST_DATA_CONFIG_VERSION, name, uuid,
plugins, Dict{String, Any}(), DataSet[],
path, AdviceAmalgamation(plugins),
Main)
newcollection = @advise init(newcollection)
!isnothing(path) && write && Base.write(newcollection)
addtostack && pushfirst!(STACK, newcollection)
if !quiet
if !isnothing(path)
printstyled(stderr, " ✓ Created new data collection '$name' at $path\n", color=:green)
else
printstyled(stderr, " ✓ Created new in-memory data collection '$name'\n", color=:green)
end
end
newcollection
end
# For advice purposes
init(dc::DataCollection) = dc
# ------------------
# Stack management
# ------------------
"""
stack_index(ident::Union{Int, String, UUID, DataCollection}; quiet::Bool=false)
Obtain the index of the data collection identified by `ident` on the stack,
if it is present. If it is not found, `nothing` is returned and unless `quiet`
is set a warning is printed.
"""
function stack_index(ident::Union{Int, String, UUID}; quiet::Bool=false)
if ident isa Int
if ident in axes(STACK, 1)
ident
end
elseif ident isa String
findfirst(c -> c.name == ident, STACK)
elseif ident isa UUID
findfirst(c -> c.uuid == ident, STACK)
elseif !quiet
printstyled(" ! ", color=:red)
println("Could not find '$ident' in the stack")
end
end
stack_index(collection::DataCollection) = findfirst(STACK .=== Ref(collection))
"""
stack_move(ident::Union{Int, String, UUID, DataCollection}, shift::Int; quiet::Bool=false)
Find `ident` in the data collection stack, and shift its position by `shift`,
returning the new index. `shift` is clamped so that the new index lies within
STACK.
If `ident` could not be resolved, then `nothing` is returned and unless `quiet`
is set a warning is printed.
"""
function stack_move(ident::Union{Int, String, UUID, DataCollection}, shift::Int; quiet::Bool=false)
current_index = stack_index(ident; quiet)
if !isnothing(current_index)
collection = STACK[current_index]
new_index = clamp(current_index + shift, 1, length(STACK))
if new_index == current_index
quiet || printstyled(" ✓ '$(collection.name)' already at #$current_index\n", color=:green)
else
deleteat!(STACK, current_index)
insert!(STACK, new_index, collection)
quiet || printstyled(" ✓ Moved '$(collection.name)': #$current_index → #$new_index\n", color=:green)
end
new_index
end
end
"""
stack_remove!(ident::Union{Int, String, UUID, DataCollection}; quiet::Bool=false)
Find `ident` in the data collection stack and remove it from the stack,
returning the index at which it was found.
If `ident` could not be resolved, then `nothing` is returned and unless `quiet`
is set a warning is printed.
"""
function stack_remove!(ident::Union{Int, String, UUID, DataCollection}; quiet::Bool=false)
index = stack_index(ident; quiet)
if !isnothing(index)
name = STACK[index].name
deleteat!(STACK, index)
quiet || printstyled(" ✓ Deleted $name\n", color=:green)
index
end
end
# ------------------
# Plugins
# ------------------
"""
plugin_add([collection::DataCollection=first(STACK)], plugins::Vector{<:AbstractString};
quiet::Bool=false)
Return a variation of `collection` with all `plugins` not currently used added
to the plugin list.
Unless `quiet` is a set an informative message is printed.
!!! warning "Side effects"
The new `collection` is written, if possible.
Should `collection` be part of `STACK`, the stack entry is updated in-place.
"""
function plugin_add(collection::DataCollection, plugins::Vector{<:AbstractString};
quiet::Bool=false)
new_plugins = setdiff(plugins, collection.plugins)
if isempty(new_plugins)
if !quiet
printstyled(" i", color=:cyan, bold=true)
println(" No new plugins added")
end
else
# It may seem overcomplicated to:
# 1. Convert `collection` to a Dict
# 2. Modify the "plugin" list there
# 3. Convert back
# instead of simply `push!`-ing to the `plugins` field
# of `collection`, however this is necessary to avoid
# asymmetric advice triggering by the plugins in question.
snapshot = convert(Dict, collection)
snapshot["plugins"] =
append!(get(snapshot, "plugins", String[]), new_plugins)
sort!(snapshot["plugins"])
newcollection =
DataCollection(snapshot; path=collection.path, mod=collection.mod)
if (sindex = findfirst(c -> c === collection, STACK)) |> !isnothing
STACK[sindex] = newcollection
end
iswritable(newcollection) && write(newcollection)
if !quiet
printstyled(" +", color=:light_green, bold=true)
print(" Added plugins: ")
printstyled(join(new_plugins, ", "), '\n', color=:green)
end
newcollection
end
end
function plugin_add(plugins::Vector{<:AbstractString}; quiet::Bool=false)
!isempty(STACK) || throw(EmptyStackError())
plugin_add(first(STACK), plugins; quiet)
end
"""
plugin_remove([collection::DataCollection=first(STACK)], plugins::Vector{<:AbstractString};
quiet::Bool=false)
Return a variation of `collection` with all `plugins` currently used removed
from the plugin list.
Unless `quiet` is a set an informative message is printed.
!!! warning "Side effects"
The new `collection` is written, if possible.
Should `collection` be part of `STACK`, the stack entry is updated in-place.
"""
function plugin_remove(collection::DataCollection, plugins::Vector{<:AbstractString};
quiet::Bool=false)
rem_plugins = intersect(plugins, collection.plugins)
if isempty(rem_plugins)
if !quiet
printstyled(" ! ", color=:yellow, bold=true)
println("No plugins removed, as $(join(plugins, ", ", ", and ")) were never used to begin with")
end
else
# It may seem overcomplicated to:
# 1. Convert `collection` to a Dict
# 2. Modify the "plugin" list there
# 3. Convert back
# instead of simply modifying the `plugins` field
# of `collection`, however this is necessary to avoid
# asymmetric advice triggering by the plugins in question.
snapshot = convert(Dict, collection)
snapshot["plugins"] =
setdiff(get(snapshot, "plugins", String[]), rem_plugins)
newcollection =
DataCollection(snapshot; path=collection.path, mod=collection.mod)
if (sindex = findfirst(c -> c === collection, STACK)) |> !isnothing
STACK[sindex] = newcollection
end
iswritable(newcollection) && write(newcollection)
if !quiet
printstyled(" -", color=:light_red, bold=true)
print(" Removed plugins: ")
printstyled(join(rem_plugins, ", "), '\n', color=:green)
end
end
newcollection
end
function plugin_remove(plugins::Vector{<:AbstractString}; quiet::Bool=false)
!isempty(STACK) || throw(EmptyStackError())
plugin_remove(first(STACK), plugins; quiet)
end
"""
plugin_info(plugin::AbstractString; quiet::Bool=false)
Fetch the documentation of `plugin`, or return `nothing` if documentation could
not be fetched.
If `quiet` is not set warning messages will be omitted when no documentation
could be fetched.
"""
function plugin_info(plugin::AbstractString; quiet::Bool=false)
if plugin ∉ getfield.(PLUGINS, :name)
if !quiet
printstyled(" ! ", color=:red, bold=true)
println("The plugin '$plugin' is not currently loaded")
end
else
documentation = get(PLUGINS_DOCUMENTATION, plugin, nothing)
if !isnothing(documentation)
quiet || printstyled(" The $plugin plugin\n\n", color=:blue, bold=true)
documentation
else
if !quiet
printstyled(" ! ", color=:yellow, bold=true)
println("The plugin '$plugin' has no documentation (naughty plugin!)")
end
end
end
end
"""
plugin_list(collection::DataCollection=first(STACK); quiet::Bool=false)
Obtain a list of plugins used in `collection`.
`quiet` is unused but accepted as an argument for the sake of consistency.
"""
plugin_list(collection::DataCollection=first(STACK); quiet::Bool=false) =
collection.plugins
# ------------------
# Configuration
# ------------------
"""
config_get(propertypath::Vector{String};
collection::DataCollection=first(STACK), quiet::Bool=false)
Obtain the configuration value at `propertypath` in `collection`.
When no value is set, `nothing` is returned instead and if `quiet` is unset
"unset" is printed.
"""
function config_get(collection::DataCollection, propertypath::Vector{String}; quiet::Bool=false)
config = collection.parameters
for segment in propertypath
config isa AbstractDict || (config = SmallDict{String, Nothing}();)
config = get(config, segment, nothing)
if isnothing(config)
quiet || printstyled(" unset\n", color=:light_black)
return nothing
end
end
config
end
config_get(propertypath::Vector{String}; quiet::Bool=false) =
config_get(first(STACK), propertypath; quiet)
"""
config_set([collection::DataCollection=first(STACK)], propertypath::Vector{String}, value::Any;
quiet::Bool=false)
Return a variation of `collection` with the configuration at `propertypath` set
to `value`.
Unless `quiet` is set, a success message is printed.
!!! warning "Side effects"
The new `collection` is written, if possible.
Should `collection` be part of `STACK`, the stack entry is updated in-place.
"""
function config_set(collection::DataCollection, propertypath::Vector{String}, value::Any;
quiet::Bool=false)
# It may seem like an unnecessary layer of indirection to set
# the configuration via a Dict conversion of `collection`,
# however this way any plugin-processing of the configuration
# will be symmetric (i.e. applied at load and write).
snapshot = convert(Dict, collection)
config = get(snapshot, "config", SmallDict{String, Any}())
window = config
for segment in propertypath[1:end-1]
if !haskey(window, segment)
window[segment] = SmallDict{String, Any}()
end
window = window[segment]
end
window[propertypath[end]] = value
snapshot["config"] = config
newcollection =
DataCollection(snapshot; path=collection.path, mod=collection.mod)
if (sindex = findfirst(c -> c === collection, STACK)) |> !isnothing
STACK[sindex] = newcollection
end
iswritable(newcollection) && write(newcollection)
quiet || printstyled(" ✓ Set $(join(propertypath, '.'))\n", color=:green)
newcollection
end
function config_set(propertypath::Vector{String}, value::Any; quiet::Bool=false)
!isempty(STACK) || throw(EmptyStackError())
config_set(first(STACK), propertypath, value; quiet)
end
"""
config_unset([collection::DataCollection=first(STACK)], propertypath::Vector{String};
quiet::Bool=false)
Return a variation of `collection` with the configuration at `propertypath`
removed.
Unless `quiet` is set, a success message is printed.
!!! warning "Side effects"
The new `collection` is written, if possible.
Should `collection` be part of `STACK`, the stack entry is updated in-place.
"""
function config_unset(collection::DataCollection, propertypath::Vector{String};
quiet::Bool=false)
# It may seem like an unnecessary layer of indirection to set
# the configuration via a Dict conversion of `collection`,
# however this way any plugin-processing of the configuration
# will be symmetric (i.e. applied at load and write).
snapshot = convert(Dict, collection)
config = get(snapshot, "config", SmallDict{String, Any}())
window = config
for segment in propertypath[1:end-1]
if !haskey(window, segment)
window[segment] = Dict{String, Any}()
end
window = window[segment]
end
delete!(window, propertypath[end])
snapshot["config"] = config
newcollection =
DataCollection(snapshot; path=collection.path, mod=collection.mod)
if (sindex = findfirst(c -> c === collection, STACK)) |> !isnothing
STACK[sindex] = newcollection
end
iswritable(newcollection) && write(newcollection)
quiet || printstyled(" ✓ Unset $(join(propertypath, '.'))\n", color=:green)
newcollection
end
function config_unset(propertypath::Vector{String}; quiet::Bool=false)
!isempty(STACK) || throw(EmptyStackError())
config_unset(first(STACK), propertypath; quiet)
end
# ------------------
# Dataset creation
# ------------------
"""
add(::Type{DataSet}, name::String, spec::Dict{String, Any}, source::String="";
collection::DataCollection=first(STACK), storage::Vector{Symbol}=Symbol[],
loaders::Vector{Symbol}=Symbol[], writers::Vector{Symbol}=Symbol[],
quiet::Bool=false)
Create a new DataSet with a `name` and `spec`, and add it to `collection`. The
data transformers will be constructed with each of the backends listed in
`storage`, `loaders`, and `writers` from `source`. If the symbol `*` is given,
all possible drivers will be searched and the highest priority driver available
(according to `createpriority`) used. Should no transformer of the specified
driver and type exist, it will be skipped.
"""
function add(::Type{DataSet}, name::String, spec::Dict{String, Any}, source::String="";
collection::DataCollection=first(STACK),
storage::Vector{Symbol}=Symbol[], loaders::Vector{Symbol}=Symbol[],
writers::Vector{Symbol}=Symbol[], quiet::Bool=false)
spec["uuid"] = uuid4()
dataset = @advise fromspec(DataSet, collection, name, spec)
for (transformer, slot, drivers) in ((DataStorage, :storage, storage),
(DataLoader, :loaders, loaders),
(DataWriter, :writers, writers))
for driver in drivers
dt = create(transformer, driver, source, dataset)
if isnothing(dt)
printstyled(" ! ", color=:yellow, bold=true)
println("Failed to create '$driver' $(string(nameof(transformer))[5:end])")
else
push!(getproperty(dataset, slot), dt)
end
end
end
push!(collection.datasets, dataset)
iswritable(collection) && write(collection)
quiet || printstyled(" ✓ Created '$name' ($(dataset.uuid))\n ", color=:green)
dataset
end
"""
create(T::Type{<:AbstractDataTransformer}, source::String, dataset::DataSet)
If `source`/`dataset` can be used to construct a data transformer of type `T`,
do so and return it. Otherwise return `nothing`.
Specific transformers should implement specialised forms of this function, that
either return `nothing` to indicate that it is not applicable, or a "create spec
form". A "create spec form" is simply a list of `key::String => value` entries,
giving properties of the to-be-created transformer, e.g.
```
["foo" => "bar",
"baz" => 2]
```
In addition to accepting TOML-representable values, a `NamedTuple` value can
be given that specifies an interactive prompt to put to the user.
```
(; prompt::String = "\$key",
type::Type{String or Bool or <:Number} = String,
default::type = false or "",
optional::Bool = false,
skipvalue::Any = nothing,
post::Function = identity)
```
The value can also be a `Function` that takes the current specification as an
argument and returns a TOML-representable value or `NamedTuple`.
Lastly `true`/`false` can be returned as a convenient way of simply indicating
whether an empty (no parameters) driver should be created.
"""
create(T::Type{<:AbstractDataTransformer}, source::String, ::DataSet) =
create(T::Type{<:AbstractDataTransformer}, source)
create(::Type{<:AbstractDataTransformer}, ::String) = nothing
"""
createpriority(T::Type{<:AbstractDataTransformer})
The priority with which a transformer of type `T` should be created.
This can be any integer, but try to keep to -100–100 (see `create`).
"""
createpriority(T::Type{<:AbstractDataTransformer}) = 0
"""
create(T::Type{<:AbstractDataTransformer}, driver::Symbol, source::String, dataset::DataSet;
minpriority::Int=-100, maxpriority::Int=100)
Create a new `T` with driver `driver` from `source`/`dataset`.
If `driver` is the symbol `*` then all possible drivers are checked and the
highest priority (according to `createpriority`) valid driver used. Drivers with
a priority outside `minpriority`–`maxpriority` will not be considered.
The created data transformer is returned, unless the given `driver` is not
valid, in which case `nothing` is returned instead.
"""
function create(T::Type{<:AbstractDataTransformer}, driver::Symbol, source::String, dataset::DataSet;
minpriority::Int=-100, maxpriority::Int=100)
T ∈ (DataStorage, DataLoader, DataWriter) ||
throw(ArgumentError("T=$T should be an driver-less Data{Storage,Loader,Writer}"))
function process_spec(spec::Vector, driver::Symbol)
final_spec = Dict{String, Any}()
function expand_value(key::String, value::Any)
if value isa Function
value = value(final_spec)
end
final_value = if value isa TOML.Internals.Printer.TOMLValue
value
elseif value isa NamedTuple
type = get(value, :type, String)
vprompt = " $(string(nameof(T))[5])($driver) " *
get(value, :prompt, "$key: ")
result = if type == Bool
confirm_yn(vprompt, get(value, :default, false))
elseif type == String
res = prompt(vprompt, get(value, :default, "");
allowempty = get(value, :optional, false))
if !isempty(res) res end
elseif type <: Number
parse(type, prompt(vprompt, string(get(value, :default, zero(type)))))
end |> get(value, :post, identity)
if get(value, :optional, false) && get(value, :skipvalue, nothing) === true && result
else
result
end
end
if !isnothing(final_value)
final_spec[key] = final_value
end
end
for (key, value) in spec
expand_value(key, value)
end
final_spec["driver"] = string(driver)
final_spec
end
if driver == :*
alldrivers = if T == DataStorage
vcat(methods(storage), methods(getstorage))
elseif T == DataLoader
methods(load)
elseif T == DataWriter
methods(save)
end |>
ms -> map(f -> Base.unwrap_unionall(
Base.unwrap_unionall(f.sig).types[2]).parameters[1], ms) |>
ds -> filter(d -> d isa Symbol, ds) |> unique |>
ds -> sort(ds, by=driver -> createpriority(T{driver})) |>
ds -> filter(driver ->
minpriority <= createpriority(T{driver}) <= maxpriority, ds)
for drv in alldrivers
spec = create(T{drv}, source, dataset)
spec isa Bool && (spec = ifelse(spec, [], nothing))
if !isnothing(spec)
return @advise fromspec(T, dataset, process_spec(spec, drv))
end
end
else
spec = create(T{driver}, source, dataset)::Union{Bool, Vector, Nothing}
spec isa Bool && (spec = ifelse(spec, [], nothing))
if !isnothing(spec)
return @advise fromspec(T, dataset, process_spec(spec, driver))
end
end
end
# ------------------
# Dataset deletion
# ------------------
"""
delete!(dataset::DataSet)
Remove `dataset` from its parent collection.
"""
function Base.delete!(dataset::DataSet)
index = findfirst(d -> d.uuid == dataset.uuid, dataset.collection.datasets)
deleteat!(dataset.collection.datasets, index)
write(dataset.collection)
end
# ------------------
# Dataset modification
# ------------------
"""
replace!(dataset::DataSet; [name, uuid, parameters, storage, loaders, writers])
Perform an in-place update of `dataset`, optionally replacing any of the `name`,
`uuid`, `parameters`, `storage`, `loaders`, or `writers` fields.
"""
function Base.replace!(dataset::DataSet;
name::String = dataset.name,
uuid::UUID = dataset.uuid,
parameters::Dict{String, Any} = dataset.parameters,
storage::Vector{DataStorage} = dataset.storage,
loaders::Vector{DataLoader} = dataset.loaders,
writers::Vector{DataWriter} = dataset.writers)
iswritable(dataset.collection) || throw(ReadonlyCollection(dataset.collection))
dsindex = findfirst(==(dataset), dataset.collection.datasets)
!isnothing(dsindex) || throw(OrphanDataSet(dataset))
replacement = DataSet(dataset.collection, name, uuid, parameters,
DataStorage[], DataLoader[], DataWriter[])
for (tfield, transformers) in zip((:storage, :loaders, :writers),
(storage, loaders, writers))
for transformer in transformers
push!(getfield(replacement, tfield),
typeof(transformer)(replacement, transformer.type,
transformer.priority, transformer.parameters))
end
end
dataset.collection.datasets[dsindex] = replacement
end
| DataToolkitBase | https://github.com/tecosaur/DataToolkitBase.jl.git |
|
[
"MIT"
] | 0.9.1 | ed1a12069bdb85038eb736b2a3bde5d59093eb0b | code | 27785 | using REPL, REPL.LineEdit
# ------------------
# Setting up the Data REPL and framework
# ------------------
@doc """
A command that can be used in the Data REPL (accessible through '$REPL_KEY').
A `ReplCmd` must have a:
- `name`, a symbol designating the command keyword.
- `trigger`, a string used as the command trigger (defaults to `String(name)`).
- `description`, a short overview of the functionality as a `string` or `display`able object.
- `execute`, either a list of sub-ReplCmds, or a function which will perform the
command's action. The function must take a single argument, the rest of the
command as an `AbstractString` (for example, 'cmd arg1 arg2' will call the
execute function with "arg1 arg2").
# Constructors
```julia
ReplCmd{name::Symbol}(trigger::String, description::Any, execute::Function)
ReplCmd{name::Symbol}(description::Any, execute::Function)
ReplCmd(name::Union{Symbol, String}, trigger::String, description::Any, execute::Function)
ReplCmd(name::Union{Symbol, String}, description::Any, execute::Function)
```
# Examples
```julia
ReplCmd(:echo, "print the argument", identity)
ReplCmd(:addone, "return the input plus one", v -> 1 + parse(Int, v))
ReplCmd(:math, "A collection of basic integer arithmetic",
[ReplCmd(:add, "a + b + ...", nums -> sum(parse.(Int, split(nums))))],
ReplCmd(:mul, "a * b * ...", nums -> prod(parse.(Int, split(nums)))))
```
# Methods
```julia
help(::ReplCmd) # -> print detailed help
allcompletions(::ReplCmd) # -> list all candidates
completions(::ReplCmd, sofar::AbstractString) # -> list relevant candidates
```
""" ReplCmd
ReplCmd{name}(description::Any, execute::Union{Function, Vector{ReplCmd}}) where {name} =
ReplCmd{name}(String(name), description, execute)
ReplCmd(name::Union{Symbol, String}, args...) =
ReplCmd{Symbol(name)}(args...)
"""
help(r::ReplCmd)
Print the help string for `r`.
help(r::ReplCmd{<:Any, Vector{ReplCmd}})
Print the help string and subcommand table for `r`.
"""
function help(r::ReplCmd)
if r.description isa AbstractString
for line in eachsplit(rstrip(r.description), '\n')
println(" ", line)
end
else
display(r.description)
end
end
function help(r::ReplCmd{<:Any, Vector{ReplCmd}})
if r.description isa AbstractString
for line in eachsplit(rstrip(r.description), '\n')
println(" ", line)
end
else
display(r.description)
end
print('\n')
help_cmd_table(commands = r.execute, sub=true)
end
"""
completions(r::ReplCmd, sofar::AbstractString)
Obtain a list of `String` completion candidates based on `sofar`.
All candidates should begin with `sofar`.
Should this function not be implemented for the specific ReplCmd `r`,
`allcompletions(r)` will be called and filter to candidates that begin
with `sofar`.
If `r` has subcommands, then the subcommand prefix will be removed and
`completions` re-called on the relevant subcommand.
"""
completions(r::ReplCmd, sofar::AbstractString) =
sort(filter(s -> startswith(s, sofar), allcompletions(r)))
completions(r::ReplCmd{<:Any, Vector{ReplCmd}}, sofar::AbstractString) =
complete_repl_cmd(sofar, commands = r.execute)
"""
allcompletions(r::ReplCmd)
Obtain all possible `String` completion candidates for `r`.
This defaults to the empty vector `String[]`.
`allcompletions` is only called when `completions(r, sofar::AbstractString)` is
not implemented.
"""
allcompletions(::ReplCmd) = String[]
"""
The help-string for the help command itself.
This contains the template string \"<SCOPE>\", which
is replaced with the relevant scope at runtime.
"""
const HELP_CMD_HELP =
"""Display help information on the available <SCOPE> commands
For convenience, help information can also be accessed via '?', e.g. '?help'.
Help for data transformers can also be accessed by asking for the help of the
transformer name prefixed by ':' (i.e. ':transformer'), and a list of documented
transformers can be pulled up with just ':'.
Usage
=====
$REPL_PROMPT help
$REPL_PROMPT help CMD
$REPL_PROMPT help PARENT CMD
$REPL_PROMPT PARENT help CMD
$REPL_PROMPT help :
$REPL_PROMPT help :TRANSFORMER
"""
"""
find_repl_cmd(cmd::AbstractString; warn::Bool=false,
commands::Vector{ReplCmd}=REPL_CMDS,
scope::String="Data REPL")
Examine the command string `cmd`, and look for a command from `commands` that is
uniquely identified. Either the identified command or `nothing` will be returned.
Should `cmd` start with `help` or `?` then a `ReplCmd{:help}` command is returned.
If `cmd` is ambiguous and `warn` is true, then a message listing all potentially
matching commands is printed.
If `cmd` does not match any of `commands` and `warn` is true, then a warning
message is printed. Additionally, should the named command in `cmd` have more
than a 3/5th longest common subsequence overlap with any of `commands`, then
those commands are printed as suggestions.
"""
function find_repl_cmd(cmd::AbstractString; warn::Bool=false,
commands::Vector{ReplCmd}=REPL_CMDS,
scope::String="Data REPL")
replcmds = let candidates = filter(c -> startswith(c.trigger, cmd), commands)
if isempty(candidates)
candidates = filter(c -> issubseq(cmd, c.trigger), commands)
if !isempty(candidates)
char_filtered = filter(c -> startswith(c.trigger, first(cmd)), candidates)
if !isempty(char_filtered)
candidates = char_filtered
end
end
end
candidates
end
subcommands = Dict{String, Vector{String}}()
for command in commands
if command.execute isa Vector{ReplCmd}
for subcmd in command.execute
if haskey(subcommands, subcmd.trigger)
push!(subcommands[subcmd.trigger], command.trigger)
else
subcommands[subcmd.trigger] = [command.trigger]
end
end
end
end
all_cmd_names = getproperty.(commands, :trigger)
if cmd == "" && "" in all_cmd_names
replcmds[findfirst("" .== all_cmd_names)]
elseif length(replcmds) == 0 && (cmd == "?" || startswith("help", cmd)) || length(cmd) == 0
ReplCmd{:help}("help", replace(HELP_CMD_HELP, "<SCOPE>" => scope),
cmd -> help_show(cmd; commands))
elseif length(replcmds) == 1
first(replcmds)
elseif length(replcmds) > 1 &&
sum(cmd .== getproperty.(replcmds, :trigger)) == 1 # single exact match
replcmds[findfirst(c -> c.trigger == cmd, replcmds)]
elseif warn && length(replcmds) > 1
printstyled(" ! ", color=:red, bold=true)
print("Multiple matching $scope commands: ")
candidates = filter(!=(""), getproperty.(replcmds, :trigger))
for cand in candidates
highlight_lcs(stdout, cand, String(cmd), before="\e[4m", after="\e[24m")
cand === last(candidates) || print(", ")
end
print('\n')
elseif warn && haskey(subcommands, cmd)
printstyled(" ! ", color=:red, bold=true)
println("The $scope command '$cmd' is not defined.")
printstyled(" i ", color=:cyan, bold=true)
println("Perhaps you want the '$(join(subcommands[cmd], '/')) $cmd' subcommand?")
elseif warn # no matching commands
printstyled(" ! ", color=:red, bold=true)
println("The $scope command '$cmd' is not defined.")
push!(all_cmd_names, "help")
cmdsims = stringsimilarity.(cmd, all_cmd_names)
if maximum(cmdsims, init=0) >= 0.5
printstyled(" i ", color=:cyan, bold=true)
println("Perhaps you meant '$(all_cmd_names[argmax(cmdsims)])'?")
end
end
end
"""
execute_repl_cmd(line::AbstractString;
commands::Vector{ReplCmd}=REPL_CMDS,
scope::String="Data REPL")
Examine `line` and identify the leading command, then:
- Show an error if the command is not given in `commands`
- Show help, if help is asked for (see `help_show`)
- Call the command's execute function, if applicable
- Call `execute_repl_cmd` on the argument with `commands`
set to the command's subcommands and `scope` set to the command's trigger,
if applicable
"""
function execute_repl_cmd(line::AbstractString;
commands::Vector{ReplCmd}=REPL_CMDS,
scope::String="Data REPL")
cmd_parts = split(line, limit = 2)
cmd, rest = if length(cmd_parts) == 0
"", ""
elseif length(cmd_parts) == 1
cmd_parts[1], ""
else
cmd_parts
end
if startswith(cmd, "?")
execute_repl_cmd(string("help ", line[2:end]); commands, scope)
elseif startswith("help", cmd) && !isempty(cmd) # help is special
rest_parts = split(rest, limit=2)
if length(rest_parts) == 1 && startswith(first(rest_parts), ':')
help_show(Symbol(first(rest_parts)[2:end]))
elseif length(rest_parts) <= 1
help_show(rest; commands)
elseif find_repl_cmd(rest_parts[1]; commands) isa ReplCmd{<:Any, Vector{ReplCmd}}
execute_repl_cmd(string(rest_parts[1], " help ", rest_parts[2]);
commands, scope)
else
help_show(rest_parts[1]; commands)
end
else
repl_cmd = find_repl_cmd(cmd; warn=true, commands, scope)
if isnothing(repl_cmd)
elseif repl_cmd isa ReplCmd{<:Any, Function}
repl_cmd.execute(rest)
elseif repl_cmd isa ReplCmd{<:Any, Vector{ReplCmd}}
execute_repl_cmd(rest, commands = repl_cmd.execute, scope = repl_cmd.trigger)
end
end
end
"""
toplevel_execute_repl_cmd(line::AbstractString)
Call `execute_repl_cmd(line)`, but gracefully catch an InterruptException if
thrown.
This is the main entrypoint for command execution.
"""
function toplevel_execute_repl_cmd(line::AbstractString)
try
execute_repl_cmd(line)
catch e
if e isa InterruptException
printstyled(" !", color=:red, bold=true)
print(" Aborted\n")
else
rethrow()
end
end
end
"""
complete_repl_cmd(line::AbstractString; commands::Vector{ReplCmd}=REPL_CMDS)
Return potential completion candidates for `line` provided by `commands`.
More specifically, the command being completed is identified and
`completions(cmd::ReplCmd{:cmd}, sofar::AbstractString)` called.
Special behaviour is implemented for the help command.
"""
function complete_repl_cmd(line::AbstractString; commands::Vector{ReplCmd}=REPL_CMDS)
if isempty(line)
(sort(vcat(getfield.(commands, :trigger), "help")),
"",
true)
else
cmd_parts = split(line, limit = 2)
cmd_name, rest = if length(cmd_parts) == 1
cmd_parts[1], ""
else
cmd_parts
end
repl_cmd = find_repl_cmd(cmd_name; commands)
complete = if !isnothing(repl_cmd) && line != cmd_name
if repl_cmd isa ReplCmd{:help}
# This can't be a `completions(...)` call because we
# need to access `commands`.
if startswith(rest, ':') # transformer help
filter(t -> startswith(t, rest),
string.(':', TRANSFORMER_DOCUMENTATION .|>
first .|> last |> unique))
else # command help
Vector{String}(filter(ns -> startswith(ns, rest),
getfield.(commands, :trigger)))
end
else
completions(repl_cmd, rest)
end
else
all_cmd_names = vcat(getfield.(commands, :trigger), "help")
# Keep any ?-prefix if getting help, otherwise it would be nice
# to end with a space to get straight to the sub-command/argument.
all_cmd_names = if startswith(cmd_name, "?")
'?' .* all_cmd_names
else
all_cmd_names .* ' '
end
cmds = filter(ns -> startswith(ns, cmd_name), all_cmd_names)
(sort(cmds),
String(line),
!isempty(cmds))
end
if complete isa Tuple{Vector{String}, String, Bool}
complete
elseif complete isa Vector{String}
(sort(complete),
String(rest),
!isempty(complete))
else
error("REPL completions for $cmd_name returned strange result, $(typeof(complete))")
end
end
end
"""
A singleton to allow for for Data REPL specific completion dispatching.
"""
struct DataCompletionProvider <: REPL.LineEdit.CompletionProvider end
function REPL.complete_line(::DataCompletionProvider,
state::REPL.LineEdit.PromptState)
# See REPL.jl complete_line(c::REPLCompletionProvider, s::PromptState)
partial = REPL.beforecursor(state.input_buffer)
full = REPL.LineEdit.input_string(state)
if partial != full
# For now, only complete at end of line
return ([], "", false)
end
complete_repl_cmd(full)
end
"""
init_repl()
Construct the Data REPL `LineEdit.Prompt` and configure it and the REPL to
behave appropriately. Other than boilerplate, this basically consists of:
- Setting the prompt style
- Setting the execution function (`toplevel_execute_repl_cmd`)
- Setting the completion to use `DataCompletionProvider`
"""
function init_repl()
# With *heavy* inspiration from https://github.com/MasonProtter/ReplMaker.jl
repl = Base.active_repl
if !isdefined(repl, :interface)
repl.interface = REPL.setup_interface(repl)
end
julia_mode = repl.interface.modes[1]
prompt_prefix, prompt_suffix = if repl.hascolor
REPL_PROMPTSTYLE, "\e[m"
else
"", ""
end
data_mode = LineEdit.Prompt(
() -> if isempty(STACK)
"(⋅) $REPL_PROMPT "
else
"($(first(STACK).name)) $REPL_PROMPT "
end;
prompt_prefix,
prompt_suffix,
keymap_dict = LineEdit.default_keymap_dict,
on_enter = LineEdit.default_enter_cb,
complete = DataCompletionProvider(),
sticky = true)
data_mode.on_done = REPL.respond(toplevel_execute_repl_cmd, repl, data_mode)
push!(repl.interface.modes, data_mode)
history_provider = julia_mode.hist
history_provider.mode_mapping[REPL_NAME] = data_mode
data_mode.hist = history_provider
_, search_keymap = LineEdit.setup_search_keymap(history_provider)
_, prefix_keymap = LineEdit.setup_prefix_keymap(history_provider, data_mode)
julia_keymap = REPL.mode_keymap(julia_mode)
data_mode.keymap_dict = LineEdit.keymap(Dict{Any, Any}[
search_keymap,
julia_keymap,
prefix_keymap,
LineEdit.history_keymap,
LineEdit.default_keymap,
LineEdit.escape_defaults
])
key_alt_action =
something(deepcopy(get(julia_mode.keymap_dict, REPL_KEY, nothing)),
(state, args...) -> LineEdit.edit_insert(state, REPL_KEY))
function key_action(state, args...)
if isempty(state) || position(LineEdit.buffer(state)) == 0
function transition_action()
LineEdit.state(state, data_mode).input_buffer =
copy(LineEdit.buffer(state))
end
LineEdit.transition(transition_action, state, data_mode)
else
key_alt_action(state, args...)
end
end
data_keymap = Dict{Any, Any}(REPL_KEY => key_action)
julia_mode.keymap_dict =
LineEdit.keymap_merge(julia_mode.keymap_dict, data_keymap)
data_mode
end
# ------------------
# Interaction utilities
# ------------------
"""
prompt(question::AbstractString, default::AbstractString="",
allowempty::Bool=false, cleardefault::Bool=true,
multiline::Bool=false)
Interactively ask `question` and return the response string, optionally
with a `default` value. If `multiline` is true, `RET` must be pressed
twice consecutively to submit a value.
Unless `allowempty` is set an empty response is not accepted.
If `cleardefault` is set, then an initial backspace will clear the default value.
The prompt supports the following line-edit-y keys:
- left arrow
- right arrow
- home
- end
- delete forwards
- delete backwards
### Example
```julia-repl
julia> prompt("What colour is the sky? ")
What colour is the sky? Blue
"Blue"
```
"""
function prompt(question::AbstractString, default::AbstractString="";
allowempty::Bool=false, cleardefault::Bool=true,
multiline::Bool=false)
firstinput = true
# Set `:color` to `false` in the stdout, so that the
# terminal doesn't report color support, and the
# prompt isn't bold.
term = REPL.Terminals.TTYTerminal(
get(ENV, "TERM", Sys.iswindows() ? "" : "dumb"),
stdin, IOContext(stdout, :color => false), stderr)
keymap = REPL.LineEdit.keymap([
Dict{Any, Any}(
"^C" => (_...) -> throw(InterruptException()),
# Backspace
'\b' => function (s::REPL.LineEdit.MIState, o...)
if firstinput && cleardefault
REPL.LineEdit.edit_clear(s)
else
REPL.LineEdit.edit_backspace(s)
end
end,
# Delete
"\e[3~" => function (s::REPL.LineEdit.MIState, o...)
if firstinput && cleardefault
REPL.LineEdit.edit_clear(s)
else
REPL.LineEdit.edit_delete(s)
end
end,
# Return
'\r' => function (s::REPL.LineEdit.MIState, o...)
if multiline
if eof(REPL.LineEdit.buffer(s)) && s.key_repeats >= 1
REPL.LineEdit.commit_line(s)
:done
else
REPL.LineEdit.edit_insert_newline(s)
end
else
if REPL.LineEdit.on_enter(s) &&
(allowempty || REPL.LineEdit.buffer(s).size != 0)
REPL.LineEdit.commit_line(s)
:done
else
REPL.LineEdit.beep(s)
end
end
end),
REPL.LineEdit.default_keymap,
REPL.LineEdit.escape_defaults
])
prompt = REPL.LineEdit.Prompt(
question;
prompt_prefix = Base.text_colors[REPL_QUESTION_COLOR],
prompt_suffix = Base.text_colors[ifelse(
isempty(default), REPL_USER_INPUT_COLOUR, :light_black)],
keymap_dict = keymap,
complete = REPL.LatexCompletions(),
on_enter = _ -> true)
interface = REPL.LineEdit.ModalInterface([prompt])
istate = REPL.LineEdit.init_state(term, interface)
pstate = istate.mode_state[prompt]
if !isempty(default)
write(pstate.input_buffer, default)
end
Base.reseteof(term)
REPL.LineEdit.raw!(term, true)
REPL.LineEdit.enable_bracketed_paste(term)
try
pstate.ias = REPL.LineEdit.InputAreaState(0, 0)
REPL.LineEdit.refresh_multi_line(term, pstate)
while true
kmap = REPL.LineEdit.keymap(pstate, prompt)
matchfn = REPL.LineEdit.match_input(kmap, istate)
kdata = REPL.LineEdit.keymap_data(pstate, prompt)
status = matchfn(istate, kdata)
if status === :ok
elseif status === :ignore
istate.last_action = istate.current_action
elseif status === :done
print("\e[F")
if firstinput
pstate.p.prompt_suffix = Base.text_colors[REPL_USER_INPUT_COLOUR]
REPL.LineEdit.refresh_multi_line(term, pstate)
end
print("\e[39m\n")
return String(rstrip(REPL.LineEdit.input_string(pstate), '\n'))
else
return nothing
end
if firstinput
pstate.p.prompt_suffix = Base.text_colors[REPL_USER_INPUT_COLOUR]
REPL.LineEdit.refresh_multi_line(term, pstate)
firstinput = false
end
end
finally
REPL.LineEdit.raw!(term, false) &&
REPL.LineEdit.disable_bracketed_paste(term)
end
end
"""
prompt_char(question::AbstractString, options::Vector{Char},
default::Union{Char, Nothing}=nothing)
Interactively ask `question`, only accepting `options` keys as answers.
All keys are converted to lower case on input. If `default` is not nothing and
'RET' is hit, then `default` will be returned.
Should '^C' be pressed, an InterruptException will be thrown.
"""
function prompt_char(question::AbstractString, options::Vector{Char},
default::Union{Char, Nothing}=nothing)
printstyled(question, color=REPL_QUESTION_COLOR)
term_env = get(ENV, "TERM", @static Sys.iswindows() ? "" : "dumb")
term = REPL.Terminals.TTYTerminal(term_env, stdin, stdout, stderr)
REPL.Terminals.raw!(term, true)
char = '\x01'
while char ∉ options
char = lowercase(Char(REPL.TerminalMenus.readkey(stdin)))
if char == '\r' && !isnothing(default)
char = default
elseif char == '\x03' # ^C
print("\e[m^C\n")
throw(InterruptException())
end
end
REPL.Terminals.raw!(term, false)
get(stdout, :color, false) && print(Base.text_colors[REPL_USER_INPUT_COLOUR])
print(stdout, char, '\n')
get(stdout, :color, false) && print("\e[m")
char
end
"""
confirm_yn(question::AbstractString, default::Bool=false)
Interactively ask `question` and accept y/Y/n/N as the response.
If any other key is pressed, then `default` will be taken as the response.
A " [y/n]: " string will be appended to the question, with y/n capitalised
to indicate the default value.
### Example
```julia-repl
julia> confirm_yn("Do you like chocolate?", true)
Do you like chocolate? [Y/n]: y
true
```
"""
function confirm_yn(question::AbstractString, default::Bool=false)
char = prompt_char(question * (" [y/N]: ", " [Y/n]: ")[1+ default],
['y', 'n'], ('n', 'y')[1+default])
char == 'y'
end
"""
peelword(input::AbstractString)
Read the next 'word' from `input`. If `input` starts with a quote, this is the
unescaped text between the opening and closing quote. Other wise this is simply
the next word.
Returns a tuple of the form `(word, rest)`.
### Example
```julia-repl
julia> peelword("one two")
("one", "two")
julia> peelword("\"one two\" three")
("one two", "three")
```
"""
function peelword(input::AbstractString; allowdot::Bool=true)
if isempty(input)
("", "")
elseif first(lstrip(input)) != '"' || count(==('"'), input) < 2
Tuple(match(ifelse(allowdot,
r"^\s*([^\s][^\s]*)\s*(.*?|)$",
r"^\s*([^\s][^\s.]*)\s*(.*?|)$"),
input).captures .|> String)
else # Starts with " and at least two " in `input`.
start = findfirst(!isspace, input)::Int
stop = nextind(input, start)
maxstop = lastindex(input)
word = Char[]
while input[stop] != '"' && stop <= maxstop
push!(word, input[stop + Int(input[stop] == '\\')])
stop = nextind(input, stop, 1 + Int(input[stop] == '\\'))
end
stop = nextind(input, stop)
if stop <= maxstop && isspace(input[stop])
stop = nextind(input, stop)
end
(String(word), input[stop:end])
end
end
# ------------------
# The help command
# ------------------
"""
help_cmd_table(; maxwidth::Int=displaysize(stdout)[2],
commands::Vector{ReplCmd}=REPL_CMDS,
sub::Bool=false)
Print a table showing the triggers and descriptions (limited to the first line)
of `commands`, under the headers "Command" and "Action" (or "Subcommand" if
`sub` is set). The table is truncated if necessary so it is no wider than
`maxwidth`.
"""
function help_cmd_table(; maxwidth::Int=displaysize(stdout)[2]-2,
commands::Vector{ReplCmd}=REPL_CMDS,
sub::Bool=false)
help_headings = [if sub "Subcommand" else "Command" end, "Action"]
help_lines = map(commands) do replcmd
String[replcmd.trigger,
first(split(string(replcmd.description), '\n'))]
end
push!(help_lines, ["help", "Display help text for commands and transformers"])
map(displaytable(help_headings, help_lines; maxwidth)) do row
print(stderr, " ", row, '\n')
end
end
"""
help_show(cmd::AbstractString; commands::Vector{ReplCmd}=REPL_CMDS)
If `cmd` refers to a command in `commands`, show its help (via `help`).
If `cmd` is empty, list `commands` via `help_cmd_table`.
"""
function help_show(cmd::AbstractString; commands::Vector{ReplCmd}=REPL_CMDS)
if all(isspace, cmd)
help_cmd_table(; commands)
println("\n \e[2;3mCommands can also be triggered by unique prefixes or substrings.\e[22;23m")
else
repl_cmd = find_repl_cmd(strip(cmd); commands, warn=true)
if !isnothing(repl_cmd)
help(repl_cmd)
end
end
nothing
end
"""
transformer_docs(name::Symbol, type::Symbol=:any)
Return the documentation for the transformer identified by `name`,
or `nothing` if no documentation entry could be found.
"""
function transformer_docs(name::Symbol, type::Symbol=:any)
tindex = findfirst(
t -> first(t)[2] === name && (type === :any || first(t)[1] === type),
TRANSFORMER_DOCUMENTATION)
if !isnothing(tindex)
last(TRANSFORMER_DOCUMENTATION[tindex])
end
end
"""
transformers_printall()
Print a list of all documented data transformers, by category.
"""
function transformers_printall()
docs = (storage = Pair{Symbol, Any}[],
loader = Pair{Symbol, Any}[],
writer = Pair{Symbol, Any}[])
for ((type, name), doc) in TRANSFORMER_DOCUMENTATION
if type ∈ (:storage, :loader, :writer)
push!(getfield(docs, type), name => doc)
else
@warn "Documentation entry for $name gives invalid transformer type $type (should be 'storage', 'loader', or 'writer')"
end
end
sort!.(values(docs), by = first)
for type in (:storage, :loader, :writer)
entries = getfield(docs, type)
printstyled(" $type transformers ($(length(entries)))\n",
color=:blue, bold=true)
for (name, doc) in entries
printstyled(" • ", color=:blue)
println(name)
end
type === :writer || print('\n')
end
end
"""
help_show(transformer::Symbol)
Show documentation of a particular data `transformer` (should it exist).
In the special case that `transformer` is `Symbol("")`, a list of all documented
transformers is printed.
"""
function help_show(transformer::Symbol)
if transformer === Symbol("") # List all documented transformers
transformers_printall()
else
tdocs = transformer_docs(transformer)
if isnothing(tdocs)
printstyled(" ! ", color=:red, bold=true)
println("There is no documentation for the '$transformer' transformer")
else
display(tdocs)
end
end
end
| DataToolkitBase | https://github.com/tecosaur/DataToolkitBase.jl.git |
|
[
"MIT"
] | 0.9.1 | ed1a12069bdb85038eb736b2a3bde5d59093eb0b | code | 7247 | Advice(@nospecialize(f::Function)) = Advice(DEFAULT_DATA_ADVISOR_PRIORITY, f)
Base.methods(dt::Advice) = methods(dt.f)
"""
(advice::Advice)((post::Function, func::Function, args::Tuple, kwargs::NamedTuple))
Apply `advice` to the function call `func(args...; kwargs...)`, and return the
new `(post, func, args, kwargs)` tuple.
"""
function (dt::Advice{F, C})(callform::Tuple{Function, Function, Tuple, NamedTuple}) where {F, C}
@nospecialize callform
post, func, args, kwargs = callform
# Abstract-y `typeof`.
atypeof(val::Any) = typeof(val)
atypeof(val::Type) = Type{val}
if hasmethod(dt.f, Tuple{typeof(func), atypeof.(args)...}, keys(kwargs))
result = invokepkglatest(dt.f, func, args...; kwargs...)
after, func, args, kwargs = if result isa Tuple{Function, Function, Tuple, NamedTuple}
result # Fully specified form
elseif result isa Tuple{Function, Function, Tuple}
# Default kwargs
result[1], result[2], result[3], NamedTuple()
elseif result isa Tuple{Function, Tuple, NamedTuple}
# Default post function
identity, result[1], result[2], result[3]
elseif result isa Tuple{Function, Tuple}
# Default post function and kwargs
identity, result[1], result[2], NamedTuple()
else
throw(ErrorException("Advice function produced invalid result: $(typeof(result))"))
end
if after !== identity
post = post ∘ after
end
(post, func, args, kwargs)
else
(post, func, args, kwargs) # act as the identity fiction
end
end
function (dt::Advice)(func::Function, args...; kwargs...)
@nospecialize func args kwargs
atypeof(val::Any) = typeof(val)
atypeof(val::Type) = Type{val}
if hasmethod(dt.f, Tuple{typeof(func), atypeof.(args)...}, keys(kwargs))
post, func, args, kwargs = dt((identity, func, args, merge(NamedTuple(), kwargs)))
result = func(args...; kwargs...)
post(result)
else
func(args...; kwargs...)
end
end
Base.empty(::Type{AdviceAmalgamation}) =
AdviceAmalgamation(identity, Advice[], String[], String[])
# When getting a property of a `AdviceAmalgamation`, first check
# if the `:plugins_wanted` field is satisfied. Should it not be,
# regenerate the `:advisors`, `:adviseall`, and `:plugins_used` fields
# based on the currently available plugins and `:plugins_wanted`.
function Base.getproperty(dta::AdviceAmalgamation, prop::Symbol)
if getfield(dta, :plugins_wanted) != getfield(dta, :plugins_used)
plugins_available =
filter(plugin -> plugin.name in getfield(dta, :plugins_wanted), PLUGINS)
if getfield.(plugins_available, :name) != getfield(dta, :plugins_used)
advisors = getfield.(plugins_available, :advisors) |>
Iterators.flatten |> collect |> Vector{Advice}
sort!(advisors, by = t -> t.priority)
setfield!(dta, :advisors, advisors)
setfield!(dta, :adviseall, ∘(reverse(advisors)...))
setfield!(dta, :plugins_used, getfield.(plugins_available, :name))
end
end
getfield(dta, prop)
end
AdviceAmalgamation(plugins::Vector{String}) =
AdviceAmalgamation(identity, Advice[], plugins, String[])
AdviceAmalgamation(collection::DataCollection) =
AdviceAmalgamation(collection.plugins)
AdviceAmalgamation(dta::AdviceAmalgamation) = # for re-building
AdviceAmalgamation(dta.plugins_wanted)
function AdviceAmalgamation(advisors::Vector{<:Advice})
advisors = sort(advisors, by = t -> t.priority)
AdviceAmalgamation(∘(reverse(advisors)...), advisors, String[], String[])
end
function (dta::AdviceAmalgamation)(@nospecialize(
annotated_func_call::Tuple{Function, Function, Tuple, NamedTuple}))
dta.adviseall(annotated_func_call)
end
function (dta::AdviceAmalgamation)(func::Function, args...; kwargs...)
@nospecialize func args kwargs
post::Function, func2::Function, args2::Tuple, kwargs2::NamedTuple =
dta((identity, func, args, merge(NamedTuple(), kwargs)))
invokepkglatest(func2, args2...; kwargs2...) |> post
end
# Utility functions/macros
"""
_dataadvise(thing::AdviceAmalgamation)
_dataadvise(thing::Vector{Advice})
_dataadvise(thing::Advice)
_dataadvise(thing::DataCollection)
_dataadvise(thing::DataSet)
_dataadvise(thing::AbstractDataTransformer)
Obtain the relevant `AdviceAmalgamation` for `thing`.
"""
_dataadvise(amalg::AdviceAmalgamation) = amalg
_dataadvise(advs::Vector{<:Advice}) = AdviceAmalgamation(advs)
_dataadvise(adv::Advice) = AdviceAmalgamation([adv])
_dataadvise(col::DataCollection) = col.advise
_dataadvise(ds::DataSet) = _dataadvise(ds.collection)
_dataadvise(adt::AbstractDataTransformer) = _dataadvise(adt.dataset)
"""
_dataadvisecall(func::Function, args...; kwargs...)
Identify the first data-like argument of `args` (i.e. a `DataCollection`,
`DataSet`, or `AbstractDataTransformer`), obtain its advise, and perform
an advised call of `func(args...; kwargs...)`.
"""
@generated function _dataadvisecall(func::Function, args...; kwargs...)
dataarg = findfirst(
a -> a <: DataCollection || a <: DataSet || a <: AbstractDataTransformer,
args)
if isnothing(dataarg)
@warn """Attempted to generate advised function call for $(func.instance),
however none of the provided arguments were advisable.
Arguments types: $args
This function call will not be advised."""
:(func(args...; kwargs...))
else
:(_dataadvise(args[$dataarg])(func, args...; kwargs...))
end
end
"""
@advise [source] f(args...; kwargs...)
Convert a function call `f(args...; kwargs...)` to an *advised* function call,
where the advise collection is obtained from `source` or the first data-like\\*
value of `args`.
\\* i.e. a `DataCollection`, `DataSet`, or `AbstractDataTransformer`
For example, `@advise myfunc(other, somedataset, rest...)` is equivalent to
`somedataset.collection.advise(myfunc, other, somedataset, rest...)`.
This macro performs a fairly minor code transformation, but should improve
clarity.
"""
macro advise(source::Union{Symbol, Expr}, funcall::Union{Expr, Nothing}=nothing)
# Handle @advice(funcall), and ensure `source` is correct both ways.
if isnothing(funcall)
funcall = source
source = GlobalRef(@__MODULE__, :_dataadvisecall)
else
source = Expr(:call,
GlobalRef(@__MODULE__, :_dataadvise),
source)
end
if funcall isa Symbol || funcall.head != :call
# Symbol if `source` was a symbol, and `funcall` nothing.
throw(ArgumentError("Cannot advise non-function call $funcall"))
elseif length(funcall.args) < 2
throw(ArgumentError("Cannot advise function call without arguments $funcall"))
else
args = if funcall.args[2] isa Expr && funcall.args[2].head == :parameters
vcat(funcall.args[2], funcall.args[1], funcall.args[3:end])
else funcall.args end
Expr(:escape,
Expr(:call,
source,
args...))
end
end
| DataToolkitBase | https://github.com/tecosaur/DataToolkitBase.jl.git |
|
[
"MIT"
] | 0.9.1 | ed1a12069bdb85038eb736b2a3bde5d59093eb0b | code | 1101 | """
@dataplugin plugin_variable
@dataplugin plugin_variable :default
Register the plugin given by the variable `plugin_variable`, along with its
documentation (fetched by `@doc`). Should `:default` be given as the second
argument the plugin is also added to the list of default plugins.
This effectievly serves as a minor, but appreciable, convenience for the
following pattern:
```julia
push!(PLUGINS, myplugin)
PLUGINS_DOCUMENTATION[myplugin.name] = @doc myplugin
push!(DEFAULT_PLUGINS, myplugin.name) # when also adding to defaults
```
"""
macro dataplugin(pluginvar::QuoteNode)
quote
push!(PLUGINS, $(esc(pluginvar.value)))
PLUGINS_DOCUMENTATION[$(esc(pluginvar.value)).name] = @doc($(esc(pluginvar.value)))
end
end
macro dataplugin(pluginvar::Symbol)
:(@dataplugin($(QuoteNode(esc(pluginvar)))))
end
macro dataplugin(pluginvar::Symbol, usebydefault::QuoteNode)
quote
@dataplugin($(QuoteNode(esc(pluginvar))))
$(if usebydefault.value == :default
:(push!(DEFAULT_PLUGINS, $(esc(pluginvar)).name))
end)
end
end
| DataToolkitBase | https://github.com/tecosaur/DataToolkitBase.jl.git |
|
[
"MIT"
] | 0.9.1 | ed1a12069bdb85038eb736b2a3bde5d59093eb0b | code | 19150 | abstract type IdentifierException <: Exception end
"""
UnresolveableIdentifier{T}(identifier::Union{String, UUID}, [collection::DataCollection])
No `T` (optionally from `collection`) could be found that matches `identifier`.
# Example occurrences
```julia-repl
julia> d"iirs"
ERROR: UnresolveableIdentifier: "iirs" does not match any available data sets
Did you perhaps mean to refer to one of these data sets?
■:iris (75% match)
Stacktrace: [...]
julia> d"iris::Int"
ERROR: UnresolveableIdentifier: "iris::Int" does not match any available data sets
Without the type restriction, however, the following data sets match:
dataset:iris, which is available as a DataFrame, Matrix, CSV.File
Stacktrace: [...]
```
"""
struct UnresolveableIdentifier{T, I} <: IdentifierException where {T, I <: Union{String, UUID}}
target::Type{T} # Prevent "unused type variable" warning
identifier::I
collection::Union{DataCollection, Nothing}
end
UnresolveableIdentifier{T}(ident::I, collection::Union{DataCollection, Nothing}=nothing) where {T, I <: Union{String, UUID}} =
UnresolveableIdentifier{T, I}(T, ident, collection)
function Base.showerror(io::IO, err::UnresolveableIdentifier{DataSet, String})
print(io, "UnresolveableIdentifier: ", sprint(show, err.identifier),
" does not match any available data sets")
if !isnothing(err.collection)
print(io, " in ", sprint(show, err.collection.name))
end
# Check to see if there are any matches without the
# type restriction (if applicable).
notypematches = Vector{DataSet}()
if err.identifier isa String
if !isnothing(err.collection)
ident = @advise err.collection parse_ident(err.identifier)
if !isnothing(ident.type)
identnotype = Identifier(ident.collection, ident.dataset,
nothing, ident.parameters)
notypematches = refine(
err.collection, err.collection.datasets, identnotype)
end
else
for collection in STACK
ident = @advise collection parse_ident(err.identifier)
if !isnothing(ident.type)
identnotype = Identifier(ident.collection, ident.dataset,
nothing, ident.parameters)
append!(notypematches, refine(
collection, collection.datasets, identnotype))
end
end
end
end
# If there are any no-type matches then display them,
# otherwise look for data sets with similar names.
if !isempty(notypematches)
print(io, "\n Without the type restriction, however, the following data sets match:")
for dataset in notypematches
print(io, "\n ")
show(io, MIME("text/plain"), Identifier(dataset); dataset.collection)
print(io, ", which is available as a ")
types = getfield.(dataset.loaders, :type) |> Iterators.flatten |> unique
for type in types
printstyled(io, string(type), color=:yellow)
type === last(types) || print(io, ", ")
end
end
else
candidates = Tuple{Identifier, DataCollection, Float64}[]
if !isnothing(err.collection) || !isempty(STACK)
let collection = @something(err.collection, first(STACK))
for ident in Identifier.(collection.datasets, nothing)
istr = @advise collection string(ident)
push!(candidates,
(ident, collection, stringsimilarity(err.identifier, istr; halfcase=true)))
end
end
elseif isnothing(err.collection) && !isempty(STACK)
for collection in last(Iterators.peel(STACK))
for ident in Identifier.(collection.datasets)
istr = @advise collection string(ident)
push!(candidates,
(ident, collection, stringsimilarity(err.identifier, istr; halfcase=true)))
end
end
end
maxsimilarity = maximum(last.(candidates), init=0.0)
if maxsimilarity >= 0.2
print(io, "\n Did you perhaps mean to refer to one of these data sets?")
threshold = maxsimilarity * 0.9
for (ident, collection, sim) in sort(candidates[last.(candidates) .>= threshold],
by=last, rev=true)
print(io, "\n ")
irep = IOContext(IOBuffer(), :color => true)
show(irep, MIME("text/plain"), ident; collection)
highlight_lcs(io, String(take!(irep.io)), err.identifier,
before="\e[2m", invert=true)
printstyled(io, " (", round(Int, 100*sim), "% match)",
color=:light_black)
end
end
end
end
function Base.showerror(io::IO, err::UnresolveableIdentifier{DataCollection})
print(io, "UnresolveableCollection: No collections within the stack matched the ",
ifelse(err.identifier isa UUID, "identifier ", "name "), string(err.identifier))
if err.identifier isa String
candidates = Tuple{DataCollection, Float64}[]
for collection in STACK
if !isnothing(collection.name)
push!(candidates,
(collection, stringsimilarity(err.identifier, collection.name; halfcase=true)))
end
end
if maximum(last.(candidates), init=0.0) >= 0.5
print(io, "\n Did you perhaps mean to refer to one of these data collections?")
for (collection, similarity) in candidates
print(io, "\n • ")
crep = IOContext(IOBuffer(), :color => true, :compact => true)
show(crep, MIME("text/plain"), collection)
highlight_lcs(io, String(take!(crep.io)), err.identifier,
before="\e[2m", invert=true)
printstyled(io, " (", round(Int, 100*similarity), "% similarity)",
color=:light_black)
end
end
end
end
"""
AmbiguousIdentifier(identifier::Union{String, UUID}, matches::Vector, [collection])
Searching for `identifier` (optionally within `collection`), found multiple
matches (provided as `matches`).
# Example occurrence
```julia-repl
julia> d"multimatch"
ERROR: AmbiguousIdentifier: "multimatch" matches multiple data sets
■:multimatch [45685f5f-e6ff-4418-aaf6-084b847236a8]
■:multimatch [92be4bda-55e9-4317-aff4-8d52ee6a5f2c]
Stacktrace: [...]
```
"""
struct AmbiguousIdentifier{T, I} <: IdentifierException where {T, I <: Union{String, UUID}}
identifier::I
matches::Vector{T}
collection::Union{DataCollection, Nothing}
end
AmbiguousIdentifier(identifier::Union{String, UUID}, matches::Vector{T}) where {T} =
AmbiguousIdentifier{T}(identifier, matches, nothing)
function Base.showerror(io::IO, err::AmbiguousIdentifier{DataSet, I}) where {I}
print(io, "AmbiguousIdentifier: ", sprint(show, err.identifier),
" matches multiple data sets")
if I == String
for dataset in err.matches
ident = Identifier(dataset, ifelse(err.collection === dataset.collection,
nothing, :name))
print(io, "\n ")
show(io, MIME("text/plain"), ident; collection=dataset.collection)
printstyled(io, " [", dataset.uuid, ']', color=:light_black)
end
else
print(io, ". There is likely some kind of accidental ID duplication occurring.")
end
end
function Base.showerror(io::IO, err::AmbiguousIdentifier{DataCollection})
print(io, "AmbiguousIdentifier: ", sprint(show, err.identifier),
" matches multiple data collections in the stack")
if I == String
for collection in err.matches
print(io, "\n • ")
printstyled(io, collection.name, color=:magenta)
printstyled(io, " [", collection.uuid, "]", color=:light_magenta)
end
print(io, "\n Consider referring to the data collection by UUID instead of name.")
else
print(io, ". Have you loaded the same data collection twice?")
end
end
abstract type PackageException <: Exception end
"""
UnregisteredPackage(pkg::Symbol, mod::Module)
The package `pkg` was asked for within `mod`, but has not been
registered by `mod`, and so cannot be loaded.
# Example occurrence
```julia-repl
julia> @import Foo
ERROR: UnregisteredPackage: Foo has not been registered by Main, see @addpkg for more information
Stacktrace: [...]
```
"""
struct UnregisteredPackage <: PackageException
pkg::Symbol
mod::Module
end
function Base.showerror(io::IO, err::UnregisteredPackage)
print(io, "UnregisteredPackage: ", err.pkg,
" has not been registered by ", err.mod)
project_deps = let proj_file = if isnothing(pathof(err.mod)) # Main, etc.
Base.active_project()
else abspath(pathof(err.mod), "..", "..", "Project.toml") end
if isfile(proj_file)
Dict{String, Base.UUID}(
pkg => Base.UUID(id)
for (pkg, id) in get(Base.parsed_toml(proj_file),
"deps", Dict{String, Any}()))
else
Dict{String, Base.UUID}()
end
end
dtk = "DataToolkit" => Base.UUID("dc83c90b-d41d-4e55-bdb7-0fc919659999")
has_dtk = dtk in project_deps
print(io, ", see ",
ifelse(has_dtk, "@addpkg", "addpkg"),
" for more information")
if haskey(project_deps, String(err.pkg))
println(io, "\n The package is present as a dependency of $(err.mod), and so this issue can likely be fixed by invoking:")
if has_dtk
print(io, " @addpkg $(err.pkg)")
else
print(io, " addpkg($(err.mod), :$(err.pkg), $(sprint(show, project_deps[String(err.pkg)])))")
end
else
print(io, " (it is also worth noting that the package does not seem to be present as a dependency of $(err.mod))")
end
end
"""
MissingPackage(pkg::Base.PkgId)
The package `pkg` was asked for, but does not seem to be available in the
current environment.
# Example occurrence
```julia-repl
julia> @addpkg Bar "00000000-0000-0000-0000-000000000000"
Bar [00000000-0000-0000-0000-000000000000]
julia> @import Bar
[ Info: Lazy-loading Bar [00000000-0000-0000-0000-000000000001]
ERROR: MissingPackage: Bar [00000000-0000-0000-0000-000000000001] has been required, but does not seem to be installed.
Stacktrace: [...]
```
"""
struct MissingPackage <: PackageException
pkg::Base.PkgId
end
Base.showerror(io::IO, err::MissingPackage) =
print(io, "MissingPackage: ", err.pkg.name, " [", err.pkg.uuid,
"] has been required, but does not seem to be installed.")
abstract type DataOperationException <: Exception end
"""
CollectionVersionMismatch(version::Int)
The `version` of the collection currently being acted on is not supported
by the current version of $(@__MODULE__).
# Example occurrence
```julia-repl
julia> fromspec(DataCollection, SmallDict{String, Any}("data_config_version" => -1))
ERROR: CollectionVersionMismatch: -1 (specified) ≠ $LATEST_DATA_CONFIG_VERSION (current)
The data collection specification uses the v-1 data collection format, however
the installed DataToolkitBase version expects the v$LATEST_DATA_CONFIG_VERSION version of the format.
In the future, conversion facilities may be implemented, for now though you
will need to manually upgrade the file to the v$LATEST_DATA_CONFIG_VERSION format.
Stacktrace: [...]
```
"""
struct CollectionVersionMismatch <: DataOperationException
version::Int
end
function Base.showerror(io::IO, err::CollectionVersionMismatch)
print(io, "CollectionVersionMismatch: ", err.version, " (specified) ≠ ",
LATEST_DATA_CONFIG_VERSION, " (current)\n")
print(io, " The data collection specification uses the v$(err.version) data collection format, however\n",
" the installed $(@__MODULE__) version expects the v$LATEST_DATA_CONFIG_VERSION version of the format.\n",
" In the future, conversion facilities may be implemented, for now though you\n will need to ",
ifelse(err.version < LATEST_DATA_CONFIG_VERSION,
"manually upgrade the file to the v$LATEST_DATA_CONFIG_VERSION format.",
"use a newer version of $(@__MODULE__)."))
end
"""
EmptyStackError()
An attempt was made to perform an operation on a collection within the data
stack, but the data stack is empty.
# Example occurrence
```julia-repl
julia> getlayer(nothing) # with an empty STACK
ERROR: EmptyStackError: The data collection stack is empty
Stacktrace: [...]
```
"""
struct EmptyStackError <: DataOperationException end
Base.showerror(io::IO, err::EmptyStackError) =
print(io, "EmptyStackError: The data collection stack is empty")
"""
ReadonlyCollection(collection::DataCollection)
Modification of `collection` is not viable, as it is read-only.
# Example Occurrence
```julia-repl
julia> lockedcollection = DataCollection(SmallDict{String, Any}("uuid" => Base.UUID(rand(UInt128)), "config" => SmallDict{String, Any}("locked" => true)))
julia> write(lockedcollection)
ERROR: ReadonlyCollection: The data collection unnamed#298 is locked
Stacktrace: [...]
```
"""
struct ReadonlyCollection <: DataOperationException
collection::DataCollection
end
Base.showerror(io::IO, err::ReadonlyCollection) =
print(io, "ReadonlyCollection: The data collection ", err.collection.name,
" is ", ifelse(get(err.collection, "locked", false) === true,
"locked", "backed by a read-only file"))
"""
TransformerError(msg::String)
A catch-all for issues involving data transformers, with details given in `msg`.
# Example occurrence
```julia-repl
julia> emptydata = DataSet(DataCollection(), "empty", SmallDict{String, Any}("uuid" => Base.UUID(rand(UInt128))))
DataSet empty
julia> read(emptydata)
ERROR: TransformerError: Data set "empty" could not be loaded in any form.
Stacktrace: [...]
```
"""
struct TransformerError <: DataOperationException
msg::String
end
Base.showerror(io::IO, err::TransformerError) =
print(io, "TransformerError: ", err.msg)
"""
UnsatisfyableTransformer{T}(dataset::DataSet, types::Vector{QualifiedType})
A transformer (of type `T`) that could provide any of `types` was asked for, but
there is no transformer that satisfies this restriction.
# Example occurrence
```julia-repl
julia> emptydata = DataSet(DataCollection(), "empty", SmallDict{String, Any}("uuid" => Base.UUID(rand(UInt128))))
DataSet empty
julia> read(emptydata, String)
ERROR: UnsatisfyableTransformer: There are no loaders for "empty" that can provide a String. The defined loaders are as follows:
Stacktrace: [...]
```
"""
struct UnsatisfyableTransformer{T} <: DataOperationException where { T <: AbstractDataTransformer }
dataset::DataSet
transformer::Type{T}
wanted::Vector{QualifiedType}
end
function Base.showerror(io::IO, err::UnsatisfyableTransformer)
transformer_type = lowercase(replace(string(nameof(err.transformer)), "Data" => ""))
print(io, "UnsatisfyableTransformer: There are no $(transformer_type)s for ",
sprint(show, err.dataset.name), " that can provide a ",
join(string.(err.wanted), ", ", ", or "),
".\n The defined $(transformer_type)s are as follows:")
transformers = if err.transformer isa DataLoader
err.dataset.loaders
else
err.dataset.storage
end
for transformer in transformers
print(io, "\n ")
show(io, transformer)
end
end
"""
OrphanDataSet(dataset::DataSet)
The data set (`dataset`) is no longer a child of its parent collection.
This error should not occur, and is intended as a sanity check should
something go quite wrong.
"""
struct OrphanDataSet <: DataOperationException
dataset::DataSet
end
function Base.showerror(io::IO, err::OrphanDataSet)
print(io, "OrphanDataSet: The data set ", err.dataset.name,
" [", err.dataset.uuid, "] is no longer a child of of its parent collection.\n",
"This should not occur, and indicates that something fundamental has gone wrong.")
end
"""
ImpossibleTypeException(qt::QualifiedType, mod::Union{Module, Nothing})
The qualified type `qt` could not be converted to a `Type`, for some reason or
another (`mod` is the parent module used in the attempt, should it be successfully
identified, and `nothing` otherwise).
"""
struct ImpossibleTypeException <: Exception
qt::QualifiedType
mod::Union{Module, Nothing}
end
function Base.showerror(io::IO, err::ImpossibleTypeException)
print(io, "ImpossibleTypeException: Could not realise the type ", string(err.qt))
if isnothing(err.mod)
print(io, ", as the parent module ", err.qt.root,
" is not loaded.")
elseif !isdefined(err.mod, err.qt.name)
print(io, ", as the parent module ", err.qt.root,
" has no property ", err.qt.name, '.')
else
print(io, ", for unknown reasons, possibly an issue with the type parameters?")
end
end
struct InvalidParameterType{T <: Union{<:AbstractDataTransformer, DataSet, DataCollection}}
thing::T
parameter::String
type::Type
end
function Base.showerror(io::IO, err::InvalidParameterType{<:AbstractDataTransformer})
print(io, "InvalidParameterType: '", err.parameter, "' parameter of ",
err.thing.dataset.name, "'s ", nameof(typeof(err.thing)),
"{:", string(first(typeof(err.thing).parameters)), "} must be a ",
string(err.type), " not a ",
string(typeof(get(err.thing, err.parameter))), ".")
end
function Base.showerror(io::IO, err::InvalidParameterType)
print(io, "InvalidParameterType: '", err.parameter, "' parameter of ",
string(err.thing), " must be a ", string(err.type), " not a ",
string(typeof(get(err.thing, err.parameter))), ".")
end
macro getparam(expr::Expr, default=nothing)
thing, type = if Meta.isexpr(expr, :(::)) expr.args else (expr, :Any) end
Meta.isexpr(thing, :.) || error("Malformed expression passed to @getparam")
root, param = thing.args
if isnothing(default)
typename = if type isa Symbol type
elseif Meta.isexpr(type, :curly) first(type.args)
else :Any end
default = if typename ∈ (:Vector, :Dict, :SmallDict)
:($type())
else :nothing end
end
if type == :Any
:(get($(esc(root)), $(esc(param)), $(esc(default))))
else
quote
let value = get($(esc(root)), $(esc(param)), $(esc(default)))
if !(value isa $(esc(type)))
throw(InvalidParameterType(
$(esc(root)), $(esc(param)), $(esc(type))))
end
value
end
end
end
end
| DataToolkitBase | https://github.com/tecosaur/DataToolkitBase.jl.git |
|
[
"MIT"
] | 0.9.1 | ed1a12069bdb85038eb736b2a3bde5d59093eb0b | code | 5190 | """
The `DataCollection.version` set on all created `DataCollection`s, and assumed
when reading any Data.toml files which do not set `data_config_version`.
"""
const LATEST_DATA_CONFIG_VERSION = 0 # while in alpha
"""
The set of data collections currently available.
"""
const STACK = DataCollection[]
"""
The set of plugins currently available.
"""
const PLUGINS = Plugin[]
"""
A mapping from Plugin names to the documentation of said plugin.
"""
const PLUGINS_DOCUMENTATION = Dict{String, Any}()
"""
The set of plugins (by name) that should used by default when creating a
new data collection.
"""
const DEFAULT_PLUGINS = String[]
"""
The set of packages loaded by each module via `@addpkg`, for import with `@import`.
More specifically, when a module M invokes `@addpkg pkg id` then
`EXTRA_PACKAGES[M][pkg] = id` is set, and then this information is used
with `@import` to obtain the package from the root module.
"""
const EXTRA_PACKAGES = Dict{Module, Dict{Symbol, Base.PkgId}}()
# For use in construction
"""
The default `priority` field value for instances of `AbstractDataTransformer`.
"""
const DEFAULT_DATATRANSFORMER_PRIORITY = 1
"""
The default `priority` field value for `Advice`s.
"""
const DEFAULT_DATA_ADVISOR_PRIORITY = 1
"""
A tuple of delimiters defining a dataset reference.
For example, if set to `("{", "}")` then `{abc}` would
be recognised as a dataset reference for `abc`.
"""
const DATASET_REFERENCE_WRAPPER = ("📇DATASET<<", ">>")
"""
A regex which matches dataset references.
This is constructed from `DATASET_REFERENCE_WRAPPER`.
"""
const DATASET_REFERENCE_REGEX =
Regex(string("^", DATASET_REFERENCE_WRAPPER[1],
"(.+)", DATASET_REFERENCE_WRAPPER[2],
"\$"))
# For plugins / general information
"""
The data specification TOML format constructs a DataCollection, which itself
contains DataSets, comprised of metadata and AbstractDataTransformers.
```
DataCollection
├─ DataSet
│ ├─ AbstractDataTransformer
│ └─ AbstractDataTransformer
├─ DataSet
⋮
```
Within each scope, there are certain reserved attributes. They are listed in
this Dict under the following keys:
- `:collection` for `DataCollection`
- `:dataset` for `DataSet`
- `:transformer` for `AbstractDataTransformer`
"""
const DATA_CONFIG_RESERVED_ATTRIBUTES =
Dict(:collection => ["data_config_version", "name", "uuid", "plugins", "config"],
:dataset => ["uuid", "storage", "loader", "writer"],
:transformer => ["driver", "type", "priority"])
"""
When writing data configuration TOML file, the keys are (recursively) sorted.
Some keys are particularly important though, and so to ensure they are placed
higher a mappings from such keys to a higher sort priority string can be
registered here.
For example, `"config" => "\0x01"` ensures that the special configuration
section is placed before all of the data sets.
This can cause odd behaviour if somebody gives a dataset the same name as a
special key, but frankly that would be a bit silly (given the key names, e.g.
"uuid") and so this is of minimal concern.
"""
const DATA_CONFIG_KEY_SORT_MAPPING =
Dict("config" => "\0x01",
"data_config_version" => "\0x01",
"uuid" => "\0x02",
"name" => "\0x03",
"driver" => "\0x03",
"description" => "\0x04",
"storage" => "\0x05",
"loader" => "\0x06",
"writer" => "\0x07")
# Linting
"""
A mapping from severity symbols to integers.
This is used to assist with more readable construction of `LintItem`s.
"""
const LINT_SEVERITY_MAPPING =
Dict(:debug => 0x05,
:info => 0x04,
:suggestion => 0x03,
:warning => 0x02,
:error => 0x01)
"""
A mapping from severity numbers (see `LINT_SEVERITY_MAPPING`) to a tuple
giving the color the message should be accented with and the severity
title string.
"""
const LINT_SEVERITY_MESSAGES =
Dict(0x01 => (:red, "Error"),
0x02 => (:yellow, "Warning"),
0x03 => (:light_yellow, "Suggestion"),
0x04 => (:light_blue, "Info"),
0x05 => (:light_black, "Debug"))
# REPL things
"""
The key that is used to enter the data REPL.
"""
const REPL_KEY = '}'
"""
A symbol identifying the Data REPL. This is used in a few places,
such as the command history.
"""
const REPL_NAME = :data_toolkit
"""
The REPL prompt shown.
"""
const REPL_PROMPT = "data>"
"""
An ANSI control sequence string that sets the style of the "$REPL_PROMPT"
REPL prompt.
"""
const REPL_PROMPTSTYLE = Base.text_colors[:magenta]
"""
The color that should be used for question text presented in a REPL context.
This should be a symbol present in `Base.text_colors`.
"""
const REPL_QUESTION_COLOR = :light_magenta
"""
The color that should be set for user response text in a REPL context.
This should be a symbol present in `Base.text_colors`.
"""
const REPL_USER_INPUT_COLOUR = :light_yellow
"""
The set of commands available directly in the Data REPL.
"""
const REPL_CMDS = ReplCmd[]
"""
List of `(category::Symbol, named::Symbol) => docs::Any` forms.
`category` can be `:storage`, `:loader`, or `:writer`.
"""
const TRANSFORMER_DOCUMENTATION = Pair{Tuple{Symbol, Symbol}, Any}[]
| DataToolkitBase | https://github.com/tecosaur/DataToolkitBase.jl.git |
|
[
"MIT"
] | 0.9.1 | ed1a12069bdb85038eb736b2a3bde5d59093eb0b | code | 7830 | Identifier(ident::Identifier, params::SmallDict{String, Any}; replace::Bool=false) =
Identifier(ident.collection, ident.dataset, ident.type,
if replace || isempty(ident.parameters);
params
else
merge(ident.parameters, params)
end)
Identifier(ident::Identifier, ::Nothing; replace::Bool=false) =
if replace
Identifier(ident, SmallDict{String, Any}(); replace)
else
ident
end
"""
Identifier(dataset::DataSet, collection::Union{Symbol, Nothing}=:name,
name::Symbol=something(collection, :name))
Create an `Identifier` referring to `dataset`, specifying the collection
`dataset` comes from as well (when `collection` is not `nothing`) as all of its
parameters, but without any type information.
Should `collection` and `name` default to the symbol `:name`, which signals that
the collection and dataset reference of the generated `Identifier` should use
the *name*s of the collection/dataset. If set to `:uuid`, the UUID is used
instead. No other value symbols are supported.
"""
function Identifier(ds::DataSet, collection::Union{Symbol, Nothing}=:name,
name::Symbol=something(collection, :name))
Identifier(
if collection == :uuid
ds.collection.uuid
elseif collection == :name
ds.collection.name
elseif isnothing(collection)
else
throw(ArgumentError("collection argument must be :uuid, :name, or nothing — not $collection"))
end,
if name == :uuid
ds.uuid
elseif name == :name
ds.name
else
throw(ArgumentError("name argument must be :uuid or :name — not $name"))
end,
nothing,
ds.parameters)
end
# Identifier(spec::AbstractString) = parse(Identifier, spec)
Identifier(spec::AbstractString, params::SmallDict{String, Any}) =
Identifier(parse(Identifier, spec), params)
Base.:(==)(a::Identifier, b::Identifier) =
getfield.(Ref(a), fieldnames(Identifier)) ==
getfield.(Ref(b), fieldnames(Identifier))
function Base.string(ident::Identifier)
string(if !isnothing(ident.collection)
string(ident.collection, ':')
else "" end,
ident.dataset,
if !isnothing(ident.type)
"::" * string(ident.type)
else "" end)
end
"""
resolve(collection::DataCollection, ident::Identifier;
resolvetype::Bool=true, requirematch::Bool=true)
Attempt to resolve an identifier (`ident`) to a particular data set.
Matching data sets will searched for from `collection`.
When `resolvetype` is set and `ident` specifies a datatype, the identified data
set will be read to that type.
When `requirematch` is set an error is raised should no dataset match `ident`.
Otherwise, `nothing` is returned.
"""
function resolve(collection::DataCollection, ident::Identifier;
resolvetype::Bool=true, requirematch::Bool=true)
collection_mismatch = !isnothing(ident.collection) &&
if ident.collection isa UUID
collection.uuid != ident.collection
else
collection.name != ident.collection
end
if collection_mismatch
return resolve(getlayer(ident.collection), ident)
end
matchingdatasets = refine(collection, collection.datasets, ident)
if length(matchingdatasets) == 1
dataset = first(matchingdatasets)
if !isnothing(ident.type) && resolvetype
read(dataset, typeify(ident.type, mod=collection.mod, shoulderror=true))
else
dataset
end
elseif length(matchingdatasets) == 0 && requirematch
notypeident = Identifier(ident.collection, ident.dataset, nothing, ident.parameters)
notypematches = refine(collection, collection.datasets, notypeident)
if !isempty(notypematches)
throw(UnsatisfyableTransformer(first(notypematches), DataLoader, ident.type))
else
throw(UnresolveableIdentifier{DataSet}(string(notypeident), collection))
end
elseif length(matchingdatasets) > 1
throw(AmbiguousIdentifier((@advise collection string(ident)),
matchingdatasets, collection))
end
end
"""
refine(collection::DataCollection, datasets::Vector{DataSet}, ident::Identifier)
Filter `datasets` (from `collection`) to data sets than match the identifier `ident`.
This function contains an advise entrypoint where plugins can apply further filtering,
applied to the method `refine(::Vector{DataSet}, ::Identifier, ::Vector{String})`.
"""
function refine(collection::DataCollection, datasets::Vector{DataSet}, ident::Identifier)
filter_nameid(datasets) =
if ident.dataset isa UUID
filter(d -> d.uuid == ident.dataset, datasets)
else
filter(d -> d.name == ident.dataset, datasets)
end
filter_type(datasets) =
if isnothing(ident.type)
datasets
else
filter(d -> any(l -> any(t -> ⊆(t, ident.type, mod=collection.mod), l.type),
d.loaders), datasets)
end
filter_parameters(datasets, ignore) =
filter(datasets) do d
all((param, value)::Pair ->
param in ignore || get(d, param) == value,
ident.parameters)
end
matchingdatasets = datasets |> filter_nameid |> filter_type
matchingdatasets, ignoreparams =
@advise collection refine(matchingdatasets, ident, String[])
filter_parameters(matchingdatasets, ignoreparams)
end
"""
refine(datasets::Vector{DataSet}, ::Identifier, ignoreparams::Vector{String})
This is a stub function that exists soley as as an advise point for data set
filtering during resolution of an identifier.
"""
refine(datasets::Vector{DataSet}, ::Identifier, ignoreparams::Vector{String}) =
(datasets, ignoreparams)
"""
resolve(ident::Identifier; resolvetype::Bool=true, stack=STACK)
Attempt to resolve `ident` using the specified data layer, if present, trying
every layer of the data stack in turn otherwise.
"""
resolve(ident::Identifier; resolvetype::Bool=true, stack::Vector{DataCollection}=STACK) =
if !isnothing(ident.collection)
resolve(getlayer(ident.collection), ident; resolvetype)
else
for collection in stack
result = resolve(collection, ident; resolvetype, requirematch=false)
if !isnothing(result)
return result
end
end
throw(UnresolveableIdentifier{DataSet}(string(ident)))
end
"""
resolve(identstr::AbstractString, parameters::Union{SmallDict{String, Any}, Nothing}=nothing;
resolvetype::Bool=true, stack::Vector{DataCollection}=STACK)
Attempt to resolve the identifier given by `identstr` and `parameters` against
each layer of the data `stack` in turn.
"""
function resolve(identstr::AbstractString, parameters::Union{SmallDict{String, Any}, Nothing}=nothing;
resolvetype::Bool=true, stack::Vector{DataCollection}=STACK)
isempty(stack) && throw(EmptyStackError())
if (cname = parse(Identifier, identstr).collection) |> !isnothing
collection = getlayer(cname)
ident = Identifier((@advise collection parse_ident(identstr)),
parameters)
resolve(collection, ident; resolvetype)
else
for collection in stack
ident = Identifier((@advise collection parse_ident(identstr)),
parameters)
result = resolve(collection, ident; resolvetype, requirematch=false)
!isnothing(result) && return result
end
throw(UnresolveableIdentifier{DataSet}(String(identstr)))
end
end
| DataToolkitBase | https://github.com/tecosaur/DataToolkitBase.jl.git |
|
[
"MIT"
] | 0.9.1 | ed1a12069bdb85038eb736b2a3bde5d59093eb0b | code | 3484 | """
dataset_parameters(source::Union{DataCollection, DataSet, AbstractDataTransformer},
action::Val{:extract|:resolve|:encode}, value::Any)
Obtain a form (depending on `action`) of `value`, a property within `source`.
## Actions
**`:extract`** Look for DataSet references ("$(DATASET_REFERENCE_WRAPPER[1])...$(DATASET_REFERENCE_WRAPPER[2])") within
`value`, and turn them into `Identifier`s (the inverse of `:encode`).
**`:resolve`** Look for `Identifier`s in `value`, and resolve them to the
referenced DataSet/value.
**`:encode`** Look for `Identifier`s in `value`, and turn them into DataSet references
(the inverse of `:extract`).
"""
function dataset_parameters(collection::DataCollection, action::Val, params::SmallDict{String,Any})
SmallDict{String, Any}(
keys(params) |> collect,
[dataset_parameters(collection, action, value)
for value in values(params)])
end
function dataset_parameters(collection::DataCollection, action::Val, param::Vector)
map(value -> dataset_parameters(collection, action, value), param)
end
dataset_parameters(::DataCollection, ::Val, value::Any) = value
dataset_parameters(dataset::DataSet, action::Val, params::Any) =
dataset_parameters(dataset.collection, action, params)
dataset_parameters(adt::AbstractDataTransformer, action::Val, params::Any) =
dataset_parameters(adt.dataset.collection, action, params)
function dataset_parameters(collection::DataCollection, ::Val{:extract}, param::String)
dsid_match = match(DATASET_REFERENCE_REGEX, param)
if !isnothing(dsid_match)
@advise collection parse_ident(dsid_match.captures[1])
else
param
end
end
function dataset_parameters(collection::DataCollection, ::Val{:resolve}, param::Identifier)
resolve(collection, param)
end
function dataset_parameters(collection::DataCollection, ::Val{:encode}, param::Identifier)
string(DATASET_REFERENCE_WRAPPER[1],
(@advise collection string(param)),
DATASET_REFERENCE_WRAPPER[2])
end
function Base.get(dataobj::Union{DataSet, DataCollection, <:AbstractDataTransformer},
property::AbstractString, default=nothing)
if haskey(dataobj.parameters, property)
dataset_parameters(dataobj, Val(:resolve), dataobj.parameters[property])
else
default
end
end
Base.get(dataobj::Union{DataSet, DataCollection, <:AbstractDataTransformer},
::typeof(:)) =
dataset_parameters(dataobj, Val(:resolve), dataobj.parameters)
# Nice extra
function referenced_datasets(dataset::DataSet)
dataset_references = Identifier[]
add_dataset_refs!(dataset_references, dataset.parameters)
for paramsource in vcat(dataset.storage, dataset.loaders, dataset.writers)
add_dataset_refs!(dataset_references, paramsource)
end
map(r -> resolve(dataset.collection, r, resolvetype=false), dataset_references) |> unique!
end
add_dataset_refs!(acc::Vector{Identifier}, @nospecialize(adt::AbstractDataTransformer)) =
add_dataset_refs!(acc, adt.parameters)
add_dataset_refs!(acc::Vector{Identifier}, props::SmallDict) =
for val in values(props)
add_dataset_refs!(acc, val)
end
add_dataset_refs!(acc::Vector{Identifier}, props::Vector) =
for val in props
add_dataset_refs!(acc, val)
end
add_dataset_refs!(acc::Vector{Identifier}, ident::Identifier) =
push!(acc, ident)
add_dataset_refs!(::Vector{Identifier}, ::Any) = nothing
| DataToolkitBase | https://github.com/tecosaur/DataToolkitBase.jl.git |
|
[
"MIT"
] | 0.9.1 | ed1a12069bdb85038eb736b2a3bde5d59093eb0b | code | 11645 | # ---------------
# QualifiedType
# ---------------
function Base.parse(::Type{QualifiedType}, spec::AbstractString)
if haskey(QUALIFIED_TYPE_SHORTHANDS.forward, spec)
return QUALIFIED_TYPE_SHORTHANDS.forward[spec]
end
components, parameters = let cbsplit = split(spec, '{', limit=2)
function destruct(param)
if param isa Number
param
elseif param isa QuoteNode
param.value
elseif param isa Expr && param.head == :tuple
Tuple(destruct.(param.args))
elseif param isa Symbol
if haskey(QUALIFIED_TYPE_SHORTHANDS.forward, string(param))
QUALIFIED_TYPE_SHORTHANDS.forward[string(param)]
else
QualifiedType(Symbol(Base.binding_module(Main, param)),
Symbol[], param, Tuple{}())
end
elseif Meta.isexpr(param, :.)
parse(QualifiedType, string(param))
elseif Meta.isexpr(param, :<:) && last(param.args) isa Symbol
TypeVar(if length(param.args) == 2
first(param.args)
else Symbol("#s0") end,
getfield(Main, last(param.args)))
elseif Meta.isexpr(param, :<:) && (val = typeify(parse(QualifiedType, string(last(param.args))))) |> !isnothing
TypeVar(if length(param.args) == 2
first(param.args)
else Symbol("#s0") end,
val)
elseif Meta.isexpr(param, :curly)
base = parse(QualifiedType, string(first(param.args)))
QualifiedType(base.root, Symbol[], base.name, Tuple(destruct.(param.args[2:end])))
else
throw(ArgumentError("Invalid QualifiedType parameter $(sprint(show, param)) in $(sprint(show, spec))"))
end
end
if length(cbsplit) == 1
split(cbsplit[1], '.'), Tuple{}()
else
typeparams = Meta.parse(spec[1+length(cbsplit[1]):end])
split(cbsplit[1], '.'), Tuple(destruct.(typeparams.args))
end
end
root, parents, name = if length(components) == 1
n = Symbol(components[1])
Symbol(Base.binding_module(Main, n)), Symbol[], n
elseif length(components) == 2
Symbol(components[1]), Symbol[], Symbol(components[2])
else
Symbol(components[1]), Symbol.(components[2:end-1]), Symbol(components[end])
end
QualifiedType(root, parents, name, parameters)
end
# ---------------
# Identifier
# ---------------
function Base.parse(::Type{Identifier}, spec::AbstractString)
isempty(STACK) && return parse_ident(spec)
mark = findfirst(':', spec)
collection = if !isnothing(mark) && (mark == length(spec) || spec[mark+1] != ':')
cstring = spec[1:prevind(spec, mark)]
something(tryparse(UUID, cstring), cstring)
end
@advise getlayer(collection) parse_ident(spec)
end
function parse_ident(spec::AbstractString)
mark = findfirst(':', spec)
collection = if !isnothing(mark) && (mark == length(spec) || spec[mark+1] != ':')
cstring, spec = spec[begin:prevind(spec, mark)], spec[mark+1:end]
something(tryparse(UUID, cstring), cstring)
end
mark = findfirst(':', spec)
dataset = if isnothing(mark)
_, spec = spec, ""
else
_, spec = spec[begin:prevind(spec, mark)], spec[mark:end]
end |> first
dtype = if startswith(spec, "::") && length(spec) > 2
parse(QualifiedType, spec[3:end])
end
Identifier(collection, something(tryparse(UUID, dataset), dataset),
dtype, SmallDict{String,Any}())
end
# ---------------
# DataTransformers
# ---------------
"""
supportedtypes(ADT::Type{<:AbstractDataTransformer})::Vector{QualifiedType}
Return a list of types supported by the data transformer `ADT`.
This is used as the default value for the `type` key in the Data TOML.
The list of types is dynamically generated based on the available methods for
the data transformer.
In some cases, it makes sense for this to be explicitly defined for a particular
transformer. """
function supportedtypes end # See `interaction/externals.jl` for method definitions.
supportedtypes(ADT::Type{<:AbstractDataTransformer}, spec::SmallDict{String, Any}, _::DataSet) =
supportedtypes(ADT, spec)
supportedtypes(ADT::Type{<:AbstractDataTransformer}, _::SmallDict{String, Any}) =
supportedtypes(ADT)
(ADT::Type{<:AbstractDataTransformer})(dataset::DataSet, spec::Dict{String, Any}) =
@advise fromspec(ADT, dataset, spec)
(ADT::Type{<:AbstractDataTransformer})(dataset::DataSet, spec::String) =
ADT(dataset, Dict{String, Any}("driver" => spec))
"""
fromspec(ADT::Type{<:AbstractDataTransformer}, dataset::DataSet, spec::Dict{String, Any})
Create an `ADT` of `dataset` according to `spec`.
`ADT` can either contain the driver name as a type parameter, or it will be read
from the `"driver"` key in `spec`.
"""
function fromspec(ADT::Type{<:AbstractDataTransformer}, dataset::DataSet, spec::Dict{String, Any})
parameters = smallify(spec)
driver = if ADT isa DataType
first(ADT.parameters)
elseif haskey(parameters, "driver")
Symbol(lowercase(parameters["driver"]))
else
@warn "$ADT for $(sprint(show, dataset.name)) has no driver!"
:MISSING
end
if !(ADT isa DataType)
ADT = ADT{driver}
end
ttype = let spec_type = get(parameters, "type", nothing)
if isnothing(spec_type)
supportedtypes(ADT, parameters, dataset)
elseif spec_type isa Vector
parse.(QualifiedType, spec_type)
elseif spec_type isa String
[parse(QualifiedType, spec_type)]
else
@warn "Invalid ADT type '$spec_type', ignoring"
end
end
if isempty(ttype)
@warn """Could not find any types that $ADT of $(sprint(show, dataset.name)) supports.
Consider adding a 'type' parameter."""
end
priority = get(parameters, "priority", DEFAULT_DATATRANSFORMER_PRIORITY)
delete!(parameters, "driver")
delete!(parameters, "type")
delete!(parameters, "priority")
@advise dataset identity(
ADT(dataset, ttype, priority,
dataset_parameters(dataset, Val(:extract), parameters)))
end
# function (ADT::Type{<:AbstractDataTransformer})(collection::DataCollection, spec::Dict{String, Any})
# @advise fromspec(ADT, collection, spec)
# end
DataStorage{driver}(dataset::Union{DataSet, DataCollection},
type::Vector{<:QualifiedType}, priority::Int,
parameters::SmallDict{String, Any}) where {driver} =
DataStorage{driver, typeof(dataset)}(dataset, type, priority, parameters)
# ---------------
# DataCollection
# ---------------
DataCollection(name::Union{String, Nothing}=nothing; path::Union{String, Nothing}=nothing) =
DataCollection(LATEST_DATA_CONFIG_VERSION, name, uuid4(), String[],
SmallDict{String, Any}(), DataSet[], path,
AdviceAmalgamation(String[]), Main)
function DataCollection(spec::Dict{String, Any}; path::Union{String, Nothing}=nothing, mod::Module=Base.Main)
plugins::Vector{String} = get(get(spec, "config", Dict("config" => Dict())), "plugins", String[])
AdviceAmalgamation(plugins)(fromspec, DataCollection, spec; path, mod)
end
"""
fromspec(::Type{DataCollection}, spec::Dict{String, Any};
path::Union{String, Nothing}=nothing, mod::Module=Base.Main)
Create a `DataCollection` from `spec`.
The `path` and `mod` keywords are used as the values for the corresponding
fields in the DataCollection.
"""
function fromspec(::Type{DataCollection}, spec::Dict{String, Any};
path::Union{String, Nothing}=nothing, mod::Module=Base.Main)
version = get(spec, "data_config_version", LATEST_DATA_CONFIG_VERSION)
if version != LATEST_DATA_CONFIG_VERSION
throw(CollectionVersionMismatch(version))
end
name = @something(get(spec, "name", nothing),
if !isnothing(path)
toml_name = path |> basename |> splitext |> first
if toml_name != "Data"
toml_name
else
basename(dirname(path))
end
end,
string(gensym("unnamed"))[3:end])
uuid = UUID(@something get(spec, "uuid", nothing) begin
@info "Data collection '$(something(name, "<unnamed>"))' had no UUID, one has been generated."
uuid4()
end)
plugins::Vector{String} = get(spec, "plugins", String[])
parameters = get(spec, "config", Dict{String, Any}()) |> smallify
unavailable_plugins = setdiff(plugins, getproperty.(PLUGINS, :name))
if length(unavailable_plugins) > 0
@warn string("The ", join(unavailable_plugins, ", ", ", and "),
" plugin", if length(unavailable_plugins) == 1
" is" else "s are" end,
" not available at the time of loading '$name'.",
"\n It is highly recommended that all plugins are loaded",
" prior to DataCollections.")
end
collection = DataCollection(version, name, uuid, plugins,
parameters, DataSet[], path,
AdviceAmalgamation(plugins),
mod)
# Construct the data sets
datasets = copy(spec)
for reservedname in DATA_CONFIG_RESERVED_ATTRIBUTES[:collection]
delete!(datasets, reservedname)
end
for (name, dspecs) in datasets
for dspec in if dspecs isa Vector dspecs else [dspecs] end
push!(collection.datasets, DataSet(collection, name, dspec))
end
end
@advise identity(collection)
end
# ---------------
# DataSet
# ---------------
function DataSet(collection::DataCollection, name::String, spec::Dict{String, Any})
@advise fromspec(DataSet, collection, name, spec)
end
"""
fromspec(::Type{DataSet}, collection::DataCollection, name::String, spec::Dict{String, Any})
Create a `DataSet` for `collection` called `name`, according to `spec`.
"""
function fromspec(::Type{DataSet}, collection::DataCollection, name::String, spec::Dict{String, Any})
uuid = UUID(@something get(spec, "uuid", nothing) begin
@info "Data set '$name' had no UUID, one has been generated."
uuid4()
end)
parameters = smallify(spec)
for reservedname in DATA_CONFIG_RESERVED_ATTRIBUTES[:dataset]
delete!(parameters, reservedname)
end
dataset = DataSet(collection, name, uuid,
dataset_parameters(collection, Val(:extract), parameters),
DataStorage[], DataLoader[], DataWriter[])
for (attr, afield, atype) in [("storage", :storage, DataStorage),
("loader", :loaders, DataLoader),
("writer", :writers, DataWriter)]
specs = get(spec, attr, Dict{String, Any}[]) |>
s -> if s isa Vector s else [s] end
for aspec::Union{String, Dict{String, Any}} in specs
push!(getfield(dataset, afield), atype(dataset, aspec))
end
sort!(getfield(dataset, afield), by=a->a.priority)
end
@advise identity(dataset)
end
| DataToolkitBase | https://github.com/tecosaur/DataToolkitBase.jl.git |
|
[
"MIT"
] | 0.9.1 | ed1a12069bdb85038eb736b2a3bde5d59093eb0b | code | 3213 | QualifiedType(m::Symbol, name::Symbol, params::Tuple=()) =
QualifiedType(m, Symbol[], name, params)
QualifiedType(m::Symbol, parents::Vector{Symbol}, name::Symbol) =
QualifiedType(m, parents, name, ())
function QualifiedType(::Type{T0}) where {T0}
T = Base.unwrap_unionall(T0)
params = map(p -> if p isa Type
QualifiedType(p)
else p end,
T.parameters)
parents = Symbol[]
root = parentmodule(T)
while root != parentmodule(root)
push!(parents, Symbol(root))
root = parentmodule(root)
end
QualifiedType(Symbol(root), parents, nameof(T), Tuple(params))
end
Base.:(==)(a::QualifiedType, b::QualifiedType) =
a.root == b.root && a.parents == b.parents &&
a.name == b.name && a.parameters == b.parameters
QualifiedType(qt::QualifiedType) = qt
QualifiedType(t::AbstractString) = parse(QualifiedType, t) # remove?
function Base.show(io::IO, ::MIME"text/plain", qt::QualifiedType)
print(io, "QualifiedType(", string(qt), ")")
end
"""
typeify(qt::QualifiedType; mod::Module=Main)
Convert `qt` to a `Type` available in `mod`, if possible.
If this cannot be done, `nothing` is returned instead.
"""
function typeify(qt::QualifiedType; mod::Module=Main, shoulderror::Bool=false)
mod = if qt.root === :Main
mod
elseif isdefined(mod, qt.root)
getfield(mod, qt.root)
else
hmod = nothing
for (pkgid, pmod) in Base.loaded_modules
if pkgid.name == String(qt.root)
hmod = pmod
break
end
end
hmod
end
for parent in qt.parents
mod = if isdefined(mod, parent)
getfield(mod, parent)
end
end
# For the sake of the `catch` statement:
if !isnothing(mod) && isdefined(mod, qt.name)
T = getfield(mod, qt.name)
isempty(qt.parameters) && return T
tparams = map(qt.parameters) do p
if p isa QualifiedType
typeify(p; mod)
else p end
end
if any(@. tparams isa TypeVar)
foldl((t, p) -> UnionAll(p, t),
tparams[reverse(findall(@. tparams isa TypeVar))],
init = T{tparams...})
else
T{tparams...}
end
elseif shoulderror
throw(ImpossibleTypeException(qt, mod))
end
end
function Base.issubset(a::QualifiedType, b::QualifiedType; mod::Module=Main)
if a == b
true
else
A, B = typeify(a; mod), typeify(b; mod)
!any(isnothing, (A, B)) && A <: B
end
end
Base.issubset(a::QualifiedType, b::Type; mod::Module=Main) =
issubset(a, QualifiedType(b); mod)
Base.issubset(a::Type, b::QualifiedType; mod::Module=Main) =
issubset(QualifiedType(a), b; mod)
# For the sake of convenience when parsing(foward)/writing(reverse).
const QUALIFIED_TYPE_SHORTHANDS = let forward =
Dict{String, QualifiedType}(
"FilePath" => QualifiedType(FilePath),
"DataSet" => QualifiedType(Symbol(@__MODULE__), :DataSet),
"DataFrame" => QualifiedType(:DataFrames, :DataFrame))
(; forward, reverse = Dict(val => key for (key, val) in forward))
end
| DataToolkitBase | https://github.com/tecosaur/DataToolkitBase.jl.git |
|
[
"MIT"
] | 0.9.1 | ed1a12069bdb85038eb736b2a3bde5d59093eb0b | code | 2758 | # SmallDict implementation
SmallDict{K, V}() where {K, V} =
SmallDict{K, V}(Vector{K}(), Vector{V}())
SmallDict() = SmallDict{Any, Any}()
SmallDict{K, V}(kv::Vector{<:Pair}) where {K, V} =
SmallDict{K, V}(Vector{K}(first.(kv)), Vector{V}(last.(kv)))
SmallDict(kv::Vector{Pair{K, V}}) where {K, V} =
SmallDict{K, V}(first.(kv), last.(kv))
SmallDict{K, V}(kv::Pair...) where {K, V} =
SmallDict{K, V}(Vector{K}(first.(kv) |> collect),
Vector{V}(last.(kv) |> collect))
SmallDict(kv::Pair{K, V}...) where {K, V} = SmallDict{K, V}(kv...)
SmallDict(kv::Pair...) = SmallDict(collect(first.(kv)), collect(last.(kv)))
Base.convert(::Type{SmallDict{K, V}}, dict::Dict) where {K, V} =
SmallDict{K, V}(Vector{K}(keys(dict) |> collect),
Vector{V}(values(dict) |> collect))
Base.convert(::Type{SmallDict}, dict::Dict{K, V}) where {K, V} =
convert(SmallDict{K, V}, dict)
"""
smallify(dict::Dict)
Create a `SmallDict` version of `dict`, with all contained `Dict`s recursively
converted into `SmallDict`s.
"""
function smallify(dict::Dict{K, V}) where {K, V}
stype(v) = v
stype(::Type{Dict{Kv, Vv}}) where {Kv, Vv} = SmallDict{Kv, stype(Vv)}
if V <: Dict || Dict <: V
SmallDict{K, stype(V)}(Vector{K}(keys(dict) |> collect),
Vector{stype(V)}([
if v isa Dict smallify(v) else v end
for v in values(dict)]))
else
convert(SmallDict{K, V}, dict)
end
end
smallify(dict::SmallDict) = dict
Base.length(d::SmallDict) = length(d.keys)
Base.keys(d::SmallDict) = d.keys
Base.values(d::SmallDict) = d.values
Base.iterate(d::SmallDict, index=1) = if index <= length(d)
(d.keys[index] => d.values[index], index+1)
end
function Base.get(d::SmallDict{K}, key::K, default) where {K}
@inbounds for (i, k) in enumerate(d.keys)
k == key && return d.values[i]
end
default
end
function Base.setindex!(d::SmallDict{K, V}, value::V, key::K) where {K, V}
@inbounds for (i, k) in enumerate(d.keys)
if k == key
d.values[i] = value
return d
end
end
push!(d.keys, key)
push!(d.values, value)
d
end
function Base.delete!(d::SmallDict{K}, key::K) where {K}
@inbounds for (i, k) in enumerate(d.keys)
if k == key
deleteat!(d.keys, i)
deleteat!(d.values, i)
return d
end
end
d
end
function Base.sizehint!(d::SmallDict, size::Integer)
sizehint!(d.keys, size)
sizehint!(d.values, size)
end
Base.empty(::SmallDict{K, V}) where {K, V} = SmallDict{K, V}()
function Base.empty!(d::SmallDict)
empty!(d.keys)
empty!(d.values)
d
end
| DataToolkitBase | https://github.com/tecosaur/DataToolkitBase.jl.git |
|
[
"MIT"
] | 0.9.1 | ed1a12069bdb85038eb736b2a3bde5d59093eb0b | code | 1108 | """
getlayer(::Nothing)
Return the first `DataCollection` on the `STACK`.
"""
function getlayer(::Nothing)
length(STACK) == 0 && throw(EmptyStackError())
first(STACK)
end
"""
getlayer(name::AbstractString)
getlayer(uuid::UUID)
Find the `DataCollection` in `STACK` with `name`/`uuid`.
"""
function getlayer(name::AbstractString)
length(STACK) == 0 && throw(EmptyStackError())
matchinglayers = filter(c -> c.name == name, STACK)
if length(matchinglayers) == 0
throw(UnresolveableIdentifier{DataCollection}(String(name)))
elseif length(matchinglayers) > 1
throw(AmbiguousIdentifier(name, matchinglayers))
else
first(matchinglayers)
end
end
# Documented above
function getlayer(uuid::UUID)
length(STACK) == 0 && throw(EmptyStackError())
matchinglayers = filter(c -> c.uuid == uuid, STACK)
if length(matchinglayers) == 1
first(matchinglayers)
elseif length(matchinglayers) == 0
throw(UnresolveableIdentifier{DataCollection}(uuid))
else
throw(AmbiguousIdentifier(uuid, matchinglayers))
end
end
| DataToolkitBase | https://github.com/tecosaur/DataToolkitBase.jl.git |
|
[
"MIT"
] | 0.9.1 | ed1a12069bdb85038eb736b2a3bde5d59093eb0b | code | 9990 | """
A representation of a Julia type that does not need the type to be defined in
the Julia session, and can be stored as a string. This is done by storing the
type name and the module it belongs to as Symbols.
!!! warning
While `QualifiedType` is currently quite capable, it is not currently
able to express the full gamut of Julia types. In future this will be improved,
but it will likely always be restricted to a certain subset.
# Subtyping
While the subtype operator cannot work on QualifiedTypes (`<:` is a built-in),
when the Julia types are defined the subset operator `⊆` can be used instead.
This works by simply `convert`ing the QualifiedTypes to the corresponding Type
and then applying the subtype operator.
```julia-repl
julia> QualifiedTypes(:Base, :Vector) ⊆ QualifiedTypes(:Core, :Array)
true
julia> Matrix ⊆ QualifiedTypes(:Core, :Array)
true
julia> QualifiedTypes(:Base, :Vector) ⊆ AbstractVector
true
julia> QualifiedTypes(:Base, :Foobar) ⊆ AbstractVector
false
```
# Constructors
```julia
QualifiedType(parentmodule::Symbol, typename::Symbol)
QualifiedType(t::Type)
```
# Parsing
A QualifiedType can be expressed as a string as `"\$parentmodule.\$typename"`.
This can be easily `parse`d as a QualifiedType, e.g. `parse(QualifiedType,
"Core.IO")`.
"""
struct QualifiedType
root::Symbol
parents::Vector{Symbol}
name::Symbol
parameters::Tuple
end
"""
SmallDict{K, V}
A little (ordered) dictionary type for a small number of keys.
Rather than doing anything clever with hashes, it just keeps
a list of keys, and a list of values.
For a small number of items, this has time and particularly space advantages
over a standard dictionary — a Dict{String, Any}("a" => 1) is about 4x larger
than the equivalent SmallDict. Further testing indicates this provides a ~40%
reduction on the overall in-memory size of a `DataCollection`.
"""
struct SmallDict{K, V} <: AbstractDict{K, V}
keys::Vector{K}
values::Vector{V}
end
"""
A description that can be used to uniquely identify a DataSet.
Four fields are used to describe the target DataSet:
- `collection`, the name or UUID of the collection (optional).
- `dataset`, the name or UUID of the dataset.
- `type`, the type that should be loaded from the dataset.
- `parameters`, any extra parameters of the dataset that should match.
# Constructors
```julia
Identifier(collection::Union{AbstractString, UUID, Nothing},
dataset::Union{AbstractString, UUID},
type::Union{QualifiedType, Nothing},
parameters::SmallDict{String, Any})
```
# Parsing
An Identifier can be represented as a string with the following form,
with the optional components enclosed by square brackets:
```
[COLLECTION:]DATASET[::TYPE]
```
Such forms can be parsed to an Identifier by simply calling the `parse`
function, i.e. `parse(Identifier, "mycollection:dataset")`.
"""
struct Identifier
collection::Union{AbstractString, UUID, Nothing}
dataset::Union{AbstractString, UUID}
type::Union{<:QualifiedType, Nothing}
parameters::SmallDict{String, Any}
end
"""
The supertype for methods producing or consuming data.
```
╭────loader─────╮
╵ ▼
Storage ◀────▶ Data Information
▲ ╷
╰────writer─────╯
```
There are three subtypes:
- `DataStorage`
- `DataLoader`
- `DataWrite`
Each subtype takes a `Symbol` type parameter designating
the driver which should be used to perform the data operation.
In addition, each subtype has the following fields:
- `dataset::DataSet`, the data set the method operates on
- `type::Vector{<:QualifiedType}`, the Julia types the method supports
- `priority::Int`, the priority with which this method should be used,
compared to alternatives. Lower values have higher priority.
- `parameters::SmallDict{String, Any}`, any parameters applied to the method.
"""
abstract type AbstractDataTransformer{driver} end
struct DataStorage{driver, T} <: AbstractDataTransformer{driver}
dataset::T
type::Vector{<:QualifiedType}
priority::Int
parameters::SmallDict{String, Any}
end
struct DataLoader{driver} <: AbstractDataTransformer{driver}
dataset
type::Vector{<:QualifiedType}
priority::Int
parameters::SmallDict{String, Any}
end
struct DataWriter{driver} <: AbstractDataTransformer{driver}
dataset
type::Vector{<:QualifiedType}
priority::Int
parameters::SmallDict{String, Any}
end
"""
Advice{func, context} <: Function
Advices allow for composable, highly flexible modifications of data by
encapsulating a function call. They are inspired by elisp's advice system,
namely the most versatile form — `:around` advice, and Clojure's advisors.
A `Advice` is essentially a function wrapper, with a `priority::Int`
attribute. The wrapped functions should be of the form:
```julia
(action::Function, args...; kargs...) ->
([post::Function], action::Function, args::Tuple, [kargs::NamedTuple])
```
Short-hand return values with `post` or `kargs` omitted are also accepted, in
which case default values (the `identity` function and `(;)` respectively) will
be automatically substituted in.
```
input=(action args kwargs)
┃ ┏╸post=identity
╭─╂────advisor 1────╂─╮
╰─╂─────────────────╂─╯
╭─╂────advisor 2────╂─╮
╰─╂─────────────────╂─╯
╭─╂────advisor 3────╂─╮
╰─╂─────────────────╂─╯
┃ ┃
▼ ▽
action(args; kargs) ━━━━▶ post╺━━▶ result
```
To specify which transforms a Advice should be applied to, ensure you
add the relevant type parameters to your transducing function. In cases where
the transducing function is not applicable, the Advice will simply act
as the identity function.
After all applicable `Advice`s have been applied, `action(args...;
kargs...) |> post` is called to produce the final result.
The final `post` function is created by rightwards-composition with every `post`
entry of the advice forms (i.e. at each stage `post = post ∘ extra` is run).
The overall behaviour can be thought of as *shells* of advice.
```
╭╌ advisor 1 ╌╌╌╌╌╌╌╌─╮
┆ ╭╌ advisor 2 ╌╌╌╌╌╮ ┆
┆ ┆ ┆ ┆
input ━━┿━┿━━━▶ function ━━━┿━┿━━▶ result
┆ ╰╌╌╌╌╌╌╌╌╌╌╌╌╌╌╌╌╌╯ ┆
╰╌╌╌╌╌╌╌╌╌╌╌╌╌╌╌╌╌╌╌╌╌╯
```
# Constructors
```julia
Advice(priority::Int, f::Function)
Advice(f::Function) # priority is set to 1
```
# Examples
**1. Logging every time a DataSet is loaded.**
```julia
loggingadvisor = Advice(
function(post::Function, f::typeof(load), loader::DataLoader, input, outtype)
@info "Loading \$(loader.data.name)"
(post, f, (loader, input, outtype))
end)
```
**2. Automatically committing each data file write.**
```julia
writecommitadvisor = Advice(
function(post::Function, f::typeof(write), writer::DataWriter{:filesystem}, output, info)
function writecommit(result)
run(`git add \$output`)
run(`git commit -m "update \$output"`)
result
end
(post ∘ writecommit, writefn, (output, info))
end)
```
"""
struct Advice{func, context} <: Function
priority::Int # REVIEW should this be an Int?
f::Function
function Advice(priority::Int, f::Function)
validmethods = methods(f, Tuple{Function, Any, Vararg{Any}})
if length(validmethods) === 0
throw(ArgumentError("Transducing function $f had no valid methods."))
end
functype, context = first(validmethods).sig.types[[2, 3:end]]
new{functype, Tuple{context...}}(priority, f)
end
end
struct Plugin
name::String
advisors::Vector{Advice}
end
Plugin(name::String, advisors::Vector{<:Function}) =
Plugin(name, Advice.(advisors))
Plugin(name::String, advisors::Vector{<:Advice}) =
Plugin(name, Vector{Advice}(advisors))
struct DataSet
collection
name::String
uuid::UUID
parameters::SmallDict{String, Any}
storage::Vector{DataStorage}
loaders::Vector{DataLoader}
writers::Vector{DataWriter}
end
"""
A collection of `Advices` sourced from available Plugins.
Like individual `Advices`, a `AdviceAmalgamation` can be called
as a function. However, it also supports the following convenience syntax:
```
(::AdviceAmalgamation)(f::Function, args...; kargs...) # -> result
```
# Constructors
```
AdviceAmalgamation(adviseall::Function, advisors::Vector{Advice},
plugins_wanted::Vector{String}, plugins_used::Vector{String})
AdviceAmalgamation(plugins::Vector{String})
AdviceAmalgamation(collection::DataCollection)
```
"""
mutable struct AdviceAmalgamation
adviseall::Function
advisors::Vector{Advice}
plugins_wanted::Vector{String}
plugins_used::Vector{String}
end
struct DataCollection
version::Int
name::Union{String, Nothing}
uuid::UUID
plugins::Vector{String}
parameters::SmallDict{String, Any}
datasets::Vector{DataSet}
path::Union{String, Nothing}
advise::AdviceAmalgamation
mod::Module
end
"""
struct FilePath path::String end
Crude stand in for a file path type, which is strangely absent from Base.
This allows for load/write method dispatch, and the distinguishing of
file content (as a String) from file paths.
# Examples
```julia-repl
julia> string(FilePath("some/path"))
"some/path"
```
"""
struct FilePath path::String end
Base.string(fp::FilePath) = fp.path
# `ReplCmd` is documented in interaction/repl.jl
struct ReplCmd{name, E <: Union{Function, Vector}}
trigger::String
description::Any
execute::E
function ReplCmd{name}(trigger::String, description::Any,
execute::Union{Function, Vector{<:ReplCmd}}) where { name }
if execute isa Function
new{name, Function}(trigger, description, execute)
else
new{name, Vector{ReplCmd}}(trigger, description, execute)
end
end
end
| DataToolkitBase | https://github.com/tecosaur/DataToolkitBase.jl.git |
|
[
"MIT"
] | 0.9.1 | ed1a12069bdb85038eb736b2a3bde5d59093eb0b | code | 13874 | struct PkgRequiredRerunNeeded end
"""
get_package(pkg::Base.PkgId)
get_package(from::Module, name::Symbol)
Obtain a module specified by either `pkg` or identified by `name` and declared
by `from`. Should the package not be currently loaded, in Julia ≥ 1.7
DataToolkit will attempt to lazy-load the package and return its module.
Failure to either locate `name` or require `pkg` will result in an exception
being thrown.
"""
function get_package(pkg::Base.PkgId)
if !Base.root_module_exists(pkg)
fmtpkg = string(pkg.name, '[', pkg.uuid, ']')
if VERSION < v"1.7" # Before `Base.invokelatest`
@error string(
"The package $fmtpkg is required for the operation of DataToolkit.\n",
"DataToolkit can not do this for you, so please add `using $(pkg.name)`\n",
"as appropriate then re-trying this operation.")
throw(MissingPackage(pkg))
end
@info "Lazy-loading $fmtpkg"
try
Base.require(pkg)
true
catch err
pkgmsg = "is required but does not seem to be installed"
err isa ArgumentError && isinteractive() && occursin(pkgmsg, err.msg) &&
try_install_pkg(pkg)
end || throw(MissingPackage(pkg))
PkgRequiredRerunNeeded()
else
Base.root_module(pkg)
end
end
const PKG_ID = Base.PkgId(Base.UUID("44cfe95a-1eb2-52ea-b672-e2afdf69b78f"), "Pkg")
@static if VERSION > v"1.11-alpha1"
function try_install_pkg(pkg::Base.PkgId)
Pkg = try
@something get(Base.loaded_modules, PKG_ID, nothing) Base.require(PKG_ID)
catch _ end
isnothing(Pkg) && return false
repl_ext = Base.get_extension(Pkg, :REPLExt)
!isnothing(repl_ext) &&
isdefined(repl_ext, :try_prompt_pkg_add) &&
Base.get_extension(Pkg, :REPLExt).try_prompt_pkg_add([Symbol(pkg.name)])
end
else
function try_install_pkg(pkg::Base.PkgId)
Pkg = get(Base.loaded_modules, PKG_ID, nothing)
!isnothing(Pkg) && isdefined(Pkg, :REPLMode) &&
isdefined(Pkg.REPLMode, :try_prompt_pkg_add) &&
Pkg.REPLMode.try_prompt_pkg_add([Symbol(pkg.name)])
end
end
function get_package(from::Module, name::Symbol)
pkgid = get(get(EXTRA_PACKAGES, from, Dict()), name, nothing)
if !isnothing(pkgid)
get_package(pkgid)
else
throw(UnregisteredPackage(name, from))
end
end
"""
@addpkg name::Symbol uuid::String
Register the package identified by `name` with UUID `uuid`.
This package may now be used with `@import \$name`.
All @addpkg statements should lie within a module's `__init__` function.
# Example
```
@addpkg CSV "336ed68f-0bac-5ca0-87d4-7b16caf5d00b"
```
"""
macro addpkg(name::Symbol, uuid::String)
:(addpkg(@__MODULE__, Symbol($(String(name))), $uuid))
end
function addpkg(mod::Module, name::Symbol, uuid::Union{UUID, String})
if !haskey(EXTRA_PACKAGES, mod)
EXTRA_PACKAGES[mod] = Dict{Symbol, Vector{Base.PkgId}}()
end
EXTRA_PACKAGES[mod][name] = Base.PkgId(UUID(uuid), String(name))
end
"""
invokepkglatest(f, args...; kwargs...)
Call `f(args...; kwargs...)` via `invokelatest`, and re-run if
PkgRequiredRerunNeeded is returned.
"""
function invokepkglatest(@nospecialize(f), @nospecialize args...; kwargs...)
result = Base.invokelatest(f, args...; kwargs...)
if result isa PkgRequiredRerunNeeded
invokepkglatest(f, args...; kwargs...)
else
result
end
end
# ------------
# @import
# ------------
module ImportParser
const PkgTerm = NamedTuple{(:name, :as), Tuple{Symbol, Symbol}}
const ImportTerm = NamedTuple{(:pkg, :property, :as), # TODO support :type
Tuple{Symbol, Union{Symbol, Expr}, Symbol}}
const PkgList = Vector{PkgTerm}
const ImportList = Vector{ImportTerm}
struct InvalidImportForm <: Exception
msg::String
end
Base.showerror(io::IO, err::InvalidImportForm) =
print(io, "InvalidImportForm: ", err.msg)
"""
addimport!(pkg::Symbol, property::Symbol,
alias::Union{Symbol, Nothing}=nothing; imports::ImportList)
addimport!(pkg::Union{Expr, Symbol}, property::Expr,
alias::Union{Symbol, Nothing}=nothing; imports::ImportList)
Add the appropriate information to `import` for `property` to be loaded from `pkg`,
either by the default name, or as `alias` if specified.
"""
function addimport!(pkg::Symbol, property::Symbol,
alias::Union{Symbol, Nothing}=nothing;
imports::ImportList)
push!(imports, (; pkg, property, as=something(alias, property)))
something(alias, property)
end
function addimport!(pkg::Union{Expr, Symbol}, property::Expr,
alias::Union{Symbol, Nothing}=nothing;
imports::ImportList)
if property.head == :.
as = something(alias, last(property.args).value)
push!(imports, (; pkg, property, as))
as
elseif property.head == :macrocall
if length(property.args) == 2
as = something(alias, first(property.args))
push!(imports, (; pkg, property=first(property.args), as))
as
else
throw(InvalidImportForm("invalid macro import from $pkg: $property"))
end
else
throw(InvalidImportForm("$property is not valid property of $pkg"))
end
end
"""
addpkg!(name::Union{Expr, Symbol}, alias::Union{Symbol, Nothing}=nothing;
pkgs::PkgList, imports::ImportList)
Add the appropriate information to `pkgs` and perhaps `imports` for the package
`name` to be loaded, either using the default name, or as `alias` if provided.
"""
function addpkg!(name::Symbol, alias::Union{Symbol, Nothing}=nothing;
pkgs::PkgList, imports::ImportList)
push!(pkgs, (; name, as=something(alias, name)))
something(alias, name)
end
function addpkg!(name::Expr, alias::Union{Symbol, Nothing}=nothing;
pkgs::PkgList, imports::ImportList)
rootpkg(s::Symbol) = s
rootpkg(e::Expr) = rootpkg(first(e.args))
if name.head == :.
# `name` (e.g. `Pkg.a.b.c`) is of the structure:
# `E.(E.(E.(Symbol(:Pkg), Q(:a)), Q(:b)), Q(:c))`
# where `E.(x, y)` is shorthand for `Expr(:., x, y)`,
# and `Q(x)` is shorthand for `QuoteNode(x)`.
pkgas = if (pkgindex = findfirst(p -> p.name == rootpkg(name), pkgs)) |> !isnothing
pkgs[pkgindex].as
else
as = gensym(rootpkg(name))
push!(pkgs, (; name=rootpkg(name), as))
as
end
property = copy(name)
_, prop = splitfirst(name)
addimport!(pkgas, prop, alias; imports)
else
throw(InvalidImportForm("$name is not a valid package name"))
end
end
"""
splitfirst(prop::Expr)
Split an nested property expression into the first term and the remaining terms
## Example
```jldoctest; setup = :(import DataToolkitBase.ImportParser.splitfirst)
julia> splitfirst(:(a.b.c.d))
(:a, :(b.c.d))
```
"""
function splitfirst(prop::Expr)
function splitfirst!(ex::Expr)
if ex.head != :.
throw(ArgumentError("Expected a property node, not $ex"))
elseif ex.args[1] isa Expr && ex.args[1].args[1] isa Symbol
leaf = ex.args[1]
first, second = leaf.args[1], leaf.args[2].value
ex.args[1] = second
first
elseif ex.args[1] isa Expr
splitfirst!(ex.args[1])
end
end
if prop.head != :.
throw(ArgumentError("Expected a property node, not $prop"))
elseif prop.args[1] isa Symbol
prop.args[1], prop.args[2].value
else
prop_copy = copy(prop)
splitfirst!(prop_copy), prop_copy
end
end
"""
propertylist(prop::Expr)
Represent a nested property expression as a vector of symbols.
## Example
```jldoctest; setup = :(import DataToolkitBase.ImportParser.propertylist)
julia> propertylist(:(a.b.c.d))
4-element Vector{Symbol}:
:a
:b
:c
:d
```
"""
function propertylist(prop::Expr)
properties = Vector{Symbol}()
while prop isa Expr
prop, peel = prop.args[1], prop.args[2].value
push!(properties, peel)
end
push!(properties, prop)
reverse(properties)
end
"""
graft(parent::Symbol, child::Union{Expr, Symbol})
Return a "grafted" expression takes the `child` property of `parent`.
## Example
```jldoctest; setup = :(import DataToolkitBase.ImportParser.graft)
julia> graft(:a, :(b.c.d))
:(a.b.c.d)
julia> graft(:a, :b)
:(a.b)
```
"""
function graft(parent::Symbol, child::Expr)
properties = propertylist(child)
gexpr = Expr(:., parent, QuoteNode(popfirst!(properties)))
while !isempty(properties)
gexpr = Expr(:., gexpr, QuoteNode(popfirst!(properties)))
end
gexpr
end
graft(parent::Symbol, child::Symbol) =
Expr(:., parent, QuoteNode(child))
"""
flattenterms(terms)
Where `terms` is a set of terms produced from parsing an expression like
`foo as bar, baz`, flatten out the individual tokens.
## Example
```jldoctest; setup = :(import DataToolkitBase.ImportParser.flattenterms)
julia> flattenterms((:(foo.bar), :as, :((bar, baz, baz.foo, other.thing)), :as, :((other, more))))
9-element Vector{Union{Expr, Symbol}}:
:(foo.bar)
:as
:bar
:baz
:(baz.foo)
:(other.thing)
:as
:other
:more
```
"""
function flattenterms(terms)
stack = Vector{Union{Symbol, Expr}}()
for term in terms
if term isa Symbol || term.head == :.
push!(stack, term)
elseif term isa Expr && term.head == :tuple
append!(stack, term.args)
end
end
stack
end
"""
extractterms(terms)
Convert an import expression (`terms`), to a named tuple giving a `PkgList` (as
`pkgs`) and `ImportList` (as `imports`).
"""
function extractterms(@nospecialize(terms))
pkgs = PkgList()
imports = ImportList()
if length(terms) == 1 && (terms[1] isa Symbol || terms[1].head == :.)
# Case 1: @import Pkg(.thing)?
addpkg!(first(terms); pkgs, imports)
elseif terms[1] isa Expr &&
((terms[1].head == :call && terms[1].args[1] == :(:)) ||
(terms[1].head == :tuple && terms[1].args[1] isa Expr &&
terms[1].args[1].head == :call && terms[1].args[1].args[1] == :(:)))
# Case 2: @import pkg: a, b as c, d, e, f as g, h, ...
stack = Union{Symbol, Expr}[]
pkg = if terms[1].head == :call
append!(stack, terms[1].args[3:end])
terms[1].args[2]
else
push!(stack, terms[1].args[1].args[3])
append!(stack, terms[1].args[2:end])
terms[1].args[1].args[2]
end
pkgalias = gensym(if pkg isa Symbol pkg else last(pkg.args).value end)
addpkg!(pkg, pkgalias; pkgs, imports)
append!(stack, flattenterms(terms[2:end]))
while !isempty(stack)
if length(stack) > 2 && stack[2] == :as
addimport!(pkgalias, stack[1], stack[3]; imports)
deleteat!(stack, 1:3)
else
addimport!(pkgalias, stack[1]; imports)
deleteat!(stack, 1)
end
end
elseif length(terms) == 1 && terms[1] isa Expr && terms[1].head == :tuple
# Case 3: @import Pkg1, Pkg2(.thing)?, ...
for term in terms[1].args
addpkg!(term; pkgs, imports)
end
else
# Case: @import pkg1 as pkg2, pkg3, ...
stack = flattenterms(terms)
while !isempty(stack)
if length(stack) > 2 && stack[2] == :as
addpkg!(stack[1], stack[3]; pkgs, imports)
deleteat!(stack, 1:3)
else
addpkg!(stack[1]; pkgs, imports)
deleteat!(stack, 1)
end
end
end
(; pkgs, imports)
end
"""
genloadstatement(pkgs::PkgList, imports::ImportList)
Create an `Expr` that sets up `pkgs` and `imports`.
"""
function genloadstatement(pkgs::PkgList, imports::ImportList)
Expr(:block,
Iterators.flatten(
map(pkgs) do (; name, as)
(Expr(:(=), as,
Expr(:call,
GlobalRef(parentmodule(@__MODULE__), :get_package),
:(@__MODULE__),
QuoteNode(name))),
Expr(:||,
Expr(:call, :isa, as, GlobalRef(Core, :Module)),
Expr(:return, as)))
end)...,
map(imports) do (; pkg, property, as)
if property isa Symbol
Expr(:(=), as, Expr(:., pkg, QuoteNode(property)))
elseif property isa Expr
Expr(:(=), as, graft(pkg, property))
end
end...)
end
"""
@import pkg1, pkg2...
@import pkg1 as name1, pkg2 as name2...
@import pkg: foo, bar...
@import pkg: foo as bar, bar as baz...
Fetch modules previously registered with `@addpkg`, and import them into the
current namespace. This macro tries to largely mirror the syntax of `using`.
For the sake of type inference, it is assumed that all bindings that start with
a lower case letter are functions, and bindings that start with an upper case
letter are types. Exceptions must be manually annotated with type assertions.
If a required package had to be loaded for the `@import` statement, a
`PkgRequiredRerunNeeded` singleton will be returned.
# Example
```julia
@import pkg
pkg.dothing(...)
# Alternative form
@import pkg: dothing
dothing(...)
```
"""
macro localimport(terms::Union{Expr, Symbol}...)
(; pkgs, imports) = extractterms(terms)
genloadstatement(pkgs, imports) |> esc
end
end
using .ImportParser
# To get around ERROR: syntax: invalid name "import"
const var"@import" = ImportParser.var"@localimport"
| DataToolkitBase | https://github.com/tecosaur/DataToolkitBase.jl.git |
|
[
"MIT"
] | 0.9.1 | ed1a12069bdb85038eb736b2a3bde5d59093eb0b | code | 7106 | # Utility functions that don't belong to any particular file
"""
natkeygen(key::String)
Generate a sorting key for `key` that when used with `sort` will put the
collection in "natural order".
```jldoctest; setup = :(import DataToolkitBase.natkeygen)
julia> natkeygen.(["A1", "A10", "A02", "A1.5"])
4-element Vector{Vector{AbstractString}}:
["a", "0\\x01"]
["a", "0\\n"]
["a", "0\\x02"]
["a", "0\\x015"]
julia> sort(["A1", "A10", "A02", "A1.5"], by=natkeygen)
4-element Vector{String}:
"A1"
"A1.5"
"A02"
"A10"
```
"""
function natkeygen(key::String)
map(eachmatch(r"(\d*\.\d+)|(\d+)|([^\d]+)", lowercase(key))) do (; captures)
float, int, str = captures
if !isnothing(float)
f = parse(Float64, float)
fint, dec = floor(Int, f), mod(f, 1)
'0' * Char(fint) * string(dec)[3:end]
elseif !isnothing(int)
'0' * Char(parse(Int, int))
else
str
end
end
end
"""
stringdist(a::AbstractString, b::AbstractString; halfcase::Bool=false)
Calculate the Restricted Damerau-Levenshtein distance (aka. Optimal String
Alignment) between `a` and `b`.
This is the minimum number of edits required to transform `a` to `b`, where each
edit is a *deletion*, *insertion*, *substitution*, or *transposition* of a
character, with the restriction that no substring is edited more than once.
When `halfcase` is true, substitutions that just switch the case of a character
cost half as much.
# Examples
```jldoctest; setup = :(import DataToolkitBase.stringdist)
julia> stringdist("The quick brown fox jumps over the lazy dog",
"The quack borwn fox leaps ovver the lzy dog")
7
julia> stringdist("typo", "tpyo")
1
julia> stringdist("frog", "cat")
4
julia> stringdist("Thing", "thing", halfcase=true)
0.5
```
"""
function stringdist(a::AbstractString, b::AbstractString; halfcase::Bool=false)
if length(a) > length(b)
a, b = b, a
end
start = 0
for (i, j) in zip(eachindex(a), eachindex(b))
if a[i] == b[j]
start += 1
else
break
end
end
start == length(a) && return length(b) - start
v₀ = collect(2:2:2*(length(b) - start))
v₁ = similar(v₀)
aᵢ₋₁, bⱼ₋₁ = first(a), first(b)
current = 0
for (i, aᵢ) in enumerate(a)
i > start || (aᵢ₋₁ = aᵢ; continue)
left = 2*(i - start - 1)
current = 2*(i - start)
transition_next = 0
@inbounds for (j, bⱼ) in enumerate(b)
j > start || (bⱼ₋₁ = bⱼ; continue)
# No need to look beyond window of lower right diagonal
above = current
this_transition = transition_next
transition_next = v₁[j - start]
v₁[j - start] = current = left
left = v₀[j - start]
if aᵢ != bⱼ
# (Potentially) cheaper substitution when just
# switching case.
substitutecost = if halfcase
aᵢswitchcap = if isuppercase(aᵢ)
lowercase(aᵢ)
elseif islowercase(aᵢ)
uppercase(aᵢ)
else aᵢ end
ifelse(aᵢswitchcap == bⱼ, 1, 2)
else
2
end
# Minimum between substitution, deletion and insertion
current = min(current + substitutecost,
above + 2, left + 2) # deletion or insertion
if i > start + 1 && j > start + 1 && aᵢ == bⱼ₋₁ && aᵢ₋₁ == bⱼ
current = min(current, (this_transition += 2))
end
end
v₀[j - start] = current
bⱼ₋₁ = bⱼ
end
aᵢ₋₁ = aᵢ
end
if halfcase current/2 else current÷2 end
end
"""
stringsimilarity(a::AbstractString, b::AbstractString; halfcase::Bool=false)
Return the `stringdist` as a proportion of the maximum length of `a` and `b`,
take one. When `halfcase` is true, case switches cost half as much.
# Example
```jldoctest; setup = :(import DataToolkitBase.stringsimilarity)
julia> stringsimilarity("same", "same")
1.0
julia> stringsimilarity("semi", "demi")
0.75
julia> stringsimilarity("Same", "same", halfcase=true)
0.875
```
"""
stringsimilarity(a::AbstractString, b::AbstractString; halfcase::Bool=false) =
1 - stringdist(a, b; halfcase) / max(length(a), length(b))
"""
longest_common_subsequence(a, b)
Find the longest common subsequence of `b` within `a`, returning the indices of
`a` that comprise the subsequence.
This function is intended for strings, but will work for any indexable objects
with `==` equality defined for their elements.
# Example
```jldoctest; setup = :(import DataToolkitBase.longest_common_subsequence)
julia> longest_common_subsequence("same", "same")
4-element Vector{Int64}:
1
2
3
4
julia> longest_common_subsequence("fooandbar", "foobar")
6-element Vector{Int64}:
1
2
3
7
8
9
```
"""
function longest_common_subsequence(a, b)
lengths = zeros(Int, length(a) + 1, length(b) + 1)
for (i, x) in enumerate(a), (j, y) in enumerate(b)
lengths[i+1, j+1] = if x == y
lengths[i, j] + 1
else
max(lengths[i+1, j], lengths[i, j+1])
end
end
subsequence = Int[]
x, y = size(lengths)
aind, bind = eachindex(a) |> collect, eachindex(b) |> collect
while lengths[x,y] > 0
if a[aind[x-1]] == b[bind[y-1]]
push!(subsequence, x-1)
x -=1; y -= 1
elseif lengths[x, y-1] > lengths[x-1, y]
y -= 1
else
x -= 1
end
end
reverse(subsequence)
end
"""
issubseq(a, b)
Return `true` if `a` is a subsequence of `b`, `false` otherwise.
## Examples
```jldoctest; setup = :(import DataToolkitBase.issubseq)
julia> issubseq("abc", "abc")
true
julia> issubseq("adg", "abcdefg")
true
julia> issubseq("gda", "abcdefg")
false
```
"""
issubseq(a, b) = length(longest_common_subsequence(a, b)) == length(a)
"""
highlight_lcs(io::IO, a::String, b::String;
before::String="\\e[1m", after::String="\\e[22m",
invert::Bool=false)
Print `a`, highlighting the longest common subsequence between `a` and `b` by
inserting `before` prior to each subsequence region and `after` afterwards.
If `invert` is set, the `before`/`after` behaviour is switched.
"""
function highlight_lcs(io::IO, a::String, b::String;
before::String="\e[1m", after::String="\e[22m",
invert::Bool=false)
seq = longest_common_subsequence(collect(a), collect(b))
seq_pos = firstindex(seq)
in_lcs = invert
for (i, char) in enumerate(a)
if seq_pos < length(seq) && seq[seq_pos] < i
seq_pos += 1
end
if in_lcs != (i == seq[seq_pos])
in_lcs = !in_lcs
get(io, :color, false) && print(io, ifelse(in_lcs ⊻ invert, before, after))
end
print(io, char)
end
get(io, :color, false) && print(io, after)
end
| DataToolkitBase | https://github.com/tecosaur/DataToolkitBase.jl.git |
|
[
"MIT"
] | 0.9.1 | ed1a12069bdb85038eb736b2a3bde5d59093eb0b | code | 5880 | """
iswritable(dc::DataCollection)
Check whether a data collection is backed by a writable file.
"""
Base.iswritable(dc::DataCollection) =
!isnothing(dc.path) &&
get(dc, "locked", false) !== true &&
try # why is this such a hassle?
open(io -> iswritable(io), dc.path, "a")
catch e
if e isa SystemError
false
else
rethrow()
end
end
function Base.string(q::QualifiedType)
if haskey(QUALIFIED_TYPE_SHORTHANDS.reverse, q)
return QUALIFIED_TYPE_SHORTHANDS.reverse[q]
end
qname = if q.root == :Base && Base.isexported(Base, q.name)
string(q.name)
elseif q.root == :Core && Base.isexported(Core, q.name)
string(q.name)
elseif isempty(q.parents)
string(q.root, '.', q.name)
else
string(q.root, '.', join(q.parents, '.'), '.', q.name)
end
if isempty(q.parameters)
qname
else
parstr = map(q.parameters) do p
if p isa Symbol
string(':', p)
elseif p isa TypeVar
string(ifelse(first(String(p.name)) == '#',
"", String(p.name)),
"<:", string(p.ub))
else
string(p)
end
end
string(qname, '{', join(parstr, ','), '}')
end
end
function Base.convert(::Type{Dict}, adt::AbstractDataTransformer)
@advise tospec(adt)
end
"""
tospec(thing::AbstractDataTransformer)
tospec(thing::DataSet)
tospec(thing::DataCollection)
Return a `Dict` representation of `thing` for writing as TOML.
"""
function tospec(adt::AbstractDataTransformer)
merge(Dict("driver" => string(first(typeof(adt).parameters)),
"type" => if length(adt.type) == 1
string(first(adt.type))
else
string.(adt.type)
end,
"priority" => adt.priority),
dataset_parameters(adt.dataset, Val(:encode), adt.parameters))
end
function Base.convert(::Type{Dict}, ds::DataSet)
@advise tospec(ds)
end
# Documented above
function tospec(ds::DataSet)
merge(Dict("uuid" => string(ds.uuid),
"storage" => convert.(Dict, ds.storage),
"loader" => convert.(Dict, ds.loaders),
"writer" => convert.(Dict, ds.writers)) |>
d -> filter(((k, v)::Pair) -> !isempty(v), d),
dataset_parameters(ds, Val(:encode), ds.parameters))
end
function Base.convert(::Type{Dict}, dc::DataCollection)
@advise tospec(dc)
end
function tospec(dc::DataCollection)
merge(Dict("data_config_version" => dc.version,
"name" => dc.name,
"uuid" => string(dc.uuid),
"plugins" => dc.plugins,
"config" => dataset_parameters(dc, Val(:encode), dc.parameters)),
let datasets = Dict{String, Any}()
for ds in dc.datasets
if haskey(datasets, ds.name)
push!(datasets[ds.name], convert(Dict, ds))
else
datasets[ds.name] = [convert(Dict, ds)]
end
end
datasets
end)
end
"""
tomlreformat!(io::IO)
Consume `io` representing a TOML file, and reformat it to improve readability.
Currently this takes the form of the following changes:
- Replace inline multi-line strings with multi-line toml strings.
An IOBuffer containing the reformatted content is returned.
The processing assumes that `io` contains `TOML.print`-formatted content.
Should this not be the case, mangled TOML may be emitted.
"""
function tomlreformat!(io::IO)
out = IOBuffer()
bytesavailable(io) == 0 && seekstart(io)
for line in eachline(io)
# Check for multi-line candidates. Cases:
# 1. key = "string..."
# 2. 'key...' = "string..."
# 3. "key..." = "string..."
if !isnothing(match(r"^\s*(?:[A-Za-z0-9_-]+|\'[ \"A-Za-z0-9_-]+\'|\"[ 'A-Za-z0-9_-]+\") *= * \".*\"$", line))
write(out, line[1:findfirst(!isspace, line)-1]) # apply indent
key, value = first(TOML.parse(line))
if length(value) < 40 || count('\n', value) == 0 || (count('\n', value) < 3 && length(value) < 90)
TOML.print(out, Dict{String, Any}(key => value))
elseif !occursin("'''", value) && count('"', value) > 4 &&
!any(c -> c != '\n' && Base.iscntrl(c), value)
TOML.Internals.Printer.printkey(out, [key])
write(out, " = '''\n", value, "'''\n")
else
TOML.Internals.Printer.printkey(out, [key])
write(out, " = \"\"\"\n",
replace(sprint(TOML.Internals.Printer.print_toml_escaped, value),
"\\n" => '\n'),
"\"\"\"\n")
end
else
write(out, line, '\n')
end
end
out
end
function Base.write(io::IO, dc::DataCollection)
datakeygen(key) = if haskey(DATA_CONFIG_KEY_SORT_MAPPING, key)
[DATA_CONFIG_KEY_SORT_MAPPING[key]]
else
natkeygen(key)
end
intermediate = IOBuffer()
TOML.print(intermediate,
filter(((_, value),) -> !isnothing(value) && !isempty(value),
convert(Dict, dc));
sorted = true, by = datakeygen)
write(io, take!(tomlreformat!(intermediate)))
end
function Base.write(dc::DataCollection)
if !iswritable(dc)
if !isnothing(dc.path)
throw(ArgumentError("No collection writer is provided, so an IO argument must be given."))
else
throw(ReadonlyCollection(dc))
end
end
write(dc.path, dc)
end
Base.write(ds::DataSet) = write(ds.collection)
Base.write(adt::AbstractDataTransformer) = write(adt.dataset)
| DataToolkitBase | https://github.com/tecosaur/DataToolkitBase.jl.git |
|
[
"MIT"
] | 0.9.1 | ed1a12069bdb85038eb736b2a3bde5d59093eb0b | code | 26665 | using DataToolkitBase
using Test
import DataToolkitBase: natkeygen, stringdist, stringsimilarity,
longest_common_subsequence, highlight_lcs, referenced_datasets,
stack_index, plugin_add, plugin_list, plugin_remove, config_get,
config_set, config_unset, DATASET_REFERENCE_WRAPPER
@testset "Utils" begin
@testset "Doctests" begin
@test natkeygen.(["A1", "A10", "A02", "A1.5"]) ==
[["a", "0\x01"], ["a", "0\n"], ["a", "0\x02"], ["a", "0\x015"]]
@test sort(["A1", "A10", "A02", "A1.5"], by=natkeygen) ==
["A1", "A1.5", "A02", "A10"]
@test stringdist("The quick brown fox jumps over the lazy dog",
"The quack borwn fox leaps ovver the lzy dog") == 7
@test stringdist("typo", "tpyo") == 1
@test stringdist("frog", "cat") == 4
@test stringsimilarity("same", "same") == 1.0
@test stringsimilarity("semi", "demi") == 0.75
@test longest_common_subsequence("same", "same") == [1:4;]
@test longest_common_subsequence("fooandbar", "foobar") == vcat(1:3, 7:9)
end
@testset "Multi-codepoint unicode" begin
@test stringdist("ÆaÆb", "ÆacÆb") == 1
@test stringsimilarity("ÆaÆb", "ÆacÆb") == 0.8
@test longest_common_subsequence("heyÆsop", "hiÆsop") == vcat(1, 4:7)
end
@testset "Highlighting LCS" begin
io = IOContext(IOBuffer(), :color => true)
highlight_lcs(io, "hey", "hey")
@test String(take!(io.io)) == "\e[1mhey\e[22m"
highlight_lcs(io, "hey", "hey", invert=true)
@test String(take!(io.io)) == "hey\e[22m"
highlight_lcs(io, "hey", "hey", before="^", after="_")
@test String(take!(io.io)) == "^hey_"
highlight_lcs(io, "xxheyyy", "aaheybb")
@test String(take!(io.io)) == "xx\e[1mhey\e[22myy\e[22m"
highlight_lcs(io, "xxheyyy", "aaheybb", before="^", after="_", invert=true)
@test String(take!(io.io)) == "^xx_hey^yy_"
highlight_lcs(io, "xxheyyy", "xx___yy")
@test String(take!(io.io)) == "\e[1mxx\e[22mhey\e[1myy\e[22m"
end
end
@testset "Advice" begin
# Some advice to use
sump1 = Advice(2, (f::typeof(sum), i::Int) -> (f, (i+1,)))
sump1a = Advice(2, (f::typeof(sum), i::Int) -> (f, (i+1,), (;)))
sump1b = Advice(2, (f::typeof(sum), i::Int) -> (identity, f, (i+1,)))
sump1c = Advice(2, (f::typeof(sum), i::Int) -> (identity, f, (i+1,), (;)))
sump1x = Advice(2, (f::typeof(sum), i::Int) -> ())
sumx2 = Advice(1, (f::typeof(sum), i::Int) -> (f, (2*i,)))
summ3 = Advice(1, (f::typeof(sum), i::Int) -> (x -> x-3, f, (i,)))
@testset "Basic advice" begin
# Application of advice
@test sump1((identity, sum, (1,), (;))) ==
(identity, sum, (2,), (;))
@test sump1(sum, 1) == 2
@test sump1a(sum, 1) == 2
@test sump1b(sum, 1) == 2
@test sump1c(sum, 1) == 2
@test_throws ErrorException sump1x(sum, 1) == 2
# Invalid advice function
@test_throws ArgumentError Advice(() -> ())
# Pass-through of `post`
@test sump1((sqrt, sum, (1,), (;))) ==
(sqrt, sum, (2,), (;))
# Matching the argument
@test sump1((identity, sum, ([1],), (;))) ==
(identity, sum, ([1],), (;))
@test sump1(sum, [1]) == 1
# Matching the kwargs
@test sump1((identity, sum, (1,), (dims=3,))) ==
(identity, sum, (1,), (dims = 3,))
# Matching the function
@test sump1((identity, sqrt, (1,), (;))) ==
(identity, sqrt, (1,), (;))
let # Using invokelatest on the advice function
thing(x) = x^2
h(x) = x+1
thing_a = Advice((f::typeof(thing), i::Int) -> (f, (h(i),)))
@test thing_a((identity, thing, (2,), (;))) ==
(identity, thing, (3,), (;))
h(x) = x+2
@test thing_a((identity, thing, (2,), (;))) ==
(identity, thing, (4,), (;))
end
end
@testset "Amalgamation" begin
amlg12 = AdviceAmalgamation(
sump1 ∘ sumx2, [sumx2, sump1], String[], String[])
@test amlg12.advisors == AdviceAmalgamation([sumx2, sump1]).advisors
@test AdviceAmalgamation(amlg12).advisors == Advice[] # no plugins
amlg21 = AdviceAmalgamation(
sumx2 ∘ sump1, [sump1, sumx2], String[], String[])
amlg321 = AdviceAmalgamation(
summ3 ∘ sumx2 ∘ sump1, [sump1, sumx2, summ3], String[], String[])
amlg213 = AdviceAmalgamation(
sumx2 ∘ sump1 ∘ summ3, [summ3, sump1, sumx2], String[], String[])
@test amlg12((identity, sum, (2,), (;))) == (identity, sum, (5,), (;))
@test amlg12(sum, 2) == 5
@test amlg21(sum, 2) == 6
@test amlg321(sum, 2) == 3
@test amlg213(sum, 2) == 3
end
@testset "Plugin loading" begin
# Empty state
amlg = empty(AdviceAmalgamation)
@test amlg.adviseall == identity
@test amlg.advisors == Advice[]
@test amlg.plugins_wanted == String[]
@test amlg.plugins_used == String[]
# Create a plugin
plg = Plugin(string(gensym()), [sump1, sumx2])
push!(PLUGINS, plg)
@test Plugin("", [sumx2.f]).advisors == Plugin("", [sumx2]).advisors
# Desire the plugin, then check the advice is incorperated correctly
push!(amlg.plugins_wanted, plg.name)
@test amlg.adviseall == sump1 ∘ sumx2
@test amlg.advisors == [sumx2, sump1]
@test amlg.plugins_wanted == [plg.name]
@test amlg.plugins_used == [plg.name]
@test AdviceAmalgamation(amlg).advisors == amlg.advisors
let cltn = DataCollection()
push!(cltn.plugins, plg.name)
@test AdviceAmalgamation(cltn).advisors == amlg.advisors
end
# Display
@test sprint(show, amlg) == "AdviceAmalgamation($(plg.name) ✔)"
end
@testset "Advice macro" begin
@test :($(GlobalRef(DataToolkitBase, :_dataadvisecall))(func, x)) ==
@macroexpand @advise func(x)
@test :($(GlobalRef(DataToolkitBase, :_dataadvisecall))(func, x, y, z)) ==
@macroexpand @advise func(x, y, z)
@test :($(GlobalRef(DataToolkitBase, :_dataadvisecall))(func; a=1, b)) ==
@macroexpand @advise func(; a=1, b)
@test :($(GlobalRef(DataToolkitBase, :_dataadvisecall))(func, x, y, z; a=1, b)) ==
@macroexpand @advise func(x, y, z; a=1, b)
@test :(($(GlobalRef(DataToolkitBase, :_dataadvise))(a))(func, x)) ==
@macroexpand @advise a func(x)
@test :(($(GlobalRef(DataToolkitBase, :_dataadvise))(source(a)))(func, x)) ==
@macroexpand @advise source(a) func(x)
@test_throws LoadError eval(:(@advise (1, 2)))
@test_throws LoadError eval(:(@advise f()))
@test 2 == @advise sump1 sum(1)
@test 2 == @advise [sump1] sum(1)
@test 2 == @advise AdviceAmalgamation([sump1]) sum(1)
end
deleteat!(PLUGINS, length(PLUGINS)) # remove `plg`
end
import DataToolkitBase: smallify
@testset "SmallDict" begin
@testset "Construction" begin
@test SmallDict() == SmallDict{Any, Any}([], [])
@test SmallDict{Any, Any}() == SmallDict{Any, Any}([], [])
@test SmallDict(:a => 1) == SmallDict{Symbol, Int}([:a], [1])
@test SmallDict([:a => 1]) == SmallDict{Symbol, Int}([:a], [1])
@test SmallDict{Symbol, Int}(:a => 1) == SmallDict{Symbol, Int}([:a], [1])
@test_throws MethodError SmallDict{String, Int}(:a => 1)
@test SmallDict(:a => 1, :b => 2) == SmallDict{Symbol, Int}([:a, :b], [1, 2])
@test SmallDict(:a => 1, :b => '1') == SmallDict{Symbol, Any}([:a, :b], [1, '1'])
@test SmallDict(:a => 1, "b" => '1') == SmallDict{Any, Any}([:a, "b"], [1, '1'])
end
@testset "Conversion" begin
@test convert(SmallDict, Dict(:a => 1)) == SmallDict{Symbol, Int}([:a], [1])
@test convert(SmallDict, Dict{Symbol, Any}(:a => 1)) == SmallDict{Symbol, Any}([:a], [1])
@test convert(SmallDict, Dict(:a => 1, :b => '1')) == SmallDict{Symbol, Any}([:a, :b], [1, '1'])
@test smallify(Dict(:a => Dict(:b => Dict(:c => 3)))) ==
SmallDict(:a => SmallDict(:b => SmallDict(:c => 3)))
end
@testset "AbstractDict interface" begin
d = SmallDict{Symbol, Int}()
@test length(d) == 0
@test haskey(d, :a) == false
@test get(d, :a, nothing) === nothing
@test iterate(d) === nothing
@test (d[:a] = 1) == 1
@test d[:a] == 1
@test collect(d) == [:a => 1]
@test length(d) == 1
@test haskey(d, :a) == true
@test get(d, :a, nothing) == 1
@test iterate(d) == (:a => 1, 2)
@test iterate(d, 2) === nothing
@test (d[:b] = 2) == 2
@test length(d) == 2
@test keys(d) == [:a, :b]
@test values(d) == [1, 2]
@test iterate(d, 2) === (:b => 2, 3)
@test Dict(d) == Dict(:a => 1, :b => 2)
@test (d[:a] = 3) == 3
@test d[:a] == 3
@test values(d) == [3, 2]
@test_throws KeyError d[:c]
delete!(d, :a)
@test keys(d) == [:b]
delete!(d, :b)
@test d == empty(d)
end
end
@testset "QualifiedType" begin
@testset "Construction" begin
@test QualifiedType(:a, :b) == QualifiedType(:a, :b, ())
@test QualifiedType(Any) == QualifiedType(:Core, :Any, ())
@test QualifiedType(Int) == QualifiedType(:Core, nameof(Int), ())
@test QualifiedType(IO) == QualifiedType(:Core, :IO, ())
# This test currently fails due to typevar inequivalence
# @test QualifiedType(QualifiedType) ==
# QualifiedType(:DataToolkitBase, :QualifiedType, (TypeVar(:T, Union{}, Tuple),))
@test QualifiedType(QualifiedType(:a, :b)) == QualifiedType(:a, :b, ())
end
@testset "Typeification" begin
@test typeify(QualifiedType(:a, :b)) === nothing
@test typeify(QualifiedType(:Core, :Int)) == Int
@test typeify(QualifiedType(:Core, :IO)) == IO
@test typeify(QualifiedType(:DataToolkitBase, :QualifiedType, ())) == QualifiedType
@test typeify(QualifiedType(:Core, :Array, (QualifiedType(:Core, :Integer, ()), 1))) ==
Vector{Integer}
# Test module expansion with unexported type
@test typeify(QualifiedType(:Main, :AnyDict, ())) === nothing
@test typeify(QualifiedType(:Main, :AnyDict, ()), mod=Base) == Base.AnyDict
end
@testset "Subtyping" begin
@test QualifiedType(Int) ⊆ QualifiedType(Integer)
@test Int ⊆ QualifiedType(Integer)
@test QualifiedType(Int) ⊆ Integer
@test !(QualifiedType(Integer) ⊆ QualifiedType(Int))
@test !(Integer ⊆ QualifiedType(Int))
@test !(QualifiedType(Integer) ⊆ Int)
@test !(QualifiedType(:a, :b) ⊆ Integer)
@test !(Integer ⊆ QualifiedType(:a, :b))
@test QualifiedType(:a, :b) ⊆ QualifiedType(:a, :b)
@test !(QualifiedType(:Main, :AnyDict, ()) ⊆ AbstractDict)
@test ⊆(QualifiedType(:Main, :AnyDict, ()), AbstractDict, mod = Base)
end
end
import DataToolkitBase: get_package, addpkg
@testset "UsePkg" begin
@testset "add/get package" begin
test = Base.PkgId(Base.UUID("8dfed614-e22c-5e08-85e1-65c5234f0b40"), "Test")
@test get_package(test) === Test
@test_throws UnregisteredPackage get_package(@__MODULE__, :Test)
@test addpkg(@__MODULE__, :Test, "8dfed614-e22c-5e08-85e1-65c5234f0b40") isa Any
@test @addpkg(Test, "8dfed614-e22c-5e08-85e1-65c5234f0b40") isa Any
@test get_package(@__MODULE__, :Test) === Test
end
@testset "@import" begin
nolinenum(blk) = Expr(:block, filter(e -> !(e isa LineNumberNode), blk.args)...)
getpkg = GlobalRef(DataToolkitBase, :get_package)
mod = GlobalRef(Core, :Module)
gensymidx() = parse(Int, last(split(String(gensym()), '#')))
function nextgensym(tag::String, next::Int=1)
Symbol("##$tag#$(gensymidx()+next)")
end
@test quote
A = $getpkg($Main, :A)
A isa $mod || return A
end |> nolinenum == @macroexpand @import A
@test quote
A = $getpkg($Main, :A)
A isa $mod || return A
B = $getpkg($Main, :B)
B isa $mod || return B
C = $getpkg($Main, :C)
C isa $mod || return C
end |> nolinenum == @macroexpand @import A, B, C
@test quote
B = $getpkg($Main, :A)
B isa $mod || return B
end |> nolinenum == @macroexpand @import A as B
@test quote
A = $getpkg($Main, :A)
A isa $mod || return A
C = $getpkg($Main, :B)
C isa $mod || return C
D = $getpkg($Main, :D)
D isa $mod || return D
end |> nolinenum == @macroexpand @import A, B as C, D
sA = nextgensym("A")
@test quote
$sA = $getpkg($Main, :A)
$sA isa $mod || return $sA
B = ($sA).B
end |> nolinenum == @macroexpand @import A.B
@test quote
A = $getpkg($Main, :A)
A isa $mod || return A
B = A.B
end |> nolinenum == @macroexpand @import A, A.B
@test quote
A = $getpkg($Main, :A)
A isa $mod || return A
C = A.B
end |> nolinenum == @macroexpand @import A, A.B as C
@test quote
B = $getpkg($Main, :A)
B isa $mod || return B
C = B.B.C
end |> nolinenum == @macroexpand @import A as B, A.B.C
sA = nextgensym("A")
@test quote
$sA = $getpkg($Main, :A)
$sA isa $mod || return $sA
B = ($sA).B
end |> nolinenum == @macroexpand @import A: B
sA = nextgensym("A")
@test quote
$sA = $getpkg($Main, :A)
$sA isa $mod || return $sA
C = ($sA).B.C
end |> nolinenum == @macroexpand @import A: B.C
sA = nextgensym("A", 3)
sB = nextgensym("B")
@test quote
$sA = $getpkg($Main, :A)
$sA isa $mod || return $sA
$sB = ($sA).B
C = ($sB).C
end |> nolinenum == @macroexpand @import A.B: C
sA = nextgensym("A")
@test quote
$sA = $getpkg($Main, :A)
$sA isa $mod || return $sA
C = ($sA).B
end |> nolinenum == @macroexpand @import A: B as C
sA = nextgensym("A")
@test quote
$sA = $getpkg($Main, :A)
$sA isa $mod || return $sA
C = ($sA).B
E = ($sA).D
end |> nolinenum == @macroexpand @import A: B as C, D as E
sA = nextgensym("A")
@test quote
$sA = $getpkg($Main, :A)
$sA isa $mod || return $sA
B = ($sA).B
C = ($sA).C
D = ($sA).D
end |> nolinenum == @macroexpand @import A: B, C, D
sA = nextgensym("A")
@test quote
$sA = $getpkg($Main, :A)
$sA isa $mod || return $sA
C = ($sA).B
D = ($sA).D
F = ($sA).E
G = ($sA).G
end |> nolinenum == @macroexpand @import A: B as C, D, E as F, G
end
end
@testset "stringification" begin
@testset "QualifiedType" begin
for (str, qt) in [("a.b", QualifiedType(:a, :b)),
("a.b.c", QualifiedType(:a, [:b], :c)),
("a.b.c.d", QualifiedType(:a, [:b, :c], :d)),
("String", QualifiedType(String)),
("a.b{c.d}", QualifiedType(:a, :b, (QualifiedType(:c, :d),))),
("a.b.c{d.e.f}", QualifiedType(:a, [:b], :c, (QualifiedType(:d, [:e], :f),))),
("Array{Bool,2}", QualifiedType(Array{Bool, 2})),
("Array{Array{Array{<:Integer,1},1},1}",
QualifiedType(Array{Array{Array{<:Integer,1},1},1})),
("Ref{I<:Integer}", QualifiedType(Ref{I} where {I <: Integer}))]
@test str == string(qt)
# Due to TypeVar comparison issues, instead of
# the following test, we'll do a round-trip instead.
# @test parse(QualifiedType, str) == qt
@test str == string(parse(QualifiedType, str))
end
end
@testset "Identifiers" begin
for (istr, ident) in [("a", Identifier(nothing, "a", nothing, SmallDict{String, Any}())),
("a:b", Identifier("a", "b", nothing, SmallDict{String, Any}())),
("a::Main.sometype", Identifier(nothing, "a", QualifiedType(:Main, :sometype), SmallDict{String, Any}())),
("a:b::Bool", Identifier("a", "b", QualifiedType(:Core, :Bool), SmallDict{String, Any}()))]
@test parse_ident(istr) == ident
@test istr == string(ident)
end
end
end
@testset "DataSet Parameters" begin
refpre, refpost = DATASET_REFERENCE_WRAPPER
datatoml = """
data_config_version = 0
uuid = "1c59ad24-f655-4903-b791-f3ef3afc5df1"
name = "datatest"
config.ref = "$(refpre)adataset$(refpost)"
[[adataset]]
uuid = "8c12e6b4-6987-44e9-a33d-efe2ad60f501"
self = "$(refpre)adataset$(refpost)"
others = { b = "$(refpre)bdataset$(refpost)" }
[[bdataset]]
uuid = "aa5ba7ab-cabd-4c08-8e4e-78d516e15801"
other = ["$(refpre)adataset$(refpost)"]
"""
collection = read(IOBuffer(datatoml), DataCollection)
adataset, bdataset = sort(collection.datasets, by=d -> d.name)
@test referenced_datasets(adataset) == [adataset, bdataset]
@test referenced_datasets(bdataset) == [adataset]
@test get(adataset, "self") == adataset
@test adataset == @getparam adataset."self"
@test get(adataset, "others")["b"] == bdataset
@test get(bdataset, "other") == [adataset]
# Collection config cannot hold data set refs
@test get(collection, "ref") == "$(refpre)adataset$(refpost)"
end
# Ensure this runs at the end (because it defines new methods, and may affect
# state). It should simulate a basic workflow.
@testset "Dry run" begin
# Basic storage/loader implementation for testing
@eval begin
import DataToolkitBase: getstorage, load, supportedtypes
function getstorage(storage::DataStorage{:raw}, T::Type)
get(storage, "value", nothing)::Union{T, Nothing}
end
supportedtypes(::Type{DataStorage{:raw}}, spec::SmallDict{String, Any}) =
[QualifiedType(typeof(get(spec, "value", nothing)))]
function load(::DataLoader{:passthrough}, from::T, ::Type{T}) where {T <: Any}
from
end
supportedtypes(::Type{DataLoader{:passthrough}}, _::SmallDict{String, Any}, dataset::DataSet) =
reduce(vcat, getproperty.(dataset.storage, :type)) |> unique
end
fieldeqn_parent_stack = []
function fieldeqn(a::T, b::T) where {T} # field equal nested
push!(fieldeqn_parent_stack, a)
if T <: Vector
eq = all([fieldeqn(ai, bi) for (ai, bi) in zip(a, b)])
pop!(fieldeqn_parent_stack)
eq
elseif isempty(fieldnames(T))
a == b || begin
@info "[fieldeqn] $T differs" a b
pop!(fieldeqn_parent_stack)
false
end
else
for field in fieldnames(T)
if getfield(a, field) in fieldeqn_parent_stack
elseif hasmethod(iterate, Tuple{fieldtype(T, field)}) &&
!all([fieldeqn(af, bf) for (af, bf) in
zip(getfield(a, field), getfield(b, field))])
@info "[fieldeqn] iterable $field of $T differs" a b
pop!(fieldeqn_parent_stack)
return false
elseif getfield(a, field) !== a && !fieldeqn(getfield(a, field), getfield(b, field))
@info "[fieldeqn] $field of $T differs" a b
pop!(fieldeqn_parent_stack)
return false
end
end
pop!(fieldeqn_parent_stack)
true
end
end
datatoml = """
data_config_version = 0
uuid = "84068d44-24db-4e28-b693-58d2e1f59d05"
name = "datatest"
config.setting = 123
config.nested.value = 4
[[dataset]]
uuid = "d9826666-5049-4051-8d2e-fe306c20802c"
property = 456
[[dataset.storage]]
driver = "raw"
value = [1, 2, 3]
[[dataset.loader]]
driver = "passthrough"
"""
datatoml_full = """
data_config_version = 0
uuid = "84068d44-24db-4e28-b693-58d2e1f59d05"
name = "datatest"
[config]
setting = 123
[config.nested]
value = 4
[[dataset]]
uuid = "d9826666-5049-4051-8d2e-fe306c20802c"
property = 456
[[dataset.storage]]
driver = "raw"
priority = 1
type = "Array{Int64,1}"
value = [1, 2, 3]
[[dataset.loader]]
driver = "passthrough"
priority = 1
type = "Array{Int64,1}"
"""
@test fieldeqn(read(IOBuffer(datatoml), DataCollection),
read(IOBuffer(datatoml_full), DataCollection))
collection = read(IOBuffer(datatoml), DataCollection)
@testset "Collection parsed properties" begin
@test collection.version == 0
@test collection.uuid == Base.UUID("84068d44-24db-4e28-b693-58d2e1f59d05")
@test collection.name == "datatest"
@test collection.parameters ==
SmallDict{String, Any}("setting" => 123, "nested" => SmallDict{String, Any}("value" => 4))
@test collection.plugins == String[]
@test collection.path === nothing
@test collection.mod == Main
@test length(collection.datasets) == 1
end
@test_throws EmptyStackError dataset("dataset")
@test_throws EmptyStackError getlayer(nothing)
@test (collection = loadcollection!(IOBuffer(datatoml))) isa Any # if this actually goes wrong, it should be caught by subsequent tests
@test getlayer(nothing) === collection
@test_throws UnresolveableIdentifier getlayer("nope")
@test_throws UnresolveableIdentifier getlayer(Base.UUID("11111111-24db-4e28-b693-58d2e1f59d05"))
@testset "DataSet parsed properties" begin
@test dataset("dataset") isa DataSet
@test dataset("dataset").name == "dataset"
@test dataset("dataset").uuid == Base.UUID("d9826666-5049-4051-8d2e-fe306c20802c")
@test dataset("dataset").parameters == SmallDict{String, Any}("property" => 456)
end
@testset "Store/Load" begin
@test length(dataset("dataset").storage) == 1
@test dataset("dataset").storage[1].dataset === dataset("dataset")
@test dataset("dataset").storage[1].parameters == SmallDict{String, Any}("value" => [1, 2, 3])
@test dataset("dataset").storage[1].type == [QualifiedType(Vector{Int})]
@test length(dataset("dataset").loaders) == 1
@test dataset("dataset").loaders[1].dataset === dataset("dataset")
@test dataset("dataset").loaders[1].parameters == SmallDict{String, Any}()
@test dataset("dataset").loaders[1].type == [QualifiedType(Vector{Int})]
@test open(dataset("dataset"), Vector{Int}) == [1, 2, 3]
@test read(dataset("dataset"), Vector{Int}) == [1, 2, 3]
@test read(dataset("dataset")) == [1, 2, 3]
@test read(parse(Identifier, "dataset"), Vector{Int}) == [1, 2, 3]
@test read(parse(Identifier, "dataset::Vector{Int}")) == [1, 2, 3]
end
@testset "Identifier" begin
@test_throws UnresolveableIdentifier dataset("nonexistent")
@test_throws UnresolveableIdentifier resolve(parse(Identifier, "nonexistent"))
@test resolve(parse(Identifier, "dataset")) == dataset("dataset")
@test resolve(parse(Identifier, "datatest:dataset")) == dataset("dataset")
@test resolve(parse(Identifier, "dataset")) == dataset("dataset")
@test resolve(parse(Identifier, "dataset::Vector{Int}")) == read(dataset("dataset"))
@test resolve(parse(Identifier, "dataset::Vector{Int}"), resolvetype=false) == dataset("dataset")
for (iargs, (col, ds)) in [((), ("datatest", "dataset")),
((:name,), ("datatest", "dataset")),
((:uuid,), (Base.UUID("84068d44-24db-4e28-b693-58d2e1f59d05"), Base.UUID("d9826666-5049-4051-8d2e-fe306c20802c"))),
((:uuid, :name), (Base.UUID("84068d44-24db-4e28-b693-58d2e1f59d05"), "dataset")),
((:name, :uuid), ("datatest", Base.UUID("d9826666-5049-4051-8d2e-fe306c20802c")))]
ident = Identifier(dataset("dataset"), iargs...)
@test ident == Identifier(col, ds, nothing, SmallDict{String, Any}("property" => 456))
@test dataset("dataset") === resolve(ident)
@test parse(Identifier, string(ident)) == Identifier(col, ds, nothing, SmallDict{String, Any}())
end
@test_throws ArgumentError Identifier(dataset("dataset"), :err)
@test dataset("dataset") == dataset("dataset", "property" => 456)
@test_throws UnresolveableIdentifier dataset("dataset", "property" => 321)
let io = IOBuffer()
write(io, collection)
@test String(take!(io)) == datatoml_full
end
end
@testset "Manipulation" begin
@test stack_index(collection.uuid) == stack_index(collection.name) == stack_index(1)
@test stack_index(2) === nothing
@test plugin_add(["test"]).plugins == ["test"]
@test plugin_add(["test2"]).plugins == ["test", "test2"]
@test plugin_list() == ["test", "test2"]
@test plugin_remove(["test"]).plugins == ["test2"]
@test plugin_remove(["test2"]).plugins == String[]
@test config_get(collection, ["setting"]) == 123
@test config_get(collection, ["setting", "none"]) ===nothing
@test config_get(collection, ["nested", "value"]) == 4
@test config_get(collection, ["nested", "nope"]) ===nothing
@test config_set(["some", "nested", "val"], 5).parameters["some"] ==
SmallDict{String, Any}("nested" => SmallDict{String, Any}("val" => 5))
@test config_get(["some", "nested", "val"]) == 5
@test get(config_unset(collection, ["some"]), "some") === nothing
end
end
| DataToolkitBase | https://github.com/tecosaur/DataToolkitBase.jl.git |
|
[
"MIT"
] | 0.8.3 | 6338b3ac7b0f1c55f5f2a457df49529a9287aca2 | code | 1869 | using Documenter
using DocStringExtensions
using UnitfulAstrodynamics
makedocs(
modules=[
UnitfulAstrodynamics,
UnitfulAstrodynamics.CommonTypes,
UnitfulAstrodynamics.TwoBody,
UnitfulAstrodynamics.NBody,
UnitfulAstrodynamics.ThreeBody,
UnitfulAstrodynamics.Propagators,
UnitfulAstrodynamics.AstroPlots
],
format=Documenter.HTML(),
sitename="UnitfulAstrodynamics.jl",
authors = "Joey Carpinelli",
pages=[
"Guide" => "index.md",
"Overview" => Any[
"About" => "Overview/about.md",
"Getting Stated" => "Overview/getting-started.md"
],
"Two-body" => Any[
"Data Structures and Types" => "TwoBody/types.md",
"Functions" => "TwoBody/functions.md"
],
"Three-body" => Any[
"Data Structures and Types" => "ThreeBody/types.md",
"Functions" => "ThreeBody/functions.md"
],
"N-body" => Any[
"Data Structures and Types" => "NBody/types.md",
"Functions" => "NBody/functions.md"
],
"Propagators" => Any[
"Data Structures and Types" => "Propagators/types.md",
"Functions" => "Propagators/functions.md"
],
"Maneuvers" => Any[
"Data Structures and Types" => "Maneuvers/types.md",
"Functions" => "Maneuvers/functions.md"
],
"Plotting" => Any[
"Functions" => "AstroPlots/functions.md"
],
"Common Types" => Any[
"Types" => "CommonTypes/types.md"
]
]
)
deploydocs(
target = "build",
repo="github.com/cadojo/UnitfulAstrodynamics.jl.git",
branch = "gh-pages",
deps = nothing,
make = nothing,
devbranch = "main",
versions = ["stable" => "v^", "manual", "v#.#", "v#.#.#"],
)
| UnitfulAstrodynamics | https://github.com/cadojo/UnitfulAstrodynamics.jl.git |
|
[
"MIT"
] | 0.8.3 | 6338b3ac7b0f1c55f5f2a457df49529a9287aca2 | code | 1033 | """
Julia package developed alongside ENAE601 at the University of Maryland.
Includes structures and functions to handle common Astrodynamics problems.
"""
module UnitfulAstrodynamics
# References:
#
# I troubleshooted Julia's module scope/export rules for a while.
# Ultimately, I referenced [1] as an example for how to structure
# submodules, and how to using `Reexport.jl` to export required
# package dependencies.
#
# [1] https://github.com/JuliaAstro/AstroBase.jl/blob/master/src/AstroBase.jl
using Reexport
include("CommonTypes/CommonTypes.jl")
include("TwoBody/TwoBody.jl")
include("ThreeBody/ThreeBody.jl")
include("NBody/NBody.jl")
include("Propagators/Propagators.jl")
include("Maneuvers/Maneuvers.jl")
include("AstroPlots/AstroPlots.jl")
@reexport using .CommonTypes
@reexport using .TwoBody
@reexport using .ThreeBody
@reexport using .NBody
@reexport using .Propagators
@reexport using .Maneuvers
@reexport using .AstroPlots
include("Misc/DocStringExtensions.jl")
include("Misc/UnitfulAliases.jl")
end # module
| UnitfulAstrodynamics | https://github.com/cadojo/UnitfulAstrodynamics.jl.git |
|
[
"MIT"
] | 0.8.3 | 6338b3ac7b0f1c55f5f2a457df49529a9287aca2 | code | 486 | """
Uses `Plots.jl` to plot propagation results for the n-body problem,
and the two-body problem. Uses `Plots.jl`
"""
module AstroPlots
using Reexport
@reexport using ..CommonTypes
include("../Misc/DocStringExtensions.jl")
include("../Misc/UnitfulAliases.jl")
using ..TwoBody
using ..ThreeBody
using ..NBody
using ..Propagators
using Plots
using Plots.PlotMeasures
export orbitplot, lagrangeplot
include("PlotTwoBody.jl")
include("PlotNBody.jl")
include("PlotThreeBody.jl")
end
| UnitfulAstrodynamics | https://github.com/cadojo/UnitfulAstrodynamics.jl.git |
Subsets and Splits
No community queries yet
The top public SQL queries from the community will appear here once available.