title
stringlengths 1
100
| titleSlug
stringlengths 3
77
| Java
int64 0
1
| Python3
int64 1
1
| content
stringlengths 28
44.4k
| voteCount
int64 0
3.67k
| question_content
stringlengths 65
5k
| question_hints
stringclasses 970
values |
---|---|---|---|---|---|---|---|
Python || recursion || backtracking | maximum-rows-covered-by-columns | 0 | 1 | # Backtracking + recursion\n## Time -> O(2^N) {N is length of columns}\n## Space -> O(cols)\n\n```\nclass Solution:\n def maximumRows(self, mat: List[List[int]], cols: int) -> int:\n res = []\n M = len(mat)\n N = len(mat[0])\n def check(seen):\n count = 0\n for row in mat:\n flag = True\n for c in range(N): \n if row[c] == 1:\n if c in seen:\n continue\n else:\n flag = False\n break\n if flag: \n count +=1 \n res.append(count)\n \n def solve(c,seen,cols):\n if cols == 0:\n check(seen)\n return\n if c == N:\n return\n else:\n seen.add(c)\n solve(c+1,seen,cols-1)\n seen.remove(c)\n solve(c+1,seen,cols)\n seen = set()\n solve(0,seen,cols)\n return max(res)\n``` | 3 | You are given a **0-indexed** `m x n` binary matrix `matrix` and an integer `numSelect`, which denotes the number of **distinct** columns you must select from `matrix`.
Let us consider `s = {c1, c2, ...., cnumSelect}` as the set of columns selected by you. A row `row` is **covered** by `s` if:
* For each cell `matrix[row][col]` (`0 <= col <= n - 1`) where `matrix[row][col] == 1`, `col` is present in `s` or,
* **No cell** in `row` has a value of `1`.
You need to choose `numSelect` columns such that the number of rows that are covered is **maximized**.
Return _the **maximum** number of rows that can be **covered** by a set of_ `numSelect` _columns._
**Example 1:**
**Input:** matrix = \[\[0,0,0\],\[1,0,1\],\[0,1,1\],\[0,0,1\]\], numSelect = 2
**Output:** 3
**Explanation:** One possible way to cover 3 rows is shown in the diagram above.
We choose s = {0, 2}.
- Row 0 is covered because it has no occurrences of 1.
- Row 1 is covered because the columns with value 1, i.e. 0 and 2 are present in s.
- Row 2 is not covered because matrix\[2\]\[1\] == 1 but 1 is not present in s.
- Row 3 is covered because matrix\[2\]\[2\] == 1 and 2 is present in s.
Thus, we can cover three rows.
Note that s = {1, 2} will also cover 3 rows, but it can be shown that no more than three rows can be covered.
**Example 2:**
**Input:** matrix = \[\[1\],\[0\]\], numSelect = 1
**Output:** 2
**Explanation:** Selecting the only column will result in both rows being covered since the entire matrix is selected.
Therefore, we return 2.
**Constraints:**
* `m == matrix.length`
* `n == matrix[i].length`
* `1 <= m, n <= 12`
* `matrix[i][j]` is either `0` or `1`.
* `1 <= numSelect <= n` | null |
Python Simple Solution | maximum-rows-covered-by-columns | 0 | 1 | ```\nclass Solution:\n def maximumRows(self, mat: List[List[int]], cols: int) -> int:\n n,m = len(mat),len(mat[0])\n ans = 0\n\n def check(state,row,rowIncludedCount):\n nonlocal ans\n if row==n:\n if sum(state)<=cols:\n ans = max(ans,rowIncludedCount)\n return\n \n check(state[::],row+1,rowIncludedCount)\n for j in range(m):\n if mat[row][j]==1:\n state[j] = 1\n check(state,row+1,rowIncludedCount+1)\n \n check([0]*m,0,0)\n return ans\n``` | 5 | You are given a **0-indexed** `m x n` binary matrix `matrix` and an integer `numSelect`, which denotes the number of **distinct** columns you must select from `matrix`.
Let us consider `s = {c1, c2, ...., cnumSelect}` as the set of columns selected by you. A row `row` is **covered** by `s` if:
* For each cell `matrix[row][col]` (`0 <= col <= n - 1`) where `matrix[row][col] == 1`, `col` is present in `s` or,
* **No cell** in `row` has a value of `1`.
You need to choose `numSelect` columns such that the number of rows that are covered is **maximized**.
Return _the **maximum** number of rows that can be **covered** by a set of_ `numSelect` _columns._
**Example 1:**
**Input:** matrix = \[\[0,0,0\],\[1,0,1\],\[0,1,1\],\[0,0,1\]\], numSelect = 2
**Output:** 3
**Explanation:** One possible way to cover 3 rows is shown in the diagram above.
We choose s = {0, 2}.
- Row 0 is covered because it has no occurrences of 1.
- Row 1 is covered because the columns with value 1, i.e. 0 and 2 are present in s.
- Row 2 is not covered because matrix\[2\]\[1\] == 1 but 1 is not present in s.
- Row 3 is covered because matrix\[2\]\[2\] == 1 and 2 is present in s.
Thus, we can cover three rows.
Note that s = {1, 2} will also cover 3 rows, but it can be shown that no more than three rows can be covered.
**Example 2:**
**Input:** matrix = \[\[1\],\[0\]\], numSelect = 1
**Output:** 2
**Explanation:** Selecting the only column will result in both rows being covered since the entire matrix is selected.
Therefore, we return 2.
**Constraints:**
* `m == matrix.length`
* `n == matrix[i].length`
* `1 <= m, n <= 12`
* `matrix[i][j]` is either `0` or `1`.
* `1 <= numSelect <= n` | null |
Python | Simple and straightforward | Combinations | Explained | maximum-rows-covered-by-columns | 0 | 1 | ```\nfrom itertools import combinations\nclass Solution:\n def maximumRows(self, mat: List[List[int]], cols: int) -> int:\n rows = 0\n \n # iterate over each combintion of the given numer of columns\n # the first argument passed to combinations is the list of columns\' indices\n # the second argumnet is the number of columns we need to consider\n # e.g., if mat consists of 3 columns, we\'ll iterate over the following list [(0,1), (0,2), (1,2)]\n for c in combinations(range(len(mat[0])), cols):\n \n # initialise temp which is the number of valid rows for the current iteration\n temp = 0\n \n # iterate over each row in mat\n for r in mat:\n valid = True\n \n # iterate over each number in the row (we use enumerate; the index of the number is the column\'s index)\n # check if each number 1 present in the row is also present among columns we consider in the current most outer iteration (c)\n # if that\'s not the case, the row is not valid\n for col, n in enumerate(r):\n if valid and n == 1 and col not in c:\n valid = False\n if valid:\n temp += 1\n \n # at the end of the current most outer iteration (c), check if the current number of valid rows (temp) is bigger then the previous one\n rows = max(rows, temp)\n return rows\n```\n | 1 | You are given a **0-indexed** `m x n` binary matrix `matrix` and an integer `numSelect`, which denotes the number of **distinct** columns you must select from `matrix`.
Let us consider `s = {c1, c2, ...., cnumSelect}` as the set of columns selected by you. A row `row` is **covered** by `s` if:
* For each cell `matrix[row][col]` (`0 <= col <= n - 1`) where `matrix[row][col] == 1`, `col` is present in `s` or,
* **No cell** in `row` has a value of `1`.
You need to choose `numSelect` columns such that the number of rows that are covered is **maximized**.
Return _the **maximum** number of rows that can be **covered** by a set of_ `numSelect` _columns._
**Example 1:**
**Input:** matrix = \[\[0,0,0\],\[1,0,1\],\[0,1,1\],\[0,0,1\]\], numSelect = 2
**Output:** 3
**Explanation:** One possible way to cover 3 rows is shown in the diagram above.
We choose s = {0, 2}.
- Row 0 is covered because it has no occurrences of 1.
- Row 1 is covered because the columns with value 1, i.e. 0 and 2 are present in s.
- Row 2 is not covered because matrix\[2\]\[1\] == 1 but 1 is not present in s.
- Row 3 is covered because matrix\[2\]\[2\] == 1 and 2 is present in s.
Thus, we can cover three rows.
Note that s = {1, 2} will also cover 3 rows, but it can be shown that no more than three rows can be covered.
**Example 2:**
**Input:** matrix = \[\[1\],\[0\]\], numSelect = 1
**Output:** 2
**Explanation:** Selecting the only column will result in both rows being covered since the entire matrix is selected.
Therefore, we return 2.
**Constraints:**
* `m == matrix.length`
* `n == matrix[i].length`
* `1 <= m, n <= 12`
* `matrix[i][j]` is either `0` or `1`.
* `1 <= numSelect <= n` | null |
Simple Solution | check-distances-between-same-letters | 0 | 1 | # Intuition\n<!-- Describe your first thoughts on how to solve this problem. -->\n\n# Approach\n<!-- Describe your approach to solving the problem. -->\n\n# Complexity\n- Time complexity:\n<!-- Add your time complexity here, e.g. $$O(n)$$ -->\n\n- Space complexity:\n<!-- Add your space complexity here, e.g. $$O(n)$$ -->\n\n# Code\n```\nclass Solution:\n def checkDistances(self, s: str, distance: List[int]) -> bool:\n d = s[::-1]\n if len(s)%2!=0:\n return False\n else:\n for i in s:\n #print((len(s)-d.index(i))-s.index(i)-1)\n if (len(s)-d.index(i)-1)-s.index(i)-1 != distance[ord(i)-ord(\'a\')]:\n return False\n return True\n\n``` | 1 | You are given a **0-indexed** string `s` consisting of only lowercase English letters, where each letter in `s` appears **exactly** **twice**. You are also given a **0-indexed** integer array `distance` of length `26`.
Each letter in the alphabet is numbered from `0` to `25` (i.e. `'a' -> 0`, `'b' -> 1`, `'c' -> 2`, ... , `'z' -> 25`).
In a **well-spaced** string, the number of letters between the two occurrences of the `ith` letter is `distance[i]`. If the `ith` letter does not appear in `s`, then `distance[i]` can be **ignored**.
Return `true` _if_ `s` _is a **well-spaced** string, otherwise return_ `false`.
**Example 1:**
**Input:** s = "abaccb ", distance = \[1,3,0,5,0,0,0,0,0,0,0,0,0,0,0,0,0,0,0,0,0,0,0,0,0,0\]
**Output:** true
**Explanation:**
- 'a' appears at indices 0 and 2 so it satisfies distance\[0\] = 1.
- 'b' appears at indices 1 and 5 so it satisfies distance\[1\] = 3.
- 'c' appears at indices 3 and 4 so it satisfies distance\[2\] = 0.
Note that distance\[3\] = 5, but since 'd' does not appear in s, it can be ignored.
Return true because s is a well-spaced string.
**Example 2:**
**Input:** s = "aa ", distance = \[1,0,0,0,0,0,0,0,0,0,0,0,0,0,0,0,0,0,0,0,0,0,0,0,0,0\]
**Output:** false
**Explanation:**
- 'a' appears at indices 0 and 1 so there are zero letters between them.
Because distance\[0\] = 1, s is not a well-spaced string.
**Constraints:**
* `2 <= s.length <= 52`
* `s` consists only of lowercase English letters.
* Each letter appears in `s` exactly twice.
* `distance.length == 26`
* `0 <= distance[i] <= 50` | null |
Python3 easy solution | check-distances-between-same-letters | 0 | 1 | \n\n# Complexity\n- Time complexity: O(n)\n<!-- Add your time complexity here, e.g. $$O(n)$$ -->\n\n- Space complexity: O(1)\n<!-- Add your space complexity here, e.g. $$O(n)$$ -->\n\n# Code\n```\nclass Solution:\n def checkDistances(self, s: str, dis: List[int]) -> bool:\n d = {}\n for i in range(len(s)):\n if s[i] not in d:\n d[s[i]] = i + 1\n else:\n if dis[ord(s[i])-ord(\'a\')] != i - d[s[i]]:\n return False\n return True\n\n``` | 1 | You are given a **0-indexed** string `s` consisting of only lowercase English letters, where each letter in `s` appears **exactly** **twice**. You are also given a **0-indexed** integer array `distance` of length `26`.
Each letter in the alphabet is numbered from `0` to `25` (i.e. `'a' -> 0`, `'b' -> 1`, `'c' -> 2`, ... , `'z' -> 25`).
In a **well-spaced** string, the number of letters between the two occurrences of the `ith` letter is `distance[i]`. If the `ith` letter does not appear in `s`, then `distance[i]` can be **ignored**.
Return `true` _if_ `s` _is a **well-spaced** string, otherwise return_ `false`.
**Example 1:**
**Input:** s = "abaccb ", distance = \[1,3,0,5,0,0,0,0,0,0,0,0,0,0,0,0,0,0,0,0,0,0,0,0,0,0\]
**Output:** true
**Explanation:**
- 'a' appears at indices 0 and 2 so it satisfies distance\[0\] = 1.
- 'b' appears at indices 1 and 5 so it satisfies distance\[1\] = 3.
- 'c' appears at indices 3 and 4 so it satisfies distance\[2\] = 0.
Note that distance\[3\] = 5, but since 'd' does not appear in s, it can be ignored.
Return true because s is a well-spaced string.
**Example 2:**
**Input:** s = "aa ", distance = \[1,0,0,0,0,0,0,0,0,0,0,0,0,0,0,0,0,0,0,0,0,0,0,0,0,0\]
**Output:** false
**Explanation:**
- 'a' appears at indices 0 and 1 so there are zero letters between them.
Because distance\[0\] = 1, s is not a well-spaced string.
**Constraints:**
* `2 <= s.length <= 52`
* `s` consists only of lowercase English letters.
* Each letter appears in `s` exactly twice.
* `distance.length == 26`
* `0 <= distance[i] <= 50` | null |
Python Solution | Easy Understanding | check-distances-between-same-letters | 0 | 1 | ```\nclass Solution:\n def mapper(self, s): # finds difference of indices\n result = dict()\n for letter in set(s):\n indices = []\n for idx, value in enumerate(s):\n if value == letter:\n indices.append(idx)\n result[letter] = indices[1] - indices[0] - 1\n return result # dict like {\'a\':1, \'b\':0}\n\n def checkDistances(self, s, distance) -> bool:\n alphabet = \'abcdefghijklmnopqrstuvwxyz\'\n for key, value in self.mapper(s).items():\n if distance[alphabet.index(key)] != value:\n return False\n return True\n``` | 1 | You are given a **0-indexed** string `s` consisting of only lowercase English letters, where each letter in `s` appears **exactly** **twice**. You are also given a **0-indexed** integer array `distance` of length `26`.
Each letter in the alphabet is numbered from `0` to `25` (i.e. `'a' -> 0`, `'b' -> 1`, `'c' -> 2`, ... , `'z' -> 25`).
In a **well-spaced** string, the number of letters between the two occurrences of the `ith` letter is `distance[i]`. If the `ith` letter does not appear in `s`, then `distance[i]` can be **ignored**.
Return `true` _if_ `s` _is a **well-spaced** string, otherwise return_ `false`.
**Example 1:**
**Input:** s = "abaccb ", distance = \[1,3,0,5,0,0,0,0,0,0,0,0,0,0,0,0,0,0,0,0,0,0,0,0,0,0\]
**Output:** true
**Explanation:**
- 'a' appears at indices 0 and 2 so it satisfies distance\[0\] = 1.
- 'b' appears at indices 1 and 5 so it satisfies distance\[1\] = 3.
- 'c' appears at indices 3 and 4 so it satisfies distance\[2\] = 0.
Note that distance\[3\] = 5, but since 'd' does not appear in s, it can be ignored.
Return true because s is a well-spaced string.
**Example 2:**
**Input:** s = "aa ", distance = \[1,0,0,0,0,0,0,0,0,0,0,0,0,0,0,0,0,0,0,0,0,0,0,0,0,0\]
**Output:** false
**Explanation:**
- 'a' appears at indices 0 and 1 so there are zero letters between them.
Because distance\[0\] = 1, s is not a well-spaced string.
**Constraints:**
* `2 <= s.length <= 52`
* `s` consists only of lowercase English letters.
* Each letter appears in `s` exactly twice.
* `distance.length == 26`
* `0 <= distance[i] <= 50` | null |
Python3 || 3 lines, T/M: 36ms/13.8MB | check-distances-between-same-letters | 0 | 1 | ```\nclass Solution: # Pretty much explains itself.\n def checkDistances(self, s: str, distance: List[int]) -> bool:\n \n d = defaultdict(list)\n\n for i, ch in enumerate(s):\n d[ch].append(i)\n\n return all(b-a-1 == distance[ord(ch)-97] for ch, (a,b) in d.items()) | 16 | You are given a **0-indexed** string `s` consisting of only lowercase English letters, where each letter in `s` appears **exactly** **twice**. You are also given a **0-indexed** integer array `distance` of length `26`.
Each letter in the alphabet is numbered from `0` to `25` (i.e. `'a' -> 0`, `'b' -> 1`, `'c' -> 2`, ... , `'z' -> 25`).
In a **well-spaced** string, the number of letters between the two occurrences of the `ith` letter is `distance[i]`. If the `ith` letter does not appear in `s`, then `distance[i]` can be **ignored**.
Return `true` _if_ `s` _is a **well-spaced** string, otherwise return_ `false`.
**Example 1:**
**Input:** s = "abaccb ", distance = \[1,3,0,5,0,0,0,0,0,0,0,0,0,0,0,0,0,0,0,0,0,0,0,0,0,0\]
**Output:** true
**Explanation:**
- 'a' appears at indices 0 and 2 so it satisfies distance\[0\] = 1.
- 'b' appears at indices 1 and 5 so it satisfies distance\[1\] = 3.
- 'c' appears at indices 3 and 4 so it satisfies distance\[2\] = 0.
Note that distance\[3\] = 5, but since 'd' does not appear in s, it can be ignored.
Return true because s is a well-spaced string.
**Example 2:**
**Input:** s = "aa ", distance = \[1,0,0,0,0,0,0,0,0,0,0,0,0,0,0,0,0,0,0,0,0,0,0,0,0,0\]
**Output:** false
**Explanation:**
- 'a' appears at indices 0 and 1 so there are zero letters between them.
Because distance\[0\] = 1, s is not a well-spaced string.
**Constraints:**
* `2 <= s.length <= 52`
* `s` consists only of lowercase English letters.
* Each letter appears in `s` exactly twice.
* `distance.length == 26`
* `0 <= distance[i] <= 50` | null |
Python | S.C. and T.C. = O(n) | number-of-ways-to-reach-a-position-after-exactly-k-steps | 0 | 1 | \n\n# Complexity\n- Time complexity : $$O(n)$$\n<!-- Add your time complexity here, e.g. $$O(n)$$ -->\n\n- Space complexity : $$O(n)$$\n<!-- Add your space complexity here, e.g. $$O(n)$$ -->\n\n# Code\n```\nclass Solution:\n\n def numberOfWays(self, startPos: int, endPos: int, k: int) -> int:\n mod = 10**9+7\n def nCr(n: int, r:int) ->int:\n if(r == 0):\n return 1\n v = [0] * (n+1)\n v[0] = 1\n for i in range(1, n+1):\n for j in range(r, 0, -1):\n v[j] = ((v[j] % mod) + (v[j-1] % mod)) % mod\n return v[r]\n diff = abs(startPos - endPos)\n if(diff > k or diff + k & 1):\n return 0\n r = (diff + k)//2\n return(nCr(k, r))\n``` | 1 | You are given two **positive** integers `startPos` and `endPos`. Initially, you are standing at position `startPos` on an **infinite** number line. With one step, you can move either one position to the left, or one position to the right.
Given a positive integer `k`, return _the number of **different** ways to reach the position_ `endPos` _starting from_ `startPos`_, such that you perform **exactly**_ `k` _steps_. Since the answer may be very large, return it **modulo** `109 + 7`.
Two ways are considered different if the order of the steps made is not exactly the same.
**Note** that the number line includes negative integers.
**Example 1:**
**Input:** startPos = 1, endPos = 2, k = 3
**Output:** 3
**Explanation:** We can reach position 2 from 1 in exactly 3 steps in three ways:
- 1 -> 2 -> 3 -> 2.
- 1 -> 2 -> 1 -> 2.
- 1 -> 0 -> 1 -> 2.
It can be proven that no other way is possible, so we return 3.
**Example 2:**
**Input:** startPos = 2, endPos = 5, k = 10
**Output:** 0
**Explanation:** It is impossible to reach position 5 from position 2 in exactly 10 steps.
**Constraints:**
* `1 <= startPos, endPos, k <= 1000` | null |
DP }| Recursion || Memoization || Python3 | number-of-ways-to-reach-a-position-after-exactly-k-steps | 0 | 1 | ```\nclass Solution(object):\n def numberOfWays(self, startPos, endPos, k):\n """\n :type startPos: int\n :type endPos: int\n :type k: int\n :rtype: int\n """\n MOD=1e9+7\n \n dp={}\n \n def A(currpos,k):\n if (currpos,k) in dp:\n return dp[(currpos,k)]\n\t\t\t\t\n\t\t\t#base case \n if currpos==endPos and k==0:\n return 1\n\t\t\t#base case\n if k<=0:\n return 0\n \n \n ans1,ans2=0,0\n\t\t\t#move left\n ans1+=A(currpos-1,k-1)\n #move right\n ans2+=A(currpos+1,k-1)\n #sum of left + right possibilities\n dp[(currpos,k)]=(ans1+ans2)%MOD\n\t\t\t#return the result\n return dp[(currpos,k)]\n \n return int(A(startPos,k)%MOD)\n \n \n ``` | 2 | You are given two **positive** integers `startPos` and `endPos`. Initially, you are standing at position `startPos` on an **infinite** number line. With one step, you can move either one position to the left, or one position to the right.
Given a positive integer `k`, return _the number of **different** ways to reach the position_ `endPos` _starting from_ `startPos`_, such that you perform **exactly**_ `k` _steps_. Since the answer may be very large, return it **modulo** `109 + 7`.
Two ways are considered different if the order of the steps made is not exactly the same.
**Note** that the number line includes negative integers.
**Example 1:**
**Input:** startPos = 1, endPos = 2, k = 3
**Output:** 3
**Explanation:** We can reach position 2 from 1 in exactly 3 steps in three ways:
- 1 -> 2 -> 3 -> 2.
- 1 -> 2 -> 1 -> 2.
- 1 -> 0 -> 1 -> 2.
It can be proven that no other way is possible, so we return 3.
**Example 2:**
**Input:** startPos = 2, endPos = 5, k = 10
**Output:** 0
**Explanation:** It is impossible to reach position 5 from position 2 in exactly 10 steps.
**Constraints:**
* `1 <= startPos, endPos, k <= 1000` | null |
[Java/Python 3] Sliding window & bit manipulation, w/ explanation, comments and analysis. | longest-nice-subarray | 1 | 1 | **Q & A**\n\n*Q*: How to think of such approach in interview?\n*A*: I believe the key point is how to understand and then use the constraint "bitwise AND of every pair of elements that are in different positions in the subarray is equal to 0."\n\nThe other point is that a subarray is a **contiguous** part of an array, which reminds me of sliding window.\n\n**End of Q & A**\n\n----\n\n\n**Intuition:**\n\nAccording to the problem description: "bitwise AND of every pair of elements that are in different positions in the subarray is equal to 0.", we can conclude that **the elements within the subarray have distinct set (`1`) bits.**, which implies that we can use an integer as a mask to record the set bits of elements within the sliding window.\n\nTherefore, we can maintain a sliding window such that the set bits of all elements in the window are distinct.\n\n**Algorithm**\n\n1. Use an integer to represent the set bits within the sliding window; use bitwise OR operation to roll an element into window;\n2. If there are duplicate set bits in current sliding window, their bitwise AND must be greater than `0`; use bitwise XOR to keep rolling the element at left bound out of the sliding window till bitwise AND becomes `0`;\n3. Update the maximum window size.\n\n```java\n public int longestNiceSubarray(int[] nums) {\n int ans = 0, mask = 0;\n for (int lo = -1, hi = 0; hi < nums.length; ++hi) {\n while ((mask & nums[hi]) != 0) { // nums[hi] has duplicate set bits for current sliding window.\n mask ^= nums[++lo]; // shrink left bound of current sliding window, and remove the corresponding element.\n }\n mask |= nums[hi]; // Expand right bound and put nums[hi] into window.\n ans = Math.max(ans, hi - lo); // update the max window size.\n }\n return ans;\n }\n```\n```python\n def longestNiceSubarray(self, nums: List[int]) -> int:\n lo, mask, ans = -1, 0, 1\n for hi, n in enumerate(nums):\n while (mask & n): # n has duplicate set bits for current sliding window.\n lo += 1 # shrink left bound of current sliding window.\n mask ^= nums[lo] # remove the corresponding element out of the window.\n mask |= n # Expand right bound and put n into window.\n ans = max(ans, hi - lo) # update the max window size.\n return ans\n```\n\n**Analysis:**\n\nTime: `O(n)`, space: `O(1)`, where `n = nums.length`.\n\n----\n\n\nSimilar Sliding Window Problems:\n\n[159. Longest Substring with At Most Two Distinct Characters](https://leetcode.com/problems/longest-substring-with-at-most-two-distinct-characters/) (Premium)\n[209. Minimum Size Subarray Sum](https://leetcode.com/problems/minimum-size-subarray-sum/description/)\n[424. Longest Repeating Character Replacement](https://leetcode.com/problems/longest-repeating-character-replacement/)\n[904. Fruit Into Baskets](https://leetcode.com/problems/fruit-into-baskets/)\n[992. Subarrays with K Different Integers](https://leetcode.com/problems/subarrays-with-k-different-integers/)\n[1004. Max Consecutive Ones III](https://leetcode.com/problems/max-consecutive-ones-iii/)\n[1234. Replace the Substring for Balanced String](https://leetcode.com/problems/replace-the-substring-for-balanced-string/)\n[1248. Count Number of Nice Subarrays](https://leetcode.com/problems/count-number-of-nice-subarrays/discuss/419321/JavaPython-3-1-pass-Sliding-Window-O(n)-time-O(1)-space-w-brief-explanation.)\n[1838. Frequency of the Most Frequent Element](https://leetcode.com/problems/frequency-of-the-most-frequent-element/discuss/1175081/JavaPython-3-Sort-then-slide-window)\n[2398. Maximum Number of Robots Within Budget](https://leetcode.com/problems/maximum-number-of-robots-within-budget/)\n[2401. Longest Nice Subarray](https://leetcode.com/problems/longest-nice-subarray/discuss/2527281/JavaPython-3-Sliding-window-w-bit-manipulation.)\n\n | 35 | You are given an array `nums` consisting of **positive** integers.
We call a subarray of `nums` **nice** if the bitwise **AND** of every pair of elements that are in **different** positions in the subarray is equal to `0`.
Return _the length of the **longest** nice subarray_.
A **subarray** is a **contiguous** part of an array.
**Note** that subarrays of length `1` are always considered nice.
**Example 1:**
**Input:** nums = \[1,3,8,48,10\]
**Output:** 3
**Explanation:** The longest nice subarray is \[3,8,48\]. This subarray satisfies the conditions:
- 3 AND 8 = 0.
- 3 AND 48 = 0.
- 8 AND 48 = 0.
It can be proven that no longer nice subarray can be obtained, so we return 3.
**Example 2:**
**Input:** nums = \[3,1,5,11,13\]
**Output:** 1
**Explanation:** The length of the longest nice subarray is 1. Any subarray of length 1 can be chosen.
**Constraints:**
* `1 <= nums.length <= 105`
* `1 <= nums[i] <= 109` | null |
[Python3] Sliding Window | longest-nice-subarray | 0 | 1 | Intuition:\n\nElements in a nice subarray should not have same bits of 1s\nFor example, we can not have 1 and 3 in a nice subarray, because bit #0 in both numbers is 1\n1 -> 0001\n3 -> 0011\n\nSo we use sliding window and keep tracking of all bits in the current window by using OR operator.\nAnd when we encounter a number that would make our current window not nice, we shrink the window by removing the the left most number in the window until it is no longer conflicting.\n\nTo remove bits of element from current window, we use XOR operator.\n\n```\nclass Solution:\n def longestNiceSubarray(self, nums: List[int]) -> int:\n l = 0\n r = 0\n n = len(nums)\n \n curr_mask = 0\n ans = 1\n while r < n:\n while l < r and curr_mask & nums[r] != 0:\n curr_mask = curr_mask ^ nums[l]\n l += 1\n \n curr_mask = curr_mask | nums[r]\n r += 1\n ans = max(ans, r - l)\n return ans\n ``` | 3 | You are given an array `nums` consisting of **positive** integers.
We call a subarray of `nums` **nice** if the bitwise **AND** of every pair of elements that are in **different** positions in the subarray is equal to `0`.
Return _the length of the **longest** nice subarray_.
A **subarray** is a **contiguous** part of an array.
**Note** that subarrays of length `1` are always considered nice.
**Example 1:**
**Input:** nums = \[1,3,8,48,10\]
**Output:** 3
**Explanation:** The longest nice subarray is \[3,8,48\]. This subarray satisfies the conditions:
- 3 AND 8 = 0.
- 3 AND 48 = 0.
- 8 AND 48 = 0.
It can be proven that no longer nice subarray can be obtained, so we return 3.
**Example 2:**
**Input:** nums = \[3,1,5,11,13\]
**Output:** 1
**Explanation:** The length of the longest nice subarray is 1. Any subarray of length 1 can be chosen.
**Constraints:**
* `1 <= nums.length <= 105`
* `1 <= nums[i] <= 109` | null |
100% Python Solution | longest-nice-subarray | 0 | 1 | \n\n# Complexity\n- Time complexity:\nO(n)\n\n- Space complexity:\nO(1)\n\n# Code\n```\nclass Solution:\n def longestNiceSubarray(self, nums: List[int]) -> int:\n l,r = 0,1\n res = 0\n\n while r < len(nums):\n i = l\n\n """do the bitwise AND for the entire window so far"""\n while i < r and ((nums[r] & nums[i]) == 0):\n # print(f"AND passed {nums[r],nums[i]}")\n i += 1\n\n \n\n if i == r:\n res = max(res, r-l+1)\n r += 1\n else:\n l = i + 1 \n r = l + 1\n\n \n\n return max(res,1)\n``` | 1 | You are given an array `nums` consisting of **positive** integers.
We call a subarray of `nums` **nice** if the bitwise **AND** of every pair of elements that are in **different** positions in the subarray is equal to `0`.
Return _the length of the **longest** nice subarray_.
A **subarray** is a **contiguous** part of an array.
**Note** that subarrays of length `1` are always considered nice.
**Example 1:**
**Input:** nums = \[1,3,8,48,10\]
**Output:** 3
**Explanation:** The longest nice subarray is \[3,8,48\]. This subarray satisfies the conditions:
- 3 AND 8 = 0.
- 3 AND 48 = 0.
- 8 AND 48 = 0.
It can be proven that no longer nice subarray can be obtained, so we return 3.
**Example 2:**
**Input:** nums = \[3,1,5,11,13\]
**Output:** 1
**Explanation:** The length of the longest nice subarray is 1. Any subarray of length 1 can be chosen.
**Constraints:**
* `1 <= nums.length <= 105`
* `1 <= nums[i] <= 109` | null |
Bit Magic with Explanation and Complexities | longest-nice-subarray | 0 | 1 | ##### Rationale\n* We need to do check whether we could include a number in the current window in constant time\n* We could represent the group with bitwise OR for the all the numbers in the current window\n\t* Given a window, `[3, 8, 48]`, we need to know whether we could include the next number in the window in constant time. Notice that the next number must have pairwise bitwise AND zero with all the members in the group. In other words, it should have not any set bit at a position where any member of the group already has a set bit. How do we know at what positions the group members have a set bit? The group\'s bitwise OR would have that information \n\t* Consider the next number to be `4`, we could easily include it in the window as all the pair wise AND would be 0. How do we know that? The group, `[3, 8, 48]` could be represented with `3 | 8 | 48` or `111011`. Any number that could be included in the group must not have a set bit at the positions where the bit is set in the group. 4 or `100` is such a number. If we AND it with group, we would get `0` as the result\n\t* Since 4 could be included, the group would increase to `[3, 8, 48, 4]` or `111111`. Notice how a bitwise OR with the previous group would give us the mentioned bitmask\n* If the bitwise AND for the group and the current number is 0, we could include the number\n* To remove an element when we shrink the window, we could do a bitwise AND for the ~(element that is to be reomved) and the group\n\t* Since we need to remove the contribution from the number that is to be removed from the window, we need to unset the bits that came from the to-be-removed number\n\t* Note that this would never to lead ambiguity. You might think that, "Hey, what if the to-be-removed number unsets the bits that were set by another number?". It can never happen. Notice how we only include numbers in the group when they do not have any set bits in common\n* Rest is trivial if you\'re familiar with sliding window\n\n##### Complexities\n* Time: `O(n)`\n* Space: `O(1)`\n\n\n```python\nclass Solution:\n def longestNiceSubarray(self, nums: List[int]) -> int:\n maximum_length = 1\n n = len(nums)\n \n current_group = 0\n left = 0\n \n for right in range(n):\n\t\t\t# If the number at the right point is safe to include, include it in the group and update the maximum length.\n if nums[right] & current_group == 0:\n current_group |=nums[right]\n maximum_length = max(maximum_length, right - left + 1)\n continue\n \n\t\t\t# Shrink the window until the number at the right pointer is safe to include.\n while left < right and nums[right] & current_group != 0: \n current_group &= (~nums[left]) \n left += 1\n \n\t\t\t# Include the number at the right pointer in the group.\n current_group |= nums[right]\n \n return maximum_length\n``` | 29 | You are given an array `nums` consisting of **positive** integers.
We call a subarray of `nums` **nice** if the bitwise **AND** of every pair of elements that are in **different** positions in the subarray is equal to `0`.
Return _the length of the **longest** nice subarray_.
A **subarray** is a **contiguous** part of an array.
**Note** that subarrays of length `1` are always considered nice.
**Example 1:**
**Input:** nums = \[1,3,8,48,10\]
**Output:** 3
**Explanation:** The longest nice subarray is \[3,8,48\]. This subarray satisfies the conditions:
- 3 AND 8 = 0.
- 3 AND 48 = 0.
- 8 AND 48 = 0.
It can be proven that no longer nice subarray can be obtained, so we return 3.
**Example 2:**
**Input:** nums = \[3,1,5,11,13\]
**Output:** 1
**Explanation:** The length of the longest nice subarray is 1. Any subarray of length 1 can be chosen.
**Constraints:**
* `1 <= nums.length <= 105`
* `1 <= nums[i] <= 109` | null |
Python | Sliding window | longest-nice-subarray | 0 | 1 | We start with the window `[0, 0]`. At each step we try to see if adding one more element to the contiguous subarray would overlap some bit to the used bits among the numbers present in the current subarray.
* When we can make the window larger to the right, we update the used bits as well
* When we cannot make the window larget to the right, we shorten it from the left, making sure to update the used bits. We also check the size of the window, to see if we found a larger window.
∎
```python
class Solution:
def longestNiceSubarray(self, nums: List[int]) -> int:
n = len(nums)
left, right = 0, 1
usedBits = nums[0]
longestNice = 1
while right < n:
while right < n and usedBits & nums[right] == 0: # while there is no bit overlap keep making longer to the right
usedBits |= nums[right] # turn on bits from right num
right += 1
longestNice = max(longestNice, right - left)
usedBits ^= nums[left] # turn off bits from left num
left += 1
return longestNice
``` | 2 | You are given an array `nums` consisting of **positive** integers.
We call a subarray of `nums` **nice** if the bitwise **AND** of every pair of elements that are in **different** positions in the subarray is equal to `0`.
Return _the length of the **longest** nice subarray_.
A **subarray** is a **contiguous** part of an array.
**Note** that subarrays of length `1` are always considered nice.
**Example 1:**
**Input:** nums = \[1,3,8,48,10\]
**Output:** 3
**Explanation:** The longest nice subarray is \[3,8,48\]. This subarray satisfies the conditions:
- 3 AND 8 = 0.
- 3 AND 48 = 0.
- 8 AND 48 = 0.
It can be proven that no longer nice subarray can be obtained, so we return 3.
**Example 2:**
**Input:** nums = \[3,1,5,11,13\]
**Output:** 1
**Explanation:** The length of the longest nice subarray is 1. Any subarray of length 1 can be chosen.
**Constraints:**
* `1 <= nums.length <= 105`
* `1 <= nums[i] <= 109` | null |
Python 3 | 2 heaps — O(m log(max(m, n)) + n), O(m+n) | meeting-rooms-iii | 0 | 1 | # Intuition\nMaintain rooms sorted by their time of being available again.\nMaintain available rooms sorted by their nubmer to pick the smallest one.\n\n# Approach\n<!-- Describe your approach to solving the problem. -->\n\n# Complexity\n- Time complexity: $O(m\\log (\\max(m, n)) + n)$\n\n- Space complexity: $O(m + n)$\n\n# Code\n```\nclass Solution:\n def mostBooked(self, n: int, meetings: List[List[int]]) -> int:\n meetings.sort()\n available_rooms = list(range(n))\n heapq.heapify(available_rooms)\n occupied_rooms = []\n cnt = defaultdict(int)\n for start, end in meetings:\n while len(occupied_rooms) > 0 and occupied_rooms[0][0] <= start:\n heapq.heappush(available_rooms, heapq.heappop(occupied_rooms)[1])\n if len(available_rooms) == 0:\n available_time, room = heapq.heappop(occupied_rooms)\n else:\n room = heapq.heappop(available_rooms)\n available_time = start\n cnt[room] += 1\n heapq.heappush(occupied_rooms, (max(start, available_time) + (end-start), room))\n max_meetings = max(cnt.values())\n return min(filter(lambda room: cnt[room] == max_meetings, range(n)))\n``` | 2 | You are given an integer `n`. There are `n` rooms numbered from `0` to `n - 1`.
You are given a 2D integer array `meetings` where `meetings[i] = [starti, endi]` means that a meeting will be held during the **half-closed** time interval `[starti, endi)`. All the values of `starti` are **unique**.
Meetings are allocated to rooms in the following manner:
1. Each meeting will take place in the unused room with the **lowest** number.
2. If there are no available rooms, the meeting will be delayed until a room becomes free. The delayed meeting should have the **same** duration as the original meeting.
3. When a room becomes unused, meetings that have an earlier original **start** time should be given the room.
Return _the **number** of the room that held the most meetings._ If there are multiple rooms, return _the room with the **lowest** number._
A **half-closed interval** `[a, b)` is the interval between `a` and `b` **including** `a` and **not including** `b`.
**Example 1:**
**Input:** n = 2, meetings = \[\[0,10\],\[1,5\],\[2,7\],\[3,4\]\]
**Output:** 0
**Explanation:**
- At time 0, both rooms are not being used. The first meeting starts in room 0.
- At time 1, only room 1 is not being used. The second meeting starts in room 1.
- At time 2, both rooms are being used. The third meeting is delayed.
- At time 3, both rooms are being used. The fourth meeting is delayed.
- At time 5, the meeting in room 1 finishes. The third meeting starts in room 1 for the time period \[5,10).
- At time 10, the meetings in both rooms finish. The fourth meeting starts in room 0 for the time period \[10,11).
Both rooms 0 and 1 held 2 meetings, so we return 0.
**Example 2:**
**Input:** n = 3, meetings = \[\[1,20\],\[2,10\],\[3,5\],\[4,9\],\[6,8\]\]
**Output:** 1
**Explanation:**
- At time 1, all three rooms are not being used. The first meeting starts in room 0.
- At time 2, rooms 1 and 2 are not being used. The second meeting starts in room 1.
- At time 3, only room 2 is not being used. The third meeting starts in room 2.
- At time 4, all three rooms are being used. The fourth meeting is delayed.
- At time 5, the meeting in room 2 finishes. The fourth meeting starts in room 2 for the time period \[5,10).
- At time 6, all three rooms are being used. The fifth meeting is delayed.
- At time 10, the meetings in rooms 1 and 2 finish. The fifth meeting starts in room 1 for the time period \[10,12).
Room 0 held 1 meeting while rooms 1 and 2 each held 2 meetings, so we return 1.
**Constraints:**
* `1 <= n <= 100`
* `1 <= meetings.length <= 105`
* `meetings[i].length == 2`
* `0 <= starti < endi <= 5 * 105`
* All the values of `starti` are **unique**. | null |
87% TC easy python solution | meeting-rooms-iii | 0 | 1 | ```\ndef mostBooked(self, n: int, meet: List[List[int]]) -> int:\n\tcount = [0] * n\n\tmeet.sort()\n\tavlbl = SortedList([i for i in range(n)])\n\thold = []\n\tfor i, j in meet:\n\t\twhile(hold and hold[0][0] <= i):\n\t\t\t_, room = heappop(hold)\n\t\t\tavlbl.add(room)\n\t\tif(avlbl):\n\t\t\troom = avlbl.pop(0)\n\t\t\tstart = i\n\t\telse:\n\t\t\tstart, room = heappop(hold)\n\t\theappush(hold, (start + (j-i), room))\n\t\tcount[room] += 1\n\tm, ans = -1, -1\n\tfor i in range(n):\n\t\tif(m < count[i]):\n\t\t\tm = count[i]\n\t\t\tans = i\n\treturn ans\n``` | 2 | You are given an integer `n`. There are `n` rooms numbered from `0` to `n - 1`.
You are given a 2D integer array `meetings` where `meetings[i] = [starti, endi]` means that a meeting will be held during the **half-closed** time interval `[starti, endi)`. All the values of `starti` are **unique**.
Meetings are allocated to rooms in the following manner:
1. Each meeting will take place in the unused room with the **lowest** number.
2. If there are no available rooms, the meeting will be delayed until a room becomes free. The delayed meeting should have the **same** duration as the original meeting.
3. When a room becomes unused, meetings that have an earlier original **start** time should be given the room.
Return _the **number** of the room that held the most meetings._ If there are multiple rooms, return _the room with the **lowest** number._
A **half-closed interval** `[a, b)` is the interval between `a` and `b` **including** `a` and **not including** `b`.
**Example 1:**
**Input:** n = 2, meetings = \[\[0,10\],\[1,5\],\[2,7\],\[3,4\]\]
**Output:** 0
**Explanation:**
- At time 0, both rooms are not being used. The first meeting starts in room 0.
- At time 1, only room 1 is not being used. The second meeting starts in room 1.
- At time 2, both rooms are being used. The third meeting is delayed.
- At time 3, both rooms are being used. The fourth meeting is delayed.
- At time 5, the meeting in room 1 finishes. The third meeting starts in room 1 for the time period \[5,10).
- At time 10, the meetings in both rooms finish. The fourth meeting starts in room 0 for the time period \[10,11).
Both rooms 0 and 1 held 2 meetings, so we return 0.
**Example 2:**
**Input:** n = 3, meetings = \[\[1,20\],\[2,10\],\[3,5\],\[4,9\],\[6,8\]\]
**Output:** 1
**Explanation:**
- At time 1, all three rooms are not being used. The first meeting starts in room 0.
- At time 2, rooms 1 and 2 are not being used. The second meeting starts in room 1.
- At time 3, only room 2 is not being used. The third meeting starts in room 2.
- At time 4, all three rooms are being used. The fourth meeting is delayed.
- At time 5, the meeting in room 2 finishes. The fourth meeting starts in room 2 for the time period \[5,10).
- At time 6, all three rooms are being used. The fifth meeting is delayed.
- At time 10, the meetings in rooms 1 and 2 finish. The fifth meeting starts in room 1 for the time period \[10,12).
Room 0 held 1 meeting while rooms 1 and 2 each held 2 meetings, so we return 1.
**Constraints:**
* `1 <= n <= 100`
* `1 <= meetings.length <= 105`
* `meetings[i].length == 2`
* `0 <= starti < endi <= 5 * 105`
* All the values of `starti` are **unique**. | null |
Simple and Detailed comments, readable, beats 99% | meeting-rooms-iii | 0 | 1 | # Intuition\n<!-- Describe your first thoughts on how to solve this problem. -->\n\n# Approach\nUsing Min heap\n\n# Complexity\n- Time complexity: \nO(nlogn)\n- Space complexity:\nO(n)\n\n# Code\n```\nclass Solution:\n def mostBooked(self, num_rooms, meetings):\n # Create a list of available rooms using indices from 0 to num_rooms-1\n available_rooms = [room for room in range(num_rooms)]\n occupied_rooms = [] # Stores rooms that are currently occupied\n heapify(available_rooms) # Convert the available_rooms list into a heap\n booking_counts = [0] * num_rooms # Initialize a list to keep track of booking counts per room\n\n # Sort the meetings in ascending order based on the start time\n sorted_meetings = sorted(meetings, key=lambda x: x[0])\n for start_time, end_time in sorted_meetings:\n # Check if there are any available rooms at the start time of the meeting\n while occupied_rooms and occupied_rooms[0][0] <= start_time:\n # Room becomes available, add it back to the available_rooms heap\n end, room = heappop(occupied_rooms)\n heappush(available_rooms, room)\n\n if available_rooms:\n # Assign an available room from the available_rooms heap to the meeting\n room = heappop(available_rooms)\n heappush(occupied_rooms, [end_time, room]) # Add the meeting to the occupied_rooms heap\n else:\n # All rooms are occupied, find the room with the earliest end time\n current_end, room = heappop(occupied_rooms)\n new_end = current_end + end_time - start_time # Update the room\'s end time\n heappush(occupied_rooms, [new_end, room])\n\n booking_counts[room] += 1 # Increment the booking count for the assigned room\n\n # Find the room with the maximum booking count and return its index\n max_booking_count = max(booking_counts)\n most_booked_room = booking_counts.index(max_booking_count)\n return most_booked_room\n\n\n\n\n\n\n\n``` | 7 | You are given an integer `n`. There are `n` rooms numbered from `0` to `n - 1`.
You are given a 2D integer array `meetings` where `meetings[i] = [starti, endi]` means that a meeting will be held during the **half-closed** time interval `[starti, endi)`. All the values of `starti` are **unique**.
Meetings are allocated to rooms in the following manner:
1. Each meeting will take place in the unused room with the **lowest** number.
2. If there are no available rooms, the meeting will be delayed until a room becomes free. The delayed meeting should have the **same** duration as the original meeting.
3. When a room becomes unused, meetings that have an earlier original **start** time should be given the room.
Return _the **number** of the room that held the most meetings._ If there are multiple rooms, return _the room with the **lowest** number._
A **half-closed interval** `[a, b)` is the interval between `a` and `b` **including** `a` and **not including** `b`.
**Example 1:**
**Input:** n = 2, meetings = \[\[0,10\],\[1,5\],\[2,7\],\[3,4\]\]
**Output:** 0
**Explanation:**
- At time 0, both rooms are not being used. The first meeting starts in room 0.
- At time 1, only room 1 is not being used. The second meeting starts in room 1.
- At time 2, both rooms are being used. The third meeting is delayed.
- At time 3, both rooms are being used. The fourth meeting is delayed.
- At time 5, the meeting in room 1 finishes. The third meeting starts in room 1 for the time period \[5,10).
- At time 10, the meetings in both rooms finish. The fourth meeting starts in room 0 for the time period \[10,11).
Both rooms 0 and 1 held 2 meetings, so we return 0.
**Example 2:**
**Input:** n = 3, meetings = \[\[1,20\],\[2,10\],\[3,5\],\[4,9\],\[6,8\]\]
**Output:** 1
**Explanation:**
- At time 1, all three rooms are not being used. The first meeting starts in room 0.
- At time 2, rooms 1 and 2 are not being used. The second meeting starts in room 1.
- At time 3, only room 2 is not being used. The third meeting starts in room 2.
- At time 4, all three rooms are being used. The fourth meeting is delayed.
- At time 5, the meeting in room 2 finishes. The fourth meeting starts in room 2 for the time period \[5,10).
- At time 6, all three rooms are being used. The fifth meeting is delayed.
- At time 10, the meetings in rooms 1 and 2 finish. The fifth meeting starts in room 1 for the time period \[10,12).
Room 0 held 1 meeting while rooms 1 and 2 each held 2 meetings, so we return 1.
**Constraints:**
* `1 <= n <= 100`
* `1 <= meetings.length <= 105`
* `meetings[i].length == 2`
* `0 <= starti < endi <= 5 * 105`
* All the values of `starti` are **unique**. | null |
Python | More heap extravaganza | meeting-rooms-iii | 0 | 1 | We will keep a heap of available rooms and a keep of occupied rooms.
* At the beginning the heap of available rooms includes all rooms. It will include only the room numbers
* The heap of occupied rooms will include tuples `(endTime, roomNumber)`
* For each meeting, we
* Check if by the time it starts any meetings would have ended by that time, and free the corresponding rooms (making them available again).
* Check if there is an available room
* If not, we look at the next room that would get free and use that one, we recalculate the ending time of that meeting keeping the same duration
* If yes, we assign the room. We make sure to increas the meeting count of the room.
Note problem makes no mention about the start times being sorted, so we need to make sure to sorte them at first for this solution to work.
∎
```python
class Solution:
def mostBooked(self, n: int, meetings: List[List[int]]) -> int:
meetings.sort() # make sure start times are sorted!!
meetingCount = [0 for _ in range(n)]
availableRooms = list(range(n)); heapify(availableRooms)
occupiedRooms = []
for start, end in meetings:
while occupiedRooms and start >= occupiedRooms[0][0]:
heappush(availableRooms, heappop(occupiedRooms)[1]) # frees room and makes it available
if availableRooms:
roomNumber = heappop(availableRooms) # assigns next available room
else:
freedEnd, roomNumber = heappop(occupiedRooms) # waits until the next room that would be available gets free
end += freedEnd - start
heappush(occupiedRooms, (end,roomNumber)) # make note that the ruom is occupied and when the assigned meeting ends
meetingCount[roomNumber] += 1 # update meeting counter
return sorted([(count, i) for i, count in enumerate(meetingCount)], key = lambda x: (-x[0], x[1]))[0][1] # find room with most meetings
``` | 2 | You are given an integer `n`. There are `n` rooms numbered from `0` to `n - 1`.
You are given a 2D integer array `meetings` where `meetings[i] = [starti, endi]` means that a meeting will be held during the **half-closed** time interval `[starti, endi)`. All the values of `starti` are **unique**.
Meetings are allocated to rooms in the following manner:
1. Each meeting will take place in the unused room with the **lowest** number.
2. If there are no available rooms, the meeting will be delayed until a room becomes free. The delayed meeting should have the **same** duration as the original meeting.
3. When a room becomes unused, meetings that have an earlier original **start** time should be given the room.
Return _the **number** of the room that held the most meetings._ If there are multiple rooms, return _the room with the **lowest** number._
A **half-closed interval** `[a, b)` is the interval between `a` and `b` **including** `a` and **not including** `b`.
**Example 1:**
**Input:** n = 2, meetings = \[\[0,10\],\[1,5\],\[2,7\],\[3,4\]\]
**Output:** 0
**Explanation:**
- At time 0, both rooms are not being used. The first meeting starts in room 0.
- At time 1, only room 1 is not being used. The second meeting starts in room 1.
- At time 2, both rooms are being used. The third meeting is delayed.
- At time 3, both rooms are being used. The fourth meeting is delayed.
- At time 5, the meeting in room 1 finishes. The third meeting starts in room 1 for the time period \[5,10).
- At time 10, the meetings in both rooms finish. The fourth meeting starts in room 0 for the time period \[10,11).
Both rooms 0 and 1 held 2 meetings, so we return 0.
**Example 2:**
**Input:** n = 3, meetings = \[\[1,20\],\[2,10\],\[3,5\],\[4,9\],\[6,8\]\]
**Output:** 1
**Explanation:**
- At time 1, all three rooms are not being used. The first meeting starts in room 0.
- At time 2, rooms 1 and 2 are not being used. The second meeting starts in room 1.
- At time 3, only room 2 is not being used. The third meeting starts in room 2.
- At time 4, all three rooms are being used. The fourth meeting is delayed.
- At time 5, the meeting in room 2 finishes. The fourth meeting starts in room 2 for the time period \[5,10).
- At time 6, all three rooms are being used. The fifth meeting is delayed.
- At time 10, the meetings in rooms 1 and 2 finish. The fifth meeting starts in room 1 for the time period \[10,12).
Room 0 held 1 meeting while rooms 1 and 2 each held 2 meetings, so we return 1.
**Constraints:**
* `1 <= n <= 100`
* `1 <= meetings.length <= 105`
* `meetings[i].length == 2`
* `0 <= starti < endi <= 5 * 105`
* All the values of `starti` are **unique**. | null |
simple code using dictionary | most-frequent-even-element | 0 | 1 | # Intuition\n<!-- Describe your first thoughts on how to solve this problem. -->\n\n# Approach\n<!-- Describe your approach to solving the problem. -->\n\n# Complexity\n- Time complexity:\n<!-- Add your time complexity here, e.g. $$O(n)$$ -->\n\n- Space complexity:\n<!-- Add your space complexity here, e.g. $$O(n)$$ -->\n\n# Code\n```\nclass Solution:\n def mostFrequentEven(self, nums: List[int]) -> int:\n maxx = 0 #max count intially zero\n even_element = -1 #even_element intially -1\n d={} #creation of dictionary\n for i in nums:\n if i%2==0:\n if i not in d:d[i]=1 \n else:d[i]+=1\n for val,count in d.items(): #key=val and count=values\n if count>maxx: #comparing count is greater then maxx \n maxx=count\n even_element=val \n elif count==maxx: #if the count is tie \n if even_element>val: #checking the even-element is lesser or not \n even_element=val\n return even_element\n \n``` | 2 | Given an integer array `nums`, return _the most frequent even element_.
If there is a tie, return the **smallest** one. If there is no such element, return `-1`.
**Example 1:**
**Input:** nums = \[0,1,2,2,4,4,1\]
**Output:** 2
**Explanation:**
The even elements are 0, 2, and 4. Of these, 2 and 4 appear the most.
We return the smallest one, which is 2.
**Example 2:**
**Input:** nums = \[4,4,4,9,2,4\]
**Output:** 4
**Explanation:** 4 is the even element appears the most.
**Example 3:**
**Input:** nums = \[29,47,21,41,13,37,25,7\]
**Output:** -1
**Explanation:** There is no even element.
**Constraints:**
* `1 <= nums.length <= 2000`
* `0 <= nums[i] <= 105` | null |
Python Simple Code || 97.6% Beats || HashMap | most-frequent-even-element | 0 | 1 | **If you got help from this,... Plz Upvote .. it encourage me**\n\nHashMap Solution:\n# Code\n```\nclass Solution:\n def mostFrequentEven(self, nums: List[int]) -> int:\n d = {}\n for ele in nums:\n if ele%2 == 0:\n d[ele] = d.get(ele,0) + 1\n\n if not d:\n return -1\n\n maxv = max(d.values())\n\n return min([ele for ele in d if d[ele] == maxv])\n # Or\n maxe = [ele for ele in d if d[ele] == maxv]\n return min(maxe)\n\n\n``` | 2 | Given an integer array `nums`, return _the most frequent even element_.
If there is a tie, return the **smallest** one. If there is no such element, return `-1`.
**Example 1:**
**Input:** nums = \[0,1,2,2,4,4,1\]
**Output:** 2
**Explanation:**
The even elements are 0, 2, and 4. Of these, 2 and 4 appear the most.
We return the smallest one, which is 2.
**Example 2:**
**Input:** nums = \[4,4,4,9,2,4\]
**Output:** 4
**Explanation:** 4 is the even element appears the most.
**Example 3:**
**Input:** nums = \[29,47,21,41,13,37,25,7\]
**Output:** -1
**Explanation:** There is no even element.
**Constraints:**
* `1 <= nums.length <= 2000`
* `0 <= nums[i] <= 105` | null |
Python Solution | Easy Understanding | most-frequent-even-element | 0 | 1 | ```\nfrom collections import Counter\n\nclass Solution:\n def mostFrequentEven(self, nums) -> int:\n counter = Counter([i for i in nums if i % 2 == 0])\n if not counter:\n return -1\n res = {x: count for x, count in counter.items() if count == max(counter.values())}\n return min(res.keys())\n``` | 3 | Given an integer array `nums`, return _the most frequent even element_.
If there is a tie, return the **smallest** one. If there is no such element, return `-1`.
**Example 1:**
**Input:** nums = \[0,1,2,2,4,4,1\]
**Output:** 2
**Explanation:**
The even elements are 0, 2, and 4. Of these, 2 and 4 appear the most.
We return the smallest one, which is 2.
**Example 2:**
**Input:** nums = \[4,4,4,9,2,4\]
**Output:** 4
**Explanation:** 4 is the even element appears the most.
**Example 3:**
**Input:** nums = \[29,47,21,41,13,37,25,7\]
**Output:** -1
**Explanation:** There is no even element.
**Constraints:**
* `1 <= nums.length <= 2000`
* `0 <= nums[i] <= 105` | null |
Hashmap (Counter) Simple 4 line code | most-frequent-even-element | 0 | 1 | ```\nclass Solution:\n def mostFrequentEven(self, nums: List[int]) -> int:\n nums.sort()\n a=Counter(nums).most_common()\n a.sort(key=lambda x:-x[1])\n for i in range(len(a)):\n if a[i][0]%2==0:\n return a[i][0]\n return -1\n``` | 2 | Given an integer array `nums`, return _the most frequent even element_.
If there is a tie, return the **smallest** one. If there is no such element, return `-1`.
**Example 1:**
**Input:** nums = \[0,1,2,2,4,4,1\]
**Output:** 2
**Explanation:**
The even elements are 0, 2, and 4. Of these, 2 and 4 appear the most.
We return the smallest one, which is 2.
**Example 2:**
**Input:** nums = \[4,4,4,9,2,4\]
**Output:** 4
**Explanation:** 4 is the even element appears the most.
**Example 3:**
**Input:** nums = \[29,47,21,41,13,37,25,7\]
**Output:** -1
**Explanation:** There is no even element.
**Constraints:**
* `1 <= nums.length <= 2000`
* `0 <= nums[i] <= 105` | null |
Hashmap Python3 | optimal-partition-of-string | 0 | 1 | # Intuition\n<!-- Describe your first thoughts on how to solve this problem. -->\n\n# Approach\n<!-- Describe your approach to solving the problem. -->\n\n# Complexity\n- Time complexity:\n<!-- Add your time complexity here, e.g. $$O(n)$$ -->\n\n- Space complexity:\n<!-- Add your space complexity here, e.g. $$O(n)$$ -->\n\n# Code\n```\nclass Solution:\n def partitionString(self, s: str) -> int:\n curset=set()\n res=1\n for c in s:\n if c in curset:\n res+=1\n curset=set()\n curset.add(c)\n return res\n``` | 1 | Given a string `s`, partition the string into one or more **substrings** such that the characters in each substring are **unique**. That is, no letter appears in a single substring more than **once**.
Return _the **minimum** number of substrings in such a partition._
Note that each character should belong to exactly one substring in a partition.
**Example 1:**
**Input:** s = "abacaba "
**Output:** 4
**Explanation:**
Two possible partitions are ( "a ", "ba ", "cab ", "a ") and ( "ab ", "a ", "ca ", "ba ").
It can be shown that 4 is the minimum number of substrings needed.
**Example 2:**
**Input:** s = "ssssss "
**Output:** 6
**Explanation:**
The only valid partition is ( "s ", "s ", "s ", "s ", "s ", "s ").
**Constraints:**
* `1 <= s.length <= 105`
* `s` consists of only English lowercase letters. | null |
Python Simple Solution O(n) | optimal-partition-of-string | 0 | 1 | # Intuition\n<!-- Describe your first thoughts on how to solve this problem. -->\n\n# Approach\n<!-- Describe your approach to solving the problem. -->\n\n# Complexity\n- Time complexity: O(n)\n<!-- Add your time complexity here, e.g. $$O(n)$$ -->\n\n- Space complexity:O(1)\n<!-- Add your space complexity here, e.g. $$O(n)$$ -->\n\n# Code\n```\nclass Solution(object):\n def partitionString(self, s):\n """\n :type s: str\n :rtype: int\n """\n count = 1\n seen = set()\n for char in s:\n if char not in seen:\n seen.add(char)\n continue\n seen.clear()\n seen.add(char)\n count +=1\n return count\n``` | 1 | Given a string `s`, partition the string into one or more **substrings** such that the characters in each substring are **unique**. That is, no letter appears in a single substring more than **once**.
Return _the **minimum** number of substrings in such a partition._
Note that each character should belong to exactly one substring in a partition.
**Example 1:**
**Input:** s = "abacaba "
**Output:** 4
**Explanation:**
Two possible partitions are ( "a ", "ba ", "cab ", "a ") and ( "ab ", "a ", "ca ", "ba ").
It can be shown that 4 is the minimum number of substrings needed.
**Example 2:**
**Input:** s = "ssssss "
**Output:** 6
**Explanation:**
The only valid partition is ( "s ", "s ", "s ", "s ", "s ", "s ").
**Constraints:**
* `1 <= s.length <= 105`
* `s` consists of only English lowercase letters. | null |
Very Easy Solution | Python3 | Greedy | optimal-partition-of-string | 0 | 1 | # Very easy solution with Greedy\ncreate substring (`ss`).\nIterate through each character `c` in `s` and check whether `c` is in `ss`. \nIf `c` is in `ss`, that means we can\'t add the `c` to `ss`. \nSo increment `t` and make `ss` empty. \n\nFinally, return `t+1` (because we started to count from 0 and we have not counted the first `ss`).\n\n# Code\n```\nclass Solution:\n def partitionString(self, s: str) -> int:\n t = 0\n ss = ""\n for c in s:\n if c in ss: \n t += 1\n ss = ""\n ss += c\n \n return t+1\n``` | 1 | Given a string `s`, partition the string into one or more **substrings** such that the characters in each substring are **unique**. That is, no letter appears in a single substring more than **once**.
Return _the **minimum** number of substrings in such a partition._
Note that each character should belong to exactly one substring in a partition.
**Example 1:**
**Input:** s = "abacaba "
**Output:** 4
**Explanation:**
Two possible partitions are ( "a ", "ba ", "cab ", "a ") and ( "ab ", "a ", "ca ", "ba ").
It can be shown that 4 is the minimum number of substrings needed.
**Example 2:**
**Input:** s = "ssssss "
**Output:** 6
**Explanation:**
The only valid partition is ( "s ", "s ", "s ", "s ", "s ", "s ").
**Constraints:**
* `1 <= s.length <= 105`
* `s` consists of only English lowercase letters. | null |
Min Heap | divide-intervals-into-minimum-number-of-groups | 0 | 1 | We use a min heap to track the rightmost number of each group.\n\nFirst, we sort the intervals. Then, for each interval, we check if the top of the heap is less than **left**.\n\nIf it is, we can add that interval to an existing group: pop from the heap, and push **right**, updating the rightmost number of that group.\n\nIf not, we need a new group: push **right** into the heap.\n\nIn the end, the size of the heap is the number of groups we need.\n\n**Python 3**\n```python\nclass Solution:\n def minGroups(self, intervals: List[List[int]]) -> int:\n pq = []\n for left, right in sorted(intervals):\n if pq and pq[0] < left:\n heappop(pq)\n heappush(pq, right)\n return len(pq)\n```\n\n**C++**\n```cpp\nint minGroups(vector<vector<int>>& ints) {\n sort(begin(ints), end(ints));\n priority_queue<int, vector<int>, greater<int>> pq;\n for (const auto &i : ints) {\n if (!pq.empty() && pq.top() < i[0])\n pq.pop();\n pq.push(i[1]);\n }\n return pq.size();\n}\n``` | 261 | You are given a 2D integer array `intervals` where `intervals[i] = [lefti, righti]` represents the **inclusive** interval `[lefti, righti]`.
You have to divide the intervals into one or more **groups** such that each interval is in **exactly** one group, and no two intervals that are in the same group **intersect** each other.
Return _the **minimum** number of groups you need to make_.
Two intervals **intersect** if there is at least one common number between them. For example, the intervals `[1, 5]` and `[5, 8]` intersect.
**Example 1:**
**Input:** intervals = \[\[5,10\],\[6,8\],\[1,5\],\[2,3\],\[1,10\]\]
**Output:** 3
**Explanation:** We can divide the intervals into the following groups:
- Group 1: \[1, 5\], \[6, 8\].
- Group 2: \[2, 3\], \[5, 10\].
- Group 3: \[1, 10\].
It can be proven that it is not possible to divide the intervals into fewer than 3 groups.
**Example 2:**
**Input:** intervals = \[\[1,3\],\[5,6\],\[8,10\],\[11,13\]\]
**Output:** 1
**Explanation:** None of the intervals overlap, so we can put all of them in one group.
**Constraints:**
* `1 <= intervals.length <= 105`
* `intervals[i].length == 2`
* `1 <= lefti <= righti <= 106` | null |
[Python 3] Line Sweep - Difference Array | divide-intervals-into-minimum-number-of-groups | 0 | 1 | # Intuition\n<!-- Describe your first thoughts on how to solve this problem. -->\n\n# Approach\n<!-- Describe your approach to solving the problem. -->\n\n# Complexity\n- Time complexity: $$O(n)$$\n<!-- Add your time complexity here, e.g. $$O(n)$$ -->\n\n- Space complexity: $$O(n)$$\n<!-- Add your space complexity here, e.g. $$O(n)$$ -->\n\n# Code\n```\nclass Solution:\n def minGroups(self, intervals: List[List[int]]) -> int:\n d = collections.defaultdict(int)\n for l, r in intervals:\n d[l] += 1\n d[r + 1] -= 1\n overlaps = res = 0\n for _, v in sorted(d.items()):\n overlaps += v\n res = max(res, overlaps)\n return res\n``` | 3 | You are given a 2D integer array `intervals` where `intervals[i] = [lefti, righti]` represents the **inclusive** interval `[lefti, righti]`.
You have to divide the intervals into one or more **groups** such that each interval is in **exactly** one group, and no two intervals that are in the same group **intersect** each other.
Return _the **minimum** number of groups you need to make_.
Two intervals **intersect** if there is at least one common number between them. For example, the intervals `[1, 5]` and `[5, 8]` intersect.
**Example 1:**
**Input:** intervals = \[\[5,10\],\[6,8\],\[1,5\],\[2,3\],\[1,10\]\]
**Output:** 3
**Explanation:** We can divide the intervals into the following groups:
- Group 1: \[1, 5\], \[6, 8\].
- Group 2: \[2, 3\], \[5, 10\].
- Group 3: \[1, 10\].
It can be proven that it is not possible to divide the intervals into fewer than 3 groups.
**Example 2:**
**Input:** intervals = \[\[1,3\],\[5,6\],\[8,10\],\[11,13\]\]
**Output:** 1
**Explanation:** None of the intervals overlap, so we can put all of them in one group.
**Constraints:**
* `1 <= intervals.length <= 105`
* `intervals[i].length == 2`
* `1 <= lefti <= righti <= 106` | null |
[Python3/Rust] Max DP Overlap | divide-intervals-into-minimum-number-of-groups | 0 | 1 | **Weekly Contest 310 Submission**\n\n**Disclaimer** \nMy contest submission was written in ```Python3```. I attempted submissions after the contest and noticed that this solution will TLE on average (as noted by ```xavier-xia-99``` it seems to be an approximate 10% acceptance chance in ```Python3```). Following the contest I wrote the same solution in ```Rust``` which does not TLE as of writing.\n\n**Intuition**\nIf we add a new interval to the input array that does not overlap with any other interval we can always group it with an existing group (no effect on the answer, unless added to an empty array). Furthermore, if an additional interval is added and does not overlap the current largest group it can be added to another group (resulting in the answer remaining the same). The problem becomes: **At what frequency does the most common subinterval occur?**\n\n**Max DP Overlap**\n1. Find the maximum ending value in all intervals (```max_val```).\n2. Create a ```dp``` array of size ```max_val``` that represents the occurrence of each number in the intervals from 1 to ```max_val```.\n3. Linearly compute the occurrences through a prefix sum.\n4. Return the maximum occurrence.\n\n**Python3 (will TLE on average)**\n```Python3 []\nclass Solution:\n def minGroups(self, I: List[List[int]]) -> int:\n max_val = max([v for _, v in I])\n dp = [0]*(max_val + 2)\n \n #Define intervals\n for u, v in I:\n dp[u] += 1\n dp[v + 1] -= 1\n \n #Compute prefix sum to get frequency\n for idx in range(1, len(dp)):\n dp[idx] += dp[idx - 1]\n \n #Return maximum overlap\n return max(dp)\n```\n**Rust (passes)**\n```Rust []\nimpl Solution {\n pub fn min_groups(I: Vec<Vec<i32>>) -> i32 {\n let mut max_val = 0;\n let mut res = 0;\n \n for idx in 0..I.len() {\n max_val = max_val.max(I[idx][1]);\n }\n \n let mut dp = vec![0; (max_val) as usize + 2];\n \n //Define intervals\n for idx in 0..I.len() {\n dp[I[idx][0] as usize] += 1;\n dp[I[idx][1] as usize + 1] -= 1;\n }\n \n //Compute prefix sum to get frequency\n for idx in 1..dp.len() {\n dp[idx] += dp[idx - 1];\n res = res.max(dp[idx]);\n }\n \n //Return maximum overlap\n res\n }\n}\n``` | 3 | You are given a 2D integer array `intervals` where `intervals[i] = [lefti, righti]` represents the **inclusive** interval `[lefti, righti]`.
You have to divide the intervals into one or more **groups** such that each interval is in **exactly** one group, and no two intervals that are in the same group **intersect** each other.
Return _the **minimum** number of groups you need to make_.
Two intervals **intersect** if there is at least one common number between them. For example, the intervals `[1, 5]` and `[5, 8]` intersect.
**Example 1:**
**Input:** intervals = \[\[5,10\],\[6,8\],\[1,5\],\[2,3\],\[1,10\]\]
**Output:** 3
**Explanation:** We can divide the intervals into the following groups:
- Group 1: \[1, 5\], \[6, 8\].
- Group 2: \[2, 3\], \[5, 10\].
- Group 3: \[1, 10\].
It can be proven that it is not possible to divide the intervals into fewer than 3 groups.
**Example 2:**
**Input:** intervals = \[\[1,3\],\[5,6\],\[8,10\],\[11,13\]\]
**Output:** 1
**Explanation:** None of the intervals overlap, so we can put all of them in one group.
**Constraints:**
* `1 <= intervals.length <= 105`
* `intervals[i].length == 2`
* `1 <= lefti <= righti <= 106` | null |
[Python3] Sort + Heap | divide-intervals-into-minimum-number-of-groups | 0 | 1 | ```\nclass Solution:\n def minGroups(self, intervals: List[List[int]]) -> int:\n intervals.sort()\n pq = []\n for interval in intervals:\n if not pq or interval[0] <= pq[0]:\n heapq.heappush(pq, interval[1])\n else:\n\t\t\t\theapq.heappop(pq)\n\t\t\t\theapq.heappush(pq, interval[1])\n return len(pq)\n``` | 3 | You are given a 2D integer array `intervals` where `intervals[i] = [lefti, righti]` represents the **inclusive** interval `[lefti, righti]`.
You have to divide the intervals into one or more **groups** such that each interval is in **exactly** one group, and no two intervals that are in the same group **intersect** each other.
Return _the **minimum** number of groups you need to make_.
Two intervals **intersect** if there is at least one common number between them. For example, the intervals `[1, 5]` and `[5, 8]` intersect.
**Example 1:**
**Input:** intervals = \[\[5,10\],\[6,8\],\[1,5\],\[2,3\],\[1,10\]\]
**Output:** 3
**Explanation:** We can divide the intervals into the following groups:
- Group 1: \[1, 5\], \[6, 8\].
- Group 2: \[2, 3\], \[5, 10\].
- Group 3: \[1, 10\].
It can be proven that it is not possible to divide the intervals into fewer than 3 groups.
**Example 2:**
**Input:** intervals = \[\[1,3\],\[5,6\],\[8,10\],\[11,13\]\]
**Output:** 1
**Explanation:** None of the intervals overlap, so we can put all of them in one group.
**Constraints:**
* `1 <= intervals.length <= 105`
* `intervals[i].length == 2`
* `1 <= lefti <= righti <= 106` | null |
✔ Python3 Solution | O(nlogn) | divide-intervals-into-minimum-number-of-groups | 0 | 1 | `Time Complexity` : `O(nlogn)`\n```\nclass Solution:\n def minGroups(self, A):\n A = list(map(sorted, zip(*A)))\n ans, j = 1, 0\n for i in range(1, len(A[0])):\n if A[0][i] > A[1][j]: j += 1\n else: ans += 1\n return ans\n``` | 1 | You are given a 2D integer array `intervals` where `intervals[i] = [lefti, righti]` represents the **inclusive** interval `[lefti, righti]`.
You have to divide the intervals into one or more **groups** such that each interval is in **exactly** one group, and no two intervals that are in the same group **intersect** each other.
Return _the **minimum** number of groups you need to make_.
Two intervals **intersect** if there is at least one common number between them. For example, the intervals `[1, 5]` and `[5, 8]` intersect.
**Example 1:**
**Input:** intervals = \[\[5,10\],\[6,8\],\[1,5\],\[2,3\],\[1,10\]\]
**Output:** 3
**Explanation:** We can divide the intervals into the following groups:
- Group 1: \[1, 5\], \[6, 8\].
- Group 2: \[2, 3\], \[5, 10\].
- Group 3: \[1, 10\].
It can be proven that it is not possible to divide the intervals into fewer than 3 groups.
**Example 2:**
**Input:** intervals = \[\[1,3\],\[5,6\],\[8,10\],\[11,13\]\]
**Output:** 1
**Explanation:** None of the intervals overlap, so we can put all of them in one group.
**Constraints:**
* `1 <= intervals.length <= 105`
* `intervals[i].length == 2`
* `1 <= lefti <= righti <= 106` | null |
Python Easy Solution Well Explained | longest-increasing-subsequence-ii | 0 | 1 | ```\n# Iterative Segment Tree\nclass SegmentTree:\n def __init__(self, n, fn):\n self.n = n\n self.fn = fn\n self.tree = [0] * (2 * n)\n \n def query(self, l, r):\n l += self.n\n r += self.n\n res = 0\n while l < r:\n if l & 1:\n res = self.fn(res, self.tree[l])\n l += 1\n if r & 1:\n r -= 1\n res = self.fn(res, self.tree[r])\n l >>= 1\n r >>= 1\n return res\n \n def update(self, i, val):\n i += self.n\n self.tree[i] = val\n while i > 1:\n i >>= 1\n self.tree[i] = self.fn(self.tree[i * 2], self.tree[i * 2 + 1])\nclass Solution:\n # Inspired from the solution of Bakerston\n # Basically we make an array to store nums in increasing order and find what is the size of the LIS ending there\n\t# Our array is 0 to max element from the given nums\n # We need to find the elements smaller than n till the limit k which are in nums before this num\n # so simply all the elements previous to our current num are smaller(till k smaller)\n # now how to find LIS\'s size?\n # Since we are updating elements left to right in our array we know the LIS\'s size of elements smaller\n # than our current num\n # we just need to find the largest LIS size in range (num - k, num) from our array\n # This is a range query and can be efficiently answered using Segment Trees.\n \n def lengthOfLIS(self, nums: List[int], k: int) -> int:\n n = max(nums)\n res = 1\n tree = SegmentTree(n, max)\n for num in nums:\n num -= 1\n mm = tree.query(max(0, num - k), num)\n tree.update(num, mm + 1)\n res = max(res, mm + 1)\n return res\n``` | 6 | You are given an integer array `nums` and an integer `k`.
Find the longest subsequence of `nums` that meets the following requirements:
* The subsequence is **strictly increasing** and
* The difference between adjacent elements in the subsequence is **at most** `k`.
Return _the length of the **longest** **subsequence** that meets the requirements._
A **subsequence** is an array that can be derived from another array by deleting some or no elements without changing the order of the remaining elements.
**Example 1:**
**Input:** nums = \[4,2,1,4,3,4,5,8,15\], k = 3
**Output:** 5
**Explanation:**
The longest subsequence that meets the requirements is \[1,3,4,5,8\].
The subsequence has a length of 5, so we return 5.
Note that the subsequence \[1,3,4,5,8,15\] does not meet the requirements because 15 - 8 = 7 is larger than 3.
**Example 2:**
**Input:** nums = \[7,4,5,1,8,12,4,7\], k = 5
**Output:** 4
**Explanation:**
The longest subsequence that meets the requirements is \[4,5,8,12\].
The subsequence has a length of 4, so we return 4.
**Example 3:**
**Input:** nums = \[1,5\], k = 1
**Output:** 1
**Explanation:**
The longest subsequence that meets the requirements is \[1\].
The subsequence has a length of 1, so we return 1.
**Constraints:**
* `1 <= nums.length <= 105`
* `1 <= nums[i], k <= 105` | null |
[Python3] segment tree | longest-increasing-subsequence-ii | 0 | 1 | Please pull this [commit](https://github.com/gaosanyong/leetcode/commit/b89b2c654a0b8ced9885ecdb433ad5f929a1d4b4) for solutions of weekly 310. \n\n```\nclass SegTree: \n\n def __init__(self, arr: List[int]): \n self.n = len(arr)\n self.tree = [0] * (2*self.n)\n # for i in range(2*self.n-1, 0, -1): \n # if i >= self.n: self.tree[i] = arr[i - self.n]\n # else: self.tree[i] = max(self.tree[i<<1], self.tree[i<<1|1])\n\n def query(self, lo: int, hi: int) -> int: \n ans = 0 \n lo += self.n \n hi += self.n\n while lo < hi: \n if lo & 1: \n ans = max(ans, self.tree[lo])\n lo += 1\n if hi & 1: \n hi -= 1\n ans = max(ans, self.tree[hi])\n lo >>= 1\n hi >>= 1\n return ans \n\n def update(self, i: int, val: int) -> None: \n i += self.n \n self.tree[i] = val\n while i > 1: \n self.tree[i>>1] = max(self.tree[i], self.tree[i^1])\n i >>= 1\n\nclass Solution:\n def lengthOfLIS(self, nums: List[int], k: int) -> int:\n m = max(nums)\n ans = 0 \n tree = SegTree([0] * (m+1))\n for x in nums: \n val = tree.query(max(0, x-k), x) + 1\n ans = max(ans, val)\n tree.update(x, val)\n return ans \n``` | 1 | You are given an integer array `nums` and an integer `k`.
Find the longest subsequence of `nums` that meets the following requirements:
* The subsequence is **strictly increasing** and
* The difference between adjacent elements in the subsequence is **at most** `k`.
Return _the length of the **longest** **subsequence** that meets the requirements._
A **subsequence** is an array that can be derived from another array by deleting some or no elements without changing the order of the remaining elements.
**Example 1:**
**Input:** nums = \[4,2,1,4,3,4,5,8,15\], k = 3
**Output:** 5
**Explanation:**
The longest subsequence that meets the requirements is \[1,3,4,5,8\].
The subsequence has a length of 5, so we return 5.
Note that the subsequence \[1,3,4,5,8,15\] does not meet the requirements because 15 - 8 = 7 is larger than 3.
**Example 2:**
**Input:** nums = \[7,4,5,1,8,12,4,7\], k = 5
**Output:** 4
**Explanation:**
The longest subsequence that meets the requirements is \[4,5,8,12\].
The subsequence has a length of 4, so we return 4.
**Example 3:**
**Input:** nums = \[1,5\], k = 1
**Output:** 1
**Explanation:**
The longest subsequence that meets the requirements is \[1\].
The subsequence has a length of 1, so we return 1.
**Constraints:**
* `1 <= nums.length <= 105`
* `1 <= nums[i], k <= 105` | null |
[Python] Segment Tree - Clean & Concise | longest-increasing-subsequence-ii | 0 | 1 | # Intuition\n- Use Segment Tree to query maximum of element in range [num-k, num-1], which mean to find the maximum existed Longest Increase Subsequence such that the maximum difference between consecutives element <= k.\n\n# Complexity\n- Time: `O(N * logMAX + MAX)`, where `N <= 10^5` is length of nums array, `MAX <= 10^5` is maximum element in nums array.\n- Space complexity: `O(MAX)`\n\n# Code\n```python\nclass SegmentTree:\n def __init__(self, n):\n self.n = n\n self.tree = [0] * 4 * self.n\n\n def query(self, left, right, index, qleft, qright):\n if qright < left or qleft > right:\n return 0\n\n if qleft <= left and right <= qright:\n return self.tree[index]\n\n mid = (left + right) // 2\n resLeft = self.query(left, mid, 2*index+1, qleft, qright)\n resRight = self.query(mid+1, right, 2*index+2, qleft, qright)\n return max(resLeft, resRight)\n\n def update(self, left, right, index, pos, val):\n if left == right:\n self.tree[index] = val\n return\n\n mid = (left + right) // 2\n if pos <= mid:\n self.update(left, mid, 2*index+1, pos, val)\n else:\n self.update(mid+1, right, 2*index+2, pos, val)\n self.tree[index] = max(self.tree[2*index+1], self.tree[2*index+2])\n\n\nclass Solution:\n def lengthOfLIS(self, nums: List[int], k: int) -> int:\n mx = max(nums)\n segmentTree = SegmentTree(mx + 1)\n ans = 0\n for num in nums:\n subLongest = segmentTree.query(0, segmentTree.n-1, 0, num-k, num-1) + 1\n ans = max(ans, subLongest)\n segmentTree.update(0, segmentTree.n-1, 0, num, subLongest)\n return ans\n``` | 3 | You are given an integer array `nums` and an integer `k`.
Find the longest subsequence of `nums` that meets the following requirements:
* The subsequence is **strictly increasing** and
* The difference between adjacent elements in the subsequence is **at most** `k`.
Return _the length of the **longest** **subsequence** that meets the requirements._
A **subsequence** is an array that can be derived from another array by deleting some or no elements without changing the order of the remaining elements.
**Example 1:**
**Input:** nums = \[4,2,1,4,3,4,5,8,15\], k = 3
**Output:** 5
**Explanation:**
The longest subsequence that meets the requirements is \[1,3,4,5,8\].
The subsequence has a length of 5, so we return 5.
Note that the subsequence \[1,3,4,5,8,15\] does not meet the requirements because 15 - 8 = 7 is larger than 3.
**Example 2:**
**Input:** nums = \[7,4,5,1,8,12,4,7\], k = 5
**Output:** 4
**Explanation:**
The longest subsequence that meets the requirements is \[4,5,8,12\].
The subsequence has a length of 4, so we return 4.
**Example 3:**
**Input:** nums = \[1,5\], k = 1
**Output:** 1
**Explanation:**
The longest subsequence that meets the requirements is \[1\].
The subsequence has a length of 1, so we return 1.
**Constraints:**
* `1 <= nums.length <= 105`
* `1 <= nums[i], k <= 105` | null |
DP to Segment Tree (Python) | longest-increasing-subsequence-ii | 0 | 1 | # Intuition\n<!-- Describe your first thoughts on how to solve this problem. -->\nTo make sense of the accepted segment tree approach, we first need to briefly discuss the relevant dynamic programming approach with $$O(nk)$$ time complexity and $$O(n)$$ space complexity.\n\nWe\'re going to traverse our array nums. For each element $x$ of $nums$, we\'re going to check for the longest increasing subsequence (LIS) that terminated at a value in the following set/interval: {$x - k, x - k + 1, ..., x - 1$}. We will track the prior LIS\'s that terminate at each value $x$ in an array of length equal to max($nums$).\n\nThis looks like:\n```python []\ndef lengthOfLIS(self, nums: List[int], k: int) -> int:\n lis = [0] * max(nums)\n for x in nums:\n intervalStart = max(0, x-1-k)\n for idx in range(intervalStart, x-1):\n lis[x-1] = max(lis[x-1], lis[idx]+1)\n \n return max(lis)\n```\n\nThis approach is TLE, so we need to use some extra space (still maintaining linear complexity) in order to improve our time complexity. This leads us to the segment tree.\n\n# Approach\n<!-- Describe your approach to solving the problem. -->\nWe build a binary tree where each node of our tree contains the length of LIS that terminates at a value in some interval $[l, r)$. Naturally, the root node of our tree corresponds to the interval $[1, \\max(nums)+1)$. \n\nConsider a node corresponding to the interval $[l, r)$. Let the midpoint of this interval, $c$, equal the floor of $(l + r) / 2$. Then the left child of this node corresponds to the interval $[l, c)$ and the right child corresponds to the interval $[c, r)$.\n\nWe will represent our tree implicitly by using the Eytzinger layout to map values to an array. Given a node whose value is at array index $m$, its left child is at index $2m$ and its right child is at index $2m + 1$. The root of our tree is at array index 1. \n\nI chose recursive implementations for the update and query methods because those made the most sense to me (I could justify to myself that the node to array index mapping was working and I could easily see how we traversed the tree to find our desired intervals). But I\'m definitely not an expert on segment trees and there are much faster implementations!\n\nPlease consult the following resources:\n- [Segment Trees (Recursive, Iterative, Best Optimizations)](https://en.algorithmica.org/hpc/data-structures/segment-trees/)\n- [Iterative Segment Tree](https://codeforces.com/blog/entry/18051)\n- [Iterative Segment Tree (python implementation)](https://leetcode.com/problems/longest-increasing-subsequence-ii/solutions/2560085/python-explanation-with-pictures-segment-tree/)\n\n# Complexity\n- Time complexity:\n<!-- Add your time complexity here, e.g. $$O(n)$$ -->\n$$O(n \\log(n)$$\n\n- Space complexity:\n<!-- Add your space complexity here, e.g. $$O(n)$$ -->\n$$O(n)$$\n\n# Code\n```python []\nclass SegmentTree:\n def __init__(self, n):\n self.tree = [0] * 4 * n\n self.n = n\n \n def update(self, l, r, x, val, treeIdx):\n if l == r - 1:\n self.tree[treeIdx] = max(self.tree[treeIdx], val)\n return \n \n c = (l + r) // 2\n if x < c:\n self.update(l, c, x, val, 2 * treeIdx)\n else:\n self.update(c, r, x, val, 2 * treeIdx + 1)\n\n self.tree[treeIdx] = max(self.tree[2 * treeIdx], self.tree[2 * treeIdx + 1])\n \n def query(self, lDesired, rDesired, l, r, treeIdx):\n if lDesired <= l and rDesired >= r:\n return self.tree[treeIdx]\n \n c = (l + r) // 2\n leftMax, rightMax = 0, 0\n if lDesired < c:\n leftMax = self.query(lDesired, rDesired, l, c, 2 * treeIdx)\n if rDesired > c:\n rightMax = self.query(lDesired, rDesired, c, r, 2 * treeIdx + 1)\n \n return max(leftMax, rightMax) \n \nclass Solution:\n def lengthOfLIS(self, nums: List[int], k: int) -> int:\n n = max(nums)\n seg = SegmentTree(n)\n for num in nums:\n lis = seg.query(max(1, num - k), num, 1, n+1, 1)\n seg.update(1, n+1, num, lis+1, 1)\n\n #print(f\'seg.tree = {seg.tree}\')\n return seg.tree[1] \n \n``` | 1 | You are given an integer array `nums` and an integer `k`.
Find the longest subsequence of `nums` that meets the following requirements:
* The subsequence is **strictly increasing** and
* The difference between adjacent elements in the subsequence is **at most** `k`.
Return _the length of the **longest** **subsequence** that meets the requirements._
A **subsequence** is an array that can be derived from another array by deleting some or no elements without changing the order of the remaining elements.
**Example 1:**
**Input:** nums = \[4,2,1,4,3,4,5,8,15\], k = 3
**Output:** 5
**Explanation:**
The longest subsequence that meets the requirements is \[1,3,4,5,8\].
The subsequence has a length of 5, so we return 5.
Note that the subsequence \[1,3,4,5,8,15\] does not meet the requirements because 15 - 8 = 7 is larger than 3.
**Example 2:**
**Input:** nums = \[7,4,5,1,8,12,4,7\], k = 5
**Output:** 4
**Explanation:**
The longest subsequence that meets the requirements is \[4,5,8,12\].
The subsequence has a length of 4, so we return 4.
**Example 3:**
**Input:** nums = \[1,5\], k = 1
**Output:** 1
**Explanation:**
The longest subsequence that meets the requirements is \[1\].
The subsequence has a length of 1, so we return 1.
**Constraints:**
* `1 <= nums.length <= 105`
* `1 <= nums[i], k <= 105` | null |
Python Elegant & Short | Built-In date | count-days-spent-together | 0 | 1 | \tfrom datetime import date\n\n\n\tclass Solution:\n\t\t"""\n\t\tTime: O(1)\n\t\tMemory: O(1)\n\t\t"""\n\n\t\tdef countDaysTogether(self, arrive_alice: str, leave_alice: str, arrive_bob: str, leave_bob: str) -> int:\n\t\t\tstart = max(self.to_date(arrive_alice), self.to_date(arrive_bob))\n\t\t\tend = min(self.to_date(leave_alice), self.to_date(leave_bob))\n\t\t\treturn max((end - start).days + 1, 0)\n\n\t\t@staticmethod\n\t\tdef to_date(month_day: str) -> date:\n\t\t\treturn date(2001, *map(int, month_day.split(\'-\')))\n | 2 | Alice and Bob are traveling to Rome for separate business meetings.
You are given 4 strings `arriveAlice`, `leaveAlice`, `arriveBob`, and `leaveBob`. Alice will be in the city from the dates `arriveAlice` to `leaveAlice` (**inclusive**), while Bob will be in the city from the dates `arriveBob` to `leaveBob` (**inclusive**). Each will be a 5-character string in the format `"MM-DD "`, corresponding to the month and day of the date.
Return _the total number of days that Alice and Bob are in Rome together._
You can assume that all dates occur in the **same** calendar year, which is **not** a leap year. Note that the number of days per month can be represented as: `[31, 28, 31, 30, 31, 30, 31, 31, 30, 31, 30, 31]`.
**Example 1:**
**Input:** arriveAlice = "08-15 ", leaveAlice = "08-18 ", arriveBob = "08-16 ", leaveBob = "08-19 "
**Output:** 3
**Explanation:** Alice will be in Rome from August 15 to August 18. Bob will be in Rome from August 16 to August 19. They are both in Rome together on August 16th, 17th, and 18th, so the answer is 3.
**Example 2:**
**Input:** arriveAlice = "10-01 ", leaveAlice = "10-31 ", arriveBob = "11-01 ", leaveBob = "12-31 "
**Output:** 0
**Explanation:** There is no day when Alice and Bob are in Rome together, so we return 0.
**Constraints:**
* All dates are provided in the format `"MM-DD "`.
* Alice and Bob's arrival dates are **earlier than or equal to** their leaving dates.
* The given dates are valid dates of a **non-leap** year. | null |
✅ Python simple solution | easy to understand | explained | count-days-spent-together | 0 | 1 | #### Understanding\n* Find the minimum date of leave between Alice & Bob.\n* Find the maximum date of arrival between Alice & Bob.\n* The desire date will be between these two limit.\n\n#### Example\n* Let the input be `arriveAlice = "08-15", leaveAlice = "08-18", arriveBob = "08-16", leaveBob = "08-19"`\n* The minimum date of leave between Alice & Bob is `"08-18"`.\n* The max date of arrival between Alice & Bob is `"08-16"`.\n* Total number of days together will be between these days.\n\t* Calculate from `"08-18"` the total days in a year using `getDate(date)` method and we get **230** days.\n\t* Calculate from `"08-16"` the total days in a year using `getDate(date)` method and we get **228** days.\n* Now substract `230 - 228 + 1`. As days are inclusive so we add 1.\n* Return the max value between substranct value and 0.\n\n##### Code\n<iframe src="https://leetcode.com/playground/6BEiBvaS/shared" frameBorder="0" width="800" height="300"></iframe>\n | 23 | Alice and Bob are traveling to Rome for separate business meetings.
You are given 4 strings `arriveAlice`, `leaveAlice`, `arriveBob`, and `leaveBob`. Alice will be in the city from the dates `arriveAlice` to `leaveAlice` (**inclusive**), while Bob will be in the city from the dates `arriveBob` to `leaveBob` (**inclusive**). Each will be a 5-character string in the format `"MM-DD "`, corresponding to the month and day of the date.
Return _the total number of days that Alice and Bob are in Rome together._
You can assume that all dates occur in the **same** calendar year, which is **not** a leap year. Note that the number of days per month can be represented as: `[31, 28, 31, 30, 31, 30, 31, 31, 30, 31, 30, 31]`.
**Example 1:**
**Input:** arriveAlice = "08-15 ", leaveAlice = "08-18 ", arriveBob = "08-16 ", leaveBob = "08-19 "
**Output:** 3
**Explanation:** Alice will be in Rome from August 15 to August 18. Bob will be in Rome from August 16 to August 19. They are both in Rome together on August 16th, 17th, and 18th, so the answer is 3.
**Example 2:**
**Input:** arriveAlice = "10-01 ", leaveAlice = "10-31 ", arriveBob = "11-01 ", leaveBob = "12-31 "
**Output:** 0
**Explanation:** There is no day when Alice and Bob are in Rome together, so we return 0.
**Constraints:**
* All dates are provided in the format `"MM-DD "`.
* Alice and Bob's arrival dates are **earlier than or equal to** their leaving dates.
* The given dates are valid dates of a **non-leap** year. | null |
Beginner Friendly Python Approach | maximum-matching-of-players-with-trainers | 0 | 1 | # Intuition\nSimple\n\n# Approach\nTwo pointer approach is the better approach for this question when considering the constraints.\n\n# Complexity\n- Time complexity:\nO(n) to O(n^2)\n\n- Space complexity:\nO(N)\n\n# Code\n```\nclass Solution:\n def matchPlayersAndTrainers(self, players: List[int], trainers: List[int]) -> int:\n i=0;j=0\n c=0\n p=sorted(players,reverse=True)\n t=sorted(trainers,reverse=True)\n while i<len(players) and j<len(trainers):\n if p[i]<=t[j]:\n c+=1\n i+=1\n j+=1\n else:\n i+=1\n return c\n \n\n \n``` | 2 | You are given a **0-indexed** integer array `players`, where `players[i]` represents the **ability** of the `ith` player. You are also given a **0-indexed** integer array `trainers`, where `trainers[j]` represents the **training capacity** of the `jth` trainer.
The `ith` player can **match** with the `jth` trainer if the player's ability is **less than or equal to** the trainer's training capacity. Additionally, the `ith` player can be matched with at most one trainer, and the `jth` trainer can be matched with at most one player.
Return _the **maximum** number of matchings between_ `players` _and_ `trainers` _that satisfy these conditions._
**Example 1:**
**Input:** players = \[4,7,9\], trainers = \[8,2,5,8\]
**Output:** 2
**Explanation:**
One of the ways we can form two matchings is as follows:
- players\[0\] can be matched with trainers\[0\] since 4 <= 8.
- players\[1\] can be matched with trainers\[3\] since 7 <= 8.
It can be proven that 2 is the maximum number of matchings that can be formed.
**Example 2:**
**Input:** players = \[1,1,1\], trainers = \[10\]
**Output:** 1
**Explanation:**
The trainer can be matched with any of the 3 players.
Each player can only be matched with one trainer, so the maximum answer is 1.
**Constraints:**
* `1 <= players.length, trainers.length <= 105`
* `1 <= players[i], trainers[j] <= 109` | null |
Python3 Greedy & Two pointers Solution - Beats 100% | maximum-matching-of-players-with-trainers | 0 | 1 | 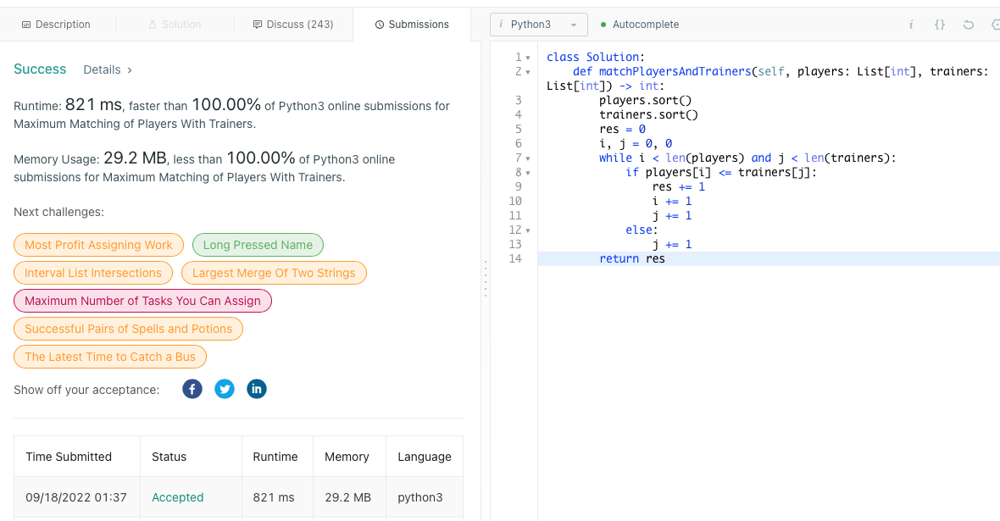\n\n```\nclass Solution:\n def matchPlayersAndTrainers(self, players: List[int], trainers: List[int]) -> int:\n players.sort()\n trainers.sort()\n res = 0\n i, j = 0, 0\n while i < len(players) and j < len(trainers):\n if players[i] <= trainers[j]:\n res += 1\n i += 1\n j += 1\n return res\n```\n\n- Sort players and trainers\n- Use two pointers i and j to scan players and trainers\n- We scan both in ascending order because we want to match them greedily | 1 | You are given a **0-indexed** integer array `players`, where `players[i]` represents the **ability** of the `ith` player. You are also given a **0-indexed** integer array `trainers`, where `trainers[j]` represents the **training capacity** of the `jth` trainer.
The `ith` player can **match** with the `jth` trainer if the player's ability is **less than or equal to** the trainer's training capacity. Additionally, the `ith` player can be matched with at most one trainer, and the `jth` trainer can be matched with at most one player.
Return _the **maximum** number of matchings between_ `players` _and_ `trainers` _that satisfy these conditions._
**Example 1:**
**Input:** players = \[4,7,9\], trainers = \[8,2,5,8\]
**Output:** 2
**Explanation:**
One of the ways we can form two matchings is as follows:
- players\[0\] can be matched with trainers\[0\] since 4 <= 8.
- players\[1\] can be matched with trainers\[3\] since 7 <= 8.
It can be proven that 2 is the maximum number of matchings that can be formed.
**Example 2:**
**Input:** players = \[1,1,1\], trainers = \[10\]
**Output:** 1
**Explanation:**
The trainer can be matched with any of the 3 players.
Each player can only be matched with one trainer, so the maximum answer is 1.
**Constraints:**
* `1 <= players.length, trainers.length <= 105`
* `1 <= players[i], trainers[j] <= 109` | null |
Extremely Simple Implementation python3. Faster than 100% solutions | maximum-matching-of-players-with-trainers | 0 | 1 | ```py\nclass Solution:\n def matchPlayersAndTrainers(self, players: List[int], t: List[int]) -> int:\n \n c = j = i = 0\n players.sort()\n t.sort()\n while i <= len(players) - 1 and j <= len(t) - 1:\n if players[i] <= t[j]:\n \n c += 1\n i += 1\n j += 1\n return c\n```\nThe solution is pretty self explanatory. But if still anyone have any doubt feel free to comment below. | 1 | You are given a **0-indexed** integer array `players`, where `players[i]` represents the **ability** of the `ith` player. You are also given a **0-indexed** integer array `trainers`, where `trainers[j]` represents the **training capacity** of the `jth` trainer.
The `ith` player can **match** with the `jth` trainer if the player's ability is **less than or equal to** the trainer's training capacity. Additionally, the `ith` player can be matched with at most one trainer, and the `jth` trainer can be matched with at most one player.
Return _the **maximum** number of matchings between_ `players` _and_ `trainers` _that satisfy these conditions._
**Example 1:**
**Input:** players = \[4,7,9\], trainers = \[8,2,5,8\]
**Output:** 2
**Explanation:**
One of the ways we can form two matchings is as follows:
- players\[0\] can be matched with trainers\[0\] since 4 <= 8.
- players\[1\] can be matched with trainers\[3\] since 7 <= 8.
It can be proven that 2 is the maximum number of matchings that can be formed.
**Example 2:**
**Input:** players = \[1,1,1\], trainers = \[10\]
**Output:** 1
**Explanation:**
The trainer can be matched with any of the 3 players.
Each player can only be matched with one trainer, so the maximum answer is 1.
**Constraints:**
* `1 <= players.length, trainers.length <= 105`
* `1 <= players[i], trainers[j] <= 109` | null |
[Secret Python Answer🤫🐍👌😍] Sliding Window with Double Dictionary of Binary Count | smallest-subarrays-with-maximum-bitwise-or | 0 | 1 | ```\nclass Solution:\n def smallestSubarrays(self, nums: List[int]) -> List[int]:\n\n def create(m):\n t = 0\n for n in m:\n if m[n] > 0:\n t = t | (1 << n)\n return t\n \n def add(a,m):\n ans = bin( a )\n s = str(ans)[2:]\n for i, b in enumerate( s[::-1]):\n if b == \'1\':\n m[i] += 1\n\n def remove(a,m):\n ans = bin( a )\n s = str(ans)[2:]\n for i, b in enumerate( s[::-1]):\n if b == \'1\':\n m[i] -= 1\n \n res = []\n\n \n n = defaultdict(int)\n for i in nums:\n add(i,n)\n\n \n m = defaultdict(int)\n r = 0\n c = 0\n\n for i,v in enumerate(nums):\n # The last check is for if nums[i] == 0, in that case we still want to add to the map\n while r < len(nums) and (create(m) != create(n) or (c==0 and nums[i] ==0)):\n add(nums[r],m)\n r+=1\n c+=1\n\n res.append(c)\n\n remove(nums[i],m)\n remove(nums[i],n)\n c-=1\n\n return res\n``` | 1 | You are given a **0-indexed** array `nums` of length `n`, consisting of non-negative integers. For each index `i` from `0` to `n - 1`, you must determine the size of the **minimum sized** non-empty subarray of `nums` starting at `i` (**inclusive**) that has the **maximum** possible **bitwise OR**.
* In other words, let `Bij` be the bitwise OR of the subarray `nums[i...j]`. You need to find the smallest subarray starting at `i`, such that bitwise OR of this subarray is equal to `max(Bik)` where `i <= k <= n - 1`.
The bitwise OR of an array is the bitwise OR of all the numbers in it.
Return _an integer array_ `answer` _of size_ `n` _where_ `answer[i]` _is the length of the **minimum** sized subarray starting at_ `i` _with **maximum** bitwise OR._
A **subarray** is a contiguous non-empty sequence of elements within an array.
**Example 1:**
**Input:** nums = \[1,0,2,1,3\]
**Output:** \[3,3,2,2,1\]
**Explanation:**
The maximum possible bitwise OR starting at any index is 3.
- Starting at index 0, the shortest subarray that yields it is \[1,0,2\].
- Starting at index 1, the shortest subarray that yields the maximum bitwise OR is \[0,2,1\].
- Starting at index 2, the shortest subarray that yields the maximum bitwise OR is \[2,1\].
- Starting at index 3, the shortest subarray that yields the maximum bitwise OR is \[1,3\].
- Starting at index 4, the shortest subarray that yields the maximum bitwise OR is \[3\].
Therefore, we return \[3,3,2,2,1\].
**Example 2:**
**Input:** nums = \[1,2\]
**Output:** \[2,1\]
**Explanation:**
Starting at index 0, the shortest subarray that yields the maximum bitwise OR is of length 2.
Starting at index 1, the shortest subarray that yields the maximum bitwise OR is of length 1.
Therefore, we return \[2,1\].
**Constraints:**
* `n == nums.length`
* `1 <= n <= 105`
* `0 <= nums[i] <= 109` | null |
The easiest possible explanation you are looking for | minimum-money-required-before-transactions | 0 | 1 | It took me a while to get this through my head. So take your time but make sure you understand this.\n\n**Alse note that this is not the perfect O(N) solution. You can see Lee215 soln for that. This is O(NlogN) solution but this is what was easier for me to understand**\n\n**Now lets start**\n\n1. First, know that there are 2 kinds of transaction, one where you earn more than you spend (good transactions), and one where you earn less than you spend (bad transactions).\n2. In the worst case, you will need to perform all the bad transactions at first. What would be even worse? You will have to perform the worst bad transaction first. What does that mean? It means, the transaction, that gives you the least cashback among all bad transactions.\n3. Now that the bad transactions are over, now we will perform the good transactions. Now what can be the worst case here? It can be that you need to perform the costliest transaction among the good transactions first. But once you have done the costliest transaction, you no longer need to check the further transactions. Why so? Because this is a good transaction. If you spend say 10$, you are guaranteed to receive a cashback of 10$ or more. And since this was the costliest transaction among the good transaction, you will now have money for the rest of them anyway, so no need to consider them.\n\n**Lets take an example to understand even better**\n\nwe are given this transaction array :`[[7,2],[0,10],[5,0],[4,1],[5,8],[5,9]]`\nLets separate the good and bad transactions.\n\n**good transactions** :`[[0,10],[5,8],[5,9]]`\n**bad transactions** :`[[7,2],[5,0],[4,1]]`\n\nNow cosider the **bad transactions**:\n\tWhich one among `[[7,2],[5,0],[4,1]]` is the worst transaction you might have to make? The answer to that is `[5,0]`. **Why?** because you are spending 5$ and getting nothing in return. What will be the next worst transaction? It will be `[4,1]` for the same reason. Do you see the **pattern**?\n\t\nWe do the transaction with the least amount of cashback first. Because there we need to have our own money and can\'t rely on cashback. So if we follow this,\n**First transaction:** `cur += 5` = 5; `need = max(need,cur)` = 5; `cur -= 0` = 5\n**Second transaction:** `cur += 4` = 9; `need = max(need,cur)` = 9; `cur -= 1` = 8 ( **NOTE** the need will first become 9, because we get a cashback only after paying the cost first. So in order to make the first and the second transaction, you need minimum of 9$)\n**Third transaction:** `cur += 7` = 15; `need = max(need,cur)` = 15; `cur -= 2` = 13\n\n**Okay so at this point all the bad transactions are over and everything bad that could have happened has happened... Except one\uD83D\uDE05**\nEven though we only have good transactions now, we need to pay the cost for them to recieve the cashback. This is simpler though. We only need to figure out which is the costliest good transaction. **Why?** because once we pay that amount, we know since its a good transaction, we will get atleast the same amount back if not more.\n\nSo the ***worst*** **good transaction** is `[5,8] or [5,9]`. `cur += 5` = 18; `need = max(need,cur)` = 18\n\nAnd we got our answer. We need a minimum of 18$ in order to complete all transaction in any order!\n\n**Let\'s finally have a look at the code to solidify what I just explained**\n\n```\ndef minimumMoney(self, transactions: List[List[int]]) -> int:\n \n\tgoodTransactions = [txn for txn in transactions if txn[0] <= txn[1]]\n\tbadTransactions = [txn for txn in transactions if txn[0] > txn[1]]\n\n\tbadTransactions.sort(key=lambda x: x[1])\n\n\tneed = 0\n\tcur_amount = 0\n\n\tfor cost,cashback in badTransactions:\n\t\tcur_amount += cost\n\t\tneed = max(need,cur_amount)\n\t\tcur_amount -= cashback\n\n\tif goodTransactions:\n\t\tcostliest_good_transaction = max(goodTransactions, key=lambda x: x[0])\n\t\tcur_amount += costliest_good_transaction[0] \n\t\tneed = max(need, cur_amount)\n\n\treturn need\n```\t\t\n\n***If this post was helpful at all, pls do upvote : )*** | 5 | You are given a **0-indexed** 2D integer array `transactions`, where `transactions[i] = [costi, cashbacki]`.
The array describes transactions, where each transaction must be completed exactly once in **some order**. At any given moment, you have a certain amount of `money`. In order to complete transaction `i`, `money >= costi` must hold true. After performing a transaction, `money` becomes `money - costi + cashbacki`.
Return _the minimum amount of_ `money` _required before any transaction so that all of the transactions can be completed **regardless of the order** of the transactions._
**Example 1:**
**Input:** transactions = \[\[2,1\],\[5,0\],\[4,2\]\]
**Output:** 10
**Explanation:**
Starting with money = 10, the transactions can be performed in any order.
It can be shown that starting with money < 10 will fail to complete all transactions in some order.
**Example 2:**
**Input:** transactions = \[\[3,0\],\[0,3\]\]
**Output:** 3
**Explanation:**
- If transactions are in the order \[\[3,0\],\[0,3\]\], the minimum money required to complete the transactions is 3.
- If transactions are in the order \[\[0,3\],\[3,0\]\], the minimum money required to complete the transactions is 0.
Thus, starting with money = 3, the transactions can be performed in any order.
**Constraints:**
* `1 <= transactions.length <= 105`
* `transactions[i].length == 2`
* `0 <= costi, cashbacki <= 109` | null |
Python: Follow the Hints | minimum-money-required-before-transactions | 0 | 1 | # Intuition\n<!-- Describe your first thoughts on how to solve this problem. -->\nOverall, we want to figure out how much money we need up front to ensure that we have enough money before we try processing any transaction to pay for its cost, regardless of whether we get a big "cashback" or not. And, we want to do this for *any* order of transaction. Does that mean we have to try all orders? No, but we need to figure out what the worst order would be.\n\nThe hints describe how to split the transactions into two classes which I called ```incomers``` (cost <= cashback) and ```outgoers``` (cost > cashback). It *appears* that the worst order is sorting the two classes as suggested and then doing all the ```outgoers``` and then all the ```incomers```; I actually tried the opposite way at first and found that it passed some but not most of the test cases.\n\nSo, first the ```outgoers```: Since every transaction has a cost greater than the cashback, as we do more and more of them, our cash on hand gets less and less. In fact, it hits a low point just after we pay the cost for the last outgoer but before we get its cashback.\nThat gives us a first estimate about how much we need up front, which is called ```min_ante``` in the code. If we have ```min_ante``` to start with, we can process all the ```outgoers``` and end up with the cashback from the last outgoer in our pocket.\n\nNext, we have to process the ```incomers```. Because these transactions all have cost no more than cashback, as we process more and more of the ```incomers``` we never fall behind and may even make up some cash in the process. The only provision is that we have to have up front the cost of the most expensive incomer, called ```min_incomer_available``` in the code If the money we had after processing the ```outgoers``` doesn\'t cover that amount, then we have to increase the up front investment ```min_ante``` by the shortfall.\n# Approach\n<!-- Describe your approach to solving the problem. -->\nThe code is a pretty straightforward implementation of the Hints and Intuition. One thing I learned during the implementation was that it was possible that the ```incomers``` or the ```outgoers``` might be empty.\n# Complexity\n- Time complexity:\n<!-- Add your time complexity here, e.g. $$O(n)$$ -->\nWe have to process all the ```outgoers``` to compute ```min_ante``` and ```after_outgoers```. However, we only need to look at the first of the ```incomers```. The overall time complexity is $$O(n)$$ where $$n$$ is the number of transactions.\n- Space complexity:\n<!-- Add your space complexity here, e.g. $$O(n)$$ -->\nThe non-scalar storage used includes space for ```incomers``` and ```outgoers```, which gives a space complexity of $$O(n)$$.\n# Code\n```\nclass Solution:\n def minimumMoney(self, transactions: list[list[int]]) -> int:\n # First, split and sort the input "transactions" per the hint\n incomers = [transaction for transaction in transactions if transaction[0] <= transaction[1]]\n outgoers = [transaction for transaction in transactions if transaction[0] > transaction[1]]\n incomers.sort(key=lambda transaction: transaction[0], reverse=True)\n outgoers.sort(key=lambda transaction: transaction[1])\n\n # Second, process "outgoers", transactions where cost > cashback: The question is: how\n # much money do we need up front in order to complete processing of\n # the outgoers. The latter amount is the sum of the net cost of all but the last\n # transaction plus the cost of the last transaction.\n if outgoers:\n outgoer_cost = sum(map(lambda transaction: transaction[0] - transaction[1], outgoers) )\n assert outgoer_cost > 0\n min_ante = outgoer_cost + outgoers[-1][1]\n after_outgoers = min_ante - outgoer_cost\n # What this means: If we start with "min_ante", we can process all the "outgoers" and\n # end up with "after_outgoers".\n else:\n min_ante = 0\n after_outgoers = 0\n\n # Third, process the "incomers", transactions where cashback is >= cost. I *think* it\'s enough\n # to have enough cash on hand to satisfy the first "cost" since then, by construction, we\'ll\n # have enough for all subsequent "incomers".\n if incomers:\n min_incomer_available = incomers[0][0]\n else:\n min_incomer_available = 0\n\n # Fourth, put together the two components:\n if min_incomer_available > after_outgoers:\n min_ante += min_incomer_available - after_outgoers\n return min_ante\n``` | 0 | You are given a **0-indexed** 2D integer array `transactions`, where `transactions[i] = [costi, cashbacki]`.
The array describes transactions, where each transaction must be completed exactly once in **some order**. At any given moment, you have a certain amount of `money`. In order to complete transaction `i`, `money >= costi` must hold true. After performing a transaction, `money` becomes `money - costi + cashbacki`.
Return _the minimum amount of_ `money` _required before any transaction so that all of the transactions can be completed **regardless of the order** of the transactions._
**Example 1:**
**Input:** transactions = \[\[2,1\],\[5,0\],\[4,2\]\]
**Output:** 10
**Explanation:**
Starting with money = 10, the transactions can be performed in any order.
It can be shown that starting with money < 10 will fail to complete all transactions in some order.
**Example 2:**
**Input:** transactions = \[\[3,0\],\[0,3\]\]
**Output:** 3
**Explanation:**
- If transactions are in the order \[\[3,0\],\[0,3\]\], the minimum money required to complete the transactions is 3.
- If transactions are in the order \[\[0,3\],\[3,0\]\], the minimum money required to complete the transactions is 0.
Thus, starting with money = 3, the transactions can be performed in any order.
**Constraints:**
* `1 <= transactions.length <= 105`
* `transactions[i].length == 2`
* `0 <= costi, cashbacki <= 109` | null |
🔥 [LeetCode The Hard Way] 🔥 Explained Line By Line | smallest-even-multiple | 0 | 1 | Please check out [LeetCode The Hard Way](https://wingkwong.github.io/leetcode-the-hard-way/) for more solution explanations and tutorials. \nIf you like it, please give a star, watch my [Github Repository](https://github.com/wingkwong/leetcode-the-hard-way) and upvote this post. You may also join my [Discord](https://discord.gg/Nqm4jJcyBf) for more updates.\n\n---\n\n**Python**\n\n```py\nclass Solution:\n def smallestEvenMultiple(self, n: int) -> int:\n\t\t# it\'s just asking for LCM of 2 and n\n return lcm(2, n)\n```\n\n```py\nclass Solution:\n def smallestEvenMultiple(self, n: int) -> int:\n\t\t# alternatively, we can use GCD to calculate LCM\n return (2 * n) // gcd(2, n)\n```\n\n```py\nclass Solution:\n def smallestEvenMultiple(self, n: int) -> int:\n\t\t# or simply check if it is divisable by 2, if so return n\n\t\t# else return its double\n return 2 * n if n & 1 else n\n``` | 5 | Given a **positive** integer `n`, return _the smallest positive integer that is a multiple of **both**_ `2` _and_ `n`.
**Example 1:**
**Input:** n = 5
**Output:** 10
**Explanation:** The smallest multiple of both 5 and 2 is 10.
**Example 2:**
**Input:** n = 6
**Output:** 6
**Explanation:** The smallest multiple of both 6 and 2 is 6. Note that a number is a multiple of itself.
**Constraints:**
* `1 <= n <= 150` | null |
Solution of smallest even multiple problem | smallest-even-multiple | 0 | 1 | # **There are two cases:**\n- if number is even: return number\n- if number is odd(else): return double number value\n\n# Code\n```\nclass Solution:\n def smallestEvenMultiple(self, n: int) -> int:\n if n % 2 == 0:\n return n\n else:\n return n*2\n \n``` | 1 | Given a **positive** integer `n`, return _the smallest positive integer that is a multiple of **both**_ `2` _and_ `n`.
**Example 1:**
**Input:** n = 5
**Output:** 10
**Explanation:** The smallest multiple of both 5 and 2 is 10.
**Example 2:**
**Input:** n = 6
**Output:** 6
**Explanation:** The smallest multiple of both 6 and 2 is 6. Note that a number is a multiple of itself.
**Constraints:**
* `1 <= n <= 150` | null |
Brute Force and Logic Methods | Python3 | smallest-even-multiple | 0 | 1 | # Complexity\n- Time complexity:\n***$$O(n)$$ and $$O(1)$$***\n\n- Space complexity:\n***$$O(n)$$ and $$O(1)$$***\n\n# Code:\n#### ***Brute Force:***\n```python [Brute Force]\nclass Solution:\n def smallestEvenMultiple(self, n: int) -> int:\n for i in range(n, n*2+1):\n if i % n == 0 and i % 2 == 0:\n return i \n```\n#### ***Logic:***\n```python [Logic]\nclass Solution:\n def smallestEvenMultiple(self, n: int) -> int:\n return n * 2 if n % 2 else n\n```\n# ***Please upvote for this solution!***\n | 1 | Given a **positive** integer `n`, return _the smallest positive integer that is a multiple of **both**_ `2` _and_ `n`.
**Example 1:**
**Input:** n = 5
**Output:** 10
**Explanation:** The smallest multiple of both 5 and 2 is 10.
**Example 2:**
**Input:** n = 6
**Output:** 6
**Explanation:** The smallest multiple of both 6 and 2 is 6. Note that a number is a multiple of itself.
**Constraints:**
* `1 <= n <= 150` | null |
[Short Python Answer🤫🐍👌😍] One Line and 15 Characters | smallest-even-multiple | 0 | 1 | ```\nclass Solution:\n def smallestEvenMultiple(self, n: int) -> int:\n return lcm(n,2) | 2 | Given a **positive** integer `n`, return _the smallest positive integer that is a multiple of **both**_ `2` _and_ `n`.
**Example 1:**
**Input:** n = 5
**Output:** 10
**Explanation:** The smallest multiple of both 5 and 2 is 10.
**Example 2:**
**Input:** n = 6
**Output:** 6
**Explanation:** The smallest multiple of both 6 and 2 is 6. Note that a number is a multiple of itself.
**Constraints:**
* `1 <= n <= 150` | null |
✅ Explained - Simple and Clear Python3 Code✅ | length-of-the-longest-alphabetical-continuous-substring | 0 | 1 | # Intuition\nThe problem asks us to find the length of the longest alphabetical continuous substring in a given string. To solve this, we can iterate through the string and keep track of the current count of consecutive alphabetical characters. Whenever we encounter a character that breaks the alphabetical sequence, we update the maximum count found so far. Finally, we return the maximum count as the result.\n\n\n# Approach\n1.\tInitialize variables: We start by initializing a count variable to keep track of the current count of consecutive alphabetical characters and a result variable to store the maximum count found so far. We also set the index variable \'i\' to 1, representing the current index in the string.\n2.\tIteration: We iterate through the string starting from the second character (index 1) until the end.\n3.\tChecking alphabetical sequence: For each character, we compare its ASCII value with the ASCII value of the previous character. If the ASCII value of the current character is one more than the previous character, it means the alphabetical sequence is continuing. In that case, we increment the count by 1.\n4.\tBreaking the sequence: If the ASCII value of the current character does not satisfy the condition mentioned above, it means the alphabetical sequence is broken. We update the result by taking the maximum of the current count and the previous result. Additionally, we reset the count to 1, as we start counting a new alphabetical sequence.\n5.\tIncrement index: After each iteration, we increment the index variable \'i\' by 1 to move to the next character in the string.\n6.\tFinalizing the result: After the loop ends, we update the result one final time by taking the maximum of the last count and the previous result, as there might be a valid alphabetical sequence ending at the last character.\n7.\tReturn the result: Finally, we return the result as the length of the longest alphabetical continuous substring in the given string.\n\n\n# Complexity\n- Time complexity:\nThe algorithm iterates through the input string once, resulting in a linear time complexity of O(n), where n is the length of the string.\n\n\n- Space complexity:\nThe algorithm uses a constant amount of extra space to store variables, resulting in a space complexity of O(1).\n\n\n# Code\n```\nclass Solution:\n def longestContinuousSubstring(self, s: str) -> int:\n count=1\n i=1\n res=1\n while i<len(s):\n if ord(s[i-1])+1==ord(s[i]):\n count+=1\n else:\n res=max(res,count)\n count=1\n i+=1\n res=max(res,count)\n return res \n``` | 6 | An **alphabetical continuous string** is a string consisting of consecutive letters in the alphabet. In other words, it is any substring of the string `"abcdefghijklmnopqrstuvwxyz "`.
* For example, `"abc "` is an alphabetical continuous string, while `"acb "` and `"za "` are not.
Given a string `s` consisting of lowercase letters only, return the _length of the **longest** alphabetical continuous substring._
**Example 1:**
**Input:** s = "abacaba "
**Output:** 2
**Explanation:** There are 4 distinct continuous substrings: "a ", "b ", "c " and "ab ".
"ab " is the longest continuous substring.
**Example 2:**
**Input:** s = "abcde "
**Output:** 5
**Explanation:** "abcde " is the longest continuous substring.
**Constraints:**
* `1 <= s.length <= 105`
* `s` consists of only English lowercase letters. | null |
Python3 O(n) solution | length-of-the-longest-alphabetical-continuous-substring | 0 | 1 | # Complexity\n- Time complexity: O(n)\n\n- Space complexity: O(1)\n\n# Code\n```\nclass Solution:\n def longestContinuousSubstring(self, s: str) -> int:\n m = 0\n curr = 1\n for i in range(len(s)-1):\n if ord(s[i])+1==ord(s[i+1]):\n curr += 1\n else:\n if curr > m:\n m = curr\n curr = 1\n if curr > m:\n m = curr\n return m\n``` | 1 | An **alphabetical continuous string** is a string consisting of consecutive letters in the alphabet. In other words, it is any substring of the string `"abcdefghijklmnopqrstuvwxyz "`.
* For example, `"abc "` is an alphabetical continuous string, while `"acb "` and `"za "` are not.
Given a string `s` consisting of lowercase letters only, return the _length of the **longest** alphabetical continuous substring._
**Example 1:**
**Input:** s = "abacaba "
**Output:** 2
**Explanation:** There are 4 distinct continuous substrings: "a ", "b ", "c " and "ab ".
"ab " is the longest continuous substring.
**Example 2:**
**Input:** s = "abcde "
**Output:** 5
**Explanation:** "abcde " is the longest continuous substring.
**Constraints:**
* `1 <= s.length <= 105`
* `s` consists of only English lowercase letters. | null |
Self Understandable solution: | length-of-the-longest-alphabetical-continuous-substring | 0 | 1 | ```\nclass Solution:\n def longestContinuousSubstring(self, s: str) -> int:\n x=set()\n p=[s[0]]\n for i in s[1:]:\n if ord(p[-1])+1==ord(i):\n p.append(i)\n else:\n x.add("".join(p))\n p=[i]\n x.add("".join(p))\n ans=sorted(x,key=len)[-1]\n return len(ans)\n``` | 1 | An **alphabetical continuous string** is a string consisting of consecutive letters in the alphabet. In other words, it is any substring of the string `"abcdefghijklmnopqrstuvwxyz "`.
* For example, `"abc "` is an alphabetical continuous string, while `"acb "` and `"za "` are not.
Given a string `s` consisting of lowercase letters only, return the _length of the **longest** alphabetical continuous substring._
**Example 1:**
**Input:** s = "abacaba "
**Output:** 2
**Explanation:** There are 4 distinct continuous substrings: "a ", "b ", "c " and "ab ".
"ab " is the longest continuous substring.
**Example 2:**
**Input:** s = "abcde "
**Output:** 5
**Explanation:** "abcde " is the longest continuous substring.
**Constraints:**
* `1 <= s.length <= 105`
* `s` consists of only English lowercase letters. | null |
Python3 || Two-Pointer | length-of-the-longest-alphabetical-continuous-substring | 0 | 1 | \n# Approach\nInitialize i with index 0 and j with index 1.\nFix the pointer \'i\' and keep on moving pointer \'j\' until the difference between ascii value of s[j] and s[j-1] equals 1. Keep updating the answer with max length obtained till the current step. once the difference between ascii value of s[j] and s[j-1] not equals 1, i.e s[j] and s[j-1] are not consecutive update the pointer \'i\' to pointer \'j\' and pointer \'j\' to j+1. Here updation indicates that we are trying to find the possible solutions starting at index \'i\'.\n# Complexity\n- Time complexity:\nO(n)\n- Space complexity:\nO(1)\n# Code\n```\nclass Solution:\n def longestContinuousSubstring(self, s: str) -> int:\n i = 0\n j = 1\n n = len(s)\n ans = 1\n while j<n:\n while j<n and ord(s[j])-ord(s[j-1])==1:\n ans = max(ans,j-i+1)\n j+=1\n if j<n and ord(s[j])-ord(s[j-1])!=1:\n i = j\n j+=1\n return ans\n``` | 1 | An **alphabetical continuous string** is a string consisting of consecutive letters in the alphabet. In other words, it is any substring of the string `"abcdefghijklmnopqrstuvwxyz "`.
* For example, `"abc "` is an alphabetical continuous string, while `"acb "` and `"za "` are not.
Given a string `s` consisting of lowercase letters only, return the _length of the **longest** alphabetical continuous substring._
**Example 1:**
**Input:** s = "abacaba "
**Output:** 2
**Explanation:** There are 4 distinct continuous substrings: "a ", "b ", "c " and "ab ".
"ab " is the longest continuous substring.
**Example 2:**
**Input:** s = "abcde "
**Output:** 5
**Explanation:** "abcde " is the longest continuous substring.
**Constraints:**
* `1 <= s.length <= 105`
* `s` consists of only English lowercase letters. | null |
python3 solution using queue beats 100% | reverse-odd-levels-of-binary-tree | 0 | 1 | # stats\n<!-- Describe your first thoughts on how to solve this problem. -->\n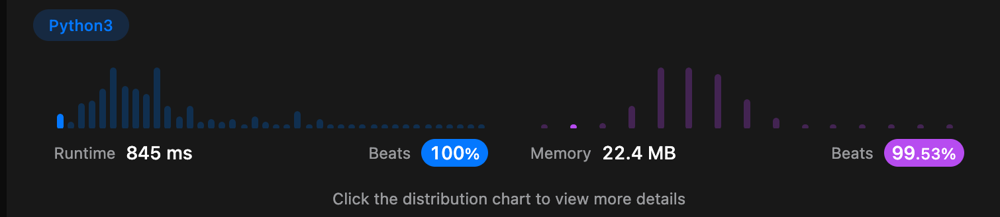\n\n# Approach\n<!-- Describe your approach to solving the problem. -->\nwe have a queue and we are using level order traversal and a counter L \nif left node exists then both node exist and we can append to queue\ncounter L plays a crucial role as when it becomes odd power of 2 like 2,8,32,128 etc\nthis is detected by `(l&(l-1))==0 and l%3==2` where `l&l-1==0` checks if it is power of 2 `2^n`and l%3==0 checks if it is odd power of 2 `2^2n-1`and if so we reverse all values in the nodes by using `q[i].val` and keep swapping for half the length of queue\nat end queue will be empty and we can return\n\n# Complexity\n- Time complexity:\n<!-- Add your time complexity here, e.g. $$O(n)$$ -->\n\n- Space complexity:\n<!-- Add your space complexity here, e.g. $$O(n)$$ -->\n\n# Code\n```\n# Definition for a binary tree node.\n# class TreeNode:\n# def __init__(self, val=0, left=None, right=None):\n# self.val = val\n# self.left = left\n# self.right = right\nclass Solution:\n def reverseOddLevels(self, root: Optional[TreeNode]) -> Optional[TreeNode]:\n\n l=1\n q=[root]\n while q:\n node=q.pop(0)\n l+=1\n if node.left:\n q.append(node.left)\n q.append(node.right)\n if (l&(l-1))==0 and l%3==2:\n for i in range(len(q)//2):\n q[i].val,q[-1-i].val=q[-1-i].val,q[i].val\n return root\n``` | 1 | Given the `root` of a **perfect** binary tree, reverse the node values at each **odd** level of the tree.
* For example, suppose the node values at level 3 are `[2,1,3,4,7,11,29,18]`, then it should become `[18,29,11,7,4,3,1,2]`.
Return _the root of the reversed tree_.
A binary tree is **perfect** if all parent nodes have two children and all leaves are on the same level.
The **level** of a node is the number of edges along the path between it and the root node.
**Example 1:**
**Input:** root = \[2,3,5,8,13,21,34\]
**Output:** \[2,5,3,8,13,21,34\]
**Explanation:**
The tree has only one odd level.
The nodes at level 1 are 3, 5 respectively, which are reversed and become 5, 3.
**Example 2:**
**Input:** root = \[7,13,11\]
**Output:** \[7,11,13\]
**Explanation:**
The nodes at level 1 are 13, 11, which are reversed and become 11, 13.
**Example 3:**
**Input:** root = \[0,1,2,0,0,0,0,1,1,1,1,2,2,2,2\]
**Output:** \[0,2,1,0,0,0,0,2,2,2,2,1,1,1,1\]
**Explanation:**
The odd levels have non-zero values.
The nodes at level 1 were 1, 2, and are 2, 1 after the reversal.
The nodes at level 3 were 1, 1, 1, 1, 2, 2, 2, 2, and are 2, 2, 2, 2, 1, 1, 1, 1 after the reversal.
**Constraints:**
* The number of nodes in the tree is in the range `[1, 214]`.
* `0 <= Node.val <= 105`
* `root` is a **perfect** binary tree. | null |
Python3 || Beats 93.89% | reverse-odd-levels-of-binary-tree | 0 | 1 | 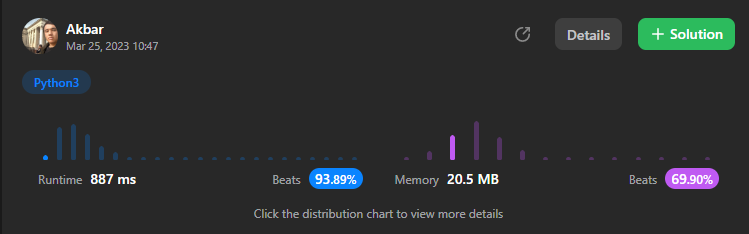\n# Please UPVOTE\uD83D\uDE0A\n\n# Python3\n```\n# Definition for a binary tree node.\n# class TreeNode:\n# def __init__(self, val=0, left=None, right=None):\n# self.val = val\n# self.left = left\n# self.right = right\nclass Solution:\n def reverseOddLevels(self, root: Optional[TreeNode]) -> Optional[TreeNode]:\n v=[root]\n l=0\n while v:\n l+=1\n vc=[]\n for i in v:\n if(i.left):\n vc+=[i.left]\n if(i.right):\n vc+=[i.right]\n if(l%2==1):\n for i in range((len(vc)+1)//2):\n vc[i].val,vc[len(vc)-i-1].val=vc[len(vc)-i-1].val,vc[i].val\n v=vc\n return root\n\n\n``` | 16 | Given the `root` of a **perfect** binary tree, reverse the node values at each **odd** level of the tree.
* For example, suppose the node values at level 3 are `[2,1,3,4,7,11,29,18]`, then it should become `[18,29,11,7,4,3,1,2]`.
Return _the root of the reversed tree_.
A binary tree is **perfect** if all parent nodes have two children and all leaves are on the same level.
The **level** of a node is the number of edges along the path between it and the root node.
**Example 1:**
**Input:** root = \[2,3,5,8,13,21,34\]
**Output:** \[2,5,3,8,13,21,34\]
**Explanation:**
The tree has only one odd level.
The nodes at level 1 are 3, 5 respectively, which are reversed and become 5, 3.
**Example 2:**
**Input:** root = \[7,13,11\]
**Output:** \[7,11,13\]
**Explanation:**
The nodes at level 1 are 13, 11, which are reversed and become 11, 13.
**Example 3:**
**Input:** root = \[0,1,2,0,0,0,0,1,1,1,1,2,2,2,2\]
**Output:** \[0,2,1,0,0,0,0,2,2,2,2,1,1,1,1\]
**Explanation:**
The odd levels have non-zero values.
The nodes at level 1 were 1, 2, and are 2, 1 after the reversal.
The nodes at level 3 were 1, 1, 1, 1, 2, 2, 2, 2, and are 2, 2, 2, 2, 1, 1, 1, 1 after the reversal.
**Constraints:**
* The number of nodes in the tree is in the range `[1, 214]`.
* `0 <= Node.val <= 105`
* `root` is a **perfect** binary tree. | null |
Easy Python Solution With Dictionary | sum-of-prefix-scores-of-strings | 0 | 1 | ```\nclass Solution:\n def sumPrefixScores(self, words: List[str]) -> List[int]:\n d = dict()\n for i in words:\n s=""\n for j in range(len(i)):\n s+=i[j]\n if s in d:\n d[s]+=1\n else:\n d[s]=1\n l=[]\n for i in words:\n c = 0\n s=""\n for j in range(len(i)):\n s+=i[j]\n c+=d[s]\n l.append(c)\n return l\n```\n***Please Upvote if you like this.*** | 1 | You are given an array `words` of size `n` consisting of **non-empty** strings.
We define the **score** of a string `word` as the **number** of strings `words[i]` such that `word` is a **prefix** of `words[i]`.
* For example, if `words = [ "a ", "ab ", "abc ", "cab "]`, then the score of `"ab "` is `2`, since `"ab "` is a prefix of both `"ab "` and `"abc "`.
Return _an array_ `answer` _of size_ `n` _where_ `answer[i]` _is the **sum** of scores of every **non-empty** prefix of_ `words[i]`.
**Note** that a string is considered as a prefix of itself.
**Example 1:**
**Input:** words = \[ "abc ", "ab ", "bc ", "b "\]
**Output:** \[5,4,3,2\]
**Explanation:** The answer for each string is the following:
- "abc " has 3 prefixes: "a ", "ab ", and "abc ".
- There are 2 strings with the prefix "a ", 2 strings with the prefix "ab ", and 1 string with the prefix "abc ".
The total is answer\[0\] = 2 + 2 + 1 = 5.
- "ab " has 2 prefixes: "a " and "ab ".
- There are 2 strings with the prefix "a ", and 2 strings with the prefix "ab ".
The total is answer\[1\] = 2 + 2 = 4.
- "bc " has 2 prefixes: "b " and "bc ".
- There are 2 strings with the prefix "b ", and 1 string with the prefix "bc ".
The total is answer\[2\] = 2 + 1 = 3.
- "b " has 1 prefix: "b ".
- There are 2 strings with the prefix "b ".
The total is answer\[3\] = 2.
**Example 2:**
**Input:** words = \[ "abcd "\]
**Output:** \[4\]
**Explanation:**
"abcd " has 4 prefixes: "a ", "ab ", "abc ", and "abcd ".
Each prefix has a score of one, so the total is answer\[0\] = 1 + 1 + 1 + 1 = 4.
**Constraints:**
* `1 <= words.length <= 1000`
* `1 <= words[i].length <= 1000`
* `words[i]` consists of lowercase English letters. | null |
Simple Easy to Read trie solution | Python3 | sum-of-prefix-scores-of-strings | 0 | 1 | ```\nclass TrieNode:\n \n def __init__(self, ch: str) -> None:\n self.ch = ch\n self.endOfWord = 0\n self.vstd = 0\n self.children = {}\n\n\nclass Solution:\n # O(n*m) time, n --> len(words), m --> len(words[i]) \n # O(n*m) space,\n # Approach: trie, hashtable\n def sumPrefixScores(self, words: List[str]) -> List[int]:\n trie = TrieNode(\'\')\n ans = [] \n \n def addWord(word: str) -> None:\n curr = trie\n for c in word:\n if c not in curr.children:\n nod = TrieNode(c)\n curr.children[c] = nod\n curr = curr.children[c]\n curr.vstd +=1\n \n curr.endOfWord +=1 \n \n \n def calcScore(word: str) -> int:\n score = 0\n curr = trie\n \n for c in word:\n curr = curr.children[c]\n score += curr.vstd\n return score\n \n \n for word in words:\n addWord(word)\n \n for word in words:\n score = calcScore(word)\n ans.append(score)\n \n return ans\n``` | 1 | You are given an array `words` of size `n` consisting of **non-empty** strings.
We define the **score** of a string `word` as the **number** of strings `words[i]` such that `word` is a **prefix** of `words[i]`.
* For example, if `words = [ "a ", "ab ", "abc ", "cab "]`, then the score of `"ab "` is `2`, since `"ab "` is a prefix of both `"ab "` and `"abc "`.
Return _an array_ `answer` _of size_ `n` _where_ `answer[i]` _is the **sum** of scores of every **non-empty** prefix of_ `words[i]`.
**Note** that a string is considered as a prefix of itself.
**Example 1:**
**Input:** words = \[ "abc ", "ab ", "bc ", "b "\]
**Output:** \[5,4,3,2\]
**Explanation:** The answer for each string is the following:
- "abc " has 3 prefixes: "a ", "ab ", and "abc ".
- There are 2 strings with the prefix "a ", 2 strings with the prefix "ab ", and 1 string with the prefix "abc ".
The total is answer\[0\] = 2 + 2 + 1 = 5.
- "ab " has 2 prefixes: "a " and "ab ".
- There are 2 strings with the prefix "a ", and 2 strings with the prefix "ab ".
The total is answer\[1\] = 2 + 2 = 4.
- "bc " has 2 prefixes: "b " and "bc ".
- There are 2 strings with the prefix "b ", and 1 string with the prefix "bc ".
The total is answer\[2\] = 2 + 1 = 3.
- "b " has 1 prefix: "b ".
- There are 2 strings with the prefix "b ".
The total is answer\[3\] = 2.
**Example 2:**
**Input:** words = \[ "abcd "\]
**Output:** \[4\]
**Explanation:**
"abcd " has 4 prefixes: "a ", "ab ", "abc ", and "abcd ".
Each prefix has a score of one, so the total is answer\[0\] = 1 + 1 + 1 + 1 = 4.
**Constraints:**
* `1 <= words.length <= 1000`
* `1 <= words[i].length <= 1000`
* `words[i]` consists of lowercase English letters. | null |
[ Python ] ✅✅ Simple Python Solution Using Dictionary 🥳✌👍 | sum-of-prefix-scores-of-strings | 0 | 1 | # If You like the Solution, Don\'t Forget To UpVote Me, Please UpVote! \uD83D\uDD3C\uD83D\uDE4F\n# Runtime: 8638 ms, faster than 9.09% of Python3 online submissions for Sum of Prefix Scores of Strings.\n# Memory Usage: 243.6 MB, less than 9.09% of Python3 online submissions for Sum of Prefix Scores of Strings.\n\n\tclass Solution:\n\t\tdef sumPrefixScores(self, words: List[str]) -> List[int]:\n\n\t\t\td = defaultdict(int)\n\n\t\t\tfor word in words:\n\t\t\t\tfor index in range(1, len(word) + 1):\n\t\t\t\t\td[word[:index]] += 1 \n\n\t\t\tresult = []\n\n\t\t\tfor word in words:\n\t\t\t\tcurrent_sum = 0\n\n\t\t\t\tfor index in range(1, len(word) + 1):\n\t\t\t\t\tcurrent_sum = current_sum + d[word[:index]]\n\n\t\t\t\tresult.append(current_sum)\n\n\t\t\treturn result\n\n# Thank You \uD83E\uDD73\u270C\uD83D\uDC4D | 1 | You are given an array `words` of size `n` consisting of **non-empty** strings.
We define the **score** of a string `word` as the **number** of strings `words[i]` such that `word` is a **prefix** of `words[i]`.
* For example, if `words = [ "a ", "ab ", "abc ", "cab "]`, then the score of `"ab "` is `2`, since `"ab "` is a prefix of both `"ab "` and `"abc "`.
Return _an array_ `answer` _of size_ `n` _where_ `answer[i]` _is the **sum** of scores of every **non-empty** prefix of_ `words[i]`.
**Note** that a string is considered as a prefix of itself.
**Example 1:**
**Input:** words = \[ "abc ", "ab ", "bc ", "b "\]
**Output:** \[5,4,3,2\]
**Explanation:** The answer for each string is the following:
- "abc " has 3 prefixes: "a ", "ab ", and "abc ".
- There are 2 strings with the prefix "a ", 2 strings with the prefix "ab ", and 1 string with the prefix "abc ".
The total is answer\[0\] = 2 + 2 + 1 = 5.
- "ab " has 2 prefixes: "a " and "ab ".
- There are 2 strings with the prefix "a ", and 2 strings with the prefix "ab ".
The total is answer\[1\] = 2 + 2 = 4.
- "bc " has 2 prefixes: "b " and "bc ".
- There are 2 strings with the prefix "b ", and 1 string with the prefix "bc ".
The total is answer\[2\] = 2 + 1 = 3.
- "b " has 1 prefix: "b ".
- There are 2 strings with the prefix "b ".
The total is answer\[3\] = 2.
**Example 2:**
**Input:** words = \[ "abcd "\]
**Output:** \[4\]
**Explanation:**
"abcd " has 4 prefixes: "a ", "ab ", "abc ", and "abcd ".
Each prefix has a score of one, so the total is answer\[0\] = 1 + 1 + 1 + 1 = 4.
**Constraints:**
* `1 <= words.length <= 1000`
* `1 <= words[i].length <= 1000`
* `words[i]` consists of lowercase English letters. | null |
Solution of sort the people problem | sort-the-people | 0 | 1 | # Approach\n- Step one: zip 2 list into one\n- Step two: sort it with function `.sorted()`\n- Step three: return sorted list in descending order by the people\'s heights.\n# Complexity\n- Time complexity:\n$$O(N * log(N))$$ - as sorted function takes O(N * log(N)) time and loop takes linear time -> $$O(N * log(N) + N)$$ -> $$O(N * log(N))$$\n\n\n# Code\n```\nclass Solution:\n def sortPeople(self, names: List[str], heights: List[int]) -> List[str]:\n heights_dict = zip(heights, names)\n sorted_dict = sorted(heights_dict, reverse=True)\n return [name for height, name in sorted_dict]\n``` | 1 | You are given an array of strings `names`, and an array `heights` that consists of **distinct** positive integers. Both arrays are of length `n`.
For each index `i`, `names[i]` and `heights[i]` denote the name and height of the `ith` person.
Return `names` _sorted in **descending** order by the people's heights_.
**Example 1:**
**Input:** names = \[ "Mary ", "John ", "Emma "\], heights = \[180,165,170\]
**Output:** \[ "Mary ", "Emma ", "John "\]
**Explanation:** Mary is the tallest, followed by Emma and John.
**Example 2:**
**Input:** names = \[ "Alice ", "Bob ", "Bob "\], heights = \[155,185,150\]
**Output:** \[ "Bob ", "Alice ", "Bob "\]
**Explanation:** The first Bob is the tallest, followed by Alice and the second Bob.
**Constraints:**
* `n == names.length == heights.length`
* `1 <= n <= 103`
* `1 <= names[i].length <= 20`
* `1 <= heights[i] <= 105`
* `names[i]` consists of lower and upper case English letters.
* All the values of `heights` are distinct. | null |
Sort Names | sort-the-people | 0 | 1 | # Intuition\n<!-- Describe your first thoughts on how to solve this problem. -->\n\n# Approach\n<!-- Describe your approach to solving the problem. -->\n\n# Complexity\n- Time complexity:\n<!-- Add your time complexity here, e.g. $$O(n)$$ -->\n\n- Space complexity:\n<!-- Add your space complexity here, e.g. $$O(n)$$ -->\n\n# Code\n```\nclass Solution:\n def sortPeople(self, names: List[str], heights: List[int]) -> List[str]:\n lis=[]\n for i in range(len(names)):\n lis.append([heights[i],names[i]])\n lis.sort(reverse=True)\n lis1=[]\n for i in range(len(names)):\n lis1.append(lis[i][1])\n return lis1\n``` | 1 | You are given an array of strings `names`, and an array `heights` that consists of **distinct** positive integers. Both arrays are of length `n`.
For each index `i`, `names[i]` and `heights[i]` denote the name and height of the `ith` person.
Return `names` _sorted in **descending** order by the people's heights_.
**Example 1:**
**Input:** names = \[ "Mary ", "John ", "Emma "\], heights = \[180,165,170\]
**Output:** \[ "Mary ", "Emma ", "John "\]
**Explanation:** Mary is the tallest, followed by Emma and John.
**Example 2:**
**Input:** names = \[ "Alice ", "Bob ", "Bob "\], heights = \[155,185,150\]
**Output:** \[ "Bob ", "Alice ", "Bob "\]
**Explanation:** The first Bob is the tallest, followed by Alice and the second Bob.
**Constraints:**
* `n == names.length == heights.length`
* `1 <= n <= 103`
* `1 <= names[i].length <= 20`
* `1 <= heights[i] <= 105`
* `names[i]` consists of lower and upper case English letters.
* All the values of `heights` are distinct. | null |
[Java/Python 3] Longest consecutive max subarray. | longest-subarray-with-maximum-bitwise-and | 1 | 1 | Max bitwise `AND` subarray must include maximum element(s) **ONLY**. Therefore, we only need to find the longest subarray including max only.\n\n**Method1: Two Pass:**\n\n```java\n public int longestSubarray(int[] nums) {\n int max = 0, longest = 1, cur = 0;\n for (int num : nums) {\n max = Math.max(max, num);\n }\n for (int num : nums) {\n if (num == max) {\n longest = Math.max(longest, ++cur);\n }else {\n cur = 0;\n }\n }\n return longest;\n }\n```\n```python\n def longestSubarray(self, nums: List[int]) -> int:\n mx = max(nums)\n longest = cur = 0\n for num in nums:\n if num == mx:\n cur += 1\n longest = max(longest, cur)\n else:\n cur = 0\n return longest\n```\n\n----\n\n\n**Method 2: 1 Pass** - inspired by **@conchwu**\n\n```java\n public int longestSubarray(int[] nums) {\n int max = 0, longest = 0, cur = 0;\n for (int num : nums) {\n if (num == max) {\n longest = Math.max(longest, ++cur);\n }else if (num > max) {\n max = num;\n cur = longest = 1;\n }else {\n cur = 0;\n }\n }\n return longest;\n }\n```\nor \n```java\n public int longestSubarray(int[] nums) {\n int max = 0, longest = 0, cur = 0;\n for (int num : nums) {\n if (num > max) {\n max = num;\n longest = cur = 0;\n }\n cur = num == max ? ++cur : 0;\n longest = Math.max(longest, cur);\n }\n return longest;\n }\n```\n\n----\n\n```python\n def longestSubarray(self, nums: List[int]) -> int:\n longest = cur = mx = 0\n for num in nums:\n if num > mx:\n mx = num\n longest = cur = 1\n elif num == mx:\n cur += 1\n longest = max(longest, cur)\n else:\n cur = 0 \n return longest\n```\nor\n\n```python\n def longestSubarray(self, nums: List[int]) -> int:\n longest = cur = mx = 0\n for num in nums:\n if num > mx:\n mx = num\n longest = cur = 0\n cur = cur + 1 if num == mx else 0\n longest = max(longest, cur)\n return longest\n```\n**Analysis:**\n\nTime: `O(n)`, space: `O(1)`. | 28 | You are given an integer array `nums` of size `n`.
Consider a **non-empty** subarray from `nums` that has the **maximum** possible **bitwise AND**.
* In other words, let `k` be the maximum value of the bitwise AND of **any** subarray of `nums`. Then, only subarrays with a bitwise AND equal to `k` should be considered.
Return _the length of the **longest** such subarray_.
The bitwise AND of an array is the bitwise AND of all the numbers in it.
A **subarray** is a contiguous sequence of elements within an array.
**Example 1:**
**Input:** nums = \[1,2,3,3,2,2\]
**Output:** 2
**Explanation:**
The maximum possible bitwise AND of a subarray is 3.
The longest subarray with that value is \[3,3\], so we return 2.
**Example 2:**
**Input:** nums = \[1,2,3,4\]
**Output:** 1
**Explanation:**
The maximum possible bitwise AND of a subarray is 4.
The longest subarray with that value is \[4\], so we return 1.
**Constraints:**
* `1 <= nums.length <= 105`
* `1 <= nums[i] <= 106` | null |
Group By and One-Pass | longest-subarray-with-maximum-bitwise-and | 0 | 1 | The maximum possible bitwise AND should not be less than a largest element.\n\nTherefore, the subarray must only include one or more largest elements.\n\n**Python 3**\n```python\nclass Solution:\n def longestSubarray(self, nums: List[int]) -> int:\n max_n = max(nums)\n return max(len(list(it)) for n, it in groupby(nums) if n == max_n)\n```\n\n**C++**\nOne-pass solution.\n```cpp\nint longestSubarray(vector<int>& nums) {\n int max_n = 0, cnt = 0, res = 0;\n for (int n : nums) {\n if (max_n < n) {\n max_n = n;\n res = cnt = 0;\n }\n cnt = n == max_n ? cnt + 1 : 0;\n res = max(res, cnt);\n }\n return res;\n}\n``` | 10 | You are given an integer array `nums` of size `n`.
Consider a **non-empty** subarray from `nums` that has the **maximum** possible **bitwise AND**.
* In other words, let `k` be the maximum value of the bitwise AND of **any** subarray of `nums`. Then, only subarrays with a bitwise AND equal to `k` should be considered.
Return _the length of the **longest** such subarray_.
The bitwise AND of an array is the bitwise AND of all the numbers in it.
A **subarray** is a contiguous sequence of elements within an array.
**Example 1:**
**Input:** nums = \[1,2,3,3,2,2\]
**Output:** 2
**Explanation:**
The maximum possible bitwise AND of a subarray is 3.
The longest subarray with that value is \[3,3\], so we return 2.
**Example 2:**
**Input:** nums = \[1,2,3,4\]
**Output:** 1
**Explanation:**
The maximum possible bitwise AND of a subarray is 4.
The longest subarray with that value is \[4\], so we return 1.
**Constraints:**
* `1 <= nums.length <= 105`
* `1 <= nums[i] <= 106` | null |
PYTHON-3, BEATS 100%, UNIQUE SOLUTION, SLIDING- WINDOW, ROLLING-HASH, UNORDERED-SET, ARRAYS | find-all-good-indices | 0 | 1 | # Intuition\n<!-- Describe your first thoughts on how to solve this problem. -->\n\n# Approach\nIf len(nums) == 3, ans will always be [1]. If k == 1, ans will always have all indices from 1 to len(nums) - 2. Instead of using two k-sized loops for every index, we will use two windows of size k to accomplish the goal in one pass. The first k sized window starting from the left is analyzed. It should have elements in non-increasing manner. If we find a pair of indices not following this rule, how about we insert the left index of the two into a set named left to indicate breakage of rule at this index. Similarly, analyzing the first right k-sized window, we insert indices at which the rule is broken into a set named right. The right sided windows need to have elements in non-decreasing order. Now for every possible index that can be a part of the ans list, if size of left and right sets is zero, this means that to the left and right of current index, the k- sized windows are following their corresponding rules. Hence we add the index to our ans list. As the windows will shift for the next index, we will handle troublesome indices that will no longer be part of our k-sized windows. For both the left and right sets, if i - k index and i + 1 index were troublesome before, we will remove them as they will no longer be a part of the k-sized windows for the next index. Next, for the two new indices that will become a part of the k-sized windows on both sides, we again check for breaking of rules and add the elements accordingly. There are multiple conditions controlling access to invalid indexes.\n\n# Complexity\n- Time complexity:\n<!-- Add your time complexity here, e.g. $$O(n)$$ -->\n\n- Space complexity:\n<!-- Add your space complexity here, e.g. $$O(n)$$ -->\n\n# Code\n```\nclass Solution:\n def goodIndices(self, nums: List[int], k: int) -> List[int]:\n\n n = len(nums)\n\n ans = []\n\n if n == 3:\n\n return [1]\n\n if k == 1:\n\n for i in range(1, n - 1):\n\n ans.append(i)\n\n return ans\n\n left = set()\n\n right = set()\n\n for i in range (k - 1):\n\n if nums[i] < nums[i + 1]:\n\n left.add(i)\n\n for i in range(k + 1, 2 * k):\n\n if i + 1 >= n:\n \n break\n\n if nums[i] > nums[i + 1]:\n\n right.add(i)\n\n for i in range(k, n - k + 1):\n\n if i + k >= n:\n\n break\n\n if len(left) == 0 and len(right) == 0:\n\n ans.append(i)\n\n if i - k in left:\n\n left.remove(i - k)\n\n if i + 1 in right:\n\n right.remove(i + 1)\n\n if nums[i] > nums[i - 1]:\n\n left.add(i - 1)\n\n if i + k + 1 < n and nums[i + k] > nums[i + k + 1]:\n\n right.add(i + k)\n\n \n return ans\n``` | 1 | You are given a **0-indexed** integer array `nums` of size `n` and a positive integer `k`.
We call an index `i` in the range `k <= i < n - k` **good** if the following conditions are satisfied:
* The `k` elements that are just **before** the index `i` are in **non-increasing** order.
* The `k` elements that are just **after** the index `i` are in **non-decreasing** order.
Return _an array of all good indices sorted in **increasing** order_.
**Example 1:**
**Input:** nums = \[2,1,1,1,3,4,1\], k = 2
**Output:** \[2,3\]
**Explanation:** There are two good indices in the array:
- Index 2. The subarray \[2,1\] is in non-increasing order, and the subarray \[1,3\] is in non-decreasing order.
- Index 3. The subarray \[1,1\] is in non-increasing order, and the subarray \[3,4\] is in non-decreasing order.
Note that the index 4 is not good because \[4,1\] is not non-decreasing.
**Example 2:**
**Input:** nums = \[2,1,1,2\], k = 2
**Output:** \[\]
**Explanation:** There are no good indices in this array.
**Constraints:**
* `n == nums.length`
* `3 <= n <= 105`
* `1 <= nums[i] <= 106`
* `1 <= k <= n / 2` | null |
「Python3」🧼Clean "Two-Sum" Style | find-all-good-indices | 0 | 1 | # Approach\n<!-- Describe your approach to solving the problem. -->\nSimilar to using a $$Hashmap$$ in to store "target num & current num", we store all the length "target INC index & current DEC index".\n\n1. store all `k` length INC index, eg. if `k = 2`, and index[0:1] is INC, store {3: 0} in $$Hashmap$$, because you would need 2 as valley, and DEC index starting at 3\n2. find all `k` length DEC index, eg. if index[3:4] is DEC, see if 3 is in $$Hashmap$$, if so, append index 2 to `ans[]`\n\n# Complexity\n- Time complexity:\n- \u2705\u231B Time complexity: $$O(N)$$\u231B --> When k is small, you go through the `nums` list twice.\n\n\n# Code\n```\nclass Solution:\n def goodIndices(self, nums: List[int], k: int) -> List[int]:\n if k == 1: \n return list(range(1,len(nums)-1))\n\n decMap = {}\n decCurrInd = 0\n incCurrInd = k+1\n ans = []\n \n <!-- Save hashmap[target INC index] = DEC index -->\n for i in range(1, len(nums) - k - 1):\n if nums[i] > nums[i-1]:\n decCurrInd = i\n if i - decCurrInd + 1== k:\n decMap[decCurrInd+k+1] = decCurrInd\n decCurrInd += 1\n\n <!-- Check if [target INC index] in the hashmap -->\n for i in range(k+2,len(nums)):\n if nums[i] < nums[i-1]:\n incCurrInd = i\n if i - incCurrInd + 1 == k:\n <!-- Append the "valley index" to ans -->\n if incCurrInd in decMap:\n ans.append(incCurrInd - 1)\n incCurrInd += 1\n\n return ans\n\n``` | 1 | You are given a **0-indexed** integer array `nums` of size `n` and a positive integer `k`.
We call an index `i` in the range `k <= i < n - k` **good** if the following conditions are satisfied:
* The `k` elements that are just **before** the index `i` are in **non-increasing** order.
* The `k` elements that are just **after** the index `i` are in **non-decreasing** order.
Return _an array of all good indices sorted in **increasing** order_.
**Example 1:**
**Input:** nums = \[2,1,1,1,3,4,1\], k = 2
**Output:** \[2,3\]
**Explanation:** There are two good indices in the array:
- Index 2. The subarray \[2,1\] is in non-increasing order, and the subarray \[1,3\] is in non-decreasing order.
- Index 3. The subarray \[1,1\] is in non-increasing order, and the subarray \[3,4\] is in non-decreasing order.
Note that the index 4 is not good because \[4,1\] is not non-decreasing.
**Example 2:**
**Input:** nums = \[2,1,1,2\], k = 2
**Output:** \[\]
**Explanation:** There are no good indices in this array.
**Constraints:**
* `n == nums.length`
* `3 <= n <= 105`
* `1 <= nums[i] <= 106`
* `1 <= k <= n / 2` | null |
Three Pass Python solution - Easy to Understand | find-all-good-indices | 0 | 1 | # Intuition\n<!-- Describe your first thoughts on how to solve this problem. -->\n\n# Approach\n<!-- Describe your approach to solving the problem. -->\n\n# Complexity\n- Time complexity:\n<!-- Add your time complexity here, e.g. $$O(n)$$ -->\n\n- Space complexity:\n<!-- Add your space complexity here, e.g. $$O(n)$$ -->\n\n# Code\n```\nclass Solution:\n def goodIndices(self, nums: List[int], k: int) -> List[int]:\n leftIndices=[]\n rightIndices=collections.deque()\n ref = float(\'inf\')\n init=0\n for i in range(0,len(nums)):\n if nums[i]<=ref:\n leftIndices.append(init)\n else:\n init=i\n leftIndices.append(init)\n ref=nums[i]\n\n ref = -float(\'inf\')\n init=len(nums)-1\n #print(leftIndices)\n for i in range(len(nums)-1,-1,-1):\n\n if nums[i]>ref:\n init=i\n rightIndices.appendleft(init)\n else:\n rightIndices.appendleft(init)\n ref = nums[i]\n #print(rightIndices)\n ans=[]\n for i in range(0+k,len(nums)-k):\n if leftIndices[i-1]<=i-k and rightIndices[i+1]>=i+k:\n ans.append(i)\n\n\n return ans\n\n\n\n\n\n \n``` | 1 | You are given a **0-indexed** integer array `nums` of size `n` and a positive integer `k`.
We call an index `i` in the range `k <= i < n - k` **good** if the following conditions are satisfied:
* The `k` elements that are just **before** the index `i` are in **non-increasing** order.
* The `k` elements that are just **after** the index `i` are in **non-decreasing** order.
Return _an array of all good indices sorted in **increasing** order_.
**Example 1:**
**Input:** nums = \[2,1,1,1,3,4,1\], k = 2
**Output:** \[2,3\]
**Explanation:** There are two good indices in the array:
- Index 2. The subarray \[2,1\] is in non-increasing order, and the subarray \[1,3\] is in non-decreasing order.
- Index 3. The subarray \[1,1\] is in non-increasing order, and the subarray \[3,4\] is in non-decreasing order.
Note that the index 4 is not good because \[4,1\] is not non-decreasing.
**Example 2:**
**Input:** nums = \[2,1,1,2\], k = 2
**Output:** \[\]
**Explanation:** There are no good indices in this array.
**Constraints:**
* `n == nums.length`
* `3 <= n <= 105`
* `1 <= nums[i] <= 106`
* `1 <= k <= n / 2` | null |
Simple Python Solution | find-all-good-indices | 0 | 1 | # Intuition\n<!-- Describe your first thoughts on how to solve this problem. -->\n\n# Approach\n<!-- Describe your approach to solving the problem. -->\n\n# Complexity\n- Time complexity:\n<!-- Add your time complexity here, e.g. $$O(n)$$ -->\n\n- Space complexity:\n<!-- Add your space complexity here, e.g. $$O(n)$$ -->\n\n# Code\n```\nclass Solution:\n def goodIndices(self, nums: List[int], k: int) -> List[int]:\n n = len(nums)\n stack = []\n decre = [False] * n\n incre = [False] * n\n ans = []\n for i in range(n):\n if len(stack) >= k:\n decre[i] = True \n\n if not stack:\n stack.append(nums[i])\n\n else:\n if nums[i] <= stack[-1]:\n stack.append(nums[i])\n else:\n stack = [nums[i]]\n\n stack = []\n for i in range(n-1,-1,-1):\n if len(stack) >= k and decre[i]:\n ans.append(i)\n\n if not stack:\n stack.append(nums[i])\n\n else:\n if nums[i] <= stack[-1]:\n stack.append(nums[i])\n else:\n stack = [nums[i]]\n return ans[::-1] \n\n\n``` | 1 | You are given a **0-indexed** integer array `nums` of size `n` and a positive integer `k`.
We call an index `i` in the range `k <= i < n - k` **good** if the following conditions are satisfied:
* The `k` elements that are just **before** the index `i` are in **non-increasing** order.
* The `k` elements that are just **after** the index `i` are in **non-decreasing** order.
Return _an array of all good indices sorted in **increasing** order_.
**Example 1:**
**Input:** nums = \[2,1,1,1,3,4,1\], k = 2
**Output:** \[2,3\]
**Explanation:** There are two good indices in the array:
- Index 2. The subarray \[2,1\] is in non-increasing order, and the subarray \[1,3\] is in non-decreasing order.
- Index 3. The subarray \[1,1\] is in non-increasing order, and the subarray \[3,4\] is in non-decreasing order.
Note that the index 4 is not good because \[4,1\] is not non-decreasing.
**Example 2:**
**Input:** nums = \[2,1,1,2\], k = 2
**Output:** \[\]
**Explanation:** There are no good indices in this array.
**Constraints:**
* `n == nums.length`
* `3 <= n <= 105`
* `1 <= nums[i] <= 106`
* `1 <= k <= n / 2` | null |
[Python3] Two passes O(n) with line by line comments. | find-all-good-indices | 0 | 1 | To solve the problem, we will need to traverse the array left to right and then right to left. From left to right, at each index i, we will compute how many elements that are just before the index i are in non-increasing order. To do this, we will use a non-increasing stack to store the elements before i, at each step we just check if the length of the stack is greater or equal to k and if it is, this index i is True for the forward pass. Doing the simillar thing from right to left, this time, we will check if the length of the stack is greater or equal to k and also if the forward[i] is Ture, if it is, add this index to the result. If you are doing left to right and then right to left like I did, you will need to return result in the reversed order.\n\n```\nclass Solution:\n def goodIndices(self, nums: List[int], k: int) -> List[int]:\n ### forward pass.\n forward = [False]*len(nums) ### For the forward pass, store if index i is good or not.\n stack = []\n for i in range(len(nums)):\n \t### if the leangth of stack is greater or equal to k, it means this index is good.\n if len(stack)>=k:\n forward[i] = True\n ### if the stack is empty, just add the current number to it.\n if not stack:\n stack.append(nums[i])\n ### check to see if the current number is smaller or equal to the last number in stack, if it is not, put this number into the stack.\n else:\n if nums[i]<=stack[-1]:\n stack.append(nums[i])\n else:\n stack = [nums[i]]\n ### backward pass\n res = []\n stack = []\n for i in reversed(range(len(nums))):\n \t### Check to see if the length of stack is greater or equal to k and also check if the forward pass at this index is Ture.\n if len(stack)>=k and forward[i]:\n res.append(i)\n if not stack:\n stack.append(nums[i])\n else:\n if nums[i]<=stack[-1]:\n stack.append(nums[i])\n else:\n stack = [nums[i]]\n return res[::-1]\n```\n\n**Upvote** if you like this post.\n\n**Connect with me on [LinkedIn](https://www.linkedin.com/in/meida-chen-938a265b/)** if you\'d like to discuss other related topics\n\nJust in case if you are working on **ML/DL 3D data-related projects** or are interested in the topic, please check out our project **[HERE](https://github.com/meidachen/STPLS3D)** | 35 | You are given a **0-indexed** integer array `nums` of size `n` and a positive integer `k`.
We call an index `i` in the range `k <= i < n - k` **good** if the following conditions are satisfied:
* The `k` elements that are just **before** the index `i` are in **non-increasing** order.
* The `k` elements that are just **after** the index `i` are in **non-decreasing** order.
Return _an array of all good indices sorted in **increasing** order_.
**Example 1:**
**Input:** nums = \[2,1,1,1,3,4,1\], k = 2
**Output:** \[2,3\]
**Explanation:** There are two good indices in the array:
- Index 2. The subarray \[2,1\] is in non-increasing order, and the subarray \[1,3\] is in non-decreasing order.
- Index 3. The subarray \[1,1\] is in non-increasing order, and the subarray \[3,4\] is in non-decreasing order.
Note that the index 4 is not good because \[4,1\] is not non-decreasing.
**Example 2:**
**Input:** nums = \[2,1,1,2\], k = 2
**Output:** \[\]
**Explanation:** There are no good indices in this array.
**Constraints:**
* `n == nums.length`
* `3 <= n <= 105`
* `1 <= nums[i] <= 106`
* `1 <= k <= n / 2` | null |
Video Explanation | Easiest Solution using Python | number-of-good-paths | 0 | 1 | # Approach\nhttps://youtu.be/EIoHwUL5Efk\n\n# Code\n```\nclass UnionFind:\n def __init__(self, size):\n self.parent = list(range(size))\n self.rank = [0] * size\n\n def find(self, x):\n if self.parent[x] != x:\n self.parent[x] = self.find(self.parent[x])\n return self.parent[x]\n\n def union_set(self, x, y):\n xset = self.find(x)\n yset = self.find(y)\n if xset == yset:\n return\n elif self.rank[xset] < self.rank[yset]:\n self.parent[xset] = yset\n elif self.rank[xset] > self.rank[yset]:\n self.parent[yset] = xset\n else:\n self.parent[yset] = xset\n self.rank[xset] += 1\n\nclass Solution:\n def numberOfGoodPaths(self, vals: List[int], edges: List[List[int]]) -> int:\n n = len(vals)\n adj = [[] for _ in range(n)]\n\n for edge in edges:\n adj[edge[0]].append(edge[1])\n adj[edge[1]].append(edge[0])\n\n values_to_nodes = {}\n for i, value in enumerate(vals):\n values_to_nodes.setdefault(value, []).append(i)\n\n\n dsu = UnionFind(n)\n good_paths = 0\n\n for value, nodes in sorted(values_to_nodes.items()):\n for node in nodes:\n for neig in adj[node]:\n if vals[node] >= vals[neig]:\n dsu.union_set(node, neig)\n\n\n group = {}\n for node in nodes:\n group[dsu.find(node)] = group.get(dsu.find(node), 0) + 1\n\n for size in group.values():\n good_paths += (size * (size + 1)) //2\n\n\n return good_paths\n\n\n\n\n\n\n\n\n\n\n\n\n\n\n\n``` | 1 | There is a tree (i.e. a connected, undirected graph with no cycles) consisting of `n` nodes numbered from `0` to `n - 1` and exactly `n - 1` edges.
You are given a **0-indexed** integer array `vals` of length `n` where `vals[i]` denotes the value of the `ith` node. You are also given a 2D integer array `edges` where `edges[i] = [ai, bi]` denotes that there exists an **undirected** edge connecting nodes `ai` and `bi`.
A **good path** is a simple path that satisfies the following conditions:
1. The starting node and the ending node have the **same** value.
2. All nodes between the starting node and the ending node have values **less than or equal to** the starting node (i.e. the starting node's value should be the maximum value along the path).
Return _the number of distinct good paths_.
Note that a path and its reverse are counted as the **same** path. For example, `0 -> 1` is considered to be the same as `1 -> 0`. A single node is also considered as a valid path.
**Example 1:**
**Input:** vals = \[1,3,2,1,3\], edges = \[\[0,1\],\[0,2\],\[2,3\],\[2,4\]\]
**Output:** 6
**Explanation:** There are 5 good paths consisting of a single node.
There is 1 additional good path: 1 -> 0 -> 2 -> 4.
(The reverse path 4 -> 2 -> 0 -> 1 is treated as the same as 1 -> 0 -> 2 -> 4.)
Note that 0 -> 2 -> 3 is not a good path because vals\[2\] > vals\[0\].
**Example 2:**
**Input:** vals = \[1,1,2,2,3\], edges = \[\[0,1\],\[1,2\],\[2,3\],\[2,4\]\]
**Output:** 7
**Explanation:** There are 5 good paths consisting of a single node.
There are 2 additional good paths: 0 -> 1 and 2 -> 3.
**Example 3:**
**Input:** vals = \[1\], edges = \[\]
**Output:** 1
**Explanation:** The tree consists of only one node, so there is one good path.
**Constraints:**
* `n == vals.length`
* `1 <= n <= 3 * 104`
* `0 <= vals[i] <= 105`
* `edges.length == n - 1`
* `edges[i].length == 2`
* `0 <= ai, bi < n`
* `ai != bi`
* `edges` represents a valid tree. | null |
Python3 🐍 concise solution beats 96.9% | number-of-good-paths | 0 | 1 | # Code\n```\nclass Solution:\n def numberOfGoodPaths(self, vals: List[int], edges: List[List[int]]) -> int:\n n = len(vals)\n p = list(range(n))\n count = [Counter({vals[i]:1}) for i in range(n)]\n edges = sorted((max(vals[i],vals[j]),i,j) for i,j in edges)\n res = n\n def find(i):\n if p[i] != i:p[i] = find(p[i])\n return p[i]\n for val, i, j in edges:\n pi, pj = find(i), find(j)\n res += count[pi][val]*count[pj][val]\n p[pi] = pj\n count[pj][val] += count[pi][val]\n return res\n``` | 5 | There is a tree (i.e. a connected, undirected graph with no cycles) consisting of `n` nodes numbered from `0` to `n - 1` and exactly `n - 1` edges.
You are given a **0-indexed** integer array `vals` of length `n` where `vals[i]` denotes the value of the `ith` node. You are also given a 2D integer array `edges` where `edges[i] = [ai, bi]` denotes that there exists an **undirected** edge connecting nodes `ai` and `bi`.
A **good path** is a simple path that satisfies the following conditions:
1. The starting node and the ending node have the **same** value.
2. All nodes between the starting node and the ending node have values **less than or equal to** the starting node (i.e. the starting node's value should be the maximum value along the path).
Return _the number of distinct good paths_.
Note that a path and its reverse are counted as the **same** path. For example, `0 -> 1` is considered to be the same as `1 -> 0`. A single node is also considered as a valid path.
**Example 1:**
**Input:** vals = \[1,3,2,1,3\], edges = \[\[0,1\],\[0,2\],\[2,3\],\[2,4\]\]
**Output:** 6
**Explanation:** There are 5 good paths consisting of a single node.
There is 1 additional good path: 1 -> 0 -> 2 -> 4.
(The reverse path 4 -> 2 -> 0 -> 1 is treated as the same as 1 -> 0 -> 2 -> 4.)
Note that 0 -> 2 -> 3 is not a good path because vals\[2\] > vals\[0\].
**Example 2:**
**Input:** vals = \[1,1,2,2,3\], edges = \[\[0,1\],\[1,2\],\[2,3\],\[2,4\]\]
**Output:** 7
**Explanation:** There are 5 good paths consisting of a single node.
There are 2 additional good paths: 0 -> 1 and 2 -> 3.
**Example 3:**
**Input:** vals = \[1\], edges = \[\]
**Output:** 1
**Explanation:** The tree consists of only one node, so there is one good path.
**Constraints:**
* `n == vals.length`
* `1 <= n <= 3 * 104`
* `0 <= vals[i] <= 105`
* `edges.length == n - 1`
* `edges[i].length == 2`
* `0 <= ai, bi < n`
* `ai != bi`
* `edges` represents a valid tree. | null |
Python O(n) Solution using Nested `Counter()` (Detailed Comments) | remove-letter-to-equalize-frequency | 0 | 1 | ```\nclass Solution:\n def equalFrequency(self, word: str) -> bool:\n word_counter = Counter(word)\n # All characters in `word` are the same character, or all characters are different (each only occur once)\n if len(word_counter) == 1 or len(word_counter) == len(word):\n return True\n frequency_counter = Counter(word_counter.values())\n # There are more than 2 type of counts\n if len(frequency_counter) != 2:\n return False\n max_count = max(frequency_counter.keys())\n min_count = min(frequency_counter.keys())\n # 1st Case: The character with different count than others only occur once\n # 2nd Case: The character with different count occur one more time than others\n return min_count == 1 and frequency_counter[min_count] == 1 or max_count - min_count == 1 and frequency_counter[max_count] == 1\n``` | 1 | You are given a **0-indexed** string `word`, consisting of lowercase English letters. You need to select **one** index and **remove** the letter at that index from `word` so that the **frequency** of every letter present in `word` is equal.
Return `true` _if it is possible to remove one letter so that the frequency of all letters in_ `word` _are equal, and_ `false` _otherwise_.
**Note:**
* The **frequency** of a letter `x` is the number of times it occurs in the string.
* You **must** remove exactly one letter and cannot chose to do nothing.
**Example 1:**
**Input:** word = "abcc "
**Output:** true
**Explanation:** Select index 3 and delete it: word becomes "abc " and each character has a frequency of 1.
**Example 2:**
**Input:** word = "aazz "
**Output:** false
**Explanation:** We must delete a character, so either the frequency of "a " is 1 and the frequency of "z " is 2, or vice versa. It is impossible to make all present letters have equal frequency.
**Constraints:**
* `2 <= word.length <= 100`
* `word` consists of lowercase English letters only. | null |
[Python3] One-Pass Solution O(n), Clean & Concise | longest-uploaded-prefix | 0 | 1 | ```\nclass LUPrefix:\n\n def __init__(self, n: int):\n self.videos = [False] * (n + 1)\n self.ans = 0\n\n def upload(self, video: int) -> None:\n self.videos[video] = True\n if video == self.ans + 1:\n while video < len(self.videos):\n if not self.videos[video]:\n break\n self.ans += 1\n video += 1\n\n def longest(self) -> int:\n return self.ans\n``` | 2 | You are given a stream of `n` videos, each represented by a **distinct** number from `1` to `n` that you need to "upload " to a server. You need to implement a data structure that calculates the length of the **longest uploaded prefix** at various points in the upload process.
We consider `i` to be an uploaded prefix if all videos in the range `1` to `i` (**inclusive**) have been uploaded to the server. The longest uploaded prefix is the **maximum** value of `i` that satisfies this definition.
Implement the `LUPrefix` class:
* `LUPrefix(int n)` Initializes the object for a stream of `n` videos.
* `void upload(int video)` Uploads `video` to the server.
* `int longest()` Returns the length of the **longest uploaded prefix** defined above.
**Example 1:**
**Input**
\[ "LUPrefix ", "upload ", "longest ", "upload ", "longest ", "upload ", "longest "\]
\[\[4\], \[3\], \[\], \[1\], \[\], \[2\], \[\]\]
**Output**
\[null, null, 0, null, 1, null, 3\]
**Explanation**
LUPrefix server = new LUPrefix(4); // Initialize a stream of 4 videos.
server.upload(3); // Upload video 3.
server.longest(); // Since video 1 has not been uploaded yet, there is no prefix.
// So, we return 0.
server.upload(1); // Upload video 1.
server.longest(); // The prefix \[1\] is the longest uploaded prefix, so we return 1.
server.upload(2); // Upload video 2.
server.longest(); // The prefix \[1,2,3\] is the longest uploaded prefix, so we return 3.
**Constraints:**
* `1 <= n <= 105`
* `1 <= video <= n`
* All values of `video` are **distinct**.
* At most `2 * 105` calls **in total** will be made to `upload` and `longest`.
* At least one call will be made to `longest`. | null |
Python Elegant & Short | Amortized O(1) | Commented | longest-uploaded-prefix | 0 | 1 | ```\nclass LUPrefix:\n """\n Memory: O(n)\n Time: O(1) per upload call, because adding to the set takes O(1) time, and the prefix\n\t\t\t\t can be increased no more than n times for all n calls to the upload function\n """\n\n def __init__(self, n: int):\n self._longest = 0\n self._nums = set()\n\n def upload(self, video: int) -> None:\n self._nums.add(video)\n # Since the prefix cannot decrease, it is enough for us to increase it\n # until we reach the number that has not yet been added\n while self._longest + 1 in self._nums:\n self._longest += 1\n\n def longest(self) -> int:\n return self._longest\n```\n\nIf you like this solution remember to **upvote it** to let me know.\n | 68 | You are given a stream of `n` videos, each represented by a **distinct** number from `1` to `n` that you need to "upload " to a server. You need to implement a data structure that calculates the length of the **longest uploaded prefix** at various points in the upload process.
We consider `i` to be an uploaded prefix if all videos in the range `1` to `i` (**inclusive**) have been uploaded to the server. The longest uploaded prefix is the **maximum** value of `i` that satisfies this definition.
Implement the `LUPrefix` class:
* `LUPrefix(int n)` Initializes the object for a stream of `n` videos.
* `void upload(int video)` Uploads `video` to the server.
* `int longest()` Returns the length of the **longest uploaded prefix** defined above.
**Example 1:**
**Input**
\[ "LUPrefix ", "upload ", "longest ", "upload ", "longest ", "upload ", "longest "\]
\[\[4\], \[3\], \[\], \[1\], \[\], \[2\], \[\]\]
**Output**
\[null, null, 0, null, 1, null, 3\]
**Explanation**
LUPrefix server = new LUPrefix(4); // Initialize a stream of 4 videos.
server.upload(3); // Upload video 3.
server.longest(); // Since video 1 has not been uploaded yet, there is no prefix.
// So, we return 0.
server.upload(1); // Upload video 1.
server.longest(); // The prefix \[1\] is the longest uploaded prefix, so we return 1.
server.upload(2); // Upload video 2.
server.longest(); // The prefix \[1,2,3\] is the longest uploaded prefix, so we return 3.
**Constraints:**
* `1 <= n <= 105`
* `1 <= video <= n`
* All values of `video` are **distinct**.
* At most `2 * 105` calls **in total** will be made to `upload` and `longest`.
* At least one call will be made to `longest`. | null |
Easy and optimized python3 solution | longest-uploaded-prefix | 0 | 1 | class LUPrefix:\n\n def __init__(self, n: int):\n \n self.v = [0]*(n+1)\n self.cur = 1\n \n\n def upload(self, video: int) -> None:\n self.v[video] = 1\n if video == self.cur:\n self.cur+=1\n \n while self.cur<len(self.v) and self.v[self.cur]!=0:\n self.cur+=1\n\n\n def longest(self) -> int:\n return self.cur-1 | 1 | You are given a stream of `n` videos, each represented by a **distinct** number from `1` to `n` that you need to "upload " to a server. You need to implement a data structure that calculates the length of the **longest uploaded prefix** at various points in the upload process.
We consider `i` to be an uploaded prefix if all videos in the range `1` to `i` (**inclusive**) have been uploaded to the server. The longest uploaded prefix is the **maximum** value of `i` that satisfies this definition.
Implement the `LUPrefix` class:
* `LUPrefix(int n)` Initializes the object for a stream of `n` videos.
* `void upload(int video)` Uploads `video` to the server.
* `int longest()` Returns the length of the **longest uploaded prefix** defined above.
**Example 1:**
**Input**
\[ "LUPrefix ", "upload ", "longest ", "upload ", "longest ", "upload ", "longest "\]
\[\[4\], \[3\], \[\], \[1\], \[\], \[2\], \[\]\]
**Output**
\[null, null, 0, null, 1, null, 3\]
**Explanation**
LUPrefix server = new LUPrefix(4); // Initialize a stream of 4 videos.
server.upload(3); // Upload video 3.
server.longest(); // Since video 1 has not been uploaded yet, there is no prefix.
// So, we return 0.
server.upload(1); // Upload video 1.
server.longest(); // The prefix \[1\] is the longest uploaded prefix, so we return 1.
server.upload(2); // Upload video 2.
server.longest(); // The prefix \[1,2,3\] is the longest uploaded prefix, so we return 3.
**Constraints:**
* `1 <= n <= 105`
* `1 <= video <= n`
* All values of `video` are **distinct**.
* At most `2 * 105` calls **in total** will be made to `upload` and `longest`.
* At least one call will be made to `longest`. | null |
O(nlogn) using merging lower upper bound intervals | longest-uploaded-prefix | 0 | 1 | **Main Idea**:\n+ Managing lower/upper bound intervals\n+ Uploading method:\n + vmin, vmax = video, video\n + Find upper bound interval: video - 1 -> found: vmin = min(interval), delete lower/upper bound intervals corresponding\n + Find lower bound interval: video + 1 -> found: vmax = max(interval), delete lower/upper bound intervals corresponding\n + Updating lower/upper bound intervals\n+ Longest method: max(upper bound(1) \n\n**Examples**:\n```\n+ Current Status:\nlower bound intervals = {1: [1,3], 5: [5,8]}\nupper bound intervals: {3:[1,3], 8:[5,8]}\n\n+ Upload(4):\nvideo 4 -> vmin=4, vmax = 4\n\nvideo-1 = 3 => found upper bound 3: [1,3]\n-> vmin = 1, upper: {8:[5,8]}, lower: {5: [5,8]}\n\nvideo+1 = 5 => found lower bound 5: [5,8]\n-> vmax = 8, upper: {}, lower: {}\n\nUpdating: lower: {1: [1,8]}, upper: {8: [1,8]}\n\n+ Longest: lower[1][1] -> 8\n```\n\n**Code**:\n```python\nclass LUPrefix:\n def __init__(self, n: int):\n self.check = [0] * (n+1)\n self.n = n\n self.hmin = {}\n self.hmax = {}\n\n def upload(self, video: int) -> None:\n if video>=1 and video<=self.n and self.check[video]==0:\n q1 = self.hmax.get(video-1)\n q2 = self.hmin.get(video+1)\n vmin, vmax = [video, video]\n if q1 is not None and q2 is not None:\n vmin, vmax = q1[0], q2[1]\n del self.hmax[video-1]\n del self.hmin[video+1]\n elif q1 is not None:\n vmin, vmax = q1[0], video\n del self.hmax[q1[1]]\n elif q2 is not None:\n vmin, vmax = video, q2[1]\n del self.hmin[q2[0]]\n self.hmax[vmax] = [vmin, vmax]\n self.hmin[vmin] = [vmin, vmax] \n self.check[video] = 1\n \n # print(q1, q2, self.check, self.hmin, self.hmax)\n \n def longest(self) -> int:\n if self.hmin.get(1) is None:\n return 0\n else:\n return self.hmin[1][1]\n\n\n# Your LUPrefix object will be instantiated and called as such:\n# obj = LUPrefix(n)\n# obj.upload(video)\n# param_2 = obj.longest()\n``` | 1 | You are given a stream of `n` videos, each represented by a **distinct** number from `1` to `n` that you need to "upload " to a server. You need to implement a data structure that calculates the length of the **longest uploaded prefix** at various points in the upload process.
We consider `i` to be an uploaded prefix if all videos in the range `1` to `i` (**inclusive**) have been uploaded to the server. The longest uploaded prefix is the **maximum** value of `i` that satisfies this definition.
Implement the `LUPrefix` class:
* `LUPrefix(int n)` Initializes the object for a stream of `n` videos.
* `void upload(int video)` Uploads `video` to the server.
* `int longest()` Returns the length of the **longest uploaded prefix** defined above.
**Example 1:**
**Input**
\[ "LUPrefix ", "upload ", "longest ", "upload ", "longest ", "upload ", "longest "\]
\[\[4\], \[3\], \[\], \[1\], \[\], \[2\], \[\]\]
**Output**
\[null, null, 0, null, 1, null, 3\]
**Explanation**
LUPrefix server = new LUPrefix(4); // Initialize a stream of 4 videos.
server.upload(3); // Upload video 3.
server.longest(); // Since video 1 has not been uploaded yet, there is no prefix.
// So, we return 0.
server.upload(1); // Upload video 1.
server.longest(); // The prefix \[1\] is the longest uploaded prefix, so we return 1.
server.upload(2); // Upload video 2.
server.longest(); // The prefix \[1,2,3\] is the longest uploaded prefix, so we return 3.
**Constraints:**
* `1 <= n <= 105`
* `1 <= video <= n`
* All values of `video` are **distinct**.
* At most `2 * 105` calls **in total** will be made to `upload` and `longest`.
* At least one call will be made to `longest`. | null |
Python Elegant & Short | O(n + m) | bitwise-xor-of-all-pairings | 0 | 1 | ```\nfrom functools import reduce\nfrom operator import xor\n\n\nclass Solution:\n def xorAllNums(self, a: List[int], b: List[int]) -> int:\n return reduce(xor, a * (len(b) & 1) + b * (len(a) & 1), 0)\n```\n\nIf you like this solution remember to **upvote it** to let me know. | 3 | You are given two **0-indexed** arrays, `nums1` and `nums2`, consisting of non-negative integers. There exists another array, `nums3`, which contains the bitwise XOR of **all pairings** of integers between `nums1` and `nums2` (every integer in `nums1` is paired with every integer in `nums2` **exactly once**).
Return _the **bitwise XOR** of all integers in_ `nums3`.
**Example 1:**
**Input:** nums1 = \[2,1,3\], nums2 = \[10,2,5,0\]
**Output:** 13
**Explanation:**
A possible nums3 array is \[8,0,7,2,11,3,4,1,9,1,6,3\].
The bitwise XOR of all these numbers is 13, so we return 13.
**Example 2:**
**Input:** nums1 = \[1,2\], nums2 = \[3,4\]
**Output:** 0
**Explanation:**
All possible pairs of bitwise XORs are nums1\[0\] ^ nums2\[0\], nums1\[0\] ^ nums2\[1\], nums1\[1\] ^ nums2\[0\],
and nums1\[1\] ^ nums2\[1\].
Thus, one possible nums3 array is \[2,5,1,6\].
2 ^ 5 ^ 1 ^ 6 = 0, so we return 0.
**Constraints:**
* `1 <= nums1.length, nums2.length <= 105`
* `0 <= nums1[i], nums2[j] <= 109` | null |
[python3] math sol for reference | bitwise-xor-of-all-pairings | 0 | 1 | # Intuition\nXOR follows identity, associative and commutative rules. \n\n# Approach\n[a,b] [c,d,e] results in the following.\na^c ^ a^d ^ a^e ^ b^c ^ b^d b^e\n\nDepending on the even or odd number of the second array, number of \'a\' can be decided. \n\na^a = 0\na^a^a = a \n\nThe same rule can be applied reverse from second to first array. \n\n# Complexity\n- Time complexity: O(N)\n- Space complexity: O(1)\n<!-- Add your space complexity here, e.g. $$O(n)$$ -->\n\n# Code\n```\nclass Solution:\n def xorAllNums(self, nums1: List[int], nums2: List[int]) -> int:\n n1 = len(nums1)%2\n n2 = len(nums2)%2\n\n n1xor = 0 \n n2xor = 0 \n \n if n2 == 1:\n for n in nums1:\n n1xor ^= n\n \n if n1 == 1:\n for n in nums2:\n n2xor ^= n \n\n return n1xor ^ n2xor\n \n \n``` | 1 | You are given two **0-indexed** arrays, `nums1` and `nums2`, consisting of non-negative integers. There exists another array, `nums3`, which contains the bitwise XOR of **all pairings** of integers between `nums1` and `nums2` (every integer in `nums1` is paired with every integer in `nums2` **exactly once**).
Return _the **bitwise XOR** of all integers in_ `nums3`.
**Example 1:**
**Input:** nums1 = \[2,1,3\], nums2 = \[10,2,5,0\]
**Output:** 13
**Explanation:**
A possible nums3 array is \[8,0,7,2,11,3,4,1,9,1,6,3\].
The bitwise XOR of all these numbers is 13, so we return 13.
**Example 2:**
**Input:** nums1 = \[1,2\], nums2 = \[3,4\]
**Output:** 0
**Explanation:**
All possible pairs of bitwise XORs are nums1\[0\] ^ nums2\[0\], nums1\[0\] ^ nums2\[1\], nums1\[1\] ^ nums2\[0\],
and nums1\[1\] ^ nums2\[1\].
Thus, one possible nums3 array is \[2,5,1,6\].
2 ^ 5 ^ 1 ^ 6 = 0, so we return 0.
**Constraints:**
* `1 <= nums1.length, nums2.length <= 105`
* `0 <= nums1[i], nums2[j] <= 109` | null |
O(n) using checking odd and even attributes of length array (Examples) | bitwise-xor-of-all-pairings | 0 | 1 | ***Main Idea***:\n+ XOR attribute: a ^ a = 0, a ^ a ^ a = a\n+ Counting odd and even attributes of the number of occuring every elements in nums1 and nums2\n + nums1[i] occurs n2 times \n + nums2[i] occurs n1 times\n+ Calculating ans as follow:\n + ans1 <- xor(all nums1[i]) if n2%1==1 or 0\n + ans2 <- xor(all nums2[i]) if n1%1==1 or 0 \n + ans <- ans1 xor ans2\n\n**Examples**:\n```\nExampe1: nums1 = [2,1,3], nums2 = [10,2,5,0]\nn2 = 4: ans1 = 0\nn1 = 3: ans2 = 10 ^ 2 ^ 5 ^ 0 = 13\nans = ans1 ^ ans2 = 0 ^ 13 = 13\n\nExample 2: nums1 = [1,2], nums2 = [3,4]\nn2 = 2: ans1 = 0\nn1 = 2: ans2 = 0\nans = ans1 ^ ans2 = 0 ^ 0 = 0\n```\n\n**Code**:\n```\nclass Solution:\n def xorAllNums(self, nums1: List[int], nums2: List[int]) -> int:\n# nums3 = []\n# for n1 in nums1:\n# for n2 in nums2:\n# nums3.append(n1 ^ n2)\n \n# ans1 = 0\n# for i in range(len(nums3)):\n# ans1 = ans1 ^ nums3[i]\n \n n1, n2 = len(nums1), len(nums2)\n ans = 0\n if n2%2==1:\n for i in range(n1):\n ans = ans ^ nums1[i]\n \n if n1%2==1:\n for i in range(n2):\n ans = ans ^ nums2[i]\n \n # print(ans1, ans)\n return ans\n``` | 1 | You are given two **0-indexed** arrays, `nums1` and `nums2`, consisting of non-negative integers. There exists another array, `nums3`, which contains the bitwise XOR of **all pairings** of integers between `nums1` and `nums2` (every integer in `nums1` is paired with every integer in `nums2` **exactly once**).
Return _the **bitwise XOR** of all integers in_ `nums3`.
**Example 1:**
**Input:** nums1 = \[2,1,3\], nums2 = \[10,2,5,0\]
**Output:** 13
**Explanation:**
A possible nums3 array is \[8,0,7,2,11,3,4,1,9,1,6,3\].
The bitwise XOR of all these numbers is 13, so we return 13.
**Example 2:**
**Input:** nums1 = \[1,2\], nums2 = \[3,4\]
**Output:** 0
**Explanation:**
All possible pairs of bitwise XORs are nums1\[0\] ^ nums2\[0\], nums1\[0\] ^ nums2\[1\], nums1\[1\] ^ nums2\[0\],
and nums1\[1\] ^ nums2\[1\].
Thus, one possible nums3 array is \[2,5,1,6\].
2 ^ 5 ^ 1 ^ 6 = 0, so we return 0.
**Constraints:**
* `1 <= nums1.length, nums2.length <= 105`
* `0 <= nums1[i], nums2[j] <= 109` | null |
Python3 + In place MergeSort + Explanation | number-of-pairs-satisfying-inequality | 0 | 1 | \n\n# Code\n```\nclass Solution:\n def numberOfPairs(self, nums1: List[int], nums2: List[int], diff: int) -> int:\n # See Merge Sort and 493. Reverse Pairs before this\n # This problem is exactly as 493, but we need to transform the two input\n # arrays to one first\n # nums1[i] - nums1[j] <= nums2[i] - nums2[j] + diff, can be written as\n # nums1[i] - nums2[i] <= nums1[j] - nums2[j]\n # Say nums1 - nums2 = arr\n # then above can be written as \n # a[i] <= a[j] + diff -- exactly like Reverse pairs but with <= instead of >\n # This <= instead of > affect the calculation of count only\n # see c += len(right) - j below, since if left[i] <= right[i] + diff\n # this will be true for all remaing j, since they are greater than this value\n\n def merge_sort(nums):\n if len(nums) <= 1: return 0\n mid = len(nums) // 2\n left = nums[:mid]\n right = nums[mid:]\n\n c_l = merge_sort(left)\n c_r = merge_sort(right)\n\n # This part we count the pairs\n i = j = c = 0\n while i < len(left) and j < len(right):\n if left[i] <= right[j] + diff:\n c += (len(right) - j)\n i += 1\n else:\n j += 1\n \n # here we do the regular merge of merge sort \n i = j = k = 0\n while i < len(left) and j < len(right):\n if right[j] < left[i]:\n nums[k] = right[j]\n j += 1\n else:\n nums[k] = left[i]\n i += 1\n k += 1\n \n while i < len(left):\n nums[k] = left[i]\n i += 1\n k += 1\n \n while j < len(right):\n nums[k] = right[j]\n j += 1\n k += 1\n \n return c_l + c_r + c\n\n diff_arr = [a - b for (a, b) in zip(nums1, nums2)]\n return merge_sort(diff_arr) \n\n``` | 1 | You are given two **0-indexed** integer arrays `nums1` and `nums2`, each of size `n`, and an integer `diff`. Find the number of **pairs** `(i, j)` such that:
* `0 <= i < j <= n - 1` **and**
* `nums1[i] - nums1[j] <= nums2[i] - nums2[j] + diff`.
Return _the **number of pairs** that satisfy the conditions._
**Example 1:**
**Input:** nums1 = \[3,2,5\], nums2 = \[2,2,1\], diff = 1
**Output:** 3
**Explanation:**
There are 3 pairs that satisfy the conditions:
1. i = 0, j = 1: 3 - 2 <= 2 - 2 + 1. Since i < j and 1 <= 1, this pair satisfies the conditions.
2. i = 0, j = 2: 3 - 5 <= 2 - 1 + 1. Since i < j and -2 <= 2, this pair satisfies the conditions.
3. i = 1, j = 2: 2 - 5 <= 2 - 1 + 1. Since i < j and -3 <= 2, this pair satisfies the conditions.
Therefore, we return 3.
**Example 2:**
**Input:** nums1 = \[3,-1\], nums2 = \[-2,2\], diff = -1
**Output:** 0
**Explanation:**
Since there does not exist any pair that satisfies the conditions, we return 0.
**Constraints:**
* `n == nums1.length == nums2.length`
* `2 <= n <= 105`
* `-104 <= nums1[i], nums2[i] <= 104`
* `-104 <= diff <= 104` | null |
Python3 Segment tree Solution | number-of-pairs-satisfying-inequality | 0 | 1 | ```\nclass segment_tree:\n \n def __init__(self, arr):\n self.len_ = len(arr)\n \n self.data_ = [0] * (self.len_ * 2)\n \n for t in range(self.len_, self.len_ * 2, 1):\n self.data_[t] = arr[t - self.len_]\n \n for t in range(self.len_-1, -1, -1): \n self.data_[t] = self.data_[t * 2] + self.data_[t * 2 + 1]\n \n def update(self, index, target):\n self.data_[index + self.len_] = target\n \n start = index + self.len_\n start = start // 2\n \n while start >= 1:\n self.data_[start] = self.data_[start*2] + self.data_[start*2+1]\n start = start // 2\n \n def query(self, left, right):\n left += self.len_\n right += self.len_\n \n res = 0\n \n while left < right:\n if left & 1:\n res += self.data_[left]\n left += 1\n if right & 1:\n right -= 1\n res += self.data_[right]\n left = left // 2\n right = right //2\n \n return res\n\nclass Solution:\n def numberOfPairs(self, nums1: List[int], nums2: List[int], diff: int) -> int:\n \n data = [ x-y for (x,y) in zip(nums1, nums2)]\n \n data2 = [(data[i] + diff, i) for i in range(len(data))]\n \n data2.sort(key = lambda x : x[0])\n \n st = segment_tree([1 for x in range(len(data2))])\n \n lookup = {}\n \n for t in range(len(data2)):\n lookup[data2[t][1]] = t \n \n data3 = [x[0] for x in data2]\n \n res = 0\n \n n = len(data)\n \n for i in range(len(data)):\n element = data[i]\n index = bisect.bisect_left(data3, element)\n index2 = lookup[i]\n st.update(index2, 0)\n if index < n:\n res += st.query(index, n)\n return res\n``` | 1 | You are given two **0-indexed** integer arrays `nums1` and `nums2`, each of size `n`, and an integer `diff`. Find the number of **pairs** `(i, j)` such that:
* `0 <= i < j <= n - 1` **and**
* `nums1[i] - nums1[j] <= nums2[i] - nums2[j] + diff`.
Return _the **number of pairs** that satisfy the conditions._
**Example 1:**
**Input:** nums1 = \[3,2,5\], nums2 = \[2,2,1\], diff = 1
**Output:** 3
**Explanation:**
There are 3 pairs that satisfy the conditions:
1. i = 0, j = 1: 3 - 2 <= 2 - 2 + 1. Since i < j and 1 <= 1, this pair satisfies the conditions.
2. i = 0, j = 2: 3 - 5 <= 2 - 1 + 1. Since i < j and -2 <= 2, this pair satisfies the conditions.
3. i = 1, j = 2: 2 - 5 <= 2 - 1 + 1. Since i < j and -3 <= 2, this pair satisfies the conditions.
Therefore, we return 3.
**Example 2:**
**Input:** nums1 = \[3,-1\], nums2 = \[-2,2\], diff = -1
**Output:** 0
**Explanation:**
Since there does not exist any pair that satisfies the conditions, we return 0.
**Constraints:**
* `n == nums1.length == nums2.length`
* `2 <= n <= 105`
* `-104 <= nums1[i], nums2[i] <= 104`
* `-104 <= diff <= 104` | null |
Clean, Fast Python3 | SortedList | O(n log(n)) | number-of-pairs-satisfying-inequality | 0 | 1 | Please upvote if it helps!\n```\nfrom sortedcontainers import SortedList\nclass Solution:\n def numberOfPairs(self, nums1: List[int], nums2: List[int], diff: int) -> int:\n n, pairs = len(nums1), 0\n # simplify the problem by rearranging equations: find all pairs of i, j in nums such that nums[i] - nums[j] <= diff\n nums = [x1 - x2 for x1, x2 in zip(nums1, nums2)] # condense to one list\n # look back thru sorted list to find number of past i\'s such that nums[i] <= nums[j] + diff\n i_nums = SortedList() \n for j_num in nums:\n pairs += i_nums.bisect_right(j_num + diff)\n i_nums.add(j_num)\n\t\t\t\n return pairs\n | 1 | You are given two **0-indexed** integer arrays `nums1` and `nums2`, each of size `n`, and an integer `diff`. Find the number of **pairs** `(i, j)` such that:
* `0 <= i < j <= n - 1` **and**
* `nums1[i] - nums1[j] <= nums2[i] - nums2[j] + diff`.
Return _the **number of pairs** that satisfy the conditions._
**Example 1:**
**Input:** nums1 = \[3,2,5\], nums2 = \[2,2,1\], diff = 1
**Output:** 3
**Explanation:**
There are 3 pairs that satisfy the conditions:
1. i = 0, j = 1: 3 - 2 <= 2 - 2 + 1. Since i < j and 1 <= 1, this pair satisfies the conditions.
2. i = 0, j = 2: 3 - 5 <= 2 - 1 + 1. Since i < j and -2 <= 2, this pair satisfies the conditions.
3. i = 1, j = 2: 2 - 5 <= 2 - 1 + 1. Since i < j and -3 <= 2, this pair satisfies the conditions.
Therefore, we return 3.
**Example 2:**
**Input:** nums1 = \[3,-1\], nums2 = \[-2,2\], diff = -1
**Output:** 0
**Explanation:**
Since there does not exist any pair that satisfies the conditions, we return 0.
**Constraints:**
* `n == nums1.length == nums2.length`
* `2 <= n <= 105`
* `-104 <= nums1[i], nums2[i] <= 104`
* `-104 <= diff <= 104` | null |
Beats 45.67% || Number of common factors | number-of-common-factors | 0 | 1 | # Intuition\n<!-- Describe your first thoughts on how to solve this problem. -->\n\n# Approach\n<!-- Describe your approach to solving the problem. -->\n\n# Complexity\n- Time complexity:\n<!-- Add your time complexity here, e.g. $$O(n)$$ -->\n\n- Space complexity:\n<!-- Add your space complexity here, e.g. $$O(n)$$ -->\n\n# Code\n```\nclass Solution:\n def commonFactors(self, a: int, b: int) -> int:\n min_ele=min(a,b)\n count=0\n while min_ele>0:\n if ( a%min_ele==0 and b%min_ele==0):\n count+=1\n min_ele-=1\n return count\n \n``` | 1 | Given two positive integers `a` and `b`, return _the number of **common** factors of_ `a` _and_ `b`.
An integer `x` is a **common factor** of `a` and `b` if `x` divides both `a` and `b`.
**Example 1:**
**Input:** a = 12, b = 6
**Output:** 4
**Explanation:** The common factors of 12 and 6 are 1, 2, 3, 6.
**Example 2:**
**Input:** a = 25, b = 30
**Output:** 2
**Explanation:** The common factors of 25 and 30 are 1, 5.
**Constraints:**
* `1 <= a, b <= 1000` | null |
Python / JS one-liners | number-of-common-factors | 0 | 1 | **Python**\n```\nclass Solution:\n def commonFactors(self, a: int, b: int) -> int:\n return sum(a % n == 0 and b % n == 0 for n in range(1, min(a, b) + 1))\n```\n**Javascript**\n```\nconst commonFactors = (a, b) => (\n new Array(Math.min(a, b)+1).fill(0)\n .reduce((total, _, i) => total + (a % i == 0 && b % i == 0), 0)\n)\n``` | 2 | Given two positive integers `a` and `b`, return _the number of **common** factors of_ `a` _and_ `b`.
An integer `x` is a **common factor** of `a` and `b` if `x` divides both `a` and `b`.
**Example 1:**
**Input:** a = 12, b = 6
**Output:** 4
**Explanation:** The common factors of 12 and 6 are 1, 2, 3, 6.
**Example 2:**
**Input:** a = 25, b = 30
**Output:** 2
**Explanation:** The common factors of 25 and 30 are 1, 5.
**Constraints:**
* `1 <= a, b <= 1000` | null |
Python 100% run time | number-of-common-factors | 0 | 1 | ```\nclass Solution:\n def commonFactors(self, a: int, b: int) -> int:\n count = 0\n if max(a,b) % min(a,b) == 0:\n count += 1\n for i in range(1,(min(a,b)//2) + 1):\n if a % i == 0 and b % i == 0:\n count += 1\n return count\n``` | 1 | Given two positive integers `a` and `b`, return _the number of **common** factors of_ `a` _and_ `b`.
An integer `x` is a **common factor** of `a` and `b` if `x` divides both `a` and `b`.
**Example 1:**
**Input:** a = 12, b = 6
**Output:** 4
**Explanation:** The common factors of 12 and 6 are 1, 2, 3, 6.
**Example 2:**
**Input:** a = 25, b = 30
**Output:** 2
**Explanation:** The common factors of 25 and 30 are 1, 5.
**Constraints:**
* `1 <= a, b <= 1000` | null |
Python Elegant & Short | maximum-sum-of-an-hourglass | 0 | 1 | ```\nfrom typing import List\n\n\nclass Solution:\n """\n Time: O(n*m)\n Memory: O(n*m)\n """\n\n def maxSum(self, grid: List[List[int]]) -> int:\n n, m = len(grid), len(grid[0])\n return max(\n self.get_hourglass(grid, i, j)\n for i in range(1, n - 1)\n for j in range(1, m - 1)\n )\n\n @staticmethod\n def get_hourglass(grid: List[List[int]], i: int, j: int) -> int:\n return grid[i - 1][j - 1] + grid[i - 1][j] + grid[i - 1][j + 1] + \\\n grid[i][j] + \\\n grid[i + 1][j - 1] + grid[i + 1][j] + grid[i + 1][j + 1]\n```\n\nIf you like this solution remember to **upvote it** to let me know.\n | 2 | You are given an `m x n` integer matrix `grid`.
We define an **hourglass** as a part of the matrix with the following form:
Return _the **maximum** sum of the elements of an hourglass_.
**Note** that an hourglass cannot be rotated and must be entirely contained within the matrix.
**Example 1:**
**Input:** grid = \[\[6,2,1,3\],\[4,2,1,5\],\[9,2,8,7\],\[4,1,2,9\]\]
**Output:** 30
**Explanation:** The cells shown above represent the hourglass with the maximum sum: 6 + 2 + 1 + 2 + 9 + 2 + 8 = 30.
**Example 2:**
**Input:** grid = \[\[1,2,3\],\[4,5,6\],\[7,8,9\]\]
**Output:** 35
**Explanation:** There is only one hourglass in the matrix, with the sum: 1 + 2 + 3 + 5 + 7 + 8 + 9 = 35.
**Constraints:**
* `m == grid.length`
* `n == grid[i].length`
* `3 <= m, n <= 150`
* `0 <= grid[i][j] <= 106` | null |
Simple Python Solution || O(MxN) beats 83.88% | maximum-sum-of-an-hourglass | 0 | 1 | ```\nclass Solution:\n def maxSum(self, grid: List[List[int]]) -> int:\n res=0\n cur=0\n \n for i in range(len(grid)-2):\n for j in range(1,len(grid[0])-1):\n \n cur=sum(grid[i][j-1:j+2]) +grid[i+1][j] + sum(grid[i+2][j-1:j+2])\n res = max(res,cur)\n \n return res\n \n``` | 1 | You are given an `m x n` integer matrix `grid`.
We define an **hourglass** as a part of the matrix with the following form:
Return _the **maximum** sum of the elements of an hourglass_.
**Note** that an hourglass cannot be rotated and must be entirely contained within the matrix.
**Example 1:**
**Input:** grid = \[\[6,2,1,3\],\[4,2,1,5\],\[9,2,8,7\],\[4,1,2,9\]\]
**Output:** 30
**Explanation:** The cells shown above represent the hourglass with the maximum sum: 6 + 2 + 1 + 2 + 9 + 2 + 8 = 30.
**Example 2:**
**Input:** grid = \[\[1,2,3\],\[4,5,6\],\[7,8,9\]\]
**Output:** 35
**Explanation:** There is only one hourglass in the matrix, with the sum: 1 + 2 + 3 + 5 + 7 + 8 + 9 = 35.
**Constraints:**
* `m == grid.length`
* `n == grid[i].length`
* `3 <= m, n <= 150`
* `0 <= grid[i][j] <= 106` | null |
Python Solution | NumPy | The Easiest Way | maximum-sum-of-an-hourglass | 0 | 1 | ```\nimport numpy as np\nclass Solution:\n def maxSum(self, grid) -> int:\n l, r, m, n = 0, 0, len(grid), len(grid[0])\n arr = np.array(grid)\n max_sum = 0\n while l < m - 2:\n while r < n - 2:\n current_sum = np.sum(arr[l:l + 3, r:r + 3]) - (arr[l + 1, r] + arr[l + 1, r + 2]) # summing 3x3 matrix and subtracting 2 values\n max_sum = max(max_sum, current_sum)\n r += 1\n l += 1\n r = 0\n return max_sum\n``` | 1 | You are given an `m x n` integer matrix `grid`.
We define an **hourglass** as a part of the matrix with the following form:
Return _the **maximum** sum of the elements of an hourglass_.
**Note** that an hourglass cannot be rotated and must be entirely contained within the matrix.
**Example 1:**
**Input:** grid = \[\[6,2,1,3\],\[4,2,1,5\],\[9,2,8,7\],\[4,1,2,9\]\]
**Output:** 30
**Explanation:** The cells shown above represent the hourglass with the maximum sum: 6 + 2 + 1 + 2 + 9 + 2 + 8 = 30.
**Example 2:**
**Input:** grid = \[\[1,2,3\],\[4,5,6\],\[7,8,9\]\]
**Output:** 35
**Explanation:** There is only one hourglass in the matrix, with the sum: 1 + 2 + 3 + 5 + 7 + 8 + 9 = 35.
**Constraints:**
* `m == grid.length`
* `n == grid[i].length`
* `3 <= m, n <= 150`
* `0 <= grid[i][j] <= 106` | null |
O(n^2) using sliding window for finding houg-glass matrix 3x3 | maximum-sum-of-an-hourglass | 0 | 1 | **Main Idea**:\n+ Processing 3x3 by sliding window\n\n**Code**:\n```\nclass Solution:\n def maxSum(self, grid: List[List[int]]) -> int:\n m, n = len(grid), len(grid[0])\n ans = 0\n for i in range(m-2):\n for j in range(n-2):\n vsum = 0\n for k in range(3):\n for t in range(3):\n # print(grid[i+k][j+t], end = " ")\n if k==0 or k==2 or (k==1 and t==1):\n vsum = vsum + grid[i+k][j+t]\n # print()\n ans = max(ans, vsum)\n # print("--")\n # print("=" * 20)\n return ans\n``` | 1 | You are given an `m x n` integer matrix `grid`.
We define an **hourglass** as a part of the matrix with the following form:
Return _the **maximum** sum of the elements of an hourglass_.
**Note** that an hourglass cannot be rotated and must be entirely contained within the matrix.
**Example 1:**
**Input:** grid = \[\[6,2,1,3\],\[4,2,1,5\],\[9,2,8,7\],\[4,1,2,9\]\]
**Output:** 30
**Explanation:** The cells shown above represent the hourglass with the maximum sum: 6 + 2 + 1 + 2 + 9 + 2 + 8 = 30.
**Example 2:**
**Input:** grid = \[\[1,2,3\],\[4,5,6\],\[7,8,9\]\]
**Output:** 35
**Explanation:** There is only one hourglass in the matrix, with the sum: 1 + 2 + 3 + 5 + 7 + 8 + 9 = 35.
**Constraints:**
* `m == grid.length`
* `n == grid[i].length`
* `3 <= m, n <= 150`
* `0 <= grid[i][j] <= 106` | null |
🔥[Python 3] Simple brute-force, beats 89% | maximum-sum-of-an-hourglass | 0 | 1 | ```python3 []\nclass Solution:\n def maxSum(self, grid: List[List[int]]) -> int:\n res = 0\n for i in range(len(grid)-2):\n for j in range(len(grid[0])-2):\n sm = grid[i][j] + grid[i][j+1] + grid[i][j+2] + grid[i+1][j+1] + \\\n grid[i+2][j] + grid[i+2][j+1] + grid[i+2][j+2]\n res = max(res, sm)\n return res\n```\n\n | 2 | You are given an `m x n` integer matrix `grid`.
We define an **hourglass** as a part of the matrix with the following form:
Return _the **maximum** sum of the elements of an hourglass_.
**Note** that an hourglass cannot be rotated and must be entirely contained within the matrix.
**Example 1:**
**Input:** grid = \[\[6,2,1,3\],\[4,2,1,5\],\[9,2,8,7\],\[4,1,2,9\]\]
**Output:** 30
**Explanation:** The cells shown above represent the hourglass with the maximum sum: 6 + 2 + 1 + 2 + 9 + 2 + 8 = 30.
**Example 2:**
**Input:** grid = \[\[1,2,3\],\[4,5,6\],\[7,8,9\]\]
**Output:** 35
**Explanation:** There is only one hourglass in the matrix, with the sum: 1 + 2 + 3 + 5 + 7 + 8 + 9 = 35.
**Constraints:**
* `m == grid.length`
* `n == grid[i].length`
* `3 <= m, n <= 150`
* `0 <= grid[i][j] <= 106` | null |
Easy to understand Python code using bit counting. | minimize-xor | 0 | 1 | The approach is simple,\n1. First count the number of 1\'s in num2 and store in c\n2. We want to set c no. of 1\'s in the required num wherever we find a set bit num1(this is because 1^1 = 0. \n3. Remember we go from MSB because we want to use c no.of 1\'s most effectively to reduce more value.\n4. After setting c 1\'s if you are still left with some 1\'s then place them at unset bits from LSB.\n5. This way we get the minimum XOR value.\n```\n c = 0\n for i in range(32):\n if num2 & (1<<i):\n c+=1\n num = 0\n for i in range(31,-1,-1):\n if (num1 & (1<<i)) and c>0:\n num+=pow(2,i)\n c-=1\n s = str(bin(num)[2:])\n s = "0"*(32-len(s))+s\n i = 31\n while(c>0 and i>0):\n if (s[i]=="0"):\n s = s[:i]+"1"+s[i+1:]\n c-=1\n else:\n i-=1\n return int(s,2)\n``` | 6 | Given two positive integers `num1` and `num2`, find the positive integer `x` such that:
* `x` has the same number of set bits as `num2`, and
* The value `x XOR num1` is **minimal**.
Note that `XOR` is the bitwise XOR operation.
Return _the integer_ `x`. The test cases are generated such that `x` is **uniquely determined**.
The number of **set bits** of an integer is the number of `1`'s in its binary representation.
**Example 1:**
**Input:** num1 = 3, num2 = 5
**Output:** 3
**Explanation:**
The binary representations of num1 and num2 are 0011 and 0101, respectively.
The integer **3** has the same number of set bits as num2, and the value `3 XOR 3 = 0` is minimal.
**Example 2:**
**Input:** num1 = 1, num2 = 12
**Output:** 3
**Explanation:**
The binary representations of num1 and num2 are 0001 and 1100, respectively.
The integer **3** has the same number of set bits as num2, and the value `3 XOR 1 = 2` is minimal.
**Constraints:**
* `1 <= num1, num2 <= 109` | null |
O(log(n)) using set and clear bit (examples) | minimize-xor | 0 | 1 | **Main Idea**:\n+ Counting the number of bit 1 of num2 (**nbit1**)\n\n+ Clearing bit 1 of num1 using **nbit1** from position high to lower \n + Setting bit 1 to ans for clearing positions \n+ Setting remain nbit 1 to ans for position from low to high excluding clearing positions\n\n**Examples**:\n```\nnum2 = 28 = 1111 1100\nnum1 = 09 = 0000 1001\n\n-> nbit1 = 6\n\n+ clear bit1 \nx = 0000 1001\nnum1 = 0000 0000\n x--x\n-> remain nbit1 = 4\n+ set remain nbit1 = 4\nx = 0011 1111\n ss xssx\n```\n\n**Code**:\n```\nclass Solution:\n def minimizeXor(self, num1: int, num2: int) -> int: \n nbit1 = 0\n while num2>0:\n nbit1 = nbit1 + (num2&1)\n num2 = num2 >> 1\n # print(nbit1)\n \n chk = []\n ans = 0\n # print(bin(num1), bin(ans))\n for i in range(31, -1, -1):\n biti = (num1>>i)&1\n if biti==1 and nbit1>0:\n num1 = num1 & ~(1<<i)\n ans = ans | (1<<i)\n chk.append(i)\n nbit1 -= 1\n # print(bin(num1), bin(ans))\n \n if nbit1>0:\n for i in range(0, 32, 1):\n biti = (num1>>i)&1\n if i not in chk and nbit1>0:\n num1 = num1 | (1<<i)\n ans = ans | (1<<i)\n nbit1 -= 1\n # print(bin(num1), bin(ans))\n # print("=" * 20)\n \n return ans\n``` | 1 | Given two positive integers `num1` and `num2`, find the positive integer `x` such that:
* `x` has the same number of set bits as `num2`, and
* The value `x XOR num1` is **minimal**.
Note that `XOR` is the bitwise XOR operation.
Return _the integer_ `x`. The test cases are generated such that `x` is **uniquely determined**.
The number of **set bits** of an integer is the number of `1`'s in its binary representation.
**Example 1:**
**Input:** num1 = 3, num2 = 5
**Output:** 3
**Explanation:**
The binary representations of num1 and num2 are 0011 and 0101, respectively.
The integer **3** has the same number of set bits as num2, and the value `3 XOR 3 = 0` is minimal.
**Example 2:**
**Input:** num1 = 1, num2 = 12
**Output:** 3
**Explanation:**
The binary representations of num1 and num2 are 0001 and 1100, respectively.
The integer **3** has the same number of set bits as num2, and the value `3 XOR 1 = 2` is minimal.
**Constraints:**
* `1 <= num1, num2 <= 109` | null |
✔ Python3 Solution | DP | Clean & Concise | maximum-deletions-on-a-string | 0 | 1 | # Complexity\n- Time complexity: $$O(n^2)$$\n- Space complexity: $$O(n)$$\n\n# Code\n```python\nclass Solution:\n def deleteString(self, S):\n N = len(S)\n if S.count(S[0]) == N: return N\n P = [0] * (N + 1)\n dp = [1] * N\n for i in range(N - 1, -1, -1):\n for j in range(i + 1, N):\n P[j] = P[j + 1] + 1 if S[i] == S[j] else 0\n if P[j] >= j - i:\n dp[i] = max(dp[i], dp[j] + 1)\n return dp[0]\n``` | 1 | You are given a string `s` consisting of only lowercase English letters. In one operation, you can:
* Delete **the entire string** `s`, or
* Delete the **first** `i` letters of `s` if the first `i` letters of `s` are **equal** to the following `i` letters in `s`, for any `i` in the range `1 <= i <= s.length / 2`.
For example, if `s = "ababc "`, then in one operation, you could delete the first two letters of `s` to get `"abc "`, since the first two letters of `s` and the following two letters of `s` are both equal to `"ab "`.
Return _the **maximum** number of operations needed to delete all of_ `s`.
**Example 1:**
**Input:** s = "abcabcdabc "
**Output:** 2
**Explanation:**
- Delete the first 3 letters ( "abc ") since the next 3 letters are equal. Now, s = "abcdabc ".
- Delete all the letters.
We used 2 operations so return 2. It can be proven that 2 is the maximum number of operations needed.
Note that in the second operation we cannot delete "abc " again because the next occurrence of "abc " does not happen in the next 3 letters.
**Example 2:**
**Input:** s = "aaabaab "
**Output:** 4
**Explanation:**
- Delete the first letter ( "a ") since the next letter is equal. Now, s = "aabaab ".
- Delete the first 3 letters ( "aab ") since the next 3 letters are equal. Now, s = "aab ".
- Delete the first letter ( "a ") since the next letter is equal. Now, s = "ab ".
- Delete all the letters.
We used 4 operations so return 4. It can be proven that 4 is the maximum number of operations needed.
**Example 3:**
**Input:** s = "aaaaa "
**Output:** 5
**Explanation:** In each operation, we can delete the first letter of s.
**Constraints:**
* `1 <= s.length <= 4000`
* `s` consists only of lowercase English letters. | null |
[✅ Python] DP top-down Clean & Concise | maximum-deletions-on-a-string | 0 | 1 | ```\n def deleteString(self, s: str) -> int:\n n = len(s)\n if n == 1: return 1\n\t\tif (len(set(s)) == 1): return n\n \n @cache\n def dp(i):\n if i == n - 1: return 1\n res = 0\n l = (n - i) // 2\n temp = ""\n for j in range(1, l + 1):\n temp += s[i + j - 1]\n if temp == s[i+j:i+2*j]:\n res = max(res, dp(i+j))\n return res + 1 \n \n return dp(0)\n``` | 3 | You are given a string `s` consisting of only lowercase English letters. In one operation, you can:
* Delete **the entire string** `s`, or
* Delete the **first** `i` letters of `s` if the first `i` letters of `s` are **equal** to the following `i` letters in `s`, for any `i` in the range `1 <= i <= s.length / 2`.
For example, if `s = "ababc "`, then in one operation, you could delete the first two letters of `s` to get `"abc "`, since the first two letters of `s` and the following two letters of `s` are both equal to `"ab "`.
Return _the **maximum** number of operations needed to delete all of_ `s`.
**Example 1:**
**Input:** s = "abcabcdabc "
**Output:** 2
**Explanation:**
- Delete the first 3 letters ( "abc ") since the next 3 letters are equal. Now, s = "abcdabc ".
- Delete all the letters.
We used 2 operations so return 2. It can be proven that 2 is the maximum number of operations needed.
Note that in the second operation we cannot delete "abc " again because the next occurrence of "abc " does not happen in the next 3 letters.
**Example 2:**
**Input:** s = "aaabaab "
**Output:** 4
**Explanation:**
- Delete the first letter ( "a ") since the next letter is equal. Now, s = "aabaab ".
- Delete the first 3 letters ( "aab ") since the next 3 letters are equal. Now, s = "aab ".
- Delete the first letter ( "a ") since the next letter is equal. Now, s = "ab ".
- Delete all the letters.
We used 4 operations so return 4. It can be proven that 4 is the maximum number of operations needed.
**Example 3:**
**Input:** s = "aaaaa "
**Output:** 5
**Explanation:** In each operation, we can delete the first letter of s.
**Constraints:**
* `1 <= s.length <= 4000`
* `s` consists only of lowercase English letters. | null |
Python 3, DP bottom-up + Substr Hash | maximum-deletions-on-a-string | 0 | 1 | # Intuition\n<!-- Describe your first thoughts on how to solve this problem. -->\nBrute-force approach runs O(S**3) time, S = s.length().\n\n# Approach\n<!-- Describe your approach to solving the problem. -->\nTo reduce run-time to O(S**2), this comparison (s[i:j] == s[j+k])within DP loops needs to be reduced from linear to constant time. I use Rabin-Karp hash approach to reduce run-time to constant.\nThe hash BASE value is 26 (only lower case alphas in s).\n\n# Complexity\n- Time complexity: O(S**2).\n<!-- Add your time complexity here, e.g. $$O(n)$$ -->\n\n- Space complexity: O(S), linear space due to caching recursive DP function + substr hashes.\n<!-- Add your space complexity here, e.g. $$O(n)$$ -->\n\n# Code\n```\nclass Solution:\n def deleteString(self, s: str) -> int:\n if len(set(s)) == 1: return len(s)\n\n MOD = 10**10 + 7 #use MODulus to avoid large int in python.\n BASE = 26 + 1 #base 26, lower case alphas only.\n s_hash = [0] #prefix hash of s.\n for char in s:\n ordChar = ord(char.lower()) - ord(\'a\') + 1\n s_hash.append( (s_hash[-1] * BASE + ordChar) % MOD )\n\n #caching pow function to reduce the runtime.\n @functools.lru_cache( maxsize = None)\n def my_pow( power):\n return pow( BASE, power, MOD)\n\n #get the hash value for substr s. Assuming average constant runtime for my_pow().\n @functools.lru_cache( maxsize = None)\n def get_substr_hash( startIndex, endIndex):\n #return substr[startIndex: endIndex] hash value.\n nonlocal s_hash, s\n if endIndex > len(s): return -1\n return (s_hash[endIndex] - s_hash[startIndex] * my_pow( endIndex - startIndex))%MOD\n\n #DP bottom-up using recursive function calls.\n #RUNTIME: O(S**2), SPACE: O(S). S = s.length(), assuming the get_substr_hash() has constant run-time averagely.\n @functools.lru_cache( maxsize = None)\n def traverse_str( startIndex = 0):\n nonlocal s\n\n if startIndex >= len(s): return 0\n numOps = 1\n for midIndex in range( startIndex+1, len(s)):\n substrLength = midIndex-startIndex\n #if s[startIndex:startIndex+substrLength] == s[midIndex:midIndex+substrLength]:\n if get_substr_hash( startIndex, startIndex+substrLength) == get_substr_hash( midIndex, midIndex+substrLength):\n numOps= max( numOps, 1 + traverse_str( midIndex) )\n return numOps\n \n maxOps = traverse_str()\n\n #cleaning up memory before exit.\n traverse_str.cache_clear()\n my_pow.cache_clear()\n get_substr_hash.cache_clear()\n \n return maxOps\n\n``` | 1 | You are given a string `s` consisting of only lowercase English letters. In one operation, you can:
* Delete **the entire string** `s`, or
* Delete the **first** `i` letters of `s` if the first `i` letters of `s` are **equal** to the following `i` letters in `s`, for any `i` in the range `1 <= i <= s.length / 2`.
For example, if `s = "ababc "`, then in one operation, you could delete the first two letters of `s` to get `"abc "`, since the first two letters of `s` and the following two letters of `s` are both equal to `"ab "`.
Return _the **maximum** number of operations needed to delete all of_ `s`.
**Example 1:**
**Input:** s = "abcabcdabc "
**Output:** 2
**Explanation:**
- Delete the first 3 letters ( "abc ") since the next 3 letters are equal. Now, s = "abcdabc ".
- Delete all the letters.
We used 2 operations so return 2. It can be proven that 2 is the maximum number of operations needed.
Note that in the second operation we cannot delete "abc " again because the next occurrence of "abc " does not happen in the next 3 letters.
**Example 2:**
**Input:** s = "aaabaab "
**Output:** 4
**Explanation:**
- Delete the first letter ( "a ") since the next letter is equal. Now, s = "aabaab ".
- Delete the first 3 letters ( "aab ") since the next 3 letters are equal. Now, s = "aab ".
- Delete the first letter ( "a ") since the next letter is equal. Now, s = "ab ".
- Delete all the letters.
We used 4 operations so return 4. It can be proven that 4 is the maximum number of operations needed.
**Example 3:**
**Input:** s = "aaaaa "
**Output:** 5
**Explanation:** In each operation, we can delete the first letter of s.
**Constraints:**
* `1 <= s.length <= 4000`
* `s` consists only of lowercase English letters. | null |
✅✅✅ 95% faster solution and easy to understand | the-employee-that-worked-on-the-longest-task | 0 | 1 | 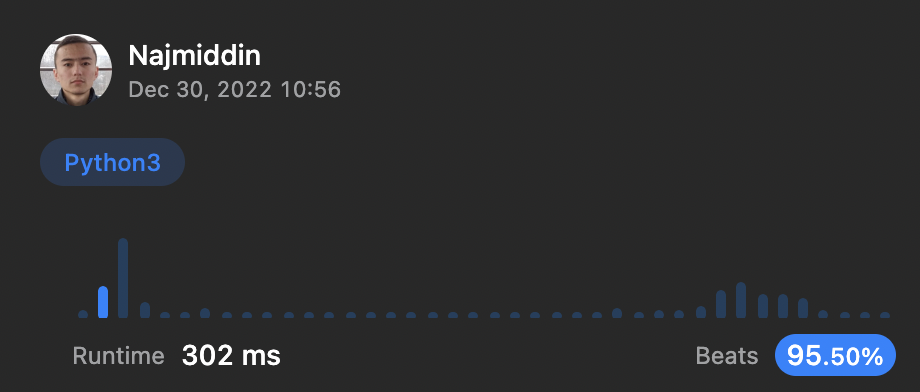\n\n```\nclass Solution:\n def hardestWorker(self, n: int, logs: List[List[int]]) -> int:\n ans = logs[0]\n for i in range(1, len(logs)):\n d = logs[i][1]-logs[i-1][1]\n if d>ans[1] or d==ans[1] and logs[i][0]<ans[0]: ans = [logs[i][0], d]\n return ans[0]\n``` | 1 | There are `n` employees, each with a unique id from `0` to `n - 1`.
You are given a 2D integer array `logs` where `logs[i] = [idi, leaveTimei]` where:
* `idi` is the id of the employee that worked on the `ith` task, and
* `leaveTimei` is the time at which the employee finished the `ith` task. All the values `leaveTimei` are **unique**.
Note that the `ith` task starts the moment right after the `(i - 1)th` task ends, and the `0th` task starts at time `0`.
Return _the id of the employee that worked the task with the longest time._ If there is a tie between two or more employees, return _the **smallest** id among them_.
**Example 1:**
**Input:** n = 10, logs = \[\[0,3\],\[2,5\],\[0,9\],\[1,15\]\]
**Output:** 1
**Explanation:**
Task 0 started at 0 and ended at 3 with 3 units of times.
Task 1 started at 3 and ended at 5 with 2 units of times.
Task 2 started at 5 and ended at 9 with 4 units of times.
Task 3 started at 9 and ended at 15 with 6 units of times.
The task with the longest time is task 3 and the employee with id 1 is the one that worked on it, so we return 1.
**Example 2:**
**Input:** n = 26, logs = \[\[1,1\],\[3,7\],\[2,12\],\[7,17\]\]
**Output:** 3
**Explanation:**
Task 0 started at 0 and ended at 1 with 1 unit of times.
Task 1 started at 1 and ended at 7 with 6 units of times.
Task 2 started at 7 and ended at 12 with 5 units of times.
Task 3 started at 12 and ended at 17 with 5 units of times.
The tasks with the longest time is task 1. The employees that worked on it is 3, so we return 3.
**Example 3:**
**Input:** n = 2, logs = \[\[0,10\],\[1,20\]\]
**Output:** 0
**Explanation:**
Task 0 started at 0 and ended at 10 with 10 units of times.
Task 1 started at 10 and ended at 20 with 10 units of times.
The tasks with the longest time are tasks 0 and 1. The employees that worked on them are 0 and 1, so we return the smallest id 0.
**Constraints:**
* `2 <= n <= 500`
* `1 <= logs.length <= 500`
* `logs[i].length == 2`
* `0 <= idi <= n - 1`
* `1 <= leaveTimei <= 500`
* `idi != idi+1`
* `leaveTimei` are sorted in a strictly increasing order. | null |
Python Elegant & Short | No indexes | 99.32% faster | the-employee-that-worked-on-the-longest-task | 0 | 1 | 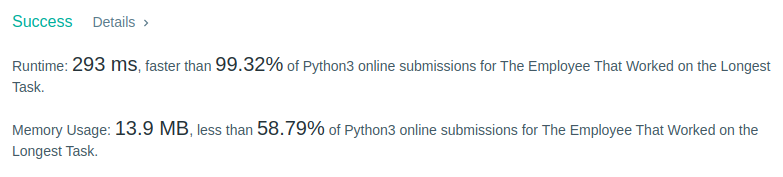\n\n```\nclass Solution:\n """\n Time: O(n)\n Memory: O(1)\n """\n\n def hardestWorker(self, n: int, logs: List[List[int]]) -> int:\n best_id = best_time = start = 0\n\n for emp_id, end in logs:\n time = end - start\n if time > best_time or (time == best_time and best_id > emp_id):\n best_id = emp_id\n best_time = time\n start = end\n\n return best_id\n```\n\nIf you like this solution remember to **upvote it** to let me know.\n | 10 | There are `n` employees, each with a unique id from `0` to `n - 1`.
You are given a 2D integer array `logs` where `logs[i] = [idi, leaveTimei]` where:
* `idi` is the id of the employee that worked on the `ith` task, and
* `leaveTimei` is the time at which the employee finished the `ith` task. All the values `leaveTimei` are **unique**.
Note that the `ith` task starts the moment right after the `(i - 1)th` task ends, and the `0th` task starts at time `0`.
Return _the id of the employee that worked the task with the longest time._ If there is a tie between two or more employees, return _the **smallest** id among them_.
**Example 1:**
**Input:** n = 10, logs = \[\[0,3\],\[2,5\],\[0,9\],\[1,15\]\]
**Output:** 1
**Explanation:**
Task 0 started at 0 and ended at 3 with 3 units of times.
Task 1 started at 3 and ended at 5 with 2 units of times.
Task 2 started at 5 and ended at 9 with 4 units of times.
Task 3 started at 9 and ended at 15 with 6 units of times.
The task with the longest time is task 3 and the employee with id 1 is the one that worked on it, so we return 1.
**Example 2:**
**Input:** n = 26, logs = \[\[1,1\],\[3,7\],\[2,12\],\[7,17\]\]
**Output:** 3
**Explanation:**
Task 0 started at 0 and ended at 1 with 1 unit of times.
Task 1 started at 1 and ended at 7 with 6 units of times.
Task 2 started at 7 and ended at 12 with 5 units of times.
Task 3 started at 12 and ended at 17 with 5 units of times.
The tasks with the longest time is task 1. The employees that worked on it is 3, so we return 3.
**Example 3:**
**Input:** n = 2, logs = \[\[0,10\],\[1,20\]\]
**Output:** 0
**Explanation:**
Task 0 started at 0 and ended at 10 with 10 units of times.
Task 1 started at 10 and ended at 20 with 10 units of times.
The tasks with the longest time are tasks 0 and 1. The employees that worked on them are 0 and 1, so we return the smallest id 0.
**Constraints:**
* `2 <= n <= 500`
* `1 <= logs.length <= 500`
* `logs[i].length == 2`
* `0 <= idi <= n - 1`
* `1 <= leaveTimei <= 500`
* `idi != idi+1`
* `leaveTimei` are sorted in a strictly increasing order. | null |
Python [Explained] | O(n) | the-employee-that-worked-on-the-longest-task | 0 | 1 | # Approach\n- We can iterate through the logs while calculating current task-duration as `logs[i][1]-logs[i-1][1]` for each task and largest task duration as `max_time`.\n- Now we will find smallest `id` among all the largest task duration.\n\n# Complexity\n- Time complexity: O(n)\n\n- Space complexity: O(n)\n\n# Code\n```\nclass Solution:\n def hardestWorker(self, n: int, logs: List[List[int]]) -> int:\n times = [logs[0][1]]\n max_time = times[0]\n for i in range(1, len(logs)):\n times.append(logs[i][1]-logs[i-1][1])\n max_time = max(max_time, times[i])\n \n id = 500 \n for i in range(len(times)):\n if times[i] == max_time:\n id = min(id, logs[i][0])\n \n return id\n```\n----------------\n**Upvote the post if you find it helpful.\nHappy coding.** | 4 | There are `n` employees, each with a unique id from `0` to `n - 1`.
You are given a 2D integer array `logs` where `logs[i] = [idi, leaveTimei]` where:
* `idi` is the id of the employee that worked on the `ith` task, and
* `leaveTimei` is the time at which the employee finished the `ith` task. All the values `leaveTimei` are **unique**.
Note that the `ith` task starts the moment right after the `(i - 1)th` task ends, and the `0th` task starts at time `0`.
Return _the id of the employee that worked the task with the longest time._ If there is a tie between two or more employees, return _the **smallest** id among them_.
**Example 1:**
**Input:** n = 10, logs = \[\[0,3\],\[2,5\],\[0,9\],\[1,15\]\]
**Output:** 1
**Explanation:**
Task 0 started at 0 and ended at 3 with 3 units of times.
Task 1 started at 3 and ended at 5 with 2 units of times.
Task 2 started at 5 and ended at 9 with 4 units of times.
Task 3 started at 9 and ended at 15 with 6 units of times.
The task with the longest time is task 3 and the employee with id 1 is the one that worked on it, so we return 1.
**Example 2:**
**Input:** n = 26, logs = \[\[1,1\],\[3,7\],\[2,12\],\[7,17\]\]
**Output:** 3
**Explanation:**
Task 0 started at 0 and ended at 1 with 1 unit of times.
Task 1 started at 1 and ended at 7 with 6 units of times.
Task 2 started at 7 and ended at 12 with 5 units of times.
Task 3 started at 12 and ended at 17 with 5 units of times.
The tasks with the longest time is task 1. The employees that worked on it is 3, so we return 3.
**Example 3:**
**Input:** n = 2, logs = \[\[0,10\],\[1,20\]\]
**Output:** 0
**Explanation:**
Task 0 started at 0 and ended at 10 with 10 units of times.
Task 1 started at 10 and ended at 20 with 10 units of times.
The tasks with the longest time are tasks 0 and 1. The employees that worked on them are 0 and 1, so we return the smallest id 0.
**Constraints:**
* `2 <= n <= 500`
* `1 <= logs.length <= 500`
* `logs[i].length == 2`
* `0 <= idi <= n - 1`
* `1 <= leaveTimei <= 500`
* `idi != idi+1`
* `leaveTimei` are sorted in a strictly increasing order. | null |
✅ Python Straightforward & Easy to Understand Solution | the-employee-that-worked-on-the-longest-task | 0 | 1 | `O(n)` \n1 - change logs by substituting the end and start times\n2 - find the longest one between durations\n```\nlogs = [[log[0], log[1] - logs[idx-1][1] if idx > 0 else log[1]] for idx, log in enumerate(logs)]\nlongest = max(logs, key=lambda x: (x[1], -x[0]))\nreturn longest[0]\n``` | 3 | There are `n` employees, each with a unique id from `0` to `n - 1`.
You are given a 2D integer array `logs` where `logs[i] = [idi, leaveTimei]` where:
* `idi` is the id of the employee that worked on the `ith` task, and
* `leaveTimei` is the time at which the employee finished the `ith` task. All the values `leaveTimei` are **unique**.
Note that the `ith` task starts the moment right after the `(i - 1)th` task ends, and the `0th` task starts at time `0`.
Return _the id of the employee that worked the task with the longest time._ If there is a tie between two or more employees, return _the **smallest** id among them_.
**Example 1:**
**Input:** n = 10, logs = \[\[0,3\],\[2,5\],\[0,9\],\[1,15\]\]
**Output:** 1
**Explanation:**
Task 0 started at 0 and ended at 3 with 3 units of times.
Task 1 started at 3 and ended at 5 with 2 units of times.
Task 2 started at 5 and ended at 9 with 4 units of times.
Task 3 started at 9 and ended at 15 with 6 units of times.
The task with the longest time is task 3 and the employee with id 1 is the one that worked on it, so we return 1.
**Example 2:**
**Input:** n = 26, logs = \[\[1,1\],\[3,7\],\[2,12\],\[7,17\]\]
**Output:** 3
**Explanation:**
Task 0 started at 0 and ended at 1 with 1 unit of times.
Task 1 started at 1 and ended at 7 with 6 units of times.
Task 2 started at 7 and ended at 12 with 5 units of times.
Task 3 started at 12 and ended at 17 with 5 units of times.
The tasks with the longest time is task 1. The employees that worked on it is 3, so we return 3.
**Example 3:**
**Input:** n = 2, logs = \[\[0,10\],\[1,20\]\]
**Output:** 0
**Explanation:**
Task 0 started at 0 and ended at 10 with 10 units of times.
Task 1 started at 10 and ended at 20 with 10 units of times.
The tasks with the longest time are tasks 0 and 1. The employees that worked on them are 0 and 1, so we return the smallest id 0.
**Constraints:**
* `2 <= n <= 500`
* `1 <= logs.length <= 500`
* `logs[i].length == 2`
* `0 <= idi <= n - 1`
* `1 <= leaveTimei <= 500`
* `idi != idi+1`
* `leaveTimei` are sorted in a strictly increasing order. | null |
✅ 92.93% One Line XORing | find-the-original-array-of-prefix-xor | 1 | 1 | # Intuition\nUpon reading the problem, the first thing that might come to mind is how the XOR operation behaves. Since the prefix XOR array is essentially an accumulation of XOR operations, the inverse process would involve \'undoing\' the XOR operation. We remember that XOR has the following properties:\n\n1. $ a \\oplus a = 0 $\n2. $ a \\oplus 0 = a $\n3. XOR operation is both associative and commutative.\n\nThese properties hint towards the approach of using previous prefix values to deduce the current value.\n\n## Live Coding in Python & Go?\nhttps://youtu.be/nyRgqGYnAf4?si=8YeN7ukMB6uEdXSi\n\n# Approach\n1. The first observation is that the first element of the original array is equal to the first element of the prefix array. This is because there\'s no prior value to XOR with.\n2. For every subsequent element in the prefix array, we can obtain the corresponding element in the original array by XORing the current prefix value with the previous prefix value. This is derived from the properties of XOR, which allows us to \'cancel out\' values, essentially \'undoing\' the accumulation.\n\nUsing this approach, we iterate through the prefix array and populate our original array.\n\n# Complexity\n- Time complexity: $O(n)$\n - We iterate through the prefix array exactly once, resulting in a linear time complexity.\n\n- Space complexity: $O(n)$\n - We are creating a new list that has the same size as the input prefix array, resulting in a linear space complexity.\n\n# Code\n```python []\nclass Solution:\n def findArray(self, pref: List[int]) -> List[int]:\n return [pref[0]] + [pref[i] ^ pref[i-1] for i in range(1, len(pref))]\n```\n``` Java []\npublic class Solution {\n public int[] findArray(int[] pref) {\n int[] result = new int[pref.length];\n result[0] = pref[0];\n for(int i = 1; i < pref.length; i++) {\n result[i] = pref[i] ^ pref[i-1];\n }\n return result;\n }\n}\n```\n``` Go []\nfunc findArray(pref []int) []int {\n result := make([]int, len(pref))\n result[0] = pref[0]\n for i := 1; i < len(pref); i++ {\n result[i] = pref[i] ^ pref[i-1]\n }\n return result\n}\n```\n``` Rust []\nimpl Solution {\n pub fn find_array(pref: Vec<i32>) -> Vec<i32> {\n let mut result = Vec::with_capacity(pref.len());\n result.push(pref[0]);\n for i in 1..pref.len() {\n result.push(pref[i] ^ pref[i-1]);\n }\n result\n }\n}\n```\n``` C++ []\nclass Solution {\npublic:\n vector<int> findArray(vector<int>& pref) {\n vector<int> result(pref.size());\n result[0] = pref[0];\n for(int i = 1; i < pref.size(); i++) {\n result[i] = pref[i] ^ pref[i-1];\n }\n return result;\n }\n};\n```\n``` C# []\npublic class Solution {\n public int[] FindArray(int[] pref) {\n int[] result = new int[pref.Length];\n result[0] = pref[0];\n for(int i = 1; i < pref.Length; i++) {\n result[i] = pref[i] ^ pref[i-1];\n }\n return result;\n }\n}\n```\n``` JavaScript []\nvar findArray = function(pref) {\n let result = [pref[0]];\n for(let i = 1; i < pref.length; i++) {\n result.push(pref[i] ^ pref[i-1]);\n }\n return result;\n }\n```\n``` PHP []\nclass Solution {\n function findArray($pref) {\n $result = [];\n $result[] = $pref[0];\n for($i = 1; $i < count($pref); $i++) {\n $result[] = $pref[$i] ^ $pref[$i-1];\n }\n return $result;\n }\n}\n```\n\n---\n\n## Performance\n\n| Language | Execution Time (ms) | Memory Usage (MB) |\n|------------|---------------------|-------------------|\n| Java | 1 | 58.4 |\n| Rust | 39 | 3.5 |\n| Go | 85 | 9.6 |\n| C++ | 86 | 76.3 |\n| JavaScript | 184 | 71.2 |\n| C# | 243 | 60.2 |\n| PHP | 253 | 39.4 |\n| Python3 | 655 | 36.5 |\n\n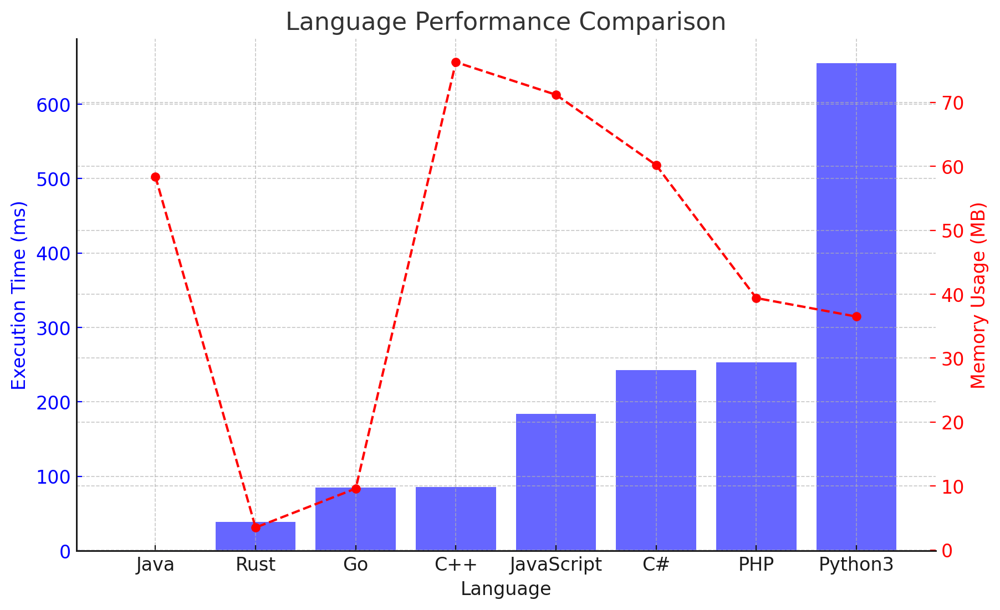\n\n\n## What We Have Learned?\n\nFrom this problem, we\'ve reinforced our understanding of the XOR operation and its properties, particularly how it can be used to deduce information from accumulated data. The solution is optimal because it utilizes the inherent properties of XOR to deduce the original values in a single pass through the prefix array. The approach is both space and time efficient, and showcases how sometimes, understanding the underlying mathematics or logic (in this case, bitwise operations) can lead to very efficient solutions. | 29 | You are given an **integer** array `pref` of size `n`. Find and return _the array_ `arr` _of size_ `n` _that satisfies_:
* `pref[i] = arr[0] ^ arr[1] ^ ... ^ arr[i]`.
Note that `^` denotes the **bitwise-xor** operation.
It can be proven that the answer is **unique**.
**Example 1:**
**Input:** pref = \[5,2,0,3,1\]
**Output:** \[5,7,2,3,2\]
**Explanation:** From the array \[5,7,2,3,2\] we have the following:
- pref\[0\] = 5.
- pref\[1\] = 5 ^ 7 = 2.
- pref\[2\] = 5 ^ 7 ^ 2 = 0.
- pref\[3\] = 5 ^ 7 ^ 2 ^ 3 = 3.
- pref\[4\] = 5 ^ 7 ^ 2 ^ 3 ^ 2 = 1.
**Example 2:**
**Input:** pref = \[13\]
**Output:** \[13\]
**Explanation:** We have pref\[0\] = arr\[0\] = 13.
**Constraints:**
* `1 <= pref.length <= 105`
* `0 <= pref[i] <= 106` | null |
✅ Beats 99.24% 🔥 || 3 Approaches 💡 || | find-the-original-array-of-prefix-xor | 1 | 1 | # Approach 1: Prefix Sum\n\n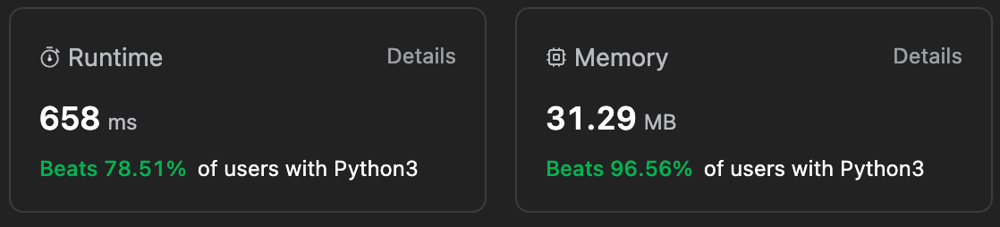\n\n# Intuition:\n\n**If we have an array of prefix sums pref, then the original array arr can be recovered by taking the prefix sum of pref. That is, arr[i] = pref[i] ^ pref[i-1] for i > 0 and arr[0] = pref[0]. This is because pref[i] = arr[0] ^ arr[1] ^ ... ^ arr[i-1] ^ arr[i], so pref[i] ^ pref[i-1] = arr[i].** \n\n# Algorithm:\n1. Initialize ans = [0] * n, where n is the length of pref.\n2. ans[0] = pref[0] because pref[0] = arr[0] ^ arr[1] ^ ... ^ arr[n-1] = arr[0].\n3. For i in range(1, n), ans[i] = pref[i] ^ pref[i-1]. This is because pref[i] = arr[0] ^ arr[1] ^ ... ^ arr[i-1] ^ arr[i], so pref[i] ^ pref[i-1] = arr[i].\n4. Return ans.\n\n# Complexity Analysis:\n- Time Complexity: **O(n)**, where n is the length of pref.\n- Space Complexity: **O(n)**, the space used by ans.\n\n# Code\n``` Python []\nclass Solution:\n def findArray(self, pref: List[int]) -> List[int]:\n n = len(pref)\n ans = [0] * n\n ans[0]=pref[0]\n for i in range(1,n):\n ans[i]=pref[i]^pref[i-1]\n return ans\n```\n``` C++ []\nclass Solution {\npublic:\n vector<int> findArray(vector<int>& pref) {\n int n = pref.size();\n vector<int> ans(n, 0);\n ans[0] = pref[0];\n for (int i = 1; i < n; i++) {\n ans[i] = pref[i] ^ pref[i - 1];\n }\n return ans;\n }\n};\n```\n``` Java []\nclass Solution {\n public int[] findArray(int[] pref) {\n int n = pref.length;\n int[] ans = new int[n];\n ans[0] = pref[0];\n for (int i = 1; i < n; i++) {\n ans[i] = pref[i] ^ pref[i - 1];\n }\n return ans;\n }\n}\n```\n# Approach 2: Prefix Sum (In-Place)\n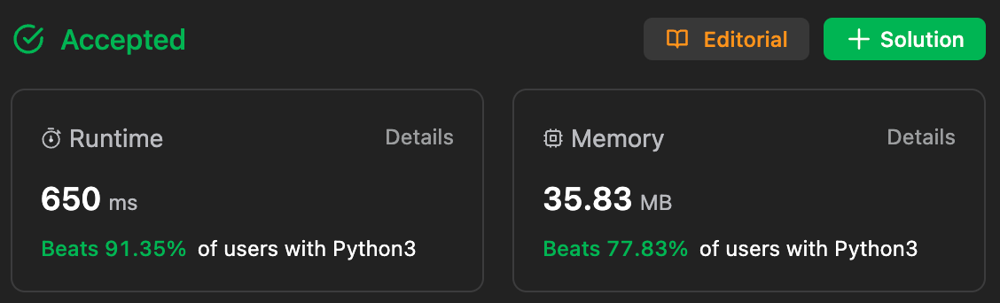\n\n# Intuition:\n**If we have an array of prefix sums pref, then the original array arr can be recovered by taking the prefix sum of pref. That is, arr[i] = pref[i] ^ pref[i-1] for i > 0 and arr[0] = pref[0]. This is because pref[i] = arr[0] ^ arr[1] ^ ... ^ arr[i-1] ^ arr[i], so pref[i] ^ pref[i-1] = arr[i].**\n\n# Algorithm:\n1. For i in range(1, n), pref[i] ^= pref[i-1]. This is because pref[i] = arr[0] ^ arr[1] ^ ... ^ arr[i-1] ^ arr[i], so pref[i] ^ pref[i-1] = arr[i].\n2. Return pref.\n\n# Complexity Analysis:\n- Time Complexity: **O(n)**, where n is the length of pref.\n- Space Complexity: **O(1)**, the space used by pref.\n\n\n# Code\n``` Python []\nclass Solution:\n def findArray(self, pref: List[int]) -> List[int]:\n return [pref[0]] + [pref[i] ^ pref[i-1] for i in range(1, len(pref))]\n```\n``` C++ []\nclass Solution {\npublic:\n vector<int> findArray(vector<int>& pref) {\n vector<int> result(pref.size());\n result[0] = pref[0];\n for (int i = 1; i < pref.size(); i++) {\n result[i] = pref[i] ^ pref[i-1];\n }\n return result;\n }\n};\n```\n``` Java []\nclass Solution {\n public int[] findArray(int[] pref) {\n int[] result = new int[pref.length];\n result[0] = pref[0];\n for (int i = 1; i < pref.length; i++) {\n result[i] = pref[i] ^ pref[i-1];\n }\n return result;\n }\n}\n```\n\n# Approach 3: Reverse Prefix Sum (In-Place)\n\n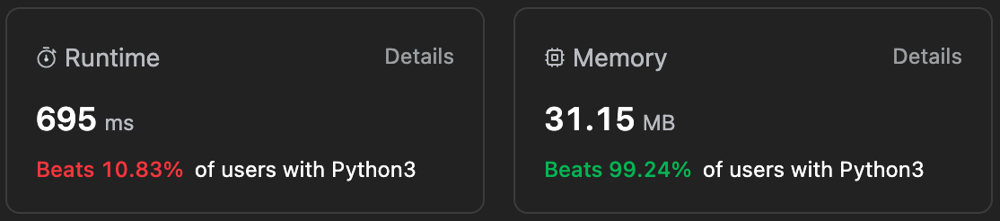\n\n# Intuition:\n**If we to find out the prefix sum of pref, we will be doing it in by pref[i]=pref[i]^pref[i-1]. But when we do it in reverse order. We don\'t \nhave to create a new array. We can just do it in place.**\n\n# Algorithm:\n1. For i in range(n-1, 0, -1), pref[i] ^= pref[i-1]. This is because pref[i] = arr[0] ^ arr[1] ^ ... ^ arr[i-1] ^ arr[i], so pref[i] ^ pref[i-1] = arr[i].\n2. Return pref.\n\n# Complexity Analysis:\n- Time Complexity: **O(n)**, where n is the length of pref.\n- Space Complexity: **O(1)**, the space used by pref.\n# Code\n``` Python []\nclass Solution:\n def findArray(self, pref):\n for i in range(len(pref) - 1, 0, -1):\n pref[i] = (pref[i] ^ pref[i-1])\n return pref\n```\n``` C++ []\nclass Solution {\npublic:\n vector<int> findArray(vector<int>& pref) {\n for (int i = pref.size() - 1; i > 0; i--) {\n pref[i] = (pref[i] ^ pref[i-1]);\n }\n return pref;\n }\n};\n```\n``` Java []\nclass Solution {\n public int[] findArray(int[] pref) {\n for (int i = pref.length - 1; i > 0; i--) {\n pref[i] = (pref[i] ^ pref[i-1]);\n }\n return pref;\n }\n}\n``` | 2 | You are given an **integer** array `pref` of size `n`. Find and return _the array_ `arr` _of size_ `n` _that satisfies_:
* `pref[i] = arr[0] ^ arr[1] ^ ... ^ arr[i]`.
Note that `^` denotes the **bitwise-xor** operation.
It can be proven that the answer is **unique**.
**Example 1:**
**Input:** pref = \[5,2,0,3,1\]
**Output:** \[5,7,2,3,2\]
**Explanation:** From the array \[5,7,2,3,2\] we have the following:
- pref\[0\] = 5.
- pref\[1\] = 5 ^ 7 = 2.
- pref\[2\] = 5 ^ 7 ^ 2 = 0.
- pref\[3\] = 5 ^ 7 ^ 2 ^ 3 = 3.
- pref\[4\] = 5 ^ 7 ^ 2 ^ 3 ^ 2 = 1.
**Example 2:**
**Input:** pref = \[13\]
**Output:** \[13\]
**Explanation:** We have pref\[0\] = arr\[0\] = 13.
**Constraints:**
* `1 <= pref.length <= 105`
* `0 <= pref[i] <= 106` | null |
【Video】Give me 5 minutes - How we think about a solution - Python, JavaScript, Java, C++ | find-the-original-array-of-prefix-xor | 1 | 1 | # Intuition\nThis is similar to prefix sum\n\n---\n\n# Solution Video\n\nhttps://youtu.be/ck4dJURKAlA\n\n\u25A0 Timeline of the video\n`0:05` Solution code with build-in function\n`0:06` quick recap of XOR calculation\n`0:28` Demonstrate how to solve the question\n`1:37` How you keep the original previous number\n`2:29` Continue to demonstrate how to solve the question\n`3:59` Coding\n`4:43` Time Complexity and Space Complexity\n\n### \u2B50\uFE0F\u2B50\uFE0F Don\'t forget to subscribe to my channel! \u2B50\uFE0F\u2B50\uFE0F\n\n**\u25A0 Subscribe URL**\nhttp://www.youtube.com/channel/UC9RMNwYTL3SXCP6ShLWVFww?sub_confirmation=1\n\nSubscribers: 2,898\nMy initial goal is 10,000\nThank you for your support!\n\n---\n\n# Approach\n\n## How we think about a solution\n\nTo be honest, there is no complicated logic this time. This is similar to prefix sum, so we can calculate `XOR` with the input numbers one by one.\n\n```\nInput: pref = [5,2,0,3,1]\n```\n\n##### Quick recap of XOR caluclation\n---\nIf one of numbers is 1 and the other is 0 \u2192 1\nIf both numbers are 1 \u2192 0\nIf both numbers are 0 \u2192 0\n\n---\n\n\n\nFirst of all, we calculate `5`, but there is no previous number, so it is considered as `0`\n\n```\n101 (= 5)\n000 (= 0)\n---\n101 (= 5)\n\n```\nActually, we can start from `index 1` in real solution code, becuase the number at index 0 never change.\n\nMy solution starts from here. In the solution code, I update input array `pref` itself.\n```\nindex = 1\n\n010 (= 2)\n101 (= 5)\n---\n111 (= 7)\n\npref = [5, 7, 0, 3, 1]\n```\nBut problem is we need `2` for the next calculation, because `2` is the previous original number of intput array. How do you get `2`.\n\nIt\'s simple.\n```\n2 ^ 5 = 7 \n```\nThis is similar to \n```\n80 + 20 = 100\n\u2193\n80 = 100 - 20\n```\n```\n2 ^ 5 = 7 \n\u2193\n2 = 7 ^ 5\n\n111 (= 7)\n101 (= 5)\n---\n010 (= 2)\n\n```\n---\n\n\u2B50\uFE0F Points\n\nCalculate a previous number we need for the next calculation before we move the next iteration.\n```\n(next)prev = pref[i] ^ (current)prev\n\npref[i] is a current result\n```\n---\n\nWe use `prev` for the next calculation.\n```\nprev = 2\npref = [5, 7, 0, 3, 1]\n```\n```\nindex = 2\n\n000 (= 0 from input array)\n010 (= 2 from prev)\n---\n010 (= 2)\n\npref = [5, 7, 2, 3, 1]\nprev = 0 (= 2 ^ 2) \n```\n```\nindex = 3\n\n011 (= 3 from input array)\n000 (= 0 from prev)\n---\n011 (= 3)\n\npref = [5, 7, 2, 3, 1]\nprev = 3 (= 0 ^ 3) \n```\n\n```\nindex = 4\n\n001 (= 1 from input array)\n011 (= 3 from prev)\n---\n010 (= 2)\n\npref = [5, 7, 2, 3, 2]\nprev = 1 (= 3 ^ 2) \n```\n```\npref: [5,7,2,3,2] (Output)\n```\n\n---\n\n\n\n### Algorithm Overview:\nThe algorithm takes an input list `pref` of integers and transforms it in-place.\n\n### Detailed Explanation:\n1. Initialize a variable `prev` with the value of the first element in the `pref` list.\n2. Start a loop from the second element (index 1) to the last element of the `pref` list.\n3. Inside the loop, perform a bitwise XOR operation (`^`) between the current element at index `i` and the `prev` value. This replaces the current element with the XOR result.\n4. Update the `prev` value by performing a bitwise XOR operation between the previous `prev` value and the updated current element.\n5. Repeat steps 3-4 for all elements in the `pref` list.\n6. Return the modified `pref` list. The input list has been transformed in-place, with each element being XORed with the cumulative XOR of all previous elements.\n\n\n---\n\n\n\n# Complexity\n- Time complexity: $$O(n)$$\n<!-- Add your time complexity here, e.g. $$O(n)$$ -->\n\n- Space complexity: $$O(1)$$\nIf we can update input array. My solution code updates it directly. But if we can\'t, space complexity should be $$O(n)$$ for result array. You need to ask interviewers about it in real interviews.\n\n\n```python []\nclass Solution:\n def findArray(self, pref: List[int]) -> List[int]:\n \n prev = pref[0]\n\n for i in range(1, len(pref)):\n pref[i] ^= prev\n prev ^= pref[i]\n \n return pref\n```\n```javascript []\n/**\n * @param {number[]} pref\n * @return {number[]}\n */\nvar findArray = function(pref) {\n let prev = pref[0];\n \n for (let i = 1; i < pref.length; i++) {\n pref[i] ^= prev;\n prev ^= pref[i];\n }\n \n return pref; \n};\n```\n```java []\nclass Solution {\n public int[] findArray(int[] pref) {\n int prev = pref[0];\n \n for (int i = 1; i < pref.length; i++) {\n pref[i] ^= prev;\n prev ^= pref[i];\n }\n \n return pref; \n }\n}\n```\n```C++ []\nclass Solution {\npublic:\n vector<int> findArray(vector<int>& pref) {\n int prev = pref[0];\n \n for (int i = 1; i < pref.size(); i++) {\n pref[i] ^= prev;\n prev ^= pref[i];\n }\n \n return pref; \n }\n};\n```\n\n---\n\nThank you for reading my post.\n\u2B50\uFE0F Please upvote it and don\'t forget to subscribe to my channel!\n\n\u25A0 Subscribe URL\nhttp://www.youtube.com/channel/UC9RMNwYTL3SXCP6ShLWVFww?sub_confirmation=1\n\n\u25A0 Twitter\nhttps://twitter.com/CodingNinjaAZ\n\n### My next daily coding challenge post and video.\n\npost\nhttps://leetcode.com/problems/find-mode-in-binary-search-tree/solutions/4233557/video-give-me-5-minutes-how-we-think-about-a-solution-why-do-we-use-inorder-traversal/\n\nvideo\nhttps://youtu.be/0i1Ze62pTuU\n\n\u25A0 Timeline of the video\n`0:04` Which traversal do you use for this question?\n`0:34` Properties of a Binary Search Tree\n`1:31` Key point of my solution code\n`3:09` How to write Inorder, Preorder, Postorder\n`4:08` Coding\n`7:13` Time Complexity and Space Complexity\n\n### \u2B50\uFE0F\u2B50\uFE0F Don\'t forget to subscribe to my channel! \u2B50\uFE0F\u2B50\uFE0F\n\n**\u25A0 Subscribe URL**\nhttp://www.youtube.com/channel/UC9RMNwYTL3SXCP6ShLWVFww?sub_confirmation=1\n\nSubscribers: 2,908\nMy initial goal is 10,000\nThank you for your support!\n\n\n### My previous daily coding challenge post and video.\n\npost\nhttps://leetcode.com/problems/sort-integers-by-the-number-of-1-bits/solutions/4225041/video-give-me-10-minutes-using-heap-without-build-in-function-such-as-sort-or-bit-count/\n\nvideo\nhttps://youtu.be/oqV8Ai0_UWA\n\n\u25A0 Timeline\n`0:05` Solution code with build-in function\n`0:26` Two difficulties to solve the question\n`0:41` How do you count 1 bits?\n`1:05` Understanding to count 1 bits with an example\n`3:02` How do you keep numbers in ascending order when multiple numbers have the same number of 1 bits?\n`5:29` Coding\n`7:54` Time Complexity and Space Complexity | 50 | You are given an **integer** array `pref` of size `n`. Find and return _the array_ `arr` _of size_ `n` _that satisfies_:
* `pref[i] = arr[0] ^ arr[1] ^ ... ^ arr[i]`.
Note that `^` denotes the **bitwise-xor** operation.
It can be proven that the answer is **unique**.
**Example 1:**
**Input:** pref = \[5,2,0,3,1\]
**Output:** \[5,7,2,3,2\]
**Explanation:** From the array \[5,7,2,3,2\] we have the following:
- pref\[0\] = 5.
- pref\[1\] = 5 ^ 7 = 2.
- pref\[2\] = 5 ^ 7 ^ 2 = 0.
- pref\[3\] = 5 ^ 7 ^ 2 ^ 3 = 3.
- pref\[4\] = 5 ^ 7 ^ 2 ^ 3 ^ 2 = 1.
**Example 2:**
**Input:** pref = \[13\]
**Output:** \[13\]
**Explanation:** We have pref\[0\] = arr\[0\] = 13.
**Constraints:**
* `1 <= pref.length <= 105`
* `0 <= pref[i] <= 106` | null |
✅☑[C++/Java/Python/JavaScript] || 1 line Code || EXPLAINED🔥 | find-the-original-array-of-prefix-xor | 1 | 1 | # PLEASE UPVOTE IF IT HELPED\n\n---\n\n\n# Approaches\n**(Also explained in the code)**\n\n\n1. It checks if the size of the `pref` vector is less than or equal to 1. If so, it returns the pref vector as it is, as there\'s not enough information to reconstruct an original array in such cases.\n\n1. If the `pref` vector has more than one element, it initializes an empty vector called `ans` to store the reconstructed original array.\n\n1. It then pushes the first element of the `pref` vector onto the `ans` vector. This is done because the first element of the original array is the same as the first element of the prefix array.\n\n1. The code enters a loop that iterates through the elements of the `pref` vector starting from the second element (at index 1).\n\n1. Inside the loop, it reconstructs the original array element by element. To find the next element in the original array, it takes the `XOR` (bitwise exclusive OR) of the current prefix element (**at index i**) and the previous one (**at index i - 1**).\n\n1. The result of the `XOR` operation is then pushed onto the `ans` vector, which is essentially building the original array element by element.\n\n1. After the loop has processed all elements in the `pref` vector, the reconstructed original array is stored in the `ans` vector.\n\n1. The function returns the `ans` vector, which now contains the reconstructed original array based on the given prefix array.\n\n\n\n# Complexity\n- *Time complexity:*\n $$O(n)$$\n \n\n- *Space complexity:*\n $$O(n)$$\n \n\n\n# Code\n```C++ []\n\nclass Solution {\npublic:\n // Function to find the original array from the prefix array.\n vector<int> findArray(vector<int>& pref) {\n // If the prefix array has one or zero elements, return it as is.\n if (pref.size() <= 1) {\n return pref;\n }\n\n vector<int> ans; // Initialize a vector to store the original array.\n ans.push_back(pref[0]); // The first element in the original array is the same as the first element in the prefix array.\n\n // Iterate through the prefix array to reconstruct the original array.\n for (int i = 1; i < pref.size(); i++) {\n // To find the next element in the original array, take the XOR (bitwise exclusive OR) of the current prefix element and the previous one.\n ans.push_back(pref[i - 1] ^ pref[i]);\n }\n\n return ans; // Return the reconstructed original array.\n }\n};\n\n\n```\n```C []\n\n\nint* findArray(int* pref, int prefSize, int* returnSize) {\n if (prefSize <= 1) {\n *returnSize = prefSize;\n return pref;\n }\n\n int* ans = (int*)malloc(prefSize * sizeof(int));\n ans[0] = pref[0];\n\n for (int i = 1; i < prefSize; i++) {\n ans[i] = pref[i - 1] ^ pref[i];\n }\n\n *returnSize = prefSize;\n return ans;\n}\n\n\n\n```\n\n```Java []\n\n\npublic class Solution {\n public int[] findArray(int[] pref) {\n if (pref.length <= 1) {\n return pref;\n }\n\n int[] ans = new int[pref.length];\n ans[0] = pref[0];\n\n for (int i = 1; i < pref.length; i++) {\n ans[i] = pref[i - 1] ^ pref[i];\n }\n\n return ans;\n }\n}\n\n\n```\n```python3 []\n\nclass Solution:\n def findArray(self, pref):\n if len(pref) <= 1:\n return pref\n\n ans = [pref[0]]\n for i in range(1, len(pref)):\n ans.append(pref[i - 1] ^ pref[i])\n\n return ans\n\n```\n\n\n```javascript []\nclass Solution {\n findArray(pref) {\n if (pref.length <= 1) {\n return pref;\n }\n\n const ans = [pref[0]];\n for (let i = 1; i < pref.length; i++) {\n ans.push(pref[i - 1] ^ pref[i]);\n }\n\n return ans;\n }\n}\n\n\n```\n\n\n---\n\n\n\n# PLEASE UPVOTE IF IT HELPED\n\n---\n---\n\n\n--- | 2 | You are given an **integer** array `pref` of size `n`. Find and return _the array_ `arr` _of size_ `n` _that satisfies_:
* `pref[i] = arr[0] ^ arr[1] ^ ... ^ arr[i]`.
Note that `^` denotes the **bitwise-xor** operation.
It can be proven that the answer is **unique**.
**Example 1:**
**Input:** pref = \[5,2,0,3,1\]
**Output:** \[5,7,2,3,2\]
**Explanation:** From the array \[5,7,2,3,2\] we have the following:
- pref\[0\] = 5.
- pref\[1\] = 5 ^ 7 = 2.
- pref\[2\] = 5 ^ 7 ^ 2 = 0.
- pref\[3\] = 5 ^ 7 ^ 2 ^ 3 = 3.
- pref\[4\] = 5 ^ 7 ^ 2 ^ 3 ^ 2 = 1.
**Example 2:**
**Input:** pref = \[13\]
**Output:** \[13\]
**Explanation:** We have pref\[0\] = arr\[0\] = 13.
**Constraints:**
* `1 <= pref.length <= 105`
* `0 <= pref[i] <= 106` | null |
✅Easy Solution🔥Java/C++/C/Python3/Python/JavaScript🔥 | find-the-original-array-of-prefix-xor | 1 | 1 | 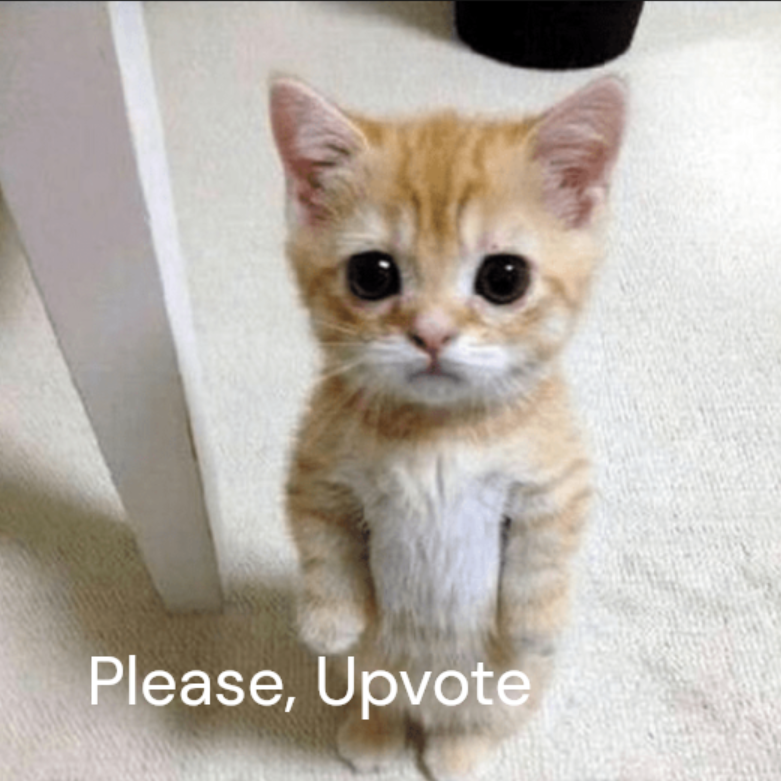\n\n\n# Code\n```Java []\nclass Solution {\n public int[] findArray(int[] pref) {\n int[] ans = new int[pref.length];\n ans[0] = pref[0];\n for(int i=1; i<ans.length; i++){\n ans[i] = pref[i] ^ pref[i-1];\n }\n return ans;\n }\n}\n```\n```C++ []\nclass Solution {\npublic:\n std::vector<int> findArray(std::vector<int>& pref) {\n std::vector<int> ans(pref.size());\n ans[0] = pref[0];\n for (int i = 1; i < ans.size(); i++) {\n ans[i] = pref[i] ^ pref[i - 1];\n }\n return ans;\n }\n};\n```\n```C []\nint* findArray(int* pref, int prefSize, int* returnSize){\n int* ans = (int*)malloc(prefSize * sizeof(int));\n ans[0] = pref[0];\n for (int i = 1; i < prefSize; i++) {\n ans[i] = pref[i] ^ pref[i - 1];\n }\n \n *returnSize = prefSize;\n return ans;\n}\n```\n```Python3 []\nclass Solution:\n def findArray(self, pref: List[int]) -> List[int]:\n ans = [0] * len(pref)\n ans[0] = pref[0]\n for i in range(1, len(ans)):\n ans[i] = pref[i] ^ pref[i - 1]\n return ans\n```\n```Python []\nclass Solution(object):\n def findArray(self, pref):\n ans = [0] * len(pref)\n ans[0] = pref[0]\n for i in range(1, len(ans)):\n ans[i] = pref[i] ^ pref[i - 1]\n return ans\n```\n\n```JavaScript []\n/**\n * @param {number[]} pref\n * @return {number[]}\n */\nvar findArray = function(pref) {\n let ans = new Array(pref.length);\n ans[0] = pref[0];\n for (let i = 1; i < ans.length; i++) {\n ans[i] = pref[i] ^ pref[i - 1];\n }\n return ans;\n};\n\n```\n | 2 | You are given an **integer** array `pref` of size `n`. Find and return _the array_ `arr` _of size_ `n` _that satisfies_:
* `pref[i] = arr[0] ^ arr[1] ^ ... ^ arr[i]`.
Note that `^` denotes the **bitwise-xor** operation.
It can be proven that the answer is **unique**.
**Example 1:**
**Input:** pref = \[5,2,0,3,1\]
**Output:** \[5,7,2,3,2\]
**Explanation:** From the array \[5,7,2,3,2\] we have the following:
- pref\[0\] = 5.
- pref\[1\] = 5 ^ 7 = 2.
- pref\[2\] = 5 ^ 7 ^ 2 = 0.
- pref\[3\] = 5 ^ 7 ^ 2 ^ 3 = 3.
- pref\[4\] = 5 ^ 7 ^ 2 ^ 3 ^ 2 = 1.
**Example 2:**
**Input:** pref = \[13\]
**Output:** \[13\]
**Explanation:** We have pref\[0\] = arr\[0\] = 13.
**Constraints:**
* `1 <= pref.length <= 105`
* `0 <= pref[i] <= 106` | null |
Python3 Solution | find-the-original-array-of-prefix-xor | 0 | 1 | \n```\nclass Solution:\n def findArray(self, pref: List[int]) -> List[int]:\n N=len(pref)\n arr=[pref[0]]\n \n for i in range(1,N):\n arr.append(pref[i-1]^pref[i])\n \n return arr \n``` | 1 | You are given an **integer** array `pref` of size `n`. Find and return _the array_ `arr` _of size_ `n` _that satisfies_:
* `pref[i] = arr[0] ^ arr[1] ^ ... ^ arr[i]`.
Note that `^` denotes the **bitwise-xor** operation.
It can be proven that the answer is **unique**.
**Example 1:**
**Input:** pref = \[5,2,0,3,1\]
**Output:** \[5,7,2,3,2\]
**Explanation:** From the array \[5,7,2,3,2\] we have the following:
- pref\[0\] = 5.
- pref\[1\] = 5 ^ 7 = 2.
- pref\[2\] = 5 ^ 7 ^ 2 = 0.
- pref\[3\] = 5 ^ 7 ^ 2 ^ 3 = 3.
- pref\[4\] = 5 ^ 7 ^ 2 ^ 3 ^ 2 = 1.
**Example 2:**
**Input:** pref = \[13\]
**Output:** \[13\]
**Explanation:** We have pref\[0\] = arr\[0\] = 13.
**Constraints:**
* `1 <= pref.length <= 105`
* `0 <= pref[i] <= 106` | null |
Subsets and Splits