text
stringlengths 7
318k
| id
stringlengths 14
166
| metadata
dict | __index_level_0__
int64 0
439
|
---|---|---|---|
<!--Copyright 2022 The HuggingFace Team. All rights reserved.
Licensed under the Apache License, Version 2.0 (the "License"); you may not use this file except in compliance with
the License. You may obtain a copy of the License at
http://www.apache.org/licenses/LICENSE-2.0
Unless required by applicable law or agreed to in writing, software distributed under the License is distributed on
an "AS IS" BASIS, WITHOUT WARRANTIES OR CONDITIONS OF ANY KIND, either express or implied. See the License for the
specific language governing permissions and limitations under the License.
โ ๏ธ Note that this file is in Markdown but contain specific syntax for our doc-builder (similar to MDX) that may not be
rendered properly in your Markdown viewer.
-->
# ํจ๋ฉ๊ณผ ์๋ผ๋ด๊ธฐ[[padding-and-truncation]]
๋ฐฐ์น ์
๋ ฅ์ ๊ธธ์ด๊ฐ ๋ค๋ฅธ ๊ฒฝ์ฐ๊ฐ ๋ง์์ ๊ณ ์ ํฌ๊ธฐ ํ
์๋ก ๋ณํํ ์ ์์ต๋๋ค. ํจ๋ฉ๊ณผ ์๋ผ๋ด๊ธฐ๋ ๋ค์ํ ๊ธธ์ด์ ๋ฐฐ์น์์ ์ง์ฌ๊ฐํ ํ
์๋ฅผ ์์ฑํ ์ ์๋๋ก ์ด ๋ฌธ์ ๋ฅผ ํด๊ฒฐํ๋ ์ ๋ต์
๋๋ค. ํจ๋ฉ์ ํน์ํ **ํจ๋ฉ ํ ํฐ**์ ์ถ๊ฐํ์ฌ ์งง์ ์ํ์ค๊ฐ ๋ฐฐ์น์์ ๊ฐ์ฅ ๊ธด ์ํ์ค ๋๋ ๋ชจ๋ธ์์ ํ์ฉํ๋ ์ต๋ ๊ธธ์ด์ ๋์ผํ ๊ธธ์ด๋ฅผ ๊ฐ๋๋ก ํฉ๋๋ค. ์๋ผ๋ด๊ธฐ๋ ๊ธด ์ํ์ค๋ฅผ ์๋ผ๋ด์ด ํจ๋ฉ๊ณผ ๋ค๋ฅธ ๋ฐฉ์์ผ๋ก ์ํ์ค์ ๊ธธ์ด๋ฅผ ๋์ผํ๊ฒ ํฉ๋๋ค.
๋๋ถ๋ถ์ ๊ฒฝ์ฐ ๋ฐฐ์น์ ๊ฐ์ฅ ๊ธด ์ํ์ค์ ๊ธธ์ด๋ก ํจ๋ฉํ๊ณ ๋ชจ๋ธ์ด ํ์ฉํ ์ ์๋ ์ต๋ ๊ธธ์ด๋ก ์๋ผ๋ด๋ ๊ฒ์ด ์ ์๋ํฉ๋๋ค. ๊ทธ๋ฌ๋ ํ์ํ๋ค๋ฉด API๊ฐ ์ง์ํ๋ ๋ ๋ง์ ์ ๋ต์ ์ฌ์ฉํ ์ ์์ต๋๋ค. ํ์ํ ์ธ์๋ `padding`, `truncation`, `max_length` ์ธ ๊ฐ์ง์
๋๋ค.
`padding` ์ธ์๋ ํจ๋ฉ์ ์ ์ดํฉ๋๋ค. ๋ถ๋ฆฌ์ธ ๋๋ ๋ฌธ์์ด์ผ ์ ์์ต๋๋ค:
- `True` ๋๋ `'longest'`: ๋ฐฐ์น์์ ๊ฐ์ฅ ๊ธด ์ํ์ค๋ก ํจ๋ฉํฉ๋๋ค(๋จ์ผ ์ํ์ค๋ง ์ ๊ณตํ๋ ๊ฒฝ์ฐ ํจ๋ฉ์ด ์ ์ฉ๋์ง ์์ต๋๋ค).
- `'max_length'`: `max_length` ์ธ์๊ฐ ์ง์ ํ ๊ธธ์ด๋ก ํจ๋ฉํ๊ฑฐ๋, `max_length`๊ฐ ์ ๊ณต๋์ง ์์ ๊ฒฝ์ฐ(`max_length=None`) ๋ชจ๋ธ์์ ํ์ฉ๋๋ ์ต๋ ๊ธธ์ด๋ก ํจ๋ฉํฉ๋๋ค. ๋จ์ผ ์ํ์ค๋ง ์ ๊ณตํ๋ ๊ฒฝ์ฐ์๋ ํจ๋ฉ์ด ์ ์ฉ๋ฉ๋๋ค.
- `False` ๋๋ `'do_not_pad'`: ํจ๋ฉ์ด ์ ์ฉ๋์ง ์์ต๋๋ค. ์ด๊ฒ์ด ๊ธฐ๋ณธ ๋์์
๋๋ค.
`truncation` ์ธ์๋ ์๋ผ๋ผ ๋ฐฉ๋ฒ์ ์ ํฉ๋๋ค. ๋ถ๋ฆฌ์ธ ๋๋ ๋ฌธ์์ด์ผ ์ ์์ต๋๋ค:
- `True` ๋๋ `longest_first`: `max_length` ์ธ์๊ฐ ์ง์ ํ ์ต๋ ๊ธธ์ด๋ก ์๋ผ๋ด๊ฑฐ๋,
`max_length`๊ฐ ์ ๊ณต๋์ง ์์ ๊ฒฝ์ฐ(`max_length=None`) ๋ชจ๋ธ์์ ํ์ฉ๋๋ ์ต๋ ๊ธธ์ด๋ก ์๋ผ๋
๋๋ค.
์ํ์ค ์์์ ๊ฐ์ฅ ๊ธด ์ํ์ค์ ํ ํฐ์ ์ ์ ํ ๊ธธ์ด์ ๋๋ฌํ ๋๊น์ง ํ๋์ฉ ์ ๊ฑฐํฉ๋๋ค.
- `'only_second'`: `max_length` ์ธ์๊ฐ ์ง์ ํ ์ต๋ ๊ธธ์ด๋ก ์๋ผ๋ด๊ฑฐ๋,
`max_length`๊ฐ ์ ๊ณต๋์ง ์์ ๊ฒฝ์ฐ(`max_length=None`) ๋ชจ๋ธ์์ ํ์ฉ๋๋ ์ต๋ ๊ธธ์ด๋ก ์๋ผ๋
๋๋ค.
์ํ์ค ์(๋๋ ์ํ์ค ์์ ๋ฐฐ์น)๊ฐ ์ ๊ณต๋ ๊ฒฝ์ฐ ์์ ๋ ๋ฒ์งธ ๋ฌธ์ฅ๋ง ์๋ผ๋
๋๋ค.
- `'only_first'`: `max_length` ์ธ์๊ฐ ์ง์ ํ ์ต๋ ๊ธธ์ด๋ก ์๋ผ๋ด๊ฑฐ๋,
`max_length`๊ฐ ์ ๊ณต๋์ง ์์ ๊ฒฝ์ฐ(`max_length=None`) ๋ชจ๋ธ์์ ํ์ฉ๋๋ ์ต๋ ๊ธธ์ด๋ก ์๋ผ๋
๋๋ค.
์ํ์ค ์(๋๋ ์ํ์ค ์์ ๋ฐฐ์น)๊ฐ ์ ๊ณต๋ ๊ฒฝ์ฐ ์์ ์ฒซ ๋ฒ์งธ ๋ฌธ์ฅ๋ง ์๋ผ๋
๋๋ค.
- `False` ๋๋ `'do_not_truncate'`: ์๋ผ๋ด๊ธฐ๋ฅผ ์ ์ฉํ์ง ์์ต๋๋ค. ์ด๊ฒ์ด ๊ธฐ๋ณธ ๋์์
๋๋ค.
`max_length` ์ธ์๋ ํจ๋ฉ ๋ฐ ์๋ผ๋ด๊ธฐ๋ฅผ ์ ์ฉํ ๊ธธ์ด๋ฅผ ์ ์ดํฉ๋๋ค. ์ด ์ธ์๋ ์ ์ ๋๋ `None`์ผ ์ ์์ผ๋ฉฐ, `None`์ผ ๊ฒฝ์ฐ ๋ชจ๋ธ์ด ํ์ฉํ ์ ์๋ ์ต๋ ๊ธธ์ด๋ก ๊ธฐ๋ณธ๊ฐ์ด ์ค์ ๋ฉ๋๋ค. ๋ชจ๋ธ์ ํน์ ํ ์ต๋ ์
๋ ฅ ๊ธธ์ด๊ฐ ์๋ ๊ฒฝ์ฐ `max_length`์ ๋ํ ์๋ผ๋ด๊ธฐ ๋๋ ํจ๋ฉ์ด ๋นํ์ฑํ๋ฉ๋๋ค.
๋ค์ ํ์๋ ํจ๋ฉ ๋ฐ ์๋ผ๋ด๊ธฐ๋ฅผ ์ค์ ํ๋ ๊ถ์ฅ ๋ฐฉ๋ฒ์ด ์์ฝ๋์ด ์์ต๋๋ค.
์
๋ ฅ์ผ๋ก ์ํ์ค ์์ ์ฌ์ฉํ๋ ๊ฒฝ์ฐ, ๋ค์ ์์ ์์ `truncation=True`๋ฅผ `['only_first', 'only_second', 'longest_first']`์์ ์ ํํ `STRATEGY`, ์ฆ `truncation='only_second'` ๋๋ `truncation='longest_first'`๋ก ๋ฐ๊พธ๋ฉด ์์ ์ค๋ช
ํ ๋๋ก ์์ ๋ ์ํ์ค๊ฐ ์๋ฆฌ๋ ๋ฐฉ์์ ์ ์ดํ ์ ์์ต๋๋ค.
| ์๋ผ๋ด๊ธฐ | ํจ๋ฉ | ์ฌ์ฉ ๋ฐฉ๋ฒ |
|--------------------------------------|-----------------------------------|------------------------------------------------------------------------------------------|
| ์๋ผ๋ด๊ธฐ ์์ | ํจ๋ฉ ์์ | `tokenizer(batch_sentences)` |
| | ๋ฐฐ์น ๋ด ์ต๋ ๊ธธ์ด๋ก ํจ๋ฉ | `tokenizer(batch_sentences, padding=True)` ๋๋ |
| | | `tokenizer(batch_sentences, padding='longest')` |
| | ๋ชจ๋ธ์ ์ต๋ ์
๋ ฅ ๊ธธ์ด๋ก ํจ๋ฉ | `tokenizer(batch_sentences, padding='max_length')` |
| | ํน์ ๊ธธ์ด๋ก ํจ๋ฉ | `tokenizer(batch_sentences, padding='max_length', max_length=42)` |
| | ๋ค์ํ ๊ธธ์ด๋ก ํจ๋ฉ | `tokenizer(batch_sentences, padding=True, pad_to_multiple_of=8) |
| ๋ชจ๋ธ์ ์ต๋ ์
๋ ฅ ๊ธธ์ด๋ก ์๋ผ๋ด๊ธฐ | ํจ๋ฉ ์์ | `tokenizer(batch_sentences, truncation=True)` ๋๋ |
| | | `tokenizer(batch_sentences, truncation=STRATEGY)` |
| | ๋ฐฐ์น ๋ด ์ต๋ ๊ธธ์ด๋ก ํจ๋ฉ | `tokenizer(batch_sentences, padding=True, truncation=True)` ๋๋ |
| | | `tokenizer(batch_sentences, padding=True, truncation=STRATEGY)` |
| | ๋ชจ๋ธ์ ์ต๋ ์
๋ ฅ ๊ธธ์ด๋ก ํจ๋ฉ | `tokenizer(batch_sentences, padding='max_length', truncation=True)` ๋๋ |
| | | `tokenizer(batch_sentences, padding='max_length', truncation=STRATEGY)` |
| | ํน์ ๊ธธ์ด๋ก ํจ๋ฉ | ์ฌ์ฉ ๋ถ๊ฐ |
| ํน์ ๊ธธ์ด๋ก ์๋ผ๋ด๊ธฐ | ํจ๋ฉ ์์ | `tokenizer(batch_sentences, truncation=True, max_length=42)` ๋๋ |
| | | `tokenizer(batch_sentences, truncation=STRATEGY, max_length=42)` |
| | ๋ฐฐ์น ๋ด ์ต๋ ๊ธธ์ด๋ก ํจ๋ฉ | `tokenizer(batch_sentences, padding=True, truncation=True, max_length=42)` ๋๋ |
| | | `tokenizer(batch_sentences, padding=True, truncation=STRATEGY, max_length=42)` |
| | ๋ชจ๋ธ์ ์ต๋ ์
๋ ฅ ๊ธธ์ด๋ก ํจ๋ฉ | ์ฌ์ฉ ๋ถ๊ฐ |
| | ํน์ ๊ธธ์ด๋ก ํจ๋ฉ | `tokenizer(batch_sentences, padding='max_length', truncation=True, max_length=42)` ๋๋ |
| | | `tokenizer(batch_sentences, padding='max_length', truncation=STRATEGY, max_length=42)` |
| transformers/docs/source/ko/pad_truncation.md/0 | {
"file_path": "transformers/docs/source/ko/pad_truncation.md",
"repo_id": "transformers",
"token_count": 5964
} | 271 |
<!--Copyright 2023 The HuggingFace Team. All rights reserved.
Licensed under the Apache License, Version 2.0 (the "License"); you may not use this file except in compliance with
the License. You may obtain a copy of the License at
http://www.apache.org/licenses/LICENSE-2.0
Unless required by applicable law or agreed to in writing, software distributed under the License is distributed on
an "AS IS" BASIS, WITHOUT WARRANTIES OR CONDITIONS OF ANY KIND, either express or implied. See the License for the
specific language governing permissions and limitations under the License.
โ ๏ธ Note that this file is in Markdown but contain specific syntax for our doc-builder (similar to MDX) that may not be
rendered properly in your Markdown viewer.
-->
# ์ ์ฒ๋ฆฌ[[preprocess]]
[[open-in-colab]]
๋ชจ๋ธ์ ํ๋ จํ๋ ค๋ฉด ๋ฐ์ดํฐ ์ธํธ๋ฅผ ๋ชจ๋ธ์ ๋ง๋ ์
๋ ฅ ํ์์ผ๋ก ์ ์ฒ๋ฆฌํด์ผ ํฉ๋๋ค. ํ
์คํธ, ์ด๋ฏธ์ง ๋๋ ์ค๋์ค์ธ์ง ๊ด๊ณ์์ด ๋ฐ์ดํฐ๋ฅผ ํ
์ ๋ฐฐ์น๋ก ๋ณํํ๊ณ ์กฐ๋ฆฝํ ํ์๊ฐ ์์ต๋๋ค. ๐ค Transformers๋ ๋ชจ๋ธ์ ๋ํ ๋ฐ์ดํฐ๋ฅผ ์ค๋นํ๋ ๋ฐ ๋์์ด ๋๋ ์ผ๋ จ์ ์ ์ฒ๋ฆฌ ํด๋์ค๋ฅผ ์ ๊ณตํฉ๋๋ค. ์ด ํํ ๋ฆฌ์ผ์์๋ ๋ค์ ๋ด์ฉ์ ๋ฐฐ์ธ ์ ์์ต๋๋ค:
* ํ
์คํธ๋ [Tokenizer](./main_classes/tokenizer)๋ฅผ ์ฌ์ฉํ์ฌ ํ ํฐ ์ํ์ค๋ก ๋ณํํ๊ณ ํ ํฐ์ ์ซ์ ํํ์ ๋ง๋ ํ ํ
์๋ก ์กฐ๋ฆฝํฉ๋๋ค.
* ์์ฑ ๋ฐ ์ค๋์ค๋ [Feature extractor](./main_classes/feature_extractor)๋ฅผ ์ฌ์ฉํ์ฌ ์ค๋์ค ํํ์์ ์ํ์ค ํน์ฑ์ ํ์
ํ์ฌ ํ
์๋ก ๋ณํํฉ๋๋ค.
* ์ด๋ฏธ์ง ์
๋ ฅ์ [ImageProcessor](./main_classes/image)์ ์ฌ์ฉํ์ฌ ์ด๋ฏธ์ง๋ฅผ ํ
์๋ก ๋ณํํฉ๋๋ค.
* ๋ฉํฐ๋ชจ๋ฌ ์
๋ ฅ์ [Processor](./main_classes/processors)์ ์ฌ์ฉํ์ฌ ํ ํฌ๋์ด์ ์ ํน์ฑ ์ถ์ถ๊ธฐ ๋๋ ์ด๋ฏธ์ง ํ๋ก์ธ์๋ฅผ ๊ฒฐํฉํฉ๋๋ค.
<Tip>
`AutoProcessor`๋ **์ธ์ ๋** ์๋ํ์ฌ ํ ํฌ๋์ด์ , ์ด๋ฏธ์ง ํ๋ก์ธ์, ํน์ฑ ์ถ์ถ๊ธฐ ๋๋ ํ๋ก์ธ์ ๋ฑ ์ฌ์ฉ ์ค์ธ ๋ชจ๋ธ์ ๋ง๋ ํด๋์ค๋ฅผ ์๋์ผ๋ก ์ ํํฉ๋๋ค.
</Tip>
์์ํ๊ธฐ ์ ์ ๐ค Datasets๋ฅผ ์ค์นํ์ฌ ์คํ์ ์ฌ์ฉํ ๋ฐ์ดํฐ๋ฅผ ๋ถ๋ฌ์ฌ ์ ์์ต๋๋ค:
```bash
pip install datasets
```
## ์์ฐ์ด์ฒ๋ฆฌ[[natural-language-processing]]
<Youtube id="Yffk5aydLzg"/>
ํ
์คํธ ๋ฐ์ดํฐ๋ฅผ ์ ์ฒ๋ฆฌํ๊ธฐ ์ํ ๊ธฐ๋ณธ ๋๊ตฌ๋ [tokenizer](main_classes/tokenizer)์
๋๋ค. ํ ํฌ๋์ด์ ๋ ์ผ๋ จ์ ๊ท์น์ ๋ฐ๋ผ ํ
์คํธ๋ฅผ *ํ ํฐ*์ผ๋ก ๋๋๋๋ค. ํ ํฐ์ ์ซ์๋ก ๋ณํ๋๊ณ ํ
์๋ ๋ชจ๋ธ ์
๋ ฅ์ด ๋ฉ๋๋ค. ๋ชจ๋ธ์ ํ์ํ ์ถ๊ฐ ์
๋ ฅ์ ํ ํฌ๋์ด์ ์ ์ํด ์ถ๊ฐ๋ฉ๋๋ค.
<Tip>
์ฌ์ ํ๋ จ๋ ๋ชจ๋ธ์ ์ฌ์ฉํ ๊ณํ์ด๋ผ๋ฉด ๋ชจ๋ธ๊ณผ ํจ๊ป ์ฌ์ ํ๋ จ๋ ํ ํฌ๋์ด์ ๋ฅผ ์ฌ์ฉํ๋ ๊ฒ์ด ์ค์ํฉ๋๋ค. ์ด๋ ๊ฒ ํ๋ฉด ํ
์คํธ๊ฐ ์ฌ์ ํ๋ จ ๋ง๋ญ์น์ ๋์ผํ ๋ฐฉ์์ผ๋ก ๋ถํ ๋๊ณ ์ฌ์ ํ๋ จ ์ค์ ๋์ผํ ํด๋น ํ ํฐ-์ธ๋ฑ์ค ์(์ผ๋ฐ์ ์ผ๋ก *vocab*์ด๋ผ๊ณ ํจ)์ ์ฌ์ฉํฉ๋๋ค.
</Tip>
์์ํ๋ ค๋ฉด [`AutoTokenizer.from_pretrained`] ๋ฉ์๋๋ฅผ ์ฌ์ฉํ์ฌ ์ฌ์ ํ๋ จ๋ ํ ํฌ๋์ด์ ๋ฅผ ๋ถ๋ฌ์ค์ธ์. ๋ชจ๋ธ๊ณผ ํจ๊ป ์ฌ์ ํ๋ จ๋ *vocab*์ ๋ค์ด๋ก๋ํฉ๋๋ค:
```py
>>> from transformers import AutoTokenizer
>>> tokenizer = AutoTokenizer.from_pretrained("bert-base-cased")
```
๊ทธ ๋ค์์ผ๋ก ํ
์คํธ๋ฅผ ํ ํฌ๋์ด์ ์ ๋ฃ์ด์ฃผ์ธ์:
```py
>>> encoded_input = tokenizer("Do not meddle in the affairs of wizards, for they are subtle and quick to anger.")
>>> print(encoded_input)
{'input_ids': [101, 2079, 2025, 19960, 10362, 1999, 1996, 3821, 1997, 16657, 1010, 2005, 2027, 2024, 11259, 1998, 4248, 2000, 4963, 1012, 102],
'token_type_ids': [0, 0, 0, 0, 0, 0, 0, 0, 0, 0, 0, 0, 0, 0, 0, 0, 0, 0, 0, 0, 0],
'attention_mask': [1, 1, 1, 1, 1, 1, 1, 1, 1, 1, 1, 1, 1, 1, 1, 1, 1, 1, 1, 1, 1]}
```
ํ ํฌ๋์ด์ ๋ ์ธ ๊ฐ์ง ์ค์ํ ํญ๋ชฉ์ ํฌํจํ ๋์
๋๋ฆฌ๋ฅผ ๋ฐํํฉ๋๋ค:
* [input_ids](glossary#input-ids)๋ ๋ฌธ์ฅ์ ๊ฐ ํ ํฐ์ ํด๋นํ๋ ์ธ๋ฑ์ค์
๋๋ค.
* [attention_mask](glossary#attention-mask)๋ ํ ํฐ์ ์ฒ๋ฆฌํด์ผ ํ๋์ง ์ฌ๋ถ๋ฅผ ๋ํ๋
๋๋ค.
* [token_type_ids](glossary#token-type-ids)๋ ๋ ๊ฐ ์ด์์ ์ํ์ค๊ฐ ์์ ๋ ํ ํฐ์ด ์ํ ์ํ์ค๋ฅผ ์๋ณํฉ๋๋ค.
`input_ids`๋ฅผ ๋์ฝ๋ฉํ์ฌ ์
๋ ฅ์ ๋ฐํํฉ๋๋ค:
```py
>>> tokenizer.decode(encoded_input["input_ids"])
'[CLS] Do not meddle in the affairs of wizards, for they are subtle and quick to anger. [SEP]'
```
ํ ํฌ๋์ด์ ๊ฐ ๋ ๊ฐ์ ํน์ํ ํ ํฐ(๋ถ๋ฅ ํ ํฐ `CLS`์ ๋ถํ ํ ํฐ `SEP`)์ ๋ฌธ์ฅ์ ์ถ๊ฐํ์ต๋๋ค.
๋ชจ๋ ๋ชจ๋ธ์ ํน์ํ ํ ํฐ์ด ํ์ํ ๊ฒ์ ์๋์ง๋ง, ํ์ํ๋ค๋ฉด ํ ํฌ๋์ด์ ๊ฐ ์๋์ผ๋ก ์ถ๊ฐํฉ๋๋ค.
์ ์ฒ๋ฆฌํ ๋ฌธ์ฅ์ด ์ฌ๋ฌ ๊ฐ ์๋ ๊ฒฝ์ฐ์๋ ๋ฆฌ์คํธ๋ก ํ ํฌ๋์ด์ ์ ์ ๋ฌํฉ๋๋ค:
```py
>>> batch_sentences = [
... "But what about second breakfast?",
... "Don't think he knows about second breakfast, Pip.",
... "What about elevensies?",
... ]
>>> encoded_inputs = tokenizer(batch_sentences)
>>> print(encoded_inputs)
{'input_ids': [[101, 1252, 1184, 1164, 1248, 6462, 136, 102],
[101, 1790, 112, 189, 1341, 1119, 3520, 1164, 1248, 6462, 117, 21902, 1643, 119, 102],
[101, 1327, 1164, 5450, 23434, 136, 102]],
'token_type_ids': [[0, 0, 0, 0, 0, 0, 0, 0],
[0, 0, 0, 0, 0, 0, 0, 0, 0, 0, 0, 0, 0, 0, 0],
[0, 0, 0, 0, 0, 0, 0]],
'attention_mask': [[1, 1, 1, 1, 1, 1, 1, 1],
[1, 1, 1, 1, 1, 1, 1, 1, 1, 1, 1, 1, 1, 1, 1],
[1, 1, 1, 1, 1, 1, 1]]}
```
### ํจ๋ฉ[[pad]]
๋ชจ๋ธ ์
๋ ฅ์ธ ํ
์๋ ๋ชจ์์ด ๊ท ์ผํด์ผ ํ์ง๋ง, ๋ฌธ์ฅ์ ๊ธธ์ด๊ฐ ํญ์ ๊ฐ์ง๋ ์๊ธฐ ๋๋ฌธ์ ๋ฌธ์ ๊ฐ ๋ ์ ์์ต๋๋ค. ํจ๋ฉ์ ์งง์ ๋ฌธ์ฅ์ ํน์ํ *ํจ๋ฉ ํ ํฐ*์ ์ถ๊ฐํ์ฌ ํ
์๋ฅผ ์ง์ฌ๊ฐํ ๋ชจ์์ด ๋๋๋ก ํ๋ ์ ๋ต์
๋๋ค.
`padding` ๋งค๊ฐ๋ณ์๋ฅผ `True`๋ก ์ค์ ํ์ฌ ๋ฐฐ์น ๋ด์ ์งง์ ์ํ์ค๋ฅผ ๊ฐ์ฅ ๊ธด ์ํ์ค์ ๋ง์ถฐ ํจ๋ฉํฉ๋๋ค.
```py
>>> batch_sentences = [
... "But what about second breakfast?",
... "Don't think he knows about second breakfast, Pip.",
... "What about elevensies?",
... ]
>>> encoded_input = tokenizer(batch_sentences, padding=True)
>>> print(encoded_input)
{'input_ids': [[101, 1252, 1184, 1164, 1248, 6462, 136, 102, 0, 0, 0, 0, 0, 0, 0],
[101, 1790, 112, 189, 1341, 1119, 3520, 1164, 1248, 6462, 117, 21902, 1643, 119, 102],
[101, 1327, 1164, 5450, 23434, 136, 102, 0, 0, 0, 0, 0, 0, 0, 0]],
'token_type_ids': [[0, 0, 0, 0, 0, 0, 0, 0, 0, 0, 0, 0, 0, 0, 0],
[0, 0, 0, 0, 0, 0, 0, 0, 0, 0, 0, 0, 0, 0, 0],
[0, 0, 0, 0, 0, 0, 0, 0, 0, 0, 0, 0, 0, 0, 0]],
'attention_mask': [[1, 1, 1, 1, 1, 1, 1, 1, 0, 0, 0, 0, 0, 0, 0],
[1, 1, 1, 1, 1, 1, 1, 1, 1, 1, 1, 1, 1, 1, 1],
[1, 1, 1, 1, 1, 1, 1, 0, 0, 0, 0, 0, 0, 0, 0]]}
```
๊ธธ์ด๊ฐ ์งง์ ์ฒซ ๋ฌธ์ฅ๊ณผ ์ธ ๋ฒ์งธ ๋ฌธ์ฅ์ด ์ด์ `0`์ผ๋ก ์ฑ์์ก์ต๋๋ค.
### ์๋ผ๋ด๊ธฐ[[truncation]]
ํํธ, ๋๋ก๋ ์ํ์ค๊ฐ ๋ชจ๋ธ์์ ์ฒ๋ฆฌํ๊ธฐ์ ๋๋ฌด ๊ธธ ์๋ ์์ต๋๋ค. ์ด ๊ฒฝ์ฐ, ์ํ์ค๋ฅผ ๋ ์งง๊ฒ ์ค์ผ ํ์๊ฐ ์์ต๋๋ค.
๋ชจ๋ธ์์ ํ์ฉํ๋ ์ต๋ ๊ธธ์ด๋ก ์ํ์ค๋ฅผ ์๋ฅด๋ ค๋ฉด `truncation` ๋งค๊ฐ๋ณ์๋ฅผ `True`๋ก ์ค์ ํ์ธ์:
```py
>>> batch_sentences = [
... "But what about second breakfast?",
... "Don't think he knows about second breakfast, Pip.",
... "What about elevensies?",
... ]
>>> encoded_input = tokenizer(batch_sentences, padding=True, truncation=True)
>>> print(encoded_input)
{'input_ids': [[101, 1252, 1184, 1164, 1248, 6462, 136, 102, 0, 0, 0, 0, 0, 0, 0],
[101, 1790, 112, 189, 1341, 1119, 3520, 1164, 1248, 6462, 117, 21902, 1643, 119, 102],
[101, 1327, 1164, 5450, 23434, 136, 102, 0, 0, 0, 0, 0, 0, 0, 0]],
'token_type_ids': [[0, 0, 0, 0, 0, 0, 0, 0, 0, 0, 0, 0, 0, 0, 0],
[0, 0, 0, 0, 0, 0, 0, 0, 0, 0, 0, 0, 0, 0, 0],
[0, 0, 0, 0, 0, 0, 0, 0, 0, 0, 0, 0, 0, 0, 0]],
'attention_mask': [[1, 1, 1, 1, 1, 1, 1, 1, 0, 0, 0, 0, 0, 0, 0],
[1, 1, 1, 1, 1, 1, 1, 1, 1, 1, 1, 1, 1, 1, 1],
[1, 1, 1, 1, 1, 1, 1, 0, 0, 0, 0, 0, 0, 0, 0]]}
```
<Tip>
๋ค์ํ ํจ๋ฉ๊ณผ ์๋ผ๋ด๊ธฐ ์ธ์์ ๋ํด ๋ ์์๋ณด๋ ค๋ฉด [ํจ๋ฉ๊ณผ ์๋ผ๋ด๊ธฐ](./pad_truncation) ๊ฐ๋
๊ฐ์ด๋๋ฅผ ํ์ธํด๋ณด์ธ์.
</Tip>
### ํ
์ ๋ง๋ค๊ธฐ[[build-tensors]]
๋ง์ง๋ง์ผ๋ก, ํ ํฌ๋์ด์ ๊ฐ ๋ชจ๋ธ์ ๊ณต๊ธ๋๋ ์ค์ ํ
์๋ฅผ ๋ฐํํ๋๋ก ํฉ๋๋ค.
`return_tensors` ๋งค๊ฐ๋ณ์๋ฅผ PyTorch์ ๊ฒฝ์ฐ `pt`, TensorFlow์ ๊ฒฝ์ฐ `tf`๋ก ์ค์ ํ์ธ์:
<frameworkcontent>
<pt>
```py
>>> batch_sentences = [
... "But what about second breakfast?",
... "Don't think he knows about second breakfast, Pip.",
... "What about elevensies?",
... ]
>>> encoded_input = tokenizer(batch_sentences, padding=True, truncation=True, return_tensors="pt")
>>> print(encoded_input)
{'input_ids': tensor([[101, 1252, 1184, 1164, 1248, 6462, 136, 102, 0, 0, 0, 0, 0, 0, 0],
[101, 1790, 112, 189, 1341, 1119, 3520, 1164, 1248, 6462, 117, 21902, 1643, 119, 102],
[101, 1327, 1164, 5450, 23434, 136, 102, 0, 0, 0, 0, 0, 0, 0, 0]]),
'token_type_ids': tensor([[0, 0, 0, 0, 0, 0, 0, 0, 0, 0, 0, 0, 0, 0, 0],
[0, 0, 0, 0, 0, 0, 0, 0, 0, 0, 0, 0, 0, 0, 0],
[0, 0, 0, 0, 0, 0, 0, 0, 0, 0, 0, 0, 0, 0, 0]]),
'attention_mask': tensor([[1, 1, 1, 1, 1, 1, 1, 1, 0, 0, 0, 0, 0, 0, 0],
[1, 1, 1, 1, 1, 1, 1, 1, 1, 1, 1, 1, 1, 1, 1],
[1, 1, 1, 1, 1, 1, 1, 0, 0, 0, 0, 0, 0, 0, 0]])}
```
</pt>
<tf>
```py
>>> batch_sentences = [
... "But what about second breakfast?",
... "Don't think he knows about second breakfast, Pip.",
... "What about elevensies?",
... ]
>>> encoded_input = tokenizer(batch_sentences, padding=True, truncation=True, return_tensors="tf")
>>> print(encoded_input)
{'input_ids': <tf.Tensor: shape=(2, 9), dtype=int32, numpy=
array([[101, 1252, 1184, 1164, 1248, 6462, 136, 102, 0, 0, 0, 0, 0, 0, 0],
[101, 1790, 112, 189, 1341, 1119, 3520, 1164, 1248, 6462, 117, 21902, 1643, 119, 102],
[101, 1327, 1164, 5450, 23434, 136, 102, 0, 0, 0, 0, 0, 0, 0, 0]],
dtype=int32)>,
'token_type_ids': <tf.Tensor: shape=(2, 9), dtype=int32, numpy=
array([[0, 0, 0, 0, 0, 0, 0, 0, 0, 0, 0, 0, 0, 0, 0],
[0, 0, 0, 0, 0, 0, 0, 0, 0, 0, 0, 0, 0, 0, 0],
[0, 0, 0, 0, 0, 0, 0, 0, 0, 0, 0, 0, 0, 0, 0]], dtype=int32)>,
'attention_mask': <tf.Tensor: shape=(2, 9), dtype=int32, numpy=
array([[1, 1, 1, 1, 1, 1, 1, 1, 0, 0, 0, 0, 0, 0, 0],
[1, 1, 1, 1, 1, 1, 1, 1, 1, 1, 1, 1, 1, 1, 1],
[1, 1, 1, 1, 1, 1, 1, 0, 0, 0, 0, 0, 0, 0, 0]], dtype=int32)>}
```
</tf>
</frameworkcontent>
## ์ค๋์ค[[audio]]
์ค๋์ค ์์
์ ๋ชจ๋ธ์ ๋ง๋ ๋ฐ์ดํฐ ์ธํธ๋ฅผ ์ค๋นํ๊ธฐ ์ํด [ํน์ฑ ์ถ์ถ๊ธฐ](main_classes/feature_extractor)๊ฐ ํ์ํฉ๋๋ค. ํน์ฑ ์ถ์ถ๊ธฐ๋ ์์ ์ค๋์ค ๋ฐ์ดํฐ์์ ํน์ฑ๋ฅผ ์ถ์ถํ๊ณ ์ด๋ฅผ ํ
์๋ก ๋ณํํ๋ ๊ฒ์ด ๋ชฉ์ ์
๋๋ค.
์ค๋์ค ๋ฐ์ดํฐ ์ธํธ์ ํน์ฑ ์ถ์ถ๊ธฐ๋ฅผ ์ฌ์ฉํ๋ ๋ฐฉ๋ฒ์ ๋ณด๊ธฐ ์ํด [MInDS-14](https://huggingface.co/datasets/PolyAI/minds14) ๋ฐ์ดํฐ ์ธํธ๋ฅผ ๊ฐ์ ธ์ค์ธ์. (๋ฐ์ดํฐ ์ธํธ๋ฅผ ๊ฐ์ ธ์ค๋ ๋ฐฉ๋ฒ์ ๐ค [๋ฐ์ดํฐ ์ธํธ ํํ ๋ฆฌ์ผ](https://huggingface.co/docs/datasets/load_hub)์์ ์์ธํ ์ค๋ช
ํ๊ณ ์์ต๋๋ค.)
```py
>>> from datasets import load_dataset, Audio
>>> dataset = load_dataset("PolyAI/minds14", name="en-US", split="train")
```
`audio` ์ด์ ์ฒซ ๋ฒ์งธ ์์์ ์ ๊ทผํ์ฌ ์
๋ ฅ์ ์ดํด๋ณด์ธ์. `audio` ์ด์ ํธ์ถํ๋ฉด ์ค๋์ค ํ์ผ์ ์๋์ผ๋ก ๊ฐ์ ธ์ค๊ณ ๋ฆฌ์ํ๋งํฉ๋๋ค.
```py
>>> dataset[0]["audio"]
{'array': array([ 0. , 0.00024414, -0.00024414, ..., -0.00024414,
0. , 0. ], dtype=float32),
'path': '/root/.cache/huggingface/datasets/downloads/extracted/f14948e0e84be638dd7943ac36518a4cf3324e8b7aa331c5ab11541518e9368c/en-US~JOINT_ACCOUNT/602ba55abb1e6d0fbce92065.wav',
'sampling_rate': 8000}
```
์ด๋ ๊ฒ ํ๋ฉด ์ธ ๊ฐ์ง ํญ๋ชฉ์ด ๋ฐํ๋ฉ๋๋ค:
* `array`๋ 1D ๋ฐฐ์ด๋ก ๊ฐ์ ธ์์ (ํ์ํ ๊ฒฝ์ฐ) ๋ฆฌ์ํ๋ง๋ ์์ฑ ์ ํธ์
๋๋ค.
* `path`๋ ์ค๋์ค ํ์ผ์ ์์น๋ฅผ ๊ฐ๋ฆฌํต๋๋ค.
* `sampling_rate`๋ ์์ฑ ์ ํธ์์ ์ด๋น ์ธก์ ๋๋ ๋ฐ์ดํฐ ํฌ์ธํธ ์๋ฅผ ๋ํ๋
๋๋ค.
์ด ํํ ๋ฆฌ์ผ์์๋ [Wav2Vec2](https://huggingface.co/facebook/wav2vec2-base) ๋ชจ๋ธ์ ์ฌ์ฉํฉ๋๋ค. ๋ชจ๋ธ ์นด๋๋ฅผ ๋ณด๋ฉด Wav2Vec2๊ฐ 16kHz ์ํ๋ง๋ ์์ฑ ์ค๋์ค๋ฅผ ๊ธฐ๋ฐ์ผ๋ก ์ฌ์ ํ๋ จ๋ ๊ฒ์ ์ ์ ์์ต๋๋ค.
๋ชจ๋ธ์ ์ฌ์ ํ๋ จํ๋ ๋ฐ ์ฌ์ฉ๋ ๋ฐ์ดํฐ ์ธํธ์ ์ํ๋ง ๋ ์ดํธ์ ์ค๋์ค ๋ฐ์ดํฐ์ ์ํ๋ง ๋ ์ดํธ๊ฐ ์ผ์นํด์ผ ํฉ๋๋ค. ๋ฐ์ดํฐ์ ์ํ๋ง ๋ ์ดํธ๊ฐ ๋ค๋ฅด๋ฉด ๋ฐ์ดํฐ๋ฅผ ๋ฆฌ์ํ๋งํด์ผ ํฉ๋๋ค.
1. ๐ค Datasets์ [`~datasets.Dataset.cast_column`] ๋ฉ์๋๋ฅผ ์ฌ์ฉํ์ฌ ์ํ๋ง ๋ ์ดํธ๋ฅผ 16kHz๋ก ์
์ํ๋งํ์ธ์:
```py
>>> dataset = dataset.cast_column("audio", Audio(sampling_rate=16_000))
```
2. ์ค๋์ค ํ์ผ์ ๋ฆฌ์ํ๋งํ๊ธฐ ์ํด `audio` ์ด์ ๋ค์ ํธ์ถํฉ๋๋ค:
```py
>>> dataset[0]["audio"]
{'array': array([ 2.3443763e-05, 2.1729663e-04, 2.2145823e-04, ...,
3.8356509e-05, -7.3497440e-06, -2.1754686e-05], dtype=float32),
'path': '/root/.cache/huggingface/datasets/downloads/extracted/f14948e0e84be638dd7943ac36518a4cf3324e8b7aa331c5ab11541518e9368c/en-US~JOINT_ACCOUNT/602ba55abb1e6d0fbce92065.wav',
'sampling_rate': 16000}
```
๋ค์์ผ๋ก, ์
๋ ฅ์ ์ ๊ทํํ๊ณ ํจ๋ฉํ ํน์ฑ ์ถ์ถ๊ธฐ๋ฅผ ๊ฐ์ ธ์ค์ธ์. ํ
์คํธ ๋ฐ์ดํฐ์ ๊ฒฝ์ฐ, ๋ ์งง์ ์ํ์ค์ ๋ํด `0`์ด ์ถ๊ฐ๋ฉ๋๋ค. ์ค๋์ค ๋ฐ์ดํฐ์๋ ๊ฐ์ ๊ฐ๋
์ด ์ ์ฉ๋ฉ๋๋ค.
ํน์ฑ ์ถ์ถ๊ธฐ๋ ๋ฐฐ์ด์ `0`(๋ฌต์์ผ๋ก ํด์)์ ์ถ๊ฐํฉ๋๋ค.
[`AutoFeatureExtractor.from_pretrained`]๋ฅผ ์ฌ์ฉํ์ฌ ํน์ฑ ์ถ์ถ๊ธฐ๋ฅผ ๊ฐ์ ธ์ค์ธ์:
```py
>>> from transformers import AutoFeatureExtractor
>>> feature_extractor = AutoFeatureExtractor.from_pretrained("facebook/wav2vec2-base")
```
์ค๋์ค `array`๋ฅผ ํน์ฑ ์ถ์ถ๊ธฐ์ ์ ๋ฌํ์ธ์. ๋ํ, ๋ฐ์ํ ์ ์๋ ์กฐ์ฉํ ์ค๋ฅ(silent errors)๋ฅผ ๋ ์ ๋๋ฒ๊น
ํ ์ ์๋๋ก ํน์ฑ ์ถ์ถ๊ธฐ์ `sampling_rate` ์ธ์๋ฅผ ์ถ๊ฐํ๋ ๊ฒ์ ๊ถ์ฅํฉ๋๋ค.
```py
>>> audio_input = [dataset[0]["audio"]["array"]]
>>> feature_extractor(audio_input, sampling_rate=16000)
{'input_values': [array([ 3.8106556e-04, 2.7506407e-03, 2.8015103e-03, ...,
5.6335266e-04, 4.6588284e-06, -1.7142107e-04], dtype=float32)]}
```
ํ ํฌ๋์ด์ ์ ๋ง์ฐฌ๊ฐ์ง๋ก ๋ฐฐ์น ๋ด์์ ๊ฐ๋ณ์ ์ธ ์ํ์ค๋ฅผ ์ฒ๋ฆฌํ๊ธฐ ์ํด ํจ๋ฉ ๋๋ ์๋ผ๋ด๊ธฐ๋ฅผ ์ ์ฉํ ์ ์์ต๋๋ค. ์ด ๋ ๊ฐ์ ์ค๋์ค ์ํ์ ์ํ์ค ๊ธธ์ด๋ฅผ ํ์ธํด๋ณด์ธ์:
```py
>>> dataset[0]["audio"]["array"].shape
(173398,)
>>> dataset[1]["audio"]["array"].shape
(106496,)
```
์ค๋์ค ์ํ์ ๊ธธ์ด๊ฐ ๋์ผํ๋๋ก ๋ฐ์ดํฐ ์ธํธ๋ฅผ ์ ์ฒ๋ฆฌํ๋ ํจ์๋ฅผ ๋ง๋์ธ์. ์ต๋ ์ํ ๊ธธ์ด๋ฅผ ์ง์ ํ๋ฉด ํน์ฑ ์ถ์ถ๊ธฐ๊ฐ ํด๋น ๊ธธ์ด์ ๋ง์ถฐ ์ํ์ค๋ฅผ ํจ๋ฉํ๊ฑฐ๋ ์๋ผ๋
๋๋ค:
```py
>>> def preprocess_function(examples):
... audio_arrays = [x["array"] for x in examples["audio"]]
... inputs = feature_extractor(
... audio_arrays,
... sampling_rate=16000,
... padding=True,
... max_length=100000,
... truncation=True,
... )
... return inputs
```
`preprocess_function`์ ๋ฐ์ดํฐ ์ธํธ์ ์ฒ์ ์์ ๋ช ๊ฐ์ ์ ์ฉํด๋ณด์ธ์:
```py
>>> processed_dataset = preprocess_function(dataset[:5])
```
์ด์ ์ํ ๊ธธ์ด๊ฐ ๋ชจ๋ ๊ฐ๊ณ ์ง์ ๋ ์ต๋ ๊ธธ์ด์ ๋ง๊ฒ ๋์์ต๋๋ค. ๋๋์ด ์ ์ฒ๋ฆฌ๋ ๋ฐ์ดํฐ ์ธํธ๋ฅผ ๋ชจ๋ธ์ ์ ๋ฌํ ์ ์์ต๋๋ค!
```py
>>> processed_dataset["input_values"][0].shape
(100000,)
>>> processed_dataset["input_values"][1].shape
(100000,)
```
## ์ปดํจํฐ ๋น์ [[computer-vision]]
์ปดํจํฐ ๋น์ ์์
์ ๊ฒฝ์ฐ, ๋ชจ๋ธ์ ๋ํ ๋ฐ์ดํฐ ์ธํธ๋ฅผ ์ค๋นํ๊ธฐ ์ํด [์ด๋ฏธ์ง ํ๋ก์ธ์](main_classes/image_processor)๊ฐ ํ์ํฉ๋๋ค.
์ด๋ฏธ์ง ์ ์ฒ๋ฆฌ๋ ์ด๋ฏธ์ง๋ฅผ ๋ชจ๋ธ์ด ์์ํ๋ ์
๋ ฅ์ผ๋ก ๋ณํํ๋ ์ฌ๋ฌ ๋จ๊ณ๋ก ์ด๋ฃจ์ด์ง๋๋ค.
์ด๋ฌํ ๋จ๊ณ์๋ ํฌ๊ธฐ ์กฐ์ , ์ ๊ทํ, ์์ ์ฑ๋ ๋ณด์ , ์ด๋ฏธ์ง์ ํ
์ ๋ณํ ๋ฑ์ด ํฌํจ๋ฉ๋๋ค.
<Tip>
์ด๋ฏธ์ง ์ ์ฒ๋ฆฌ๋ ์ด๋ฏธ์ง ์ฆ๊ฐ ๊ธฐ๋ฒ์ ๋ช ๊ฐ์ง ์ ์ฉํ ๋ค์ ํ ์๋ ์์ต๋๋ค.
์ด๋ฏธ์ง ์ ์ฒ๋ฆฌ ๋ฐ ์ด๋ฏธ์ง ์ฆ๊ฐ์ ๋ชจ๋ ์ด๋ฏธ์ง ๋ฐ์ดํฐ๋ฅผ ๋ณํํ์ง๋ง, ์๋ก ๋ค๋ฅธ ๋ชฉ์ ์ ๊ฐ์ง๊ณ ์์ต๋๋ค:
* ์ด๋ฏธ์ง ์ฆ๊ฐ์ ๊ณผ์ ํฉ(over-fitting)์ ๋ฐฉ์งํ๊ณ ๋ชจ๋ธ์ ๊ฒฌ๊ณ ํจ(resiliency)์ ๋์ด๋ ๋ฐ ๋์์ด ๋๋ ๋ฐฉ์์ผ๋ก ์ด๋ฏธ์ง๋ฅผ ์์ ํฉ๋๋ค.
๋ฐ๊ธฐ์ ์์ ์กฐ์ , ์๋ฅด๊ธฐ, ํ์ , ํฌ๊ธฐ ์กฐ์ , ํ๋/์ถ์ ๋ฑ ๋ค์ํ ๋ฐฉ๋ฒ์ผ๋ก ๋ฐ์ดํฐ๋ฅผ ์ฆ๊ฐํ ์ ์์ต๋๋ค.
๊ทธ๋ฌ๋ ์ฆ๊ฐ์ผ๋ก ์ด๋ฏธ์ง์ ์๋ฏธ๊ฐ ๋ฐ๋์ง ์๋๋ก ์ฃผ์ํด์ผ ํฉ๋๋ค.
* ์ด๋ฏธ์ง ์ ์ฒ๋ฆฌ๋ ์ด๋ฏธ์ง๊ฐ ๋ชจ๋ธ์ด ์์ํ๋ ์
๋ ฅ ํ์๊ณผ ์ผ์นํ๋๋ก ๋ณด์ฅํฉ๋๋ค.
์ปดํจํฐ ๋น์ ๋ชจ๋ธ์ ๋ฏธ์ธ ์กฐ์ ํ ๋ ์ด๋ฏธ์ง๋ ๋ชจ๋ธ์ด ์ด๊ธฐ์ ํ๋ จ๋ ๋์ ์ ํํ ๊ฐ์ ๋ฐฉ์์ผ๋ก ์ ์ฒ๋ฆฌ๋์ด์ผ ํฉ๋๋ค.
์ด๋ฏธ์ง ์ฆ๊ฐ์๋ ์ํ๋ ๋ผ์ด๋ธ๋ฌ๋ฆฌ๋ฅผ ๋ฌด์์ด๋ ์ฌ์ฉํ ์ ์์ต๋๋ค. ์ด๋ฏธ์ง ์ ์ฒ๋ฆฌ์๋ ๋ชจ๋ธ๊ณผ ์ฐ๊ฒฐ๋ `ImageProcessor`๋ฅผ ์ฌ์ฉํฉ๋๋ค.
</Tip>
[food101](https://huggingface.co/datasets/food101) ๋ฐ์ดํฐ ์ธํธ๋ฅผ ๊ฐ์ ธ์์ ์ปดํจํฐ ๋น์ ๋ฐ์ดํฐ ์ธํธ์์ ์ด๋ฏธ์ง ํ๋ก์ธ์๋ฅผ ์ด๋ป๊ฒ ์ฌ์ฉํ๋์ง ์์๋ณด์ธ์.
๋ฐ์ดํฐ ์ธํธ๋ฅผ ๋ถ๋ฌ์ค๋ ๋ฐฉ๋ฒ์ ๐ค [๋ฐ์ดํฐ ์ธํธ ํํ ๋ฆฌ์ผ](https://huggingface.co/docs/datasets/load_hub)์ ์ฐธ๊ณ ํ์ธ์.
<Tip>
๋ฐ์ดํฐ ์ธํธ๊ฐ ์๋นํ ํฌ๊ธฐ ๋๋ฌธ์ ๐ค Datasets์ `split` ๋งค๊ฐ๋ณ์๋ฅผ ์ฌ์ฉํ์ฌ ํ๋ จ ์ธํธ์์ ์์ ์ํ๋ง ๊ฐ์ ธ์ค์ธ์!
</Tip>
```py
>>> from datasets import load_dataset
>>> dataset = load_dataset("food101", split="train[:100]")
```
๋ค์์ผ๋ก, ๐ค Datasets์ [`image`](https://huggingface.co/docs/datasets/package_reference/main_classes?highlight=image#datasets.Image)๋ก ์ด๋ฏธ์ง๋ฅผ ํ์ธํด๋ณด์ธ์:
```py
>>> dataset[0]["image"]
```
<div class="flex justify-center">
<img src="https://huggingface.co/datasets/huggingface/documentation-images/resolve/main/vision-preprocess-tutorial.png"/>
</div>
[`AutoImageProcessor.from_pretrained`]๋ก ์ด๋ฏธ์ง ํ๋ก์ธ์๋ฅผ ๊ฐ์ ธ์ค์ธ์:
```py
>>> from transformers import AutoImageProcessor
>>> image_processor = AutoImageProcessor.from_pretrained("google/vit-base-patch16-224")
```
๋จผ์ ์ด๋ฏธ์ง ์ฆ๊ฐ ๋จ๊ณ๋ฅผ ์ถ๊ฐํด ๋ด
์๋ค. ์๋ฌด ๋ผ์ด๋ธ๋ฌ๋ฆฌ๋ ์ฌ์ฉํด๋ ๊ด์ฐฎ์ง๋ง, ์ด๋ฒ ํํ ๋ฆฌ์ผ์์๋ torchvision์ [`transforms`](https://pytorch.org/vision/stable/transforms.html) ๋ชจ๋์ ์ฌ์ฉํ๊ฒ ์ต๋๋ค.
๋ค๋ฅธ ๋ฐ์ดํฐ ์ฆ๊ฐ ๋ผ์ด๋ธ๋ฌ๋ฆฌ๋ฅผ ์ฌ์ฉํด๋ณด๊ณ ์ถ๋ค๋ฉด, [Albumentations](https://colab.research.google.com/github/huggingface/notebooks/blob/main/examples/image_classification_albumentations.ipynb) ๋๋ [Kornia notebooks](https://colab.research.google.com/github/huggingface/notebooks/blob/main/examples/image_classification_kornia.ipynb)์์ ์ด๋ป๊ฒ ์ฌ์ฉํ๋์ง ๋ฐฐ์ธ ์ ์์ต๋๋ค.
1. [`Compose`](https://pytorch.org/vision/master/generated/torchvision.transforms.Compose.html)๋ก [`RandomResizedCrop`](https://pytorch.org/vision/main/generated/torchvision.transforms.RandomResizedCrop.html)์ [`ColorJitter`](https://pytorch.org/vision/main/generated/torchvision.transforms.ColorJitter.html) ๋ฑ ๋ณํ์ ๋ช ๊ฐ์ง ์ฐ๊ฒฐํ์ธ์.
์ฐธ๊ณ ๋ก ํฌ๊ธฐ ์กฐ์ ์ ํ์ํ ์ด๋ฏธ์ง์ ํฌ๊ธฐ ์๊ตฌ์ฌํญ์ `image_processor`์์ ๊ฐ์ ธ์ฌ ์ ์์ต๋๋ค.
์ผ๋ถ ๋ชจ๋ธ์ ์ ํํ ๋์ด์ ๋๋น๋ฅผ ์๊ตฌํ์ง๋ง, ์ ์ผ ์งง์ ๋ณ์ ๊ธธ์ด(`shortest_edge`)๋ง ์ ์๋ ๋ชจ๋ธ๋ ์์ต๋๋ค.
```py
>>> from torchvision.transforms import RandomResizedCrop, ColorJitter, Compose
>>> size = (
... image_processor.size["shortest_edge"]
... if "shortest_edge" in image_processor.size
... else (image_processor.size["height"], image_processor.size["width"])
... )
>>> _transforms = Compose([RandomResizedCrop(size), ColorJitter(brightness=0.5, hue=0.5)])
```
2. ๋ชจ๋ธ์ ์
๋ ฅ์ผ๋ก [`pixel_values`](model_doc/visionencoderdecoder#transformers.VisionEncoderDecoderModel.forward.pixel_values)๋ฅผ ๋ฐ์ต๋๋ค.
`ImageProcessor`๋ ์ด๋ฏธ์ง ์ ๊ทํ ๋ฐ ์ ์ ํ ํ
์ ์์ฑ์ ์ฒ๋ฆฌํ ์ ์์ต๋๋ค.
๋ฐฐ์น ์ด๋ฏธ์ง์ ๋ํ ์ด๋ฏธ์ง ์ฆ๊ฐ ๋ฐ ์ด๋ฏธ์ง ์ ์ฒ๋ฆฌ๋ฅผ ๊ฒฐํฉํ๊ณ `pixel_values`๋ฅผ ์์ฑํ๋ ํจ์๋ฅผ ๋ง๋ญ๋๋ค:
```py
>>> def transforms(examples):
... images = [_transforms(img.convert("RGB")) for img in examples["image"]]
... examples["pixel_values"] = image_processor(images, do_resize=False, return_tensors="pt")["pixel_values"]
... return examples
```
<Tip>
์์ ์์์๋ ์ด๋ฏธ์ง ์ฆ๊ฐ ์ค์ ์ด๋ฏธ์ง ํฌ๊ธฐ๋ฅผ ์กฐ์ ํ๊ธฐ ๋๋ฌธ์ `do_resize=False`๋ก ์ค์ ํ๊ณ , ํด๋น `image_processor`์์ `size` ์์ฑ์ ํ์ฉํ์ต๋๋ค.
์ด๋ฏธ์ง ์ฆ๊ฐ ์ค์ ์ด๋ฏธ์ง ํฌ๊ธฐ๋ฅผ ์กฐ์ ํ์ง ์์ ๊ฒฝ์ฐ ์ด ๋งค๊ฐ๋ณ์๋ฅผ ์๋ตํ์ธ์.
๊ธฐ๋ณธ์ ์ผ๋ก๋ `ImageProcessor`๊ฐ ํฌ๊ธฐ ์กฐ์ ์ ์ฒ๋ฆฌํฉ๋๋ค.
์ฆ๊ฐ ๋ณํ ๊ณผ์ ์์ ์ด๋ฏธ์ง๋ฅผ ์ ๊ทํํ๋ ค๋ฉด `image_processor.image_mean` ๋ฐ `image_processor.image_std` ๊ฐ์ ์ฌ์ฉํ์ธ์.
</Tip>
3. ๐ค Datasets์ [`set_transform`](https://huggingface.co/docs/datasets/process#format-transform)๋ฅผ ์ฌ์ฉํ์ฌ ์ค์๊ฐ์ผ๋ก ๋ณํ์ ์ ์ฉํฉ๋๋ค:
```py
>>> dataset.set_transform(transforms)
```
4. ์ด์ ์ด๋ฏธ์ง์ ์ ๊ทผํ๋ฉด ์ด๋ฏธ์ง ํ๋ก์ธ์๊ฐ `pixel_values`๋ฅผ ์ถ๊ฐํ ๊ฒ์ ์ ์ ์์ต๋๋ค.
๋๋์ด ์ฒ๋ฆฌ๋ ๋ฐ์ดํฐ ์ธํธ๋ฅผ ๋ชจ๋ธ์ ์ ๋ฌํ ์ ์์ต๋๋ค!
```py
>>> dataset[0].keys()
```
๋ค์์ ๋ณํ์ด ์ ์ฉ๋ ํ์ ์ด๋ฏธ์ง์
๋๋ค. ์ด๋ฏธ์ง๊ฐ ๋ฌด์์๋ก ์๋ ค๋๊ฐ๊ณ ์์ ์์ฑ์ด ๋ค๋ฆ
๋๋ค.
```py
>>> import numpy as np
>>> import matplotlib.pyplot as plt
>>> img = dataset[0]["pixel_values"]
>>> plt.imshow(img.permute(1, 2, 0))
```
<div class="flex justify-center">
<img src="https://huggingface.co/datasets/huggingface/documentation-images/resolve/main/preprocessed_image.png"/>
</div>
<Tip>
`ImageProcessor`๋ ๊ฐ์ฒด ๊ฐ์ง, ์๋งจํฑ ์ธ๊ทธ๋ฉํ
์ด์
(semantic segmentation), ์ธ์คํด์ค ์ธ๊ทธ๋ฉํ
์ด์
(instance segmentation), ํ๋ํฑ ์ธ๊ทธ๋ฉํ
์ด์
(panoptic segmentation)๊ณผ ๊ฐ์ ์์
์ ๋ํ ํ์ฒ๋ฆฌ ๋ฐฉ๋ฒ์ ์ ๊ณตํฉ๋๋ค.
์ด๋ฌํ ๋ฐฉ๋ฒ์ ๋ชจ๋ธ์ ์์ ์ถ๋ ฅ์ ๊ฒฝ๊ณ ์์๋ ์ธ๊ทธ๋ฉํ
์ด์
๋งต๊ณผ ๊ฐ์ ์๋ฏธ ์๋ ์์ธก์ผ๋ก ๋ณํํด์ค๋๋ค.
</Tip>
### ํจ๋ฉ[[pad]]
์๋ฅผ ๋ค์ด, [DETR](./model_doc/detr)์ ๊ฐ์ ๊ฒฝ์ฐ์๋ ๋ชจ๋ธ์ด ํ๋ จํ ๋ ํฌ๊ธฐ ์กฐ์ ์ฆ๊ฐ์ ์ ์ฉํฉ๋๋ค.
์ด๋ก ์ธํด ๋ฐฐ์น ๋ด ์ด๋ฏธ์ง ํฌ๊ธฐ๊ฐ ๋ฌ๋ผ์ง ์ ์์ต๋๋ค.
[`DetrImageProcessor`]์ [`DetrImageProcessor.pad`]๋ฅผ ์ฌ์ฉํ๊ณ ์ฌ์ฉ์ ์ ์ `collate_fn`์ ์ ์ํด์ ๋ฐฐ์น ์ด๋ฏธ์ง๋ฅผ ์ฒ๋ฆฌํ ์ ์์ต๋๋ค.
```py
>>> def collate_fn(batch):
... pixel_values = [item["pixel_values"] for item in batch]
... encoding = image_processor.pad(pixel_values, return_tensors="pt")
... labels = [item["labels"] for item in batch]
... batch = {}
... batch["pixel_values"] = encoding["pixel_values"]
... batch["pixel_mask"] = encoding["pixel_mask"]
... batch["labels"] = labels
... return batch
```
## ๋ฉํฐ๋ชจ๋ฌ[[multimodal]]
๋ฉํฐ๋ชจ๋ฌ ์
๋ ฅ์ด ํ์ํ ์์
์ ๊ฒฝ์ฐ, ๋ชจ๋ธ์ ๋ฐ์ดํฐ ์ธํธ๋ฅผ ์ค๋นํ๊ธฐ ์ํ [ํ๋ก์ธ์](main_classes/processors)๊ฐ ํ์ํฉ๋๋ค.
ํ๋ก์ธ์๋ ํ ํฌ๋์ด์ ์ ํน์ฑ ์ถ์ถ๊ธฐ์ ๊ฐ์ ๋ ๊ฐ์ง ์ฒ๋ฆฌ ๊ฐ์ฒด๋ฅผ ๊ฒฐํฉํฉ๋๋ค.
[LJ Speech](https://huggingface.co/datasets/lj_speech) ๋ฐ์ดํฐ ์ธํธ๋ฅผ ๊ฐ์ ธ์์ ์๋ ์์ฑ ์ธ์(ASR)์ ์ํ ํ๋ก์ธ์๋ฅผ ์ฌ์ฉํ๋ ๋ฐฉ๋ฒ์ ํ์ธํ์ธ์.
(๋ฐ์ดํฐ ์ธํธ๋ฅผ ๊ฐ์ ธ์ค๋ ๋ฐฉ๋ฒ์ ๋ํ ์์ธํ ๋ด์ฉ์ ๐ค [๋ฐ์ดํฐ ์ธํธ ํํ ๋ฆฌ์ผ](https://huggingface.co/docs/datasets/load_hub)์์ ๋ณผ ์ ์์ต๋๋ค.)
```py
>>> from datasets import load_dataset
>>> lj_speech = load_dataset("lj_speech", split="train")
```
์๋ ์์ฑ ์ธ์(ASR)์์๋ `audio`์ `text`์๋ง ์ง์คํ๋ฉด ๋๋ฏ๋ก, ๋ค๋ฅธ ์ด๋ค์ ์ ๊ฑฐํ ์ ์์ต๋๋ค:
```py
>>> lj_speech = lj_speech.map(remove_columns=["file", "id", "normalized_text"])
```
์ด์ `audio`์ `text`์ด์ ์ดํด๋ณด์ธ์:
```py
>>> lj_speech[0]["audio"]
{'array': array([-7.3242188e-04, -7.6293945e-04, -6.4086914e-04, ...,
7.3242188e-04, 2.1362305e-04, 6.1035156e-05], dtype=float32),
'path': '/root/.cache/huggingface/datasets/downloads/extracted/917ece08c95cf0c4115e45294e3cd0dee724a1165b7fc11798369308a465bd26/LJSpeech-1.1/wavs/LJ001-0001.wav',
'sampling_rate': 22050}
>>> lj_speech[0]["text"]
'Printing, in the only sense with which we are at present concerned, differs from most if not from all the arts and crafts represented in the Exhibition'
```
๊ธฐ์กด์ ์ฌ์ ํ๋ จ๋ ๋ชจ๋ธ์์ ์ฌ์ฉ๋ ๋ฐ์ดํฐ ์ธํธ์ ์๋ก์ด ์ค๋์ค ๋ฐ์ดํฐ ์ธํธ์ ์ํ๋ง ๋ ์ดํธ๋ฅผ ์ผ์น์ํค๊ธฐ ์ํด ์ค๋์ค ๋ฐ์ดํฐ ์ธํธ์ ์ํ๋ง ๋ ์ดํธ๋ฅผ [๋ฆฌ์ํ๋ง](preprocessing#audio)ํด์ผ ํฉ๋๋ค!
```py
>>> lj_speech = lj_speech.cast_column("audio", Audio(sampling_rate=16_000))
```
[`AutoProcessor.from_pretrained`]๋ก ํ๋ก์ธ์๋ฅผ ๊ฐ์ ธ์ค์ธ์:
```py
>>> from transformers import AutoProcessor
>>> processor = AutoProcessor.from_pretrained("facebook/wav2vec2-base-960h")
```
1. `array`์ ๋ค์ด ์๋ ์ค๋์ค ๋ฐ์ดํฐ๋ฅผ `input_values`๋ก ๋ณํํ๊ณ `text`๋ฅผ ํ ํฐํํ์ฌ `labels`๋ก ๋ณํํ๋ ํจ์๋ฅผ ๋ง๋ญ๋๋ค.
๋ชจ๋ธ์ ์
๋ ฅ์ ๋ค์๊ณผ ๊ฐ์ต๋๋ค:
```py
>>> def prepare_dataset(example):
... audio = example["audio"]
... example.update(processor(audio=audio["array"], text=example["text"], sampling_rate=16000))
... return example
```
2. ์ํ์ `prepare_dataset` ํจ์์ ์ ์ฉํ์ธ์:
```py
>>> prepare_dataset(lj_speech[0])
```
์ด์ ํ๋ก์ธ์๊ฐ `input_values`์ `labels`๋ฅผ ์ถ๊ฐํ๊ณ , ์ํ๋ง ๋ ์ดํธ๋ ์ฌ๋ฐ๋ฅด๊ฒ 16kHz๋ก ๋ค์ด์ํ๋งํ์ต๋๋ค.
๋๋์ด ์ฒ๋ฆฌ๋ ๋ฐ์ดํฐ ์ธํธ๋ฅผ ๋ชจ๋ธ์ ์ ๋ฌํ ์ ์์ต๋๋ค!
| transformers/docs/source/ko/preprocessing.md/0 | {
"file_path": "transformers/docs/source/ko/preprocessing.md",
"repo_id": "transformers",
"token_count": 17104
} | 272 |
<!--Copyright 2022 The HuggingFace Team. All rights reserved.
Licensed under the Apache License, Version 2.0 (the "License"); you may not use this file except in compliance with
the License. You may obtain a copy of the License at
http://www.apache.org/licenses/LICENSE-2.0
Unless required by applicable law or agreed to in writing, software distributed under the License is distributed on
an "AS IS" BASIS, WITHOUT WARRANTIES OR CONDITIONS OF ANY KIND, either express or implied. See the License for the
specific language governing permissions and limitations under the License.
โ ๏ธ Note that this file is in Markdown but contain specific syntax for our doc-builder (similar to MDX) that may not be
rendered properly in your Markdown viewer.
-->
# Tour rรกpido
[[open-in-colab]]
Comece a trabalhar com ๐ค Transformers! Comece usando [`pipeline`] para rรกpida inferรชncia e facilmente carregue um modelo prรฉ-treinado e um tokenizer com [AutoClass](./model_doc/auto) para resolver tarefas de texto, visรฃo ou รกudio.
<Tip>
Todos os exemplos de cรณdigo apresentados na documentaรงรฃo tรชm um botรฃo no canto superior direito para escolher se vocรช deseja ocultar ou mostrar o cรณdigo no Pytorch ou no TensorFlow. Caso contrรกrio, รฉ esperado que funcione para ambos back-ends sem nenhuma alteraรงรฃo.
</Tip>
## Pipeline
[`pipeline`] รฉ a maneira mais fรกcil de usar um modelo prรฉ-treinado para uma dada tarefa.
<Youtube id="tiZFewofSLM"/>
A [`pipeline`] apoia diversas tarefas fora da caixa:
**Texto**:
* Anรกlise sentimental: classifica a polaridade de um texto.
* Geraรงรฃo de texto (em Inglรชs): gera texto a partir de uma entrada.
* Reconhecimento de entidade mencionada: legenda cada palavra com uma classe que a representa (pessoa, data, local, etc...)
* Respostas: extrai uma resposta dado algum contexto e uma questรฃo
* Mรกscara de preenchimento: preenche o espaรงo, dado um texto com mรกscaras de palavras.
* Sumarizaรงรฃo: gera o resumo de um texto longo ou documento.
* Traduรงรฃo: traduz texto para outra lรญngua.
* Extraรงรฃo de caracterรญsticas: cria um tensor que representa o texto.
**Imagem**:
* Classificaรงรฃo de imagens: classifica uma imagem.
* Segmentaรงรฃo de imagem: classifica cada pixel da imagem.
* Detecรงรฃo de objetos: detecta objetos em uma imagem.
**Audio**:
* Classficaรงรฃo de รกudio: legenda um trecho de รกudio fornecido.
* Reconhecimento de fala automรกtico: transcreve audio em texto.
<Tip>
Para mais detalhes sobre a [`pipeline`] e tarefas associadas, siga a documentaรงรฃo [aqui](./main_classes/pipelines).
</Tip>
### Uso da pipeline
No exemplo a seguir, vocรช usarรก [`pipeline`] para anรกlise sentimental.
Instale as seguintes dependรชncias se vocรช ainda nรฃo o fez:
<frameworkcontent>
<pt>
```bash
pip install torch
```
</pt>
<tf>
```bash
pip install tensorflow
```
</tf>
</frameworkcontent>
Importe [`pipeline`] e especifique a tarefa que deseja completar:
```py
>>> from transformers import pipeline
>>> classifier = pipeline("sentiment-analysis")
```
A pipeline baixa and armazena um [modelo prรฉ-treinado](https://huggingface.co/distilbert-base-uncased-finetuned-sst-2-english) padrรฃo e tokenizer para anรกlise sentimental. Agora vocรช pode usar `classifier` no texto alvo:
```py
>>> classifier("We are very happy to show you the ๐ค Transformers library.")
[{'label': 'POSITIVE', 'score': 0.9998}]
```
Para mais de uma sentenรงa, passe uma lista para a [`pipeline`], a qual retornarรก uma lista de dicionรกrios:
```py
>>> results = classifier(["We are very happy to show you the ๐ค Transformers library.", "We hope you don't hate it."])
>>> for result in results:
... print(f"label: {result['label']}, with score: {round(result['score'], 4)}")
label: POSITIVE, with score: 0.9998
label: NEGATIVE, with score: 0.5309
```
A [`pipeline`] tambรฉm pode iterar sobre um Dataset inteiro. Comece instalando a biblioteca de [๐ค Datasets](https://huggingface.co/docs/datasets/):
```bash
pip install datasets
```
Crie uma [`pipeline`] com a tarefa que deseja resolver e o modelo que deseja usar.
```py
>>> import torch
>>> from transformers import pipeline
>>> speech_recognizer = pipeline("automatic-speech-recognition", model="facebook/wav2vec2-base-960h")
```
A seguir, carregue uma base de dados (confira a ๐ค [Iniciaรงรฃo em Datasets](https://huggingface.co/docs/datasets/quickstart) para mais detalhes) que vocรช gostaria de iterar sobre. Por exemplo, vamos carregar o dataset [MInDS-14](https://huggingface.co/datasets/PolyAI/minds14):
```py
>>> from datasets import load_dataset, Audio
>>> dataset = load_dataset("PolyAI/minds14", name="en-US", split="train") # doctest: +IGNORE_RESULT
```
Precisamos garantir que a taxa de amostragem do conjunto de dados corresponda ร taxa de amostragem em que o facebook/wav2vec2-base-960h foi treinado.
```py
>>> dataset = dataset.cast_column("audio", Audio(sampling_rate=speech_recognizer.feature_extractor.sampling_rate))
```
Os arquivos de รกudio sรฃo carregados e re-amostrados automaticamente ao chamar a coluna `"audio"`.
Vamos extrair as arrays de formas de onda originais das primeiras 4 amostras e passรก-las como uma lista para o pipeline:
```py
>>> result = speech_recognizer(dataset[:4]["audio"])
>>> print([d["text"] for d in result])
['I WOULD LIKE TO SET UP A JOINT ACCOUNT WITH MY PARTNER HOW DO I PROCEED WITH DOING THAT', "FONDERING HOW I'D SET UP A JOIN TO HET WITH MY WIFE AND WHERE THE AP MIGHT BE", "I I'D LIKE TOY SET UP A JOINT ACCOUNT WITH MY PARTNER I'M NOT SEEING THE OPTION TO DO IT ON THE APSO I CALLED IN TO GET SOME HELP CAN I JUST DO IT OVER THE PHONE WITH YOU AND GIVE YOU THE INFORMATION OR SHOULD I DO IT IN THE AP AND I'M MISSING SOMETHING UQUETTE HAD PREFERRED TO JUST DO IT OVER THE PHONE OF POSSIBLE THINGS", 'HOW DO I TURN A JOIN A COUNT']
```
Para um conjunto de dados maior onde as entradas sรฃo maiores (como em fala ou visรฃo), serรก necessรกrio passar um gerador em vez de uma lista que carregue todas as entradas na memรณria. Consulte a [documentaรงรฃo do pipeline](./main_classes/pipelines) para mais informaรงรตes.
### Use outro modelo e tokenizer na pipeline
A [`pipeline`] pode acomodar qualquer modelo do [Model Hub](https://huggingface.co/models), facilitando sua adaptaรงรฃo para outros casos de uso. Por exemplo, se vocรช quiser um modelo capaz de lidar com texto em francรชs, use as tags no Model Hub para filtrar um modelo apropriado. O principal resultado filtrado retorna um [modelo BERT](https://huggingface.co/nlptown/bert-base-multilingual-uncased-sentiment) bilรญngue ajustado para anรกlise de sentimentos. รtimo, vamos usar este modelo!
```py
>>> model_name = "nlptown/bert-base-multilingual-uncased-sentiment"
```
<frameworkcontent>
<pt>
Use o [`AutoModelForSequenceClassification`] e [`AutoTokenizer`] para carregar o modelo prรฉ-treinado e seu tokenizer associado (mais em `AutoClass` abaixo):
```py
>>> from transformers import AutoTokenizer, AutoModelForSequenceClassification
>>> model = AutoModelForSequenceClassification.from_pretrained(model_name)
>>> tokenizer = AutoTokenizer.from_pretrained(model_name)
```
</pt>
<tf>
Use o [`TFAutoModelForSequenceClassification`] and [`AutoTokenizer`] para carregar o modelo prรฉ-treinado e o tokenizer associado (mais em `TFAutoClass` abaixo):
```py
>>> from transformers import AutoTokenizer, TFAutoModelForSequenceClassification
>>> model = TFAutoModelForSequenceClassification.from_pretrained(model_name)
>>> tokenizer = AutoTokenizer.from_pretrained(model_name)
```
</tf>
</frameworkcontent>
Entรฃo vocรช pode especificar o modelo e o tokenizador na [`pipeline`] e aplicar o `classifier` no seu texto alvo:
```py
>>> classifier = pipeline("sentiment-analysis", model=model, tokenizer=tokenizer)
>>> classifier("Nous sommes trรจs heureux de vous prรฉsenter la bibliothรจque ๐ค Transformers.")
[{'label': '5 stars', 'score': 0.7273}]
```
Se vocรช nรฃo conseguir achar um modelo para o seu caso de uso, precisarรก usar fine-tune em um modelo prรฉ-treinado nos seus dados. Veja nosso [tutorial de fine-tuning](./training) para descobrir como. Finalmente, depois que vocรช tiver usado esse processo em seu modelo, considere compartilhรก-lo conosco (veja o tutorial [aqui](./model_sharing)) na plataforma Model Hub afim de democratizar NLP! ๐ค
## AutoClass
<Youtube id="AhChOFRegn4"/>
Por baixo dos panos, as classes [`AutoModelForSequenceClassification`] e [`AutoTokenizer`] trabalham juntas para fortificar o [`pipeline`]. Um [AutoClass](./model_doc/auto) รฉ um atalho que automaticamente recupera a arquitetura de um modelo prรฉ-treinado a partir de seu nome ou caminho. Basta selecionar a `AutoClass` apropriada para sua tarefa e seu tokenizer associado com [`AutoTokenizer`].
Vamos voltar ao nosso exemplo e ver como vocรช pode usar a `AutoClass` para replicar os resultados do [`pipeline`].
### AutoTokenizer
Um tokenizer รฉ responsรกvel por prรฉ-processar o texto em um formato que seja compreensรญvel para o modelo. Primeiro, o tokenizer dividirรก o texto em palavras chamadas *tokens*. Existem vรกrias regras que regem o processo de tokenizaรงรฃo, incluindo como dividir uma palavra e em que nรญvel (saiba mais sobre tokenizaรงรฃo [aqui](./tokenizer_summary)). A coisa mais importante a lembrar, porรฉm, รฉ que vocรช precisa instanciar o tokenizer com o mesmo nome do modelo para garantir que estรก usando as mesmas regras de tokenizaรงรฃo com as quais um modelo foi prรฉ-treinado.
Carregue um tokenizer com [`AutoTokenizer`]:
```py
>>> from transformers import AutoTokenizer
>>> model_name = "nlptown/bert-base-multilingual-uncased-sentiment"
>>> tokenizer = AutoTokenizer.from_pretrained(model_name)
```
Em seguida, o tokenizer converte os tokens em nรบmeros para construir um tensor como entrada para o modelo. Isso รฉ conhecido como o *vocabulรกrio* do modelo.
Passe o texto para o tokenizer:
```py
>>> encoding = tokenizer("We are very happy to show you the ๐ค Transformers library.")
>>> print(encoding)
{'input_ids': [101, 11312, 10320, 12495, 19308, 10114, 11391, 10855, 10103, 100, 58263, 13299, 119, 102],
'token_type_ids': [0, 0, 0, 0, 0, 0, 0, 0, 0, 0, 0, 0, 0, 0],
'attention_mask': [1, 1, 1, 1, 1, 1, 1, 1, 1, 1, 1, 1, 1, 1]}
```
O tokenizer retornarรก um dicionรกrio contendo:
* [input_ids](./glossary#input-ids): representaรงรตes numรฉricas de seus tokens.
* [atttention_mask](.glossary#attention-mask): indica quais tokens devem ser atendidos.
Assim como o [`pipeline`], o tokenizer aceitarรก uma lista de entradas. Alรฉm disso, o tokenizer tambรฉm pode preencher e truncar o texto para retornar um lote com comprimento uniforme:
<frameworkcontent>
<pt>
```py
>>> pt_batch = tokenizer(
... ["We are very happy to show you the ๐ค transformers library.", "We hope you don't hate it."],
... padding=True,
... truncation=True,
... max_length=512,
... return_tensors="pt",
... )
```
</pt>
<tf>
```py
>>> tf_batch = tokenizer(
... ["We are very happy to show you the ๐ค Transformers library.", "We hope you don't hate it."],
... padding=True,
... truncation=True,
... max_length=512,
... return_tensors="tf",
... )
```
</tf>
</frameworkcontent>
Leia o tutorial de [prรฉ-processamento](./prรฉ-processamento) para obter mais detalhes sobre tokenizaรงรฃo.
### AutoModel
<frameworkcontent>
<pt>
๐ค Transformers fornecem uma maneira simples e unificada de carregar instรขncias prรฉ-treinadas. Isso significa que vocรช pode carregar um [`AutoModel`] como carregaria um [`AutoTokenizer`]. A รบnica diferenรงa รฉ selecionar o [`AutoModel`] correto para a tarefa. Como vocรช estรก fazendo classificaรงรฃo de texto ou sequรชncia, carregue [`AutoModelForSequenceClassification`]:
```py
>>> from transformers import AutoModelForSequenceClassification
>>> model_name = "nlptown/bert-base-multilingual-uncased-sentiment"
>>> pt_model = AutoModelForSequenceClassification.from_pretrained(model_name)
```
<Tip>
Veja o [sumรกrio de tarefas](./task_summary) para qual classe de [`AutoModel`] usar para cada tarefa.
</Tip>
Agora vocรช pode passar seu grupo de entradas prรฉ-processadas diretamente para o modelo. Vocรช apenas tem que descompactar o dicionรกrio usando `**`:
```py
>>> pt_outputs = pt_model(**pt_batch)
```
O modelo gera as ativaรงรตes finais no atributo `logits`. Aplique a funรงรฃo softmax aos `logits` para recuperar as probabilidades:
```py
>>> from torch import nn
>>> pt_predictions = nn.functional.softmax(pt_outputs.logits, dim=-1)
>>> print(pt_predictions)
tensor([[0.0021, 0.0018, 0.0115, 0.2121, 0.7725],
[0.2084, 0.1826, 0.1969, 0.1755, 0.2365]], grad_fn=<SoftmaxBackward0>)
```
</pt>
<tf>
๐ค Transformers fornecem uma maneira simples e unificada de carregar instรขncias prรฉ-treinadas. Isso significa que vocรช pode carregar um [`TFAutoModel`] como carregaria um [`AutoTokenizer`]. A รบnica diferenรงa รฉ selecionar o [`TFAutoModel`] correto para a tarefa. Como vocรช estรก fazendo classificaรงรฃo de texto ou sequรชncia, carregue [`TFAutoModelForSequenceClassification`]:
```py
>>> from transformers import TFAutoModelForSequenceClassification
>>> model_name = "nlptown/bert-base-multilingual-uncased-sentiment"
>>> tf_model = TFAutoModelForSequenceClassification.from_pretrained(model_name)
```
<Tip>
Veja o [sumรกrio de tarefas](./task_summary) para qual classe de [`AutoModel`] usar para cada tarefa.
</Tip>
Agora vocรช pode passar seu grupo de entradas prรฉ-processadas diretamente para o modelo atravรฉs da passagem de chaves de dicionรกrios ao tensor.
```py
>>> tf_outputs = tf_model(tf_batch)
```
O modelo gera as ativaรงรตes finais no atributo `logits`. Aplique a funรงรฃo softmax aos `logits` para recuperar as probabilidades:
```py
>>> import tensorflow as tf
>>> tf_predictions = tf.nn.softmax(tf_outputs.logits, axis=-1)
>>> tf_predictions # doctest: +IGNORE_RESULT
```
</tf>
</frameworkcontent>
<Tip>
Todos os modelos de ๐ค Transformers (PyTorch ou TensorFlow) geram tensores *antes* da funรงรฃo de ativaรงรฃo final (como softmax) pois essa funรงรฃo algumas vezes รฉ fundida com a perda.
</Tip>
Os modelos sรฃo um standard [`torch.nn.Module`](https://pytorch.org/docs/stable/nn.html#torch.nn.Module) ou um [`tf.keras.Model`](https: //www.tensorflow.org/api_docs/python/tf/keras/Model) para que vocรช possa usรก-los em seu loop de treinamento habitual. No entanto, para facilitar as coisas, ๐ค Transformers fornece uma classe [`Trainer`] para PyTorch que adiciona funcionalidade para treinamento distribuรญdo, precisรฃo mista e muito mais. Para o TensorFlow, vocรช pode usar o mรฉtodo `fit` de [Keras](https://keras.io/). Consulte o [tutorial de treinamento](./training) para obter mais detalhes.
<Tip>
As saรญdas do modelo ๐ค Transformers sรฃo classes de dados especiais para que seus atributos sejam preenchidos automaticamente em um IDE.
As saรญdas do modelo tambรฉm se comportam como uma tupla ou um dicionรกrio (por exemplo, vocรช pode indexar com um inteiro, uma parte ou uma string), caso em que os atributos `None` sรฃo ignorados.
</Tip>
### Salvar um modelo
<frameworkcontent>
<pt>
Uma vez que seu modelo estiver afinado, vocรช pode salvรก-lo com seu Tokenizer usando [`PreTrainedModel.save_pretrained`]:
```py
>>> pt_save_directory = "./pt_save_pretrained"
>>> tokenizer.save_pretrained(pt_save_directory) # doctest: +IGNORE_RESULT
>>> pt_model.save_pretrained(pt_save_directory)
```
Quando vocรช estiver pronto para usรก-lo novamente, recarregue com [`PreTrainedModel.from_pretrained`]:
```py
>>> pt_model = AutoModelForSequenceClassification.from_pretrained("./pt_save_pretrained")
```
</pt>
<tf>
Uma vez que seu modelo estiver afinado, vocรช pode salvรก-lo com seu Tokenizer usando [`TFPreTrainedModel.save_pretrained`]:
```py
>>> tf_save_directory = "./tf_save_pretrained"
>>> tokenizer.save_pretrained(tf_save_directory) # doctest: +IGNORE_RESULT
>>> tf_model.save_pretrained(tf_save_directory)
```
Quando vocรช estiver pronto para usรก-lo novamente, recarregue com [`TFPreTrainedModel.from_pretrained`]
```py
>>> tf_model = TFAutoModelForSequenceClassification.from_pretrained("./tf_save_pretrained")
```
</tf>
</frameworkcontent>
Um recurso particularmente interessante dos ๐ค Transformers รฉ a capacidade de salvar um modelo e recarregรก-lo como um modelo PyTorch ou TensorFlow. Use `from_pt` ou `from_tf` para converter o modelo de um framework para outro:
<frameworkcontent>
<pt>
```py
>>> from transformers import AutoModel
>>> tokenizer = AutoTokenizer.from_pretrained(tf_save_directory)
>>> pt_model = AutoModelForSequenceClassification.from_pretrained(tf_save_directory, from_tf=True)
```
</pt>
<tf>
```py
>>> from transformers import TFAutoModel
>>> tokenizer = AutoTokenizer.from_pretrained(pt_save_directory)
>>> tf_model = TFAutoModelForSequenceClassification.from_pretrained(pt_save_directory, from_pt=True)
```
</tf>
</frameworkcontent> | transformers/docs/source/pt/quicktour.md/0 | {
"file_path": "transformers/docs/source/pt/quicktour.md",
"repo_id": "transformers",
"token_count": 6107
} | 273 |
<!--Copyright 2022 The HuggingFace Team. All rights reserved.
Licensed under the Apache License, Version 2.0 (the "License"); you may not use this file except in compliance with
the License. You may obtain a copy of the License at
http://www.apache.org/licenses/LICENSE-2.0
Unless required by applicable law or agreed to in writing, software distributed under the License is distributed on
an "AS IS" BASIS, WITHOUT WARRANTIES OR CONDITIONS OF ANY KIND, either express or implied. See the License for the
specific language governing permissions and limitations under the License.
โ ๏ธ Note that this file is in Markdown but contain specific syntax for our doc-builder (similar to MDX) that may not be
rendered properly in your Markdown viewer.
-->
# ๅๅปบ่ชๅฎไนๆถๆ
[`AutoClass`](model_doc/auto) ่ชๅจๆจๆญๆจกๅๆถๆๅนถไธ่ฝฝ้ข่ฎญ็ป็้
็ฝฎๅๆ้ใไธ่ฌๆฅ่ฏด๏ผๆไปฌๅปบ่ฎฎไฝฟ็จ `AutoClass` ็ๆไธๆฃๆฅ็น๏ผcheckpoint๏ผๆ ๅ
ณ็ไปฃ็ ใๅธๆๅฏน็นๅฎๆจกๅๅๆฐๆๆดๅคๆงๅถ็็จๆท๏ผๅฏไปฅไป
ไปๅ ไธชๅบ็ฑปๅๅปบ่ชๅฎไน็ ๐ค Transformers ๆจกๅใ่ฟๅฏนไบไปปไฝๆๅ
ด่ถฃๅญฆไน ใ่ฎญ็ปๆ่ฏ้ช ๐ค Transformers ๆจกๅ็ไบบๅฏ่ฝ็นๅซๆ็จใ้่ฟๆฌๆๅ๏ผๆทฑๅ
ฅไบ่งฃๅฆไฝไธ้่ฟ `AutoClass` ๅๅปบ่ชๅฎไนๆจกๅใไบ่งฃๅฆไฝ๏ผ
- ๅ ่ฝฝๅนถ่ชๅฎไนๆจกๅ้
็ฝฎใ
- ๅๅปบๆจกๅๆถๆใ
- ไธบๆๆฌๅๅปบๆ
ข้ๅๅฟซ้ๅ่ฏๅจใ
- ไธบ่ง่งไปปๅกๅๅปบๅพๅๅค็ๅจใ
- ไธบ้ณ้ขไปปๅกๅๅปบ็นๅพๆๅๅจใ
- ไธบๅคๆจกๆไปปๅกๅๅปบๅค็ๅจใ
## ้
็ฝฎ
[้
็ฝฎ](main_classes/configuration) ๆถๅๅฐๆจกๅ็ๅ
ทไฝๅฑๆงใๆฏไธชๆจกๅ้
็ฝฎ้ฝๆไธๅ็ๅฑๆง๏ผไพๅฆ๏ผๆๆ NLP ๆจกๅ้ฝๅ
ฑไบซ `hidden_size`ใ`num_attention_heads`ใ `num_hidden_layers` ๅ `vocab_size` ๅฑๆงใ่ฟไบๅฑๆง็จไบๆๅฎๆๅปบๆจกๅๆถ็ๆณจๆๅๅคดๆฐ้ๆ้่ๅฑๅฑๆฐใ
่ฎฟ้ฎ [`DistilBertConfig`] ไปฅๆด่ฟไธๆญฅไบ่งฃ [DistilBERT](model_doc/distilbert)๏ผๆฃๆฅๅฎ็ๅฑๆง๏ผ
```py
>>> from transformers import DistilBertConfig
>>> config = DistilBertConfig()
>>> print(config)
DistilBertConfig {
"activation": "gelu",
"attention_dropout": 0.1,
"dim": 768,
"dropout": 0.1,
"hidden_dim": 3072,
"initializer_range": 0.02,
"max_position_embeddings": 512,
"model_type": "distilbert",
"n_heads": 12,
"n_layers": 6,
"pad_token_id": 0,
"qa_dropout": 0.1,
"seq_classif_dropout": 0.2,
"sinusoidal_pos_embds": false,
"transformers_version": "4.16.2",
"vocab_size": 30522
}
```
[`DistilBertConfig`] ๆพ็คบไบๆๅปบๅบ็ก [`DistilBertModel`] ๆไฝฟ็จ็ๆๆ้ป่ฎคๅฑๆงใๆๆๅฑๆง้ฝๅฏไปฅ่ฟ่ก่ชๅฎไน๏ผไธบๅฎ้ชๅ้ ไบ็ฉบ้ดใไพๅฆ๏ผๆจๅฏไปฅๅฐ้ป่ฎคๆจกๅ่ชๅฎไนไธบ๏ผ
- ไฝฟ็จ `activation` ๅๆฐๅฐ่ฏไธๅ็ๆฟๆดปๅฝๆฐใ
- ไฝฟ็จ `attention_dropout` ๅๆฐไธบ attention probabilities ไฝฟ็จๆด้ซ็ dropout ratioใ
```py
>>> my_config = DistilBertConfig(activation="relu", attention_dropout=0.4)
>>> print(my_config)
DistilBertConfig {
"activation": "relu",
"attention_dropout": 0.4,
"dim": 768,
"dropout": 0.1,
"hidden_dim": 3072,
"initializer_range": 0.02,
"max_position_embeddings": 512,
"model_type": "distilbert",
"n_heads": 12,
"n_layers": 6,
"pad_token_id": 0,
"qa_dropout": 0.1,
"seq_classif_dropout": 0.2,
"sinusoidal_pos_embds": false,
"transformers_version": "4.16.2",
"vocab_size": 30522
}
```
้ข่ฎญ็ปๆจกๅ็ๅฑๆงๅฏไปฅๅจ [`~PretrainedConfig.from_pretrained`] ๅฝๆฐไธญ่ฟ่กไฟฎๆน๏ผ
```py
>>> my_config = DistilBertConfig.from_pretrained("distilbert-base-uncased", activation="relu", attention_dropout=0.4)
```
ๅฝไฝ ๅฏนๆจกๅ้
็ฝฎๆปกๆๆถ๏ผๅฏไปฅไฝฟ็จ [`~PretrainedConfig.save_pretrained`] ๆฅไฟๅญ้
็ฝฎใไฝ ็้
็ฝฎๆไปถๅฐไปฅ JSON ๆไปถ็ๅฝขๅผๅญๅจๅจๆๅฎ็ไฟๅญ็ฎๅฝไธญ๏ผ
```py
>>> my_config.save_pretrained(save_directory="./your_model_save_path")
```
่ฆ้็จ้
็ฝฎๆไปถ๏ผ่ฏทไฝฟ็จ [`~PretrainedConfig.from_pretrained`] ่ฟ่กๅ ่ฝฝ๏ผ
```py
>>> my_config = DistilBertConfig.from_pretrained("./your_model_save_path/config.json")
```
<Tip>
ไฝ ่ฟๅฏไปฅๅฐ้
็ฝฎๆไปถไฟๅญไธบๅญๅ
ธ๏ผ็่ณๅชไฟๅญ่ชๅฎไน้
็ฝฎๅฑๆงไธ้ป่ฎค้
็ฝฎๅฑๆงไน้ด็ๅทฎๅผ๏ผๆๅ
ณๆดๅค่ฏฆ็ปไฟกๆฏ๏ผ่ฏทๅ้
[้
็ฝฎ](main_classes/configuration) ๆๆกฃใ
</Tip>
## ๆจกๅ
ๆฅไธๆฅ๏ผๅๅปบไธไธช[ๆจกๅ](main_classes/models)ใๆจกๅ๏ผไนๅฏๆณๆๆถๆ๏ผๅฎไนไบๆฏไธๅฑ็ฝ็ป็่กไธบไปฅๅ่ฟ่ก็ๆไฝใ้
็ฝฎไธญ็ `num_hidden_layers` ็ญๅฑๆง็จไบๅฎไนๆถๆใๆฏไธชๆจกๅ้ฝๅ
ฑไบซๅบ็ฑป [`PreTrainedModel`] ๅไธไบๅธธ็จๆนๆณ๏ผไพๅฆ่ฐๆด่พๅ
ฅๅตๅ
ฅ็ๅคงๅฐๅไฟฎๅช่ชๆณจๆๅๅคดใๆญคๅค๏ผๆๆๆจกๅ้ฝๆฏ [`torch.nn.Module`](https://pytorch.org/docs/stable/generated/torch.nn.Module.html)ใ[`tf.keras.Model`](https://www.tensorflow.org/api_docs/python/tf/keras/Model) ๆ [`flax.linen.Module`](https://flax.readthedocs.io/en/latest/api_reference/flax.linen/module.html) ็ๅญ็ฑปใ่ฟๆๅณ็ๆจกๅไธๅ่ชๆกๆถ็็จๆณๅ
ผๅฎนใ
<frameworkcontent>
<pt>
ๅฐ่ชๅฎไน้
็ฝฎๅฑๆงๅ ่ฝฝๅฐๆจกๅไธญ๏ผ
```py
>>> from transformers import DistilBertModel
>>> my_config = DistilBertConfig.from_pretrained("./your_model_save_path/config.json")
>>> model = DistilBertModel(my_config)
```
่ฟๆฎตไปฃ็ ๅๅปบไบไธไธชๅ
ทๆ้ๆบๅๆฐ่ไธๆฏ้ข่ฎญ็ปๆ้็ๆจกๅใๅจ่ฎญ็ป่ฏฅๆจกๅไนๅ๏ผๆจ่ฟๆ ๆณๅฐ่ฏฅๆจกๅ็จไบไปปไฝ็จ้ใ่ฎญ็ปๆฏไธ้กนๆ่ดตไธ่ๆถ็่ฟ็จใ้ๅธธๆฅ่ฏด๏ผๆๅฅฝไฝฟ็จ้ข่ฎญ็ปๆจกๅๆฅๆดๅฟซๅฐ่ทๅพๆดๅฅฝ็็ปๆ๏ผๅๆถไป
ไฝฟ็จ่ฎญ็ปๆ้่ตๆบ็ไธๅฐ้จๅใ
ไฝฟ็จ [`~PreTrainedModel.from_pretrained`] ๅๅปบ้ข่ฎญ็ปๆจกๅ๏ผ
```py
>>> model = DistilBertModel.from_pretrained("distilbert-base-uncased")
```
ๅฝๅ ่ฝฝ้ข่ฎญ็ปๆ้ๆถ๏ผๅฆๆๆจกๅๆฏ็ฑ ๐ค Transformers ๆไพ็๏ผๅฐ่ชๅจๅ ่ฝฝ้ป่ฎคๆจกๅ้
็ฝฎใ็ถ่๏ผๅฆๆไฝ ๆฟๆ๏ผไป็ถๅฏไปฅๅฐ้ป่ฎคๆจกๅ้
็ฝฎ็ๆไบๆ่
ๆๆๅฑๆงๆฟๆขๆไฝ ่ชๅทฑ็้
็ฝฎ๏ผ
```py
>>> model = DistilBertModel.from_pretrained("distilbert-base-uncased", config=my_config)
```
</pt>
<tf>
ๅฐ่ชๅฎไน้
็ฝฎๅฑๆงๅ ่ฝฝๅฐๆจกๅไธญ๏ผ
```py
>>> from transformers import TFDistilBertModel
>>> my_config = DistilBertConfig.from_pretrained("./your_model_save_path/my_config.json")
>>> tf_model = TFDistilBertModel(my_config)
```
่ฟๆฎตไปฃ็ ๅๅปบไบไธไธชๅ
ทๆ้ๆบๅๆฐ่ไธๆฏ้ข่ฎญ็ปๆ้็ๆจกๅใๅจ่ฎญ็ป่ฏฅๆจกๅไนๅ๏ผๆจ่ฟๆ ๆณๅฐ่ฏฅๆจกๅ็จไบไปปไฝ็จ้ใ่ฎญ็ปๆฏไธ้กนๆ่ดตไธ่ๆถ็่ฟ็จใ้ๅธธๆฅ่ฏด๏ผๆๅฅฝไฝฟ็จ้ข่ฎญ็ปๆจกๅๆฅๆดๅฟซๅฐ่ทๅพๆดๅฅฝ็็ปๆ๏ผๅๆถไป
ไฝฟ็จ่ฎญ็ปๆ้่ตๆบ็ไธๅฐ้จๅใ
ไฝฟ็จ [`~TFPreTrainedModel.from_pretrained`] ๅๅปบ้ข่ฎญ็ปๆจกๅ๏ผ
```py
>>> tf_model = TFDistilBertModel.from_pretrained("distilbert-base-uncased")
```
ๅฝๅ ่ฝฝ้ข่ฎญ็ปๆ้ๆถ๏ผๅฆๆๆจกๅๆฏ็ฑ ๐ค Transformers ๆไพ็๏ผๅฐ่ชๅจๅ ่ฝฝ้ป่ฎคๆจกๅ้
็ฝฎใ็ถ่๏ผๅฆๆไฝ ๆฟๆ๏ผไป็ถๅฏไปฅๅฐ้ป่ฎคๆจกๅ้
็ฝฎ็ๆไบๆ่
ๆๆๅฑๆงๆฟๆขๆ่ชๅทฑ็้
็ฝฎ๏ผ
```py
>>> tf_model = TFDistilBertModel.from_pretrained("distilbert-base-uncased", config=my_config)
```
</tf>
</frameworkcontent>
### ๆจกๅๅคด๏ผModel heads๏ผ
ๆญคๆถ๏ผไฝ ๅทฒ็ปๆไบไธไธช่พๅบ*้่็ถๆ*็ๅบ็ก DistilBERT ๆจกๅใ้่็ถๆไฝไธบ่พๅ
ฅไผ ้ๅฐๆจกๅๅคดไปฅ็ๆๆ็ป่พๅบใ๐ค Transformers ไธบๆฏไธชไปปๅกๆไพไธๅ็ๆจกๅๅคด๏ผๅช่ฆๆจกๅๆฏๆ่ฏฅไปปๅก๏ผๅณ๏ผๆจไธ่ฝไฝฟ็จ DistilBERT ๆฅๆง่กๅ็ฟป่ฏ่ฟๆ ท็ๅบๅๅฐๅบๅไปปๅก๏ผใ
<frameworkcontent>
<pt>
ไพๅฆ๏ผ[`DistilBertForSequenceClassification`] ๆฏไธไธชๅธฆๆๅบๅๅ็ฑปๅคด๏ผsequence classification head๏ผ็ๅบ็ก DistilBERT ๆจกๅใๅบๅๅ็ฑปๅคดๆฏๆฑ ๅ่พๅบไนไธ็็บฟๆงๅฑใ
```py
>>> from transformers import DistilBertForSequenceClassification
>>> model = DistilBertForSequenceClassification.from_pretrained("distilbert-base-uncased")
```
้่ฟๅๆขๅฐไธๅ็ๆจกๅๅคด๏ผๅฏไปฅ่ฝปๆพๅฐๅฐๆญคๆฃๆฅ็น้ๅค็จไบๅ
ถไปไปปๅกใๅฏนไบ้ฎ็ญไปปๅก๏ผไฝ ๅฏไปฅไฝฟ็จ [`DistilBertForQuestionAnswering`] ๆจกๅๅคดใ้ฎ็ญๅคด๏ผquestion answering head๏ผไธๅบๅๅ็ฑปๅคด็ฑปไผผ๏ผไธๅ็นๅจไบๅฎๆฏ้่็ถๆ่พๅบไนไธ็็บฟๆงๅฑใ
```py
>>> from transformers import DistilBertForQuestionAnswering
>>> model = DistilBertForQuestionAnswering.from_pretrained("distilbert-base-uncased")
```
</pt>
<tf>
ไพๅฆ๏ผ[`TFDistilBertForSequenceClassification`] ๆฏไธไธชๅธฆๆๅบๅๅ็ฑปๅคด๏ผsequence classification head๏ผ็ๅบ็ก DistilBERT ๆจกๅใๅบๅๅ็ฑปๅคดๆฏๆฑ ๅ่พๅบไนไธ็็บฟๆงๅฑใ
```py
>>> from transformers import TFDistilBertForSequenceClassification
>>> tf_model = TFDistilBertForSequenceClassification.from_pretrained("distilbert-base-uncased")
```
้่ฟๅๆขๅฐไธๅ็ๆจกๅๅคด,ๅฏไปฅ่ฝปๆพๅฐๅฐๆญคๆฃๆฅ็น้ๅค็จไบๅ
ถไปไปปๅกใๅฏนไบ้ฎ็ญไปปๅก๏ผไฝ ๅฏไปฅไฝฟ็จ [`TFDistilBertForQuestionAnswering`] ๆจกๅๅคดใ้ฎ็ญๅคด๏ผquestion answering head๏ผไธๅบๅๅ็ฑปๅคด็ฑปไผผ๏ผไธๅ็นๅจไบๅฎๆฏ้่็ถๆ่พๅบไนไธ็็บฟๆงๅฑใ
```py
>>> from transformers import TFDistilBertForQuestionAnswering
>>> tf_model = TFDistilBertForQuestionAnswering.from_pretrained("distilbert-base-uncased")
```
</tf>
</frameworkcontent>
## ๅ่ฏๅจ
ๅจๅฐๆจกๅ็จไบๆๆฌๆฐๆฎไนๅ๏ผไฝ ้่ฆ็ๆๅไธไธชๅบ็ฑปๆฏ [tokenizer](main_classes/tokenizer)๏ผๅฎ็จไบๅฐๅๅงๆๆฌ่ฝฌๆขไธบๅผ ้ใ๐ค Transformers ๆฏๆไธค็ง็ฑปๅ็ๅ่ฏๅจ๏ผ
- [`PreTrainedTokenizer`]๏ผๅ่ฏๅจ็Pythonๅฎ็ฐ
- [`PreTrainedTokenizerFast`]๏ผๆฅ่ชๆไปฌๅบไบ Rust ็ [๐ค Tokenizer](https://huggingface.co/docs/tokenizers/python/latest/) ๅบ็ๅ่ฏๅจใๅ ไธบๅ
ถไฝฟ็จไบ Rust ๅฎ็ฐ๏ผ่ฟ็งๅ่ฏๅจ็ฑปๅ็้ๅบฆ่ฆๅฟซๅพๅค๏ผๅฐคๅ
ถๆฏๅจๆน้ๅ่ฏ๏ผbatch tokenization๏ผ็ๆถๅใๅฟซ้ๅ่ฏๅจ่ฟๆไพๅ
ถไป็ๆนๆณ๏ผไพๅฆ*ๅ็งปๆ ๅฐ๏ผoffset mapping๏ผ*๏ผๅฎๅฐๆ ่ฎฐ๏ผtoken๏ผๆ ๅฐๅฐๅ
ถๅๅงๅ่ฏๆๅญ็ฌฆใ
่ฟไธค็งๅ่ฏๅจ้ฝๆฏๆๅธธ็จ็ๆนๆณ๏ผๅฆ็ผ็ ๅ่งฃ็ ใๆทปๅ ๆฐๆ ่ฎฐไปฅๅ็ฎก็็นๆฎๆ ่ฎฐใ
<Tip warning={true}>
ๅนถ้ๆฏไธชๆจกๅ้ฝๆฏๆๅฟซ้ๅ่ฏๅจใๅ็
ง่ฟๅผ [่กจๆ ผ](index#supported-frameworks) ๆฅ็ๆจกๅๆฏๅฆๆฏๆๅฟซ้ๅ่ฏๅจใ
</Tip>
ๅฆๆๆจ่ฎญ็ปไบ่ชๅทฑ็ๅ่ฏๅจ๏ผๅๅฏไปฅไป*่ฏ่กจ*ๆไปถๅๅปบไธไธชๅ่ฏๅจ๏ผ
```py
>>> from transformers import DistilBertTokenizer
>>> my_tokenizer = DistilBertTokenizer(vocab_file="my_vocab_file.txt", do_lower_case=False, padding_side="left")
```
่ฏทๅกๅฟ
่ฎฐไฝ๏ผ่ชๅฎไนๅ่ฏๅจ็ๆ็่ฏ่กจไธ้ข่ฎญ็ปๆจกๅๅ่ฏๅจ็ๆ็่ฏ่กจๆฏไธๅ็ใๅฆๆไฝฟ็จ้ข่ฎญ็ปๆจกๅ๏ผๅ้่ฆไฝฟ็จ้ข่ฎญ็ปๆจกๅ็่ฏ่กจ๏ผๅฆๅ่พๅ
ฅๅฐๆฒกๆๆไนใ ไฝฟ็จ [`DistilBertTokenizer`] ็ฑปๅๅปบๅ
ทๆ้ข่ฎญ็ปๆจกๅ่ฏ่กจ็ๅ่ฏๅจ๏ผ
```py
>>> from transformers import DistilBertTokenizer
>>> slow_tokenizer = DistilBertTokenizer.from_pretrained("distilbert-base-uncased")
```
ไฝฟ็จ [`DistilBertTokenizerFast`] ็ฑปๅๅปบๅฟซ้ๅ่ฏๅจ๏ผ
```py
>>> from transformers import DistilBertTokenizerFast
>>> fast_tokenizer = DistilBertTokenizerFast.from_pretrained("distilbert-base-uncased")
```
<Tip>
้ป่ฎคๆ
ๅตไธ๏ผ[`AutoTokenizer`] ๅฐๅฐ่ฏๅ ่ฝฝๅฟซ้ๆ ่ฎฐ็ๆๅจใไฝ ๅฏไปฅ้่ฟๅจ `from_pretrained` ไธญ่ฎพ็ฝฎ `use_fast=False` ไปฅ็ฆ็จๆญค่กไธบใ
</Tip>
## ๅพๅๅค็ๅจ
ๅพๅๅค็ๅจ็จไบๅค็่ง่ง่พๅ
ฅใๅฎ็ปงๆฟ่ช [`~image_processing_utils.ImageProcessingMixin`] ๅบ็ฑปใ
่ฆไฝฟ็จๅฎ๏ผ้่ฆๅๅปบไธไธชไธไฝ ไฝฟ็จ็ๆจกๅๅ
ณ่็ๅพๅๅค็ๅจใไพๅฆ๏ผๅฆๆไฝ ไฝฟ็จ [ViT](model_doc/vit) ่ฟ่กๅพๅๅ็ฑป๏ผๅฏไปฅๅๅปบไธไธช้ป่ฎค็ [`ViTImageProcessor`]๏ผ
```py
>>> from transformers import ViTImageProcessor
>>> vit_extractor = ViTImageProcessor()
>>> print(vit_extractor)
ViTImageProcessor {
"do_normalize": true,
"do_resize": true,
"image_processor_type": "ViTImageProcessor",
"image_mean": [
0.5,
0.5,
0.5
],
"image_std": [
0.5,
0.5,
0.5
],
"resample": 2,
"size": 224
}
```
<Tip>
ๅฆๆๆจไธ้่ฆ่ฟ่กไปปไฝ่ชๅฎไน๏ผๅช้ไฝฟ็จ `from_pretrained` ๆนๆณๅ ่ฝฝๆจกๅ็้ป่ฎคๅพๅๅค็ๅจๅๆฐใ
</Tip>
ไฟฎๆนไปปไฝ [`ViTImageProcessor`] ๅๆฐไปฅๅๅปบ่ชๅฎไนๅพๅๅค็ๅจ๏ผ
```py
>>> from transformers import ViTImageProcessor
>>> my_vit_extractor = ViTImageProcessor(resample="PIL.Image.BOX", do_normalize=False, image_mean=[0.3, 0.3, 0.3])
>>> print(my_vit_extractor)
ViTImageProcessor {
"do_normalize": false,
"do_resize": true,
"image_processor_type": "ViTImageProcessor",
"image_mean": [
0.3,
0.3,
0.3
],
"image_std": [
0.5,
0.5,
0.5
],
"resample": "PIL.Image.BOX",
"size": 224
}
```
## ็นๅพๆๅๅจ
็นๅพๆๅๅจ็จไบๅค็้ณ้ข่พๅ
ฅใๅฎ็ปงๆฟ่ช [`~feature_extraction_utils.FeatureExtractionMixin`] ๅบ็ฑป๏ผไบฆๅฏ็ปงๆฟ [`SequenceFeatureExtractor`] ็ฑปๆฅๅค็้ณ้ข่พๅ
ฅใ
่ฆไฝฟ็จๅฎ๏ผๅๅปบไธไธชไธไฝ ไฝฟ็จ็ๆจกๅๅ
ณ่็็นๅพๆๅๅจใไพๅฆ๏ผๅฆๆไฝ ไฝฟ็จ [Wav2Vec2](model_doc/wav2vec2) ่ฟ่ก้ณ้ขๅ็ฑป๏ผๅฏไปฅๅๅปบไธไธช้ป่ฎค็ [`Wav2Vec2FeatureExtractor`]๏ผ
```py
>>> from transformers import Wav2Vec2FeatureExtractor
>>> w2v2_extractor = Wav2Vec2FeatureExtractor()
>>> print(w2v2_extractor)
Wav2Vec2FeatureExtractor {
"do_normalize": true,
"feature_extractor_type": "Wav2Vec2FeatureExtractor",
"feature_size": 1,
"padding_side": "right",
"padding_value": 0.0,
"return_attention_mask": false,
"sampling_rate": 16000
}
```
<Tip>
ๅฆๆๆจไธ้่ฆ่ฟ่กไปปไฝ่ชๅฎไน๏ผๅช้ไฝฟ็จ `from_pretrained` ๆนๆณๅ ่ฝฝๆจกๅ็้ป่ฎค็นๅพๆๅๅจๅๆฐใ
</Tip>
ไฟฎๆนไปปไฝ [`Wav2Vec2FeatureExtractor`] ๅๆฐไปฅๅๅปบ่ชๅฎไน็นๅพๆๅๅจ๏ผ
```py
>>> from transformers import Wav2Vec2FeatureExtractor
>>> w2v2_extractor = Wav2Vec2FeatureExtractor(sampling_rate=8000, do_normalize=False)
>>> print(w2v2_extractor)
Wav2Vec2FeatureExtractor {
"do_normalize": false,
"feature_extractor_type": "Wav2Vec2FeatureExtractor",
"feature_size": 1,
"padding_side": "right",
"padding_value": 0.0,
"return_attention_mask": false,
"sampling_rate": 8000
}
```
## ๅค็ๅจ
ๅฏนไบๆฏๆๅคๆจกๅผไปปๅก็ๆจกๅ๏ผ๐ค Transformers ๆไพไบไธไธชๅค็ๅจ็ฑป๏ผๅฏไปฅๆนไพฟๅฐๅฐ็นๅพๆๅๅจๅๅ่ฏๅจ็ญๅค็็ฑปๅ
่ฃ
ๅฐๅไธชๅฏน่ฑกไธญใไพๅฆ๏ผ่ฎฉๆไปฌไฝฟ็จ [`Wav2Vec2Processor`] ๆฅๆง่ก่ชๅจ่ฏญ้ณ่ฏๅซไปปๅก (ASR)ใ ASR ๅฐ้ณ้ข่ฝฌๅฝไธบๆๆฌ๏ผๅ ๆญคๆจๅฐ้่ฆไธไธช็นๅพๆๅๅจๅไธไธชๅ่ฏๅจใ
ๅๅปบไธไธช็นๅพๆๅๅจๆฅๅค็้ณ้ข่พๅ
ฅ๏ผ
```py
>>> from transformers import Wav2Vec2FeatureExtractor
>>> feature_extractor = Wav2Vec2FeatureExtractor(padding_value=1.0, do_normalize=True)
```
ๅๅปบไธไธชๅ่ฏๅจๆฅๅค็ๆๆฌ่พๅ
ฅ๏ผ
```py
>>> from transformers import Wav2Vec2CTCTokenizer
>>> tokenizer = Wav2Vec2CTCTokenizer(vocab_file="my_vocab_file.txt")
```
ๅฐ็นๅพๆๅๅจๅๅ่ฏๅจๅๅนถๅฐ [`Wav2Vec2Processor`] ไธญ๏ผ
```py
>>> from transformers import Wav2Vec2Processor
>>> processor = Wav2Vec2Processor(feature_extractor=feature_extractor, tokenizer=tokenizer)
```
้่ฟไธคไธชๅบ็ฑป - ้
็ฝฎ็ฑปๅๆจกๅ็ฑป - ไปฅๅไธไธช้ๅ ็้ขๅค็็ฑป๏ผๅ่ฏๅจใๅพๅๅค็ๅจใ็นๅพๆๅๅจๆๅค็ๅจ๏ผ๏ผไฝ ๅฏไปฅๅๅปบ ๐ค Transformers ๆฏๆ็ไปปไฝๆจกๅใ ๆฏไธชๅบ็ฑป้ฝๆฏๅฏ้
็ฝฎ็๏ผๅ
่ฎธไฝ ไฝฟ็จๆ้็็นๅฎๅฑๆงใ ไฝ ๅฏไปฅ่ฝปๆพ่ฎพ็ฝฎๆจกๅ่ฟ่ก่ฎญ็ปๆไฟฎๆน็ฐๆ็้ข่ฎญ็ปๆจกๅ่ฟ่กๅพฎ่ฐใ
| transformers/docs/source/zh/create_a_model.md/0 | {
"file_path": "transformers/docs/source/zh/create_a_model.md",
"repo_id": "transformers",
"token_count": 8476
} | 274 |
<!--Copyright 2023 The HuggingFace Team. All rights reserved.
Licensed under the Apache License, Version 2.0 (the "License"); you may not use this file except in compliance with
the License. You may obtain a copy of the License at
http://www.apache.org/licenses/LICENSE-2.0
Unless required by applicable law or agreed to in writing, software distributed under the License is distributed on
an "AS IS" BASIS, WITHOUT WARRANTIES OR CONDITIONS OF ANY KIND, either express or implied. See the License for the
specific language governing permissions and limitations under the License.
โ ๏ธ Note that this file is in Markdown but contain specific syntax for our doc-builder (similar to MDX) that may not be
rendered properly in your Markdown viewer.
-->
## ไฝฟ็จLLMs่ฟ่ก็ๆ
[[open-in-colab]]
LLMs๏ผๅณๅคง่ฏญ่จๆจกๅ๏ผๆฏๆๆฌ็ๆ่ๅ็ๅ
ณ้ฎ็ปๆ้จๅใ็ฎๅๆฅ่ฏด๏ผๅฎไปฌๅ
ๅซ็ป่ฟๅคง่งๆจก้ข่ฎญ็ป็transformerๆจกๅ๏ผ็จไบๆ นๆฎ็ปๅฎ็่พๅ
ฅๆๆฌ้ขๆตไธไธไธช่ฏ๏ผๆๆดๅ็กฎๅฐ่ฏด๏ผไธไธไธช`token`๏ผใ็ฑไบๅฎไปฌไธๆฌกๅช้ขๆตไธไธช`token`๏ผๅ ๆญค้คไบ่ฐ็จๆจกๅไนๅค๏ผๆจ้่ฆๆง่กๆดๅคๆ็ๆไฝๆฅ็ๆๆฐ็ๅฅๅญโโๆจ้่ฆ่ฟ่ก่ชๅๅฝ็ๆใ
่ชๅๅฝ็ๆๆฏๅจ็ปๅฎไธไบๅๅง่พๅ
ฅ๏ผ้่ฟ่ฟญไปฃ่ฐ็จๆจกๅๅๅ
ถ่ช่บซ็็ๆ่พๅบๆฅ็ๆๆๆฌ็ๆจ็่ฟ็จ๏ผใๅจ๐ค Transformersไธญ๏ผ่ฟ็ฑ[`~generation.GenerationMixin.generate`]ๆนๆณๅค็๏ผๆๆๅ
ทๆ็ๆ่ฝๅ็ๆจกๅ้ฝๅฏไปฅไฝฟ็จ่ฏฅๆนๆณใ
ๆฌๆ็จๅฐๅๆจๅฑ็คบๅฆไฝ๏ผ
* ไฝฟ็จLLM็ๆๆๆฌ
* ้ฟๅ
ๅธธ่ง็้ท้ฑ
* ๅธฎๅฉๆจๅ
ๅๅฉ็จLLMไธไธๆญฅๆๅฏผ
ๅจๅผๅงไนๅ๏ผ่ฏท็กฎไฟๅทฒๅฎ่ฃ
ๆๆๅฟ
่ฆ็ๅบ๏ผ
```bash
pip install transformers bitsandbytes>=0.39.0 -q
```
## ็ๆๆๆฌ
ไธไธช็จไบ[ๅ ๆ่ฏญ่จๅปบๆจก](tasks/language_modeling)่ฎญ็ป็่ฏญ่จๆจกๅ๏ผๅฐๆๆฌ`tokens`ๅบๅไฝไธบ่พๅ
ฅ๏ผๅนถ่ฟๅไธไธไธช`token`็ๆฆ็ๅๅธใ
<!-- [GIF 1 -- FWD PASS] -->
<figure class="image table text-center m-0 w-full">
<video
style="max-width: 90%; margin: auto;"
autoplay loop muted playsinline
src="https://huggingface.co/datasets/huggingface/documentation-images/resolve/main/blog/assisted-generation/gif_1_1080p.mov"
></video>
<figcaption>"LLM็ๅๅไผ ้"</figcaption>
</figure>
ไฝฟ็จLLM่ฟ่ก่ชๅๅฝ็ๆ็ไธไธชๅ
ณ้ฎๆน้ขๆฏๅฆไฝไป่ฟไธชๆฆ็ๅๅธไธญ้ๆฉไธไธไธช`token`ใ่ฟไธชๆญฅ้ชคๅฏไปฅ้ๆ่ฟ่ก๏ผๅช่ฆๆ็ปๅพๅฐไธไธไธช่ฟญไปฃ็`token`ใ่ฟๆๅณ็ๅฏไปฅ็ฎๅ็ไปๆฆ็ๅๅธไธญ้ๆฉๆๅฏ่ฝ็`token`๏ผไนๅฏไปฅๅคๆ็ๅจๅฏน็ปๆๅๅธ่ฟ่ก้ๆ ทไนๅๅบ็จๅค็งๅๆข๏ผ่ฟๅๅณไบไฝ ็้ๆฑใ
<!-- [GIF 2 -- TEXT GENERATION] -->
<figure class="image table text-center m-0 w-full">
<video
style="max-width: 90%; margin: auto;"
autoplay loop muted playsinline
src="https://huggingface.co/datasets/huggingface/documentation-images/resolve/main/blog/assisted-generation/gif_2_1080p.mov"
></video>
<figcaption>"่ชๅๅฝ็ๆ่ฟญไปฃๅฐไปๆฆ็ๅๅธไธญ้ๆฉไธไธไธชtokenไปฅ็ๆๆๆฌ"</figcaption>
</figure>
ไธ่ฟฐ่ฟ็จๆฏ่ฟญไปฃ้ๅค็๏ผ็ดๅฐ่พพๅฐๆไธชๅๆญขๆกไปถใ็ๆณๆ
ๅตไธ๏ผๅๆญขๆกไปถ็ฑๆจกๅๅณๅฎ๏ผ่ฏฅๆจกๅๅบๅญฆไผๅจไฝๆถ่พๅบไธไธช็ปๆๅบๅ๏ผ`EOS`๏ผๆ ่ฎฐใๅฆๆไธๆฏ่ฟ็งๆ
ๅต๏ผ็ๆๅฐๅจ่พพๅฐๆไธช้ขๅฎไน็ๆๅคง้ฟๅบฆๆถๅๆญขใ
ๆญฃ็กฎ่ฎพ็ฝฎ`token`้ๆฉๆญฅ้ชคๅๅๆญขๆกไปถๅฏนไบ่ฎฉไฝ ็ๆจกๅๆ็
ง้ขๆ็ๆนๅผๆง่กไปปๅก่ณๅ
ณ้่ฆใ่ฟๅฐฑๆฏไธบไปไนๆไปฌไธบๆฏไธชๆจกๅ้ฝๆไธไธช[~generation.GenerationConfig]ๆไปถ๏ผๅฎๅ
ๅซไธไธชๆๆไธ้็้ป่ฎค็ๆๅๆฐ้
็ฝฎ๏ผๅนถไธๆจๆจกๅไธ่ตทๅ ่ฝฝใ
่ฎฉๆไปฌ่ฐ่ฐไปฃ็ ๏ผ
<Tip>
ๅฆๆๆจๅฏนๅบๆฌ็LLMไฝฟ็จๆๅ
ด่ถฃ๏ผๆไปฌ้ซ็บง็[`Pipeline`](pipeline_tutorial)ๆฅๅฃๆฏไธไธชๅพๅฅฝ็่ตท็นใ็ถ่๏ผLLMs้ๅธธ้่ฆๅ`quantization`ๅ`token้ๆฉๆญฅ้ชค็็ฒพ็ปๆงๅถ`็ญ้ซ็บงๅ่ฝ๏ผ่ฟๆๅฅฝ้่ฟ[`~generation.GenerationMixin.generate`]ๆฅๅฎๆใไฝฟ็จLLM่ฟ่ก่ชๅๅฝ็ๆไนๆฏ่ตๆบๅฏ้ๅ็ๆไฝ๏ผๅบ่ฏฅๅจGPUไธๆง่กไปฅ่ทๅพ่ถณๅค็ๅๅ้ใ
</Tip>
้ฆๅ
๏ผๆจ้่ฆๅ ่ฝฝๆจกๅใ
```py
>>> from transformers import AutoModelForCausalLM
>>> model = AutoModelForCausalLM.from_pretrained(
... "mistralai/Mistral-7B-v0.1", device_map="auto", load_in_4bit=True
... )
```
ๆจๅฐไผๆณจๆๅฐๅจ`from_pretrained`่ฐ็จไธญ็ไธคไธชๆ ๅฟ๏ผ
- `device_map`็กฎไฟๆจกๅ่ขซ็งปๅจๅฐๆจ็GPU(s)ไธ
- `load_in_4bit`ๅบ็จ[4ไฝๅจๆ้ๅ](main_classes/quantization)ๆฅๆๅคงๅฐๅๅฐ่ตๆบ้ๆฑ
่ฟๆๅ
ถไปๆนๅผๆฅๅๅงๅไธไธชๆจกๅ๏ผไฝ่ฟๆฏไธไธชๅผๅงไฝฟ็จLLMๅพๅฅฝ็่ตท็นใ
ๆฅไธๆฅ๏ผไฝ ้่ฆไฝฟ็จไธไธช[tokenizer](tokenizer_summary)ๆฅ้ขๅค็ไฝ ็ๆๆฌ่พๅ
ฅใ
```py
>>> from transformers import AutoTokenizer
>>> tokenizer = AutoTokenizer.from_pretrained("mistralai/Mistral-7B-v0.1", padding_side="left")
>>> model_inputs = tokenizer(["A list of colors: red, blue"], return_tensors="pt").to("cuda")
```
`model_inputs`ๅ้ไฟๅญ็ๅ่ฏๅ็ๆๆฌ่พๅ
ฅไปฅๅๆณจๆๅๆฉ็ ใๅฐฝ็ฎก[`~generation.GenerationMixin.generate`]ๅจๆชไผ ้ๆณจๆๅๆฉ็ ๆถไผๅฐฝๅ
ถๆ่ฝๆจๆญๅบๆณจๆๅๆฉ็ ๏ผไฝๅปบ่ฎฎๅฐฝๅฏ่ฝไผ ้ๅฎไปฅ่ทๅพๆไฝณ็ปๆใ
ๅจๅฏน่พๅ
ฅ่ฟ่กๅ่ฏๅ๏ผๅฏไปฅ่ฐ็จ[`~generation.GenerationMixin.generate`]ๆนๆณๆฅ่ฟๅ็ๆ็`tokens`ใ็ๆ็`tokens`ๅบ่ฏฅๅจๆๅฐไนๅ่ฝฌๆขไธบๆๆฌใ
```py
>>> generated_ids = model.generate(**model_inputs)
>>> tokenizer.batch_decode(generated_ids, skip_special_tokens=True)[0]
'A list of colors: red, blue, green, yellow, orange, purple, pink,'
```
ๆๅ๏ผๆจไธ้่ฆไธๆฌกๅค็ไธไธชๅบๅ๏ผๆจๅฏไปฅๆน้่พๅ
ฅ๏ผ่ฟๅฐๅจๅฐๅปถ่ฟๅไฝๅ
ๅญๆๆฌไธๆพ่ๆ้ซๅๅ้ใๆจๅช้่ฆ็กฎไฟๆญฃ็กฎๅฐๅกซๅ
ๆจ็่พๅ
ฅ๏ผ่ฏฆ่งไธๆ๏ผใ
```py
>>> tokenizer.pad_token = tokenizer.eos_token # Most LLMs don't have a pad token by default
>>> model_inputs = tokenizer(
... ["A list of colors: red, blue", "Portugal is"], return_tensors="pt", padding=True
... ).to("cuda")
>>> generated_ids = model.generate(**model_inputs)
>>> tokenizer.batch_decode(generated_ids, skip_special_tokens=True)
['A list of colors: red, blue, green, yellow, orange, purple, pink,',
'Portugal is a country in southwestern Europe, on the Iber']
```
ๅฐฑๆฏ่ฟๆ ท๏ผๅจๅ ่กไปฃ็ ไธญ๏ผๆจๅฐฑๅฏไปฅๅฉ็จLLM็ๅผบๅคงๅ่ฝใ
## ๅธธ่ง้ท้ฑ
ๆ่ฎธๅค[็ๆ็ญ็ฅ](generation_strategies)๏ผๆๆถ้ป่ฎคๅผๅฏ่ฝไธ้ๅๆจ็็จไพใๅฆๆๆจ็่พๅบไธๆจๆๆ็็ปๆไธๅน้
๏ผๆไปฌๅทฒ็ปๅๅปบไบไธไธชๆๅธธ่ง็้ท้ฑๅ่กจไปฅๅๅฆไฝ้ฟๅ
ๅฎไปฌใ
```py
>>> from transformers import AutoModelForCausalLM, AutoTokenizer
>>> tokenizer = AutoTokenizer.from_pretrained("mistralai/Mistral-7B-v0.1")
>>> tokenizer.pad_token = tokenizer.eos_token # Most LLMs don't have a pad token by default
>>> model = AutoModelForCausalLM.from_pretrained(
... "mistralai/Mistral-7B-v0.1", device_map="auto", load_in_4bit=True
... )
```
### ็ๆ็่พๅบๅคช็ญ/ๅคช้ฟ
ๅฆๆๅจ[`~generation.GenerationConfig`]ๆไปถไธญๆฒกๆๆๅฎ๏ผ`generate`้ป่ฎค่ฟๅ20ไธชtokensใๆไปฌๅผบ็ๅปบ่ฎฎๅจๆจ็`generate`่ฐ็จไธญๆๅจ่ฎพ็ฝฎ`max_new_tokens`ไปฅๆงๅถๅฎๅฏไปฅ่ฟๅ็ๆๅคงๆฐtokensๆฐ้ใ่ฏทๆณจๆ๏ผLLMs๏ผๆดๅ็กฎๅฐ่ฏด๏ผไป
[่งฃ็ ๅจๆจกๅ](https://huggingface.co/learn/nlp-course/chapter1/6?fw=pt)๏ผไนๅฐ่พๅ
ฅๆ็คบไฝไธบ่พๅบ็ไธ้จๅ่ฟๅใ
```py
>>> model_inputs = tokenizer(["A sequence of numbers: 1, 2"], return_tensors="pt").to("cuda")
>>> # By default, the output will contain up to 20 tokens
>>> generated_ids = model.generate(**model_inputs)
>>> tokenizer.batch_decode(generated_ids, skip_special_tokens=True)[0]
'A sequence of numbers: 1, 2, 3, 4, 5'
>>> # Setting `max_new_tokens` allows you to control the maximum length
>>> generated_ids = model.generate(**model_inputs, max_new_tokens=50)
>>> tokenizer.batch_decode(generated_ids, skip_special_tokens=True)[0]
'A sequence of numbers: 1, 2, 3, 4, 5, 6, 7, 8, 9, 10, 11, 12, 13, 14, 15, 16,'
```
### ้่ฏฏ็็ๆๆจกๅผ
้ป่ฎคๆ
ๅตไธ๏ผ้ค้ๅจ[`~generation.GenerationConfig`]ๆไปถไธญๆๅฎ๏ผๅฆๅ`generate`ไผๅจๆฏไธช่ฟญไปฃไธญ้ๆฉๆๅฏ่ฝ็token๏ผ่ดชๅฉช่งฃ็ ๏ผใๅฏนไบๆจ็ไปปๅก๏ผ่ฟๅฏ่ฝๆฏไธ็ๆณ็๏ผๅ่ๅคฉๆบๅจไบบๆๅไฝๆ็ซ ่ฟๆ ท็ๅ้ ๆงไปปๅกๅ็ไบ้ๆ ทใๅฆไธๆน้ข๏ผๅ้ณ้ข่ฝฌๅฝๆ็ฟป่ฏ่ฟๆ ท็ๅบไบ่พๅ
ฅ็ไปปๅกๅ็ไบ่ดชๅฉช่งฃ็ ใ้่ฟๅฐ`do_sample=True`ๅฏ็จ้ๆ ท๏ผๆจๅฏไปฅๅจ่ฟ็ฏ[ๅๅฎขๆ็ซ ](https://huggingface.co/blog/how-to-generate)ไธญไบ่งฃๆดๅคๅ
ณไบ่ฟไธช่ฏ้ข็ไฟกๆฏใ
```py
>>> # Set seed or reproducibility -- you don't need this unless you want full reproducibility
>>> from transformers import set_seed
>>> set_seed(42)
>>> model_inputs = tokenizer(["I am a cat."], return_tensors="pt").to("cuda")
>>> # LLM + greedy decoding = repetitive, boring output
>>> generated_ids = model.generate(**model_inputs)
>>> tokenizer.batch_decode(generated_ids, skip_special_tokens=True)[0]
'I am a cat. I am a cat. I am a cat. I am a cat'
>>> # With sampling, the output becomes more creative!
>>> generated_ids = model.generate(**model_inputs, do_sample=True)
>>> tokenizer.batch_decode(generated_ids, skip_special_tokens=True)[0]
'I am a cat. Specifically, I am an indoor-only cat. I'
```
### ้่ฏฏ็ๅกซๅ
ไฝ็ฝฎ
LLMsๆฏ[ไป
่งฃ็ ๅจ](https://huggingface.co/learn/nlp-course/chapter1/6?fw=pt)ๆถๆ๏ผๆๅณ็ๅฎไปฌไผๆ็ปญ่ฟญไปฃๆจ็่พๅ
ฅๆ็คบใๅฆๆๆจ็่พๅ
ฅ้ฟๅบฆไธ็ธๅ๏ผๅ้่ฆๅฏนๅฎไปฌ่ฟ่กๅกซๅ
ใ็ฑไบLLMsๆฒกๆๆฅๅ่ฟไป`pad tokens`็ปง็ปญ่ฎญ็ป๏ผๅ ๆญคๆจ็่พๅ
ฅ้่ฆๅทฆๅกซๅ
ใ็กฎไฟๅจ็ๆๆถไธ่ฆๅฟ่ฎฐไผ ้ๆณจๆๅๆฉ็ ๏ผ
```py
>>> # The tokenizer initialized above has right-padding active by default: the 1st sequence,
>>> # which is shorter, has padding on the right side. Generation fails to capture the logic.
>>> model_inputs = tokenizer(
... ["1, 2, 3", "A, B, C, D, E"], padding=True, return_tensors="pt"
... ).to("cuda")
>>> generated_ids = model.generate(**model_inputs)
>>> tokenizer.batch_decode(generated_ids, skip_special_tokens=True)[0]
'1, 2, 33333333333'
>>> # With left-padding, it works as expected!
>>> tokenizer = AutoTokenizer.from_pretrained("mistralai/Mistral-7B-v0.1", padding_side="left")
>>> tokenizer.pad_token = tokenizer.eos_token # Most LLMs don't have a pad token by default
>>> model_inputs = tokenizer(
... ["1, 2, 3", "A, B, C, D, E"], padding=True, return_tensors="pt"
... ).to("cuda")
>>> generated_ids = model.generate(**model_inputs)
>>> tokenizer.batch_decode(generated_ids, skip_special_tokens=True)[0]
'1, 2, 3, 4, 5, 6,'
```
### ้่ฏฏ็ๆ็คบ
ไธไบๆจกๅๅไปปๅกๆๆๆ็ง่พๅ
ฅๆ็คบๆ ผๅผๆ่ฝๆญฃๅธธๅทฅไฝใๅฝๆชๅบ็จๆญคๆ ผๅผๆถ๏ผๆจๅฐ่ทๅพๆ็ถ็ๆง่ฝไธ้๏ผๆจกๅ่ฝๅทฅไฝ๏ผไฝไธๅฆ้ขๆๆ็คบ้ฃๆ ทๅฅฝใๆๅ
ณๆ็คบ็ๆดๅคไฟกๆฏ๏ผๅ
ๆฌๅชไบๆจกๅๅไปปๅก้่ฆๅฐๅฟ๏ผๅฏๅจ[ๆๅ](tasks/prompting)ไธญๆพๅฐใ่ฎฉๆไปฌ็ไธไธชไฝฟ็จ[่ๅคฉๆจกๆฟ](chat_templating)็่ๅคฉLLM็คบไพ๏ผ
```python
>>> tokenizer = AutoTokenizer.from_pretrained("HuggingFaceH4/zephyr-7b-alpha")
>>> model = AutoModelForCausalLM.from_pretrained(
... "HuggingFaceH4/zephyr-7b-alpha", device_map="auto", load_in_4bit=True
... )
>>> set_seed(0)
>>> prompt = """How many helicopters can a human eat in one sitting? Reply as a thug."""
>>> model_inputs = tokenizer([prompt], return_tensors="pt").to("cuda")
>>> input_length = model_inputs.input_ids.shape[1]
>>> generated_ids = model.generate(**model_inputs, max_new_tokens=20)
>>> print(tokenizer.batch_decode(generated_ids[:, input_length:], skip_special_tokens=True)[0])
"I'm not a thug, but i can tell you that a human cannot eat"
>>> # Oh no, it did not follow our instruction to reply as a thug! Let's see what happens when we write
>>> # a better prompt and use the right template for this model (through `tokenizer.apply_chat_template`)
>>> set_seed(0)
>>> messages = [
... {
... "role": "system",
... "content": "You are a friendly chatbot who always responds in the style of a thug",
... },
... {"role": "user", "content": "How many helicopters can a human eat in one sitting?"},
... ]
>>> model_inputs = tokenizer.apply_chat_template(messages, add_generation_prompt=True, return_tensors="pt").to("cuda")
>>> input_length = model_inputs.shape[1]
>>> generated_ids = model.generate(model_inputs, do_sample=True, max_new_tokens=20)
>>> print(tokenizer.batch_decode(generated_ids[:, input_length:], skip_special_tokens=True)[0])
'None, you thug. How bout you try to focus on more useful questions?'
>>> # As we can see, it followed a proper thug style ๐
```
## ๆดๅค่ตๆบ
่ฝ็ถ่ชๅๅฝ็ๆ่ฟ็จ็ธๅฏน็ฎๅ๏ผไฝ่ฆๅ
ๅๅฉ็จLLMๅฏ่ฝๆฏไธไธชๅ
ทๆๆๆๆง็ไปปๅก๏ผๅ ไธบๅพๅค็ปไปถๅคๆไธๅฏๅๅ
ณ่ใไปฅไธๆฏๅธฎๅฉๆจๆทฑๅ
ฅไบ่งฃLLMไฝฟ็จๅ็่งฃ็ไธไธๆญฅ๏ผ
### ้ซ็บง็ๆ็จๆณ
1. [ๆๅ](generation_strategies)๏ผไป็ปๅฆไฝๆงๅถไธๅ็็ๆๆนๆณใๅฆไฝ่ฎพ็ฝฎ็ๆ้
็ฝฎๆไปถไปฅๅๅฆไฝ่ฟ่ก่พๅบๆตๅผไผ ่พ๏ผ
2. [ๆๅ](chat_templating)๏ผไป็ป่ๅคฉLLMs็ๆ็คบๆจกๆฟ๏ผ
3. [ๆๅ](tasks/prompting)๏ผไป็ปๅฆไฝๅ
ๅๅฉ็จๆ็คบ่ฎพ่ฎก๏ผ
4. APIๅ่ๆๆกฃ๏ผๅ
ๆฌ[`~generation.GenerationConfig`]ใ[`~generation.GenerationMixin.generate`]ๅ[ไธ็ๆ็ธๅ
ณ็็ฑป](internal/generation_utils)ใ
### LLMๆ่กๆฆ
1. [Open LLM Leaderboard](https://huggingface.co/spaces/HuggingFaceH4/open_llm_leaderboard), ไพง้ไบๅผๆบๆจกๅ็่ดจ้;
2. [Open LLM-Perf Leaderboard](https://huggingface.co/spaces/optimum/llm-perf-leaderboard), ไพง้ไบLLM็ๅๅ้.
### ๅปถ่ฟใๅๅ้ๅๅ
ๅญๅฉ็จ็
1. [ๆๅ](llm_tutorial_optimization),ๅฆไฝไผๅLLMsไปฅๆ้ซ้ๅบฆๅๅ
ๅญๅฉ็จ๏ผ
2. [ๆๅ](main_classes/quantization), ๅ
ณไบ`quantization`๏ผๅฆbitsandbytesๅautogptq็ๆๅ๏ผๆๆจๅฆไฝๅคงๅน
้ไฝๅ
ๅญ้ๆฑใ
### ็ธๅ
ณๅบ
1. [`text-generation-inference`](https://github.com/huggingface/text-generation-inference), ไธไธช้ขๅ็ไบง็LLMๆๅกๅจ๏ผ
2. [`optimum`](https://github.com/huggingface/optimum), ไธไธช๐ค Transformers็ๆฉๅฑ๏ผไผๅ็นๅฎ็กฌไปถ่ฎพๅค็ๆง่ฝ
| transformers/docs/source/zh/llm_tutorial.md/0 | {
"file_path": "transformers/docs/source/zh/llm_tutorial.md",
"repo_id": "transformers",
"token_count": 7107
} | 275 |
<!--Copyright 2023 The HuggingFace Team. All rights reserved.
Licensed under the Apache License, Version 2.0 (the "License"); you may not use this file except in compliance with
the License. You may obtain a copy of the License at
http://www.apache.org/licenses/LICENSE-2.0
Unless required by applicable law or agreed to in writing, software distributed under the License is distributed on
an "AS IS" BASIS, WITHOUT WARRANTIES OR CONDITIONS OF ANY KIND, either express or implied. See the License for the
specific language governing permissions and limitations under the License.
โ ๏ธ Note that this file is in Markdown but contain specific syntax for our doc-builder (similar to MDX) that may not be
rendered properly in your Markdown viewer.
-->
# ้ๅ ๐ค Transformers ๆจกๅ
## AWQ้ๆ
AWQๆนๆณๅทฒ็ปๅจ[*AWQ: Activation-aware Weight Quantization for LLM Compression and Acceleration*่ฎบๆ](https://arxiv.org/abs/2306.00978)ไธญๅผๅ
ฅใ้่ฟAWQ๏ผๆจๅฏไปฅไปฅ4ไฝ็ฒพๅบฆ่ฟ่กๆจกๅ๏ผๅๆถไฟ็ๅ
ถๅๅงๆง่ฝ๏ผๅณๆฒกๆๆง่ฝ้็บง๏ผ๏ผๅนถๅ
ทๆๆฏไธ้ขไป็ป็ๅ
ถไป้ๅๆนๆณๆดๅบ่ฒ็ๅๅ้ - ่พพๅฐไธ็บฏ`float16`ๆจ็็ธไผผ็ๅๅ้ใ
ๆไปฌ็ฐๅจๆฏๆไฝฟ็จไปปไฝAWQๆจกๅ่ฟ่กๆจ็๏ผ่ฟๆๅณ็ไปปไฝไบบ้ฝๅฏไปฅๅ ่ฝฝๅไฝฟ็จๅจHubไธๆจ้ๆๆฌๅฐไฟๅญ็AWQๆ้ใ่ฏทๆณจๆ๏ผไฝฟ็จAWQ้่ฆ่ฎฟ้ฎNVIDIA GPUใ็ฎๅไธๆฏๆCPUๆจ็ใ
### ้ๅไธไธชๆจกๅ
ๆไปฌๅปบ่ฎฎ็จๆทๆฅ็็ๆ็ณป็ปไธญไธๅ็็ฐๆๅทฅๅ
ท๏ผไปฅไฝฟ็จAWQ็ฎๆณๅฏนๅ
ถๆจกๅ่ฟ่ก้ๅ๏ผไพๅฆ๏ผ
- [`llm-awq`](https://github.com/mit-han-lab/llm-awq)๏ผๆฅ่ชMIT Han Lab
- [`autoawq`](https://github.com/casper-hansen/AutoAWQ)๏ผๆฅ่ช[`casper-hansen`](https://github.com/casper-hansen)
- Intel neural compressor๏ผๆฅ่ชIntel - ้่ฟ[`optimum-intel`](https://huggingface.co/docs/optimum/main/en/intel/optimization_inc)ไฝฟ็จ
็ๆ็ณป็ปไธญๅฏ่ฝๅญๅจ่ฎธๅคๅ
ถไปๅทฅๅ
ท๏ผ่ฏท้ๆถๆๅบPRๅฐๅฎไปฌๆทปๅ ๅฐๅ่กจไธญใ
็ฎๅไธ๐ค Transformers็้ๆไป
้็จไบไฝฟ็จ`autoawq`ๅ`llm-awq`้ๅๅ็ๆจกๅใๅคงๅคๆฐไฝฟ็จ`auto-awq`้ๅ็ๆจกๅๅฏไปฅๅจ๐ค Hub็[`TheBloke`](https://huggingface.co/TheBloke)ๅฝๅ็ฉบ้ดไธๆพๅฐ๏ผ่ฆไฝฟ็จ`llm-awq`ๅฏนๆจกๅ่ฟ่ก้ๅ๏ผ่ฏทๅ้
[`llm-awq`](https://github.com/mit-han-lab/llm-awq/)็็คบไพๆไปถๅคนไธญ็[`convert_to_hf.py`](https://github.com/mit-han-lab/llm-awq/blob/main/examples/convert_to_hf.py)่ๆฌใ
### ๅ ่ฝฝไธไธช้ๅ็ๆจกๅ
ๆจๅฏไปฅไฝฟ็จ`from_pretrained`ๆนๆณไปHubๅ ่ฝฝไธไธช้ๅๆจกๅใ้่ฟๆฃๆฅๆจกๅ้
็ฝฎๆไปถ๏ผ`configuration.json`๏ผไธญๆฏๅฆๅญๅจ`quantization_config`ๅฑๆง๏ผๆฅ่ฟ่ก็กฎ่ฎคๆจ้็ๆ้ๆฏ้ๅ็ใๆจๅฏไปฅ้่ฟๆฃๆฅๅญๆฎต`quantization_config.quant_method`ๆฅ็กฎ่ฎคๆจกๅๆฏๅฆไปฅAWQๆ ผๅผ่ฟ่ก้ๅ๏ผ่ฏฅๅญๆฎตๅบ่ฏฅ่ฎพ็ฝฎไธบ`"awq"`ใ่ฏทๆณจๆ๏ผไธบไบๆง่ฝๅๅ ๏ผ้ป่ฎคๆ
ๅตไธๅ ่ฝฝๆจกๅๅฐ่ฎพ็ฝฎๅ
ถไปๆ้ไธบ`float16`ใๅฆๆๆจๆณๆดๆน่ฟ็ง่ฎพ็ฝฎ๏ผๅฏไปฅ้่ฟๅฐ`torch_dtype`ๅๆฐ่ฎพ็ฝฎไธบ`torch.float32`ๆ`torch.bfloat16`ใๅจไธ้ข็้จๅไธญ๏ผๆจๅฏไปฅๆพๅฐไธไบ็คบไพ็ๆฎตๅnotebookใ
## ็คบไพไฝฟ็จ
้ฆๅ
๏ผๆจ้่ฆๅฎ่ฃ
[`autoawq`](https://github.com/casper-hansen/AutoAWQ)ๅบ
```bash
pip install autoawq
```
```python
from transformers import AutoModelForCausalLM, AutoTokenizer
model_id = "TheBloke/zephyr-7B-alpha-AWQ"
model = AutoModelForCausalLM.from_pretrained(model_id, device_map="cuda:0")
```
ๅฆๆๆจ้ฆๅ
ๅฐๆจกๅๅ ่ฝฝๅฐCPUไธ๏ผ่ฏท็กฎไฟๅจไฝฟ็จไนๅๅฐๅ
ถ็งปๅจๅฐGPU่ฎพๅคไธใ
```python
from transformers import AutoModelForCausalLM, AutoTokenizer
model_id = "TheBloke/zephyr-7B-alpha-AWQ"
model = AutoModelForCausalLM.from_pretrained(model_id).to("cuda:0")
```
### ็ปๅ AWQ ๅ Flash Attention
ๆจๅฏไปฅๅฐAWQ้ๅไธFlash Attention็ปๅ่ตทๆฅ๏ผๅพๅฐไธไธชๆข่ขซ้ๅๅๆดๅฟซ้็ๆจกๅใๅช้ไฝฟ็จ`from_pretrained`ๅ ่ฝฝๆจกๅ๏ผๅนถไผ ้`attn_implementation="flash_attention_2"`ๅๆฐใ
```python
from transformers import AutoModelForCausalLM, AutoTokenizer
model = AutoModelForCausalLM.from_pretrained("TheBloke/zephyr-7B-alpha-AWQ", attn_implementation="flash_attention_2", device_map="cuda:0")
```
### ๅบๅๆต่ฏ
ๆไปฌไฝฟ็จ[`optimum-benchmark`](https://github.com/huggingface/optimum-benchmark)ๅบ่ฟ่กไบไธไบ้ๅบฆใๅๅ้ๅๅปถ่ฟๅบๅๆต่ฏใ
่ฏทๆณจๆ๏ผๅจ็ผๅๆฌๆๆกฃ้จๅๆถ๏ผๅฏ็จ็้ๅๆนๆณๅ
ๆฌ๏ผ`awq`ใ`gptq`ๅ`bitsandbytes`ใ
ๅบๅๆต่ฏๅจไธๅฐNVIDIA-A100ๅฎไพไธ่ฟ่ก๏ผไฝฟ็จ[`TheBloke/Mistral-7B-v0.1-AWQ`](https://huggingface.co/TheBloke/Mistral-7B-v0.1-AWQ)ไฝไธบAWQๆจกๅ๏ผ[`TheBloke/Mistral-7B-v0.1-GPTQ`](https://huggingface.co/TheBloke/Mistral-7B-v0.1-GPTQ)ไฝไธบGPTQๆจกๅใๆไปฌ่ฟๅฐๅ
ถไธ`bitsandbytes`้ๅๆจกๅๅ`float16`ๆจกๅ่ฟ่กไบๅฏนๆฏใไปฅไธๆฏไธไบ็ปๆ็คบไพ๏ผ
<div style="text-align: center">
<img src="https://huggingface.co/datasets/huggingface/documentation-images/resolve/main/quantization/forward_memory_plot.png">
</div>
<div style="text-align: center">
<img src="https://huggingface.co/datasets/huggingface/documentation-images/resolve/main/quantization/generate_memory_plot.png">
</div>
<div style="text-align: center">
<img src="https://huggingface.co/datasets/huggingface/documentation-images/resolve/main/quantization/generate_throughput_plot.png">
</div>
<div style="text-align: center">
<img src="https://huggingface.co/datasets/huggingface/documentation-images/resolve/main/quantization/forward_latency_plot.png">
</div>
ไฝ ๅฏไปฅๅจ[ๆญค้พๆฅ](https://github.com/huggingface/optimum-benchmark/tree/main/examples/running-mistrals)ไธญๆพๅฐๅฎๆด็็ปๆไปฅๅๅ
็ๆฌใ
ไป็ปๆๆฅ็๏ผAWQ้ๅๆนๆณๆฏๆจ็ใๆๆฌ็ๆไธญๆๅฟซ็้ๅๆนๆณ๏ผๅนถไธๅจๆๆฌ็ๆ็ๅณฐๅผๅ
ๅญๆน้ขๅฑไบๆไฝใ็ถ่๏ผๅฏนไบๆฏๆนๆฐๆฎ๏ผAWQไผผไนๆๆๅคง็ๅๅๅปถ่ฟใ
### Google colab ๆผ็คบ
ๆฅ็ๅฆไฝๅจ[Google Colabๆผ็คบ](https://colab.research.google.com/drive/1HzZH89yAXJaZgwJDhQj9LqSBux932BvY)ไธญไฝฟ็จๆญค้ๆ๏ผ
### AwqConfig
[[autodoc]] AwqConfig
## `AutoGPTQ` ้ๆ
๐ค Transformersๅทฒ็ปๆดๅไบ`optimum` API๏ผ็จไบๅฏน่ฏญ่จๆจกๅๆง่กGPTQ้ๅใๆจๅฏไปฅไปฅ8ใ4ใ3็่ณ2ไฝๅ ่ฝฝๅ้ๅๆจ็ๆจกๅ๏ผ่ๆง่ฝๆ ๆๆพไธ้๏ผๅนถไธๆจ็้ๅบฆๆดๅฟซ๏ผ่ฟๅๅฐๅคงๅคๆฐGPU็กฌไปถ็ๆฏๆใ
่ฆไบ่งฃๆดๅคๅ
ณไบ้ๅๆจกๅ็ไฟกๆฏ๏ผ่ฏทๆฅ็๏ผ
- [GPTQ](https://arxiv.org/pdf/2210.17323.pdf)่ฎบๆ
- `optimum`ๅ
ณไบGPTQ้ๅ็[ๆๅ](https://huggingface.co/docs/optimum/llm_quantization/usage_guides/quantization)
- ็จไฝๅ็ซฏ็[`AutoGPTQ`](https://github.com/PanQiWei/AutoGPTQ)ๅบ
### ่ฆๆฑ
ไธบไบ่ฟ่กไธ้ข็ไปฃ็ ๏ผๆจ้่ฆๅฎ่ฃ
๏ผ
- ๅฎ่ฃ
ๆๆฐ็ๆฌ็ `AutoGPTQ` ๅบ
`pip install auto-gptq`
- ไปๆบไปฃ็ ๅฎ่ฃ
ๆๆฐ็ๆฌ็`optimum`
`pip install git+https://github.com/huggingface/optimum.git`
- ไปๆบไปฃ็ ๅฎ่ฃ
ๆๆฐ็ๆฌ็`transformers`
`pip install git+https://github.com/huggingface/transformers.git`
- ๅฎ่ฃ
ๆๆฐ็ๆฌ็`accelerate`ๅบ๏ผ
`pip install --upgrade accelerate`
่ฏทๆณจๆ๏ผ็ฎๅGPTQ้ๆไป
ๆฏๆๆๆฌๆจกๅ๏ผๅฏนไบ่ง่งใ่ฏญ้ณๆๅคๆจกๆๆจกๅๅฏ่ฝไผ้ๅฐ้ขๆไปฅๅค็ปๆใ
### ๅ ่ฝฝๅ้ๅๆจกๅ
GPTQๆฏไธ็งๅจไฝฟ็จ้ๅๆจกๅไนๅ้่ฆ่ฟ่กๆ้ๆ กๅ็้ๅๆนๆณใๅฆๆๆจๆณไปๅคดๅผๅงๅฏนtransformersๆจกๅ่ฟ่ก้ๅ๏ผ็ๆ้ๅๆจกๅๅฏ่ฝ้่ฆไธไบๆถ้ด๏ผๅจGoogle Colabไธๅฏน`facebook/opt-350m`ๆจกๅ้ๅ็บฆไธบ5ๅ้๏ผใ
ๅ ๆญค๏ผๆไธค็งไธๅ็ๆ
ๅตไธๆจๅฏ่ฝๆณไฝฟ็จGPTQ้ๅๆจกๅใ็ฌฌไธ็งๆ
ๅตๆฏๅ ่ฝฝๅทฒ็ป็ฑๅ
ถไป็จๆทๅจHubไธ้ๅ็ๆจกๅ๏ผ็ฌฌไบ็งๆ
ๅตๆฏไปๅคดๅผๅงๅฏนๆจ็ๆจกๅ่ฟ่ก้ๅๅนถไฟๅญๆๆจ้ๅฐHub๏ผไปฅไพฟๅ
ถไป็จๆทไนๅฏไปฅไฝฟ็จๅฎใ
#### GPTQ ้
็ฝฎ
ไธบไบๅ ่ฝฝๅ้ๅไธไธชๆจกๅ๏ผๆจ้่ฆๅๅปบไธไธช[`GPTQConfig`]ใๆจ้่ฆไผ ้`bits`็ๆฐ้๏ผไธไธช็จไบๆ กๅ้ๅ็`dataset`๏ผไปฅๅๆจกๅ็`tokenizer`ไปฅๅๅคๆฐๆฎ้ใ
```python
model_id = "facebook/opt-125m"
tokenizer = AutoTokenizer.from_pretrained(model_id)
gptq_config = GPTQConfig(bits=4, dataset = "c4", tokenizer=tokenizer)
```
่ฏทๆณจๆ๏ผๆจๅฏไปฅๅฐ่ชๅทฑ็ๆฐๆฎ้ไปฅๅญ็ฌฆไธฒๅ่กจๅฝขๅผไผ ้ๅฐๆจกๅใ็ถ่๏ผๅผบ็ๅปบ่ฎฎๆจไฝฟ็จGPTQ่ฎบๆไธญๆไพ็ๆฐๆฎ้ใ
```python
dataset = ["auto-gptq is an easy-to-use model quantization library with user-friendly apis, based on GPTQ algorithm."]
quantization = GPTQConfig(bits=4, dataset = dataset, tokenizer=tokenizer)
```
#### ้ๅ
ๆจๅฏไปฅ้่ฟไฝฟ็จ`from_pretrained`ๅนถ่ฎพ็ฝฎ`quantization_config`ๆฅๅฏนๆจกๅ่ฟ่ก้ๅใ
```python
from transformers import AutoModelForCausalLM
model = AutoModelForCausalLM.from_pretrained(model_id, quantization_config=gptq_config)
```
่ฏทๆณจๆ๏ผๆจ้่ฆไธไธชGPUๆฅ้ๅๆจกๅใๆไปฌๅฐๆจกๅๆพๅจcpuไธญ๏ผๅนถๅฐๆจกๅๆฅๅ็งปๅจๅฐgpuไธญ๏ผไปฅไพฟๅฏนๅ
ถ่ฟ่ก้ๅใ
ๅฆๆๆจๆณๅจไฝฟ็จ CPU ๅธ่ฝฝ็ๅๆถๆๅคงๅ GPU ไฝฟ็จ็๏ผๆจๅฏไปฅ่ฎพ็ฝฎ `device_map = "auto"`ใ
```python
from transformers import AutoModelForCausalLM
model = AutoModelForCausalLM.from_pretrained(model_id, device_map="auto", quantization_config=gptq_config)
```
่ฏทๆณจๆ๏ผไธๆฏๆ็ฃ็ๅธ่ฝฝใๆญคๅค๏ผๅฆๆ็ฑไบๆฐๆฎ้่ๅ
ๅญไธ่ถณ๏ผๆจๅฏ่ฝ้่ฆๅจ`from_pretrained`ไธญ่ฎพ็ฝฎ`max_memory`ใๆฅ็่ฟไธช[ๆๅ](https://huggingface.co/docs/accelerate/usage_guides/big_modeling#designing-a-device-map)ไปฅไบ่งฃๆๅ
ณ`device_map`ๅ`max_memory`็ๆดๅคไฟกๆฏใ
<Tip warning={true}>
็ฎๅ๏ผGPTQ้ๅไป
้็จไบๆๆฌๆจกๅใๆญคๅค๏ผ้ๅ่ฟ็จๅฏ่ฝไผ่ฑ่ดนๅพๅคๆถ้ด๏ผๅ
ทไฝๅๅณไบ็กฌไปถๆง่ฝ๏ผ175BๆจกๅๅจNVIDIA A100ไธ้่ฆ4ๅฐๆถ๏ผใ่ฏทๅจHubไธๆฃๆฅๆฏๅฆๆๆจกๅ็GPTQ้ๅ็ๆฌใๅฆๆๆฒกๆ๏ผๆจๅฏไปฅๅจGitHubไธๆไบค้ๆฑใ
</Tip>
### ๆจ้้ๅๆจกๅๅฐ ๐ค Hub
ๆจๅฏไปฅไฝฟ็จ`push_to_hub`ๅฐ้ๅๆจกๅๅไปปไฝๆจกๅไธๆ ทๆจ้ๅฐHubใ้ๅ้
็ฝฎๅฐไธๆจกๅไธ่ตทไฟๅญๅๆจ้ใ
```python
quantized_model.push_to_hub("opt-125m-gptq")
tokenizer.push_to_hub("opt-125m-gptq")
```
ๅฆๆๆจๆณๅจๆฌๅฐ่ฎก็ฎๆบไธไฟๅญ้ๅๆจกๅ๏ผๆจไนๅฏไปฅไฝฟ็จ`save_pretrained`ๆฅๅฎๆ๏ผ
```python
quantized_model.save_pretrained("opt-125m-gptq")
tokenizer.save_pretrained("opt-125m-gptq")
```
่ฏทๆณจๆ๏ผๅฆๆๆจ้ๅๆจกๅๆถๆณไฝฟ็จ`device_map`๏ผ่ฏท็กฎไฟๅจไฟๅญไนๅๅฐๆดไธชๆจกๅ็งปๅจๅฐๆจ็GPUๆCPUไนไธใ
```python
quantized_model.to("cpu")
quantized_model.save_pretrained("opt-125m-gptq")
```
### ไป ๐ค Hub ๅ ่ฝฝไธไธช้ๅๆจกๅ
ๆจๅฏไปฅไฝฟ็จ`from_pretrained`ไปHubๅ ่ฝฝ้ๅๆจกๅใ
่ฏท็กฎไฟๆจ้ๆ้ๆฏ้ๅ็๏ผๆฃๆฅๆจกๅ้
็ฝฎๅฏน่ฑกไธญๆฏๅฆๅญๅจ`quantization_config`ๅฑๆงใ
```python
from transformers import AutoModelForCausalLM
model = AutoModelForCausalLM.from_pretrained("{your_username}/opt-125m-gptq")
```
ๅฆๆๆจๆณๆดๅฟซๅฐๅ ่ฝฝๆจกๅ๏ผๅนถไธไธ้่ฆๅ้
ๆฏๅฎ้
้่ฆๅ
ๅญๆดๅค็ๅ
ๅญ๏ผ้ๅๆจกๅไนไฝฟ็จ`device_map`ๅๆฐใ็กฎไฟๆจๅทฒๅฎ่ฃ
`accelerate`ๅบใ
```python
from transformers import AutoModelForCausalLM
model = AutoModelForCausalLM.from_pretrained("{your_username}/opt-125m-gptq", device_map="auto")
```
### Exllamaๅ
ๆ ธๅ ๅฟซๆจ็้ๅบฆ
ไฟ็ๆ ผๅผ๏ผๅฏนไบ 4 ไฝๆจกๅ๏ผๆจๅฏไปฅไฝฟ็จ exllama ๅ
ๆ ธๆฅๆ้ซๆจ็้ๅบฆใ้ป่ฎคๆ
ๅตไธ๏ผๅฎๅคไบๅฏ็จ็ถๆใๆจๅฏไปฅ้่ฟๅจ [`GPTQConfig`] ไธญไผ ้ `use_exllama` ๆฅๆดๆนๆญค้
็ฝฎใ่ฟๅฐ่ฆ็ๅญๅจๅจ้
็ฝฎไธญ็้ๅ้
็ฝฎใ่ฏทๆณจๆ๏ผๆจๅช่ฝ่ฆ็ไธๅ
ๆ ธ็ธๅ
ณ็ๅฑๆงใๆญคๅค๏ผๅฆๆๆจๆณไฝฟ็จ exllama ๅ
ๆ ธ๏ผๆดไธชๆจกๅ้่ฆๅ
จ้จ้จ็ฝฒๅจ gpus ไธใๆญคๅค๏ผๆจๅฏไปฅไฝฟ็จ ็ๆฌ > 0.4.2 ็ Auto-GPTQ ๅนถไผ ้ `device_map` = "cpu" ๆฅๆง่ก CPU ๆจ็ใๅฏนไบ CPU ๆจ็๏ผๆจๅฟ
้กปๅจ `GPTQConfig` ไธญไผ ้ `use_exllama = False`ใ
```py
import torch
gptq_config = GPTQConfig(bits=4)
model = AutoModelForCausalLM.from_pretrained("{your_username}/opt-125m-gptq", device_map="auto", quantization_config=gptq_config)
```
้็ exllamav2 ๅ
ๆ ธ็ๅๅธ๏ผไธ exllama ๅ
ๆ ธ็ธๆฏ๏ผๆจๅฏไปฅ่ทๅพๆดๅฟซ็ๆจ็้ๅบฆใๆจๅช้ๅจ [`GPTQConfig`] ไธญไผ ้ `exllama_config={"version": 2}`๏ผ
```py
import torch
gptq_config = GPTQConfig(bits=4, exllama_config={"version":2})
model = AutoModelForCausalLM.from_pretrained("{your_username}/opt-125m-gptq", device_map="auto", quantization_config = gptq_config)
```
่ฏทๆณจๆ๏ผ็ฎๅไป
ๆฏๆ 4 ไฝๆจกๅใๆญคๅค๏ผๅฆๆๆจๆญฃๅจไฝฟ็จ peft ๅฏน้ๅๆจกๅ่ฟ่กๅพฎ่ฐ๏ผๅปบ่ฎฎ็ฆ็จ exllama ๅ
ๆ ธใ
ๆจๅฏไปฅๅจๆญคๆพๅฐ่ฟไบๅ
ๆ ธ็ๅบๅๆต่ฏ [่ฟ้](https://github.com/huggingface/optimum/tree/main/tests/benchmark#gptq-benchmark)
#### ๅพฎ่ฐไธไธช้ๅๆจกๅ
ๅจHugging Face็ๆ็ณป็ป็ๅฎๆนๆฏๆไธ๏ผๆจๅฏไปฅไฝฟ็จGPTQ่ฟ่ก้ๅๅ็ๆจกๅ่ฟ่กๅพฎ่ฐใ
่ฏทๆฅ็`peft`ๅบไบ่งฃๆดๅค่ฏฆๆ
ใ
### ็คบไพๆผ็คบ
่ฏทๆฅ็ Google Colab [notebook](https://colab.research.google.com/drive/1_TIrmuKOFhuRRiTWN94ilkUFu6ZX4ceb?usp=sharing)๏ผไบ่งฃๅฆไฝไฝฟ็จGPTQ้ๅๆจ็ๆจกๅไปฅๅๅฆไฝไฝฟ็จpeftๅพฎ่ฐ้ๅๆจกๅใ
### GPTQConfig
[[autodoc]] GPTQConfig
## `bitsandbytes` ้ๆ
๐ค Transformers ไธ `bitsandbytes` ไธๆๅธธ็จ็ๆจกๅ็ดงๅฏ้ๆใๆจๅฏไปฅไฝฟ็จๅ ่กไปฃ็ ไปฅ 8 ไฝ็ฒพๅบฆๅ ่ฝฝๆจ็ๆจกๅใ
่ชbitsandbytes็0.37.0็ๆฌๅๅธไปฅๆฅ๏ผๅคงๅคๆฐGPU็กฌไปถ้ฝๆฏๆ่ฟไธ็นใ
ๅจ[LLM.int8()](https://arxiv.org/abs/2208.07339)่ฎบๆไธญไบ่งฃๆดๅคๅ
ณไบ้ๅๆนๆณ็ไฟกๆฏ๏ผๆ่
ๅจ[ๅๅฎขๆ็ซ ](https://huggingface.co/blog/hf-bitsandbytes-integration)ไธญไบ่งฃๅ
ณไบๅไฝ็ๆดๅคไฟกๆฏใ
่ชๅ
ถโ0.39.0โ็ๆฌๅๅธไปฅๆฅ๏ผๆจๅฏไปฅไฝฟ็จFP4ๆฐๆฎ็ฑปๅ๏ผ้่ฟ4ไฝ้ๅๅ ่ฝฝไปปไฝๆฏๆโdevice_mapโ็ๆจกๅใ
ๅฆๆๆจๆณ้ๅ่ชๅทฑ็ pytorch ๆจกๅ๏ผ่ฏทๆฅ็ ๐ค Accelerate ็[ๆๆกฃ](https://huggingface.co/docs/accelerate/main/en/usage_guides/quantization)ใ
ไปฅไธๆฏๆจๅฏไปฅไฝฟ็จโbitsandbytesโ้ๆๅฎๆ็ไบๆ
### ้็จ็จๆณ
ๅช่ฆๆจ็ๆจกๅๆฏๆไฝฟ็จ ๐ค Accelerate ่ฟ่กๅ ่ฝฝๅนถๅ
ๅซ `torch.nn.Linear` ๅฑ๏ผๆจๅฏไปฅๅจ่ฐ็จ [`~PreTrainedModel.from_pretrained`] ๆนๆณๆถไฝฟ็จ `load_in_8bit` ๆ `load_in_4bit` ๅๆฐๆฅ้ๅๆจกๅใ่ฟไนๅบ่ฏฅ้็จไบไปปไฝๆจกๆใ
```python
from transformers import AutoModelForCausalLM
model_8bit = AutoModelForCausalLM.from_pretrained("facebook/opt-350m", load_in_8bit=True)
model_4bit = AutoModelForCausalLM.from_pretrained("facebook/opt-350m", load_in_4bit=True)
```
้ป่ฎคๆ
ๅตไธ๏ผๆๆๅ
ถไปๆจกๅ๏ผไพๅฆ `torch.nn.LayerNorm`๏ผๅฐ่ขซ่ฝฌๆขไธบ `torch.float16` ็ฑปๅใไฝๅฆๆๆจๆณๆดๆนๅฎไปฌ็ `dtype`๏ผๅฏไปฅ้่ฝฝ `torch_dtype` ๅๆฐ๏ผ
```python
>>> import torch
>>> from transformers import AutoModelForCausalLM
>>> model_8bit = AutoModelForCausalLM.from_pretrained("facebook/opt-350m", load_in_8bit=True, torch_dtype=torch.float32)
>>> model_8bit.model.decoder.layers[-1].final_layer_norm.weight.dtype
torch.float32
```
### FP4 ้ๅ
#### ่ฆๆฑ
็กฎไฟๅจ่ฟ่กไปฅไธไปฃ็ ๆฎตไนๅๅทฒๅฎๆไปฅไธ่ฆๆฑ๏ผ
- ๆๆฐ็ๆฌ `bitsandbytes` ๅบ
`pip install bitsandbytes>=0.39.0`
- ๅฎ่ฃ
ๆๆฐ็ๆฌ `accelerate`
`pip install --upgrade accelerate`
- ๅฎ่ฃ
ๆๆฐ็ๆฌ `transformers`
`pip install --upgrade transformers`
#### ๆ็คบๅๆไฝณๅฎ่ทต
- **้ซ็บง็จๆณ๏ผ** ่ฏทๅ่ [ๆญค Google Colab notebook](https://colab.research.google.com/drive/1ge2F1QSK8Q7h0hn3YKuBCOAS0bK8E0wf) ไปฅ่ทๅ 4 ไฝ้ๅ้ซ็บง็จๆณๅๆๆๅฏ้้้กนใ
- **ไฝฟ็จ `batch_size=1` ๅฎ็ฐๆดๅฟซ็ๆจ็๏ผ** ่ช `bitsandbytes` ็ `0.40.0` ็ๆฌไปฅๆฅ๏ผ่ฎพ็ฝฎ `batch_size=1`๏ผๆจๅฏไปฅไปๅฟซ้ๆจ็ไธญๅ็ใ่ฏทๆฅ็ [่ฟไบๅๅธ่ฏดๆ](https://github.com/TimDettmers/bitsandbytes/releases/tag/0.40.0) ๏ผๅนถ็กฎไฟไฝฟ็จๅคงไบ `0.40.0` ็็ๆฌไปฅ็ดๆฅๅฉ็จๆญคๅ่ฝใ
- **่ฎญ็ป๏ผ** ๆ นๆฎ [QLoRA ่ฎบๆ](https://arxiv.org/abs/2305.14314)๏ผๅฏนไบ4ไฝๅบๆจกๅ่ฎญ็ป๏ผไฝฟ็จ LoRA ้้
ๅจ๏ผ๏ผๅบไฝฟ็จ `bnb_4bit_quant_type='nf4'`ใ
- **ๆจ็๏ผ** ๅฏนไบๆจ็๏ผ`bnb_4bit_quant_type` ๅฏนๆง่ฝๅฝฑๅไธๅคงใไฝๆฏไธบไบไธๆจกๅ็ๆ้ไฟๆไธ่ด๏ผ่ฏท็กฎไฟไฝฟ็จ็ธๅ็ `bnb_4bit_compute_dtype` ๅ `torch_dtype` ๅๆฐใ
#### ๅ ่ฝฝ 4 ไฝ้ๅ็ๅคงๆจกๅ
ๅจ่ฐ็จ `.from_pretrained` ๆนๆณๆถไฝฟ็จ `load_in_4bit=True`๏ผๅฏไปฅๅฐๆจ็ๅ
ๅญไฝฟ็จ้ๅๅฐๅฐๅคง็บฆๅๆฅ็ 1/4ใ
```python
# pip install transformers accelerate bitsandbytes
from transformers import AutoModelForCausalLM, AutoTokenizer
model_id = "bigscience/bloom-1b7"
tokenizer = AutoTokenizer.from_pretrained(model_id)
model = AutoModelForCausalLM.from_pretrained(model_id, device_map="auto", load_in_4bit=True)
```
<Tip warning={true}>
้่ฆๆณจๆ็ๆฏ๏ผไธๆฆๆจกๅไปฅ 4 ไฝ้ๅๆนๅผๅ ่ฝฝ๏ผๅฐฑๆ ๆณๅฐ้ๅๅ็ๆ้ๆจ้ๅฐ Hub ไธใๆญคๅค๏ผๆจไธ่ฝ่ฎญ็ป 4 ไฝ้ๅๆ้๏ผๅ ไธบ็ฎๅๅฐไธๆฏๆๆญคๅ่ฝใไฝๆฏ๏ผๆจๅฏไปฅไฝฟ็จ 4 ไฝ้ๅๆจกๅๆฅ่ฎญ็ป้ขๅคๅๆฐ๏ผ่ฟๅฐๅจไธไธ้จๅไธญไป็ปใ
</Tip>
### ๅ ่ฝฝ 8 ไฝ้ๅ็ๅคงๆจกๅ
ๆจๅฏไปฅ้่ฟๅจ่ฐ็จ `.from_pretrained` ๆนๆณๆถไฝฟ็จ `load_in_8bit=True` ๅๆฐ๏ผๅฐๅ
ๅญ้ๆฑๅคง่ดๅๅๆฅๅ ่ฝฝๆจกๅ
```python
# pip install transformers accelerate bitsandbytes
from transformers import AutoModelForCausalLM, AutoTokenizer
model_id = "bigscience/bloom-1b7"
tokenizer = AutoTokenizer.from_pretrained(model_id)
model = AutoModelForCausalLM.from_pretrained(model_id, device_map="auto", load_in_8bit=True)
```
็ถๅ๏ผๅ้ๅธธไฝฟ็จ `PreTrainedModel` ไธๆ ทไฝฟ็จๆจ็ๆจกๅใ
ๆจๅฏไปฅไฝฟ็จ `get_memory_footprint` ๆนๆณๆฃๆฅๆจกๅ็ๅ
ๅญๅ ็จใ
```python
print(model.get_memory_footprint())
```
้่ฟ่ฟ็ง้ๆ๏ผๆไปฌ่ฝๅคๅจ่พๅฐ็่ฎพๅคไธๅ ่ฝฝๅคงๆจกๅๅนถ่ฟ่กๅฎไปฌ่ๆฒกๆไปปไฝ้ฎ้ขใ
<Tip warning={true}>
้่ฆๆณจๆ็ๆฏ๏ผไธๆฆๆจกๅไปฅ 8 ไฝ้ๅๆนๅผๅ ่ฝฝ๏ผ้คไบไฝฟ็จๆๆฐ็ `transformers` ๅ `bitsandbytes` ไนๅค๏ผ็ฎๅๅฐๆ ๆณๅฐ้ๅๅ็ๆ้ๆจ้ๅฐ Hub ไธใๆญคๅค๏ผๆจไธ่ฝ่ฎญ็ป 8 ไฝ้ๅๆ้๏ผๅ ไธบ็ฎๅๅฐไธๆฏๆๆญคๅ่ฝใไฝๆฏ๏ผๆจๅฏไปฅไฝฟ็จ 8 ไฝ้ๅๆจกๅๆฅ่ฎญ็ป้ขๅคๅๆฐ๏ผ่ฟๅฐๅจไธไธ้จๅไธญไป็ปใ
ๆณจๆ๏ผ`device_map` ๆฏๅฏ้็๏ผไฝ่ฎพ็ฝฎ `device_map = 'auto'` ๆด้ๅ็จไบๆจ็๏ผๅ ไธบๅฎๅฐๆดๆๆๅฐ่ฐๅบฆๅฏ็จ่ตๆบไธ็ๆจกๅใ
</Tip>
#### ้ซ็บง็จไพ
ๅจ่ฟ้๏ผๆไปฌๅฐไป็ปไฝฟ็จ FP4 ้ๅ็ไธไบ้ซ็บง็จไพใ
##### ๆดๆน่ฎก็ฎๆฐๆฎ็ฑปๅ
่ฎก็ฎๆฐๆฎ็ฑปๅ็จไบๆนๅๅจ่ฟ่ก่ฎก็ฎๆถไฝฟ็จ็ๆฐๆฎ็ฑปๅใไพๅฆ๏ผhidden statesๅฏไปฅๆฏ `float32`๏ผไฝไธบไบๅ ้๏ผ่ฎก็ฎๆถๅฏไปฅ่ขซ่ฎพ็ฝฎไธบ `bf16`ใ้ป่ฎคๆ
ๅตไธ๏ผ่ฎก็ฎๆฐๆฎ็ฑปๅ่ขซ่ฎพ็ฝฎไธบ `float32`ใ
```python
import torch
from transformers import BitsAndBytesConfig
quantization_config = BitsAndBytesConfig(load_in_4bit=True, bnb_4bit_compute_dtype=torch.bfloat16)
```
#### ไฝฟ็จ NF4๏ผๆฎ้ๆตฎ็นๆฐ 4๏ผๆฐๆฎ็ฑปๅ
ๆจ่ฟๅฏไปฅไฝฟ็จ NF4 ๆฐๆฎ็ฑปๅ๏ผ่ฟๆฏไธ็ง้ๅฏนไฝฟ็จๆญฃๆๅๅธๅๅงๅ็ๆ้่้ๅบ็ๆฐๅ 4 ไฝๆฐๆฎ็ฑปๅใ่ฆ่ฟ่ก๏ผ
```python
from transformers import BitsAndBytesConfig
nf4_config = BitsAndBytesConfig(
load_in_4bit=True,
bnb_4bit_quant_type="nf4",
)
model_nf4 = AutoModelForCausalLM.from_pretrained(model_id, quantization_config=nf4_config)
```
#### ไฝฟ็จๅตๅฅ้ๅ่ฟ่กๆด้ซๆ็ๅ
ๅญๆจ็
ๆไปฌ่ฟๅปบ่ฎฎ็จๆทไฝฟ็จๅตๅฅ้ๅๆๆฏใไปๆไปฌ็็ป้ช่งๅฏๆฅ็๏ผ่ฟ็งๆนๆณๅจไธๅขๅ ้ขๅคๆง่ฝ็ๆ
ๅตไธ่็ๆดๅคๅ
ๅญใ่ฟไฝฟๅพ llama-13b ๆจกๅ่ฝๅคๅจๅ
ทๆ 1024 ไธชๅบๅ้ฟๅบฆใ1 ไธชๆนๆฌกๅคงๅฐๅ 4 ไธชๆขฏๅบฆ็ดฏ็งฏๆญฅ้ชค็ NVIDIA-T4 16GB ไธ่ฟ่ก fine-tuningใ
```python
from transformers import BitsAndBytesConfig
double_quant_config = BitsAndBytesConfig(
load_in_4bit=True,
bnb_4bit_use_double_quant=True,
)
model_double_quant = AutoModelForCausalLM.from_pretrained(model_id, quantization_config=double_quant_config)
```
### ๅฐ้ๅๆจกๅๆจ้ๅฐ๐ค Hub
ๆจๅฏไปฅไฝฟ็จ `push_to_hub` ๆนๆณๅฐ้ๅๆจกๅๆจ้ๅฐ Hub ไธใ่ฟๅฐ้ฆๅ
ๆจ้้ๅ้
็ฝฎๆไปถ๏ผ็ถๅๆจ้้ๅๆจกๅๆ้ใ
่ฏท็กฎไฟไฝฟ็จ `bitsandbytes>0.37.2`๏ผๅจๆฐๅๆฌๆๆถ๏ผๆไปฌไฝฟ็จ็ๆฏ `bitsandbytes==0.38.0.post1`๏ผๆ่ฝไฝฟ็จๆญคๅ่ฝใ
```python
from transformers import AutoModelForCausalLM, AutoTokenizer
model = AutoModelForCausalLM.from_pretrained("bigscience/bloom-560m", device_map="auto", load_in_8bit=True)
tokenizer = AutoTokenizer.from_pretrained("bigscience/bloom-560m")
model.push_to_hub("bloom-560m-8bit")
```
<Tip warning={true}>
ๅฏนๅคงๆจกๅ๏ผๅผบ็้ผๅฑๅฐ 8 ไฝ้ๅๆจกๅๆจ้ๅฐ Hub ไธ๏ผไปฅไพฟ่ฎฉ็คพๅบ่ฝๅคไปๅ
ๅญๅ ็จๅๅฐๅๅ ่ฝฝไธญๅ็๏ผไพๅฆๅจ Google Colab ไธๅ ่ฝฝๅคงๆจกๅใ
</Tip>
### ไป๐ค Hubๅ ่ฝฝ้ๅๆจกๅ
ๆจๅฏไปฅไฝฟ็จ `from_pretrained` ๆนๆณไป Hub ๅ ่ฝฝ้ๅๆจกๅใ่ฏท็กฎไฟๆจ้็ๆ้ๆฏ้ๅ็๏ผๆฃๆฅๆจกๅ้
็ฝฎๅฏน่ฑกไธญๆฏๅฆๅญๅจ `quantization_config` ๅฑๆงใ
```python
from transformers import AutoModelForCausalLM, AutoTokenizer
model = AutoModelForCausalLM.from_pretrained("{your_username}/bloom-560m-8bit", device_map="auto")
```
่ฏทๆณจๆ๏ผๅจ่ฟ็งๆ
ๅตไธ๏ผๆจไธ้่ฆๆๅฎ `load_in_8bit=True` ๅๆฐ๏ผไฝ้่ฆ็กฎไฟ `bitsandbytes` ๅ `accelerate` ๅทฒๅฎ่ฃ
ใ
ๆ
ๆณจๆ๏ผ`device_map` ๆฏๅฏ้็๏ผไฝ่ฎพ็ฝฎ `device_map = 'auto'` ๆด้ๅ็จไบๆจ็๏ผๅ ไธบๅฎๅฐๆดๆๆๅฐ่ฐๅบฆๅฏ็จ่ตๆบไธ็ๆจกๅใ
### ้ซ็บง็จไพ
ๆฌ่้ขๅๅธๆๆข็ดข้คไบๅ ่ฝฝๅ่ฟ่ก 8 ไฝๆจกๅไนๅค่ฟ่ฝๅไปไน็่ฟ้ถ็จๆทใ
#### ๅจ `cpu` ๅ `gpu` ไน้ดๅธ่ฝฝ
ๆญค้ซ็บง็จไพไนไธๆฏ่ฝๅคๅ ่ฝฝๆจกๅๅนถๅฐๆ้ๅๆดพๅฐ `CPU` ๅ `GPU` ไน้ดใ่ฏทๆณจๆ๏ผๅฐๅจ CPU ไธๅๆดพ็ๆ้ **ไธไผ** ่ฝฌๆขไธบ 8 ไฝ๏ผๅ ๆญคไผไฟ็ไธบ `float32`ใๆญคๅ่ฝ้็จไบๆณ่ฆ้ๅบ้ๅธธๅคง็ๆจกๅๅนถๅฐๆจกๅๅๆดพๅฐ GPU ๅ CPU ไน้ด็็จๆทใ
้ฆๅ
๏ผไป `transformers` ไธญๅ ่ฝฝไธไธช [`BitsAndBytesConfig`]๏ผๅนถๅฐๅฑๆง `llm_int8_enable_fp32_cpu_offload` ่ฎพ็ฝฎไธบ `True`๏ผ
```python
from transformers import AutoModelForCausalLM, AutoTokenizer, BitsAndBytesConfig
quantization_config = BitsAndBytesConfig(llm_int8_enable_fp32_cpu_offload=True)
```
ๅ่ฎพๆจๆณๅ ่ฝฝ `bigscience/bloom-1b7` ๆจกๅ๏ผๆจ็ GPUๆพๅญไป
่ถณๅคๅฎน็บณ้คไบ`lm_head`ๅค็ๆดไธชๆจกๅใๅ ๆญค๏ผๆจๅฏไปฅๆ็
งไปฅไธๆนๅผ็ผๅ่ชๅฎไน็ device_map๏ผ
```python
device_map = {
"transformer.word_embeddings": 0,
"transformer.word_embeddings_layernorm": 0,
"lm_head": "cpu",
"transformer.h": 0,
"transformer.ln_f": 0,
}
```
็ถๅๅฆไธๅ ่ฝฝๆจกๅ๏ผ
```python
model_8bit = AutoModelForCausalLM.from_pretrained(
"bigscience/bloom-1b7",
device_map=device_map,
quantization_config=quantization_config,
)
```
่ฟๅฐฑๆฏๅ
จ้จๅ
ๅฎน๏ผไบซๅๆจ็ๆจกๅๅง๏ผ
#### ไฝฟ็จ`llm_int8_threshold`
ๆจๅฏไปฅไฝฟ็จ `llm_int8_threshold` ๅๆฐๆฅๆดๆนๅผๅธธๅผ็้ๅผใโๅผๅธธๅผโๆฏไธไธชๅคงไบ็นๅฎ้ๅผ็`hidden state`ๅผใ
่ฟๅฏนๅบไบ`LLM.int8()`่ฎบๆไธญๆ่ฟฐ็ๅผๅธธๆฃๆต็ๅผๅธธ้ๅผใไปปไฝ้ซไบๆญค้ๅผ็`hidden state`ๅผ้ฝๅฐ่ขซ่งไธบๅผๅธธๅผ๏ผๅฏน่ฟไบๅผ็ๆไฝๅฐๅจ fp16 ไธญๅฎๆใๅผ้ๅธธๆฏๆญฃๆๅๅธ็๏ผไนๅฐฑๆฏ่ฏด๏ผๅคงๅคๆฐๅผๅจ [-3.5, 3.5] ่ๅดๅ
๏ผไฝๆไธไบ้ขๅค็็ณป็ปๅผๅธธๅผ๏ผๅฏนไบๅคงๆจกๅๆฅ่ฏด๏ผๅฎไปฌ็ๅๅธ้ๅธธไธๅใ่ฟไบๅผๅธธๅผ้ๅธธๅจๅบ้ด [-60, -6] ๆ [6, 60] ๅ
ใInt8 ้ๅๅฏนไบๅน
ๅบฆไธบ ~5 ็ๅผๆๆๅพๅฅฝ๏ผไฝ่ถ
ๅบ่ฟไธช่ๅด๏ผๆง่ฝๅฐฑไผๆๆพไธ้ใไธไธชๅฅฝ็้ป่ฎค้ๅผๆฏ 6๏ผไฝๅฏนไบๆดไธ็จณๅฎ็ๆจกๅ๏ผๅฐๆจกๅใๅพฎ่ฐ๏ผๅฏ่ฝ้่ฆๆดไฝ็้ๅผใ
่ฟไธชๅๆฐไผๅฝฑๅๆจกๅ็ๆจ็้ๅบฆใๆไปฌๅปบ่ฎฎๅฐ่ฏ่ฟไธชๅๆฐ๏ผไปฅๆพๅฐๆ้ๅๆจ็็จไพ็ๅๆฐใ
```python
from transformers import AutoModelForCausalLM, AutoTokenizer, BitsAndBytesConfig
model_id = "bigscience/bloom-1b7"
quantization_config = BitsAndBytesConfig(
llm_int8_threshold=10,
)
model_8bit = AutoModelForCausalLM.from_pretrained(
model_id,
device_map=device_map,
quantization_config=quantization_config,
)
tokenizer = AutoTokenizer.from_pretrained(model_id)
```
#### ่ทณ่ฟๆไบๆจกๅ็่ฝฌๆข
ไธไบๆจกๅๆๅ ไธช้่ฆไฟๆๆช่ฝฌๆข็ถๆไปฅ็กฎไฟ็จณๅฎๆง็ๆจกๅใไพๅฆ๏ผJukebox ๆจกๅๆๅ ไธช `lm_head` ๆจกๅ้่ฆ่ทณ่ฟใไฝฟ็จ `llm_int8_skip_modules` ๅๆฐ่ฟ่ก็ธๅบๆไฝใ
```python
from transformers import AutoModelForCausalLM, AutoTokenizer, BitsAndBytesConfig
model_id = "bigscience/bloom-1b7"
quantization_config = BitsAndBytesConfig(
llm_int8_skip_modules=["lm_head"],
)
model_8bit = AutoModelForCausalLM.from_pretrained(
model_id,
device_map=device_map,
quantization_config=quantization_config,
)
tokenizer = AutoTokenizer.from_pretrained(model_id)
```
#### ๅพฎ่ฐๅทฒๅ ่ฝฝไธบ8ไฝ็ฒพๅบฆ็ๆจกๅ
ๅๅฉHugging Face็ๆ็ณป็ปไธญ้้
ๅจ๏ผadapters๏ผ็ๅฎๆนๆฏๆ๏ผๆจๅฏไปฅๅจ8ไฝ็ฒพๅบฆไธๅพฎ่ฐๆจกๅใ่ฟไฝฟๅพๅฏไปฅๅจๅไธชGoogle Colabไธญๅพฎ่ฐๅคงๆจกๅ๏ผไพๅฆ`flan-t5-large`ๆ`facebook/opt-6.7b`ใ่ฏทๆฅ็[`peft`](https://github.com/huggingface/peft)ๅบไบ่งฃๆดๅค่ฏฆๆ
ใ
ๆณจๆ๏ผๅ ่ฝฝๆจกๅ่ฟ่ก่ฎญ็ปๆถๆ ้ไผ ้`device_map`ใๅฎๅฐ่ชๅจๅฐๆจ็ๆจกๅๅ ่ฝฝๅฐGPUไธใๅฆๆ้่ฆ๏ผๆจๅฏไปฅๅฐ่ฎพๅคๆ ๅฐไธบ็นๅฎ่ฎพๅค๏ผไพๅฆ`cuda:0`ใ`0`ใ`torch.device('cuda:0')`๏ผใ่ฏทๆณจๆ๏ผ`device_map=auto`ไป
ๅบ็จไบๆจ็ใ
### BitsAndBytesConfig
[[autodoc]] BitsAndBytesConfig
## ไฝฟ็จ ๐ค `optimum` ่ฟ่ก้ๅ
่ฏทๆฅ็[Optimum ๆๆกฃ](https://huggingface.co/docs/optimum/index)ไปฅไบ่งฃๆดๅคๅ
ณไบ`optimum`ๆฏๆ็้ๅๆนๆณ๏ผๅนถๆฅ็่ฟไบๆนๆณๆฏๅฆ้็จไบๆจ็็จไพใ
| transformers/docs/source/zh/main_classes/quantization.md/0 | {
"file_path": "transformers/docs/source/zh/main_classes/quantization.md",
"repo_id": "transformers",
"token_count": 14178
} | 276 |
<!--Copyright 2023 The HuggingFace Team. All rights reserved.
Licensed under the Apache License, Version 2.0 (the "License"); you may not use this file except in compliance with
the License. You may obtain a copy of the License at
http://www.apache.org/licenses/LICENSE-2.0
Unless required by applicable law or agreed to in writing, software distributed under the License is distributed on
an "AS IS" BASIS, WITHOUT WARRANTIES OR CONDITIONS OF ANY KIND, either express or implied. See the License for the
specific language governing permissions and limitations under the License.
โ ๏ธ Note that this file is in Markdown but contain specific syntax for our doc-builder (similar to MDX) that may not be
rendered properly in your Markdown viewer.
-->
# ็จไบ TensorFlow ๆจกๅ็ XLA ้ๆ
[[open-in-colab]]
ๅ ้็บฟๆงไปฃๆฐ๏ผไน็งฐไธบXLA๏ผๆฏไธไธช็จไบๅ ้TensorFlowๆจกๅ่ฟ่กๆถ้ด็็ผ่ฏๅจใไป[ๅฎๆนๆๆกฃ](https://www.tensorflow.org/xla)ไธญๅฏไปฅ็ๅฐ๏ผ
XLA๏ผๅ ้็บฟๆงไปฃๆฐ๏ผๆฏไธ็ง้ๅฏน็บฟๆงไปฃๆฐ็็นๅฎ้ขๅ็ผ่ฏๅจ๏ผๅฏไปฅๅจๅฏ่ฝไธ้่ฆๆดๆนๆบไปฃ็ ็ๆ
ๅตไธๅ ้TensorFlowๆจกๅใ
ๅจTensorFlowไธญไฝฟ็จXLA้ๅธธ็ฎๅโโๅฎๅ
ๅซๅจ`tensorflow`ๅบไธญ๏ผๅนถไธๅฏไปฅไฝฟ็จไปปไฝๅพๅๅปบๅฝๆฐไธญ็`jit_compile`ๅๆฐๆฅ่งฆๅ๏ผไพๅฆ[`tf.function`](https://www.tensorflow.org/guide/intro_to_graphs)ใๅจไฝฟ็จKerasๆนๆณๅฆ`fit()`ๅ`predict()`ๆถ๏ผๅช้ๅฐ`jit_compile`ๅๆฐไผ ้็ป`model.compile()`ๅณๅฏๅฏ็จXLAใ็ถ่๏ผXLAไธไป
้ไบ่ฟไบๆนๆณ - ๅฎ่ฟๅฏไปฅ็จไบๅ ้ไปปไฝไปปๆ็`tf.function`ใ
ๅจ๐ค Transformersไธญ๏ผๅ ไธชTensorFlowๆนๆณๅทฒ็ป่ขซ้ๅไธบไธXLAๅ
ผๅฎน๏ผๅ
ๆฌ[GPT2](https://huggingface.co/docs/transformers/model_doc/gpt2)ใ[T5](https://huggingface.co/docs/transformers/model_doc/t5)ๅ[OPT](https://huggingface.co/docs/transformers/model_doc/opt)็ญๆๆฌ็ๆๆจกๅ๏ผไปฅๅ[Whisper](https://huggingface.co/docs/transformers/model_doc/whisper)็ญ่ฏญ้ณๅค็ๆจกๅใ
่ฝ็ถ็กฎๅ็ๅ ้ๅๆฐๅพๅคง็จๅบฆไธๅๅณไบๆจกๅ๏ผไฝๅฏนไบ๐ค Transformersไธญ็TensorFlowๆๆฌ็ๆๆจกๅ๏ผๆไปฌๆณจๆๅฐ้ๅบฆๆ้ซไบ็บฆ100ๅใๆฌๆๆกฃๅฐ่งฃ้ๅฆไฝๅจ่ฟไบๆจกๅไธไฝฟ็จXLA่ทๅพๆๅคง็ๆง่ฝใๅฆๆๆจๆๅ
ด่ถฃไบ่งฃๆดๅคๅ
ณไบๅบๅๆต่ฏๅๆไปฌๅจXLA้ๆ่ๅ็่ฎพ่ฎกๅฒๅญฆ็ไฟกๆฏ๏ผๆไปฌ่ฟๅฐๆไพ้ขๅค็่ตๆบ้พๆฅใ
## ไฝฟ็จ XLA ่ฟ่ก TensorFlow ๅฝๆฐ
่ฎฉๆไปฌ่่ไปฅไธTensorFlow ไธญ็ๆจกๅ๏ผ
```py
import tensorflow as tf
model = tf.keras.Sequential(
[tf.keras.layers.Dense(10, input_shape=(10,), activation="relu"), tf.keras.layers.Dense(5, activation="softmax")]
)
```
ไธ่ฟฐๆจกๅๆฅๅ็ปดๅบฆไธบ `(10,)` ็่พๅ
ฅใๆไปฌๅฏไปฅๅไธ้ข่ฟๆ ทไฝฟ็จๆจกๅ่ฟ่กๅๅไผ ๆญ๏ผ
```py
# Generate random inputs for the model.
batch_size = 16
input_vector_dim = 10
random_inputs = tf.random.normal((batch_size, input_vector_dim))
# Run a forward pass.
_ = model(random_inputs)
```
ไธบไบไฝฟ็จ XLA ็ผ่ฏ็ๅฝๆฐ่ฟ่กๅๅไผ ๆญ๏ผๆไปฌ้่ฆๆง่กไปฅไธๆไฝ๏ผ
```py
xla_fn = tf.function(model, jit_compile=True)
_ = xla_fn(random_inputs)
```
`model`็้ป่ฎค`call()`ๅฝๆฐ็จไบ็ผ่ฏXLAๅพใไฝๅฆๆไฝ ๆณๅฐๅ
ถไปๆจกๅๅฝๆฐ็ผ่ฏๆXLA๏ผไนๆฏๅฏไปฅ็๏ผๅฆไธๆ็คบ๏ผ
```py
my_xla_fn = tf.function(model.my_xla_fn, jit_compile=True)
```
## ๅจ๐ค Transformersๅบไธญไฝฟ็จXLA่ฟ่กTensorFlowๆๆฌ็ๆๆจกๅ
่ฆๅจ๐ค Transformersไธญๅฏ็จXLAๅ ้็ๆ๏ผๆจ้่ฆๅฎ่ฃ
ๆๆฐ็ๆฌ็`transformers`ใๆจๅฏไปฅ้่ฟ่ฟ่กไปฅไธๅฝไปคๆฅๅฎ่ฃ
ๅฎ๏ผ
```bash
pip install transformers --upgrade
```
็ถๅๆจๅฏไปฅ่ฟ่กไปฅไธไปฃ็ ๏ผ
```py
import tensorflow as tf
from transformers import AutoTokenizer, TFAutoModelForCausalLM
# Will error if the minimal version of Transformers is not installed.
from transformers.utils import check_min_version
check_min_version("4.21.0")
tokenizer = AutoTokenizer.from_pretrained("gpt2", padding_side="left", pad_token="</s>")
model = TFAutoModelForCausalLM.from_pretrained("gpt2")
input_string = ["TensorFlow is"]
# One line to create an XLA generation function
xla_generate = tf.function(model.generate, jit_compile=True)
tokenized_input = tokenizer(input_string, return_tensors="tf")
generated_tokens = xla_generate(**tokenized_input, num_beams=2)
decoded_text = tokenizer.decode(generated_tokens[0], skip_special_tokens=True)
print(f"Generated -- {decoded_text}")
# Generated -- TensorFlow is an open-source, open-source, distributed-source application # framework for the
```
ๆญฃๅฆๆจๆๆณจๆๅฐ็๏ผๅจ`generate()`ไธๅฏ็จXLAๅช้่ฆไธ่กไปฃ็ ใๅ
ถไฝ้จๅไปฃ็ ไฟๆไธๅใ็ถ่๏ผไธ้ข็ไปฃ็ ็ๆฎตไธญๆไธไบไธXLA็ธๅ
ณ็ๆณจๆไบ้กนใๆจ้่ฆไบ่งฃ่ฟไบๆณจๆไบ้กน๏ผไปฅๅ
ๅๅฉ็จXLAๅฏ่ฝๅธฆๆฅ็ๆง่ฝๆๅใๆไปฌๅฐๅจไธ้ข็้จๅ่ฎจ่ฎบ่ฟไบๅ
ๅฎนใ
## ้่ฆๅ
ณๆณจ็ๆณจๆไบ้กน
ๅฝๆจ้ฆๆฌกๆง่กๅฏ็จXLA็ๅฝๆฐ๏ผๅฆไธ้ข็`xla_generate()`๏ผๆถ๏ผๅฎๅฐๅจๅ
้จๅฐ่ฏๆจๆญ่ฎก็ฎๅพ๏ผ่ฟๆฏไธไธช่ๆถ็่ฟ็จใ่ฟไธช่ฟ็จ่ขซ็งฐไธบ[โtracingโ](https://www.tensorflow.org/guide/intro_to_graphs#when_is_a_function_tracing)ใ
ๆจๅฏ่ฝไผๆณจๆๅฐ็ๆๆถ้ดๅนถไธๅฟซใ่ฟ็ปญ่ฐ็จ`xla_generate()`๏ผๆไปปไฝๅ
ถไปๅฏ็จไบXLA็ๅฝๆฐ๏ผไธ้่ฆๅๆฌกๆจๆญ่ฎก็ฎๅพ๏ผๅช่ฆๅฝๆฐ็่พๅ
ฅไธๆๅๆๅปบ่ฎก็ฎๅพๆถ็ๅฝข็ถ็ธๅน้
ใๅฏนไบๅ
ทๆๅบๅฎ่พๅ
ฅๅฝข็ถ็ๆจกๆ๏ผไพๅฆๅพๅ๏ผ๏ผ่ฟไธๆฏ้ฎ้ข๏ผไฝๅฆๆๆจๆญฃๅจๅค็ๅ
ทๆๅฏๅ่พๅ
ฅๅฝข็ถ็ๆจกๆ๏ผไพๅฆๆๆฌ๏ผ๏ผๅๅฟ
้กปๆณจๆใ
ไธบไบ็กฎไฟ`xla_generate()`ๅง็ปไฝฟ็จ็ธๅ็่พๅ
ฅๅฝข็ถ๏ผๆจๅฏไปฅๅจ่ฐ็จ`tokenizer`ๆถๆๅฎ`padding`ๅๆฐใ
```py
import tensorflow as tf
from transformers import AutoTokenizer, TFAutoModelForCausalLM
tokenizer = AutoTokenizer.from_pretrained("gpt2", padding_side="left", pad_token="</s>")
model = TFAutoModelForCausalLM.from_pretrained("gpt2")
input_string = ["TensorFlow is"]
xla_generate = tf.function(model.generate, jit_compile=True)
# Here, we call the tokenizer with padding options.
tokenized_input = tokenizer(input_string, pad_to_multiple_of=8, padding=True, return_tensors="tf")
generated_tokens = xla_generate(**tokenized_input, num_beams=2)
decoded_text = tokenizer.decode(generated_tokens[0], skip_special_tokens=True)
print(f"Generated -- {decoded_text}")
```
้่ฟ่ฟ็งๆนๅผ๏ผๆจๅฏไปฅ็กฎไฟ`xla_generate()`็่พๅ
ฅๅง็ปๅ
ทๆๅฎ่ท่ธช็ๅฝข็ถ๏ผไป่ๅ ้็ๆๆถ้ดใๆจๅฏไปฅไฝฟ็จไปฅไธไปฃ็ ๆฅ้ช่ฏ่ฟไธ็น๏ผ
```py
import time
import tensorflow as tf
from transformers import AutoTokenizer, TFAutoModelForCausalLM
tokenizer = AutoTokenizer.from_pretrained("gpt2", padding_side="left", pad_token="</s>")
model = TFAutoModelForCausalLM.from_pretrained("gpt2")
xla_generate = tf.function(model.generate, jit_compile=True)
for input_string in ["TensorFlow is", "TensorFlow is a", "TFLite is a"]:
tokenized_input = tokenizer(input_string, pad_to_multiple_of=8, padding=True, return_tensors="tf")
start = time.time_ns()
generated_tokens = xla_generate(**tokenized_input, num_beams=2)
end = time.time_ns()
print(f"Execution time -- {(end - start) / 1e6:.1f} ms\n")
```
ๅจTesla T4 GPUไธ๏ผๆจๅฏไปฅๆๆๅฆไธ็่พๅบ๏ผ
```bash
Execution time -- 30819.6 ms
Execution time -- 79.0 ms
Execution time -- 78.9 ms
```
็ฌฌไธๆฌก่ฐ็จ`xla_generate()`ไผๅ ไธบ`tracing`่่ๆถ๏ผไฝๅ็ปญ็่ฐ็จไผๅฟซๅพๅคใ่ฏทๆณจๆ๏ผไปปไฝๆถๅๅฏน็ๆ้้กน็ๆดๆน้ฝไผ่งฆๅ้ๆฐ`tracing`๏ผไป่ๅฏผ่ด็ๆๆถ้ดๅๆ
ขใ
ๅจๆฌๆๆกฃไธญ๏ผๆไปฌๆฒกๆๆถต็๐ค Transformersๆไพ็ๆๆๆๆฌ็ๆ้้กนใๆไปฌ้ผๅฑๆจ้
่ฏปๆๆกฃไปฅไบ่งฃ้ซ็บง็จไพใ
## ้ๅ ่ตๆบ
ไปฅไธๆฏไธไบ้ๅ ่ตๆบ๏ผๅฆๆๆจๆณๆทฑๅ
ฅไบ่งฃๅจ๐ค Transformersๅๅ
ถไปๅบไธไฝฟ็จXLA๏ผ
* [่ฟไธชColab Notebook](https://colab.research.google.com/github/huggingface/blog/blob/main/notebooks/91_tf_xla_generate.ipynb) ๆไพไบไธไธชไบๅจๆผ็คบ๏ผ่ฎฉๆจๅฏไปฅๅฐ่ฏไฝฟ็จXLAๅ
ผๅฎน็็ผ็ ๅจ-่งฃ็ ๅจ๏ผไพๅฆ[T5](https://huggingface.co/docs/transformers/model_doc/t5)๏ผๅไป
่งฃ็ ๅจ๏ผไพๅฆ[GPT2](https://huggingface.co/docs/transformers/model_doc/gpt2)๏ผๆๆฌ็ๆๆจกๅใ
* [่ฟ็ฏๅๅฎขๆ็ซ ](https://huggingface.co/blog/tf-xla-generate) ๆไพไบXLAๅ
ผๅฎนๆจกๅ็ๆฏ่พๅบๅๆฆ่ฟฐ๏ผไปฅๅๅ
ณไบๅจTensorFlowไธญไฝฟ็จXLA็ๅๅฅฝไป็ปใ
* [่ฟ็ฏๅๅฎขๆ็ซ ](https://blog.tensorflow.org/2022/11/how-hugging-face-improved-text-generation-performance-with-xla.html) ่ฎจ่ฎบไบๆไปฌๅจ๐ค TransformersไธญไธบTensorFlowๆจกๅๆทปๅ XLAๆฏๆ็่ฎพ่ฎก็ๅฟตใ
* ๆจ่็จไบๆดๅคๅญฆไน XLAๅTensorFlowๅพ็่ตๆบ๏ผ
* [XLA๏ผ้ขๅๆบๅจๅญฆไน ็ไผๅ็ผ่ฏๅจ](https://www.tensorflow.org/xla)
* [ๅพๅtf.function็ฎไป](https://www.tensorflow.org/guide/intro_to_graphs)
* [ไฝฟ็จtf.function่ทๅพๆดๅฅฝ็ๆง่ฝ](https://www.tensorflow.org/guide/function) | transformers/docs/source/zh/tf_xla.md/0 | {
"file_path": "transformers/docs/source/zh/tf_xla.md",
"repo_id": "transformers",
"token_count": 4519
} | 277 |
#!/usr/bin/env python
# coding=utf-8
# Copyright 2021 The HuggingFace Team All rights reserved.
#
# Licensed under the Apache License, Version 2.0 (the "License");
# you may not use this file except in compliance with the License.
# You may obtain a copy of the License at
#
# http://www.apache.org/licenses/LICENSE-2.0
#
# Unless required by applicable law or agreed to in writing, software
# distributed under the License is distributed on an "AS IS" BASIS,
# WITHOUT WARRANTIES OR CONDITIONS OF ANY KIND, either express or implied.
# See the License for the specific language governing permissions and
# limitations under the License.
"""
Fine-tuning the library models for masked language modeling (BERT, ALBERT, RoBERTa...) with whole word masking on a
text file or a dataset.
Here is the full list of checkpoints on the hub that can be fine-tuned by this script:
https://huggingface.co/models?filter=fill-mask
"""
import json
import logging
import math
import os
import sys
import time
import warnings
from dataclasses import asdict, dataclass, field
from enum import Enum
from itertools import chain
# You can also adapt this script on your own masked language modeling task. Pointers for this are left as comments.
from pathlib import Path
from typing import Dict, List, Optional, Tuple
import flax
import jax
import jax.numpy as jnp
import numpy as np
import optax
from datasets import load_dataset
from flax import jax_utils, traverse_util
from flax.jax_utils import pad_shard_unpad
from flax.training import train_state
from flax.training.common_utils import get_metrics, onehot, shard
from huggingface_hub import Repository, create_repo
from tqdm import tqdm
from transformers import (
CONFIG_MAPPING,
FLAX_MODEL_FOR_MASKED_LM_MAPPING,
AutoConfig,
AutoTokenizer,
FlaxAutoModelForMaskedLM,
HfArgumentParser,
PreTrainedTokenizerBase,
TensorType,
is_tensorboard_available,
set_seed,
)
from transformers.utils import send_example_telemetry
MODEL_CONFIG_CLASSES = list(FLAX_MODEL_FOR_MASKED_LM_MAPPING.keys())
MODEL_TYPES = tuple(conf.model_type for conf in MODEL_CONFIG_CLASSES)
@dataclass
class TrainingArguments:
output_dir: str = field(
metadata={"help": "The output directory where the model predictions and checkpoints will be written."},
)
overwrite_output_dir: bool = field(
default=False,
metadata={
"help": (
"Overwrite the content of the output directory. "
"Use this to continue training if output_dir points to a checkpoint directory."
)
},
)
do_train: bool = field(default=False, metadata={"help": "Whether to run training."})
do_eval: bool = field(default=False, metadata={"help": "Whether to run eval on the dev set."})
per_device_train_batch_size: int = field(
default=8, metadata={"help": "Batch size per GPU/TPU core/CPU for training."}
)
per_device_eval_batch_size: int = field(
default=8, metadata={"help": "Batch size per GPU/TPU core/CPU for evaluation."}
)
learning_rate: float = field(default=5e-5, metadata={"help": "The initial learning rate for AdamW."})
weight_decay: float = field(default=0.0, metadata={"help": "Weight decay for AdamW if we apply some."})
adam_beta1: float = field(default=0.9, metadata={"help": "Beta1 for AdamW optimizer"})
adam_beta2: float = field(default=0.999, metadata={"help": "Beta2 for AdamW optimizer"})
adam_epsilon: float = field(default=1e-8, metadata={"help": "Epsilon for AdamW optimizer."})
adafactor: bool = field(default=False, metadata={"help": "Whether or not to replace AdamW by Adafactor."})
num_train_epochs: float = field(default=3.0, metadata={"help": "Total number of training epochs to perform."})
warmup_steps: int = field(default=0, metadata={"help": "Linear warmup over warmup_steps."})
logging_steps: int = field(default=500, metadata={"help": "Log every X updates steps."})
save_steps: int = field(default=500, metadata={"help": "Save checkpoint every X updates steps."})
eval_steps: int = field(default=None, metadata={"help": "Run an evaluation every X steps."})
seed: int = field(default=42, metadata={"help": "Random seed that will be set at the beginning of training."})
push_to_hub: bool = field(
default=False, metadata={"help": "Whether or not to upload the trained model to the model hub after training."}
)
hub_model_id: str = field(
default=None, metadata={"help": "The name of the repository to keep in sync with the local `output_dir`."}
)
hub_token: str = field(default=None, metadata={"help": "The token to use to push to the Model Hub."})
gradient_checkpointing: bool = field(
default=False,
metadata={
"help": "If True, use gradient checkpointing to save memory at the expense of slower backward pass."
},
)
def __post_init__(self):
if self.output_dir is not None:
self.output_dir = os.path.expanduser(self.output_dir)
def to_dict(self):
"""
Serializes this instance while replace `Enum` by their values (for JSON serialization support). It obfuscates
the token values by removing their value.
"""
d = asdict(self)
for k, v in d.items():
if isinstance(v, Enum):
d[k] = v.value
if isinstance(v, list) and len(v) > 0 and isinstance(v[0], Enum):
d[k] = [x.value for x in v]
if k.endswith("_token"):
d[k] = f"<{k.upper()}>"
return d
@dataclass
class ModelArguments:
"""
Arguments pertaining to which model/config/tokenizer we are going to fine-tune, or train from scratch.
"""
model_name_or_path: Optional[str] = field(
default=None,
metadata={
"help": (
"The model checkpoint for weights initialization. Don't set if you want to train a model from scratch."
)
},
)
model_type: Optional[str] = field(
default=None,
metadata={"help": "If training from scratch, pass a model type from the list: " + ", ".join(MODEL_TYPES)},
)
config_name: Optional[str] = field(
default=None, metadata={"help": "Pretrained config name or path if not the same as model_name"}
)
tokenizer_name: Optional[str] = field(
default=None, metadata={"help": "Pretrained tokenizer name or path if not the same as model_name"}
)
cache_dir: Optional[str] = field(
default=None, metadata={"help": "Where do you want to store the pretrained models downloaded from s3"}
)
use_fast_tokenizer: bool = field(
default=True,
metadata={"help": "Whether to use one of the fast tokenizer (backed by the tokenizers library) or not."},
)
dtype: Optional[str] = field(
default="float32",
metadata={
"help": (
"Floating-point format in which the model weights should be initialized and trained. Choose one of"
" `[float32, float16, bfloat16]`."
)
},
)
token: str = field(
default=None,
metadata={
"help": (
"The token to use as HTTP bearer authorization for remote files. If not specified, will use the token "
"generated when running `huggingface-cli login` (stored in `~/.huggingface`)."
)
},
)
use_auth_token: bool = field(
default=None,
metadata={
"help": "The `use_auth_token` argument is deprecated and will be removed in v4.34. Please use `token` instead."
},
)
trust_remote_code: bool = field(
default=False,
metadata={
"help": (
"Whether or not to allow for custom models defined on the Hub in their own modeling files. This option "
"should only be set to `True` for repositories you trust and in which you have read the code, as it will "
"execute code present on the Hub on your local machine."
)
},
)
@dataclass
class DataTrainingArguments:
"""
Arguments pertaining to what data we are going to input our model for training and eval.
"""
dataset_name: Optional[str] = field(
default=None, metadata={"help": "The name of the dataset to use (via the datasets library)."}
)
dataset_config_name: Optional[str] = field(
default=None, metadata={"help": "The configuration name of the dataset to use (via the datasets library)."}
)
train_file: Optional[str] = field(default=None, metadata={"help": "The input training data file (a text file)."})
validation_file: Optional[str] = field(
default=None,
metadata={"help": "An optional input evaluation data file to evaluate the perplexity on (a text file)."},
)
train_ref_file: Optional[str] = field(
default=None,
metadata={"help": "An optional input train ref data file for whole word masking in Chinese."},
)
validation_ref_file: Optional[str] = field(
default=None,
metadata={"help": "An optional input validation ref data file for whole word masking in Chinese."},
)
overwrite_cache: bool = field(
default=False, metadata={"help": "Overwrite the cached training and evaluation sets"}
)
validation_split_percentage: Optional[int] = field(
default=5,
metadata={
"help": "The percentage of the train set used as validation set in case there's no validation split"
},
)
max_seq_length: Optional[int] = field(
default=None,
metadata={
"help": (
"The maximum total input sequence length after tokenization. Sequences longer "
"than this will be truncated. Default to the max input length of the model."
)
},
)
preprocessing_num_workers: Optional[int] = field(
default=None,
metadata={"help": "The number of processes to use for the preprocessing."},
)
mlm_probability: float = field(
default=0.15, metadata={"help": "Ratio of tokens to mask for masked language modeling loss"}
)
pad_to_max_length: bool = field(
default=False,
metadata={
"help": (
"Whether to pad all samples to `max_seq_length`. "
"If False, will pad the samples dynamically when batching to the maximum length in the batch."
)
},
)
line_by_line: bool = field(
default=False,
metadata={"help": "Whether distinct lines of text in the dataset are to be handled as distinct sequences."},
)
def __post_init__(self):
if self.dataset_name is None and self.train_file is None and self.validation_file is None:
raise ValueError("Need either a dataset name or a training/validation file.")
else:
if self.train_file is not None:
extension = self.train_file.split(".")[-1]
assert extension in ["csv", "json", "txt"], "`train_file` should be a csv, a json or a txt file."
if self.validation_file is not None:
extension = self.validation_file.split(".")[-1]
assert extension in ["csv", "json", "txt"], "`validation_file` should be a csv, a json or a txt file."
@flax.struct.dataclass
class FlaxDataCollatorForLanguageModeling:
"""
Data collator used for language modeling. Inputs are dynamically padded to the maximum length of a batch if they
are not all of the same length.
Args:
tokenizer (:class:`~transformers.PreTrainedTokenizer` or :class:`~transformers.PreTrainedTokenizerFast`):
The tokenizer used for encoding the data.
mlm_probability (:obj:`float`, `optional`, defaults to 0.15):
The probability with which to (randomly) mask tokens in the input.
.. note::
For best performance, this data collator should be used with a dataset having items that are dictionaries or
BatchEncoding, with the :obj:`"special_tokens_mask"` key, as returned by a
:class:`~transformers.PreTrainedTokenizer` or a :class:`~transformers.PreTrainedTokenizerFast` with the
argument :obj:`return_special_tokens_mask=True`.
"""
tokenizer: PreTrainedTokenizerBase
mlm_probability: float = 0.15
def __post_init__(self):
if self.tokenizer.mask_token is None:
raise ValueError(
"This tokenizer does not have a mask token which is necessary for masked language modeling. "
"You should pass `mlm=False` to train on causal language modeling instead."
)
def __call__(self, examples: List[Dict[str, np.ndarray]], pad_to_multiple_of: int) -> Dict[str, np.ndarray]:
# Handle dict or lists with proper padding and conversion to tensor.
batch = self.tokenizer.pad(examples, pad_to_multiple_of=pad_to_multiple_of, return_tensors=TensorType.NUMPY)
# If special token mask has been preprocessed, pop it from the dict.
special_tokens_mask = batch.pop("special_tokens_mask", None)
batch["input_ids"], batch["labels"] = self.mask_tokens(
batch["input_ids"], special_tokens_mask=special_tokens_mask
)
return batch
def mask_tokens(
self, inputs: np.ndarray, special_tokens_mask: Optional[np.ndarray]
) -> Tuple[np.ndarray, np.ndarray]:
"""
Prepare masked tokens inputs/labels for masked language modeling: 80% MASK, 10% random, 10% original.
"""
labels = inputs.copy()
# We sample a few tokens in each sequence for MLM training (with probability `self.mlm_probability`)
probability_matrix = np.full(labels.shape, self.mlm_probability)
special_tokens_mask = special_tokens_mask.astype("bool")
probability_matrix[special_tokens_mask] = 0.0
masked_indices = np.random.binomial(1, probability_matrix).astype("bool")
labels[~masked_indices] = -100 # We only compute loss on masked tokens
# 80% of the time, we replace masked input tokens with tokenizer.mask_token ([MASK])
indices_replaced = np.random.binomial(1, np.full(labels.shape, 0.8)).astype("bool") & masked_indices
inputs[indices_replaced] = self.tokenizer.convert_tokens_to_ids(self.tokenizer.mask_token)
# 10% of the time, we replace masked input tokens with random word
indices_random = np.random.binomial(1, np.full(labels.shape, 0.5)).astype("bool")
indices_random &= masked_indices & ~indices_replaced
random_words = np.random.randint(self.tokenizer.vocab_size, size=labels.shape, dtype="i4")
inputs[indices_random] = random_words[indices_random]
# The rest of the time (10% of the time) we keep the masked input tokens unchanged
return inputs, labels
def generate_batch_splits(samples_idx: np.ndarray, batch_size: int, drop_last=True) -> np.ndarray:
"""Generate batches of data for a specified batch size from sample indices. If the dataset size is not divisible by
the batch size and `drop_last` is `True`, the last incomplete batch is dropped. Else, it is returned."""
num_samples = len(samples_idx)
if drop_last:
samples_to_remove = num_samples % batch_size
if samples_to_remove != 0:
samples_idx = samples_idx[:-samples_to_remove]
sections_split = num_samples // batch_size
samples_idx = samples_idx.reshape((sections_split, batch_size))
else:
sections_split = math.ceil(num_samples / batch_size)
samples_idx = np.array_split(samples_idx, sections_split)
return samples_idx
def write_train_metric(summary_writer, train_metrics, train_time, step):
summary_writer.scalar("train_time", train_time, step)
train_metrics = get_metrics(train_metrics)
for key, vals in train_metrics.items():
tag = f"train_{key}"
for i, val in enumerate(vals):
summary_writer.scalar(tag, val, step - len(vals) + i + 1)
def write_eval_metric(summary_writer, eval_metrics, step):
for metric_name, value in eval_metrics.items():
summary_writer.scalar(f"eval_{metric_name}", value, step)
def main():
# See all possible arguments in src/transformers/training_args.py
# or by passing the --help flag to this script.
# We now keep distinct sets of args, for a cleaner separation of concerns.
parser = HfArgumentParser((ModelArguments, DataTrainingArguments, TrainingArguments))
if len(sys.argv) == 2 and sys.argv[1].endswith(".json"):
# If we pass only one argument to the script and it's the path to a json file,
# let's parse it to get our arguments.
model_args, data_args, training_args = parser.parse_json_file(json_file=os.path.abspath(sys.argv[1]))
else:
model_args, data_args, training_args = parser.parse_args_into_dataclasses()
if model_args.use_auth_token is not None:
warnings.warn(
"The `use_auth_token` argument is deprecated and will be removed in v4.34. Please use `token` instead.",
FutureWarning,
)
if model_args.token is not None:
raise ValueError("`token` and `use_auth_token` are both specified. Please set only the argument `token`.")
model_args.token = model_args.use_auth_token
# Sending telemetry. Tracking the example usage helps us better allocate resources to maintain them. The
# information sent is the one passed as arguments along with your Python/PyTorch versions.
send_example_telemetry("run_mlm", model_args, data_args, framework="flax")
if (
os.path.exists(training_args.output_dir)
and os.listdir(training_args.output_dir)
and training_args.do_train
and not training_args.overwrite_output_dir
):
raise ValueError(
f"Output directory ({training_args.output_dir}) already exists and is not empty. "
"Use --overwrite_output_dir to overcome."
)
# Setup logging
logging.basicConfig(
format="%(asctime)s - %(levelname)s - %(name)s - %(message)s",
level=logging.INFO,
datefmt="[%X]",
)
# Log on each process the small summary:
logger = logging.getLogger(__name__)
# Set the verbosity to info of the Transformers logger (on main process only):
logger.info(f"Training/evaluation parameters {training_args}")
# Set seed before initializing model.
set_seed(training_args.seed)
# Handle the repository creation
if training_args.push_to_hub:
# Retrieve of infer repo_name
repo_name = training_args.hub_model_id
if repo_name is None:
repo_name = Path(training_args.output_dir).absolute().name
# Create repo and retrieve repo_id
repo_id = create_repo(repo_name, exist_ok=True, token=training_args.hub_token).repo_id
# Clone repo locally
repo = Repository(training_args.output_dir, clone_from=repo_id, token=training_args.hub_token)
# Get the datasets: you can either provide your own CSV/JSON/TXT training and evaluation files (see below)
# or just provide the name of one of the public datasets available on the hub at https://huggingface.co/datasets/
# (the dataset will be downloaded automatically from the datasets Hub).
#
# For CSV/JSON files, this script will use the column called 'text' or the first column if no column called
# 'text' is found. You can easily tweak this behavior (see below).
#
# In distributed training, the load_dataset function guarantees that only one local process can concurrently
# download the dataset.
if data_args.dataset_name is not None:
# Downloading and loading a dataset from the hub.
datasets = load_dataset(
data_args.dataset_name,
data_args.dataset_config_name,
cache_dir=model_args.cache_dir,
token=model_args.token,
num_proc=data_args.preprocessing_num_workers,
)
if "validation" not in datasets.keys():
datasets["validation"] = load_dataset(
data_args.dataset_name,
data_args.dataset_config_name,
split=f"train[:{data_args.validation_split_percentage}%]",
cache_dir=model_args.cache_dir,
token=model_args.token,
num_proc=data_args.preprocessing_num_workers,
)
datasets["train"] = load_dataset(
data_args.dataset_name,
data_args.dataset_config_name,
split=f"train[{data_args.validation_split_percentage}%:]",
cache_dir=model_args.cache_dir,
token=model_args.token,
num_proc=data_args.preprocessing_num_workers,
)
else:
data_files = {}
if data_args.train_file is not None:
data_files["train"] = data_args.train_file
extension = data_args.train_file.split(".")[-1]
if data_args.validation_file is not None:
data_files["validation"] = data_args.validation_file
extension = data_args.validation_file.split(".")[-1]
if extension == "txt":
extension = "text"
datasets = load_dataset(
extension,
data_files=data_files,
cache_dir=model_args.cache_dir,
token=model_args.token,
num_proc=data_args.preprocessing_num_workers,
)
if "validation" not in datasets.keys():
datasets["validation"] = load_dataset(
extension,
data_files=data_files,
split=f"train[:{data_args.validation_split_percentage}%]",
cache_dir=model_args.cache_dir,
token=model_args.token,
num_proc=data_args.preprocessing_num_workers,
)
datasets["train"] = load_dataset(
extension,
data_files=data_files,
split=f"train[{data_args.validation_split_percentage}%:]",
cache_dir=model_args.cache_dir,
token=model_args.token,
num_proc=data_args.preprocessing_num_workers,
)
# See more about loading any type of standard or custom dataset (from files, python dict, pandas DataFrame, etc) at
# https://huggingface.co/docs/datasets/loading_datasets.
# Load pretrained model and tokenizer
# Distributed training:
# The .from_pretrained methods guarantee that only one local process can concurrently
# download model & vocab.
if model_args.config_name:
config = AutoConfig.from_pretrained(
model_args.config_name,
cache_dir=model_args.cache_dir,
token=model_args.token,
trust_remote_code=model_args.trust_remote_code,
)
elif model_args.model_name_or_path:
config = AutoConfig.from_pretrained(
model_args.model_name_or_path,
cache_dir=model_args.cache_dir,
token=model_args.token,
trust_remote_code=model_args.trust_remote_code,
)
else:
config = CONFIG_MAPPING[model_args.model_type]()
logger.warning("You are instantiating a new config instance from scratch.")
if model_args.tokenizer_name:
tokenizer = AutoTokenizer.from_pretrained(
model_args.tokenizer_name,
cache_dir=model_args.cache_dir,
use_fast=model_args.use_fast_tokenizer,
token=model_args.token,
trust_remote_code=model_args.trust_remote_code,
)
elif model_args.model_name_or_path:
tokenizer = AutoTokenizer.from_pretrained(
model_args.model_name_or_path,
cache_dir=model_args.cache_dir,
use_fast=model_args.use_fast_tokenizer,
token=model_args.token,
trust_remote_code=model_args.trust_remote_code,
)
else:
raise ValueError(
"You are instantiating a new tokenizer from scratch. This is not supported by this script. "
"You can do it from another script, save it, and load it from here, using --tokenizer_name."
)
# Preprocessing the datasets.
# First we tokenize all the texts.
if training_args.do_train:
column_names = datasets["train"].column_names
else:
column_names = datasets["validation"].column_names
text_column_name = "text" if "text" in column_names else column_names[0]
max_seq_length = min(data_args.max_seq_length, tokenizer.model_max_length)
if data_args.line_by_line:
# When using line_by_line, we just tokenize each nonempty line.
padding = "max_length" if data_args.pad_to_max_length else False
def tokenize_function(examples):
# Remove empty lines
examples = [line for line in examples if len(line) > 0 and not line.isspace()]
return tokenizer(
examples,
return_special_tokens_mask=True,
padding=padding,
truncation=True,
max_length=max_seq_length,
)
tokenized_datasets = datasets.map(
tokenize_function,
input_columns=[text_column_name],
batched=True,
num_proc=data_args.preprocessing_num_workers,
remove_columns=column_names,
load_from_cache_file=not data_args.overwrite_cache,
)
else:
# Otherwise, we tokenize every text, then concatenate them together before splitting them in smaller parts.
# We use `return_special_tokens_mask=True` because DataCollatorForLanguageModeling (see below) is more
# efficient when it receives the `special_tokens_mask`.
def tokenize_function(examples):
return tokenizer(examples[text_column_name], return_special_tokens_mask=True)
tokenized_datasets = datasets.map(
tokenize_function,
batched=True,
num_proc=data_args.preprocessing_num_workers,
remove_columns=column_names,
load_from_cache_file=not data_args.overwrite_cache,
)
# Main data processing function that will concatenate all texts from our dataset and generate chunks of
# max_seq_length.
def group_texts(examples):
# Concatenate all texts.
concatenated_examples = {k: list(chain(*examples[k])) for k in examples.keys()}
total_length = len(concatenated_examples[list(examples.keys())[0]])
# We drop the small remainder, we could add padding if the model supported it instead of this drop, you can
# customize this part to your needs.
if total_length >= max_seq_length:
total_length = (total_length // max_seq_length) * max_seq_length
# Split by chunks of max_len.
result = {
k: [t[i : i + max_seq_length] for i in range(0, total_length, max_seq_length)]
for k, t in concatenated_examples.items()
}
return result
# Note that with `batched=True`, this map processes 1,000 texts together, so group_texts throws away a
# remainder for each of those groups of 1,000 texts. You can adjust that batch_size here but a higher value
# might be slower to preprocess.
#
# To speed up this part, we use multiprocessing. See the documentation of the map method for more information:
# https://huggingface.co/docs/datasets/process#map
tokenized_datasets = tokenized_datasets.map(
group_texts,
batched=True,
num_proc=data_args.preprocessing_num_workers,
load_from_cache_file=not data_args.overwrite_cache,
)
# Enable tensorboard only on the master node
has_tensorboard = is_tensorboard_available()
if has_tensorboard and jax.process_index() == 0:
try:
from flax.metrics.tensorboard import SummaryWriter
summary_writer = SummaryWriter(log_dir=Path(training_args.output_dir))
except ImportError as ie:
has_tensorboard = False
logger.warning(
f"Unable to display metrics through TensorBoard because some package are not installed: {ie}"
)
else:
logger.warning(
"Unable to display metrics through TensorBoard because the package is not installed: "
"Please run pip install tensorboard to enable."
)
# Data collator
# This one will take care of randomly masking the tokens.
data_collator = FlaxDataCollatorForLanguageModeling(tokenizer=tokenizer, mlm_probability=data_args.mlm_probability)
# Initialize our training
rng = jax.random.PRNGKey(training_args.seed)
dropout_rngs = jax.random.split(rng, jax.local_device_count())
if model_args.model_name_or_path:
model = FlaxAutoModelForMaskedLM.from_pretrained(
model_args.model_name_or_path,
config=config,
seed=training_args.seed,
dtype=getattr(jnp, model_args.dtype),
token=model_args.token,
trust_remote_code=model_args.trust_remote_code,
)
else:
model = FlaxAutoModelForMaskedLM.from_config(
config,
seed=training_args.seed,
dtype=getattr(jnp, model_args.dtype),
trust_remote_code=model_args.trust_remote_code,
)
if training_args.gradient_checkpointing:
model.enable_gradient_checkpointing()
# Store some constant
num_epochs = int(training_args.num_train_epochs)
train_batch_size = int(training_args.per_device_train_batch_size) * jax.device_count()
per_device_eval_batch_size = int(training_args.per_device_eval_batch_size)
eval_batch_size = per_device_eval_batch_size * jax.device_count()
num_train_steps = len(tokenized_datasets["train"]) // train_batch_size * num_epochs
# Create learning rate schedule
warmup_fn = optax.linear_schedule(
init_value=0.0, end_value=training_args.learning_rate, transition_steps=training_args.warmup_steps
)
decay_fn = optax.linear_schedule(
init_value=training_args.learning_rate,
end_value=0,
transition_steps=num_train_steps - training_args.warmup_steps,
)
linear_decay_lr_schedule_fn = optax.join_schedules(
schedules=[warmup_fn, decay_fn], boundaries=[training_args.warmup_steps]
)
# We use Optax's "masking" functionality to not apply weight decay
# to bias and LayerNorm scale parameters. decay_mask_fn returns a
# mask boolean with the same structure as the parameters.
# The mask is True for parameters that should be decayed.
def decay_mask_fn(params):
flat_params = traverse_util.flatten_dict(params)
# find out all LayerNorm parameters
layer_norm_candidates = ["layernorm", "layer_norm", "ln"]
layer_norm_named_params = {
layer[-2:]
for layer_norm_name in layer_norm_candidates
for layer in flat_params.keys()
if layer_norm_name in "".join(layer).lower()
}
flat_mask = {path: (path[-1] != "bias" and path[-2:] not in layer_norm_named_params) for path in flat_params}
return traverse_util.unflatten_dict(flat_mask)
# create adam optimizer
if training_args.adafactor:
# We use the default parameters here to initialize adafactor,
# For more details about the parameters please check https://github.com/deepmind/optax/blob/ed02befef9bf81cbbf236be3d2b0e032e9ed4a40/optax/_src/alias.py#L74
optimizer = optax.adafactor(
learning_rate=linear_decay_lr_schedule_fn,
)
else:
optimizer = optax.adamw(
learning_rate=linear_decay_lr_schedule_fn,
b1=training_args.adam_beta1,
b2=training_args.adam_beta2,
eps=training_args.adam_epsilon,
weight_decay=training_args.weight_decay,
mask=decay_mask_fn,
)
# Setup train state
state = train_state.TrainState.create(apply_fn=model.__call__, params=model.params, tx=optimizer)
# Define gradient update step fn
def train_step(state, batch, dropout_rng):
dropout_rng, new_dropout_rng = jax.random.split(dropout_rng)
def loss_fn(params):
labels = batch.pop("labels")
logits = state.apply_fn(**batch, params=params, dropout_rng=dropout_rng, train=True)[0]
# compute loss, ignore padded input tokens
label_mask = jnp.where(labels > 0, 1.0, 0.0)
loss = optax.softmax_cross_entropy(logits, onehot(labels, logits.shape[-1])) * label_mask
# take average
loss = loss.sum()
num_labels = label_mask.sum()
return loss, num_labels
grad_fn = jax.value_and_grad(loss_fn, has_aux=True)
(loss, num_labels), grad = grad_fn(state.params)
num_labels = jax.lax.psum(num_labels, "batch")
# true loss = total loss / total samples
loss = jax.lax.psum(loss, "batch")
loss = jax.tree_util.tree_map(lambda x: x / num_labels, loss)
# true grad = total grad / total samples
grad = jax.lax.psum(grad, "batch")
grad = jax.tree_util.tree_map(lambda x: x / num_labels, grad)
new_state = state.apply_gradients(grads=grad)
metrics = {"loss": loss, "learning_rate": linear_decay_lr_schedule_fn(state.step)}
return new_state, metrics, new_dropout_rng
# Create parallel version of the train step
p_train_step = jax.pmap(train_step, "batch", donate_argnums=(0,))
# Define eval fn
def eval_step(params, batch):
labels = batch.pop("labels")
logits = model(**batch, params=params, train=False)[0]
# compute loss, ignore padded input tokens
label_mask = jnp.where(labels > 0, 1.0, 0.0)
loss = optax.softmax_cross_entropy(logits, onehot(labels, logits.shape[-1])) * label_mask
# compute accuracy
accuracy = jnp.equal(jnp.argmax(logits, axis=-1), labels) * label_mask
# summarize metrics
metrics = {"loss": loss.sum(), "accuracy": accuracy.sum(), "normalizer": label_mask.sum()}
metrics = jax.lax.psum(metrics, axis_name="batch")
return metrics
p_eval_step = jax.pmap(eval_step, "batch", donate_argnums=(0,))
# Replicate the train state on each device
state = jax_utils.replicate(state)
train_time = 0
epochs = tqdm(range(num_epochs), desc=f"Epoch ... (1/{num_epochs})", position=0)
for epoch in epochs:
# ======================== Training ================================
train_start = time.time()
train_metrics = []
# Create sampling rng
rng, input_rng = jax.random.split(rng)
# Generate an epoch by shuffling sampling indices from the train dataset
num_train_samples = len(tokenized_datasets["train"])
# Avoid using jax.numpy here in case of TPU training
train_samples_idx = np.random.permutation(np.arange(num_train_samples))
train_batch_idx = generate_batch_splits(train_samples_idx, train_batch_size)
# Gather the indexes for creating the batch and do a training step
for step, batch_idx in enumerate(tqdm(train_batch_idx, desc="Training...", position=1)):
samples = [tokenized_datasets["train"][int(idx)] for idx in batch_idx]
model_inputs = data_collator(samples, pad_to_multiple_of=16)
# Model forward
model_inputs = shard(model_inputs.data)
state, train_metric, dropout_rngs = p_train_step(state, model_inputs, dropout_rngs)
train_metrics.append(train_metric)
cur_step = epoch * (num_train_samples // train_batch_size) + step
if cur_step % training_args.logging_steps == 0 and cur_step > 0:
# Save metrics
train_metric = jax_utils.unreplicate(train_metric)
train_time += time.time() - train_start
if has_tensorboard and jax.process_index() == 0:
write_train_metric(summary_writer, train_metrics, train_time, cur_step)
epochs.write(
f"Step... ({cur_step} | Loss: {train_metric['loss']}, Learning Rate:"
f" {train_metric['learning_rate']})"
)
train_metrics = []
if cur_step % training_args.eval_steps == 0 and cur_step > 0:
# ======================== Evaluating ==============================
num_eval_samples = len(tokenized_datasets["validation"])
# Avoid using jax.numpy here in case of TPU training
eval_samples_idx = np.arange(num_eval_samples)
eval_batch_idx = generate_batch_splits(eval_samples_idx, eval_batch_size, drop_last=False)
eval_metrics = []
for i, batch_idx in enumerate(tqdm(eval_batch_idx, desc="Evaluating ...", position=2)):
samples = [tokenized_datasets["validation"][int(idx)] for idx in batch_idx]
model_inputs = data_collator(samples, pad_to_multiple_of=16)
# Model forward
metrics = pad_shard_unpad(p_eval_step, static_return=True)(
state.params, model_inputs.data, min_device_batch=per_device_eval_batch_size
)
eval_metrics.append(metrics)
# normalize eval metrics
eval_metrics = get_metrics(eval_metrics)
eval_metrics = jax.tree_util.tree_map(jnp.sum, eval_metrics)
eval_normalizer = eval_metrics.pop("normalizer")
eval_metrics = jax.tree_util.tree_map(lambda x: x / eval_normalizer, eval_metrics)
# Update progress bar
epochs.desc = f"Step... ({cur_step} | Loss: {eval_metrics['loss']}, Acc: {eval_metrics['accuracy']})"
# Save metrics
if has_tensorboard and jax.process_index() == 0:
write_eval_metric(summary_writer, eval_metrics, cur_step)
if cur_step % training_args.save_steps == 0 and cur_step > 0:
# save checkpoint after each epoch and push checkpoint to the hub
if jax.process_index() == 0:
params = jax.device_get(jax.tree_util.tree_map(lambda x: x[0], state.params))
model.save_pretrained(training_args.output_dir, params=params)
tokenizer.save_pretrained(training_args.output_dir)
if training_args.push_to_hub:
repo.push_to_hub(commit_message=f"Saving weights and logs of step {cur_step}", blocking=False)
# Eval after training
if training_args.do_eval:
num_eval_samples = len(tokenized_datasets["validation"])
# Avoid using jax.numpy here in case of TPU training
eval_samples_idx = np.arange(num_eval_samples)
eval_batch_idx = generate_batch_splits(eval_samples_idx, eval_batch_size, drop_last=False)
eval_metrics = []
for _, batch_idx in enumerate(tqdm(eval_batch_idx, desc="Evaluating ...", position=2)):
samples = [tokenized_datasets["validation"][int(idx)] for idx in batch_idx]
model_inputs = data_collator(samples, pad_to_multiple_of=16)
# Model forward
metrics = pad_shard_unpad(p_eval_step, static_return=True)(
state.params, model_inputs.data, min_device_batch=per_device_eval_batch_size
)
eval_metrics.append(metrics)
# normalize eval metrics
eval_metrics = get_metrics(eval_metrics)
eval_metrics = jax.tree_util.tree_map(lambda metric: jnp.sum(metric).item(), eval_metrics)
eval_normalizer = eval_metrics.pop("normalizer")
eval_metrics = jax.tree_util.tree_map(lambda x: x / eval_normalizer, eval_metrics)
try:
perplexity = math.exp(eval_metrics["loss"])
except OverflowError:
perplexity = float("inf")
eval_metrics["perplexity"] = perplexity
if jax.process_index() == 0:
eval_metrics = {f"eval_{metric_name}": value for metric_name, value in eval_metrics.items()}
path = os.path.join(training_args.output_dir, "eval_results.json")
with open(path, "w") as f:
json.dump(eval_metrics, f, indent=4, sort_keys=True)
if __name__ == "__main__":
main()
| transformers/examples/flax/language-modeling/run_mlm_flax.py/0 | {
"file_path": "transformers/examples/flax/language-modeling/run_mlm_flax.py",
"repo_id": "transformers",
"token_count": 17080
} | 278 |
#!/usr/bin/env python
# coding=utf-8
# Copyright 2021 The HuggingFace Inc. team. All rights reserved.
#
# Licensed under the Apache License, Version 2.0 (the "License");
# you may not use this file except in compliance with the License.
# You may obtain a copy of the License at
#
# http://www.apache.org/licenses/LICENSE-2.0
#
# Unless required by applicable law or agreed to in writing, software
# distributed under the License is distributed on an "AS IS" BASIS,
# WITHOUT WARRANTIES OR CONDITIONS OF ANY KIND, either express or implied.
# See the License for the specific language governing permissions and
# limitations under the License.
""" Finetuning a ๐ค Flax Transformers model for sequence classification on GLUE."""
import json
import logging
import math
import os
import random
import sys
import time
import warnings
from dataclasses import dataclass, field
from pathlib import Path
from typing import Any, Callable, Dict, Optional, Tuple
import datasets
import evaluate
import jax
import jax.numpy as jnp
import numpy as np
import optax
from datasets import load_dataset
from flax import struct, traverse_util
from flax.jax_utils import pad_shard_unpad, replicate, unreplicate
from flax.training import train_state
from flax.training.common_utils import get_metrics, onehot, shard
from huggingface_hub import Repository, create_repo
from tqdm import tqdm
import transformers
from transformers import (
AutoConfig,
AutoTokenizer,
FlaxAutoModelForSequenceClassification,
HfArgumentParser,
PretrainedConfig,
TrainingArguments,
is_tensorboard_available,
)
from transformers.utils import check_min_version, send_example_telemetry
logger = logging.getLogger(__name__)
# Will error if the minimal version of Transformers is not installed. Remove at your own risks.
check_min_version("4.38.0.dev0")
Array = Any
Dataset = datasets.arrow_dataset.Dataset
PRNGKey = Any
task_to_keys = {
"cola": ("sentence", None),
"mnli": ("premise", "hypothesis"),
"mrpc": ("sentence1", "sentence2"),
"qnli": ("question", "sentence"),
"qqp": ("question1", "question2"),
"rte": ("sentence1", "sentence2"),
"sst2": ("sentence", None),
"stsb": ("sentence1", "sentence2"),
"wnli": ("sentence1", "sentence2"),
}
@dataclass
class ModelArguments:
"""
Arguments pertaining to which model/config/tokenizer we are going to fine-tune from.
"""
model_name_or_path: str = field(
metadata={"help": "Path to pretrained model or model identifier from huggingface.co/models"}
)
config_name: Optional[str] = field(
default=None, metadata={"help": "Pretrained config name or path if not the same as model_name"}
)
tokenizer_name: Optional[str] = field(
default=None, metadata={"help": "Pretrained tokenizer name or path if not the same as model_name"}
)
use_slow_tokenizer: Optional[bool] = field(
default=False,
metadata={"help": "If passed, will use a slow tokenizer (not backed by the ๐ค Tokenizers library)."},
)
cache_dir: Optional[str] = field(
default=None,
metadata={"help": "Where do you want to store the pretrained models downloaded from huggingface.co"},
)
model_revision: str = field(
default="main",
metadata={"help": "The specific model version to use (can be a branch name, tag name or commit id)."},
)
token: str = field(
default=None,
metadata={
"help": (
"The token to use as HTTP bearer authorization for remote files. If not specified, will use the token "
"generated when running `huggingface-cli login` (stored in `~/.huggingface`)."
)
},
)
use_auth_token: bool = field(
default=None,
metadata={
"help": "The `use_auth_token` argument is deprecated and will be removed in v4.34. Please use `token` instead."
},
)
trust_remote_code: bool = field(
default=False,
metadata={
"help": (
"Whether or not to allow for custom models defined on the Hub in their own modeling files. This option "
"should only be set to `True` for repositories you trust and in which you have read the code, as it will "
"execute code present on the Hub on your local machine."
)
},
)
@dataclass
class DataTrainingArguments:
"""
Arguments pertaining to what data we are going to input our model for training and eval.
"""
task_name: Optional[str] = field(
default=None, metadata={"help": f"The name of the glue task to train on. choices {list(task_to_keys.keys())}"}
)
dataset_config_name: Optional[str] = field(
default=None, metadata={"help": "The configuration name of the dataset to use (via the datasets library)."}
)
train_file: Optional[str] = field(
default=None, metadata={"help": "The input training data file (a csv or JSON file)."}
)
validation_file: Optional[str] = field(
default=None,
metadata={"help": "An optional input evaluation data file to evaluate on (a csv or JSON file)."},
)
test_file: Optional[str] = field(
default=None,
metadata={"help": "An optional input test data file to predict on (a csv or JSON file)."},
)
text_column_name: Optional[str] = field(
default=None, metadata={"help": "The column name of text to input in the file (a csv or JSON file)."}
)
label_column_name: Optional[str] = field(
default=None, metadata={"help": "The column name of label to input in the file (a csv or JSON file)."}
)
overwrite_cache: bool = field(
default=False, metadata={"help": "Overwrite the cached training and evaluation sets"}
)
preprocessing_num_workers: Optional[int] = field(
default=None,
metadata={"help": "The number of processes to use for the preprocessing."},
)
max_seq_length: int = field(
default=None,
metadata={
"help": (
"The maximum total input sequence length after tokenization. If set, sequences longer "
"than this will be truncated, sequences shorter will be padded."
)
},
)
max_train_samples: Optional[int] = field(
default=None,
metadata={
"help": (
"For debugging purposes or quicker training, truncate the number of training examples to this "
"value if set."
)
},
)
max_eval_samples: Optional[int] = field(
default=None,
metadata={
"help": (
"For debugging purposes or quicker training, truncate the number of evaluation examples to this "
"value if set."
)
},
)
max_predict_samples: Optional[int] = field(
default=None,
metadata={
"help": (
"For debugging purposes or quicker training, truncate the number of prediction examples to this "
"value if set."
)
},
)
def __post_init__(self):
if self.task_name is None and self.train_file is None and self.validation_file is None:
raise ValueError("Need either a dataset name or a training/validation file.")
else:
if self.train_file is not None:
extension = self.train_file.split(".")[-1]
assert extension in ["csv", "json"], "`train_file` should be a csv or a json file."
if self.validation_file is not None:
extension = self.validation_file.split(".")[-1]
assert extension in ["csv", "json"], "`validation_file` should be a csv or a json file."
self.task_name = self.task_name.lower() if isinstance(self.task_name, str) else self.task_name
def create_train_state(
model: FlaxAutoModelForSequenceClassification,
learning_rate_fn: Callable[[int], float],
is_regression: bool,
num_labels: int,
weight_decay: float,
) -> train_state.TrainState:
"""Create initial training state."""
class TrainState(train_state.TrainState):
"""Train state with an Optax optimizer.
The two functions below differ depending on whether the task is classification
or regression.
Args:
logits_fn: Applied to last layer to obtain the logits.
loss_fn: Function to compute the loss.
"""
logits_fn: Callable = struct.field(pytree_node=False)
loss_fn: Callable = struct.field(pytree_node=False)
# We use Optax's "masking" functionality to not apply weight decay
# to bias and LayerNorm scale parameters. decay_mask_fn returns a
# mask boolean with the same structure as the parameters.
# The mask is True for parameters that should be decayed.
def decay_mask_fn(params):
flat_params = traverse_util.flatten_dict(params)
# find out all LayerNorm parameters
layer_norm_candidates = ["layernorm", "layer_norm", "ln"]
layer_norm_named_params = {
layer[-2:]
for layer_norm_name in layer_norm_candidates
for layer in flat_params.keys()
if layer_norm_name in "".join(layer).lower()
}
flat_mask = {path: (path[-1] != "bias" and path[-2:] not in layer_norm_named_params) for path in flat_params}
return traverse_util.unflatten_dict(flat_mask)
tx = optax.adamw(
learning_rate=learning_rate_fn, b1=0.9, b2=0.999, eps=1e-6, weight_decay=weight_decay, mask=decay_mask_fn
)
if is_regression:
def mse_loss(logits, labels):
return jnp.mean((logits[..., 0] - labels) ** 2)
return TrainState.create(
apply_fn=model.__call__,
params=model.params,
tx=tx,
logits_fn=lambda logits: logits[..., 0],
loss_fn=mse_loss,
)
else: # Classification.
def cross_entropy_loss(logits, labels):
xentropy = optax.softmax_cross_entropy(logits, onehot(labels, num_classes=num_labels))
return jnp.mean(xentropy)
return TrainState.create(
apply_fn=model.__call__,
params=model.params,
tx=tx,
logits_fn=lambda logits: logits.argmax(-1),
loss_fn=cross_entropy_loss,
)
def create_learning_rate_fn(
train_ds_size: int, train_batch_size: int, num_train_epochs: int, num_warmup_steps: int, learning_rate: float
) -> Callable[[int], jnp.ndarray]:
"""Returns a linear warmup, linear_decay learning rate function."""
steps_per_epoch = train_ds_size // train_batch_size
num_train_steps = steps_per_epoch * num_train_epochs
warmup_fn = optax.linear_schedule(init_value=0.0, end_value=learning_rate, transition_steps=num_warmup_steps)
decay_fn = optax.linear_schedule(
init_value=learning_rate, end_value=0, transition_steps=num_train_steps - num_warmup_steps
)
schedule_fn = optax.join_schedules(schedules=[warmup_fn, decay_fn], boundaries=[num_warmup_steps])
return schedule_fn
def glue_train_data_collator(rng: PRNGKey, dataset: Dataset, batch_size: int):
"""Returns shuffled batches of size `batch_size` from truncated `train dataset`, sharded over all local devices."""
steps_per_epoch = len(dataset) // batch_size
perms = jax.random.permutation(rng, len(dataset))
perms = perms[: steps_per_epoch * batch_size] # Skip incomplete batch.
perms = perms.reshape((steps_per_epoch, batch_size))
for perm in perms:
batch = dataset[perm]
batch = {k: np.array(v) for k, v in batch.items()}
batch = shard(batch)
yield batch
def glue_eval_data_collator(dataset: Dataset, batch_size: int):
"""Returns batches of size `batch_size` from `eval dataset`. Sharding handled by `pad_shard_unpad` in the eval loop."""
batch_idx = np.arange(len(dataset))
steps_per_epoch = math.ceil(len(dataset) / batch_size)
batch_idx = np.array_split(batch_idx, steps_per_epoch)
for idx in batch_idx:
batch = dataset[idx]
batch = {k: np.array(v) for k, v in batch.items()}
yield batch
def main():
parser = HfArgumentParser((ModelArguments, DataTrainingArguments, TrainingArguments))
if len(sys.argv) == 2 and sys.argv[1].endswith(".json"):
# If we pass only one argument to the script and it's the path to a json file,
# let's parse it to get our arguments.
model_args, data_args, training_args = parser.parse_json_file(json_file=os.path.abspath(sys.argv[1]))
else:
model_args, data_args, training_args = parser.parse_args_into_dataclasses()
if model_args.use_auth_token is not None:
warnings.warn(
"The `use_auth_token` argument is deprecated and will be removed in v4.34. Please use `token` instead.",
FutureWarning,
)
if model_args.token is not None:
raise ValueError("`token` and `use_auth_token` are both specified. Please set only the argument `token`.")
model_args.token = model_args.use_auth_token
# Sending telemetry. Tracking the example usage helps us better allocate resources to maintain them. The
# information sent is the one passed as arguments along with your Python/PyTorch versions.
send_example_telemetry("run_glue", model_args, data_args, framework="flax")
# Make one log on every process with the configuration for debugging.
logging.basicConfig(
format="%(asctime)s - %(levelname)s - %(name)s - %(message)s",
datefmt="%m/%d/%Y %H:%M:%S",
level=logging.INFO,
)
# Setup logging, we only want one process per machine to log things on the screen.
logger.setLevel(logging.INFO if jax.process_index() == 0 else logging.ERROR)
if jax.process_index() == 0:
datasets.utils.logging.set_verbosity_warning()
transformers.utils.logging.set_verbosity_info()
else:
datasets.utils.logging.set_verbosity_error()
transformers.utils.logging.set_verbosity_error()
# Handle the repository creation
if training_args.push_to_hub:
# Retrieve of infer repo_name
repo_name = training_args.hub_model_id
if repo_name is None:
repo_name = Path(training_args.output_dir).absolute().name
# Create repo and retrieve repo_id
repo_id = create_repo(repo_name, exist_ok=True, token=training_args.hub_token).repo_id
# Clone repo locally
repo = Repository(training_args.output_dir, clone_from=repo_id, token=training_args.hub_token)
# Get the datasets: you can either provide your own CSV/JSON training and evaluation files (see below)
# or specify a GLUE benchmark task (the dataset will be downloaded automatically from the datasets Hub).
# For CSV/JSON files, this script will use as labels the column called 'label' and as pair of sentences the
# sentences in columns called 'sentence1' and 'sentence2' if such column exists or the first two columns not named
# label if at least two columns are provided.
# If the CSVs/JSONs contain only one non-label column, the script does single sentence classification on this
# single column. You can easily tweak this behavior (see below)
# In distributed training, the load_dataset function guarantee that only one local process can concurrently
# download the dataset.
if data_args.task_name is not None:
# Downloading and loading a dataset from the hub.
raw_datasets = load_dataset(
"glue",
data_args.task_name,
token=model_args.token,
)
else:
# Loading the dataset from local csv or json file.
data_files = {}
if data_args.train_file is not None:
data_files["train"] = data_args.train_file
if data_args.validation_file is not None:
data_files["validation"] = data_args.validation_file
extension = (data_args.train_file if data_args.train_file is not None else data_args.valid_file).split(".")[-1]
raw_datasets = load_dataset(
extension,
data_files=data_files,
token=model_args.token,
)
# See more about loading any type of standard or custom dataset at
# https://huggingface.co/docs/datasets/loading_datasets.
# Labels
if data_args.task_name is not None:
is_regression = data_args.task_name == "stsb"
if not is_regression:
label_list = raw_datasets["train"].features["label"].names
num_labels = len(label_list)
else:
num_labels = 1
else:
# Trying to have good defaults here, don't hesitate to tweak to your needs.
is_regression = raw_datasets["train"].features["label"].dtype in ["float32", "float64"]
if is_regression:
num_labels = 1
else:
# A useful fast method:
# https://huggingface.co/docs/datasets/package_reference/main_classes#datasets.Dataset.unique
label_list = raw_datasets["train"].unique("label")
label_list.sort() # Let's sort it for determinism
num_labels = len(label_list)
# Load pretrained model and tokenizer
config = AutoConfig.from_pretrained(
model_args.model_name_or_path,
num_labels=num_labels,
finetuning_task=data_args.task_name,
token=model_args.token,
trust_remote_code=model_args.trust_remote_code,
)
tokenizer = AutoTokenizer.from_pretrained(
model_args.model_name_or_path,
use_fast=not model_args.use_slow_tokenizer,
token=model_args.token,
trust_remote_code=model_args.trust_remote_code,
)
model = FlaxAutoModelForSequenceClassification.from_pretrained(
model_args.model_name_or_path,
config=config,
token=model_args.token,
trust_remote_code=model_args.trust_remote_code,
)
# Preprocessing the datasets
if data_args.task_name is not None:
sentence1_key, sentence2_key = task_to_keys[data_args.task_name]
else:
# Again, we try to have some nice defaults but don't hesitate to tweak to your use case.
non_label_column_names = [name for name in raw_datasets["train"].column_names if name != "label"]
if "sentence1" in non_label_column_names and "sentence2" in non_label_column_names:
sentence1_key, sentence2_key = "sentence1", "sentence2"
else:
if len(non_label_column_names) >= 2:
sentence1_key, sentence2_key = non_label_column_names[:2]
else:
sentence1_key, sentence2_key = non_label_column_names[0], None
# Some models have set the order of the labels to use, so let's make sure we do use it.
label_to_id = None
if (
model.config.label2id != PretrainedConfig(num_labels=num_labels).label2id
and data_args.task_name is not None
and not is_regression
):
# Some have all caps in their config, some don't.
label_name_to_id = {k.lower(): v for k, v in model.config.label2id.items()}
if sorted(label_name_to_id.keys()) == sorted(label_list):
logger.info(
f"The configuration of the model provided the following label correspondence: {label_name_to_id}. "
"Using it!"
)
label_to_id = {i: label_name_to_id[label_list[i]] for i in range(num_labels)}
else:
logger.warning(
"Your model seems to have been trained with labels, but they don't match the dataset: ",
f"model labels: {sorted(label_name_to_id.keys())}, dataset labels: {sorted(label_list)}."
"\nIgnoring the model labels as a result.",
)
elif data_args.task_name is None:
label_to_id = {v: i for i, v in enumerate(label_list)}
def preprocess_function(examples):
# Tokenize the texts
texts = (
(examples[sentence1_key],) if sentence2_key is None else (examples[sentence1_key], examples[sentence2_key])
)
result = tokenizer(*texts, padding="max_length", max_length=data_args.max_seq_length, truncation=True)
if "label" in examples:
if label_to_id is not None:
# Map labels to IDs (not necessary for GLUE tasks)
result["labels"] = [label_to_id[l] for l in examples["label"]]
else:
# In all cases, rename the column to labels because the model will expect that.
result["labels"] = examples["label"]
return result
processed_datasets = raw_datasets.map(
preprocess_function, batched=True, remove_columns=raw_datasets["train"].column_names
)
train_dataset = processed_datasets["train"]
eval_dataset = processed_datasets["validation_matched" if data_args.task_name == "mnli" else "validation"]
# Log a few random samples from the training set:
for index in random.sample(range(len(train_dataset)), 3):
logger.info(f"Sample {index} of the training set: {train_dataset[index]}.")
# Define a summary writer
has_tensorboard = is_tensorboard_available()
if has_tensorboard and jax.process_index() == 0:
try:
from flax.metrics.tensorboard import SummaryWriter
summary_writer = SummaryWriter(training_args.output_dir)
summary_writer.hparams({**training_args.to_dict(), **vars(model_args), **vars(data_args)})
except ImportError as ie:
has_tensorboard = False
logger.warning(
f"Unable to display metrics through TensorBoard because some package are not installed: {ie}"
)
else:
logger.warning(
"Unable to display metrics through TensorBoard because the package is not installed: "
"Please run pip install tensorboard to enable."
)
def write_train_metric(summary_writer, train_metrics, train_time, step):
summary_writer.scalar("train_time", train_time, step)
train_metrics = get_metrics(train_metrics)
for key, vals in train_metrics.items():
tag = f"train_{key}"
for i, val in enumerate(vals):
summary_writer.scalar(tag, val, step - len(vals) + i + 1)
def write_eval_metric(summary_writer, eval_metrics, step):
for metric_name, value in eval_metrics.items():
summary_writer.scalar(f"eval_{metric_name}", value, step)
num_epochs = int(training_args.num_train_epochs)
rng = jax.random.PRNGKey(training_args.seed)
dropout_rngs = jax.random.split(rng, jax.local_device_count())
train_batch_size = int(training_args.per_device_train_batch_size) * jax.local_device_count()
per_device_eval_batch_size = int(training_args.per_device_eval_batch_size)
eval_batch_size = per_device_eval_batch_size * jax.device_count()
learning_rate_fn = create_learning_rate_fn(
len(train_dataset),
train_batch_size,
training_args.num_train_epochs,
training_args.warmup_steps,
training_args.learning_rate,
)
state = create_train_state(
model, learning_rate_fn, is_regression, num_labels=num_labels, weight_decay=training_args.weight_decay
)
# define step functions
def train_step(
state: train_state.TrainState, batch: Dict[str, Array], dropout_rng: PRNGKey
) -> Tuple[train_state.TrainState, float]:
"""Trains model with an optimizer (both in `state`) on `batch`, returning a pair `(new_state, loss)`."""
dropout_rng, new_dropout_rng = jax.random.split(dropout_rng)
targets = batch.pop("labels")
def loss_fn(params):
logits = state.apply_fn(**batch, params=params, dropout_rng=dropout_rng, train=True)[0]
loss = state.loss_fn(logits, targets)
return loss
grad_fn = jax.value_and_grad(loss_fn)
loss, grad = grad_fn(state.params)
grad = jax.lax.pmean(grad, "batch")
new_state = state.apply_gradients(grads=grad)
metrics = jax.lax.pmean({"loss": loss, "learning_rate": learning_rate_fn(state.step)}, axis_name="batch")
return new_state, metrics, new_dropout_rng
p_train_step = jax.pmap(train_step, axis_name="batch", donate_argnums=(0,))
def eval_step(state, batch):
logits = state.apply_fn(**batch, params=state.params, train=False)[0]
return state.logits_fn(logits)
p_eval_step = jax.pmap(eval_step, axis_name="batch")
if data_args.task_name is not None:
metric = evaluate.load("glue", data_args.task_name, cache_dir=model_args.cache_dir)
else:
metric = evaluate.load("accuracy", cache_dir=model_args.cache_dir)
logger.info(f"===== Starting training ({num_epochs} epochs) =====")
train_time = 0
# make sure weights are replicated on each device
state = replicate(state)
steps_per_epoch = len(train_dataset) // train_batch_size
total_steps = steps_per_epoch * num_epochs
epochs = tqdm(range(num_epochs), desc=f"Epoch ... (0/{num_epochs})", position=0)
for epoch in epochs:
train_start = time.time()
train_metrics = []
# Create sampling rng
rng, input_rng = jax.random.split(rng)
# train
train_loader = glue_train_data_collator(input_rng, train_dataset, train_batch_size)
for step, batch in enumerate(
tqdm(
train_loader,
total=steps_per_epoch,
desc="Training...",
position=1,
),
):
state, train_metric, dropout_rngs = p_train_step(state, batch, dropout_rngs)
train_metrics.append(train_metric)
cur_step = (epoch * steps_per_epoch) + (step + 1)
if cur_step % training_args.logging_steps == 0 and cur_step > 0:
# Save metrics
train_metric = unreplicate(train_metric)
train_time += time.time() - train_start
if has_tensorboard and jax.process_index() == 0:
write_train_metric(summary_writer, train_metrics, train_time, cur_step)
epochs.write(
f"Step... ({cur_step}/{total_steps} | Training Loss: {train_metric['loss']}, Learning Rate:"
f" {train_metric['learning_rate']})"
)
train_metrics = []
if (cur_step % training_args.eval_steps == 0 or cur_step % steps_per_epoch == 0) and cur_step > 0:
# evaluate
eval_loader = glue_eval_data_collator(eval_dataset, eval_batch_size)
for batch in tqdm(
eval_loader,
total=math.ceil(len(eval_dataset) / eval_batch_size),
desc="Evaluating ...",
position=2,
):
labels = batch.pop("labels")
predictions = pad_shard_unpad(p_eval_step)(
state, batch, min_device_batch=per_device_eval_batch_size
)
metric.add_batch(predictions=np.array(predictions), references=labels)
eval_metric = metric.compute()
logger.info(f"Step... ({cur_step}/{total_steps} | Eval metrics: {eval_metric})")
if has_tensorboard and jax.process_index() == 0:
write_eval_metric(summary_writer, eval_metric, cur_step)
if (cur_step % training_args.save_steps == 0 and cur_step > 0) or (cur_step == total_steps):
# save checkpoint after each epoch and push checkpoint to the hub
if jax.process_index() == 0:
params = jax.device_get(unreplicate(state.params))
model.save_pretrained(training_args.output_dir, params=params)
tokenizer.save_pretrained(training_args.output_dir)
if training_args.push_to_hub:
repo.push_to_hub(commit_message=f"Saving weights and logs of step {cur_step}", blocking=False)
epochs.desc = f"Epoch ... {epoch + 1}/{num_epochs}"
# save the eval metrics in json
if jax.process_index() == 0:
eval_metric = {f"eval_{metric_name}": value for metric_name, value in eval_metric.items()}
path = os.path.join(training_args.output_dir, "eval_results.json")
with open(path, "w") as f:
json.dump(eval_metric, f, indent=4, sort_keys=True)
if __name__ == "__main__":
main()
| transformers/examples/flax/text-classification/run_flax_glue.py/0 | {
"file_path": "transformers/examples/flax/text-classification/run_flax_glue.py",
"repo_id": "transformers",
"token_count": 11980
} | 279 |
import argparse
import glob
import logging
import os
import time
from argparse import Namespace
import numpy as np
import torch
from lightning_base import BaseTransformer, add_generic_args, generic_train
from torch.utils.data import DataLoader, TensorDataset
from transformers import glue_compute_metrics as compute_metrics
from transformers import glue_convert_examples_to_features as convert_examples_to_features
from transformers import glue_output_modes, glue_tasks_num_labels
from transformers import glue_processors as processors
logger = logging.getLogger(__name__)
class GLUETransformer(BaseTransformer):
mode = "sequence-classification"
def __init__(self, hparams):
if isinstance(hparams, dict):
hparams = Namespace(**hparams)
hparams.glue_output_mode = glue_output_modes[hparams.task]
num_labels = glue_tasks_num_labels[hparams.task]
super().__init__(hparams, num_labels, self.mode)
def forward(self, **inputs):
return self.model(**inputs)
def training_step(self, batch, batch_idx):
inputs = {"input_ids": batch[0], "attention_mask": batch[1], "labels": batch[3]}
if self.config.model_type not in ["distilbert", "bart"]:
inputs["token_type_ids"] = batch[2] if self.config.model_type in ["bert", "xlnet", "albert"] else None
outputs = self(**inputs)
loss = outputs[0]
lr_scheduler = self.trainer.lr_schedulers[0]["scheduler"]
tensorboard_logs = {"loss": loss, "rate": lr_scheduler.get_last_lr()[-1]}
return {"loss": loss, "log": tensorboard_logs}
def prepare_data(self):
"Called to initialize data. Use the call to construct features"
args = self.hparams
processor = processors[args.task]()
self.labels = processor.get_labels()
for mode in ["train", "dev"]:
cached_features_file = self._feature_file(mode)
if os.path.exists(cached_features_file) and not args.overwrite_cache:
logger.info("Loading features from cached file %s", cached_features_file)
else:
logger.info("Creating features from dataset file at %s", args.data_dir)
examples = (
processor.get_dev_examples(args.data_dir)
if mode == "dev"
else processor.get_train_examples(args.data_dir)
)
features = convert_examples_to_features(
examples,
self.tokenizer,
max_length=args.max_seq_length,
label_list=self.labels,
output_mode=args.glue_output_mode,
)
logger.info("Saving features into cached file %s", cached_features_file)
torch.save(features, cached_features_file)
def get_dataloader(self, mode: str, batch_size: int, shuffle: bool = False) -> DataLoader:
"Load datasets. Called after prepare data."
# We test on dev set to compare to benchmarks without having to submit to GLUE server
mode = "dev" if mode == "test" else mode
cached_features_file = self._feature_file(mode)
logger.info("Loading features from cached file %s", cached_features_file)
features = torch.load(cached_features_file)
all_input_ids = torch.tensor([f.input_ids for f in features], dtype=torch.long)
all_attention_mask = torch.tensor([f.attention_mask for f in features], dtype=torch.long)
all_token_type_ids = torch.tensor([f.token_type_ids for f in features], dtype=torch.long)
if self.hparams.glue_output_mode == "classification":
all_labels = torch.tensor([f.label for f in features], dtype=torch.long)
elif self.hparams.glue_output_mode == "regression":
all_labels = torch.tensor([f.label for f in features], dtype=torch.float)
return DataLoader(
TensorDataset(all_input_ids, all_attention_mask, all_token_type_ids, all_labels),
batch_size=batch_size,
shuffle=shuffle,
)
def validation_step(self, batch, batch_idx):
inputs = {"input_ids": batch[0], "attention_mask": batch[1], "labels": batch[3]}
if self.config.model_type not in ["distilbert", "bart"]:
inputs["token_type_ids"] = batch[2] if self.config.model_type in ["bert", "xlnet", "albert"] else None
outputs = self(**inputs)
tmp_eval_loss, logits = outputs[:2]
preds = logits.detach().cpu().numpy()
out_label_ids = inputs["labels"].detach().cpu().numpy()
return {"val_loss": tmp_eval_loss.detach().cpu(), "pred": preds, "target": out_label_ids}
def _eval_end(self, outputs) -> tuple:
val_loss_mean = torch.stack([x["val_loss"] for x in outputs]).mean().detach().cpu().item()
preds = np.concatenate([x["pred"] for x in outputs], axis=0)
if self.hparams.glue_output_mode == "classification":
preds = np.argmax(preds, axis=1)
elif self.hparams.glue_output_mode == "regression":
preds = np.squeeze(preds)
out_label_ids = np.concatenate([x["target"] for x in outputs], axis=0)
out_label_list = [[] for _ in range(out_label_ids.shape[0])]
preds_list = [[] for _ in range(out_label_ids.shape[0])]
results = {**{"val_loss": val_loss_mean}, **compute_metrics(self.hparams.task, preds, out_label_ids)}
ret = dict(results.items())
ret["log"] = results
return ret, preds_list, out_label_list
def validation_epoch_end(self, outputs: list) -> dict:
ret, preds, targets = self._eval_end(outputs)
logs = ret["log"]
return {"val_loss": logs["val_loss"], "log": logs, "progress_bar": logs}
def test_epoch_end(self, outputs) -> dict:
ret, predictions, targets = self._eval_end(outputs)
logs = ret["log"]
# `val_loss` is the key returned by `self._eval_end()` but actually refers to `test_loss`
return {"avg_test_loss": logs["val_loss"], "log": logs, "progress_bar": logs}
@staticmethod
def add_model_specific_args(parser, root_dir):
BaseTransformer.add_model_specific_args(parser, root_dir)
parser.add_argument(
"--max_seq_length",
default=128,
type=int,
help=(
"The maximum total input sequence length after tokenization. Sequences longer "
"than this will be truncated, sequences shorter will be padded."
),
)
parser.add_argument(
"--task",
default="",
type=str,
required=True,
help="The GLUE task to run",
)
parser.add_argument(
"--gpus",
default=0,
type=int,
help="The number of GPUs allocated for this, it is by default 0 meaning none",
)
parser.add_argument(
"--overwrite_cache", action="store_true", help="Overwrite the cached training and evaluation sets"
)
return parser
def main():
parser = argparse.ArgumentParser()
add_generic_args(parser, os.getcwd())
parser = GLUETransformer.add_model_specific_args(parser, os.getcwd())
args = parser.parse_args()
# If output_dir not provided, a folder will be generated in pwd
if args.output_dir is None:
args.output_dir = os.path.join(
"./results",
f"{args.task}_{time.strftime('%Y%m%d_%H%M%S')}",
)
os.makedirs(args.output_dir)
model = GLUETransformer(args)
trainer = generic_train(model, args)
# Optionally, predict on dev set and write to output_dir
if args.do_predict:
checkpoints = sorted(glob.glob(os.path.join(args.output_dir, "checkpoint-epoch=*.ckpt"), recursive=True))
model = model.load_from_checkpoint(checkpoints[-1])
return trainer.test(model)
if __name__ == "__main__":
main()
| transformers/examples/legacy/pytorch-lightning/run_glue.py/0 | {
"file_path": "transformers/examples/legacy/pytorch-lightning/run_glue.py",
"repo_id": "transformers",
"token_count": 3476
} | 280 |
#!/usr/bin/env python
# Copyright 2020 The HuggingFace Team. All rights reserved.
#
# Licensed under the Apache License, Version 2.0 (the "License");
# you may not use this file except in compliance with the License.
# You may obtain a copy of the License at
#
# http://www.apache.org/licenses/LICENSE-2.0
#
# Unless required by applicable law or agreed to in writing, software
# distributed under the License is distributed on an "AS IS" BASIS,
# WITHOUT WARRANTIES OR CONDITIONS OF ANY KIND, either express or implied.
# See the License for the specific language governing permissions and
# limitations under the License.
from typing import Union
import fire
import torch
from tqdm import tqdm
def convert(src_path: str, map_location: str = "cpu", save_path: Union[str, None] = None) -> None:
"""Convert a pytorch_model.bin or model.pt file to torch.float16 for faster downloads, less disk space."""
state_dict = torch.load(src_path, map_location=map_location)
for k, v in tqdm(state_dict.items()):
if not isinstance(v, torch.Tensor):
raise TypeError("FP16 conversion only works on paths that are saved state dicts, like pytorch_model.bin")
state_dict[k] = v.half()
if save_path is None: # overwrite src_path
save_path = src_path
torch.save(state_dict, save_path)
if __name__ == "__main__":
fire.Fire(convert)
| transformers/examples/legacy/seq2seq/convert_model_to_fp16.py/0 | {
"file_path": "transformers/examples/legacy/seq2seq/convert_model_to_fp16.py",
"repo_id": "transformers",
"token_count": 450
} | 281 |
#!/usr/bin/env python
# Copyright 2020 The HuggingFace Team. All rights reserved.
#
# Licensed under the Apache License, Version 2.0 (the "License");
# you may not use this file except in compliance with the License.
# You may obtain a copy of the License at
#
# http://www.apache.org/licenses/LICENSE-2.0
#
# Unless required by applicable law or agreed to in writing, software
# distributed under the License is distributed on an "AS IS" BASIS,
# WITHOUT WARRANTIES OR CONDITIONS OF ANY KIND, either express or implied.
# See the License for the specific language governing permissions and
# limitations under the License.
import argparse
import shutil
import time
from json import JSONDecodeError
from logging import getLogger
from pathlib import Path
from typing import Dict, List
import torch
from torch.utils.data import DataLoader
from tqdm import tqdm
from transformers import AutoModelForSeq2SeqLM, AutoTokenizer
from utils import (
Seq2SeqDataset,
calculate_bleu,
calculate_rouge,
chunks,
lmap,
load_json,
parse_numeric_n_bool_cl_kwargs,
save_json,
use_task_specific_params,
write_txt_file,
)
logger = getLogger(__name__)
def eval_data_dir(
data_dir,
save_dir: str,
model_name: str,
bs: int = 8,
max_source_length: int = 1024,
type_path="val",
n_obs=None,
fp16=False,
task="summarization",
local_rank=None,
num_return_sequences=1,
dataset_kwargs: Dict = None,
prefix="",
**generate_kwargs,
) -> Dict:
"""Run evaluation on part of the data for one gpu and save to {save_dir}/rank_{rank}_output.json"""
model_name = str(model_name)
assert local_rank is not None
torch.distributed.init_process_group(backend="nccl", rank=local_rank)
save_dir = Path(save_dir)
save_path = save_dir.joinpath(f"rank_{local_rank}_output.json")
torch.cuda.set_device(local_rank)
model = AutoModelForSeq2SeqLM.from_pretrained(model_name).cuda()
if fp16:
model = model.half()
# determine if we need to increase num_beams
use_task_specific_params(model, task) # update config with task specific params
num_beams = generate_kwargs.pop("num_beams", model.config.num_beams) # AttributeError risk?
if num_return_sequences > num_beams:
num_beams = num_return_sequences
tokenizer = AutoTokenizer.from_pretrained(model_name)
logger.info(f"Inferred tokenizer type: {tokenizer.__class__}") # if this is wrong, check config.model_type.
if max_source_length is None:
max_source_length = tokenizer.model_max_length
if prefix is None:
prefix = prefix or getattr(model.config, "prefix", "") or ""
ds = Seq2SeqDataset(
tokenizer,
data_dir,
max_source_length,
max_target_length=1024,
type_path=type_path,
n_obs=n_obs,
prefix=prefix,
**dataset_kwargs,
)
# I set shuffle=True for a more accurate progress bar.
# If all the longest samples are first, the prog bar estimate is too high at the beginning.
sampler = ds.make_sortish_sampler(bs, distributed=True, add_extra_examples=False, shuffle=True)
data_loader = DataLoader(ds, sampler=sampler, batch_size=bs, collate_fn=ds.collate_fn)
results = []
for batch in tqdm(data_loader):
summaries = model.generate(
input_ids=batch["input_ids"].to(model.device),
attention_mask=batch["attention_mask"].to(model.device),
num_return_sequences=num_return_sequences,
num_beams=num_beams,
**generate_kwargs,
)
preds = tokenizer.batch_decode(summaries, skip_special_tokens=True, clean_up_tokenization_spaces=False)
ids = batch["ids"]
if num_return_sequences > 1:
preds = chunks(preds, num_return_sequences) # batch size chunks, each of size num_return_seq
for i, pred in enumerate(preds):
results.append({"pred": pred, "id": ids[i].item()})
save_json(results, save_path)
return results, sampler.num_replicas
def run_generate():
parser = argparse.ArgumentParser(
epilog="Unspecified args like --num_beams=2 --decoder_start_token_id=4 are passed to model.generate"
)
parser.add_argument("--data_dir", type=str, help="like cnn_dm/test.source")
parser.add_argument(
"--model_name",
type=str,
help="like facebook/bart-large-cnn,t5-base, etc.",
default="sshleifer/distilbart-xsum-12-3",
)
parser.add_argument("--save_dir", type=str, help="where to save", default="tmp_gen")
parser.add_argument("--max_source_length", type=int, default=None)
parser.add_argument(
"--type_path", type=str, default="test", help="which subset to evaluate typically train/val/test"
)
parser.add_argument("--task", type=str, default="summarization", help="used for task_specific_params + metrics")
parser.add_argument("--bs", type=int, default=8, required=False, help="batch size")
parser.add_argument(
"--local_rank", type=int, default=-1, required=False, help="should be passed by distributed.launch"
)
parser.add_argument(
"--n_obs", type=int, default=None, required=False, help="How many observations. Defaults to all."
)
parser.add_argument(
"--num_return_sequences", type=int, default=1, required=False, help="How many sequences to return"
)
parser.add_argument(
"--sync_timeout",
type=int,
default=600,
required=False,
help="How long should master process wait for other processes to finish.",
)
parser.add_argument("--src_lang", type=str, default=None, required=False)
parser.add_argument("--tgt_lang", type=str, default=None, required=False)
parser.add_argument(
"--prefix", type=str, required=False, default=None, help="will be added to the beginning of src examples"
)
parser.add_argument("--fp16", action="store_true")
parser.add_argument("--debug", action="store_true")
start_time = time.time()
args, rest = parser.parse_known_args()
generate_kwargs = parse_numeric_n_bool_cl_kwargs(rest)
if generate_kwargs and args.local_rank <= 0:
print(f"parsed the following generate kwargs: {generate_kwargs}")
json_save_dir = Path(args.save_dir + "_tmp")
Path(json_save_dir).mkdir(exist_ok=True) # this handles locking.
intermediate_files = list(json_save_dir.glob("rank_*.json"))
if intermediate_files:
raise ValueError(f"Found files at {json_save_dir} please move or remove them.")
# In theory, a node could finish and save before another node hits this. If this happens, we can address later.
dataset_kwargs = {}
if args.src_lang is not None:
dataset_kwargs["src_lang"] = args.src_lang
if args.tgt_lang is not None:
dataset_kwargs["tgt_lang"] = args.tgt_lang
Path(args.save_dir).mkdir(exist_ok=True)
results, num_replicas = eval_data_dir(
args.data_dir,
json_save_dir,
args.model_name,
type_path=args.type_path,
bs=args.bs,
fp16=args.fp16,
task=args.task,
local_rank=args.local_rank,
n_obs=args.n_obs,
max_source_length=args.max_source_length,
num_return_sequences=args.num_return_sequences,
prefix=args.prefix,
dataset_kwargs=dataset_kwargs,
**generate_kwargs,
)
if args.local_rank <= 0:
save_dir = Path(args.save_dir)
save_dir.mkdir(exist_ok=True)
partial_results = gather_results_from_each_node(num_replicas, json_save_dir, args.sync_timeout)
preds = combine_partial_results(partial_results)
if args.num_return_sequences > 1:
save_path = save_dir.joinpath("pseudolabel_results.json")
print(f"Saving aggregated results at {save_path}, intermediate in {json_save_dir}/")
save_json(preds, save_path)
return
tgt_file = Path(args.data_dir).joinpath(args.type_path + ".target")
with open(tgt_file) as f:
labels = [x.rstrip() for x in f.readlines()][: len(preds)]
# Calculate metrics, save metrics, and save _generations.txt
calc_bleu = "translation" in args.task
score_fn = calculate_bleu if calc_bleu else calculate_rouge
metric_name = "bleu" if calc_bleu else "rouge"
metrics: Dict = score_fn(preds, labels)
metrics["n_obs"] = len(preds)
runtime = time.time() - start_time
metrics["seconds_per_sample"] = round(runtime / metrics["n_obs"], 4)
metrics["n_gpus"] = num_replicas
# TODO(@stas00): add whatever metadata to metrics
metrics_save_path = save_dir.joinpath(f"{args.type_path}_{metric_name}.json")
save_json(metrics, metrics_save_path, indent=None)
print(metrics)
write_txt_file(preds, save_dir.joinpath(f"{args.type_path}_generations.txt"))
if args.debug:
write_txt_file(labels, save_dir.joinpath(f"{args.type_path}.target"))
else:
shutil.rmtree(json_save_dir)
def combine_partial_results(partial_results) -> List:
"""Concatenate partial results into one file, then sort it by id."""
records = []
for partial_result in partial_results:
records.extend(partial_result)
records = sorted(records, key=lambda x: x["id"])
preds = [x["pred"] for x in records]
return preds
def gather_results_from_each_node(num_replicas, save_dir, timeout) -> List[Dict[str, List]]:
# WAIT FOR lots of .json files
start_wait = time.time()
logger.info("waiting for all nodes to finish")
json_data = None
while (time.time() - start_wait) < timeout:
json_files = list(save_dir.glob("rank_*.json"))
if len(json_files) < num_replicas:
continue
try:
# make sure all json files are fully saved
json_data = lmap(load_json, json_files)
return json_data
except JSONDecodeError:
continue
else:
raise TimeoutError("Rank 0 gave up on waiting for other processes")
# Unreachable
if __name__ == "__main__":
# Usage for MT:
run_generate()
| transformers/examples/legacy/seq2seq/run_distributed_eval.py/0 | {
"file_path": "transformers/examples/legacy/seq2seq/run_distributed_eval.py",
"repo_id": "transformers",
"token_count": 4159
} | 282 |
<!---
Copyright 2020 The HuggingFace Team. All rights reserved.
Licensed under the Apache License, Version 2.0 (the "License");
you may not use this file except in compliance with the License.
You may obtain a copy of the License at
http://www.apache.org/licenses/LICENSE-2.0
Unless required by applicable law or agreed to in writing, software
distributed under the License is distributed on an "AS IS" BASIS,
WITHOUT WARRANTIES OR CONDITIONS OF ANY KIND, either express or implied.
See the License for the specific language governing permissions and
limitations under the License.
-->
# Examples
This folder contains actively maintained examples of use of ๐ค Transformers using the PyTorch backend, organized by ML task.
## The Big Table of Tasks
Here is the list of all our examples:
- with information on whether they are **built on top of `Trainer`** (if not, they still work, they might
just lack some features),
- whether or not they have a version using the [๐ค Accelerate](https://github.com/huggingface/accelerate) library.
- whether or not they leverage the [๐ค Datasets](https://github.com/huggingface/datasets) library.
- links to **Colab notebooks** to walk through the scripts and run them easily,
<!--
Coming soon!
- links to **Cloud deployments** to be able to deploy large-scale trainings in the Cloud with little to no setup.
-->
| Task | Example datasets | Trainer support | ๐ค Accelerate | ๐ค Datasets | Colab
|---|---|:---:|:---:|:---:|:---:|
| [**`language-modeling`**](https://github.com/huggingface/transformers/tree/main/examples/pytorch/language-modeling) | [WikiText-2](https://huggingface.co/datasets/wikitext) | โ
| โ
| โ
| [](https://colab.research.google.com/github/huggingface/notebooks/blob/main/examples/language_modeling.ipynb)
| [**`multiple-choice`**](https://github.com/huggingface/transformers/tree/main/examples/pytorch/multiple-choice) | [SWAG](https://huggingface.co/datasets/swag) | โ
| โ
| โ
| [](https://colab.research.google.com/github/huggingface/notebooks/blob/main/examples/multiple_choice.ipynb)
| [**`question-answering`**](https://github.com/huggingface/transformers/tree/main/examples/pytorch/question-answering) | [SQuAD](https://huggingface.co/datasets/squad) | โ
| โ
| โ
| [](https://colab.research.google.com/github/huggingface/notebooks/blob/main/examples/question_answering.ipynb)
| [**`summarization`**](https://github.com/huggingface/transformers/tree/main/examples/pytorch/summarization) | [XSum](https://huggingface.co/datasets/xsum) | โ
| โ
| โ
| [](https://colab.research.google.com/github/huggingface/notebooks/blob/main/examples/summarization.ipynb)
| [**`text-classification`**](https://github.com/huggingface/transformers/tree/main/examples/pytorch/text-classification) | [GLUE](https://huggingface.co/datasets/glue) | โ
| โ
| โ
| [](https://colab.research.google.com/github/huggingface/notebooks/blob/main/examples/text_classification.ipynb)
| [**`text-generation`**](https://github.com/huggingface/transformers/tree/main/examples/pytorch/text-generation) | - | n/a | - | - | [](https://colab.research.google.com/github/huggingface/blog/blob/main/notebooks/02_how_to_generate.ipynb)
| [**`token-classification`**](https://github.com/huggingface/transformers/tree/main/examples/pytorch/token-classification) | [CoNLL NER](https://huggingface.co/datasets/conll2003) | โ
|โ
| โ
| [](https://colab.research.google.com/github/huggingface/notebooks/blob/main/examples/token_classification.ipynb)
| [**`translation`**](https://github.com/huggingface/transformers/tree/main/examples/pytorch/translation) | [WMT](https://huggingface.co/datasets/wmt17) | โ
| โ
|โ
| [](https://colab.research.google.com/github/huggingface/notebooks/blob/main/examples/translation.ipynb)
| [**`speech-recognition`**](https://github.com/huggingface/transformers/tree/main/examples/pytorch/speech-recognition) | [TIMIT](https://huggingface.co/datasets/timit_asr) | โ
| - |โ
| [](https://colab.research.google.com/github/huggingface/notebooks/blob/main/examples/speech_recognition.ipynb)
| [**`multi-lingual speech-recognition`**](https://github.com/huggingface/transformers/tree/main/examples/pytorch/speech-recognition) | [Common Voice](https://huggingface.co/datasets/common_voice) | โ
| - |โ
| [](https://colab.research.google.com/github/huggingface/notebooks/blob/main/examples/multi_lingual_speech_recognition.ipynb)
| [**`audio-classification`**](https://github.com/huggingface/transformers/tree/main/examples/pytorch/audio-classification) | [SUPERB KS](https://huggingface.co/datasets/superb) | โ
| - |โ
| [](https://colab.research.google.com/github/huggingface/notebooks/blob/main/examples/audio_classification.ipynb)
| [**`image-pretraining`**](https://github.com/huggingface/transformers/tree/main/examples/pytorch/image-pretraining) | [ImageNet-1k](https://huggingface.co/datasets/imagenet-1k) | โ
| - |โ
| /
| [**`image-classification`**](https://github.com/huggingface/transformers/tree/main/examples/pytorch/image-classification) | [CIFAR-10](https://huggingface.co/datasets/cifar10) | โ
| โ
|โ
| [](https://colab.research.google.com/github/huggingface/notebooks/blob/main/examples/image_classification.ipynb)
| [**`semantic-segmentation`**](https://github.com/huggingface/transformers/tree/main/examples/pytorch/semantic-segmentation) | [SCENE_PARSE_150](https://huggingface.co/datasets/scene_parse_150) | โ
| โ
|โ
| [](https://colab.research.google.com/github/huggingface/notebooks/blob/main/examples/semantic_segmentation.ipynb)
## Running quick tests
Most examples are equipped with a mechanism to truncate the number of dataset samples to the desired length. This is useful for debugging purposes, for example to quickly check that all stages of the programs can complete, before running the same setup on the full dataset which may take hours to complete.
For example here is how to truncate all three splits to just 50 samples each:
```
examples/pytorch/token-classification/run_ner.py \
--max_train_samples 50 \
--max_eval_samples 50 \
--max_predict_samples 50 \
[...]
```
Most example scripts should have the first two command line arguments and some have the third one. You can quickly check if a given example supports any of these by passing a `-h` option, e.g.:
```
examples/pytorch/token-classification/run_ner.py -h
```
## Resuming training
You can resume training from a previous checkpoint like this:
1. Pass `--output_dir previous_output_dir` without `--overwrite_output_dir` to resume training from the latest checkpoint in `output_dir` (what you would use if the training was interrupted, for instance).
2. Pass `--resume_from_checkpoint path_to_a_specific_checkpoint` to resume training from that checkpoint folder.
Should you want to turn an example into a notebook where you'd no longer have access to the command
line, ๐ค Trainer supports resuming from a checkpoint via `trainer.train(resume_from_checkpoint)`.
1. If `resume_from_checkpoint` is `True` it will look for the last checkpoint in the value of `output_dir` passed via `TrainingArguments`.
2. If `resume_from_checkpoint` is a path to a specific checkpoint it will use that saved checkpoint folder to resume the training from.
### Upload the trained/fine-tuned model to the Hub
All the example scripts support automatic upload of your final model to the [Model Hub](https://huggingface.co/models) by adding a `--push_to_hub` argument. It will then create a repository with your username slash the name of the folder you are using as `output_dir`. For instance, `"sgugger/test-mrpc"` if your username is `sgugger` and you are working in the folder `~/tmp/test-mrpc`.
To specify a given repository name, use the `--hub_model_id` argument. You will need to specify the whole repository name (including your username), for instance `--hub_model_id sgugger/finetuned-bert-mrpc`. To upload to an organization you are a member of, just use the name of that organization instead of your username: `--hub_model_id huggingface/finetuned-bert-mrpc`.
A few notes on this integration:
- you will need to be logged in to the Hugging Face website locally for it to work, the easiest way to achieve this is to run `huggingface-cli login` and then type your username and password when prompted. You can also pass along your authentication token with the `--hub_token` argument.
- the `output_dir` you pick will either need to be a new folder or a local clone of the distant repository you are using.
## Distributed training and mixed precision
All the PyTorch scripts mentioned above work out of the box with distributed training and mixed precision, thanks to
the [Trainer API](https://huggingface.co/transformers/main_classes/trainer.html). To launch one of them on _n_ GPUs,
use the following command:
```bash
torchrun \
--nproc_per_node number_of_gpu_you_have path_to_script.py \
--all_arguments_of_the_script
```
As an example, here is how you would fine-tune the BERT large model (with whole word masking) on the text
classification MNLI task using the `run_glue` script, with 8 GPUs:
```bash
torchrun \
--nproc_per_node 8 pytorch/text-classification/run_glue.py \
--model_name_or_path bert-large-uncased-whole-word-masking \
--task_name mnli \
--do_train \
--do_eval \
--max_seq_length 128 \
--per_device_train_batch_size 8 \
--learning_rate 2e-5 \
--num_train_epochs 3.0 \
--output_dir /tmp/mnli_output/
```
If you have a GPU with mixed precision capabilities (architecture Pascal or more recent), you can use mixed precision
training with PyTorch 1.6.0 or latest, or by installing the [Apex](https://github.com/NVIDIA/apex) library for previous
versions. Just add the flag `--fp16` to your command launching one of the scripts mentioned above!
Using mixed precision training usually results in 2x-speedup for training with the same final results (as shown in
[this table](https://github.com/huggingface/transformers/tree/main/examples/text-classification#mixed-precision-training)
for text classification).
## Running on TPUs
When using Tensorflow, TPUs are supported out of the box as a `tf.distribute.Strategy`.
When using PyTorch, we support TPUs thanks to `pytorch/xla`. For more context and information on how to setup your TPU environment refer to Google's documentation and to the
very detailed [pytorch/xla README](https://github.com/pytorch/xla/blob/master/README.md).
In this repo, we provide a very simple launcher script named
[xla_spawn.py](https://github.com/huggingface/transformers/tree/main/examples/pytorch/xla_spawn.py) that lets you run our
example scripts on multiple TPU cores without any boilerplate. Just pass a `--num_cores` flag to this script, then your
regular training script with its arguments (this is similar to the `torch.distributed.launch` helper for
`torch.distributed`):
```bash
python xla_spawn.py --num_cores num_tpu_you_have \
path_to_script.py \
--all_arguments_of_the_script
```
As an example, here is how you would fine-tune the BERT large model (with whole word masking) on the text
classification MNLI task using the `run_glue` script, with 8 TPUs (from this folder):
```bash
python xla_spawn.py --num_cores 8 \
text-classification/run_glue.py \
--model_name_or_path bert-large-uncased-whole-word-masking \
--task_name mnli \
--do_train \
--do_eval \
--max_seq_length 128 \
--per_device_train_batch_size 8 \
--learning_rate 2e-5 \
--num_train_epochs 3.0 \
--output_dir /tmp/mnli_output/
```
## Using Accelerate
Most PyTorch example scripts have a version using the [๐ค Accelerate](https://github.com/huggingface/accelerate) library
that exposes the training loop so it's easy for you to customize or tweak them to your needs. They all require you to
install `accelerate` with the latest development version
```bash
pip install git+https://github.com/huggingface/accelerate
```
Then you can easily launch any of the scripts by running
```bash
accelerate config
```
and reply to the questions asked. Then
```bash
accelerate test
```
that will check everything is ready for training. Finally, you can launch training with
```bash
accelerate launch path_to_script.py --args_to_script
```
## Logging & Experiment tracking
You can easily log and monitor your runs code. The following are currently supported:
* [TensorBoard](https://www.tensorflow.org/tensorboard)
* [Weights & Biases](https://docs.wandb.ai/integrations/huggingface)
* [Comet ML](https://www.comet.ml/docs/python-sdk/huggingface/)
* [Neptune](https://docs.neptune.ai/integrations-and-supported-tools/model-training/hugging-face)
* [ClearML](https://clear.ml/docs/latest/docs/getting_started/ds/ds_first_steps)
* [DVCLive](https://dvc.org/doc/dvclive/ml-frameworks/huggingface)
### Weights & Biases
To use Weights & Biases, install the wandb package with:
```bash
pip install wandb
```
Then log in the command line:
```bash
wandb login
```
If you are in Jupyter or Colab, you should login with:
```python
import wandb
wandb.login()
```
To enable logging to W&B, include `"wandb"` in the `report_to` of your `TrainingArguments` or script. Or just pass along `--report_to_all` if you have `wandb` installed.
Whenever you use the `Trainer` class, your losses, evaluation metrics, model topology and gradients will automatically be logged.
Advanced configuration is possible by setting environment variables:
| Environment Variable | Value |
|---|---|
| WANDB_LOG_MODEL | Log the model as artifact (log the model as artifact at the end of training) (`false` by default) |
| WANDB_WATCH | one of `gradients` (default) to log histograms of gradients, `all` to log histograms of both gradients and parameters, or `false` for no histogram logging |
| WANDB_PROJECT | Organize runs by project |
Set run names with `run_name` argument present in scripts or as part of `TrainingArguments`.
Additional configuration options are available through generic [wandb environment variables](https://docs.wandb.com/library/environment-variables).
Refer to related [documentation & examples](https://docs.wandb.ai/integrations/huggingface).
### Comet.ml
To use `comet_ml`, install the Python package with:
```bash
pip install comet_ml
```
or if in a Conda environment:
```bash
conda install -c comet_ml -c anaconda -c conda-forge comet_ml
```
### Neptune
First, install the Neptune client library. You can do it with either `pip` or `conda`:
`pip`:
```bash
pip install neptune
```
`conda`:
```bash
conda install -c conda-forge neptune
```
Next, in your model training script, import `NeptuneCallback`:
```python
from transformers.integrations import NeptuneCallback
```
To enable Neptune logging, in your `TrainingArguments`, set the `report_to` argument to `"neptune"`:
```python
training_args = TrainingArguments(
"quick-training-distilbert-mrpc",
evaluation_strategy="steps",
eval_steps=20,
report_to="neptune",
)
trainer = Trainer(
model,
training_args,
...
)
```
**Note:** This method requires saving your Neptune credentials as environment variables (see the bottom of the section).
Alternatively, for more logging options, create a Neptune callback:
```python
neptune_callback = NeptuneCallback()
```
To add more detail to the tracked run, you can supply optional arguments to `NeptuneCallback`.
Some examples:
```python
neptune_callback = NeptuneCallback(
name = "DistilBERT",
description = "DistilBERT fine-tuned on GLUE/MRPC",
tags = ["args-callback", "fine-tune", "MRPC"], # tags help you manage runs in Neptune
base_namespace="callback", # the default is "finetuning"
log_checkpoints = "best", # other options are "last", "same", and None
capture_hardware_metrics = False, # additional keyword arguments for a Neptune run
)
```
Pass the callback to the Trainer:
```python
training_args = TrainingArguments(..., report_to=None)
trainer = Trainer(
model,
training_args,
...
callbacks=[neptune_callback],
)
```
Now, when you start the training with `trainer.train()`, your metadata will be logged in Neptune.
**Note:** Although you can pass your **Neptune API token** and **project name** as arguments when creating the callback, the recommended way is to save them as environment variables:
| Environment variable | Value |
| :------------------- | :--------------------------------------------------- |
| `NEPTUNE_API_TOKEN` | Your Neptune API token. To find and copy it, click your Neptune avatar and select **Get your API token**. |
| `NEPTUNE_PROJECT` | The full name of your Neptune project (`workspace-name/project-name`). To find and copy it, head to **project settings** → **Properties**. |
For detailed instructions and examples, see the [Neptune docs](https://docs.neptune.ai/integrations/transformers/).
### ClearML
To use ClearML, install the clearml package with:
```bash
pip install clearml
```
Then [create new credentials]() from the ClearML Server. You can get a free hosted server [here]() or [self-host your own]()!
After creating your new credentials, you can either copy the local snippet which you can paste after running:
```bash
clearml-init
```
Or you can copy the jupyter snippet if you are in Jupyter or Colab:
```python
%env CLEARML_WEB_HOST=https://app.clear.ml
%env CLEARML_API_HOST=https://api.clear.ml
%env CLEARML_FILES_HOST=https://files.clear.ml
%env CLEARML_API_ACCESS_KEY=***
%env CLEARML_API_SECRET_KEY=***
```
To enable logging to ClearML, include `"clearml"` in the `report_to` of your `TrainingArguments` or script. Or just pass along `--report_to all` if you have `clearml` already installed.
Advanced configuration is possible by setting environment variables:
| Environment Variable | Value |
|---|---|
| CLEARML_PROJECT | Name of the project in ClearML. (default: `"HuggingFace Transformers"`) |
| CLEARML_TASK | Name of the task in ClearML. (default: `"Trainer"`) |
Additional configuration options are available through generic [clearml environment variables](https://clear.ml/docs/latest/docs/configs/env_vars).
| transformers/examples/pytorch/README.md/0 | {
"file_path": "transformers/examples/pytorch/README.md",
"repo_id": "transformers",
"token_count": 6242
} | 283 |
#!/usr/bin/env python
# coding=utf-8
# Copyright 2022 The HuggingFace Inc. team. All rights reserved.
#
# Licensed under the Apache License, Version 2.0 (the "License");
# you may not use this file except in compliance with the License.
# You may obtain a copy of the License at
#
# http://www.apache.org/licenses/LICENSE-2.0
#
# Unless required by applicable law or agreed to in writing, software
# distributed under the License is distributed on an "AS IS" BASIS,
# WITHOUT WARRANTIES OR CONDITIONS OF ANY KIND, either express or implied.
# See the License for the specific language governing permissions and
import logging
import os
import sys
import warnings
from dataclasses import dataclass, field
from typing import Optional
import numpy as np
import torch
from datasets import load_dataset
from torchvision.transforms import Compose, Lambda, Normalize, RandomHorizontalFlip, RandomResizedCrop, ToTensor
import transformers
from transformers import (
CONFIG_MAPPING,
IMAGE_PROCESSOR_MAPPING,
MODEL_FOR_MASKED_IMAGE_MODELING_MAPPING,
AutoConfig,
AutoImageProcessor,
AutoModelForMaskedImageModeling,
HfArgumentParser,
Trainer,
TrainingArguments,
)
from transformers.trainer_utils import get_last_checkpoint
from transformers.utils import check_min_version, send_example_telemetry
from transformers.utils.versions import require_version
""" Pre-training a ๐ค Transformers model for simple masked image modeling (SimMIM).
Any model supported by the AutoModelForMaskedImageModeling API can be used.
"""
logger = logging.getLogger(__name__)
# Will error if the minimal version of Transformers is not installed. Remove at your own risks.
check_min_version("4.38.0.dev0")
require_version("datasets>=1.8.0", "To fix: pip install -r examples/pytorch/image-pretraining/requirements.txt")
MODEL_CONFIG_CLASSES = list(MODEL_FOR_MASKED_IMAGE_MODELING_MAPPING.keys())
MODEL_TYPES = tuple(conf.model_type for conf in MODEL_CONFIG_CLASSES)
@dataclass
class DataTrainingArguments:
"""
Arguments pertaining to what data we are going to input our model for training and eval.
Using `HfArgumentParser` we can turn this class into argparse arguments to be able to
specify them on the command line.
"""
dataset_name: Optional[str] = field(
default="cifar10", metadata={"help": "Name of a dataset from the datasets package"}
)
dataset_config_name: Optional[str] = field(
default=None, metadata={"help": "The configuration name of the dataset to use (via the datasets library)."}
)
image_column_name: Optional[str] = field(
default=None,
metadata={"help": "The column name of the images in the files. If not set, will try to use 'image' or 'img'."},
)
train_dir: Optional[str] = field(default=None, metadata={"help": "A folder containing the training data."})
validation_dir: Optional[str] = field(default=None, metadata={"help": "A folder containing the validation data."})
train_val_split: Optional[float] = field(
default=0.15, metadata={"help": "Percent to split off of train for validation."}
)
mask_patch_size: int = field(default=32, metadata={"help": "The size of the square patches to use for masking."})
mask_ratio: float = field(
default=0.6,
metadata={"help": "Percentage of patches to mask."},
)
max_train_samples: Optional[int] = field(
default=None,
metadata={
"help": (
"For debugging purposes or quicker training, truncate the number of training examples to this "
"value if set."
)
},
)
max_eval_samples: Optional[int] = field(
default=None,
metadata={
"help": (
"For debugging purposes or quicker training, truncate the number of evaluation examples to this "
"value if set."
)
},
)
def __post_init__(self):
data_files = {}
if self.train_dir is not None:
data_files["train"] = self.train_dir
if self.validation_dir is not None:
data_files["val"] = self.validation_dir
self.data_files = data_files if data_files else None
@dataclass
class ModelArguments:
"""
Arguments pertaining to which model/config/image processor we are going to pre-train.
"""
model_name_or_path: str = field(
default=None,
metadata={
"help": (
"The model checkpoint for weights initialization. Can be a local path to a pytorch_model.bin or a "
"checkpoint identifier on the hub. "
"Don't set if you want to train a model from scratch."
)
},
)
model_type: Optional[str] = field(
default=None,
metadata={"help": "If training from scratch, pass a model type from the list: " + ", ".join(MODEL_TYPES)},
)
config_name_or_path: Optional[str] = field(
default=None, metadata={"help": "Pretrained config name or path if not the same as model_name"}
)
config_overrides: Optional[str] = field(
default=None,
metadata={
"help": (
"Override some existing default config settings when a model is trained from scratch. Example: "
"n_embd=10,resid_pdrop=0.2,scale_attn_weights=false,summary_type=cls_index"
)
},
)
cache_dir: Optional[str] = field(
default=None,
metadata={"help": "Where do you want to store (cache) the pretrained models/datasets downloaded from the hub"},
)
model_revision: str = field(
default="main",
metadata={"help": "The specific model version to use (can be a branch name, tag name or commit id)."},
)
image_processor_name: str = field(default=None, metadata={"help": "Name or path of preprocessor config."})
token: str = field(
default=None,
metadata={
"help": (
"The token to use as HTTP bearer authorization for remote files. If not specified, will use the token "
"generated when running `huggingface-cli login` (stored in `~/.huggingface`)."
)
},
)
use_auth_token: bool = field(
default=None,
metadata={
"help": "The `use_auth_token` argument is deprecated and will be removed in v4.34. Please use `token` instead."
},
)
trust_remote_code: bool = field(
default=False,
metadata={
"help": (
"Whether or not to allow for custom models defined on the Hub in their own modeling files. This option "
"should only be set to `True` for repositories you trust and in which you have read the code, as it will "
"execute code present on the Hub on your local machine."
)
},
)
image_size: Optional[int] = field(
default=None,
metadata={
"help": (
"The size (resolution) of each image. If not specified, will use `image_size` of the configuration."
)
},
)
patch_size: Optional[int] = field(
default=None,
metadata={
"help": (
"The size (resolution) of each patch. If not specified, will use `patch_size` of the configuration."
)
},
)
encoder_stride: Optional[int] = field(
default=None,
metadata={"help": "Stride to use for the encoder."},
)
class MaskGenerator:
"""
A class to generate boolean masks for the pretraining task.
A mask is a 1D tensor of shape (model_patch_size**2,) where the value is either 0 or 1,
where 1 indicates "masked".
"""
def __init__(self, input_size=192, mask_patch_size=32, model_patch_size=4, mask_ratio=0.6):
self.input_size = input_size
self.mask_patch_size = mask_patch_size
self.model_patch_size = model_patch_size
self.mask_ratio = mask_ratio
if self.input_size % self.mask_patch_size != 0:
raise ValueError("Input size must be divisible by mask patch size")
if self.mask_patch_size % self.model_patch_size != 0:
raise ValueError("Mask patch size must be divisible by model patch size")
self.rand_size = self.input_size // self.mask_patch_size
self.scale = self.mask_patch_size // self.model_patch_size
self.token_count = self.rand_size**2
self.mask_count = int(np.ceil(self.token_count * self.mask_ratio))
def __call__(self):
mask_idx = np.random.permutation(self.token_count)[: self.mask_count]
mask = np.zeros(self.token_count, dtype=int)
mask[mask_idx] = 1
mask = mask.reshape((self.rand_size, self.rand_size))
mask = mask.repeat(self.scale, axis=0).repeat(self.scale, axis=1)
return torch.tensor(mask.flatten())
def collate_fn(examples):
pixel_values = torch.stack([example["pixel_values"] for example in examples])
mask = torch.stack([example["mask"] for example in examples])
return {"pixel_values": pixel_values, "bool_masked_pos": mask}
def main():
# See all possible arguments in src/transformers/training_args.py
# or by passing the --help flag to this script.
# We now keep distinct sets of args, for a cleaner separation of concerns.
parser = HfArgumentParser((ModelArguments, DataTrainingArguments, TrainingArguments))
if len(sys.argv) == 2 and sys.argv[1].endswith(".json"):
# If we pass only one argument to the script and it's the path to a json file,
# let's parse it to get our arguments.
model_args, data_args, training_args = parser.parse_json_file(json_file=os.path.abspath(sys.argv[1]))
else:
model_args, data_args, training_args = parser.parse_args_into_dataclasses()
if model_args.use_auth_token is not None:
warnings.warn(
"The `use_auth_token` argument is deprecated and will be removed in v4.34. Please use `token` instead.",
FutureWarning,
)
if model_args.token is not None:
raise ValueError("`token` and `use_auth_token` are both specified. Please set only the argument `token`.")
model_args.token = model_args.use_auth_token
# Sending telemetry. Tracking the example usage helps us better allocate resources to maintain them. The
# information sent is the one passed as arguments along with your Python/PyTorch versions.
send_example_telemetry("run_mim", model_args, data_args)
# Setup logging
logging.basicConfig(
format="%(asctime)s - %(levelname)s - %(name)s - %(message)s",
datefmt="%m/%d/%Y %H:%M:%S",
handlers=[logging.StreamHandler(sys.stdout)],
)
if training_args.should_log:
# The default of training_args.log_level is passive, so we set log level at info here to have that default.
transformers.utils.logging.set_verbosity_info()
log_level = training_args.get_process_log_level()
logger.setLevel(log_level)
transformers.utils.logging.set_verbosity(log_level)
transformers.utils.logging.enable_default_handler()
transformers.utils.logging.enable_explicit_format()
# Log on each process the small summary:
logger.warning(
f"Process rank: {training_args.local_rank}, device: {training_args.device}, n_gpu: {training_args.n_gpu}, "
+ f"distributed training: {training_args.parallel_mode.value == 'distributed'}, 16-bits training: {training_args.fp16}"
)
logger.info(f"Training/evaluation parameters {training_args}")
# Detecting last checkpoint.
last_checkpoint = None
if os.path.isdir(training_args.output_dir) and training_args.do_train and not training_args.overwrite_output_dir:
last_checkpoint = get_last_checkpoint(training_args.output_dir)
if last_checkpoint is None and len(os.listdir(training_args.output_dir)) > 0:
raise ValueError(
f"Output directory ({training_args.output_dir}) already exists and is not empty. "
"Use --overwrite_output_dir to overcome."
)
elif last_checkpoint is not None and training_args.resume_from_checkpoint is None:
logger.info(
f"Checkpoint detected, resuming training at {last_checkpoint}. To avoid this behavior, change "
"the `--output_dir` or add `--overwrite_output_dir` to train from scratch."
)
# Initialize our dataset.
ds = load_dataset(
data_args.dataset_name,
data_args.dataset_config_name,
data_files=data_args.data_files,
cache_dir=model_args.cache_dir,
token=model_args.token,
)
# If we don't have a validation split, split off a percentage of train as validation.
data_args.train_val_split = None if "validation" in ds.keys() else data_args.train_val_split
if isinstance(data_args.train_val_split, float) and data_args.train_val_split > 0.0:
split = ds["train"].train_test_split(data_args.train_val_split)
ds["train"] = split["train"]
ds["validation"] = split["test"]
# Create config
# Distributed training:
# The .from_pretrained methods guarantee that only one local process can concurrently
# download model & vocab.
config_kwargs = {
"cache_dir": model_args.cache_dir,
"revision": model_args.model_revision,
"token": model_args.token,
"trust_remote_code": model_args.trust_remote_code,
}
if model_args.config_name_or_path:
config = AutoConfig.from_pretrained(model_args.config_name_or_path, **config_kwargs)
elif model_args.model_name_or_path:
config = AutoConfig.from_pretrained(model_args.model_name_or_path, **config_kwargs)
else:
config = CONFIG_MAPPING[model_args.model_type]()
logger.warning("You are instantiating a new config instance from scratch.")
if model_args.config_overrides is not None:
logger.info(f"Overriding config: {model_args.config_overrides}")
config.update_from_string(model_args.config_overrides)
logger.info(f"New config: {config}")
# make sure the decoder_type is "simmim" (only relevant for BEiT)
if hasattr(config, "decoder_type"):
config.decoder_type = "simmim"
# adapt config
model_args.image_size = model_args.image_size if model_args.image_size is not None else config.image_size
model_args.patch_size = model_args.patch_size if model_args.patch_size is not None else config.patch_size
model_args.encoder_stride = (
model_args.encoder_stride if model_args.encoder_stride is not None else config.encoder_stride
)
config.update(
{
"image_size": model_args.image_size,
"patch_size": model_args.patch_size,
"encoder_stride": model_args.encoder_stride,
}
)
# create image processor
if model_args.image_processor_name:
image_processor = AutoImageProcessor.from_pretrained(model_args.image_processor_name, **config_kwargs)
elif model_args.model_name_or_path:
image_processor = AutoImageProcessor.from_pretrained(model_args.model_name_or_path, **config_kwargs)
else:
IMAGE_PROCESSOR_TYPES = {
conf.model_type: image_processor_class for conf, image_processor_class in IMAGE_PROCESSOR_MAPPING.items()
}
image_processor = IMAGE_PROCESSOR_TYPES[model_args.model_type]()
# create model
if model_args.model_name_or_path:
model = AutoModelForMaskedImageModeling.from_pretrained(
model_args.model_name_or_path,
from_tf=bool(".ckpt" in model_args.model_name_or_path),
config=config,
cache_dir=model_args.cache_dir,
revision=model_args.model_revision,
token=model_args.token,
trust_remote_code=model_args.trust_remote_code,
)
else:
logger.info("Training new model from scratch")
model = AutoModelForMaskedImageModeling.from_config(config, trust_remote_code=model_args.trust_remote_code)
if training_args.do_train:
column_names = ds["train"].column_names
else:
column_names = ds["validation"].column_names
if data_args.image_column_name is not None:
image_column_name = data_args.image_column_name
elif "image" in column_names:
image_column_name = "image"
elif "img" in column_names:
image_column_name = "img"
else:
image_column_name = column_names[0]
# transformations as done in original SimMIM paper
# source: https://github.com/microsoft/SimMIM/blob/main/data/data_simmim.py
transforms = Compose(
[
Lambda(lambda img: img.convert("RGB") if img.mode != "RGB" else img),
RandomResizedCrop(model_args.image_size, scale=(0.67, 1.0), ratio=(3.0 / 4.0, 4.0 / 3.0)),
RandomHorizontalFlip(),
ToTensor(),
Normalize(mean=image_processor.image_mean, std=image_processor.image_std),
]
)
# create mask generator
mask_generator = MaskGenerator(
input_size=model_args.image_size,
mask_patch_size=data_args.mask_patch_size,
model_patch_size=model_args.patch_size,
mask_ratio=data_args.mask_ratio,
)
def preprocess_images(examples):
"""Preprocess a batch of images by applying transforms + creating a corresponding mask, indicating
which patches to mask."""
examples["pixel_values"] = [transforms(image) for image in examples[image_column_name]]
examples["mask"] = [mask_generator() for i in range(len(examples[image_column_name]))]
return examples
if training_args.do_train:
if "train" not in ds:
raise ValueError("--do_train requires a train dataset")
if data_args.max_train_samples is not None:
ds["train"] = ds["train"].shuffle(seed=training_args.seed).select(range(data_args.max_train_samples))
# Set the training transforms
ds["train"].set_transform(preprocess_images)
if training_args.do_eval:
if "validation" not in ds:
raise ValueError("--do_eval requires a validation dataset")
if data_args.max_eval_samples is not None:
ds["validation"] = (
ds["validation"].shuffle(seed=training_args.seed).select(range(data_args.max_eval_samples))
)
# Set the validation transforms
ds["validation"].set_transform(preprocess_images)
# Initialize our trainer
trainer = Trainer(
model=model,
args=training_args,
train_dataset=ds["train"] if training_args.do_train else None,
eval_dataset=ds["validation"] if training_args.do_eval else None,
tokenizer=image_processor,
data_collator=collate_fn,
)
# Training
if training_args.do_train:
checkpoint = None
if training_args.resume_from_checkpoint is not None:
checkpoint = training_args.resume_from_checkpoint
elif last_checkpoint is not None:
checkpoint = last_checkpoint
train_result = trainer.train(resume_from_checkpoint=checkpoint)
trainer.save_model()
trainer.log_metrics("train", train_result.metrics)
trainer.save_metrics("train", train_result.metrics)
trainer.save_state()
# Evaluation
if training_args.do_eval:
metrics = trainer.evaluate()
trainer.log_metrics("eval", metrics)
trainer.save_metrics("eval", metrics)
# Write model card and (optionally) push to hub
kwargs = {
"finetuned_from": model_args.model_name_or_path,
"tasks": "masked-image-modeling",
"dataset": data_args.dataset_name,
"tags": ["masked-image-modeling"],
}
if training_args.push_to_hub:
trainer.push_to_hub(**kwargs)
else:
trainer.create_model_card(**kwargs)
if __name__ == "__main__":
main()
| transformers/examples/pytorch/image-pretraining/run_mim.py/0 | {
"file_path": "transformers/examples/pytorch/image-pretraining/run_mim.py",
"repo_id": "transformers",
"token_count": 8028
} | 284 |
<!---
Copyright 2021 The HuggingFace Team. All rights reserved.
Licensed under the Apache License, Version 2.0 (the "License");
you may not use this file except in compliance with the License.
You may obtain a copy of the License at
http://www.apache.org/licenses/LICENSE-2.0
Unless required by applicable law or agreed to in writing, software
distributed under the License is distributed on an "AS IS" BASIS,
WITHOUT WARRANTIES OR CONDITIONS OF ANY KIND, either express or implied.
See the License for the specific language governing permissions and
limitations under the License.
-->
# Automatic Speech Recognition Examples
## Table of Contents
- [Automatic Speech Recognition with CTC](#connectionist-temporal-classification)
- [Single GPU example](#single-gpu-ctc)
- [Multi GPU example](#multi-gpu-ctc)
- [Examples](#examples-ctc)
- [TIMIT](#timit-ctc)
- [Librispeech](#librispeech-ctc)
- [Common Voice](#common-voice-ctc)
- [Multilingual Librispeech](#multilingual-librispeech-ctc)
- [Automatic Speech Recognition with CTC and Adapter Layers](#connectionist-temporal-classification-with-adapters)
- [Massive Multilingual Speech (MMS)](#mms-model)
- [Examples](#examples-ctc-adapter)
- [Common Voice](#common-voice-ctc-adapter)
- [Automatic Speech Recognition with Sequence-to-Sequence](#sequence-to-sequence)
- [Whisper Model](#whisper-model)
- [Speech-Encoder-Decoder Model](#warm-started-speech-encoder-decoder-model)
- [Examples](#examples-seq2seq)
- [Librispeech](#librispeech-seq2seq)
## Connectionist Temporal Classification
The script [`run_speech_recognition_ctc.py`](https://github.com/huggingface/transformers/blob/main/examples/pytorch/speech-recognition/run_speech_recognition_ctc.py) can be used to fine-tune any pretrained [Connectionist Temporal Classification Model](https://huggingface.co/docs/transformers/main/en/model_doc/auto#transformers.AutoModelForCTC) for automatic speech
recognition on one of the [official speech recognition datasets](https://huggingface.co/datasets?task_ids=task_ids:automatic-speech-recognition) or a custom dataset.
Speech recognition models that have been pretrained in unsupervised fashion on audio data alone, *e.g.* [Wav2Vec2](https://huggingface.co/transformers/main/model_doc/wav2vec2.html), [HuBERT](https://huggingface.co/transformers/main/model_doc/hubert.html), [XLSR-Wav2Vec2](https://huggingface.co/transformers/main/model_doc/xlsr_wav2vec2.html), have shown to require only
very little annotated data to yield good performance on automatic speech recognition datasets.
In the script [`run_speech_recognition_ctc`], we first create a vocabulary from all unique characters of both the training data and evaluation data. Then, we preprocesses the speech recognition dataset, which includes correct resampling, normalization and padding. Finally, the pretrained speech recognition model is fine-tuned on the annotated speech recognition datasets using CTC loss.
---
**NOTE**
If you encounter problems with data preprocessing by setting `--preprocessing_num_workers` > 1,
you might want to set the environment variable `OMP_NUM_THREADS` to 1 as follows:
```bash
OMP_NUM_THREADS=1 python run_speech_recognition_ctc ...
```
If the environment variable is not set, the training script might freeze, *i.e.* see: https://github.com/pytorch/audio/issues/1021#issuecomment-726915239
---
### Single GPU CTC
The following command shows how to fine-tune [XLSR-Wav2Vec2](https://huggingface.co/transformers/main/model_doc/xlsr_wav2vec2.html) on [Common Voice](https://huggingface.co/datasets/common_voice) using a single GPU in half-precision.
```bash
python run_speech_recognition_ctc.py \
--dataset_name="common_voice" \
--model_name_or_path="facebook/wav2vec2-large-xlsr-53" \
--dataset_config_name="tr" \
--output_dir="./wav2vec2-common_voice-tr-demo" \
--overwrite_output_dir \
--num_train_epochs="15" \
--per_device_train_batch_size="16" \
--gradient_accumulation_steps="2" \
--learning_rate="3e-4" \
--warmup_steps="500" \
--evaluation_strategy="steps" \
--text_column_name="sentence" \
--length_column_name="input_length" \
--save_steps="400" \
--eval_steps="100" \
--layerdrop="0.0" \
--save_total_limit="3" \
--freeze_feature_encoder \
--gradient_checkpointing \
--chars_to_ignore , ? . ! - \; \: \" โ % โ โ ๏ฟฝ \
--fp16 \
--group_by_length \
--push_to_hub \
--do_train --do_eval
```
On a single V100 GPU, this script should run in *ca.* 1 hour 20 minutes and yield a CTC loss of **0.39** and word error rate
of **0.35**.
### Multi GPU CTC
The following command shows how to fine-tune [XLSR-Wav2Vec2](https://huggingface.co/transformers/main/model_doc/xlsr_wav2vec2.html) on [Common Voice](https://huggingface.co/datasets/common_voice) using 8 GPUs in half-precision.
```bash
torchrun \
--nproc_per_node 8 run_speech_recognition_ctc.py \
--dataset_name="common_voice" \
--model_name_or_path="facebook/wav2vec2-large-xlsr-53" \
--dataset_config_name="tr" \
--output_dir="./wav2vec2-common_voice-tr-demo-dist" \
--overwrite_output_dir \
--num_train_epochs="15" \
--per_device_train_batch_size="4" \
--learning_rate="3e-4" \
--warmup_steps="500" \
--evaluation_strategy="steps" \
--text_column_name="sentence" \
--length_column_name="input_length" \
--save_steps="400" \
--eval_steps="100" \
--logging_steps="1" \
--layerdrop="0.0" \
--save_total_limit="3" \
--freeze_feature_encoder \
--gradient_checkpointing \
--chars_to_ignore , ? . ! - \; \: \" โ % โ โ ๏ฟฝ \
--fp16 \
--group_by_length \
--push_to_hub \
--do_train --do_eval
```
On 8 V100 GPUs, this script should run in *ca.* 18 minutes and yield a CTC loss of **0.39** and word error rate
of **0.36**.
### Multi GPU CTC with Dataset Streaming
The following command shows how to use [Dataset Streaming mode](https://huggingface.co/docs/datasets/dataset_streaming)
to fine-tune [XLS-R](https://huggingface.co/transformers/main/model_doc/xls_r.html)
on [Common Voice](https://huggingface.co/datasets/common_voice) using 4 GPUs in half-precision.
Streaming mode imposes several constraints on training:
1. We need to construct a tokenizer beforehand and define it via `--tokenizer_name_or_path`.
2. `--num_train_epochs` has to be replaced by `--max_steps`. Similarly, all other epoch-based arguments have to be
replaced by step-based ones.
3. Full dataset shuffling on each epoch is not possible, since we don't have the whole dataset available at once.
However, the `--shuffle_buffer_size` argument controls how many examples we can pre-download before shuffling them.
```bash
**torchrun \
--nproc_per_node 4 run_speech_recognition_ctc_streaming.py \
--dataset_name="common_voice" \
--model_name_or_path="facebook/wav2vec2-xls-r-300m" \
--tokenizer_name_or_path="anton-l/wav2vec2-tokenizer-turkish" \
--dataset_config_name="tr" \
--train_split_name="train+validation" \
--eval_split_name="test" \
--output_dir="wav2vec2-xls-r-common_voice-tr-ft" \
--overwrite_output_dir \
--max_steps="5000" \
--per_device_train_batch_size="8" \
--gradient_accumulation_steps="2" \
--learning_rate="5e-4" \
--warmup_steps="500" \
--evaluation_strategy="steps" \
--text_column_name="sentence" \
--save_steps="500" \
--eval_steps="500" \
--logging_steps="1" \
--layerdrop="0.0" \
--eval_metrics wer cer \
--save_total_limit="1" \
--mask_time_prob="0.3" \
--mask_time_length="10" \
--mask_feature_prob="0.1" \
--mask_feature_length="64" \
--freeze_feature_encoder \
--chars_to_ignore , ? . ! - \; \: \" โ % โ โ ๏ฟฝ \
--max_duration_in_seconds="20" \
--shuffle_buffer_size="500" \
--fp16 \
--push_to_hub \
--do_train --do_eval \
--gradient_checkpointing**
```
On 4 V100 GPUs, this script should run in *ca.* 3h 31min and yield a CTC loss of **0.35** and word error rate
of **0.29**.
### Examples CTC
The following tables present a couple of example runs on the most popular speech-recognition datasets.
The presented performances are by no means optimal as no hyper-parameter tuning was done. Nevertheless,
they can serve as a baseline to improve upon.
#### TIMIT CTC
- [TIMIT](https://huggingface.co/datasets/timit_asr)
| Dataset | Dataset Config | Pretrained Model | Word error rate on eval | Phoneme error rate on eval | GPU setup | Training time | Fine-tuned Model & Logs | Command to reproduce |
|-------|------------------------------|-------------|---------------|---------------|----------------------|-------------| -------------| ------- |
| [TIMIT](https://huggingface.co/datasets/timit_asr)| - | [wav2vec2-base](https://huggingface.co/facebook/wav2vec2-base) | 0.21 | - | 1 GPU TITAN RTX | 32min | [here](https://huggingface.co/patrickvonplaten/wav2vec2-base-timit-fine-tuned) | [run.sh](https://huggingface.co/patrickvonplaten/wav2vec2-base-timit-fine-tuned/blob/main/run.sh) |
| [TIMIT](https://huggingface.co/datasets/timit_asr)| - | [wav2vec2-base](https://huggingface.co/facebook/wav2vec2-base) | 0.21 | - | 1 GPU TITAN RTX | 32min | [here](https://huggingface.co/patrickvonplaten/wav2vec2-base-timit-fine-tuned) | [run.sh](https://huggingface.co/patrickvonplaten/wav2vec2-base-timit-fine-tuned/blob/main/run.sh) |
| [TIMIT](https://huggingface.co/datasets/timit_asr)| - | [unispeech-large-1500h-cv](https://huggingface.co/microsoft/unispeech-large-1500h-cv) | 0.22 | - | 1 GPU TITAN RTX | 35min | [here](https://huggingface.co/patrickvonplaten/unispeech-large-1500h-cv-timit) | [run.sh](https://huggingface.co/patrickvonplaten/unispeech-large-1500h-cv-timit/blob/main/run.sh) |
| [TIMIT](https://huggingface.co/datasets/timit_asr)| - | [asapp/sew-mid-100k](https://huggingface.co/asapp/sew-mid-100k) | 0.30 | - | 1 GPU TITAN RTX | 28min | [here](https://huggingface.co/patrickvonplaten/sew-small-100k-timit) | [run.sh](https://huggingface.co/patrickvonplaten/sew-small-100k-timit/blob/main/run.sh) |
| [TIMIT](https://huggingface.co/datasets/timit_asr)| - | [ntu-spml/distilhubert](https://huggingface.co/ntu-spml/distilhubert) | 0.68 | - | 1 GPU TITAN RTX | 26min | [here](https://huggingface.co/patrickvonplaten/distilhubert-timit) | [run.sh](https://huggingface.co/patrickvonplaten/distilhubert-timit/blob/main/run.sh) |
#### Librispeech CTC
- [Librispeech](https://huggingface.co/datasets/librispeech_asr)
| Dataset | Dataset Config | Pretrained Model | Word error rate on eval | Phoneme error rate on eval | GPU setup | Training time | Fine-tuned Model & Logs | Command to reproduce |
|-------|------------------------------|-------------|---------------|---------------|----------------------|-------------| -------------| ------- |
| [Librispeech](https://huggingface.co/datasets/librispeech_asr)| `"clean"` - `"train.100"` | [microsoft/wavlm-large](https://huggingface.co/microsoft/wavlm-large) | 0.049 | - | 8 GPU V100 | 1h30min | [here](https://huggingface.co/patrickvonplaten/wavlm-libri-clean-100h-large) | [run.sh](https://huggingface.co/patrickvonplaten/wavlm-libri-clean-100h-large/blob/main/run.sh) |
| [Librispeech](https://huggingface.co/datasets/librispeech_asr)| `"clean"` - `"train.100"` | [microsoft/wavlm-base-plus](https://huggingface.co/microsoft/wavlm-base-plus) | 0.068 | - | 8 GPU V100 | 1h30min | [here](https://huggingface.co/patrickvonplaten/wavlm-libri-clean-100h-base-plus) | [run.sh](https://huggingface.co/patrickvonplaten/wavlm-libri-clean-100h-base-plus/blob/main/run.sh) |
| [Librispeech](https://huggingface.co/datasets/librispeech_asr)| `"clean"` - `"train.100"` | [facebook/wav2vec2-large-lv60](https://huggingface.co/facebook/wav2vec2-large-lv60) | 0.042 | - | 8 GPU V100 | 1h30min | [here](https://huggingface.co/patrickvonplaten/wav2vec2-librispeech-clean-100h-demo-dist) | [run.sh](https://huggingface.co/patrickvonplaten/wav2vec2-librispeech-clean-100h-demo-dist/blob/main/run.sh) |
| [Librispeech](https://huggingface.co/datasets/librispeech_asr)| `"clean"` - `"train.100"` | [facebook/wav2vec2-large-lv60](https://huggingface.co/facebook/wav2vec2-large-lv60) | 0.042 | - | 8 GPU V100 | 1h30min | [here](https://huggingface.co/patrickvonplaten/wav2vec2-librispeech-clean-100h-demo-dist) | [run.sh](https://huggingface.co/patrickvonplaten/wav2vec2-librispeech-clean-100h-demo-dist/blob/main/run.sh) |
| [Librispeech](https://huggingface.co/datasets/librispeech_asr)| `"clean"` - `"train.100"` | [facebook/hubert-large-ll60k](https://huggingface.co/facebook/hubert-large-ll60k) | 0.088 | - | 8 GPU V100 | 1h30min | [here](https://huggingface.co/patrickvonplaten/hubert-librispeech-clean-100h-demo-dist) | [run.sh](https://huggingface.co/patrickvonplaten/hubert-librispeech-clean-100h-demo-dist/blob/main/run.sh) |
| [Librispeech](https://huggingface.co/datasets/librispeech_asr)| `"clean"` - `"train.100"` | [asapp/sew-mid-100k](https://huggingface.co/asapp/sew-mid-100k) | 0.167 | | 8 GPU V100 | 54min | [here](https://huggingface.co/patrickvonplaten/sew-mid-100k-librispeech-clean-100h-ft) | [run.sh](https://huggingface.co/patrickvonplaten/sew-mid-100k-librispeech-clean-100h-ft/blob/main/run.sh) |
#### Common Voice CTC
- [Common Voice](https://huggingface.co/datasets/common_voice)
| Dataset | Dataset Config | Pretrained Model | Word error rate on eval | Phoneme error rate on eval | GPU setup | Training time | Fine-tuned Model & Logs | Command to reproduce |
|-------|------------------------------|-------------|---------------|---------------|----------------------|-------------| -------------| ------- |
| [Common Voice](https://huggingface.co/datasets/mozilla-foundation/common_voice_3_0)| `"tr"` | [facebook/wav2vec2-large-xls-r-300m](https://huggingface.co/facebook/wav2vec2-xls-r-300m) | - | 0.099 | 8 GPU V100 | 23min | [here](https://huggingface.co/patrickvonplaten/xls-r-300m-tr-phoneme) | [run.sh](https://huggingface.co/patrickvonplaten/xls-r-300m-tr-phoneme/blob/main/run.sh) |
| [Common Voice](https://huggingface.co/datasets/mozilla-foundation/common_voice_3_0)| `"it"` | [facebook/wav2vec2-large-xls-r-300m](https://huggingface.co/facebook/wav2vec2-xls-r-300m) | - | 0.077 | 8 GPU V100 | 23min | [here](https://huggingface.co/patrickvonplaten/xls-r-300m-it-phoneme) | [run.sh](https://huggingface.co/patrickvonplaten/xls-r-300m-it-phoneme/blob/main/run.sh) |
| [Common Voice](https://huggingface.co/datasets/mozilla-foundation/common_voice_3_0)| `"sv-SE"` | [facebook/wav2vec2-large-xls-r-300m](https://huggingface.co/facebook/wav2vec2-xls-r-300m) | - | 0.099 | 8 GPU V100 | 23min | [here](https://huggingface.co/patrickvonplaten/xls-r-300m-sv-phoneme) | [run.sh](https://huggingface.co/patrickvonplaten/xls-r-300m-sv-phoneme/blob/main/run.sh) |
| [Common Voice](https://huggingface.co/datasets/common_voice)| `"tr"` | [facebook/wav2vec2-large-xlsr-53](https://huggingface.co/facebook/wav2vec2-large-xlsr-53) | 0.36 | - | 8 GPU V100 | 18min | [here](https://huggingface.co/patrickvonplaten/wav2vec2-common_voice-tr-demo-dist) | [run.sh](https://huggingface.co/patrickvonplaten/wav2vec2-common_voice-tr-demo-dist/blob/main/run_dist.sh) |
| [Common Voice](https://huggingface.co/datasets/common_voice)| `"tr"` | [facebook/wav2vec2-large-xlsr-53](https://huggingface.co/facebook/wav2vec2-large-xlsr-53) | 0.31 | - | 8 GPU V100 | 1h05 | [here](https://huggingface.co/patrickvonplaten/wav2vec2-large-xlsr-53-common_voice-tr-ft) | [run.sh](https://huggingface.co/patrickvonplaten/wav2vec2-large-xlsr-53-common_voice-tr-ft/blob/main/run.sh) |
| [Common Voice](https://huggingface.co/datasets/common_voice)| `"tr"` | [facebook/wav2vec2-large-xlsr-53](https://huggingface.co/facebook/wav2vec2-large-xlsr-53) | 0.35 | - | 1 GPU V100 | 1h20min | [here](https://huggingface.co/patrickvonplaten/wav2vec2-common_voice-tr-demo) | [run.sh](https://huggingface.co/patrickvonplaten/wav2vec2-common_voice-tr-demo/blob/main/run.sh) |
| [Common Voice](https://huggingface.co/datasets/common_voice)| `"tr"` | [facebook/wav2vec2-xls-r-300m](https://huggingface.co/facebook/wav2vec2-xls-r-300m) | 0.31 | - | 8 GPU V100 | 1h05 | [here](https://huggingface.co/patrickvonplaten/wav2vec2-large-xls-r-300m-common_voice-tr-ft) | [run.sh](https://huggingface.co/patrickvonplaten/wav2vec2-large-xls-r-300m-common_voice-tr-ft/blob/main/run.sh) |
| [Common Voice](https://huggingface.co/datasets/common_voice)| `"tr"` | [facebook/wav2vec2-xls-r-1b](https://huggingface.co/facebook/wav2vec2-xls-r-1b) | 0.21 | - | 2 GPU Titan 24 GB RAM | 15h10 | [here](https://huggingface.co/patrickvonplaten/wav2vec2-xls-r-1b-common_voice-tr-ft) | [run.sh](https://huggingface.co/patrickvonplaten/wav2vec2-large-xls-r-1b-common_voice-tr-ft/blob/main/run.sh) |
| [Common Voice](https://huggingface.co/datasets/common_voice)| `"tr"` in streaming mode | [facebook/wav2vec2-xls-r-300m](https://huggingface.co/facebook/wav2vec2-xls-r-300m) | 0.29 | - | 4 GPU V100 | 3h31 | [here](https://huggingface.co/anton-l/wav2vec2-xls-r-common_voice-tr-ft-stream) | [run.sh](https://huggingface.co/anton-l/wav2vec2-xls-r-common_voice-tr-ft-stream/blob/main/run.sh) |
#### Multilingual Librispeech CTC
- [Multilingual Librispeech](https://huggingface.co/datasets/multilingual_librispeech)
| Dataset | Dataset Config | Pretrained Model | Word error rate on eval | Phoneme error rate on eval | GPU setup | Training time | Fine-tuned Model & Logs | Command to reproduce |
|-------|------------------------------|-------------|---------------|---------------|----------------------|-------------| -------------| ------- |
| [Multilingual Librispeech](https://huggingface.co/datasets/multilingual_librispeech)| `"german"` | [facebook/wav2vec2-large-xlsr-53](https://huggingface.co/facebook/wav2vec2-large-xlsr-53) | 0.13 | - | 1 GPU Titan 24 GB RAM | 15h04 | [here](https://huggingface.co/patrickvonplaten/wav2vec2-xlsr-53-300m-mls-german-ft) | [run.sh](https://huggingface.co/patrickvonplaten/wav2vec2-xlsr-53-300m-mls-german-ft/blob/main/run.sh) |
| [Multilingual Librispeech](https://huggingface.co/datasets/multilingual_librispeech)| `"german"` | [facebook/wav2vec2-xls-r-300m](https://huggingface.co/facebook/wav2vec2-xls-r-300m) | 0.15 | - | 1 GPU Titan 24 GB RAM | 15h04 | [here](https://huggingface.co/patrickvonplaten/wav2vec2-300m-mls-german-ft) | [run.sh](https://huggingface.co/patrickvonplaten/wav2vec2-300m-mls-german-ft/blob/main/run.sh) |
## Connectionist Temporal Classification With Adapters
The script [`run_speech_recognition_ctc_adapter.py`](https://github.com/huggingface/transformers/blob/main/examples/pytorch/speech-recognition/run_speech_recognition_ctc_adapter.py) can be used to fine-tune adapter layers for [Wav2Vec2-like models like MMS](https://huggingface.co/docs/transformers/main/en/model_doc/mms) for automatic speech recognition.
### MMS Model
The [Massive Multilingual Speech (MMS) model](https://huggingface.co/facebook/mms-1b-all) has been pre-trained and fine-tuned
on 1000+ languages. The model makes use of adapter attention layers to fine-tune only a small part
of the model on a specific language. The model already comes with fine-tuned adapter layers for 1000+ languages and
can be used for inference for 1000+ languages out of the box.
However, for improved performance or more specific use cases one can re-initialize the adapter weights, freeze all
other weights and fine-tune them on a specific dataset as shown in the [example below](#examples-ctc-adapter).
Note that the adapter weights include low dimensional linear layers for every attention block as well as the final language
model head layers.
### Examples CTC Adapter
In the following we will look at how one can fine-tune adapter weights for any of the
[MMS CTC checkpoints](https://huggingface.co/models?pipeline_tag=automatic-speech-recognition&other=mms&sort=downloads) in less than 1 hour.
#### Common Voice CTC Adapter
As in the examples [above](#examples-ctc), we fine-tune on Common Voice's 6 dataset in Turkish as an example.
Contrary to [`run_speech_recognition_ctc.py`](https://github.com/huggingface/transformers/blob/main/examples/pytorch/speech-recognition/run_speech_recognition_ctc.py) before there is a `--target_language` which has to be defined to state for which
language or concept the adapter layers shall be trained. The adapter weights will then
accordingly be called `adapter.{<target_language}.safetensors`.
Let's run an example script. Make sure to be logged in so that your model can be directly uploaded to the Hub.
```
huggingface-cli login
```
Now, let's run an example and upload it to the Hub under `wav2vec2-common_voice-tr-mms-demo`.
```sh
python run_speech_recognition_ctc.py \
--dataset_name="common_voice" \
--model_name_or_path="facebook/mms-1b-all" \
--dataset_config_name="tr" \
--output_dir="./wav2vec2-common_voice-tr-mms-demo" \
--num_train_epochs="4" \
--per_device_train_batch_size="32" \
--learning_rate="1e-3" \
--warmup_steps="100" \
--evaluation_strategy="steps" \
--text_column_name="sentence" \
--length_column_name="input_length" \
--save_steps="200" \
--eval_steps="100" \
--save_total_limit="3" \
--target_language="tur" \
--gradient_checkpointing \
--chars_to_ignore , ? . ! - \; \: \" โ % โ โ ๏ฟฝ \
--fp16 \
--group_by_length \
--do_train --do_eval \
--push_to_hub
```
This should take less than 10 minutes on most GPUs and you should very quickly get word error rates
below 27%.
For an example run, you can have a look at [`patrickvonplaten/wav2vec2-common_voice-tr-mms-demo`](https://huggingface.co/patrickvonplaten/wav2vec2-common_voice-tr-mms-demo).
If you'd like to train another adapter model with the same base model, you can simply re-use the same `--output_dir`,
but make sure to pass the `--output_dir` folder also to `--tokenizer_name_or_path` so that the vocabulary is not
overwritten but **extended**. Assuming you would like to train adapter weights on Swedish in addition to Turkish and save
the adapter weights in the same model repo, you can run:
```sh
python run_speech_recognition_ctc.py \
--dataset_name="common_voice" \
--model_name_or_path="facebook/mms-1b-all" \
--dataset_config_name="sw" \
--output_dir="./wav2vec2-common_voice-tr-mms-demo" \
--tokenizer_name_or_path="./wav2vec2-common_voice-tr-mms-demo" \
--num_train_epochs="4" \
--per_device_train_batch_size="32" \
--learning_rate="1e-3" \
--warmup_steps="100" \
--evaluation_strategy="steps" \
--text_column_name="sentence" \
--length_column_name="input_length" \
--save_steps="200" \
--eval_steps="100" \
--save_total_limit="3" \
--target_language="swe" \
--gradient_checkpointing \
--chars_to_ignore , ? . ! - \; \: \" โ % โ โ ๏ฟฝ \
--fp16 \
--group_by_length \
--do_train --do_eval \
--push_to_hub
```
Now you should have both `adapter.tur.safetensors` and `adapter.swe.safetensors` in the model repo
and you can load the respective language with:
```py
model.load_adapter("tur") # or "swe"
```
respectively.
## Sequence to Sequence
The script [`run_speech_recognition_seq2seq.py`](https://github.com/huggingface/transformers/blob/main/examples/pytorch/speech-recognition/run_speech_recognition_seq2seq.py) can be used to fine-tune any [Speech Sequence-to-Sequence Model](https://huggingface.co/docs/transformers/main/en/model_doc/auto#transformers.AutoModelForSpeechSeq2Seq) for automatic speech
recognition on one of the [official speech recognition datasets](https://huggingface.co/datasets?task_ids=task_ids:automatic-speech-recognition) or a custom dataset. This includes the Whisper model from OpenAI or a warm-started Speech-Encoder-Decoder Model, examples for which are included below.
### Whisper Model
We can load all components of the Whisper model directly from the pretrained checkpoint, including the pretrained model weights, feature extractor and tokenizer. We simply have to specify our fine-tuning dataset and training hyperparameters.
#### Single GPU Whisper Training
The following example shows how to fine-tune the [Whisper small](https://huggingface.co/openai/whisper-small) checkpoint on the Hindi subset of [Common Voice 11](https://huggingface.co/datasets/mozilla-foundation/common_voice_11_0) using a single GPU device in half-precision:
```bash
python run_speech_recognition_seq2seq.py \
--model_name_or_path="openai/whisper-small" \
--dataset_name="mozilla-foundation/common_voice_11_0" \
--dataset_config_name="hi" \
--language="hindi" \
--train_split_name="train+validation" \
--eval_split_name="test" \
--max_steps="5000" \
--output_dir="./whisper-small-hi" \
--per_device_train_batch_size="16" \
--gradient_accumulation_steps="2" \
--per_device_eval_batch_size="16" \
--logging_steps="25" \
--learning_rate="1e-5" \
--warmup_steps="500" \
--evaluation_strategy="steps" \
--eval_steps="1000" \
--save_strategy="steps" \
--save_steps="1000" \
--generation_max_length="225" \
--preprocessing_num_workers="16" \
--length_column_name="input_length" \
--max_duration_in_seconds="30" \
--text_column_name="sentence" \
--freeze_feature_encoder="False" \
--gradient_checkpointing \
--group_by_length \
--fp16 \
--overwrite_output_dir \
--do_train \
--do_eval \
--predict_with_generate \
--use_auth_token
```
On a single V100, training should take approximately 8 hours, with a final cross-entropy loss of **1e-4** and word error rate of **32.6%**.
If training on a different language, you should be sure to change the `language` argument. The `language` argument should be omitted for English speech recognition.
#### Multi GPU Whisper Training
The following example shows how to fine-tune the [Whisper small](https://huggingface.co/openai/whisper-small) checkpoint on the Hindi subset of [Common Voice 11](https://huggingface.co/datasets/mozilla-foundation/common_voice_11_0) using 2 GPU devices in half-precision:
```bash
torchrun \
--nproc_per_node 2 run_speech_recognition_seq2seq.py \
--model_name_or_path="openai/whisper-small" \
--dataset_name="mozilla-foundation/common_voice_11_0" \
--dataset_config_name="hi" \
--language="hindi" \
--train_split_name="train+validation" \
--eval_split_name="test" \
--max_steps="5000" \
--output_dir="./whisper-small-hi" \
--per_device_train_batch_size="16" \
--per_device_eval_batch_size="16" \
--logging_steps="25" \
--learning_rate="1e-5" \
--warmup_steps="500" \
--evaluation_strategy="steps" \
--eval_steps="1000" \
--save_strategy="steps" \
--save_steps="1000" \
--generation_max_length="225" \
--preprocessing_num_workers="16" \
--length_column_name="input_length" \
--max_duration_in_seconds="30" \
--text_column_name="sentence" \
--freeze_feature_encoder="False" \
--gradient_checkpointing \
--group_by_length \
--fp16 \
--overwrite_output_dir \
--do_train \
--do_eval \
--predict_with_generate \
--use_auth_token
```
On two V100s, training should take approximately 4 hours, with a final cross-entropy loss of **1e-4** and word error rate of **32.6%**.
### Warm-Started Speech-Encoder-Decoder Model
A very common use case is to leverage a pretrained speech encoder model,
*e.g.* [Wav2Vec2](https://huggingface.co/transformers/main/model_doc/wav2vec2.html), [HuBERT](https://huggingface.co/transformers/main/model_doc/hubert.html) or [XLSR-Wav2Vec2](https://huggingface.co/transformers/main/model_doc/xlsr_wav2vec2.html), with a pretrained text decoder model, *e.g.* [BART](https://huggingface.co/docs/transformers/main/en/model_doc/bart#transformers.BartForCausalLM) or [GPT-2](https://huggingface.co/docs/transformers/main/en/model_doc/gpt2#transformers.GPT2ForCausalLM), to create a [Speech-Encoder-Decoder Model](https://huggingface.co/docs/transformers/main/en/model_doc/speech-encoder-decoder#speech-encoder-decoder-models).
By pairing a pretrained speech model with a pretrained text model, the warm-started model has prior knowledge of both the source audio and target text domains. However, the cross-attention weights between the encoder and decoder are randomly initialised. Thus, the model requires fine-tuning to learn the cross-attention weights and align the encoder mapping with that of the decoder. We can perform this very fine-tuning procedure using the example script.
As an example, let's instantiate a *Wav2Vec2-2-Bart* model with the `SpeechEncoderDecoderModel` framework. First create an empty repo on `hf.co`:
```bash
huggingface-cli repo create wav2vec2-2-bart-base
git clone https://huggingface.co/<your-user-name>/wav2vec2-2-bart-base
cd wav2vec2-2-bart-base
```
Next, run the following script **inside** the just cloned repo:
```python
from transformers import SpeechEncoderDecoderModel, AutoFeatureExtractor, AutoTokenizer, Wav2Vec2Processor
# checkpoints to leverage
encoder_id = "facebook/wav2vec2-base"
decoder_id = "facebook/bart-base"
# load and save speech-encoder-decoder model
# set some hyper-parameters for training and evaluation
model = SpeechEncoderDecoderModel.from_encoder_decoder_pretrained(encoder_id, decoder_id, encoder_add_adapter=True, encoder_feat_proj_dropout=0.0, encoder_layerdrop=0.0, max_length=200, num_beams=5)
model.config.decoder_start_token_id = model.decoder.config.bos_token_id
model.config.pad_token_id = model.decoder.config.pad_token_id
model.config.eos_token_id = model.decoder.config.eos_token_id
model.save_pretrained("./")
# load and save processor
feature_extractor = AutoFeatureExtractor.from_pretrained(encoder_id)
tokenizer = AutoTokenizer.from_pretrained(decoder_id)
processor = Wav2Vec2Processor(feature_extractor, tokenizer)
processor.save_pretrained("./")
```
Finally, we can upload all files:
```bash
git lfs install
git add . && git commit -m "upload model files" && git push
```
and link the official `run_speech_recognition_seq2seq.py` script to the folder:
```bash
ln -s $(realpath <path/to/transformers>/examples/pytorch/speech-recognition/run_speech_recognition_seq2seq.py) ./
```
Note that we have added a randomly initialized _adapter layer_ to `wav2vec2-base` with the argument
`encoder_add_adapter=True`. This adapter sub-samples the output sequence of
`wav2vec2-base` along the time dimension. By default, a single
output vector of `wav2vec2-base` has a receptive field of *ca.* 25ms (*cf.*
Section *4.2* of the [official Wav2Vec2 paper](https://arxiv.org/pdf/2006.11477.pdf)), which represents a little less a single character. On the other hand, BART
makes use of a sentence-piece tokenizer as an input processor, so that a single
hidden vector of `bart-base` represents *ca.* 4 characters. To better align the
receptive field of the *Wav2Vec2* output vectors with *BART*'s hidden-states in the cross-attention
mechanism, we further subsample *Wav2Vec2*'s output by a factor of 8 by
adding a convolution-based adapter.
Having warm-started the speech-encoder-decoder model under `<your-user-name>/wav2vec2-2-bart`, we can now fine-tune it on the task of speech recognition.
In the script [`run_speech_recognition_seq2seq`], we load the warm-started model,
feature extractor, and tokenizer, process a speech recognition dataset,
and subsequently make use of the [`Seq2SeqTrainer`](https://huggingface.co/docs/transformers/main/en/main_classes/trainer#transformers.Seq2SeqTrainer) to train our system.
Note that it is important to align the target transcriptions with the decoder's vocabulary. For example, the [`Librispeech`](https://huggingface.co/datasets/librispeech_asr) dataset only contains capitalized letters in the transcriptions,
whereas BART was pretrained mostly on normalized text. Thus, it is recommended to add the argument
`--do_lower_case` to the fine-tuning script when using a warm-started `SpeechEncoderDecoderModel`.
The model is fine-tuned on the standard cross-entropy language modeling
loss for sequence-to-sequence (just like *T5* or *BART* in natural language processing).
---
**NOTE**
If you encounter problems with data preprocessing by setting `--preprocessing_num_workers` > 1,
you might want to set the environment variable `OMP_NUM_THREADS` to 1 as follows:
```bash
OMP_NUM_THREADS=1 python run_speech_recognition_ctc ...
```
If the environment variable is not set, the training script might freeze, *i.e.* see: https://github.com/pytorch/audio/issues/1021#issuecomment-726915239.
---
#### Single GPU Seq2Seq
The following command shows how to fine-tune [XLSR-Wav2Vec2](https://huggingface.co/transformers/main/model_doc/xlsr_wav2vec2.html) on [Common Voice](https://huggingface.co/datasets/common_voice) using a single GPU in half-precision.
```bash
python run_speech_recognition_seq2seq.py \
--dataset_name="librispeech_asr" \
--model_name_or_path="./" \
--dataset_config_name="clean" \
--train_split_name="train.100" \
--eval_split_name="validation" \
--output_dir="./" \
--preprocessing_num_workers="16" \
--length_column_name="input_length" \
--overwrite_output_dir \
--num_train_epochs="5" \
--per_device_train_batch_size="8" \
--per_device_eval_batch_size="8" \
--gradient_accumulation_steps="8" \
--learning_rate="3e-4" \
--warmup_steps="400" \
--evaluation_strategy="steps" \
--text_column_name="text" \
--save_steps="400" \
--eval_steps="400" \
--logging_steps="10" \
--save_total_limit="1" \
--freeze_feature_encoder \
--gradient_checkpointing \
--fp16 \
--group_by_length \
--predict_with_generate \
--generation_max_length="40" \
--generation_num_beams="1" \
--do_train --do_eval \
--do_lower_case
```
On a single V100 GPU, this script should run in *ca.* 5 hours and yield a
cross-entropy loss of **0.405** and word error rate of **0.0728**.
#### Multi GPU Seq2Seq
The following command shows how to fine-tune [XLSR-Wav2Vec2](https://huggingface.co/transformers/main/model_doc/xlsr_wav2vec2.html) on [Common Voice](https://huggingface.co/datasets/common_voice) using 8 GPUs in half-precision.
```bash
torchrun \
--nproc_per_node 8 run_speech_recognition_seq2seq.py \
--dataset_name="librispeech_asr" \
--model_name_or_path="./" \
--dataset_config_name="clean" \
--train_split_name="train.100" \
--eval_split_name="validation" \
--output_dir="./" \
--preprocessing_num_workers="16" \
--length_column_name="input_length" \
--overwrite_output_dir \
--num_train_epochs="5" \
--per_device_train_batch_size="8" \
--per_device_eval_batch_size="8" \
--gradient_accumulation_steps="1" \
--learning_rate="3e-4" \
--warmup_steps="400" \
--evaluation_strategy="steps" \
--text_column_name="text" \
--save_steps="400" \
--eval_steps="400" \
--logging_steps="10" \
--save_total_limit="1" \
--freeze_feature_encoder \
--gradient_checkpointing \
--fp16 \
--group_by_length \
--predict_with_generate \
--do_train --do_eval \
--do_lower_case
```
On 8 V100 GPUs, this script should run in *ca.* 45 minutes and yield a cross-entropy loss of **0.405** and word error rate of **0.0728**
### Examples Seq2Seq
#### Librispeech Seq2Seq
- [Librispeech](https://huggingface.co/datasets/librispeech_asr)
| Dataset | Dataset Config | Pretrained Model | Word error rate on eval | Phoneme error rate on eval | GPU setup | Training time | Fine-tuned Model & Logs | Command to reproduce |
|----------------------------------------------------------------|---------------------------|-----------------------------------------------------------------------------------------------------------------------------------------------------------|-------------------------|----------------------------|------------|---------------|-----------------------------------------------------------------------|-------------------------------------------------------------------------------------------------------------------------------------------------------------------------------------------------------------------|
| [Librispeech](https://huggingface.co/datasets/librispeech_asr) | `"clean"` - `"train.100"` | [facebook/wav2vec2-base](https://huggingface.co/facebook/wav2vec2-base) and [facebook/bart-base](https://huggingface.co/facebook/bart-base) | 0.0728 | - | 8 GPU V100 | 45min | [here](https://huggingface.co/patrickvonplaten/wav2vec2-2-bart-base) | [create_model.py](https://huggingface.co/patrickvonplaten/wav2vec2-2-bart-base/blob/main/create_model.py) & [run.sh](https://huggingface.co/patrickvonplaten/wav2vec2-2-bart-base/blob/main/run_librispeech.sh) |
| [Librispeech](https://huggingface.co/datasets/librispeech_asr) | `"clean"` - `"train.100"` | [facebook/wav2vec2-large-lv60](https://huggingface.co/facebook/wav2vec2-large-lv60) and [facebook/bart-large](https://huggingface.co/facebook/bart-large) | 0.0486 | - | 8 GPU V100 | 1h20min | [here](https://huggingface.co/patrickvonplaten/wav2vec2-2-bart-large) | [create_model.py](https://huggingface.co/patrickvonplaten/wav2vec2-2-bart-large/blob/main/create_model.py) & [run.sh](https://huggingface.co/patrickvonplaten/wav2vec2-2-bart-large/blob/main/run_librispeech.sh) |
| transformers/examples/pytorch/speech-recognition/README.md/0 | {
"file_path": "transformers/examples/pytorch/speech-recognition/README.md",
"repo_id": "transformers",
"token_count": 14259
} | 285 |
#!/usr/bin/env python
# coding=utf-8
# Copyright 2018 The Google AI Language Team Authors and The HuggingFace Inc. team.
# Copyright (c) 2018, NVIDIA CORPORATION. All rights reserved.
#
# Licensed under the Apache License, Version 2.0 (the "License");
# you may not use this file except in compliance with the License.
# You may obtain a copy of the License at
#
# http://www.apache.org/licenses/LICENSE-2.0
#
# Unless required by applicable law or agreed to in writing, software
# distributed under the License is distributed on an "AS IS" BASIS,
# WITHOUT WARRANTIES OR CONDITIONS OF ANY KIND, either express or implied.
# See the License for the specific language governing permissions and
# limitations under the License.
""" Finetuning multi-lingual models on XNLI (e.g. Bert, DistilBERT, XLM).
Adapted from `examples/text-classification/run_glue.py`"""
import logging
import os
import random
import sys
import warnings
from dataclasses import dataclass, field
from typing import Optional
import datasets
import evaluate
import numpy as np
from datasets import load_dataset
import transformers
from transformers import (
AutoConfig,
AutoModelForSequenceClassification,
AutoTokenizer,
DataCollatorWithPadding,
EvalPrediction,
HfArgumentParser,
Trainer,
TrainingArguments,
default_data_collator,
set_seed,
)
from transformers.trainer_utils import get_last_checkpoint
from transformers.utils import check_min_version, send_example_telemetry
from transformers.utils.versions import require_version
# Will error if the minimal version of Transformers is not installed. Remove at your own risks.
check_min_version("4.38.0.dev0")
require_version("datasets>=1.8.0", "To fix: pip install -r examples/pytorch/text-classification/requirements.txt")
logger = logging.getLogger(__name__)
@dataclass
class DataTrainingArguments:
"""
Arguments pertaining to what data we are going to input our model for training and eval.
Using `HfArgumentParser` we can turn this class
into argparse arguments to be able to specify them on
the command line.
"""
max_seq_length: Optional[int] = field(
default=128,
metadata={
"help": (
"The maximum total input sequence length after tokenization. Sequences longer "
"than this will be truncated, sequences shorter will be padded."
)
},
)
overwrite_cache: bool = field(
default=False, metadata={"help": "Overwrite the cached preprocessed datasets or not."}
)
pad_to_max_length: bool = field(
default=True,
metadata={
"help": (
"Whether to pad all samples to `max_seq_length`. "
"If False, will pad the samples dynamically when batching to the maximum length in the batch."
)
},
)
max_train_samples: Optional[int] = field(
default=None,
metadata={
"help": (
"For debugging purposes or quicker training, truncate the number of training examples to this "
"value if set."
)
},
)
max_eval_samples: Optional[int] = field(
default=None,
metadata={
"help": (
"For debugging purposes or quicker training, truncate the number of evaluation examples to this "
"value if set."
)
},
)
max_predict_samples: Optional[int] = field(
default=None,
metadata={
"help": (
"For debugging purposes or quicker training, truncate the number of prediction examples to this "
"value if set."
)
},
)
@dataclass
class ModelArguments:
"""
Arguments pertaining to which model/config/tokenizer we are going to fine-tune from.
"""
model_name_or_path: str = field(
default=None, metadata={"help": "Path to pretrained model or model identifier from huggingface.co/models"}
)
language: str = field(
default=None, metadata={"help": "Evaluation language. Also train language if `train_language` is set to None."}
)
train_language: Optional[str] = field(
default=None, metadata={"help": "Train language if it is different from the evaluation language."}
)
config_name: Optional[str] = field(
default=None, metadata={"help": "Pretrained config name or path if not the same as model_name"}
)
tokenizer_name: Optional[str] = field(
default=None, metadata={"help": "Pretrained tokenizer name or path if not the same as model_name"}
)
cache_dir: Optional[str] = field(
default=None,
metadata={"help": "Where do you want to store the pretrained models downloaded from huggingface.co"},
)
do_lower_case: Optional[bool] = field(
default=False,
metadata={"help": "arg to indicate if tokenizer should do lower case in AutoTokenizer.from_pretrained()"},
)
use_fast_tokenizer: bool = field(
default=True,
metadata={"help": "Whether to use one of the fast tokenizer (backed by the tokenizers library) or not."},
)
model_revision: str = field(
default="main",
metadata={"help": "The specific model version to use (can be a branch name, tag name or commit id)."},
)
token: str = field(
default=None,
metadata={
"help": (
"The token to use as HTTP bearer authorization for remote files. If not specified, will use the token "
"generated when running `huggingface-cli login` (stored in `~/.huggingface`)."
)
},
)
use_auth_token: bool = field(
default=None,
metadata={
"help": "The `use_auth_token` argument is deprecated and will be removed in v4.34. Please use `token` instead."
},
)
trust_remote_code: bool = field(
default=False,
metadata={
"help": (
"Whether or not to allow for custom models defined on the Hub in their own modeling files. This option "
"should only be set to `True` for repositories you trust and in which you have read the code, as it will "
"execute code present on the Hub on your local machine."
)
},
)
ignore_mismatched_sizes: bool = field(
default=False,
metadata={"help": "Will enable to load a pretrained model whose head dimensions are different."},
)
def main():
# See all possible arguments in src/transformers/training_args.py
# or by passing the --help flag to this script.
# We now keep distinct sets of args, for a cleaner separation of concerns.
parser = HfArgumentParser((ModelArguments, DataTrainingArguments, TrainingArguments))
model_args, data_args, training_args = parser.parse_args_into_dataclasses()
if model_args.use_auth_token is not None:
warnings.warn(
"The `use_auth_token` argument is deprecated and will be removed in v4.34. Please use `token` instead.",
FutureWarning,
)
if model_args.token is not None:
raise ValueError("`token` and `use_auth_token` are both specified. Please set only the argument `token`.")
model_args.token = model_args.use_auth_token
# Sending telemetry. Tracking the example usage helps us better allocate resources to maintain them. The
# information sent is the one passed as arguments along with your Python/PyTorch versions.
send_example_telemetry("run_xnli", model_args)
# Setup logging
logging.basicConfig(
format="%(asctime)s - %(levelname)s - %(name)s - %(message)s",
datefmt="%m/%d/%Y %H:%M:%S",
handlers=[logging.StreamHandler(sys.stdout)],
)
if training_args.should_log:
# The default of training_args.log_level is passive, so we set log level at info here to have that default.
transformers.utils.logging.set_verbosity_info()
log_level = training_args.get_process_log_level()
logger.setLevel(log_level)
datasets.utils.logging.set_verbosity(log_level)
transformers.utils.logging.set_verbosity(log_level)
transformers.utils.logging.enable_default_handler()
transformers.utils.logging.enable_explicit_format()
# Log on each process the small summary:
logger.warning(
f"Process rank: {training_args.local_rank}, device: {training_args.device}, n_gpu: {training_args.n_gpu}, "
+ f"distributed training: {training_args.parallel_mode.value == 'distributed'}, 16-bits training: {training_args.fp16}"
)
logger.info(f"Training/evaluation parameters {training_args}")
# Detecting last checkpoint.
last_checkpoint = None
if os.path.isdir(training_args.output_dir) and training_args.do_train and not training_args.overwrite_output_dir:
last_checkpoint = get_last_checkpoint(training_args.output_dir)
if last_checkpoint is None and len(os.listdir(training_args.output_dir)) > 0:
raise ValueError(
f"Output directory ({training_args.output_dir}) already exists and is not empty. "
"Use --overwrite_output_dir to overcome."
)
elif last_checkpoint is not None:
logger.info(
f"Checkpoint detected, resuming training at {last_checkpoint}. To avoid this behavior, change "
"the `--output_dir` or add `--overwrite_output_dir` to train from scratch."
)
# Set seed before initializing model.
set_seed(training_args.seed)
# In distributed training, the load_dataset function guarantees that only one local process can concurrently
# download the dataset.
# Downloading and loading xnli dataset from the hub.
if training_args.do_train:
if model_args.train_language is None:
train_dataset = load_dataset(
"xnli",
model_args.language,
split="train",
cache_dir=model_args.cache_dir,
token=model_args.token,
)
else:
train_dataset = load_dataset(
"xnli",
model_args.train_language,
split="train",
cache_dir=model_args.cache_dir,
token=model_args.token,
)
label_list = train_dataset.features["label"].names
if training_args.do_eval:
eval_dataset = load_dataset(
"xnli",
model_args.language,
split="validation",
cache_dir=model_args.cache_dir,
token=model_args.token,
)
label_list = eval_dataset.features["label"].names
if training_args.do_predict:
predict_dataset = load_dataset(
"xnli",
model_args.language,
split="test",
cache_dir=model_args.cache_dir,
token=model_args.token,
)
label_list = predict_dataset.features["label"].names
# Labels
num_labels = len(label_list)
# Load pretrained model and tokenizer
# In distributed training, the .from_pretrained methods guarantee that only one local process can concurrently
# download model & vocab.
config = AutoConfig.from_pretrained(
model_args.config_name if model_args.config_name else model_args.model_name_or_path,
num_labels=num_labels,
id2label={str(i): label for i, label in enumerate(label_list)},
label2id={label: i for i, label in enumerate(label_list)},
finetuning_task="xnli",
cache_dir=model_args.cache_dir,
revision=model_args.model_revision,
token=model_args.token,
trust_remote_code=model_args.trust_remote_code,
)
tokenizer = AutoTokenizer.from_pretrained(
model_args.tokenizer_name if model_args.tokenizer_name else model_args.model_name_or_path,
do_lower_case=model_args.do_lower_case,
cache_dir=model_args.cache_dir,
use_fast=model_args.use_fast_tokenizer,
revision=model_args.model_revision,
token=model_args.token,
trust_remote_code=model_args.trust_remote_code,
)
model = AutoModelForSequenceClassification.from_pretrained(
model_args.model_name_or_path,
from_tf=bool(".ckpt" in model_args.model_name_or_path),
config=config,
cache_dir=model_args.cache_dir,
revision=model_args.model_revision,
token=model_args.token,
trust_remote_code=model_args.trust_remote_code,
ignore_mismatched_sizes=model_args.ignore_mismatched_sizes,
)
# Preprocessing the datasets
# Padding strategy
if data_args.pad_to_max_length:
padding = "max_length"
else:
# We will pad later, dynamically at batch creation, to the max sequence length in each batch
padding = False
def preprocess_function(examples):
# Tokenize the texts
return tokenizer(
examples["premise"],
examples["hypothesis"],
padding=padding,
max_length=data_args.max_seq_length,
truncation=True,
)
if training_args.do_train:
if data_args.max_train_samples is not None:
max_train_samples = min(len(train_dataset), data_args.max_train_samples)
train_dataset = train_dataset.select(range(max_train_samples))
with training_args.main_process_first(desc="train dataset map pre-processing"):
train_dataset = train_dataset.map(
preprocess_function,
batched=True,
load_from_cache_file=not data_args.overwrite_cache,
desc="Running tokenizer on train dataset",
)
# Log a few random samples from the training set:
for index in random.sample(range(len(train_dataset)), 3):
logger.info(f"Sample {index} of the training set: {train_dataset[index]}.")
if training_args.do_eval:
if data_args.max_eval_samples is not None:
max_eval_samples = min(len(eval_dataset), data_args.max_eval_samples)
eval_dataset = eval_dataset.select(range(max_eval_samples))
with training_args.main_process_first(desc="validation dataset map pre-processing"):
eval_dataset = eval_dataset.map(
preprocess_function,
batched=True,
load_from_cache_file=not data_args.overwrite_cache,
desc="Running tokenizer on validation dataset",
)
if training_args.do_predict:
if data_args.max_predict_samples is not None:
max_predict_samples = min(len(predict_dataset), data_args.max_predict_samples)
predict_dataset = predict_dataset.select(range(max_predict_samples))
with training_args.main_process_first(desc="prediction dataset map pre-processing"):
predict_dataset = predict_dataset.map(
preprocess_function,
batched=True,
load_from_cache_file=not data_args.overwrite_cache,
desc="Running tokenizer on prediction dataset",
)
# Get the metric function
metric = evaluate.load("xnli", cache_dir=model_args.cache_dir)
# You can define your custom compute_metrics function. It takes an `EvalPrediction` object (a namedtuple with a
# predictions and label_ids field) and has to return a dictionary string to float.
def compute_metrics(p: EvalPrediction):
preds = p.predictions[0] if isinstance(p.predictions, tuple) else p.predictions
preds = np.argmax(preds, axis=1)
return metric.compute(predictions=preds, references=p.label_ids)
# Data collator will default to DataCollatorWithPadding, so we change it if we already did the padding.
if data_args.pad_to_max_length:
data_collator = default_data_collator
elif training_args.fp16:
data_collator = DataCollatorWithPadding(tokenizer, pad_to_multiple_of=8)
else:
data_collator = None
# Initialize our Trainer
trainer = Trainer(
model=model,
args=training_args,
train_dataset=train_dataset if training_args.do_train else None,
eval_dataset=eval_dataset if training_args.do_eval else None,
compute_metrics=compute_metrics,
tokenizer=tokenizer,
data_collator=data_collator,
)
# Training
if training_args.do_train:
checkpoint = None
if training_args.resume_from_checkpoint is not None:
checkpoint = training_args.resume_from_checkpoint
elif last_checkpoint is not None:
checkpoint = last_checkpoint
train_result = trainer.train(resume_from_checkpoint=checkpoint)
metrics = train_result.metrics
max_train_samples = (
data_args.max_train_samples if data_args.max_train_samples is not None else len(train_dataset)
)
metrics["train_samples"] = min(max_train_samples, len(train_dataset))
trainer.save_model() # Saves the tokenizer too for easy upload
trainer.log_metrics("train", metrics)
trainer.save_metrics("train", metrics)
trainer.save_state()
# Evaluation
if training_args.do_eval:
logger.info("*** Evaluate ***")
metrics = trainer.evaluate(eval_dataset=eval_dataset)
max_eval_samples = data_args.max_eval_samples if data_args.max_eval_samples is not None else len(eval_dataset)
metrics["eval_samples"] = min(max_eval_samples, len(eval_dataset))
trainer.log_metrics("eval", metrics)
trainer.save_metrics("eval", metrics)
# Prediction
if training_args.do_predict:
logger.info("*** Predict ***")
predictions, labels, metrics = trainer.predict(predict_dataset, metric_key_prefix="predict")
max_predict_samples = (
data_args.max_predict_samples if data_args.max_predict_samples is not None else len(predict_dataset)
)
metrics["predict_samples"] = min(max_predict_samples, len(predict_dataset))
trainer.log_metrics("predict", metrics)
trainer.save_metrics("predict", metrics)
predictions = np.argmax(predictions, axis=1)
output_predict_file = os.path.join(training_args.output_dir, "predictions.txt")
if trainer.is_world_process_zero():
with open(output_predict_file, "w") as writer:
writer.write("index\tprediction\n")
for index, item in enumerate(predictions):
item = label_list[item]
writer.write(f"{index}\t{item}\n")
if __name__ == "__main__":
main()
| transformers/examples/pytorch/text-classification/run_xnli.py/0 | {
"file_path": "transformers/examples/pytorch/text-classification/run_xnli.py",
"repo_id": "transformers",
"token_count": 7663
} | 286 |
<!---
Copyright 2020 The HuggingFace Team. All rights reserved.
Licensed under the Apache License, Version 2.0 (the "License");
you may not use this file except in compliance with the License.
You may obtain a copy of the License at
http://www.apache.org/licenses/LICENSE-2.0
Unless required by applicable law or agreed to in writing, software
distributed under the License is distributed on an "AS IS" BASIS,
WITHOUT WARRANTIES OR CONDITIONS OF ANY KIND, either express or implied.
See the License for the specific language governing permissions and
limitations under the License.
-->
# Research projects
This folder contains various research projects using ๐ค Transformers. They are not maintained and require a specific
version of ๐ค Transformers that is indicated in the requirements file of each folder. Updating them to the most recent version of the library will require some work.
To use any of them, just run the command
```
pip install -r requirements.txt
```
inside the folder of your choice.
If you need help with any of those, contact the author(s), indicated at the top of the `README` of each folder.
| transformers/examples/research_projects/README.md/0 | {
"file_path": "transformers/examples/research_projects/README.md",
"repo_id": "transformers",
"token_count": 279
} | 287 |
# MIT License
# Copyright (c) 2019 Yang Liu and the HuggingFace team
# Permission is hereby granted, free of charge, to any person obtaining a copy
# of this software and associated documentation files (the "Software"), to deal
# in the Software without restriction, including without limitation the rights
# to use, copy, modify, merge, publish, distribute, sublicense, and/or sell
# copies of the Software, and to permit persons to whom the Software is
# furnished to do so, subject to the following conditions:
# The above copyright notice and this permission notice shall be included in all
# copies or substantial portions of the Software.
# THE SOFTWARE IS PROVIDED "AS IS", WITHOUT WARRANTY OF ANY KIND, EXPRESS OR
# IMPLIED, INCLUDING BUT NOT LIMITED TO THE WARRANTIES OF MERCHANTABILITY,
# FITNESS FOR A PARTICULAR PURPOSE AND NONINFRINGEMENT. IN NO EVENT SHALL THE
# AUTHORS OR COPYRIGHT HOLDERS BE LIABLE FOR ANY CLAIM, DAMAGES OR OTHER
# LIABILITY, WHETHER IN AN ACTION OF CONTRACT, TORT OR OTHERWISE, ARISING FROM,
# OUT OF OR IN CONNECTION WITH THE SOFTWARE OR THE USE OR OTHER DEALINGS IN THE
# SOFTWARE.
import copy
import math
import numpy as np
import torch
from configuration_bertabs import BertAbsConfig
from torch import nn
from torch.nn.init import xavier_uniform_
from transformers import BertConfig, BertModel, PreTrainedModel
MAX_SIZE = 5000
BERTABS_FINETUNED_MODEL_ARCHIVE_LIST = [
"remi/bertabs-finetuned-cnndm-extractive-abstractive-summarization",
]
class BertAbsPreTrainedModel(PreTrainedModel):
config_class = BertAbsConfig
load_tf_weights = False
base_model_prefix = "bert"
class BertAbs(BertAbsPreTrainedModel):
def __init__(self, args, checkpoint=None, bert_extractive_checkpoint=None):
super().__init__(args)
self.args = args
self.bert = Bert()
# If pre-trained weights are passed for Bert, load these.
load_bert_pretrained_extractive = True if bert_extractive_checkpoint else False
if load_bert_pretrained_extractive:
self.bert.model.load_state_dict(
{n[11:]: p for n, p in bert_extractive_checkpoint.items() if n.startswith("bert.model")},
strict=True,
)
self.vocab_size = self.bert.model.config.vocab_size
if args.max_pos > 512:
my_pos_embeddings = nn.Embedding(args.max_pos, self.bert.model.config.hidden_size)
my_pos_embeddings.weight.data[:512] = self.bert.model.embeddings.position_embeddings.weight.data
my_pos_embeddings.weight.data[512:] = self.bert.model.embeddings.position_embeddings.weight.data[-1][
None, :
].repeat(args.max_pos - 512, 1)
self.bert.model.embeddings.position_embeddings = my_pos_embeddings
tgt_embeddings = nn.Embedding(self.vocab_size, self.bert.model.config.hidden_size, padding_idx=0)
tgt_embeddings.weight = copy.deepcopy(self.bert.model.embeddings.word_embeddings.weight)
self.decoder = TransformerDecoder(
self.args.dec_layers,
self.args.dec_hidden_size,
heads=self.args.dec_heads,
d_ff=self.args.dec_ff_size,
dropout=self.args.dec_dropout,
embeddings=tgt_embeddings,
vocab_size=self.vocab_size,
)
gen_func = nn.LogSoftmax(dim=-1)
self.generator = nn.Sequential(nn.Linear(args.dec_hidden_size, args.vocab_size), gen_func)
self.generator[0].weight = self.decoder.embeddings.weight
load_from_checkpoints = False if checkpoint is None else True
if load_from_checkpoints:
self.load_state_dict(checkpoint)
def init_weights(self):
for module in self.decoder.modules():
if isinstance(module, (nn.Linear, nn.Embedding)):
module.weight.data.normal_(mean=0.0, std=0.02)
elif isinstance(module, nn.LayerNorm):
module.bias.data.zero_()
module.weight.data.fill_(1.0)
if isinstance(module, nn.Linear) and module.bias is not None:
module.bias.data.zero_()
for p in self.generator.parameters():
if p.dim() > 1:
xavier_uniform_(p)
else:
p.data.zero_()
def forward(
self,
encoder_input_ids,
decoder_input_ids,
token_type_ids,
encoder_attention_mask,
decoder_attention_mask,
):
encoder_output = self.bert(
input_ids=encoder_input_ids,
token_type_ids=token_type_ids,
attention_mask=encoder_attention_mask,
)
encoder_hidden_states = encoder_output[0]
dec_state = self.decoder.init_decoder_state(encoder_input_ids, encoder_hidden_states)
decoder_outputs, _ = self.decoder(decoder_input_ids[:, :-1], encoder_hidden_states, dec_state)
return decoder_outputs
class Bert(nn.Module):
"""This class is not really necessary and should probably disappear."""
def __init__(self):
super().__init__()
config = BertConfig.from_pretrained("bert-base-uncased")
self.model = BertModel(config)
def forward(self, input_ids, attention_mask=None, token_type_ids=None, **kwargs):
self.eval()
with torch.no_grad():
encoder_outputs, _ = self.model(
input_ids, token_type_ids=token_type_ids, attention_mask=attention_mask, **kwargs
)
return encoder_outputs
class TransformerDecoder(nn.Module):
"""
The Transformer decoder from "Attention is All You Need".
Args:
num_layers (int): number of encoder layers.
d_model (int): size of the model
heads (int): number of heads
d_ff (int): size of the inner FF layer
dropout (float): dropout parameters
embeddings (:obj:`onmt.modules.Embeddings`):
embeddings to use, should have positional encodings
attn_type (str): if using a separate copy attention
"""
def __init__(self, num_layers, d_model, heads, d_ff, dropout, embeddings, vocab_size):
super().__init__()
# Basic attributes.
self.decoder_type = "transformer"
self.num_layers = num_layers
self.embeddings = embeddings
self.pos_emb = PositionalEncoding(dropout, self.embeddings.embedding_dim)
# Build TransformerDecoder.
self.transformer_layers = nn.ModuleList(
[TransformerDecoderLayer(d_model, heads, d_ff, dropout) for _ in range(num_layers)]
)
self.layer_norm = nn.LayerNorm(d_model, eps=1e-6)
# forward(input_ids, attention_mask, encoder_hidden_states, encoder_attention_mask)
# def forward(self, input_ids, state, attention_mask=None, memory_lengths=None,
# step=None, cache=None, encoder_attention_mask=None, encoder_hidden_states=None, memory_masks=None):
def forward(
self,
input_ids,
encoder_hidden_states=None,
state=None,
attention_mask=None,
memory_lengths=None,
step=None,
cache=None,
encoder_attention_mask=None,
):
"""
See :obj:`onmt.modules.RNNDecoderBase.forward()`
memory_bank = encoder_hidden_states
"""
# Name conversion
tgt = input_ids
memory_bank = encoder_hidden_states
memory_mask = encoder_attention_mask
# src_words = state.src
src_words = state.src
src_batch, src_len = src_words.size()
padding_idx = self.embeddings.padding_idx
# Decoder padding mask
tgt_words = tgt
tgt_batch, tgt_len = tgt_words.size()
tgt_pad_mask = tgt_words.data.eq(padding_idx).unsqueeze(1).expand(tgt_batch, tgt_len, tgt_len)
# Encoder padding mask
if memory_mask is not None:
src_len = memory_mask.size(-1)
src_pad_mask = memory_mask.expand(src_batch, tgt_len, src_len)
else:
src_pad_mask = src_words.data.eq(padding_idx).unsqueeze(1).expand(src_batch, tgt_len, src_len)
# Pass through the embeddings
emb = self.embeddings(input_ids)
output = self.pos_emb(emb, step)
assert emb.dim() == 3 # len x batch x embedding_dim
if state.cache is None:
saved_inputs = []
for i in range(self.num_layers):
prev_layer_input = None
if state.cache is None:
if state.previous_input is not None:
prev_layer_input = state.previous_layer_inputs[i]
output, all_input = self.transformer_layers[i](
output,
memory_bank,
src_pad_mask,
tgt_pad_mask,
previous_input=prev_layer_input,
layer_cache=state.cache["layer_{}".format(i)] if state.cache is not None else None,
step=step,
)
if state.cache is None:
saved_inputs.append(all_input)
if state.cache is None:
saved_inputs = torch.stack(saved_inputs)
output = self.layer_norm(output)
if state.cache is None:
state = state.update_state(tgt, saved_inputs)
# Decoders in transformers return a tuple. Beam search will fail
# if we don't follow this convention.
return output, state # , state
def init_decoder_state(self, src, memory_bank, with_cache=False):
"""Init decoder state"""
state = TransformerDecoderState(src)
if with_cache:
state._init_cache(memory_bank, self.num_layers)
return state
class PositionalEncoding(nn.Module):
def __init__(self, dropout, dim, max_len=5000):
pe = torch.zeros(max_len, dim)
position = torch.arange(0, max_len).unsqueeze(1)
div_term = torch.exp((torch.arange(0, dim, 2, dtype=torch.float) * -(math.log(10000.0) / dim)))
pe[:, 0::2] = torch.sin(position.float() * div_term)
pe[:, 1::2] = torch.cos(position.float() * div_term)
pe = pe.unsqueeze(0)
super().__init__()
self.register_buffer("pe", pe)
self.dropout = nn.Dropout(p=dropout)
self.dim = dim
def forward(self, emb, step=None):
emb = emb * math.sqrt(self.dim)
if step:
emb = emb + self.pe[:, step][:, None, :]
else:
emb = emb + self.pe[:, : emb.size(1)]
emb = self.dropout(emb)
return emb
def get_emb(self, emb):
return self.pe[:, : emb.size(1)]
class TransformerDecoderLayer(nn.Module):
"""
Args:
d_model (int): the dimension of keys/values/queries in
MultiHeadedAttention, also the input size of
the first-layer of the PositionwiseFeedForward.
heads (int): the number of heads for MultiHeadedAttention.
d_ff (int): the second-layer of the PositionwiseFeedForward.
dropout (float): dropout probability(0-1.0).
self_attn_type (string): type of self-attention scaled-dot, average
"""
def __init__(self, d_model, heads, d_ff, dropout):
super().__init__()
self.self_attn = MultiHeadedAttention(heads, d_model, dropout=dropout)
self.context_attn = MultiHeadedAttention(heads, d_model, dropout=dropout)
self.feed_forward = PositionwiseFeedForward(d_model, d_ff, dropout)
self.layer_norm_1 = nn.LayerNorm(d_model, eps=1e-6)
self.layer_norm_2 = nn.LayerNorm(d_model, eps=1e-6)
self.drop = nn.Dropout(dropout)
mask = self._get_attn_subsequent_mask(MAX_SIZE)
# Register self.mask as a saved_state in TransformerDecoderLayer, so
# it gets TransformerDecoderLayer's cuda behavior automatically.
self.register_buffer("mask", mask)
def forward(
self,
inputs,
memory_bank,
src_pad_mask,
tgt_pad_mask,
previous_input=None,
layer_cache=None,
step=None,
):
"""
Args:
inputs (`FloatTensor`): `[batch_size x 1 x model_dim]`
memory_bank (`FloatTensor`): `[batch_size x src_len x model_dim]`
src_pad_mask (`LongTensor`): `[batch_size x 1 x src_len]`
tgt_pad_mask (`LongTensor`): `[batch_size x 1 x 1]`
Returns:
(`FloatTensor`, `FloatTensor`, `FloatTensor`):
* output `[batch_size x 1 x model_dim]`
* attn `[batch_size x 1 x src_len]`
* all_input `[batch_size x current_step x model_dim]`
"""
dec_mask = torch.gt(tgt_pad_mask + self.mask[:, : tgt_pad_mask.size(1), : tgt_pad_mask.size(1)], 0)
input_norm = self.layer_norm_1(inputs)
all_input = input_norm
if previous_input is not None:
all_input = torch.cat((previous_input, input_norm), dim=1)
dec_mask = None
query = self.self_attn(
all_input,
all_input,
input_norm,
mask=dec_mask,
layer_cache=layer_cache,
type="self",
)
query = self.drop(query) + inputs
query_norm = self.layer_norm_2(query)
mid = self.context_attn(
memory_bank,
memory_bank,
query_norm,
mask=src_pad_mask,
layer_cache=layer_cache,
type="context",
)
output = self.feed_forward(self.drop(mid) + query)
return output, all_input
# return output
def _get_attn_subsequent_mask(self, size):
"""
Get an attention mask to avoid using the subsequent info.
Args:
size: int
Returns:
(`LongTensor`):
* subsequent_mask `[1 x size x size]`
"""
attn_shape = (1, size, size)
subsequent_mask = np.triu(np.ones(attn_shape), k=1).astype("uint8")
subsequent_mask = torch.from_numpy(subsequent_mask)
return subsequent_mask
class MultiHeadedAttention(nn.Module):
"""
Multi-Head Attention module from
"Attention is All You Need"
:cite:`DBLP:journals/corr/VaswaniSPUJGKP17`.
Similar to standard `dot` attention but uses
multiple attention distributions simulataneously
to select relevant items.
.. mermaid::
graph BT
A[key]
B[value]
C[query]
O[output]
subgraph Attn
D[Attn 1]
E[Attn 2]
F[Attn N]
end
A --> D
C --> D
A --> E
C --> E
A --> F
C --> F
D --> O
E --> O
F --> O
B --> O
Also includes several additional tricks.
Args:
head_count (int): number of parallel heads
model_dim (int): the dimension of keys/values/queries,
must be divisible by head_count
dropout (float): dropout parameter
"""
def __init__(self, head_count, model_dim, dropout=0.1, use_final_linear=True):
assert model_dim % head_count == 0
self.dim_per_head = model_dim // head_count
self.model_dim = model_dim
super().__init__()
self.head_count = head_count
self.linear_keys = nn.Linear(model_dim, head_count * self.dim_per_head)
self.linear_values = nn.Linear(model_dim, head_count * self.dim_per_head)
self.linear_query = nn.Linear(model_dim, head_count * self.dim_per_head)
self.softmax = nn.Softmax(dim=-1)
self.dropout = nn.Dropout(dropout)
self.use_final_linear = use_final_linear
if self.use_final_linear:
self.final_linear = nn.Linear(model_dim, model_dim)
def forward(
self,
key,
value,
query,
mask=None,
layer_cache=None,
type=None,
predefined_graph_1=None,
):
"""
Compute the context vector and the attention vectors.
Args:
key (`FloatTensor`): set of `key_len`
key vectors `[batch, key_len, dim]`
value (`FloatTensor`): set of `key_len`
value vectors `[batch, key_len, dim]`
query (`FloatTensor`): set of `query_len`
query vectors `[batch, query_len, dim]`
mask: binary mask indicating which keys have
non-zero attention `[batch, query_len, key_len]`
Returns:
(`FloatTensor`, `FloatTensor`) :
* output context vectors `[batch, query_len, dim]`
* one of the attention vectors `[batch, query_len, key_len]`
"""
batch_size = key.size(0)
dim_per_head = self.dim_per_head
head_count = self.head_count
def shape(x):
"""projection"""
return x.view(batch_size, -1, head_count, dim_per_head).transpose(1, 2)
def unshape(x):
"""compute context"""
return x.transpose(1, 2).contiguous().view(batch_size, -1, head_count * dim_per_head)
# 1) Project key, value, and query.
if layer_cache is not None:
if type == "self":
query, key, value = (
self.linear_query(query),
self.linear_keys(query),
self.linear_values(query),
)
key = shape(key)
value = shape(value)
if layer_cache is not None:
device = key.device
if layer_cache["self_keys"] is not None:
key = torch.cat((layer_cache["self_keys"].to(device), key), dim=2)
if layer_cache["self_values"] is not None:
value = torch.cat((layer_cache["self_values"].to(device), value), dim=2)
layer_cache["self_keys"] = key
layer_cache["self_values"] = value
elif type == "context":
query = self.linear_query(query)
if layer_cache is not None:
if layer_cache["memory_keys"] is None:
key, value = self.linear_keys(key), self.linear_values(value)
key = shape(key)
value = shape(value)
else:
key, value = (
layer_cache["memory_keys"],
layer_cache["memory_values"],
)
layer_cache["memory_keys"] = key
layer_cache["memory_values"] = value
else:
key, value = self.linear_keys(key), self.linear_values(value)
key = shape(key)
value = shape(value)
else:
key = self.linear_keys(key)
value = self.linear_values(value)
query = self.linear_query(query)
key = shape(key)
value = shape(value)
query = shape(query)
# 2) Calculate and scale scores.
query = query / math.sqrt(dim_per_head)
scores = torch.matmul(query, key.transpose(2, 3))
if mask is not None:
mask = mask.unsqueeze(1).expand_as(scores)
scores = scores.masked_fill(mask, -1e18)
# 3) Apply attention dropout and compute context vectors.
attn = self.softmax(scores)
if predefined_graph_1 is not None:
attn_masked = attn[:, -1] * predefined_graph_1
attn_masked = attn_masked / (torch.sum(attn_masked, 2).unsqueeze(2) + 1e-9)
attn = torch.cat([attn[:, :-1], attn_masked.unsqueeze(1)], 1)
drop_attn = self.dropout(attn)
if self.use_final_linear:
context = unshape(torch.matmul(drop_attn, value))
output = self.final_linear(context)
return output
else:
context = torch.matmul(drop_attn, value)
return context
class DecoderState(object):
"""Interface for grouping together the current state of a recurrent
decoder. In the simplest case just represents the hidden state of
the model. But can also be used for implementing various forms of
input_feeding and non-recurrent models.
Modules need to implement this to utilize beam search decoding.
"""
def detach(self):
"""Need to document this"""
self.hidden = tuple([_.detach() for _ in self.hidden])
self.input_feed = self.input_feed.detach()
def beam_update(self, idx, positions, beam_size):
"""Need to document this"""
for e in self._all:
sizes = e.size()
br = sizes[1]
if len(sizes) == 3:
sent_states = e.view(sizes[0], beam_size, br // beam_size, sizes[2])[:, :, idx]
else:
sent_states = e.view(sizes[0], beam_size, br // beam_size, sizes[2], sizes[3])[:, :, idx]
sent_states.data.copy_(sent_states.data.index_select(1, positions))
def map_batch_fn(self, fn):
raise NotImplementedError()
class TransformerDecoderState(DecoderState):
"""Transformer Decoder state base class"""
def __init__(self, src):
"""
Args:
src (FloatTensor): a sequence of source words tensors
with optional feature tensors, of size (len x batch).
"""
self.src = src
self.previous_input = None
self.previous_layer_inputs = None
self.cache = None
@property
def _all(self):
"""
Contains attributes that need to be updated in self.beam_update().
"""
if self.previous_input is not None and self.previous_layer_inputs is not None:
return (self.previous_input, self.previous_layer_inputs, self.src)
else:
return (self.src,)
def detach(self):
if self.previous_input is not None:
self.previous_input = self.previous_input.detach()
if self.previous_layer_inputs is not None:
self.previous_layer_inputs = self.previous_layer_inputs.detach()
self.src = self.src.detach()
def update_state(self, new_input, previous_layer_inputs):
state = TransformerDecoderState(self.src)
state.previous_input = new_input
state.previous_layer_inputs = previous_layer_inputs
return state
def _init_cache(self, memory_bank, num_layers):
self.cache = {}
for l in range(num_layers):
layer_cache = {"memory_keys": None, "memory_values": None}
layer_cache["self_keys"] = None
layer_cache["self_values"] = None
self.cache["layer_{}".format(l)] = layer_cache
def repeat_beam_size_times(self, beam_size):
"""Repeat beam_size times along batch dimension."""
self.src = self.src.data.repeat(1, beam_size, 1)
def map_batch_fn(self, fn):
def _recursive_map(struct, batch_dim=0):
for k, v in struct.items():
if v is not None:
if isinstance(v, dict):
_recursive_map(v)
else:
struct[k] = fn(v, batch_dim)
self.src = fn(self.src, 0)
if self.cache is not None:
_recursive_map(self.cache)
def gelu(x):
return 0.5 * x * (1 + torch.tanh(math.sqrt(2 / math.pi) * (x + 0.044715 * torch.pow(x, 3))))
class PositionwiseFeedForward(nn.Module):
"""A two-layer Feed-Forward-Network with residual layer norm.
Args:
d_model (int): the size of input for the first-layer of the FFN.
d_ff (int): the hidden layer size of the second-layer
of the FNN.
dropout (float): dropout probability in :math:`[0, 1)`.
"""
def __init__(self, d_model, d_ff, dropout=0.1):
super().__init__()
self.w_1 = nn.Linear(d_model, d_ff)
self.w_2 = nn.Linear(d_ff, d_model)
self.layer_norm = nn.LayerNorm(d_model, eps=1e-6)
self.actv = gelu
self.dropout_1 = nn.Dropout(dropout)
self.dropout_2 = nn.Dropout(dropout)
def forward(self, x):
inter = self.dropout_1(self.actv(self.w_1(self.layer_norm(x))))
output = self.dropout_2(self.w_2(inter))
return output + x
#
# TRANSLATOR
# The following code is used to generate summaries using the
# pre-trained weights and beam search.
#
def build_predictor(args, tokenizer, symbols, model, logger=None):
# we should be able to refactor the global scorer a lot
scorer = GNMTGlobalScorer(args.alpha, length_penalty="wu")
translator = Translator(args, model, tokenizer, symbols, global_scorer=scorer, logger=logger)
return translator
class GNMTGlobalScorer(object):
"""
NMT re-ranking score from
"Google's Neural Machine Translation System" :cite:`wu2016google`
Args:
alpha (float): length parameter
beta (float): coverage parameter
"""
def __init__(self, alpha, length_penalty):
self.alpha = alpha
penalty_builder = PenaltyBuilder(length_penalty)
self.length_penalty = penalty_builder.length_penalty()
def score(self, beam, logprobs):
"""
Rescores a prediction based on penalty functions
"""
normalized_probs = self.length_penalty(beam, logprobs, self.alpha)
return normalized_probs
class PenaltyBuilder(object):
"""
Returns the Length and Coverage Penalty function for Beam Search.
Args:
length_pen (str): option name of length pen
cov_pen (str): option name of cov pen
"""
def __init__(self, length_pen):
self.length_pen = length_pen
def length_penalty(self):
if self.length_pen == "wu":
return self.length_wu
elif self.length_pen == "avg":
return self.length_average
else:
return self.length_none
"""
Below are all the different penalty terms implemented so far
"""
def length_wu(self, beam, logprobs, alpha=0.0):
"""
NMT length re-ranking score from
"Google's Neural Machine Translation System" :cite:`wu2016google`.
"""
modifier = ((5 + len(beam.next_ys)) ** alpha) / ((5 + 1) ** alpha)
return logprobs / modifier
def length_average(self, beam, logprobs, alpha=0.0):
"""
Returns the average probability of tokens in a sequence.
"""
return logprobs / len(beam.next_ys)
def length_none(self, beam, logprobs, alpha=0.0, beta=0.0):
"""
Returns unmodified scores.
"""
return logprobs
class Translator(object):
"""
Uses a model to translate a batch of sentences.
Args:
model (:obj:`onmt.modules.NMTModel`):
NMT model to use for translation
fields (dict of Fields): data fields
beam_size (int): size of beam to use
n_best (int): number of translations produced
max_length (int): maximum length output to produce
global_scores (:obj:`GlobalScorer`):
object to rescore final translations
copy_attn (bool): use copy attention during translation
beam_trace (bool): trace beam search for debugging
logger(logging.Logger): logger.
"""
def __init__(self, args, model, vocab, symbols, global_scorer=None, logger=None):
self.logger = logger
self.args = args
self.model = model
self.generator = self.model.generator
self.vocab = vocab
self.symbols = symbols
self.start_token = symbols["BOS"]
self.end_token = symbols["EOS"]
self.global_scorer = global_scorer
self.beam_size = args.beam_size
self.min_length = args.min_length
self.max_length = args.max_length
def translate(self, batch, step, attn_debug=False):
"""Generates summaries from one batch of data."""
self.model.eval()
with torch.no_grad():
batch_data = self.translate_batch(batch)
translations = self.from_batch(batch_data)
return translations
def translate_batch(self, batch, fast=False):
"""
Translate a batch of sentences.
Mostly a wrapper around :obj:`Beam`.
Args:
batch (:obj:`Batch`): a batch from a dataset object
fast (bool): enables fast beam search (may not support all features)
"""
with torch.no_grad():
return self._fast_translate_batch(batch, self.max_length, min_length=self.min_length)
# Where the beam search lives
# I have no idea why it is being called from the method above
def _fast_translate_batch(self, batch, max_length, min_length=0):
"""Beam Search using the encoder inputs contained in `batch`."""
# The batch object is funny
# Instead of just looking at the size of the arguments we encapsulate
# a size argument.
# Where is it defined?
beam_size = self.beam_size
batch_size = batch.batch_size
src = batch.src
segs = batch.segs
mask_src = batch.mask_src
src_features = self.model.bert(src, segs, mask_src)
dec_states = self.model.decoder.init_decoder_state(src, src_features, with_cache=True)
device = src_features.device
# Tile states and memory beam_size times.
dec_states.map_batch_fn(lambda state, dim: tile(state, beam_size, dim=dim))
src_features = tile(src_features, beam_size, dim=0)
batch_offset = torch.arange(batch_size, dtype=torch.long, device=device)
beam_offset = torch.arange(0, batch_size * beam_size, step=beam_size, dtype=torch.long, device=device)
alive_seq = torch.full([batch_size * beam_size, 1], self.start_token, dtype=torch.long, device=device)
# Give full probability to the first beam on the first step.
topk_log_probs = torch.tensor([0.0] + [float("-inf")] * (beam_size - 1), device=device).repeat(batch_size)
# Structure that holds finished hypotheses.
hypotheses = [[] for _ in range(batch_size)] # noqa: F812
results = {}
results["predictions"] = [[] for _ in range(batch_size)] # noqa: F812
results["scores"] = [[] for _ in range(batch_size)] # noqa: F812
results["gold_score"] = [0] * batch_size
results["batch"] = batch
for step in range(max_length):
decoder_input = alive_seq[:, -1].view(1, -1)
# Decoder forward.
decoder_input = decoder_input.transpose(0, 1)
dec_out, dec_states = self.model.decoder(decoder_input, src_features, dec_states, step=step)
# Generator forward.
log_probs = self.generator(dec_out.transpose(0, 1).squeeze(0))
vocab_size = log_probs.size(-1)
if step < min_length:
log_probs[:, self.end_token] = -1e20
# Multiply probs by the beam probability.
log_probs += topk_log_probs.view(-1).unsqueeze(1)
alpha = self.global_scorer.alpha
length_penalty = ((5.0 + (step + 1)) / 6.0) ** alpha
# Flatten probs into a list of possibilities.
curr_scores = log_probs / length_penalty
if self.args.block_trigram:
cur_len = alive_seq.size(1)
if cur_len > 3:
for i in range(alive_seq.size(0)):
fail = False
words = [int(w) for w in alive_seq[i]]
words = [self.vocab.ids_to_tokens[w] for w in words]
words = " ".join(words).replace(" ##", "").split()
if len(words) <= 3:
continue
trigrams = [(words[i - 1], words[i], words[i + 1]) for i in range(1, len(words) - 1)]
trigram = tuple(trigrams[-1])
if trigram in trigrams[:-1]:
fail = True
if fail:
curr_scores[i] = -10e20
curr_scores = curr_scores.reshape(-1, beam_size * vocab_size)
topk_scores, topk_ids = curr_scores.topk(beam_size, dim=-1)
# Recover log probs.
topk_log_probs = topk_scores * length_penalty
# Resolve beam origin and true word ids.
topk_beam_index = topk_ids.div(vocab_size)
topk_ids = topk_ids.fmod(vocab_size)
# Map beam_index to batch_index in the flat representation.
batch_index = topk_beam_index + beam_offset[: topk_beam_index.size(0)].unsqueeze(1)
select_indices = batch_index.view(-1)
# Append last prediction.
alive_seq = torch.cat([alive_seq.index_select(0, select_indices), topk_ids.view(-1, 1)], -1)
is_finished = topk_ids.eq(self.end_token)
if step + 1 == max_length:
is_finished.fill_(1)
# End condition is top beam is finished.
end_condition = is_finished[:, 0].eq(1)
# Save finished hypotheses.
if is_finished.any():
predictions = alive_seq.view(-1, beam_size, alive_seq.size(-1))
for i in range(is_finished.size(0)):
b = batch_offset[i]
if end_condition[i]:
is_finished[i].fill_(1)
finished_hyp = is_finished[i].nonzero().view(-1)
# Store finished hypotheses for this batch.
for j in finished_hyp:
hypotheses[b].append((topk_scores[i, j], predictions[i, j, 1:]))
# If the batch reached the end, save the n_best hypotheses.
if end_condition[i]:
best_hyp = sorted(hypotheses[b], key=lambda x: x[0], reverse=True)
score, pred = best_hyp[0]
results["scores"][b].append(score)
results["predictions"][b].append(pred)
non_finished = end_condition.eq(0).nonzero().view(-1)
# If all sentences are translated, no need to go further.
if len(non_finished) == 0:
break
# Remove finished batches for the next step.
topk_log_probs = topk_log_probs.index_select(0, non_finished)
batch_index = batch_index.index_select(0, non_finished)
batch_offset = batch_offset.index_select(0, non_finished)
alive_seq = predictions.index_select(0, non_finished).view(-1, alive_seq.size(-1))
# Reorder states.
select_indices = batch_index.view(-1)
src_features = src_features.index_select(0, select_indices)
dec_states.map_batch_fn(lambda state, dim: state.index_select(dim, select_indices))
return results
def from_batch(self, translation_batch):
batch = translation_batch["batch"]
assert len(translation_batch["gold_score"]) == len(translation_batch["predictions"])
batch_size = batch.batch_size
preds, _, _, tgt_str, src = (
translation_batch["predictions"],
translation_batch["scores"],
translation_batch["gold_score"],
batch.tgt_str,
batch.src,
)
translations = []
for b in range(batch_size):
pred_sents = self.vocab.convert_ids_to_tokens([int(n) for n in preds[b][0]])
pred_sents = " ".join(pred_sents).replace(" ##", "")
gold_sent = " ".join(tgt_str[b].split())
raw_src = [self.vocab.ids_to_tokens[int(t)] for t in src[b]][:500]
raw_src = " ".join(raw_src)
translation = (pred_sents, gold_sent, raw_src)
translations.append(translation)
return translations
def tile(x, count, dim=0):
"""
Tiles x on dimension dim count times.
"""
perm = list(range(len(x.size())))
if dim != 0:
perm[0], perm[dim] = perm[dim], perm[0]
x = x.permute(perm).contiguous()
out_size = list(x.size())
out_size[0] *= count
batch = x.size(0)
x = x.view(batch, -1).transpose(0, 1).repeat(count, 1).transpose(0, 1).contiguous().view(*out_size)
if dim != 0:
x = x.permute(perm).contiguous()
return x
#
# Optimizer for training. We keep this here in case we want to add
# a finetuning script.
#
class BertSumOptimizer(object):
"""Specific optimizer for BertSum.
As described in [1], the authors fine-tune BertSum for abstractive
summarization using two Adam Optimizers with different warm-up steps and
learning rate. They also use a custom learning rate scheduler.
[1] Liu, Yang, and Mirella Lapata. "Text summarization with pretrained encoders."
arXiv preprint arXiv:1908.08345 (2019).
"""
def __init__(self, model, lr, warmup_steps, beta_1=0.99, beta_2=0.999, eps=1e-8):
self.encoder = model.encoder
self.decoder = model.decoder
self.lr = lr
self.warmup_steps = warmup_steps
self.optimizers = {
"encoder": torch.optim.Adam(
model.encoder.parameters(),
lr=lr["encoder"],
betas=(beta_1, beta_2),
eps=eps,
),
"decoder": torch.optim.Adam(
model.decoder.parameters(),
lr=lr["decoder"],
betas=(beta_1, beta_2),
eps=eps,
),
}
self._step = 0
self.current_learning_rates = {}
def _update_rate(self, stack):
return self.lr[stack] * min(self._step ** (-0.5), self._step * self.warmup_steps[stack] ** (-1.5))
def zero_grad(self):
self.optimizer_decoder.zero_grad()
self.optimizer_encoder.zero_grad()
def step(self):
self._step += 1
for stack, optimizer in self.optimizers.items():
new_rate = self._update_rate(stack)
for param_group in optimizer.param_groups:
param_group["lr"] = new_rate
optimizer.step()
self.current_learning_rates[stack] = new_rate
| transformers/examples/research_projects/bertabs/modeling_bertabs.py/0 | {
"file_path": "transformers/examples/research_projects/bertabs/modeling_bertabs.py",
"repo_id": "transformers",
"token_count": 17886
} | 288 |
import json
import multiprocessing
import os
import re
from collections import defaultdict
import torch
from accelerate import Accelerator
from accelerate.utils import set_seed
from arguments import HumanEvalArguments
from datasets import load_dataset, load_metric
from torch.utils.data import IterableDataset
from torch.utils.data.dataloader import DataLoader
from tqdm import tqdm
import transformers
from transformers import AutoModelForCausalLM, AutoTokenizer, HfArgumentParser, StoppingCriteria, StoppingCriteriaList
EOF_STRINGS = ["\nclass", "\ndef", "\n#", "\n@", "\nprint", "\nif"]
class TokenizedDataset(IterableDataset):
"""Tokenize and preprocess the dataset
Multiple copies of the same prompt are sent sequentially.
See compute_code for more details.
"""
def __init__(self, tokenizer, dataset, n_tasks=None, n_copies=1):
self.tokenizer = tokenizer
self.dataset = dataset
self.n_tasks = len(dataset) if n_tasks is None else n_tasks
self.n_copies = n_copies
def __iter__(self):
prompts = []
for task in range(self.n_tasks):
# without strip, the model generate commented codes ...
prompts.append(self.tokenizer.eos_token + self.dataset[task]["prompt"].strip())
outputs = self.tokenizer(prompts, padding=True, return_tensors="pt")
for task in range(self.n_tasks):
for _ in range(self.n_copies):
yield {
"ids": outputs.input_ids[task],
"task_id": task,
"input_len": outputs.attention_mask[task].sum(),
}
class EndOfFunctionCriteria(StoppingCriteria):
"""Custom `StoppingCriteria` which checks if all generated functions in the batch are completed."""
def __init__(self, start_length, eof_strings, tokenizer):
self.start_length = start_length
self.eof_strings = eof_strings
self.tokenizer = tokenizer
def __call__(self, input_ids, scores, **kwargs):
"""Returns true if all generated sequences contain any of the end-of-function strings."""
decoded_generations = self.tokenizer.batch_decode(input_ids[:, self.start_length :])
done = []
for decoded_generation in decoded_generations:
done.append(any(stop_string in decoded_generation for stop_string in self.eof_strings))
return all(done)
def remove_last_block(string):
"""Remove the last block of the code containing EOF_STRINGS"""
string_list = re.split("(%s)" % "|".join(EOF_STRINGS), string)
# last string should be ""
return "".join(string_list[:-2])
def complete_code(accelerator, model, tokenizer, dataloader, n_tasks, batch_size=20, **gen_kwargs):
"""Generate multiple codes for each task in the dataset. This function leverage accelerator to distribute
the processing to multiple GPUs.
dataloader, a wrapper around a TokenizeDataset objectm is supposed to send all the prompts from
the evalution dataset to the modelm as the following:
[p_0_0, p_0_1, ..., p_0_nc-1, p_1_0, ..., p_nt-1_nc-1]
where nc is the number of copies of the prompt, and nt is the number of tasks.
nc is such that num_sample = nc * batch_size
Parameters
----------
accelerator: Accelerator
model: transformers.PreTrainedModel
Code generation model. AutoTokenizer.from_pretrained(model_ckpt), ex model_ckpt = "lvwerra/codeparrot"
tokenizer: transformers.AutoTokenizer
The tokenizer used to train model
dataloader: DataLoader
The dataloader is a wrapper around a TokenizeDataset object. It is designed to be used with multiple GPUs.
n_tasks: int
The number of tasks in the dataset. It is used to determine the length of the output.
Should be aligned with the number of tasks in the TokenizeDataset.
batch_size: int
num_return_sequences per copy of the prompt such that num_sample = batch_size * n_copies
gen_kwargs: dict
Keyword arguments for the generation function of the model.
Returns
-------
code_gens: list of list of str, of length n_tasks
List of generated codes for each task.
Each element is a list of generated codes for each task, with length num_samples
"""
gen_token_dict = defaultdict(list) # dict of list of generated tokens
for step, batch in tqdm(enumerate(dataloader)):
with torch.no_grad():
gen_kwargs["stopping_criteria"][0].start_length = batch["ids"].shape[-1]
generated_tokens = accelerator.unwrap_model(model).generate(
input_ids=batch["ids"][:, : batch["input_len"]], num_return_sequences=batch_size, **gen_kwargs
)
# each task is generated batch_size times
generated_tasks = batch["task_id"].repeat(batch_size)
generated_tokens = accelerator.pad_across_processes(
generated_tokens, dim=1, pad_index=tokenizer.pad_token_id
)
generated_tokens, generated_tasks = accelerator.gather((generated_tokens, generated_tasks))
generated_tokens = generated_tokens.cpu().numpy()
generated_tasks = generated_tasks.cpu().numpy()
for task, generated_tokens in zip(generated_tasks, generated_tokens):
gen_token_dict[task].append(generated_tokens)
code_gens = [[] for _ in range(n_tasks)]
for task, generated_tokens in gen_token_dict.items():
for s in generated_tokens:
gen_code = tokenizer.decode(s, skip_special_tokens=True, clean_up_tokenization_spaces=True)
code_gens[task].append(remove_last_block(gen_code))
return code_gens
def main():
# Setup configuration
parser = HfArgumentParser(HumanEvalArguments)
args = parser.parse_args()
transformers.logging.set_verbosity_error()
# enables code execution in code_eval metric
os.environ["HF_ALLOW_CODE_EVAL"] = args.HF_ALLOW_CODE_EVAL
# make sure tokenizer plays nice with multiprocessing
os.environ["TOKENIZERS_PARALLELISM"] = "false"
if args.num_workers is None:
args.num_workers = multiprocessing.cpu_count()
# Use dataset load to feed to accelerate
accelerator = Accelerator()
set_seed(args.seed, device_specific=True)
# Load model and tokenizer
tokenizer = AutoTokenizer.from_pretrained(args.model_ckpt)
tokenizer.pad_token = tokenizer.eos_token
model = AutoModelForCausalLM.from_pretrained(args.model_ckpt)
# Generation settings
gen_kwargs = {
"do_sample": args.do_sample,
"temperature": args.temperature,
"max_new_tokens": args.max_new_tokens,
"top_p": args.top_p,
"top_k": args.top_k,
"stopping_criteria": StoppingCriteriaList([EndOfFunctionCriteria(0, EOF_STRINGS, tokenizer)]),
}
# Load evaluation dataset and metric
human_eval = load_dataset("openai_humaneval")
code_eval_metric = load_metric("code_eval")
n_tasks = args.num_tasks if args.num_tasks is not None else len(human_eval["test"])
n_copies = args.n_samples // args.batch_size
human_eval_tokenized = TokenizedDataset(tokenizer, human_eval["test"], n_copies=n_copies, n_tasks=n_tasks)
# do not confuse args.batch_size, which is actually the num_return_sequences
human_eval_loader = DataLoader(human_eval_tokenized, batch_size=1)
# Run a quick test to see if code evaluation is enabled
try:
_ = code_eval_metric.compute(references=[""], predictions=[[""]])
except ValueError as exception:
print(
'Code evaluation not enabled. Read the warning below carefully and then use `--HF_ALLOW_CODE_EVAL="1"`'
" flag to enable code evaluation."
)
raise exception
model, human_eval_loader = accelerator.prepare(model, human_eval_loader)
generations = complete_code(
accelerator,
model,
tokenizer,
human_eval_loader,
n_tasks=n_tasks,
batch_size=args.batch_size,
**gen_kwargs,
)
if accelerator.is_main_process:
references = []
for task in tqdm(range(n_tasks)):
test_func = human_eval["test"][task]["test"]
entry_point = f"check({human_eval['test'][task]['entry_point']})"
references.append("\n" + test_func + "\n" + entry_point)
# Evaluate completions with "code_eval" metric
pass_at_k, _ = code_eval_metric.compute(
references=references, predictions=generations, num_workers=args.num_workers
)
print(f"Results: {pass_at_k}")
# Save results to json file
with open(args.output_file, "w") as fp:
json.dump(pass_at_k, fp)
# For some reason the folliwng seems to be necessary sometimes for code_eval to work nice with multiprocessing
# https://stackoverflow.com/questions/60804599/python-multiprocessing-keeps-spawning-the-whole-script
if __name__ == "__main__":
main()
| transformers/examples/research_projects/codeparrot/scripts/human_eval.py/0 | {
"file_path": "transformers/examples/research_projects/codeparrot/scripts/human_eval.py",
"repo_id": "transformers",
"token_count": 3551
} | 289 |
import torch
from torch import nn
from torch.nn import CrossEntropyLoss, MSELoss
from transformers.file_utils import add_start_docstrings, add_start_docstrings_to_model_forward
from transformers.models.bert.modeling_bert import (
BERT_INPUTS_DOCSTRING,
BERT_START_DOCSTRING,
BertEmbeddings,
BertLayer,
BertPooler,
BertPreTrainedModel,
)
def entropy(x):
"""Calculate entropy of a pre-softmax logit Tensor"""
exp_x = torch.exp(x)
A = torch.sum(exp_x, dim=1) # sum of exp(x_i)
B = torch.sum(x * exp_x, dim=1) # sum of x_i * exp(x_i)
return torch.log(A) - B / A
class DeeBertEncoder(nn.Module):
def __init__(self, config):
super().__init__()
self.output_attentions = config.output_attentions
self.output_hidden_states = config.output_hidden_states
self.layer = nn.ModuleList([BertLayer(config) for _ in range(config.num_hidden_layers)])
self.highway = nn.ModuleList([BertHighway(config) for _ in range(config.num_hidden_layers)])
self.early_exit_entropy = [-1 for _ in range(config.num_hidden_layers)]
def set_early_exit_entropy(self, x):
if isinstance(x, (float, int)):
for i in range(len(self.early_exit_entropy)):
self.early_exit_entropy[i] = x
else:
self.early_exit_entropy = x
def init_highway_pooler(self, pooler):
loaded_model = pooler.state_dict()
for highway in self.highway:
for name, param in highway.pooler.state_dict().items():
param.copy_(loaded_model[name])
def forward(
self,
hidden_states,
attention_mask=None,
head_mask=None,
encoder_hidden_states=None,
encoder_attention_mask=None,
):
all_hidden_states = ()
all_attentions = ()
all_highway_exits = ()
for i, layer_module in enumerate(self.layer):
if self.output_hidden_states:
all_hidden_states = all_hidden_states + (hidden_states,)
layer_outputs = layer_module(
hidden_states, attention_mask, head_mask[i], encoder_hidden_states, encoder_attention_mask
)
hidden_states = layer_outputs[0]
if self.output_attentions:
all_attentions = all_attentions + (layer_outputs[1],)
current_outputs = (hidden_states,)
if self.output_hidden_states:
current_outputs = current_outputs + (all_hidden_states,)
if self.output_attentions:
current_outputs = current_outputs + (all_attentions,)
highway_exit = self.highway[i](current_outputs)
# logits, pooled_output
if not self.training:
highway_logits = highway_exit[0]
highway_entropy = entropy(highway_logits)
highway_exit = highway_exit + (highway_entropy,) # logits, hidden_states(?), entropy
all_highway_exits = all_highway_exits + (highway_exit,)
if highway_entropy < self.early_exit_entropy[i]:
new_output = (highway_logits,) + current_outputs[1:] + (all_highway_exits,)
raise HighwayException(new_output, i + 1)
else:
all_highway_exits = all_highway_exits + (highway_exit,)
# Add last layer
if self.output_hidden_states:
all_hidden_states = all_hidden_states + (hidden_states,)
outputs = (hidden_states,)
if self.output_hidden_states:
outputs = outputs + (all_hidden_states,)
if self.output_attentions:
outputs = outputs + (all_attentions,)
outputs = outputs + (all_highway_exits,)
return outputs # last-layer hidden state, (all hidden states), (all attentions), all highway exits
@add_start_docstrings(
"The Bert Model transformer with early exiting (DeeBERT). ",
BERT_START_DOCSTRING,
)
class DeeBertModel(BertPreTrainedModel):
def __init__(self, config):
super().__init__(config)
self.config = config
self.embeddings = BertEmbeddings(config)
self.encoder = DeeBertEncoder(config)
self.pooler = BertPooler(config)
self.init_weights()
def init_highway_pooler(self):
self.encoder.init_highway_pooler(self.pooler)
def get_input_embeddings(self):
return self.embeddings.word_embeddings
def set_input_embeddings(self, value):
self.embeddings.word_embeddings = value
def _prune_heads(self, heads_to_prune):
"""Prunes heads of the model.
heads_to_prune: dict of {layer_num: list of heads to prune in this layer}
See base class PreTrainedModel
"""
for layer, heads in heads_to_prune.items():
self.encoder.layer[layer].attention.prune_heads(heads)
@add_start_docstrings_to_model_forward(BERT_INPUTS_DOCSTRING)
def forward(
self,
input_ids=None,
attention_mask=None,
token_type_ids=None,
position_ids=None,
head_mask=None,
inputs_embeds=None,
encoder_hidden_states=None,
encoder_attention_mask=None,
):
r"""
Return:
:obj:`tuple(torch.FloatTensor)` comprising various elements depending on the configuration (:class:`~transformers.BertConfig`) and inputs:
last_hidden_state (:obj:`torch.FloatTensor` of shape :obj:`(batch_size, sequence_length, hidden_size)`):
Sequence of hidden-states at the output of the last layer of the model.
pooler_output (:obj:`torch.FloatTensor`: of shape :obj:`(batch_size, hidden_size)`):
Last layer hidden-state of the first token of the sequence (classification token)
further processed by a Linear layer and a Tanh activation function. The Linear
layer weights are trained from the next sentence prediction (classification)
objective during pre-training.
This output is usually *not* a good summary
of the semantic content of the input, you're often better with averaging or pooling
the sequence of hidden-states for the whole input sequence.
hidden_states (:obj:`tuple(torch.FloatTensor)`, `optional`, returned when ``output_hidden_states=True`` is passed or when ``config.output_hidden_states=True``):
Tuple of :obj:`torch.FloatTensor` (one for the output of the embeddings + one for the output of each layer)
of shape :obj:`(batch_size, sequence_length, hidden_size)`.
Hidden-states of the model at the output of each layer plus the initial embedding outputs.
attentions (:obj:`tuple(torch.FloatTensor)`, `optional`, returned when ``output_attentions=True`` is passed or when ``config.output_attentions=True``):
Tuple of :obj:`torch.FloatTensor` (one for each layer) of shape
:obj:`(batch_size, num_heads, sequence_length, sequence_length)`.
Attentions weights after the attention softmax, used to compute the weighted average in the self-attention
heads.
highway_exits (:obj:`tuple(tuple(torch.Tensor))`:
Tuple of each early exit's results (total length: number of layers)
Each tuple is again, a tuple of length 2 - the first entry is logits and the second entry is hidden states.
"""
if input_ids is not None and inputs_embeds is not None:
raise ValueError("You cannot specify both input_ids and inputs_embeds at the same time")
elif input_ids is not None:
input_shape = input_ids.size()
elif inputs_embeds is not None:
input_shape = inputs_embeds.size()[:-1]
else:
raise ValueError("You have to specify either input_ids or inputs_embeds")
device = input_ids.device if input_ids is not None else inputs_embeds.device
if attention_mask is None:
attention_mask = torch.ones(input_shape, device=device)
if encoder_attention_mask is None:
encoder_attention_mask = torch.ones(input_shape, device=device)
if token_type_ids is None:
token_type_ids = torch.zeros(input_shape, dtype=torch.long, device=device)
# We can provide a self-attention mask of dimensions [batch_size, from_seq_length, to_seq_length]
# ourselves in which case we just need to make it broadcastable to all heads.
extended_attention_mask: torch.Tensor = self.get_extended_attention_mask(attention_mask, input_shape, device)
# If a 2D ou 3D attention mask is provided for the cross-attention
# we need to make broadcastable to [batch_size, num_heads, seq_length, seq_length]
if encoder_attention_mask.dim() == 3:
encoder_extended_attention_mask = encoder_attention_mask[:, None, :, :]
if encoder_attention_mask.dim() == 2:
encoder_extended_attention_mask = encoder_attention_mask[:, None, None, :]
encoder_extended_attention_mask = encoder_extended_attention_mask.to(
dtype=next(self.parameters()).dtype
) # fp16 compatibility
encoder_extended_attention_mask = (1.0 - encoder_extended_attention_mask) * -10000.0
# Prepare head mask if needed
# 1.0 in head_mask indicate we keep the head
# attention_probs has shape bsz x n_heads x N x N
# input head_mask has shape [num_heads] or [num_hidden_layers x num_heads]
# and head_mask is converted to shape [num_hidden_layers x batch x num_heads x seq_length x seq_length]
head_mask = self.get_head_mask(head_mask, self.config.num_hidden_layers)
embedding_output = self.embeddings(
input_ids=input_ids, position_ids=position_ids, token_type_ids=token_type_ids, inputs_embeds=inputs_embeds
)
encoder_outputs = self.encoder(
embedding_output,
attention_mask=extended_attention_mask,
head_mask=head_mask,
encoder_hidden_states=encoder_hidden_states,
encoder_attention_mask=encoder_extended_attention_mask,
)
sequence_output = encoder_outputs[0]
pooled_output = self.pooler(sequence_output)
outputs = (
sequence_output,
pooled_output,
) + encoder_outputs[1:] # add hidden_states and attentions if they are here
return outputs # sequence_output, pooled_output, (hidden_states), (attentions), highway exits
class HighwayException(Exception):
def __init__(self, message, exit_layer):
self.message = message
self.exit_layer = exit_layer # start from 1!
class BertHighway(nn.Module):
"""A module to provide a shortcut
from (the output of one non-final BertLayer in BertEncoder) to (cross-entropy computation in BertForSequenceClassification)
"""
def __init__(self, config):
super().__init__()
self.pooler = BertPooler(config)
self.dropout = nn.Dropout(config.hidden_dropout_prob)
self.classifier = nn.Linear(config.hidden_size, config.num_labels)
def forward(self, encoder_outputs):
# Pooler
pooler_input = encoder_outputs[0]
pooler_output = self.pooler(pooler_input)
# "return" pooler_output
# BertModel
bmodel_output = (pooler_input, pooler_output) + encoder_outputs[1:]
# "return" bmodel_output
# Dropout and classification
pooled_output = bmodel_output[1]
pooled_output = self.dropout(pooled_output)
logits = self.classifier(pooled_output)
return logits, pooled_output
@add_start_docstrings(
"""Bert Model (with early exiting - DeeBERT) with a classifier on top,
also takes care of multi-layer training. """,
BERT_START_DOCSTRING,
)
class DeeBertForSequenceClassification(BertPreTrainedModel):
def __init__(self, config):
super().__init__(config)
self.num_labels = config.num_labels
self.num_layers = config.num_hidden_layers
self.bert = DeeBertModel(config)
self.dropout = nn.Dropout(config.hidden_dropout_prob)
self.classifier = nn.Linear(config.hidden_size, self.config.num_labels)
self.init_weights()
@add_start_docstrings_to_model_forward(BERT_INPUTS_DOCSTRING)
def forward(
self,
input_ids=None,
attention_mask=None,
token_type_ids=None,
position_ids=None,
head_mask=None,
inputs_embeds=None,
labels=None,
output_layer=-1,
train_highway=False,
):
r"""
labels (:obj:`torch.LongTensor` of shape :obj:`(batch_size,)`, `optional`):
Labels for computing the sequence classification/regression loss.
Indices should be in :obj:`[0, ..., config.num_labels - 1]`.
If :obj:`config.num_labels == 1` a regression loss is computed (Mean-Square loss),
If :obj:`config.num_labels > 1` a classification loss is computed (Cross-Entropy).
Returns:
:obj:`tuple(torch.FloatTensor)` comprising various elements depending on the configuration (:class:`~transformers.BertConfig`) and inputs:
loss (:obj:`torch.FloatTensor` of shape :obj:`(1,)`, `optional`, returned when :obj:`label` is provided):
Classification (or regression if config.num_labels==1) loss.
logits (:obj:`torch.FloatTensor` of shape :obj:`(batch_size, config.num_labels)`):
Classification (or regression if config.num_labels==1) scores (before SoftMax).
hidden_states (:obj:`tuple(torch.FloatTensor)`, `optional`, returned when ``output_hidden_states=True`` is passed or when ``config.output_hidden_states=True``):
Tuple of :obj:`torch.FloatTensor` (one for the output of the embeddings + one for the output of each layer)
of shape :obj:`(batch_size, sequence_length, hidden_size)`.
Hidden-states of the model at the output of each layer plus the initial embedding outputs.
attentions (:obj:`tuple(torch.FloatTensor)`, `optional`, returned when ``output_attentions=True`` is passed or when ``config.output_attentions=True``):
Tuple of :obj:`torch.FloatTensor` (one for each layer) of shape
:obj:`(batch_size, num_heads, sequence_length, sequence_length)`.
Attentions weights after the attention softmax, used to compute the weighted average in the self-attention
heads.
highway_exits (:obj:`tuple(tuple(torch.Tensor))`:
Tuple of each early exit's results (total length: number of layers)
Each tuple is again, a tuple of length 2 - the first entry is logits and the second entry is hidden states.
"""
exit_layer = self.num_layers
try:
outputs = self.bert(
input_ids,
attention_mask=attention_mask,
token_type_ids=token_type_ids,
position_ids=position_ids,
head_mask=head_mask,
inputs_embeds=inputs_embeds,
)
# sequence_output, pooled_output, (hidden_states), (attentions), highway exits
pooled_output = outputs[1]
pooled_output = self.dropout(pooled_output)
logits = self.classifier(pooled_output)
outputs = (logits,) + outputs[2:] # add hidden states and attention if they are here
except HighwayException as e:
outputs = e.message
exit_layer = e.exit_layer
logits = outputs[0]
if not self.training:
original_entropy = entropy(logits)
highway_entropy = []
highway_logits_all = []
if labels is not None:
if self.num_labels == 1:
# We are doing regression
loss_fct = MSELoss()
loss = loss_fct(logits.view(-1), labels.view(-1))
else:
loss_fct = CrossEntropyLoss()
loss = loss_fct(logits.view(-1, self.num_labels), labels.view(-1))
# work with highway exits
highway_losses = []
for highway_exit in outputs[-1]:
highway_logits = highway_exit[0]
if not self.training:
highway_logits_all.append(highway_logits)
highway_entropy.append(highway_exit[2])
if self.num_labels == 1:
# We are doing regression
loss_fct = MSELoss()
highway_loss = loss_fct(highway_logits.view(-1), labels.view(-1))
else:
loss_fct = CrossEntropyLoss()
highway_loss = loss_fct(highway_logits.view(-1, self.num_labels), labels.view(-1))
highway_losses.append(highway_loss)
if train_highway:
outputs = (sum(highway_losses[:-1]),) + outputs
# exclude the final highway, of course
else:
outputs = (loss,) + outputs
if not self.training:
outputs = outputs + ((original_entropy, highway_entropy), exit_layer)
if output_layer >= 0:
outputs = (
(outputs[0],) + (highway_logits_all[output_layer],) + outputs[2:]
) # use the highway of the last layer
return outputs # (loss), logits, (hidden_states), (attentions), (highway_exits)
| transformers/examples/research_projects/deebert/src/modeling_highway_bert.py/0 | {
"file_path": "transformers/examples/research_projects/deebert/src/modeling_highway_bert.py",
"repo_id": "transformers",
"token_count": 7800
} | 290 |
#!/usr/bin/env python
# coding=utf-8
# Copyright 2022 The HuggingFace Inc. team. All rights reserved.
#
# Licensed under the Apache License, Version 2.0 (the "License");
# you may not use this file except in compliance with the License.
# You may obtain a copy of the License at
#
# http://www.apache.org/licenses/LICENSE-2.0
#
# Unless required by applicable law or agreed to in writing, software
# distributed under the License is distributed on an "AS IS" BASIS,
# WITHOUT WARRANTIES OR CONDITIONS OF ANY KIND, either express or implied.
# See the License for the specific language governing permissions and
# limitations under the License.
"""
Fine-tuning (m)LUKE model on token classification tasks (NER, POS, CHUNKS) relying on the accelerate library ๐ค
without using a Trainer.
"""
import argparse
import logging
import math
import os
import random
from pathlib import Path
import datasets
import torch
from accelerate import Accelerator, DistributedDataParallelKwargs
from datasets import ClassLabel, load_dataset, load_metric
from huggingface_hub import Repository, create_repo
from luke_utils import DataCollatorForLukeTokenClassification, is_punctuation, padding_tensor
from torch.utils.data import DataLoader
from tqdm.auto import tqdm
import transformers
from transformers import (
AdamW,
LukeConfig,
LukeForEntitySpanClassification,
LukeTokenizer,
SchedulerType,
default_data_collator,
get_scheduler,
set_seed,
)
from transformers.utils.versions import require_version
logger = logging.getLogger(__name__)
require_version("datasets>=1.8.0", "To fix: pip install -r examples/pytorch/token-classification/requirements.txt")
def parse_args():
parser = argparse.ArgumentParser(
description="Finetune (m)LUKE on a token classification task (such as NER) with the accelerate library"
)
parser.add_argument(
"--dataset_name",
type=str,
default=None,
help="The name of the dataset to use (via the datasets library).",
)
parser.add_argument(
"--dataset_config_name",
type=str,
default=None,
help="The configuration name of the dataset to use (via the datasets library).",
)
parser.add_argument(
"--train_file", type=str, default=None, help="A csv or a json file containing the training data."
)
parser.add_argument(
"--validation_file", type=str, default=None, help="A csv or a json file containing the validation data."
)
parser.add_argument(
"--text_column_name",
type=str,
default=None,
help="The column name of text to input in the file (a csv or JSON file).",
)
parser.add_argument(
"--label_column_name",
type=str,
default=None,
help="The column name of label to input in the file (a csv or JSON file).",
)
parser.add_argument(
"--max_length",
type=int,
default=128,
help=(
"The maximum total input sequence length after tokenization. Sequences longer than this will be truncated,"
" sequences shorter will be padded if `--pad_to_max_length` is passed."
),
)
parser.add_argument(
"--max_entity_length",
type=int,
default=32,
help=(
"The maximum total input entity length after tokenization (Used only for (M)Luke models). Sequences longer"
" than this will be truncated, sequences shorter will be padded if `--pad_to_max_length` is passed."
),
)
parser.add_argument(
"--max_mention_length",
type=int,
default=30,
help=(
"The maximum total input mention length after tokenization (Used only for (M)Luke models). Sequences"
" longer than this will be truncated, sequences shorter will be padded if `--pad_to_max_length` is passed."
),
)
parser.add_argument(
"--pad_to_max_length",
action="store_true",
help="If passed, pad all samples to `max_length`. Otherwise, dynamic padding is used.",
)
parser.add_argument(
"--model_name_or_path",
type=str,
help="Path to pretrained model or model identifier from huggingface.co/models.",
required=True,
)
parser.add_argument(
"--config_name",
type=str,
default=None,
help="Pretrained config name or path if not the same as model_name",
)
parser.add_argument(
"--tokenizer_name",
type=str,
default=None,
help="Pretrained tokenizer name or path if not the same as model_name",
)
parser.add_argument(
"--per_device_train_batch_size",
type=int,
default=8,
help="Batch size (per device) for the training dataloader.",
)
parser.add_argument(
"--per_device_eval_batch_size",
type=int,
default=8,
help="Batch size (per device) for the evaluation dataloader.",
)
parser.add_argument(
"--learning_rate",
type=float,
default=5e-5,
help="Initial learning rate (after the potential warmup period) to use.",
)
parser.add_argument("--weight_decay", type=float, default=0.0, help="Weight decay to use.")
parser.add_argument("--num_train_epochs", type=int, default=3, help="Total number of training epochs to perform.")
parser.add_argument(
"--max_train_steps",
type=int,
default=None,
help="Total number of training steps to perform. If provided, overrides num_train_epochs.",
)
parser.add_argument(
"--gradient_accumulation_steps",
type=int,
default=1,
help="Number of updates steps to accumulate before performing a backward/update pass.",
)
parser.add_argument(
"--lr_scheduler_type",
type=SchedulerType,
default="linear",
help="The scheduler type to use.",
choices=["linear", "cosine", "cosine_with_restarts", "polynomial", "constant", "constant_with_warmup"],
)
parser.add_argument(
"--num_warmup_steps", type=int, default=0, help="Number of steps for the warmup in the lr scheduler."
)
parser.add_argument("--output_dir", type=str, default=None, help="Where to store the final model.")
parser.add_argument("--seed", type=int, default=None, help="A seed for reproducible training.")
parser.add_argument(
"--label_all_tokens",
action="store_true",
help="Setting labels of all special tokens to -100 and thus PyTorch will ignore them.",
)
parser.add_argument(
"--return_entity_level_metrics",
action="store_true",
help="Indication whether entity level metrics are to be returner.",
)
parser.add_argument(
"--task_name",
type=str,
default="ner",
choices=["ner", "pos", "chunk"],
help="The name of the task.",
)
parser.add_argument(
"--debug",
action="store_true",
help="Activate debug mode and run training only with a subset of data.",
)
parser.add_argument("--push_to_hub", action="store_true", help="Whether or not to push the model to the Hub.")
parser.add_argument(
"--hub_model_id", type=str, help="The name of the repository to keep in sync with the local `output_dir`."
)
parser.add_argument("--hub_token", type=str, help="The token to use to push to the Model Hub.")
args = parser.parse_args()
# Sanity checks
if args.task_name is None and args.train_file is None and args.validation_file is None:
raise ValueError("Need either a task name or a training/validation file.")
else:
if args.train_file is not None:
extension = args.train_file.split(".")[-1]
assert extension in ["csv", "json"], "`train_file` should be a csv or a json file."
if args.validation_file is not None:
extension = args.validation_file.split(".")[-1]
assert extension in ["csv", "json"], "`validation_file` should be a csv or a json file."
if args.push_to_hub:
assert args.output_dir is not None, "Need an `output_dir` to create a repo when `--push_to_hub` is passed."
return args
def main():
args = parse_args()
# Initialize the accelerator. We will let the accelerator handle device placement for us in this example.
handler = DistributedDataParallelKwargs(find_unused_parameters=True)
accelerator = Accelerator(kwargs_handlers=[handler])
# Make one log on every process with the configuration for debugging.
logging.basicConfig(
format="%(asctime)s - %(levelname)s - %(name)s - %(message)s",
datefmt="%m/%d/%Y %H:%M:%S",
level=logging.INFO,
)
logger.info(accelerator.state)
# Setup logging, we only want one process per machine to log things on the screen.
# accelerator.is_local_main_process is only True for one process per machine.
logger.setLevel(logging.INFO if accelerator.is_local_main_process else logging.ERROR)
if accelerator.is_local_main_process:
datasets.utils.logging.set_verbosity_warning()
transformers.utils.logging.set_verbosity_info()
else:
datasets.utils.logging.set_verbosity_error()
transformers.utils.logging.set_verbosity_error()
# If passed along, set the training seed now.
if args.seed is not None:
set_seed(args.seed)
# Handle the repository creation
if accelerator.is_main_process:
if args.push_to_hub:
# Retrieve of infer repo_name
repo_name = args.hub_model_id
if repo_name is None:
repo_name = Path(args.output_dir).absolute().name
# Create repo and retrieve repo_id
repo_id = create_repo(repo_name, exist_ok=True, token=args.hub_token).repo_id
# Clone repo locally
repo = Repository(args.output_dir, clone_from=repo_id, token=args.hub_token)
elif args.output_dir is not None:
os.makedirs(args.output_dir, exist_ok=True)
accelerator.wait_for_everyone()
# Get the datasets: you can either provide your own CSV/JSON/TXT training and evaluation files (see below)
# or just provide the name of one of the public datasets for token classification task available on the hub at https://huggingface.co/datasets/
# (the dataset will be downloaded automatically from the datasets Hub).
#
# For CSV/JSON files, this script will use the column called 'tokens' or the first column if no column called
# 'tokens' is found. You can easily tweak this behavior (see below).
#
# In distributed training, the load_dataset function guarantee that only one local process can concurrently
# download the dataset.
if args.dataset_name is not None:
# Downloading and loading a dataset from the hub.
raw_datasets = load_dataset(args.dataset_name, args.dataset_config_name)
else:
data_files = {}
if args.train_file is not None:
data_files["train"] = args.train_file
extension = args.train_file.split(".")[-1]
if args.validation_file is not None:
data_files["validation"] = args.validation_file
extension = args.validation_file.split(".")[-1]
raw_datasets = load_dataset(extension, data_files=data_files)
# Trim a number of training examples
if args.debug:
for split in raw_datasets.keys():
raw_datasets[split] = raw_datasets[split].select(range(100))
# See more about loading any type of standard or custom dataset (from files, python dict, pandas DataFrame, etc) at
# https://huggingface.co/docs/datasets/loading_datasets.
if raw_datasets["train"] is not None:
column_names = raw_datasets["train"].column_names
features = raw_datasets["train"].features
else:
column_names = raw_datasets["validation"].column_names
features = raw_datasets["validation"].features
if args.text_column_name is not None:
text_column_name = args.text_column_name
elif "tokens" in column_names:
text_column_name = "tokens"
else:
text_column_name = column_names[0]
if args.label_column_name is not None:
label_column_name = args.label_column_name
elif f"{args.task_name}_tags" in column_names:
label_column_name = f"{args.task_name}_tags"
else:
label_column_name = column_names[1]
# In the event the labels are not a `Sequence[ClassLabel]`, we will need to go through the dataset to get the
# unique labels.
def get_label_list(labels):
unique_labels = set()
for label in labels:
unique_labels = unique_labels | set(label)
label_list = list(unique_labels)
label_list.sort()
return label_list
if isinstance(features[label_column_name].feature, ClassLabel):
label_list = features[label_column_name].feature.names
# No need to convert the labels since they are already ints.
else:
label_list = get_label_list(raw_datasets["train"][label_column_name])
num_labels = len(label_list)
# Map that sends B-Xxx label to its I-Xxx counterpart
b_to_i_label = []
for idx, label in enumerate(label_list):
if label.startswith("B-") and label.replace("B-", "I-") in label_list:
b_to_i_label.append(label_list.index(label.replace("B-", "I-")))
else:
b_to_i_label.append(idx)
# Load pretrained model and tokenizer
#
# In distributed training, the .from_pretrained methods guarantee that only one local process can concurrently
# download model & vocab.
if args.config_name:
config = LukeConfig.from_pretrained(args.config_name, num_labels=num_labels)
elif args.model_name_or_path:
config = LukeConfig.from_pretrained(args.model_name_or_path, num_labels=num_labels)
else:
logger.warning("You are instantiating a new config instance from scratch.")
tokenizer_name_or_path = args.tokenizer_name if args.tokenizer_name else args.model_name_or_path
if not tokenizer_name_or_path:
raise ValueError(
"You are instantiating a new tokenizer from scratch. This is not supported by this script. "
"You can do it from another script, save it, and load it from here, using --tokenizer_name."
)
tokenizer = LukeTokenizer.from_pretrained(
tokenizer_name_or_path,
use_fast=False,
task="entity_span_classification",
max_entity_length=args.max_entity_length,
max_mention_length=args.max_mention_length,
)
if args.model_name_or_path:
model = LukeForEntitySpanClassification.from_pretrained(
args.model_name_or_path,
from_tf=bool(".ckpt" in args.model_name_or_path),
config=config,
)
else:
logger.info("Training new model from scratch")
model = LukeForEntitySpanClassification.from_config(config)
model.resize_token_embeddings(len(tokenizer))
# Preprocessing the datasets.
# First we tokenize all the texts.
padding = "max_length" if args.pad_to_max_length else False
def compute_sentence_boundaries_for_luke(examples):
sentence_boundaries = []
for tokens in examples[text_column_name]:
sentence_boundaries.append([0, len(tokens)])
examples["sentence_boundaries"] = sentence_boundaries
return examples
def compute_entity_spans_for_luke(examples):
all_entity_spans = []
texts = []
all_labels_entity_spans = []
all_original_entity_spans = []
for labels, tokens, sentence_boundaries in zip(
examples[label_column_name], examples[text_column_name], examples["sentence_boundaries"]
):
subword_lengths = [len(tokenizer.tokenize(token)) for token in tokens]
total_subword_length = sum(subword_lengths)
_, context_end = sentence_boundaries
if total_subword_length > args.max_length - 2:
cur_length = sum(subword_lengths[:context_end])
idx = context_end - 1
while cur_length > args.max_length - 2:
cur_length -= subword_lengths[idx]
context_end -= 1
idx -= 1
text = ""
sentence_words = tokens[:context_end]
sentence_subword_lengths = subword_lengths[:context_end]
word_start_char_positions = []
word_end_char_positions = []
labels_positions = {}
for word, label in zip(sentence_words, labels):
if word[0] == "'" or (len(word) == 1 and is_punctuation(word)):
text = text.rstrip()
word_start_char_positions.append(len(text))
text += word
word_end_char_positions.append(len(text))
text += " "
labels_positions[(word_start_char_positions[-1], word_end_char_positions[-1])] = label
text = text.rstrip()
texts.append(text)
entity_spans = []
labels_entity_spans = []
original_entity_spans = []
for word_start in range(len(sentence_words)):
for word_end in range(word_start, len(sentence_words)):
if (
sum(sentence_subword_lengths[word_start:word_end]) <= tokenizer.max_mention_length
and len(entity_spans) < tokenizer.max_entity_length
):
entity_spans.append((word_start_char_positions[word_start], word_end_char_positions[word_end]))
original_entity_spans.append((word_start, word_end + 1))
if (
word_start_char_positions[word_start],
word_end_char_positions[word_end],
) in labels_positions:
labels_entity_spans.append(
labels_positions[
(word_start_char_positions[word_start], word_end_char_positions[word_end])
]
)
else:
labels_entity_spans.append(0)
all_entity_spans.append(entity_spans)
all_labels_entity_spans.append(labels_entity_spans)
all_original_entity_spans.append(original_entity_spans)
examples["entity_spans"] = all_entity_spans
examples["text"] = texts
examples["labels_entity_spans"] = all_labels_entity_spans
examples["original_entity_spans"] = all_original_entity_spans
return examples
def tokenize_and_align_labels(examples):
entity_spans = []
for v in examples["entity_spans"]:
entity_spans.append(list(map(tuple, v)))
tokenized_inputs = tokenizer(
examples["text"],
entity_spans=entity_spans,
max_length=args.max_length,
padding=padding,
truncation=True,
)
if padding == "max_length":
tokenized_inputs["labels"] = padding_tensor(
examples["labels_entity_spans"], -100, tokenizer.padding_side, tokenizer.max_entity_length
)
tokenized_inputs["original_entity_spans"] = padding_tensor(
examples["original_entity_spans"], (-1, -1), tokenizer.padding_side, tokenizer.max_entity_length
)
tokenized_inputs[label_column_name] = padding_tensor(
examples[label_column_name], -1, tokenizer.padding_side, tokenizer.max_entity_length
)
else:
tokenized_inputs["labels"] = [ex[: tokenizer.max_entity_length] for ex in examples["labels_entity_spans"]]
tokenized_inputs["original_entity_spans"] = [
ex[: tokenizer.max_entity_length] for ex in examples["original_entity_spans"]
]
tokenized_inputs[label_column_name] = [
ex[: tokenizer.max_entity_length] for ex in examples[label_column_name]
]
return tokenized_inputs
with accelerator.main_process_first():
raw_datasets = raw_datasets.map(
compute_sentence_boundaries_for_luke,
batched=True,
desc="Adding sentence boundaries",
)
raw_datasets = raw_datasets.map(
compute_entity_spans_for_luke,
batched=True,
desc="Adding sentence spans",
)
processed_raw_datasets = raw_datasets.map(
tokenize_and_align_labels,
batched=True,
remove_columns=raw_datasets["train"].column_names,
desc="Running tokenizer on dataset",
)
train_dataset = processed_raw_datasets["train"]
eval_dataset = processed_raw_datasets["validation"]
# Log a few random samples from the training set:
for index in random.sample(range(len(train_dataset)), 3):
logger.info(f"Sample {index} of the training set: {train_dataset[index]}.")
# DataLoaders creation:
if args.pad_to_max_length:
# If padding was already done ot max length, we use the default data collator that will just convert everything
# to tensors.
data_collator = default_data_collator
else:
# Otherwise, `DataCollatorForTokenClassification` will apply dynamic padding for us (by padding to the maximum length of
# the samples passed). When using mixed precision, we add `pad_to_multiple_of=8` to pad all tensors to multiple
# of 8s, which will enable the use of Tensor Cores on NVIDIA hardware with compute capability >= 7.5 (Volta).
data_collator = DataCollatorForLukeTokenClassification(
tokenizer, pad_to_multiple_of=(8 if accelerator.use_fp16 else None)
)
train_dataloader = DataLoader(
train_dataset, shuffle=True, collate_fn=data_collator, batch_size=args.per_device_train_batch_size
)
eval_dataloader = DataLoader(eval_dataset, collate_fn=data_collator, batch_size=args.per_device_eval_batch_size)
# Optimizer
# Split weights in two groups, one with weight decay and the other not.
no_decay = ["bias", "LayerNorm.weight"]
optimizer_grouped_parameters = [
{
"params": [p for n, p in model.named_parameters() if not any(nd in n for nd in no_decay)],
"weight_decay": args.weight_decay,
},
{
"params": [p for n, p in model.named_parameters() if any(nd in n for nd in no_decay)],
"weight_decay": 0.0,
},
]
optimizer = AdamW(optimizer_grouped_parameters, lr=args.learning_rate)
# Use the device given by the `accelerator` object.
device = accelerator.device
model.to(device)
# Prepare everything with our `accelerator`.
model, optimizer, train_dataloader, eval_dataloader = accelerator.prepare(
model, optimizer, train_dataloader, eval_dataloader
)
# Note -> the training dataloader needs to be prepared before we grab his length below (cause its length will be
# shorter in multiprocess)
# Scheduler and math around the number of training steps.
num_update_steps_per_epoch = math.ceil(len(train_dataloader) / args.gradient_accumulation_steps)
if args.max_train_steps is None:
args.max_train_steps = args.num_train_epochs * num_update_steps_per_epoch
else:
args.num_train_epochs = math.ceil(args.max_train_steps / num_update_steps_per_epoch)
lr_scheduler = get_scheduler(
name=args.lr_scheduler_type,
optimizer=optimizer,
num_warmup_steps=args.num_warmup_steps,
num_training_steps=args.max_train_steps,
)
# Metrics
metric = load_metric("seqeval")
def get_luke_labels(outputs, ner_tags, original_entity_spans):
true_predictions = []
true_labels = []
for output, original_spans, tags in zip(outputs.logits, original_entity_spans, ner_tags):
true_tags = [val for val in tags if val != -1]
true_original_spans = [val for val in original_spans if val != (-1, -1)]
max_indices = torch.argmax(output, axis=1)
max_logits = torch.max(output, axis=1).values
predictions = []
for logit, index, span in zip(max_logits, max_indices, true_original_spans):
if index != 0:
predictions.append((logit, span, label_list[index]))
predicted_sequence = [label_list[0]] * len(true_tags)
for _, span, label in sorted(predictions, key=lambda o: o[0], reverse=True):
if all(o == label_list[0] for o in predicted_sequence[span[0] : span[1]]):
predicted_sequence[span[0]] = label
if span[1] - span[0] > 1:
predicted_sequence[span[0] + 1 : span[1]] = [label] * (span[1] - span[0] - 1)
true_predictions.append(predicted_sequence)
true_labels.append([label_list[tag_id] for tag_id in true_tags])
return true_predictions, true_labels
def compute_metrics():
results = metric.compute()
if args.return_entity_level_metrics:
# Unpack nested dictionaries
final_results = {}
for key, value in results.items():
if isinstance(value, dict):
for n, v in value.items():
final_results[f"{key}_{n}"] = v
else:
final_results[key] = value
return final_results
else:
return {
"precision": results["overall_precision"],
"recall": results["overall_recall"],
"f1": results["overall_f1"],
"accuracy": results["overall_accuracy"],
}
# Train!
total_batch_size = args.per_device_train_batch_size * accelerator.num_processes * args.gradient_accumulation_steps
logger.info("***** Running training *****")
logger.info(f" Num examples = {len(train_dataset)}")
logger.info(f" Num Epochs = {args.num_train_epochs}")
logger.info(f" Instantaneous batch size per device = {args.per_device_train_batch_size}")
logger.info(f" Total train batch size (w. parallel, distributed & accumulation) = {total_batch_size}")
logger.info(f" Gradient Accumulation steps = {args.gradient_accumulation_steps}")
logger.info(f" Total optimization steps = {args.max_train_steps}")
# Only show the progress bar once on each machine.
progress_bar = tqdm(range(args.max_train_steps), disable=not accelerator.is_local_main_process)
completed_steps = 0
for epoch in range(args.num_train_epochs):
model.train()
for step, batch in enumerate(train_dataloader):
_ = batch.pop("original_entity_spans")
outputs = model(**batch)
loss = outputs.loss
loss = loss / args.gradient_accumulation_steps
accelerator.backward(loss)
if step % args.gradient_accumulation_steps == 0 or step == len(train_dataloader) - 1:
optimizer.step()
lr_scheduler.step()
optimizer.zero_grad()
progress_bar.update(1)
completed_steps += 1
if completed_steps >= args.max_train_steps:
break
model.eval()
for step, batch in enumerate(eval_dataloader):
original_entity_spans = batch.pop("original_entity_spans")
with torch.no_grad():
outputs = model(**batch)
preds, refs = get_luke_labels(outputs, batch[label_column_name], original_entity_spans)
metric.add_batch(
predictions=preds,
references=refs,
) # predictions and preferences are expected to be a nested list of labels, not label_ids
eval_metric = compute_metrics()
accelerator.print(f"epoch {epoch}:", eval_metric)
if args.push_to_hub and epoch < args.num_train_epochs - 1:
accelerator.wait_for_everyone()
unwrapped_model = accelerator.unwrap_model(model)
unwrapped_model.save_pretrained(args.output_dir, save_function=accelerator.save)
if accelerator.is_main_process:
tokenizer.save_pretrained(args.output_dir)
repo.push_to_hub(
commit_message=f"Training in progress epoch {epoch}", blocking=False, auto_lfs_prune=True
)
if args.output_dir is not None:
accelerator.wait_for_everyone()
unwrapped_model = accelerator.unwrap_model(model)
unwrapped_model.save_pretrained(args.output_dir, save_function=accelerator.save)
if accelerator.is_main_process:
tokenizer.save_pretrained(args.output_dir)
if args.push_to_hub:
repo.push_to_hub(commit_message="End of training", auto_lfs_prune=True)
if __name__ == "__main__":
main()
| transformers/examples/research_projects/luke/run_luke_ner_no_trainer.py/0 | {
"file_path": "transformers/examples/research_projects/luke/run_luke_ner_no_trainer.py",
"repo_id": "transformers",
"token_count": 12486
} | 291 |
# Movement Pruning: Adaptive Sparsity by Fine-Tuning
Author: @VictorSanh
*Magnitude pruning is a widely used strategy for reducing model size in pure supervised learning; however, it is less effective in the transfer learning regime that has become standard for state-of-the-art natural language processing applications. We propose the use of *movement pruning*, a simple, deterministic first-order weight pruning method that is more adaptive to pretrained model fine-tuning. Experiments show that when pruning large pretrained language models, movement pruning shows significant improvements in high-sparsity regimes. When combined with distillation, the approach achieves minimal accuracy loss with down to only 3% of the model parameters:*
| Fine-pruning+Distillation<br>(Teacher=BERT-base fine-tuned) | BERT base<br>fine-tuned | Remaining<br>Weights (%) | Magnitude Pruning | L0 Regularization | Movement Pruning | Soft Movement Pruning |
| :---: | :---: | :---: | :---: | :---: | :---: | :---: |
| SQuAD - Dev<br>EM/F1 | 80.4/88.1 | 10%<br>3% | 70.2/80.1<br>45.5/59.6 | 72.4/81.9<br>64.3/75.8 | 75.6/84.3<br>67.5/78.0 | **76.6/84.9**<br>**72.7/82.3** |
| MNLI - Dev<br>acc/MM acc | 84.5/84.9 | 10%<br>3% | 78.3/79.3<br>69.4/70.6 | 78.7/79.7<br>76.0/76.2 | 80.1/80.4<br>76.5/77.4 | **81.2/81.8**<br>**79.5/80.1** |
| QQP - Dev<br>acc/F1 | 91.4/88.4 | 10%<br>3% | 79.8/65.0<br>72.4/57.8 | 88.1/82.8<br>87.0/81.9 | 89.7/86.2<br>86.1/81.5 | **90.2/86.8**<br>**89.1/85.5** |
This page contains information on how to fine-prune pre-trained models such as `BERT` to obtain extremely sparse models with movement pruning. In contrast to magnitude pruning which selects weights that are far from 0, movement pruning retains weights that are moving away from 0.
For more information, we invite you to check out [our paper](https://arxiv.org/abs/2005.07683).
You can also have a look at this fun *Explain Like I'm Five* introductory [slide deck](https://www.slideshare.net/VictorSanh/movement-pruning-explain-like-im-five-234205241).
<div align="center">
<img src="https://www.seekpng.com/png/detail/166-1669328_how-to-make-emmental-cheese-at-home-icooker.png" width="400">
</div>
## Extreme sparsity and efficient storage
One promise of extreme pruning is to obtain extremely small models that can be easily sent (and stored) on edge devices. By setting weights to 0., we reduce the amount of information we need to store, and thus decreasing the memory size. We are able to obtain extremely sparse fine-pruned models with movement pruning: ~95% of the dense performance with ~5% of total remaining weights in the BERT encoder.
In [this notebook](https://github.com/huggingface/transformers/blob/main/examples/research_projects/movement-pruning/Saving_PruneBERT.ipynb), we showcase how we can leverage standard tools that exist out-of-the-box to efficiently store an extremely sparse question answering model (only 6% of total remaining weights in the encoder). We are able to reduce the memory size of the encoder **from the 340MB (the original dense BERT) to 11MB**, without any additional training of the model (every operation is performed *post fine-pruning*). It is sufficiently small to store it on a [91' floppy disk](https://en.wikipedia.org/wiki/Floptical) ๐!
While movement pruning does not directly optimize for memory footprint (but rather the number of non-null weights), we hypothetize that further memory compression ratios can be achieved with specific quantization aware trainings (see for instance [Q8BERT](https://arxiv.org/abs/1910.06188), [And the Bit Goes Down](https://arxiv.org/abs/1907.05686) or [Quant-Noise](https://arxiv.org/abs/2004.07320)).
## Fine-pruned models
As examples, we release two English PruneBERT checkpoints (models fine-pruned from a pre-trained `BERT` checkpoint), one on SQuAD and the other on MNLI.
- **`prunebert-base-uncased-6-finepruned-w-distil-squad`**<br/>
Pre-trained `BERT-base-uncased` fine-pruned with soft movement pruning on SQuAD v1.1. We use an additional distillation signal from `BERT-base-uncased` finetuned on SQuAD. The encoder counts 6% of total non-null weights and reaches 83.8 F1 score. The model can be accessed with: `pruned_bert = BertForQuestionAnswering.from_pretrained("huggingface/prunebert-base-uncased-6-finepruned-w-distil-squad")`
- **`prunebert-base-uncased-6-finepruned-w-distil-mnli`**<br/>
Pre-trained `BERT-base-uncased` fine-pruned with soft movement pruning on MNLI. We use an additional distillation signal from `BERT-base-uncased` finetuned on MNLI. The encoder counts 6% of total non-null weights and reaches 80.7 (matched) accuracy. The model can be accessed with: `pruned_bert = BertForSequenceClassification.from_pretrained("huggingface/prunebert-base-uncased-6-finepruned-w-distil-mnli")`
## How to fine-prune?
### Setup
The code relies on the ๐ค Transformers library. In addition to the dependencies listed in the [`examples`](https://github.com/huggingface/transformers/tree/main/examples) folder, you should install a few additional dependencies listed in the `requirements.txt` file: `pip install -r requirements.txt`.
Note that we built our experiments on top of a stabilized version of the library (commit https://github.com/huggingface/transformers/commit/352d5472b0c1dec0f420d606d16747d851b4bda8): we do not guarantee that everything is still compatible with the latest version of the main branch.
### Fine-pruning with movement pruning
Below, we detail how to reproduce the results reported in the paper. We use SQuAD as a running example. Commands (and scripts) can be easily adapted for other tasks.
The following command fine-prunes a pre-trained `BERT-base` on SQuAD using movement pruning towards 15% of remaining weights (85% sparsity). Note that we freeze all the embeddings modules (from their pre-trained value) and only prune the Fully Connected layers in the encoder (12 layers of Transformer Block).
```bash
SERIALIZATION_DIR=<OUTPUT_DIR>
SQUAD_DATA=<SQUAD_DATA>
python examples/movement-pruning/masked_run_squad.py \
--output_dir $SERIALIZATION_DIR \
--data_dir $SQUAD_DATA \
--train_file train-v1.1.json \
--predict_file dev-v1.1.json \
--do_train --do_eval --do_lower_case \
--model_type masked_bert \
--model_name_or_path bert-base-uncased \
--per_gpu_train_batch_size 16 \
--warmup_steps 5400 \
--num_train_epochs 10 \
--learning_rate 3e-5 --mask_scores_learning_rate 1e-2 \
--initial_threshold 1 --final_threshold 0.15 \
--initial_warmup 1 --final_warmup 2 \
--pruning_method topK --mask_init constant --mask_scale 0.
```
### Fine-pruning with other methods
We can also explore other fine-pruning methods by changing the `pruning_method` parameter:
Soft movement pruning
```bash
python examples/movement-pruning/masked_run_squad.py \
--output_dir $SERIALIZATION_DIR \
--data_dir $SQUAD_DATA \
--train_file train-v1.1.json \
--predict_file dev-v1.1.json \
--do_train --do_eval --do_lower_case \
--model_type masked_bert \
--model_name_or_path bert-base-uncased \
--per_gpu_train_batch_size 16 \
--warmup_steps 5400 \
--num_train_epochs 10 \
--learning_rate 3e-5 --mask_scores_learning_rate 1e-2 \
--initial_threshold 0 --final_threshold 0.1 \
--initial_warmup 1 --final_warmup 2 \
--pruning_method sigmoied_threshold --mask_init constant --mask_scale 0. \
--regularization l1 --final_lambda 400.
```
L0 regularization
```bash
python examples/movement-pruning/masked_run_squad.py \
--output_dir $SERIALIZATION_DIR \
--data_dir $SQUAD_DATA \
--train_file train-v1.1.json \
--predict_file dev-v1.1.json \
--do_train --do_eval --do_lower_case \
--model_type masked_bert \
--model_name_or_path bert-base-uncased \
--per_gpu_train_batch_size 16 \
--warmup_steps 5400 \
--num_train_epochs 10 \
--learning_rate 3e-5 --mask_scores_learning_rate 1e-1 \
--initial_threshold 1. --final_threshold 1. \
--initial_warmup 1 --final_warmup 1 \
--pruning_method l0 --mask_init constant --mask_scale 2.197 \
--regularization l0 --final_lambda 125.
```
Iterative Magnitude Pruning
```bash
python examples/movement-pruning/masked_run_squad.py \
--output_dir ./dbg \
--data_dir examples/distillation/data/squad_data \
--train_file train-v1.1.json \
--predict_file dev-v1.1.json \
--do_train --do_eval --do_lower_case \
--model_type masked_bert \
--model_name_or_path bert-base-uncased \
--per_gpu_train_batch_size 16 \
--warmup_steps 5400 \
--num_train_epochs 10 \
--learning_rate 3e-5 \
--initial_threshold 1 --final_threshold 0.15 \
--initial_warmup 1 --final_warmup 2 \
--pruning_method magnitude
```
### After fine-pruning
**Counting parameters**
Regularization based pruning methods (soft movement pruning and L0 regularization) rely on the penalty to induce sparsity. The multiplicative coefficient controls the sparsity level.
To obtain the effective sparsity level in the encoder, we simply count the number of activated (non-null) weights:
```bash
python examples/movement-pruning/counts_parameters.py \
--pruning_method sigmoied_threshold \
--threshold 0.1 \
--serialization_dir $SERIALIZATION_DIR
```
**Pruning once for all**
Once the model has been fine-pruned, the pruned weights can be set to 0. once for all (reducing the amount of information to store). In our running experiments, we can convert a `MaskedBertForQuestionAnswering` (a BERT model augmented to enable on-the-fly pruning capabilities) to a standard `BertForQuestionAnswering`:
```bash
python examples/movement-pruning/bertarize.py \
--pruning_method sigmoied_threshold \
--threshold 0.1 \
--model_name_or_path $SERIALIZATION_DIR
```
## Hyper-parameters
For reproducibility purposes, we share the detailed results presented in the paper. These [tables](https://docs.google.com/spreadsheets/d/17JgRq_OFFTniUrz6BZWW_87DjFkKXpI1kYDSsseT_7g/edit?usp=sharing) exhaustively describe the individual hyper-parameters used for each data point.
## Inference speed
Early experiments show that even though models fine-pruned with (soft) movement pruning are extremely sparse, they do not benefit from significant improvement in terms of inference speed when using the standard PyTorch inference.
We are currently benchmarking and exploring inference setups specifically for sparse architectures.
In particular, hardware manufacturers are announcing devices that will speedup inference for sparse networks considerably.
## Citation
If you find this resource useful, please consider citing the following paper:
```
@article{sanh2020movement,
title={Movement Pruning: Adaptive Sparsity by Fine-Tuning},
author={Victor Sanh and Thomas Wolf and Alexander M. Rush},
year={2020},
eprint={2005.07683},
archivePrefix={arXiv},
primaryClass={cs.CL}
}
```
| transformers/examples/research_projects/movement-pruning/README.md/0 | {
"file_path": "transformers/examples/research_projects/movement-pruning/README.md",
"repo_id": "transformers",
"token_count": 4030
} | 292 |
<!---
Copyright 2021 NVIDIA Corporation. All rights reserved.
Licensed under the Apache License, Version 2.0 (the "License");
you may not use this file except in compliance with the License.
You may obtain a copy of the License at
http://www.apache.org/licenses/LICENSE-2.0
Unless required by applicable law or agreed to in writing, software
distributed under the License is distributed on an "AS IS" BASIS,
WITHOUT WARRANTIES OR CONDITIONS OF ANY KIND, either express or implied.
See the License for the specific language governing permissions and
limitations under the License.
-->
# Huggingface QDQBERT Quantization Example
The QDQBERT model adds fake quantization (pair of QuantizeLinear/DequantizeLinear ops) to:
* linear layer inputs and weights
* matmul inputs
* residual add inputs
In this example, we use QDQBERT model to do quantization on SQuAD task, including Quantization Aware Training (QAT), Post Training Quantization (PTQ) and inferencing using TensorRT.
Required:
- [pytorch-quantization toolkit](https://github.com/NVIDIA/TensorRT/tree/master/tools/pytorch-quantization)
- [TensorRT >= 8.2](https://developer.nvidia.com/tensorrt)
- PyTorch >= 1.10.0
## Setup the environment with Dockerfile
Under the directory of `transformers/`, build the docker image:
```
docker build . -f examples/research_projects/quantization-qdqbert/Dockerfile -t bert_quantization:latest
```
Run the docker:
```
docker run --gpus all --privileged --rm -it --shm-size=1g --ulimit memlock=-1 --ulimit stack=67108864 bert_quantization:latest
```
In the container:
```
cd transformers/examples/research_projects/quantization-qdqbert/
```
## Quantization Aware Training (QAT)
Calibrate the pretrained model and finetune with quantization awared:
```
python3 run_quant_qa.py \
--model_name_or_path bert-base-uncased \
--dataset_name squad \
--max_seq_length 128 \
--doc_stride 32 \
--output_dir calib/bert-base-uncased \
--do_calib \
--calibrator percentile \
--percentile 99.99
```
```
python3 run_quant_qa.py \
--model_name_or_path calib/bert-base-uncased \
--dataset_name squad \
--do_train \
--do_eval \
--per_device_train_batch_size 12 \
--learning_rate 4e-5 \
--num_train_epochs 2 \
--max_seq_length 128 \
--doc_stride 32 \
--output_dir finetuned_int8/bert-base-uncased \
--tokenizer_name bert-base-uncased \
--save_steps 0
```
### Export QAT model to ONNX
To export the QAT model finetuned above:
```
python3 run_quant_qa.py \
--model_name_or_path finetuned_int8/bert-base-uncased \
--output_dir ./ \
--save_onnx \
--per_device_eval_batch_size 1 \
--max_seq_length 128 \
--doc_stride 32 \
--dataset_name squad \
--tokenizer_name bert-base-uncased
```
Use `--recalibrate-weights` to calibrate the weight ranges according to the quantizer axis. Use `--quant-per-tensor` for per tensor quantization (default is per channel).
Recalibrating will affect the accuracy of the model, but the change should be minimal (< 0.5 F1).
### Benchmark the INT8 QAT ONNX model inference with TensorRT using dummy input
```
trtexec --onnx=model.onnx --explicitBatch --workspace=16384 --int8 --shapes=input_ids:64x128,attention_mask:64x128,token_type_ids:64x128 --verbose
```
### Benchmark the INT8 QAT ONNX model inference with [ONNX Runtime-TRT](https://onnxruntime.ai/docs/execution-providers/TensorRT-ExecutionProvider.html) using dummy input
```
python3 ort-infer-benchmark.py
```
### Evaluate the INT8 QAT ONNX model inference with TensorRT
```
python3 evaluate-hf-trt-qa.py \
--onnx_model_path=./model.onnx \
--output_dir ./ \
--per_device_eval_batch_size 64 \
--max_seq_length 128 \
--doc_stride 32 \
--dataset_name squad \
--tokenizer_name bert-base-uncased \
--int8 \
--seed 42
```
## Fine-tuning of FP32 model for comparison
Finetune a fp32 precision model with [transformers/examples/pytorch/question-answering/](../../pytorch/question-answering/):
```
python3 ../../pytorch/question-answering/run_qa.py \
--model_name_or_path bert-base-uncased \
--dataset_name squad \
--per_device_train_batch_size 12 \
--learning_rate 3e-5 \
--num_train_epochs 2 \
--max_seq_length 128 \
--doc_stride 32 \
--output_dir ./finetuned_fp32/bert-base-uncased \
--save_steps 0 \
--do_train \
--do_eval
```
## Post Training Quantization (PTQ)
### PTQ by calibrating and evaluating the finetuned FP32 model above:
```
python3 run_quant_qa.py \
--model_name_or_path ./finetuned_fp32/bert-base-uncased \
--dataset_name squad \
--calibrator percentile \
--percentile 99.99 \
--max_seq_length 128 \
--doc_stride 32 \
--output_dir ./calib/bert-base-uncased \
--save_steps 0 \
--do_calib \
--do_eval
```
### Export the INT8 PTQ model to ONNX
```
python3 run_quant_qa.py \
--model_name_or_path ./calib/bert-base-uncased \
--output_dir ./ \
--save_onnx \
--per_device_eval_batch_size 1 \
--max_seq_length 128 \
--doc_stride 32 \
--dataset_name squad \
--tokenizer_name bert-base-uncased
```
### Evaluate the INT8 PTQ ONNX model inference with TensorRT
```
python3 evaluate-hf-trt-qa.py \
--onnx_model_path=./model.onnx \
--output_dir ./ \
--per_device_eval_batch_size 64 \
--max_seq_length 128 \
--doc_stride 32 \
--dataset_name squad \
--tokenizer_name bert-base-uncased \
--int8 \
--seed 42
```
### Quantization options
Some useful options to support different implementations and optimizations. These should be specified for both calibration and finetuning.
|argument|description|
|--------|-----------|
|`--quant-per-tensor`| quantize weights with one quantization range per tensor |
|`--fuse-qkv` | use a single range (the max) for quantizing QKV weights and output activations |
|`--clip-gelu N` | clip the output of GELU to a maximum of N when quantizing (e.g. 10) |
|`--disable-dropout` | disable dropout for consistent activation ranges |
| transformers/examples/research_projects/quantization-qdqbert/README.md/0 | {
"file_path": "transformers/examples/research_projects/quantization-qdqbert/README.md",
"repo_id": "transformers",
"token_count": 2081
} | 293 |
import logging
import os
from typing import List, Tuple
import numpy as np
import psutil
import torch
import torch.distributed as dist
from transformers import RagRetriever
logger = logging.getLogger(__name__)
class RagPyTorchDistributedRetriever(RagRetriever):
"""
A distributed retriever built on top of the ``torch.distributed`` communication package. During training all workers
initialize their own instance of the retriever, however, only the main worker loads the index into memory. The index is stored
in cpu memory. The index will also work well in a non-distributed setup.
Args:
config (:class:`~transformers.RagConfig`):
The configuration of the RAG model this Retriever is used with. Contains parameters indicating which ``Index`` to build.
question_encoder_tokenizer (:class:`~transformers.PreTrainedTokenizer`):
The tokenizer that was used to tokenize the question.
It is used to decode the question and then use the generator_tokenizer.
generator_tokenizer (:class:`~transformers.PreTrainedTokenizer`):
The tokenizer used for the generator part of the RagModel.
index (:class:`~transformers.models.rag.retrieval_rag.Index`, optional, defaults to the one defined by the configuration):
If specified, use this index instead of the one built using the configuration
"""
def __init__(self, config, question_encoder_tokenizer, generator_tokenizer, index=None):
super().__init__(
config,
question_encoder_tokenizer=question_encoder_tokenizer,
generator_tokenizer=generator_tokenizer,
index=index,
init_retrieval=False,
)
self.process_group = None
def init_retrieval(self, distributed_port: int):
"""
Retriever initialization function, needs to be called from the training process. The function sets some common parameters
and environment variables. On top of that, (only) the main process in the process group loads the index into memory.
Args:
distributed_port (:obj:`int`):
The port on which the main communication of the training run is carried out. We set the port for retrieval-related
communication as ``distributed_port + 1``.
"""
logger.info("initializing retrieval")
# initializing a separate process group for retrieval as the default
# nccl backend doesn't support gather/scatter operations while gloo
# is too slow to replace nccl for the core gpu communication
if dist.is_initialized():
logger.info("dist initialized")
# needs to be set manually
os.environ["GLOO_SOCKET_IFNAME"] = self._infer_socket_ifname()
# avoid clash with the NCCL port
os.environ["MASTER_PORT"] = str(distributed_port + 1)
self.process_group = dist.new_group(ranks=None, backend="gloo")
# initialize retriever only on the main worker
if not dist.is_initialized() or self._is_main():
logger.info("dist not initialized / main")
self.index.init_index()
# all processes wait untill the retriever is initialized by the main process
if dist.is_initialized():
torch.distributed.barrier(group=self.process_group)
def _is_main(self):
return dist.get_rank(group=self.process_group) == 0
def _scattered(self, scatter_list, target_shape, target_type=torch.float32):
target_tensor = torch.empty(target_shape, dtype=target_type)
dist.scatter(target_tensor, src=0, scatter_list=scatter_list, group=self.process_group)
return target_tensor
def _infer_socket_ifname(self):
addrs = psutil.net_if_addrs()
# a hacky way to deal with varying network interface names
ifname = next((addr for addr in addrs if addr.startswith("e")), None)
return ifname
def retrieve(self, question_hidden_states: np.ndarray, n_docs: int) -> Tuple[np.ndarray, List[dict]]:
"""
Retrieves documents for specified ``question_hidden_states``. The main process, which has the access to the index stored in memory, gathers queries
from all the processes in the main training process group, performs the retrieval and scatters back the results.
Args:
question_hidden_states (:obj:`np.ndarray` of shape :obj:`(batch_size, vector_size)`):
A batch of query vectors to retrieve with.
n_docs (:obj:`int`):
The number of docs retrieved per query.
Output:
retrieved_doc_embeds (:obj:`np.ndarray` of shape :obj:`(batch_size, n_docs, dim)`
The retrieval embeddings of the retrieved docs per query.
doc_ids (:obj:`np.ndarray` of shape :obj:`batch_size, n_docs`)
The ids of the documents in the index
doc_dicts (:obj:`List[dict]`):
The retrieved_doc_embeds examples per query.
"""
# single GPU training
if not dist.is_initialized():
doc_ids, retrieved_doc_embeds = self._main_retrieve(question_hidden_states, n_docs)
return retrieved_doc_embeds, doc_ids, self.index.get_doc_dicts(doc_ids)
# distributed training
world_size = dist.get_world_size(group=self.process_group)
# gather logic
gather_list = None
if self._is_main():
gather_list = [torch.empty(question_hidden_states.shape, dtype=torch.float32) for _ in range(world_size)]
dist.gather(torch.tensor(question_hidden_states), dst=0, gather_list=gather_list, group=self.process_group)
# scatter logic
n_queries = question_hidden_states.shape[0]
scatter_ids = []
scatter_vectors = []
if self._is_main():
assert len(gather_list) == world_size
ids, vectors = self._main_retrieve(torch.cat(gather_list).numpy(), n_docs)
ids, vectors = torch.tensor(ids), torch.tensor(vectors)
scatter_ids = self._chunk_tensor(ids, n_queries)
scatter_vectors = self._chunk_tensor(vectors, n_queries)
doc_ids = self._scattered(scatter_ids, [n_queries, n_docs], target_type=torch.int64)
retrieved_doc_embeds = self._scattered(scatter_vectors, [n_queries, n_docs, question_hidden_states.shape[1]])
return retrieved_doc_embeds.numpy(), doc_ids.numpy(), self.index.get_doc_dicts(doc_ids)
| transformers/examples/research_projects/rag/distributed_pytorch_retriever.py/0 | {
"file_path": "transformers/examples/research_projects/rag/distributed_pytorch_retriever.py",
"repo_id": "transformers",
"token_count": 2561
} | 294 |
#!/usr/bin/env bash
export PYTHONPATH="../":"${PYTHONPATH}"
export WANDB_PROJECT=dmar
export MAX_LEN=128
export m=sshleifer/student_marian_en_ro_6_1
python finetune.py \
--learning_rate=3e-4 \
--do_train \
--fp16 \
--data_dir wmt_en_ro \
--max_source_length $MAX_LEN --max_target_length $MAX_LEN --val_max_target_length $MAX_LEN --test_max_target_length $MAX_LEN \
--freeze_encoder --freeze_embeds \
--train_batch_size=48 --eval_batch_size=64 \
--tokenizer_name $m --model_name_or_path $m --num_train_epochs=1 \
--warmup_steps 500 --logger_name wandb --gpus 1 \
--fp16_opt_level=O1 --task translation \
"$@"
| transformers/examples/research_projects/seq2seq-distillation/dynamic_bs_example.sh/0 | {
"file_path": "transformers/examples/research_projects/seq2seq-distillation/dynamic_bs_example.sh",
"repo_id": "transformers",
"token_count": 267
} | 295 |
<!---
Copyright 2022 The Microsoft Inc. and The HuggingFace Inc. Team. All rights reserved.
Licensed under the Apache License, Version 2.0 (the "License");
you may not use this file except in compliance with the License.
You may obtain a copy of the License at
http://www.apache.org/licenses/LICENSE-2.0
Unless required by applicable law or agreed to in writing, software
distributed under the License is distributed on an "AS IS" BASIS,
WITHOUT WARRANTIES OR CONDITIONS OF ANY KIND, either express or implied.
See the License for the specific language governing permissions and
limitations under the License.
-->
# Run Table Tasks with TAPEX
TAPEX is a table pre-training approach for table-related tasks. By learning a neural SQL executor over a synthetic corpus based on generative language models (e.g., BART), it achieves state-of-the-art performance on several table-based question answering benchmarks and table-based fact verification benchmark. More details can be found in the original paper [TAPEX: Table Pre-training via Learning a Neural SQL Executor](https://arxiv.org/pdf/2107.07653.pdf).
> If you are also familiar with [fairseq](https://github.com/pytorch/fairseq), you may also find [the official implementation](https://github.com/microsoft/Table-Pretraining) useful, which leverages the framework.
## Table Question Answering Tasks
### What is Table Question Answering
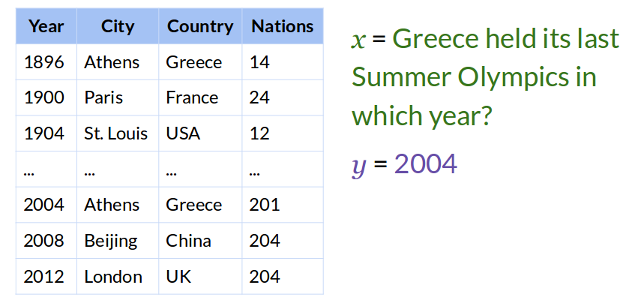
The task of Table Question Answering (TableQA) is to empower machines to answer users' questions over a given table. The resulting answer(s) can be a region in the table, or a number calculated by applying aggregation operators to a specific region.
### What Questions Can be Answered
Benefiting from the powerfulness of generative models, TAPEX can deal with almost all kinds of questions over tables (if there is training data). Below are some typical question and their answers taken from [WikiTableQuestion](https://nlp.stanford.edu/blog/wikitablequestions-a-complex-real-world-question-understanding-dataset).
| Question | Answer |
| :---: | :---: |
| What is the years won for each team? | 2004, 2008, 2012 |
| How long did Taiki Tsuchiya last? | 4:27 |
| What is the total amount of matches drawn? | 1 |
| Besides Tiger Woods, what other player won between 2007 and 2009? | Camilo Villegas |
| What was the last Baekje Temple? | Uija |
| What is the difference between White voters and Black voters in 1948? | 0 |
| What is the average number of sailors for each country during the worlds qualification tournament? | 2 |
### How to Fine-tune TAPEX on TableQA
We provide a fine-tuning script of tapex for TableQA on the WikiSQL benchmark: [WikiSQL](https://github.com/salesforce/WikiSQL).
This script is customized for tapex models, and can be easily adapted to other benchmarks such as WikiTableQuestion
(only some tweaks in the function `preprocess_tableqa_function`).
#### TAPEX-Base on WikiSQL
Here is how to run the script on the WikiSQL with `tapex-base`:
> The default hyper-parameter may allow you to reproduce our reported tapex-base results within the memory budget of 16GB and 1 GPU card. If you have more GPU cards, you could reduce `gradient_accumulation_steps` accordingly.
```bash
export EXP_NAME=wikisql_tapex_base
python run_wikisql_with_tapex.py \
--do_train \
--do_eval \
--output_dir $EXP_NAME \
--model_name_or_path microsoft/tapex-base \
--overwrite_output_dir \
--per_device_train_batch_size 4 \
--gradient_accumulation_steps 8 \
--per_device_eval_batch_size 4 \
--learning_rate 3e-5 \
--logging_steps 10 \
--eval_steps 1000 \
--save_steps 1000 \
--warmup_steps 1000 \
--evaluation_strategy steps \
--predict_with_generate \
--num_beams 5 \
--weight_decay 1e-2 \
--label_smoothing_factor 0.1 \
--max_steps 20000
```
#### TAPEX-Large on WikiSQL
Here is how to run the script on the WikiSQL with `tapex-large`:
> The default hyper-parameter may allow you to reproduce our reported tapex-large results within the memory budget of 16GB and 1 GPU card with fp16. If you have more GPU cards, you could reduce `gradient_accumulation_steps` accordingly. If you do not install apex or other mixed-precision-training libs, you could disable the `predict_with_generate` option to save GPU memory and manually evaluate the model once the fine-tuning finished. Or just pick up the last checkpoint, which usually performs good enough on the dataset.
```bash
export EXP_NAME=wikisql_tapex_large
python run_wikisql_with_tapex.py \
--do_train \
--do_eval \
--output_dir $EXP_NAME \
--model_name_or_path microsoft/tapex-large \
--overwrite_output_dir \
--per_device_train_batch_size 1 \
--gradient_accumulation_steps 32 \
--per_device_eval_batch_size 4 \
--learning_rate 3e-5 \
--logging_steps 10 \
--eval_steps 1000 \
--save_steps 1000 \
--warmup_steps 1000 \
--evaluation_strategy steps \
--predict_with_generate \
--num_beams 5 \
--weight_decay 1e-2 \
--label_smoothing_factor 0.1 \
--max_steps 20000 \
--fp16
```
#### TAPEX-Base on WikiTableQuestions
Here is how to run the script on the WikiTableQuestions with `tapex-base`:
> The default hyper-parameter may allow you to reproduce our reported tapex-base results within the memory budget of 16GB and 1 GPU card. If you have more GPU cards, you could reduce `gradient_accumulation_steps` accordingly.
```bash
export EXP_NAME=wikitablequestions_tapex_base
python run_wikitablequestions_with_tapex.py \
--do_train \
--do_eval \
--output_dir $EXP_NAME \
--model_name_or_path microsoft/tapex-base \
--overwrite_output_dir \
--per_device_train_batch_size 4 \
--gradient_accumulation_steps 8 \
--per_device_eval_batch_size 4 \
--learning_rate 3e-5 \
--logging_steps 10 \
--eval_steps 1000 \
--save_steps 1000 \
--warmup_steps 1000 \
--evaluation_strategy steps \
--predict_with_generate \
--num_beams 5 \
--weight_decay 1e-2 \
--label_smoothing_factor 0.1 \
--max_steps 20000
```
#### TAPEX-Large on WikiTableQuestions
Here is how to run the script on the WikiTableQuestions with `tapex-large`:
> The default hyper-parameter may allow you to reproduce our reported tapex-large results within the memory budget of 16GB and 1 GPU card with fp16. If you have more GPU cards, you could reduce `gradient_accumulation_steps` accordingly. If you do not install apex or other mixed-precision-training libs, you could reduce the `per_device_train_batch_size` and `per_device_eval_batch_size` and have another try. Or you could disable the `predict_with_generate` option to save GPU memory and manually evaluate the model once the fine-tuning finished. Or just pick up the last checkpoint, which usually performs good enough on the dataset.
```bash
export EXP_NAME=wikitablequestions_tapex_large
python run_wikitablequestions_with_tapex.py \
--do_train \
--do_eval \
--output_dir $EXP_NAME \
--model_name_or_path microsoft/tapex-large \
--overwrite_output_dir \
--per_device_train_batch_size 2 \
--gradient_accumulation_steps 12 \
--per_device_eval_batch_size 4 \
--learning_rate 3e-5 \
--logging_steps 10 \
--eval_steps 1000 \
--save_steps 1000 \
--warmup_steps 1000 \
--evaluation_strategy steps \
--predict_with_generate \
--num_beams 5 \
--weight_decay 1e-2 \
--label_smoothing_factor 0.1 \
--max_steps 20000 \
--fp16
```
### How to Evaluate TAPEX Fine-tuned Models on TableQA
We provide fine-tuned model weights to reproduce our results. You can evaluate them using the following command:
> You can also replace `microsoft/tapex-base-finetuned-wikisql` with your local directory to evaluate your fine-tuned models. Notice that if the model has a larger size, you should reduce `per_device_eval_batch_size` to fit the memory requirement.
```bash
export EXP_NAME=wikisql_tapex_base_eval
python run_wikisql_with_tapex.py \
--do_eval \
--model_name_or_path microsoft/tapex-base-finetuned-wikisql \
--output_dir $EXP_NAME \
--per_device_eval_batch_size 4 \
--predict_with_generate \
--num_beams 5
```
## Table Fact Verification Tasks
### What is Table Fact Verification
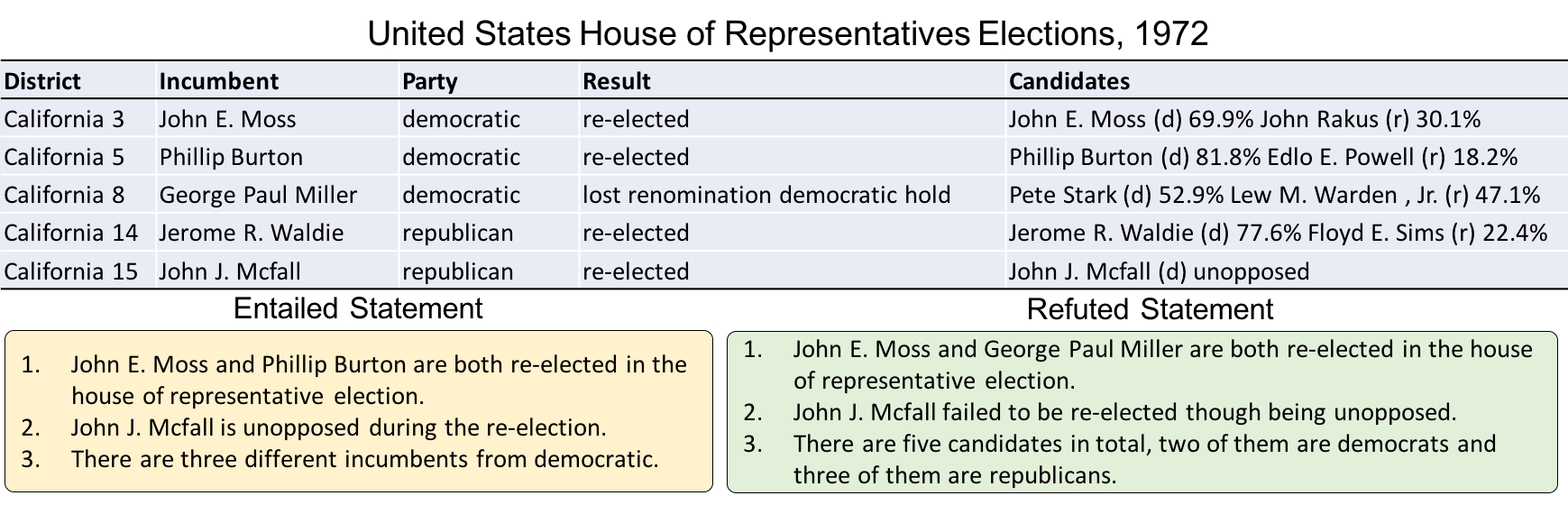
The task of Table Fact Verification (TableFV) is to empower machines to justify if a statement follows facts in a given table. The result is a binary classification belonging to `1` (entailed) or `0` (refused).
### How to Fine-tune TAPEX on TableFV
#### TAPEX-Base on TabFact
We provide a fine-tuning script of tapex for TableFV on the TabFact benchmark: [TabFact](https://github.com/wenhuchen/Table-Fact-Checking).
Here is how to run the script on the TabFact:
> The default hyper-parameter may allow you to reproduce our reported tapex-base results within the memory budget of 16GB and 1 GPU card. If you have more GPU cards, you could reduce `gradient_accumulation_steps` accordingly. Note that the `eval_accumulation_steps` is necessary, otherwise GPU memory leaks will occur during the evaluation.
```bash
export EXP_NAME=tabfact_tapex_base
python run_tabfact_with_tapex.py \
--do_train \
--do_eval \
--output_dir $EXP_NAME \
--model_name_or_path microsoft/tapex-base \
--overwrite_output_dir \
--per_device_train_batch_size 3 \
--gradient_accumulation_steps 16 \
--per_device_eval_batch_size 12 \
--eval_accumulation_steps 6 \
--warm_steps 1000 \
--logging_steps 10 \
--learning_rate 3e-5 \
--eval_steps 1000 \
--save_steps 1000 \
--evaluation_strategy steps \
--weight_decay 1e-2 \
--max_steps 30000 \
--max_grad_norm 0.1
```
#### TAPEX-Large on TabFact
Here is how to run the script on the TabFact:
> The default hyper-parameter may allow you to reproduce our reported tapex-base results within the memory budget of 24GB and 1 GPU card. Sorry we cannot reduce the memory consumption since the model input in TabFact usually contains nearly ~1000 tokens. If you have more GPU cards, you could reduce `gradient_accumulation_steps` accordingly. Note that the `eval_accumulation_steps` is necessary, otherwise GPU memory leaks will occur during the evaluation.
```bash
export EXP_NAME=tabfact_tapex_large
python run_tabfact_with_tapex.py \
--do_train \
--do_eval \
--output_dir $EXP_NAME \
--model_name_or_path microsoft/tapex-large \
--overwrite_output_dir \
--per_device_train_batch_size 2 \
--gradient_accumulation_steps 18 \
--per_device_eval_batch_size 4 \
--eval_accumulation_steps 12 \
--warm_steps 1000 \
--logging_steps 10 \
--learning_rate 3e-5 \
--eval_steps 1000 \
--save_steps 1000 \
--evaluation_strategy steps \
--weight_decay 1e-2 \
--max_steps 30000 \
--max_grad_norm 0.1
```
### How to Evaluate TAPEX Fine-tuned Models on TableFV
We provide fine-tuned model weights to reproduce our results. You can evaluate them using the following command:
> You can also replace `microsoft/tapex-base-finetuned-tabfact` with your local directory to evaluate your fine-tuned models. Notice that if the model has a larger size, you should reduce `per_device_eval_batch_size` to fit the memory requirement.
```bash
export EXP_NAME=tabfact_tapex_base_eval
python run_tabfact_with_tapex.py \
--do_eval \
--model_name_or_path microsoft/tapex-base-finetuned-tabfact \
--output_dir $EXP_NAME \
--per_device_eval_batch_size 12 \
--eval_accumulation_steps 6
```
## Reproduced Results
We get the following results on the dev set of the benchmark with the previous commands:
| Task | Model Size | Metric | Result |
|:---:|:---:|:---:|:---:|
| WikiSQL (Weak) | Base | Denotation Accuracy | 88.1 |
| WikiSQL (Weak) | Large | Denotation Accuracy | 89.5 |
| WikiTableQuestion | Base | Denotation Accuracy | 47.1 |
| WikiTableQuestion | Large | Denotation Accuracy | 57.2 |
| TabFact | Base | Accuracy | 78.7 |
| TabFact | Large | Accuracy | 83.6 |
| transformers/examples/research_projects/tapex/README.md/0 | {
"file_path": "transformers/examples/research_projects/tapex/README.md",
"repo_id": "transformers",
"token_count": 3719
} | 296 |
import numpy as np
import PIL
import torch
import torchvision.transforms as T
import torchvision.transforms.functional as TF
from PIL import Image
def preprocess(img, target_image_size=256):
s = min(img.size)
if s < target_image_size:
raise ValueError(f"min dim for image {s} < {target_image_size}")
r = target_image_size / s
s = (round(r * img.size[1]), round(r * img.size[0]))
img = TF.resize(img, s, interpolation=PIL.Image.LANCZOS)
img = TF.center_crop(img, output_size=2 * [target_image_size])
img = torch.unsqueeze(T.ToTensor()(img), 0)
return img
def preprocess_vqgan(x):
x = 2.0 * x - 1.0
return x
def custom_to_pil(x, process=True, mode="RGB"):
x = x.detach().cpu()
if process:
x = post_process_tensor(x)
x = x.numpy()
if process:
x = (255 * x).astype(np.uint8)
x = Image.fromarray(x)
if not x.mode == mode:
x = x.convert(mode)
return x
def post_process_tensor(x):
x = torch.clamp(x, -1.0, 1.0)
x = (x + 1.0) / 2.0
x = x.permute(1, 2, 0)
return x
def loop_post_process(x):
x = post_process_tensor(x.squeeze())
return x.permute(2, 0, 1).unsqueeze(0)
| transformers/examples/research_projects/vqgan-clip/img_processing.py/0 | {
"file_path": "transformers/examples/research_projects/vqgan-clip/img_processing.py",
"repo_id": "transformers",
"token_count": 545
} | 297 |
#!/usr/bin/env bash
python alignment.py \
--model_name="arijitx/wav2vec2-xls-r-300m-bengali" \
--wav_dir="./wavs" \
--text_file="script.txt" \
--input_wavs_sr=48000 \
--output_dir="./out_alignment" \
--cuda
| transformers/examples/research_projects/wav2vec2/run_alignment.sh/0 | {
"file_path": "transformers/examples/research_projects/wav2vec2/run_alignment.sh",
"repo_id": "transformers",
"token_count": 97
} | 298 |
#!/usr/bin/env python
# coding=utf-8
# Copyright 2021 The HuggingFace Inc. team. All rights reserved.
#
# Licensed under the Apache License, Version 2.0 (the "License");
# you may not use this file except in compliance with the License.
# You may obtain a copy of the License at
#
# http://www.apache.org/licenses/LICENSE-2.0
#
# Unless required by applicable law or agreed to in writing, software
# distributed under the License is distributed on an "AS IS" BASIS,
# WITHOUT WARRANTIES OR CONDITIONS OF ANY KIND, either express or implied.
# See the License for the specific language governing permissions and
# limitations under the License.
"""
Fine-tuning the library models for masked language modeling (BERT, ALBERT, RoBERTa...)
on a text file or a dataset without using HuggingFace Trainer.
Here is the full list of checkpoints on the hub that can be fine-tuned by this script:
https://huggingface.co/models?filter=fill-mask
"""
# You can also adapt this script on your own mlm task. Pointers for this are left as comments.
import json
import logging
import math
import os
import random
import sys
import warnings
from dataclasses import dataclass, field
from itertools import chain
from pathlib import Path
from typing import Optional
import datasets
import tensorflow as tf
from datasets import load_dataset
from sklearn.model_selection import train_test_split
import transformers
from transformers import (
CONFIG_MAPPING,
CONFIG_NAME,
TF2_WEIGHTS_NAME,
TF_MODEL_FOR_MASKED_LM_MAPPING,
AutoConfig,
AutoTokenizer,
DataCollatorForLanguageModeling,
HfArgumentParser,
PushToHubCallback,
TFAutoModelForMaskedLM,
TFTrainingArguments,
create_optimizer,
set_seed,
)
from transformers.utils import send_example_telemetry
from transformers.utils.versions import require_version
logger = logging.getLogger(__name__)
require_version("datasets>=1.8.0", "To fix: pip install -r examples/tensorflow/language-modeling/requirements.txt")
MODEL_CONFIG_CLASSES = list(TF_MODEL_FOR_MASKED_LM_MAPPING.keys())
MODEL_TYPES = tuple(conf.model_type for conf in MODEL_CONFIG_CLASSES)
# region Command-line arguments
@dataclass
class ModelArguments:
"""
Arguments pertaining to which model/config/tokenizer we are going to fine-tune, or train from scratch.
"""
model_name_or_path: Optional[str] = field(
default=None,
metadata={
"help": (
"The model checkpoint for weights initialization. Don't set if you want to train a model from scratch."
)
},
)
model_type: Optional[str] = field(
default=None,
metadata={"help": "If training from scratch, pass a model type from the list: " + ", ".join(MODEL_TYPES)},
)
config_overrides: Optional[str] = field(
default=None,
metadata={
"help": (
"Override some existing default config settings when a model is trained from scratch. Example: "
"n_embd=10,resid_pdrop=0.2,scale_attn_weights=false,summary_type=cls_index"
)
},
)
config_name: Optional[str] = field(
default=None, metadata={"help": "Pretrained config name or path if not the same as model_name"}
)
tokenizer_name: Optional[str] = field(
default=None, metadata={"help": "Pretrained tokenizer name or path if not the same as model_name"}
)
cache_dir: Optional[str] = field(
default=None,
metadata={"help": "Where do you want to store the pretrained models downloaded from huggingface.co"},
)
use_fast_tokenizer: bool = field(
default=True,
metadata={"help": "Whether to use one of the fast tokenizer (backed by the tokenizers library) or not."},
)
model_revision: str = field(
default="main",
metadata={"help": "The specific model version to use (can be a branch name, tag name or commit id)."},
)
token: str = field(
default=None,
metadata={
"help": (
"The token to use as HTTP bearer authorization for remote files. If not specified, will use the token "
"generated when running `huggingface-cli login` (stored in `~/.huggingface`)."
)
},
)
use_auth_token: bool = field(
default=None,
metadata={
"help": "The `use_auth_token` argument is deprecated and will be removed in v4.34. Please use `token` instead."
},
)
trust_remote_code: bool = field(
default=False,
metadata={
"help": (
"Whether or not to allow for custom models defined on the Hub in their own modeling files. This option "
"should only be set to `True` for repositories you trust and in which you have read the code, as it will "
"execute code present on the Hub on your local machine."
)
},
)
def __post_init__(self):
if self.config_overrides is not None and (self.config_name is not None or self.model_name_or_path is not None):
raise ValueError(
"--config_overrides can't be used in combination with --config_name or --model_name_or_path"
)
@dataclass
class DataTrainingArguments:
"""
Arguments pertaining to what data we are going to input our model for training and eval.
"""
dataset_name: Optional[str] = field(
default=None, metadata={"help": "The name of the dataset to use (via the datasets library)."}
)
dataset_config_name: Optional[str] = field(
default=None, metadata={"help": "The configuration name of the dataset to use (via the datasets library)."}
)
train_file: Optional[str] = field(default=None, metadata={"help": "The input training data file (a text file)."})
validation_file: Optional[str] = field(
default=None,
metadata={"help": "An optional input evaluation data file to evaluate the perplexity on (a text file)."},
)
overwrite_cache: bool = field(
default=False, metadata={"help": "Overwrite the cached training and evaluation sets"}
)
validation_split_percentage: Optional[int] = field(
default=5,
metadata={
"help": "The percentage of the train set used as validation set in case there's no validation split"
},
)
max_seq_length: Optional[int] = field(
default=None,
metadata={
"help": (
"The maximum total input sequence length after tokenization. Sequences longer "
"than this will be truncated."
)
},
)
preprocessing_num_workers: Optional[int] = field(
default=None,
metadata={"help": "The number of processes to use for the preprocessing."},
)
mlm_probability: float = field(
default=0.15, metadata={"help": "Ratio of tokens to mask for masked language modeling loss"}
)
line_by_line: bool = field(
default=False,
metadata={"help": "Whether distinct lines of text in the dataset are to be handled as distinct sequences."},
)
pad_to_max_length: bool = field(
default=False,
metadata={
"help": (
"Whether to pad all samples to `max_seq_length`. "
"If False, will pad the samples dynamically when batching to the maximum length in the batch."
)
},
)
max_train_samples: Optional[int] = field(
default=None,
metadata={
"help": (
"For debugging purposes or quicker training, truncate the number of training examples to this "
"value if set."
)
},
)
max_eval_samples: Optional[int] = field(
default=None,
metadata={
"help": (
"For debugging purposes or quicker training, truncate the number of evaluation examples to this "
"value if set."
)
},
)
def __post_init__(self):
if self.dataset_name is None and self.train_file is None and self.validation_file is None:
raise ValueError("Need either a dataset name or a training/validation file.")
else:
if self.train_file is not None:
extension = self.train_file.split(".")[-1]
assert extension in ["csv", "json", "txt"], "`train_file` should be a csv, a json or a txt file."
if self.validation_file is not None:
extension = self.validation_file.split(".")[-1]
assert extension in ["csv", "json", "txt"], "`validation_file` should be a csv, a json or a txt file."
# endregion
def main():
# region Argument Parsing
parser = HfArgumentParser((ModelArguments, DataTrainingArguments, TFTrainingArguments))
if len(sys.argv) == 2 and sys.argv[1].endswith(".json"):
# If we pass only one argument to the script and it's the path to a json file,
# let's parse it to get our arguments.
model_args, data_args, training_args = parser.parse_json_file(json_file=os.path.abspath(sys.argv[1]))
else:
model_args, data_args, training_args = parser.parse_args_into_dataclasses()
if model_args.use_auth_token is not None:
warnings.warn(
"The `use_auth_token` argument is deprecated and will be removed in v4.34. Please use `token` instead.",
FutureWarning,
)
if model_args.token is not None:
raise ValueError("`token` and `use_auth_token` are both specified. Please set only the argument `token`.")
model_args.token = model_args.use_auth_token
# Sending telemetry. Tracking the example usage helps us better allocate resources to maintain them. The
# information sent is the one passed as arguments along with your Python/PyTorch versions.
send_example_telemetry("run_mlm", model_args, data_args, framework="tensorflow")
# Sanity checks
if data_args.dataset_name is None and data_args.train_file is None and data_args.validation_file is None:
raise ValueError("Need either a dataset name or a training/validation file.")
else:
if data_args.train_file is not None:
extension = data_args.train_file.split(".")[-1]
assert extension in ["csv", "json", "txt"], "`train_file` should be a csv, json or txt file."
if data_args.validation_file is not None:
extension = data_args.validation_file.split(".")[-1]
assert extension in ["csv", "json", "txt"], "`validation_file` should be a csv, json or txt file."
if training_args.output_dir is not None:
training_args.output_dir = Path(training_args.output_dir)
os.makedirs(training_args.output_dir, exist_ok=True)
if isinstance(training_args.strategy, tf.distribute.TPUStrategy) and not data_args.pad_to_max_length:
logger.warning("We are training on TPU - forcing pad_to_max_length")
data_args.pad_to_max_length = True
# endregion
# region Checkpoints
# Detecting last checkpoint.
checkpoint = None
if len(os.listdir(training_args.output_dir)) > 0 and not training_args.overwrite_output_dir:
config_path = training_args.output_dir / CONFIG_NAME
weights_path = training_args.output_dir / TF2_WEIGHTS_NAME
if config_path.is_file() and weights_path.is_file():
checkpoint = training_args.output_dir
logger.warning(
f"Checkpoint detected, resuming training from checkpoint in {training_args.output_dir}. To avoid this"
" behavior, change the `--output_dir` or add `--overwrite_output_dir` to train from scratch."
)
else:
raise ValueError(
f"Output directory ({training_args.output_dir}) already exists and is not empty. "
"Use --overwrite_output_dir to continue regardless."
)
# endregion
# region Setup logging
# accelerator.is_local_main_process is only True for one process per machine.
logger.setLevel(logging.INFO)
datasets.utils.logging.set_verbosity_warning()
transformers.utils.logging.set_verbosity_info()
# endregion
# If passed along, set the training seed now.
if training_args.seed is not None:
set_seed(training_args.seed)
# region Load datasets
# Get the datasets: you can either provide your own CSV/JSON/TXT training and evaluation files (see below)
# or just provide the name of one of the public datasets available on the hub at https://huggingface.co/datasets/
# (the dataset will be downloaded automatically from the datasets Hub).
#
# For CSV/JSON files, this script will use the column called 'text' or the first column if no column called
# 'text' is found. You can easily tweak this behavior (see below).
#
# In distributed training, the load_dataset function guarantee that only one local process can concurrently
# download the dataset.
if data_args.dataset_name is not None:
# Downloading and loading a dataset from the hub.
raw_datasets = load_dataset(
data_args.dataset_name,
data_args.dataset_config_name,
token=model_args.token,
)
if "validation" not in raw_datasets.keys():
raw_datasets["validation"] = load_dataset(
data_args.dataset_name,
data_args.dataset_config_name,
split=f"train[:{data_args.validation_split_percentage}%]",
token=model_args.token,
)
raw_datasets["train"] = load_dataset(
data_args.dataset_name,
data_args.dataset_config_name,
split=f"train[{data_args.validation_split_percentage}%:]",
token=model_args.token,
)
else:
data_files = {}
if data_args.train_file is not None:
data_files["train"] = data_args.train_file
extension = data_args.train_file.split(".")[-1]
if data_args.validation_file is not None:
data_files["validation"] = data_args.validation_file
extension = data_args.validation_file.split(".")[-1]
if extension == "txt":
extension = "text"
raw_datasets = load_dataset(
extension,
data_files=data_files,
token=model_args.token,
)
# See more about loading any type of standard or custom dataset (from files, python dict, pandas DataFrame, etc) at
# https://huggingface.co/docs/datasets/loading_datasets.
# endregion
# region Load pretrained model and tokenizer
#
# In distributed training, the .from_pretrained methods guarantee that only one local process can concurrently
# download model & vocab.
if checkpoint is not None:
config = AutoConfig.from_pretrained(
checkpoint, token=model_args.token, trust_remote_code=model_args.trust_remote_code
)
elif model_args.config_name:
config = AutoConfig.from_pretrained(
model_args.config_name, token=model_args.token, trust_remote_code=model_args.trust_remote_code
)
elif model_args.model_name_or_path:
config = AutoConfig.from_pretrained(
model_args.model_name_or_path, token=model_args.token, trust_remote_code=model_args.trust_remote_code
)
else:
config = CONFIG_MAPPING[model_args.model_type]()
logger.warning("You are instantiating a new config instance from scratch.")
if model_args.tokenizer_name:
tokenizer = AutoTokenizer.from_pretrained(
model_args.tokenizer_name, token=model_args.token, trust_remote_code=model_args.trust_remote_code
)
elif model_args.model_name_or_path:
tokenizer = AutoTokenizer.from_pretrained(
model_args.model_name_or_path, token=model_args.token, trust_remote_code=model_args.trust_remote_code
)
else:
raise ValueError(
"You are instantiating a new tokenizer from scratch. This is not supported by this script. "
"You can do it from another script, save it, and load it from here, using --tokenizer_name."
)
# endregion
# region Dataset preprocessing
# First we tokenize all the texts.
column_names = raw_datasets["train"].column_names
text_column_name = "text" if "text" in column_names else column_names[0]
if data_args.max_seq_length is None:
max_seq_length = tokenizer.model_max_length
if max_seq_length > 1024:
logger.warning(
f"The tokenizer picked seems to have a very large `model_max_length` ({tokenizer.model_max_length}). "
"Picking 1024 instead. You can reduce that default value by passing --max_seq_length xxx."
)
max_seq_length = 1024
else:
if data_args.max_seq_length > tokenizer.model_max_length:
logger.warning(
f"The max_seq_length passed ({data_args.max_seq_length}) is larger than the maximum length for the "
f"model ({tokenizer.model_max_length}). Using max_seq_length={tokenizer.model_max_length}."
)
max_seq_length = min(data_args.max_seq_length, tokenizer.model_max_length)
if data_args.line_by_line:
# When using line_by_line, we just tokenize each nonempty line.
padding = "max_length" if data_args.pad_to_max_length else False
def tokenize_function(examples):
# Remove empty lines
examples[text_column_name] = [
line for line in examples[text_column_name] if len(line) > 0 and not line.isspace()
]
return tokenizer(
examples[text_column_name],
padding=padding,
truncation=True,
max_length=max_seq_length,
# We use this option because DataCollatorForLanguageModeling (see below) is more efficient when it
# receives the `special_tokens_mask`.
return_special_tokens_mask=True,
)
tokenized_datasets = raw_datasets.map(
tokenize_function,
batched=True,
num_proc=data_args.preprocessing_num_workers,
remove_columns=[text_column_name],
load_from_cache_file=not data_args.overwrite_cache,
desc="Running tokenizer on dataset line_by_line",
)
else:
# Otherwise, we tokenize every text, then concatenate them together before splitting them in smaller parts.
# We use `return_special_tokens_mask=True` because DataCollatorForLanguageModeling (see below) is more
# efficient when it receives the `special_tokens_mask`.
def tokenize_function(examples):
return tokenizer(examples[text_column_name], return_special_tokens_mask=True)
tokenized_datasets = raw_datasets.map(
tokenize_function,
batched=True,
num_proc=data_args.preprocessing_num_workers,
remove_columns=column_names,
load_from_cache_file=not data_args.overwrite_cache,
desc="Running tokenizer on every text in dataset",
)
# Main data processing function that will concatenate all texts from our dataset and generate chunks of
# max_seq_length.
def group_texts(examples):
# Concatenate all texts.
concatenated_examples = {k: list(chain(*examples[k])) for k in examples.keys()}
total_length = len(concatenated_examples[list(examples.keys())[0]])
# We drop the small remainder, we could add padding if the model supported it instead of this drop, you can
# customize this part to your needs.
if total_length >= max_seq_length:
total_length = (total_length // max_seq_length) * max_seq_length
# Split by chunks of max_len.
result = {
k: [t[i : i + max_seq_length] for i in range(0, total_length, max_seq_length)]
for k, t in concatenated_examples.items()
}
return result
# Note that with `batched=True`, this map processes 1,000 texts together, so group_texts throws away a
# remainder for each of those groups of 1,000 texts. You can adjust that batch_size here but a higher value
# might be slower to preprocess.
#
# To speed up this part, we use multiprocessing. See the documentation of the map method for more information:
# https://huggingface.co/docs/datasets/process#map
tokenized_datasets = tokenized_datasets.map(
group_texts,
batched=True,
num_proc=data_args.preprocessing_num_workers,
load_from_cache_file=not data_args.overwrite_cache,
desc=f"Grouping texts in chunks of {max_seq_length}",
)
train_dataset = tokenized_datasets["train"]
if data_args.validation_file is not None:
eval_dataset = tokenized_datasets["validation"]
else:
logger.info(
f"Validation file not found: using {data_args.validation_split_percentage}% of the dataset as validation"
" as provided in data_args"
)
train_indices, val_indices = train_test_split(
list(range(len(train_dataset))), test_size=data_args.validation_split_percentage / 100
)
eval_dataset = train_dataset.select(val_indices)
train_dataset = train_dataset.select(train_indices)
if data_args.max_train_samples is not None:
max_train_samples = min(len(train_dataset), data_args.max_train_samples)
train_dataset = train_dataset.select(range(max_train_samples))
if data_args.max_eval_samples is not None:
max_eval_samples = min(len(eval_dataset), data_args.max_eval_samples)
eval_dataset = eval_dataset.select(range(max_eval_samples))
# Log a few random samples from the training set:
for index in random.sample(range(len(train_dataset)), min(3, len(train_dataset))):
logger.info(f"Sample {index} of the training set: {train_dataset[index]}.")
# endregion
with training_args.strategy.scope():
# region Prepare model
if checkpoint is not None:
model = TFAutoModelForMaskedLM.from_pretrained(
checkpoint, config=config, token=model_args.token, trust_remote_code=model_args.trust_remote_code
)
elif model_args.model_name_or_path:
model = TFAutoModelForMaskedLM.from_pretrained(
model_args.model_name_or_path,
config=config,
token=model_args.token,
trust_remote_code=model_args.trust_remote_code,
)
else:
logger.info("Training new model from scratch")
model = TFAutoModelForMaskedLM.from_config(
config, token=model_args.token, trust_remote_code=model_args.trust_remote_code
)
# We resize the embeddings only when necessary to avoid index errors. If you are creating a model from scratch
# on a small vocab and want a smaller embedding size, remove this test.
embeddings = model.get_input_embeddings()
# Matt: This is a temporary workaround as we transition our models to exclusively using Keras embeddings.
# As soon as the transition is complete, all embeddings should be keras.Embeddings layers, and
# the weights will always be in embeddings.embeddings.
if hasattr(embeddings, "embeddings"):
embedding_size = embeddings.embeddings.shape[0]
else:
embedding_size = embeddings.weight.shape[0]
if len(tokenizer) > embedding_size:
model.resize_token_embeddings(len(tokenizer))
# endregion
# region TF Dataset preparation
num_replicas = training_args.strategy.num_replicas_in_sync
data_collator = DataCollatorForLanguageModeling(
tokenizer=tokenizer, mlm_probability=data_args.mlm_probability, return_tensors="np"
)
options = tf.data.Options()
options.experimental_distribute.auto_shard_policy = tf.data.experimental.AutoShardPolicy.OFF
# model.prepare_tf_dataset() wraps a Hugging Face dataset in a tf.data.Dataset which is ready to use in
# training. This is the recommended way to use a Hugging Face dataset when training with Keras. You can also
# use the lower-level dataset.to_tf_dataset() method, but you will have to specify things like column names
# yourself if you use this method, whereas they are automatically inferred from the model input names when
# using model.prepare_tf_dataset()
# For more info see the docs:
# https://huggingface.co/docs/transformers/main/en/main_classes/model#transformers.TFPreTrainedModel.prepare_tf_dataset
# https://huggingface.co/docs/datasets/main/en/package_reference/main_classes#datasets.Dataset.to_tf_dataset
tf_train_dataset = model.prepare_tf_dataset(
train_dataset,
shuffle=True,
batch_size=num_replicas * training_args.per_device_train_batch_size,
collate_fn=data_collator,
).with_options(options)
tf_eval_dataset = model.prepare_tf_dataset(
eval_dataset,
# labels are passed as input, as we will use the model's internal loss
shuffle=False,
batch_size=num_replicas * training_args.per_device_eval_batch_size,
collate_fn=data_collator,
drop_remainder=True,
).with_options(options)
# endregion
# region Optimizer and loss
num_train_steps = len(tf_train_dataset) * int(training_args.num_train_epochs)
if training_args.warmup_steps > 0:
num_warmup_steps = training_args.warmup_steps
elif training_args.warmup_ratio > 0:
num_warmup_steps = int(num_train_steps * training_args.warmup_ratio)
else:
num_warmup_steps = 0
# Bias and layernorm weights are automatically excluded from the decay
optimizer, lr_schedule = create_optimizer(
init_lr=training_args.learning_rate,
num_train_steps=num_train_steps,
num_warmup_steps=num_warmup_steps,
adam_beta1=training_args.adam_beta1,
adam_beta2=training_args.adam_beta2,
adam_epsilon=training_args.adam_epsilon,
weight_decay_rate=training_args.weight_decay,
adam_global_clipnorm=training_args.max_grad_norm,
)
# Transformers models compute the right loss for their task by default when labels are passed, and will
# use this for training unless you specify your own loss function in compile().
model.compile(optimizer=optimizer, jit_compile=training_args.xla)
# endregion
# region Preparing push_to_hub and model card
push_to_hub_model_id = training_args.push_to_hub_model_id
model_name = model_args.model_name_or_path.split("/")[-1]
if not push_to_hub_model_id:
if data_args.dataset_name is not None:
push_to_hub_model_id = f"{model_name}-finetuned-{data_args.dataset_name}"
else:
push_to_hub_model_id = f"{model_name}-finetuned-mlm"
model_card_kwargs = {"finetuned_from": model_args.model_name_or_path, "tasks": "fill-mask"}
if data_args.dataset_name is not None:
model_card_kwargs["dataset_tags"] = data_args.dataset_name
if data_args.dataset_config_name is not None:
model_card_kwargs["dataset_args"] = data_args.dataset_config_name
model_card_kwargs["dataset"] = f"{data_args.dataset_name} {data_args.dataset_config_name}"
else:
model_card_kwargs["dataset"] = data_args.dataset_name
if training_args.push_to_hub:
callbacks = [
PushToHubCallback(
output_dir=training_args.output_dir,
hub_model_id=push_to_hub_model_id,
hub_token=training_args.push_to_hub_token,
tokenizer=tokenizer,
**model_card_kwargs,
)
]
else:
callbacks = []
# endregion
# region Training and validation
logger.info("***** Running training *****")
logger.info(f" Num examples = {len(train_dataset)}")
logger.info(f" Num Epochs = {training_args.num_train_epochs}")
logger.info(f" Instantaneous batch size per device = {training_args.per_device_train_batch_size}")
logger.info(f" Total train batch size = {training_args.per_device_train_batch_size * num_replicas}")
# For long training runs, you may wish to use the PushToHub() callback here to save intermediate checkpoints
# to the Hugging Face Hub rather than just pushing the finished model.
# See https://huggingface.co/docs/transformers/main_classes/keras_callbacks#transformers.PushToHubCallback
history = model.fit(
tf_train_dataset,
validation_data=tf_eval_dataset,
epochs=int(training_args.num_train_epochs),
callbacks=callbacks,
)
train_loss = history.history["loss"][-1]
try:
train_perplexity = math.exp(train_loss)
except OverflowError:
train_perplexity = math.inf
logger.info(f" Final train loss: {train_loss:.3f}")
logger.info(f" Final train perplexity: {train_perplexity:.3f}")
validation_loss = history.history["val_loss"][-1]
try:
validation_perplexity = math.exp(validation_loss)
except OverflowError:
validation_perplexity = math.inf
logger.info(f" Final validation loss: {validation_loss:.3f}")
logger.info(f" Final validation perplexity: {validation_perplexity:.3f}")
if training_args.output_dir is not None:
output_eval_file = os.path.join(training_args.output_dir, "all_results.json")
results_dict = {}
results_dict["train_loss"] = train_loss
results_dict["train_perplexity"] = train_perplexity
results_dict["eval_loss"] = validation_loss
results_dict["eval_perplexity"] = validation_perplexity
with open(output_eval_file, "w") as writer:
writer.write(json.dumps(results_dict))
# endregion
if training_args.output_dir is not None and not training_args.push_to_hub:
# If we're not pushing to hub, at least save a local copy when we're done
model.save_pretrained(training_args.output_dir)
if __name__ == "__main__":
main()
| transformers/examples/tensorflow/language-modeling/run_mlm.py/0 | {
"file_path": "transformers/examples/tensorflow/language-modeling/run_mlm.py",
"repo_id": "transformers",
"token_count": 12796
} | 299 |
<!---
Copyright 2021 The HuggingFace Team. All rights reserved.
Licensed under the Apache License, Version 2.0 (the "License");
you may not use this file except in compliance with the License.
You may obtain a copy of the License at
http://www.apache.org/licenses/LICENSE-2.0
Unless required by applicable law or agreed to in writing, software
distributed under the License is distributed on an "AS IS" BASIS,
WITHOUT WARRANTIES OR CONDITIONS OF ANY KIND, either express or implied.
See the License for the specific language governing permissions and
limitations under the License.
-->
# Token classification
Fine-tuning the library models for token classification task such as Named Entity Recognition (NER), Parts-of-speech
tagging (POS) or phrase extraction (CHUNKS). The main script `run_ner.py` leverages the [๐ค Datasets](https://github.com/huggingface/datasets) library. You can easily
customize it to your needs if you need extra processing on your datasets.
It will either run on a datasets hosted on our [hub](https://huggingface.co/datasets) or with your own text files for
training and validation, you might just need to add some tweaks in the data preprocessing.
The following example fine-tunes BERT on CoNLL-2003:
```bash
python run_ner.py \
--model_name_or_path bert-base-uncased \
--dataset_name conll2003 \
--output_dir /tmp/test-ner
```
To run on your own training and validation files, use the following command:
```bash
python run_ner.py \
--model_name_or_path bert-base-uncased \
--train_file path_to_train_file \
--validation_file path_to_validation_file \
--output_dir /tmp/test-ner
```
**Note:** This script only works with models that have a fast tokenizer (backed by the [๐ค Tokenizers](https://github.com/huggingface/tokenizers) library) as it
uses special features of those tokenizers. You can check if your favorite model has a fast tokenizer in
[this table](https://huggingface.co/transformers/index.html#supported-frameworks).
| transformers/examples/tensorflow/token-classification/README.md/0 | {
"file_path": "transformers/examples/tensorflow/token-classification/README.md",
"repo_id": "transformers",
"token_count": 573
} | 300 |
#!/usr/bin/env bash
# Copyright 2020 The HuggingFace Team. All rights reserved.
#
# Licensed under the Apache License, Version 2.0 (the "License");
# you may not use this file except in compliance with the License.
# You may obtain a copy of the License at
#
# http://www.apache.org/licenses/LICENSE-2.0
#
# Unless required by applicable law or agreed to in writing, software
# distributed under the License is distributed on an "AS IS" BASIS,
# WITHOUT WARRANTIES OR CONDITIONS OF ANY KIND, either express or implied.
# See the License for the specific language governing permissions and
# limitations under the License.
# this script evals the following fsmt models
# it covers:
# - allenai/wmt16-en-de-dist-12-1
# - allenai/wmt16-en-de-dist-6-1
# - allenai/wmt16-en-de-12-1
# this script needs to be run from the top level of the transformers repo
if [ ! -d "src/transformers" ]; then
echo "Error: This script needs to be run from the top of the transformers repo"
exit 1
fi
# In these scripts you may have to lower BS if you get CUDA OOM (or increase it if you have a large GPU)
### Normal eval ###
export PAIR=en-de
export DATA_DIR=data/$PAIR
export SAVE_DIR=data/$PAIR
export BS=64
export NUM_BEAMS=5
mkdir -p $DATA_DIR
sacrebleu -t wmt19 -l $PAIR --echo src > $DATA_DIR/val.source
sacrebleu -t wmt19 -l $PAIR --echo ref > $DATA_DIR/val.target
MODEL_PATH=allenai/wmt16-en-de-dist-12-1
echo $PAIR $MODEL_PATH
PYTHONPATH="src:examples/seq2seq" python examples/seq2seq/run_eval.py $MODEL_PATH $DATA_DIR/val.source $SAVE_DIR/test_translations.txt --reference_path $DATA_DIR/val.target --score_path $SAVE_DIR/test_bleu.json --bs $BS --task translation --num_beams $NUM_BEAMS
MODEL_PATH=allenai/wmt16-en-de-dist-6-1
echo $PAIR $MODEL_PATH
PYTHONPATH="src:examples/seq2seq" python examples/seq2seq/run_eval.py $MODEL_PATH $DATA_DIR/val.source $SAVE_DIR/test_translations.txt --reference_path $DATA_DIR/val.target --score_path $SAVE_DIR/test_bleu.json --bs $BS --task translation --num_beams $NUM_BEAMS
MODEL_PATH=allenai/wmt16-en-de-12-1
echo $PAIR $MODEL_PATH
PYTHONPATH="src:examples/seq2seq" python examples/seq2seq/run_eval.py $MODEL_PATH $DATA_DIR/val.source $SAVE_DIR/test_translations.txt --reference_path $DATA_DIR/val.target --score_path $SAVE_DIR/test_bleu.json --bs $BS --task translation --num_beams $NUM_BEAMS
### Searching hparams eval ###
export PAIR=en-de
export DATA_DIR=data/$PAIR
export SAVE_DIR=data/$PAIR
export BS=32
export NUM_BEAMS=5
mkdir -p $DATA_DIR
sacrebleu -t wmt19 -l $PAIR --echo src > $DATA_DIR/val.source
sacrebleu -t wmt19 -l $PAIR --echo ref > $DATA_DIR/val.target
MODEL_PATH=allenai/wmt16-en-de-dist-12-1
echo $PAIR $MODEL_PATH
PYTHONPATH="src:examples/seq2seq" python examples/seq2seq/run_eval_search.py $MODEL_PATH $DATA_DIR/val.source $SAVE_DIR/test_translations.txt --reference_path $DATA_DIR/val.target --score_path $SAVE_DIR/test_bleu.json --bs $BS --task translation --search="num_beams=5:10:15 length_penalty=0.6:0.7:0.8:0.9:1.0:1.1"
MODEL_PATH=allenai/wmt16-en-de-dist-6-1
echo $PAIR $MODEL_PATH
PYTHONPATH="src:examples/seq2seq" python examples/seq2seq/run_eval_search.py $MODEL_PATH $DATA_DIR/val.source $SAVE_DIR/test_translations.txt --reference_path $DATA_DIR/val.target --score_path $SAVE_DIR/test_bleu.json --bs $BS --task translation --search="num_beams=5:10:15 length_penalty=0.6:0.7:0.8:0.9:1.0:1.1"
MODEL_PATH=allenai/wmt16-en-de-12-1
echo $PAIR $MODEL_PATH
PYTHONPATH="src:examples/seq2seq" python examples/seq2seq/run_eval_search.py $MODEL_PATH $DATA_DIR/val.source $SAVE_DIR/test_translations.txt --reference_path $DATA_DIR/val.target --score_path $SAVE_DIR/test_bleu.json --bs $BS --task translation --search="num_beams=5:10:15 length_penalty=0.6:0.7:0.8:0.9:1.0:1.1"
| transformers/scripts/fsmt/eval-allenai-wmt16.sh/0 | {
"file_path": "transformers/scripts/fsmt/eval-allenai-wmt16.sh",
"repo_id": "transformers",
"token_count": 1452
} | 301 |
# Copyright 2020 The HuggingFace Team. All rights reserved.
#
# Licensed under the Apache License, Version 2.0 (the "License");
# you may not use this file except in compliance with the License.
# You may obtain a copy of the License at
#
# http://www.apache.org/licenses/LICENSE-2.0
#
# Unless required by applicable law or agreed to in writing, software
# distributed under the License is distributed on an "AS IS" BASIS,
# WITHOUT WARRANTIES OR CONDITIONS OF ANY KIND, either express or implied.
# See the License for the specific language governing permissions and
# limitations under the License.
import math
import warnings
from collections import OrderedDict
import torch
from packaging import version
from torch import Tensor, nn
from .utils import logging
logger = logging.get_logger(__name__)
class PytorchGELUTanh(nn.Module):
"""
A fast C implementation of the tanh approximation of the GeLU activation function. See
https://arxiv.org/abs/1606.08415.
This implementation is equivalent to NewGELU and FastGELU but much faster. However, it is not an exact numerical
match due to rounding errors.
"""
def __init__(self):
super().__init__()
if version.parse(torch.__version__) < version.parse("1.12.0"):
raise ImportError(
f"You are using torch=={torch.__version__}, but torch>=1.12.0 is required to use "
"PytorchGELUTanh. Please upgrade torch."
)
def forward(self, input: Tensor) -> Tensor:
return nn.functional.gelu(input, approximate="tanh")
class NewGELUActivation(nn.Module):
"""
Implementation of the GELU activation function currently in Google BERT repo (identical to OpenAI GPT). Also see
the Gaussian Error Linear Units paper: https://arxiv.org/abs/1606.08415
"""
def forward(self, input: Tensor) -> Tensor:
return 0.5 * input * (1.0 + torch.tanh(math.sqrt(2.0 / math.pi) * (input + 0.044715 * torch.pow(input, 3.0))))
class GELUActivation(nn.Module):
"""
Original Implementation of the GELU activation function in Google BERT repo when initially created. For
information: OpenAI GPT's GELU is slightly different (and gives slightly different results): 0.5 * x * (1 +
torch.tanh(math.sqrt(2 / math.pi) * (x + 0.044715 * torch.pow(x, 3)))) This is now written in C in nn.functional
Also see the Gaussian Error Linear Units paper: https://arxiv.org/abs/1606.08415
"""
def __init__(self, use_gelu_python: bool = False):
super().__init__()
if use_gelu_python:
self.act = self._gelu_python
else:
self.act = nn.functional.gelu
def _gelu_python(self, input: Tensor) -> Tensor:
return input * 0.5 * (1.0 + torch.erf(input / math.sqrt(2.0)))
def forward(self, input: Tensor) -> Tensor:
return self.act(input)
class FastGELUActivation(nn.Module):
"""
Applies GELU approximation that is slower than QuickGELU but more accurate. See: https://github.com/hendrycks/GELUs
"""
def forward(self, input: Tensor) -> Tensor:
return 0.5 * input * (1.0 + torch.tanh(input * 0.7978845608 * (1.0 + 0.044715 * input * input)))
class QuickGELUActivation(nn.Module):
"""
Applies GELU approximation that is fast but somewhat inaccurate. See: https://github.com/hendrycks/GELUs
"""
def forward(self, input: Tensor) -> Tensor:
return input * torch.sigmoid(1.702 * input)
class ClippedGELUActivation(nn.Module):
"""
Clip the range of possible GeLU outputs between [min, max]. This is especially useful for quantization purpose, as
it allows mapping negatives values in the GeLU spectrum. For more information on this trick, please refer to
https://arxiv.org/abs/2004.09602.
Gaussian Error Linear Unit. Original Implementation of the gelu activation function in Google Bert repo when
initially created.
For information: OpenAI GPT's gelu is slightly different (and gives slightly different results): 0.5 * x * (1 +
torch.tanh(math.sqrt(2 / math.pi) * (x + 0.044715 * torch.pow(x, 3)))). See https://arxiv.org/abs/1606.08415
"""
def __init__(self, min: float, max: float):
if min > max:
raise ValueError(f"min should be < max (got min: {min}, max: {max})")
super().__init__()
self.min = min
self.max = max
def forward(self, x: Tensor) -> Tensor:
return torch.clip(gelu(x), self.min, self.max)
class AccurateGELUActivation(nn.Module):
"""
Applies GELU approximation that is faster than default and more accurate than QuickGELU. See:
https://github.com/hendrycks/GELUs
Implemented along with MEGA (Moving Average Equipped Gated Attention)
"""
def __init__(self):
super().__init__()
self.precomputed_constant = math.sqrt(2 / math.pi)
def forward(self, input: Tensor) -> Tensor:
return 0.5 * input * (1 + torch.tanh(self.precomputed_constant * (input + 0.044715 * torch.pow(input, 3))))
class SiLUActivation(nn.SiLU):
def __init__(self, *args, **kwargs):
warnings.warn(
"The SiLUActivation class has been deprecated and will be removed in v4.39. Please use nn.SiLU instead.",
)
super().__init__(*args, **kwargs)
class MishActivation(nn.Module):
"""
See Mish: A Self-Regularized Non-Monotonic Activation Function (Misra., https://arxiv.org/abs/1908.08681). Also
visit the official repository for the paper: https://github.com/digantamisra98/Mish
"""
def __init__(self):
super().__init__()
if version.parse(torch.__version__) < version.parse("1.9.0"):
self.act = self._mish_python
else:
self.act = nn.functional.mish
def _mish_python(self, input: Tensor) -> Tensor:
return input * torch.tanh(nn.functional.softplus(input))
def forward(self, input: Tensor) -> Tensor:
return self.act(input)
class LinearActivation(nn.Module):
"""
Applies the linear activation function, i.e. forwarding input directly to output.
"""
def forward(self, input: Tensor) -> Tensor:
return input
class LaplaceActivation(nn.Module):
"""
Applies elementwise activation based on Laplace function, introduced in MEGA as an attention activation. See
https://arxiv.org/abs/2209.10655
Inspired by squared relu, but with bounded range and gradient for better stability
"""
def forward(self, input, mu=0.707107, sigma=0.282095):
input = (input - mu).div(sigma * math.sqrt(2.0))
return 0.5 * (1.0 + torch.erf(input))
class ReLUSquaredActivation(nn.Module):
"""
Applies the relu^2 activation introduced in https://arxiv.org/abs/2109.08668v2
"""
def forward(self, input):
relu_applied = nn.functional.relu(input)
squared = torch.square(relu_applied)
return squared
class ClassInstantier(OrderedDict):
def __getitem__(self, key):
content = super().__getitem__(key)
cls, kwargs = content if isinstance(content, tuple) else (content, {})
return cls(**kwargs)
ACT2CLS = {
"gelu": GELUActivation,
"gelu_10": (ClippedGELUActivation, {"min": -10, "max": 10}),
"gelu_fast": FastGELUActivation,
"gelu_new": NewGELUActivation,
"gelu_python": (GELUActivation, {"use_gelu_python": True}),
"gelu_pytorch_tanh": PytorchGELUTanh,
"gelu_accurate": AccurateGELUActivation,
"laplace": LaplaceActivation,
"leaky_relu": nn.LeakyReLU,
"linear": LinearActivation,
"mish": MishActivation,
"quick_gelu": QuickGELUActivation,
"relu": nn.ReLU,
"relu2": ReLUSquaredActivation,
"relu6": nn.ReLU6,
"sigmoid": nn.Sigmoid,
"silu": nn.SiLU,
"swish": nn.SiLU,
"tanh": nn.Tanh,
}
ACT2FN = ClassInstantier(ACT2CLS)
def get_activation(activation_string):
if activation_string in ACT2FN:
return ACT2FN[activation_string]
else:
raise KeyError(f"function {activation_string} not found in ACT2FN mapping {list(ACT2FN.keys())}")
# For backwards compatibility with: from activations import gelu_python
gelu_python = get_activation("gelu_python")
gelu_new = get_activation("gelu_new")
gelu = get_activation("gelu")
gelu_fast = get_activation("gelu_fast")
quick_gelu = get_activation("quick_gelu")
silu = get_activation("silu")
mish = get_activation("mish")
linear_act = get_activation("linear")
| transformers/src/transformers/activations.py/0 | {
"file_path": "transformers/src/transformers/activations.py",
"repo_id": "transformers",
"token_count": 3234
} | 302 |
# Copyright 2020 The HuggingFace Team. All rights reserved.
#
# Licensed under the Apache License, Version 2.0 (the "License");
# you may not use this file except in compliance with the License.
# You may obtain a copy of the License at
#
# http://www.apache.org/licenses/LICENSE-2.0
#
# Unless required by applicable law or agreed to in writing, software
# distributed under the License is distributed on an "AS IS" BASIS,
# WITHOUT WARRANTIES OR CONDITIONS OF ANY KIND, either express or implied.
# See the License for the specific language governing permissions and
# limitations under the License.
import importlib.util
import os
import platform
from argparse import ArgumentParser
import huggingface_hub
from .. import __version__ as version
from ..utils import (
is_accelerate_available,
is_flax_available,
is_safetensors_available,
is_tf_available,
is_torch_available,
)
from . import BaseTransformersCLICommand
def info_command_factory(_):
return EnvironmentCommand()
def download_command_factory(args):
return EnvironmentCommand(args.accelerate_config_file)
class EnvironmentCommand(BaseTransformersCLICommand):
@staticmethod
def register_subcommand(parser: ArgumentParser):
download_parser = parser.add_parser("env")
download_parser.set_defaults(func=info_command_factory)
download_parser.add_argument(
"--accelerate-config_file",
default=None,
help="The accelerate config file to use for the default values in the launching script.",
)
download_parser.set_defaults(func=download_command_factory)
def __init__(self, accelerate_config_file, *args) -> None:
self._accelerate_config_file = accelerate_config_file
def run(self):
safetensors_version = "not installed"
if is_safetensors_available():
import safetensors
safetensors_version = safetensors.__version__
elif importlib.util.find_spec("safetensors") is not None:
import safetensors
safetensors_version = f"{safetensors.__version__} but is ignored because of PyTorch version too old."
accelerate_version = "not installed"
accelerate_config = accelerate_config_str = "not found"
if is_accelerate_available():
import accelerate
from accelerate.commands.config import default_config_file, load_config_from_file
accelerate_version = accelerate.__version__
# Get the default from the config file.
if self._accelerate_config_file is not None or os.path.isfile(default_config_file):
accelerate_config = load_config_from_file(self._accelerate_config_file).to_dict()
accelerate_config_str = (
"\n".join([f"\t- {prop}: {val}" for prop, val in accelerate_config.items()])
if isinstance(accelerate_config, dict)
else f"\t{accelerate_config}"
)
pt_version = "not installed"
pt_cuda_available = "NA"
if is_torch_available():
import torch
pt_version = torch.__version__
pt_cuda_available = torch.cuda.is_available()
tf_version = "not installed"
tf_cuda_available = "NA"
if is_tf_available():
import tensorflow as tf
tf_version = tf.__version__
try:
# deprecated in v2.1
tf_cuda_available = tf.test.is_gpu_available()
except AttributeError:
# returns list of devices, convert to bool
tf_cuda_available = bool(tf.config.list_physical_devices("GPU"))
flax_version = "not installed"
jax_version = "not installed"
jaxlib_version = "not installed"
jax_backend = "NA"
if is_flax_available():
import flax
import jax
import jaxlib
flax_version = flax.__version__
jax_version = jax.__version__
jaxlib_version = jaxlib.__version__
jax_backend = jax.lib.xla_bridge.get_backend().platform
info = {
"`transformers` version": version,
"Platform": platform.platform(),
"Python version": platform.python_version(),
"Huggingface_hub version": huggingface_hub.__version__,
"Safetensors version": f"{safetensors_version}",
"Accelerate version": f"{accelerate_version}",
"Accelerate config": f"{accelerate_config_str}",
"PyTorch version (GPU?)": f"{pt_version} ({pt_cuda_available})",
"Tensorflow version (GPU?)": f"{tf_version} ({tf_cuda_available})",
"Flax version (CPU?/GPU?/TPU?)": f"{flax_version} ({jax_backend})",
"Jax version": f"{jax_version}",
"JaxLib version": f"{jaxlib_version}",
"Using GPU in script?": "<fill in>",
"Using distributed or parallel set-up in script?": "<fill in>",
}
print("\nCopy-and-paste the text below in your GitHub issue and FILL OUT the two last points.\n")
print(self.format_dict(info))
return info
@staticmethod
def format_dict(d):
return "\n".join([f"- {prop}: {val}" for prop, val in d.items()]) + "\n"
| transformers/src/transformers/commands/env.py/0 | {
"file_path": "transformers/src/transformers/commands/env.py",
"repo_id": "transformers",
"token_count": 2227
} | 303 |
# coding=utf-8
# Copyright 2021 The HuggingFace Inc. team.
#
# Licensed under the Apache License, Version 2.0 (the "License");
# you may not use this file except in compliance with the License.
# You may obtain a copy of the License at
#
# http://www.apache.org/licenses/LICENSE-2.0
#
# Unless required by applicable law or agreed to in writing, software
# distributed under the License is distributed on an "AS IS" BASIS,
# WITHOUT WARRANTIES OR CONDITIONS OF ANY KIND, either express or implied.
# See the License for the specific language governing permissions and
# limitations under the License.
"""
Sequence feature extraction class for common feature extractors to preprocess sequences.
"""
from typing import Dict, List, Optional, Union
import numpy as np
from .feature_extraction_utils import BatchFeature, FeatureExtractionMixin
from .utils import PaddingStrategy, TensorType, is_tf_tensor, is_torch_tensor, logging, to_numpy
logger = logging.get_logger(__name__)
class SequenceFeatureExtractor(FeatureExtractionMixin):
"""
This is a general feature extraction class for speech recognition.
Args:
feature_size (`int`):
The feature dimension of the extracted features.
sampling_rate (`int`):
The sampling rate at which the audio files should be digitalized expressed in hertz (Hz).
padding_value (`float`):
The value that is used to fill the padding values / vectors.
"""
def __init__(self, feature_size: int, sampling_rate: int, padding_value: float, **kwargs):
self.feature_size = feature_size
self.sampling_rate = sampling_rate
self.padding_value = padding_value
self.padding_side = kwargs.pop("padding_side", "right")
self.return_attention_mask = kwargs.pop("return_attention_mask", True)
super().__init__(**kwargs)
def pad(
self,
processed_features: Union[
BatchFeature,
List[BatchFeature],
Dict[str, BatchFeature],
Dict[str, List[BatchFeature]],
List[Dict[str, BatchFeature]],
],
padding: Union[bool, str, PaddingStrategy] = True,
max_length: Optional[int] = None,
truncation: bool = False,
pad_to_multiple_of: Optional[int] = None,
return_attention_mask: Optional[bool] = None,
return_tensors: Optional[Union[str, TensorType]] = None,
) -> BatchFeature:
"""
Pad input values / input vectors or a batch of input values / input vectors up to predefined length or to the
max sequence length in the batch.
Padding side (left/right) padding values are defined at the feature extractor level (with `self.padding_side`,
`self.padding_value`)
<Tip>
If the `processed_features` passed are dictionary of numpy arrays, PyTorch tensors or TensorFlow tensors, the
result will use the same type unless you provide a different tensor type with `return_tensors`. In the case of
PyTorch tensors, you will lose the specific device of your tensors however.
</Tip>
Args:
processed_features ([`BatchFeature`], list of [`BatchFeature`], `Dict[str, List[float]]`, `Dict[str, List[List[float]]` or `List[Dict[str, List[float]]]`):
Processed inputs. Can represent one input ([`BatchFeature`] or `Dict[str, List[float]]`) or a batch of
input values / vectors (list of [`BatchFeature`], *Dict[str, List[List[float]]]* or *List[Dict[str,
List[float]]]*) so you can use this method during preprocessing as well as in a PyTorch Dataloader
collate function.
Instead of `List[float]` you can have tensors (numpy arrays, PyTorch tensors or TensorFlow tensors),
see the note above for the return type.
padding (`bool`, `str` or [`~utils.PaddingStrategy`], *optional*, defaults to `True`):
Select a strategy to pad the returned sequences (according to the model's padding side and padding
index) among:
- `True` or `'longest'`: Pad to the longest sequence in the batch (or no padding if only a single
sequence if provided).
- `'max_length'`: Pad to a maximum length specified with the argument `max_length` or to the maximum
acceptable input length for the model if that argument is not provided.
- `False` or `'do_not_pad'` (default): No padding (i.e., can output a batch with sequences of different
lengths).
max_length (`int`, *optional*):
Maximum length of the returned list and optionally padding length (see above).
truncation (`bool`):
Activates truncation to cut input sequences longer than `max_length` to `max_length`.
pad_to_multiple_of (`int`, *optional*):
If set will pad the sequence to a multiple of the provided value.
This is especially useful to enable the use of Tensor Cores on NVIDIA hardware with compute capability
`>= 7.5` (Volta), or on TPUs which benefit from having sequence lengths be a multiple of 128.
return_attention_mask (`bool`, *optional*):
Whether to return the attention mask. If left to the default, will return the attention mask according
to the specific feature_extractor's default.
[What are attention masks?](../glossary#attention-mask)
return_tensors (`str` or [`~utils.TensorType`], *optional*):
If set, will return tensors instead of list of python integers. Acceptable values are:
- `'tf'`: Return TensorFlow `tf.constant` objects.
- `'pt'`: Return PyTorch `torch.Tensor` objects.
- `'np'`: Return Numpy `np.ndarray` objects.
"""
# If we have a list of dicts, let's convert it in a dict of lists
# We do this to allow using this method as a collate_fn function in PyTorch Dataloader
if isinstance(processed_features, (list, tuple)) and isinstance(processed_features[0], (dict, BatchFeature)):
processed_features = {
key: [example[key] for example in processed_features] for key in processed_features[0].keys()
}
# The model's main input name, usually `input_values`, has be passed for padding
if self.model_input_names[0] not in processed_features:
raise ValueError(
"You should supply an instance of `transformers.BatchFeature` or list of `transformers.BatchFeature`"
f" to this method that includes {self.model_input_names[0]}, but you provided"
f" {list(processed_features.keys())}"
)
required_input = processed_features[self.model_input_names[0]]
return_attention_mask = (
return_attention_mask if return_attention_mask is not None else self.return_attention_mask
)
if len(required_input) == 0:
if return_attention_mask:
processed_features["attention_mask"] = []
return processed_features
# If we have PyTorch/TF tensors or lists as inputs, we cast them as Numpy arrays
# and rebuild them afterwards if no return_tensors is specified
# Note that we lose the specific device the tensor may be on for PyTorch
first_element = required_input[0]
if isinstance(first_element, (list, tuple)):
# first_element might be an empty list/tuple in some edge cases so we grab the first non empty element.
index = 0
while len(required_input[index]) == 0:
index += 1
if index < len(required_input):
first_element = required_input[index][0]
if return_tensors is None:
if is_tf_tensor(first_element):
return_tensors = "tf"
elif is_torch_tensor(first_element):
return_tensors = "pt"
elif isinstance(first_element, (int, float, list, tuple, np.ndarray)):
return_tensors = "np"
else:
raise ValueError(
f"type of {first_element} unknown: {type(first_element)}. "
"Should be one of a python, numpy, pytorch or tensorflow object."
)
for key, value in processed_features.items():
if isinstance(value[0], (int, float)):
processed_features[key] = to_numpy(value)
else:
processed_features[key] = [to_numpy(v) for v in value]
# Convert padding_strategy in PaddingStrategy
padding_strategy = self._get_padding_strategies(padding=padding, max_length=max_length)
required_input = processed_features[self.model_input_names[0]]
batch_size = len(required_input)
if not all(len(v) == batch_size for v in processed_features.values()):
raise ValueError("Some items in the output dictionary have a different batch size than others.")
truncated_inputs = []
for i in range(batch_size):
inputs = {k: v[i] for k, v in processed_features.items()}
# truncation
inputs_slice = self._truncate(
inputs,
max_length=max_length,
pad_to_multiple_of=pad_to_multiple_of,
truncation=truncation,
)
truncated_inputs.append(inputs_slice)
if padding_strategy == PaddingStrategy.LONGEST:
# make sure that `max_length` cannot be longer than the longest truncated length
max_length = max(len(input_slice[self.model_input_names[0]]) for input_slice in truncated_inputs)
padding_strategy = PaddingStrategy.MAX_LENGTH
batch_outputs = {}
for i in range(batch_size):
# padding
outputs = self._pad(
truncated_inputs[i],
max_length=max_length,
padding_strategy=padding_strategy,
pad_to_multiple_of=pad_to_multiple_of,
return_attention_mask=return_attention_mask,
)
for key, value in outputs.items():
if key not in batch_outputs:
batch_outputs[key] = []
if value.dtype is np.dtype(np.float64):
value = value.astype(np.float32)
batch_outputs[key].append(value)
return BatchFeature(batch_outputs, tensor_type=return_tensors)
def _pad(
self,
processed_features: Union[Dict[str, np.ndarray], BatchFeature],
max_length: Optional[int] = None,
padding_strategy: PaddingStrategy = PaddingStrategy.DO_NOT_PAD,
pad_to_multiple_of: Optional[int] = None,
return_attention_mask: Optional[bool] = None,
) -> dict:
"""
Pad inputs (on left/right and up to predefined length or max length in the batch)
Args:
processed_features (`Union[Dict[str, np.ndarray], BatchFeature]`):
Dictionary of input values (`np.ndarray[float]`) / input vectors (`List[np.ndarray[float]]`) or batch
of inputs values (`List[np.ndarray[int]]`) / input vectors (`List[np.ndarray[int]]`)
max_length (`int`, *optional*):
Maximum length of the returned list and optionally padding length (see below)
padding_strategy (`PaddingStrategy`, *optional*, default to `PaddingStrategy.DO_NOT_PAD`):
PaddingStrategy to use for padding.
- PaddingStrategy.LONGEST Pad to the longest sequence in the batch
- PaddingStrategy.MAX_LENGTH: Pad to the max length (default)
- PaddingStrategy.DO_NOT_PAD: Do not pad
The feature_extractor padding sides are defined in self.padding_side:
- 'left': pads on the left of the sequences
- 'right': pads on the right of the sequences
pad_to_multiple_of (`int`, *optional*):
Integer if set will pad the sequence to a multiple of the provided value. This is especially useful to
enable the use of Tensor Core on NVIDIA hardware with compute capability `>= 7.5` (Volta), or on TPUs
which benefit from having sequence lengths be a multiple of 128.
return_attention_mask (`bool`, *optional*):
Set to False to avoid returning attention mask (default: set to model specifics)
"""
required_input = processed_features[self.model_input_names[0]]
if padding_strategy == PaddingStrategy.LONGEST:
max_length = len(required_input)
if max_length is not None and pad_to_multiple_of is not None and (max_length % pad_to_multiple_of != 0):
max_length = ((max_length // pad_to_multiple_of) + 1) * pad_to_multiple_of
needs_to_be_padded = padding_strategy != PaddingStrategy.DO_NOT_PAD and len(required_input) < max_length
if return_attention_mask and "attention_mask" not in processed_features:
processed_features["attention_mask"] = np.ones(len(required_input), dtype=np.int32)
if needs_to_be_padded:
difference = max_length - len(required_input)
if self.padding_side == "right":
if return_attention_mask:
processed_features["attention_mask"] = np.pad(
processed_features["attention_mask"], (0, difference)
)
padding_shape = ((0, difference), (0, 0)) if self.feature_size > 1 else (0, difference)
processed_features[self.model_input_names[0]] = np.pad(
required_input, padding_shape, "constant", constant_values=self.padding_value
)
elif self.padding_side == "left":
if return_attention_mask:
processed_features["attention_mask"] = np.pad(
processed_features["attention_mask"], (difference, 0)
)
padding_shape = ((difference, 0), (0, 0)) if self.feature_size > 1 else (difference, 0)
processed_features[self.model_input_names[0]] = np.pad(
required_input, padding_shape, "constant", constant_values=self.padding_value
)
else:
raise ValueError("Invalid padding strategy:" + str(self.padding_side))
return processed_features
def _truncate(
self,
processed_features: Union[Dict[str, np.ndarray], BatchFeature],
max_length: Optional[int] = None,
pad_to_multiple_of: Optional[int] = None,
truncation: Optional[bool] = None,
):
"""
Truncate inputs to predefined length or max length in the batch
Args:
processed_features(`Union[Dict[str, np.ndarray], BatchFeature]`):
Dictionary of input values (`np.ndarray[float]`) / input vectors (`List[np.ndarray[float]]`) or batch
of inputs values (`List[np.ndarray[int]]`) / input vectors (`List[np.ndarray[int]]`)
max_length (`int`, *optional*):
maximum length of the returned list and optionally padding length (see below)
pad_to_multiple_of (`int`, *optional*) :
Integer if set will pad the sequence to a multiple of the provided value. This is especially useful to
enable the use of Tensor Core on NVIDIA hardware with compute capability `>= 7.5` (Volta), or on TPUs
which benefit from having sequence lengths be a multiple of 128.
truncation (`bool`, *optional*):
Activates truncation to cut input sequences longer than `max_length` to `max_length`.
"""
if not truncation:
return processed_features
elif truncation and max_length is None:
raise ValueError("When setting ``truncation=True``, make sure that ``max_length`` is defined.")
required_input = processed_features[self.model_input_names[0]]
# find `max_length` that fits `pad_to_multiple_of`
if max_length is not None and pad_to_multiple_of is not None and (max_length % pad_to_multiple_of != 0):
max_length = ((max_length // pad_to_multiple_of) + 1) * pad_to_multiple_of
needs_to_be_truncated = len(required_input) > max_length
if needs_to_be_truncated:
processed_features[self.model_input_names[0]] = processed_features[self.model_input_names[0]][:max_length]
if "attention_mask" in processed_features:
processed_features["attention_mask"] = processed_features["attention_mask"][:max_length]
return processed_features
def _get_padding_strategies(self, padding=False, max_length=None):
"""
Find the correct padding strategy
"""
# Get padding strategy
if padding is not False:
if padding is True:
padding_strategy = PaddingStrategy.LONGEST # Default to pad to the longest sequence in the batch
elif not isinstance(padding, PaddingStrategy):
padding_strategy = PaddingStrategy(padding)
elif isinstance(padding, PaddingStrategy):
padding_strategy = padding
else:
padding_strategy = PaddingStrategy.DO_NOT_PAD
# Set max length if needed
if max_length is None:
if padding_strategy == PaddingStrategy.MAX_LENGTH:
raise ValueError(
f"When setting ``padding={PaddingStrategy.MAX_LENGTH}``, make sure that max_length is defined"
)
# Test if we have a padding value
if padding_strategy != PaddingStrategy.DO_NOT_PAD and (self.padding_value is None):
raise ValueError(
"Asking to pad but the feature_extractor does not have a padding value. Please select a value to use"
" as `padding_value`. For example: `feature_extractor.padding_value = 0.0`."
)
return padding_strategy
| transformers/src/transformers/feature_extraction_sequence_utils.py/0 | {
"file_path": "transformers/src/transformers/feature_extraction_sequence_utils.py",
"repo_id": "transformers",
"token_count": 7735
} | 304 |
/*!
**************************************************************************************************
* Deformable DETR
* Copyright (c) 2020 SenseTime. All Rights Reserved.
* Licensed under the Apache License, Version 2.0 [see LICENSE for details]
**************************************************************************************************
* Modified from https://github.com/chengdazhi/Deformable-Convolution-V2-PyTorch/tree/pytorch_1.0.0
**************************************************************************************************
*/
#pragma once
#include <torch/extension.h>
at::Tensor
ms_deform_attn_cpu_forward(
const at::Tensor &value,
const at::Tensor &spatial_shapes,
const at::Tensor &level_start_index,
const at::Tensor &sampling_loc,
const at::Tensor &attn_weight,
const int im2col_step);
std::vector<at::Tensor>
ms_deform_attn_cpu_backward(
const at::Tensor &value,
const at::Tensor &spatial_shapes,
const at::Tensor &level_start_index,
const at::Tensor &sampling_loc,
const at::Tensor &attn_weight,
const at::Tensor &grad_output,
const int im2col_step);
| transformers/src/transformers/kernels/deformable_detr/cpu/ms_deform_attn_cpu.h/0 | {
"file_path": "transformers/src/transformers/kernels/deformable_detr/cpu/ms_deform_attn_cpu.h",
"repo_id": "transformers",
"token_count": 353
} | 305 |
#define MAX_THREADS_PER_BLOCK 1024
#define OPTIMAL_THREADS_PER_BLOCK 256
#define WARP_SIZE 32
#define MAX_NUM_BLOCK_X 2147483647
#define MAX_NUM_BLOCK_Y 65535
#define MAX_NUM_BLOCK_Z 65535
#define MAX_SHARED_MEM_PER_BLOCK 48000
#define FULL_MASK 0xffffffff
| transformers/src/transformers/kernels/yoso/common_cuda.h/0 | {
"file_path": "transformers/src/transformers/kernels/yoso/common_cuda.h",
"repo_id": "transformers",
"token_count": 110
} | 306 |
# coding=utf-8
# Copyright 2018 The Google AI Language Team Authors, Facebook AI Research authors and The HuggingFace Inc. team.
# Copyright (c) 2018, NVIDIA CORPORATION. All rights reserved.
#
# Licensed under the Apache License, Version 2.0 (the "License");
# you may not use this file except in compliance with the License.
# You may obtain a copy of the License at
#
# http://www.apache.org/licenses/LICENSE-2.0
#
# Unless required by applicable law or agreed to in writing, software
# distributed under the License is distributed on an "AS IS" BASIS,
# WITHOUT WARRANTIES OR CONDITIONS OF ANY KIND, either express or implied.
# See the License for the specific language governing permissions and
# limitations under the License.
import collections
import copy
import functools
import gc
import importlib.metadata
import inspect
import itertools
import json
import os
import re
import shutil
import tempfile
import warnings
from contextlib import contextmanager
from dataclasses import dataclass
from functools import partial, wraps
from typing import Any, Callable, Dict, List, Optional, Tuple, Union
from zipfile import is_zipfile
import torch
from packaging import version
from torch import Tensor, nn
from torch.nn import CrossEntropyLoss, Identity
from torch.utils.checkpoint import checkpoint
from .activations import get_activation
from .configuration_utils import PretrainedConfig
from .dynamic_module_utils import custom_object_save
from .generation import GenerationConfig, GenerationMixin
from .integrations import PeftAdapterMixin, deepspeed_config, is_deepspeed_zero3_enabled
from .pytorch_utils import ( # noqa: F401
Conv1D,
apply_chunking_to_forward,
find_pruneable_heads_and_indices,
id_tensor_storage,
is_torch_greater_or_equal_than_1_13,
prune_conv1d_layer,
prune_layer,
prune_linear_layer,
)
from .quantizers import AutoHfQuantizer, HfQuantizer
from .safetensors_conversion import auto_conversion
from .utils import (
ADAPTER_SAFE_WEIGHTS_NAME,
ADAPTER_WEIGHTS_NAME,
CONFIG_NAME,
DUMMY_INPUTS,
FLAX_WEIGHTS_NAME,
SAFE_WEIGHTS_INDEX_NAME,
SAFE_WEIGHTS_NAME,
TF2_WEIGHTS_NAME,
TF_WEIGHTS_NAME,
WEIGHTS_INDEX_NAME,
WEIGHTS_NAME,
ContextManagers,
ModelOutput,
PushToHubMixin,
cached_file,
copy_func,
download_url,
extract_commit_hash,
has_file,
is_accelerate_available,
is_bitsandbytes_available,
is_flash_attn_2_available,
is_offline_mode,
is_optimum_available,
is_peft_available,
is_remote_url,
is_safetensors_available,
is_torch_sdpa_available,
is_torch_tpu_available,
logging,
replace_return_docstrings,
strtobool,
)
from .utils.hub import convert_file_size_to_int, create_and_tag_model_card, get_checkpoint_shard_files
from .utils.import_utils import (
ENV_VARS_TRUE_VALUES,
is_sagemaker_mp_enabled,
is_torch_fx_proxy,
is_torchdynamo_compiling,
)
from .utils.quantization_config import BitsAndBytesConfig, QuantizationMethod
XLA_USE_BF16 = os.environ.get("XLA_USE_BF16", "0").upper()
XLA_DOWNCAST_BF16 = os.environ.get("XLA_DOWNCAST_BF16", "0").upper()
if is_accelerate_available():
from accelerate import dispatch_model, infer_auto_device_map, init_empty_weights
from accelerate.hooks import add_hook_to_module
from accelerate.utils import (
check_tied_parameters_on_same_device,
find_tied_parameters,
get_balanced_memory,
get_max_memory,
load_offloaded_weights,
offload_weight,
save_offload_index,
set_module_tensor_to_device,
)
if is_safetensors_available():
from safetensors import safe_open
from safetensors.torch import load_file as safe_load_file
from safetensors.torch import save_file as safe_save_file
logger = logging.get_logger(__name__)
_init_weights = True
def is_fsdp_enabled():
return (
torch.distributed.is_available()
and torch.distributed.is_initialized()
and strtobool(os.environ.get("ACCELERATE_USE_FSDP", "False")) == 1
and strtobool(os.environ.get("FSDP_CPU_RAM_EFFICIENT_LOADING", "False")) == 1
)
def is_local_dist_rank_0():
return (
torch.distributed.is_available()
and torch.distributed.is_initialized()
and int(os.environ.get("LOCAL_RANK", -1)) == 0
)
if is_sagemaker_mp_enabled():
import smdistributed.modelparallel.torch as smp
from smdistributed.modelparallel import __version__ as SMP_VERSION
IS_SAGEMAKER_MP_POST_1_10 = version.parse(SMP_VERSION) >= version.parse("1.10")
else:
IS_SAGEMAKER_MP_POST_1_10 = False
if is_peft_available():
from .utils import find_adapter_config_file
TORCH_INIT_FUNCTIONS = {
"uniform_": nn.init.uniform_,
"normal_": nn.init.normal_,
"trunc_normal_": nn.init.trunc_normal_,
"constant_": nn.init.constant_,
"xavier_uniform_": nn.init.xavier_uniform_,
"xavier_normal_": nn.init.xavier_normal_,
"kaiming_uniform_": nn.init.kaiming_uniform_,
"kaiming_normal_": nn.init.kaiming_normal_,
"uniform": nn.init.uniform,
"normal": nn.init.normal,
"xavier_uniform": nn.init.xavier_uniform,
"xavier_normal": nn.init.xavier_normal,
"kaiming_uniform": nn.init.kaiming_uniform,
"kaiming_normal": nn.init.kaiming_normal,
}
@contextmanager
def no_init_weights(_enable=True):
"""
Context manager to globally disable weight initialization to speed up loading large models.
TODO(Patrick): Delete safety argument `_enable=True` at next major version. .
"""
global _init_weights
old_init_weights = _init_weights
if _enable:
_init_weights = False
def _skip_init(*args, **kwargs):
pass
# # Save the original initialization functions
for name, init_func in TORCH_INIT_FUNCTIONS.items():
setattr(torch.nn.init, name, _skip_init)
try:
yield
finally:
_init_weights = old_init_weights
if _enable:
# # Restore the original initialization functions
for name, init_func in TORCH_INIT_FUNCTIONS.items():
setattr(torch.nn.init, name, init_func)
def get_parameter_device(parameter: Union[nn.Module, GenerationMixin, "ModuleUtilsMixin"]):
try:
return next(parameter.parameters()).device
except StopIteration:
# For nn.DataParallel compatibility in PyTorch 1.5
def find_tensor_attributes(module: nn.Module) -> List[Tuple[str, Tensor]]:
tuples = [(k, v) for k, v in module.__dict__.items() if torch.is_tensor(v)]
return tuples
gen = parameter._named_members(get_members_fn=find_tensor_attributes)
first_tuple = next(gen)
return first_tuple[1].device
def get_first_parameter_dtype(parameter: Union[nn.Module, GenerationMixin, "ModuleUtilsMixin"]):
"""
Returns the first parameter dtype (can be non-floating) or asserts if none were found.
"""
try:
return next(parameter.parameters()).dtype
except StopIteration:
# For nn.DataParallel compatibility in PyTorch > 1.5
def find_tensor_attributes(module: nn.Module) -> List[Tuple[str, Tensor]]:
tuples = [(k, v) for k, v in module.__dict__.items() if torch.is_tensor(v)]
return tuples
gen = parameter._named_members(get_members_fn=find_tensor_attributes)
first_tuple = next(gen)
return first_tuple[1].dtype
def get_parameter_dtype(parameter: Union[nn.Module, GenerationMixin, "ModuleUtilsMixin"]):
"""
Returns the first found floating dtype in parameters if there is one, otherwise returns the last dtype it found.
"""
last_dtype = None
for t in parameter.parameters():
last_dtype = t.dtype
if t.is_floating_point():
# Adding fix for https://github.com/pytorch/xla/issues/4152
# Fixes issue where the model code passes a value that is out of range for XLA_USE_BF16=1
# and XLA_DOWNCAST_BF16=1 so the conversion would cast it to -inf
# NOTE: `is_torch_tpu_available()` is checked last as it induces a graph break in torch dynamo
if XLA_USE_BF16 in ENV_VARS_TRUE_VALUES and is_torch_tpu_available():
return torch.bfloat16
if XLA_DOWNCAST_BF16 in ENV_VARS_TRUE_VALUES and is_torch_tpu_available():
if t.dtype == torch.float:
return torch.bfloat16
if t.dtype == torch.double:
return torch.float32
return t.dtype
if last_dtype is not None:
# if no floating dtype was found return whatever the first dtype is
return last_dtype
# For nn.DataParallel compatibility in PyTorch > 1.5
def find_tensor_attributes(module: nn.Module) -> List[Tuple[str, Tensor]]:
tuples = [(k, v) for k, v in module.__dict__.items() if torch.is_tensor(v)]
return tuples
gen = parameter._named_members(get_members_fn=find_tensor_attributes)
last_tuple = None
for tuple in gen:
last_tuple = tuple
if tuple[1].is_floating_point():
return tuple[1].dtype
if last_tuple is not None:
# fallback to the last dtype
return last_tuple[1].dtype
# fallback to buffer dtype
for t in parameter.buffers():
last_dtype = t.dtype
if t.is_floating_point():
return t.dtype
return last_dtype
def get_state_dict_float_dtype(state_dict):
"""
Returns the first found floating dtype in `state_dict` or asserts if none were found.
"""
for t in state_dict.values():
if t.is_floating_point():
return t.dtype
raise ValueError("couldn't find any floating point dtypes in state_dict")
def get_state_dict_dtype(state_dict):
"""
Returns the first found floating dtype in `state_dict` if there is one, otherwise returns the first dtype.
"""
for t in state_dict.values():
if t.is_floating_point():
return t.dtype
# if no floating dtype was found return whatever the first dtype is
else:
return next(state_dict.values()).dtype
def dtype_byte_size(dtype):
"""
Returns the size (in bytes) occupied by one parameter of type `dtype`.
Example:
```py
>>> dtype_byte_size(torch.float32)
4
```
"""
if dtype == torch.bool:
return 1 / 8
bit_search = re.search(r"[^\d](\d+)$", str(dtype))
if bit_search is None:
raise ValueError(f"`dtype` is not a valid dtype: {dtype}.")
bit_size = int(bit_search.groups()[0])
return bit_size // 8
def shard_checkpoint(
state_dict: Dict[str, torch.Tensor], max_shard_size: Union[int, str] = "10GB", weights_name: str = WEIGHTS_NAME
):
"""
Splits a model state dictionary in sub-checkpoints so that the final size of each sub-checkpoint does not exceed a
given size.
The sub-checkpoints are determined by iterating through the `state_dict` in the order of its keys, so there is no
optimization made to make each sub-checkpoint as close as possible to the maximum size passed. For example, if the
limit is 10GB and we have weights of sizes [6GB, 6GB, 2GB, 6GB, 2GB, 2GB] they will get sharded as [6GB], [6+2GB],
[6+2+2GB] and not [6+2+2GB], [6+2GB], [6GB].
<Tip warning={true}>
If one of the model's weight is bigger than `max_shard_size`, it will end up in its own sub-checkpoint which will
have a size greater than `max_shard_size`.
</Tip>
Args:
state_dict (`Dict[str, torch.Tensor]`): The state dictionary of a model to save.
max_shard_size (`int` or `str`, *optional*, defaults to `"10GB"`):
The maximum size of each sub-checkpoint. If expressed as a string, needs to be digits followed by a unit
(like `"5MB"`).
weights_name (`str`, *optional*, defaults to `"pytorch_model.bin"`):
The name of the model save file.
"""
max_shard_size = convert_file_size_to_int(max_shard_size)
sharded_state_dicts = [{}]
last_block_size = 0
total_size = 0
storage_id_to_block = {}
for key, weight in state_dict.items():
# when bnb serialization is used the weights in the state dict can be strings
# check: https://github.com/huggingface/transformers/pull/24416 for more details
if isinstance(weight, str):
continue
else:
storage_id = id_tensor_storage(weight)
# If a `weight` shares the same underlying storage as another tensor, we put `weight` in the same `block`
if storage_id in storage_id_to_block:
block_id = storage_id_to_block[storage_id]
sharded_state_dicts[block_id][key] = weight
continue
weight_size = weight.numel() * dtype_byte_size(weight.dtype)
# If this weight is going to tip up over the maximal size, we split, but only if we have put at least one
# weight in the current shard.
if last_block_size + weight_size > max_shard_size and len(sharded_state_dicts[-1]) > 0:
sharded_state_dicts.append({})
last_block_size = 0
sharded_state_dicts[-1][key] = weight
last_block_size += weight_size
total_size += weight_size
storage_id_to_block[storage_id] = len(sharded_state_dicts) - 1
# If we only have one shard, we return it
if len(sharded_state_dicts) == 1:
return {weights_name: sharded_state_dicts[0]}, None
# Otherwise, let's build the index
weight_map = {}
shards = {}
for idx, shard in enumerate(sharded_state_dicts):
shard_file = weights_name.replace(".bin", f"-{idx+1:05d}-of-{len(sharded_state_dicts):05d}.bin")
shard_file = shard_file.replace(
".safetensors", f"-{idx + 1:05d}-of-{len(sharded_state_dicts):05d}.safetensors"
)
shards[shard_file] = shard
for key in shard.keys():
weight_map[key] = shard_file
# Add the metadata
metadata = {"total_size": total_size}
index = {"metadata": metadata, "weight_map": weight_map}
return shards, index
def load_sharded_checkpoint(model, folder, strict=True, prefer_safe=True):
"""
This is the same as
[`torch.nn.Module.load_state_dict`](https://pytorch.org/docs/stable/generated/torch.nn.Module.html?highlight=load_state_dict#torch.nn.Module.load_state_dict)
but for a sharded checkpoint.
This load is performed efficiently: each checkpoint shard is loaded one by one in RAM and deleted after being
loaded in the model.
Args:
model (`torch.nn.Module`): The model in which to load the checkpoint.
folder (`str` or `os.PathLike`): A path to a folder containing the sharded checkpoint.
strict (`bool`, *optional`, defaults to `True`):
Whether to strictly enforce that the keys in the model state dict match the keys in the sharded checkpoint.
prefer_safe (`bool`, *optional*, defaults to `False`)
If both safetensors and PyTorch save files are present in checkpoint and `prefer_safe` is True, the
safetensors files will be loaded. Otherwise, PyTorch files are always loaded when possible.
Returns:
`NamedTuple`: A named tuple with `missing_keys` and `unexpected_keys` fields
- `missing_keys` is a list of str containing the missing keys
- `unexpected_keys` is a list of str containing the unexpected keys
"""
# Load the index
index_file = os.path.join(folder, WEIGHTS_INDEX_NAME)
safe_index_file = os.path.join(folder, SAFE_WEIGHTS_INDEX_NAME)
index_present = os.path.isfile(index_file)
safe_index_present = os.path.isfile(safe_index_file)
if not index_present and not (safe_index_present and is_safetensors_available()):
filenames = (
(WEIGHTS_INDEX_NAME, SAFE_WEIGHTS_INDEX_NAME) if is_safetensors_available() else (WEIGHTS_INDEX_NAME,)
)
raise ValueError(f"Can't find a checkpoint index ({' or '.join(filenames)}) in {folder}.")
load_safe = False
if safe_index_present:
if prefer_safe:
if is_safetensors_available():
load_safe = True # load safe due to preference
else:
logger.warning(
f"Cannot load sharded checkpoint at {folder} safely since safetensors is not installed!"
)
elif not index_present:
load_safe = True # load safe since we have no other choice
load_index = safe_index_file if load_safe else index_file
with open(load_index, "r", encoding="utf-8") as f:
index = json.load(f)
shard_files = list(set(index["weight_map"].values()))
# If strict=True, error before loading any of the state dicts.
loaded_keys = index["weight_map"].keys()
model_keys = model.state_dict().keys()
missing_keys = [key for key in model_keys if key not in loaded_keys]
unexpected_keys = [key for key in loaded_keys if key not in model_keys]
if strict and (len(missing_keys) > 0 or len(unexpected_keys) > 0):
error_message = f"Error(s) in loading state_dict for {model.__class__.__name__}"
if len(missing_keys) > 0:
str_missing_keys = ",".join([f'"{k}"' for k in missing_keys])
error_message += f"\nMissing key(s): {str_missing_keys}."
if len(unexpected_keys) > 0:
str_unexpected_keys = ",".join([f'"{k}"' for k in unexpected_keys])
error_message += f"\nMissing key(s): {str_unexpected_keys}."
raise RuntimeError(error_message)
weights_only_kwarg = {"weights_only": True} if is_torch_greater_or_equal_than_1_13 else {}
loader = safe_load_file if load_safe else partial(torch.load, map_location="cpu", **weights_only_kwarg)
for shard_file in shard_files:
state_dict = loader(os.path.join(folder, shard_file))
model.load_state_dict(state_dict, strict=False)
# Make sure memory is freed before we load the next state dict.
del state_dict
gc.collect()
# Return the same thing as PyTorch load_state_dict function.
return torch.nn.modules.module._IncompatibleKeys(missing_keys, unexpected_keys)
def load_state_dict(checkpoint_file: Union[str, os.PathLike]):
"""
Reads a PyTorch checkpoint file, returning properly formatted errors if they arise.
"""
if checkpoint_file.endswith(".safetensors") and is_safetensors_available():
# Check format of the archive
with safe_open(checkpoint_file, framework="pt") as f:
metadata = f.metadata()
if metadata.get("format") not in ["pt", "tf", "flax"]:
raise OSError(
f"The safetensors archive passed at {checkpoint_file} does not contain the valid metadata. Make sure "
"you save your model with the `save_pretrained` method."
)
return safe_load_file(checkpoint_file)
try:
if (
is_deepspeed_zero3_enabled() and torch.distributed.is_initialized() and torch.distributed.get_rank() > 0
) or (is_fsdp_enabled() and not is_local_dist_rank_0()):
map_location = "meta"
else:
map_location = "cpu"
extra_args = {}
# mmap can only be used with files serialized with zipfile-based format.
if (
isinstance(checkpoint_file, str)
and map_location != "meta"
and version.parse(torch.__version__) >= version.parse("2.1.0")
and is_zipfile(checkpoint_file)
):
extra_args = {"mmap": True}
weights_only_kwarg = {"weights_only": True} if is_torch_greater_or_equal_than_1_13 else {}
return torch.load(
checkpoint_file,
map_location=map_location,
**weights_only_kwarg,
**extra_args,
)
except Exception as e:
try:
with open(checkpoint_file) as f:
if f.read(7) == "version":
raise OSError(
"You seem to have cloned a repository without having git-lfs installed. Please install "
"git-lfs and run `git lfs install` followed by `git lfs pull` in the folder "
"you cloned."
)
else:
raise ValueError(
f"Unable to locate the file {checkpoint_file} which is necessary to load this pretrained "
"model. Make sure you have saved the model properly."
) from e
except (UnicodeDecodeError, ValueError):
raise OSError(
f"Unable to load weights from pytorch checkpoint file for '{checkpoint_file}' "
f"at '{checkpoint_file}'. "
"If you tried to load a PyTorch model from a TF 2.0 checkpoint, please set from_tf=True."
)
def set_initialized_submodules(model, state_dict_keys):
"""
Sets the `_is_hf_initialized` flag in all submodules of a given model when all its weights are in the loaded state
dict.
"""
not_initialized_submodules = {}
for module_name, module in model.named_modules():
loaded_keys = {k.replace(f"{module_name}.", "") for k in state_dict_keys if k.startswith(f"{module_name}.")}
if loaded_keys.issuperset(module.state_dict()):
module._is_hf_initialized = True
else:
not_initialized_submodules[module_name] = module
return not_initialized_submodules
def _load_state_dict_into_model(model_to_load, state_dict, start_prefix):
# Convert old format to new format if needed from a PyTorch state_dict
old_keys = []
new_keys = []
for key in state_dict.keys():
new_key = None
if "gamma" in key:
new_key = key.replace("gamma", "weight")
if "beta" in key:
new_key = key.replace("beta", "bias")
if new_key:
old_keys.append(key)
new_keys.append(new_key)
for old_key, new_key in zip(old_keys, new_keys):
state_dict[new_key] = state_dict.pop(old_key)
# copy state_dict so _load_from_state_dict can modify it
metadata = getattr(state_dict, "_metadata", None)
state_dict = state_dict.copy()
if metadata is not None:
state_dict._metadata = metadata
error_msgs = []
# PyTorch's `_load_from_state_dict` does not copy parameters in a module's descendants
# so we need to apply the function recursively.
def load(module: nn.Module, state_dict, prefix=""):
local_metadata = {} if metadata is None else metadata.get(prefix[:-1], {})
args = (state_dict, prefix, local_metadata, True, [], [], error_msgs)
# Parameters of module and children will start with prefix. We can exit early if there are none in this
# state_dict
if len([key for key in state_dict if key.startswith(prefix)]) > 0:
if is_deepspeed_zero3_enabled():
import deepspeed
# In sharded models, each shard has only part of the full state_dict, so only gather
# parameters that are in the current state_dict.
named_parameters = dict(module.named_parameters(prefix=prefix[:-1], recurse=False))
params_to_gather = [named_parameters[k] for k in state_dict.keys() if k in named_parameters]
if len(params_to_gather) > 0:
# because zero3 puts placeholders in model params, this context
# manager gathers (unpartitions) the params of the current layer, then loads from
# the state dict and then re-partitions them again
with deepspeed.zero.GatheredParameters(params_to_gather, modifier_rank=0):
if torch.distributed.get_rank() == 0:
module._load_from_state_dict(*args)
else:
module._load_from_state_dict(*args)
for name, child in module._modules.items():
if child is not None:
load(child, state_dict, prefix + name + ".")
load(model_to_load, state_dict, prefix=start_prefix)
# Delete `state_dict` so it could be collected by GC earlier. Note that `state_dict` is a copy of the argument, so
# it's safe to delete it.
del state_dict
return error_msgs
def find_submodule_and_param_name(model, long_key, start_prefix):
"""
A helper util to find the last sub-module and the param/buffer name. If `start_prefix` is supplied it'll be removed
from the start of the key
"""
if len(start_prefix) > 0 and long_key.startswith(start_prefix):
long_key = ".".join(long_key.split(".")[1:])
split_key = long_key.split(".")
submodule = model
while len(split_key) > 1:
if hasattr(submodule, split_key[0]):
submodule = getattr(submodule, split_key[0])
del split_key[0]
else:
submodule = None
break
if submodule == model:
submodule = None
return submodule, split_key[0]
def _move_model_to_meta(model, loaded_state_dict_keys, start_prefix):
"""
Moves `loaded_state_dict_keys` in model to meta device which frees up the memory taken by those params.
`start_prefix` is used for models which insert their name into model keys, e.g. `bert` in
`bert.pooler.dense.weight`
"""
# dematerialize param storage for keys that are going to be replaced by state_dict, by
# putting those on the meta device
for k in loaded_state_dict_keys:
submodule, param_name = find_submodule_and_param_name(model, k, start_prefix)
if submodule is not None:
# selectively switch to the meta device only those params/buffers that will
# be next replaced from state_dict. This a complex way to do p.to_("meta")
# since we have no in-place to_ for tensors.
new_val = getattr(submodule, param_name)
if isinstance(new_val, torch.nn.Parameter):
# isinstance returns False for Params on meta device, so switch after the check
new_val = torch.nn.Parameter(new_val.to("meta"))
else:
new_val = new_val.to("meta")
setattr(submodule, param_name, new_val)
def _load_state_dict_into_meta_model(
model,
state_dict,
loaded_state_dict_keys, # left for now but could be removed, see below
start_prefix,
expected_keys,
device_map=None,
offload_folder=None,
offload_index=None,
state_dict_folder=None,
state_dict_index=None,
dtype=None,
hf_quantizer=None,
is_safetensors=False,
keep_in_fp32_modules=None,
unexpected_keys=None, # passing `unexpected` for cleanup from quantization items
):
"""
This is somewhat similar to `_load_state_dict_into_model`, but deals with a model that has some or all of its
params on a `meta` device. It replaces the model params with the data from the `state_dict`, while moving the
params back to the normal device, but only for `loaded_state_dict_keys`.
`start_prefix` is used for models which insert their name into model keys, e.g. `bert` in
`bert.pooler.dense.weight`
"""
# XXX: remaining features to implement to be fully compatible with _load_state_dict_into_model
# - deepspeed zero 3 support
# - need to copy metadata if any - see _load_state_dict_into_model
# - handling error_msgs - mimicking the error handling in module._load_from_state_dict()
# - Is there a situation where some keys aren't in `loaded_state_dict_keys` and in which case
# they won't get loaded.
error_msgs = []
old_keys = []
new_keys = []
for key in state_dict.keys():
new_key = None
if "gamma" in key:
new_key = key.replace("gamma", "weight")
if "beta" in key:
new_key = key.replace("beta", "bias")
if new_key:
old_keys.append(key)
new_keys.append(new_key)
for old_key, new_key in zip(old_keys, new_keys):
state_dict[new_key] = state_dict.pop(old_key)
for param_name, param in state_dict.items():
# First part of the test is always true as load_state_dict_keys always contains state_dict keys.
if param_name not in loaded_state_dict_keys or param_name not in expected_keys:
continue
if param_name.startswith(start_prefix):
param_name = param_name[len(start_prefix) :]
module_name = param_name
set_module_kwargs = {}
# We convert floating dtypes to the `dtype` passed. We want to keep the buffers/params
# in int/uint/bool and not cast them.
if dtype is not None and torch.is_floating_point(param):
if (
keep_in_fp32_modules is not None
and any(
module_to_keep_in_fp32 in param_name.split(".") for module_to_keep_in_fp32 in keep_in_fp32_modules
)
and dtype == torch.float16
):
param = param.to(torch.float32)
# For backward compatibility with older versions of `accelerate`
# TODO: @sgugger replace this check with version check at the next `accelerate` release
if "dtype" in list(inspect.signature(set_module_tensor_to_device).parameters):
set_module_kwargs["dtype"] = torch.float32
else:
param = param.to(dtype)
# For compatibility with PyTorch load_state_dict which converts state dict dtype to existing dtype in model, and which
# uses `param.copy_(input_param)` that preserves the contiguity of the parameter in the model.
# Reference: https://github.com/pytorch/pytorch/blob/db79ceb110f6646523019a59bbd7b838f43d4a86/torch/nn/modules/module.py#L2040C29-L2040C29
old_param = model
splits = param_name.split(".")
for split in splits:
old_param = getattr(old_param, split)
if old_param is None:
break
if old_param is not None:
if dtype is None:
param = param.to(old_param.dtype)
if old_param.is_contiguous():
param = param.contiguous()
set_module_kwargs["value"] = param
if device_map is None:
param_device = "cpu"
else:
# find next higher level module that is defined in device_map:
# bert.lm_head.weight -> bert.lm_head -> bert -> ''
while len(module_name) > 0 and module_name not in device_map:
module_name = ".".join(module_name.split(".")[:-1])
if module_name == "" and "" not in device_map:
# TODO: group all errors and raise at the end.
raise ValueError(f"{param_name} doesn't have any device set.")
param_device = device_map[module_name]
if param_device == "disk":
if not is_safetensors:
offload_index = offload_weight(param, param_name, offload_folder, offload_index)
elif param_device == "cpu" and state_dict_index is not None:
state_dict_index = offload_weight(param, param_name, model, state_dict_folder, state_dict_index)
elif (
hf_quantizer is None
or (not hf_quantizer.requires_parameters_quantization)
or (not hf_quantizer.check_quantized_param(model, param, param_name, state_dict))
):
# For backward compatibility with older versions of `accelerate` and for non-quantized params
set_module_tensor_to_device(model, param_name, param_device, **set_module_kwargs)
else:
hf_quantizer.create_quantized_param(model, param, param_name, param_device, state_dict, unexpected_keys)
# TODO: consider removing used param_parts from state_dict before return
return error_msgs, offload_index, state_dict_index
def _add_variant(weights_name: str, variant: Optional[str] = None) -> str:
if variant is not None:
splits = weights_name.split(".")
splits = splits[:-1] + [variant] + splits[-1:]
weights_name = ".".join(splits)
return weights_name
class ModuleUtilsMixin:
"""
A few utilities for `torch.nn.Modules`, to be used as a mixin.
"""
@staticmethod
def _hook_rss_memory_pre_forward(module, *args, **kwargs):
try:
import psutil
except ImportError:
raise ImportError("You need to install psutil (pip install psutil) to use memory tracing.")
process = psutil.Process(os.getpid())
mem = process.memory_info()
module.mem_rss_pre_forward = mem.rss
return None
@staticmethod
def _hook_rss_memory_post_forward(module, *args, **kwargs):
try:
import psutil
except ImportError:
raise ImportError("You need to install psutil (pip install psutil) to use memory tracing.")
process = psutil.Process(os.getpid())
mem = process.memory_info()
module.mem_rss_post_forward = mem.rss
mem_rss_diff = module.mem_rss_post_forward - module.mem_rss_pre_forward
module.mem_rss_diff = mem_rss_diff + (module.mem_rss_diff if hasattr(module, "mem_rss_diff") else 0)
return None
def add_memory_hooks(self):
"""
Add a memory hook before and after each sub-module forward pass to record increase in memory consumption.
Increase in memory consumption is stored in a `mem_rss_diff` attribute for each module and can be reset to zero
with `model.reset_memory_hooks_state()`.
"""
for module in self.modules():
module.register_forward_pre_hook(self._hook_rss_memory_pre_forward)
module.register_forward_hook(self._hook_rss_memory_post_forward)
self.reset_memory_hooks_state()
def reset_memory_hooks_state(self):
"""
Reset the `mem_rss_diff` attribute of each module (see [`~modeling_utils.ModuleUtilsMixin.add_memory_hooks`]).
"""
for module in self.modules():
module.mem_rss_diff = 0
module.mem_rss_post_forward = 0
module.mem_rss_pre_forward = 0
@property
def device(self) -> torch.device:
"""
`torch.device`: The device on which the module is (assuming that all the module parameters are on the same
device).
"""
return get_parameter_device(self)
@property
def dtype(self) -> torch.dtype:
"""
`torch.dtype`: The dtype of the module (assuming that all the module parameters have the same dtype).
"""
return get_parameter_dtype(self)
def invert_attention_mask(self, encoder_attention_mask: Tensor) -> Tensor:
"""
Invert an attention mask (e.g., switches 0. and 1.).
Args:
encoder_attention_mask (`torch.Tensor`): An attention mask.
Returns:
`torch.Tensor`: The inverted attention mask.
"""
if encoder_attention_mask.dim() == 3:
encoder_extended_attention_mask = encoder_attention_mask[:, None, :, :]
if encoder_attention_mask.dim() == 2:
encoder_extended_attention_mask = encoder_attention_mask[:, None, None, :]
# T5 has a mask that can compare sequence ids, we can simulate this here with this transposition
# Cf. https://github.com/tensorflow/mesh/blob/8d2465e9bc93129b913b5ccc6a59aa97abd96ec6/mesh_tensorflow
# /transformer/transformer_layers.py#L270
# encoder_extended_attention_mask = (encoder_extended_attention_mask ==
# encoder_extended_attention_mask.transpose(-1, -2))
encoder_extended_attention_mask = encoder_extended_attention_mask.to(dtype=self.dtype) # fp16 compatibility
encoder_extended_attention_mask = (1.0 - encoder_extended_attention_mask) * torch.finfo(self.dtype).min
return encoder_extended_attention_mask
@staticmethod
def create_extended_attention_mask_for_decoder(input_shape, attention_mask, device=None):
if device is not None:
warnings.warn(
"The `device` argument is deprecated and will be removed in v5 of Transformers.", FutureWarning
)
else:
device = attention_mask.device
batch_size, seq_length = input_shape
seq_ids = torch.arange(seq_length, device=device)
causal_mask = seq_ids[None, None, :].repeat(batch_size, seq_length, 1) <= seq_ids[None, :, None]
# in case past_key_values are used we need to add a prefix ones mask to the causal mask
# causal and attention masks must have same type with pytorch version < 1.3
causal_mask = causal_mask.to(attention_mask.dtype)
if causal_mask.shape[1] < attention_mask.shape[1]:
prefix_seq_len = attention_mask.shape[1] - causal_mask.shape[1]
causal_mask = torch.cat(
[
torch.ones((batch_size, seq_length, prefix_seq_len), device=device, dtype=causal_mask.dtype),
causal_mask,
],
axis=-1,
)
extended_attention_mask = causal_mask[:, None, :, :] * attention_mask[:, None, None, :]
return extended_attention_mask
def get_extended_attention_mask(
self, attention_mask: Tensor, input_shape: Tuple[int], device: torch.device = None, dtype: torch.float = None
) -> Tensor:
"""
Makes broadcastable attention and causal masks so that future and masked tokens are ignored.
Arguments:
attention_mask (`torch.Tensor`):
Mask with ones indicating tokens to attend to, zeros for tokens to ignore.
input_shape (`Tuple[int]`):
The shape of the input to the model.
Returns:
`torch.Tensor` The extended attention mask, with a the same dtype as `attention_mask.dtype`.
"""
if dtype is None:
dtype = self.dtype
if not (attention_mask.dim() == 2 and self.config.is_decoder):
# show warning only if it won't be shown in `create_extended_attention_mask_for_decoder`
if device is not None:
warnings.warn(
"The `device` argument is deprecated and will be removed in v5 of Transformers.", FutureWarning
)
# We can provide a self-attention mask of dimensions [batch_size, from_seq_length, to_seq_length]
# ourselves in which case we just need to make it broadcastable to all heads.
if attention_mask.dim() == 3:
extended_attention_mask = attention_mask[:, None, :, :]
elif attention_mask.dim() == 2:
# Provided a padding mask of dimensions [batch_size, seq_length]
# - if the model is a decoder, apply a causal mask in addition to the padding mask
# - if the model is an encoder, make the mask broadcastable to [batch_size, num_heads, seq_length, seq_length]
if self.config.is_decoder:
extended_attention_mask = ModuleUtilsMixin.create_extended_attention_mask_for_decoder(
input_shape, attention_mask, device
)
else:
extended_attention_mask = attention_mask[:, None, None, :]
else:
raise ValueError(
f"Wrong shape for input_ids (shape {input_shape}) or attention_mask (shape {attention_mask.shape})"
)
# Since attention_mask is 1.0 for positions we want to attend and 0.0 for
# masked positions, this operation will create a tensor which is 0.0 for
# positions we want to attend and the dtype's smallest value for masked positions.
# Since we are adding it to the raw scores before the softmax, this is
# effectively the same as removing these entirely.
extended_attention_mask = extended_attention_mask.to(dtype=dtype) # fp16 compatibility
extended_attention_mask = (1.0 - extended_attention_mask) * torch.finfo(dtype).min
return extended_attention_mask
def get_head_mask(
self, head_mask: Optional[Tensor], num_hidden_layers: int, is_attention_chunked: bool = False
) -> Tensor:
"""
Prepare the head mask if needed.
Args:
head_mask (`torch.Tensor` with shape `[num_heads]` or `[num_hidden_layers x num_heads]`, *optional*):
The mask indicating if we should keep the heads or not (1.0 for keep, 0.0 for discard).
num_hidden_layers (`int`):
The number of hidden layers in the model.
is_attention_chunked (`bool`, *optional*, defaults to `False`):
Whether or not the attentions scores are computed by chunks or not.
Returns:
`torch.Tensor` with shape `[num_hidden_layers x batch x num_heads x seq_length x seq_length]` or list with
`[None]` for each layer.
"""
if head_mask is not None:
head_mask = self._convert_head_mask_to_5d(head_mask, num_hidden_layers)
if is_attention_chunked is True:
head_mask = head_mask.unsqueeze(-1)
else:
head_mask = [None] * num_hidden_layers
return head_mask
def _convert_head_mask_to_5d(self, head_mask, num_hidden_layers):
"""-> [num_hidden_layers x batch x num_heads x seq_length x seq_length]"""
if head_mask.dim() == 1:
head_mask = head_mask.unsqueeze(0).unsqueeze(0).unsqueeze(-1).unsqueeze(-1)
head_mask = head_mask.expand(num_hidden_layers, -1, -1, -1, -1)
elif head_mask.dim() == 2:
head_mask = head_mask.unsqueeze(1).unsqueeze(-1).unsqueeze(-1) # We can specify head_mask for each layer
assert head_mask.dim() == 5, f"head_mask.dim != 5, instead {head_mask.dim()}"
head_mask = head_mask.to(dtype=self.dtype) # switch to float if need + fp16 compatibility
return head_mask
def num_parameters(self, only_trainable: bool = False, exclude_embeddings: bool = False) -> int:
"""
Get number of (optionally, trainable or non-embeddings) parameters in the module.
Args:
only_trainable (`bool`, *optional*, defaults to `False`):
Whether or not to return only the number of trainable parameters
exclude_embeddings (`bool`, *optional*, defaults to `False`):
Whether or not to return only the number of non-embeddings parameters
Returns:
`int`: The number of parameters.
"""
if exclude_embeddings:
embedding_param_names = [
f"{name}.weight" for name, module_type in self.named_modules() if isinstance(module_type, nn.Embedding)
]
total_parameters = [
parameter for name, parameter in self.named_parameters() if name not in embedding_param_names
]
else:
total_parameters = list(self.parameters())
total_numel = []
is_loaded_in_4bit = getattr(self, "is_loaded_in_4bit", False)
if is_loaded_in_4bit:
if is_bitsandbytes_available():
import bitsandbytes as bnb
else:
raise ValueError(
"bitsandbytes is not installed but it seems that the model has been loaded in 4bit precision, something went wrong"
" make sure to install bitsandbytes with `pip install bitsandbytes`. You also need a GPU. "
)
for param in total_parameters:
if param.requires_grad or not only_trainable:
# For 4bit models, we need to multiply the number of parameters by 2 as half of the parameters are
# used for the 4bit quantization (uint8 tensors are stored)
if is_loaded_in_4bit and isinstance(param, bnb.nn.Params4bit):
total_numel.append(param.numel() * 2)
else:
total_numel.append(param.numel())
return sum(total_numel)
def estimate_tokens(self, input_dict: Dict[str, Union[torch.Tensor, Any]]) -> int:
"""
Helper function to estimate the total number of tokens from the model inputs.
Args:
inputs (`dict`): The model inputs.
Returns:
`int`: The total number of tokens.
"""
if not hasattr(self, "warnings_issued"):
self.warnings_issued = {}
if self.main_input_name in input_dict:
return input_dict[self.main_input_name].numel()
elif "estimate_tokens" not in self.warnings_issued:
logger.warning(
"Could not estimate the number of tokens of the input, floating-point operations will not be computed"
)
self.warnings_issued["estimate_tokens"] = True
return 0
def floating_point_ops(
self, input_dict: Dict[str, Union[torch.Tensor, Any]], exclude_embeddings: bool = True
) -> int:
"""
Get number of (optionally, non-embeddings) floating-point operations for the forward and backward passes of a
batch with this transformer model. Default approximation neglects the quadratic dependency on the number of
tokens (valid if `12 * d_model << sequence_length`) as laid out in [this
paper](https://arxiv.org/pdf/2001.08361.pdf) section 2.1. Should be overridden for transformers with parameter
re-use e.g. Albert or Universal Transformers, or if doing long-range modeling with very high sequence lengths.
Args:
batch_size (`int`):
The batch size for the forward pass.
sequence_length (`int`):
The number of tokens in each line of the batch.
exclude_embeddings (`bool`, *optional*, defaults to `True`):
Whether or not to count embedding and softmax operations.
Returns:
`int`: The number of floating-point operations.
"""
return 6 * self.estimate_tokens(input_dict) * self.num_parameters(exclude_embeddings=exclude_embeddings)
class PreTrainedModel(nn.Module, ModuleUtilsMixin, GenerationMixin, PushToHubMixin, PeftAdapterMixin):
r"""
Base class for all models.
[`PreTrainedModel`] takes care of storing the configuration of the models and handles methods for loading,
downloading and saving models as well as a few methods common to all models to:
- resize the input embeddings,
- prune heads in the self-attention heads.
Class attributes (overridden by derived classes):
- **config_class** ([`PretrainedConfig`]) -- A subclass of [`PretrainedConfig`] to use as configuration class
for this model architecture.
- **load_tf_weights** (`Callable`) -- A python *method* for loading a TensorFlow checkpoint in a PyTorch model,
taking as arguments:
- **model** ([`PreTrainedModel`]) -- An instance of the model on which to load the TensorFlow checkpoint.
- **config** ([`PreTrainedConfig`]) -- An instance of the configuration associated to the model.
- **path** (`str`) -- A path to the TensorFlow checkpoint.
- **base_model_prefix** (`str`) -- A string indicating the attribute associated to the base model in derived
classes of the same architecture adding modules on top of the base model.
- **is_parallelizable** (`bool`) -- A flag indicating whether this model supports model parallelization.
- **main_input_name** (`str`) -- The name of the principal input to the model (often `input_ids` for NLP
models, `pixel_values` for vision models and `input_values` for speech models).
"""
config_class = None
base_model_prefix = ""
main_input_name = "input_ids"
model_tags = None
_auto_class = None
_no_split_modules = None
_skip_keys_device_placement = None
_keep_in_fp32_modules = None
# a list of `re` patterns of `state_dict` keys that should be removed from the list of missing
# keys we find (keys inside the model but not in the checkpoint) and avoid unnecessary warnings.
_keys_to_ignore_on_load_missing = None
# a list of `re` patterns of `state_dict` keys that should be removed from the list of
# unexpected keys we find (keys inside the checkpoint but not the model) and avoid unnecessary
# warnings.
_keys_to_ignore_on_load_unexpected = None
# a list of `state_dict` keys to ignore when saving the model (useful for keys that aren't
# trained, but which are either deterministic or tied variables)
_keys_to_ignore_on_save = None
# a list of `state_dict` keys that are potentially tied to another key in the state_dict.
_tied_weights_keys = None
is_parallelizable = False
supports_gradient_checkpointing = False
# Flash Attention 2 support
_supports_flash_attn_2 = False
# SDPA support
_supports_sdpa = False
# Has support for a `Cache` instance as `past_key_values`
_supports_cache_class = False
@property
def dummy_inputs(self) -> Dict[str, torch.Tensor]:
"""
`Dict[str, torch.Tensor]`: Dummy inputs to do a forward pass in the network.
"""
return {"input_ids": torch.tensor(DUMMY_INPUTS)}
@property
def framework(self) -> str:
"""
:str: Identifies that this is a PyTorch model.
"""
return "pt"
def __init__(self, config: PretrainedConfig, *inputs, **kwargs):
super().__init__()
if not isinstance(config, PretrainedConfig):
raise ValueError(
f"Parameter config in `{self.__class__.__name__}(config)` should be an instance of class "
"`PretrainedConfig`. To create a model from a pretrained model use "
f"`model = {self.__class__.__name__}.from_pretrained(PRETRAINED_MODEL_NAME)`"
)
# Save config and origin of the pretrained weights if given in model
config = self._autoset_attn_implementation(
config, torch_dtype=torch.get_default_dtype(), check_device_map=False
)
self.config = config
self.name_or_path = config.name_or_path
self.warnings_issued = {}
self.generation_config = GenerationConfig.from_model_config(config) if self.can_generate() else None
# Overwrite the class attribute to make it an instance attribute, so models like
# `InstructBlipForConditionalGeneration` can dynamically update it without modifying the class attribute
# when a different component (e.g. language_model) is used.
self._keep_in_fp32_modules = copy.copy(self.__class__._keep_in_fp32_modules)
def post_init(self):
"""
A method executed at the end of each Transformer model initialization, to execute code that needs the model's
modules properly initialized (such as weight initialization).
"""
self.init_weights()
self._backward_compatibility_gradient_checkpointing()
def _backward_compatibility_gradient_checkpointing(self):
if self.supports_gradient_checkpointing and getattr(self.config, "gradient_checkpointing", False):
self.gradient_checkpointing_enable()
# Remove the attribute now that is has been consumed, so it's no saved in the config.
delattr(self.config, "gradient_checkpointing")
def add_model_tags(self, tags: Union[List[str], str]) -> None:
r"""
Add custom tags into the model that gets pushed to the Hugging Face Hub. Will
not overwrite existing tags in the model.
Args:
tags (`Union[List[str], str]`):
The desired tags to inject in the model
Examples:
```python
from transformers import AutoModel
model = AutoModel.from_pretrained("bert-base-cased")
model.add_model_tags(["custom", "custom-bert"])
# Push the model to your namespace with the name "my-custom-bert".
model.push_to_hub("my-custom-bert")
```
"""
if isinstance(tags, str):
tags = [tags]
if self.model_tags is None:
self.model_tags = []
for tag in tags:
if tag not in self.model_tags:
self.model_tags.append(tag)
@classmethod
def _from_config(cls, config, **kwargs):
"""
All context managers that the model should be initialized under go here.
Args:
torch_dtype (`torch.dtype`, *optional*):
Override the default `torch.dtype` and load the model under this dtype.
"""
torch_dtype = kwargs.pop("torch_dtype", None)
use_flash_attention_2 = kwargs.pop("use_flash_attention_2", False)
# override default dtype if needed
dtype_orig = None
if torch_dtype is not None:
dtype_orig = cls._set_default_torch_dtype(torch_dtype)
config = copy.deepcopy(config) # We do not want to modify the config inplace in _from_config.
config._attn_implementation = kwargs.pop("attn_implementation", None)
config = cls._autoset_attn_implementation(
config,
use_flash_attention_2=use_flash_attention_2,
check_device_map=False,
torch_dtype=torch_dtype,
)
if is_deepspeed_zero3_enabled():
import deepspeed
logger.info("Detected DeepSpeed ZeRO-3: activating zero.init() for this model")
# this immediately partitions the model across all gpus, to avoid the overhead in time
# and memory copying it on CPU or each GPU first
with deepspeed.zero.Init(config_dict_or_path=deepspeed_config()):
model = cls(config, **kwargs)
else:
model = cls(config, **kwargs)
# restore default dtype if it was modified
if dtype_orig is not None:
torch.set_default_dtype(dtype_orig)
return model
@classmethod
def _autoset_attn_implementation(
cls,
config,
use_flash_attention_2: bool = False,
torch_dtype: Optional[torch.dtype] = None,
device_map: Optional[Union[str, Dict[str, int]]] = None,
check_device_map: bool = True,
):
"""
Automatically checks and dispatches to a default attention implementation. In order of priority:
1. An implementation specified in `config._attn_implementation` (due for example to the argument attn_implementation="sdpa" in from_pretrained).
2. DEPRECATED: if use_flash_attention_2 is set to `True` and `flash_attn` is available, flash attention. (`LlamaFlashAttention` for example)
3. SDPA implementation, if available and supported by the model type. (`LlamaSdpaAttention` for example)
4. The default model's implementation otherwise (`LlamaAttention` for example) .
"""
# Here we use config._attn_implementation_internal to check whether the attention implementation was explicitely set by the user.
# The property `PretrainedConfig._attn_implementation` is never `None`, for backward compatibility (always fall back on "eager").
# The `hasattr` here is used as some Transformers tests for some reason do not call PretrainedConfig __init__ (e.g. test_no_super_init_config_and_model)
requested_attn_implementation = None
if hasattr(config, "_attn_implementation_internal") and config._attn_implementation_internal is not None:
if config._attn_implementation != "flash_attention_2" and use_flash_attention_2:
raise ValueError(
f'Both attn_implementation="{config._attn_implementation}" and `use_flash_attention_2=True` were used when loading the model, which are not compatible.'
' We recommend to just use `attn_implementation="flash_attention_2"` when loading the model.'
)
if config._attn_implementation not in ["eager", "sdpa", "flash_attention_2"]:
message = f'Specified `attn_implementation="{config._attn_implementation}"` is not supported. The only possible arguments are `attn_implementation="eager"` (manual attention implementation)'
if cls._supports_flash_attn_2:
message += ', `"attn_implementation=flash_attention_2"` (implementation using flash attention 2)'
if cls._supports_sdpa:
message += ', `"attn_implementation=sdpa"` (implementation using torch.nn.functional.scaled_dot_product_attention)'
raise ValueError(message + ".")
# If a config is passed with a preset attn_implementation, we skip the automatic dispatch and use the user-provided config, with hard checks that the requested attention implementation is available.
requested_attn_implementation = config._attn_implementation_internal
if use_flash_attention_2:
logger.warning_once(
'The model was loaded with use_flash_attention_2=True, which is deprecated and may be removed in a future release. Please use `attn_implementation="flash_attention_2"` instead.'
)
config._attn_implementation = "flash_attention_2"
if config._attn_implementation == "flash_attention_2":
cls._check_and_enable_flash_attn_2(
config,
torch_dtype=torch_dtype,
device_map=device_map,
hard_check_only=False,
check_device_map=check_device_map,
)
elif requested_attn_implementation in [None, "sdpa"]:
# use_flash_attention_2 takes priority over SDPA, hence SDPA treated in this elif.
config = cls._check_and_enable_sdpa(
config,
hard_check_only=False if requested_attn_implementation is None else True,
)
else:
config._attn_implementation = "eager"
return config
@classmethod
def _set_default_torch_dtype(cls, dtype: torch.dtype) -> torch.dtype:
"""
Change the default dtype and return the previous one. This is needed when wanting to instantiate the model
under specific dtype.
Args:
dtype (`torch.dtype`):
a floating dtype to set to.
Returns:
`torch.dtype`: the original `dtype` that can be used to restore `torch.set_default_dtype(dtype)` if it was
modified. If it wasn't, returns `None`.
Note `set_default_dtype` currently only works with floating-point types and asserts if for example,
`torch.int64` is passed. So if a non-float `dtype` is passed this functions will throw an exception.
"""
if not dtype.is_floating_point:
raise ValueError(
f"Can't instantiate {cls.__name__} model under dtype={dtype} since it is not a floating point dtype"
)
logger.info(f"Instantiating {cls.__name__} model under default dtype {dtype}.")
dtype_orig = torch.get_default_dtype()
torch.set_default_dtype(dtype)
return dtype_orig
@property
def base_model(self) -> nn.Module:
"""
`torch.nn.Module`: The main body of the model.
"""
return getattr(self, self.base_model_prefix, self)
@classmethod
def can_generate(cls) -> bool:
"""
Returns whether this model can generate sequences with `.generate()`.
Returns:
`bool`: Whether this model can generate sequences with `.generate()`.
"""
# Detects whether `prepare_inputs_for_generation` has been overwritten, which is a requirement for generation.
# Alternativelly, the model can also have a custom `generate` function.
if "GenerationMixin" in str(cls.prepare_inputs_for_generation) and "GenerationMixin" in str(cls.generate):
return False
return True
@classmethod
def _check_and_enable_flash_attn_2(
cls,
config,
torch_dtype: Optional[torch.dtype] = None,
device_map: Optional[Union[str, Dict[str, int]]] = None,
check_device_map: bool = True,
hard_check_only: bool = False,
) -> PretrainedConfig:
"""
Checks the availability of Flash Attention 2 and compatibility with the current model.
If all checks pass and `hard_check_only` is False, the method will set the config attribute `attn_implementation` to "flash_attention_2" so that the model can initialize the correct attention module.
"""
if not cls._supports_flash_attn_2:
raise ValueError(
f"{cls.__name__} does not support Flash Attention 2.0 yet. Please request to add support where"
f" the model is hosted, on its model hub page: https://huggingface.co/{config._name_or_path}/discussions/new"
" or in the Transformers GitHub repo: https://github.com/huggingface/transformers/issues/new"
)
if not is_flash_attn_2_available():
preface = "FlashAttention2 has been toggled on, but it cannot be used due to the following error:"
install_message = "Please refer to the documentation of https://huggingface.co/docs/transformers/perf_infer_gpu_one#flashattention-2 to install Flash Attention 2."
if importlib.util.find_spec("flash_attn") is None:
raise ImportError(f"{preface} the package flash_attn seems to be not installed. {install_message}")
flash_attention_version = version.parse(importlib.metadata.version("flash_attn"))
if torch.version.cuda:
if flash_attention_version < version.parse("2.1.0"):
raise ImportError(
f"{preface} you need flash_attn package version to be greater or equal than 2.1.0. Detected version {flash_attention_version}. {install_message}"
)
else:
raise ImportError(f"{preface} Flash Attention 2 is not available. {install_message}")
elif torch.version.hip:
if flash_attention_version < version.parse("2.0.4"):
raise ImportError(
f"{preface} you need flash_attn package version to be greater or equal than 2.0.4. Make sure to have that version installed - detected version {flash_attention_version}. {install_message}"
)
else:
raise ImportError(f"{preface} Flash Attention 2 is not available. {install_message}")
_is_bettertransformer = getattr(cls, "use_bettertransformer", False)
if _is_bettertransformer:
raise ValueError(
"Flash Attention 2 and BetterTransformer API are not compatible. Please make sure to disable BetterTransformers by doing model.reverse_bettertransformer()"
)
if torch_dtype is None:
logger.warning_once(
"You are attempting to use Flash Attention 2.0 without specifying a torch dtype. This might lead to unexpected behaviour"
)
elif torch_dtype is not None and torch_dtype not in [torch.float16, torch.bfloat16]:
logger.warning_once(
"Flash Attention 2.0 only supports torch.float16 and torch.bfloat16 dtypes, but"
f" the current dype in {cls.__name__} is {torch_dtype}. You should run training or inference using Automatic Mixed-Precision via the `with torch.autocast(device_type='torch_device'):` decorator,"
' or load the model with the `torch_dtype` argument. Example: `model = AutoModel.from_pretrained("openai/whisper-tiny", attn_implementation="flash_attention_2", torch_dtype=torch.float16)`'
)
# The check `torch.empty(0).device.type != "cuda"` is needed as the model may be initialized after `torch.set_default_device` has been called,
# or the model may be initialized under the context manager `with torch.device("cuda"):`.
if check_device_map and device_map is None and torch.empty(0).device.type != "cuda":
if torch.cuda.is_available():
logger.warning_once(
"You are attempting to use Flash Attention 2.0 with a model not initialized on GPU. Make sure to move the model to GPU"
" after initializing it on CPU with `model.to('cuda')`."
)
else:
raise ValueError(
"You are attempting to use Flash Attention 2.0 with a model not initialized on GPU and with no GPU available. "
"This is not supported yet. Please make sure to have access to a GPU and either initialise the model on a GPU by passing a device_map "
"or initialising the model on CPU and then moving it to GPU."
)
elif (
check_device_map
and device_map is not None
and isinstance(device_map, dict)
and ("cpu" in device_map.values() or "disk" in device_map.values())
):
raise ValueError(
"You are attempting to use Flash Attention 2.0 with a model dispatched on CPU or disk. This is not supported. Please make sure to "
"initialise the model on a GPU by passing a device_map that contains only GPU devices as keys."
)
if not hard_check_only:
config._attn_implementation = "flash_attention_2"
return config
@classmethod
def _check_and_enable_sdpa(cls, config, hard_check_only: bool = False) -> PretrainedConfig:
"""
Checks the availability of SDPA for a given model.
If all checks pass and `hard_check_only` is False, the method will set the config attribute `_attn_implementation` to "flash_attention_2" so that the model can initialize the correct attention module.
"""
if hard_check_only:
if not cls._supports_sdpa:
raise ValueError(
f"{cls.__name__} does not support an attention implementation through torch.nn.functional.scaled_dot_product_attention yet."
" Please request the support for this architecture: https://github.com/huggingface/transformers/issues/28005. If you believe"
' this error is a bug, please open an issue in Transformers GitHub repository and load your model with the argument `attn_implementation="eager"` meanwhile. Example: `model = AutoModel.from_pretrained("openai/whisper-tiny", attn_implementation="eager")`'
)
if not is_torch_sdpa_available():
raise ImportError(
"PyTorch SDPA requirements in Transformers are not met. Please install torch>=2.1.1."
)
if not is_torch_sdpa_available() or not cls._supports_sdpa:
return config
_is_bettertransformer = getattr(cls, "use_bettertransformer", False)
if _is_bettertransformer:
return config
if not hard_check_only:
config._attn_implementation = "sdpa"
return config
def enable_input_require_grads(self):
"""
Enables the gradients for the input embeddings. This is useful for fine-tuning adapter weights while keeping
the model weights fixed.
"""
def make_inputs_require_grads(module, input, output):
output.requires_grad_(True)
self._require_grads_hook = self.get_input_embeddings().register_forward_hook(make_inputs_require_grads)
def disable_input_require_grads(self):
"""
Removes the `_require_grads_hook`.
"""
self._require_grads_hook.remove()
def get_input_embeddings(self) -> nn.Module:
"""
Returns the model's input embeddings.
Returns:
`nn.Module`: A torch module mapping vocabulary to hidden states.
"""
base_model = getattr(self, self.base_model_prefix, self)
if base_model is not self:
return base_model.get_input_embeddings()
else:
raise NotImplementedError
def set_input_embeddings(self, value: nn.Module):
"""
Set model's input embeddings.
Args:
value (`nn.Module`): A module mapping vocabulary to hidden states.
"""
base_model = getattr(self, self.base_model_prefix, self)
if base_model is not self:
base_model.set_input_embeddings(value)
else:
raise NotImplementedError
def get_output_embeddings(self) -> nn.Module:
"""
Returns the model's output embeddings.
Returns:
`nn.Module`: A torch module mapping hidden states to vocabulary.
"""
return None # Overwrite for models with output embeddings
def _init_weights(self, module):
"""
Initialize the weights. This method should be overridden by derived class and is
the only initialization method that will be called when loading a checkpoint
using `from_pretrained`. Any attempt to initialize outside of this function
will be useless as the torch.nn.init function are all replaced with skip.
"""
pass
def _initialize_weights(self, module):
"""
Initialize the weights if they are not already initialized.
"""
if getattr(module, "_is_hf_initialized", False):
return
self._init_weights(module)
module._is_hf_initialized = True
def tie_weights(self):
"""
Tie the weights between the input embeddings and the output embeddings.
If the `torchscript` flag is set in the configuration, can't handle parameter sharing so we are cloning the
weights instead.
"""
if getattr(self.config, "tie_word_embeddings", True):
output_embeddings = self.get_output_embeddings()
if output_embeddings is not None:
self._tie_or_clone_weights(output_embeddings, self.get_input_embeddings())
if getattr(self.config, "is_encoder_decoder", False) and getattr(self.config, "tie_encoder_decoder", False):
if hasattr(self, self.base_model_prefix):
self = getattr(self, self.base_model_prefix)
self._tie_encoder_decoder_weights(self.encoder, self.decoder, self.base_model_prefix)
for module in self.modules():
if hasattr(module, "_tie_weights"):
module._tie_weights()
@staticmethod
def _tie_encoder_decoder_weights(encoder: nn.Module, decoder: nn.Module, base_model_prefix: str):
uninitialized_encoder_weights: List[str] = []
if decoder.__class__ != encoder.__class__:
logger.info(
f"{decoder.__class__} and {encoder.__class__} are not equal. In this case make sure that all encoder"
" weights are correctly initialized."
)
def tie_encoder_to_decoder_recursively(
decoder_pointer: nn.Module,
encoder_pointer: nn.Module,
module_name: str,
uninitialized_encoder_weights: List[str],
depth=0,
):
assert isinstance(decoder_pointer, nn.Module) and isinstance(
encoder_pointer, nn.Module
), f"{decoder_pointer} and {encoder_pointer} have to be of type nn.Module"
if hasattr(decoder_pointer, "weight"):
assert hasattr(encoder_pointer, "weight")
encoder_pointer.weight = decoder_pointer.weight
if hasattr(decoder_pointer, "bias"):
assert hasattr(encoder_pointer, "bias")
encoder_pointer.bias = decoder_pointer.bias
return
encoder_modules = encoder_pointer._modules
decoder_modules = decoder_pointer._modules
if len(decoder_modules) > 0:
assert (
len(encoder_modules) > 0
), f"Encoder module {encoder_pointer} does not match decoder module {decoder_pointer}"
all_encoder_weights = {module_name + "/" + sub_name for sub_name in encoder_modules.keys()}
encoder_layer_pos = 0
for name, module in decoder_modules.items():
if name.isdigit():
encoder_name = str(int(name) + encoder_layer_pos)
decoder_name = name
if not isinstance(decoder_modules[decoder_name], type(encoder_modules[encoder_name])) and len(
encoder_modules
) != len(decoder_modules):
# this can happen if the name corresponds to the position in a list module list of layers
# in this case the decoder has added a cross-attention that the encoder does not have
# thus skip this step and subtract one layer pos from encoder
encoder_layer_pos -= 1
continue
elif name not in encoder_modules:
continue
elif depth > 500:
raise ValueError(
"Max depth of recursive function `tie_encoder_to_decoder` reached. It seems that there is"
" a circular dependency between two or more `nn.Modules` of your model."
)
else:
decoder_name = encoder_name = name
tie_encoder_to_decoder_recursively(
decoder_modules[decoder_name],
encoder_modules[encoder_name],
module_name + "/" + name,
uninitialized_encoder_weights,
depth=depth + 1,
)
all_encoder_weights.remove(module_name + "/" + encoder_name)
uninitialized_encoder_weights += list(all_encoder_weights)
# tie weights recursively
tie_encoder_to_decoder_recursively(decoder, encoder, base_model_prefix, uninitialized_encoder_weights)
if len(uninitialized_encoder_weights) > 0:
logger.warning(
f"The following encoder weights were not tied to the decoder {uninitialized_encoder_weights}"
)
def _tie_or_clone_weights(self, output_embeddings, input_embeddings):
"""Tie or clone module weights depending of whether we are using TorchScript or not"""
if self.config.torchscript:
output_embeddings.weight = nn.Parameter(input_embeddings.weight.clone())
else:
output_embeddings.weight = input_embeddings.weight
if getattr(output_embeddings, "bias", None) is not None:
output_embeddings.bias.data = nn.functional.pad(
output_embeddings.bias.data,
(
0,
output_embeddings.weight.shape[0] - output_embeddings.bias.shape[0],
),
"constant",
0,
)
if hasattr(output_embeddings, "out_features") and hasattr(input_embeddings, "num_embeddings"):
output_embeddings.out_features = input_embeddings.num_embeddings
def _get_no_split_modules(self, device_map: str):
"""
Get the modules of the model that should not be spit when using device_map. We iterate through the modules to
get the underlying `_no_split_modules`.
Args:
device_map (`str`):
The device map value. Options are ["auto", "balanced", "balanced_low_0", "sequential"]
Returns:
`List[str]`: List of modules that should not be split
"""
_no_split_modules = set()
modules_to_check = [self]
while len(modules_to_check) > 0:
module = modules_to_check.pop(-1)
# if the module does not appear in _no_split_modules, we also check the children
if module.__class__.__name__ not in _no_split_modules:
if isinstance(module, PreTrainedModel):
if module._no_split_modules is None:
raise ValueError(
f"{module.__class__.__name__} does not support `device_map='{device_map}'`. To implement support, the model "
"class needs to implement the `_no_split_modules` attribute."
)
else:
_no_split_modules = _no_split_modules | set(module._no_split_modules)
modules_to_check += list(module.children())
return list(_no_split_modules)
def resize_token_embeddings(
self, new_num_tokens: Optional[int] = None, pad_to_multiple_of: Optional[int] = None
) -> nn.Embedding:
"""
Resizes input token embeddings matrix of the model if `new_num_tokens != config.vocab_size`.
Takes care of tying weights embeddings afterwards if the model class has a `tie_weights()` method.
Arguments:
new_num_tokens (`int`, *optional*):
The new number of tokens in the embedding matrix. Increasing the size will add newly initialized
vectors at the end. Reducing the size will remove vectors from the end. If not provided or `None`, just
returns a pointer to the input tokens `torch.nn.Embedding` module of the model without doing anything.
pad_to_multiple_of (`int`, *optional*):
If set will pad the embedding matrix to a multiple of the provided value.If `new_num_tokens` is set to
`None` will just pad the embedding to a multiple of `pad_to_multiple_of`.
This is especially useful to enable the use of Tensor Cores on NVIDIA hardware with compute capability
`>= 7.5` (Volta), or on TPUs which benefit from having sequence lengths be a multiple of 128. For more
details about this, or help on choosing the correct value for resizing, refer to this guide:
https://docs.nvidia.com/deeplearning/performance/dl-performance-matrix-multiplication/index.html#requirements-tc
Return:
`torch.nn.Embedding`: Pointer to the input tokens Embeddings Module of the model.
"""
model_embeds = self._resize_token_embeddings(new_num_tokens, pad_to_multiple_of)
if new_num_tokens is None and pad_to_multiple_of is None:
return model_embeds
# Update base model and current model config
self.config.vocab_size = model_embeds.weight.shape[0]
self.vocab_size = model_embeds.weight.shape[0]
# Tie weights again if needed
self.tie_weights()
return model_embeds
def _resize_token_embeddings(self, new_num_tokens, pad_to_multiple_of=None):
old_embeddings = self.get_input_embeddings()
new_embeddings = self._get_resized_embeddings(old_embeddings, new_num_tokens, pad_to_multiple_of)
if hasattr(old_embeddings, "_hf_hook"):
hook = old_embeddings._hf_hook
add_hook_to_module(new_embeddings, hook)
old_embeddings_requires_grad = old_embeddings.weight.requires_grad
new_embeddings.requires_grad_(old_embeddings_requires_grad)
self.set_input_embeddings(new_embeddings)
# Update new_num_tokens with the actual size of new_embeddings
if pad_to_multiple_of is not None:
if is_deepspeed_zero3_enabled():
import deepspeed
with deepspeed.zero.GatheredParameters(new_embeddings.weight, modifier_rank=None):
new_num_tokens = new_embeddings.weight.shape[0]
else:
new_num_tokens = new_embeddings.weight.shape[0]
# if word embeddings are not tied, make sure that lm head is resized as well
if self.get_output_embeddings() is not None and not self.config.tie_word_embeddings:
old_lm_head = self.get_output_embeddings()
new_lm_head = self._get_resized_lm_head(old_lm_head, new_num_tokens)
if hasattr(old_lm_head, "_hf_hook"):
hook = old_lm_head._hf_hook
add_hook_to_module(new_lm_head, hook)
old_lm_head_requires_grad = old_lm_head.weight.requires_grad
new_lm_head.requires_grad_(old_lm_head_requires_grad)
self.set_output_embeddings(new_lm_head)
return self.get_input_embeddings()
def _get_resized_embeddings(
self,
old_embeddings: nn.Embedding,
new_num_tokens: Optional[int] = None,
pad_to_multiple_of: Optional[int] = None,
) -> nn.Embedding:
"""
Build a resized Embedding Module from a provided token Embedding Module. Increasing the size will add newly
initialized vectors at the end. Reducing the size will remove vectors from the end
Args:
old_embeddings (`torch.nn.Embedding`):
Old embeddings to be resized.
new_num_tokens (`int`, *optional*):
New number of tokens in the embedding matrix.
Increasing the size will add newly initialized vectors at the end. Reducing the size will remove
vectors from the end. If not provided or `None`, just returns a pointer to the input tokens
`torch.nn.Embedding` module of the model without doing anything.
pad_to_multiple_of (`int`, *optional*):
If set will pad the embedding matrix to a multiple of the provided value. If `new_num_tokens` is set to
`None` will just pad the embedding to a multiple of `pad_to_multiple_of`.
This is especially useful to enable the use of Tensor Cores on NVIDIA hardware with compute capability
`>= 7.5` (Volta), or on TPUs which benefit from having sequence lengths be a multiple of 128. For more
details about this, or help on choosing the correct value for resizing, refer to this guide:
https://docs.nvidia.com/deeplearning/performance/dl-performance-matrix-multiplication/index.html#requirements-tc
Return:
`torch.nn.Embedding`: Pointer to the resized Embedding Module or the old Embedding Module if
`new_num_tokens` is `None`
"""
if pad_to_multiple_of is not None:
if not isinstance(pad_to_multiple_of, int):
raise ValueError(
f"Asking to pad the embedding matrix to a multiple of `{pad_to_multiple_of}`, which is not and integer. Please make sure to pass an integer"
)
if new_num_tokens is None:
new_num_tokens = old_embeddings.weight.shape[0]
new_num_tokens = ((new_num_tokens + pad_to_multiple_of - 1) // pad_to_multiple_of) * pad_to_multiple_of
else:
logger.info(
"You are resizing the embedding layer without providing a `pad_to_multiple_of` parameter. This means that the new embedding"
f" dimension will be {new_num_tokens}. This might induce some performance reduction as *Tensor Cores* will not be available."
" For more details about this, or help on choosing the correct value for resizing, refer to this guide:"
" https://docs.nvidia.com/deeplearning/performance/dl-performance-matrix-multiplication/index.html#requirements-tc"
)
if new_num_tokens is None:
return old_embeddings
if is_deepspeed_zero3_enabled():
import deepspeed
with deepspeed.zero.GatheredParameters(old_embeddings.weight, modifier_rank=None):
old_num_tokens, old_embedding_dim = old_embeddings.weight.size()
else:
old_num_tokens, old_embedding_dim = old_embeddings.weight.size()
if old_num_tokens == new_num_tokens and not is_deepspeed_zero3_enabled():
return old_embeddings
if not isinstance(old_embeddings, nn.Embedding):
raise TypeError(
f"Old embeddings are of type {type(old_embeddings)}, which is not an instance of {nn.Embedding}. You"
" should either use a different resize function or make sure that `old_embeddings` are an instance of"
f" {nn.Embedding}."
)
# Build new embeddings
# When using DeepSpeed ZeRO-3, we shouldn't create new embeddings with DeepSpeed init
# because the shape of the new embedding layer is used across various modeling files
# as well as to update config vocab size. Shape will be 0 when using DeepSpeed init leading
# to errors when training.
new_embeddings = nn.Embedding(
new_num_tokens,
old_embedding_dim,
device=old_embeddings.weight.device,
dtype=old_embeddings.weight.dtype,
)
# initialize all new embeddings (in particular added tokens)
self._init_weights(new_embeddings)
# Copy token embeddings from the previous weights
# numbers of tokens to copy
n = min(old_num_tokens, new_num_tokens)
if is_deepspeed_zero3_enabled():
import deepspeed
params = [old_embeddings.weight, new_embeddings.weight]
with deepspeed.zero.GatheredParameters(params, modifier_rank=0):
new_embeddings.weight.data[:n, :] = old_embeddings.weight.data[:n, :]
else:
new_embeddings.weight.data[:n, :] = old_embeddings.weight.data[:n, :]
return new_embeddings
def _get_resized_lm_head(
self, old_lm_head: nn.Linear, new_num_tokens: Optional[int] = None, transposed: Optional[bool] = False
) -> nn.Linear:
"""
Build a resized Linear Module from a provided old Linear Module. Increasing the size will add newly initialized
vectors at the end. Reducing the size will remove vectors from the end
Args:
old_lm_head (`torch.nn.Linear`):
Old lm head liner layer to be resized.
new_num_tokens (`int`, *optional*):
New number of tokens in the linear matrix.
Increasing the size will add newly initialized vectors at the end. Reducing the size will remove
vectors from the end. If not provided or `None`, just returns a pointer to the input tokens
`torch.nn.Linear` module of the model without doing anything. transposed (`bool`, *optional*, defaults
to `False`): Whether `old_lm_head` is transposed or not. If True `old_lm_head.size()` is `lm_head_dim,
vocab_size` else `vocab_size, lm_head_dim`.
Return:
`torch.nn.Linear`: Pointer to the resized Linear Module or the old Linear Module if `new_num_tokens` is
`None`
"""
if new_num_tokens is None:
return old_lm_head
if is_deepspeed_zero3_enabled():
import deepspeed
with deepspeed.zero.GatheredParameters(old_lm_head.weight, modifier_rank=None):
old_num_tokens, old_lm_head_dim = (
old_lm_head.weight.size() if not transposed else old_lm_head.weight.t().size()
)
else:
old_num_tokens, old_lm_head_dim = (
old_lm_head.weight.size() if not transposed else old_lm_head.weight.t().size()
)
if old_num_tokens == new_num_tokens and not is_deepspeed_zero3_enabled():
return old_lm_head
if not isinstance(old_lm_head, nn.Linear):
raise TypeError(
f"Old language model head is of type {type(old_lm_head)}, which is not an instance of {nn.Linear}. You"
" should either use a different resize function or make sure that `old_lm_head` are an instance of"
f" {nn.Linear}."
)
# Build new lm head
new_lm_head_shape = (old_lm_head_dim, new_num_tokens) if not transposed else (new_num_tokens, old_lm_head_dim)
has_new_lm_head_bias = old_lm_head.bias is not None
# When using DeepSpeed ZeRO-3, we shouldn't create new embeddings with DeepSpeed init
# because the shape of the new embedding layer is used across various modeling files
# as well as to update config vocab size. Shape will be 0 when using DeepSpeed init leading
# to errors when training.
new_lm_head = nn.Linear(
*new_lm_head_shape,
bias=has_new_lm_head_bias,
device=old_lm_head.weight.device,
dtype=old_lm_head.weight.dtype,
)
# initialize new lm head (in particular added tokens)
self._init_weights(new_lm_head)
num_tokens_to_copy = min(old_num_tokens, new_num_tokens)
if is_deepspeed_zero3_enabled():
import deepspeed
params = [old_lm_head.weight, old_lm_head.bias, new_lm_head.weight, new_lm_head.bias]
with deepspeed.zero.GatheredParameters(params, modifier_rank=0):
self._copy_lm_head_original_to_resized(
new_lm_head, old_lm_head, num_tokens_to_copy, transposed, has_new_lm_head_bias
)
else:
self._copy_lm_head_original_to_resized(
new_lm_head, old_lm_head, num_tokens_to_copy, transposed, has_new_lm_head_bias
)
return new_lm_head
def _copy_lm_head_original_to_resized(
self, new_lm_head, old_lm_head, num_tokens_to_copy, transposed, has_new_lm_head_bias
):
# Copy old lm head weights to new lm head
if not transposed:
new_lm_head.weight.data[:num_tokens_to_copy, :] = old_lm_head.weight.data[:num_tokens_to_copy, :]
else:
new_lm_head.weight.data[:, :num_tokens_to_copy] = old_lm_head.weight.data[:, :num_tokens_to_copy]
# Copy bias weights to new lm head
if has_new_lm_head_bias:
new_lm_head.bias.data[:num_tokens_to_copy] = old_lm_head.bias.data[:num_tokens_to_copy]
def resize_position_embeddings(self, new_num_position_embeddings: int):
raise NotImplementedError(
f"`resize_position_embeddings` is not implemented for {self.__class__}`. To implement it, you should "
f"overwrite this method in the class {self.__class__} in `modeling_{self.__class__.__module__}.py`"
)
def get_position_embeddings(self) -> Union[nn.Embedding, Tuple[nn.Embedding]]:
raise NotImplementedError(
f"`get_position_embeddings` is not implemented for {self.__class__}`. To implement it, you should "
f"overwrite this method in the class {self.__class__} in `modeling_{self.__class__.__module__}.py`"
)
def init_weights(self):
"""
If needed prunes and maybe initializes weights. If using a custom `PreTrainedModel`, you need to implement any
initialization logic in `_init_weights`.
"""
# Prune heads if needed
if self.config.pruned_heads:
self.prune_heads(self.config.pruned_heads)
if _init_weights:
# Initialize weights
self.apply(self._initialize_weights)
# Tie weights should be skipped when not initializing all weights
# since from_pretrained(...) calls tie weights anyways
self.tie_weights()
def prune_heads(self, heads_to_prune: Dict[int, List[int]]):
"""
Prunes heads of the base model.
Arguments:
heads_to_prune (`Dict[int, List[int]]`):
Dictionary with keys being selected layer indices (`int`) and associated values being the list of heads
to prune in said layer (list of `int`). For instance {1: [0, 2], 2: [2, 3]} will prune heads 0 and 2 on
layer 1 and heads 2 and 3 on layer 2.
"""
# save new sets of pruned heads as union of previously stored pruned heads and newly pruned heads
for layer, heads in heads_to_prune.items():
union_heads = set(self.config.pruned_heads.get(layer, [])) | set(heads)
self.config.pruned_heads[layer] = list(union_heads) # Unfortunately we have to store it as list for JSON
self.base_model._prune_heads(heads_to_prune)
def gradient_checkpointing_enable(self, gradient_checkpointing_kwargs=None):
"""
Activates gradient checkpointing for the current model.
Note that in other frameworks this feature can be referred to as "activation checkpointing" or "checkpoint
activations".
We pass the `__call__` method of the modules instead of `forward` because `__call__` attaches all the hooks of
the module. https://discuss.pytorch.org/t/any-different-between-model-input-and-model-forward-input/3690/2
Args:
gradient_checkpointing_kwargs (dict, *optional*):
Additional keyword arguments passed along to the `torch.utils.checkpoint.checkpoint` function.
"""
if not self.supports_gradient_checkpointing:
raise ValueError(f"{self.__class__.__name__} does not support gradient checkpointing.")
if gradient_checkpointing_kwargs is None:
gradient_checkpointing_kwargs = {}
gradient_checkpointing_func = functools.partial(checkpoint, **gradient_checkpointing_kwargs)
# For old GC format (transformers < 4.35.0) for models that live on the Hub
# we will fall back to the overwritten `_set_gradient_checkpointing` method
_is_using_old_format = "value" in inspect.signature(self._set_gradient_checkpointing).parameters
if not _is_using_old_format:
self._set_gradient_checkpointing(enable=True, gradient_checkpointing_func=gradient_checkpointing_func)
else:
self.apply(partial(self._set_gradient_checkpointing, value=True))
logger.warn(
"You are using an old version of the checkpointing format that is deprecated (We will also silently ignore `gradient_checkpointing_kwargs` in case you passed it)."
"Please update to the new format on your modeling file. To use the new format, you need to completely remove the definition of the method `_set_gradient_checkpointing` in your model."
)
if getattr(self, "_hf_peft_config_loaded", False):
# When using PEFT + gradient checkpointing + Trainer we need to make sure the input has requires_grad=True
# we do it also on PEFT: https://github.com/huggingface/peft/blob/85013987aa82aa1af3da1236b6902556ce3e483e/src/peft/peft_model.py#L334
# When training with PEFT, only LoRA layers will have requires grad set to True, but the output of frozen layers need to propagate
# the gradients to make sure the gradient flows.
self.enable_input_require_grads()
def _set_gradient_checkpointing(self, enable: bool = True, gradient_checkpointing_func: Callable = checkpoint):
is_gradient_checkpointing_set = False
# Apply it on the top-level module in case the top-level modules supports it
# for example, LongT5Stack inherits from `PreTrainedModel`.
if hasattr(self, "gradient_checkpointing"):
self._gradient_checkpointing_func = gradient_checkpointing_func
self.gradient_checkpointing = enable
is_gradient_checkpointing_set = True
for module in self.modules():
if hasattr(module, "gradient_checkpointing"):
module._gradient_checkpointing_func = gradient_checkpointing_func
module.gradient_checkpointing = enable
is_gradient_checkpointing_set = True
if not is_gradient_checkpointing_set:
raise ValueError(
f"{self.__class__.__name__} is not compatible with gradient checkpointing. Make sure all the architecture support it by setting a boolean attribute"
" `gradient_checkpointing` to modules of the model that uses checkpointing."
)
def gradient_checkpointing_disable(self):
"""
Deactivates gradient checkpointing for the current model.
Note that in other frameworks this feature can be referred to as "activation checkpointing" or "checkpoint
activations".
"""
if self.supports_gradient_checkpointing:
# For old GC format (transformers < 4.35.0) for models that live on the Hub
# we will fall back to the overwritten `_set_gradient_checkpointing` methid
_is_using_old_format = "value" in inspect.signature(self._set_gradient_checkpointing).parameters
if not _is_using_old_format:
self._set_gradient_checkpointing(enable=False)
else:
logger.warn(
"You are using an old version of the checkpointing format that is deprecated (We will also silently ignore `gradient_checkpointing_kwargs` in case you passed it)."
"Please update to the new format on your modeling file. To use the new format, you need to completely remove the definition of the method `_set_gradient_checkpointing` in your model."
)
self.apply(partial(self._set_gradient_checkpointing, value=False))
if getattr(self, "_hf_peft_config_loaded", False):
self.disable_input_require_grads()
@property
def is_gradient_checkpointing(self) -> bool:
"""
Whether gradient checkpointing is activated for this model or not.
Note that in other frameworks this feature can be referred to as "activation checkpointing" or "checkpoint
activations".
"""
return any(hasattr(m, "gradient_checkpointing") and m.gradient_checkpointing for m in self.modules())
def save_pretrained(
self,
save_directory: Union[str, os.PathLike],
is_main_process: bool = True,
state_dict: Optional[dict] = None,
save_function: Callable = torch.save,
push_to_hub: bool = False,
max_shard_size: Union[int, str] = "5GB",
safe_serialization: bool = True,
variant: Optional[str] = None,
token: Optional[Union[str, bool]] = None,
save_peft_format: bool = True,
**kwargs,
):
"""
Save a model and its configuration file to a directory, so that it can be re-loaded using the
[`~PreTrainedModel.from_pretrained`] class method.
Arguments:
save_directory (`str` or `os.PathLike`):
Directory to which to save. Will be created if it doesn't exist.
is_main_process (`bool`, *optional*, defaults to `True`):
Whether the process calling this is the main process or not. Useful when in distributed training like
TPUs and need to call this function on all processes. In this case, set `is_main_process=True` only on
the main process to avoid race conditions.
state_dict (nested dictionary of `torch.Tensor`):
The state dictionary of the model to save. Will default to `self.state_dict()`, but can be used to only
save parts of the model or if special precautions need to be taken when recovering the state dictionary
of a model (like when using model parallelism).
save_function (`Callable`):
The function to use to save the state dictionary. Useful on distributed training like TPUs when one
need to replace `torch.save` by another method.
push_to_hub (`bool`, *optional*, defaults to `False`):
Whether or not to push your model to the Hugging Face model hub after saving it. You can specify the
repository you want to push to with `repo_id` (will default to the name of `save_directory` in your
namespace).
max_shard_size (`int` or `str`, *optional*, defaults to `"5GB"`):
The maximum size for a checkpoint before being sharded. Checkpoints shard will then be each of size
lower than this size. If expressed as a string, needs to be digits followed by a unit (like `"5MB"`).
We default it to 5GB in order for models to be able to run easily on free-tier google colab instances
without CPU OOM issues.
<Tip warning={true}>
If a single weight of the model is bigger than `max_shard_size`, it will be in its own checkpoint shard
which will be bigger than `max_shard_size`.
</Tip>
safe_serialization (`bool`, *optional*, defaults to `True`):
Whether to save the model using `safetensors` or the traditional PyTorch way (that uses `pickle`).
variant (`str`, *optional*):
If specified, weights are saved in the format pytorch_model.<variant>.bin.
token (`str` or `bool`, *optional*):
The token to use as HTTP bearer authorization for remote files. If `True`, or not specified, will use
the token generated when running `huggingface-cli login` (stored in `~/.huggingface`).
save_peft_format (`bool`, *optional*, defaults to `True`):
For backward compatibility with PEFT library, in case adapter weights are attached to the model, all
keys of the state dict of adapters needs to be pre-pended with `base_model.model`. Advanced users can
disable this behaviours by setting `save_peft_format` to `False`.
kwargs (`Dict[str, Any]`, *optional*):
Additional key word arguments passed along to the [`~utils.PushToHubMixin.push_to_hub`] method.
"""
use_auth_token = kwargs.pop("use_auth_token", None)
ignore_metadata_errors = kwargs.pop("ignore_metadata_errors", False)
if use_auth_token is not None:
warnings.warn(
"The `use_auth_token` argument is deprecated and will be removed in v5 of Transformers. Please use `token` instead.",
FutureWarning,
)
if token is not None:
raise ValueError(
"`token` and `use_auth_token` are both specified. Please set only the argument `token`."
)
token = use_auth_token
if token is not None:
kwargs["token"] = token
_hf_peft_config_loaded = getattr(self, "_hf_peft_config_loaded", False)
hf_quantizer = getattr(self, "hf_quantizer", None)
quantization_serializable = (
hf_quantizer is not None and isinstance(hf_quantizer, HfQuantizer) and hf_quantizer.is_serializable
)
if hf_quantizer is not None and not _hf_peft_config_loaded and not quantization_serializable:
raise ValueError(
f"The model is quantized with {hf_quantizer.quantization_config.quant_method} and is not serializable - check out the warnings from"
" the logger on the traceback to understand the reason why the quantized model is not serializable."
)
if "save_config" in kwargs:
warnings.warn(
"`save_config` is deprecated and will be removed in v5 of Transformers. Use `is_main_process` instead."
)
is_main_process = kwargs.pop("save_config")
if safe_serialization and not is_safetensors_available():
raise ImportError("`safe_serialization` requires the `safetensors library: `pip install safetensors`.")
if os.path.isfile(save_directory):
logger.error(f"Provided path ({save_directory}) should be a directory, not a file")
return
os.makedirs(save_directory, exist_ok=True)
if push_to_hub:
commit_message = kwargs.pop("commit_message", None)
repo_id = kwargs.pop("repo_id", save_directory.split(os.path.sep)[-1])
repo_id = self._create_repo(repo_id, **kwargs)
files_timestamps = self._get_files_timestamps(save_directory)
# Only save the model itself if we are using distributed training
model_to_save = unwrap_model(self)
# save the string version of dtype to the config, e.g. convert torch.float32 => "float32"
# we currently don't use this setting automatically, but may start to use with v5
dtype = get_parameter_dtype(model_to_save)
model_to_save.config.torch_dtype = str(dtype).split(".")[1]
# Attach architecture to the config
model_to_save.config.architectures = [model_to_save.__class__.__name__]
# If we have a custom model, we copy the file defining it in the folder and set the attributes so it can be
# loaded from the Hub.
if self._auto_class is not None:
custom_object_save(self, save_directory, config=self.config)
# Save the config
if is_main_process:
if not _hf_peft_config_loaded:
model_to_save.config.save_pretrained(save_directory)
if self.can_generate():
# generation config built from the model config + the model config holds generation kwargs -> generate
# may revert to legacy behavior if the two don't match
if (
model_to_save.generation_config._from_model_config
and model_to_save.config._has_non_default_generation_parameters()
):
new_generation_config = GenerationConfig.from_model_config(model_to_save.config)
if new_generation_config != model_to_save.generation_config:
logger.warning(
"Your generation config was originally created from the model config, but the model "
"config has changed since then. Unless you pass the `generation_config` argument to this "
"model's `generate` calls, they will revert to the legacy behavior where the base "
"`generate` parameterization is loaded from the model config instead. "
"To avoid this behavior and this warning, we recommend you to overwrite the generation "
"config model attribute before calling the model's `save_pretrained`, preferably also "
"removing any generation kwargs from the model config. This warning will be raised to an "
"exception in v4.41."
)
model_to_save.generation_config.save_pretrained(save_directory)
if _hf_peft_config_loaded:
logger.info(
"Detected adapters on the model, saving the model in the PEFT format, only adapter weights will be saved."
)
state_dict = model_to_save.get_adapter_state_dict()
if save_peft_format:
logger.info(
"To match the expected format of the PEFT library, all keys of the state dict of adapters will be pre-pended with `base_model.model`."
)
peft_state_dict = {}
for key, value in state_dict.items():
peft_state_dict[f"base_model.model.{key}"] = value
state_dict = peft_state_dict
active_adapter = self.active_adapters()
if len(active_adapter) > 1:
raise ValueError(
"Multiple active adapters detected, saving multiple active adapters is not supported yet. You can save adapters separately one by one "
"by iteratively calling `model.set_adapter(adapter_name)` then `model.save_pretrained(...)`"
)
active_adapter = active_adapter[0]
current_peft_config = self.peft_config[active_adapter]
current_peft_config.save_pretrained(save_directory)
# Save the model
if state_dict is None:
state_dict = model_to_save.state_dict()
# Translate state_dict from smp to hf if saving with smp >= 1.10
if IS_SAGEMAKER_MP_POST_1_10:
for smp_to_hf, _ in smp.state.module_manager.translate_functions:
state_dict = smp_to_hf(state_dict)
# Handle the case where some state_dict keys shouldn't be saved
if self._keys_to_ignore_on_save is not None:
for ignore_key in self._keys_to_ignore_on_save:
if ignore_key in state_dict.keys():
del state_dict[ignore_key]
if safe_serialization:
# Safetensors does not allow tensor aliasing.
# We're going to remove aliases before saving
ptrs = collections.defaultdict(list)
for name, tensor in state_dict.items():
# Sometimes in the state_dict we have non-tensor objects.
# e.g. in bitsandbytes we have some `str` objects in the state_dict
if isinstance(tensor, torch.Tensor):
ptrs[id_tensor_storage(tensor)].append(name)
else:
# In the non-tensor case, fall back to the pointer of the object itself
ptrs[id(tensor)].append(name)
# These are all the pointers of shared tensors.
shared_ptrs = {ptr: names for ptr, names in ptrs.items() if len(names) > 1}
warn_names = set()
for names in shared_ptrs.values():
# Removing the keys which are declared as known duplicates on
# load. This allows to make sure the name which is kept is consistent.
if self._tied_weights_keys is not None:
found = 0
for name in sorted(names):
matches_pattern = any(re.search(pat, name) for pat in self._tied_weights_keys)
if matches_pattern and name in state_dict:
found += 1
if found < len(names):
del state_dict[name]
# When not all duplicates have been cleaned, still remove those keys, but put a clear warning.
# If the link between tensors was done at runtime then `from_pretrained` will not get
# the key back leading to random tensor. A proper warning will be shown
# during reload (if applicable), but since the file is not necessarily compatible with
# the config, better show a proper warning.
found = 0
for name in names:
if name in state_dict:
found += 1
if found > 1:
del state_dict[name]
warn_names.add(name)
if len(warn_names) > 0:
logger.warning_once(
f"Removed shared tensor {warn_names} while saving. This should be OK, but check by verifying that you don't receive any warning while reloading",
)
# Shard the model if it is too big.
if not _hf_peft_config_loaded:
weights_name = SAFE_WEIGHTS_NAME if safe_serialization else WEIGHTS_NAME
weights_name = _add_variant(weights_name, variant)
else:
weights_name = ADAPTER_SAFE_WEIGHTS_NAME if safe_serialization else ADAPTER_WEIGHTS_NAME
shards, index = shard_checkpoint(state_dict, max_shard_size=max_shard_size, weights_name=weights_name)
# Clean the folder from a previous save
for filename in os.listdir(save_directory):
full_filename = os.path.join(save_directory, filename)
# If we have a shard file that is not going to be replaced, we delete it, but only from the main process
# in distributed settings to avoid race conditions.
weights_no_suffix = weights_name.replace(".bin", "").replace(".safetensors", "")
# make sure that file to be deleted matches format of sharded file, e.g. pytorch_model-00001-of-00005
filename_no_suffix = filename.replace(".bin", "").replace(".safetensors", "")
reg = re.compile(r"(.*?)-\d{5}-of-\d{5}")
if (
filename.startswith(weights_no_suffix)
and os.path.isfile(full_filename)
and filename not in shards.keys()
and is_main_process
and reg.fullmatch(filename_no_suffix) is not None
):
os.remove(full_filename)
# Save the model
for shard_file, shard in shards.items():
if safe_serialization:
# At some point we will need to deal better with save_function (used for TPU and other distributed
# joyfulness), but for now this enough.
safe_save_file(shard, os.path.join(save_directory, shard_file), metadata={"format": "pt"})
else:
save_function(shard, os.path.join(save_directory, shard_file))
if index is None:
path_to_weights = os.path.join(save_directory, weights_name)
logger.info(f"Model weights saved in {path_to_weights}")
else:
save_index_file = SAFE_WEIGHTS_INDEX_NAME if safe_serialization else WEIGHTS_INDEX_NAME
save_index_file = os.path.join(save_directory, _add_variant(save_index_file, variant))
# Save the index as well
with open(save_index_file, "w", encoding="utf-8") as f:
content = json.dumps(index, indent=2, sort_keys=True) + "\n"
f.write(content)
logger.info(
f"The model is bigger than the maximum size per checkpoint ({max_shard_size}) and is going to be "
f"split in {len(shards)} checkpoint shards. You can find where each parameters has been saved in the "
f"index located at {save_index_file}."
)
if push_to_hub:
# Eventually create an empty model card
model_card = create_and_tag_model_card(
repo_id, self.model_tags, token=token, ignore_metadata_errors=ignore_metadata_errors
)
# Update model card if needed:
model_card.save(os.path.join(save_directory, "README.md"))
self._upload_modified_files(
save_directory,
repo_id,
files_timestamps,
commit_message=commit_message,
token=token,
)
@wraps(PushToHubMixin.push_to_hub)
def push_to_hub(self, *args, **kwargs):
tags = self.model_tags if self.model_tags is not None else []
tags_kwargs = kwargs.get("tags", [])
if isinstance(tags_kwargs, str):
tags_kwargs = [tags_kwargs]
for tag in tags_kwargs:
if tag not in tags:
tags.append(tag)
if tags:
kwargs["tags"] = tags
return super().push_to_hub(*args, **kwargs)
def get_memory_footprint(self, return_buffers=True):
r"""
Get the memory footprint of a model. This will return the memory footprint of the current model in bytes.
Useful to benchmark the memory footprint of the current model and design some tests. Solution inspired from the
PyTorch discussions: https://discuss.pytorch.org/t/gpu-memory-that-model-uses/56822/2
Arguments:
return_buffers (`bool`, *optional*, defaults to `True`):
Whether to return the size of the buffer tensors in the computation of the memory footprint. Buffers
are tensors that do not require gradients and not registered as parameters. E.g. mean and std in batch
norm layers. Please see: https://discuss.pytorch.org/t/what-pytorch-means-by-buffers/120266/2
"""
mem = sum([param.nelement() * param.element_size() for param in self.parameters()])
if return_buffers:
mem_bufs = sum([buf.nelement() * buf.element_size() for buf in self.buffers()])
mem = mem + mem_bufs
return mem
@wraps(torch.nn.Module.cuda)
def cuda(self, *args, **kwargs):
# Checks if the model has been loaded in 8-bit
if getattr(self, "quantization_method", None) == QuantizationMethod.BITS_AND_BYTES:
raise ValueError(
"Calling `cuda()` is not supported for `4-bit` or `8-bit` quantized models. Please use the model as it is, since the"
" model has already been set to the correct devices and casted to the correct `dtype`."
)
else:
return super().cuda(*args, **kwargs)
@wraps(torch.nn.Module.to)
def to(self, *args, **kwargs):
# Checks if the model has been loaded in 8-bit
if getattr(self, "quantization_method", None) == QuantizationMethod.BITS_AND_BYTES:
raise ValueError(
"`.to` is not supported for `4-bit` or `8-bit` bitsandbytes models. Please use the model as it is, since the"
" model has already been set to the correct devices and casted to the correct `dtype`."
)
elif getattr(self, "quantization_method", None) == QuantizationMethod.GPTQ:
# For GPTQ models, we prevent users from casting the model to another dytpe to restrict unwanted behaviours.
# the correct API should be to load the model with the desired dtype directly through `from_pretrained`.
dtype_present_in_args = False
if "dtype" not in kwargs:
for arg in args:
if isinstance(arg, torch.dtype):
dtype_present_in_args = True
break
else:
dtype_present_in_args = True
if dtype_present_in_args:
raise ValueError(
"You cannot cast a GPTQ model in a new `dtype`. Make sure to load the model using `from_pretrained` using the desired"
" `dtype` by passing the correct `torch_dtype` argument."
)
return super().to(*args, **kwargs)
def half(self, *args):
# Checks if the model is quantized
if getattr(self, "is_quantized", False):
raise ValueError(
"`.half()` is not supported for quantized model. Please use the model as it is, since the"
" model has already been casted to the correct `dtype`."
)
else:
return super().half(*args)
def float(self, *args):
# Checks if the model is quantized
if getattr(self, "is_quantized", False):
raise ValueError(
"`.float()` is not supported for quantized model. Please use the model as it is, since the"
" model has already been casted to the correct `dtype`."
)
else:
return super().float(*args)
@classmethod
def from_pretrained(
cls,
pretrained_model_name_or_path: Optional[Union[str, os.PathLike]],
*model_args,
config: Optional[Union[PretrainedConfig, str, os.PathLike]] = None,
cache_dir: Optional[Union[str, os.PathLike]] = None,
ignore_mismatched_sizes: bool = False,
force_download: bool = False,
local_files_only: bool = False,
token: Optional[Union[str, bool]] = None,
revision: str = "main",
use_safetensors: bool = None,
**kwargs,
):
r"""
Instantiate a pretrained pytorch model from a pre-trained model configuration.
The model is set in evaluation mode by default using `model.eval()` (Dropout modules are deactivated). To train
the model, you should first set it back in training mode with `model.train()`.
The warning *Weights from XXX not initialized from pretrained model* means that the weights of XXX do not come
pretrained with the rest of the model. It is up to you to train those weights with a downstream fine-tuning
task.
The warning *Weights from XXX not used in YYY* means that the layer XXX is not used by YYY, therefore those
weights are discarded.
Parameters:
pretrained_model_name_or_path (`str` or `os.PathLike`, *optional*):
Can be either:
- A string, the *model id* of a pretrained model hosted inside a model repo on huggingface.co.
Valid model ids can be located at the root-level, like `bert-base-uncased`, or namespaced under a
user or organization name, like `dbmdz/bert-base-german-cased`.
- A path to a *directory* containing model weights saved using
[`~PreTrainedModel.save_pretrained`], e.g., `./my_model_directory/`.
- A path or url to a *tensorflow index checkpoint file* (e.g, `./tf_model/model.ckpt.index`). In
this case, `from_tf` should be set to `True` and a configuration object should be provided as
`config` argument. This loading path is slower than converting the TensorFlow checkpoint in a
PyTorch model using the provided conversion scripts and loading the PyTorch model afterwards.
- A path or url to a model folder containing a *flax checkpoint file* in *.msgpack* format (e.g,
`./flax_model/` containing `flax_model.msgpack`). In this case, `from_flax` should be set to
`True`.
- `None` if you are both providing the configuration and state dictionary (resp. with keyword
arguments `config` and `state_dict`).
model_args (sequence of positional arguments, *optional*):
All remaining positional arguments will be passed to the underlying model's `__init__` method.
config (`Union[PretrainedConfig, str, os.PathLike]`, *optional*):
Can be either:
- an instance of a class derived from [`PretrainedConfig`],
- a string or path valid as input to [`~PretrainedConfig.from_pretrained`].
Configuration for the model to use instead of an automatically loaded configuration. Configuration can
be automatically loaded when:
- The model is a model provided by the library (loaded with the *model id* string of a pretrained
model).
- The model was saved using [`~PreTrainedModel.save_pretrained`] and is reloaded by supplying the
save directory.
- The model is loaded by supplying a local directory as `pretrained_model_name_or_path` and a
configuration JSON file named *config.json* is found in the directory.
state_dict (`Dict[str, torch.Tensor]`, *optional*):
A state dictionary to use instead of a state dictionary loaded from saved weights file.
This option can be used if you want to create a model from a pretrained configuration but load your own
weights. In this case though, you should check if using [`~PreTrainedModel.save_pretrained`] and
[`~PreTrainedModel.from_pretrained`] is not a simpler option.
cache_dir (`Union[str, os.PathLike]`, *optional*):
Path to a directory in which a downloaded pretrained model configuration should be cached if the
standard cache should not be used.
from_tf (`bool`, *optional*, defaults to `False`):
Load the model weights from a TensorFlow checkpoint save file (see docstring of
`pretrained_model_name_or_path` argument).
from_flax (`bool`, *optional*, defaults to `False`):
Load the model weights from a Flax checkpoint save file (see docstring of
`pretrained_model_name_or_path` argument).
ignore_mismatched_sizes (`bool`, *optional*, defaults to `False`):
Whether or not to raise an error if some of the weights from the checkpoint do not have the same size
as the weights of the model (if for instance, you are instantiating a model with 10 labels from a
checkpoint with 3 labels).
force_download (`bool`, *optional*, defaults to `False`):
Whether or not to force the (re-)download of the model weights and configuration files, overriding the
cached versions if they exist.
resume_download (`bool`, *optional*, defaults to `False`):
Whether or not to delete incompletely received files. Will attempt to resume the download if such a
file exists.
proxies (`Dict[str, str]`, *optional*):
A dictionary of proxy servers to use by protocol or endpoint, e.g., `{'http': 'foo.bar:3128',
'http://hostname': 'foo.bar:4012'}`. The proxies are used on each request.
output_loading_info(`bool`, *optional*, defaults to `False`):
Whether ot not to also return a dictionary containing missing keys, unexpected keys and error messages.
local_files_only(`bool`, *optional*, defaults to `False`):
Whether or not to only look at local files (i.e., do not try to download the model).
token (`str` or `bool`, *optional*):
The token to use as HTTP bearer authorization for remote files. If `True`, or not specified, will use
the token generated when running `huggingface-cli login` (stored in `~/.huggingface`).
revision (`str`, *optional*, defaults to `"main"`):
The specific model version to use. It can be a branch name, a tag name, or a commit id, since we use a
git-based system for storing models and other artifacts on huggingface.co, so `revision` can be any
identifier allowed by git.
<Tip>
To test a pull request you made on the Hub, you can pass `revision="refs/pr/<pr_number>".
</Tip>
mirror (`str`, *optional*):
Mirror source to accelerate downloads in China. If you are from China and have an accessibility
problem, you can set this option to resolve it. Note that we do not guarantee the timeliness or safety.
Please refer to the mirror site for more information.
_fast_init(`bool`, *optional*, defaults to `True`):
Whether or not to disable fast initialization.
<Tip warning={true}>
One should only disable *_fast_init* to ensure backwards compatibility with `transformers.__version__ <
4.6.0` for seeded model initialization. This argument will be removed at the next major version. See
[pull request 11471](https://github.com/huggingface/transformers/pull/11471) for more information.
</Tip>
> Parameters for big model inference
low_cpu_mem_usage(`bool`, *optional*):
Tries to not use more than 1x model size in CPU memory (including peak memory) while loading the model.
This is an experimental feature and a subject to change at any moment.
torch_dtype (`str` or `torch.dtype`, *optional*):
Override the default `torch.dtype` and load the model under a specific `dtype`. The different options
are:
1. `torch.float16` or `torch.bfloat16` or `torch.float`: load in a specified
`dtype`, ignoring the model's `config.torch_dtype` if one exists. If not specified
- the model will get loaded in `torch.float` (fp32).
2. `"auto"` - A `torch_dtype` entry in the `config.json` file of the model will be
attempted to be used. If this entry isn't found then next check the `dtype` of the first weight in
the checkpoint that's of a floating point type and use that as `dtype`. This will load the model
using the `dtype` it was saved in at the end of the training. It can't be used as an indicator of how
the model was trained. Since it could be trained in one of half precision dtypes, but saved in fp32.
<Tip>
For some models the `dtype` they were trained in is unknown - you may try to check the model's paper or
reach out to the authors and ask them to add this information to the model's card and to insert the
`torch_dtype` entry in `config.json` on the hub.
</Tip>
device_map (`str` or `Dict[str, Union[int, str, torch.device]]` or `int` or `torch.device`, *optional*):
A map that specifies where each submodule should go. It doesn't need to be refined to each
parameter/buffer name, once a given module name is inside, every submodule of it will be sent to the
same device. If we only pass the device (*e.g.*, `"cpu"`, `"cuda:1"`, `"mps"`, or a GPU ordinal rank
like `1`) on which the model will be allocated, the device map will map the entire model to this
device. Passing `device_map = 0` means put the whole model on GPU 0.
To have Accelerate compute the most optimized `device_map` automatically, set `device_map="auto"`. For
more information about each option see [designing a device
map](https://hf.co/docs/accelerate/main/en/usage_guides/big_modeling#designing-a-device-map).
max_memory (`Dict`, *optional*):
A dictionary device identifier to maximum memory. Will default to the maximum memory available for each
GPU and the available CPU RAM if unset.
offload_folder (`str` or `os.PathLike`, *optional*):
If the `device_map` contains any value `"disk"`, the folder where we will offload weights.
offload_state_dict (`bool`, *optional*):
If `True`, will temporarily offload the CPU state dict to the hard drive to avoid getting out of CPU
RAM if the weight of the CPU state dict + the biggest shard of the checkpoint does not fit. Defaults to
`True` when there is some disk offload.
quantization_config (`Union[QuantizationConfigMixin,Dict]`, *optional*):
A dictionary of configuration parameters or a QuantizationConfigMixin object for quantization (e.g
bitsandbytes, gptq). There may be other quantization-related kwargs, including `load_in_4bit` and
`load_in_8bit`, which are parsed by QuantizationConfigParser. Supported only for bitsandbytes
quantizations and not preferred. consider inserting all such arguments into quantization_config
instead.
subfolder (`str`, *optional*, defaults to `""`):
In case the relevant files are located inside a subfolder of the model repo on huggingface.co, you can
specify the folder name here.
variant (`str`, *optional*):
If specified load weights from `variant` filename, *e.g.* pytorch_model.<variant>.bin. `variant` is
ignored when using `from_tf` or `from_flax`.
use_safetensors (`bool`, *optional*, defaults to `None`):
Whether or not to use `safetensors` checkpoints. Defaults to `None`. If not specified and `safetensors`
is not installed, it will be set to `False`.
kwargs (remaining dictionary of keyword arguments, *optional*):
Can be used to update the configuration object (after it being loaded) and initiate the model (e.g.,
`output_attentions=True`). Behaves differently depending on whether a `config` is provided or
automatically loaded:
- If a configuration is provided with `config`, `**kwargs` will be directly passed to the
underlying model's `__init__` method (we assume all relevant updates to the configuration have
already been done)
- If a configuration is not provided, `kwargs` will be first passed to the configuration class
initialization function ([`~PretrainedConfig.from_pretrained`]). Each key of `kwargs` that
corresponds to a configuration attribute will be used to override said attribute with the
supplied `kwargs` value. Remaining keys that do not correspond to any configuration attribute
will be passed to the underlying model's `__init__` function.
<Tip>
Activate the special ["offline-mode"](https://huggingface.co/transformers/installation.html#offline-mode) to
use this method in a firewalled environment.
</Tip>
Examples:
```python
>>> from transformers import BertConfig, BertModel
>>> # Download model and configuration from huggingface.co and cache.
>>> model = BertModel.from_pretrained("bert-base-uncased")
>>> # Model was saved using *save_pretrained('./test/saved_model/')* (for example purposes, not runnable).
>>> model = BertModel.from_pretrained("./test/saved_model/")
>>> # Update configuration during loading.
>>> model = BertModel.from_pretrained("bert-base-uncased", output_attentions=True)
>>> assert model.config.output_attentions == True
>>> # Loading from a TF checkpoint file instead of a PyTorch model (slower, for example purposes, not runnable).
>>> config = BertConfig.from_json_file("./tf_model/my_tf_model_config.json")
>>> model = BertModel.from_pretrained("./tf_model/my_tf_checkpoint.ckpt.index", from_tf=True, config=config)
>>> # Loading from a Flax checkpoint file instead of a PyTorch model (slower)
>>> model = BertModel.from_pretrained("bert-base-uncased", from_flax=True)
```
* `low_cpu_mem_usage` algorithm:
This is an experimental function that loads the model using ~1x model size CPU memory
Here is how it works:
1. save which state_dict keys we have
2. drop state_dict before the model is created, since the latter takes 1x model size CPU memory
3. after the model has been instantiated switch to the meta device all params/buffers that
are going to be replaced from the loaded state_dict
4. load state_dict 2nd time
5. replace the params/buffers from the state_dict
Currently, it can't handle deepspeed ZeRO stage 3 and ignores loading errors
"""
state_dict = kwargs.pop("state_dict", None)
from_tf = kwargs.pop("from_tf", False)
from_flax = kwargs.pop("from_flax", False)
resume_download = kwargs.pop("resume_download", False)
proxies = kwargs.pop("proxies", None)
output_loading_info = kwargs.pop("output_loading_info", False)
use_auth_token = kwargs.pop("use_auth_token", None)
trust_remote_code = kwargs.pop("trust_remote_code", None)
_ = kwargs.pop("mirror", None)
from_pipeline = kwargs.pop("_from_pipeline", None)
from_auto_class = kwargs.pop("_from_auto", False)
_fast_init = kwargs.pop("_fast_init", True)
torch_dtype = kwargs.pop("torch_dtype", None)
low_cpu_mem_usage = kwargs.pop("low_cpu_mem_usage", None)
device_map = kwargs.pop("device_map", None)
max_memory = kwargs.pop("max_memory", None)
offload_folder = kwargs.pop("offload_folder", None)
offload_state_dict = kwargs.pop("offload_state_dict", False)
load_in_8bit = kwargs.pop("load_in_8bit", False)
load_in_4bit = kwargs.pop("load_in_4bit", False)
quantization_config = kwargs.pop("quantization_config", None)
subfolder = kwargs.pop("subfolder", "")
commit_hash = kwargs.pop("_commit_hash", None)
variant = kwargs.pop("variant", None)
adapter_kwargs = kwargs.pop("adapter_kwargs", {})
adapter_name = kwargs.pop("adapter_name", "default")
use_flash_attention_2 = kwargs.pop("use_flash_attention_2", False)
if is_fsdp_enabled():
low_cpu_mem_usage = True
if use_auth_token is not None:
warnings.warn(
"The `use_auth_token` argument is deprecated and will be removed in v5 of Transformers. Please use `token` instead.",
FutureWarning,
)
if token is not None:
raise ValueError(
"`token` and `use_auth_token` are both specified. Please set only the argument `token`."
)
token = use_auth_token
if token is not None and adapter_kwargs is not None and "token" not in adapter_kwargs:
adapter_kwargs["token"] = token
if use_safetensors is None and not is_safetensors_available():
use_safetensors = False
if trust_remote_code is True:
logger.warning(
"The argument `trust_remote_code` is to be used with Auto classes. It has no effect here and is"
" ignored."
)
if commit_hash is None:
if not isinstance(config, PretrainedConfig):
# We make a call to the config file first (which may be absent) to get the commit hash as soon as possible
resolved_config_file = cached_file(
pretrained_model_name_or_path,
CONFIG_NAME,
cache_dir=cache_dir,
force_download=force_download,
resume_download=resume_download,
proxies=proxies,
local_files_only=local_files_only,
token=token,
revision=revision,
subfolder=subfolder,
_raise_exceptions_for_gated_repo=False,
_raise_exceptions_for_missing_entries=False,
_raise_exceptions_for_connection_errors=False,
)
commit_hash = extract_commit_hash(resolved_config_file, commit_hash)
else:
commit_hash = getattr(config, "_commit_hash", None)
if is_peft_available():
_adapter_model_path = adapter_kwargs.pop("_adapter_model_path", None)
if _adapter_model_path is None:
_adapter_model_path = find_adapter_config_file(
pretrained_model_name_or_path,
cache_dir=cache_dir,
force_download=force_download,
resume_download=resume_download,
proxies=proxies,
local_files_only=local_files_only,
_commit_hash=commit_hash,
**adapter_kwargs,
)
if _adapter_model_path is not None and os.path.isfile(_adapter_model_path):
with open(_adapter_model_path, "r", encoding="utf-8") as f:
_adapter_model_path = pretrained_model_name_or_path
pretrained_model_name_or_path = json.load(f)["base_model_name_or_path"]
else:
_adapter_model_path = None
# change device_map into a map if we passed an int, a str or a torch.device
if isinstance(device_map, torch.device):
device_map = {"": device_map}
elif isinstance(device_map, str) and device_map not in ["auto", "balanced", "balanced_low_0", "sequential"]:
try:
device_map = {"": torch.device(device_map)}
except RuntimeError:
raise ValueError(
"When passing device_map as a string, the value needs to be a device name (e.g. cpu, cuda:0) or "
f"'auto', 'balanced', 'balanced_low_0', 'sequential' but found {device_map}."
)
elif isinstance(device_map, int):
if device_map < 0:
raise ValueError(
"You can't pass device_map as a negative int. If you want to put the model on the cpu, pass device_map = 'cpu' "
)
else:
device_map = {"": device_map}
if device_map is not None:
if low_cpu_mem_usage is None:
low_cpu_mem_usage = True
elif not low_cpu_mem_usage:
raise ValueError("Passing along a `device_map` requires `low_cpu_mem_usage=True`")
if low_cpu_mem_usage:
if is_deepspeed_zero3_enabled():
raise ValueError(
"DeepSpeed Zero-3 is not compatible with `low_cpu_mem_usage=True` or with passing a `device_map`."
)
elif not is_accelerate_available():
raise ImportError(
"Using `low_cpu_mem_usage=True` or a `device_map` requires Accelerate: `pip install accelerate`"
)
# handling bnb config from kwargs, remove after `load_in_{4/8}bit` deprecation.
if load_in_4bit or load_in_8bit:
if quantization_config is not None:
raise ValueError(
"You can't pass `load_in_4bit`or `load_in_8bit` as a kwarg when passing "
"`quantization_config` argument at the same time."
)
# preparing BitsAndBytesConfig from kwargs
config_dict = {k: v for k, v in kwargs.items() if k in inspect.signature(BitsAndBytesConfig).parameters}
config_dict = {**config_dict, "load_in_4bit": load_in_4bit, "load_in_8bit": load_in_8bit}
quantization_config, kwargs = BitsAndBytesConfig.from_dict(
config_dict=config_dict, return_unused_kwargs=True, **kwargs
)
logger.warning(
"The `load_in_4bit` and `load_in_8bit` arguments are deprecated and will be removed in the future versions. "
"Please, pass a `BitsAndBytesConfig` object in `quantization_config` argument instead."
)
from_pt = not (from_tf | from_flax)
user_agent = {"file_type": "model", "framework": "pytorch", "from_auto_class": from_auto_class}
if from_pipeline is not None:
user_agent["using_pipeline"] = from_pipeline
if is_offline_mode() and not local_files_only:
logger.info("Offline mode: forcing local_files_only=True")
local_files_only = True
# Load config if we don't provide a configuration
if not isinstance(config, PretrainedConfig):
config_path = config if config is not None else pretrained_model_name_or_path
config, model_kwargs = cls.config_class.from_pretrained(
config_path,
cache_dir=cache_dir,
return_unused_kwargs=True,
force_download=force_download,
resume_download=resume_download,
proxies=proxies,
local_files_only=local_files_only,
token=token,
revision=revision,
subfolder=subfolder,
_from_auto=from_auto_class,
_from_pipeline=from_pipeline,
**kwargs,
)
else:
# In case one passes a config to `from_pretrained` + "attn_implementation"
# override the `_attn_implementation` attribute to `attn_implementation` of the kwargs
# Please see: https://github.com/huggingface/transformers/issues/28038
# Overwrite `config._attn_implementation` by the one from the kwargs --> in auto-factory
# we pop attn_implementation from the kwargs but this handles the case where users
# passes manually the config to `from_pretrained`.
config = copy.deepcopy(config)
kwarg_attn_imp = kwargs.pop("attn_implementation", None)
if kwarg_attn_imp is not None and config._attn_implementation != kwarg_attn_imp:
config._attn_implementation = kwarg_attn_imp
model_kwargs = kwargs
pre_quantized = getattr(config, "quantization_config", None) is not None
if pre_quantized or quantization_config is not None:
if pre_quantized:
config.quantization_config = AutoHfQuantizer.merge_quantization_configs(
config.quantization_config, quantization_config
)
else:
config.quantization_config = quantization_config
hf_quantizer = AutoHfQuantizer.from_config(config.quantization_config, pre_quantized=pre_quantized)
else:
hf_quantizer = None
if hf_quantizer is not None:
hf_quantizer.validate_environment(
torch_dtype=torch_dtype, from_tf=from_tf, from_flax=from_flax, device_map=device_map
)
torch_dtype = hf_quantizer.update_torch_dtype(torch_dtype)
device_map = hf_quantizer.update_device_map(device_map)
# Force-set to `True` for more mem efficiency
if low_cpu_mem_usage is None:
low_cpu_mem_usage = True
logger.warning("`low_cpu_mem_usage` was None, now set to True since model is quantized.")
# This variable will flag if we're loading a sharded checkpoint. In this case the archive file is just the
# index of the files.
is_sharded = False
sharded_metadata = None
# Load model
loading_info = None
# Keep in fp32 modules
keep_in_fp32_modules = None
use_keep_in_fp32_modules = False
if pretrained_model_name_or_path is not None:
pretrained_model_name_or_path = str(pretrained_model_name_or_path)
is_local = os.path.isdir(pretrained_model_name_or_path)
if is_local:
if from_tf and os.path.isfile(
os.path.join(pretrained_model_name_or_path, subfolder, TF_WEIGHTS_NAME + ".index")
):
# Load from a TF 1.0 checkpoint in priority if from_tf
archive_file = os.path.join(pretrained_model_name_or_path, subfolder, TF_WEIGHTS_NAME + ".index")
elif from_tf and os.path.isfile(
os.path.join(pretrained_model_name_or_path, subfolder, TF2_WEIGHTS_NAME)
):
# Load from a TF 2.0 checkpoint in priority if from_tf
archive_file = os.path.join(pretrained_model_name_or_path, subfolder, TF2_WEIGHTS_NAME)
elif from_flax and os.path.isfile(
os.path.join(pretrained_model_name_or_path, subfolder, FLAX_WEIGHTS_NAME)
):
# Load from a Flax checkpoint in priority if from_flax
archive_file = os.path.join(pretrained_model_name_or_path, subfolder, FLAX_WEIGHTS_NAME)
elif use_safetensors is not False and os.path.isfile(
os.path.join(pretrained_model_name_or_path, subfolder, _add_variant(SAFE_WEIGHTS_NAME, variant))
):
# Load from a safetensors checkpoint
archive_file = os.path.join(
pretrained_model_name_or_path, subfolder, _add_variant(SAFE_WEIGHTS_NAME, variant)
)
elif use_safetensors is not False and os.path.isfile(
os.path.join(
pretrained_model_name_or_path, subfolder, _add_variant(SAFE_WEIGHTS_INDEX_NAME, variant)
)
):
# Load from a sharded safetensors checkpoint
archive_file = os.path.join(
pretrained_model_name_or_path, subfolder, _add_variant(SAFE_WEIGHTS_INDEX_NAME, variant)
)
is_sharded = True
elif os.path.isfile(
os.path.join(pretrained_model_name_or_path, subfolder, _add_variant(WEIGHTS_NAME, variant))
):
# Load from a PyTorch checkpoint
archive_file = os.path.join(
pretrained_model_name_or_path, subfolder, _add_variant(WEIGHTS_NAME, variant)
)
elif os.path.isfile(
os.path.join(pretrained_model_name_or_path, subfolder, _add_variant(WEIGHTS_INDEX_NAME, variant))
):
# Load from a sharded PyTorch checkpoint
archive_file = os.path.join(
pretrained_model_name_or_path, subfolder, _add_variant(WEIGHTS_INDEX_NAME, variant)
)
is_sharded = True
# At this stage we don't have a weight file so we will raise an error.
elif os.path.isfile(
os.path.join(pretrained_model_name_or_path, subfolder, TF_WEIGHTS_NAME + ".index")
) or os.path.isfile(os.path.join(pretrained_model_name_or_path, subfolder, TF2_WEIGHTS_NAME)):
raise EnvironmentError(
f"Error no file named {_add_variant(WEIGHTS_NAME, variant)} found in directory"
f" {pretrained_model_name_or_path} but there is a file for TensorFlow weights. Use"
" `from_tf=True` to load this model from those weights."
)
elif os.path.isfile(os.path.join(pretrained_model_name_or_path, subfolder, FLAX_WEIGHTS_NAME)):
raise EnvironmentError(
f"Error no file named {_add_variant(WEIGHTS_NAME, variant)} found in directory"
f" {pretrained_model_name_or_path} but there is a file for Flax weights. Use `from_flax=True`"
" to load this model from those weights."
)
elif use_safetensors:
raise EnvironmentError(
f"Error no file named {_add_variant(SAFE_WEIGHTS_NAME, variant)} found in directory"
f" {pretrained_model_name_or_path}."
)
else:
raise EnvironmentError(
f"Error no file named {_add_variant(WEIGHTS_NAME, variant)}, {TF2_WEIGHTS_NAME},"
f" {TF_WEIGHTS_NAME + '.index'} or {FLAX_WEIGHTS_NAME} found in directory"
f" {pretrained_model_name_or_path}."
)
elif os.path.isfile(os.path.join(subfolder, pretrained_model_name_or_path)):
archive_file = pretrained_model_name_or_path
is_local = True
elif os.path.isfile(os.path.join(subfolder, pretrained_model_name_or_path + ".index")):
if not from_tf:
raise ValueError(
f"We found a TensorFlow checkpoint at {pretrained_model_name_or_path + '.index'}, please set "
"from_tf to True to load from this checkpoint."
)
archive_file = os.path.join(subfolder, pretrained_model_name_or_path + ".index")
is_local = True
elif is_remote_url(pretrained_model_name_or_path):
filename = pretrained_model_name_or_path
resolved_archive_file = download_url(pretrained_model_name_or_path)
else:
# set correct filename
if from_tf:
filename = TF2_WEIGHTS_NAME
elif from_flax:
filename = FLAX_WEIGHTS_NAME
elif use_safetensors is not False:
filename = _add_variant(SAFE_WEIGHTS_NAME, variant)
else:
filename = _add_variant(WEIGHTS_NAME, variant)
try:
# Load from URL or cache if already cached
cached_file_kwargs = {
"cache_dir": cache_dir,
"force_download": force_download,
"proxies": proxies,
"resume_download": resume_download,
"local_files_only": local_files_only,
"token": token,
"user_agent": user_agent,
"revision": revision,
"subfolder": subfolder,
"_raise_exceptions_for_gated_repo": False,
"_raise_exceptions_for_missing_entries": False,
"_commit_hash": commit_hash,
}
resolved_archive_file = cached_file(pretrained_model_name_or_path, filename, **cached_file_kwargs)
# Since we set _raise_exceptions_for_missing_entries=False, we don't get an exception but a None
# result when internet is up, the repo and revision exist, but the file does not.
if resolved_archive_file is None and filename == _add_variant(SAFE_WEIGHTS_NAME, variant):
# Maybe the checkpoint is sharded, we try to grab the index name in this case.
resolved_archive_file = cached_file(
pretrained_model_name_or_path,
_add_variant(SAFE_WEIGHTS_INDEX_NAME, variant),
**cached_file_kwargs,
)
if resolved_archive_file is not None:
is_sharded = True
elif use_safetensors:
if revision == "main":
resolved_archive_file, revision, is_sharded = auto_conversion(
pretrained_model_name_or_path, **cached_file_kwargs
)
cached_file_kwargs["revision"] = revision
if resolved_archive_file is None:
raise EnvironmentError(
f"{pretrained_model_name_or_path} does not appear to have a file named"
f" {_add_variant(SAFE_WEIGHTS_NAME, variant)} or {_add_variant(SAFE_WEIGHTS_INDEX_NAME, variant)} "
"and thus cannot be loaded with `safetensors`. Please make sure that the model has "
"been saved with `safe_serialization=True` or do not set `use_safetensors=True`."
)
else:
# This repo has no safetensors file of any kind, we switch to PyTorch.
filename = _add_variant(WEIGHTS_NAME, variant)
resolved_archive_file = cached_file(
pretrained_model_name_or_path, filename, **cached_file_kwargs
)
if resolved_archive_file is None and filename == _add_variant(WEIGHTS_NAME, variant):
# Maybe the checkpoint is sharded, we try to grab the index name in this case.
resolved_archive_file = cached_file(
pretrained_model_name_or_path,
_add_variant(WEIGHTS_INDEX_NAME, variant),
**cached_file_kwargs,
)
if resolved_archive_file is not None:
is_sharded = True
if resolved_archive_file is None:
# Otherwise, maybe there is a TF or Flax model file. We try those to give a helpful error
# message.
has_file_kwargs = {
"revision": revision,
"proxies": proxies,
"token": token,
}
if has_file(pretrained_model_name_or_path, TF2_WEIGHTS_NAME, **has_file_kwargs):
raise EnvironmentError(
f"{pretrained_model_name_or_path} does not appear to have a file named"
f" {_add_variant(WEIGHTS_NAME, variant)} but there is a file for TensorFlow weights."
" Use `from_tf=True` to load this model from those weights."
)
elif has_file(pretrained_model_name_or_path, FLAX_WEIGHTS_NAME, **has_file_kwargs):
raise EnvironmentError(
f"{pretrained_model_name_or_path} does not appear to have a file named"
f" {_add_variant(WEIGHTS_NAME, variant)} but there is a file for Flax weights. Use"
" `from_flax=True` to load this model from those weights."
)
elif variant is not None and has_file(
pretrained_model_name_or_path, WEIGHTS_NAME, **has_file_kwargs
):
raise EnvironmentError(
f"{pretrained_model_name_or_path} does not appear to have a file named"
f" {_add_variant(WEIGHTS_NAME, variant)} but there is a file without the variant"
f" {variant}. Use `variant=None` to load this model from those weights."
)
else:
raise EnvironmentError(
f"{pretrained_model_name_or_path} does not appear to have a file named"
f" {_add_variant(WEIGHTS_NAME, variant)}, {TF2_WEIGHTS_NAME}, {TF_WEIGHTS_NAME} or"
f" {FLAX_WEIGHTS_NAME}."
)
except EnvironmentError:
# Raise any environment error raise by `cached_file`. It will have a helpful error message adapted
# to the original exception.
raise
except Exception as e:
# For any other exception, we throw a generic error.
raise EnvironmentError(
f"Can't load the model for '{pretrained_model_name_or_path}'. If you were trying to load it"
" from 'https://huggingface.co/models', make sure you don't have a local directory with the"
f" same name. Otherwise, make sure '{pretrained_model_name_or_path}' is the correct path to a"
f" directory containing a file named {_add_variant(WEIGHTS_NAME, variant)},"
f" {TF2_WEIGHTS_NAME}, {TF_WEIGHTS_NAME} or {FLAX_WEIGHTS_NAME}."
) from e
if is_local:
logger.info(f"loading weights file {archive_file}")
resolved_archive_file = archive_file
else:
logger.info(f"loading weights file {filename} from cache at {resolved_archive_file}")
else:
resolved_archive_file = None
# We'll need to download and cache each checkpoint shard if the checkpoint is sharded.
if is_sharded:
# rsolved_archive_file becomes a list of files that point to the different checkpoint shards in this case.
resolved_archive_file, sharded_metadata = get_checkpoint_shard_files(
pretrained_model_name_or_path,
resolved_archive_file,
cache_dir=cache_dir,
force_download=force_download,
proxies=proxies,
resume_download=resume_download,
local_files_only=local_files_only,
token=token,
user_agent=user_agent,
revision=revision,
subfolder=subfolder,
_commit_hash=commit_hash,
)
if (
is_safetensors_available()
and isinstance(resolved_archive_file, str)
and resolved_archive_file.endswith(".safetensors")
):
with safe_open(resolved_archive_file, framework="pt") as f:
metadata = f.metadata()
if metadata.get("format") == "pt":
pass
elif metadata.get("format") == "tf":
from_tf = True
logger.info("A TensorFlow safetensors file is being loaded in a PyTorch model.")
elif metadata.get("format") == "flax":
from_flax = True
logger.info("A Flax safetensors file is being loaded in a PyTorch model.")
else:
raise ValueError(
f"Incompatible safetensors file. File metadata is not ['pt', 'tf', 'flax'] but {metadata.get('format')}"
)
from_pt = not (from_tf | from_flax)
# load pt weights early so that we know which dtype to init the model under
if from_pt:
if not is_sharded and state_dict is None:
# Time to load the checkpoint
state_dict = load_state_dict(resolved_archive_file)
# set dtype to instantiate the model under:
# 1. If torch_dtype is not None, we use that dtype
# 2. If torch_dtype is "auto", we auto-detect dtype from the loaded state_dict, by checking its first
# weights entry that is of a floating type - we assume all floating dtype weights are of the same dtype
# we also may have config.torch_dtype available, but we won't rely on it till v5
dtype_orig = None
if torch_dtype is not None:
if isinstance(torch_dtype, str):
if torch_dtype == "auto":
if hasattr(config, "torch_dtype") and config.torch_dtype is not None:
torch_dtype = config.torch_dtype
logger.info(f"Will use torch_dtype={torch_dtype} as defined in model's config object")
else:
if is_sharded and "dtype" in sharded_metadata:
torch_dtype = sharded_metadata["dtype"]
elif not is_sharded:
torch_dtype = get_state_dict_dtype(state_dict)
else:
one_state_dict = load_state_dict(resolved_archive_file[0])
torch_dtype = get_state_dict_dtype(one_state_dict)
del one_state_dict # free CPU memory
logger.info(
"Since the `torch_dtype` attribute can't be found in model's config object, "
"will use torch_dtype={torch_dtype} as derived from model's weights"
)
else:
raise ValueError(
f'`torch_dtype` can be either `torch.dtype` or `"auto"`, but received {torch_dtype}'
)
dtype_orig = cls._set_default_torch_dtype(torch_dtype)
# Check if `_keep_in_fp32_modules` is not None
use_keep_in_fp32_modules = (cls._keep_in_fp32_modules is not None) and (
(torch_dtype == torch.float16) or hasattr(hf_quantizer, "use_keep_in_fp32_modules")
)
if is_sharded:
loaded_state_dict_keys = sharded_metadata["all_checkpoint_keys"]
else:
loaded_state_dict_keys = list(state_dict.keys())
if low_cpu_mem_usage or (use_keep_in_fp32_modules and is_accelerate_available()):
# In case some weights need to be kept in float32 and accelerate is not installed,
# we later on want to take the path where state_dict is not None, that is the one
# that do not require accelerate.
state_dict = None
config.name_or_path = pretrained_model_name_or_path
# Instantiate model.
init_contexts = [no_init_weights(_enable=_fast_init)]
if is_deepspeed_zero3_enabled():
import deepspeed
logger.info("Detected DeepSpeed ZeRO-3: activating zero.init() for this model")
init_contexts = [deepspeed.zero.Init(config_dict_or_path=deepspeed_config())] + init_contexts
elif low_cpu_mem_usage:
init_contexts.append(init_empty_weights())
config = copy.deepcopy(config) # We do not want to modify the config inplace in from_pretrained.
config = cls._autoset_attn_implementation(
config, use_flash_attention_2=use_flash_attention_2, torch_dtype=torch_dtype, device_map=device_map
)
with ContextManagers(init_contexts):
# Let's make sure we don't run the init function of buffer modules
model = cls(config, *model_args, **model_kwargs)
# make sure we use the model's config since the __init__ call might have copied it
config = model.config
# Check first if we are `from_pt`
if use_keep_in_fp32_modules:
if is_accelerate_available() and not is_deepspeed_zero3_enabled():
low_cpu_mem_usage = True
keep_in_fp32_modules = model._keep_in_fp32_modules
else:
keep_in_fp32_modules = []
if hf_quantizer is not None:
hf_quantizer.preprocess_model(
model=model, device_map=device_map, keep_in_fp32_modules=keep_in_fp32_modules
)
# We store the original dtype for quantized models as we cannot easily retrieve it
# once the weights have been quantized
# Note that once you have loaded a quantized model, you can't change its dtype so this will
# remain a single source of truth
config._pre_quantization_dtype = torch_dtype
if isinstance(device_map, str):
special_dtypes = {}
if hf_quantizer is not None:
special_dtypes.update(hf_quantizer.get_special_dtypes_update(model, torch_dtype))
special_dtypes.update(
{
name: torch.float32
for name, _ in model.named_parameters()
if any(m in name for m in keep_in_fp32_modules)
}
)
target_dtype = torch_dtype
if hf_quantizer is not None:
target_dtype = hf_quantizer.adjust_target_dtype(target_dtype)
no_split_modules = model._get_no_split_modules(device_map)
if device_map not in ["auto", "balanced", "balanced_low_0", "sequential"]:
raise ValueError(
"If passing a string for `device_map`, please choose 'auto', 'balanced', 'balanced_low_0' or "
"'sequential'."
)
device_map_kwargs = {"no_split_module_classes": no_split_modules}
if "special_dtypes" in inspect.signature(infer_auto_device_map).parameters:
device_map_kwargs["special_dtypes"] = special_dtypes
elif len(special_dtypes) > 0:
logger.warning(
"This model has some weights that should be kept in higher precision, you need to upgrade "
"`accelerate` to properly deal with them (`pip install --upgrade accelerate`)."
)
if device_map != "sequential":
max_memory = get_balanced_memory(
model,
dtype=target_dtype,
low_zero=(device_map == "balanced_low_0"),
max_memory=max_memory,
**device_map_kwargs,
)
else:
max_memory = get_max_memory(max_memory)
if hf_quantizer is not None:
max_memory = hf_quantizer.adjust_max_memory(max_memory)
device_map_kwargs["max_memory"] = max_memory
# Make sure tied weights are tied before creating the device map.
model.tie_weights()
device_map = infer_auto_device_map(model, dtype=target_dtype, **device_map_kwargs)
if hf_quantizer is not None:
hf_quantizer.validate_environment(device_map=device_map)
elif device_map is not None:
model.tie_weights()
tied_params = find_tied_parameters(model)
# check if we don't have tied param in different devices
check_tied_parameters_on_same_device(tied_params, device_map)
if from_tf:
if resolved_archive_file.endswith(".index"):
# Load from a TensorFlow 1.X checkpoint - provided by original authors
model = cls.load_tf_weights(model, config, resolved_archive_file[:-6]) # Remove the '.index'
else:
# Load from our TensorFlow 2.0 checkpoints
try:
from .modeling_tf_pytorch_utils import load_tf2_checkpoint_in_pytorch_model
model, loading_info = load_tf2_checkpoint_in_pytorch_model(
model, resolved_archive_file, allow_missing_keys=True, output_loading_info=True
)
except ImportError:
logger.error(
"Loading a TensorFlow model in PyTorch, requires both PyTorch and TensorFlow to be installed."
" Please see https://pytorch.org/ and https://www.tensorflow.org/install/ for installation"
" instructions."
)
raise
elif from_flax:
try:
from .modeling_flax_pytorch_utils import load_flax_checkpoint_in_pytorch_model
model = load_flax_checkpoint_in_pytorch_model(model, resolved_archive_file)
except ImportError:
logger.error(
"Loading a Flax model in PyTorch, requires both PyTorch and Flax to be installed. Please see"
" https://pytorch.org/ and https://flax.readthedocs.io/en/latest/installation.html for"
" installation instructions."
)
raise
elif from_pt:
# restore default dtype
if dtype_orig is not None:
torch.set_default_dtype(dtype_orig)
(
model,
missing_keys,
unexpected_keys,
mismatched_keys,
offload_index,
error_msgs,
) = cls._load_pretrained_model(
model,
state_dict,
loaded_state_dict_keys, # XXX: rename?
resolved_archive_file,
pretrained_model_name_or_path,
ignore_mismatched_sizes=ignore_mismatched_sizes,
sharded_metadata=sharded_metadata,
_fast_init=_fast_init,
low_cpu_mem_usage=low_cpu_mem_usage,
device_map=device_map,
offload_folder=offload_folder,
offload_state_dict=offload_state_dict,
dtype=torch_dtype,
hf_quantizer=hf_quantizer,
keep_in_fp32_modules=keep_in_fp32_modules,
)
# make sure token embedding weights are still tied if needed
model.tie_weights()
# Set model in evaluation mode to deactivate DropOut modules by default
model.eval()
# If it is a model with generation capabilities, attempt to load the generation config
if model.can_generate() and pretrained_model_name_or_path is not None:
try:
model.generation_config = GenerationConfig.from_pretrained(
pretrained_model_name_or_path,
cache_dir=cache_dir,
force_download=force_download,
resume_download=resume_download,
proxies=proxies,
local_files_only=local_files_only,
token=token,
revision=revision,
subfolder=subfolder,
_from_auto=from_auto_class,
_from_pipeline=from_pipeline,
**kwargs,
)
except OSError:
logger.info(
"Generation config file not found, using a generation config created from the model config."
)
pass
# Dispatch model with hooks on all devices if necessary
if device_map is not None:
device_map_kwargs = {
"device_map": device_map,
"offload_dir": offload_folder,
"offload_index": offload_index,
}
if "skip_keys" in inspect.signature(dispatch_model).parameters:
device_map_kwargs["skip_keys"] = model._skip_keys_device_placement
dispatch_model(model, **device_map_kwargs)
if hf_quantizer is not None:
hf_quantizer.postprocess_model(model)
model.hf_quantizer = hf_quantizer
if _adapter_model_path is not None:
model.load_adapter(
_adapter_model_path,
adapter_name=adapter_name,
token=token,
adapter_kwargs=adapter_kwargs,
)
if output_loading_info:
if loading_info is None:
loading_info = {
"missing_keys": missing_keys,
"unexpected_keys": unexpected_keys,
"mismatched_keys": mismatched_keys,
"error_msgs": error_msgs,
}
return model, loading_info
return model
@classmethod
def _load_pretrained_model(
cls,
model,
state_dict,
loaded_keys,
resolved_archive_file,
pretrained_model_name_or_path,
ignore_mismatched_sizes=False,
sharded_metadata=None,
_fast_init=True,
low_cpu_mem_usage=False,
device_map=None,
offload_folder=None,
offload_state_dict=None,
dtype=None,
hf_quantizer=None,
keep_in_fp32_modules=None,
):
is_safetensors = False
if device_map is not None and "disk" in device_map.values():
archive_file = (
resolved_archive_file[0] if isinstance(resolved_archive_file, (list, tuple)) else resolved_archive_file
)
is_safetensors = archive_file.endswith(".safetensors")
if offload_folder is None and not is_safetensors:
raise ValueError(
"The current `device_map` had weights offloaded to the disk. Please provide an `offload_folder`"
" for them. Alternatively, make sure you have `safetensors` installed if the model you are using"
" offers the weights in this format."
)
if offload_folder is not None:
os.makedirs(offload_folder, exist_ok=True)
if offload_state_dict is None:
offload_state_dict = True
is_sharded_safetensors = is_safetensors and sharded_metadata is not None
# tie the model weights before retrieving the state_dict
model.tie_weights()
# Retrieve missing & unexpected_keys
model_state_dict = model.state_dict()
expected_keys = list(model_state_dict.keys())
prefix = model.base_model_prefix
def _fix_key(key):
if "beta" in key:
return key.replace("beta", "bias")
if "gamma" in key:
return key.replace("gamma", "weight")
return key
original_loaded_keys = loaded_keys
loaded_keys = [_fix_key(key) for key in loaded_keys]
if len(prefix) > 0:
has_prefix_module = any(s.startswith(prefix) for s in loaded_keys)
expects_prefix_module = any(s.startswith(prefix) for s in expected_keys)
else:
has_prefix_module = False
expects_prefix_module = False
# key re-naming operations are never done on the keys
# that are loaded, but always on the keys of the newly initialized model
remove_prefix_from_model = not has_prefix_module and expects_prefix_module
add_prefix_to_model = has_prefix_module and not expects_prefix_module
if remove_prefix_from_model:
_prefix = f"{prefix}."
expected_keys_not_prefixed = [s for s in expected_keys if not s.startswith(_prefix)]
expected_keys = [s[len(_prefix) :] if s.startswith(_prefix) else s for s in expected_keys]
elif add_prefix_to_model:
expected_keys = [".".join([prefix, s]) for s in expected_keys]
missing_keys = sorted(set(expected_keys) - set(loaded_keys))
unexpected_keys = set(loaded_keys) - set(expected_keys)
# Remove nonpersistent buffers from unexpected keys: they are not in the state dict but will be in the model
# buffers
model_buffers = {n for n, _ in model.named_buffers()}
if remove_prefix_from_model:
model_buffers = {key[len(_prefix) :] if key.startswith(_prefix) else key for key in model_buffers}
elif add_prefix_to_model:
model_buffers = {".".join([prefix, key]) for key in model_buffers}
unexpected_keys = sorted(unexpected_keys - model_buffers)
model.tie_weights()
if device_map is None and not is_fsdp_enabled() and not is_deepspeed_zero3_enabled():
ptrs = collections.defaultdict(list)
for name, tensor in model.state_dict().items():
id_tensor = id_tensor_storage(tensor)
ptrs[id_tensor].append(name)
# These are all the pointers of shared tensors.
tied_params = [names for _, names in ptrs.items() if len(names) > 1]
else:
# id function doesn't work for meta tensor so we need this function
tied_params = find_tied_parameters(model)
for group in tied_params:
if remove_prefix_from_model:
group = [key[len(_prefix) :] if key.startswith(_prefix) else key for key in group]
elif add_prefix_to_model:
group = [".".join([prefix, key]) for key in group]
missing_in_group = [k for k in missing_keys if k in group]
if len(missing_in_group) > 0 and len(missing_in_group) < len(group):
missing_keys = [k for k in missing_keys if k not in missing_in_group]
# Some models may have keys that are not in the state by design, removing them before needlessly warning
# the user.
if cls._keys_to_ignore_on_load_missing is not None:
for pat in cls._keys_to_ignore_on_load_missing:
missing_keys = [k for k in missing_keys if re.search(pat, k) is None]
if cls._keys_to_ignore_on_load_unexpected is not None:
for pat in cls._keys_to_ignore_on_load_unexpected:
unexpected_keys = [k for k in unexpected_keys if re.search(pat, k) is None]
# retrieve weights on meta device and put them back on CPU.
# This is not ideal in terms of memory, but if we don't do that not, we can't initialize them in the next step
if low_cpu_mem_usage:
for key in missing_keys:
if key in list(model_state_dict.keys()):
key = key
elif f"{prefix}.{key}" in list(model_state_dict.keys()):
key = f"{prefix}.{key}"
elif key.startswith(prefix) and ".".join(key.split(".")[1:]) in list(model_state_dict.keys()):
key = ".".join(key.split(".")[1:])
param = model_state_dict[key]
# upcast in fp32 if any
target_dtype = dtype
if (
keep_in_fp32_modules is not None
and dtype == torch.float16
and any(
module_to_keep_in_fp32 in key.split(".") for module_to_keep_in_fp32 in keep_in_fp32_modules
)
):
target_dtype = torch.float32
if param.device == torch.device("meta"):
value = torch.empty(*param.size(), dtype=target_dtype)
if (
hf_quantizer is None
or getattr(hf_quantizer, "requires_parameters_quantization", False)
or not hf_quantizer.check_quantized_param(
model, param_value=value, param_name=key, state_dict={}
)
):
set_module_tensor_to_device(model, key, "cpu", value)
else:
hf_quantizer.create_quantized_param(model, value, key, "cpu", state_dict)
# retrieve uninitialized modules and initialize before maybe overriding that with the pretrained weights.
if _fast_init:
if not ignore_mismatched_sizes:
if remove_prefix_from_model:
_loaded_keys = [f"{prefix}.{k}" for k in loaded_keys]
elif add_prefix_to_model:
_loaded_keys = [k[len(prefix) + 1 :] for k in loaded_keys]
else:
_loaded_keys = loaded_keys
not_initialized_submodules = set_initialized_submodules(model, _loaded_keys)
# if we're about to tie the output embeds to the input embeds we don't need to init them
if hasattr(model.config, "tie_word_embeddings") and model.config.tie_word_embeddings:
output_embeddings = model.get_output_embeddings()
if output_embeddings is not None:
output_embeddings._is_hf_initialized = True
else:
not_initialized_submodules = dict(model.named_modules())
# This will only initialize submodules that are not marked as initialized by the line above.
if is_deepspeed_zero3_enabled():
import deepspeed
not_initialized_parameters = list(
set(
itertools.chain.from_iterable(
submodule.parameters(recurse=False) for submodule in not_initialized_submodules.values()
)
)
)
with deepspeed.zero.GatheredParameters(not_initialized_parameters, modifier_rank=0):
model.apply(model._initialize_weights)
else:
model.apply(model._initialize_weights)
# Set some modules to fp32 if any
if keep_in_fp32_modules is not None:
for name, param in model.named_parameters():
if any(module_to_keep_in_fp32 in name.split(".") for module_to_keep_in_fp32 in keep_in_fp32_modules):
# param = param.to(torch.float32) does not work here as only in the local scope.
param.data = param.data.to(torch.float32)
# Make sure we are able to load base models as well as derived models (with heads)
start_prefix = ""
model_to_load = model
if len(cls.base_model_prefix) > 0 and not hasattr(model, cls.base_model_prefix) and has_prefix_module:
start_prefix = cls.base_model_prefix + "."
if len(cls.base_model_prefix) > 0 and hasattr(model, cls.base_model_prefix) and not has_prefix_module:
model_to_load = getattr(model, cls.base_model_prefix)
base_model_expected_keys = list(model_to_load.state_dict().keys())
if any(key in expected_keys_not_prefixed and key not in base_model_expected_keys for key in loaded_keys):
raise ValueError(
"The state dictionary of the model you are trying to load is corrupted. Are you sure it was "
"properly saved?"
)
if device_map is not None:
device_map = {k.replace(f"{cls.base_model_prefix}.", ""): v for k, v in device_map.items()}
def _find_mismatched_keys(
state_dict,
model_state_dict,
loaded_keys,
add_prefix_to_model,
remove_prefix_from_model,
ignore_mismatched_sizes,
):
mismatched_keys = []
if ignore_mismatched_sizes:
for checkpoint_key in loaded_keys:
# If the checkpoint is sharded, we may not have the key here.
if checkpoint_key not in state_dict:
continue
model_key = checkpoint_key
if remove_prefix_from_model:
# The model key starts with `prefix` but `checkpoint_key` doesn't so we add it.
model_key = f"{prefix}.{checkpoint_key}"
elif add_prefix_to_model:
# The model key doesn't start with `prefix` but `checkpoint_key` does so we remove it.
model_key = ".".join(checkpoint_key.split(".")[1:])
if (
model_key in model_state_dict
and state_dict[checkpoint_key].shape != model_state_dict[model_key].shape
):
if (
state_dict[checkpoint_key].shape[-1] == 1
and state_dict[checkpoint_key].numel() * 2 == model_state_dict[model_key].numel()
):
# This skips size mismatches for 4-bit weights. Two 4-bit values share an 8-bit container, causing size differences.
# Without matching with module type or paramter type it seems like a practical way to detect valid 4bit weights.
pass
else:
mismatched_keys.append(
(checkpoint_key, state_dict[checkpoint_key].shape, model_state_dict[model_key].shape)
)
del state_dict[checkpoint_key]
return mismatched_keys
if resolved_archive_file is not None:
folder = os.path.sep.join(resolved_archive_file[0].split(os.path.sep)[:-1])
else:
folder = None
if device_map is not None and is_safetensors:
param_device_map = expand_device_map(device_map, original_loaded_keys, start_prefix)
str_dtype = str(dtype).replace("torch.", "") if dtype is not None else "float32"
if sharded_metadata is None:
archive_file = (
resolved_archive_file[0]
if isinstance(resolved_archive_file, (list, tuple))
else resolved_archive_file
)
weight_map = {p: archive_file for p in original_loaded_keys}
else:
weight_map = {p: os.path.join(folder, f) for p, f in sharded_metadata["weight_map"].items()}
offload_index = {
p[len(start_prefix) :]: {"safetensors_file": f, "weight_name": p, "dtype": str_dtype}
for p, f in weight_map.items()
if p.startswith(start_prefix) and param_device_map[p[len(start_prefix) :]] == "disk"
}
if state_dict is not None:
# Whole checkpoint
mismatched_keys = _find_mismatched_keys(
state_dict,
model_state_dict,
original_loaded_keys,
add_prefix_to_model,
remove_prefix_from_model,
ignore_mismatched_sizes,
)
error_msgs = _load_state_dict_into_model(model_to_load, state_dict, start_prefix)
offload_index = None
else:
# Sharded checkpoint or whole but low_cpu_mem_usage==True
# This should always be a list but, just to be sure.
if not isinstance(resolved_archive_file, list):
resolved_archive_file = [resolved_archive_file]
error_msgs = []
mismatched_keys = []
if not is_safetensors:
offload_index = {} if device_map is not None and "disk" in device_map.values() else None
if offload_state_dict:
state_dict_folder = tempfile.mkdtemp()
state_dict_index = {}
else:
state_dict_folder = None
state_dict_index = None
if is_sharded_safetensors:
disk_only_shard_files = get_disk_only_shard_files(
device_map, sharded_metadata=sharded_metadata, start_prefix=start_prefix
)
disk_only_shard_files = [os.path.join(folder, f) for f in disk_only_shard_files]
else:
disk_only_shard_files = []
if len(resolved_archive_file) > 1:
resolved_archive_file = logging.tqdm(resolved_archive_file, desc="Loading checkpoint shards")
for shard_file in resolved_archive_file:
# Skip the load for shards that only contain disk-offloaded weights when using safetensors for the offload.
if shard_file in disk_only_shard_files:
continue
state_dict = load_state_dict(shard_file)
# Mistmatched keys contains tuples key/shape1/shape2 of weights in the checkpoint that have a shape not
# matching the weights in the model.
mismatched_keys += _find_mismatched_keys(
state_dict,
model_state_dict,
original_loaded_keys,
add_prefix_to_model,
remove_prefix_from_model,
ignore_mismatched_sizes,
)
if low_cpu_mem_usage:
if is_fsdp_enabled() and not is_local_dist_rank_0():
for key, param in model_to_load.state_dict().items():
if param.device == torch.device("meta"):
if hf_quantizer is None:
set_module_tensor_to_device(
model_to_load, key, "cpu", torch.empty(*param.size(), dtype=dtype)
)
else:
hf_quantizer.create_quantized_param(model, param, key, "cpu", state_dict)
else:
new_error_msgs, offload_index, state_dict_index = _load_state_dict_into_meta_model(
model_to_load,
state_dict,
loaded_keys,
start_prefix,
expected_keys,
device_map=device_map,
offload_folder=offload_folder,
offload_index=offload_index,
state_dict_folder=state_dict_folder,
state_dict_index=state_dict_index,
dtype=dtype,
hf_quantizer=hf_quantizer,
is_safetensors=is_safetensors,
keep_in_fp32_modules=keep_in_fp32_modules,
unexpected_keys=unexpected_keys,
)
error_msgs += new_error_msgs
else:
error_msgs += _load_state_dict_into_model(model_to_load, state_dict, start_prefix)
# force memory release
del state_dict
gc.collect()
if offload_index is not None and len(offload_index) > 0:
if model != model_to_load:
# We need to add the prefix of the base model
prefix = cls.base_model_prefix
if not is_safetensors:
for weight_name in offload_index:
shutil.move(
os.path.join(offload_folder, f"{weight_name}.dat"),
os.path.join(offload_folder, f"{prefix}.{weight_name}.dat"),
)
offload_index = {f"{prefix}.{key}": value for key, value in offload_index.items()}
if not is_safetensors:
save_offload_index(offload_index, offload_folder)
offload_index = None
if offload_state_dict:
# Load back temporarily offloaded state dict
load_offloaded_weights(model_to_load, state_dict_index, state_dict_folder)
shutil.rmtree(state_dict_folder)
if len(error_msgs) > 0:
error_msg = "\n\t".join(error_msgs)
if "size mismatch" in error_msg:
error_msg += (
"\n\tYou may consider adding `ignore_mismatched_sizes=True` in the model `from_pretrained` method."
)
raise RuntimeError(f"Error(s) in loading state_dict for {model.__class__.__name__}:\n\t{error_msg}")
if len(unexpected_keys) > 0:
archs = [] if model.config.architectures is None else model.config.architectures
warner = logger.warning if model.__class__.__name__ in archs else logger.info
warner(
f"Some weights of the model checkpoint at {pretrained_model_name_or_path} were not used when"
f" initializing {model.__class__.__name__}: {unexpected_keys}\n- This IS expected if you are"
f" initializing {model.__class__.__name__} from the checkpoint of a model trained on another task or"
" with another architecture (e.g. initializing a BertForSequenceClassification model from a"
" BertForPreTraining model).\n- This IS NOT expected if you are initializing"
f" {model.__class__.__name__} from the checkpoint of a model that you expect to be exactly identical"
" (initializing a BertForSequenceClassification model from a BertForSequenceClassification model)."
)
else:
logger.info(f"All model checkpoint weights were used when initializing {model.__class__.__name__}.\n")
if len(missing_keys) > 0:
logger.warning(
f"Some weights of {model.__class__.__name__} were not initialized from the model checkpoint at"
f" {pretrained_model_name_or_path} and are newly initialized: {missing_keys}\nYou should probably"
" TRAIN this model on a down-stream task to be able to use it for predictions and inference."
)
elif len(mismatched_keys) == 0:
logger.info(
f"All the weights of {model.__class__.__name__} were initialized from the model checkpoint at"
f" {pretrained_model_name_or_path}.\nIf your task is similar to the task the model of the checkpoint"
f" was trained on, you can already use {model.__class__.__name__} for predictions without further"
" training."
)
if len(mismatched_keys) > 0:
mismatched_warning = "\n".join(
[
f"- {key}: found shape {shape1} in the checkpoint and {shape2} in the model instantiated"
for key, shape1, shape2 in mismatched_keys
]
)
logger.warning(
f"Some weights of {model.__class__.__name__} were not initialized from the model checkpoint at"
f" {pretrained_model_name_or_path} and are newly initialized because the shapes did not"
f" match:\n{mismatched_warning}\nYou should probably TRAIN this model on a down-stream task to be able"
" to use it for predictions and inference."
)
return model, missing_keys, unexpected_keys, mismatched_keys, offload_index, error_msgs
def retrieve_modules_from_names(self, names, add_prefix=False, remove_prefix=False):
module_keys = {".".join(key.split(".")[:-1]) for key in names}
# torch.nn.ParameterList is a special case where two parameter keywords
# are appended to the module name, *e.g.* bert.special_embeddings.0
module_keys = module_keys.union(
{".".join(key.split(".")[:-2]) for key in names if len(key) > 0 and key[-1].isdigit()}
)
retrieved_modules = []
# retrieve all modules that has at least one missing weight name
for name, module in self.named_modules():
if remove_prefix:
_prefix = f"{self.base_model_prefix}."
name = name[len(_prefix) :] if name.startswith(_prefix) else name
elif add_prefix:
name = ".".join([self.base_model_prefix, name]) if len(name) > 0 else self.base_model_prefix
if name in module_keys:
retrieved_modules.append(module)
return retrieved_modules
@staticmethod
def _load_pretrained_model_low_mem(
model, loaded_state_dict_keys, resolved_archive_file, start_prefix="", hf_quantizer=None
):
"""
This is an experimental function that loads the model using ~1.x model size CPU memory
Before you call it do:
1. save which state_dict keys are available
2. drop state_dict before model is created, since the latter takes 1x model size memory
Here then we continue:
3. switch to the meta device all params/buffers that are going to be replaced from the loaded state_dict
4. load state_dict 2nd time
5. replace the params/buffers from the state_dict
Currently, it doesn't handle missing_keys, unexpected_keys, mismatched_keys. It can't handle deepspeed. To
handle bitsandbytes, needs non-empty hf_quantizer argument.
"""
_move_model_to_meta(model, loaded_state_dict_keys, start_prefix)
state_dict = load_state_dict(resolved_archive_file)
expected_keys = loaded_state_dict_keys # plug for missing expected_keys. TODO: replace with proper keys
error_msgs = _load_state_dict_into_meta_model(
model,
state_dict,
loaded_state_dict_keys,
start_prefix,
expected_keys=expected_keys,
hf_quantizer=hf_quantizer,
)
return error_msgs
@classmethod
def register_for_auto_class(cls, auto_class="AutoModel"):
"""
Register this class with a given auto class. This should only be used for custom models as the ones in the
library are already mapped with an auto class.
<Tip warning={true}>
This API is experimental and may have some slight breaking changes in the next releases.
</Tip>
Args:
auto_class (`str` or `type`, *optional*, defaults to `"AutoModel"`):
The auto class to register this new model with.
"""
if not isinstance(auto_class, str):
auto_class = auto_class.__name__
import transformers.models.auto as auto_module
if not hasattr(auto_module, auto_class):
raise ValueError(f"{auto_class} is not a valid auto class.")
cls._auto_class = auto_class
def to_bettertransformer(self) -> "PreTrainedModel":
"""
Converts the model to use [PyTorch's native attention
implementation](https://pytorch.org/docs/stable/generated/torch.nn.MultiheadAttention.html), integrated to
Transformers through [Optimum library](https://huggingface.co/docs/optimum/bettertransformer/overview). Only a
subset of all Transformers models are supported.
PyTorch's attention fastpath allows to speed up inference through kernel fusions and the use of [nested
tensors](https://pytorch.org/docs/stable/nested.html). Detailed benchmarks can be found in [this blog
post](https://medium.com/pytorch/bettertransformer-out-of-the-box-performance-for-huggingface-transformers-3fbe27d50ab2).
Returns:
[`PreTrainedModel`]: The model converted to BetterTransformer.
"""
if not is_optimum_available():
raise ImportError("The package `optimum` is required to use Better Transformer.")
from optimum.version import __version__ as optimum_version
if version.parse(optimum_version) < version.parse("1.7.0"):
raise ImportError(
f"Please install optimum>=1.7.0 to use Better Transformer. The version {optimum_version} was found."
)
from optimum.bettertransformer import BetterTransformer
return BetterTransformer.transform(self)
def reverse_bettertransformer(self):
"""
Reverts the transformation from [`~PreTrainedModel.to_bettertransformer`] so that the original modeling is
used, for example in order to save the model.
Returns:
[`PreTrainedModel`]: The model converted back to the original modeling.
"""
if not is_optimum_available():
raise ImportError("The package `optimum` is required to use Better Transformer.")
from optimum.version import __version__ as optimum_version
if version.parse(optimum_version) < version.parse("1.7.0"):
raise ImportError(
f"Please install optimum>=1.7.0 to use Better Transformer. The version {optimum_version} was found."
)
from optimum.bettertransformer import BetterTransformer
return BetterTransformer.reverse(self)
def warn_if_padding_and_no_attention_mask(self, input_ids, attention_mask):
"""
Shows a one-time warning if the input_ids appear to contain padding and no attention mask was given.
"""
# Skip the check during tracing.
if is_torch_fx_proxy(input_ids) or torch.jit.is_tracing() or is_torchdynamo_compiling():
return
if (attention_mask is not None) or (self.config.pad_token_id is None):
return
# Check only the first and last input IDs to reduce overhead.
if self.config.pad_token_id in input_ids[:, [-1, 0]]:
warn_string = (
"We strongly recommend passing in an `attention_mask` since your input_ids may be padded. See "
"https://huggingface.co/docs/transformers/troubleshooting"
"#incorrect-output-when-padding-tokens-arent-masked."
)
# If the pad token is equal to either BOS, EOS, or SEP, we do not know whether the user should use an
# attention_mask or not. In this case, we should still show a warning because this is a rare case.
if (
(self.config.bos_token_id is not None and self.config.bos_token_id == self.config.pad_token_id)
or (self.config.eos_token_id is not None and self.config.eos_token_id == self.config.pad_token_id)
or (self.config.sep_token_id is not None and self.config.sep_token_id == self.config.pad_token_id)
):
warn_string += (
f"\nYou may ignore this warning if your `pad_token_id` ({self.config.pad_token_id}) is identical "
f"to the `bos_token_id` ({self.config.bos_token_id}), `eos_token_id` ({self.config.eos_token_id}), "
f"or the `sep_token_id` ({self.config.sep_token_id}), and your input is not padded."
)
logger.warning_once(warn_string)
PreTrainedModel.push_to_hub = copy_func(PreTrainedModel.push_to_hub)
if PreTrainedModel.push_to_hub.__doc__ is not None:
PreTrainedModel.push_to_hub.__doc__ = PreTrainedModel.push_to_hub.__doc__.format(
object="model", object_class="AutoModel", object_files="model file"
)
class PoolerStartLogits(nn.Module):
"""
Compute SQuAD start logits from sequence hidden states.
Args:
config ([`PretrainedConfig`]):
The config used by the model, will be used to grab the `hidden_size` of the model.
"""
def __init__(self, config: PretrainedConfig):
super().__init__()
self.dense = nn.Linear(config.hidden_size, 1)
def forward(
self, hidden_states: torch.FloatTensor, p_mask: Optional[torch.FloatTensor] = None
) -> torch.FloatTensor:
"""
Args:
hidden_states (`torch.FloatTensor` of shape `(batch_size, seq_len, hidden_size)`):
The final hidden states of the model.
p_mask (`torch.FloatTensor` of shape `(batch_size, seq_len)`, *optional*):
Mask for tokens at invalid position, such as query and special symbols (PAD, SEP, CLS). 1.0 means token
should be masked.
Returns:
`torch.FloatTensor`: The start logits for SQuAD.
"""
x = self.dense(hidden_states).squeeze(-1)
if p_mask is not None:
if get_parameter_dtype(self) == torch.float16:
x = x * (1 - p_mask) - 65500 * p_mask
else:
x = x * (1 - p_mask) - 1e30 * p_mask
return x
class PoolerEndLogits(nn.Module):
"""
Compute SQuAD end logits from sequence hidden states.
Args:
config ([`PretrainedConfig`]):
The config used by the model, will be used to grab the `hidden_size` of the model and the `layer_norm_eps`
to use.
"""
def __init__(self, config: PretrainedConfig):
super().__init__()
self.dense_0 = nn.Linear(config.hidden_size * 2, config.hidden_size)
self.activation = nn.Tanh()
self.LayerNorm = nn.LayerNorm(config.hidden_size, eps=config.layer_norm_eps)
self.dense_1 = nn.Linear(config.hidden_size, 1)
def forward(
self,
hidden_states: torch.FloatTensor,
start_states: Optional[torch.FloatTensor] = None,
start_positions: Optional[torch.LongTensor] = None,
p_mask: Optional[torch.FloatTensor] = None,
) -> torch.FloatTensor:
"""
Args:
hidden_states (`torch.FloatTensor` of shape `(batch_size, seq_len, hidden_size)`):
The final hidden states of the model.
start_states (`torch.FloatTensor` of shape `(batch_size, seq_len, hidden_size)`, *optional*):
The hidden states of the first tokens for the labeled span.
start_positions (`torch.LongTensor` of shape `(batch_size,)`, *optional*):
The position of the first token for the labeled span.
p_mask (`torch.FloatTensor` of shape `(batch_size, seq_len)`, *optional*):
Mask for tokens at invalid position, such as query and special symbols (PAD, SEP, CLS). 1.0 means token
should be masked.
<Tip>
One of `start_states` or `start_positions` should be not `None`. If both are set, `start_positions` overrides
`start_states`.
</Tip>
Returns:
`torch.FloatTensor`: The end logits for SQuAD.
"""
assert (
start_states is not None or start_positions is not None
), "One of start_states, start_positions should be not None"
if start_positions is not None:
slen, hsz = hidden_states.shape[-2:]
start_positions = start_positions[:, None, None].expand(-1, -1, hsz) # shape (bsz, 1, hsz)
start_states = hidden_states.gather(-2, start_positions) # shape (bsz, 1, hsz)
start_states = start_states.expand(-1, slen, -1) # shape (bsz, slen, hsz)
x = self.dense_0(torch.cat([hidden_states, start_states], dim=-1))
x = self.activation(x)
x = self.LayerNorm(x)
x = self.dense_1(x).squeeze(-1)
if p_mask is not None:
if get_parameter_dtype(self) == torch.float16:
x = x * (1 - p_mask) - 65500 * p_mask
else:
x = x * (1 - p_mask) - 1e30 * p_mask
return x
class PoolerAnswerClass(nn.Module):
"""
Compute SQuAD 2.0 answer class from classification and start tokens hidden states.
Args:
config ([`PretrainedConfig`]):
The config used by the model, will be used to grab the `hidden_size` of the model.
"""
def __init__(self, config):
super().__init__()
self.dense_0 = nn.Linear(config.hidden_size * 2, config.hidden_size)
self.activation = nn.Tanh()
self.dense_1 = nn.Linear(config.hidden_size, 1, bias=False)
def forward(
self,
hidden_states: torch.FloatTensor,
start_states: Optional[torch.FloatTensor] = None,
start_positions: Optional[torch.LongTensor] = None,
cls_index: Optional[torch.LongTensor] = None,
) -> torch.FloatTensor:
"""
Args:
hidden_states (`torch.FloatTensor` of shape `(batch_size, seq_len, hidden_size)`):
The final hidden states of the model.
start_states (`torch.FloatTensor` of shape `(batch_size, seq_len, hidden_size)`, *optional*):
The hidden states of the first tokens for the labeled span.
start_positions (`torch.LongTensor` of shape `(batch_size,)`, *optional*):
The position of the first token for the labeled span.
cls_index (`torch.LongTensor` of shape `(batch_size,)`, *optional*):
Position of the CLS token for each sentence in the batch. If `None`, takes the last token.
<Tip>
One of `start_states` or `start_positions` should be not `None`. If both are set, `start_positions` overrides
`start_states`.
</Tip>
Returns:
`torch.FloatTensor`: The SQuAD 2.0 answer class.
"""
# No dependency on end_feature so that we can obtain one single `cls_logits` for each sample.
hsz = hidden_states.shape[-1]
assert (
start_states is not None or start_positions is not None
), "One of start_states, start_positions should be not None"
if start_positions is not None:
start_positions = start_positions[:, None, None].expand(-1, -1, hsz) # shape (bsz, 1, hsz)
start_states = hidden_states.gather(-2, start_positions).squeeze(-2) # shape (bsz, hsz)
if cls_index is not None:
cls_index = cls_index[:, None, None].expand(-1, -1, hsz) # shape (bsz, 1, hsz)
cls_token_state = hidden_states.gather(-2, cls_index).squeeze(-2) # shape (bsz, hsz)
else:
cls_token_state = hidden_states[:, -1, :] # shape (bsz, hsz)
x = self.dense_0(torch.cat([start_states, cls_token_state], dim=-1))
x = self.activation(x)
x = self.dense_1(x).squeeze(-1)
return x
@dataclass
class SquadHeadOutput(ModelOutput):
"""
Base class for outputs of question answering models using a [`~modeling_utils.SQuADHead`].
Args:
loss (`torch.FloatTensor` of shape `(1,)`, *optional*, returned if both `start_positions` and `end_positions` are provided):
Classification loss as the sum of start token, end token (and is_impossible if provided) classification
losses.
start_top_log_probs (`torch.FloatTensor` of shape `(batch_size, config.start_n_top)`, *optional*, returned if `start_positions` or `end_positions` is not provided):
Log probabilities for the top config.start_n_top start token possibilities (beam-search).
start_top_index (`torch.LongTensor` of shape `(batch_size, config.start_n_top)`, *optional*, returned if `start_positions` or `end_positions` is not provided):
Indices for the top config.start_n_top start token possibilities (beam-search).
end_top_log_probs (`torch.FloatTensor` of shape `(batch_size, config.start_n_top * config.end_n_top)`, *optional*, returned if `start_positions` or `end_positions` is not provided):
Log probabilities for the top `config.start_n_top * config.end_n_top` end token possibilities
(beam-search).
end_top_index (`torch.LongTensor` of shape `(batch_size, config.start_n_top * config.end_n_top)`, *optional*, returned if `start_positions` or `end_positions` is not provided):
Indices for the top `config.start_n_top * config.end_n_top` end token possibilities (beam-search).
cls_logits (`torch.FloatTensor` of shape `(batch_size,)`, *optional*, returned if `start_positions` or `end_positions` is not provided):
Log probabilities for the `is_impossible` label of the answers.
"""
loss: Optional[torch.FloatTensor] = None
start_top_log_probs: Optional[torch.FloatTensor] = None
start_top_index: Optional[torch.LongTensor] = None
end_top_log_probs: Optional[torch.FloatTensor] = None
end_top_index: Optional[torch.LongTensor] = None
cls_logits: Optional[torch.FloatTensor] = None
class SQuADHead(nn.Module):
r"""
A SQuAD head inspired by XLNet.
Args:
config ([`PretrainedConfig`]):
The config used by the model, will be used to grab the `hidden_size` of the model and the `layer_norm_eps`
to use.
"""
def __init__(self, config):
super().__init__()
self.start_n_top = config.start_n_top
self.end_n_top = config.end_n_top
self.start_logits = PoolerStartLogits(config)
self.end_logits = PoolerEndLogits(config)
self.answer_class = PoolerAnswerClass(config)
@replace_return_docstrings(output_type=SquadHeadOutput, config_class=PretrainedConfig)
def forward(
self,
hidden_states: torch.FloatTensor,
start_positions: Optional[torch.LongTensor] = None,
end_positions: Optional[torch.LongTensor] = None,
cls_index: Optional[torch.LongTensor] = None,
is_impossible: Optional[torch.LongTensor] = None,
p_mask: Optional[torch.FloatTensor] = None,
return_dict: bool = False,
) -> Union[SquadHeadOutput, Tuple[torch.FloatTensor]]:
"""
Args:
hidden_states (`torch.FloatTensor` of shape `(batch_size, seq_len, hidden_size)`):
Final hidden states of the model on the sequence tokens.
start_positions (`torch.LongTensor` of shape `(batch_size,)`, *optional*):
Positions of the first token for the labeled span.
end_positions (`torch.LongTensor` of shape `(batch_size,)`, *optional*):
Positions of the last token for the labeled span.
cls_index (`torch.LongTensor` of shape `(batch_size,)`, *optional*):
Position of the CLS token for each sentence in the batch. If `None`, takes the last token.
is_impossible (`torch.LongTensor` of shape `(batch_size,)`, *optional*):
Whether the question has a possible answer in the paragraph or not.
p_mask (`torch.FloatTensor` of shape `(batch_size, seq_len)`, *optional*):
Mask for tokens at invalid position, such as query and special symbols (PAD, SEP, CLS). 1.0 means token
should be masked.
return_dict (`bool`, *optional*, defaults to `False`):
Whether or not to return a [`~utils.ModelOutput`] instead of a plain tuple.
Returns:
"""
start_logits = self.start_logits(hidden_states, p_mask=p_mask)
if start_positions is not None and end_positions is not None:
# If we are on multi-GPU, let's remove the dimension added by batch splitting
for x in (start_positions, end_positions, cls_index, is_impossible):
if x is not None and x.dim() > 1:
x.squeeze_(-1)
# during training, compute the end logits based on the ground truth of the start position
end_logits = self.end_logits(hidden_states, start_positions=start_positions, p_mask=p_mask)
loss_fct = CrossEntropyLoss()
start_loss = loss_fct(start_logits, start_positions)
end_loss = loss_fct(end_logits, end_positions)
total_loss = (start_loss + end_loss) / 2
if cls_index is not None and is_impossible is not None:
# Predict answerability from the representation of CLS and START
cls_logits = self.answer_class(hidden_states, start_positions=start_positions, cls_index=cls_index)
loss_fct_cls = nn.BCEWithLogitsLoss()
cls_loss = loss_fct_cls(cls_logits, is_impossible)
# note(zhiliny): by default multiply the loss by 0.5 so that the scale is comparable to start_loss and end_loss
total_loss += cls_loss * 0.5
return SquadHeadOutput(loss=total_loss) if return_dict else (total_loss,)
else:
# during inference, compute the end logits based on beam search
bsz, slen, hsz = hidden_states.size()
start_log_probs = nn.functional.softmax(start_logits, dim=-1) # shape (bsz, slen)
start_top_log_probs, start_top_index = torch.topk(
start_log_probs, self.start_n_top, dim=-1
) # shape (bsz, start_n_top)
start_top_index_exp = start_top_index.unsqueeze(-1).expand(-1, -1, hsz) # shape (bsz, start_n_top, hsz)
start_states = torch.gather(hidden_states, -2, start_top_index_exp) # shape (bsz, start_n_top, hsz)
start_states = start_states.unsqueeze(1).expand(-1, slen, -1, -1) # shape (bsz, slen, start_n_top, hsz)
hidden_states_expanded = hidden_states.unsqueeze(2).expand_as(
start_states
) # shape (bsz, slen, start_n_top, hsz)
p_mask = p_mask.unsqueeze(-1) if p_mask is not None else None
end_logits = self.end_logits(hidden_states_expanded, start_states=start_states, p_mask=p_mask)
end_log_probs = nn.functional.softmax(end_logits, dim=1) # shape (bsz, slen, start_n_top)
end_top_log_probs, end_top_index = torch.topk(
end_log_probs, self.end_n_top, dim=1
) # shape (bsz, end_n_top, start_n_top)
end_top_log_probs = end_top_log_probs.view(-1, self.start_n_top * self.end_n_top)
end_top_index = end_top_index.view(-1, self.start_n_top * self.end_n_top)
start_states = torch.einsum("blh,bl->bh", hidden_states, start_log_probs)
cls_logits = self.answer_class(hidden_states, start_states=start_states, cls_index=cls_index)
if not return_dict:
return (start_top_log_probs, start_top_index, end_top_log_probs, end_top_index, cls_logits)
else:
return SquadHeadOutput(
start_top_log_probs=start_top_log_probs,
start_top_index=start_top_index,
end_top_log_probs=end_top_log_probs,
end_top_index=end_top_index,
cls_logits=cls_logits,
)
class SequenceSummary(nn.Module):
r"""
Compute a single vector summary of a sequence hidden states.
Args:
config ([`PretrainedConfig`]):
The config used by the model. Relevant arguments in the config class of the model are (refer to the actual
config class of your model for the default values it uses):
- **summary_type** (`str`) -- The method to use to make this summary. Accepted values are:
- `"last"` -- Take the last token hidden state (like XLNet)
- `"first"` -- Take the first token hidden state (like Bert)
- `"mean"` -- Take the mean of all tokens hidden states
- `"cls_index"` -- Supply a Tensor of classification token position (GPT/GPT-2)
- `"attn"` -- Not implemented now, use multi-head attention
- **summary_use_proj** (`bool`) -- Add a projection after the vector extraction.
- **summary_proj_to_labels** (`bool`) -- If `True`, the projection outputs to `config.num_labels` classes
(otherwise to `config.hidden_size`).
- **summary_activation** (`Optional[str]`) -- Set to `"tanh"` to add a tanh activation to the output,
another string or `None` will add no activation.
- **summary_first_dropout** (`float`) -- Optional dropout probability before the projection and activation.
- **summary_last_dropout** (`float`)-- Optional dropout probability after the projection and activation.
"""
def __init__(self, config: PretrainedConfig):
super().__init__()
self.summary_type = getattr(config, "summary_type", "last")
if self.summary_type == "attn":
# We should use a standard multi-head attention module with absolute positional embedding for that.
# Cf. https://github.com/zihangdai/xlnet/blob/master/modeling.py#L253-L276
# We can probably just use the multi-head attention module of PyTorch >=1.1.0
raise NotImplementedError
self.summary = Identity()
if hasattr(config, "summary_use_proj") and config.summary_use_proj:
if hasattr(config, "summary_proj_to_labels") and config.summary_proj_to_labels and config.num_labels > 0:
num_classes = config.num_labels
else:
num_classes = config.hidden_size
self.summary = nn.Linear(config.hidden_size, num_classes)
activation_string = getattr(config, "summary_activation", None)
self.activation: Callable = get_activation(activation_string) if activation_string else Identity()
self.first_dropout = Identity()
if hasattr(config, "summary_first_dropout") and config.summary_first_dropout > 0:
self.first_dropout = nn.Dropout(config.summary_first_dropout)
self.last_dropout = Identity()
if hasattr(config, "summary_last_dropout") and config.summary_last_dropout > 0:
self.last_dropout = nn.Dropout(config.summary_last_dropout)
def forward(
self, hidden_states: torch.FloatTensor, cls_index: Optional[torch.LongTensor] = None
) -> torch.FloatTensor:
"""
Compute a single vector summary of a sequence hidden states.
Args:
hidden_states (`torch.FloatTensor` of shape `[batch_size, seq_len, hidden_size]`):
The hidden states of the last layer.
cls_index (`torch.LongTensor` of shape `[batch_size]` or `[batch_size, ...]` where ... are optional leading dimensions of `hidden_states`, *optional*):
Used if `summary_type == "cls_index"` and takes the last token of the sequence as classification token.
Returns:
`torch.FloatTensor`: The summary of the sequence hidden states.
"""
if self.summary_type == "last":
output = hidden_states[:, -1]
elif self.summary_type == "first":
output = hidden_states[:, 0]
elif self.summary_type == "mean":
output = hidden_states.mean(dim=1)
elif self.summary_type == "cls_index":
if cls_index is None:
cls_index = torch.full_like(
hidden_states[..., :1, :],
hidden_states.shape[-2] - 1,
dtype=torch.long,
)
else:
cls_index = cls_index.unsqueeze(-1).unsqueeze(-1)
cls_index = cls_index.expand((-1,) * (cls_index.dim() - 1) + (hidden_states.size(-1),))
# shape of cls_index: (bsz, XX, 1, hidden_size) where XX are optional leading dim of hidden_states
output = hidden_states.gather(-2, cls_index).squeeze(-2) # shape (bsz, XX, hidden_size)
elif self.summary_type == "attn":
raise NotImplementedError
output = self.first_dropout(output)
output = self.summary(output)
output = self.activation(output)
output = self.last_dropout(output)
return output
def unwrap_model(model: nn.Module) -> nn.Module:
"""
Recursively unwraps a model from potential containers (as used in distributed training).
Args:
model (`torch.nn.Module`): The model to unwrap.
"""
# since there could be multiple levels of wrapping, unwrap recursively
if hasattr(model, "module"):
return unwrap_model(model.module)
else:
return model
def expand_device_map(device_map, param_names, start_prefix):
"""
Expand a device map to return the correspondance parameter name to device.
"""
new_device_map = {}
param_names = [p[len(start_prefix) :] for p in param_names if p.startswith(start_prefix)]
for module, device in device_map.items():
new_device_map.update(
{p: device for p in param_names if p == module or p.startswith(f"{module}.") or module == ""}
)
return new_device_map
def get_disk_only_shard_files(device_map, sharded_metadata, start_prefix):
"""
Returns the list of shard files containing only weights offloaded to disk.
"""
weight_map = {
p[len(start_prefix) :]: v for p, v in sharded_metadata["weight_map"].items() if p.startswith(start_prefix)
}
files_content = collections.defaultdict(list)
for weight_name, filename in weight_map.items():
while len(weight_name) > 0 and weight_name not in device_map:
weight_name = ".".join(weight_name.split(".")[:-1])
files_content[filename].append(device_map[weight_name])
return [fname for fname, devices in files_content.items() if set(devices) == {"disk"}]
| transformers/src/transformers/modeling_utils.py/0 | {
"file_path": "transformers/src/transformers/modeling_utils.py",
"repo_id": "transformers",
"token_count": 101876
} | 307 |
# coding=utf-8
# Copyright 2022 WenXiang ZhongzhiCheng LedellWu LiuGuang BoWenZhang and The HuggingFace Inc. team. All rights reserved.
#
# Licensed under the Apache License, Version 2.0 (the "License");
# you may not use this file except in compliance with the License.
# You may obtain a copy of the License at
#
# http://www.apache.org/licenses/LICENSE-2.0
#
# Unless required by applicable law or agreed to in writing, software
# distributed under the License is distributed on an "AS IS" BASIS,
# WITHOUT WARRANTIES OR CONDITIONS OF ANY KIND, either express or implied.
# See the License for the specific language governing permissions and
# limitations under the License.
""" AltCLIP model configuration"""
import os
from typing import Union
from ...configuration_utils import PretrainedConfig
from ...utils import logging
logger = logging.get_logger(__name__)
ALTCLIP_PRETRAINED_CONFIG_ARCHIVE_MAP = {
"BAAI/AltCLIP": "https://huggingface.co/BAAI/AltCLIP/resolve/main/config.json",
# See all AltCLIP models at https://huggingface.co/models?filter=altclip
}
class AltCLIPTextConfig(PretrainedConfig):
r"""
This is the configuration class to store the configuration of a [`AltCLIPTextModel`]. It is used to instantiate a
AltCLIP text model according to the specified arguments, defining the model architecture. Instantiating a
configuration with the defaults will yield a similar configuration to that of the AltCLIP
[BAAI/AltCLIP](https://huggingface.co/BAAI/AltCLIP) architecture.
Configuration objects inherit from [`PretrainedConfig`] and can be used to control the model outputs. Read the
documentation from [`PretrainedConfig`] for more information.
Args:
vocab_size (`int`, *optional*, defaults to 250002):
Vocabulary size of the AltCLIP model. Defines the number of different tokens that can be represented by the
`inputs_ids` passed when calling [`AltCLIPTextModel`].
hidden_size (`int`, *optional*, defaults to 1024):
Dimensionality of the encoder layers and the pooler layer.
num_hidden_layers (`int`, *optional*, defaults to 24):
Number of hidden layers in the Transformer encoder.
num_attention_heads (`int`, *optional*, defaults to 16):
Number of attention heads for each attention layer in the Transformer encoder.
intermediate_size (`int`, *optional*, defaults to 4096):
Dimensionality of the "intermediate" (often named feed-forward) layer in the Transformer encoder.
hidden_act (`str` or `Callable`, *optional*, defaults to `"gelu"`):
The non-linear activation function (function or string) in the encoder and pooler. If string, `"gelu"`,
`"relu"`, `"silu"` and `"gelu_new"` are supported.
hidden_dropout_prob (`float`, *optional*, defaults to 0.1):
The dropout probability for all fully connected layers in the embeddings, encoder, and pooler.
attention_probs_dropout_prob (`float`, *optional*, defaults to 0.1):
The dropout ratio for the attention probabilities.
max_position_embeddings (`int`, *optional*, defaults to 514):
The maximum sequence length that this model might ever be used with. Typically set this to something large
just in case (e.g., 512 or 1024 or 2048).
type_vocab_size (`int`, *optional*, defaults to 1):
The vocabulary size of the `token_type_ids` passed when calling [`AltCLIPTextModel`]
initializer_range (`float`, *optional*, defaults to 0.02):
The standard deviation of the truncated_normal_initializer for initializing all weight matrices.
initializer_factor (`float`, *optional*, defaults to 0.02):
A factor for initializing all weight matrices (should be kept to 1, used internally for initialization
testing).
layer_norm_eps (`float`, *optional*, defaults to 1e-05):
The epsilon used by the layer normalization layers.
pad_token_id (`int`, *optional*, defaults to 1): The id of the *padding* token.
bos_token_id (`int`, *optional*, defaults to 0): The id of the *beginning-of-sequence* token.
eos_token_id (`Union[int, List[int]]`, *optional*, defaults to 2):
The id of the *end-of-sequence* token. Optionally, use a list to set multiple *end-of-sequence* tokens.
position_embedding_type (`str`, *optional*, defaults to `"absolute"`):
Type of position embedding. Choose one of `"absolute"`, `"relative_key"`, `"relative_key_query"`. For
positional embeddings use `"absolute"`. For more information on `"relative_key"`, please refer to
[Self-Attention with Relative Position Representations (Shaw et al.)](https://arxiv.org/abs/1803.02155).
For more information on `"relative_key_query"`, please refer to *Method 4* in [Improve Transformer Models
with Better Relative Position Embeddings (Huang et al.)](https://arxiv.org/abs/2009.13658).
use_cache (`bool`, *optional*, defaults to `True`):
Whether or not the model should return the last key/values attentions (not used by all models). Only
relevant if `config.is_decoder=True`.
project_dim (`int`, *optional*, defaults to 768):
The dimentions of the teacher model before the mapping layer.
Examples:
```python
>>> from transformers import AltCLIPTextModel, AltCLIPTextConfig
>>> # Initializing a AltCLIPTextConfig with BAAI/AltCLIP style configuration
>>> configuration = AltCLIPTextConfig()
>>> # Initializing a AltCLIPTextModel (with random weights) from the BAAI/AltCLIP style configuration
>>> model = AltCLIPTextModel(configuration)
>>> # Accessing the model configuration
>>> configuration = model.config
```"""
model_type = "altclip_text_model"
def __init__(
self,
vocab_size=250002,
hidden_size=1024,
num_hidden_layers=24,
num_attention_heads=16,
intermediate_size=4096,
hidden_act="gelu",
hidden_dropout_prob=0.1,
attention_probs_dropout_prob=0.1,
max_position_embeddings=514,
type_vocab_size=1,
initializer_range=0.02,
initializer_factor=0.02,
layer_norm_eps=1e-05,
pad_token_id=1,
bos_token_id=0,
eos_token_id=2,
position_embedding_type="absolute",
use_cache=True,
project_dim=768,
**kwargs,
):
super().__init__(pad_token_id=pad_token_id, bos_token_id=bos_token_id, eos_token_id=eos_token_id, **kwargs)
self.vocab_size = vocab_size
self.hidden_size = hidden_size
self.num_hidden_layers = num_hidden_layers
self.num_attention_heads = num_attention_heads
self.hidden_act = hidden_act
self.intermediate_size = intermediate_size
self.hidden_dropout_prob = hidden_dropout_prob
self.attention_probs_dropout_prob = attention_probs_dropout_prob
self.max_position_embeddings = max_position_embeddings
self.type_vocab_size = type_vocab_size
self.initializer_range = initializer_range
self.initializer_factor = initializer_factor
self.layer_norm_eps = layer_norm_eps
self.position_embedding_type = position_embedding_type
self.use_cache = use_cache
self.project_dim = project_dim
class AltCLIPVisionConfig(PretrainedConfig):
r"""
This is the configuration class to store the configuration of a [`AltCLIPModel`]. It is used to instantiate an
AltCLIP model according to the specified arguments, defining the model architecture. Instantiating a configuration
with the defaults will yield a similar configuration to that of the AltCLIP
[BAAI/AltCLIP](https://huggingface.co/BAAI/AltCLIP) architecture.
Configuration objects inherit from [`PretrainedConfig`] and can be used to control the model outputs. Read the
documentation from [`PretrainedConfig`] for more information.
Args:
hidden_size (`int`, *optional*, defaults to 768):
Dimensionality of the encoder layers and the pooler layer.
intermediate_size (`int`, *optional*, defaults to 3072):
Dimensionality of the "intermediate" (i.e., feed-forward) layer in the Transformer encoder.
projection_dim (`int`, *optional*, defaults to 512):
Dimentionality of text and vision projection layers.
num_hidden_layers (`int`, *optional*, defaults to 12):
Number of hidden layers in the Transformer encoder.
num_attention_heads (`int`, *optional*, defaults to 12):
Number of attention heads for each attention layer in the Transformer encoder.
num_channels (`int`, *optional*, defaults to 3):
The number of input channels.
image_size (`int`, *optional*, defaults to 224):
The size (resolution) of each image.
patch_size (`int`, *optional*, defaults to 32):
The size (resolution) of each patch.
hidden_act (`str` or `function`, *optional*, defaults to `"quick_gelu"`):
The non-linear activation function (function or string) in the encoder and pooler. If string, `"gelu"`,
`"relu"`, `"selu"` and `"gelu_new"` ``"quick_gelu"` are supported.
layer_norm_eps (`float`, *optional*, defaults to 1e-05):
The epsilon used by the layer normalization layers.
attention_dropout (`float`, *optional*, defaults to 0.0):
The dropout ratio for the attention probabilities.
initializer_range (`float`, *optional*, defaults to 0.02):
The standard deviation of the truncated_normal_initializer for initializing all weight matrices.
initializer_factor (`float`, *optional*, defaults to 1.0):
A factor for initializing all weight matrices (should be kept to 1, used internally for initialization
testing).
Example:
```python
>>> from transformers import AltCLIPVisionConfig, AltCLIPVisionModel
>>> # Initializing a AltCLIPVisionConfig with BAAI/AltCLIP style configuration
>>> configuration = AltCLIPVisionConfig()
>>> # Initializing a AltCLIPVisionModel (with random weights) from the BAAI/AltCLIP style configuration
>>> model = AltCLIPVisionModel(configuration)
>>> # Accessing the model configuration
>>> configuration = model.config
```"""
model_type = "altclip_vision_model"
def __init__(
self,
hidden_size=768,
intermediate_size=3072,
projection_dim=512,
num_hidden_layers=12,
num_attention_heads=12,
num_channels=3,
image_size=224,
patch_size=32,
hidden_act="quick_gelu",
layer_norm_eps=1e-5,
attention_dropout=0.0,
initializer_range=0.02,
initializer_factor=1.0,
**kwargs,
):
super().__init__(**kwargs)
self.hidden_size = hidden_size
self.intermediate_size = intermediate_size
self.projection_dim = projection_dim
self.num_hidden_layers = num_hidden_layers
self.num_attention_heads = num_attention_heads
self.num_channels = num_channels
self.patch_size = patch_size
self.image_size = image_size
self.initializer_range = initializer_range
self.initializer_factor = initializer_factor
self.attention_dropout = attention_dropout
self.layer_norm_eps = layer_norm_eps
self.hidden_act = hidden_act
@classmethod
def from_pretrained(cls, pretrained_model_name_or_path: Union[str, os.PathLike], **kwargs) -> "PretrainedConfig":
cls._set_token_in_kwargs(kwargs)
config_dict, kwargs = cls.get_config_dict(pretrained_model_name_or_path, **kwargs)
# get the vision config dict if we are loading from AltCLIPConfig
if config_dict.get("model_type") == "altclip":
config_dict = config_dict["vision_config"]
if "model_type" in config_dict and hasattr(cls, "model_type") and config_dict["model_type"] != cls.model_type:
logger.warning(
f"You are using a model of type {config_dict['model_type']} to instantiate a model of type "
f"{cls.model_type}. This is not supported for all configurations of models and can yield errors."
)
return cls.from_dict(config_dict, **kwargs)
class AltCLIPConfig(PretrainedConfig):
r"""
This is the configuration class to store the configuration of a [`AltCLIPModel`]. It is used to instantiate an
AltCLIP model according to the specified arguments, defining the model architecture. Instantiating a configuration
with the defaults will yield a similar configuration to that of the AltCLIP
[BAAI/AltCLIP](https://huggingface.co/BAAI/AltCLIP) architecture.
Configuration objects inherit from [`PretrainedConfig`] and can be used to control the model outputs. Read the
documentation from [`PretrainedConfig`] for more information.
Args:
text_config (`dict`, *optional*):
Dictionary of configuration options used to initialize [`AltCLIPTextConfig`].
vision_config (`dict`, *optional*):
Dictionary of configuration options used to initialize [`AltCLIPVisionConfig`].
projection_dim (`int`, *optional*, defaults to 768):
Dimentionality of text and vision projection layers.
logit_scale_init_value (`float`, *optional*, defaults to 2.6592):
The inital value of the *logit_scale* paramter. Default is used as per the original CLIP implementation.
kwargs (*optional*):
Dictionary of keyword arguments.
Example:
```python
>>> from transformers import AltCLIPConfig, AltCLIPModel
>>> # Initializing a AltCLIPConfig with BAAI/AltCLIP style configuration
>>> configuration = AltCLIPConfig()
>>> # Initializing a AltCLIPModel (with random weights) from the BAAI/AltCLIP style configuration
>>> model = AltCLIPModel(configuration)
>>> # Accessing the model configuration
>>> configuration = model.config
>>> # We can also initialize a AltCLIPConfig from a AltCLIPTextConfig and a AltCLIPVisionConfig
>>> # Initializing a AltCLIPText and AltCLIPVision configuration
>>> config_text = AltCLIPTextConfig()
>>> config_vision = AltCLIPVisionConfig()
>>> config = AltCLIPConfig.from_text_vision_configs(config_text, config_vision)
```"""
model_type = "altclip"
def __init__(
self, text_config=None, vision_config=None, projection_dim=768, logit_scale_init_value=2.6592, **kwargs
):
# If `_config_dict` exist, we use them for the backward compatibility.
# We pop out these 2 attributes before calling `super().__init__` to avoid them being saved (which causes a lot
# of confusion!).
text_config_dict = kwargs.pop("text_config_dict", None)
vision_config_dict = kwargs.pop("vision_config_dict", None)
super().__init__(**kwargs)
# Instead of simply assigning `[text|vision]_config_dict` to `[text|vision]_config`, we use the values in
# `[text|vision]_config_dict` to update the values in `[text|vision]_config`. The values should be same in most
# cases, but we don't want to break anything regarding `_config_dict` that existed before commit `8827e1b2`.
if text_config_dict is not None:
if text_config is None:
text_config = {}
# This is the complete result when using `text_config_dict`.
_text_config_dict = AltCLIPTextConfig(**text_config_dict).to_dict()
# Give a warning if the values exist in both `_text_config_dict` and `text_config` but being different.
for key, value in _text_config_dict.items():
if key in text_config and value != text_config[key] and key not in ["transformers_version"]:
# If specified in `text_config_dict`
if key in text_config_dict:
message = (
f"`{key}` is found in both `text_config_dict` and `text_config` but with different values. "
f'The value `text_config_dict["{key}"]` will be used instead.'
)
# If inferred from default argument values (just to be super careful)
else:
message = (
f"`text_config_dict` is provided which will be used to initialize `AltCLIPTextConfig`. The "
f'value `text_config["{key}"]` will be overriden.'
)
logger.info(message)
# Update all values in `text_config` with the ones in `_text_config_dict`.
text_config.update(_text_config_dict)
if vision_config_dict is not None:
if vision_config is None:
vision_config = {}
# This is the complete result when using `vision_config_dict`.
_vision_config_dict = AltCLIPVisionConfig(**vision_config_dict).to_dict()
# convert keys to string instead of integer
if "id2label" in _vision_config_dict:
_vision_config_dict["id2label"] = {
str(key): value for key, value in _vision_config_dict["id2label"].items()
}
# Give a warning if the values exist in both `_vision_config_dict` and `vision_config` but being different.
for key, value in _vision_config_dict.items():
if key in vision_config and value != vision_config[key] and key not in ["transformers_version"]:
# If specified in `vision_config_dict`
if key in vision_config_dict:
message = (
f"`{key}` is found in both `vision_config_dict` and `vision_config` but with different "
f'values. The value `vision_config_dict["{key}"]` will be used instead.'
)
# If inferred from default argument values (just to be super careful)
else:
message = (
f"`vision_config_dict` is provided which will be used to initialize `AltCLIPVisionConfig`. "
f'The value `vision_config["{key}"]` will be overriden.'
)
logger.info(message)
# Update all values in `vision_config` with the ones in `_vision_config_dict`.
vision_config.update(_vision_config_dict)
if text_config is None:
text_config = {}
logger.info("`text_config` is `None`. Initializing the `AltCLIPTextConfig` with default values.")
if vision_config is None:
vision_config = {}
logger.info("`vision_config` is `None`. initializing the `AltCLIPVisionConfig` with default values.")
self.text_config = AltCLIPTextConfig(**text_config)
self.vision_config = AltCLIPVisionConfig(**vision_config)
self.projection_dim = projection_dim
self.logit_scale_init_value = logit_scale_init_value
self.initializer_factor = 1.0
@classmethod
def from_text_vision_configs(cls, text_config: AltCLIPTextConfig, vision_config: AltCLIPVisionConfig, **kwargs):
r"""
Instantiate a [`AltCLIPConfig`] (or a derived class) from altclip text model configuration and altclip vision
model configuration.
Returns:
[`AltCLIPConfig`]: An instance of a configuration object
"""
return cls(text_config=text_config.to_dict(), vision_config=vision_config.to_dict(), **kwargs)
| transformers/src/transformers/models/altclip/configuration_altclip.py/0 | {
"file_path": "transformers/src/transformers/models/altclip/configuration_altclip.py",
"repo_id": "transformers",
"token_count": 7757
} | 308 |
# coding=utf-8
# Copyright 2021 The HuggingFace Inc. team.
#
# Licensed under the Apache License, Version 2.0 (the "License");
# you may not use this file except in compliance with the License.
# You may obtain a copy of the License at
#
# http://www.apache.org/licenses/LICENSE-2.0
#
# Unless required by applicable law or agreed to in writing, software
# distributed under the License is distributed on an "AS IS" BASIS,
# WITHOUT WARRANTIES OR CONDITIONS OF ANY KIND, either express or implied.
# See the License for the specific language governing permissions and
# limitations under the License.
""" AutoProcessor class."""
import importlib
import inspect
import json
import os
import warnings
from collections import OrderedDict
# Build the list of all feature extractors
from ...configuration_utils import PretrainedConfig
from ...dynamic_module_utils import get_class_from_dynamic_module, resolve_trust_remote_code
from ...feature_extraction_utils import FeatureExtractionMixin
from ...image_processing_utils import ImageProcessingMixin
from ...processing_utils import ProcessorMixin
from ...tokenization_utils import TOKENIZER_CONFIG_FILE
from ...utils import FEATURE_EXTRACTOR_NAME, PROCESSOR_NAME, get_file_from_repo, logging
from .auto_factory import _LazyAutoMapping
from .configuration_auto import (
CONFIG_MAPPING_NAMES,
AutoConfig,
model_type_to_module_name,
replace_list_option_in_docstrings,
)
from .feature_extraction_auto import AutoFeatureExtractor
from .image_processing_auto import AutoImageProcessor
from .tokenization_auto import AutoTokenizer
logger = logging.get_logger(__name__)
PROCESSOR_MAPPING_NAMES = OrderedDict(
[
("align", "AlignProcessor"),
("altclip", "AltCLIPProcessor"),
("bark", "BarkProcessor"),
("blip", "BlipProcessor"),
("blip-2", "Blip2Processor"),
("bridgetower", "BridgeTowerProcessor"),
("chinese_clip", "ChineseCLIPProcessor"),
("clap", "ClapProcessor"),
("clip", "CLIPProcessor"),
("clipseg", "CLIPSegProcessor"),
("clvp", "ClvpProcessor"),
("flava", "FlavaProcessor"),
("fuyu", "FuyuProcessor"),
("git", "GitProcessor"),
("groupvit", "CLIPProcessor"),
("hubert", "Wav2Vec2Processor"),
("idefics", "IdeficsProcessor"),
("instructblip", "InstructBlipProcessor"),
("kosmos-2", "Kosmos2Processor"),
("layoutlmv2", "LayoutLMv2Processor"),
("layoutlmv3", "LayoutLMv3Processor"),
("llava", "LlavaProcessor"),
("markuplm", "MarkupLMProcessor"),
("mctct", "MCTCTProcessor"),
("mgp-str", "MgpstrProcessor"),
("oneformer", "OneFormerProcessor"),
("owlv2", "Owlv2Processor"),
("owlvit", "OwlViTProcessor"),
("pix2struct", "Pix2StructProcessor"),
("pop2piano", "Pop2PianoProcessor"),
("sam", "SamProcessor"),
("seamless_m4t", "SeamlessM4TProcessor"),
("sew", "Wav2Vec2Processor"),
("sew-d", "Wav2Vec2Processor"),
("siglip", "SiglipProcessor"),
("speech_to_text", "Speech2TextProcessor"),
("speech_to_text_2", "Speech2Text2Processor"),
("speecht5", "SpeechT5Processor"),
("trocr", "TrOCRProcessor"),
("tvlt", "TvltProcessor"),
("tvp", "TvpProcessor"),
("unispeech", "Wav2Vec2Processor"),
("unispeech-sat", "Wav2Vec2Processor"),
("vilt", "ViltProcessor"),
("vipllava", "LlavaProcessor"),
("vision-text-dual-encoder", "VisionTextDualEncoderProcessor"),
("wav2vec2", "Wav2Vec2Processor"),
("wav2vec2-bert", "Wav2Vec2Processor"),
("wav2vec2-conformer", "Wav2Vec2Processor"),
("wavlm", "Wav2Vec2Processor"),
("whisper", "WhisperProcessor"),
("xclip", "XCLIPProcessor"),
]
)
PROCESSOR_MAPPING = _LazyAutoMapping(CONFIG_MAPPING_NAMES, PROCESSOR_MAPPING_NAMES)
def processor_class_from_name(class_name: str):
for module_name, processors in PROCESSOR_MAPPING_NAMES.items():
if class_name in processors:
module_name = model_type_to_module_name(module_name)
module = importlib.import_module(f".{module_name}", "transformers.models")
try:
return getattr(module, class_name)
except AttributeError:
continue
for processor in PROCESSOR_MAPPING._extra_content.values():
if getattr(processor, "__name__", None) == class_name:
return processor
# We did not fine the class, but maybe it's because a dep is missing. In that case, the class will be in the main
# init and we return the proper dummy to get an appropriate error message.
main_module = importlib.import_module("transformers")
if hasattr(main_module, class_name):
return getattr(main_module, class_name)
return None
class AutoProcessor:
r"""
This is a generic processor class that will be instantiated as one of the processor classes of the library when
created with the [`AutoProcessor.from_pretrained`] class method.
This class cannot be instantiated directly using `__init__()` (throws an error).
"""
def __init__(self):
raise EnvironmentError(
"AutoProcessor is designed to be instantiated "
"using the `AutoProcessor.from_pretrained(pretrained_model_name_or_path)` method."
)
@classmethod
@replace_list_option_in_docstrings(PROCESSOR_MAPPING_NAMES)
def from_pretrained(cls, pretrained_model_name_or_path, **kwargs):
r"""
Instantiate one of the processor classes of the library from a pretrained model vocabulary.
The processor class to instantiate is selected based on the `model_type` property of the config object (either
passed as an argument or loaded from `pretrained_model_name_or_path` if possible):
List options
Params:
pretrained_model_name_or_path (`str` or `os.PathLike`):
This can be either:
- a string, the *model id* of a pretrained feature_extractor hosted inside a model repo on
huggingface.co. Valid model ids can be located at the root-level, like `bert-base-uncased`, or
namespaced under a user or organization name, like `dbmdz/bert-base-german-cased`.
- a path to a *directory* containing a processor files saved using the `save_pretrained()` method,
e.g., `./my_model_directory/`.
cache_dir (`str` or `os.PathLike`, *optional*):
Path to a directory in which a downloaded pretrained model feature extractor should be cached if the
standard cache should not be used.
force_download (`bool`, *optional*, defaults to `False`):
Whether or not to force to (re-)download the feature extractor files and override the cached versions
if they exist.
resume_download (`bool`, *optional*, defaults to `False`):
Whether or not to delete incompletely received file. Attempts to resume the download if such a file
exists.
proxies (`Dict[str, str]`, *optional*):
A dictionary of proxy servers to use by protocol or endpoint, e.g., `{'http': 'foo.bar:3128',
'http://hostname': 'foo.bar:4012'}.` The proxies are used on each request.
token (`str` or *bool*, *optional*):
The token to use as HTTP bearer authorization for remote files. If `True`, will use the token generated
when running `huggingface-cli login` (stored in `~/.huggingface`).
revision (`str`, *optional*, defaults to `"main"`):
The specific model version to use. It can be a branch name, a tag name, or a commit id, since we use a
git-based system for storing models and other artifacts on huggingface.co, so `revision` can be any
identifier allowed by git.
return_unused_kwargs (`bool`, *optional*, defaults to `False`):
If `False`, then this function returns just the final feature extractor object. If `True`, then this
functions returns a `Tuple(feature_extractor, unused_kwargs)` where *unused_kwargs* is a dictionary
consisting of the key/value pairs whose keys are not feature extractor attributes: i.e., the part of
`kwargs` which has not been used to update `feature_extractor` and is otherwise ignored.
trust_remote_code (`bool`, *optional*, defaults to `False`):
Whether or not to allow for custom models defined on the Hub in their own modeling files. This option
should only be set to `True` for repositories you trust and in which you have read the code, as it will
execute code present on the Hub on your local machine.
kwargs (`Dict[str, Any]`, *optional*):
The values in kwargs of any keys which are feature extractor attributes will be used to override the
loaded values. Behavior concerning key/value pairs whose keys are *not* feature extractor attributes is
controlled by the `return_unused_kwargs` keyword parameter.
<Tip>
Passing `token=True` is required when you want to use a private model.
</Tip>
Examples:
```python
>>> from transformers import AutoProcessor
>>> # Download processor from huggingface.co and cache.
>>> processor = AutoProcessor.from_pretrained("facebook/wav2vec2-base-960h")
>>> # If processor files are in a directory (e.g. processor was saved using *save_pretrained('./test/saved_model/')*)
>>> # processor = AutoProcessor.from_pretrained("./test/saved_model/")
```"""
use_auth_token = kwargs.pop("use_auth_token", None)
if use_auth_token is not None:
warnings.warn(
"The `use_auth_token` argument is deprecated and will be removed in v5 of Transformers. Please use `token` instead.",
FutureWarning,
)
if kwargs.get("token", None) is not None:
raise ValueError(
"`token` and `use_auth_token` are both specified. Please set only the argument `token`."
)
kwargs["token"] = use_auth_token
config = kwargs.pop("config", None)
trust_remote_code = kwargs.pop("trust_remote_code", None)
kwargs["_from_auto"] = True
processor_class = None
processor_auto_map = None
# First, let's see if we have a processor or preprocessor config.
# Filter the kwargs for `get_file_from_repo`.
get_file_from_repo_kwargs = {
key: kwargs[key] for key in inspect.signature(get_file_from_repo).parameters.keys() if key in kwargs
}
# Let's start by checking whether the processor class is saved in a processor config
processor_config_file = get_file_from_repo(
pretrained_model_name_or_path, PROCESSOR_NAME, **get_file_from_repo_kwargs
)
if processor_config_file is not None:
config_dict, _ = ProcessorMixin.get_processor_dict(pretrained_model_name_or_path, **kwargs)
processor_class = config_dict.get("processor_class", None)
if "AutoProcessor" in config_dict.get("auto_map", {}):
processor_auto_map = config_dict["auto_map"]["AutoProcessor"]
if processor_class is None:
# If not found, let's check whether the processor class is saved in an image processor config
preprocessor_config_file = get_file_from_repo(
pretrained_model_name_or_path, FEATURE_EXTRACTOR_NAME, **get_file_from_repo_kwargs
)
if preprocessor_config_file is not None:
config_dict, _ = ImageProcessingMixin.get_image_processor_dict(pretrained_model_name_or_path, **kwargs)
processor_class = config_dict.get("processor_class", None)
if "AutoProcessor" in config_dict.get("auto_map", {}):
processor_auto_map = config_dict["auto_map"]["AutoProcessor"]
# If not found, let's check whether the processor class is saved in a feature extractor config
if preprocessor_config_file is not None and processor_class is None:
config_dict, _ = FeatureExtractionMixin.get_feature_extractor_dict(
pretrained_model_name_or_path, **kwargs
)
processor_class = config_dict.get("processor_class", None)
if "AutoProcessor" in config_dict.get("auto_map", {}):
processor_auto_map = config_dict["auto_map"]["AutoProcessor"]
if processor_class is None:
# Next, let's check whether the processor class is saved in a tokenizer
tokenizer_config_file = get_file_from_repo(
pretrained_model_name_or_path, TOKENIZER_CONFIG_FILE, **get_file_from_repo_kwargs
)
if tokenizer_config_file is not None:
with open(tokenizer_config_file, encoding="utf-8") as reader:
config_dict = json.load(reader)
processor_class = config_dict.get("processor_class", None)
if "AutoProcessor" in config_dict.get("auto_map", {}):
processor_auto_map = config_dict["auto_map"]["AutoProcessor"]
if processor_class is None:
# Otherwise, load config, if it can be loaded.
if not isinstance(config, PretrainedConfig):
config = AutoConfig.from_pretrained(
pretrained_model_name_or_path, trust_remote_code=trust_remote_code, **kwargs
)
# And check if the config contains the processor class.
processor_class = getattr(config, "processor_class", None)
if hasattr(config, "auto_map") and "AutoProcessor" in config.auto_map:
processor_auto_map = config.auto_map["AutoProcessor"]
if processor_class is not None:
processor_class = processor_class_from_name(processor_class)
has_remote_code = processor_auto_map is not None
has_local_code = processor_class is not None or type(config) in PROCESSOR_MAPPING
trust_remote_code = resolve_trust_remote_code(
trust_remote_code, pretrained_model_name_or_path, has_local_code, has_remote_code
)
if has_remote_code and trust_remote_code:
processor_class = get_class_from_dynamic_module(
processor_auto_map, pretrained_model_name_or_path, **kwargs
)
_ = kwargs.pop("code_revision", None)
if os.path.isdir(pretrained_model_name_or_path):
processor_class.register_for_auto_class()
return processor_class.from_pretrained(
pretrained_model_name_or_path, trust_remote_code=trust_remote_code, **kwargs
)
elif processor_class is not None:
return processor_class.from_pretrained(
pretrained_model_name_or_path, trust_remote_code=trust_remote_code, **kwargs
)
# Last try: we use the PROCESSOR_MAPPING.
elif type(config) in PROCESSOR_MAPPING:
return PROCESSOR_MAPPING[type(config)].from_pretrained(pretrained_model_name_or_path, **kwargs)
# At this stage, there doesn't seem to be a `Processor` class available for this model, so let's try a
# tokenizer.
try:
return AutoTokenizer.from_pretrained(
pretrained_model_name_or_path, trust_remote_code=trust_remote_code, **kwargs
)
except Exception:
try:
return AutoImageProcessor.from_pretrained(
pretrained_model_name_or_path, trust_remote_code=trust_remote_code, **kwargs
)
except Exception:
pass
try:
return AutoFeatureExtractor.from_pretrained(
pretrained_model_name_or_path, trust_remote_code=trust_remote_code, **kwargs
)
except Exception:
pass
raise ValueError(
f"Unrecognized processing class in {pretrained_model_name_or_path}. Can't instantiate a processor, a "
"tokenizer, an image processor or a feature extractor for this model. Make sure the repository contains "
"the files of at least one of those processing classes."
)
@staticmethod
def register(config_class, processor_class, exist_ok=False):
"""
Register a new processor for this class.
Args:
config_class ([`PretrainedConfig`]):
The configuration corresponding to the model to register.
processor_class ([`FeatureExtractorMixin`]): The processor to register.
"""
PROCESSOR_MAPPING.register(config_class, processor_class, exist_ok=exist_ok)
| transformers/src/transformers/models/auto/processing_auto.py/0 | {
"file_path": "transformers/src/transformers/models/auto/processing_auto.py",
"repo_id": "transformers",
"token_count": 7135
} | 309 |
# coding=utf-8
# Copyright 2021 The Fairseq Authors and The HuggingFace Inc. team. All rights reserved.
#
# Licensed under the Apache License, Version 2.0 (the "License");
# you may not use this file except in compliance with the License.
# You may obtain a copy of the License at
#
# http://www.apache.org/licenses/LICENSE-2.0
#
# Unless required by applicable law or agreed to in writing, software
# distributed under the License is distributed on an "AS IS" BASIS,
# WITHOUT WARRANTIES OR CONDITIONS OF ANY KIND, either express or implied.
# See the License for the specific language governing permissions and
# limitations under the License.
""" TF 2.0 Bart model."""
from __future__ import annotations
import random
from typing import Optional, Tuple, Union
import numpy as np
import tensorflow as tf
from ...activations_tf import get_tf_activation
from ...modeling_tf_outputs import (
TFBaseModelOutput,
TFBaseModelOutputWithPastAndCrossAttentions,
TFSeq2SeqLMOutput,
TFSeq2SeqModelOutput,
TFSeq2SeqSequenceClassifierOutput,
)
# Public API
from ...modeling_tf_utils import (
TFCausalLanguageModelingLoss,
TFModelInputType,
TFPreTrainedModel,
TFSequenceClassificationLoss,
keras,
keras_serializable,
unpack_inputs,
)
from ...tf_utils import check_embeddings_within_bounds, shape_list, stable_softmax
from ...utils import (
add_code_sample_docstrings,
add_end_docstrings,
add_start_docstrings,
add_start_docstrings_to_model_forward,
logging,
replace_return_docstrings,
)
from .configuration_bart import BartConfig
logger = logging.get_logger(__name__)
_CHECKPOINT_FOR_DOC = "facebook/bart-large"
_CONFIG_FOR_DOC = "BartConfig"
LARGE_NEGATIVE = -1e8
def shift_tokens_right(input_ids: tf.Tensor, pad_token_id: int, decoder_start_token_id: int):
pad_token_id = tf.cast(pad_token_id, input_ids.dtype)
decoder_start_token_id = tf.cast(decoder_start_token_id, input_ids.dtype)
start_tokens = tf.fill(
(shape_list(input_ids)[0], 1), tf.convert_to_tensor(decoder_start_token_id, input_ids.dtype)
)
shifted_input_ids = tf.concat([start_tokens, input_ids[:, :-1]], -1)
# replace possible -100 values in labels by `pad_token_id`
shifted_input_ids = tf.where(
shifted_input_ids == -100,
tf.fill(shape_list(shifted_input_ids), tf.convert_to_tensor(pad_token_id, input_ids.dtype)),
shifted_input_ids,
)
# "Verify that `labels` has only positive values and -100"
assert_gte0 = tf.debugging.assert_greater_equal(shifted_input_ids, tf.constant(0, dtype=input_ids.dtype))
# Make sure the assertion op is called by wrapping the result in an identity no-op
with tf.control_dependencies([assert_gte0]):
shifted_input_ids = tf.identity(shifted_input_ids)
return shifted_input_ids
def _make_causal_mask(input_ids_shape: tf.TensorShape, past_key_values_length: int = 0):
"""
Make causal mask used for bi-directional self-attention.
"""
bsz = input_ids_shape[0]
tgt_len = input_ids_shape[1]
mask = tf.ones((tgt_len, tgt_len)) * LARGE_NEGATIVE
mask_cond = tf.range(shape_list(mask)[-1])
mask = tf.where(mask_cond < tf.reshape(mask_cond + 1, (shape_list(mask)[-1], 1)), 0.0, mask)
if past_key_values_length > 0:
mask = tf.concat([tf.zeros((tgt_len, past_key_values_length)), mask], axis=-1)
return tf.tile(mask[None, None, :, :], (bsz, 1, 1, 1))
def _expand_mask(mask: tf.Tensor, tgt_len: Optional[int] = None):
"""
Expands attention_mask from `[bsz, seq_len]` to `[bsz, 1, tgt_seq_len, src_seq_len]`.
"""
src_len = shape_list(mask)[1]
tgt_len = tgt_len if tgt_len is not None else src_len
one_cst = tf.constant(1.0)
mask = tf.cast(mask, dtype=one_cst.dtype)
expanded_mask = tf.tile(mask[:, None, None, :], (1, 1, tgt_len, 1))
return (one_cst - expanded_mask) * LARGE_NEGATIVE
class TFBartLearnedPositionalEmbedding(keras.layers.Embedding):
"""
This module learns positional embeddings up to a fixed maximum size.
"""
def __init__(self, num_embeddings: int, embedding_dim: int, **kwargs):
# Bart is set up so that if padding_idx is specified then offset the embedding ids by 2
# and adjust num_embeddings appropriately. Other models don't have this hack
self.offset = 2
super().__init__(num_embeddings + self.offset, embedding_dim, **kwargs)
def call(
self,
input_shape: Optional[tf.TensorShape] = None,
past_key_values_length: int = 0,
position_ids: tf.Tensor | None = None,
):
"""Input is expected to be of size [bsz x seqlen]."""
if position_ids is None:
seq_len = input_shape[1]
position_ids = tf.range(seq_len, delta=1, name="range")
position_ids += past_key_values_length
offset_dtype = position_ids.dtype if isinstance(position_ids, tf.Tensor) else tf.int32
return super().call(position_ids + tf.constant(self.offset, dtype=offset_dtype))
class TFBartAttention(keras.layers.Layer):
"""Multi-headed attention from "Attention Is All You Need"""
def __init__(
self,
embed_dim: int,
num_heads: int,
dropout: float = 0.0,
is_decoder: bool = False,
bias: bool = True,
**kwargs,
):
super().__init__(**kwargs)
self.embed_dim = embed_dim
self.num_heads = num_heads
self.dropout = keras.layers.Dropout(dropout)
self.head_dim = embed_dim // num_heads
if (self.head_dim * num_heads) != self.embed_dim:
raise ValueError(
f"embed_dim must be divisible by num_heads (got `embed_dim`: {self.embed_dim}"
f" and `num_heads`: {num_heads})."
)
self.scaling = self.head_dim**-0.5
self.is_decoder = is_decoder
self.k_proj = keras.layers.Dense(embed_dim, use_bias=bias, name="k_proj")
self.q_proj = keras.layers.Dense(embed_dim, use_bias=bias, name="q_proj")
self.v_proj = keras.layers.Dense(embed_dim, use_bias=bias, name="v_proj")
self.out_proj = keras.layers.Dense(embed_dim, use_bias=bias, name="out_proj")
def _shape(self, tensor: tf.Tensor, seq_len: int, bsz: int):
return tf.transpose(tf.reshape(tensor, (bsz, seq_len, self.num_heads, self.head_dim)), (0, 2, 1, 3))
def call(
self,
hidden_states: tf.Tensor,
key_value_states: tf.Tensor | None = None,
past_key_value: Tuple[Tuple[tf.Tensor]] | None = None,
attention_mask: tf.Tensor | None = None,
layer_head_mask: tf.Tensor | None = None,
training: Optional[bool] = False,
) -> Tuple[tf.Tensor, tf.Tensor | None]:
"""Input shape: Batch x Time x Channel"""
# if key_value_states are provided this layer is used as a cross-attention layer
# for the decoder
is_cross_attention = key_value_states is not None
bsz, tgt_len, embed_dim = shape_list(hidden_states)
# get query proj
query_states = self.q_proj(hidden_states) * self.scaling
# get key, value proj
if is_cross_attention and past_key_value is not None:
# reuse k,v, cross_attentions
key_states = past_key_value[0]
value_states = past_key_value[1]
elif is_cross_attention:
# cross_attentions
key_states = self._shape(self.k_proj(key_value_states), -1, bsz)
value_states = self._shape(self.v_proj(key_value_states), -1, bsz)
elif past_key_value is not None:
# reuse k, v, self_attention
key_states = self._shape(self.k_proj(hidden_states), -1, bsz)
value_states = self._shape(self.v_proj(hidden_states), -1, bsz)
key_states = tf.concat([past_key_value[0], key_states], axis=2)
value_states = tf.concat([past_key_value[1], value_states], axis=2)
else:
# self_attention
key_states = self._shape(self.k_proj(hidden_states), -1, bsz)
value_states = self._shape(self.v_proj(hidden_states), -1, bsz)
if self.is_decoder:
# if cross_attention save Tuple(tf.Tensor, tf.Tensor) of all cross attention key/value_states.
# Further calls to cross_attention layer can then reuse all cross-attention
# key/value_states (first "if" case)
# if uni-directional self-attention (decoder) save Tuple(tf.Tensor, tf.Tensor) of
# all previous decoder key/value_states. Further calls to uni-directional self-attention
# can concat previous decoder key/value_states to current projected key/value_states (third "elif" case)
# if encoder bi-directional self-attention `past_key_value` is always `None`
past_key_value = (key_states, value_states)
proj_shape = (bsz * self.num_heads, -1, self.head_dim)
query_states = tf.reshape(self._shape(query_states, tgt_len, bsz), proj_shape)
key_states = tf.reshape(key_states, proj_shape)
value_states = tf.reshape(value_states, proj_shape)
src_len = shape_list(key_states)[1]
attn_weights = tf.matmul(query_states, key_states, transpose_b=True)
tf.debugging.assert_equal(
shape_list(attn_weights),
[bsz * self.num_heads, tgt_len, src_len],
message=(
f"Attention weights should be of size {(bsz * self.num_heads, tgt_len, src_len)}, but is"
f" {shape_list(attn_weights)}"
),
)
if attention_mask is not None:
tf.debugging.assert_equal(
shape_list(attention_mask),
[bsz, 1, tgt_len, src_len],
message=(
f"Attention mask should be of size {(bsz, 1, tgt_len, src_len)}, but is"
f" {shape_list(attention_mask)}"
),
)
attention_mask = tf.cast(attention_mask, dtype=attn_weights.dtype)
attn_weights = tf.reshape(attn_weights, (bsz, self.num_heads, tgt_len, src_len)) + attention_mask
attn_weights = tf.reshape(attn_weights, (bsz * self.num_heads, tgt_len, src_len))
attn_weights = stable_softmax(attn_weights, axis=-1)
if layer_head_mask is not None:
tf.debugging.assert_equal(
shape_list(layer_head_mask),
[self.num_heads],
message=(
f"Head mask for a single layer should be of size {(self.num_heads)}, but is"
f" {shape_list(layer_head_mask)}"
),
)
attn_weights = tf.reshape(layer_head_mask, (1, -1, 1, 1)) * tf.reshape(
attn_weights, (bsz, self.num_heads, tgt_len, src_len)
)
attn_weights = tf.reshape(attn_weights, (bsz * self.num_heads, tgt_len, src_len))
attn_probs = self.dropout(attn_weights, training=training)
attn_output = tf.matmul(attn_probs, value_states)
tf.debugging.assert_equal(
shape_list(attn_output),
[bsz * self.num_heads, tgt_len, self.head_dim],
message=(
f"`attn_output` should be of size {(bsz, self.num_heads, tgt_len, self.head_dim)}, but is"
f" {shape_list(attn_output)}"
),
)
attn_output = tf.transpose(
tf.reshape(attn_output, (bsz, self.num_heads, tgt_len, self.head_dim)), (0, 2, 1, 3)
)
attn_output = tf.reshape(attn_output, (bsz, tgt_len, embed_dim))
attn_output = self.out_proj(attn_output)
attn_weights: tf.Tensor = tf.reshape(attn_weights, (bsz, self.num_heads, tgt_len, src_len))
return attn_output, attn_weights, past_key_value
def build(self, input_shape=None):
if self.built:
return
self.built = True
if getattr(self, "k_proj", None) is not None:
with tf.name_scope(self.k_proj.name):
self.k_proj.build([None, None, self.embed_dim])
if getattr(self, "q_proj", None) is not None:
with tf.name_scope(self.q_proj.name):
self.q_proj.build([None, None, self.embed_dim])
if getattr(self, "v_proj", None) is not None:
with tf.name_scope(self.v_proj.name):
self.v_proj.build([None, None, self.embed_dim])
if getattr(self, "out_proj", None) is not None:
with tf.name_scope(self.out_proj.name):
self.out_proj.build([None, None, self.embed_dim])
class TFBartEncoderLayer(keras.layers.Layer):
def __init__(self, config: BartConfig, **kwargs):
super().__init__(**kwargs)
self.embed_dim = config.d_model
self.self_attn = TFBartAttention(
self.embed_dim, config.encoder_attention_heads, dropout=config.attention_dropout, name="self_attn"
)
self.self_attn_layer_norm = keras.layers.LayerNormalization(epsilon=1e-5, name="self_attn_layer_norm")
self.dropout = keras.layers.Dropout(config.dropout)
self.activation_fn = get_tf_activation(config.activation_function)
self.activation_dropout = keras.layers.Dropout(config.activation_dropout)
self.fc1 = keras.layers.Dense(config.encoder_ffn_dim, name="fc1")
self.fc2 = keras.layers.Dense(self.embed_dim, name="fc2")
self.final_layer_norm = keras.layers.LayerNormalization(epsilon=1e-5, name="final_layer_norm")
self.config = config
def call(
self,
hidden_states: tf.Tensor,
attention_mask: np.ndarray | tf.Tensor | None,
layer_head_mask: tf.Tensor | None,
training: Optional[bool] = False,
) -> tf.Tensor:
"""
Args:
hidden_states (`tf.Tensor`): input to the layer of shape `(batch, seq_len, embed_dim)`
attention_mask (`tf.Tensor`): attention mask of size
`(batch, 1, tgt_len, src_len)` where padding elements are indicated by very large negative values.
layer_head_mask (`tf.Tensor`): mask for attention heads in a given layer of size
`(encoder_attention_heads,)`
"""
residual = hidden_states
hidden_states, self_attn_weights, _ = self.self_attn(
hidden_states=hidden_states, attention_mask=attention_mask, layer_head_mask=layer_head_mask
)
tf.debugging.assert_equal(
shape_list(hidden_states),
shape_list(residual),
message=f"Self attn modified the shape of query {shape_list(residual)} to {shape_list(hidden_states)}",
)
hidden_states = self.dropout(hidden_states, training=training)
hidden_states = residual + hidden_states
hidden_states = self.self_attn_layer_norm(hidden_states)
residual = hidden_states
hidden_states = self.activation_fn(self.fc1(hidden_states))
hidden_states = self.activation_dropout(hidden_states, training=training)
hidden_states = self.fc2(hidden_states)
hidden_states = self.dropout(hidden_states, training=training)
hidden_states = residual + hidden_states
hidden_states = self.final_layer_norm(hidden_states)
return hidden_states, self_attn_weights
def build(self, input_shape=None):
if self.built:
return
self.built = True
if getattr(self, "self_attn", None) is not None:
with tf.name_scope(self.self_attn.name):
self.self_attn.build(None)
if getattr(self, "self_attn_layer_norm", None) is not None:
with tf.name_scope(self.self_attn_layer_norm.name):
self.self_attn_layer_norm.build([None, None, self.embed_dim])
if getattr(self, "fc1", None) is not None:
with tf.name_scope(self.fc1.name):
self.fc1.build([None, None, self.embed_dim])
if getattr(self, "fc2", None) is not None:
with tf.name_scope(self.fc2.name):
self.fc2.build([None, None, self.config.encoder_ffn_dim])
if getattr(self, "final_layer_norm", None) is not None:
with tf.name_scope(self.final_layer_norm.name):
self.final_layer_norm.build([None, None, self.embed_dim])
class TFBartDecoderLayer(keras.layers.Layer):
def __init__(self, config: BartConfig, **kwargs):
super().__init__(**kwargs)
self.embed_dim = config.d_model
self.self_attn = TFBartAttention(
embed_dim=self.embed_dim,
num_heads=config.decoder_attention_heads,
dropout=config.attention_dropout,
name="self_attn",
is_decoder=True,
)
self.dropout = keras.layers.Dropout(config.dropout)
self.activation_fn = get_tf_activation(config.activation_function)
self.activation_dropout = keras.layers.Dropout(config.activation_dropout)
self.self_attn_layer_norm = keras.layers.LayerNormalization(epsilon=1e-5, name="self_attn_layer_norm")
self.encoder_attn = TFBartAttention(
self.embed_dim,
config.decoder_attention_heads,
dropout=config.attention_dropout,
name="encoder_attn",
is_decoder=True,
)
self.encoder_attn_layer_norm = keras.layers.LayerNormalization(epsilon=1e-5, name="encoder_attn_layer_norm")
self.fc1 = keras.layers.Dense(config.decoder_ffn_dim, name="fc1")
self.fc2 = keras.layers.Dense(self.embed_dim, name="fc2")
self.final_layer_norm = keras.layers.LayerNormalization(epsilon=1e-5, name="final_layer_norm")
self.config = config
def call(
self,
hidden_states: tf.Tensor,
attention_mask: np.ndarray | tf.Tensor | None = None,
encoder_hidden_states: np.ndarray | tf.Tensor | None = None,
encoder_attention_mask: np.ndarray | tf.Tensor | None = None,
layer_head_mask: tf.Tensor | None = None,
cross_attn_layer_head_mask: tf.Tensor | None = None,
past_key_value: Optional[Tuple[Tuple[Union[np.ndarray, tf.Tensor]]]] = None,
training: Optional[bool] = False,
) -> Tuple[tf.Tensor, tf.Tensor, Tuple[Tuple[tf.Tensor]]]:
"""
Args:
hidden_states (`tf.Tensor`): input to the layer of shape `(batch, seq_len, embed_dim)`
attention_mask (`tf.Tensor`): attention mask of size
`(batch, 1, tgt_len, src_len)` where padding elements are indicated by very large negative values.
encoder_hidden_states (`tf.Tensor`):
cross attention input to the layer of shape `(batch, seq_len, embed_dim)`
encoder_attention_mask (`tf.Tensor`): encoder attention mask of size
`(batch, 1, tgt_len, src_len)` where padding elements are indicated by very large negative values.
layer_head_mask (`tf.Tensor`): mask for attention heads in a given layer of size
`(decoder_attention_heads,)`
cross_attn_layer_head_mask (`tf.Tensor`): mask for heads of the cross-attention module.
`(decoder_attention_heads,)`
past_key_value (`Tuple(tf.Tensor)`): cached past key and value projection states
"""
residual = hidden_states
# Self Attention
# decoder uni-directional self-attention cached key/values tuple is at positions 1,2
self_attn_past_key_value = past_key_value[:2] if past_key_value is not None else None
# add present self-attn cache to positions 1,2 of present_key_value tuple
hidden_states, self_attn_weights, present_key_value = self.self_attn(
hidden_states=hidden_states,
past_key_value=self_attn_past_key_value,
attention_mask=attention_mask,
layer_head_mask=layer_head_mask,
)
hidden_states = self.dropout(hidden_states, training=training)
hidden_states = residual + hidden_states
hidden_states = self.self_attn_layer_norm(hidden_states)
# Cross-Attention Block
cross_attn_present_key_value = None
cross_attn_weights = None
if encoder_hidden_states is not None:
residual = hidden_states
# cross_attn cached key/values tuple is at positions 3,4 of present_key_value tuple
cross_attn_past_key_value = past_key_value[-2:] if past_key_value is not None else None
hidden_states, cross_attn_weights, cross_attn_present_key_value = self.encoder_attn(
hidden_states=hidden_states,
key_value_states=encoder_hidden_states,
attention_mask=encoder_attention_mask,
layer_head_mask=cross_attn_layer_head_mask,
past_key_value=cross_attn_past_key_value,
)
hidden_states = self.dropout(hidden_states, training=training)
hidden_states = residual + hidden_states
hidden_states = self.encoder_attn_layer_norm(hidden_states)
# add cross-attn to positions 3,4 of present_key_value tuple
present_key_value = present_key_value + cross_attn_present_key_value
# Fully Connected
residual = hidden_states
hidden_states = self.activation_fn(self.fc1(hidden_states))
hidden_states = self.activation_dropout(hidden_states, training=training)
hidden_states = self.fc2(hidden_states)
hidden_states = self.dropout(hidden_states, training=training)
hidden_states = residual + hidden_states
hidden_states = self.final_layer_norm(hidden_states)
return (
hidden_states,
self_attn_weights,
cross_attn_weights,
present_key_value,
)
def build(self, input_shape=None):
if self.built:
return
self.built = True
if getattr(self, "self_attn", None) is not None:
with tf.name_scope(self.self_attn.name):
self.self_attn.build(None)
if getattr(self, "self_attn_layer_norm", None) is not None:
with tf.name_scope(self.self_attn_layer_norm.name):
self.self_attn_layer_norm.build([None, None, self.embed_dim])
if getattr(self, "encoder_attn", None) is not None:
with tf.name_scope(self.encoder_attn.name):
self.encoder_attn.build(None)
if getattr(self, "encoder_attn_layer_norm", None) is not None:
with tf.name_scope(self.encoder_attn_layer_norm.name):
self.encoder_attn_layer_norm.build([None, None, self.embed_dim])
if getattr(self, "fc1", None) is not None:
with tf.name_scope(self.fc1.name):
self.fc1.build([None, None, self.embed_dim])
if getattr(self, "fc2", None) is not None:
with tf.name_scope(self.fc2.name):
self.fc2.build([None, None, self.config.decoder_ffn_dim])
if getattr(self, "final_layer_norm", None) is not None:
with tf.name_scope(self.final_layer_norm.name):
self.final_layer_norm.build([None, None, self.embed_dim])
class TFBartClassificationHead(keras.layers.Layer):
"""Head for sentence-level classification tasks."""
def __init__(self, inner_dim: int, num_classes: int, pooler_dropout: float, name: str, **kwargs):
super().__init__(name=name, **kwargs)
self.dense = keras.layers.Dense(inner_dim, name="dense")
self.dropout = keras.layers.Dropout(pooler_dropout)
self.out_proj = keras.layers.Dense(num_classes, name="out_proj")
self.input_dim = inner_dim
self.inner_dim = inner_dim
def call(self, inputs):
hidden_states = self.dropout(inputs)
hidden_states = self.dense(hidden_states)
hidden_states = keras.activations.tanh(hidden_states)
hidden_states = self.dropout(hidden_states)
hidden_states = self.out_proj(hidden_states)
return hidden_states
def build(self, input_shape=None):
if self.built:
return
self.built = True
if getattr(self, "dense", None) is not None:
with tf.name_scope(self.dense.name):
self.dense.build([None, None, self.input_dim])
if getattr(self, "out_proj", None) is not None:
with tf.name_scope(self.out_proj.name):
self.out_proj.build([None, None, self.inner_dim])
class TFBartPretrainedModel(TFPreTrainedModel):
config_class = BartConfig
base_model_prefix = "model"
@property
def dummy_inputs(self):
dummy_inputs = super().dummy_inputs
# Dummy inputs should not contain the default val of 1
# as this is the padding token and some assertions check it
dummy_inputs["input_ids"] = dummy_inputs["input_ids"] * 2
if "decoder_input_ids" in dummy_inputs:
dummy_inputs["decoder_input_ids"] = dummy_inputs["decoder_input_ids"] * 2
return dummy_inputs
def tf_to_pt_weight_rename(self, tf_weight):
if tf_weight == "model.shared.weight":
return tf_weight, "model.decoder.embed_tokens.weight"
else:
return (tf_weight,)
BART_START_DOCSTRING = r"""
This model inherits from [`TFPreTrainedModel`]. Check the superclass documentation for the generic methods the
library implements for all its model (such as downloading or saving, resizing the input embeddings, pruning heads
etc.)
This model is also a [keras.Model](https://www.tensorflow.org/api_docs/python/tf/keras/Model) subclass. Use it
as a regular TF 2.0 Keras Model and refer to the TF 2.0 documentation for all matter related to general usage and
behavior.
<Tip>
TensorFlow models and layers in `transformers` accept two formats as input:
- having all inputs as keyword arguments (like PyTorch models), or
- having all inputs as a list, tuple or dict in the first positional argument.
The reason the second format is supported is that Keras methods prefer this format when passing inputs to models
and layers. Because of this support, when using methods like `model.fit()` things should "just work" for you - just
pass your inputs and labels in any format that `model.fit()` supports! If, however, you want to use the second
format outside of Keras methods like `fit()` and `predict()`, such as when creating your own layers or models with
the Keras `Functional` API, there are three possibilities you can use to gather all the input Tensors in the first
positional argument:
- a single Tensor with `input_ids` only and nothing else: `model(input_ids)`
- a list of varying length with one or several input Tensors IN THE ORDER given in the docstring:
`model([input_ids, attention_mask])` or `model([input_ids, attention_mask, token_type_ids])`
- a dictionary with one or several input Tensors associated to the input names given in the docstring:
`model({"input_ids": input_ids, "token_type_ids": token_type_ids})`
Note that when creating models and layers with
[subclassing](https://keras.io/guides/making_new_layers_and_models_via_subclassing/) then you don't need to worry
about any of this, as you can just pass inputs like you would to any other Python function!
</Tip>
Args:
config ([`BartConfig`]): Model configuration class with all the parameters of the model.
Initializing with a config file does not load the weights associated with the model, only the
configuration. Check out the [`~TFPreTrainedModel.from_pretrained`] method to load the model weights.
"""
BART_GENERATION_EXAMPLE = r"""
Summarization example:
```python
>>> from transformers import AutoTokenizer, TFBartForConditionalGeneration
>>> model = TFBartForConditionalGeneration.from_pretrained("facebook/bart-large")
>>> tokenizer = AutoTokenizer.from_pretrained("facebook/bart-large")
>>> ARTICLE_TO_SUMMARIZE = "My friends are cool but they eat too many carbs."
>>> inputs = tokenizer([ARTICLE_TO_SUMMARIZE], max_length=1024, return_tensors="tf")
>>> # Generate Summary
>>> summary_ids = model.generate(inputs["input_ids"], num_beams=4, max_length=5)
>>> print(tokenizer.batch_decode(summary_ids, skip_special_tokens=True, clean_up_tokenization_spaces=False))
```
Mask filling example:
```python
>>> from transformers import AutoTokenizer, TFBartForConditionalGeneration
>>> tokenizer = AutoTokenizer.from_pretrained("facebook/bart-large")
>>> TXT = "My friends are <mask> but they eat too many carbs."
>>> model = TFBartForConditionalGeneration.from_pretrained("facebook/bart-large")
>>> input_ids = tokenizer([TXT], return_tensors="tf")["input_ids"]
>>> logits = model(input_ids).logits
>>> probs = tf.nn.softmax(logits[0])
>>> # probs[5] is associated with the mask token
```
"""
BART_INPUTS_DOCSTRING = r"""
Args:
input_ids (`tf.Tensor` of shape `({0})`):
Indices of input sequence tokens in the vocabulary.
Indices can be obtained using [`AutoTokenizer`]. See [`PreTrainedTokenizer.encode`] and
[`PreTrainedTokenizer.__call__`] for details.
[What are input IDs?](../glossary#input-ids)
attention_mask (`tf.Tensor` of shape `({0})`, *optional*):
Mask to avoid performing attention on padding token indices. Mask values selected in `[0, 1]`:
- 1 for tokens that are **not masked**,
- 0 for tokens that are **masked**.
[What are attention masks?](../glossary#attention-mask)
decoder_input_ids (`tf.Tensor` of shape `(batch_size, target_sequence_length)`, *optional*):
Indices of decoder input sequence tokens in the vocabulary.
Indices can be obtained using [`AutoTokenizer`]. See [`PreTrainedTokenizer.encode`] and
[`PreTrainedTokenizer.__call__`] for details.
[What are decoder input IDs?](../glossary#decoder-input-ids)
Bart uses the `eos_token_id` as the starting token for `decoder_input_ids` generation. If `past_key_values`
is used, optionally only the last `decoder_input_ids` have to be input (see `past_key_values`).
For translation and summarization training, `decoder_input_ids` should be provided. If no
`decoder_input_ids` is provided, the model will create this tensor by shifting the `input_ids` to the right
for denoising pre-training following the paper.
decoder_attention_mask (`tf.Tensor` of shape `(batch_size, target_sequence_length)`, *optional*):
will be made by default and ignore pad tokens. It is not recommended to set this for most use cases.
decoder_position_ids (`tf.Tensor` of shape `(batch_size, sequence_length)`, *optional*):
Indices of positions of each decoder input sequence tokens in the position embeddings. Selected in the
range `[0, config.max_position_embeddings - 1]`.
head_mask (`tf.Tensor` of shape `(encoder_layers, encoder_attention_heads)`, *optional*):
Mask to nullify selected heads of the attention modules in the encoder. Mask values selected in `[0, 1]`:
- 1 indicates the head is **not masked**,
- 0 indicates the head is **masked**.
decoder_head_mask (`tf.Tensor` of shape `(decoder_layers, decoder_attention_heads)`, *optional*):
Mask to nullify selected heads of the attention modules in the decoder. Mask values selected in `[0, 1]`:
- 1 indicates the head is **not masked**,
- 0 indicates the head is **masked**.
cross_attn_head_mask (`tf.Tensor` of shape `(decoder_layers, decoder_attention_heads)`, *optional*):
Mask to nullify selected heads of the cross-attention modules. Mask values selected in `[0, 1]`:
- 1 indicates the head is **not masked**,
- 0 indicates the head is **masked**.
encoder_outputs (`tf.FloatTensor`, *optional*):
hidden states at the output of the last layer of the encoder. Used in the cross-attention of the decoder.
of shape `(batch_size, sequence_length, hidden_size)` is a sequence of
past_key_values (`Tuple[Tuple[tf.Tensor]]` of length `config.n_layers`)
contains precomputed key and value hidden states of the attention blocks. Can be used to speed up decoding.
If `past_key_values` are used, the user can optionally input only the last `decoder_input_ids` (those that
don't have their past key value states given to this model) of shape `(batch_size, 1)` instead of all
`decoder_input_ids` of shape `(batch_size, sequence_length)`.
inputs_embeds (`tf.Tensor` of shape `(batch_size, sequence_length, hidden_size)`, *optional*):
Optionally, instead of passing `input_ids` you can choose to directly pass an embedded representation.
This is useful if you want more control over how to convert `input_ids` indices into associated vectors
than the model's internal embedding lookup matrix.
use_cache (`bool`, *optional*, defaults to `True`):
If set to `True`, `past_key_values` key value states are returned and can be used to speed up decoding (see
`past_key_values`). Set to `False` during training, `True` during generation
output_attentions (`bool`, *optional*):
Whether or not to return the attentions tensors of all attention layers. See `attentions` under returned
tensors for more detail. This argument can be used only in eager mode, in graph mode the value in the
config will be used instead.
output_hidden_states (`bool`, *optional*):
Whether or not to return the hidden states of all layers. See `hidden_states` under returned tensors for
more detail. This argument can be used only in eager mode, in graph mode the value in the config will be
used instead.
return_dict (`bool`, *optional*):
Whether or not to return a [`~utils.ModelOutput`] instead of a plain tuple. This argument can be used in
eager mode, in graph mode the value will always be set to True.
training (`bool`, *optional*, defaults to `False`):
Whether or not to use the model in training mode (some modules like dropout modules have different
behaviors between training and evaluation).
"""
@keras_serializable
class TFBartEncoder(keras.layers.Layer):
config_class = BartConfig
"""
Transformer encoder consisting of *config.encoder_layers* self attention layers. Each layer is a
[`TFBartEncoderLayer`].
Args:
config: BartConfig
"""
def __init__(self, config: BartConfig, embed_tokens: Optional[keras.layers.Embedding] = None, **kwargs):
super().__init__(**kwargs)
self.config = config
self.dropout = keras.layers.Dropout(config.dropout)
self.layerdrop = config.encoder_layerdrop
self.padding_idx = config.pad_token_id
self.max_source_positions = config.max_position_embeddings
self.embed_scale = tf.math.sqrt(float(config.d_model)) if config.scale_embedding else 1.0
self.embed_tokens = embed_tokens
self.embed_positions = TFBartLearnedPositionalEmbedding(
config.max_position_embeddings,
config.d_model,
name="embed_positions",
)
self.layers = [TFBartEncoderLayer(config, name=f"layers.{i}") for i in range(config.encoder_layers)]
self.layernorm_embedding = keras.layers.LayerNormalization(epsilon=1e-5, name="layernorm_embedding")
self.embed_dim = config.d_model
@unpack_inputs
def call(
self,
input_ids: TFModelInputType | None = None,
inputs_embeds: np.ndarray | tf.Tensor | None = None,
attention_mask: np.ndarray | tf.Tensor | None = None,
head_mask: np.ndarray | tf.Tensor | None = None,
output_attentions: Optional[bool] = None,
output_hidden_states: Optional[bool] = None,
return_dict: Optional[bool] = None,
training: Optional[bool] = False,
) -> Union[TFBaseModelOutput, Tuple[tf.Tensor]]:
"""
Args:
input_ids (`tf.Tensor` of shape `(batch_size, sequence_length)`):
Indices of input sequence tokens in the vocabulary. Padding will be ignored by default should you
provide it.
Indices can be obtained using [`AutoTokenizer`]. See [`PreTrainedTokenizer.encode`] and
[`PreTrainedTokenizer.__call__`] for details.
[What are input IDs?](../glossary#input-ids)
attention_mask (`tf.Tensor` of shape `(batch_size, sequence_length)`, *optional*):
Mask to avoid performing attention on padding token indices. Mask values selected in `[0, 1]`:
- 1 for tokens that are **not masked**,
- 0 for tokens that are **masked**.
[What are attention masks?](../glossary#attention-mask)
head_mask (`tf.Tensor` of shape `(encoder_layers, encoder_attention_heads)`, `optional):
Mask to nullify selected heads of the attention modules. Mask values selected in `[0, 1]`:
- 1 indicates the head is **not masked**,
- 0 indicates the head is **masked**.
inputs_embeds (`tf.Tensor` of shape `(batch_size, sequence_length, hidden_size)`, *optional*):
Optionally, instead of passing `input_ids` you can choose to directly pass an embedded representation.
This is useful if you want more control over how to convert `input_ids` indices into associated vectors
than the model's internal embedding lookup matrix.
output_attentions (`bool`, *optional*):
Whether or not to return the attentions tensors of all attention layers. See `attentions` under
returned tensors for more detail.
output_hidden_states (`bool`, *optional*):
Whether or not to return the hidden states of all layers. See `hidden_states` under returned tensors
for more detail.
return_dict (`bool`, *optional*):
Whether or not to return a [`~utils.ModelOutput`] instead of a plain tuple.
"""
if input_ids is not None and inputs_embeds is not None:
raise ValueError("You cannot specify both input_ids and inputs_embeds at the same time")
elif input_ids is not None:
input_shape = shape_list(input_ids)
elif inputs_embeds is not None:
input_shape = shape_list(inputs_embeds)[:-1]
else:
raise ValueError("You have to specify either input_ids or inputs_embeds")
if inputs_embeds is None:
check_embeddings_within_bounds(input_ids, self.embed_tokens.input_dim)
inputs_embeds = self.embed_tokens(input_ids) * self.embed_scale
embed_pos = self.embed_positions(input_shape)
hidden_states = inputs_embeds + embed_pos
hidden_states = self.layernorm_embedding(hidden_states)
hidden_states = self.dropout(hidden_states, training=training)
# check attention mask and invert
if attention_mask is not None:
# [bsz, seq_len] -> [bsz, 1, tgt_seq_len, src_seq_len]
attention_mask = _expand_mask(attention_mask)
else:
attention_mask = None
encoder_states = () if output_hidden_states else None
all_attentions = () if output_attentions else None
# check if head_mask has a correct number of layers specified if desired
if head_mask is not None:
tf.debugging.assert_equal(
shape_list(head_mask)[0],
len(self.layers),
message=(
f"The head_mask should be specified for {len(self.layers)} layers, but it is for"
f" {shape_list(head_mask)[0]}."
),
)
# encoder layers
for idx, encoder_layer in enumerate(self.layers):
if output_hidden_states:
encoder_states = encoder_states + (hidden_states,)
# add LayerDrop (see https://arxiv.org/abs/1909.11556 for description)
dropout_probability = random.uniform(0, 1)
if training and (dropout_probability < self.layerdrop): # skip the layer
continue
hidden_states, attn = encoder_layer(
hidden_states,
attention_mask,
head_mask[idx] if head_mask is not None else None,
)
if output_attentions:
all_attentions += (attn,)
if output_hidden_states:
encoder_states = encoder_states + (hidden_states,)
if not return_dict:
return tuple(v for v in [hidden_states, encoder_states, all_attentions] if v is not None)
return TFBaseModelOutput(
last_hidden_state=hidden_states, hidden_states=encoder_states, attentions=all_attentions
)
def build(self, input_shape=None):
if self.built:
return
self.built = True
if getattr(self, "embed_positions", None) is not None:
with tf.name_scope(self.embed_positions.name):
self.embed_positions.build(None)
if getattr(self, "layernorm_embedding", None) is not None:
with tf.name_scope(self.layernorm_embedding.name):
self.layernorm_embedding.build([None, None, self.embed_dim])
if getattr(self, "layers", None) is not None:
for layer in self.layers:
with tf.name_scope(layer.name):
layer.build(None)
@keras_serializable
class TFBartDecoder(keras.layers.Layer):
config_class = BartConfig
"""
Transformer decoder consisting of *config.decoder_layers* layers. Each layer is a [`TFBartDecoderLayer`]
Args:
config: BartConfig
embed_tokens: output embedding
"""
def __init__(self, config: BartConfig, embed_tokens: Optional[keras.layers.Embedding] = None, **kwargs):
super().__init__(**kwargs)
self.config = config
self.padding_idx = config.pad_token_id
self.embed_tokens = embed_tokens
self.layerdrop = config.decoder_layerdrop
self.embed_positions = TFBartLearnedPositionalEmbedding(
config.max_position_embeddings,
config.d_model,
name="embed_positions",
)
self.embed_scale = tf.math.sqrt(float(config.d_model)) if config.scale_embedding else 1.0
self.layers = [TFBartDecoderLayer(config, name=f"layers.{i}") for i in range(config.decoder_layers)]
self.layernorm_embedding = keras.layers.LayerNormalization(epsilon=1e-5, name="layernorm_embedding")
self.dropout = keras.layers.Dropout(config.dropout)
@unpack_inputs
def call(
self,
input_ids: TFModelInputType | None = None,
inputs_embeds: np.ndarray | tf.Tensor | None = None,
attention_mask: np.ndarray | tf.Tensor | None = None,
position_ids: np.ndarray | tf.Tensor | None = None,
encoder_hidden_states: np.ndarray | tf.Tensor | None = None,
encoder_attention_mask: np.ndarray | tf.Tensor | None = None,
head_mask: np.ndarray | tf.Tensor | None = None,
cross_attn_head_mask: np.ndarray | tf.Tensor | None = None,
past_key_values: Optional[Tuple[Tuple[Union[np.ndarray, tf.Tensor]]]] = None,
use_cache: Optional[bool] = None,
output_attentions: Optional[bool] = None,
output_hidden_states: Optional[bool] = None,
return_dict: Optional[bool] = None,
training: Optional[bool] = False,
) -> Union[TFBaseModelOutputWithPastAndCrossAttentions, Tuple[tf.Tensor]]:
r"""
Args:
input_ids (`tf.Tensor` of shape `(batch_size, sequence_length)`):
Indices of input sequence tokens in the vocabulary. Padding will be ignored by default should you
provide it.
Indices can be obtained using [`AutoTokenizer`]. See [`PreTrainedTokenizer.encode`] and
[`PreTrainedTokenizer.__call__`] for details.
[What are input IDs?](../glossary#input-ids)
attention_mask (`tf.Tensor` of shape `(batch_size, sequence_length)`, *optional*):
Mask to avoid performing attention on padding token indices. Mask values selected in `[0, 1]`:
- 1 for tokens that are **not masked**,
- 0 for tokens that are **masked**.
[What are attention masks?](../glossary#attention-mask)
position_ids (`tf.Tensor` of shape `(batch_size, sequence_length)`, *optional*):
Indices of positions of each decoder input sequence tokens in the position embeddings. Selected in the
range `[0, config.max_position_embeddings - 1]`.
encoder_hidden_states (`tf.Tensor` of shape `(batch_size, encoder_sequence_length, hidden_size)`, *optional*):
Sequence of hidden-states at the output of the last layer of the encoder. Used in the cross-attention
of the decoder.
encoder_attention_mask (`tf.Tensor` of shape `(batch_size, encoder_sequence_length)`, *optional*):
Mask to avoid performing cross-attention on padding tokens indices of encoder input_ids. Mask values
selected in `[0, 1]`:
- 1 for tokens that are **not masked**,
- 0 for tokens that are **masked**.
[What are attention masks?](../glossary#attention-mask)
head_mask (`tf.Tensor` of shape `(decoder_layers, decoder_attention_heads)`, *optional*):
Mask to nullify selected heads of the attention modules. Mask values selected in `[0, 1]`:
- 1 indicates the head is **not masked**,
- 0 indicates the head is **masked**.
cross_attn_head_mask (`tf.Tensor` of shape `(decoder_layers, decoder_attention_heads)`, *optional*):
Mask to nullify selected heads of the cross-attention modules. Mask values selected in `[0, 1]`:
- 1 indicates the head is **not masked**,
- 0 indicates the head is **masked**.
past_key_values (`Tuple[Tuple[tf.Tensor]]` of length `config.n_layers` with each tuple having 2 tuples each of which has 2 tensors of shape `(batch_size, num_heads, sequence_length - 1, embed_size_per_head)`):
Contains precomputed key and value hidden-states of the attention blocks. Can be used to speed up
decoding.
If `past_key_values` are used, the user can optionally input only the last `decoder_input_ids` (those
that don't have their past key value states given to this model) of shape `(batch_size, 1)` instead of
all `decoder_input_ids` of shape `(batch_size, sequence_length)`.
inputs_embeds (`tf.tTensor` of shape `(batch_size, sequence_length, hidden_size)`, *optional*):
Optionally, instead of passing `input_ids` you can choose to directly pass an embedded representation.
This is useful if you want more control over how to convert `input_ids` indices into associated vectors
than the model's internal embedding lookup matrix.
output_attentions (`bool`, *optional*):
Whether or not to return the attentions tensors of all attention layers. See `attentions` under
returned tensors for more detail.
output_hidden_states (`bool`, *optional*):
Whether or not to return the hidden states of all layers. See `hidden_states` under returned tensors
for more detail.
return_dict (`bool`, *optional*):
Whether or not to return a [`~utils.ModelOutput`] instead of a plain tuple.
"""
if input_ids is not None and inputs_embeds is not None:
raise ValueError("You cannot specify both decoder_input_ids and decoder_inputs_embeds at the same time")
elif input_ids is not None:
input_shape = shape_list(input_ids)
elif inputs_embeds is not None:
input_shape = shape_list(inputs_embeds)[:-1]
else:
raise ValueError("You have to specify either decoder_input_ids or decoder_inputs_embeds")
past_key_values_length = shape_list(past_key_values[0][0])[2] if past_key_values is not None else 0
# embed positions
if position_ids is None:
positions = self.embed_positions(input_shape, past_key_values_length)
else:
positions = self.embed_positions(input_shape, position_ids=position_ids)
if inputs_embeds is None:
check_embeddings_within_bounds(input_ids, self.embed_tokens.input_dim)
inputs_embeds = self.embed_tokens(input_ids) * self.embed_scale
hidden_states = inputs_embeds
# [bsz, seq_len] -> [bsz, 1, tgt_seq_len, src_seq_len]
if input_shape[-1] > 1:
combined_attention_mask = _make_causal_mask(input_shape, past_key_values_length=past_key_values_length)
else:
combined_attention_mask = _expand_mask(
tf.ones((input_shape[0], input_shape[1] + past_key_values_length)), tgt_len=input_shape[-1]
)
if attention_mask is not None:
combined_attention_mask = combined_attention_mask + _expand_mask(attention_mask, tgt_len=input_shape[-1])
if encoder_hidden_states is not None and encoder_attention_mask is not None:
# [bsz, seq_len] -> [bsz, 1, tgt_seq_len, src_seq_len]
encoder_attention_mask = _expand_mask(encoder_attention_mask, tgt_len=input_shape[-1])
hidden_states = self.layernorm_embedding(hidden_states + positions)
hidden_states = self.dropout(hidden_states, training=training)
# decoder layers
all_hidden_states = () if output_hidden_states else None
all_self_attns = () if output_attentions else None
all_cross_attns = () if (output_attentions and encoder_hidden_states is not None) else None
present_key_values = () if use_cache else None
# check if head_mask and cross_attn_head_mask have a correct number of layers specified if desired
for attn_mask_name, attn_mask in [("head_mask", head_mask), ("cross_attn_head_mask", cross_attn_head_mask)]:
if attn_mask is not None:
tf.debugging.assert_equal(
shape_list(attn_mask)[0],
len(self.layers),
message=(
f"The {attn_mask_name} should be specified for {len(self.layers)} layers, but it is for"
f" {shape_list(attn_mask)[0]}."
),
)
for idx, decoder_layer in enumerate(self.layers):
# add LayerDrop (see https://arxiv.org/abs/1909.11556 for description)
if output_hidden_states:
all_hidden_states += (hidden_states,)
dropout_probability = random.uniform(0, 1)
if training and (dropout_probability < self.layerdrop):
continue
past_key_value = past_key_values[idx] if past_key_values is not None else None
hidden_states, layer_self_attn, layer_cross_attn, present_key_value = decoder_layer(
hidden_states,
attention_mask=combined_attention_mask,
encoder_hidden_states=encoder_hidden_states,
encoder_attention_mask=encoder_attention_mask,
layer_head_mask=head_mask[idx] if head_mask is not None else None,
cross_attn_layer_head_mask=cross_attn_head_mask[idx] if cross_attn_head_mask is not None else None,
past_key_value=past_key_value,
)
if use_cache:
present_key_values += (present_key_value,)
if output_attentions:
all_self_attns += (layer_self_attn,)
if encoder_hidden_states is not None:
all_cross_attns += (layer_cross_attn,)
if output_hidden_states:
all_hidden_states += (hidden_states,)
if not return_dict:
return hidden_states, present_key_values, all_hidden_states, all_self_attns, all_cross_attns
else:
return TFBaseModelOutputWithPastAndCrossAttentions(
last_hidden_state=hidden_states,
past_key_values=present_key_values,
hidden_states=all_hidden_states,
attentions=all_self_attns,
cross_attentions=all_cross_attns,
)
def build(self, input_shape=None):
if self.built:
return
self.built = True
if getattr(self, "embed_positions", None) is not None:
with tf.name_scope(self.embed_positions.name):
self.embed_positions.build(None)
if getattr(self, "layernorm_embedding", None) is not None:
with tf.name_scope(self.layernorm_embedding.name):
self.layernorm_embedding.build([None, None, self.config.d_model])
if getattr(self, "layers", None) is not None:
for layer in self.layers:
with tf.name_scope(layer.name):
layer.build(None)
@keras_serializable
class TFBartMainLayer(keras.layers.Layer):
config_class = BartConfig
def __init__(self, config: BartConfig, load_weight_prefix=None, **kwargs):
super().__init__(**kwargs)
self.config = config
self.shared = keras.layers.Embedding(
input_dim=config.vocab_size,
output_dim=config.d_model,
embeddings_initializer=keras.initializers.TruncatedNormal(stddev=self.config.init_std),
name="model.shared",
)
# Additional attribute to specify the expected name scope of the layer (for loading/storing weights)
self.shared.load_weight_prefix = "model.shared" if load_weight_prefix is None else load_weight_prefix
self.encoder = TFBartEncoder(config, self.shared, name="encoder")
self.decoder = TFBartDecoder(config, self.shared, name="decoder")
def get_input_embeddings(self):
return self.shared
def set_input_embeddings(self, new_embeddings):
self.shared = new_embeddings
self.encoder.embed_tokens = self.shared
self.decoder.embed_tokens = self.shared
@unpack_inputs
def call(
self,
input_ids: TFModelInputType | None = None,
attention_mask: np.ndarray | tf.Tensor | None = None,
decoder_input_ids: np.ndarray | tf.Tensor | None = None,
decoder_attention_mask: np.ndarray | tf.Tensor | None = None,
decoder_position_ids: np.ndarray | tf.Tensor | None = None,
head_mask: np.ndarray | tf.Tensor | None = None,
decoder_head_mask: np.ndarray | tf.Tensor | None = None,
cross_attn_head_mask: np.ndarray | tf.Tensor | None = None,
encoder_outputs: Optional[Union[Tuple, TFBaseModelOutput]] = None,
past_key_values: Optional[Tuple[Tuple[Union[np.ndarray, tf.Tensor]]]] = None,
inputs_embeds: np.ndarray | tf.Tensor | None = None,
decoder_inputs_embeds: np.ndarray | tf.Tensor | None = None,
use_cache: Optional[bool] = None,
output_attentions: Optional[bool] = None,
output_hidden_states: Optional[bool] = None,
return_dict: Optional[bool] = None,
training: Optional[bool] = False,
**kwargs,
) -> Union[TFSeq2SeqModelOutput, Tuple[tf.Tensor]]:
# different to other models, Bart automatically creates decoder_input_ids from
# input_ids if no decoder_input_ids are provided
if decoder_input_ids is None and decoder_inputs_embeds is None:
if input_ids is None:
raise ValueError(
"If no `decoder_input_ids` or `decoder_inputs_embeds` are "
"passed, `input_ids` cannot be `None`. Please pass either "
"`input_ids` or `decoder_input_ids` or `decoder_inputs_embeds`."
)
decoder_input_ids = shift_tokens_right(
input_ids, self.config.pad_token_id, self.config.decoder_start_token_id
)
if encoder_outputs is None:
encoder_outputs = self.encoder(
input_ids=input_ids,
attention_mask=attention_mask,
head_mask=head_mask,
inputs_embeds=inputs_embeds,
output_attentions=output_attentions,
output_hidden_states=output_hidden_states,
return_dict=return_dict,
training=training,
)
# If the user passed a tuple for encoder_outputs, we wrap it in a TFBaseModelOutput when return_dict=True
elif return_dict and not isinstance(encoder_outputs, TFBaseModelOutput):
encoder_outputs = TFBaseModelOutput(
last_hidden_state=encoder_outputs[0],
hidden_states=encoder_outputs[1] if len(encoder_outputs) > 1 else None,
attentions=encoder_outputs[2] if len(encoder_outputs) > 2 else None,
)
# If the user passed a TFBaseModelOutput for encoder_outputs, we wrap it in a tuple when return_dict=False
elif not return_dict and not isinstance(encoder_outputs, tuple):
encoder_outputs = encoder_outputs.to_tuple()
decoder_outputs = self.decoder(
decoder_input_ids,
attention_mask=decoder_attention_mask,
position_ids=decoder_position_ids,
encoder_hidden_states=encoder_outputs[0],
encoder_attention_mask=attention_mask,
head_mask=decoder_head_mask,
cross_attn_head_mask=cross_attn_head_mask,
past_key_values=past_key_values,
inputs_embeds=decoder_inputs_embeds,
use_cache=use_cache,
output_attentions=output_attentions,
output_hidden_states=output_hidden_states,
return_dict=return_dict,
training=training,
)
if not return_dict:
return decoder_outputs + encoder_outputs
return TFSeq2SeqModelOutput(
last_hidden_state=decoder_outputs.last_hidden_state,
past_key_values=decoder_outputs.past_key_values,
decoder_hidden_states=decoder_outputs.hidden_states,
decoder_attentions=decoder_outputs.attentions,
cross_attentions=decoder_outputs.cross_attentions,
encoder_last_hidden_state=encoder_outputs.last_hidden_state,
encoder_hidden_states=encoder_outputs.hidden_states,
encoder_attentions=encoder_outputs.attentions,
)
def build(self, input_shape=None):
if self.built:
return
self.built = True
# The shared/tied weights expect to be in the model base namespace
# Adding "/" to the end (not the start!) of a tf.name_scope puts it in the root namespace rather than
# the current one.
with tf.name_scope(self.shared.load_weight_prefix + "/" + self.shared.name + "/"):
self.shared.build(None)
if getattr(self, "encoder", None) is not None:
with tf.name_scope(self.encoder.name):
self.encoder.build(None)
if getattr(self, "decoder", None) is not None:
with tf.name_scope(self.decoder.name):
self.decoder.build(None)
@add_start_docstrings(
"The bare BART Model outputting raw hidden-states without any specific head on top.",
BART_START_DOCSTRING,
)
class TFBartModel(TFBartPretrainedModel):
_requires_load_weight_prefix = True
def __init__(self, config: BartConfig, load_weight_prefix=None, *inputs, **kwargs):
super().__init__(config, *inputs, **kwargs)
self.model = TFBartMainLayer(config, load_weight_prefix=load_weight_prefix, name="model")
def get_encoder(self):
return self.model.encoder
def get_decoder(self):
return self.model.decoder
@add_start_docstrings_to_model_forward(BART_INPUTS_DOCSTRING.format("batch_size, sequence_length"))
@add_code_sample_docstrings(
checkpoint=_CHECKPOINT_FOR_DOC,
output_type=TFSeq2SeqModelOutput,
config_class=_CONFIG_FOR_DOC,
)
@unpack_inputs
def call(
self,
input_ids: TFModelInputType | None = None,
attention_mask: np.ndarray | tf.Tensor | None = None,
decoder_input_ids: np.ndarray | tf.Tensor | None = None,
decoder_attention_mask: np.ndarray | tf.Tensor | None = None,
decoder_position_ids: np.ndarray | tf.Tensor | None = None,
head_mask: np.ndarray | tf.Tensor | None = None,
decoder_head_mask: np.ndarray | tf.Tensor | None = None,
cross_attn_head_mask: np.ndarray | tf.Tensor | None = None,
encoder_outputs: Optional[Union[Tuple, TFBaseModelOutput]] = None,
past_key_values: Optional[Tuple[Tuple[Union[np.ndarray, tf.Tensor]]]] = None,
inputs_embeds: np.ndarray | tf.Tensor | None = None,
decoder_inputs_embeds: np.ndarray | tf.Tensor | None = None,
use_cache: Optional[bool] = None,
output_attentions: Optional[bool] = None,
output_hidden_states: Optional[bool] = None,
return_dict: Optional[bool] = None,
training: Optional[bool] = False,
**kwargs,
) -> Union[TFBaseModelOutput, Tuple[tf.Tensor]]:
outputs = self.model(
input_ids=input_ids,
attention_mask=attention_mask,
decoder_input_ids=decoder_input_ids,
decoder_attention_mask=decoder_attention_mask,
decoder_position_ids=decoder_position_ids,
head_mask=head_mask,
decoder_head_mask=decoder_head_mask,
cross_attn_head_mask=cross_attn_head_mask,
encoder_outputs=encoder_outputs,
past_key_values=past_key_values,
inputs_embeds=inputs_embeds,
decoder_inputs_embeds=decoder_inputs_embeds,
use_cache=use_cache,
output_attentions=output_attentions,
output_hidden_states=output_hidden_states,
return_dict=return_dict,
training=training,
)
return outputs
def serving_output(self, output):
pkv = tf.tuple(output.past_key_values)[1] if self.config.use_cache else None
dec_hs = tf.convert_to_tensor(output.decoder_hidden_states) if self.config.output_hidden_states else None
dec_attns = tf.convert_to_tensor(output.decoder_attentions) if self.config.output_attentions else None
cross_attns = tf.convert_to_tensor(output.cross_attentions) if self.config.output_attentions else None
enc_hs = tf.convert_to_tensor(output.encoder_hidden_states) if self.config.output_hidden_states else None
enc_attns = tf.convert_to_tensor(output.encoder_attentions) if self.config.output_attentions else None
return TFSeq2SeqModelOutput(
last_hidden_state=output.last_hidden_state,
past_key_values=pkv,
decoder_hidden_states=dec_hs,
decoder_attentions=dec_attns,
cross_attentions=cross_attns,
encoder_last_hidden_state=output.encoder_last_hidden_state,
encoder_hidden_states=enc_hs,
encoder_attentions=enc_attns,
)
def build(self, input_shape=None):
if self.built:
return
self.built = True
if getattr(self, "model", None) is not None:
with tf.name_scope(self.model.name):
self.model.build(None)
class BiasLayer(keras.layers.Layer):
"""
Bias as a layer. It is used for serialization purposes: `keras.Model.save_weights` stores on a per-layer basis,
so all weights have to be registered in a layer.
"""
def __init__(self, shape, initializer, trainable, name, **kwargs):
super().__init__(name=name, **kwargs)
# Note: the name of this variable will NOT be scoped when serialized, i.e. it will not be in the format of
# "outer_layer/inner_layer/.../name:0". Instead, it will be "name:0". For further details, see:
# https://github.com/huggingface/transformers/pull/18833#issuecomment-1233090214
self.bias = self.add_weight(name=name, shape=shape, initializer=initializer, trainable=trainable)
def call(self, x):
return x + self.bias
@add_start_docstrings(
"The BART Model with a language modeling head. Can be used for summarization.",
BART_START_DOCSTRING,
)
class TFBartForConditionalGeneration(TFBartPretrainedModel, TFCausalLanguageModelingLoss):
_keys_to_ignore_on_load_missing = [r"final_logits_bias"]
_requires_load_weight_prefix = True
def __init__(self, config, load_weight_prefix=None, *inputs, **kwargs):
super().__init__(config, *inputs, **kwargs)
self.model = TFBartMainLayer(config, load_weight_prefix=load_weight_prefix, name="model")
self.use_cache = config.use_cache
# final_bias_logits is registered as a buffer in pytorch, so not trainable for the sake of consistency.
self.bias_layer = BiasLayer(
name="final_logits_bias", shape=[1, config.vocab_size], initializer="zeros", trainable=False
)
def get_decoder(self):
return self.model.decoder
def get_encoder(self):
return self.model.encoder
def get_output_embeddings(self):
return self.get_input_embeddings()
def set_output_embeddings(self, value):
self.set_input_embeddings(value)
def get_bias(self):
return {"final_logits_bias": self.bias_layer.bias}
def set_bias(self, value):
# Replaces the existing layers containing bias for correct (de)serialization.
vocab_size = value["final_logits_bias"].shape[-1]
self.bias_layer = BiasLayer(
name="final_logits_bias", shape=[1, vocab_size], initializer="zeros", trainable=False
)
self.bias_layer.bias.assign(value["final_logits_bias"])
@add_start_docstrings_to_model_forward(BART_INPUTS_DOCSTRING)
@replace_return_docstrings(output_type=TFSeq2SeqLMOutput, config_class=_CONFIG_FOR_DOC)
@add_end_docstrings(BART_GENERATION_EXAMPLE)
@unpack_inputs
def call(
self,
input_ids: TFModelInputType | None = None,
attention_mask: np.ndarray | tf.Tensor | None = None,
decoder_input_ids: np.ndarray | tf.Tensor | None = None,
decoder_attention_mask: np.ndarray | tf.Tensor | None = None,
decoder_position_ids: np.ndarray | tf.Tensor | None = None,
head_mask: np.ndarray | tf.Tensor | None = None,
decoder_head_mask: np.ndarray | tf.Tensor | None = None,
cross_attn_head_mask: np.ndarray | tf.Tensor | None = None,
encoder_outputs: Optional[TFBaseModelOutput] = None,
past_key_values: Optional[Tuple[Tuple[Union[np.ndarray, tf.Tensor]]]] = None,
inputs_embeds: np.ndarray | tf.Tensor | None = None,
decoder_inputs_embeds: np.ndarray | tf.Tensor | None = None,
use_cache: Optional[bool] = None,
output_attentions: Optional[bool] = None,
output_hidden_states: Optional[bool] = None,
return_dict: Optional[bool] = None,
labels: tf.Tensor | None = None,
training: Optional[bool] = False,
) -> Union[TFSeq2SeqLMOutput, Tuple[tf.Tensor]]:
r"""
labels (`tf.Tensor` of shape `(batch_size, sequence_length)`, *optional*):
Labels for computing the masked language modeling loss. Indices should either be in `[0, ...,
config.vocab_size]` or -100 (see `input_ids` docstring). Tokens with indices set to `-100` are ignored
(masked), the loss is only computed for the tokens with labels in `[0, ..., config.vocab_size]`.
Returns:
"""
if labels is not None:
labels = tf.where(
labels == self.config.pad_token_id,
tf.cast(tf.fill(shape_list(labels), -100), labels.dtype),
labels,
)
use_cache = False
if decoder_input_ids is None and decoder_inputs_embeds is None:
decoder_input_ids = shift_tokens_right(
labels, self.config.pad_token_id, self.config.decoder_start_token_id
)
outputs = self.model(
input_ids,
attention_mask=attention_mask,
decoder_input_ids=decoder_input_ids,
encoder_outputs=encoder_outputs,
decoder_attention_mask=decoder_attention_mask,
decoder_position_ids=decoder_position_ids,
head_mask=head_mask,
decoder_head_mask=decoder_head_mask,
cross_attn_head_mask=cross_attn_head_mask,
past_key_values=past_key_values,
inputs_embeds=inputs_embeds,
decoder_inputs_embeds=decoder_inputs_embeds,
use_cache=use_cache,
output_attentions=output_attentions,
output_hidden_states=output_hidden_states,
return_dict=return_dict,
training=training,
)
lm_logits = tf.matmul(outputs[0], self.model.shared.weights, transpose_b=True)
lm_logits = self.bias_layer(lm_logits)
masked_lm_loss = None if labels is None else self.hf_compute_loss(labels, lm_logits)
if not return_dict:
output = (lm_logits,) + outputs[1:]
return ((masked_lm_loss,) + output) if masked_lm_loss is not None else output
return TFSeq2SeqLMOutput(
loss=masked_lm_loss,
logits=lm_logits,
past_key_values=outputs.past_key_values, # index 1 of d outputs
decoder_hidden_states=outputs.decoder_hidden_states, # index 2 of d outputs
decoder_attentions=outputs.decoder_attentions, # index 3 of d outputs
cross_attentions=outputs.cross_attentions, # index 4 of d outputs
encoder_last_hidden_state=outputs.encoder_last_hidden_state, # index 0 of encoder outputs
encoder_hidden_states=outputs.encoder_hidden_states, # 1 of e out
encoder_attentions=outputs.encoder_attentions, # 2 of e out
)
def serving_output(self, output):
pkv = tf.tuple(output.past_key_values)[1] if self.config.use_cache else None
dec_hs = tf.convert_to_tensor(output.decoder_hidden_states) if self.config.output_hidden_states else None
dec_attns = tf.convert_to_tensor(output.decoder_attentions) if self.config.output_attentions else None
cross_attns = tf.convert_to_tensor(output.cross_attentions) if self.config.output_attentions else None
enc_hs = tf.convert_to_tensor(output.encoder_hidden_states) if self.config.output_hidden_states else None
enc_attns = tf.convert_to_tensor(output.encoder_attentions) if self.config.output_attentions else None
return TFSeq2SeqLMOutput(
logits=output.logits,
past_key_values=pkv,
decoder_hidden_states=dec_hs,
decoder_attentions=dec_attns,
cross_attentions=cross_attns,
encoder_last_hidden_state=output.encoder_last_hidden_state,
encoder_hidden_states=enc_hs,
encoder_attentions=enc_attns,
)
def prepare_inputs_for_generation(
self,
decoder_input_ids,
past_key_values=None,
attention_mask=None,
decoder_attention_mask=None,
head_mask=None,
decoder_head_mask=None,
cross_attn_head_mask=None,
use_cache=None,
encoder_outputs=None,
**kwargs,
):
# cut decoder_input_ids if past_key_values is used
if past_key_values is not None:
decoder_input_ids = decoder_input_ids[:, -1:]
if decoder_attention_mask is not None: # xla
decoder_position_ids = tf.math.cumsum(decoder_attention_mask, axis=-1, exclusive=True)[:, -1:]
elif past_key_values is not None: # no xla + past_key_values
decoder_position_ids = past_key_values[0][0].shape[2]
else: # no xla + no past_key_values
decoder_position_ids = tf.range(decoder_input_ids.shape[1])
return {
"input_ids": None, # encoder_outputs is defined. input_ids not needed
"encoder_outputs": encoder_outputs,
"past_key_values": past_key_values,
"decoder_input_ids": decoder_input_ids,
"attention_mask": attention_mask,
"decoder_attention_mask": decoder_attention_mask,
"decoder_position_ids": decoder_position_ids,
"head_mask": head_mask,
"decoder_head_mask": decoder_head_mask,
"cross_attn_head_mask": cross_attn_head_mask,
"use_cache": use_cache, # change this to avoid caching (presumably for debugging)
}
def prepare_decoder_input_ids_from_labels(self, labels: tf.Tensor):
return shift_tokens_right(labels, self.config.pad_token_id, self.config.decoder_start_token_id)
def build(self, input_shape=None):
if self.built:
return
self.built = True
if getattr(self, "model", None) is not None:
with tf.name_scope(self.model.name):
self.model.build(None)
if getattr(self, "bias_layer", None) is not None:
with tf.name_scope(self.bias_layer.name):
self.bias_layer.build(None)
@add_start_docstrings(
"""
Bart model with a sequence classification/head on top (a linear layer on top of the pooled output) e.g. for GLUE
tasks.
""",
BART_START_DOCSTRING,
)
class TFBartForSequenceClassification(TFBartPretrainedModel, TFSequenceClassificationLoss):
def __init__(self, config: BartConfig, load_weight_prefix=None, *inputs, **kwargs):
super().__init__(config, *inputs, **kwargs)
self.model = TFBartMainLayer(config, load_weight_prefix=load_weight_prefix, name="model")
self.classification_head = TFBartClassificationHead(
config.d_model, config.num_labels, config.classifier_dropout, name="classification_head"
)
@add_start_docstrings_to_model_forward(BART_INPUTS_DOCSTRING)
@replace_return_docstrings(output_type=TFSeq2SeqSequenceClassifierOutput, config_class=_CONFIG_FOR_DOC)
@unpack_inputs
def call(
self,
input_ids: TFModelInputType | None = None,
attention_mask: np.ndarray | tf.Tensor | None = None,
decoder_input_ids: np.ndarray | tf.Tensor | None = None,
decoder_attention_mask: np.ndarray | tf.Tensor | None = None,
decoder_position_ids: np.ndarray | tf.Tensor | None = None,
head_mask: np.ndarray | tf.Tensor | None = None,
decoder_head_mask: np.ndarray | tf.Tensor | None = None,
cross_attn_head_mask: np.ndarray | tf.Tensor | None = None,
encoder_outputs: Optional[TFBaseModelOutput] = None,
past_key_values: Optional[Tuple[Tuple[Union[np.ndarray, tf.Tensor]]]] = None,
inputs_embeds: np.ndarray | tf.Tensor | None = None,
decoder_inputs_embeds: np.ndarray | tf.Tensor | None = None,
use_cache: Optional[bool] = None,
output_attentions: Optional[bool] = None,
output_hidden_states: Optional[bool] = None,
return_dict: Optional[bool] = None,
labels: tf.Tensor | None = None,
training: Optional[bool] = False,
) -> Union[TFSeq2SeqSequenceClassifierOutput, Tuple[tf.Tensor]]:
r"""
labels (`tf.Tensor` of shape `(batch_size, sequence_length)`, *optional*):
Labels for computing the sequence classification/regression loss. Indices should be in `[0, ...,
config.num_labels - 1]`. If `config.num_labels > 1` a classification loss is computed (Cross-Entropy).
Returns:
"""
return_dict = return_dict if return_dict is not None else self.config.use_return_dict
if labels is not None:
use_cache = False
if input_ids is None and inputs_embeds is not None:
raise NotImplementedError(
f"Passing input embeddings is currently not supported for {self.__class__.__name__}"
)
outputs = self.model(
input_ids=input_ids,
attention_mask=attention_mask,
decoder_input_ids=decoder_input_ids,
decoder_attention_mask=decoder_attention_mask,
decoder_position_ids=decoder_position_ids,
head_mask=head_mask,
decoder_head_mask=decoder_head_mask,
cross_attn_head_mask=cross_attn_head_mask,
encoder_outputs=encoder_outputs,
past_key_values=past_key_values,
inputs_embeds=inputs_embeds,
decoder_inputs_embeds=decoder_inputs_embeds,
use_cache=use_cache,
output_attentions=output_attentions,
output_hidden_states=output_hidden_states,
return_dict=return_dict,
training=training,
)
last_hidden_state = outputs[0]
eos_mask = tf.equal(input_ids, self.config.eos_token_id)
# out the rows with False where present. Then verify all the final
# entries are True
self_masked = tf.reshape(tf.boolean_mask(eos_mask, eos_mask), (tf.shape(input_ids)[0], -1))
tf.Assert(tf.reduce_all(self_masked[:, -1]), ["All examples must have the same number of <eos> tokens."])
masked = tf.reshape(
tf.boolean_mask(last_hidden_state, eos_mask),
(tf.shape(input_ids)[0], tf.shape(self_masked)[1], tf.shape(last_hidden_state)[-1]),
)
sentence_representation = masked[:, -1, :]
logits = self.classification_head(sentence_representation)
loss = None if labels is None else self.hf_compute_loss(labels=labels, logits=logits)
if not return_dict:
output = (logits,) + outputs[1:]
return ((loss,) + output) if loss is not None else output
return TFSeq2SeqSequenceClassifierOutput(
loss=loss,
logits=logits,
past_key_values=outputs.past_key_values,
decoder_hidden_states=outputs.decoder_hidden_states,
decoder_attentions=outputs.decoder_attentions,
cross_attentions=outputs.cross_attentions,
encoder_last_hidden_state=outputs.encoder_last_hidden_state,
encoder_hidden_states=outputs.encoder_hidden_states,
encoder_attentions=outputs.encoder_attentions,
)
def serving_output(self, output):
logits = tf.convert_to_tensor(output.logits)
pkv = tf.tuple(output.past_key_values)[1] if self.config.use_cache else None
dec_hs = tf.convert_to_tensor(output.decoder_hidden_states) if self.config.output_hidden_states else None
dec_attns = tf.convert_to_tensor(output.decoder_attentions) if self.config.output_attentions else None
cross_attns = tf.convert_to_tensor(output.cross_attentions) if self.config.output_attentions else None
enc_hs = tf.convert_to_tensor(output.encoder_hidden_states) if self.config.output_hidden_states else None
enc_attns = tf.convert_to_tensor(output.encoder_attentions) if self.config.output_attentions else None
return TFSeq2SeqSequenceClassifierOutput(
logits=logits,
past_key_values=pkv,
decoder_hidden_states=dec_hs,
decoder_attentions=dec_attns,
cross_attentions=cross_attns,
encoder_last_hidden_state=output.encoder_last_hidden_state,
encoder_hidden_states=enc_hs,
encoder_attentions=enc_attns,
)
def build(self, input_shape=None):
if self.built:
return
self.built = True
if getattr(self, "model", None) is not None:
with tf.name_scope(self.model.name):
self.model.build(None)
if getattr(self, "classification_head", None) is not None:
with tf.name_scope(self.classification_head.name):
self.classification_head.build(None)
| transformers/src/transformers/models/bart/modeling_tf_bart.py/0 | {
"file_path": "transformers/src/transformers/models/bart/modeling_tf_bart.py",
"repo_id": "transformers",
"token_count": 35465
} | 310 |
# coding=utf-8
# Copyright 2018 The Google AI Language Team Authors and The HuggingFace Inc. team.
# Copyright (c) 2018, NVIDIA CORPORATION. All rights reserved.
#
# Licensed under the Apache License, Version 2.0 (the "License");
# you may not use this file except in compliance with the License.
# You may obtain a copy of the License at
#
# http://www.apache.org/licenses/LICENSE-2.0
#
# Unless required by applicable law or agreed to in writing, software
# distributed under the License is distributed on an "AS IS" BASIS,
# WITHOUT WARRANTIES OR CONDITIONS OF ANY KIND, either express or implied.
# See the License for the specific language governing permissions and
# limitations under the License.
""" BERT model configuration"""
from collections import OrderedDict
from typing import Mapping
from ...configuration_utils import PretrainedConfig
from ...onnx import OnnxConfig
from ...utils import logging
logger = logging.get_logger(__name__)
BERT_PRETRAINED_CONFIG_ARCHIVE_MAP = {
"bert-base-uncased": "https://huggingface.co/bert-base-uncased/resolve/main/config.json",
"bert-large-uncased": "https://huggingface.co/bert-large-uncased/resolve/main/config.json",
"bert-base-cased": "https://huggingface.co/bert-base-cased/resolve/main/config.json",
"bert-large-cased": "https://huggingface.co/bert-large-cased/resolve/main/config.json",
"bert-base-multilingual-uncased": "https://huggingface.co/bert-base-multilingual-uncased/resolve/main/config.json",
"bert-base-multilingual-cased": "https://huggingface.co/bert-base-multilingual-cased/resolve/main/config.json",
"bert-base-chinese": "https://huggingface.co/bert-base-chinese/resolve/main/config.json",
"bert-base-german-cased": "https://huggingface.co/bert-base-german-cased/resolve/main/config.json",
"bert-large-uncased-whole-word-masking": (
"https://huggingface.co/bert-large-uncased-whole-word-masking/resolve/main/config.json"
),
"bert-large-cased-whole-word-masking": (
"https://huggingface.co/bert-large-cased-whole-word-masking/resolve/main/config.json"
),
"bert-large-uncased-whole-word-masking-finetuned-squad": (
"https://huggingface.co/bert-large-uncased-whole-word-masking-finetuned-squad/resolve/main/config.json"
),
"bert-large-cased-whole-word-masking-finetuned-squad": (
"https://huggingface.co/bert-large-cased-whole-word-masking-finetuned-squad/resolve/main/config.json"
),
"bert-base-cased-finetuned-mrpc": "https://huggingface.co/bert-base-cased-finetuned-mrpc/resolve/main/config.json",
"bert-base-german-dbmdz-cased": "https://huggingface.co/bert-base-german-dbmdz-cased/resolve/main/config.json",
"bert-base-german-dbmdz-uncased": "https://huggingface.co/bert-base-german-dbmdz-uncased/resolve/main/config.json",
"cl-tohoku/bert-base-japanese": "https://huggingface.co/cl-tohoku/bert-base-japanese/resolve/main/config.json",
"cl-tohoku/bert-base-japanese-whole-word-masking": (
"https://huggingface.co/cl-tohoku/bert-base-japanese-whole-word-masking/resolve/main/config.json"
),
"cl-tohoku/bert-base-japanese-char": (
"https://huggingface.co/cl-tohoku/bert-base-japanese-char/resolve/main/config.json"
),
"cl-tohoku/bert-base-japanese-char-whole-word-masking": (
"https://huggingface.co/cl-tohoku/bert-base-japanese-char-whole-word-masking/resolve/main/config.json"
),
"TurkuNLP/bert-base-finnish-cased-v1": (
"https://huggingface.co/TurkuNLP/bert-base-finnish-cased-v1/resolve/main/config.json"
),
"TurkuNLP/bert-base-finnish-uncased-v1": (
"https://huggingface.co/TurkuNLP/bert-base-finnish-uncased-v1/resolve/main/config.json"
),
"wietsedv/bert-base-dutch-cased": "https://huggingface.co/wietsedv/bert-base-dutch-cased/resolve/main/config.json",
# See all BERT models at https://huggingface.co/models?filter=bert
}
class BertConfig(PretrainedConfig):
r"""
This is the configuration class to store the configuration of a [`BertModel`] or a [`TFBertModel`]. It is used to
instantiate a BERT model according to the specified arguments, defining the model architecture. Instantiating a
configuration with the defaults will yield a similar configuration to that of the BERT
[bert-base-uncased](https://huggingface.co/bert-base-uncased) architecture.
Configuration objects inherit from [`PretrainedConfig`] and can be used to control the model outputs. Read the
documentation from [`PretrainedConfig`] for more information.
Args:
vocab_size (`int`, *optional*, defaults to 30522):
Vocabulary size of the BERT model. Defines the number of different tokens that can be represented by the
`inputs_ids` passed when calling [`BertModel`] or [`TFBertModel`].
hidden_size (`int`, *optional*, defaults to 768):
Dimensionality of the encoder layers and the pooler layer.
num_hidden_layers (`int`, *optional*, defaults to 12):
Number of hidden layers in the Transformer encoder.
num_attention_heads (`int`, *optional*, defaults to 12):
Number of attention heads for each attention layer in the Transformer encoder.
intermediate_size (`int`, *optional*, defaults to 3072):
Dimensionality of the "intermediate" (often named feed-forward) layer in the Transformer encoder.
hidden_act (`str` or `Callable`, *optional*, defaults to `"gelu"`):
The non-linear activation function (function or string) in the encoder and pooler. If string, `"gelu"`,
`"relu"`, `"silu"` and `"gelu_new"` are supported.
hidden_dropout_prob (`float`, *optional*, defaults to 0.1):
The dropout probability for all fully connected layers in the embeddings, encoder, and pooler.
attention_probs_dropout_prob (`float`, *optional*, defaults to 0.1):
The dropout ratio for the attention probabilities.
max_position_embeddings (`int`, *optional*, defaults to 512):
The maximum sequence length that this model might ever be used with. Typically set this to something large
just in case (e.g., 512 or 1024 or 2048).
type_vocab_size (`int`, *optional*, defaults to 2):
The vocabulary size of the `token_type_ids` passed when calling [`BertModel`] or [`TFBertModel`].
initializer_range (`float`, *optional*, defaults to 0.02):
The standard deviation of the truncated_normal_initializer for initializing all weight matrices.
layer_norm_eps (`float`, *optional*, defaults to 1e-12):
The epsilon used by the layer normalization layers.
position_embedding_type (`str`, *optional*, defaults to `"absolute"`):
Type of position embedding. Choose one of `"absolute"`, `"relative_key"`, `"relative_key_query"`. For
positional embeddings use `"absolute"`. For more information on `"relative_key"`, please refer to
[Self-Attention with Relative Position Representations (Shaw et al.)](https://arxiv.org/abs/1803.02155).
For more information on `"relative_key_query"`, please refer to *Method 4* in [Improve Transformer Models
with Better Relative Position Embeddings (Huang et al.)](https://arxiv.org/abs/2009.13658).
is_decoder (`bool`, *optional*, defaults to `False`):
Whether the model is used as a decoder or not. If `False`, the model is used as an encoder.
use_cache (`bool`, *optional*, defaults to `True`):
Whether or not the model should return the last key/values attentions (not used by all models). Only
relevant if `config.is_decoder=True`.
classifier_dropout (`float`, *optional*):
The dropout ratio for the classification head.
Examples:
```python
>>> from transformers import BertConfig, BertModel
>>> # Initializing a BERT bert-base-uncased style configuration
>>> configuration = BertConfig()
>>> # Initializing a model (with random weights) from the bert-base-uncased style configuration
>>> model = BertModel(configuration)
>>> # Accessing the model configuration
>>> configuration = model.config
```"""
model_type = "bert"
def __init__(
self,
vocab_size=30522,
hidden_size=768,
num_hidden_layers=12,
num_attention_heads=12,
intermediate_size=3072,
hidden_act="gelu",
hidden_dropout_prob=0.1,
attention_probs_dropout_prob=0.1,
max_position_embeddings=512,
type_vocab_size=2,
initializer_range=0.02,
layer_norm_eps=1e-12,
pad_token_id=0,
position_embedding_type="absolute",
use_cache=True,
classifier_dropout=None,
**kwargs,
):
super().__init__(pad_token_id=pad_token_id, **kwargs)
self.vocab_size = vocab_size
self.hidden_size = hidden_size
self.num_hidden_layers = num_hidden_layers
self.num_attention_heads = num_attention_heads
self.hidden_act = hidden_act
self.intermediate_size = intermediate_size
self.hidden_dropout_prob = hidden_dropout_prob
self.attention_probs_dropout_prob = attention_probs_dropout_prob
self.max_position_embeddings = max_position_embeddings
self.type_vocab_size = type_vocab_size
self.initializer_range = initializer_range
self.layer_norm_eps = layer_norm_eps
self.position_embedding_type = position_embedding_type
self.use_cache = use_cache
self.classifier_dropout = classifier_dropout
class BertOnnxConfig(OnnxConfig):
@property
def inputs(self) -> Mapping[str, Mapping[int, str]]:
if self.task == "multiple-choice":
dynamic_axis = {0: "batch", 1: "choice", 2: "sequence"}
else:
dynamic_axis = {0: "batch", 1: "sequence"}
return OrderedDict(
[
("input_ids", dynamic_axis),
("attention_mask", dynamic_axis),
("token_type_ids", dynamic_axis),
]
)
| transformers/src/transformers/models/bert/configuration_bert.py/0 | {
"file_path": "transformers/src/transformers/models/bert/configuration_bert.py",
"repo_id": "transformers",
"token_count": 4005
} | 311 |
# coding=utf-8
# Copyright 2018 The Google AI Language Team Authors and The HuggingFace Inc. team.
#
# Licensed under the Apache License, Version 2.0 (the "License");
# you may not use this file except in compliance with the License.
# You may obtain a copy of the License at
#
# http://www.apache.org/licenses/LICENSE-2.0
#
# Unless required by applicable law or agreed to in writing, software
# distributed under the License is distributed on an "AS IS" BASIS,
# WITHOUT WARRANTIES OR CONDITIONS OF ANY KIND, either express or implied.
# See the License for the specific language governing permissions and
# limitations under the License.
"""Tokenization classes."""
import collections
import copy
import os
import unicodedata
from typing import Any, Dict, List, Optional, Tuple
from ...tokenization_utils import PreTrainedTokenizer, _is_control, _is_punctuation, _is_whitespace
from ...utils import is_sentencepiece_available, logging
if is_sentencepiece_available():
import sentencepiece as spm
else:
spm = None
logger = logging.get_logger(__name__)
VOCAB_FILES_NAMES = {"vocab_file": "vocab.txt", "spm_file": "spiece.model"}
SPIECE_UNDERLINE = "โ"
PRETRAINED_VOCAB_FILES_MAP = {
"vocab_file": {
"cl-tohoku/bert-base-japanese": "https://huggingface.co/cl-tohoku/bert-base-japanese/resolve/main/vocab.txt",
"cl-tohoku/bert-base-japanese-whole-word-masking": (
"https://huggingface.co/cl-tohoku/bert-base-japanese-whole-word-masking/resolve/main/vocab.txt"
),
"cl-tohoku/bert-base-japanese-char": (
"https://huggingface.co/cl-tohoku/bert-base-japanese-char/resolve/main/vocab.txt"
),
"cl-tohoku/bert-base-japanese-char-whole-word-masking": (
"https://huggingface.co/cl-tohoku/bert-base-japanese-char-whole-word-masking/resolve/main/vocab.txt"
),
}
}
PRETRAINED_POSITIONAL_EMBEDDINGS_SIZES = {
"cl-tohoku/bert-base-japanese": 512,
"cl-tohoku/bert-base-japanese-whole-word-masking": 512,
"cl-tohoku/bert-base-japanese-char": 512,
"cl-tohoku/bert-base-japanese-char-whole-word-masking": 512,
}
PRETRAINED_INIT_CONFIGURATION = {
"cl-tohoku/bert-base-japanese": {
"do_lower_case": False,
"word_tokenizer_type": "mecab",
"subword_tokenizer_type": "wordpiece",
},
"cl-tohoku/bert-base-japanese-whole-word-masking": {
"do_lower_case": False,
"word_tokenizer_type": "mecab",
"subword_tokenizer_type": "wordpiece",
},
"cl-tohoku/bert-base-japanese-char": {
"do_lower_case": False,
"word_tokenizer_type": "mecab",
"subword_tokenizer_type": "character",
},
"cl-tohoku/bert-base-japanese-char-whole-word-masking": {
"do_lower_case": False,
"word_tokenizer_type": "mecab",
"subword_tokenizer_type": "character",
},
}
# Copied from transformers.models.bert.tokenization_bert.load_vocab
def load_vocab(vocab_file):
"""Loads a vocabulary file into a dictionary."""
vocab = collections.OrderedDict()
with open(vocab_file, "r", encoding="utf-8") as reader:
tokens = reader.readlines()
for index, token in enumerate(tokens):
token = token.rstrip("\n")
vocab[token] = index
return vocab
# Copied from transformers.models.bert.tokenization_bert.whitespace_tokenize
def whitespace_tokenize(text):
"""Runs basic whitespace cleaning and splitting on a piece of text."""
text = text.strip()
if not text:
return []
tokens = text.split()
return tokens
class BertJapaneseTokenizer(PreTrainedTokenizer):
r"""
Construct a BERT tokenizer for Japanese text.
This tokenizer inherits from [`PreTrainedTokenizer`] which contains most of the main methods. Users should refer
to: this superclass for more information regarding those methods.
Args:
vocab_file (`str`):
Path to a one-wordpiece-per-line vocabulary file.
spm_file (`str`, *optional*):
Path to [SentencePiece](https://github.com/google/sentencepiece) file (generally has a .spm or .model
extension) that contains the vocabulary.
do_lower_case (`bool`, *optional*, defaults to `True`):
Whether to lower case the input. Only has an effect when do_basic_tokenize=True.
do_word_tokenize (`bool`, *optional*, defaults to `True`):
Whether to do word tokenization.
do_subword_tokenize (`bool`, *optional*, defaults to `True`):
Whether to do subword tokenization.
word_tokenizer_type (`str`, *optional*, defaults to `"basic"`):
Type of word tokenizer. Choose from ["basic", "mecab", "sudachi", "jumanpp"].
subword_tokenizer_type (`str`, *optional*, defaults to `"wordpiece"`):
Type of subword tokenizer. Choose from ["wordpiece", "character", "sentencepiece",].
mecab_kwargs (`dict`, *optional*):
Dictionary passed to the `MecabTokenizer` constructor.
sudachi_kwargs (`dict`, *optional*):
Dictionary passed to the `SudachiTokenizer` constructor.
jumanpp_kwargs (`dict`, *optional*):
Dictionary passed to the `JumanppTokenizer` constructor.
"""
vocab_files_names = VOCAB_FILES_NAMES
pretrained_vocab_files_map = PRETRAINED_VOCAB_FILES_MAP
pretrained_init_configuration = PRETRAINED_INIT_CONFIGURATION
max_model_input_sizes = PRETRAINED_POSITIONAL_EMBEDDINGS_SIZES
def __init__(
self,
vocab_file,
spm_file=None,
do_lower_case=False,
do_word_tokenize=True,
do_subword_tokenize=True,
word_tokenizer_type="basic",
subword_tokenizer_type="wordpiece",
never_split=None,
unk_token="[UNK]",
sep_token="[SEP]",
pad_token="[PAD]",
cls_token="[CLS]",
mask_token="[MASK]",
mecab_kwargs=None,
sudachi_kwargs=None,
jumanpp_kwargs=None,
**kwargs,
):
if subword_tokenizer_type == "sentencepiece":
if not os.path.isfile(spm_file):
raise ValueError(
f"Can't find a vocabulary file at path '{spm_file}'. To load the vocabulary from a Google"
" pretrained model use `tokenizer = AutoTokenizer.from_pretrained(PRETRAINED_MODEL_NAME)`"
)
self.spm_file = spm_file
else:
if not os.path.isfile(vocab_file):
raise ValueError(
f"Can't find a vocabulary file at path '{vocab_file}'. To load the vocabulary from a Google"
" pretrained model use `tokenizer = AutoTokenizer.from_pretrained(PRETRAINED_MODEL_NAME)`"
)
self.vocab = load_vocab(vocab_file)
self.ids_to_tokens = collections.OrderedDict([(ids, tok) for tok, ids in self.vocab.items()])
self.do_word_tokenize = do_word_tokenize
self.word_tokenizer_type = word_tokenizer_type
self.lower_case = do_lower_case
self.never_split = never_split
self.mecab_kwargs = copy.deepcopy(mecab_kwargs)
self.sudachi_kwargs = copy.deepcopy(sudachi_kwargs)
self.jumanpp_kwargs = copy.deepcopy(jumanpp_kwargs)
if do_word_tokenize:
if word_tokenizer_type == "basic":
self.word_tokenizer = BasicTokenizer(
do_lower_case=do_lower_case, never_split=never_split, tokenize_chinese_chars=False
)
elif word_tokenizer_type == "mecab":
self.word_tokenizer = MecabTokenizer(
do_lower_case=do_lower_case, never_split=never_split, **(mecab_kwargs or {})
)
elif word_tokenizer_type == "sudachi":
self.word_tokenizer = SudachiTokenizer(
do_lower_case=do_lower_case, never_split=never_split, **(sudachi_kwargs or {})
)
elif word_tokenizer_type == "jumanpp":
self.word_tokenizer = JumanppTokenizer(
do_lower_case=do_lower_case, never_split=never_split, **(jumanpp_kwargs or {})
)
else:
raise ValueError(f"Invalid word_tokenizer_type '{word_tokenizer_type}' is specified.")
self.do_subword_tokenize = do_subword_tokenize
self.subword_tokenizer_type = subword_tokenizer_type
if do_subword_tokenize:
if subword_tokenizer_type == "wordpiece":
self.subword_tokenizer = WordpieceTokenizer(vocab=self.vocab, unk_token=str(unk_token))
elif subword_tokenizer_type == "character":
self.subword_tokenizer = CharacterTokenizer(vocab=self.vocab, unk_token=str(unk_token))
elif subword_tokenizer_type == "sentencepiece":
self.subword_tokenizer = SentencepieceTokenizer(vocab=self.spm_file, unk_token=str(unk_token))
else:
raise ValueError(f"Invalid subword_tokenizer_type '{subword_tokenizer_type}' is specified.")
super().__init__(
spm_file=spm_file,
unk_token=unk_token,
sep_token=sep_token,
pad_token=pad_token,
cls_token=cls_token,
mask_token=mask_token,
do_lower_case=do_lower_case,
do_word_tokenize=do_word_tokenize,
do_subword_tokenize=do_subword_tokenize,
word_tokenizer_type=word_tokenizer_type,
subword_tokenizer_type=subword_tokenizer_type,
never_split=never_split,
mecab_kwargs=mecab_kwargs,
sudachi_kwargs=sudachi_kwargs,
jumanpp_kwargs=jumanpp_kwargs,
**kwargs,
)
@property
def do_lower_case(self):
return self.lower_case
def __getstate__(self):
state = dict(self.__dict__)
if self.word_tokenizer_type in ["mecab", "sudachi", "jumanpp"]:
del state["word_tokenizer"]
return state
def __setstate__(self, state):
self.__dict__ = state
if self.word_tokenizer_type == "mecab":
self.word_tokenizer = MecabTokenizer(
do_lower_case=self.do_lower_case, never_split=self.never_split, **(self.mecab_kwargs or {})
)
elif self.word_tokenizer_type == "sudachi":
self.word_tokenizer = SudachiTokenizer(
do_lower_case=self.do_lower_case, never_split=self.never_split, **(self.sudachi_kwargs or {})
)
elif self.word_tokenizer_type == "jumanpp":
self.word_tokenizer = JumanppTokenizer(
do_lower_case=self.do_lower_case, never_split=self.never_split, **(self.jumanpp_kwargs or {})
)
def _tokenize(self, text):
if self.do_word_tokenize:
tokens = self.word_tokenizer.tokenize(text, never_split=self.all_special_tokens)
else:
tokens = [text]
if self.do_subword_tokenize:
split_tokens = [sub_token for token in tokens for sub_token in self.subword_tokenizer.tokenize(token)]
else:
split_tokens = tokens
return split_tokens
@property
def vocab_size(self):
if self.subword_tokenizer_type == "sentencepiece":
return len(self.subword_tokenizer.sp_model)
return len(self.vocab)
def get_vocab(self):
if self.subword_tokenizer_type == "sentencepiece":
vocab = {self.convert_ids_to_tokens(i): i for i in range(self.vocab_size)}
vocab.update(self.added_tokens_encoder)
return vocab
return dict(self.vocab, **self.added_tokens_encoder)
def _convert_token_to_id(self, token):
"""Converts a token (str) in an id using the vocab."""
if self.subword_tokenizer_type == "sentencepiece":
return self.subword_tokenizer.sp_model.PieceToId(token)
return self.vocab.get(token, self.vocab.get(self.unk_token))
def _convert_id_to_token(self, index):
"""Converts an index (integer) in a token (str) using the vocab."""
if self.subword_tokenizer_type == "sentencepiece":
return self.subword_tokenizer.sp_model.IdToPiece(index)
return self.ids_to_tokens.get(index, self.unk_token)
def convert_tokens_to_string(self, tokens):
"""Converts a sequence of tokens (string) in a single string."""
if self.subword_tokenizer_type == "sentencepiece":
return self.subword_tokenizer.sp_model.decode(tokens)
out_string = " ".join(tokens).replace(" ##", "").strip()
return out_string
# Copied from transformers.models.bert.tokenization_bert.BertTokenizer.build_inputs_with_special_tokens
def build_inputs_with_special_tokens(
self, token_ids_0: List[int], token_ids_1: Optional[List[int]] = None
) -> List[int]:
"""
Build model inputs from a sequence or a pair of sequence for sequence classification tasks by concatenating and
adding special tokens. A BERT sequence has the following format:
- single sequence: `[CLS] X [SEP]`
- pair of sequences: `[CLS] A [SEP] B [SEP]`
Args:
token_ids_0 (`List[int]`):
List of IDs to which the special tokens will be added.
token_ids_1 (`List[int]`, *optional*):
Optional second list of IDs for sequence pairs.
Returns:
`List[int]`: List of [input IDs](../glossary#input-ids) with the appropriate special tokens.
"""
if token_ids_1 is None:
return [self.cls_token_id] + token_ids_0 + [self.sep_token_id]
cls = [self.cls_token_id]
sep = [self.sep_token_id]
return cls + token_ids_0 + sep + token_ids_1 + sep
# Copied from transformers.models.bert.tokenization_bert.BertTokenizer.get_special_tokens_mask
def get_special_tokens_mask(
self, token_ids_0: List[int], token_ids_1: Optional[List[int]] = None, already_has_special_tokens: bool = False
) -> List[int]:
"""
Retrieve sequence ids from a token list that has no special tokens added. This method is called when adding
special tokens using the tokenizer `prepare_for_model` method.
Args:
token_ids_0 (`List[int]`):
List of IDs.
token_ids_1 (`List[int]`, *optional*):
Optional second list of IDs for sequence pairs.
already_has_special_tokens (`bool`, *optional*, defaults to `False`):
Whether or not the token list is already formatted with special tokens for the model.
Returns:
`List[int]`: A list of integers in the range [0, 1]: 1 for a special token, 0 for a sequence token.
"""
if already_has_special_tokens:
return super().get_special_tokens_mask(
token_ids_0=token_ids_0, token_ids_1=token_ids_1, already_has_special_tokens=True
)
if token_ids_1 is not None:
return [1] + ([0] * len(token_ids_0)) + [1] + ([0] * len(token_ids_1)) + [1]
return [1] + ([0] * len(token_ids_0)) + [1]
# Copied from transformers.models.bert.tokenization_bert.BertTokenizer.create_token_type_ids_from_sequences
def create_token_type_ids_from_sequences(
self, token_ids_0: List[int], token_ids_1: Optional[List[int]] = None
) -> List[int]:
"""
Create a mask from the two sequences passed to be used in a sequence-pair classification task. A BERT sequence
pair mask has the following format:
```
0 0 0 0 0 0 0 0 0 0 0 1 1 1 1 1 1 1 1 1
| first sequence | second sequence |
```
If `token_ids_1` is `None`, this method only returns the first portion of the mask (0s).
Args:
token_ids_0 (`List[int]`):
List of IDs.
token_ids_1 (`List[int]`, *optional*):
Optional second list of IDs for sequence pairs.
Returns:
`List[int]`: List of [token type IDs](../glossary#token-type-ids) according to the given sequence(s).
"""
sep = [self.sep_token_id]
cls = [self.cls_token_id]
if token_ids_1 is None:
return len(cls + token_ids_0 + sep) * [0]
return len(cls + token_ids_0 + sep) * [0] + len(token_ids_1 + sep) * [1]
def save_vocabulary(self, save_directory: str, filename_prefix: Optional[str] = None) -> Tuple[str]:
if os.path.isdir(save_directory):
if self.subword_tokenizer_type == "sentencepiece":
vocab_file = os.path.join(
save_directory, (filename_prefix + "-" if filename_prefix else "") + VOCAB_FILES_NAMES["spm_file"]
)
else:
vocab_file = os.path.join(
save_directory,
(filename_prefix + "-" if filename_prefix else "") + VOCAB_FILES_NAMES["vocab_file"],
)
else:
vocab_file = (filename_prefix + "-" if filename_prefix else "") + save_directory
if self.subword_tokenizer_type == "sentencepiece":
with open(vocab_file, "wb") as writer:
content_spiece_model = self.subword_tokenizer.sp_model.serialized_model_proto()
writer.write(content_spiece_model)
else:
with open(vocab_file, "w", encoding="utf-8") as writer:
index = 0
for token, token_index in sorted(self.vocab.items(), key=lambda kv: kv[1]):
if index != token_index:
logger.warning(
f"Saving vocabulary to {vocab_file}: vocabulary indices are not consecutive."
" Please check that the vocabulary is not corrupted!"
)
index = token_index
writer.write(token + "\n")
index += 1
return (vocab_file,)
class MecabTokenizer:
"""Runs basic tokenization with MeCab morphological parser."""
def __init__(
self,
do_lower_case=False,
never_split=None,
normalize_text=True,
mecab_dic: Optional[str] = "ipadic",
mecab_option: Optional[str] = None,
):
"""
Constructs a MecabTokenizer.
Args:
**do_lower_case**: (*optional*) boolean (default True)
Whether to lowercase the input.
**never_split**: (*optional*) list of str
Kept for backward compatibility purposes. Now implemented directly at the base class level (see
[`PreTrainedTokenizer.tokenize`]) List of tokens not to split.
**normalize_text**: (*optional*) boolean (default True)
Whether to apply unicode normalization to text before tokenization.
**mecab_dic**: (*optional*) string (default "ipadic")
Name of dictionary to be used for MeCab initialization. If you are using a system-installed dictionary,
set this option to `None` and modify *mecab_option*.
**mecab_option**: (*optional*) string
String passed to MeCab constructor.
"""
self.do_lower_case = do_lower_case
self.never_split = never_split if never_split is not None else []
self.normalize_text = normalize_text
try:
import fugashi
except ModuleNotFoundError as error:
raise error.__class__(
"You need to install fugashi to use MecabTokenizer. "
"See https://pypi.org/project/fugashi/ for installation."
)
mecab_option = mecab_option or ""
if mecab_dic is not None:
if mecab_dic == "ipadic":
try:
import ipadic
except ModuleNotFoundError as error:
raise error.__class__(
"The ipadic dictionary is not installed. "
"See https://github.com/polm/ipadic-py for installation."
)
dic_dir = ipadic.DICDIR
elif mecab_dic == "unidic_lite":
try:
import unidic_lite
except ModuleNotFoundError as error:
raise error.__class__(
"The unidic_lite dictionary is not installed. "
"See https://github.com/polm/unidic-lite for installation."
)
dic_dir = unidic_lite.DICDIR
elif mecab_dic == "unidic":
try:
import unidic
except ModuleNotFoundError as error:
raise error.__class__(
"The unidic dictionary is not installed. "
"See https://github.com/polm/unidic-py for installation."
)
dic_dir = unidic.DICDIR
if not os.path.isdir(dic_dir):
raise RuntimeError(
"The unidic dictionary itself is not found. "
"See https://github.com/polm/unidic-py for installation."
)
else:
raise ValueError("Invalid mecab_dic is specified.")
mecabrc = os.path.join(dic_dir, "mecabrc")
mecab_option = f'-d "{dic_dir}" -r "{mecabrc}" ' + mecab_option
self.mecab = fugashi.GenericTagger(mecab_option)
def tokenize(self, text, never_split=None, **kwargs):
"""Tokenizes a piece of text."""
if self.normalize_text:
text = unicodedata.normalize("NFKC", text)
never_split = self.never_split + (never_split if never_split is not None else [])
tokens = []
for word in self.mecab(text):
token = word.surface
if self.do_lower_case and token not in never_split:
token = token.lower()
tokens.append(token)
return tokens
class SudachiTokenizer:
"""Runs basic tokenization with Sudachi morphological parser."""
def __init__(
self,
do_lower_case=False,
never_split=None,
normalize_text=True,
trim_whitespace=False,
sudachi_split_mode="A",
sudachi_config_path=None,
sudachi_resource_dir=None,
sudachi_dict_type="core",
):
"""
Constructs a SudachiTokenizer.
Args:
**do_lower_case**: (*optional*) boolean (default True)
Whether to lowercase the input.
**never_split**: (*optional*) list of str
Kept for backward compatibility purposes. Now implemented directly at the base class level (see
[`PreTrainedTokenizer.tokenize`]) List of tokens not to split.
**normalize_text**: (*optional*) boolean (default True)
Whether to apply unicode normalization to text before tokenization.
**trim_whitespace**: (*optional*) boolean (default False)
Whether to trim all whitespace, tab, newline from tokens.
**sudachi_split_mode**: (*optional*) string
Split mode of sudachi, choose from "A", "B", "C".
**sudachi_config_path**: (*optional*) string
**sudachi_resource_dir**: (*optional*) string
**sudachi_dict_type**: (*optional*) string
dict type of sudachi, choose from "small", "core", "full".
"""
self.do_lower_case = do_lower_case
self.never_split = never_split if never_split is not None else []
self.normalize_text = normalize_text
self.trim_whitespace = trim_whitespace
try:
from sudachipy import dictionary, tokenizer
except ImportError:
raise ImportError(
"You need to install sudachipy to use SudachiTokenizer. "
"See https://github.com/WorksApplications/SudachiPy for installation."
)
if sudachi_split_mode == "A":
self.split_mode = tokenizer.Tokenizer.SplitMode.A
elif sudachi_split_mode == "B":
self.split_mode = tokenizer.Tokenizer.SplitMode.B
elif sudachi_split_mode == "C":
self.split_mode = tokenizer.Tokenizer.SplitMode.C
else:
raise ValueError("Invalid sudachi_split_mode is specified.")
self.sudachi = dictionary.Dictionary(
config_path=sudachi_config_path, resource_dir=sudachi_resource_dir, dict=sudachi_dict_type
).create(self.split_mode)
def tokenize(self, text, never_split=None, **kwargs):
"""Tokenizes a piece of text."""
if self.normalize_text:
text = unicodedata.normalize("NFKC", text)
never_split = self.never_split + (never_split if never_split is not None else [])
tokens = []
for word in self.sudachi.tokenize(text):
token = word.surface()
if self.do_lower_case and token not in never_split:
token = token.lower()
if self.trim_whitespace:
if token.strip() == "":
continue
else:
token = token.strip()
tokens.append(token)
return tokens
class JumanppTokenizer:
"""Runs basic tokenization with jumanpp morphological parser."""
def __init__(
self,
do_lower_case=False,
never_split=None,
normalize_text=True,
trim_whitespace=False,
):
"""
Constructs a JumanppTokenizer.
Args:
**do_lower_case**: (*optional*) boolean (default True)
Whether to lowercase the input.
**never_split**: (*optional*) list of str
Kept for backward compatibility purposes. Now implemented directly at the base class level (see
[`PreTrainedTokenizer.tokenize`]) List of tokens not to split.
**normalize_text**: (*optional*) boolean (default True)
Whether to apply unicode normalization to text before tokenization.
**trim_whitespace**: (*optional*) boolean (default False)
Whether to trim all whitespace, tab, newline from tokens.
"""
self.do_lower_case = do_lower_case
self.never_split = never_split if never_split is not None else []
self.normalize_text = normalize_text
self.trim_whitespace = trim_whitespace
try:
import rhoknp
except ImportError:
raise ImportError(
"You need to install rhoknp to use JumanppTokenizer. "
"See https://github.com/ku-nlp/rhoknp for installation."
)
self.juman = rhoknp.Jumanpp()
def tokenize(self, text, never_split=None, **kwargs):
"""Tokenizes a piece of text."""
if self.normalize_text:
text = unicodedata.normalize("NFKC", text)
text = text.strip()
never_split = self.never_split + (never_split if never_split is not None else [])
tokens = []
for mrph in self.juman.apply_to_sentence(text).morphemes:
token = mrph.text
if self.do_lower_case and token not in never_split:
token = token.lower()
if self.trim_whitespace:
if token.strip() == "":
continue
else:
token = token.strip()
tokens.append(token)
return tokens
class CharacterTokenizer:
"""Runs Character tokenization."""
def __init__(self, vocab, unk_token, normalize_text=True):
"""
Constructs a CharacterTokenizer.
Args:
**vocab**:
Vocabulary object.
**unk_token**: str
A special symbol for out-of-vocabulary token.
**normalize_text**: (`optional`) boolean (default True)
Whether to apply unicode normalization to text before tokenization.
"""
self.vocab = vocab
self.unk_token = unk_token
self.normalize_text = normalize_text
def tokenize(self, text):
"""
Tokenizes a piece of text into characters.
For example, `input = "apple""` wil return as output `["a", "p", "p", "l", "e"]`.
Args:
text: A single token or whitespace separated tokens.
This should have already been passed through *BasicTokenizer*.
Returns:
A list of characters.
"""
if self.normalize_text:
text = unicodedata.normalize("NFKC", text)
output_tokens = []
for char in text:
if char not in self.vocab:
output_tokens.append(self.unk_token)
continue
output_tokens.append(char)
return output_tokens
# Copied from transformers.models.bert.tokenization_bert.BasicTokenizer
class BasicTokenizer(object):
"""
Constructs a BasicTokenizer that will run basic tokenization (punctuation splitting, lower casing, etc.).
Args:
do_lower_case (`bool`, *optional*, defaults to `True`):
Whether or not to lowercase the input when tokenizing.
never_split (`Iterable`, *optional*):
Collection of tokens which will never be split during tokenization. Only has an effect when
`do_basic_tokenize=True`
tokenize_chinese_chars (`bool`, *optional*, defaults to `True`):
Whether or not to tokenize Chinese characters.
This should likely be deactivated for Japanese (see this
[issue](https://github.com/huggingface/transformers/issues/328)).
strip_accents (`bool`, *optional*):
Whether or not to strip all accents. If this option is not specified, then it will be determined by the
value for `lowercase` (as in the original BERT).
do_split_on_punc (`bool`, *optional*, defaults to `True`):
In some instances we want to skip the basic punctuation splitting so that later tokenization can capture
the full context of the words, such as contractions.
"""
def __init__(
self,
do_lower_case=True,
never_split=None,
tokenize_chinese_chars=True,
strip_accents=None,
do_split_on_punc=True,
):
if never_split is None:
never_split = []
self.do_lower_case = do_lower_case
self.never_split = set(never_split)
self.tokenize_chinese_chars = tokenize_chinese_chars
self.strip_accents = strip_accents
self.do_split_on_punc = do_split_on_punc
def tokenize(self, text, never_split=None):
"""
Basic Tokenization of a piece of text. For sub-word tokenization, see WordPieceTokenizer.
Args:
never_split (`List[str]`, *optional*)
Kept for backward compatibility purposes. Now implemented directly at the base class level (see
[`PreTrainedTokenizer.tokenize`]) List of token not to split.
"""
# union() returns a new set by concatenating the two sets.
never_split = self.never_split.union(set(never_split)) if never_split else self.never_split
text = self._clean_text(text)
# This was added on November 1st, 2018 for the multilingual and Chinese
# models. This is also applied to the English models now, but it doesn't
# matter since the English models were not trained on any Chinese data
# and generally don't have any Chinese data in them (there are Chinese
# characters in the vocabulary because Wikipedia does have some Chinese
# words in the English Wikipedia.).
if self.tokenize_chinese_chars:
text = self._tokenize_chinese_chars(text)
# prevents treating the same character with different unicode codepoints as different characters
unicode_normalized_text = unicodedata.normalize("NFC", text)
orig_tokens = whitespace_tokenize(unicode_normalized_text)
split_tokens = []
for token in orig_tokens:
if token not in never_split:
if self.do_lower_case:
token = token.lower()
if self.strip_accents is not False:
token = self._run_strip_accents(token)
elif self.strip_accents:
token = self._run_strip_accents(token)
split_tokens.extend(self._run_split_on_punc(token, never_split))
output_tokens = whitespace_tokenize(" ".join(split_tokens))
return output_tokens
def _run_strip_accents(self, text):
"""Strips accents from a piece of text."""
text = unicodedata.normalize("NFD", text)
output = []
for char in text:
cat = unicodedata.category(char)
if cat == "Mn":
continue
output.append(char)
return "".join(output)
def _run_split_on_punc(self, text, never_split=None):
"""Splits punctuation on a piece of text."""
if not self.do_split_on_punc or (never_split is not None and text in never_split):
return [text]
chars = list(text)
i = 0
start_new_word = True
output = []
while i < len(chars):
char = chars[i]
if _is_punctuation(char):
output.append([char])
start_new_word = True
else:
if start_new_word:
output.append([])
start_new_word = False
output[-1].append(char)
i += 1
return ["".join(x) for x in output]
def _tokenize_chinese_chars(self, text):
"""Adds whitespace around any CJK character."""
output = []
for char in text:
cp = ord(char)
if self._is_chinese_char(cp):
output.append(" ")
output.append(char)
output.append(" ")
else:
output.append(char)
return "".join(output)
def _is_chinese_char(self, cp):
"""Checks whether CP is the codepoint of a CJK character."""
# This defines a "chinese character" as anything in the CJK Unicode block:
# https://en.wikipedia.org/wiki/CJK_Unified_Ideographs_(Unicode_block)
#
# Note that the CJK Unicode block is NOT all Japanese and Korean characters,
# despite its name. The modern Korean Hangul alphabet is a different block,
# as is Japanese Hiragana and Katakana. Those alphabets are used to write
# space-separated words, so they are not treated specially and handled
# like the all of the other languages.
if (
(cp >= 0x4E00 and cp <= 0x9FFF)
or (cp >= 0x3400 and cp <= 0x4DBF) #
or (cp >= 0x20000 and cp <= 0x2A6DF) #
or (cp >= 0x2A700 and cp <= 0x2B73F) #
or (cp >= 0x2B740 and cp <= 0x2B81F) #
or (cp >= 0x2B820 and cp <= 0x2CEAF) #
or (cp >= 0xF900 and cp <= 0xFAFF)
or (cp >= 0x2F800 and cp <= 0x2FA1F) #
): #
return True
return False
def _clean_text(self, text):
"""Performs invalid character removal and whitespace cleanup on text."""
output = []
for char in text:
cp = ord(char)
if cp == 0 or cp == 0xFFFD or _is_control(char):
continue
if _is_whitespace(char):
output.append(" ")
else:
output.append(char)
return "".join(output)
# Copied from transformers.models.bert.tokenization_bert.WordpieceTokenizer
class WordpieceTokenizer(object):
"""Runs WordPiece tokenization."""
def __init__(self, vocab, unk_token, max_input_chars_per_word=100):
self.vocab = vocab
self.unk_token = unk_token
self.max_input_chars_per_word = max_input_chars_per_word
def tokenize(self, text):
"""
Tokenizes a piece of text into its word pieces. This uses a greedy longest-match-first algorithm to perform
tokenization using the given vocabulary.
For example, `input = "unaffable"` wil return as output `["un", "##aff", "##able"]`.
Args:
text: A single token or whitespace separated tokens. This should have
already been passed through *BasicTokenizer*.
Returns:
A list of wordpiece tokens.
"""
output_tokens = []
for token in whitespace_tokenize(text):
chars = list(token)
if len(chars) > self.max_input_chars_per_word:
output_tokens.append(self.unk_token)
continue
is_bad = False
start = 0
sub_tokens = []
while start < len(chars):
end = len(chars)
cur_substr = None
while start < end:
substr = "".join(chars[start:end])
if start > 0:
substr = "##" + substr
if substr in self.vocab:
cur_substr = substr
break
end -= 1
if cur_substr is None:
is_bad = True
break
sub_tokens.append(cur_substr)
start = end
if is_bad:
output_tokens.append(self.unk_token)
else:
output_tokens.extend(sub_tokens)
return output_tokens
class SentencepieceTokenizer(object):
"""
Runs sentencepiece tokenization. Based on transformers.models.albert.tokenization_albert.AlbertTokenizer.
"""
def __init__(
self,
vocab,
unk_token,
do_lower_case=False,
remove_space=True,
keep_accents=True,
sp_model_kwargs: Optional[Dict[str, Any]] = None,
):
self.vocab = vocab
self.unk_token = unk_token
self.do_lower_case = do_lower_case
self.remove_space = remove_space
self.keep_accents = keep_accents
self.sp_model_kwargs = {} if sp_model_kwargs is None else sp_model_kwargs
self.sp_model = spm.SentencePieceProcessor(**self.sp_model_kwargs)
self.sp_model.Load(self.vocab)
def preprocess_text(self, inputs):
if self.remove_space:
outputs = " ".join(inputs.strip().split())
else:
outputs = inputs
outputs = outputs.replace("``", '"').replace("''", '"')
if not self.keep_accents:
outputs = unicodedata.normalize("NFKD", outputs)
outputs = "".join([c for c in outputs if not unicodedata.combining(c)])
if self.do_lower_case:
outputs = outputs.lower()
return outputs
def tokenize(self, text):
"""
Tokenizes text by sentencepiece. Based on [SentencePiece](https://github.com/google/sentencepiece).
Tokenization needs the given vocabulary.
Args:
text: A string needs to be tokenized.
Returns:
A list of sentencepiece tokens.
"""
text = self.preprocess_text(text)
pieces = self.sp_model.encode(text, out_type=str)
new_pieces = []
for piece in pieces:
if len(piece) > 1 and piece[-1] == str(",") and piece[-2].isdigit():
cur_pieces = self.sp_model.EncodeAsPieces(piece[:-1].replace(SPIECE_UNDERLINE, ""))
if piece[0] != SPIECE_UNDERLINE and cur_pieces[0][0] == SPIECE_UNDERLINE:
if len(cur_pieces[0]) == 1:
cur_pieces = cur_pieces[1:]
else:
cur_pieces[0] = cur_pieces[0][1:]
cur_pieces.append(piece[-1])
new_pieces.extend(cur_pieces)
else:
new_pieces.append(piece)
return new_pieces
| transformers/src/transformers/models/bert_japanese/tokenization_bert_japanese.py/0 | {
"file_path": "transformers/src/transformers/models/bert_japanese/tokenization_bert_japanese.py",
"repo_id": "transformers",
"token_count": 18811
} | 312 |
# coding=utf-8
# Copyright 2022 The HuggingFace Inc. team.
#
# Licensed under the Apache License, Version 2.0 (the "License");
# you may not use this file except in compliance with the License.
# You may obtain a copy of the License at
#
# http://www.apache.org/licenses/LICENSE-2.0
#
# Unless required by applicable law or agreed to in writing, software
# distributed under the License is distributed on an "AS IS" BASIS,
# WITHOUT WARRANTIES OR CONDITIONS OF ANY KIND, either express or implied.
# See the License for the specific language governing permissions and
# limitations under the License.
import argparse
import json
import os
import re
import shutil
import torch
from transformers import BioGptConfig, BioGptForCausalLM
from transformers.models.biogpt.tokenization_biogpt import VOCAB_FILES_NAMES
from transformers.tokenization_utils_base import TOKENIZER_CONFIG_FILE
from transformers.utils import WEIGHTS_NAME, logging
logging.set_verbosity_warning()
json_indent = 2
# modified from https://github.com/facebookresearch/fairseq/blob/dd74992d0d143155998e9ed4076826bcea80fb06/fairseq/data/dictionary.py#L18
class Dictionary:
"""A mapping from symbols to consecutive integers"""
def __init__(
self,
*, # begin keyword-only arguments
bos="<s>",
pad="<pad>",
eos="</s>",
unk="<unk>",
extra_special_symbols=None,
):
self.bos_word, self.unk_word, self.pad_word, self.eos_word = bos, unk, pad, eos
self.symbols = []
self.count = []
self.indices = {}
self.bos_index = self.add_symbol(bos)
self.pad_index = self.add_symbol(pad)
self.eos_index = self.add_symbol(eos)
self.unk_index = self.add_symbol(unk)
if extra_special_symbols:
for s in extra_special_symbols:
self.add_symbol(s)
self.nspecial = len(self.symbols)
def __eq__(self, other):
return self.indices == other.indices
def __getitem__(self, idx):
if idx < len(self.symbols):
return self.symbols[idx]
return self.unk_word
def __len__(self):
"""Returns the number of symbols in the dictionary"""
return len(self.symbols)
def __contains__(self, sym):
return sym in self.indices
@classmethod
def load(cls, f):
"""Loads the dictionary from a text file with the format:
```
<symbol0> <count0>
<symbol1> <count1>
...
```
"""
d = cls()
d.add_from_file(f)
return d
def add_symbol(self, word, n=1, overwrite=False):
"""Adds a word to the dictionary"""
if word in self.indices and not overwrite:
idx = self.indices[word]
self.count[idx] = self.count[idx] + n
return idx
else:
idx = len(self.symbols)
self.indices[word] = idx
self.symbols.append(word)
self.count.append(n)
return idx
def _load_meta(self, lines):
return 0
def add_from_file(self, f):
"""
Loads a pre-existing dictionary from a text file and adds its symbols to this instance.
"""
if isinstance(f, str):
try:
with open(f, "r", encoding="utf-8") as fd:
self.add_from_file(fd)
except FileNotFoundError as fnfe:
raise fnfe
except UnicodeError:
raise Exception("Incorrect encoding detected in {}, please rebuild the dataset".format(f))
return
lines = f.readlines()
indices_start_line = self._load_meta(lines)
for line in lines[indices_start_line:]:
try:
line, field = line.rstrip().rsplit(" ", 1)
if field == "#fairseq:overwrite":
overwrite = True
line, field = line.rsplit(" ", 1)
else:
overwrite = False
count = int(field)
word = line
if word in self and not overwrite:
raise RuntimeError(
"Duplicate word found when loading Dictionary: '{}'. "
"Duplicate words can overwrite earlier ones by adding the "
"#fairseq:overwrite flag at the end of the corresponding row "
"in the dictionary file. If using the Camembert model, please "
"download an updated copy of the model file.".format(word)
)
self.add_symbol(word, n=count, overwrite=overwrite)
except ValueError:
raise ValueError("Incorrect dictionary format, expected '<token> <cnt> [flags]'")
def rewrite_dict_keys(d):
# (1) remove word breaking symbol, (2) add word ending symbol where the word is not broken up,
# e.g.: d = {'le@@': 5, 'tt@@': 6, 'er': 7} => {'le': 5, 'tt': 6, 'er</w>': 7}
d2 = dict((re.sub(r"@@$", "", k), v) if k.endswith("@@") else (re.sub(r"$", "</w>", k), v) for k, v in d.items())
keep_keys = "<s> <pad> </s> <unk>".split()
# restore the special tokens
for k in keep_keys:
del d2[f"{k}</w>"]
d2[k] = d[k] # restore
return d2
def convert_biogpt_checkpoint_to_pytorch(biogpt_checkpoint_path, pytorch_dump_folder_path):
# prep
if not os.path.exists(biogpt_checkpoint_path):
raise ValueError(f"path {biogpt_checkpoint_path} does not exist!")
os.makedirs(pytorch_dump_folder_path, exist_ok=True)
print(f"Writing results to {pytorch_dump_folder_path}")
# handle various types of models
checkpoint_file = os.path.join(biogpt_checkpoint_path, "checkpoint.pt")
if not os.path.isfile(checkpoint_file):
raise ValueError(f"path to the file {checkpoint_file} does not exist!")
chkpt = torch.load(checkpoint_file, map_location="cpu")
args = chkpt["cfg"]["model"]
# dicts
dict_file = os.path.join(biogpt_checkpoint_path, "dict.txt")
if not os.path.isfile(dict_file):
raise ValueError(f"path to the file {dict_file} does not exist!")
src_dict = Dictionary.load(dict_file)
src_vocab = rewrite_dict_keys(src_dict.indices)
src_vocab_size = len(src_vocab)
src_vocab_file = os.path.join(pytorch_dump_folder_path, VOCAB_FILES_NAMES["vocab_file"])
print(f"Generating {src_vocab_file} of {src_vocab_size} records")
with open(src_vocab_file, "w", encoding="utf-8") as f:
f.write(json.dumps(src_vocab, ensure_ascii=False, indent=json_indent))
# merges_file (bpecodes)
bpecodes_file = os.path.join(biogpt_checkpoint_path, "bpecodes")
if not os.path.isfile(bpecodes_file):
raise ValueError(f"path to the file {bpecodes_file} does not exist!")
merges_file = os.path.join(pytorch_dump_folder_path, VOCAB_FILES_NAMES["merges_file"])
shutil.copyfile(bpecodes_file, merges_file)
# model config
biogpt_model_config_file = os.path.join(pytorch_dump_folder_path, "config.json")
model_conf = {
"activation_dropout": args["activation_dropout"],
"architectures": ["BioGptForCausalLM"],
"attention_probs_dropout_prob": args["attention_dropout"],
"bos_token_id": 0,
"eos_token_id": 2,
"hidden_act": args["activation_fn"],
"hidden_dropout_prob": args["dropout"],
"hidden_size": args["decoder_embed_dim"],
"initializer_range": 0.02,
"intermediate_size": args["decoder_ffn_embed_dim"],
"layer_norm_eps": 1e-12,
"layerdrop": args["decoder_layerdrop"],
"max_position_embeddings": args["max_target_positions"],
"model_type": "biogpt",
"num_attention_heads": args["decoder_attention_heads"],
"num_hidden_layers": args["decoder_layers"],
"pad_token_id": 1,
"scale_embedding": not args["no_scale_embedding"],
"tie_word_embeddings": args["share_decoder_input_output_embed"],
"vocab_size": src_vocab_size,
}
# good hparam defaults to start with
print(f"Generating {biogpt_model_config_file}")
with open(biogpt_model_config_file, "w", encoding="utf-8") as f:
f.write(json.dumps(model_conf, ensure_ascii=False, indent=json_indent))
# tokenizer config
biogpt_tokenizer_config_file = os.path.join(pytorch_dump_folder_path, TOKENIZER_CONFIG_FILE)
tokenizer_conf = {
"bos_token": "<s>",
"eos_token": "</s>",
"model_max_length": 1024,
"pad_token": "<pad>",
"special_tokens_map_file": None,
"tokenizer_class": "BioGptTokenizer",
"unk_token": "<unk>",
}
print(f"Generating {biogpt_tokenizer_config_file}")
with open(biogpt_tokenizer_config_file, "w", encoding="utf-8") as f:
f.write(json.dumps(tokenizer_conf, ensure_ascii=False, indent=json_indent))
# model
model_state_dict = chkpt["model"]
# remove unneeded keys
ignore_keys = [
"decoder.version",
]
for k in ignore_keys:
model_state_dict.pop(k, None)
layer_names = list(model_state_dict.keys())
for layer_name in layer_names:
if layer_name.endswith("output_projection.weight"):
model_state_dict[layer_name.replace("decoder.", "")] = model_state_dict.pop(layer_name)
else:
model_state_dict[layer_name.replace("decoder", "biogpt")] = model_state_dict.pop(layer_name)
config = BioGptConfig.from_pretrained(pytorch_dump_folder_path)
model_new = BioGptForCausalLM(config)
# check that it loads ok
model_new.load_state_dict(model_state_dict)
# save
pytorch_weights_dump_path = os.path.join(pytorch_dump_folder_path, WEIGHTS_NAME)
print(f"Generating {pytorch_weights_dump_path}")
torch.save(model_state_dict, pytorch_weights_dump_path)
print("Conversion is done!")
if __name__ == "__main__":
parser = argparse.ArgumentParser()
# Required parameters
parser.add_argument(
"--biogpt_checkpoint_path",
default=None,
type=str,
required=True,
help=(
"Path to the official PyTorch checkpoint file which is expected to reside in the dump dir with dicts,"
" bpecodes, etc."
),
)
parser.add_argument(
"--pytorch_dump_folder_path", default=None, type=str, required=True, help="Path to the output PyTorch model."
)
args = parser.parse_args()
convert_biogpt_checkpoint_to_pytorch(args.biogpt_checkpoint_path, args.pytorch_dump_folder_path)
| transformers/src/transformers/models/biogpt/convert_biogpt_original_pytorch_checkpoint_to_pytorch.py/0 | {
"file_path": "transformers/src/transformers/models/biogpt/convert_biogpt_original_pytorch_checkpoint_to_pytorch.py",
"repo_id": "transformers",
"token_count": 4719
} | 313 |
# Copyright 2023 The HuggingFace Team. All rights reserved.
#
# Licensed under the Apache License, Version 2.0 (the "License");
# you may not use this file except in compliance with the License.
# You may obtain a copy of the License at
#
# http://www.apache.org/licenses/LICENSE-2.0
#
# Unless required by applicable law or agreed to in writing, software
# distributed under the License is distributed on an "AS IS" BASIS,
# WITHOUT WARRANTIES OR CONDITIONS OF ANY KIND, either express or implied.
# See the License for the specific language governing permissions and
# limitations under the License.
from typing import TYPE_CHECKING
from ...utils import OptionalDependencyNotAvailable, _LazyModule, is_torch_available
_import_structure = {
"configuration_blip_2": [
"BLIP_2_PRETRAINED_CONFIG_ARCHIVE_MAP",
"Blip2Config",
"Blip2QFormerConfig",
"Blip2VisionConfig",
],
"processing_blip_2": ["Blip2Processor"],
}
try:
if not is_torch_available():
raise OptionalDependencyNotAvailable()
except OptionalDependencyNotAvailable:
pass
else:
_import_structure["modeling_blip_2"] = [
"BLIP_2_PRETRAINED_MODEL_ARCHIVE_LIST",
"Blip2Model",
"Blip2QFormerModel",
"Blip2PreTrainedModel",
"Blip2ForConditionalGeneration",
"Blip2VisionModel",
]
if TYPE_CHECKING:
from .configuration_blip_2 import (
BLIP_2_PRETRAINED_CONFIG_ARCHIVE_MAP,
Blip2Config,
Blip2QFormerConfig,
Blip2VisionConfig,
)
from .processing_blip_2 import Blip2Processor
try:
if not is_torch_available():
raise OptionalDependencyNotAvailable()
except OptionalDependencyNotAvailable:
pass
else:
from .modeling_blip_2 import (
BLIP_2_PRETRAINED_MODEL_ARCHIVE_LIST,
Blip2ForConditionalGeneration,
Blip2Model,
Blip2PreTrainedModel,
Blip2QFormerModel,
Blip2VisionModel,
)
else:
import sys
sys.modules[__name__] = _LazyModule(__name__, globals()["__file__"], _import_structure, module_spec=__spec__)
| transformers/src/transformers/models/blip_2/__init__.py/0 | {
"file_path": "transformers/src/transformers/models/blip_2/__init__.py",
"repo_id": "transformers",
"token_count": 887
} | 314 |
# coding=utf-8
# Copyright 2021 The HuggingFace Inc. team.
#
# Licensed under the Apache License, Version 2.0 (the "License");
# you may not use this file except in compliance with the License.
# You may obtain a copy of the License at
#
# http://www.apache.org/licenses/LICENSE-2.0
#
# Unless required by applicable law or agreed to in writing, software
# distributed under the License is distributed on an "AS IS" BASIS,
# WITHOUT WARRANTIES OR CONDITIONS OF ANY KIND, either express or implied.
# See the License for the specific language governing permissions and
# limitations under the License.
"""Convert CANINE checkpoint."""
import argparse
from transformers import CanineConfig, CanineModel, CanineTokenizer, load_tf_weights_in_canine
from transformers.utils import logging
logging.set_verbosity_info()
def convert_tf_checkpoint_to_pytorch(tf_checkpoint_path, pytorch_dump_path):
# Initialize PyTorch model
config = CanineConfig()
model = CanineModel(config)
model.eval()
print(f"Building PyTorch model from configuration: {config}")
# Load weights from tf checkpoint
load_tf_weights_in_canine(model, config, tf_checkpoint_path)
# Save pytorch-model (weights and configuration)
print(f"Save PyTorch model to {pytorch_dump_path}")
model.save_pretrained(pytorch_dump_path)
# Save tokenizer files
tokenizer = CanineTokenizer()
print(f"Save tokenizer files to {pytorch_dump_path}")
tokenizer.save_pretrained(pytorch_dump_path)
if __name__ == "__main__":
parser = argparse.ArgumentParser()
# Required parameters
parser.add_argument(
"--tf_checkpoint_path",
default=None,
type=str,
required=True,
help="Path to the TensorFlow checkpoint. Should end with model.ckpt",
)
parser.add_argument(
"--pytorch_dump_path",
default=None,
type=str,
required=True,
help="Path to a folder where the PyTorch model will be placed.",
)
args = parser.parse_args()
convert_tf_checkpoint_to_pytorch(args.tf_checkpoint_path, args.pytorch_dump_path)
| transformers/src/transformers/models/canine/convert_canine_original_tf_checkpoint_to_pytorch.py/0 | {
"file_path": "transformers/src/transformers/models/canine/convert_canine_original_tf_checkpoint_to_pytorch.py",
"repo_id": "transformers",
"token_count": 742
} | 315 |
# Copyright 2021 The HuggingFace Team. All rights reserved.
#
# Licensed under the Apache License, Version 2.0 (the "License");
# you may not use this file except in compliance with the License.
# You may obtain a copy of the License at
#
# http://www.apache.org/licenses/LICENSE-2.0
#
# Unless required by applicable law or agreed to in writing, software
# distributed under the License is distributed on an "AS IS" BASIS,
# WITHOUT WARRANTIES OR CONDITIONS OF ANY KIND, either express or implied.
# See the License for the specific language governing permissions and
# limitations under the License.
from typing import TYPE_CHECKING
from ...utils import (
OptionalDependencyNotAvailable,
_LazyModule,
is_flax_available,
is_tf_available,
is_tokenizers_available,
is_torch_available,
is_vision_available,
)
_import_structure = {
"configuration_clip": [
"CLIP_PRETRAINED_CONFIG_ARCHIVE_MAP",
"CLIPConfig",
"CLIPOnnxConfig",
"CLIPTextConfig",
"CLIPVisionConfig",
],
"processing_clip": ["CLIPProcessor"],
"tokenization_clip": ["CLIPTokenizer"],
}
try:
if not is_tokenizers_available():
raise OptionalDependencyNotAvailable()
except OptionalDependencyNotAvailable:
pass
else:
_import_structure["tokenization_clip_fast"] = ["CLIPTokenizerFast"]
try:
if not is_vision_available():
raise OptionalDependencyNotAvailable()
except OptionalDependencyNotAvailable:
pass
else:
_import_structure["feature_extraction_clip"] = ["CLIPFeatureExtractor"]
_import_structure["image_processing_clip"] = ["CLIPImageProcessor"]
try:
if not is_torch_available():
raise OptionalDependencyNotAvailable()
except OptionalDependencyNotAvailable:
pass
else:
_import_structure["modeling_clip"] = [
"CLIP_PRETRAINED_MODEL_ARCHIVE_LIST",
"CLIPModel",
"CLIPPreTrainedModel",
"CLIPTextModel",
"CLIPTextModelWithProjection",
"CLIPVisionModel",
"CLIPVisionModelWithProjection",
]
try:
if not is_tf_available():
raise OptionalDependencyNotAvailable()
except OptionalDependencyNotAvailable:
pass
else:
_import_structure["modeling_tf_clip"] = [
"TF_CLIP_PRETRAINED_MODEL_ARCHIVE_LIST",
"TFCLIPModel",
"TFCLIPPreTrainedModel",
"TFCLIPTextModel",
"TFCLIPVisionModel",
]
try:
if not is_flax_available():
raise OptionalDependencyNotAvailable()
except OptionalDependencyNotAvailable:
pass
else:
_import_structure["modeling_flax_clip"] = [
"FlaxCLIPModel",
"FlaxCLIPPreTrainedModel",
"FlaxCLIPTextModel",
"FlaxCLIPTextPreTrainedModel",
"FlaxCLIPTextModelWithProjection",
"FlaxCLIPVisionModel",
"FlaxCLIPVisionPreTrainedModel",
]
if TYPE_CHECKING:
from .configuration_clip import (
CLIP_PRETRAINED_CONFIG_ARCHIVE_MAP,
CLIPConfig,
CLIPOnnxConfig,
CLIPTextConfig,
CLIPVisionConfig,
)
from .processing_clip import CLIPProcessor
from .tokenization_clip import CLIPTokenizer
try:
if not is_tokenizers_available():
raise OptionalDependencyNotAvailable()
except OptionalDependencyNotAvailable:
pass
else:
from .tokenization_clip_fast import CLIPTokenizerFast
try:
if not is_vision_available():
raise OptionalDependencyNotAvailable()
except OptionalDependencyNotAvailable:
pass
else:
from .feature_extraction_clip import CLIPFeatureExtractor
from .image_processing_clip import CLIPImageProcessor
try:
if not is_torch_available():
raise OptionalDependencyNotAvailable()
except OptionalDependencyNotAvailable:
pass
else:
from .modeling_clip import (
CLIP_PRETRAINED_MODEL_ARCHIVE_LIST,
CLIPModel,
CLIPPreTrainedModel,
CLIPTextModel,
CLIPTextModelWithProjection,
CLIPVisionModel,
CLIPVisionModelWithProjection,
)
try:
if not is_tf_available():
raise OptionalDependencyNotAvailable()
except OptionalDependencyNotAvailable:
pass
else:
from .modeling_tf_clip import (
TF_CLIP_PRETRAINED_MODEL_ARCHIVE_LIST,
TFCLIPModel,
TFCLIPPreTrainedModel,
TFCLIPTextModel,
TFCLIPVisionModel,
)
try:
if not is_flax_available():
raise OptionalDependencyNotAvailable()
except OptionalDependencyNotAvailable:
pass
else:
from .modeling_flax_clip import (
FlaxCLIPModel,
FlaxCLIPPreTrainedModel,
FlaxCLIPTextModel,
FlaxCLIPTextModelWithProjection,
FlaxCLIPTextPreTrainedModel,
FlaxCLIPVisionModel,
FlaxCLIPVisionPreTrainedModel,
)
else:
import sys
sys.modules[__name__] = _LazyModule(__name__, globals()["__file__"], _import_structure, module_spec=__spec__)
| transformers/src/transformers/models/clip/__init__.py/0 | {
"file_path": "transformers/src/transformers/models/clip/__init__.py",
"repo_id": "transformers",
"token_count": 2180
} | 316 |
# Copyright 2023 The HuggingFace Team. All rights reserved.
#
# Licensed under the Apache License, Version 2.0 (the "License");
# you may not use this file except in compliance with the License.
# You may obtain a copy of the License at
#
# http://www.apache.org/licenses/LICENSE-2.0
#
# Unless required by applicable law or agreed to in writing, software
# distributed under the License is distributed on an "AS IS" BASIS,
# WITHOUT WARRANTIES OR CONDITIONS OF ANY KIND, either express or implied.
# See the License for the specific language governing permissions and
# limitations under the License.
from typing import TYPE_CHECKING
from ...utils import (
OptionalDependencyNotAvailable,
_LazyModule,
is_torch_available,
)
_import_structure = {
"configuration_clvp": [
"CLVP_PRETRAINED_CONFIG_ARCHIVE_MAP",
"ClvpConfig",
"ClvpDecoderConfig",
"ClvpEncoderConfig",
],
"feature_extraction_clvp": ["ClvpFeatureExtractor"],
"processing_clvp": ["ClvpProcessor"],
"tokenization_clvp": ["ClvpTokenizer"],
}
try:
if not is_torch_available():
raise OptionalDependencyNotAvailable()
except OptionalDependencyNotAvailable:
pass
else:
_import_structure["modeling_clvp"] = [
"CLVP_PRETRAINED_MODEL_ARCHIVE_LIST",
"ClvpModelForConditionalGeneration",
"ClvpForCausalLM",
"ClvpModel",
"ClvpPreTrainedModel",
"ClvpEncoder",
"ClvpDecoder",
]
if TYPE_CHECKING:
from .configuration_clvp import (
CLVP_PRETRAINED_CONFIG_ARCHIVE_MAP,
ClvpConfig,
ClvpDecoderConfig,
ClvpEncoderConfig,
)
from .feature_extraction_clvp import ClvpFeatureExtractor
from .processing_clvp import ClvpProcessor
from .tokenization_clvp import ClvpTokenizer
try:
if not is_torch_available():
raise OptionalDependencyNotAvailable()
except OptionalDependencyNotAvailable:
pass
else:
from .modeling_clvp import (
CLVP_PRETRAINED_MODEL_ARCHIVE_LIST,
ClvpDecoder,
ClvpEncoder,
ClvpForCausalLM,
ClvpModel,
ClvpModelForConditionalGeneration,
ClvpPreTrainedModel,
)
else:
import sys
sys.modules[__name__] = _LazyModule(__name__, globals()["__file__"], _import_structure, module_spec=__spec__)
| transformers/src/transformers/models/clvp/__init__.py/0 | {
"file_path": "transformers/src/transformers/models/clvp/__init__.py",
"repo_id": "transformers",
"token_count": 974
} | 317 |
#!/usr/bin/env python3
import argparse
import json
import torch
from huggingface_hub import hf_hub_download
from PIL import Image
from timm.models import create_model
from transformers import (
BeitImageProcessor,
Data2VecVisionConfig,
Data2VecVisionForImageClassification,
Data2VecVisionModel,
)
def create_rename_keys(config, has_lm_head=False, is_semantic=False, hf_prefix="data2vec."):
prefix = "backbone." if is_semantic else ""
rename_keys = []
for i in range(config.num_hidden_layers):
# encoder layers: output projection, 2 feedforward neural networks and 2 layernorms
rename_keys.append(
(f"{prefix}blocks.{i}.norm1.weight", f"{hf_prefix}encoder.layer.{i}.layernorm_before.weight")
)
rename_keys.append((f"{prefix}blocks.{i}.norm1.bias", f"{hf_prefix}encoder.layer.{i}.layernorm_before.bias"))
rename_keys.append(
(f"{prefix}blocks.{i}.attn.proj.weight", f"{hf_prefix}encoder.layer.{i}.attention.output.dense.weight")
)
rename_keys.append(
(f"{prefix}blocks.{i}.attn.proj.bias", f"{hf_prefix}encoder.layer.{i}.attention.output.dense.bias")
)
rename_keys.append(
(f"{prefix}blocks.{i}.norm2.weight", f"{hf_prefix}encoder.layer.{i}.layernorm_after.weight")
)
rename_keys.append((f"{prefix}blocks.{i}.norm2.bias", f"{hf_prefix}encoder.layer.{i}.layernorm_after.bias"))
rename_keys.append(
(f"{prefix}blocks.{i}.mlp.fc1.weight", f"{hf_prefix}encoder.layer.{i}.intermediate.dense.weight")
)
rename_keys.append(
(f"{prefix}blocks.{i}.mlp.fc1.bias", f"{hf_prefix}encoder.layer.{i}.intermediate.dense.bias")
)
rename_keys.append((f"{prefix}blocks.{i}.mlp.fc2.weight", f"{hf_prefix}encoder.layer.{i}.output.dense.weight"))
rename_keys.append((f"{prefix}blocks.{i}.mlp.fc2.bias", f"{hf_prefix}encoder.layer.{i}.output.dense.bias"))
# projection layer + position embeddings
rename_keys.extend(
[
(f"{prefix}cls_token", f"{hf_prefix}embeddings.cls_token"),
(f"{prefix}patch_embed.proj.weight", f"{hf_prefix}embeddings.patch_embeddings.projection.weight"),
(f"{prefix}patch_embed.proj.bias", f"{hf_prefix}embeddings.patch_embeddings.projection.bias"),
]
)
if has_lm_head:
# mask token + shared relative position bias + layernorm
rename_keys.extend(
[
("mask_token", f"{hf_prefix}embeddings.mask_token"),
(
"rel_pos_bias.relative_position_bias_table",
f"{hf_prefix}encoder.relative_position_bias.relative_position_bias_table",
),
(
"rel_pos_bias.relative_position_index",
f"{hf_prefix}encoder.relative_position_bias.relative_position_index",
),
("norm.weight", "layernorm.weight"),
("norm.bias", "layernorm.bias"),
]
)
elif is_semantic:
# semantic segmentation classification heads
rename_keys.extend(
[
("decode_head.conv_seg.weight", "decode_head.classifier.weight"),
("decode_head.conv_seg.bias", "decode_head.classifier.bias"),
("auxiliary_head.conv_seg.weight", "auxiliary_head.classifier.weight"),
("auxiliary_head.conv_seg.bias", "auxiliary_head.classifier.bias"),
]
)
else:
# layernorm + classification head
rename_keys.extend(
[
("fc_norm.weight", f"{hf_prefix}pooler.layernorm.weight"),
("fc_norm.bias", f"{hf_prefix}pooler.layernorm.bias"),
("head.weight", "classifier.weight"),
("head.bias", "classifier.bias"),
]
)
return rename_keys
def read_in_q_k_v(state_dict, config, has_lm_head=False, is_semantic=False, hf_prefix="data2vec_vision."):
for i in range(config.num_hidden_layers):
prefix = "backbone." if is_semantic else ""
# queries, keys and values
in_proj_weight = state_dict.pop(f"{prefix}blocks.{i}.attn.qkv.weight")
q_bias = state_dict.pop(f"{prefix}blocks.{i}.attn.q_bias")
v_bias = state_dict.pop(f"{prefix}blocks.{i}.attn.v_bias")
state_dict[f"{hf_prefix}encoder.layer.{i}.attention.attention.query.weight"] = in_proj_weight[
: config.hidden_size, :
]
state_dict[f"{hf_prefix}encoder.layer.{i}.attention.attention.query.bias"] = q_bias
state_dict[f"{hf_prefix}encoder.layer.{i}.attention.attention.key.weight"] = in_proj_weight[
config.hidden_size : config.hidden_size * 2, :
]
state_dict[f"{hf_prefix}encoder.layer.{i}.attention.attention.value.weight"] = in_proj_weight[
-config.hidden_size :, :
]
state_dict[f"{hf_prefix}encoder.layer.{i}.attention.attention.value.bias"] = v_bias
# gamma_1 and gamma_2
# we call them lambda because otherwise they are renamed when using .from_pretrained
gamma_1 = state_dict.pop(f"{prefix}blocks.{i}.gamma_1")
gamma_2 = state_dict.pop(f"{prefix}blocks.{i}.gamma_2")
state_dict[f"{hf_prefix}encoder.layer.{i}.lambda_1"] = gamma_1
state_dict[f"{hf_prefix}encoder.layer.{i}.lambda_2"] = gamma_2
# relative_position bias table + index
if not has_lm_head:
# each layer has its own relative position bias
table = state_dict.pop(f"{prefix}blocks.{i}.attn.relative_position_bias_table")
index = state_dict.pop(f"{prefix}blocks.{i}.attn.relative_position_index")
state_dict[
f"{hf_prefix}encoder.layer.{i}.attention.attention.relative_position_bias.relative_position_bias_table"
] = table
state_dict[
f"{hf_prefix}encoder.layer.{i}.attention.attention.relative_position_bias.relative_position_index"
] = index
def get_args():
parser = argparse.ArgumentParser(
"Convert Data2VecVision to HF for image classification and pretraining", add_help=False
)
parser.add_argument("--hf_checkpoint_name", type=str)
parser.add_argument("--input_size", default=224, type=int, help="images input size")
parser.add_argument("--beit_checkpoint", default="", help="beit checkpoint")
return parser.parse_args()
def load_beit_model(args, is_finetuned, is_large):
def load_state_dict(model, state_dict, prefix="", ignore_missing="relative_position_index"):
missing_keys = []
unexpected_keys = []
error_msgs = []
# copy state_dict so _load_from_state_dict can modify it
metadata = getattr(state_dict, "_metadata", None)
state_dict = state_dict.copy()
if metadata is not None:
state_dict._metadata = metadata
def load(module, prefix=""):
local_metadata = {} if metadata is None else metadata.get(prefix[:-1], {})
module._load_from_state_dict(
state_dict, prefix, local_metadata, True, missing_keys, unexpected_keys, error_msgs
)
for name, child in module._modules.items():
if child is not None:
load(child, prefix + name + ".")
load(model, prefix=prefix)
warn_missing_keys = []
ignore_missing_keys = []
for key in missing_keys:
keep_flag = True
for ignore_key in ignore_missing.split("|"):
if ignore_key in key:
keep_flag = False
break
if keep_flag:
warn_missing_keys.append(key)
else:
ignore_missing_keys.append(key)
missing_keys = warn_missing_keys
if len(missing_keys) > 0:
print(
"Weights of {} not initialized from pretrained model: {}".format(
model.__class__.__name__, missing_keys
)
)
if len(unexpected_keys) > 0:
print("Weights from pretrained model not used in {}: {}".format(model.__class__.__name__, unexpected_keys))
if len(ignore_missing_keys) > 0:
print(
"Ignored weights of {} not initialized from pretrained model: {}".format(
model.__class__.__name__, ignore_missing_keys
)
)
if len(error_msgs) > 0:
print("\n".join(error_msgs))
model_kwargs = {
"pretrained": False,
"use_shared_rel_pos_bias": True,
"use_abs_pos_emb": False,
"init_values": 0.1,
}
if is_finetuned:
model_kwargs.update(
{
"num_classes": 1000,
"use_mean_pooling": True,
"init_scale": 0.001,
"use_rel_pos_bias": True,
}
)
model = create_model(
"beit_large_patch16_224" if is_large else "beit_base_patch16_224",
**model_kwargs,
)
patch_size = model.patch_embed.patch_size
args.window_size = (args.input_size // patch_size[0], args.input_size // patch_size[1])
checkpoint = torch.load(args.beit_checkpoint, map_location="cpu")
print(f"Load ckpt from {args.beit_checkpoint}")
checkpoint_model = None
for model_key in ("model", "module"):
if model_key in checkpoint:
checkpoint_model = checkpoint[model_key]
print(f"Load state_dict by model_key = {model_key}")
break
all_keys = list(checkpoint_model.keys())
for key in all_keys:
if "relative_position_index" in key:
checkpoint_model.pop(key)
if "relative_position_bias_table" in key:
rel_pos_bias = checkpoint_model[key]
src_num_pos, num_attn_heads = rel_pos_bias.size()
dst_num_pos, _ = model.state_dict()[key].size()
dst_patch_shape = model.patch_embed.patch_shape
if dst_patch_shape[0] != dst_patch_shape[1]:
raise NotImplementedError()
load_state_dict(model, checkpoint_model, prefix="")
return model
def main():
args = get_args()
is_finetuned = "ft1k" in args.hf_checkpoint_name
is_large = "large" in args.hf_checkpoint_name
if is_finetuned:
# To convert Beit's data2vec_vision to HF you need to copy
# https://github.com/facebookresearch/data2vec_vision/blob/main/beit/modeling_finetune.py
# into this folder.
import modeling_finetune # noqa: F401
else:
# To convert Beit's data2vec_vision to HF you need to copy
# https://github.com/facebookresearch/data2vec_vision/blob/main/beit/modeling_cyclical.py
# into this folder
# IMPORTANT: Note that for now we've only converted the down-stream
# model and not the full pretrained model. This means for the integration
# test you need to add a `return x` after the following line:
# https://github.com/facebookresearch/data2vec_vision/blob/af9a36349aaed59ae66e69b5dabeef2d62fdc5da/beit/modeling_cyclical.py#L197
# to make the integration test pass.
import modeling_cyclical # noqa: F401
# 1. Create model config
config = Data2VecVisionConfig()
if is_finetuned:
config.use_relative_position_bias = True
config.use_shared_relative_position_bias = False
config.use_mean_pooling = True
config.num_labels = 1000
repo_id = "huggingface/label-files"
filename = "imagenet-1k-id2label.json"
id2label = json.load(open(hf_hub_download(repo_id, filename, repo_type="dataset"), "r"))
id2label = {int(k): v for k, v in id2label.items()}
config.id2label = id2label
config.label2id = {v: k for k, v in id2label.items()}
else:
config.use_relative_position_bias = False
config.use_shared_relative_position_bias = True
config.use_mean_pooling = False
if is_large:
config.hidden_size = 1024
config.intermediate_size = 4096
config.num_hidden_layers = 24
config.num_attention_heads = 16
# 2. Load Beit model
orig_model = load_beit_model(args, is_finetuned, is_large)
orig_model.eval()
# 3. Forward Beit model
image_processor = BeitImageProcessor(size=config.image_size, do_center_crop=False)
image = Image.open("../../../../tests/fixtures/tests_samples/COCO/000000039769.png")
encoding = image_processor(images=image, return_tensors="pt")
pixel_values = encoding["pixel_values"]
orig_args = (pixel_values,) if is_finetuned else (pixel_values, None)
with torch.no_grad():
orig_model_output = orig_model(*orig_args)
# 4. Load HF Data2VecVision model
if is_finetuned:
hf_model = Data2VecVisionForImageClassification(config)
hf_model.eval()
has_lm_head = False
hf_prefix = "data2vec_vision."
else:
hf_model = Data2VecVisionModel(config)
hf_model.eval()
has_lm_head = True
hf_prefix = ""
rename_keys = create_rename_keys(config, hf_prefix=hf_prefix, has_lm_head=has_lm_head)
state_dict = orig_model.state_dict()
for src, dest in rename_keys:
val = state_dict.pop(src)
state_dict[dest] = val
read_in_q_k_v(state_dict, config, hf_prefix=hf_prefix, has_lm_head=has_lm_head)
missing_keys, unexpected_keys = hf_model.load_state_dict(state_dict, strict=False)
print("HF missing", missing_keys)
print("HF unexpected_keys", unexpected_keys)
# 5. Forward HF Data2VecVision model
with torch.no_grad():
hf_model_output = hf_model(pixel_values)
hf_output = hf_model_output.logits if is_finetuned else hf_model_output.last_hidden_state
# 6. Compare
max_absolute_diff = torch.max(torch.abs(hf_output - orig_model_output)).item()
print(f"max_absolute_diff = {max_absolute_diff}")
success = torch.allclose(hf_output, orig_model_output, atol=1e-3)
print("Do both models output the same tensors?", "๐ฅ" if success else "๐ฉ")
if not success:
raise Exception("Something went wRoNg")
# 7. Save
print(f"Saving to {args.hf_checkpoint_name}")
hf_model.save_pretrained(args.hf_checkpoint_name)
image_processor.save_pretrained(args.hf_checkpoint_name)
if __name__ == "__main__":
main()
# Run the following to convert checkpoints
# python ./convert_data2vec_vision_original_pytorch_checkpoint_to_pytorch.py \
# --beit_checkpoint ./pretrained_base.pt \
# --hf_checkpoint_name "./data2vec-vision-base"
# python ./convert_data2vec_vision_original_pytorch_checkpoint_to_pytorch.py \
# --beit_checkpoint ./finetuned_base.pt \
# --hf_checkpoint_name "./data2vec-vision-base-ft1k"
# python ./convert_data2vec_vision_original_pytorch_checkpoint_to_pytorch.py \
# --beit_checkpoint ./pretrained_large.pt \
# --hf_checkpoint_name "./data2vec-vision-large"
# python ./convert_data2vec_vision_original_pytorch_checkpoint_to_pytorch.py \
# --beit_checkpoint ./finetuned_large.pt \
# --hf_checkpoint_name "./data2vec-vision-large-ft1k"
| transformers/src/transformers/models/data2vec/convert_data2vec_vision_original_pytorch_checkpoint_to_pytorch.py/0 | {
"file_path": "transformers/src/transformers/models/data2vec/convert_data2vec_vision_original_pytorch_checkpoint_to_pytorch.py",
"repo_id": "transformers",
"token_count": 7103
} | 318 |
# coding=utf-8
# Copyright 2020 Microsoft and the HuggingFace Inc. team.
#
# Licensed under the Apache License, Version 2.0 (the "License");
# you may not use this file except in compliance with the License.
# You may obtain a copy of the License at
#
# http://www.apache.org/licenses/LICENSE-2.0
#
# Unless required by applicable law or agreed to in writing, software
# distributed under the License is distributed on an "AS IS" BASIS,
# WITHOUT WARRANTIES OR CONDITIONS OF ANY KIND, either express or implied.
# See the License for the specific language governing permissions and
# limitations under the License.
"""Fast Tokenization class for model DeBERTa."""
import os
from shutil import copyfile
from typing import Optional, Tuple
from ...file_utils import is_sentencepiece_available
from ...tokenization_utils_fast import PreTrainedTokenizerFast
from ...utils import logging
if is_sentencepiece_available():
from .tokenization_deberta_v2 import DebertaV2Tokenizer
else:
DebertaV2Tokenizer = None
logger = logging.get_logger(__name__)
VOCAB_FILES_NAMES = {"vocab_file": "spm.model", "tokenizer_file": "tokenizer.json"}
PRETRAINED_VOCAB_FILES_MAP = {
"vocab_file": {
"microsoft/deberta-v2-xlarge": "https://huggingface.co/microsoft/deberta-v2-xlarge/resolve/main/spm.model",
"microsoft/deberta-v2-xxlarge": "https://huggingface.co/microsoft/deberta-v2-xxlarge/resolve/main/spm.model",
"microsoft/deberta-v2-xlarge-mnli": (
"https://huggingface.co/microsoft/deberta-v2-xlarge-mnli/resolve/main/spm.model"
),
"microsoft/deberta-v2-xxlarge-mnli": (
"https://huggingface.co/microsoft/deberta-v2-xxlarge-mnli/resolve/main/spm.model"
),
}
}
PRETRAINED_POSITIONAL_EMBEDDINGS_SIZES = {
"microsoft/deberta-v2-xlarge": 512,
"microsoft/deberta-v2-xxlarge": 512,
"microsoft/deberta-v2-xlarge-mnli": 512,
"microsoft/deberta-v2-xxlarge-mnli": 512,
}
PRETRAINED_INIT_CONFIGURATION = {
"microsoft/deberta-v2-xlarge": {"do_lower_case": False},
"microsoft/deberta-v2-xxlarge": {"do_lower_case": False},
"microsoft/deberta-v2-xlarge-mnli": {"do_lower_case": False},
"microsoft/deberta-v2-xxlarge-mnli": {"do_lower_case": False},
}
class DebertaV2TokenizerFast(PreTrainedTokenizerFast):
r"""
Constructs a DeBERTa-v2 fast tokenizer. Based on [SentencePiece](https://github.com/google/sentencepiece).
Args:
vocab_file (`str`):
[SentencePiece](https://github.com/google/sentencepiece) file (generally has a *.spm* extension) that
contains the vocabulary necessary to instantiate a tokenizer.
do_lower_case (`bool`, *optional*, defaults to `False`):
Whether or not to lowercase the input when tokenizing.
bos_token (`string`, *optional*, defaults to `"[CLS]"`):
The beginning of sequence token that was used during pre-training. Can be used a sequence classifier token.
When building a sequence using special tokens, this is not the token that is used for the beginning of
sequence. The token used is the `cls_token`.
eos_token (`string`, *optional*, defaults to `"[SEP]"`):
The end of sequence token. When building a sequence using special tokens, this is not the token that is
used for the end of sequence. The token used is the `sep_token`.
unk_token (`str`, *optional*, defaults to `"[UNK]"`):
The unknown token. A token that is not in the vocabulary cannot be converted to an ID and is set to be this
token instead.
sep_token (`str`, *optional*, defaults to `"[SEP]"`):
The separator token, which is used when building a sequence from multiple sequences, e.g. two sequences for
sequence classification or for a text and a question for question answering. It is also used as the last
token of a sequence built with special tokens.
pad_token (`str`, *optional*, defaults to `"[PAD]"`):
The token used for padding, for example when batching sequences of different lengths.
cls_token (`str`, *optional*, defaults to `"[CLS]"`):
The classifier token which is used when doing sequence classification (classification of the whole sequence
instead of per-token classification). It is the first token of the sequence when built with special tokens.
mask_token (`str`, *optional*, defaults to `"[MASK]"`):
The token used for masking values. This is the token used when training this model with masked language
modeling. This is the token which the model will try to predict.
sp_model_kwargs (`dict`, *optional*):
Will be passed to the `SentencePieceProcessor.__init__()` method. The [Python wrapper for
SentencePiece](https://github.com/google/sentencepiece/tree/master/python) can be used, among other things,
to set:
- `enable_sampling`: Enable subword regularization.
- `nbest_size`: Sampling parameters for unigram. Invalid for BPE-Dropout.
- `nbest_size = {0,1}`: No sampling is performed.
- `nbest_size > 1`: samples from the nbest_size results.
- `nbest_size < 0`: assuming that nbest_size is infinite and samples from the all hypothesis (lattice)
using forward-filtering-and-backward-sampling algorithm.
- `alpha`: Smoothing parameter for unigram sampling, and dropout probability of merge operations for
BPE-dropout.
"""
vocab_files_names = VOCAB_FILES_NAMES
pretrained_vocab_files_map = PRETRAINED_VOCAB_FILES_MAP
pretrained_init_configuration = PRETRAINED_INIT_CONFIGURATION
max_model_input_sizes = PRETRAINED_POSITIONAL_EMBEDDINGS_SIZES
slow_tokenizer_class = DebertaV2Tokenizer
def __init__(
self,
vocab_file=None,
tokenizer_file=None,
do_lower_case=False,
split_by_punct=False,
bos_token="[CLS]",
eos_token="[SEP]",
unk_token="[UNK]",
sep_token="[SEP]",
pad_token="[PAD]",
cls_token="[CLS]",
mask_token="[MASK]",
**kwargs,
) -> None:
super().__init__(
vocab_file,
tokenizer_file=tokenizer_file,
do_lower_case=do_lower_case,
bos_token=bos_token,
eos_token=eos_token,
unk_token=unk_token,
sep_token=sep_token,
pad_token=pad_token,
cls_token=cls_token,
mask_token=mask_token,
split_by_punct=split_by_punct,
**kwargs,
)
self.do_lower_case = do_lower_case
self.split_by_punct = split_by_punct
self.vocab_file = vocab_file
@property
def can_save_slow_tokenizer(self) -> bool:
return os.path.isfile(self.vocab_file) if self.vocab_file else False
def build_inputs_with_special_tokens(self, token_ids_0, token_ids_1=None):
"""
Build model inputs from a sequence or a pair of sequence for sequence classification tasks by concatenating and
adding special tokens. A DeBERTa sequence has the following format:
- single sequence: [CLS] X [SEP]
- pair of sequences: [CLS] A [SEP] B [SEP]
Args:
token_ids_0 (`List[int]`):
List of IDs to which the special tokens will be added.
token_ids_1 (`List[int]`, *optional*):
Optional second list of IDs for sequence pairs.
Returns:
`List[int]`: List of [input IDs](../glossary#input-ids) with the appropriate special tokens.
"""
if token_ids_1 is None:
return [self.cls_token_id] + token_ids_0 + [self.sep_token_id]
cls = [self.cls_token_id]
sep = [self.sep_token_id]
return cls + token_ids_0 + sep + token_ids_1 + sep
def get_special_tokens_mask(self, token_ids_0, token_ids_1=None, already_has_special_tokens=False):
"""
Retrieves sequence ids from a token list that has no special tokens added. This method is called when adding
special tokens using the tokenizer `prepare_for_model` or `encode_plus` methods.
Args:
token_ids_0 (`List[int]`):
List of IDs.
token_ids_1 (`List[int]`, *optional*):
Optional second list of IDs for sequence pairs.
already_has_special_tokens (`bool`, *optional*, defaults to `False`):
Whether or not the token list is already formatted with special tokens for the model.
Returns:
`List[int]`: A list of integers in the range [0, 1]: 1 for a special token, 0 for a sequence token.
"""
if already_has_special_tokens:
return super().get_special_tokens_mask(
token_ids_0=token_ids_0, token_ids_1=token_ids_1, already_has_special_tokens=True
)
if token_ids_1 is not None:
return [1] + ([0] * len(token_ids_0)) + [1] + ([0] * len(token_ids_1)) + [1]
return [1] + ([0] * len(token_ids_0)) + [1]
def create_token_type_ids_from_sequences(self, token_ids_0, token_ids_1=None):
"""
Create a mask from the two sequences passed to be used in a sequence-pair classification task. A DeBERTa
sequence pair mask has the following format:
```
0 0 0 0 0 0 0 0 0 0 0 1 1 1 1 1 1 1 1 1
| first sequence | second sequence |
```
If `token_ids_1` is `None`, this method only returns the first portion of the mask (0s).
Args:
token_ids_0 (`List[int]`):
List of IDs.
token_ids_1 (`List[int]`, *optional*):
Optional second list of IDs for sequence pairs.
Returns:
`List[int]`: List of [token type IDs](../glossary#token-type-ids) according to the given sequence(s).
"""
sep = [self.sep_token_id]
cls = [self.cls_token_id]
if token_ids_1 is None:
return len(cls + token_ids_0 + sep) * [0]
return len(cls + token_ids_0 + sep) * [0] + len(token_ids_1 + sep) * [1]
def save_vocabulary(self, save_directory: str, filename_prefix: Optional[str] = None) -> Tuple[str]:
if not self.can_save_slow_tokenizer:
raise ValueError(
"Your fast tokenizer does not have the necessary information to save the vocabulary for a slow "
"tokenizer."
)
if not os.path.isdir(save_directory):
logger.error(f"Vocabulary path ({save_directory}) should be a directory")
return
out_vocab_file = os.path.join(
save_directory, (filename_prefix + "-" if filename_prefix else "") + VOCAB_FILES_NAMES["vocab_file"]
)
if os.path.abspath(self.vocab_file) != os.path.abspath(out_vocab_file):
copyfile(self.vocab_file, out_vocab_file)
return (out_vocab_file,)
| transformers/src/transformers/models/deberta_v2/tokenization_deberta_v2_fast.py/0 | {
"file_path": "transformers/src/transformers/models/deberta_v2/tokenization_deberta_v2_fast.py",
"repo_id": "transformers",
"token_count": 4688
} | 319 |
# coding=utf-8
# Copyright 2021 Facebook AI Research (FAIR), Ross Wightman, The HuggingFace Inc. team. All rights reserved.
#
# Licensed under the Apache License, Version 2.0 (the "License");
# you may not use this file except in compliance with the License.
# You may obtain a copy of the License at
#
# http://www.apache.org/licenses/LICENSE-2.0
#
# Unless required by applicable law or agreed to in writing, software
# distributed under the License is distributed on an "AS IS" BASIS,
# WITHOUT WARRANTIES OR CONDITIONS OF ANY KIND, either express or implied.
# See the License for the specific language governing permissions and
# limitations under the License.
""" PyTorch DeiT model."""
import collections.abc
import math
from dataclasses import dataclass
from typing import Optional, Set, Tuple, Union
import torch
import torch.utils.checkpoint
from torch import nn
from torch.nn import BCEWithLogitsLoss, CrossEntropyLoss, MSELoss
from ...activations import ACT2FN
from ...modeling_outputs import (
BaseModelOutput,
BaseModelOutputWithPooling,
ImageClassifierOutput,
MaskedImageModelingOutput,
)
from ...modeling_utils import PreTrainedModel
from ...pytorch_utils import find_pruneable_heads_and_indices, prune_linear_layer
from ...utils import (
ModelOutput,
add_code_sample_docstrings,
add_start_docstrings,
add_start_docstrings_to_model_forward,
logging,
replace_return_docstrings,
)
from .configuration_deit import DeiTConfig
logger = logging.get_logger(__name__)
# General docstring
_CONFIG_FOR_DOC = "DeiTConfig"
# Base docstring
_CHECKPOINT_FOR_DOC = "facebook/deit-base-distilled-patch16-224"
_EXPECTED_OUTPUT_SHAPE = [1, 198, 768]
# Image classification docstring
_IMAGE_CLASS_CHECKPOINT = "facebook/deit-base-distilled-patch16-224"
_IMAGE_CLASS_EXPECTED_OUTPUT = "tabby, tabby cat"
DEIT_PRETRAINED_MODEL_ARCHIVE_LIST = [
"facebook/deit-base-distilled-patch16-224",
# See all DeiT models at https://huggingface.co/models?filter=deit
]
class DeiTEmbeddings(nn.Module):
"""
Construct the CLS token, distillation token, position and patch embeddings. Optionally, also the mask token.
"""
def __init__(self, config: DeiTConfig, use_mask_token: bool = False) -> None:
super().__init__()
self.cls_token = nn.Parameter(torch.zeros(1, 1, config.hidden_size))
self.distillation_token = nn.Parameter(torch.zeros(1, 1, config.hidden_size))
self.mask_token = nn.Parameter(torch.zeros(1, 1, config.hidden_size)) if use_mask_token else None
self.patch_embeddings = DeiTPatchEmbeddings(config)
num_patches = self.patch_embeddings.num_patches
self.position_embeddings = nn.Parameter(torch.zeros(1, num_patches + 2, config.hidden_size))
self.dropout = nn.Dropout(config.hidden_dropout_prob)
def forward(self, pixel_values: torch.Tensor, bool_masked_pos: Optional[torch.BoolTensor] = None) -> torch.Tensor:
embeddings = self.patch_embeddings(pixel_values)
batch_size, seq_length, _ = embeddings.size()
if bool_masked_pos is not None:
mask_tokens = self.mask_token.expand(batch_size, seq_length, -1)
# replace the masked visual tokens by mask_tokens
mask = bool_masked_pos.unsqueeze(-1).type_as(mask_tokens)
embeddings = embeddings * (1.0 - mask) + mask_tokens * mask
cls_tokens = self.cls_token.expand(batch_size, -1, -1)
distillation_tokens = self.distillation_token.expand(batch_size, -1, -1)
embeddings = torch.cat((cls_tokens, distillation_tokens, embeddings), dim=1)
embeddings = embeddings + self.position_embeddings
embeddings = self.dropout(embeddings)
return embeddings
class DeiTPatchEmbeddings(nn.Module):
"""
This class turns `pixel_values` of shape `(batch_size, num_channels, height, width)` into the initial
`hidden_states` (patch embeddings) of shape `(batch_size, seq_length, hidden_size)` to be consumed by a
Transformer.
"""
def __init__(self, config):
super().__init__()
image_size, patch_size = config.image_size, config.patch_size
num_channels, hidden_size = config.num_channels, config.hidden_size
image_size = image_size if isinstance(image_size, collections.abc.Iterable) else (image_size, image_size)
patch_size = patch_size if isinstance(patch_size, collections.abc.Iterable) else (patch_size, patch_size)
num_patches = (image_size[1] // patch_size[1]) * (image_size[0] // patch_size[0])
self.image_size = image_size
self.patch_size = patch_size
self.num_channels = num_channels
self.num_patches = num_patches
self.projection = nn.Conv2d(num_channels, hidden_size, kernel_size=patch_size, stride=patch_size)
def forward(self, pixel_values: torch.Tensor) -> torch.Tensor:
batch_size, num_channels, height, width = pixel_values.shape
if num_channels != self.num_channels:
raise ValueError(
"Make sure that the channel dimension of the pixel values match with the one set in the configuration."
)
if height != self.image_size[0] or width != self.image_size[1]:
raise ValueError(
f"Input image size ({height}*{width}) doesn't match model ({self.image_size[0]}*{self.image_size[1]})."
)
x = self.projection(pixel_values).flatten(2).transpose(1, 2)
return x
# Copied from transformers.models.vit.modeling_vit.ViTSelfAttention with ViT->DeiT
class DeiTSelfAttention(nn.Module):
def __init__(self, config: DeiTConfig) -> None:
super().__init__()
if config.hidden_size % config.num_attention_heads != 0 and not hasattr(config, "embedding_size"):
raise ValueError(
f"The hidden size {config.hidden_size,} is not a multiple of the number of attention "
f"heads {config.num_attention_heads}."
)
self.num_attention_heads = config.num_attention_heads
self.attention_head_size = int(config.hidden_size / config.num_attention_heads)
self.all_head_size = self.num_attention_heads * self.attention_head_size
self.query = nn.Linear(config.hidden_size, self.all_head_size, bias=config.qkv_bias)
self.key = nn.Linear(config.hidden_size, self.all_head_size, bias=config.qkv_bias)
self.value = nn.Linear(config.hidden_size, self.all_head_size, bias=config.qkv_bias)
self.dropout = nn.Dropout(config.attention_probs_dropout_prob)
def transpose_for_scores(self, x: torch.Tensor) -> torch.Tensor:
new_x_shape = x.size()[:-1] + (self.num_attention_heads, self.attention_head_size)
x = x.view(new_x_shape)
return x.permute(0, 2, 1, 3)
def forward(
self, hidden_states, head_mask: Optional[torch.Tensor] = None, output_attentions: bool = False
) -> Union[Tuple[torch.Tensor, torch.Tensor], Tuple[torch.Tensor]]:
mixed_query_layer = self.query(hidden_states)
key_layer = self.transpose_for_scores(self.key(hidden_states))
value_layer = self.transpose_for_scores(self.value(hidden_states))
query_layer = self.transpose_for_scores(mixed_query_layer)
# Take the dot product between "query" and "key" to get the raw attention scores.
attention_scores = torch.matmul(query_layer, key_layer.transpose(-1, -2))
attention_scores = attention_scores / math.sqrt(self.attention_head_size)
# Normalize the attention scores to probabilities.
attention_probs = nn.functional.softmax(attention_scores, dim=-1)
# This is actually dropping out entire tokens to attend to, which might
# seem a bit unusual, but is taken from the original Transformer paper.
attention_probs = self.dropout(attention_probs)
# Mask heads if we want to
if head_mask is not None:
attention_probs = attention_probs * head_mask
context_layer = torch.matmul(attention_probs, value_layer)
context_layer = context_layer.permute(0, 2, 1, 3).contiguous()
new_context_layer_shape = context_layer.size()[:-2] + (self.all_head_size,)
context_layer = context_layer.view(new_context_layer_shape)
outputs = (context_layer, attention_probs) if output_attentions else (context_layer,)
return outputs
# Copied from transformers.models.vit.modeling_vit.ViTSelfOutput with ViT->DeiT
class DeiTSelfOutput(nn.Module):
"""
The residual connection is defined in DeiTLayer instead of here (as is the case with other models), due to the
layernorm applied before each block.
"""
def __init__(self, config: DeiTConfig) -> None:
super().__init__()
self.dense = nn.Linear(config.hidden_size, config.hidden_size)
self.dropout = nn.Dropout(config.hidden_dropout_prob)
def forward(self, hidden_states: torch.Tensor, input_tensor: torch.Tensor) -> torch.Tensor:
hidden_states = self.dense(hidden_states)
hidden_states = self.dropout(hidden_states)
return hidden_states
# Copied from transformers.models.vit.modeling_vit.ViTAttention with ViT->DeiT
class DeiTAttention(nn.Module):
def __init__(self, config: DeiTConfig) -> None:
super().__init__()
self.attention = DeiTSelfAttention(config)
self.output = DeiTSelfOutput(config)
self.pruned_heads = set()
def prune_heads(self, heads: Set[int]) -> None:
if len(heads) == 0:
return
heads, index = find_pruneable_heads_and_indices(
heads, self.attention.num_attention_heads, self.attention.attention_head_size, self.pruned_heads
)
# Prune linear layers
self.attention.query = prune_linear_layer(self.attention.query, index)
self.attention.key = prune_linear_layer(self.attention.key, index)
self.attention.value = prune_linear_layer(self.attention.value, index)
self.output.dense = prune_linear_layer(self.output.dense, index, dim=1)
# Update hyper params and store pruned heads
self.attention.num_attention_heads = self.attention.num_attention_heads - len(heads)
self.attention.all_head_size = self.attention.attention_head_size * self.attention.num_attention_heads
self.pruned_heads = self.pruned_heads.union(heads)
def forward(
self,
hidden_states: torch.Tensor,
head_mask: Optional[torch.Tensor] = None,
output_attentions: bool = False,
) -> Union[Tuple[torch.Tensor, torch.Tensor], Tuple[torch.Tensor]]:
self_outputs = self.attention(hidden_states, head_mask, output_attentions)
attention_output = self.output(self_outputs[0], hidden_states)
outputs = (attention_output,) + self_outputs[1:] # add attentions if we output them
return outputs
# Copied from transformers.models.vit.modeling_vit.ViTIntermediate with ViT->DeiT
class DeiTIntermediate(nn.Module):
def __init__(self, config: DeiTConfig) -> None:
super().__init__()
self.dense = nn.Linear(config.hidden_size, config.intermediate_size)
if isinstance(config.hidden_act, str):
self.intermediate_act_fn = ACT2FN[config.hidden_act]
else:
self.intermediate_act_fn = config.hidden_act
def forward(self, hidden_states: torch.Tensor) -> torch.Tensor:
hidden_states = self.dense(hidden_states)
hidden_states = self.intermediate_act_fn(hidden_states)
return hidden_states
# Copied from transformers.models.vit.modeling_vit.ViTOutput with ViT->DeiT
class DeiTOutput(nn.Module):
def __init__(self, config: DeiTConfig) -> None:
super().__init__()
self.dense = nn.Linear(config.intermediate_size, config.hidden_size)
self.dropout = nn.Dropout(config.hidden_dropout_prob)
def forward(self, hidden_states: torch.Tensor, input_tensor: torch.Tensor) -> torch.Tensor:
hidden_states = self.dense(hidden_states)
hidden_states = self.dropout(hidden_states)
hidden_states = hidden_states + input_tensor
return hidden_states
# Copied from transformers.models.vit.modeling_vit.ViTLayer with ViT->DeiT
class DeiTLayer(nn.Module):
"""This corresponds to the Block class in the timm implementation."""
def __init__(self, config: DeiTConfig) -> None:
super().__init__()
self.chunk_size_feed_forward = config.chunk_size_feed_forward
self.seq_len_dim = 1
self.attention = DeiTAttention(config)
self.intermediate = DeiTIntermediate(config)
self.output = DeiTOutput(config)
self.layernorm_before = nn.LayerNorm(config.hidden_size, eps=config.layer_norm_eps)
self.layernorm_after = nn.LayerNorm(config.hidden_size, eps=config.layer_norm_eps)
def forward(
self,
hidden_states: torch.Tensor,
head_mask: Optional[torch.Tensor] = None,
output_attentions: bool = False,
) -> Union[Tuple[torch.Tensor, torch.Tensor], Tuple[torch.Tensor]]:
self_attention_outputs = self.attention(
self.layernorm_before(hidden_states), # in DeiT, layernorm is applied before self-attention
head_mask,
output_attentions=output_attentions,
)
attention_output = self_attention_outputs[0]
outputs = self_attention_outputs[1:] # add self attentions if we output attention weights
# first residual connection
hidden_states = attention_output + hidden_states
# in DeiT, layernorm is also applied after self-attention
layer_output = self.layernorm_after(hidden_states)
layer_output = self.intermediate(layer_output)
# second residual connection is done here
layer_output = self.output(layer_output, hidden_states)
outputs = (layer_output,) + outputs
return outputs
# Copied from transformers.models.vit.modeling_vit.ViTEncoder with ViT->DeiT
class DeiTEncoder(nn.Module):
def __init__(self, config: DeiTConfig) -> None:
super().__init__()
self.config = config
self.layer = nn.ModuleList([DeiTLayer(config) for _ in range(config.num_hidden_layers)])
self.gradient_checkpointing = False
def forward(
self,
hidden_states: torch.Tensor,
head_mask: Optional[torch.Tensor] = None,
output_attentions: bool = False,
output_hidden_states: bool = False,
return_dict: bool = True,
) -> Union[tuple, BaseModelOutput]:
all_hidden_states = () if output_hidden_states else None
all_self_attentions = () if output_attentions else None
for i, layer_module in enumerate(self.layer):
if output_hidden_states:
all_hidden_states = all_hidden_states + (hidden_states,)
layer_head_mask = head_mask[i] if head_mask is not None else None
if self.gradient_checkpointing and self.training:
layer_outputs = self._gradient_checkpointing_func(
layer_module.__call__,
hidden_states,
layer_head_mask,
output_attentions,
)
else:
layer_outputs = layer_module(hidden_states, layer_head_mask, output_attentions)
hidden_states = layer_outputs[0]
if output_attentions:
all_self_attentions = all_self_attentions + (layer_outputs[1],)
if output_hidden_states:
all_hidden_states = all_hidden_states + (hidden_states,)
if not return_dict:
return tuple(v for v in [hidden_states, all_hidden_states, all_self_attentions] if v is not None)
return BaseModelOutput(
last_hidden_state=hidden_states,
hidden_states=all_hidden_states,
attentions=all_self_attentions,
)
class DeiTPreTrainedModel(PreTrainedModel):
"""
An abstract class to handle weights initialization and a simple interface for downloading and loading pretrained
models.
"""
config_class = DeiTConfig
base_model_prefix = "deit"
main_input_name = "pixel_values"
supports_gradient_checkpointing = True
_no_split_modules = ["DeiTLayer"]
def _init_weights(self, module: Union[nn.Linear, nn.Conv2d, nn.LayerNorm]) -> None:
"""Initialize the weights"""
if isinstance(module, (nn.Linear, nn.Conv2d)):
# Upcast the input in `fp32` and cast it back to desired `dtype` to avoid
# `trunc_normal_cpu` not implemented in `half` issues
module.weight.data = nn.init.trunc_normal_(
module.weight.data.to(torch.float32), mean=0.0, std=self.config.initializer_range
).to(module.weight.dtype)
if module.bias is not None:
module.bias.data.zero_()
elif isinstance(module, nn.LayerNorm):
module.bias.data.zero_()
module.weight.data.fill_(1.0)
DEIT_START_DOCSTRING = r"""
This model is a PyTorch [torch.nn.Module](https://pytorch.org/docs/stable/nn.html#torch.nn.Module) subclass. Use it
as a regular PyTorch Module and refer to the PyTorch documentation for all matter related to general usage and
behavior.
Parameters:
config ([`DeiTConfig`]): Model configuration class with all the parameters of the model.
Initializing with a config file does not load the weights associated with the model, only the
configuration. Check out the [`~PreTrainedModel.from_pretrained`] method to load the model weights.
"""
DEIT_INPUTS_DOCSTRING = r"""
Args:
pixel_values (`torch.FloatTensor` of shape `(batch_size, num_channels, height, width)`):
Pixel values. Pixel values can be obtained using [`AutoImageProcessor`]. See
[`DeiTImageProcessor.__call__`] for details.
head_mask (`torch.FloatTensor` of shape `(num_heads,)` or `(num_layers, num_heads)`, *optional*):
Mask to nullify selected heads of the self-attention modules. Mask values selected in `[0, 1]`:
- 1 indicates the head is **not masked**,
- 0 indicates the head is **masked**.
output_attentions (`bool`, *optional*):
Whether or not to return the attentions tensors of all attention layers. See `attentions` under returned
tensors for more detail.
output_hidden_states (`bool`, *optional*):
Whether or not to return the hidden states of all layers. See `hidden_states` under returned tensors for
more detail.
return_dict (`bool`, *optional*):
Whether or not to return a [`~utils.ModelOutput`] instead of a plain tuple.
"""
@add_start_docstrings(
"The bare DeiT Model transformer outputting raw hidden-states without any specific head on top.",
DEIT_START_DOCSTRING,
)
class DeiTModel(DeiTPreTrainedModel):
def __init__(self, config: DeiTConfig, add_pooling_layer: bool = True, use_mask_token: bool = False) -> None:
super().__init__(config)
self.config = config
self.embeddings = DeiTEmbeddings(config, use_mask_token=use_mask_token)
self.encoder = DeiTEncoder(config)
self.layernorm = nn.LayerNorm(config.hidden_size, eps=config.layer_norm_eps)
self.pooler = DeiTPooler(config) if add_pooling_layer else None
# Initialize weights and apply final processing
self.post_init()
def get_input_embeddings(self) -> DeiTPatchEmbeddings:
return self.embeddings.patch_embeddings
def _prune_heads(self, heads_to_prune):
"""
Prunes heads of the model. heads_to_prune: dict of {layer_num: list of heads to prune in this layer} See base
class PreTrainedModel
"""
for layer, heads in heads_to_prune.items():
self.encoder.layer[layer].attention.prune_heads(heads)
@add_start_docstrings_to_model_forward(DEIT_INPUTS_DOCSTRING)
@add_code_sample_docstrings(
checkpoint=_CHECKPOINT_FOR_DOC,
output_type=BaseModelOutputWithPooling,
config_class=_CONFIG_FOR_DOC,
modality="vision",
expected_output=_EXPECTED_OUTPUT_SHAPE,
)
def forward(
self,
pixel_values: Optional[torch.Tensor] = None,
bool_masked_pos: Optional[torch.BoolTensor] = None,
head_mask: Optional[torch.Tensor] = None,
output_attentions: Optional[bool] = None,
output_hidden_states: Optional[bool] = None,
return_dict: Optional[bool] = None,
) -> Union[Tuple, BaseModelOutputWithPooling]:
r"""
bool_masked_pos (`torch.BoolTensor` of shape `(batch_size, num_patches)`, *optional*):
Boolean masked positions. Indicates which patches are masked (1) and which aren't (0).
"""
output_attentions = output_attentions if output_attentions is not None else self.config.output_attentions
output_hidden_states = (
output_hidden_states if output_hidden_states is not None else self.config.output_hidden_states
)
return_dict = return_dict if return_dict is not None else self.config.use_return_dict
if pixel_values is None:
raise ValueError("You have to specify pixel_values")
# Prepare head mask if needed
# 1.0 in head_mask indicate we keep the head
# attention_probs has shape bsz x n_heads x N x N
# input head_mask has shape [num_heads] or [num_hidden_layers x num_heads]
# and head_mask is converted to shape [num_hidden_layers x batch x num_heads x seq_length x seq_length]
head_mask = self.get_head_mask(head_mask, self.config.num_hidden_layers)
# TODO: maybe have a cleaner way to cast the input (from `ImageProcessor` side?)
expected_dtype = self.embeddings.patch_embeddings.projection.weight.dtype
if pixel_values.dtype != expected_dtype:
pixel_values = pixel_values.to(expected_dtype)
embedding_output = self.embeddings(pixel_values, bool_masked_pos=bool_masked_pos)
encoder_outputs = self.encoder(
embedding_output,
head_mask=head_mask,
output_attentions=output_attentions,
output_hidden_states=output_hidden_states,
return_dict=return_dict,
)
sequence_output = encoder_outputs[0]
sequence_output = self.layernorm(sequence_output)
pooled_output = self.pooler(sequence_output) if self.pooler is not None else None
if not return_dict:
head_outputs = (sequence_output, pooled_output) if pooled_output is not None else (sequence_output,)
return head_outputs + encoder_outputs[1:]
return BaseModelOutputWithPooling(
last_hidden_state=sequence_output,
pooler_output=pooled_output,
hidden_states=encoder_outputs.hidden_states,
attentions=encoder_outputs.attentions,
)
# Copied from transformers.models.vit.modeling_vit.ViTPooler with ViT->DeiT
class DeiTPooler(nn.Module):
def __init__(self, config: DeiTConfig):
super().__init__()
self.dense = nn.Linear(config.hidden_size, config.hidden_size)
self.activation = nn.Tanh()
def forward(self, hidden_states):
# We "pool" the model by simply taking the hidden state corresponding
# to the first token.
first_token_tensor = hidden_states[:, 0]
pooled_output = self.dense(first_token_tensor)
pooled_output = self.activation(pooled_output)
return pooled_output
@add_start_docstrings(
"""DeiT Model with a decoder on top for masked image modeling, as proposed in [SimMIM](https://arxiv.org/abs/2111.09886).
<Tip>
Note that we provide a script to pre-train this model on custom data in our [examples
directory](https://github.com/huggingface/transformers/tree/main/examples/pytorch/image-pretraining).
</Tip>
""",
DEIT_START_DOCSTRING,
)
class DeiTForMaskedImageModeling(DeiTPreTrainedModel):
def __init__(self, config: DeiTConfig) -> None:
super().__init__(config)
self.deit = DeiTModel(config, add_pooling_layer=False, use_mask_token=True)
self.decoder = nn.Sequential(
nn.Conv2d(
in_channels=config.hidden_size,
out_channels=config.encoder_stride**2 * config.num_channels,
kernel_size=1,
),
nn.PixelShuffle(config.encoder_stride),
)
# Initialize weights and apply final processing
self.post_init()
@add_start_docstrings_to_model_forward(DEIT_INPUTS_DOCSTRING)
@replace_return_docstrings(output_type=MaskedImageModelingOutput, config_class=_CONFIG_FOR_DOC)
def forward(
self,
pixel_values: Optional[torch.Tensor] = None,
bool_masked_pos: Optional[torch.BoolTensor] = None,
head_mask: Optional[torch.Tensor] = None,
output_attentions: Optional[bool] = None,
output_hidden_states: Optional[bool] = None,
return_dict: Optional[bool] = None,
) -> Union[tuple, MaskedImageModelingOutput]:
r"""
bool_masked_pos (`torch.BoolTensor` of shape `(batch_size, num_patches)`):
Boolean masked positions. Indicates which patches are masked (1) and which aren't (0).
Returns:
Examples:
```python
>>> from transformers import AutoImageProcessor, DeiTForMaskedImageModeling
>>> import torch
>>> from PIL import Image
>>> import requests
>>> url = "http://images.cocodataset.org/val2017/000000039769.jpg"
>>> image = Image.open(requests.get(url, stream=True).raw)
>>> image_processor = AutoImageProcessor.from_pretrained("facebook/deit-base-distilled-patch16-224")
>>> model = DeiTForMaskedImageModeling.from_pretrained("facebook/deit-base-distilled-patch16-224")
>>> num_patches = (model.config.image_size // model.config.patch_size) ** 2
>>> pixel_values = image_processor(images=image, return_tensors="pt").pixel_values
>>> # create random boolean mask of shape (batch_size, num_patches)
>>> bool_masked_pos = torch.randint(low=0, high=2, size=(1, num_patches)).bool()
>>> outputs = model(pixel_values, bool_masked_pos=bool_masked_pos)
>>> loss, reconstructed_pixel_values = outputs.loss, outputs.reconstruction
>>> list(reconstructed_pixel_values.shape)
[1, 3, 224, 224]
```"""
return_dict = return_dict if return_dict is not None else self.config.use_return_dict
outputs = self.deit(
pixel_values,
bool_masked_pos=bool_masked_pos,
head_mask=head_mask,
output_attentions=output_attentions,
output_hidden_states=output_hidden_states,
return_dict=return_dict,
)
sequence_output = outputs[0]
# Reshape to (batch_size, num_channels, height, width)
sequence_output = sequence_output[:, 1:-1]
batch_size, sequence_length, num_channels = sequence_output.shape
height = width = int(sequence_length**0.5)
sequence_output = sequence_output.permute(0, 2, 1).reshape(batch_size, num_channels, height, width)
# Reconstruct pixel values
reconstructed_pixel_values = self.decoder(sequence_output)
masked_im_loss = None
if bool_masked_pos is not None:
size = self.config.image_size // self.config.patch_size
bool_masked_pos = bool_masked_pos.reshape(-1, size, size)
mask = (
bool_masked_pos.repeat_interleave(self.config.patch_size, 1)
.repeat_interleave(self.config.patch_size, 2)
.unsqueeze(1)
.contiguous()
)
reconstruction_loss = nn.functional.l1_loss(pixel_values, reconstructed_pixel_values, reduction="none")
masked_im_loss = (reconstruction_loss * mask).sum() / (mask.sum() + 1e-5) / self.config.num_channels
if not return_dict:
output = (reconstructed_pixel_values,) + outputs[1:]
return ((masked_im_loss,) + output) if masked_im_loss is not None else output
return MaskedImageModelingOutput(
loss=masked_im_loss,
reconstruction=reconstructed_pixel_values,
hidden_states=outputs.hidden_states,
attentions=outputs.attentions,
)
@add_start_docstrings(
"""
DeiT Model transformer with an image classification head on top (a linear layer on top of the final hidden state of
the [CLS] token) e.g. for ImageNet.
""",
DEIT_START_DOCSTRING,
)
class DeiTForImageClassification(DeiTPreTrainedModel):
def __init__(self, config: DeiTConfig) -> None:
super().__init__(config)
self.num_labels = config.num_labels
self.deit = DeiTModel(config, add_pooling_layer=False)
# Classifier head
self.classifier = nn.Linear(config.hidden_size, config.num_labels) if config.num_labels > 0 else nn.Identity()
# Initialize weights and apply final processing
self.post_init()
@add_start_docstrings_to_model_forward(DEIT_INPUTS_DOCSTRING)
@replace_return_docstrings(output_type=ImageClassifierOutput, config_class=_CONFIG_FOR_DOC)
def forward(
self,
pixel_values: Optional[torch.Tensor] = None,
head_mask: Optional[torch.Tensor] = None,
labels: Optional[torch.Tensor] = None,
output_attentions: Optional[bool] = None,
output_hidden_states: Optional[bool] = None,
return_dict: Optional[bool] = None,
) -> Union[tuple, ImageClassifierOutput]:
r"""
labels (`torch.LongTensor` of shape `(batch_size,)`, *optional*):
Labels for computing the image classification/regression loss. Indices should be in `[0, ...,
config.num_labels - 1]`. If `config.num_labels == 1` a regression loss is computed (Mean-Square loss), If
`config.num_labels > 1` a classification loss is computed (Cross-Entropy).
Returns:
Examples:
```python
>>> from transformers import AutoImageProcessor, DeiTForImageClassification
>>> import torch
>>> from PIL import Image
>>> import requests
>>> torch.manual_seed(3) # doctest: +IGNORE_RESULT
>>> url = "http://images.cocodataset.org/val2017/000000039769.jpg"
>>> image = Image.open(requests.get(url, stream=True).raw)
>>> # note: we are loading a DeiTForImageClassificationWithTeacher from the hub here,
>>> # so the head will be randomly initialized, hence the predictions will be random
>>> image_processor = AutoImageProcessor.from_pretrained("facebook/deit-base-distilled-patch16-224")
>>> model = DeiTForImageClassification.from_pretrained("facebook/deit-base-distilled-patch16-224")
>>> inputs = image_processor(images=image, return_tensors="pt")
>>> outputs = model(**inputs)
>>> logits = outputs.logits
>>> # model predicts one of the 1000 ImageNet classes
>>> predicted_class_idx = logits.argmax(-1).item()
>>> print("Predicted class:", model.config.id2label[predicted_class_idx])
Predicted class: magpie
```"""
return_dict = return_dict if return_dict is not None else self.config.use_return_dict
outputs = self.deit(
pixel_values,
head_mask=head_mask,
output_attentions=output_attentions,
output_hidden_states=output_hidden_states,
return_dict=return_dict,
)
sequence_output = outputs[0]
logits = self.classifier(sequence_output[:, 0, :])
# we don't use the distillation token
loss = None
if labels is not None:
labels = labels.to(logits.device)
if self.config.problem_type is None:
if self.num_labels == 1:
self.config.problem_type = "regression"
elif self.num_labels > 1 and (labels.dtype == torch.long or labels.dtype == torch.int):
self.config.problem_type = "single_label_classification"
else:
self.config.problem_type = "multi_label_classification"
if self.config.problem_type == "regression":
loss_fct = MSELoss()
if self.num_labels == 1:
loss = loss_fct(logits.squeeze(), labels.squeeze())
else:
loss = loss_fct(logits, labels)
elif self.config.problem_type == "single_label_classification":
loss_fct = CrossEntropyLoss()
loss = loss_fct(logits.view(-1, self.num_labels), labels.view(-1))
elif self.config.problem_type == "multi_label_classification":
loss_fct = BCEWithLogitsLoss()
loss = loss_fct(logits, labels)
if not return_dict:
output = (logits,) + outputs[1:]
return ((loss,) + output) if loss is not None else output
return ImageClassifierOutput(
loss=loss,
logits=logits,
hidden_states=outputs.hidden_states,
attentions=outputs.attentions,
)
@dataclass
class DeiTForImageClassificationWithTeacherOutput(ModelOutput):
"""
Output type of [`DeiTForImageClassificationWithTeacher`].
Args:
logits (`torch.FloatTensor` of shape `(batch_size, config.num_labels)`):
Prediction scores as the average of the cls_logits and distillation logits.
cls_logits (`torch.FloatTensor` of shape `(batch_size, config.num_labels)`):
Prediction scores of the classification head (i.e. the linear layer on top of the final hidden state of the
class token).
distillation_logits (`torch.FloatTensor` of shape `(batch_size, config.num_labels)`):
Prediction scores of the distillation head (i.e. the linear layer on top of the final hidden state of the
distillation token).
hidden_states (`tuple(torch.FloatTensor)`, *optional*, returned when `output_hidden_states=True` is passed or when `config.output_hidden_states=True`):
Tuple of `torch.FloatTensor` (one for the output of the embeddings + one for the output of each layer) of
shape `(batch_size, sequence_length, hidden_size)`. Hidden-states of the model at the output of each layer
plus the initial embedding outputs.
attentions (`tuple(torch.FloatTensor)`, *optional*, returned when `output_attentions=True` is passed or when `config.output_attentions=True`):
Tuple of `torch.FloatTensor` (one for each layer) of shape `(batch_size, num_heads, sequence_length,
sequence_length)`. Attentions weights after the attention softmax, used to compute the weighted average in
the self-attention heads.
"""
logits: torch.FloatTensor = None
cls_logits: torch.FloatTensor = None
distillation_logits: torch.FloatTensor = None
hidden_states: Optional[Tuple[torch.FloatTensor]] = None
attentions: Optional[Tuple[torch.FloatTensor]] = None
@add_start_docstrings(
"""
DeiT Model transformer with image classification heads on top (a linear layer on top of the final hidden state of
the [CLS] token and a linear layer on top of the final hidden state of the distillation token) e.g. for ImageNet.
.. warning::
This model supports inference-only. Fine-tuning with distillation (i.e. with a teacher) is not yet
supported.
""",
DEIT_START_DOCSTRING,
)
class DeiTForImageClassificationWithTeacher(DeiTPreTrainedModel):
def __init__(self, config: DeiTConfig) -> None:
super().__init__(config)
self.num_labels = config.num_labels
self.deit = DeiTModel(config, add_pooling_layer=False)
# Classifier heads
self.cls_classifier = (
nn.Linear(config.hidden_size, config.num_labels) if config.num_labels > 0 else nn.Identity()
)
self.distillation_classifier = (
nn.Linear(config.hidden_size, config.num_labels) if config.num_labels > 0 else nn.Identity()
)
# Initialize weights and apply final processing
self.post_init()
@add_start_docstrings_to_model_forward(DEIT_INPUTS_DOCSTRING)
@add_code_sample_docstrings(
checkpoint=_IMAGE_CLASS_CHECKPOINT,
output_type=DeiTForImageClassificationWithTeacherOutput,
config_class=_CONFIG_FOR_DOC,
expected_output=_IMAGE_CLASS_EXPECTED_OUTPUT,
)
def forward(
self,
pixel_values: Optional[torch.Tensor] = None,
head_mask: Optional[torch.Tensor] = None,
output_attentions: Optional[bool] = None,
output_hidden_states: Optional[bool] = None,
return_dict: Optional[bool] = None,
) -> Union[tuple, DeiTForImageClassificationWithTeacherOutput]:
return_dict = return_dict if return_dict is not None else self.config.use_return_dict
outputs = self.deit(
pixel_values,
head_mask=head_mask,
output_attentions=output_attentions,
output_hidden_states=output_hidden_states,
return_dict=return_dict,
)
sequence_output = outputs[0]
cls_logits = self.cls_classifier(sequence_output[:, 0, :])
distillation_logits = self.distillation_classifier(sequence_output[:, 1, :])
# during inference, return the average of both classifier predictions
logits = (cls_logits + distillation_logits) / 2
if not return_dict:
output = (logits, cls_logits, distillation_logits) + outputs[1:]
return output
return DeiTForImageClassificationWithTeacherOutput(
logits=logits,
cls_logits=cls_logits,
distillation_logits=distillation_logits,
hidden_states=outputs.hidden_states,
attentions=outputs.attentions,
)
| transformers/src/transformers/models/deit/modeling_deit.py/0 | {
"file_path": "transformers/src/transformers/models/deit/modeling_deit.py",
"repo_id": "transformers",
"token_count": 15850
} | 320 |
# Copyright 2020 The HuggingFace Team. All rights reserved.
#
# Licensed under the Apache License, Version 2.0 (the "License");
# you may not use this file except in compliance with the License.
# You may obtain a copy of the License at
#
# http://www.apache.org/licenses/LICENSE-2.0
#
# Unless required by applicable law or agreed to in writing, software
# distributed under the License is distributed on an "AS IS" BASIS,
# WITHOUT WARRANTIES OR CONDITIONS OF ANY KIND, either express or implied.
# See the License for the specific language governing permissions and
# limitations under the License.
from typing import TYPE_CHECKING
from ....utils import OptionalDependencyNotAvailable, _LazyModule, is_tokenizers_available, is_torch_available
_import_structure = {
"configuration_retribert": ["RETRIBERT_PRETRAINED_CONFIG_ARCHIVE_MAP", "RetriBertConfig"],
"tokenization_retribert": ["RetriBertTokenizer"],
}
try:
if not is_tokenizers_available():
raise OptionalDependencyNotAvailable()
except OptionalDependencyNotAvailable:
pass
else:
_import_structure["tokenization_retribert_fast"] = ["RetriBertTokenizerFast"]
try:
if not is_torch_available():
raise OptionalDependencyNotAvailable()
except OptionalDependencyNotAvailable:
pass
else:
_import_structure["modeling_retribert"] = [
"RETRIBERT_PRETRAINED_MODEL_ARCHIVE_LIST",
"RetriBertModel",
"RetriBertPreTrainedModel",
]
if TYPE_CHECKING:
from .configuration_retribert import RETRIBERT_PRETRAINED_CONFIG_ARCHIVE_MAP, RetriBertConfig
from .tokenization_retribert import RetriBertTokenizer
try:
if not is_tokenizers_available():
raise OptionalDependencyNotAvailable()
except OptionalDependencyNotAvailable:
pass
else:
from .tokenization_retribert_fast import RetriBertTokenizerFast
try:
if not is_torch_available():
raise OptionalDependencyNotAvailable()
except OptionalDependencyNotAvailable:
pass
else:
from .modeling_retribert import (
RETRIBERT_PRETRAINED_MODEL_ARCHIVE_LIST,
RetriBertModel,
RetriBertPreTrainedModel,
)
else:
import sys
sys.modules[__name__] = _LazyModule(__name__, globals()["__file__"], _import_structure, module_spec=__spec__)
| transformers/src/transformers/models/deprecated/retribert/__init__.py/0 | {
"file_path": "transformers/src/transformers/models/deprecated/retribert/__init__.py",
"repo_id": "transformers",
"token_count": 861
} | 321 |
# coding=utf-8
# Copyright 2018 Google AI, Google Brain and Carnegie Mellon University Authors and the HuggingFace Inc. team.
# Copyright (c) 2018, NVIDIA CORPORATION. All rights reserved.
#
# Licensed under the Apache License, Version 2.0 (the "License");
# you may not use this file except in compliance with the License.
# You may obtain a copy of the License at
#
# http://www.apache.org/licenses/LICENSE-2.0
#
# Unless required by applicable law or agreed to in writing, software
# distributed under the License is distributed on an "AS IS" BASIS,
# WITHOUT WARRANTIES OR CONDITIONS OF ANY KIND, either express or implied.
# See the License for the specific language governing permissions and
# limitations under the License.
"""
PyTorch Transformer XL model. Adapted from https://github.com/kimiyoung/transformer-xl. In particular
https://github.com/kimiyoung/transformer-xl/blob/master/pytorch/mem_transformer.py
"""
import warnings
from dataclasses import dataclass
from typing import List, Optional, Tuple, Union
import torch
from torch import nn
from torch.nn import BCEWithLogitsLoss, CrossEntropyLoss, MSELoss
from ....modeling_utils import PreTrainedModel
from ....utils import (
ModelOutput,
add_code_sample_docstrings,
add_start_docstrings,
add_start_docstrings_to_model_forward,
logging,
)
from .configuration_transfo_xl import TransfoXLConfig
from .modeling_transfo_xl_utilities import ProjectedAdaptiveLogSoftmax
logger = logging.get_logger(__name__)
_CHECKPOINT_FOR_DOC = "transfo-xl-wt103"
_CONFIG_FOR_DOC = "TransfoXLConfig"
TRANSFO_XL_PRETRAINED_MODEL_ARCHIVE_LIST = [
"transfo-xl-wt103",
# See all Transformer XL models at https://huggingface.co/models?filter=transfo-xl
]
def build_tf_to_pytorch_map(model, config):
"""
A map of modules from TF to PyTorch. This time I use a map to keep the PyTorch model as identical to the original
PyTorch model as possible.
"""
tf_to_pt_map = {}
if hasattr(model, "transformer"):
# We are loading in a TransfoXLLMHeadModel => we will load also the Adaptive Softmax
tf_to_pt_map.update(
{
"transformer/adaptive_softmax/cutoff_0/cluster_W": model.crit.cluster_weight,
"transformer/adaptive_softmax/cutoff_0/cluster_b": model.crit.cluster_bias,
}
)
for i, (out_l, proj_l, tie_proj) in enumerate(
zip(model.crit.out_layers, model.crit.out_projs, config.tie_projs)
):
layer_str = f"transformer/adaptive_softmax/cutoff_{i}/"
if config.tie_word_embeddings:
tf_to_pt_map.update({layer_str + "b": out_l.bias})
else:
raise NotImplementedError
# I don't think this is implemented in the TF code
tf_to_pt_map.update({layer_str + "lookup_table": out_l.weight, layer_str + "b": out_l.bias})
if not tie_proj:
tf_to_pt_map.update({layer_str + "proj": proj_l})
# Now load the rest of the transformer
model = model.transformer
# Embeddings
for i, (embed_l, proj_l) in enumerate(zip(model.word_emb.emb_layers, model.word_emb.emb_projs)):
layer_str = f"transformer/adaptive_embed/cutoff_{i}/"
tf_to_pt_map.update({layer_str + "lookup_table": embed_l.weight, layer_str + "proj_W": proj_l})
# Transformer blocks
for i, b in enumerate(model.layers):
layer_str = f"transformer/layer_{i}/"
tf_to_pt_map.update(
{
layer_str + "rel_attn/LayerNorm/gamma": b.dec_attn.layer_norm.weight,
layer_str + "rel_attn/LayerNorm/beta": b.dec_attn.layer_norm.bias,
layer_str + "rel_attn/o/kernel": b.dec_attn.o_net.weight,
layer_str + "rel_attn/qkv/kernel": b.dec_attn.qkv_net.weight,
layer_str + "rel_attn/r/kernel": b.dec_attn.r_net.weight,
layer_str + "ff/LayerNorm/gamma": b.pos_ff.layer_norm.weight,
layer_str + "ff/LayerNorm/beta": b.pos_ff.layer_norm.bias,
layer_str + "ff/layer_1/kernel": b.pos_ff.CoreNet[0].weight,
layer_str + "ff/layer_1/bias": b.pos_ff.CoreNet[0].bias,
layer_str + "ff/layer_2/kernel": b.pos_ff.CoreNet[3].weight,
layer_str + "ff/layer_2/bias": b.pos_ff.CoreNet[3].bias,
}
)
# Relative positioning biases
if config.untie_r:
r_r_list = []
r_w_list = []
for b in model.layers:
r_r_list.append(b.dec_attn.r_r_bias)
r_w_list.append(b.dec_attn.r_w_bias)
else:
r_r_list = [model.r_r_bias]
r_w_list = [model.r_w_bias]
tf_to_pt_map.update({"transformer/r_r_bias": r_r_list, "transformer/r_w_bias": r_w_list})
return tf_to_pt_map
def load_tf_weights_in_transfo_xl(model, config, tf_path):
"""Load tf checkpoints in a pytorch model"""
try:
import numpy as np
import tensorflow as tf
except ImportError:
logger.error(
"Loading a TensorFlow models in PyTorch, requires TensorFlow to be installed. Please see "
"https://www.tensorflow.org/install/ for installation instructions."
)
raise
# Build TF to PyTorch weights loading map
tf_to_pt_map = build_tf_to_pytorch_map(model, config)
# Load weights from TF model
init_vars = tf.train.list_variables(tf_path)
tf_weights = {}
for name, shape in init_vars:
logger.info(f"Loading TF weight {name} with shape {shape}")
array = tf.train.load_variable(tf_path, name)
tf_weights[name] = array
for name, pointer in tf_to_pt_map.items():
assert name in tf_weights
array = tf_weights[name]
# adam_v and adam_m are variables used in AdamWeightDecayOptimizer to calculated m and v
# which are not required for using pretrained model
if "kernel" in name or "proj" in name:
array = np.transpose(array)
if ("r_r_bias" in name or "r_w_bias" in name) and len(pointer) > 1:
# Here we will split the TF weights
assert len(pointer) == array.shape[0]
for i, p_i in enumerate(pointer):
arr_i = array[i, ...]
try:
assert p_i.shape == arr_i.shape
except AssertionError as e:
e.args += (p_i.shape, arr_i.shape)
raise
logger.info(f"Initialize PyTorch weight {name} for layer {i}")
p_i.data = torch.from_numpy(arr_i)
else:
try:
assert (
pointer.shape == array.shape
), f"Pointer shape {pointer.shape} and array shape {array.shape} mismatched"
except AssertionError as e:
e.args += (pointer.shape, array.shape)
raise
logger.info(f"Initialize PyTorch weight {name}")
pointer.data = torch.from_numpy(array)
tf_weights.pop(name, None)
tf_weights.pop(name + "/Adam", None)
tf_weights.pop(name + "/Adam_1", None)
logger.info(f"Weights not copied to PyTorch model: {', '.join(tf_weights.keys())}")
return model
class PositionalEmbedding(nn.Module):
def __init__(self, demb):
super().__init__()
self.demb = demb
inv_freq = 1 / (10000 ** (torch.arange(0.0, demb, 2.0) / demb))
self.register_buffer("inv_freq", inv_freq)
def forward(self, pos_seq, bsz=None):
sinusoid_inp = torch.outer(pos_seq, self.inv_freq)
pos_emb = torch.cat([sinusoid_inp.sin(), sinusoid_inp.cos()], dim=-1)
if bsz is not None:
return pos_emb[:, None, :].expand(-1, bsz, -1)
else:
return pos_emb[:, None, :]
class PositionwiseFF(nn.Module):
def __init__(self, d_model, d_inner, dropout, pre_lnorm=False, layer_norm_epsilon=1e-5):
super().__init__()
self.d_model = d_model
self.d_inner = d_inner
self.dropout = dropout
self.CoreNet = nn.Sequential(
nn.Linear(d_model, d_inner),
nn.ReLU(inplace=True),
nn.Dropout(dropout),
nn.Linear(d_inner, d_model),
nn.Dropout(dropout),
)
self.layer_norm = nn.LayerNorm(d_model, eps=layer_norm_epsilon)
self.pre_lnorm = pre_lnorm
def forward(self, inp):
if self.pre_lnorm:
# layer normalization + positionwise feed-forward
core_out = self.CoreNet(self.layer_norm(inp))
# residual connection
output = core_out + inp
else:
# positionwise feed-forward
core_out = self.CoreNet(inp)
# residual connection + layer normalization
output = self.layer_norm(inp + core_out)
return output
class RelPartialLearnableMultiHeadAttn(nn.Module):
def __init__(
self,
n_head,
d_model,
d_head,
dropout,
dropatt=0,
pre_lnorm=False,
r_r_bias=None,
r_w_bias=None,
layer_norm_epsilon=1e-5,
):
super().__init__()
self.n_head = n_head
self.d_model = d_model
self.d_head = d_head
self.dropout = dropout
self.qkv_net = nn.Linear(d_model, 3 * n_head * d_head, bias=False)
self.drop = nn.Dropout(dropout)
self.dropatt = nn.Dropout(dropatt)
self.o_net = nn.Linear(n_head * d_head, d_model, bias=False)
self.layer_norm = nn.LayerNorm(d_model, eps=layer_norm_epsilon)
self.scale = 1 / (d_head**0.5)
self.pre_lnorm = pre_lnorm
if r_r_bias is None or r_w_bias is None: # Biases are not shared
self.r_r_bias = nn.Parameter(torch.FloatTensor(self.n_head, self.d_head))
self.r_w_bias = nn.Parameter(torch.FloatTensor(self.n_head, self.d_head))
else:
self.r_r_bias = r_r_bias
self.r_w_bias = r_w_bias
self.r_net = nn.Linear(self.d_model, self.n_head * self.d_head, bias=False)
def _rel_shift(self, x):
zero_pad_shape = (x.size(0), 1) + x.size()[2:]
zero_pad = torch.zeros(zero_pad_shape, device=x.device, dtype=x.dtype)
x_padded = torch.cat([zero_pad, x], dim=1)
x_padded_shape = (x.size(1) + 1, x.size(0)) + x.size()[2:]
x_padded = x_padded.view(*x_padded_shape)
x = x_padded[1:].view_as(x)
return x
def forward(self, w, r, attn_mask=None, mems=None, head_mask=None, output_attentions=False):
qlen, rlen, bsz = w.size(0), r.size(0), w.size(1)
if mems is not None:
cat = torch.cat([mems, w], 0)
if self.pre_lnorm:
w_heads = self.qkv_net(self.layer_norm(cat))
else:
w_heads = self.qkv_net(cat)
r_head_k = self.r_net(r)
w_head_q, w_head_k, w_head_v = torch.chunk(w_heads, 3, dim=-1)
w_head_q = w_head_q[-qlen:]
else:
if self.pre_lnorm:
w_heads = self.qkv_net(self.layer_norm(w))
else:
w_heads = self.qkv_net(w)
r_head_k = self.r_net(r)
w_head_q, w_head_k, w_head_v = torch.chunk(w_heads, 3, dim=-1)
klen = w_head_k.size(0)
w_head_q = w_head_q.view(qlen, bsz, self.n_head, self.d_head) # qlen x bsz x n_head x d_head
w_head_k = w_head_k.view(klen, bsz, self.n_head, self.d_head) # qlen x bsz x n_head x d_head
w_head_v = w_head_v.view(klen, bsz, self.n_head, self.d_head) # qlen x bsz x n_head x d_head
r_head_k = r_head_k.view(rlen, self.n_head, self.d_head) # qlen x n_head x d_head
# compute attention score
rw_head_q = w_head_q + self.r_w_bias # qlen x bsz x n_head x d_head
AC = torch.einsum("ibnd,jbnd->ijbn", (rw_head_q, w_head_k)) # qlen x klen x bsz x n_head
rr_head_q = w_head_q + self.r_r_bias
BD = torch.einsum("ibnd,jnd->ijbn", (rr_head_q, r_head_k)) # qlen x klen x bsz x n_head
BD = self._rel_shift(BD)
# [qlen x klen x bsz x n_head]
attn_score = AC + BD
attn_score.mul_(self.scale)
mask_value = torch.finfo(attn_score.dtype).min
# compute attention probability
if attn_mask is not None and torch.sum(attn_mask).item():
attn_mask = attn_mask == 1 # Switch to bool
if attn_mask.dim() == 2:
attn_score = (
attn_score.float().masked_fill(attn_mask[None, :, :, None], mask_value).type_as(attn_score)
)
elif attn_mask.dim() == 3:
attn_score = attn_score.float().masked_fill(attn_mask[:, :, :, None], mask_value).type_as(attn_score)
# [qlen x klen x bsz x n_head]
attn_prob = nn.functional.softmax(attn_score, dim=1)
attn_prob = self.dropatt(attn_prob)
# Mask heads if we want to
if head_mask is not None:
attn_prob = attn_prob * head_mask
# compute attention vector
attn_vec = torch.einsum("ijbn,jbnd->ibnd", (attn_prob, w_head_v))
# [qlen x bsz x n_head x d_head]
attn_vec = attn_vec.contiguous().view(attn_vec.size(0), attn_vec.size(1), self.n_head * self.d_head)
# linear projection
attn_out = self.o_net(attn_vec)
attn_out = self.drop(attn_out)
if self.pre_lnorm:
# residual connection
outputs = [w + attn_out]
else:
# residual connection + layer normalization
outputs = [self.layer_norm(w + attn_out)]
if output_attentions:
outputs.append(attn_prob)
return outputs
class RelPartialLearnableDecoderLayer(nn.Module):
def __init__(self, n_head, d_model, d_head, d_inner, dropout, layer_norm_epsilon=1e-5, **kwargs):
super().__init__()
self.dec_attn = RelPartialLearnableMultiHeadAttn(
n_head, d_model, d_head, dropout, layer_norm_epsilon=layer_norm_epsilon, **kwargs
)
self.pos_ff = PositionwiseFF(
d_model, d_inner, dropout, pre_lnorm=kwargs.get("pre_lnorm"), layer_norm_epsilon=layer_norm_epsilon
)
def forward(self, dec_inp, r, dec_attn_mask=None, mems=None, head_mask=None, output_attentions=False):
attn_outputs = self.dec_attn(
dec_inp,
r,
attn_mask=dec_attn_mask,
mems=mems,
head_mask=head_mask,
output_attentions=output_attentions,
)
ff_output = self.pos_ff(attn_outputs[0])
outputs = [ff_output] + attn_outputs[1:]
return outputs
class AdaptiveEmbedding(nn.Module):
def __init__(self, n_token, d_embed, d_proj, cutoffs, div_val=1, sample_softmax=False):
super().__init__()
self.n_token = n_token
self.d_embed = d_embed
self.cutoffs = cutoffs + [n_token]
self.div_val = div_val
self.d_proj = d_proj
self.emb_scale = d_proj**0.5
self.cutoff_ends = [0] + self.cutoffs
self.emb_layers = nn.ModuleList()
self.emb_projs = nn.ParameterList()
if div_val == 1:
self.emb_layers.append(nn.Embedding(n_token, d_embed, sparse=sample_softmax > 0))
if d_proj != d_embed:
self.emb_projs.append(nn.Parameter(torch.FloatTensor(d_proj, d_embed)))
else:
for i in range(len(self.cutoffs)):
l_idx, r_idx = self.cutoff_ends[i], self.cutoff_ends[i + 1]
d_emb_i = d_embed // (div_val**i)
self.emb_layers.append(nn.Embedding(r_idx - l_idx, d_emb_i))
self.emb_projs.append(nn.Parameter(torch.FloatTensor(d_proj, d_emb_i)))
def forward(self, inp):
if self.div_val == 1:
embed = self.emb_layers[0](inp)
if self.d_proj != self.d_embed:
embed = nn.functional.linear(embed, self.emb_projs[0])
else:
param = next(self.parameters())
inp_flat = inp.view(-1)
emb_flat = torch.zeros([inp_flat.size(0), self.d_proj], dtype=param.dtype, device=param.device)
for i in range(len(self.cutoffs)):
l_idx, r_idx = self.cutoff_ends[i], self.cutoff_ends[i + 1]
mask_i = (inp_flat >= l_idx) & (inp_flat < r_idx)
indices_i = mask_i.nonzero().squeeze()
if indices_i.numel() == 0:
continue
inp_i = inp_flat.index_select(0, indices_i) - l_idx
emb_i = self.emb_layers[i](inp_i)
emb_i = nn.functional.linear(emb_i, self.emb_projs[i])
emb_flat.index_copy_(0, indices_i, emb_i)
embed_shape = inp.size() + (self.d_proj,)
embed = emb_flat.view(embed_shape)
embed.mul_(self.emb_scale)
return embed
class TransfoXLPreTrainedModel(PreTrainedModel):
"""
An abstract class to handle weights initialization and a simple interface for downloading and loading pretrained
models.
"""
config_class = TransfoXLConfig
load_tf_weights = load_tf_weights_in_transfo_xl
base_model_prefix = "transformer"
def _init_weight(self, weight):
if self.config.init == "uniform":
nn.init.uniform_(weight, -self.config.init_range, self.config.init_range)
elif self.config.init == "normal":
nn.init.normal_(weight, 0.0, self.config.init_std)
def _init_bias(self, bias):
nn.init.constant_(bias, 0.0)
def _init_weights(self, m):
"""Initialize the weights."""
classname = m.__class__.__name__
if classname.find("Linear") != -1:
if hasattr(m, "weight") and m.weight is not None:
self._init_weight(m.weight)
if hasattr(m, "bias") and m.bias is not None:
self._init_bias(m.bias)
elif classname.find("AdaptiveEmbedding") != -1:
if hasattr(m, "emb_projs"):
for i in range(len(m.emb_projs)):
if m.emb_projs[i] is not None:
nn.init.normal_(m.emb_projs[i], 0.0, self.config.proj_init_std)
elif classname.find("Embedding") != -1:
if hasattr(m, "weight"):
self._init_weight(m.weight)
elif classname.find("ProjectedAdaptiveLogSoftmax") != -1:
if hasattr(m, "cluster_weight") and m.cluster_weight is not None:
self._init_weight(m.cluster_weight)
if hasattr(m, "cluster_bias") and m.cluster_bias is not None:
self._init_bias(m.cluster_bias)
if hasattr(m, "out_projs"):
for i in range(len(m.out_projs)):
if m.out_projs[i] is not None:
nn.init.normal_(m.out_projs[i], 0.0, self.config.proj_init_std)
elif classname.find("LayerNorm") != -1:
if hasattr(m, "weight"):
nn.init.normal_(m.weight, 1.0, self.config.init_std)
if hasattr(m, "bias") and m.bias is not None:
self._init_bias(m.bias)
else:
if hasattr(m, "r_emb"):
self._init_weight(m.r_emb)
if hasattr(m, "r_w_bias"):
self._init_weight(m.r_w_bias)
if hasattr(m, "r_r_bias"):
self._init_weight(m.r_r_bias)
if hasattr(m, "r_bias"):
self._init_bias(m.r_bias)
def resize_token_embeddings(self, new_num_tokens: Optional[int] = None, layer: Optional[int] = -1):
"""
Resize input token embeddings matrix of the model if new_num_tokens != config.vocab_size. Take care of tying
weights embeddings afterwards if the model class has a *tie_weights()* method.
Arguments:
new_num_tokens: (*optional*) int:
New number of tokens in the embedding matrix. Increasing the size will add newly initialized vectors at
the end. Reducing the size will remove vectors from the end. If not provided or None: does nothing and
just returns a pointer to the input tokens `torch.nn.Embeddings` Module of the model.
layer: (*optional*) int:
Layer of the *AdaptiveEmbedding* where the resizing should be done. Per default the last layer will be
resized. Be aware that when resizing other than the last layer, you have to ensure that the new
token(s) in the tokenizer are at the corresponding position.
Return: `torch.nn.Embeddings` Pointer to the input tokens Embeddings Module of the model
"""
base_model = getattr(self, self.base_model_prefix, self) # get the base model if needed
if new_num_tokens is None:
return self.get_input_embeddings()
new_num_tokens_layer, layer = self._get_new_num_tokens_layer(new_num_tokens, layer)
assert new_num_tokens_layer > 0, "The size of the new embedding layer cannot be 0 or less"
model_embeds = base_model._resize_token_embeddings(new_num_tokens_layer, layer)
# Update base model and current model config
self.config.vocab_size = new_num_tokens
base_model.vocab_size = new_num_tokens
base_model.n_token = new_num_tokens
new_embedding_shapes = self._get_embedding_shapes()
self._resize_cutoffs(new_num_tokens, new_num_tokens_layer, new_embedding_shapes, layer)
# Tie weights again if needed
self.tie_weights()
return model_embeds
def _get_new_num_tokens_layer(self, new_num_tokens, layer):
embeddings = self.get_input_embeddings()
if layer == -1:
layer = len(embeddings.emb_layers) - 1
assert 0 <= layer <= len(embeddings.emb_layers) - 1
new_num_tokens_layer = (
new_num_tokens
- sum([emb.weight.shape[0] for emb in embeddings.emb_layers[:layer]])
- sum([emb.weight.shape[0] for emb in embeddings.emb_layers[layer + 1 :]])
)
return new_num_tokens_layer, layer
def _get_embedding_shapes(self):
embeddings = self.get_input_embeddings()
return [emb.weight.shape[0] for emb in embeddings.emb_layers]
def _resize_token_embeddings(self, new_num_tokens, layer=-1):
embeddings = self.get_input_embeddings()
if new_num_tokens is None:
return embeddings
new_embeddings_layer = self._get_resized_embeddings(embeddings.emb_layers[layer], new_num_tokens)
embeddings.emb_layers[layer] = new_embeddings_layer
self.set_input_embeddings(embeddings)
return self.get_input_embeddings()
def _resize_cutoffs(self, new_num_tokens, new_emb_size, new_embedding_shapes, layer):
embeddings = self.get_input_embeddings()
for i in range(layer, len(embeddings.cutoffs)):
embeddings.cutoffs[i] = sum(new_embedding_shapes[: i + 1])
embeddings.cutoff_ends = [0] + embeddings.cutoffs
embeddings.n_token = new_num_tokens
self.config.cutoffs = embeddings.cutoffs[:-1]
return embeddings.cutoffs
@dataclass
class TransfoXLModelOutput(ModelOutput):
"""
Base class for model's outputs that may also contain a past key/values (to speed up sequential decoding).
Args:
last_hidden_state (`torch.FloatTensor` of shape `(batch_size, sequence_length, hidden_size)`):
Sequence of hidden-states at the output of the last layer of the model.
mems (`List[torch.FloatTensor]` of length `config.n_layers`):
Contains pre-computed hidden-states (key and values in the attention blocks). Can be used (see `mems`
input) to speed up sequential decoding. The token ids which have their past given to this model should not
be passed as input ids as they have already been computed.
hidden_states (`tuple(torch.FloatTensor)`, *optional*, returned when `output_hidden_states=True` is passed or when `config.output_hidden_states=True`):
Tuple of `torch.FloatTensor` (one for the output of the embeddings + one for the output of each layer) of
shape `(batch_size, sequence_length, hidden_size)`.
Hidden-states of the model at the output of each layer plus the initial embedding outputs.
attentions (`tuple(torch.FloatTensor)`, *optional*, returned when `output_attentions=True` is passed or when `config.output_attentions=True`):
Tuple of `torch.FloatTensor` (one for each layer) of shape `(batch_size, num_heads, sequence_length,
sequence_length)`.
Attentions weights after the attention softmax, used to compute the weighted average in the self-attention
heads.
"""
last_hidden_state: torch.FloatTensor
mems: List[torch.FloatTensor] = None
hidden_states: Optional[Tuple[torch.FloatTensor]] = None
attentions: Optional[Tuple[torch.FloatTensor]] = None
@dataclass
class TransfoXLSequenceClassifierOutputWithPast(ModelOutput):
"""
Base class for outputs of sentence classification models.
Args:
loss (`torch.FloatTensor` of shape `(1,)`, *optional*, returned when `labels` is provided):
Classification (or regression if config.num_labels==1) loss.
logits (`torch.FloatTensor` of shape `(batch_size, config.num_labels)`):
Classification (or regression if config.num_labels==1) scores (before SoftMax).
mems (`List[torch.FloatTensor]` of length `config.n_layers`):
Contains pre-computed hidden-states (key and values in the attention blocks). Can be used (see `mems`
input) to speed up sequential decoding. The token ids which have their past given to this model should not
be passed as input ids as they have already been computed.
hidden_states (`tuple(torch.FloatTensor)`, *optional*, returned when `output_hidden_states=True` is passed or when `config.output_hidden_states=True`):
Tuple of `torch.FloatTensor` (one for the output of the embeddings + one for the output of each layer) of
shape `(batch_size, sequence_length, hidden_size)`.
Hidden-states of the model at the output of each layer plus the initial embedding outputs.
attentions (`tuple(torch.FloatTensor)`, *optional*, returned when `output_attentions=True` is passed or when `config.output_attentions=True`):
Tuple of `torch.FloatTensor` (one for each layer) of shape `(batch_size, num_heads, sequence_length,
sequence_length)`.
Attentions weights after the attention softmax, used to compute the weighted average in the self-attention
heads.
"""
loss: Optional[torch.FloatTensor] = None
logits: torch.FloatTensor = None
mems: List[torch.FloatTensor] = None
hidden_states: Optional[Tuple[torch.FloatTensor]] = None
attentions: Optional[Tuple[torch.FloatTensor]] = None
@dataclass
class TransfoXLLMHeadModelOutput(ModelOutput):
"""
Base class for model's outputs that may also contain a past key/values (to speed up sequential decoding).
Args:
losses (`torch.FloatTensor` of shape *(batch_size, sequence_length-1)*, *optional*, returned when `labels` is provided):
Language modeling losses (not reduced).
prediction_scores (`torch.FloatTensor` of shape `(batch_size, sequence_length, config.vocab_size)`):
Prediction scores of the language modeling head (scores for each vocabulary token after SoftMax).
mems (`List[torch.FloatTensor]` of length `config.n_layers`):
Contains pre-computed hidden-states (key and values in the attention blocks). Can be used (see `mems`
input) to speed up sequential decoding. The token ids which have their past given to this model should not
be passed as input ids as they have already been computed.
hidden_states (`tuple(torch.FloatTensor)`, *optional*, returned when `output_hidden_states=True` is passed or when `config.output_hidden_states=True`):
Tuple of `torch.FloatTensor` (one for the output of the embeddings + one for the output of each layer) of
shape `(batch_size, sequence_length, hidden_size)`.
Hidden-states of the model at the output of each layer plus the initial embedding outputs.
attentions (`tuple(torch.FloatTensor)`, *optional*, returned when `output_attentions=True` is passed or when `config.output_attentions=True`):
Tuple of `torch.FloatTensor` (one for each layer) of shape `(batch_size, num_heads, sequence_length,
sequence_length)`.
Attentions weights after the attention softmax, used to compute the weighted average in the self-attention
heads.
loss (`torch.FloatTensor` of shape `()`, *optional*, returned when `labels` is provided)
Reduced language modeling loss.
"""
losses: Optional[torch.FloatTensor] = None
prediction_scores: torch.FloatTensor = None
mems: List[torch.FloatTensor] = None
hidden_states: Optional[Tuple[torch.FloatTensor]] = None
attentions: Optional[Tuple[torch.FloatTensor]] = None
loss: Optional[torch.FloatTensor] = None
@property
def logits(self):
# prediction scores are the output of the adaptive softmax, see
# the file `modeling_transfo_xl_utilities`. Since the adaptive
# softmax returns the log softmax value, `self.prediction_scores`
# are strictly speaking not exactly `logits`, but behave the same
# way logits do.
return self.prediction_scores
TRANSFO_XL_START_DOCSTRING = r"""
This model inherits from [`PreTrainedModel`]. Check the superclass documentation for the generic methods the
library implements for all its model (such as downloading or saving, resizing the input embeddings, pruning heads
etc.)
This model is also a PyTorch [torch.nn.Module](https://pytorch.org/docs/stable/nn.html#torch.nn.Module) subclass.
Use it as a regular PyTorch Module and refer to the PyTorch documentation for all matter related to general usage
and behavior.
Parameters:
config ([`TransfoXLConfig`]): Model configuration class with all the parameters of the model.
Initializing with a config file does not load the weights associated with the model, only the
configuration. Check out the [`~PreTrainedModel.from_pretrained`] method to load the model weights.
"""
TRANSFO_XL_INPUTS_DOCSTRING = r"""
Args:
input_ids (`torch.LongTensor` of shape `(batch_size, sequence_length)`):
Indices of input sequence tokens in the vocabulary.
Indices can be obtained using [`AutoTokenizer`]. See [`PreTrainedTokenizer.encode`] and
[`PreTrainedTokenizer.__call__`] for details.
[What are input IDs?](../glossary#input-ids)
mems (`List[torch.FloatTensor]` of length `config.n_layers`):
Contains pre-computed hidden-states (key and values in the attention blocks) as computed by the model (see
`mems` output below). Can be used to speed up sequential decoding. The token ids which have their mems
given to this model should not be passed as `input_ids` as they have already been computed.
head_mask (`torch.FloatTensor` of shape `(num_heads,)` or `(num_layers, num_heads)`, *optional*):
Mask to nullify selected heads of the self-attention modules. Mask values selected in `[0, 1]`:
- 1 indicates the head is **not masked**,
- 0 indicates the head is **masked**.
inputs_embeds (`torch.FloatTensor` of shape `(batch_size, sequence_length, hidden_size)`, *optional*):
Optionally, instead of passing `input_ids` you can choose to directly pass an embedded representation. This
is useful if you want more control over how to convert `input_ids` indices into associated vectors than the
model's internal embedding lookup matrix.
output_attentions (`bool`, *optional*):
Whether or not to return the attentions tensors of all attention layers. See `attentions` under returned
tensors for more detail.
output_hidden_states (`bool`, *optional*):
Whether or not to return the hidden states of all layers. See `hidden_states` under returned tensors for
more detail.
return_dict (`bool`, *optional*):
Whether or not to return a [`~utils.ModelOutput`] instead of a plain tuple.
"""
@add_start_docstrings(
"The bare Bert Model transformer outputting raw hidden-states without any specific head on top.",
TRANSFO_XL_START_DOCSTRING,
)
class TransfoXLModel(TransfoXLPreTrainedModel):
def __init__(self, config):
super().__init__(config)
self.n_token = config.vocab_size
self.d_embed = config.d_embed
self.d_model = config.d_model
self.n_head = config.n_head
self.d_head = config.d_head
self.word_emb = AdaptiveEmbedding(
config.vocab_size, config.d_embed, config.d_model, config.cutoffs, div_val=config.div_val
)
self.drop = nn.Dropout(config.dropout)
self.n_layer = config.n_layer
self.mem_len = config.mem_len
self.attn_type = config.attn_type
if not config.untie_r:
self.r_w_bias = nn.Parameter(torch.FloatTensor(self.n_head, self.d_head))
self.r_r_bias = nn.Parameter(torch.FloatTensor(self.n_head, self.d_head))
self.layers = nn.ModuleList()
if config.attn_type == 0: # the default attention
for i in range(config.n_layer):
self.layers.append(
RelPartialLearnableDecoderLayer(
config.n_head,
config.d_model,
config.d_head,
config.d_inner,
config.dropout,
dropatt=config.dropatt,
pre_lnorm=config.pre_lnorm,
r_w_bias=None if config.untie_r else self.r_w_bias,
r_r_bias=None if config.untie_r else self.r_r_bias,
layer_norm_epsilon=config.layer_norm_epsilon,
)
)
else: # learnable embeddings and absolute embeddings are not used in our pretrained checkpoints
raise NotImplementedError # Removed them to avoid maintaining dead code
self.same_length = config.same_length
self.clamp_len = config.clamp_len
if self.attn_type == 0: # default attention
self.pos_emb = PositionalEmbedding(self.d_model)
else: # learnable embeddings and absolute embeddings
raise NotImplementedError # Removed these to avoid maintaining dead code - They are not used in our pretrained checkpoint
# Initialize weights and apply final processing
self.post_init()
def get_input_embeddings(self):
return self.word_emb
def set_input_embeddings(self, new_embeddings):
self.word_emb = new_embeddings
def backward_compatible(self):
self.sample_softmax = -1
def reset_memory_length(self, mem_len):
self.mem_len = mem_len
def _prune_heads(self, heads):
logger.info("Head pruning is not implemented for Transformer-XL model")
pass
def init_mems(self, bsz):
if self.mem_len > 0:
mems = []
param = next(self.parameters())
for i in range(self.n_layer):
empty = torch.zeros(self.mem_len, bsz, self.config.d_model, dtype=param.dtype, device=param.device)
mems.append(empty)
return mems
else:
return None
def _update_mems(self, hids, mems, mlen, qlen):
# does not deal with None
if mems is None:
return None
# mems is not None
assert len(hids) == len(mems), "len(hids) != len(mems)"
# There are `mlen + qlen` steps that can be cached into mems
with torch.no_grad():
new_mems = []
end_idx = mlen + max(0, qlen)
beg_idx = max(0, end_idx - self.mem_len)
for i in range(len(hids)):
cat = torch.cat([mems[i], hids[i]], dim=0)
new_mems.append(cat[beg_idx:end_idx].detach())
return new_mems
@add_start_docstrings_to_model_forward(TRANSFO_XL_INPUTS_DOCSTRING)
@add_code_sample_docstrings(
checkpoint=_CHECKPOINT_FOR_DOC,
output_type=TransfoXLModelOutput,
config_class=_CONFIG_FOR_DOC,
)
def forward(
self,
input_ids: Optional[torch.LongTensor] = None,
mems: Optional[List[torch.FloatTensor]] = None,
head_mask: Optional[torch.FloatTensor] = None,
inputs_embeds: Optional[torch.FloatTensor] = None,
output_attentions: Optional[bool] = None,
output_hidden_states: Optional[bool] = None,
return_dict: Optional[bool] = None,
) -> Union[Tuple, TransfoXLModelOutput]:
output_attentions = output_attentions if output_attentions is not None else self.config.output_attentions
output_hidden_states = (
output_hidden_states if output_hidden_states is not None else self.config.output_hidden_states
)
return_dict = return_dict if return_dict is not None else self.config.use_return_dict
# the original code for Transformer-XL used shapes [len, bsz] but we want a unified interface in the library
# so we transpose here from shape [bsz, len] to shape [len, bsz]
if input_ids is not None and inputs_embeds is not None:
raise ValueError("You cannot specify both input_ids and inputs_embeds at the same time")
elif input_ids is not None:
input_ids = input_ids.transpose(0, 1).contiguous()
qlen, bsz = input_ids.size()
elif inputs_embeds is not None:
inputs_embeds = inputs_embeds.transpose(0, 1).contiguous()
qlen, bsz = inputs_embeds.shape[0], inputs_embeds.shape[1]
else:
raise ValueError("You have to specify either input_ids or inputs_embeds")
if mems is None:
mems = self.init_mems(bsz)
# Prepare head mask if needed
# 1.0 in head_mask indicate we keep the head
# attention_probs has shape bsz x n_heads x N x N
# input head_mask has shape [num_heads] or [num_hidden_layers x num_heads] (a head_mask for each layer)
# and head_mask is converted to shape [num_hidden_layers x qlen x klen x bsz x n_head]
if head_mask is not None:
if head_mask.dim() == 1:
head_mask = head_mask.unsqueeze(0).unsqueeze(0).unsqueeze(0).unsqueeze(0)
head_mask = head_mask.expand(self.n_layer, -1, -1, -1, -1)
elif head_mask.dim() == 2:
head_mask = head_mask.unsqueeze(1).unsqueeze(1).unsqueeze(1)
head_mask = head_mask.to(
dtype=next(self.parameters()).dtype
) # switch to float if need + fp16 compatibility
else:
head_mask = [None] * self.n_layer
if inputs_embeds is not None:
word_emb = inputs_embeds
else:
word_emb = self.word_emb(input_ids)
mlen = mems[0].size(0) if mems is not None else 0
klen = mlen + qlen
if self.same_length:
all_ones = word_emb.new_ones((qlen, klen), dtype=torch.bool)
mask_len = klen - self.mem_len
if mask_len > 0:
mask_shift_len = qlen - mask_len
else:
mask_shift_len = qlen
dec_attn_mask = (torch.triu(all_ones, 1 + mlen) + torch.tril(all_ones, -mask_shift_len))[:, :, None] # -1
else:
dec_attn_mask = torch.triu(word_emb.new_ones((qlen, klen), dtype=torch.bool), diagonal=1 + mlen)[
:, :, None
]
hids = []
attentions = [] if output_attentions else None
if self.attn_type == 0: # default
pos_seq = torch.arange(klen - 1, -1, -1.0, device=word_emb.device, dtype=torch.int64).type_as(
dtype=word_emb.dtype
)
if self.clamp_len > 0:
pos_seq.clamp_(max=self.clamp_len)
pos_emb = self.pos_emb(pos_seq)
core_out = self.drop(word_emb)
pos_emb = self.drop(pos_emb)
for i, layer in enumerate(self.layers):
hids.append(core_out)
mems_i = None if mems is None else mems[i]
layer_outputs = layer(
core_out,
pos_emb,
dec_attn_mask=dec_attn_mask,
mems=mems_i,
head_mask=head_mask[i],
output_attentions=output_attentions,
)
core_out = layer_outputs[0]
if output_attentions:
attentions.append(layer_outputs[1])
else: # learnable embeddings and absolute embeddings
raise NotImplementedError # Removed these to avoid maintaining dead code - They are not used in our pretrained checkpoint
core_out = self.drop(core_out)
new_mems = self._update_mems(hids, mems, mlen, qlen)
if output_hidden_states:
# Add last layer and transpose to library standard shape [bsz, len, hidden_dim]
hids.append(core_out)
hids = tuple(t.transpose(0, 1).contiguous() for t in hids)
else:
hids = None
if output_attentions:
# Transpose to library standard shape [bsz, n_heads, query_seq_len, key_seq_len]
attentions = tuple(t.permute(2, 3, 0, 1).contiguous() for t in attentions)
# We transpose back here to shape [bsz, len, hidden_dim]
core_out = core_out.transpose(0, 1).contiguous()
if not return_dict:
return tuple(v for v in [core_out, new_mems, hids, attentions] if v is not None)
return TransfoXLModelOutput(
last_hidden_state=core_out,
mems=new_mems,
hidden_states=hids,
attentions=attentions,
)
@add_start_docstrings(
"""
The Transformer-XL Model with a language modeling head on top (adaptive softmax with weights tied to the adaptive
input embeddings)
""",
TRANSFO_XL_START_DOCSTRING,
)
class TransfoXLLMHeadModel(TransfoXLPreTrainedModel):
_tied_weights_keys = [r"crit\.out_projs\.\d+", r"crit\.out_layers\.\d+\.weight"]
def __init__(self, config):
super().__init__(config)
self.transformer = TransfoXLModel(config)
self.sample_softmax = config.sample_softmax
self.trainer_compatible = getattr(config, "trainer_compatible", False)
if not self.trainer_compatible:
warnings.warn(
"The output of TransfoXL will be updated in v5 to support a single loss as first argument. In order "
"to use that updated output, please specify `trainer_compatible=True` as your configuration"
" attribute.",
DeprecationWarning,
)
assert self.sample_softmax <= 0, (
"Sampling from the softmax is not implemented yet. Please look at issue: #3310:"
" https://github.com/huggingface/transformers/issues/3310"
)
self.crit = ProjectedAdaptiveLogSoftmax(
config.vocab_size, config.d_embed, config.d_model, config.cutoffs, div_val=config.div_val
)
# Initialize weights and apply final processing
self.post_init()
def tie_weights(self):
"""
Run this to be sure output and input (adaptive) softmax weights are tied
"""
if self.config.tie_word_embeddings:
for i in range(len(self.crit.out_layers)):
self._tie_or_clone_weights(self.crit.out_layers[i], self.transformer.word_emb.emb_layers[i])
if self.config.tie_projs:
for i, tie_proj in enumerate(self.config.tie_projs):
if tie_proj and self.config.div_val == 1 and self.config.d_model != self.config.d_embed:
if self.config.torchscript:
self.crit.out_projs[i] = nn.Parameter(self.transformer.word_emb.emb_projs[0].clone())
else:
self.crit.out_projs[i] = self.transformer.word_emb.emb_projs[0]
elif tie_proj and self.config.div_val != 1:
if self.config.torchscript:
self.crit.out_projs[i] = nn.Parameter(self.transformer.word_emb.emb_projs[i].clone())
else:
self.crit.out_projs[i] = self.transformer.word_emb.emb_projs[i]
def reset_memory_length(self, mem_len):
self.transformer.reset_memory_length(mem_len)
def init_mems(self, bsz):
return self.transformer.init_mems(bsz)
@add_start_docstrings_to_model_forward(TRANSFO_XL_INPUTS_DOCSTRING)
@add_code_sample_docstrings(
checkpoint=_CHECKPOINT_FOR_DOC,
output_type=TransfoXLLMHeadModelOutput,
config_class=_CONFIG_FOR_DOC,
)
def forward(
self,
input_ids: Optional[torch.LongTensor] = None,
mems: Optional[List[torch.FloatTensor]] = None,
head_mask: Optional[torch.FloatTensor] = None,
inputs_embeds: Optional[torch.FloatTensor] = None,
labels: Optional[torch.LongTensor] = None,
output_attentions: Optional[bool] = None,
output_hidden_states: Optional[bool] = None,
return_dict: Optional[bool] = None,
) -> Union[Tuple, TransfoXLLMHeadModelOutput]:
r"""
labels (`torch.LongTensor` of shape `(batch_size, sequence_length)`, *optional*):
Labels for language modeling. Note that the labels **are shifted** inside the model, i.e. you can set
`labels = input_ids` Indices are selected in `[-100, 0, ..., config.vocab_size]` All labels set to `-100`
are ignored (masked), the loss is only computed for labels in `[0, ..., config.vocab_size]`
"""
return_dict = return_dict if return_dict is not None else self.config.use_return_dict
if input_ids is not None:
bsz, tgt_len = input_ids.size(0), input_ids.size(1)
elif inputs_embeds is not None:
bsz, tgt_len = inputs_embeds.size(0), inputs_embeds.size(1)
else:
raise ValueError("You have to specify either input_ids or inputs_embeds")
transformer_outputs = self.transformer(
input_ids,
mems=mems,
head_mask=head_mask,
inputs_embeds=inputs_embeds,
output_attentions=output_attentions,
output_hidden_states=output_hidden_states,
return_dict=return_dict,
)
last_hidden = transformer_outputs[0]
pred_hid = last_hidden[:, -tgt_len:]
if labels is not None:
# Prevents all labels being -100 and throwing an error
# when backwarding the loss
miss_valid_label = labels[0, 1:].sum() == (labels.size(1) - 1) * -100
if miss_valid_label:
# Sets an <EOS> token, just to prevent loss from being NaN
labels[0, 1] = self.config.eos_token_id
softmax_output = self.crit(pred_hid, labels)
prediction_scores = softmax_output.view(bsz, tgt_len, -1) if labels is None else ()
if labels is not None:
losses = softmax_output.view(bsz, tgt_len - 1)
# Avoids from incorporating padding (-100) tokens into loss value
loss = losses[losses != 0].mean()
else:
losses, loss = None, None
if not return_dict:
if self.trainer_compatible:
output = (prediction_scores, losses) if losses is not None else (prediction_scores,)
output += transformer_outputs[1:]
return ((loss,) + output) if loss is not None else output
else:
output = (prediction_scores, *transformer_outputs[1:])
output = ((losses,) + output) if losses is not None else output
return (output + (loss,)) if loss is not None else output
return TransfoXLLMHeadModelOutput(
loss=loss,
prediction_scores=prediction_scores,
losses=losses,
mems=transformer_outputs.mems,
hidden_states=transformer_outputs.hidden_states,
attentions=transformer_outputs.attentions,
)
def get_output_embeddings(self):
"""Double-check if you are using adaptive softmax."""
if self.sample_softmax > 0:
return self.out_layer
else:
return self.crit.out_layers[-1]
def prepare_inputs_for_generation(self, input_ids, past_key_values=None, **model_kwargs):
inputs = {}
# if past is defined in model kwargs then use it for faster decoding
if past_key_values:
inputs["mems"] = past_key_values
inputs["input_ids"] = input_ids[:, -1].unsqueeze(-1)
else:
inputs["input_ids"] = input_ids
return inputs
def _resize_cutoffs(self, new_num_tokens, new_emb_size, new_embedding_shapes, layer):
new_cutoffs = super()._resize_cutoffs(new_num_tokens, new_emb_size, new_embedding_shapes, layer)
self.crit.cutoffs = new_cutoffs
self.crit.cutoff_ends = [0] + new_cutoffs
self.crit.n_token = new_num_tokens
@staticmethod
def _reorder_cache(mems: List[torch.Tensor], beam_idx: torch.Tensor) -> List[torch.Tensor]:
"""
This function is used to re-order the `mems` cache if [`~PreTrainedModel.beam_search`] or
[`~PreTrainedModel.beam_sample`] is called. This is required to match `mems` with the correct beam_idx at every
generation step.
"""
return [layer_past.index_select(1, beam_idx.to(layer_past.device)) for layer_past in mems]
@add_start_docstrings(
"""
The Transformer-XL Model transformer with a sequence classification head on top (linear layer).
[`TransfoXLForSequenceClassification`] uses the last token in order to do the classification, as other causal
models (e.g. GPT-1) do.
Since it does classification on the last token, it requires to know the position of the last token. If a
`pad_token_id` is defined in the configuration, it finds the last token that is not a padding token in each row. If
no `pad_token_id` is defined, it simply takes the last value in each row of the batch. Since it cannot guess the
padding tokens when `inputs_embeds` are passed instead of `input_ids`, it does the same (take the last value in
each row of the batch).
""",
TRANSFO_XL_START_DOCSTRING,
)
class TransfoXLForSequenceClassification(TransfoXLPreTrainedModel):
def __init__(self, config):
super().__init__(config)
self.num_labels = config.num_labels
self.transformer = TransfoXLModel(config)
self.score = nn.Linear(config.d_embed, self.num_labels, bias=False)
# Initialize weights and apply final processing
self.post_init()
@add_start_docstrings_to_model_forward(TRANSFO_XL_INPUTS_DOCSTRING)
@add_code_sample_docstrings(
checkpoint=_CHECKPOINT_FOR_DOC,
output_type=TransfoXLSequenceClassifierOutputWithPast,
config_class=_CONFIG_FOR_DOC,
)
def forward(
self,
input_ids: Optional[torch.LongTensor] = None,
mems: Optional[List[torch.FloatTensor]] = None,
head_mask: Optional[torch.FloatTensor] = None,
inputs_embeds: Optional[torch.FloatTensor] = None,
labels: Optional[torch.LongTensor] = None,
output_attentions: Optional[bool] = None,
output_hidden_states: Optional[bool] = None,
return_dict: Optional[bool] = None,
) -> Union[Tuple, TransfoXLSequenceClassifierOutputWithPast]:
r"""
labels (`torch.LongTensor` of shape `(batch_size,)`, *optional*):
Labels for computing the sequence classification/regression loss. Indices should be in `[0, ...,
config.num_labels - 1]`. If `config.num_labels == 1` a regression loss is computed (Mean-Square loss), If
`config.num_labels > 1` a classification loss is computed (Cross-Entropy).
"""
return_dict = return_dict if return_dict is not None else self.config.use_return_dict
transformer_outputs = self.transformer(
input_ids,
mems=mems,
head_mask=head_mask,
inputs_embeds=inputs_embeds,
output_attentions=output_attentions,
output_hidden_states=output_hidden_states,
return_dict=return_dict,
)
hidden_states = transformer_outputs[0]
logits = self.score(hidden_states)
if input_ids is not None:
batch_size, sequence_length = input_ids.shape[:2]
else:
batch_size, sequence_length = inputs_embeds.shape[:2]
assert (
self.config.pad_token_id is not None or batch_size == 1
), "Cannot handle batch sizes > 1 if no padding token is defined."
if self.config.pad_token_id is None:
sequence_lengths = -1
else:
if input_ids is not None:
# if no pad token found, use modulo instead of reverse indexing for ONNX compatibility
sequence_lengths = torch.eq(input_ids, self.config.pad_token_id).int().argmax(-1) - 1
sequence_lengths = sequence_lengths % input_ids.shape[-1]
sequence_lengths = sequence_lengths.to(logits.device)
else:
sequence_lengths = -1
logger.warning(
f"{self.__class__.__name__} will not detect padding tokens in `inputs_embeds`. Results may be "
"unexpected if using padding tokens in conjunction with `inputs_embeds.`"
)
pooled_logits = logits[range(batch_size), sequence_lengths]
loss = None
if labels is not None:
if self.config.problem_type is None:
if self.num_labels == 1:
self.config.problem_type = "regression"
elif self.num_labels > 1 and (labels.dtype == torch.long or labels.dtype == torch.int):
self.config.problem_type = "single_label_classification"
else:
self.config.problem_type = "multi_label_classification"
if self.config.problem_type == "regression":
loss_fct = MSELoss()
if self.num_labels == 1:
loss = loss_fct(pooled_logits.squeeze(), labels.squeeze())
else:
loss = loss_fct(pooled_logits, labels)
elif self.config.problem_type == "single_label_classification":
loss_fct = CrossEntropyLoss()
loss = loss_fct(pooled_logits.view(-1, self.num_labels), labels.view(-1))
elif self.config.problem_type == "multi_label_classification":
loss_fct = BCEWithLogitsLoss()
loss = loss_fct(pooled_logits, labels)
if not return_dict:
output = (pooled_logits,) + transformer_outputs[1:]
return ((loss,) + output) if loss is not None else output
return TransfoXLSequenceClassifierOutputWithPast(
loss=loss,
logits=pooled_logits,
mems=transformer_outputs.mems,
hidden_states=transformer_outputs.hidden_states,
attentions=transformer_outputs.attentions,
)
| transformers/src/transformers/models/deprecated/transfo_xl/modeling_transfo_xl.py/0 | {
"file_path": "transformers/src/transformers/models/deprecated/transfo_xl/modeling_transfo_xl.py",
"repo_id": "transformers",
"token_count": 25754
} | 322 |
# coding=utf-8
# Copyright 2022 SenseTime and The HuggingFace Inc. team. All rights reserved.
#
# Licensed under the Apache License, Version 2.0 (the "License");
# you may not use this file except in compliance with the License.
# You may obtain a copy of the License at
#
# http://www.apache.org/licenses/LICENSE-2.0
#
# Unless required by applicable law or agreed to in writing, software
# distributed under the License is distributed on an "AS IS" BASIS,
# WITHOUT WARRANTIES OR CONDITIONS OF ANY KIND, either express or implied.
# See the License for the specific language governing permissions and
# limitations under the License.
""" PyTorch DETA model."""
import copy
import math
import warnings
from dataclasses import dataclass
from typing import Dict, List, Optional, Tuple, Union
import torch
import torch.nn.functional as F
from torch import Tensor, nn
from ...activations import ACT2FN
from ...file_utils import (
ModelOutput,
add_start_docstrings,
add_start_docstrings_to_model_forward,
is_scipy_available,
is_vision_available,
replace_return_docstrings,
)
from ...modeling_attn_mask_utils import _prepare_4d_attention_mask
from ...modeling_outputs import BaseModelOutput
from ...modeling_utils import PreTrainedModel
from ...pytorch_utils import meshgrid
from ...utils import is_accelerate_available, is_torchvision_available, logging, requires_backends
from ...utils.backbone_utils import load_backbone
from .configuration_deta import DetaConfig
logger = logging.get_logger(__name__)
if is_accelerate_available():
from accelerate import PartialState
from accelerate.utils import reduce
if is_vision_available():
from transformers.image_transforms import center_to_corners_format
if is_torchvision_available():
from torchvision.ops.boxes import batched_nms
if is_scipy_available():
from scipy.optimize import linear_sum_assignment
logger = logging.get_logger(__name__)
_CONFIG_FOR_DOC = "DetaConfig"
_CHECKPOINT_FOR_DOC = "jozhang97/deta-swin-large-o365"
DETA_PRETRAINED_MODEL_ARCHIVE_LIST = [
"jozhang97/deta-swin-large-o365",
# See all DETA models at https://huggingface.co/models?filter=deta
]
@dataclass
# Copied from transformers.models.deformable_detr.modeling_deformable_detr.DeformableDetrDecoderOutput with DeformableDetr->Deta
class DetaDecoderOutput(ModelOutput):
"""
Base class for outputs of the DetaDecoder. This class adds two attributes to
BaseModelOutputWithCrossAttentions, namely:
- a stacked tensor of intermediate decoder hidden states (i.e. the output of each decoder layer)
- a stacked tensor of intermediate reference points.
Args:
last_hidden_state (`torch.FloatTensor` of shape `(batch_size, sequence_length, hidden_size)`):
Sequence of hidden-states at the output of the last layer of the model.
intermediate_hidden_states (`torch.FloatTensor` of shape `(batch_size, config.decoder_layers, num_queries, hidden_size)`):
Stacked intermediate hidden states (output of each layer of the decoder).
intermediate_reference_points (`torch.FloatTensor` of shape `(batch_size, config.decoder_layers, sequence_length, hidden_size)`):
Stacked intermediate reference points (reference points of each layer of the decoder).
hidden_states (`tuple(torch.FloatTensor)`, *optional*, returned when `output_hidden_states=True` is passed or when `config.output_hidden_states=True`):
Tuple of `torch.FloatTensor` (one for the output of the embeddings + one for the output of each layer) of
shape `(batch_size, sequence_length, hidden_size)`. Hidden-states of the model at the output of each layer
plus the initial embedding outputs.
attentions (`tuple(torch.FloatTensor)`, *optional*, returned when `output_attentions=True` is passed or when `config.output_attentions=True`):
Tuple of `torch.FloatTensor` (one for each layer) of shape `(batch_size, num_heads, sequence_length,
sequence_length)`. Attentions weights after the attention softmax, used to compute the weighted average in
the self-attention heads.
cross_attentions (`tuple(torch.FloatTensor)`, *optional*, returned when `output_attentions=True` and `config.add_cross_attention=True` is passed or when `config.output_attentions=True`):
Tuple of `torch.FloatTensor` (one for each layer) of shape `(batch_size, num_heads, sequence_length,
sequence_length)`. Attentions weights of the decoder's cross-attention layer, after the attention softmax,
used to compute the weighted average in the cross-attention heads.
"""
last_hidden_state: torch.FloatTensor = None
intermediate_hidden_states: torch.FloatTensor = None
intermediate_reference_points: torch.FloatTensor = None
hidden_states: Optional[Tuple[torch.FloatTensor]] = None
attentions: Optional[Tuple[torch.FloatTensor]] = None
cross_attentions: Optional[Tuple[torch.FloatTensor]] = None
@dataclass
class DetaModelOutput(ModelOutput):
"""
Base class for outputs of the Deformable DETR encoder-decoder model.
Args:
init_reference_points (`torch.FloatTensor` of shape `(batch_size, num_queries, 4)`):
Initial reference points sent through the Transformer decoder.
last_hidden_state (`torch.FloatTensor` of shape `(batch_size, num_queries, hidden_size)`):
Sequence of hidden-states at the output of the last layer of the decoder of the model.
intermediate_hidden_states (`torch.FloatTensor` of shape `(batch_size, config.decoder_layers, num_queries, hidden_size)`):
Stacked intermediate hidden states (output of each layer of the decoder).
intermediate_reference_points (`torch.FloatTensor` of shape `(batch_size, config.decoder_layers, num_queries, 4)`):
Stacked intermediate reference points (reference points of each layer of the decoder).
decoder_hidden_states (`tuple(torch.FloatTensor)`, *optional*, returned when `output_hidden_states=True` is passed or when `config.output_hidden_states=True`):
Tuple of `torch.FloatTensor` (one for the output of the embeddings + one for the output of each layer) of
shape `(batch_size, num_queries, hidden_size)`. Hidden-states of the decoder at the output of each layer
plus the initial embedding outputs.
decoder_attentions (`tuple(torch.FloatTensor)`, *optional*, returned when `output_attentions=True` is passed or when `config.output_attentions=True`):
Tuple of `torch.FloatTensor` (one for each layer) of shape `(batch_size, num_heads, num_queries,
num_queries)`. Attentions weights of the decoder, after the attention softmax, used to compute the weighted
average in the self-attention heads.
cross_attentions (`tuple(torch.FloatTensor)`, *optional*, returned when `output_attentions=True` is passed or when `config.output_attentions=True`):
Tuple of `torch.FloatTensor` (one for each layer) of shape `(batch_size, num_queries, num_heads, 4, 4)`.
Attentions weights of the decoder's cross-attention layer, after the attention softmax, used to compute the
weighted average in the cross-attention heads.
encoder_last_hidden_state (`torch.FloatTensor` of shape `(batch_size, sequence_length, hidden_size)`, *optional*):
Sequence of hidden-states at the output of the last layer of the encoder of the model.
encoder_hidden_states (`tuple(torch.FloatTensor)`, *optional*, returned when `output_hidden_states=True` is passed or when `config.output_hidden_states=True`):
Tuple of `torch.FloatTensor` (one for the output of the embeddings + one for the output of each layer) of
shape `(batch_size, sequence_length, hidden_size)`. Hidden-states of the encoder at the output of each
layer plus the initial embedding outputs.
encoder_attentions (`tuple(torch.FloatTensor)`, *optional*, returned when `output_attentions=True` is passed or when `config.output_attentions=True`):
Tuple of `torch.FloatTensor` (one for each layer) of shape `(batch_size, num_queries, num_heads, 4, 4)`.
Attentions weights of the encoder, after the attention softmax, used to compute the weighted average in the
self-attention heads.
enc_outputs_class (`torch.FloatTensor` of shape `(batch_size, sequence_length, config.num_labels)`, *optional*, returned when `config.with_box_refine=True` and `config.two_stage=True`):
Predicted bounding boxes scores where the top `config.two_stage_num_proposals` scoring bounding boxes are
picked as region proposals in the first stage. Output of bounding box binary classification (i.e.
foreground and background).
enc_outputs_coord_logits (`torch.FloatTensor` of shape `(batch_size, sequence_length, 4)`, *optional*, returned when `config.with_box_refine=True` and `config.two_stage=True`):
Logits of predicted bounding boxes coordinates in the first stage.
output_proposals (`torch.FloatTensor` of shape `(batch_size, sequence_length, 4)`, *optional*, returned when `config.two_stage=True`):
Logits of proposal bounding boxes coordinates in the gen_encoder_output_proposals.
"""
init_reference_points: torch.FloatTensor = None
last_hidden_state: torch.FloatTensor = None
intermediate_hidden_states: torch.FloatTensor = None
intermediate_reference_points: torch.FloatTensor = None
decoder_hidden_states: Optional[Tuple[torch.FloatTensor]] = None
decoder_attentions: Optional[Tuple[torch.FloatTensor]] = None
cross_attentions: Optional[Tuple[torch.FloatTensor]] = None
encoder_last_hidden_state: Optional[torch.FloatTensor] = None
encoder_hidden_states: Optional[Tuple[torch.FloatTensor]] = None
encoder_attentions: Optional[Tuple[torch.FloatTensor]] = None
enc_outputs_class: Optional[torch.FloatTensor] = None
enc_outputs_coord_logits: Optional[torch.FloatTensor] = None
output_proposals: Optional[torch.FloatTensor] = None
@dataclass
class DetaObjectDetectionOutput(ModelOutput):
"""
Output type of [`DetaForObjectDetection`].
Args:
loss (`torch.FloatTensor` of shape `(1,)`, *optional*, returned when `labels` are provided)):
Total loss as a linear combination of a negative log-likehood (cross-entropy) for class prediction and a
bounding box loss. The latter is defined as a linear combination of the L1 loss and the generalized
scale-invariant IoU loss.
loss_dict (`Dict`, *optional*):
A dictionary containing the individual losses. Useful for logging.
logits (`torch.FloatTensor` of shape `(batch_size, num_queries, num_classes + 1)`):
Classification logits (including no-object) for all queries.
pred_boxes (`torch.FloatTensor` of shape `(batch_size, num_queries, 4)`):
Normalized boxes coordinates for all queries, represented as (center_x, center_y, width, height). These
values are normalized in [0, 1], relative to the size of each individual image in the batch (disregarding
possible padding). You can use [`~DetaProcessor.post_process_object_detection`] to retrieve the
unnormalized bounding boxes.
auxiliary_outputs (`list[Dict]`, *optional*):
Optional, only returned when auxilary losses are activated (i.e. `config.auxiliary_loss` is set to `True`)
and labels are provided. It is a list of dictionaries containing the two above keys (`logits` and
`pred_boxes`) for each decoder layer.
last_hidden_state (`torch.FloatTensor` of shape `(batch_size, num_queries, hidden_size)`, *optional*):
Sequence of hidden-states at the output of the last layer of the decoder of the model.
decoder_hidden_states (`tuple(torch.FloatTensor)`, *optional*, returned when `output_hidden_states=True` is passed or when `config.output_hidden_states=True`):
Tuple of `torch.FloatTensor` (one for the output of the embeddings + one for the output of each layer) of
shape `(batch_size, num_queries, hidden_size)`. Hidden-states of the decoder at the output of each layer
plus the initial embedding outputs.
decoder_attentions (`tuple(torch.FloatTensor)`, *optional*, returned when `output_attentions=True` is passed or when `config.output_attentions=True`):
Tuple of `torch.FloatTensor` (one for each layer) of shape `(batch_size, num_heads, num_queries,
num_queries)`. Attentions weights of the decoder, after the attention softmax, used to compute the weighted
average in the self-attention heads.
cross_attentions (`tuple(torch.FloatTensor)`, *optional*, returned when `output_attentions=True` is passed or when `config.output_attentions=True`):
Tuple of `torch.FloatTensor` (one for each layer) of shape `(batch_size, num_queries, num_heads, 4, 4)`.
Attentions weights of the decoder's cross-attention layer, after the attention softmax, used to compute the
weighted average in the cross-attention heads.
encoder_last_hidden_state (`torch.FloatTensor` of shape `(batch_size, sequence_length, hidden_size)`, *optional*):
Sequence of hidden-states at the output of the last layer of the encoder of the model.
encoder_hidden_states (`tuple(torch.FloatTensor)`, *optional*, returned when `output_hidden_states=True` is passed or when `config.output_hidden_states=True`):
Tuple of `torch.FloatTensor` (one for the output of the embeddings + one for the output of each layer) of
shape `(batch_size, sequence_length, hidden_size)`. Hidden-states of the encoder at the output of each
layer plus the initial embedding outputs.
encoder_attentions (`tuple(torch.FloatTensor)`, *optional*, returned when `output_attentions=True` is passed or when `config.output_attentions=True`):
Tuple of `torch.FloatTensor` (one for each layer) of shape `(batch_size, sequence_length, num_heads, 4,
4)`. Attentions weights of the encoder, after the attention softmax, used to compute the weighted average
in the self-attention heads.
intermediate_hidden_states (`torch.FloatTensor` of shape `(batch_size, config.decoder_layers, num_queries, hidden_size)`):
Stacked intermediate hidden states (output of each layer of the decoder).
intermediate_reference_points (`torch.FloatTensor` of shape `(batch_size, config.decoder_layers, num_queries, 4)`):
Stacked intermediate reference points (reference points of each layer of the decoder).
init_reference_points (`torch.FloatTensor` of shape `(batch_size, num_queries, 4)`):
Initial reference points sent through the Transformer decoder.
enc_outputs_class (`torch.FloatTensor` of shape `(batch_size, sequence_length, config.num_labels)`, *optional*, returned when `config.with_box_refine=True` and `config.two_stage=True`):
Predicted bounding boxes scores where the top `config.two_stage_num_proposals` scoring bounding boxes are
picked as region proposals in the first stage. Output of bounding box binary classification (i.e.
foreground and background).
enc_outputs_coord_logits (`torch.FloatTensor` of shape `(batch_size, sequence_length, 4)`, *optional*, returned when `config.with_box_refine=True` and `config.two_stage=True`):
Logits of predicted bounding boxes coordinates in the first stage.
output_proposals (`torch.FloatTensor` of shape `(batch_size, sequence_length, 4)`, *optional*, returned when `config.two_stage=True`):
Logits of proposal bounding boxes coordinates in the gen_encoder_output_proposals.
"""
loss: Optional[torch.FloatTensor] = None
loss_dict: Optional[Dict] = None
logits: torch.FloatTensor = None
pred_boxes: torch.FloatTensor = None
auxiliary_outputs: Optional[List[Dict]] = None
init_reference_points: Optional[torch.FloatTensor] = None
last_hidden_state: Optional[torch.FloatTensor] = None
intermediate_hidden_states: Optional[torch.FloatTensor] = None
intermediate_reference_points: Optional[torch.FloatTensor] = None
decoder_hidden_states: Optional[Tuple[torch.FloatTensor]] = None
decoder_attentions: Optional[Tuple[torch.FloatTensor]] = None
cross_attentions: Optional[Tuple[torch.FloatTensor]] = None
encoder_last_hidden_state: Optional[torch.FloatTensor] = None
encoder_hidden_states: Optional[Tuple[torch.FloatTensor]] = None
encoder_attentions: Optional[Tuple[torch.FloatTensor]] = None
enc_outputs_class: Optional = None
enc_outputs_coord_logits: Optional = None
output_proposals: Optional[torch.FloatTensor] = None
def _get_clones(module, N):
return nn.ModuleList([copy.deepcopy(module) for i in range(N)])
def inverse_sigmoid(x, eps=1e-5):
x = x.clamp(min=0, max=1)
x1 = x.clamp(min=eps)
x2 = (1 - x).clamp(min=eps)
return torch.log(x1 / x2)
# Copied from transformers.models.detr.modeling_detr.DetrFrozenBatchNorm2d with Detr->Deta
class DetaFrozenBatchNorm2d(nn.Module):
"""
BatchNorm2d where the batch statistics and the affine parameters are fixed.
Copy-paste from torchvision.misc.ops with added eps before rqsrt, without which any other models than
torchvision.models.resnet[18,34,50,101] produce nans.
"""
def __init__(self, n):
super().__init__()
self.register_buffer("weight", torch.ones(n))
self.register_buffer("bias", torch.zeros(n))
self.register_buffer("running_mean", torch.zeros(n))
self.register_buffer("running_var", torch.ones(n))
def _load_from_state_dict(
self, state_dict, prefix, local_metadata, strict, missing_keys, unexpected_keys, error_msgs
):
num_batches_tracked_key = prefix + "num_batches_tracked"
if num_batches_tracked_key in state_dict:
del state_dict[num_batches_tracked_key]
super()._load_from_state_dict(
state_dict, prefix, local_metadata, strict, missing_keys, unexpected_keys, error_msgs
)
def forward(self, x):
# move reshapes to the beginning
# to make it user-friendly
weight = self.weight.reshape(1, -1, 1, 1)
bias = self.bias.reshape(1, -1, 1, 1)
running_var = self.running_var.reshape(1, -1, 1, 1)
running_mean = self.running_mean.reshape(1, -1, 1, 1)
epsilon = 1e-5
scale = weight * (running_var + epsilon).rsqrt()
bias = bias - running_mean * scale
return x * scale + bias
# Copied from transformers.models.detr.modeling_detr.replace_batch_norm with Detr->Deta
def replace_batch_norm(model):
r"""
Recursively replace all `torch.nn.BatchNorm2d` with `DetaFrozenBatchNorm2d`.
Args:
model (torch.nn.Module):
input model
"""
for name, module in model.named_children():
if isinstance(module, nn.BatchNorm2d):
new_module = DetaFrozenBatchNorm2d(module.num_features)
if not module.weight.device == torch.device("meta"):
new_module.weight.data.copy_(module.weight)
new_module.bias.data.copy_(module.bias)
new_module.running_mean.data.copy_(module.running_mean)
new_module.running_var.data.copy_(module.running_var)
model._modules[name] = new_module
if len(list(module.children())) > 0:
replace_batch_norm(module)
class DetaBackboneWithPositionalEncodings(nn.Module):
"""
Backbone model with positional embeddings.
nn.BatchNorm2d layers are replaced by DetaFrozenBatchNorm2d as defined above.
"""
def __init__(self, config):
super().__init__()
backbone = load_backbone(config)
with torch.no_grad():
replace_batch_norm(backbone)
self.model = backbone
self.intermediate_channel_sizes = self.model.channels
# TODO fix this
if config.backbone_config.model_type == "resnet":
for name, parameter in self.model.named_parameters():
if "stages.1" not in name and "stages.2" not in name and "stages.3" not in name:
parameter.requires_grad_(False)
self.position_embedding = build_position_encoding(config)
def forward(self, pixel_values: torch.Tensor, pixel_mask: torch.Tensor):
"""
Outputs feature maps of latter stages C_3 through C_5 in ResNet if `config.num_feature_levels > 1`, otherwise
outputs feature maps of C_5.
"""
# first, send pixel_values through the backbone to get list of feature maps
features = self.model(pixel_values).feature_maps
# next, create position embeddings
out = []
pos = []
for feature_map in features:
# downsample pixel_mask to match shape of corresponding feature_map
mask = nn.functional.interpolate(pixel_mask[None].float(), size=feature_map.shape[-2:]).to(torch.bool)[0]
position_embeddings = self.position_embedding(feature_map, mask).to(feature_map.dtype)
out.append((feature_map, mask))
pos.append(position_embeddings)
return out, pos
# Copied from transformers.models.deformable_detr.modeling_deformable_detr.DeformableDetrSinePositionEmbedding with DeformableDetr->Deta
class DetaSinePositionEmbedding(nn.Module):
"""
This is a more standard version of the position embedding, very similar to the one used by the Attention is all you
need paper, generalized to work on images.
"""
def __init__(self, embedding_dim=64, temperature=10000, normalize=False, scale=None):
super().__init__()
self.embedding_dim = embedding_dim
self.temperature = temperature
self.normalize = normalize
if scale is not None and normalize is False:
raise ValueError("normalize should be True if scale is passed")
if scale is None:
scale = 2 * math.pi
self.scale = scale
def forward(self, pixel_values, pixel_mask):
if pixel_mask is None:
raise ValueError("No pixel mask provided")
y_embed = pixel_mask.cumsum(1, dtype=torch.float32)
x_embed = pixel_mask.cumsum(2, dtype=torch.float32)
if self.normalize:
eps = 1e-6
y_embed = (y_embed - 0.5) / (y_embed[:, -1:, :] + eps) * self.scale
x_embed = (x_embed - 0.5) / (x_embed[:, :, -1:] + eps) * self.scale
dim_t = torch.arange(self.embedding_dim, dtype=torch.int64, device=pixel_values.device).float()
dim_t = self.temperature ** (2 * torch.div(dim_t, 2, rounding_mode="floor") / self.embedding_dim)
pos_x = x_embed[:, :, :, None] / dim_t
pos_y = y_embed[:, :, :, None] / dim_t
pos_x = torch.stack((pos_x[:, :, :, 0::2].sin(), pos_x[:, :, :, 1::2].cos()), dim=4).flatten(3)
pos_y = torch.stack((pos_y[:, :, :, 0::2].sin(), pos_y[:, :, :, 1::2].cos()), dim=4).flatten(3)
pos = torch.cat((pos_y, pos_x), dim=3).permute(0, 3, 1, 2)
return pos
# Copied from transformers.models.detr.modeling_detr.DetrLearnedPositionEmbedding
class DetaLearnedPositionEmbedding(nn.Module):
"""
This module learns positional embeddings up to a fixed maximum size.
"""
def __init__(self, embedding_dim=256):
super().__init__()
self.row_embeddings = nn.Embedding(50, embedding_dim)
self.column_embeddings = nn.Embedding(50, embedding_dim)
def forward(self, pixel_values, pixel_mask=None):
height, width = pixel_values.shape[-2:]
width_values = torch.arange(width, device=pixel_values.device)
height_values = torch.arange(height, device=pixel_values.device)
x_emb = self.column_embeddings(width_values)
y_emb = self.row_embeddings(height_values)
pos = torch.cat([x_emb.unsqueeze(0).repeat(height, 1, 1), y_emb.unsqueeze(1).repeat(1, width, 1)], dim=-1)
pos = pos.permute(2, 0, 1)
pos = pos.unsqueeze(0)
pos = pos.repeat(pixel_values.shape[0], 1, 1, 1)
return pos
# Copied from transformers.models.detr.modeling_detr.build_position_encoding with Detr->Deta
def build_position_encoding(config):
n_steps = config.d_model // 2
if config.position_embedding_type == "sine":
# TODO find a better way of exposing other arguments
position_embedding = DetaSinePositionEmbedding(n_steps, normalize=True)
elif config.position_embedding_type == "learned":
position_embedding = DetaLearnedPositionEmbedding(n_steps)
else:
raise ValueError(f"Not supported {config.position_embedding_type}")
return position_embedding
# Copied from transformers.models.deformable_detr.modeling_deformable_detr.multi_scale_deformable_attention
def multi_scale_deformable_attention(
value: Tensor, value_spatial_shapes: Tensor, sampling_locations: Tensor, attention_weights: Tensor
) -> Tensor:
batch_size, _, num_heads, hidden_dim = value.shape
_, num_queries, num_heads, num_levels, num_points, _ = sampling_locations.shape
value_list = value.split([height.item() * width.item() for height, width in value_spatial_shapes], dim=1)
sampling_grids = 2 * sampling_locations - 1
sampling_value_list = []
for level_id, (height, width) in enumerate(value_spatial_shapes):
# batch_size, height*width, num_heads, hidden_dim
# -> batch_size, height*width, num_heads*hidden_dim
# -> batch_size, num_heads*hidden_dim, height*width
# -> batch_size*num_heads, hidden_dim, height, width
value_l_ = (
value_list[level_id].flatten(2).transpose(1, 2).reshape(batch_size * num_heads, hidden_dim, height, width)
)
# batch_size, num_queries, num_heads, num_points, 2
# -> batch_size, num_heads, num_queries, num_points, 2
# -> batch_size*num_heads, num_queries, num_points, 2
sampling_grid_l_ = sampling_grids[:, :, :, level_id].transpose(1, 2).flatten(0, 1)
# batch_size*num_heads, hidden_dim, num_queries, num_points
sampling_value_l_ = nn.functional.grid_sample(
value_l_, sampling_grid_l_, mode="bilinear", padding_mode="zeros", align_corners=False
)
sampling_value_list.append(sampling_value_l_)
# (batch_size, num_queries, num_heads, num_levels, num_points)
# -> (batch_size, num_heads, num_queries, num_levels, num_points)
# -> (batch_size, num_heads, 1, num_queries, num_levels*num_points)
attention_weights = attention_weights.transpose(1, 2).reshape(
batch_size * num_heads, 1, num_queries, num_levels * num_points
)
output = (
(torch.stack(sampling_value_list, dim=-2).flatten(-2) * attention_weights)
.sum(-1)
.view(batch_size, num_heads * hidden_dim, num_queries)
)
return output.transpose(1, 2).contiguous()
class DetaMultiscaleDeformableAttention(nn.Module):
"""
Multiscale deformable attention as proposed in Deformable DETR.
"""
def __init__(self, embed_dim: int, num_heads: int, n_levels: int, n_points: int):
super().__init__()
if embed_dim % num_heads != 0:
raise ValueError(
f"embed_dim (d_model) must be divisible by num_heads, but got {embed_dim} and {num_heads}"
)
dim_per_head = embed_dim // num_heads
# check if dim_per_head is power of 2
if not ((dim_per_head & (dim_per_head - 1) == 0) and dim_per_head != 0):
warnings.warn(
"You'd better set embed_dim (d_model) in DetaMultiscaleDeformableAttention to make the"
" dimension of each attention head a power of 2 which is more efficient in the authors' CUDA"
" implementation."
)
self.im2col_step = 64
self.d_model = embed_dim
self.n_levels = n_levels
self.n_heads = num_heads
self.n_points = n_points
self.sampling_offsets = nn.Linear(embed_dim, num_heads * n_levels * n_points * 2)
self.attention_weights = nn.Linear(embed_dim, num_heads * n_levels * n_points)
self.value_proj = nn.Linear(embed_dim, embed_dim)
self.output_proj = nn.Linear(embed_dim, embed_dim)
self._reset_parameters()
def _reset_parameters(self):
nn.init.constant_(self.sampling_offsets.weight.data, 0.0)
thetas = torch.arange(self.n_heads, dtype=torch.int64).float() * (2.0 * math.pi / self.n_heads)
grid_init = torch.stack([thetas.cos(), thetas.sin()], -1)
grid_init = (
(grid_init / grid_init.abs().max(-1, keepdim=True)[0])
.view(self.n_heads, 1, 1, 2)
.repeat(1, self.n_levels, self.n_points, 1)
)
for i in range(self.n_points):
grid_init[:, :, i, :] *= i + 1
with torch.no_grad():
self.sampling_offsets.bias = nn.Parameter(grid_init.view(-1))
nn.init.constant_(self.attention_weights.weight.data, 0.0)
nn.init.constant_(self.attention_weights.bias.data, 0.0)
nn.init.xavier_uniform_(self.value_proj.weight.data)
nn.init.constant_(self.value_proj.bias.data, 0.0)
nn.init.xavier_uniform_(self.output_proj.weight.data)
nn.init.constant_(self.output_proj.bias.data, 0.0)
def with_pos_embed(self, tensor: torch.Tensor, position_embeddings: Optional[Tensor]):
return tensor if position_embeddings is None else tensor + position_embeddings
def forward(
self,
hidden_states: torch.Tensor,
attention_mask: Optional[torch.Tensor] = None,
encoder_hidden_states=None,
encoder_attention_mask=None,
position_embeddings: Optional[torch.Tensor] = None,
reference_points=None,
spatial_shapes=None,
level_start_index=None,
output_attentions: bool = False,
):
# add position embeddings to the hidden states before projecting to queries and keys
if position_embeddings is not None:
hidden_states = self.with_pos_embed(hidden_states, position_embeddings)
batch_size, num_queries, _ = hidden_states.shape
batch_size, sequence_length, _ = encoder_hidden_states.shape
if (spatial_shapes[:, 0] * spatial_shapes[:, 1]).sum() != sequence_length:
raise ValueError(
"Make sure to align the spatial shapes with the sequence length of the encoder hidden states"
)
value = self.value_proj(encoder_hidden_states)
if attention_mask is not None:
# we invert the attention_mask
value = value.masked_fill(~attention_mask[..., None], float(0))
value = value.view(batch_size, sequence_length, self.n_heads, self.d_model // self.n_heads)
sampling_offsets = self.sampling_offsets(hidden_states).view(
batch_size, num_queries, self.n_heads, self.n_levels, self.n_points, 2
)
attention_weights = self.attention_weights(hidden_states).view(
batch_size, num_queries, self.n_heads, self.n_levels * self.n_points
)
attention_weights = F.softmax(attention_weights, -1).view(
batch_size, num_queries, self.n_heads, self.n_levels, self.n_points
)
# batch_size, num_queries, n_heads, n_levels, n_points, 2
if reference_points.shape[-1] == 2:
offset_normalizer = torch.stack([spatial_shapes[..., 1], spatial_shapes[..., 0]], -1)
sampling_locations = (
reference_points[:, :, None, :, None, :]
+ sampling_offsets / offset_normalizer[None, None, None, :, None, :]
)
elif reference_points.shape[-1] == 4:
sampling_locations = (
reference_points[:, :, None, :, None, :2]
+ sampling_offsets / self.n_points * reference_points[:, :, None, :, None, 2:] * 0.5
)
else:
raise ValueError(f"Last dim of reference_points must be 2 or 4, but got {reference_points.shape[-1]}")
# PyTorch implementation (for now)
output = multi_scale_deformable_attention(value, spatial_shapes, sampling_locations, attention_weights)
output = self.output_proj(output)
return output, attention_weights
# Copied from transformers.models.deformable_detr.modeling_deformable_detr.DeformableDetrMultiheadAttention with DeformableDetr->Deta,Deformable DETR->DETA
class DetaMultiheadAttention(nn.Module):
"""
Multi-headed attention from 'Attention Is All You Need' paper.
Here, we add position embeddings to the queries and keys (as explained in the Deformable DETR paper).
"""
def __init__(
self,
embed_dim: int,
num_heads: int,
dropout: float = 0.0,
bias: bool = True,
):
super().__init__()
self.embed_dim = embed_dim
self.num_heads = num_heads
self.dropout = dropout
self.head_dim = embed_dim // num_heads
if self.head_dim * num_heads != self.embed_dim:
raise ValueError(
f"embed_dim must be divisible by num_heads (got `embed_dim`: {self.embed_dim} and `num_heads`:"
f" {num_heads})."
)
self.scaling = self.head_dim**-0.5
self.k_proj = nn.Linear(embed_dim, embed_dim, bias=bias)
self.v_proj = nn.Linear(embed_dim, embed_dim, bias=bias)
self.q_proj = nn.Linear(embed_dim, embed_dim, bias=bias)
self.out_proj = nn.Linear(embed_dim, embed_dim, bias=bias)
def _shape(self, tensor: torch.Tensor, seq_len: int, batch_size: int):
return tensor.view(batch_size, seq_len, self.num_heads, self.head_dim).transpose(1, 2).contiguous()
def with_pos_embed(self, tensor: torch.Tensor, position_embeddings: Optional[Tensor]):
return tensor if position_embeddings is None else tensor + position_embeddings
def forward(
self,
hidden_states: torch.Tensor,
attention_mask: Optional[torch.Tensor] = None,
position_embeddings: Optional[torch.Tensor] = None,
output_attentions: bool = False,
) -> Tuple[torch.Tensor, Optional[torch.Tensor], Optional[Tuple[torch.Tensor]]]:
"""Input shape: Batch x Time x Channel"""
batch_size, target_len, embed_dim = hidden_states.size()
# add position embeddings to the hidden states before projecting to queries and keys
if position_embeddings is not None:
hidden_states_original = hidden_states
hidden_states = self.with_pos_embed(hidden_states, position_embeddings)
# get queries, keys and values
query_states = self.q_proj(hidden_states) * self.scaling
key_states = self._shape(self.k_proj(hidden_states), -1, batch_size)
value_states = self._shape(self.v_proj(hidden_states_original), -1, batch_size)
proj_shape = (batch_size * self.num_heads, -1, self.head_dim)
query_states = self._shape(query_states, target_len, batch_size).view(*proj_shape)
key_states = key_states.view(*proj_shape)
value_states = value_states.view(*proj_shape)
source_len = key_states.size(1)
attn_weights = torch.bmm(query_states, key_states.transpose(1, 2))
if attn_weights.size() != (batch_size * self.num_heads, target_len, source_len):
raise ValueError(
f"Attention weights should be of size {(batch_size * self.num_heads, target_len, source_len)}, but is"
f" {attn_weights.size()}"
)
# expand attention_mask
if attention_mask is not None:
# [batch_size, seq_len] -> [batch_size, 1, target_seq_len, source_seq_len]
attention_mask = _prepare_4d_attention_mask(attention_mask, hidden_states.dtype)
if attention_mask is not None:
if attention_mask.size() != (batch_size, 1, target_len, source_len):
raise ValueError(
f"Attention mask should be of size {(batch_size, 1, target_len, source_len)}, but is"
f" {attention_mask.size()}"
)
attn_weights = attn_weights.view(batch_size, self.num_heads, target_len, source_len) + attention_mask
attn_weights = attn_weights.view(batch_size * self.num_heads, target_len, source_len)
attn_weights = nn.functional.softmax(attn_weights, dim=-1)
if output_attentions:
# this operation is a bit awkward, but it's required to
# make sure that attn_weights keeps its gradient.
# In order to do so, attn_weights have to reshaped
# twice and have to be reused in the following
attn_weights_reshaped = attn_weights.view(batch_size, self.num_heads, target_len, source_len)
attn_weights = attn_weights_reshaped.view(batch_size * self.num_heads, target_len, source_len)
else:
attn_weights_reshaped = None
attn_probs = nn.functional.dropout(attn_weights, p=self.dropout, training=self.training)
attn_output = torch.bmm(attn_probs, value_states)
if attn_output.size() != (batch_size * self.num_heads, target_len, self.head_dim):
raise ValueError(
f"`attn_output` should be of size {(batch_size, self.num_heads, target_len, self.head_dim)}, but is"
f" {attn_output.size()}"
)
attn_output = attn_output.view(batch_size, self.num_heads, target_len, self.head_dim)
attn_output = attn_output.transpose(1, 2)
attn_output = attn_output.reshape(batch_size, target_len, embed_dim)
attn_output = self.out_proj(attn_output)
return attn_output, attn_weights_reshaped
class DetaEncoderLayer(nn.Module):
def __init__(self, config: DetaConfig):
super().__init__()
self.embed_dim = config.d_model
self.self_attn = DetaMultiscaleDeformableAttention(
embed_dim=self.embed_dim,
num_heads=config.encoder_attention_heads,
n_levels=config.num_feature_levels,
n_points=config.encoder_n_points,
)
self.self_attn_layer_norm = nn.LayerNorm(self.embed_dim)
self.dropout = config.dropout
self.activation_fn = ACT2FN[config.activation_function]
self.activation_dropout = config.activation_dropout
self.fc1 = nn.Linear(self.embed_dim, config.encoder_ffn_dim)
self.fc2 = nn.Linear(config.encoder_ffn_dim, self.embed_dim)
self.final_layer_norm = nn.LayerNorm(self.embed_dim)
def forward(
self,
hidden_states: torch.Tensor,
attention_mask: torch.Tensor,
position_embeddings: torch.Tensor = None,
reference_points=None,
spatial_shapes=None,
level_start_index=None,
output_attentions: bool = False,
):
"""
Args:
hidden_states (`torch.FloatTensor` of shape `(batch_size, sequence_length, hidden_size)`):
Input to the layer.
attention_mask (`torch.FloatTensor` of shape `(batch_size, sequence_length)`):
Attention mask.
position_embeddings (`torch.FloatTensor`, *optional*):
Position embeddings, to be added to `hidden_states`.
reference_points (`torch.FloatTensor`, *optional*):
Reference points.
spatial_shapes (`torch.LongTensor`, *optional*):
Spatial shapes of the backbone feature maps.
level_start_index (`torch.LongTensor`, *optional*):
Level start index.
output_attentions (`bool`, *optional*):
Whether or not to return the attentions tensors of all attention layers. See `attentions` under
returned tensors for more detail.
"""
residual = hidden_states
# Apply Multi-scale Deformable Attention Module on the multi-scale feature maps.
hidden_states, attn_weights = self.self_attn(
hidden_states=hidden_states,
attention_mask=attention_mask,
encoder_hidden_states=hidden_states,
encoder_attention_mask=attention_mask,
position_embeddings=position_embeddings,
reference_points=reference_points,
spatial_shapes=spatial_shapes,
level_start_index=level_start_index,
output_attentions=output_attentions,
)
hidden_states = nn.functional.dropout(hidden_states, p=self.dropout, training=self.training)
hidden_states = residual + hidden_states
hidden_states = self.self_attn_layer_norm(hidden_states)
residual = hidden_states
hidden_states = self.activation_fn(self.fc1(hidden_states))
hidden_states = nn.functional.dropout(hidden_states, p=self.activation_dropout, training=self.training)
hidden_states = self.fc2(hidden_states)
hidden_states = nn.functional.dropout(hidden_states, p=self.dropout, training=self.training)
hidden_states = residual + hidden_states
hidden_states = self.final_layer_norm(hidden_states)
if self.training:
if torch.isinf(hidden_states).any() or torch.isnan(hidden_states).any():
clamp_value = torch.finfo(hidden_states.dtype).max - 1000
hidden_states = torch.clamp(hidden_states, min=-clamp_value, max=clamp_value)
outputs = (hidden_states,)
if output_attentions:
outputs += (attn_weights,)
return outputs
class DetaDecoderLayer(nn.Module):
def __init__(self, config: DetaConfig):
super().__init__()
self.embed_dim = config.d_model
# self-attention
self.self_attn = DetaMultiheadAttention(
embed_dim=self.embed_dim,
num_heads=config.decoder_attention_heads,
dropout=config.attention_dropout,
)
self.dropout = config.dropout
self.activation_fn = ACT2FN[config.activation_function]
self.activation_dropout = config.activation_dropout
self.self_attn_layer_norm = nn.LayerNorm(self.embed_dim)
# cross-attention
self.encoder_attn = DetaMultiscaleDeformableAttention(
embed_dim=self.embed_dim,
num_heads=config.decoder_attention_heads,
n_levels=config.num_feature_levels,
n_points=config.decoder_n_points,
)
self.encoder_attn_layer_norm = nn.LayerNorm(self.embed_dim)
# feedforward neural networks
self.fc1 = nn.Linear(self.embed_dim, config.decoder_ffn_dim)
self.fc2 = nn.Linear(config.decoder_ffn_dim, self.embed_dim)
self.final_layer_norm = nn.LayerNorm(self.embed_dim)
def forward(
self,
hidden_states: torch.Tensor,
position_embeddings: Optional[torch.Tensor] = None,
reference_points=None,
spatial_shapes=None,
level_start_index=None,
encoder_hidden_states: Optional[torch.Tensor] = None,
encoder_attention_mask: Optional[torch.Tensor] = None,
output_attentions: Optional[bool] = False,
):
"""
Args:
hidden_states (`torch.FloatTensor`):
Input to the layer of shape `(batch, seq_len, embed_dim)`.
position_embeddings (`torch.FloatTensor`, *optional*):
Position embeddings that are added to the queries and keys in the self-attention layer.
reference_points (`torch.FloatTensor`, *optional*):
Reference points.
spatial_shapes (`torch.LongTensor`, *optional*):
Spatial shapes.
level_start_index (`torch.LongTensor`, *optional*):
Level start index.
encoder_hidden_states (`torch.FloatTensor`):
cross attention input to the layer of shape `(batch, seq_len, embed_dim)`
encoder_attention_mask (`torch.FloatTensor`): encoder attention mask of size
`(batch, 1, target_len, source_len)` where padding elements are indicated by very large negative
values.
output_attentions (`bool`, *optional*):
Whether or not to return the attentions tensors of all attention layers. See `attentions` under
returned tensors for more detail.
"""
residual = hidden_states
# Self Attention
hidden_states, self_attn_weights = self.self_attn(
hidden_states=hidden_states,
position_embeddings=position_embeddings,
output_attentions=output_attentions,
)
hidden_states = nn.functional.dropout(hidden_states, p=self.dropout, training=self.training)
hidden_states = residual + hidden_states
hidden_states = self.self_attn_layer_norm(hidden_states)
second_residual = hidden_states
# Cross-Attention
cross_attn_weights = None
hidden_states, cross_attn_weights = self.encoder_attn(
hidden_states=hidden_states,
attention_mask=encoder_attention_mask,
encoder_hidden_states=encoder_hidden_states,
encoder_attention_mask=encoder_attention_mask,
position_embeddings=position_embeddings,
reference_points=reference_points,
spatial_shapes=spatial_shapes,
level_start_index=level_start_index,
output_attentions=output_attentions,
)
hidden_states = nn.functional.dropout(hidden_states, p=self.dropout, training=self.training)
hidden_states = second_residual + hidden_states
hidden_states = self.encoder_attn_layer_norm(hidden_states)
# Fully Connected
residual = hidden_states
hidden_states = self.activation_fn(self.fc1(hidden_states))
hidden_states = nn.functional.dropout(hidden_states, p=self.activation_dropout, training=self.training)
hidden_states = self.fc2(hidden_states)
hidden_states = nn.functional.dropout(hidden_states, p=self.dropout, training=self.training)
hidden_states = residual + hidden_states
hidden_states = self.final_layer_norm(hidden_states)
outputs = (hidden_states,)
if output_attentions:
outputs += (self_attn_weights, cross_attn_weights)
return outputs
# Copied from transformers.models.detr.modeling_detr.DetrClassificationHead
class DetaClassificationHead(nn.Module):
"""Head for sentence-level classification tasks."""
def __init__(self, input_dim: int, inner_dim: int, num_classes: int, pooler_dropout: float):
super().__init__()
self.dense = nn.Linear(input_dim, inner_dim)
self.dropout = nn.Dropout(p=pooler_dropout)
self.out_proj = nn.Linear(inner_dim, num_classes)
def forward(self, hidden_states: torch.Tensor):
hidden_states = self.dropout(hidden_states)
hidden_states = self.dense(hidden_states)
hidden_states = torch.tanh(hidden_states)
hidden_states = self.dropout(hidden_states)
hidden_states = self.out_proj(hidden_states)
return hidden_states
class DetaPreTrainedModel(PreTrainedModel):
config_class = DetaConfig
base_model_prefix = "model"
main_input_name = "pixel_values"
_no_split_modules = [r"DetaBackboneWithPositionalEncodings", r"DetaEncoderLayer", r"DetaDecoderLayer"]
supports_gradient_checkpointing = True
def _init_weights(self, module):
std = self.config.init_std
if isinstance(module, DetaLearnedPositionEmbedding):
nn.init.uniform_(module.row_embeddings.weight)
nn.init.uniform_(module.column_embeddings.weight)
elif isinstance(module, DetaMultiscaleDeformableAttention):
module._reset_parameters()
elif isinstance(module, (nn.Linear, nn.Conv2d, nn.BatchNorm2d)):
# Slightly different from the TF version which uses truncated_normal for initialization
# cf https://github.com/pytorch/pytorch/pull/5617
module.weight.data.normal_(mean=0.0, std=std)
if module.bias is not None:
module.bias.data.zero_()
elif isinstance(module, nn.Embedding):
module.weight.data.normal_(mean=0.0, std=std)
if module.padding_idx is not None:
module.weight.data[module.padding_idx].zero_()
if hasattr(module, "reference_points") and not self.config.two_stage:
nn.init.xavier_uniform_(module.reference_points.weight.data, gain=1.0)
nn.init.constant_(module.reference_points.bias.data, 0.0)
if hasattr(module, "level_embed"):
nn.init.normal_(module.level_embed)
DETA_START_DOCSTRING = r"""
This model inherits from [`PreTrainedModel`]. Check the superclass documentation for the generic methods the
library implements for all its model (such as downloading or saving, resizing the input embeddings, pruning heads
etc.)
This model is also a PyTorch [torch.nn.Module](https://pytorch.org/docs/stable/nn.html#torch.nn.Module) subclass.
Use it as a regular PyTorch Module and refer to the PyTorch documentation for all matter related to general usage
and behavior.
Parameters:
config ([`DetaConfig`]):
Model configuration class with all the parameters of the model. Initializing with a config file does not
load the weights associated with the model, only the configuration. Check out the
[`~PreTrainedModel.from_pretrained`] method to load the model weights.
"""
DETA_INPUTS_DOCSTRING = r"""
Args:
pixel_values (`torch.FloatTensor` of shape `(batch_size, num_channels, height, width)`):
Pixel values. Padding will be ignored by default should you provide it.
Pixel values can be obtained using [`AutoImageProcessor`]. See [`AutoImageProcessor.__call__`] for details.
pixel_mask (`torch.LongTensor` of shape `(batch_size, height, width)`, *optional*):
Mask to avoid performing attention on padding pixel values. Mask values selected in `[0, 1]`:
- 1 for pixels that are real (i.e. **not masked**),
- 0 for pixels that are padding (i.e. **masked**).
[What are attention masks?](../glossary#attention-mask)
decoder_attention_mask (`torch.FloatTensor` of shape `(batch_size, num_queries)`, *optional*):
Not used by default. Can be used to mask object queries.
encoder_outputs (`tuple(tuple(torch.FloatTensor)`, *optional*):
Tuple consists of (`last_hidden_state`, *optional*: `hidden_states`, *optional*: `attentions`)
`last_hidden_state` of shape `(batch_size, sequence_length, hidden_size)`, *optional*) is a sequence of
hidden-states at the output of the last layer of the encoder. Used in the cross-attention of the decoder.
inputs_embeds (`torch.FloatTensor` of shape `(batch_size, sequence_length, hidden_size)`, *optional*):
Optionally, instead of passing the flattened feature map (output of the backbone + projection layer), you
can choose to directly pass a flattened representation of an image.
decoder_inputs_embeds (`torch.FloatTensor` of shape `(batch_size, num_queries, hidden_size)`, *optional*):
Optionally, instead of initializing the queries with a tensor of zeros, you can choose to directly pass an
embedded representation.
output_attentions (`bool`, *optional*):
Whether or not to return the attentions tensors of all attention layers. See `attentions` under returned
tensors for more detail.
output_hidden_states (`bool`, *optional*):
Whether or not to return the hidden states of all layers. See `hidden_states` under returned tensors for
more detail.
return_dict (`bool`, *optional*):
Whether or not to return a [`~file_utils.ModelOutput`] instead of a plain tuple.
"""
class DetaEncoder(DetaPreTrainedModel):
"""
Transformer encoder consisting of *config.encoder_layers* deformable attention layers. Each layer is a
[`DetaEncoderLayer`].
The encoder updates the flattened multi-scale feature maps through multiple deformable attention layers.
Args:
config: DetaConfig
"""
def __init__(self, config: DetaConfig):
super().__init__(config)
self.dropout = config.dropout
self.layers = nn.ModuleList([DetaEncoderLayer(config) for _ in range(config.encoder_layers)])
self.gradient_checkpointing = False
# Initialize weights and apply final processing
self.post_init()
@staticmethod
def get_reference_points(spatial_shapes, valid_ratios, device):
"""
Get reference points for each feature map. Used in decoder.
Args:
spatial_shapes (`torch.LongTensor` of shape `(num_feature_levels, 2)`):
Spatial shapes of each feature map.
valid_ratios (`torch.FloatTensor` of shape `(batch_size, num_feature_levels, 2)`):
Valid ratios of each feature map.
device (`torch.device`):
Device on which to create the tensors.
Returns:
`torch.FloatTensor` of shape `(batch_size, num_queries, num_feature_levels, 2)`
"""
reference_points_list = []
for level, (height, width) in enumerate(spatial_shapes):
ref_y, ref_x = meshgrid(
torch.linspace(0.5, height - 0.5, height, dtype=torch.float32, device=device),
torch.linspace(0.5, width - 0.5, width, dtype=torch.float32, device=device),
indexing="ij",
)
# TODO: valid_ratios could be useless here. check https://github.com/fundamentalvision/Deformable-DETR/issues/36
ref_y = ref_y.reshape(-1)[None] / (valid_ratios[:, None, level, 1] * height)
ref_x = ref_x.reshape(-1)[None] / (valid_ratios[:, None, level, 0] * width)
ref = torch.stack((ref_x, ref_y), -1)
reference_points_list.append(ref)
reference_points = torch.cat(reference_points_list, 1)
reference_points = reference_points[:, :, None] * valid_ratios[:, None]
return reference_points
def forward(
self,
inputs_embeds=None,
attention_mask=None,
position_embeddings=None,
spatial_shapes=None,
level_start_index=None,
valid_ratios=None,
output_attentions=None,
output_hidden_states=None,
return_dict=None,
):
r"""
Args:
inputs_embeds (`torch.FloatTensor` of shape `(batch_size, sequence_length, hidden_size)`):
Flattened feature map (output of the backbone + projection layer) that is passed to the encoder.
attention_mask (`torch.Tensor` of shape `(batch_size, sequence_length)`, *optional*):
Mask to avoid performing attention on padding pixel features. Mask values selected in `[0, 1]`:
- 1 for pixel features that are real (i.e. **not masked**),
- 0 for pixel features that are padding (i.e. **masked**).
[What are attention masks?](../glossary#attention-mask)
position_embeddings (`torch.FloatTensor` of shape `(batch_size, sequence_length, hidden_size)`):
Position embeddings that are added to the queries and keys in each self-attention layer.
spatial_shapes (`torch.LongTensor` of shape `(num_feature_levels, 2)`):
Spatial shapes of each feature map.
level_start_index (`torch.LongTensor` of shape `(num_feature_levels)`):
Starting index of each feature map.
valid_ratios (`torch.FloatTensor` of shape `(batch_size, num_feature_levels, 2)`):
Ratio of valid area in each feature level.
output_attentions (`bool`, *optional*):
Whether or not to return the attentions tensors of all attention layers. See `attentions` under
returned tensors for more detail.
output_hidden_states (`bool`, *optional*):
Whether or not to return the hidden states of all layers. See `hidden_states` under returned tensors
for more detail.
return_dict (`bool`, *optional*):
Whether or not to return a [`~file_utils.ModelOutput`] instead of a plain tuple.
"""
output_attentions = output_attentions if output_attentions is not None else self.config.output_attentions
output_hidden_states = (
output_hidden_states if output_hidden_states is not None else self.config.output_hidden_states
)
return_dict = return_dict if return_dict is not None else self.config.use_return_dict
hidden_states = inputs_embeds
hidden_states = nn.functional.dropout(hidden_states, p=self.dropout, training=self.training)
reference_points = self.get_reference_points(spatial_shapes, valid_ratios, device=inputs_embeds.device)
encoder_states = () if output_hidden_states else None
all_attentions = () if output_attentions else None
for i, encoder_layer in enumerate(self.layers):
if output_hidden_states:
encoder_states = encoder_states + (hidden_states,)
layer_outputs = encoder_layer(
hidden_states,
attention_mask,
position_embeddings=position_embeddings,
reference_points=reference_points,
spatial_shapes=spatial_shapes,
level_start_index=level_start_index,
output_attentions=output_attentions,
)
hidden_states = layer_outputs[0]
if output_attentions:
all_attentions = all_attentions + (layer_outputs[1],)
if output_hidden_states:
encoder_states = encoder_states + (hidden_states,)
if not return_dict:
return tuple(v for v in [hidden_states, encoder_states, all_attentions] if v is not None)
return BaseModelOutput(
last_hidden_state=hidden_states, hidden_states=encoder_states, attentions=all_attentions
)
class DetaDecoder(DetaPreTrainedModel):
"""
Transformer decoder consisting of *config.decoder_layers* layers. Each layer is a [`DetaDecoderLayer`].
The decoder updates the query embeddings through multiple self-attention and cross-attention layers.
Some tweaks for Deformable DETR:
- `position_embeddings`, `reference_points`, `spatial_shapes` and `valid_ratios` are added to the forward pass.
- it also returns a stack of intermediate outputs and reference points from all decoding layers.
Args:
config: DetaConfig
"""
def __init__(self, config: DetaConfig):
super().__init__(config)
self.dropout = config.dropout
self.layers = nn.ModuleList([DetaDecoderLayer(config) for _ in range(config.decoder_layers)])
self.gradient_checkpointing = False
# hack implementation for iterative bounding box refinement and two-stage Deformable DETR
self.bbox_embed = None
self.class_embed = None
# Initialize weights and apply final processing
self.post_init()
def forward(
self,
inputs_embeds=None,
encoder_hidden_states=None,
encoder_attention_mask=None,
position_embeddings=None,
reference_points=None,
spatial_shapes=None,
level_start_index=None,
valid_ratios=None,
output_attentions=None,
output_hidden_states=None,
return_dict=None,
):
r"""
Args:
inputs_embeds (`torch.FloatTensor` of shape `(batch_size, num_queries, hidden_size)`):
The query embeddings that are passed into the decoder.
encoder_hidden_states (`torch.FloatTensor` of shape `(batch_size, sequence_length, hidden_size)`, *optional*):
Sequence of hidden-states at the output of the last layer of the encoder. Used in the cross-attention
of the decoder.
encoder_attention_mask (`torch.LongTensor` of shape `(batch_size, sequence_length)`, *optional*):
Mask to avoid performing cross-attention on padding pixel_values of the encoder. Mask values selected
in `[0, 1]`:
- 1 for pixels that are real (i.e. **not masked**),
- 0 for pixels that are padding (i.e. **masked**).
position_embeddings (`torch.FloatTensor` of shape `(batch_size, num_queries, hidden_size)`, *optional*):
Position embeddings that are added to the queries and keys in each self-attention layer.
reference_points (`torch.FloatTensor` of shape `(batch_size, num_queries, 4)` is `as_two_stage` else `(batch_size, num_queries, 2)` or , *optional*):
Reference point in range `[0, 1]`, top-left (0,0), bottom-right (1, 1), including padding area.
spatial_shapes (`torch.FloatTensor` of shape `(num_feature_levels, 2)`):
Spatial shapes of the feature maps.
level_start_index (`torch.LongTensor` of shape `(num_feature_levels)`, *optional*):
Indexes for the start of each feature level. In range `[0, sequence_length]`.
valid_ratios (`torch.FloatTensor` of shape `(batch_size, num_feature_levels, 2)`, *optional*):
Ratio of valid area in each feature level.
output_attentions (`bool`, *optional*):
Whether or not to return the attentions tensors of all attention layers. See `attentions` under
returned tensors for more detail.
output_hidden_states (`bool`, *optional*):
Whether or not to return the hidden states of all layers. See `hidden_states` under returned tensors
for more detail.
return_dict (`bool`, *optional*):
Whether or not to return a [`~file_utils.ModelOutput`] instead of a plain tuple.
"""
output_attentions = output_attentions if output_attentions is not None else self.config.output_attentions
output_hidden_states = (
output_hidden_states if output_hidden_states is not None else self.config.output_hidden_states
)
return_dict = return_dict if return_dict is not None else self.config.use_return_dict
if inputs_embeds is not None:
hidden_states = inputs_embeds
# decoder layers
all_hidden_states = () if output_hidden_states else None
all_self_attns = () if output_attentions else None
all_cross_attentions = () if (output_attentions and encoder_hidden_states is not None) else None
intermediate = ()
intermediate_reference_points = ()
for idx, decoder_layer in enumerate(self.layers):
if reference_points.shape[-1] == 4:
reference_points_input = (
reference_points[:, :, None] * torch.cat([valid_ratios, valid_ratios], -1)[:, None]
)
else:
if reference_points.shape[-1] != 2:
raise ValueError("Reference points' last dimension must be of size 2")
reference_points_input = reference_points[:, :, None] * valid_ratios[:, None]
if output_hidden_states:
all_hidden_states += (hidden_states,)
if self.gradient_checkpointing and self.training:
layer_outputs = self._gradient_checkpointing_func(
decoder_layer.__call__,
hidden_states,
position_embeddings,
reference_points_input,
spatial_shapes,
level_start_index,
encoder_hidden_states,
encoder_attention_mask,
output_attentions,
)
else:
layer_outputs = decoder_layer(
hidden_states,
position_embeddings=position_embeddings,
encoder_hidden_states=encoder_hidden_states,
reference_points=reference_points_input,
spatial_shapes=spatial_shapes,
level_start_index=level_start_index,
encoder_attention_mask=encoder_attention_mask,
output_attentions=output_attentions,
)
hidden_states = layer_outputs[0]
# hack implementation for iterative bounding box refinement
if self.bbox_embed is not None:
tmp = self.bbox_embed[idx](hidden_states)
if reference_points.shape[-1] == 4:
new_reference_points = tmp + inverse_sigmoid(reference_points)
new_reference_points = new_reference_points.sigmoid()
else:
if reference_points.shape[-1] != 2:
raise ValueError(
f"Reference points' last dimension must be of size 2, but is {reference_points.shape[-1]}"
)
new_reference_points = tmp
new_reference_points[..., :2] = tmp[..., :2] + inverse_sigmoid(reference_points)
new_reference_points = new_reference_points.sigmoid()
reference_points = new_reference_points.detach()
intermediate += (hidden_states,)
intermediate_reference_points += (reference_points,)
if output_attentions:
all_self_attns += (layer_outputs[1],)
if encoder_hidden_states is not None:
all_cross_attentions += (layer_outputs[2],)
# Keep batch_size as first dimension
intermediate = torch.stack(intermediate, dim=1)
intermediate_reference_points = torch.stack(intermediate_reference_points, dim=1)
# add hidden states from the last decoder layer
if output_hidden_states:
all_hidden_states += (hidden_states,)
if not return_dict:
return tuple(
v
for v in [
hidden_states,
intermediate,
intermediate_reference_points,
all_hidden_states,
all_self_attns,
all_cross_attentions,
]
if v is not None
)
return DetaDecoderOutput(
last_hidden_state=hidden_states,
intermediate_hidden_states=intermediate,
intermediate_reference_points=intermediate_reference_points,
hidden_states=all_hidden_states,
attentions=all_self_attns,
cross_attentions=all_cross_attentions,
)
@add_start_docstrings(
"""
The bare DETA Model (consisting of a backbone and encoder-decoder Transformer) outputting raw hidden-states without
any specific head on top.
""",
DETA_START_DOCSTRING,
)
class DetaModel(DetaPreTrainedModel):
def __init__(self, config: DetaConfig):
super().__init__(config)
if config.two_stage:
requires_backends(self, ["torchvision"])
# Create backbone with positional encoding
self.backbone = DetaBackboneWithPositionalEncodings(config)
intermediate_channel_sizes = self.backbone.intermediate_channel_sizes
# Create input projection layers
if config.num_feature_levels > 1:
num_backbone_outs = len(intermediate_channel_sizes)
input_proj_list = []
for _ in range(num_backbone_outs):
in_channels = intermediate_channel_sizes[_]
input_proj_list.append(
nn.Sequential(
nn.Conv2d(in_channels, config.d_model, kernel_size=1),
nn.GroupNorm(32, config.d_model),
)
)
for _ in range(config.num_feature_levels - num_backbone_outs):
input_proj_list.append(
nn.Sequential(
nn.Conv2d(in_channels, config.d_model, kernel_size=3, stride=2, padding=1),
nn.GroupNorm(32, config.d_model),
)
)
in_channels = config.d_model
self.input_proj = nn.ModuleList(input_proj_list)
else:
self.input_proj = nn.ModuleList(
[
nn.Sequential(
nn.Conv2d(intermediate_channel_sizes[-1], config.d_model, kernel_size=1),
nn.GroupNorm(32, config.d_model),
)
]
)
if not config.two_stage:
self.query_position_embeddings = nn.Embedding(config.num_queries, config.d_model * 2)
self.encoder = DetaEncoder(config)
self.decoder = DetaDecoder(config)
self.level_embed = nn.Parameter(torch.Tensor(config.num_feature_levels, config.d_model))
if config.two_stage:
self.enc_output = nn.Linear(config.d_model, config.d_model)
self.enc_output_norm = nn.LayerNorm(config.d_model)
self.pos_trans = nn.Linear(config.d_model * 2, config.d_model * 2)
self.pos_trans_norm = nn.LayerNorm(config.d_model * 2)
self.pix_trans = nn.Linear(config.d_model, config.d_model)
self.pix_trans_norm = nn.LayerNorm(config.d_model)
else:
self.reference_points = nn.Linear(config.d_model, 2)
self.assign_first_stage = config.assign_first_stage
self.two_stage_num_proposals = config.two_stage_num_proposals
self.post_init()
# Copied from transformers.models.deformable_detr.modeling_deformable_detr.DeformableDetrModel.get_encoder
def get_encoder(self):
return self.encoder
# Copied from transformers.models.deformable_detr.modeling_deformable_detr.DeformableDetrModel.get_decoder
def get_decoder(self):
return self.decoder
def freeze_backbone(self):
for name, param in self.backbone.model.named_parameters():
param.requires_grad_(False)
def unfreeze_backbone(self):
for name, param in self.backbone.model.named_parameters():
param.requires_grad_(True)
# Copied from transformers.models.deformable_detr.modeling_deformable_detr.DeformableDetrModel.get_valid_ratio
def get_valid_ratio(self, mask):
"""Get the valid ratio of all feature maps."""
_, height, width = mask.shape
valid_height = torch.sum(mask[:, :, 0], 1)
valid_width = torch.sum(mask[:, 0, :], 1)
valid_ratio_heigth = valid_height.float() / height
valid_ratio_width = valid_width.float() / width
valid_ratio = torch.stack([valid_ratio_width, valid_ratio_heigth], -1)
return valid_ratio
# Copied from transformers.models.deformable_detr.modeling_deformable_detr.DeformableDetrModel.get_proposal_pos_embed
def get_proposal_pos_embed(self, proposals):
"""Get the position embedding of the proposals."""
num_pos_feats = self.config.d_model // 2
temperature = 10000
scale = 2 * math.pi
dim_t = torch.arange(num_pos_feats, dtype=torch.int64, device=proposals.device).float()
dim_t = temperature ** (2 * torch.div(dim_t, 2, rounding_mode="floor") / num_pos_feats)
# batch_size, num_queries, 4
proposals = proposals.sigmoid() * scale
# batch_size, num_queries, 4, 128
pos = proposals[:, :, :, None] / dim_t
# batch_size, num_queries, 4, 64, 2 -> batch_size, num_queries, 512
pos = torch.stack((pos[:, :, :, 0::2].sin(), pos[:, :, :, 1::2].cos()), dim=4).flatten(2)
return pos
def gen_encoder_output_proposals(self, enc_output, padding_mask, spatial_shapes):
"""Generate the encoder output proposals from encoded enc_output.
Args:
enc_output (Tensor[batch_size, sequence_length, hidden_size]): Output of the encoder.
padding_mask (Tensor[batch_size, sequence_length]): Padding mask for `enc_output`.
spatial_shapes (Tensor[num_feature_levels, 2]): Spatial shapes of the feature maps.
Returns:
`tuple(torch.FloatTensor)`: A tuple of feature map and bbox prediction.
- object_query (Tensor[batch_size, sequence_length, hidden_size]): Object query features. Later used to
directly predict a bounding box. (without the need of a decoder)
- output_proposals (Tensor[batch_size, sequence_length, 4]): Normalized proposals, after an inverse
sigmoid.
"""
batch_size = enc_output.shape[0]
proposals = []
_cur = 0
level_ids = []
for level, (height, width) in enumerate(spatial_shapes):
mask_flatten_ = padding_mask[:, _cur : (_cur + height * width)].view(batch_size, height, width, 1)
valid_height = torch.sum(~mask_flatten_[:, :, 0, 0], 1)
valid_width = torch.sum(~mask_flatten_[:, 0, :, 0], 1)
grid_y, grid_x = meshgrid(
torch.linspace(0, height - 1, height, dtype=torch.float32, device=enc_output.device),
torch.linspace(0, width - 1, width, dtype=torch.float32, device=enc_output.device),
indexing="ij",
)
grid = torch.cat([grid_x.unsqueeze(-1), grid_y.unsqueeze(-1)], -1)
scale = torch.cat([valid_width.unsqueeze(-1), valid_height.unsqueeze(-1)], 1).view(batch_size, 1, 1, 2)
grid = (grid.unsqueeze(0).expand(batch_size, -1, -1, -1) + 0.5) / scale
width_heigth = torch.ones_like(grid) * 0.05 * (2.0**level)
proposal = torch.cat((grid, width_heigth), -1).view(batch_size, -1, 4)
proposals.append(proposal)
_cur += height * width
level_ids.append(grid.new_ones(height * width, dtype=torch.long) * level)
output_proposals = torch.cat(proposals, 1)
output_proposals_valid = ((output_proposals > 0.01) & (output_proposals < 0.99)).all(-1, keepdim=True)
output_proposals = torch.log(output_proposals / (1 - output_proposals)) # inverse sigmoid
output_proposals = output_proposals.masked_fill(padding_mask.unsqueeze(-1), float("inf"))
output_proposals = output_proposals.masked_fill(~output_proposals_valid, float("inf"))
# assign each pixel as an object query
object_query = enc_output
object_query = object_query.masked_fill(padding_mask.unsqueeze(-1), float(0))
object_query = object_query.masked_fill(~output_proposals_valid, float(0))
object_query = self.enc_output_norm(self.enc_output(object_query))
level_ids = torch.cat(level_ids)
return object_query, output_proposals, level_ids
@add_start_docstrings_to_model_forward(DETA_INPUTS_DOCSTRING)
@replace_return_docstrings(output_type=DetaModelOutput, config_class=_CONFIG_FOR_DOC)
def forward(
self,
pixel_values: torch.FloatTensor,
pixel_mask: Optional[torch.LongTensor] = None,
decoder_attention_mask: Optional[torch.FloatTensor] = None,
encoder_outputs: Optional[torch.FloatTensor] = None,
inputs_embeds: Optional[torch.FloatTensor] = None,
decoder_inputs_embeds: Optional[torch.FloatTensor] = None,
output_attentions: Optional[bool] = None,
output_hidden_states: Optional[bool] = None,
return_dict: Optional[bool] = None,
) -> Union[Tuple[torch.FloatTensor], DetaModelOutput]:
r"""
Returns:
Examples:
```python
>>> from transformers import AutoImageProcessor, DetaModel
>>> from PIL import Image
>>> import requests
>>> url = "http://images.cocodataset.org/val2017/000000039769.jpg"
>>> image = Image.open(requests.get(url, stream=True).raw)
>>> image_processor = AutoImageProcessor.from_pretrained("jozhang97/deta-swin-large-o365")
>>> model = DetaModel.from_pretrained("jozhang97/deta-swin-large-o365", two_stage=False)
>>> inputs = image_processor(images=image, return_tensors="pt")
>>> outputs = model(**inputs)
>>> last_hidden_states = outputs.last_hidden_state
>>> list(last_hidden_states.shape)
[1, 900, 256]
```"""
output_attentions = output_attentions if output_attentions is not None else self.config.output_attentions
output_hidden_states = (
output_hidden_states if output_hidden_states is not None else self.config.output_hidden_states
)
return_dict = return_dict if return_dict is not None else self.config.use_return_dict
batch_size, num_channels, height, width = pixel_values.shape
device = pixel_values.device
if pixel_mask is None:
pixel_mask = torch.ones(((batch_size, height, width)), dtype=torch.long, device=device)
# Extract multi-scale feature maps of same resolution `config.d_model` (cf Figure 4 in paper)
# First, sent pixel_values + pixel_mask through Backbone to obtain the features
# which is a list of tuples
features, position_embeddings_list = self.backbone(pixel_values, pixel_mask)
# Then, apply 1x1 convolution to reduce the channel dimension to d_model (256 by default)
sources = []
masks = []
for level, (source, mask) in enumerate(features):
sources.append(self.input_proj[level](source))
masks.append(mask)
if mask is None:
raise ValueError("No attention mask was provided")
# Lowest resolution feature maps are obtained via 3x3 stride 2 convolutions on the final stage
if self.config.num_feature_levels > len(sources):
_len_sources = len(sources)
for level in range(_len_sources, self.config.num_feature_levels):
if level == _len_sources:
source = self.input_proj[level](features[-1][0])
else:
source = self.input_proj[level](sources[-1])
mask = nn.functional.interpolate(pixel_mask[None].float(), size=source.shape[-2:]).to(torch.bool)[0]
pos_l = self.backbone.position_embedding(source, mask).to(source.dtype)
sources.append(source)
masks.append(mask)
position_embeddings_list.append(pos_l)
# Create queries
query_embeds = None
if not self.config.two_stage:
query_embeds = self.query_position_embeddings.weight
# Prepare encoder inputs (by flattening)
spatial_shapes = [(source.shape[2:]) for source in sources]
source_flatten = [source.flatten(2).transpose(1, 2) for source in sources]
mask_flatten = [mask.flatten(1) for mask in masks]
lvl_pos_embed_flatten = []
for level, pos_embed in enumerate(position_embeddings_list):
pos_embed = pos_embed.flatten(2).transpose(1, 2)
lvl_pos_embed = pos_embed + self.level_embed[level].view(1, 1, -1)
lvl_pos_embed_flatten.append(lvl_pos_embed)
source_flatten = torch.cat(source_flatten, 1)
mask_flatten = torch.cat(mask_flatten, 1)
lvl_pos_embed_flatten = torch.cat(lvl_pos_embed_flatten, 1)
spatial_shapes = torch.as_tensor(spatial_shapes, dtype=torch.long, device=source_flatten.device)
level_start_index = torch.cat((spatial_shapes.new_zeros((1,)), spatial_shapes.prod(1).cumsum(0)[:-1]))
valid_ratios = torch.stack([self.get_valid_ratio(m) for m in masks], 1)
valid_ratios = valid_ratios.float()
# Fourth, sent source_flatten + mask_flatten + lvl_pos_embed_flatten (backbone + proj layer output) through encoder
# Also provide spatial_shapes, level_start_index and valid_ratios
if encoder_outputs is None:
encoder_outputs = self.encoder(
inputs_embeds=source_flatten,
attention_mask=mask_flatten,
position_embeddings=lvl_pos_embed_flatten,
spatial_shapes=spatial_shapes,
level_start_index=level_start_index,
valid_ratios=valid_ratios,
output_attentions=output_attentions,
output_hidden_states=output_hidden_states,
return_dict=return_dict,
)
# If the user passed a tuple for encoder_outputs, we wrap it in a BaseModelOutput when return_dict=True
elif return_dict and not isinstance(encoder_outputs, BaseModelOutput):
encoder_outputs = BaseModelOutput(
last_hidden_state=encoder_outputs[0],
hidden_states=encoder_outputs[1] if len(encoder_outputs) > 1 else None,
attentions=encoder_outputs[2] if len(encoder_outputs) > 2 else None,
)
# Fifth, prepare decoder inputs
batch_size, _, num_channels = encoder_outputs[0].shape
enc_outputs_class = None
enc_outputs_coord_logits = None
output_proposals = None
if self.config.two_stage:
object_query_embedding, output_proposals, level_ids = self.gen_encoder_output_proposals(
encoder_outputs[0], ~mask_flatten, spatial_shapes
)
# hack implementation for two-stage DETA
# apply a detection head to each pixel (A.4 in paper)
# linear projection for bounding box binary classification (i.e. foreground and background)
enc_outputs_class = self.decoder.class_embed[-1](object_query_embedding)
# 3-layer FFN to predict bounding boxes coordinates (bbox regression branch)
delta_bbox = self.decoder.bbox_embed[-1](object_query_embedding)
enc_outputs_coord_logits = delta_bbox + output_proposals
# only keep top scoring `config.two_stage_num_proposals` proposals
topk = self.two_stage_num_proposals
proposal_logit = enc_outputs_class[..., 0]
if self.assign_first_stage:
proposal_boxes = center_to_corners_format(enc_outputs_coord_logits.sigmoid().float()).clamp(0, 1)
topk_proposals = []
for b in range(batch_size):
prop_boxes_b = proposal_boxes[b]
prop_logits_b = proposal_logit[b]
# pre-nms per-level topk
pre_nms_topk = 1000
pre_nms_inds = []
for lvl in range(len(spatial_shapes)):
lvl_mask = level_ids == lvl
pre_nms_inds.append(torch.topk(prop_logits_b.sigmoid() * lvl_mask, pre_nms_topk)[1])
pre_nms_inds = torch.cat(pre_nms_inds)
# nms on topk indices
post_nms_inds = batched_nms(
prop_boxes_b[pre_nms_inds], prop_logits_b[pre_nms_inds], level_ids[pre_nms_inds], 0.9
)
keep_inds = pre_nms_inds[post_nms_inds]
if len(keep_inds) < self.two_stage_num_proposals:
print(
f"[WARNING] nms proposals ({len(keep_inds)}) < {self.two_stage_num_proposals}, running"
" naive topk"
)
keep_inds = torch.topk(proposal_logit[b], topk)[1]
# keep top Q/L indices for L levels
q_per_l = topk // len(spatial_shapes)
is_level_ordered = (
level_ids[keep_inds][None]
== torch.arange(len(spatial_shapes), device=level_ids.device)[:, None]
)
keep_inds_mask = is_level_ordered & (is_level_ordered.cumsum(1) <= q_per_l) # LS
keep_inds_mask = keep_inds_mask.any(0) # S
# pad to Q indices (might let ones filtered from pre-nms sneak by... unlikely because we pick high conf anyways)
if keep_inds_mask.sum() < topk:
num_to_add = topk - keep_inds_mask.sum()
pad_inds = (~keep_inds_mask).nonzero()[:num_to_add]
keep_inds_mask[pad_inds] = True
keep_inds_topk = keep_inds[keep_inds_mask]
topk_proposals.append(keep_inds_topk)
topk_proposals = torch.stack(topk_proposals)
else:
topk_proposals = torch.topk(enc_outputs_class[..., 0], topk, dim=1)[1]
topk_coords_logits = torch.gather(
enc_outputs_coord_logits, 1, topk_proposals.unsqueeze(-1).repeat(1, 1, 4)
)
topk_coords_logits = topk_coords_logits.detach()
reference_points = topk_coords_logits.sigmoid()
init_reference_points = reference_points
pos_trans_out = self.pos_trans_norm(self.pos_trans(self.get_proposal_pos_embed(topk_coords_logits)))
query_embed, target = torch.split(pos_trans_out, num_channels, dim=2)
topk_feats = torch.stack(
[object_query_embedding[b][topk_proposals[b]] for b in range(batch_size)]
).detach()
target = target + self.pix_trans_norm(self.pix_trans(topk_feats))
else:
query_embed, target = torch.split(query_embeds, num_channels, dim=1)
query_embed = query_embed.unsqueeze(0).expand(batch_size, -1, -1)
target = target.unsqueeze(0).expand(batch_size, -1, -1)
reference_points = self.reference_points(query_embed).sigmoid()
init_reference_points = reference_points
decoder_outputs = self.decoder(
inputs_embeds=target,
position_embeddings=query_embed,
encoder_hidden_states=encoder_outputs[0],
encoder_attention_mask=mask_flatten,
reference_points=reference_points,
spatial_shapes=spatial_shapes,
level_start_index=level_start_index,
valid_ratios=valid_ratios,
output_attentions=output_attentions,
output_hidden_states=output_hidden_states,
return_dict=return_dict,
)
if not return_dict:
enc_outputs = tuple(value for value in [enc_outputs_class, enc_outputs_coord_logits] if value is not None)
tuple_outputs = (init_reference_points,) + decoder_outputs + encoder_outputs + enc_outputs
return tuple_outputs
return DetaModelOutput(
init_reference_points=init_reference_points,
last_hidden_state=decoder_outputs.last_hidden_state,
intermediate_hidden_states=decoder_outputs.intermediate_hidden_states,
intermediate_reference_points=decoder_outputs.intermediate_reference_points,
decoder_hidden_states=decoder_outputs.hidden_states,
decoder_attentions=decoder_outputs.attentions,
cross_attentions=decoder_outputs.cross_attentions,
encoder_last_hidden_state=encoder_outputs.last_hidden_state,
encoder_hidden_states=encoder_outputs.hidden_states,
encoder_attentions=encoder_outputs.attentions,
enc_outputs_class=enc_outputs_class,
enc_outputs_coord_logits=enc_outputs_coord_logits,
output_proposals=output_proposals,
)
@add_start_docstrings(
"""
DETA Model (consisting of a backbone and encoder-decoder Transformer) with object detection heads on top, for tasks
such as COCO detection.
""",
DETA_START_DOCSTRING,
)
class DetaForObjectDetection(DetaPreTrainedModel):
# When using clones, all layers > 0 will be clones, but layer 0 *is* required
_tied_weights_keys = [r"bbox_embed\.\d+"]
# We can't initialize the model on meta device as some weights are modified during the initialization
_no_split_modules = None
# Copied from transformers.models.deformable_detr.modeling_deformable_detr.DeformableDetrForObjectDetection.__init__ with DeformableDetr->Deta
def __init__(self, config: DetaConfig):
super().__init__(config)
# Deformable DETR encoder-decoder model
self.model = DetaModel(config)
# Detection heads on top
self.class_embed = nn.Linear(config.d_model, config.num_labels)
self.bbox_embed = DetaMLPPredictionHead(
input_dim=config.d_model, hidden_dim=config.d_model, output_dim=4, num_layers=3
)
prior_prob = 0.01
bias_value = -math.log((1 - prior_prob) / prior_prob)
self.class_embed.bias.data = torch.ones(config.num_labels) * bias_value
nn.init.constant_(self.bbox_embed.layers[-1].weight.data, 0)
nn.init.constant_(self.bbox_embed.layers[-1].bias.data, 0)
# if two-stage, the last class_embed and bbox_embed is for region proposal generation
num_pred = (config.decoder_layers + 1) if config.two_stage else config.decoder_layers
if config.with_box_refine:
self.class_embed = _get_clones(self.class_embed, num_pred)
self.bbox_embed = _get_clones(self.bbox_embed, num_pred)
nn.init.constant_(self.bbox_embed[0].layers[-1].bias.data[2:], -2.0)
# hack implementation for iterative bounding box refinement
self.model.decoder.bbox_embed = self.bbox_embed
else:
nn.init.constant_(self.bbox_embed.layers[-1].bias.data[2:], -2.0)
self.class_embed = nn.ModuleList([self.class_embed for _ in range(num_pred)])
self.bbox_embed = nn.ModuleList([self.bbox_embed for _ in range(num_pred)])
self.model.decoder.bbox_embed = None
if config.two_stage:
# hack implementation for two-stage
self.model.decoder.class_embed = self.class_embed
for box_embed in self.bbox_embed:
nn.init.constant_(box_embed.layers[-1].bias.data[2:], 0.0)
# Initialize weights and apply final processing
self.post_init()
@torch.jit.unused
def _set_aux_loss(self, outputs_class, outputs_coord):
# this is a workaround to make torchscript happy, as torchscript
# doesn't support dictionary with non-homogeneous values, such
# as a dict having both a Tensor and a list.
aux_loss = [
{"logits": logits, "pred_boxes": pred_boxes}
for logits, pred_boxes in zip(outputs_class.transpose(0, 1)[:-1], outputs_coord.transpose(0, 1)[:-1])
]
return aux_loss
@add_start_docstrings_to_model_forward(DETA_INPUTS_DOCSTRING)
@replace_return_docstrings(output_type=DetaObjectDetectionOutput, config_class=_CONFIG_FOR_DOC)
def forward(
self,
pixel_values: torch.FloatTensor,
pixel_mask: Optional[torch.LongTensor] = None,
decoder_attention_mask: Optional[torch.FloatTensor] = None,
encoder_outputs: Optional[torch.FloatTensor] = None,
inputs_embeds: Optional[torch.FloatTensor] = None,
decoder_inputs_embeds: Optional[torch.FloatTensor] = None,
labels: Optional[List[dict]] = None,
output_attentions: Optional[bool] = None,
output_hidden_states: Optional[bool] = None,
return_dict: Optional[bool] = None,
) -> Union[Tuple[torch.FloatTensor], DetaObjectDetectionOutput]:
r"""
labels (`List[Dict]` of len `(batch_size,)`, *optional*):
Labels for computing the bipartite matching loss. List of dicts, each dictionary containing at least the
following 2 keys: 'class_labels' and 'boxes' (the class labels and bounding boxes of an image in the batch
respectively). The class labels themselves should be a `torch.LongTensor` of len `(number of bounding boxes
in the image,)` and the boxes a `torch.FloatTensor` of shape `(number of bounding boxes in the image, 4)`.
Returns:
Examples:
```python
>>> from transformers import AutoImageProcessor, DetaForObjectDetection
>>> from PIL import Image
>>> import requests
>>> url = "http://images.cocodataset.org/val2017/000000039769.jpg"
>>> image = Image.open(requests.get(url, stream=True).raw)
>>> image_processor = AutoImageProcessor.from_pretrained("jozhang97/deta-swin-large")
>>> model = DetaForObjectDetection.from_pretrained("jozhang97/deta-swin-large")
>>> inputs = image_processor(images=image, return_tensors="pt")
>>> outputs = model(**inputs)
>>> # convert outputs (bounding boxes and class logits) to Pascal VOC format (xmin, ymin, xmax, ymax)
>>> target_sizes = torch.tensor([image.size[::-1]])
>>> results = image_processor.post_process_object_detection(outputs, threshold=0.5, target_sizes=target_sizes)[
... 0
... ]
>>> for score, label, box in zip(results["scores"], results["labels"], results["boxes"]):
... box = [round(i, 2) for i in box.tolist()]
... print(
... f"Detected {model.config.id2label[label.item()]} with confidence "
... f"{round(score.item(), 3)} at location {box}"
... )
Detected cat with confidence 0.683 at location [345.85, 23.68, 639.86, 372.83]
Detected cat with confidence 0.683 at location [8.8, 52.49, 316.93, 473.45]
Detected remote with confidence 0.568 at location [40.02, 73.75, 175.96, 117.33]
Detected remote with confidence 0.546 at location [333.68, 77.13, 370.12, 187.51]
```"""
return_dict = return_dict if return_dict is not None else self.config.use_return_dict
# First, sent images through DETR base model to obtain encoder + decoder outputs
outputs = self.model(
pixel_values,
pixel_mask=pixel_mask,
decoder_attention_mask=decoder_attention_mask,
encoder_outputs=encoder_outputs,
inputs_embeds=inputs_embeds,
decoder_inputs_embeds=decoder_inputs_embeds,
output_attentions=output_attentions,
output_hidden_states=output_hidden_states,
return_dict=return_dict,
)
hidden_states = outputs.intermediate_hidden_states if return_dict else outputs[2]
init_reference = outputs.init_reference_points if return_dict else outputs[0]
inter_references = outputs.intermediate_reference_points if return_dict else outputs[3]
# class logits + predicted bounding boxes
outputs_classes = []
outputs_coords = []
for level in range(hidden_states.shape[1]):
if level == 0:
reference = init_reference
else:
reference = inter_references[:, level - 1]
reference = inverse_sigmoid(reference)
outputs_class = self.class_embed[level](hidden_states[:, level])
delta_bbox = self.bbox_embed[level](hidden_states[:, level])
if reference.shape[-1] == 4:
outputs_coord_logits = delta_bbox + reference
elif reference.shape[-1] == 2:
delta_bbox[..., :2] += reference
outputs_coord_logits = delta_bbox
else:
raise ValueError(f"reference.shape[-1] should be 4 or 2, but got {reference.shape[-1]}")
outputs_coord = outputs_coord_logits.sigmoid()
outputs_classes.append(outputs_class)
outputs_coords.append(outputs_coord)
# Keep batch_size as first dimension
outputs_class = torch.stack(outputs_classes, dim=1)
outputs_coord = torch.stack(outputs_coords, dim=1)
logits = outputs_class[:, -1]
pred_boxes = outputs_coord[:, -1]
loss, loss_dict, auxiliary_outputs = None, None, None
if labels is not None:
# First: create the matcher
matcher = DetaHungarianMatcher(
class_cost=self.config.class_cost, bbox_cost=self.config.bbox_cost, giou_cost=self.config.giou_cost
)
# Second: create the criterion
losses = ["labels", "boxes", "cardinality"]
criterion = DetaLoss(
matcher=matcher,
num_classes=self.config.num_labels,
focal_alpha=self.config.focal_alpha,
losses=losses,
num_queries=self.config.num_queries,
assign_first_stage=self.config.assign_first_stage,
assign_second_stage=self.config.assign_second_stage,
)
criterion.to(logits.device)
# Third: compute the losses, based on outputs and labels
outputs_loss = {}
outputs_loss["logits"] = logits
outputs_loss["pred_boxes"] = pred_boxes
outputs_loss["init_reference"] = init_reference
if self.config.auxiliary_loss:
auxiliary_outputs = self._set_aux_loss(outputs_class, outputs_coord)
outputs_loss["auxiliary_outputs"] = auxiliary_outputs
if self.config.two_stage:
enc_outputs_coord = outputs.enc_outputs_coord_logits.sigmoid()
outputs_loss["enc_outputs"] = {
"logits": outputs.enc_outputs_class,
"pred_boxes": enc_outputs_coord,
"anchors": outputs.output_proposals.sigmoid(),
}
loss_dict = criterion(outputs_loss, labels)
# Fourth: compute total loss, as a weighted sum of the various losses
weight_dict = {"loss_ce": 1, "loss_bbox": self.config.bbox_loss_coefficient}
weight_dict["loss_giou"] = self.config.giou_loss_coefficient
if self.config.auxiliary_loss:
aux_weight_dict = {}
for i in range(self.config.decoder_layers - 1):
aux_weight_dict.update({k + f"_{i}": v for k, v in weight_dict.items()})
aux_weight_dict.update({k + "_enc": v for k, v in weight_dict.items()})
weight_dict.update(aux_weight_dict)
loss = sum(loss_dict[k] * weight_dict[k] for k in loss_dict.keys() if k in weight_dict)
if not return_dict:
if auxiliary_outputs is not None:
output = (logits, pred_boxes) + auxiliary_outputs + outputs
else:
output = (logits, pred_boxes) + outputs
tuple_outputs = ((loss, loss_dict) + output) if loss is not None else output
return tuple_outputs
dict_outputs = DetaObjectDetectionOutput(
loss=loss,
loss_dict=loss_dict,
logits=logits,
pred_boxes=pred_boxes,
auxiliary_outputs=auxiliary_outputs,
last_hidden_state=outputs.last_hidden_state,
decoder_hidden_states=outputs.decoder_hidden_states,
decoder_attentions=outputs.decoder_attentions,
cross_attentions=outputs.cross_attentions,
encoder_last_hidden_state=outputs.encoder_last_hidden_state,
encoder_hidden_states=outputs.encoder_hidden_states,
encoder_attentions=outputs.encoder_attentions,
intermediate_hidden_states=outputs.intermediate_hidden_states,
intermediate_reference_points=outputs.intermediate_reference_points,
init_reference_points=outputs.init_reference_points,
enc_outputs_class=outputs.enc_outputs_class,
enc_outputs_coord_logits=outputs.enc_outputs_coord_logits,
output_proposals=outputs.output_proposals,
)
return dict_outputs
# Copied from transformers.models.detr.modeling_detr.dice_loss
def dice_loss(inputs, targets, num_boxes):
"""
Compute the DICE loss, similar to generalized IOU for masks
Args:
inputs: A float tensor of arbitrary shape.
The predictions for each example.
targets: A float tensor with the same shape as inputs. Stores the binary
classification label for each element in inputs (0 for the negative class and 1 for the positive
class).
"""
inputs = inputs.sigmoid()
inputs = inputs.flatten(1)
numerator = 2 * (inputs * targets).sum(1)
denominator = inputs.sum(-1) + targets.sum(-1)
loss = 1 - (numerator + 1) / (denominator + 1)
return loss.sum() / num_boxes
# Copied from transformers.models.detr.modeling_detr.sigmoid_focal_loss
def sigmoid_focal_loss(inputs, targets, num_boxes, alpha: float = 0.25, gamma: float = 2):
"""
Loss used in RetinaNet for dense detection: https://arxiv.org/abs/1708.02002.
Args:
inputs (`torch.FloatTensor` of arbitrary shape):
The predictions for each example.
targets (`torch.FloatTensor` with the same shape as `inputs`)
A tensor storing the binary classification label for each element in the `inputs` (0 for the negative class
and 1 for the positive class).
alpha (`float`, *optional*, defaults to `0.25`):
Optional weighting factor in the range (0,1) to balance positive vs. negative examples.
gamma (`int`, *optional*, defaults to `2`):
Exponent of the modulating factor (1 - p_t) to balance easy vs hard examples.
Returns:
Loss tensor
"""
prob = inputs.sigmoid()
ce_loss = nn.functional.binary_cross_entropy_with_logits(inputs, targets, reduction="none")
# add modulating factor
p_t = prob * targets + (1 - prob) * (1 - targets)
loss = ce_loss * ((1 - p_t) ** gamma)
if alpha >= 0:
alpha_t = alpha * targets + (1 - alpha) * (1 - targets)
loss = alpha_t * loss
return loss.mean(1).sum() / num_boxes
class DetaLoss(nn.Module):
"""
This class computes the losses for `DetaForObjectDetection`. The process happens in two steps: 1) we compute
hungarian assignment between ground truth boxes and the outputs of the model 2) we supervise each pair of matched
ground-truth / prediction (supervised class and box).
Args:
matcher (`DetaHungarianMatcher`):
Module able to compute a matching between targets and proposals.
num_classes (`int`):
Number of object categories, omitting the special no-object category.
focal_alpha (`float`):
Alpha parameter in focal loss.
losses (`List[str]`):
List of all the losses to be applied. See `get_loss` for a list of all available losses.
"""
def __init__(
self,
matcher,
num_classes,
focal_alpha,
losses,
num_queries,
assign_first_stage=False,
assign_second_stage=False,
):
super().__init__()
self.matcher = matcher
self.num_classes = num_classes
self.focal_alpha = focal_alpha
self.losses = losses
self.assign_first_stage = assign_first_stage
self.assign_second_stage = assign_second_stage
if self.assign_first_stage:
self.stg1_assigner = DetaStage1Assigner()
if self.assign_second_stage:
self.stg2_assigner = DetaStage2Assigner(num_queries)
# Copied from transformers.models.deformable_detr.modeling_deformable_detr.DeformableDetrLoss.loss_labels
def loss_labels(self, outputs, targets, indices, num_boxes):
"""
Classification loss (Binary focal loss) targets dicts must contain the key "class_labels" containing a tensor
of dim [nb_target_boxes]
"""
if "logits" not in outputs:
raise KeyError("No logits were found in the outputs")
source_logits = outputs["logits"]
idx = self._get_source_permutation_idx(indices)
target_classes_o = torch.cat([t["class_labels"][J] for t, (_, J) in zip(targets, indices)])
target_classes = torch.full(
source_logits.shape[:2], self.num_classes, dtype=torch.int64, device=source_logits.device
)
target_classes[idx] = target_classes_o
target_classes_onehot = torch.zeros(
[source_logits.shape[0], source_logits.shape[1], source_logits.shape[2] + 1],
dtype=source_logits.dtype,
layout=source_logits.layout,
device=source_logits.device,
)
target_classes_onehot.scatter_(2, target_classes.unsqueeze(-1), 1)
target_classes_onehot = target_classes_onehot[:, :, :-1]
loss_ce = (
sigmoid_focal_loss(source_logits, target_classes_onehot, num_boxes, alpha=self.focal_alpha, gamma=2)
* source_logits.shape[1]
)
losses = {"loss_ce": loss_ce}
return losses
@torch.no_grad()
# Copied from transformers.models.deformable_detr.modeling_deformable_detr.DeformableDetrLoss.loss_cardinality
def loss_cardinality(self, outputs, targets, indices, num_boxes):
"""
Compute the cardinality error, i.e. the absolute error in the number of predicted non-empty boxes.
This is not really a loss, it is intended for logging purposes only. It doesn't propagate gradients.
"""
logits = outputs["logits"]
device = logits.device
target_lengths = torch.as_tensor([len(v["class_labels"]) for v in targets], device=device)
# Count the number of predictions that are NOT "no-object" (which is the last class)
card_pred = (logits.argmax(-1) != logits.shape[-1] - 1).sum(1)
card_err = nn.functional.l1_loss(card_pred.float(), target_lengths.float())
losses = {"cardinality_error": card_err}
return losses
# Copied from transformers.models.deformable_detr.modeling_deformable_detr.DeformableDetrLoss.loss_boxes
def loss_boxes(self, outputs, targets, indices, num_boxes):
"""
Compute the losses related to the bounding boxes, the L1 regression loss and the GIoU loss.
Targets dicts must contain the key "boxes" containing a tensor of dim [nb_target_boxes, 4]. The target boxes
are expected in format (center_x, center_y, w, h), normalized by the image size.
"""
if "pred_boxes" not in outputs:
raise KeyError("No predicted boxes found in outputs")
idx = self._get_source_permutation_idx(indices)
source_boxes = outputs["pred_boxes"][idx]
target_boxes = torch.cat([t["boxes"][i] for t, (_, i) in zip(targets, indices)], dim=0)
loss_bbox = nn.functional.l1_loss(source_boxes, target_boxes, reduction="none")
losses = {}
losses["loss_bbox"] = loss_bbox.sum() / num_boxes
loss_giou = 1 - torch.diag(
generalized_box_iou(center_to_corners_format(source_boxes), center_to_corners_format(target_boxes))
)
losses["loss_giou"] = loss_giou.sum() / num_boxes
return losses
# Copied from transformers.models.deformable_detr.modeling_deformable_detr.DeformableDetrLoss._get_source_permutation_idx
def _get_source_permutation_idx(self, indices):
# permute predictions following indices
batch_idx = torch.cat([torch.full_like(source, i) for i, (source, _) in enumerate(indices)])
source_idx = torch.cat([source for (source, _) in indices])
return batch_idx, source_idx
# Copied from transformers.models.deformable_detr.modeling_deformable_detr.DeformableDetrLoss._get_target_permutation_idx
def _get_target_permutation_idx(self, indices):
# permute targets following indices
batch_idx = torch.cat([torch.full_like(target, i) for i, (_, target) in enumerate(indices)])
target_idx = torch.cat([target for (_, target) in indices])
return batch_idx, target_idx
# Copied from transformers.models.deformable_detr.modeling_deformable_detr.DeformableDetrLoss.get_loss
def get_loss(self, loss, outputs, targets, indices, num_boxes):
loss_map = {
"labels": self.loss_labels,
"cardinality": self.loss_cardinality,
"boxes": self.loss_boxes,
}
if loss not in loss_map:
raise ValueError(f"Loss {loss} not supported")
return loss_map[loss](outputs, targets, indices, num_boxes)
def forward(self, outputs, targets):
"""
This performs the loss computation.
Args:
outputs (`dict`, *optional*):
Dictionary of tensors, see the output specification of the model for the format.
targets (`List[dict]`, *optional*):
List of dicts, such that `len(targets) == batch_size`. The expected keys in each dict depends on the
losses applied, see each loss' doc.
"""
outputs_without_aux = {k: v for k, v in outputs.items() if k not in ("auxiliary_outputs", "enc_outputs")}
# Retrieve the matching between the outputs of the last layer and the targets
if self.assign_second_stage:
indices = self.stg2_assigner(outputs_without_aux, targets)
else:
indices = self.matcher(outputs_without_aux, targets)
# Compute the average number of target boxes accross all nodes, for normalization purposes
num_boxes = sum(len(t["class_labels"]) for t in targets)
num_boxes = torch.as_tensor([num_boxes], dtype=torch.float, device=next(iter(outputs.values())).device)
# Check that we have initialized the distributed state
world_size = 1
if PartialState._shared_state != {}:
num_boxes = reduce(num_boxes)
world_size = PartialState().num_processes
num_boxes = torch.clamp(num_boxes / world_size, min=1).item()
# Compute all the requested losses
losses = {}
for loss in self.losses:
losses.update(self.get_loss(loss, outputs, targets, indices, num_boxes))
# In case of auxiliary losses, we repeat this process with the output of each intermediate layer.
if "auxiliary_outputs" in outputs:
for i, auxiliary_outputs in enumerate(outputs["auxiliary_outputs"]):
if not self.assign_second_stage:
indices = self.matcher(auxiliary_outputs, targets)
for loss in self.losses:
l_dict = self.get_loss(loss, auxiliary_outputs, targets, indices, num_boxes)
l_dict = {k + f"_{i}": v for k, v in l_dict.items()}
losses.update(l_dict)
if "enc_outputs" in outputs:
enc_outputs = outputs["enc_outputs"]
bin_targets = copy.deepcopy(targets)
for bt in bin_targets:
bt["class_labels"] = torch.zeros_like(bt["class_labels"])
if self.assign_first_stage:
indices = self.stg1_assigner(enc_outputs, bin_targets)
else:
indices = self.matcher(enc_outputs, bin_targets)
for loss in self.losses:
l_dict = self.get_loss(loss, enc_outputs, bin_targets, indices, num_boxes)
l_dict = {k + "_enc": v for k, v in l_dict.items()}
losses.update(l_dict)
return losses
# Copied from transformers.models.detr.modeling_detr.DetrMLPPredictionHead
class DetaMLPPredictionHead(nn.Module):
"""
Very simple multi-layer perceptron (MLP, also called FFN), used to predict the normalized center coordinates,
height and width of a bounding box w.r.t. an image.
Copied from https://github.com/facebookresearch/detr/blob/master/models/detr.py
"""
def __init__(self, input_dim, hidden_dim, output_dim, num_layers):
super().__init__()
self.num_layers = num_layers
h = [hidden_dim] * (num_layers - 1)
self.layers = nn.ModuleList(nn.Linear(n, k) for n, k in zip([input_dim] + h, h + [output_dim]))
def forward(self, x):
for i, layer in enumerate(self.layers):
x = nn.functional.relu(layer(x)) if i < self.num_layers - 1 else layer(x)
return x
# Copied from transformers.models.deformable_detr.modeling_deformable_detr.DeformableDetrHungarianMatcher with DeformableDetr->Deta
class DetaHungarianMatcher(nn.Module):
"""
This class computes an assignment between the targets and the predictions of the network.
For efficiency reasons, the targets don't include the no_object. Because of this, in general, there are more
predictions than targets. In this case, we do a 1-to-1 matching of the best predictions, while the others are
un-matched (and thus treated as non-objects).
Args:
class_cost:
The relative weight of the classification error in the matching cost.
bbox_cost:
The relative weight of the L1 error of the bounding box coordinates in the matching cost.
giou_cost:
The relative weight of the giou loss of the bounding box in the matching cost.
"""
def __init__(self, class_cost: float = 1, bbox_cost: float = 1, giou_cost: float = 1):
super().__init__()
requires_backends(self, ["scipy"])
self.class_cost = class_cost
self.bbox_cost = bbox_cost
self.giou_cost = giou_cost
if class_cost == 0 and bbox_cost == 0 and giou_cost == 0:
raise ValueError("All costs of the Matcher can't be 0")
@torch.no_grad()
def forward(self, outputs, targets):
"""
Args:
outputs (`dict`):
A dictionary that contains at least these entries:
* "logits": Tensor of dim [batch_size, num_queries, num_classes] with the classification logits
* "pred_boxes": Tensor of dim [batch_size, num_queries, 4] with the predicted box coordinates.
targets (`List[dict]`):
A list of targets (len(targets) = batch_size), where each target is a dict containing:
* "class_labels": Tensor of dim [num_target_boxes] (where num_target_boxes is the number of
ground-truth
objects in the target) containing the class labels
* "boxes": Tensor of dim [num_target_boxes, 4] containing the target box coordinates.
Returns:
`List[Tuple]`: A list of size `batch_size`, containing tuples of (index_i, index_j) where:
- index_i is the indices of the selected predictions (in order)
- index_j is the indices of the corresponding selected targets (in order)
For each batch element, it holds: len(index_i) = len(index_j) = min(num_queries, num_target_boxes)
"""
batch_size, num_queries = outputs["logits"].shape[:2]
# We flatten to compute the cost matrices in a batch
out_prob = outputs["logits"].flatten(0, 1).sigmoid() # [batch_size * num_queries, num_classes]
out_bbox = outputs["pred_boxes"].flatten(0, 1) # [batch_size * num_queries, 4]
# Also concat the target labels and boxes
target_ids = torch.cat([v["class_labels"] for v in targets])
target_bbox = torch.cat([v["boxes"] for v in targets])
# Compute the classification cost.
alpha = 0.25
gamma = 2.0
neg_cost_class = (1 - alpha) * (out_prob**gamma) * (-(1 - out_prob + 1e-8).log())
pos_cost_class = alpha * ((1 - out_prob) ** gamma) * (-(out_prob + 1e-8).log())
class_cost = pos_cost_class[:, target_ids] - neg_cost_class[:, target_ids]
# Compute the L1 cost between boxes
bbox_cost = torch.cdist(out_bbox, target_bbox, p=1)
# Compute the giou cost between boxes
giou_cost = -generalized_box_iou(center_to_corners_format(out_bbox), center_to_corners_format(target_bbox))
# Final cost matrix
cost_matrix = self.bbox_cost * bbox_cost + self.class_cost * class_cost + self.giou_cost * giou_cost
cost_matrix = cost_matrix.view(batch_size, num_queries, -1).cpu()
sizes = [len(v["boxes"]) for v in targets]
indices = [linear_sum_assignment(c[i]) for i, c in enumerate(cost_matrix.split(sizes, -1))]
return [(torch.as_tensor(i, dtype=torch.int64), torch.as_tensor(j, dtype=torch.int64)) for i, j in indices]
# Copied from transformers.models.detr.modeling_detr._upcast
def _upcast(t: Tensor) -> Tensor:
# Protects from numerical overflows in multiplications by upcasting to the equivalent higher type
if t.is_floating_point():
return t if t.dtype in (torch.float32, torch.float64) else t.float()
else:
return t if t.dtype in (torch.int32, torch.int64) else t.int()
# Copied from transformers.models.detr.modeling_detr.box_area
def box_area(boxes: Tensor) -> Tensor:
"""
Computes the area of a set of bounding boxes, which are specified by its (x1, y1, x2, y2) coordinates.
Args:
boxes (`torch.FloatTensor` of shape `(number_of_boxes, 4)`):
Boxes for which the area will be computed. They are expected to be in (x1, y1, x2, y2) format with `0 <= x1
< x2` and `0 <= y1 < y2`.
Returns:
`torch.FloatTensor`: a tensor containing the area for each box.
"""
boxes = _upcast(boxes)
return (boxes[:, 2] - boxes[:, 0]) * (boxes[:, 3] - boxes[:, 1])
# Copied from transformers.models.detr.modeling_detr.box_iou
def box_iou(boxes1, boxes2):
area1 = box_area(boxes1)
area2 = box_area(boxes2)
left_top = torch.max(boxes1[:, None, :2], boxes2[:, :2]) # [N,M,2]
right_bottom = torch.min(boxes1[:, None, 2:], boxes2[:, 2:]) # [N,M,2]
width_height = (right_bottom - left_top).clamp(min=0) # [N,M,2]
inter = width_height[:, :, 0] * width_height[:, :, 1] # [N,M]
union = area1[:, None] + area2 - inter
iou = inter / union
return iou, union
# Copied from transformers.models.detr.modeling_detr.generalized_box_iou
def generalized_box_iou(boxes1, boxes2):
"""
Generalized IoU from https://giou.stanford.edu/. The boxes should be in [x0, y0, x1, y1] (corner) format.
Returns:
`torch.FloatTensor`: a [N, M] pairwise matrix, where N = len(boxes1) and M = len(boxes2)
"""
# degenerate boxes gives inf / nan results
# so do an early check
if not (boxes1[:, 2:] >= boxes1[:, :2]).all():
raise ValueError(f"boxes1 must be in [x0, y0, x1, y1] (corner) format, but got {boxes1}")
if not (boxes2[:, 2:] >= boxes2[:, :2]).all():
raise ValueError(f"boxes2 must be in [x0, y0, x1, y1] (corner) format, but got {boxes2}")
iou, union = box_iou(boxes1, boxes2)
top_left = torch.min(boxes1[:, None, :2], boxes2[:, :2])
bottom_right = torch.max(boxes1[:, None, 2:], boxes2[:, 2:])
width_height = (bottom_right - top_left).clamp(min=0) # [N,M,2]
area = width_height[:, :, 0] * width_height[:, :, 1]
return iou - (area - union) / area
# from https://github.com/facebookresearch/detectron2/blob/cbbc1ce26473cb2a5cc8f58e8ada9ae14cb41052/detectron2/layers/wrappers.py#L100
def nonzero_tuple(x):
"""
A 'as_tuple=True' version of torch.nonzero to support torchscript. because of
https://github.com/pytorch/pytorch/issues/38718
"""
if torch.jit.is_scripting():
if x.dim() == 0:
return x.unsqueeze(0).nonzero().unbind(1)
return x.nonzero().unbind(1)
else:
return x.nonzero(as_tuple=True)
# from https://github.com/facebookresearch/detectron2/blob/9921a2caa585d4fa66c4b534b6fab6e74d89b582/detectron2/modeling/matcher.py#L9
class DetaMatcher(object):
"""
This class assigns to each predicted "element" (e.g., a box) a ground-truth element. Each predicted element will
have exactly zero or one matches; each ground-truth element may be matched to zero or more predicted elements.
The matching is determined by the MxN match_quality_matrix, that characterizes how well each (ground-truth,
prediction)-pair match each other. For example, if the elements are boxes, this matrix may contain box
intersection-over-union overlap values.
The matcher returns (a) a vector of length N containing the index of the ground-truth element m in [0, M) that
matches to prediction n in [0, N). (b) a vector of length N containing the labels for each prediction.
"""
def __init__(self, thresholds: List[float], labels: List[int], allow_low_quality_matches: bool = False):
"""
Args:
thresholds (`list[float]`):
A list of thresholds used to stratify predictions into levels.
labels (`list[int`):
A list of values to label predictions belonging at each level. A label can be one of {-1, 0, 1}
signifying {ignore, negative class, positive class}, respectively.
allow_low_quality_matches (`bool`, *optional*, defaults to `False`):
If `True`, produce additional matches for predictions with maximum match quality lower than
high_threshold. See `set_low_quality_matches_` for more details.
For example,
thresholds = [0.3, 0.5] labels = [0, -1, 1] All predictions with iou < 0.3 will be marked with 0 and
thus will be considered as false positives while training. All predictions with 0.3 <= iou < 0.5 will
be marked with -1 and thus will be ignored. All predictions with 0.5 <= iou will be marked with 1 and
thus will be considered as true positives.
"""
# Add -inf and +inf to first and last position in thresholds
thresholds = thresholds[:]
if thresholds[0] < 0:
raise ValueError("Thresholds should be positive")
thresholds.insert(0, -float("inf"))
thresholds.append(float("inf"))
# Currently torchscript does not support all + generator
if not all(low <= high for (low, high) in zip(thresholds[:-1], thresholds[1:])):
raise ValueError("Thresholds should be sorted.")
if not all(l in [-1, 0, 1] for l in labels):
raise ValueError("All labels should be either -1, 0 or 1")
if len(labels) != len(thresholds) - 1:
raise ValueError("Number of labels should be equal to number of thresholds - 1")
self.thresholds = thresholds
self.labels = labels
self.allow_low_quality_matches = allow_low_quality_matches
def __call__(self, match_quality_matrix):
"""
Args:
match_quality_matrix (Tensor[float]): an MxN tensor, containing the
pairwise quality between M ground-truth elements and N predicted elements. All elements must be >= 0
(due to the us of `torch.nonzero` for selecting indices in `set_low_quality_matches_`).
Returns:
matches (Tensor[int64]): a vector of length N, where matches[i] is a matched
ground-truth index in [0, M)
match_labels (Tensor[int8]): a vector of length N, where pred_labels[i] indicates
whether a prediction is a true or false positive or ignored
"""
assert match_quality_matrix.dim() == 2
if match_quality_matrix.numel() == 0:
default_matches = match_quality_matrix.new_full((match_quality_matrix.size(1),), 0, dtype=torch.int64)
# When no gt boxes exist, we define IOU = 0 and therefore set labels
# to `self.labels[0]`, which usually defaults to background class 0
# To choose to ignore instead, can make labels=[-1,0,-1,1] + set appropriate thresholds
default_match_labels = match_quality_matrix.new_full(
(match_quality_matrix.size(1),), self.labels[0], dtype=torch.int8
)
return default_matches, default_match_labels
assert torch.all(match_quality_matrix >= 0)
# match_quality_matrix is M (gt) x N (predicted)
# Max over gt elements (dim 0) to find best gt candidate for each prediction
matched_vals, matches = match_quality_matrix.max(dim=0)
match_labels = matches.new_full(matches.size(), 1, dtype=torch.int8)
for l, low, high in zip(self.labels, self.thresholds[:-1], self.thresholds[1:]):
low_high = (matched_vals >= low) & (matched_vals < high)
match_labels[low_high] = l
if self.allow_low_quality_matches:
self.set_low_quality_matches_(match_labels, match_quality_matrix)
return matches, match_labels
def set_low_quality_matches_(self, match_labels, match_quality_matrix):
"""
Produce additional matches for predictions that have only low-quality matches. Specifically, for each
ground-truth G find the set of predictions that have maximum overlap with it (including ties); for each
prediction in that set, if it is unmatched, then match it to the ground-truth G.
This function implements the RPN assignment case (i) in Sec. 3.1.2 of :paper:`Faster R-CNN`.
"""
# For each gt, find the prediction with which it has highest quality
highest_quality_foreach_gt, _ = match_quality_matrix.max(dim=1)
# Find the highest quality match available, even if it is low, including ties.
# Note that the matches qualities must be positive due to the use of
# `torch.nonzero`.
_, pred_inds_with_highest_quality = nonzero_tuple(match_quality_matrix == highest_quality_foreach_gt[:, None])
# If an anchor was labeled positive only due to a low-quality match
# with gt_A, but it has larger overlap with gt_B, it's matched index will still be gt_B.
# This follows the implementation in Detectron, and is found to have no significant impact.
match_labels[pred_inds_with_highest_quality] = 1
# from https://github.com/facebookresearch/detectron2/blob/cbbc1ce26473cb2a5cc8f58e8ada9ae14cb41052/detectron2/modeling/sampling.py#L9
def subsample_labels(labels: torch.Tensor, num_samples: int, positive_fraction: float, bg_label: int):
"""
Return `num_samples` (or fewer, if not enough found) random samples from `labels` which is a mixture of positives &
negatives. It will try to return as many positives as possible without exceeding `positive_fraction * num_samples`,
and then try to fill the remaining slots with negatives.
Args:
labels (Tensor): (N, ) label vector with values:
* -1: ignore
* bg_label: background ("negative") class
* otherwise: one or more foreground ("positive") classes
num_samples (int): The total number of labels with value >= 0 to return.
Values that are not sampled will be filled with -1 (ignore).
positive_fraction (float): The number of subsampled labels with values > 0
is `min(num_positives, int(positive_fraction * num_samples))`. The number of negatives sampled is
`min(num_negatives, num_samples - num_positives_sampled)`. In order words, if there are not enough
positives, the sample is filled with negatives. If there are also not enough negatives, then as many
elements are sampled as is possible.
bg_label (int): label index of background ("negative") class.
Returns:
pos_idx, neg_idx (Tensor):
1D vector of indices. The total length of both is `num_samples` or fewer.
"""
positive = nonzero_tuple((labels != -1) & (labels != bg_label))[0]
negative = nonzero_tuple(labels == bg_label)[0]
num_pos = int(num_samples * positive_fraction)
# protect against not enough positive examples
num_pos = min(positive.numel(), num_pos)
num_neg = num_samples - num_pos
# protect against not enough negative examples
num_neg = min(negative.numel(), num_neg)
# randomly select positive and negative examples
perm1 = torch.randperm(positive.numel(), device=positive.device)[:num_pos]
perm2 = torch.randperm(negative.numel(), device=negative.device)[:num_neg]
pos_idx = positive[perm1]
neg_idx = negative[perm2]
return pos_idx, neg_idx
def sample_topk_per_gt(pr_inds, gt_inds, iou, k):
if len(gt_inds) == 0:
return pr_inds, gt_inds
# find topk matches for each gt
gt_inds2, counts = gt_inds.unique(return_counts=True)
scores, pr_inds2 = iou[gt_inds2].topk(k, dim=1)
gt_inds2 = gt_inds2[:, None].repeat(1, k)
# filter to as many matches that gt has
pr_inds3 = torch.cat([pr[:c] for c, pr in zip(counts, pr_inds2)])
gt_inds3 = torch.cat([gt[:c] for c, gt in zip(counts, gt_inds2)])
return pr_inds3, gt_inds3
# modified from https://github.com/facebookresearch/detectron2/blob/cbbc1ce26473cb2a5cc8f58e8ada9ae14cb41052/detectron2/modeling/roi_heads/roi_heads.py#L123
class DetaStage2Assigner(nn.Module):
def __init__(self, num_queries, max_k=4):
super().__init__()
self.positive_fraction = 0.25
self.bg_label = 400 # number > 91 to filter out later
self.batch_size_per_image = num_queries
self.proposal_matcher = DetaMatcher(thresholds=[0.6], labels=[0, 1], allow_low_quality_matches=True)
self.k = max_k
def _sample_proposals(self, matched_idxs: torch.Tensor, matched_labels: torch.Tensor, gt_classes: torch.Tensor):
"""
Based on the matching between N proposals and M groundtruth, sample the proposals and set their classification
labels.
Args:
matched_idxs (Tensor): a vector of length N, each is the best-matched
gt index in [0, M) for each proposal.
matched_labels (Tensor): a vector of length N, the matcher's label
(one of cfg.MODEL.ROI_HEADS.IOU_LABELS) for each proposal.
gt_classes (Tensor): a vector of length M.
Returns:
Tensor: a vector of indices of sampled proposals. Each is in [0, N). Tensor: a vector of the same length,
the classification label for
each sampled proposal. Each sample is labeled as either a category in [0, num_classes) or the
background (num_classes).
"""
has_gt = gt_classes.numel() > 0
# Get the corresponding GT for each proposal
if has_gt:
gt_classes = gt_classes[matched_idxs]
# Label unmatched proposals (0 label from matcher) as background (label=num_classes)
gt_classes[matched_labels == 0] = self.bg_label
# Label ignore proposals (-1 label)
gt_classes[matched_labels == -1] = -1
else:
gt_classes = torch.zeros_like(matched_idxs) + self.bg_label
sampled_fg_idxs, sampled_bg_idxs = subsample_labels(
gt_classes, self.batch_size_per_image, self.positive_fraction, self.bg_label
)
sampled_idxs = torch.cat([sampled_fg_idxs, sampled_bg_idxs], dim=0)
return sampled_idxs, gt_classes[sampled_idxs]
def forward(self, outputs, targets, return_cost_matrix=False):
# COCO categories are from 1 to 90. They set num_classes=91 and apply sigmoid.
bs = len(targets)
indices = []
ious = []
for b in range(bs):
iou, _ = box_iou(
center_to_corners_format(targets[b]["boxes"]),
center_to_corners_format(outputs["init_reference"][b].detach()),
)
matched_idxs, matched_labels = self.proposal_matcher(
iou
) # proposal_id -> highest_iou_gt_id, proposal_id -> [1 if iou > 0.6, 0 ow]
(
sampled_idxs,
sampled_gt_classes,
) = self._sample_proposals( # list of sampled proposal_ids, sampled_id -> [0, num_classes)+[bg_label]
matched_idxs, matched_labels, targets[b]["class_labels"]
)
pos_pr_inds = sampled_idxs[sampled_gt_classes != self.bg_label]
pos_gt_inds = matched_idxs[pos_pr_inds]
pos_pr_inds, pos_gt_inds = self.postprocess_indices(pos_pr_inds, pos_gt_inds, iou)
indices.append((pos_pr_inds, pos_gt_inds))
ious.append(iou)
if return_cost_matrix:
return indices, ious
return indices
def postprocess_indices(self, pr_inds, gt_inds, iou):
return sample_topk_per_gt(pr_inds, gt_inds, iou, self.k)
# modified from https://github.com/facebookresearch/detectron2/blob/cbbc1ce26473cb2a5cc8f58e8ada9ae14cb41052/detectron2/modeling/proposal_generator/rpn.py#L181
class DetaStage1Assigner(nn.Module):
def __init__(self, t_low=0.3, t_high=0.7, max_k=4):
super().__init__()
self.positive_fraction = 0.5
self.batch_size_per_image = 256
self.k = max_k
self.t_low = t_low
self.t_high = t_high
self.anchor_matcher = DetaMatcher(
thresholds=[t_low, t_high], labels=[0, -1, 1], allow_low_quality_matches=True
)
def _subsample_labels(self, label):
"""
Randomly sample a subset of positive and negative examples, and overwrite the label vector to the ignore value
(-1) for all elements that are not included in the sample.
Args:
labels (Tensor): a vector of -1, 0, 1. Will be modified in-place and returned.
"""
pos_idx, neg_idx = subsample_labels(label, self.batch_size_per_image, self.positive_fraction, 0)
# Fill with the ignore label (-1), then set positive and negative labels
label.fill_(-1)
label.scatter_(0, pos_idx, 1)
label.scatter_(0, neg_idx, 0)
return label
def forward(self, outputs, targets):
bs = len(targets)
indices = []
for b in range(bs):
anchors = outputs["anchors"][b]
if len(targets[b]["boxes"]) == 0:
indices.append(
(
torch.tensor([], dtype=torch.long, device=anchors.device),
torch.tensor([], dtype=torch.long, device=anchors.device),
)
)
continue
iou, _ = box_iou(
center_to_corners_format(targets[b]["boxes"]),
center_to_corners_format(anchors),
)
matched_idxs, matched_labels = self.anchor_matcher(
iou
) # proposal_id -> highest_iou_gt_id, proposal_id -> [1 if iou > 0.7, 0 if iou < 0.3, -1 ow]
matched_labels = self._subsample_labels(matched_labels)
all_pr_inds = torch.arange(len(anchors), device=matched_labels.device)
pos_pr_inds = all_pr_inds[matched_labels == 1]
pos_gt_inds = matched_idxs[pos_pr_inds]
pos_pr_inds, pos_gt_inds = self.postprocess_indices(pos_pr_inds, pos_gt_inds, iou)
pos_pr_inds, pos_gt_inds = pos_pr_inds.to(anchors.device), pos_gt_inds.to(anchors.device)
indices.append((pos_pr_inds, pos_gt_inds))
return indices
def postprocess_indices(self, pr_inds, gt_inds, iou):
return sample_topk_per_gt(pr_inds, gt_inds, iou, self.k)
| transformers/src/transformers/models/deta/modeling_deta.py/0 | {
"file_path": "transformers/src/transformers/models/deta/modeling_deta.py",
"repo_id": "transformers",
"token_count": 58219
} | 323 |
# coding=utf-8
# Copyright 2023 Meta AI and The HuggingFace Inc. team. All rights reserved.
#
# Licensed under the Apache License, Version 2.0 (the "License");
# you may not use this file except in compliance with the License.
# You may obtain a copy of the License at
#
# http://www.apache.org/licenses/LICENSE-2.0
#
# Unless required by applicable law or agreed to in writing, software
# distributed under the License is distributed on an "AS IS" BASIS,
# WITHOUT WARRANTIES OR CONDITIONS OF ANY KIND, either express or implied.
# See the License for the specific language governing permissions and
# limitations under the License.
""" PyTorch DINOv2 model."""
import collections.abc
import math
from typing import Dict, List, Optional, Set, Tuple, Union
import torch
import torch.utils.checkpoint
from torch import nn
from torch.nn import BCEWithLogitsLoss, CrossEntropyLoss, MSELoss
from ...activations import ACT2FN
from ...modeling_outputs import (
BackboneOutput,
BaseModelOutput,
BaseModelOutputWithPooling,
ImageClassifierOutput,
)
from ...modeling_utils import PreTrainedModel
from ...pytorch_utils import find_pruneable_heads_and_indices, prune_linear_layer
from ...utils import (
add_code_sample_docstrings,
add_start_docstrings,
add_start_docstrings_to_model_forward,
logging,
replace_return_docstrings,
)
from ...utils.backbone_utils import BackboneMixin
from .configuration_dinov2 import Dinov2Config
logger = logging.get_logger(__name__)
# General docstring
_CONFIG_FOR_DOC = "Dinov2Config"
# Base docstring
_CHECKPOINT_FOR_DOC = "facebook/dinov2-base"
_EXPECTED_OUTPUT_SHAPE = [1, 257, 768]
# Image classification docstring
_IMAGE_CLASS_CHECKPOINT = "facebook/dinov2-small-imagenet1k-1-layer"
_IMAGE_CLASS_EXPECTED_OUTPUT = "tabby, tabby cat"
DINOV2_PRETRAINED_MODEL_ARCHIVE_LIST = [
"facebook/dinov2-base",
# See all DINOv2 models at https://huggingface.co/models?filter=dinov2
]
class Dinov2Embeddings(nn.Module):
"""
Construct the CLS token, mask token, position and patch embeddings.
"""
def __init__(self, config: Dinov2Config) -> None:
super().__init__()
self.cls_token = nn.Parameter(torch.randn(1, 1, config.hidden_size))
self.mask_token = nn.Parameter(torch.zeros(1, config.hidden_size))
self.patch_embeddings = Dinov2PatchEmbeddings(config)
num_patches = self.patch_embeddings.num_patches
self.position_embeddings = nn.Parameter(torch.randn(1, num_patches + 1, config.hidden_size))
self.dropout = nn.Dropout(config.hidden_dropout_prob)
self.config = config
def interpolate_pos_encoding(self, embeddings: torch.Tensor, height: int, width: int) -> torch.Tensor:
"""
This method allows to interpolate the pre-trained position encodings, to be able to use the model on higher
resolution images.
Source:
https://github.com/facebookresearch/dino/blob/de9ee3df6cf39fac952ab558447af1fa1365362a/vision_transformer.py#L174
"""
num_patches = embeddings.shape[1] - 1
num_positions = self.position_embeddings.shape[1] - 1
if num_patches == num_positions and height == width:
return self.position_embeddings
class_pos_embed = self.position_embeddings[:, 0]
patch_pos_embed = self.position_embeddings[:, 1:]
dim = embeddings.shape[-1]
height = height // self.config.patch_size
width = width // self.config.patch_size
# we add a small number to avoid floating point error in the interpolation
# see discussion at https://github.com/facebookresearch/dino/issues/8
height, width = height + 0.1, width + 0.1
patch_pos_embed = patch_pos_embed.reshape(1, int(math.sqrt(num_positions)), int(math.sqrt(num_positions)), dim)
patch_pos_embed = patch_pos_embed.permute(0, 3, 1, 2)
target_dtype = patch_pos_embed.dtype
patch_pos_embed = nn.functional.interpolate(
patch_pos_embed.to(dtype=torch.float32),
scale_factor=(float(height / math.sqrt(num_positions)), float(width / math.sqrt(num_positions))),
mode="bicubic",
align_corners=False,
).to(dtype=target_dtype)
if int(height) != patch_pos_embed.shape[-2] or int(width) != patch_pos_embed.shape[-1]:
raise ValueError("Width or height does not match with the interpolated position embeddings")
patch_pos_embed = patch_pos_embed.permute(0, 2, 3, 1).view(1, -1, dim)
return torch.cat((class_pos_embed.unsqueeze(0), patch_pos_embed), dim=1)
def forward(self, pixel_values: torch.Tensor, bool_masked_pos: Optional[torch.Tensor] = None) -> torch.Tensor:
batch_size, _, height, width = pixel_values.shape
target_dtype = self.patch_embeddings.projection.weight.dtype
embeddings = self.patch_embeddings(pixel_values.to(dtype=target_dtype))
if bool_masked_pos is not None:
embeddings = torch.where(
bool_masked_pos.unsqueeze(-1), self.mask_token.to(embeddings.dtype).unsqueeze(0), embeddings
)
# add the [CLS] token to the embedded patch tokens
cls_tokens = self.cls_token.expand(batch_size, -1, -1)
embeddings = torch.cat((cls_tokens, embeddings), dim=1)
# add positional encoding to each token
embeddings = embeddings + self.interpolate_pos_encoding(embeddings, height, width)
embeddings = self.dropout(embeddings)
return embeddings
class Dinov2PatchEmbeddings(nn.Module):
"""
This class turns `pixel_values` of shape `(batch_size, num_channels, height, width)` into the initial
`hidden_states` (patch embeddings) of shape `(batch_size, seq_length, hidden_size)` to be consumed by a
Transformer.
"""
def __init__(self, config):
super().__init__()
image_size, patch_size = config.image_size, config.patch_size
num_channels, hidden_size = config.num_channels, config.hidden_size
image_size = image_size if isinstance(image_size, collections.abc.Iterable) else (image_size, image_size)
patch_size = patch_size if isinstance(patch_size, collections.abc.Iterable) else (patch_size, patch_size)
num_patches = (image_size[1] // patch_size[1]) * (image_size[0] // patch_size[0])
self.image_size = image_size
self.patch_size = patch_size
self.num_channels = num_channels
self.num_patches = num_patches
self.projection = nn.Conv2d(num_channels, hidden_size, kernel_size=patch_size, stride=patch_size)
def forward(self, pixel_values: torch.Tensor) -> torch.Tensor:
num_channels = pixel_values.shape[1]
if num_channels != self.num_channels:
raise ValueError(
"Make sure that the channel dimension of the pixel values match with the one set in the configuration."
f" Expected {self.num_channels} but got {num_channels}."
)
embeddings = self.projection(pixel_values).flatten(2).transpose(1, 2)
return embeddings
# Copied from transformers.models.vit.modeling_vit.ViTSelfAttention with ViT->Dinov2
class Dinov2SelfAttention(nn.Module):
def __init__(self, config: Dinov2Config) -> None:
super().__init__()
if config.hidden_size % config.num_attention_heads != 0 and not hasattr(config, "embedding_size"):
raise ValueError(
f"The hidden size {config.hidden_size,} is not a multiple of the number of attention "
f"heads {config.num_attention_heads}."
)
self.num_attention_heads = config.num_attention_heads
self.attention_head_size = int(config.hidden_size / config.num_attention_heads)
self.all_head_size = self.num_attention_heads * self.attention_head_size
self.query = nn.Linear(config.hidden_size, self.all_head_size, bias=config.qkv_bias)
self.key = nn.Linear(config.hidden_size, self.all_head_size, bias=config.qkv_bias)
self.value = nn.Linear(config.hidden_size, self.all_head_size, bias=config.qkv_bias)
self.dropout = nn.Dropout(config.attention_probs_dropout_prob)
def transpose_for_scores(self, x: torch.Tensor) -> torch.Tensor:
new_x_shape = x.size()[:-1] + (self.num_attention_heads, self.attention_head_size)
x = x.view(new_x_shape)
return x.permute(0, 2, 1, 3)
def forward(
self, hidden_states, head_mask: Optional[torch.Tensor] = None, output_attentions: bool = False
) -> Union[Tuple[torch.Tensor, torch.Tensor], Tuple[torch.Tensor]]:
mixed_query_layer = self.query(hidden_states)
key_layer = self.transpose_for_scores(self.key(hidden_states))
value_layer = self.transpose_for_scores(self.value(hidden_states))
query_layer = self.transpose_for_scores(mixed_query_layer)
# Take the dot product between "query" and "key" to get the raw attention scores.
attention_scores = torch.matmul(query_layer, key_layer.transpose(-1, -2))
attention_scores = attention_scores / math.sqrt(self.attention_head_size)
# Normalize the attention scores to probabilities.
attention_probs = nn.functional.softmax(attention_scores, dim=-1)
# This is actually dropping out entire tokens to attend to, which might
# seem a bit unusual, but is taken from the original Transformer paper.
attention_probs = self.dropout(attention_probs)
# Mask heads if we want to
if head_mask is not None:
attention_probs = attention_probs * head_mask
context_layer = torch.matmul(attention_probs, value_layer)
context_layer = context_layer.permute(0, 2, 1, 3).contiguous()
new_context_layer_shape = context_layer.size()[:-2] + (self.all_head_size,)
context_layer = context_layer.view(new_context_layer_shape)
outputs = (context_layer, attention_probs) if output_attentions else (context_layer,)
return outputs
# Copied from transformers.models.vit.modeling_vit.ViTSelfOutput with ViT->Dinov2
class Dinov2SelfOutput(nn.Module):
"""
The residual connection is defined in Dinov2Layer instead of here (as is the case with other models), due to the
layernorm applied before each block.
"""
def __init__(self, config: Dinov2Config) -> None:
super().__init__()
self.dense = nn.Linear(config.hidden_size, config.hidden_size)
self.dropout = nn.Dropout(config.hidden_dropout_prob)
def forward(self, hidden_states: torch.Tensor, input_tensor: torch.Tensor) -> torch.Tensor:
hidden_states = self.dense(hidden_states)
hidden_states = self.dropout(hidden_states)
return hidden_states
# Copied from transformers.models.vit.modeling_vit.ViTAttention with ViT->Dinov2
class Dinov2Attention(nn.Module):
def __init__(self, config: Dinov2Config) -> None:
super().__init__()
self.attention = Dinov2SelfAttention(config)
self.output = Dinov2SelfOutput(config)
self.pruned_heads = set()
def prune_heads(self, heads: Set[int]) -> None:
if len(heads) == 0:
return
heads, index = find_pruneable_heads_and_indices(
heads, self.attention.num_attention_heads, self.attention.attention_head_size, self.pruned_heads
)
# Prune linear layers
self.attention.query = prune_linear_layer(self.attention.query, index)
self.attention.key = prune_linear_layer(self.attention.key, index)
self.attention.value = prune_linear_layer(self.attention.value, index)
self.output.dense = prune_linear_layer(self.output.dense, index, dim=1)
# Update hyper params and store pruned heads
self.attention.num_attention_heads = self.attention.num_attention_heads - len(heads)
self.attention.all_head_size = self.attention.attention_head_size * self.attention.num_attention_heads
self.pruned_heads = self.pruned_heads.union(heads)
def forward(
self,
hidden_states: torch.Tensor,
head_mask: Optional[torch.Tensor] = None,
output_attentions: bool = False,
) -> Union[Tuple[torch.Tensor, torch.Tensor], Tuple[torch.Tensor]]:
self_outputs = self.attention(hidden_states, head_mask, output_attentions)
attention_output = self.output(self_outputs[0], hidden_states)
outputs = (attention_output,) + self_outputs[1:] # add attentions if we output them
return outputs
class Dinov2LayerScale(nn.Module):
def __init__(self, config) -> None:
super().__init__()
self.lambda1 = nn.Parameter(config.layerscale_value * torch.ones(config.hidden_size))
def forward(self, hidden_state: torch.Tensor) -> torch.Tensor:
return hidden_state * self.lambda1
# Copied from transformers.models.beit.modeling_beit.drop_path
def drop_path(input: torch.Tensor, drop_prob: float = 0.0, training: bool = False) -> torch.Tensor:
"""
Drop paths (Stochastic Depth) per sample (when applied in main path of residual blocks).
Comment by Ross Wightman: This is the same as the DropConnect impl I created for EfficientNet, etc networks,
however, the original name is misleading as 'Drop Connect' is a different form of dropout in a separate paper...
See discussion: https://github.com/tensorflow/tpu/issues/494#issuecomment-532968956 ... I've opted for changing the
layer and argument names to 'drop path' rather than mix DropConnect as a layer name and use 'survival rate' as the
argument.
"""
if drop_prob == 0.0 or not training:
return input
keep_prob = 1 - drop_prob
shape = (input.shape[0],) + (1,) * (input.ndim - 1) # work with diff dim tensors, not just 2D ConvNets
random_tensor = keep_prob + torch.rand(shape, dtype=input.dtype, device=input.device)
random_tensor.floor_() # binarize
output = input.div(keep_prob) * random_tensor
return output
# Copied from transformers.models.beit.modeling_beit.BeitDropPath
class Dinov2DropPath(nn.Module):
"""Drop paths (Stochastic Depth) per sample (when applied in main path of residual blocks)."""
def __init__(self, drop_prob: Optional[float] = None) -> None:
super().__init__()
self.drop_prob = drop_prob
def forward(self, hidden_states: torch.Tensor) -> torch.Tensor:
return drop_path(hidden_states, self.drop_prob, self.training)
def extra_repr(self) -> str:
return "p={}".format(self.drop_prob)
class Dinov2MLP(nn.Module):
def __init__(self, config) -> None:
super().__init__()
in_features = out_features = config.hidden_size
hidden_features = int(config.hidden_size * config.mlp_ratio)
self.fc1 = nn.Linear(in_features, hidden_features, bias=True)
if isinstance(config.hidden_act, str):
self.activation = ACT2FN[config.hidden_act]
else:
self.activation = config.hidden_act
self.fc2 = nn.Linear(hidden_features, out_features, bias=True)
def forward(self, hidden_state: torch.Tensor) -> torch.Tensor:
hidden_state = self.fc1(hidden_state)
hidden_state = self.activation(hidden_state)
hidden_state = self.fc2(hidden_state)
return hidden_state
class Dinov2SwiGLUFFN(nn.Module):
def __init__(self, config) -> None:
super().__init__()
in_features = out_features = config.hidden_size
hidden_features = int(config.hidden_size * config.mlp_ratio)
hidden_features = (int(hidden_features * 2 / 3) + 7) // 8 * 8
self.weights_in = nn.Linear(in_features, 2 * hidden_features, bias=True)
self.weights_out = nn.Linear(hidden_features, out_features, bias=True)
def forward(self, hidden_state: torch.Tensor) -> torch.Tensor:
hidden_state = self.weights_in(hidden_state)
x1, x2 = hidden_state.chunk(2, dim=-1)
hidden = nn.functional.silu(x1) * x2
return self.weights_out(hidden)
class Dinov2Layer(nn.Module):
"""This corresponds to the Block class in the original implementation."""
def __init__(self, config: Dinov2Config) -> None:
super().__init__()
self.norm1 = nn.LayerNorm(config.hidden_size, eps=config.layer_norm_eps)
self.attention = Dinov2Attention(config)
self.layer_scale1 = Dinov2LayerScale(config)
self.drop_path1 = Dinov2DropPath(config.drop_path_rate) if config.drop_path_rate > 0.0 else nn.Identity()
self.norm2 = nn.LayerNorm(config.hidden_size, eps=config.layer_norm_eps)
if config.use_swiglu_ffn:
self.mlp = Dinov2SwiGLUFFN(config)
else:
self.mlp = Dinov2MLP(config)
self.layer_scale2 = Dinov2LayerScale(config)
self.drop_path2 = Dinov2DropPath(config.drop_path_rate) if config.drop_path_rate > 0.0 else nn.Identity()
def forward(
self,
hidden_states: torch.Tensor,
head_mask: Optional[torch.Tensor] = None,
output_attentions: bool = False,
) -> Union[Tuple[torch.Tensor, torch.Tensor], Tuple[torch.Tensor]]:
self_attention_outputs = self.attention(
self.norm1(hidden_states), # in Dinov2, layernorm is applied before self-attention
head_mask,
output_attentions=output_attentions,
)
attention_output = self_attention_outputs[0]
attention_output = self.layer_scale1(attention_output)
outputs = self_attention_outputs[1:] # add self attentions if we output attention weights
# first residual connection
hidden_states = attention_output + hidden_states
# in Dinov2, layernorm is also applied after self-attention
layer_output = self.norm2(hidden_states)
layer_output = self.mlp(layer_output)
layer_output = self.layer_scale2(layer_output)
# second residual connection
layer_output = layer_output + hidden_states
outputs = (layer_output,) + outputs
return outputs
# Copied from transformers.models.vit.modeling_vit.ViTEncoder with ViT->Dinov2
class Dinov2Encoder(nn.Module):
def __init__(self, config: Dinov2Config) -> None:
super().__init__()
self.config = config
self.layer = nn.ModuleList([Dinov2Layer(config) for _ in range(config.num_hidden_layers)])
self.gradient_checkpointing = False
def forward(
self,
hidden_states: torch.Tensor,
head_mask: Optional[torch.Tensor] = None,
output_attentions: bool = False,
output_hidden_states: bool = False,
return_dict: bool = True,
) -> Union[tuple, BaseModelOutput]:
all_hidden_states = () if output_hidden_states else None
all_self_attentions = () if output_attentions else None
for i, layer_module in enumerate(self.layer):
if output_hidden_states:
all_hidden_states = all_hidden_states + (hidden_states,)
layer_head_mask = head_mask[i] if head_mask is not None else None
if self.gradient_checkpointing and self.training:
layer_outputs = self._gradient_checkpointing_func(
layer_module.__call__,
hidden_states,
layer_head_mask,
output_attentions,
)
else:
layer_outputs = layer_module(hidden_states, layer_head_mask, output_attentions)
hidden_states = layer_outputs[0]
if output_attentions:
all_self_attentions = all_self_attentions + (layer_outputs[1],)
if output_hidden_states:
all_hidden_states = all_hidden_states + (hidden_states,)
if not return_dict:
return tuple(v for v in [hidden_states, all_hidden_states, all_self_attentions] if v is not None)
return BaseModelOutput(
last_hidden_state=hidden_states,
hidden_states=all_hidden_states,
attentions=all_self_attentions,
)
class Dinov2PreTrainedModel(PreTrainedModel):
"""
An abstract class to handle weights initialization and a simple interface for downloading and loading pretrained
models.
"""
config_class = Dinov2Config
base_model_prefix = "dinov2"
main_input_name = "pixel_values"
supports_gradient_checkpointing = True
def _init_weights(self, module: Union[nn.Linear, nn.Conv2d, nn.LayerNorm]) -> None:
"""Initialize the weights"""
if isinstance(module, (nn.Linear, nn.Conv2d)):
# Upcast the input in `fp32` and cast it back to desired `dtype` to avoid
# `trunc_normal_cpu` not implemented in `half` issues
module.weight.data = nn.init.trunc_normal_(
module.weight.data.to(torch.float32), mean=0.0, std=self.config.initializer_range
).to(module.weight.dtype)
if module.bias is not None:
module.bias.data.zero_()
elif isinstance(module, nn.LayerNorm):
module.bias.data.zero_()
module.weight.data.fill_(1.0)
elif isinstance(module, Dinov2Embeddings):
module.position_embeddings.data = nn.init.trunc_normal_(
module.position_embeddings.data.to(torch.float32),
mean=0.0,
std=self.config.initializer_range,
).to(module.position_embeddings.dtype)
module.cls_token.data = nn.init.trunc_normal_(
module.cls_token.data.to(torch.float32),
mean=0.0,
std=self.config.initializer_range,
).to(module.cls_token.dtype)
DINOV2_START_DOCSTRING = r"""
This model is a PyTorch [torch.nn.Module](https://pytorch.org/docs/stable/nn.html#torch.nn.Module) subclass. Use it
as a regular PyTorch Module and refer to the PyTorch documentation for all matter related to general usage and
behavior.
Parameters:
config ([`Dinov2Config`]): Model configuration class with all the parameters of the model.
Initializing with a config file does not load the weights associated with the model, only the
configuration. Check out the [`~PreTrainedModel.from_pretrained`] method to load the model weights.
"""
DINOV2_BASE_INPUTS_DOCSTRING = r"""
Args:
pixel_values (`torch.FloatTensor` of shape `(batch_size, num_channels, height, width)`):
Pixel values. Pixel values can be obtained using [`AutoImageProcessor`]. See
[`BitImageProcessor.preprocess`] for details.
bool_masked_pos (`torch.BoolTensor` of shape `(batch_size, sequence_length)`):
Boolean masked positions. Indicates which patches are masked (1) and which aren't (0). Only relevant for
pre-training.
head_mask (`torch.FloatTensor` of shape `(num_heads,)` or `(num_layers, num_heads)`, *optional*):
Mask to nullify selected heads of the self-attention modules. Mask values selected in `[0, 1]`:
- 1 indicates the head is **not masked**,
- 0 indicates the head is **masked**.
output_attentions (`bool`, *optional*):
Whether or not to return the attentions tensors of all attention layers. See `attentions` under returned
tensors for more detail.
output_hidden_states (`bool`, *optional*):
Whether or not to return the hidden states of all layers. See `hidden_states` under returned tensors for
more detail.
return_dict (`bool`, *optional*):
Whether or not to return a [`~utils.ModelOutput`] instead of a plain tuple.
"""
DINOV2_INPUTS_DOCSTRING = r"""
Args:
pixel_values (`torch.FloatTensor` of shape `(batch_size, num_channels, height, width)`):
Pixel values. Pixel values can be obtained using [`AutoImageProcessor`]. See
[`BitImageProcessor.preprocess`] for details.
head_mask (`torch.FloatTensor` of shape `(num_heads,)` or `(num_layers, num_heads)`, *optional*):
Mask to nullify selected heads of the self-attention modules. Mask values selected in `[0, 1]`:
- 1 indicates the head is **not masked**,
- 0 indicates the head is **masked**.
output_attentions (`bool`, *optional*):
Whether or not to return the attentions tensors of all attention layers. See `attentions` under returned
tensors for more detail.
output_hidden_states (`bool`, *optional*):
Whether or not to return the hidden states of all layers. See `hidden_states` under returned tensors for
more detail.
return_dict (`bool`, *optional*):
Whether or not to return a [`~utils.ModelOutput`] instead of a plain tuple.
"""
@add_start_docstrings(
"The bare DINOv2 Model transformer outputting raw hidden-states without any specific head on top.",
DINOV2_START_DOCSTRING,
)
class Dinov2Model(Dinov2PreTrainedModel):
def __init__(self, config: Dinov2Config):
super().__init__(config)
self.config = config
self.embeddings = Dinov2Embeddings(config)
self.encoder = Dinov2Encoder(config)
self.layernorm = nn.LayerNorm(config.hidden_size, eps=config.layer_norm_eps)
# Initialize weights and apply final processing
self.post_init()
def get_input_embeddings(self) -> Dinov2PatchEmbeddings:
return self.embeddings.patch_embeddings
def _prune_heads(self, heads_to_prune: Dict[int, List[int]]) -> None:
"""
Prunes heads of the model. heads_to_prune: dict of {layer_num: list of heads to prune in this layer} See base
class PreTrainedModel
"""
for layer, heads in heads_to_prune.items():
self.encoder.layer[layer].attention.prune_heads(heads)
@add_start_docstrings_to_model_forward(DINOV2_BASE_INPUTS_DOCSTRING)
@add_code_sample_docstrings(
checkpoint=_CHECKPOINT_FOR_DOC,
output_type=BaseModelOutputWithPooling,
config_class=_CONFIG_FOR_DOC,
modality="vision",
expected_output=_EXPECTED_OUTPUT_SHAPE,
)
def forward(
self,
pixel_values: Optional[torch.Tensor] = None,
bool_masked_pos: Optional[torch.Tensor] = None,
head_mask: Optional[torch.Tensor] = None,
output_attentions: Optional[bool] = None,
output_hidden_states: Optional[bool] = None,
return_dict: Optional[bool] = None,
) -> Union[Tuple, BaseModelOutputWithPooling]:
output_attentions = output_attentions if output_attentions is not None else self.config.output_attentions
output_hidden_states = (
output_hidden_states if output_hidden_states is not None else self.config.output_hidden_states
)
return_dict = return_dict if return_dict is not None else self.config.use_return_dict
if pixel_values is None:
raise ValueError("You have to specify pixel_values")
# Prepare head mask if needed
# 1.0 in head_mask indicate we keep the head
# attention_probs has shape bsz x n_heads x N x N
# input head_mask has shape [num_heads] or [num_hidden_layers x num_heads]
# and head_mask is converted to shape [num_hidden_layers x batch x num_heads x seq_length x seq_length]
head_mask = self.get_head_mask(head_mask, self.config.num_hidden_layers)
embedding_output = self.embeddings(pixel_values, bool_masked_pos=bool_masked_pos)
encoder_outputs = self.encoder(
embedding_output,
head_mask=head_mask,
output_attentions=output_attentions,
output_hidden_states=output_hidden_states,
return_dict=return_dict,
)
sequence_output = encoder_outputs[0]
sequence_output = self.layernorm(sequence_output)
pooled_output = sequence_output[:, 0, :]
if not return_dict:
head_outputs = (sequence_output, pooled_output)
return head_outputs + encoder_outputs[1:]
return BaseModelOutputWithPooling(
last_hidden_state=sequence_output,
pooler_output=pooled_output,
hidden_states=encoder_outputs.hidden_states,
attentions=encoder_outputs.attentions,
)
@add_start_docstrings(
"""
Dinov2 Model transformer with an image classification head on top (a linear layer on top of the final hidden state
of the [CLS] token) e.g. for ImageNet.
""",
DINOV2_START_DOCSTRING,
)
class Dinov2ForImageClassification(Dinov2PreTrainedModel):
def __init__(self, config: Dinov2Config) -> None:
super().__init__(config)
self.num_labels = config.num_labels
self.dinov2 = Dinov2Model(config)
# Classifier head
self.classifier = (
nn.Linear(config.hidden_size * 2, config.num_labels) if config.num_labels > 0 else nn.Identity()
)
# Initialize weights and apply final processing
self.post_init()
@add_start_docstrings_to_model_forward(DINOV2_INPUTS_DOCSTRING)
@add_code_sample_docstrings(
checkpoint=_IMAGE_CLASS_CHECKPOINT,
output_type=ImageClassifierOutput,
config_class=_CONFIG_FOR_DOC,
expected_output=_IMAGE_CLASS_EXPECTED_OUTPUT,
)
def forward(
self,
pixel_values: Optional[torch.Tensor] = None,
head_mask: Optional[torch.Tensor] = None,
labels: Optional[torch.Tensor] = None,
output_attentions: Optional[bool] = None,
output_hidden_states: Optional[bool] = None,
return_dict: Optional[bool] = None,
) -> Union[tuple, ImageClassifierOutput]:
r"""
labels (`torch.LongTensor` of shape `(batch_size,)`, *optional*):
Labels for computing the image classification/regression loss. Indices should be in `[0, ...,
config.num_labels - 1]`. If `config.num_labels == 1` a regression loss is computed (Mean-Square loss), If
`config.num_labels > 1` a classification loss is computed (Cross-Entropy).
"""
return_dict = return_dict if return_dict is not None else self.config.use_return_dict
outputs = self.dinov2(
pixel_values,
head_mask=head_mask,
output_attentions=output_attentions,
output_hidden_states=output_hidden_states,
return_dict=return_dict,
)
sequence_output = outputs[0] # batch_size, sequence_length, hidden_size
cls_token = sequence_output[:, 0]
patch_tokens = sequence_output[:, 1:]
linear_input = torch.cat([cls_token, patch_tokens.mean(dim=1)], dim=1)
logits = self.classifier(linear_input)
loss = None
if labels is not None:
# move labels to correct device to enable model parallelism
labels = labels.to(logits.device)
if self.config.problem_type is None:
if self.num_labels == 1:
self.config.problem_type = "regression"
elif self.num_labels > 1 and (labels.dtype == torch.long or labels.dtype == torch.int):
self.config.problem_type = "single_label_classification"
else:
self.config.problem_type = "multi_label_classification"
if self.config.problem_type == "regression":
loss_fct = MSELoss()
if self.num_labels == 1:
loss = loss_fct(logits.squeeze(), labels.squeeze())
else:
loss = loss_fct(logits, labels)
elif self.config.problem_type == "single_label_classification":
loss_fct = CrossEntropyLoss()
loss = loss_fct(logits.view(-1, self.num_labels), labels.view(-1))
elif self.config.problem_type == "multi_label_classification":
loss_fct = BCEWithLogitsLoss()
loss = loss_fct(logits, labels)
if not return_dict:
output = (logits,) + outputs[2:]
return ((loss,) + output) if loss is not None else output
return ImageClassifierOutput(
loss=loss,
logits=logits,
hidden_states=outputs.hidden_states,
attentions=outputs.attentions,
)
@add_start_docstrings(
"""
Dinov2 backbone, to be used with frameworks like DETR and MaskFormer.
""",
DINOV2_START_DOCSTRING,
)
class Dinov2Backbone(Dinov2PreTrainedModel, BackboneMixin):
def __init__(self, config):
super().__init__(config)
super()._init_backbone(config)
self.num_features = [config.hidden_size for _ in range(config.num_hidden_layers + 1)]
self.embeddings = Dinov2Embeddings(config)
self.encoder = Dinov2Encoder(config)
self.layernorm = nn.LayerNorm(config.hidden_size, eps=config.layer_norm_eps)
# Initialize weights and apply final processing
self.post_init()
def get_input_embeddings(self) -> Dinov2PatchEmbeddings:
return self.embeddings.patch_embeddings
@add_start_docstrings_to_model_forward(DINOV2_INPUTS_DOCSTRING)
@replace_return_docstrings(output_type=BackboneOutput, config_class=_CONFIG_FOR_DOC)
def forward(
self,
pixel_values: torch.Tensor,
output_hidden_states: Optional[bool] = None,
output_attentions: Optional[bool] = None,
return_dict: Optional[bool] = None,
) -> BackboneOutput:
"""
Returns:
Examples:
```python
>>> from transformers import AutoImageProcessor, AutoBackbone
>>> import torch
>>> from PIL import Image
>>> import requests
>>> url = "http://images.cocodataset.org/val2017/000000039769.jpg"
>>> image = Image.open(requests.get(url, stream=True).raw)
>>> processor = AutoImageProcessor.from_pretrained("facebook/dinov2-base")
>>> model = AutoBackbone.from_pretrained(
... "facebook/dinov2-base", out_features=["stage2", "stage5", "stage8", "stage11"]
... )
>>> inputs = processor(image, return_tensors="pt")
>>> outputs = model(**inputs)
>>> feature_maps = outputs.feature_maps
>>> list(feature_maps[-1].shape)
[1, 768, 16, 16]
```"""
return_dict = return_dict if return_dict is not None else self.config.use_return_dict
output_hidden_states = (
output_hidden_states if output_hidden_states is not None else self.config.output_hidden_states
)
output_attentions = output_attentions if output_attentions is not None else self.config.output_attentions
embedding_output = self.embeddings(pixel_values)
outputs = self.encoder(
embedding_output, output_hidden_states=True, output_attentions=output_attentions, return_dict=return_dict
)
hidden_states = outputs.hidden_states if return_dict else outputs[1]
feature_maps = ()
for stage, hidden_state in zip(self.stage_names, hidden_states):
if stage in self.out_features:
if self.config.apply_layernorm:
hidden_state = self.layernorm(hidden_state)
if self.config.reshape_hidden_states:
hidden_state = hidden_state[:, 1:]
# this was actually a bug in the original implementation that we copied here,
# cause normally the order is height, width
batch_size, _, height, width = pixel_values.shape
patch_size = self.config.patch_size
hidden_state = hidden_state.reshape(batch_size, height // patch_size, width // patch_size, -1)
hidden_state = hidden_state.permute(0, 3, 1, 2).contiguous()
feature_maps += (hidden_state,)
if not return_dict:
if output_hidden_states:
output = (feature_maps,) + outputs[1:]
else:
output = (feature_maps,) + outputs[2:]
return output
return BackboneOutput(
feature_maps=feature_maps,
hidden_states=outputs.hidden_states if output_hidden_states else None,
attentions=outputs.attentions if output_attentions else None,
)
| transformers/src/transformers/models/dinov2/modeling_dinov2.py/0 | {
"file_path": "transformers/src/transformers/models/dinov2/modeling_dinov2.py",
"repo_id": "transformers",
"token_count": 15219
} | 324 |
# coding=utf-8
# Copyright 2022 The HuggingFace Inc. team.
#
# Licensed under the Apache License, Version 2.0 (the "License");
# you may not use this file except in compliance with the License.
# You may obtain a copy of the License at
#
# http://www.apache.org/licenses/LICENSE-2.0
#
# Unless required by applicable law or agreed to in writing, software
# distributed under the License is distributed on an "AS IS" BASIS,
# WITHOUT WARRANTIES OR CONDITIONS OF ANY KIND, either express or implied.
# See the License for the specific language governing permissions and
# limitations under the License.
"""
Processor class for Donut.
"""
import re
import warnings
from contextlib import contextmanager
from ...processing_utils import ProcessorMixin
class DonutProcessor(ProcessorMixin):
r"""
Constructs a Donut processor which wraps a Donut image processor and an XLMRoBERTa tokenizer into a single
processor.
[`DonutProcessor`] offers all the functionalities of [`DonutImageProcessor`] and
[`XLMRobertaTokenizer`/`XLMRobertaTokenizerFast`]. See the [`~DonutProcessor.__call__`] and
[`~DonutProcessor.decode`] for more information.
Args:
image_processor ([`DonutImageProcessor`], *optional*):
An instance of [`DonutImageProcessor`]. The image processor is a required input.
tokenizer ([`XLMRobertaTokenizer`/`XLMRobertaTokenizerFast`], *optional*):
An instance of [`XLMRobertaTokenizer`/`XLMRobertaTokenizerFast`]. The tokenizer is a required input.
"""
attributes = ["image_processor", "tokenizer"]
image_processor_class = "AutoImageProcessor"
tokenizer_class = "AutoTokenizer"
def __init__(self, image_processor=None, tokenizer=None, **kwargs):
feature_extractor = None
if "feature_extractor" in kwargs:
warnings.warn(
"The `feature_extractor` argument is deprecated and will be removed in v5, use `image_processor`"
" instead.",
FutureWarning,
)
feature_extractor = kwargs.pop("feature_extractor")
image_processor = image_processor if image_processor is not None else feature_extractor
if image_processor is None:
raise ValueError("You need to specify an `image_processor`.")
if tokenizer is None:
raise ValueError("You need to specify a `tokenizer`.")
super().__init__(image_processor, tokenizer)
self.current_processor = self.image_processor
self._in_target_context_manager = False
def __call__(self, *args, **kwargs):
"""
When used in normal mode, this method forwards all its arguments to AutoImageProcessor's
[`~AutoImageProcessor.__call__`] and returns its output. If used in the context
[`~DonutProcessor.as_target_processor`] this method forwards all its arguments to DonutTokenizer's
[`~DonutTokenizer.__call__`]. Please refer to the doctsring of the above two methods for more information.
"""
# For backward compatibility
if self._in_target_context_manager:
return self.current_processor(*args, **kwargs)
images = kwargs.pop("images", None)
text = kwargs.pop("text", None)
if len(args) > 0:
images = args[0]
args = args[1:]
if images is None and text is None:
raise ValueError("You need to specify either an `images` or `text` input to process.")
if images is not None:
inputs = self.image_processor(images, *args, **kwargs)
if text is not None:
encodings = self.tokenizer(text, **kwargs)
if text is None:
return inputs
elif images is None:
return encodings
else:
inputs["labels"] = encodings["input_ids"]
return inputs
def batch_decode(self, *args, **kwargs):
"""
This method forwards all its arguments to DonutTokenizer's [`~PreTrainedTokenizer.batch_decode`]. Please refer
to the docstring of this method for more information.
"""
return self.tokenizer.batch_decode(*args, **kwargs)
def decode(self, *args, **kwargs):
"""
This method forwards all its arguments to DonutTokenizer's [`~PreTrainedTokenizer.decode`]. Please refer to the
docstring of this method for more information.
"""
return self.tokenizer.decode(*args, **kwargs)
@contextmanager
def as_target_processor(self):
"""
Temporarily sets the tokenizer for processing the input. Useful for encoding the labels when fine-tuning TrOCR.
"""
warnings.warn(
"`as_target_processor` is deprecated and will be removed in v5 of Transformers. You can process your "
"labels by using the argument `text` of the regular `__call__` method (either in the same call as "
"your images inputs, or in a separate call."
)
self._in_target_context_manager = True
self.current_processor = self.tokenizer
yield
self.current_processor = self.image_processor
self._in_target_context_manager = False
def token2json(self, tokens, is_inner_value=False, added_vocab=None):
"""
Convert a (generated) token sequence into an ordered JSON format.
"""
if added_vocab is None:
added_vocab = self.tokenizer.get_added_vocab()
output = {}
while tokens:
start_token = re.search(r"<s_(.*?)>", tokens, re.IGNORECASE)
if start_token is None:
break
key = start_token.group(1)
key_escaped = re.escape(key)
end_token = re.search(rf"</s_{key_escaped}>", tokens, re.IGNORECASE)
start_token = start_token.group()
if end_token is None:
tokens = tokens.replace(start_token, "")
else:
end_token = end_token.group()
start_token_escaped = re.escape(start_token)
end_token_escaped = re.escape(end_token)
content = re.search(f"{start_token_escaped}(.*?){end_token_escaped}", tokens, re.IGNORECASE)
if content is not None:
content = content.group(1).strip()
if r"<s_" in content and r"</s_" in content: # non-leaf node
value = self.token2json(content, is_inner_value=True, added_vocab=added_vocab)
if value:
if len(value) == 1:
value = value[0]
output[key] = value
else: # leaf nodes
output[key] = []
for leaf in content.split(r"<sep/>"):
leaf = leaf.strip()
if leaf in added_vocab and leaf[0] == "<" and leaf[-2:] == "/>":
leaf = leaf[1:-2] # for categorical special tokens
output[key].append(leaf)
if len(output[key]) == 1:
output[key] = output[key][0]
tokens = tokens[tokens.find(end_token) + len(end_token) :].strip()
if tokens[:6] == r"<sep/>": # non-leaf nodes
return [output] + self.token2json(tokens[6:], is_inner_value=True, added_vocab=added_vocab)
if len(output):
return [output] if is_inner_value else output
else:
return [] if is_inner_value else {"text_sequence": tokens}
@property
def feature_extractor_class(self):
warnings.warn(
"`feature_extractor_class` is deprecated and will be removed in v5. Use `image_processor_class` instead.",
FutureWarning,
)
return self.image_processor_class
@property
def feature_extractor(self):
warnings.warn(
"`feature_extractor` is deprecated and will be removed in v5. Use `image_processor` instead.",
FutureWarning,
)
return self.image_processor
| transformers/src/transformers/models/donut/processing_donut.py/0 | {
"file_path": "transformers/src/transformers/models/donut/processing_donut.py",
"repo_id": "transformers",
"token_count": 3519
} | 325 |
# coding=utf-8
# Copyright 2022 The HuggingFace Inc. team. All rights reserved.
#
# Licensed under the Apache License, Version 2.0 (the "License");
# you may not use this file except in compliance with the License.
# You may obtain a copy of the License at
#
# http://www.apache.org/licenses/LICENSE-2.0
#
# Unless required by applicable law or agreed to in writing, software
# distributed under the License is distributed on an "AS IS" BASIS,
# WITHOUT WARRANTIES OR CONDITIONS OF ANY KIND, either express or implied.
# See the License for the specific language governing permissions and
# limitations under the License.
"""Image processor class for DPT."""
import math
from typing import Dict, Iterable, List, Optional, Tuple, Union
import numpy as np
from ...image_processing_utils import BaseImageProcessor, BatchFeature, get_size_dict
from ...image_transforms import pad, resize, to_channel_dimension_format
from ...image_utils import (
IMAGENET_STANDARD_MEAN,
IMAGENET_STANDARD_STD,
ChannelDimension,
ImageInput,
PILImageResampling,
get_image_size,
infer_channel_dimension_format,
is_scaled_image,
is_torch_available,
is_torch_tensor,
make_list_of_images,
to_numpy_array,
valid_images,
)
from ...utils import TensorType, is_vision_available, logging
if is_torch_available():
import torch
if is_vision_available():
import PIL
logger = logging.get_logger(__name__)
def get_resize_output_image_size(
input_image: np.ndarray,
output_size: Union[int, Iterable[int]],
keep_aspect_ratio: bool,
multiple: int,
input_data_format: Optional[Union[str, ChannelDimension]] = None,
) -> Tuple[int, int]:
def constraint_to_multiple_of(val, multiple, min_val=0, max_val=None):
x = round(val / multiple) * multiple
if max_val is not None and x > max_val:
x = math.floor(val / multiple) * multiple
if x < min_val:
x = math.ceil(val / multiple) * multiple
return x
output_size = (output_size, output_size) if isinstance(output_size, int) else output_size
input_height, input_width = get_image_size(input_image, input_data_format)
output_height, output_width = output_size
# determine new height and width
scale_height = output_height / input_height
scale_width = output_width / input_width
if keep_aspect_ratio:
# scale as little as possible
if abs(1 - scale_width) < abs(1 - scale_height):
# fit width
scale_height = scale_width
else:
# fit height
scale_width = scale_height
new_height = constraint_to_multiple_of(scale_height * input_height, multiple=multiple)
new_width = constraint_to_multiple_of(scale_width * input_width, multiple=multiple)
return (new_height, new_width)
class DPTImageProcessor(BaseImageProcessor):
r"""
Constructs a DPT image processor.
Args:
do_resize (`bool`, *optional*, defaults to `True`):
Whether to resize the image's (height, width) dimensions. Can be overidden by `do_resize` in `preprocess`.
size (`Dict[str, int]` *optional*, defaults to `{"height": 384, "width": 384}`):
Size of the image after resizing. Can be overidden by `size` in `preprocess`.
resample (`PILImageResampling`, *optional*, defaults to `Resampling.BICUBIC`):
Defines the resampling filter to use if resizing the image. Can be overidden by `resample` in `preprocess`.
keep_aspect_ratio (`bool`, *optional*, defaults to `False`):
If `True`, the image is resized to the largest possible size such that the aspect ratio is preserved. Can
be overidden by `keep_aspect_ratio` in `preprocess`.
ensure_multiple_of (`int`, *optional*, defaults to 1):
If `do_resize` is `True`, the image is resized to a size that is a multiple of this value. Can be overidden
by `ensure_multiple_of` in `preprocess`.
do_rescale (`bool`, *optional*, defaults to `True`):
Whether to rescale the image by the specified scale `rescale_factor`. Can be overidden by `do_rescale` in
`preprocess`.
rescale_factor (`int` or `float`, *optional*, defaults to `1/255`):
Scale factor to use if rescaling the image. Can be overidden by `rescale_factor` in `preprocess`.
do_normalize (`bool`, *optional*, defaults to `True`):
Whether to normalize the image. Can be overridden by the `do_normalize` parameter in the `preprocess`
method.
image_mean (`float` or `List[float]`, *optional*, defaults to `IMAGENET_STANDARD_MEAN`):
Mean to use if normalizing the image. This is a float or list of floats the length of the number of
channels in the image. Can be overridden by the `image_mean` parameter in the `preprocess` method.
image_std (`float` or `List[float]`, *optional*, defaults to `IMAGENET_STANDARD_STD`):
Standard deviation to use if normalizing the image. This is a float or list of floats the length of the
number of channels in the image. Can be overridden by the `image_std` parameter in the `preprocess` method.
do_pad (`bool`, *optional*, defaults to `False`):
Whether to apply center padding. This was introduced in the DINOv2 paper, which uses the model in
combination with DPT.
size_divisor (`int`, *optional*):
If `do_pad` is `True`, pads the image dimensions to be divisible by this value. This was introduced in the
DINOv2 paper, which uses the model in combination with DPT.
"""
model_input_names = ["pixel_values"]
def __init__(
self,
do_resize: bool = True,
size: Dict[str, int] = None,
resample: PILImageResampling = PILImageResampling.BICUBIC,
keep_aspect_ratio: bool = False,
ensure_multiple_of: int = 1,
do_rescale: bool = True,
rescale_factor: Union[int, float] = 1 / 255,
do_normalize: bool = True,
image_mean: Optional[Union[float, List[float]]] = None,
image_std: Optional[Union[float, List[float]]] = None,
do_pad: bool = False,
size_divisor: int = None,
**kwargs,
) -> None:
super().__init__(**kwargs)
size = size if size is not None else {"height": 384, "width": 384}
size = get_size_dict(size)
self.do_resize = do_resize
self.size = size
self.keep_aspect_ratio = keep_aspect_ratio
self.ensure_multiple_of = ensure_multiple_of
self.resample = resample
self.do_rescale = do_rescale
self.rescale_factor = rescale_factor
self.do_normalize = do_normalize
self.image_mean = image_mean if image_mean is not None else IMAGENET_STANDARD_MEAN
self.image_std = image_std if image_std is not None else IMAGENET_STANDARD_STD
self.do_pad = do_pad
self.size_divisor = size_divisor
def resize(
self,
image: np.ndarray,
size: Dict[str, int],
keep_aspect_ratio: bool = False,
ensure_multiple_of: int = 1,
resample: PILImageResampling = PILImageResampling.BICUBIC,
data_format: Optional[Union[str, ChannelDimension]] = None,
input_data_format: Optional[Union[str, ChannelDimension]] = None,
**kwargs,
) -> np.ndarray:
"""
Resize an image to target size `(size["height"], size["width"])`. If `keep_aspect_ratio` is `True`, the image
is resized to the largest possible size such that the aspect ratio is preserved. If `ensure_multiple_of` is
set, the image is resized to a size that is a multiple of this value.
Args:
image (`np.ndarray`):
Image to resize.
size (`Dict[str, int]`):
Target size of the output image.
keep_aspect_ratio (`bool`, *optional*, defaults to `False`):
If `True`, the image is resized to the largest possible size such that the aspect ratio is preserved.
ensure_multiple_of (`int`, *optional*, defaults to 1):
The image is resized to a size that is a multiple of this value.
resample (`PILImageResampling`, *optional*, defaults to `PILImageResampling.BICUBIC`):
Defines the resampling filter to use if resizing the image. Otherwise, the image is resized to size
specified in `size`.
resample (`PILImageResampling`, *optional*, defaults to `PILImageResampling.BICUBIC`):
Resampling filter to use when resiizing the image.
data_format (`str` or `ChannelDimension`, *optional*):
The channel dimension format of the image. If not provided, it will be the same as the input image.
input_data_format (`str` or `ChannelDimension`, *optional*):
The channel dimension format of the input image. If not provided, it will be inferred.
"""
size = get_size_dict(size)
if "height" not in size or "width" not in size:
raise ValueError(f"The size dictionary must contain the keys 'height' and 'width'. Got {size.keys()}")
output_size = get_resize_output_image_size(
image,
output_size=(size["height"], size["width"]),
keep_aspect_ratio=keep_aspect_ratio,
multiple=ensure_multiple_of,
input_data_format=input_data_format,
)
return resize(
image,
size=output_size,
resample=resample,
data_format=data_format,
input_data_format=input_data_format,
**kwargs,
)
def pad_image(
self,
image: np.array,
size_divisor: int,
data_format: Optional[Union[str, ChannelDimension]] = None,
input_data_format: Optional[Union[str, ChannelDimension]] = None,
):
"""
Center pad an image to be a multiple of `multiple`.
Args:
image (`np.ndarray`):
Image to pad.
size_divisor (`int`):
The width and height of the image will be padded to a multiple of this number.
data_format (`ChannelDimension` or `str`, *optional*, defaults to `ChannelDimension.FIRST`):
The channel dimension format for the output image. Can be one of:
- `"channels_first"` or `ChannelDimension.FIRST`: image in (num_channels, height, width) format.
- `"channels_last"` or `ChannelDimension.LAST`: image in (height, width, num_channels) format.
- Unset: Use the channel dimension format of the input image.
input_data_format (`ChannelDimension` or `str`, *optional*):
The channel dimension format for the input image. If unset, the channel dimension format is inferred
from the input image. Can be one of:
- `"channels_first"` or `ChannelDimension.FIRST`: image in (num_channels, height, width) format.
- `"channels_last"` or `ChannelDimension.LAST`: image in (height, width, num_channels) format.
- `"none"` or `ChannelDimension.NONE`: image in (height, width) format.
"""
def _get_pad(size, size_divisor):
new_size = math.ceil(size / size_divisor) * size_divisor
pad_size = new_size - size
pad_size_left = pad_size // 2
pad_size_right = pad_size - pad_size_left
return pad_size_left, pad_size_right
if input_data_format is None:
input_data_format = infer_channel_dimension_format(image)
height, width = get_image_size(image, input_data_format)
pad_size_left, pad_size_right = _get_pad(height, size_divisor)
pad_size_top, pad_size_bottom = _get_pad(width, size_divisor)
return pad(image, ((pad_size_left, pad_size_right), (pad_size_top, pad_size_bottom)), data_format=data_format)
def preprocess(
self,
images: ImageInput,
do_resize: bool = None,
size: int = None,
keep_aspect_ratio: bool = None,
ensure_multiple_of: int = None,
resample: PILImageResampling = None,
do_rescale: bool = None,
rescale_factor: float = None,
do_normalize: bool = None,
image_mean: Optional[Union[float, List[float]]] = None,
image_std: Optional[Union[float, List[float]]] = None,
do_pad: bool = None,
size_divisor: int = None,
return_tensors: Optional[Union[str, TensorType]] = None,
data_format: ChannelDimension = ChannelDimension.FIRST,
input_data_format: Optional[Union[str, ChannelDimension]] = None,
**kwargs,
) -> PIL.Image.Image:
"""
Preprocess an image or batch of images.
Args:
images (`ImageInput`):
Image to preprocess. Expects a single or batch of images with pixel values ranging from 0 to 255. If
passing in images with pixel values between 0 and 1, set `do_rescale=False`.
do_resize (`bool`, *optional*, defaults to `self.do_resize`):
Whether to resize the image.
size (`Dict[str, int]`, *optional*, defaults to `self.size`):
Size of the image after reszing. If `keep_aspect_ratio` is `True`, the image is resized to the largest
possible size such that the aspect ratio is preserved. If `ensure_multiple_of` is set, the image is
resized to a size that is a multiple of this value.
keep_aspect_ratio (`bool`, *optional*, defaults to `self.keep_aspect_ratio`):
Whether to keep the aspect ratio of the image. If False, the image will be resized to (size, size). If
True, the image will be resized to keep the aspect ratio and the size will be the maximum possible.
ensure_multiple_of (`int`, *optional*, defaults to `self.ensure_multiple_of`):
Ensure that the image size is a multiple of this value.
resample (`int`, *optional*, defaults to `self.resample`):
Resampling filter to use if resizing the image. This can be one of the enum `PILImageResampling`, Only
has an effect if `do_resize` is set to `True`.
do_rescale (`bool`, *optional*, defaults to `self.do_rescale`):
Whether to rescale the image values between [0 - 1].
rescale_factor (`float`, *optional*, defaults to `self.rescale_factor`):
Rescale factor to rescale the image by if `do_rescale` is set to `True`.
do_normalize (`bool`, *optional*, defaults to `self.do_normalize`):
Whether to normalize the image.
image_mean (`float` or `List[float]`, *optional*, defaults to `self.image_mean`):
Image mean.
image_std (`float` or `List[float]`, *optional*, defaults to `self.image_std`):
Image standard deviation.
return_tensors (`str` or `TensorType`, *optional*):
The type of tensors to return. Can be one of:
- Unset: Return a list of `np.ndarray`.
- `TensorType.TENSORFLOW` or `'tf'`: Return a batch of type `tf.Tensor`.
- `TensorType.PYTORCH` or `'pt'`: Return a batch of type `torch.Tensor`.
- `TensorType.NUMPY` or `'np'`: Return a batch of type `np.ndarray`.
- `TensorType.JAX` or `'jax'`: Return a batch of type `jax.numpy.ndarray`.
data_format (`ChannelDimension` or `str`, *optional*, defaults to `ChannelDimension.FIRST`):
The channel dimension format for the output image. Can be one of:
- `ChannelDimension.FIRST`: image in (num_channels, height, width) format.
- `ChannelDimension.LAST`: image in (height, width, num_channels) format.
input_data_format (`ChannelDimension` or `str`, *optional*):
The channel dimension format for the input image. If unset, the channel dimension format is inferred
from the input image. Can be one of:
- `"channels_first"` or `ChannelDimension.FIRST`: image in (num_channels, height, width) format.
- `"channels_last"` or `ChannelDimension.LAST`: image in (height, width, num_channels) format.
- `"none"` or `ChannelDimension.NONE`: image in (height, width) format.
"""
do_resize = do_resize if do_resize is not None else self.do_resize
size = size if size is not None else self.size
size = get_size_dict(size)
keep_aspect_ratio = keep_aspect_ratio if keep_aspect_ratio is not None else self.keep_aspect_ratio
ensure_multiple_of = ensure_multiple_of if ensure_multiple_of is not None else self.ensure_multiple_of
resample = resample if resample is not None else self.resample
do_rescale = do_rescale if do_rescale is not None else self.do_rescale
rescale_factor = rescale_factor if rescale_factor is not None else self.rescale_factor
do_normalize = do_normalize if do_normalize is not None else self.do_normalize
image_mean = image_mean if image_mean is not None else self.image_mean
image_std = image_std if image_std is not None else self.image_std
do_pad = do_pad if do_pad is not None else self.do_pad
size_divisor = size_divisor if size_divisor is not None else self.size_divisor
images = make_list_of_images(images)
if not valid_images(images):
raise ValueError(
"Invalid image type. Must be of type PIL.Image.Image, numpy.ndarray, "
"torch.Tensor, tf.Tensor or jax.ndarray."
)
if do_resize and size is None or resample is None:
raise ValueError("Size and resample must be specified if do_resize is True.")
if do_rescale and rescale_factor is None:
raise ValueError("Rescale factor must be specified if do_rescale is True.")
if do_normalize and (image_mean is None or image_std is None):
raise ValueError("Image mean and std must be specified if do_normalize is True.")
if do_pad and size_divisor is None:
raise ValueError("Size divisibility must be specified if do_pad is True.")
# All transformations expect numpy arrays.
images = [to_numpy_array(image) for image in images]
if is_scaled_image(images[0]) and do_rescale:
logger.warning_once(
"It looks like you are trying to rescale already rescaled images. If the input"
" images have pixel values between 0 and 1, set `do_rescale=False` to avoid rescaling them again."
)
if input_data_format is None:
# We assume that all images have the same channel dimension format.
input_data_format = infer_channel_dimension_format(images[0])
if do_resize:
images = [
self.resize(
image=image,
size=size,
resample=resample,
keep_aspect_ratio=keep_aspect_ratio,
ensure_multiple_of=ensure_multiple_of,
input_data_format=input_data_format,
)
for image in images
]
if do_rescale:
images = [
self.rescale(image=image, scale=rescale_factor, input_data_format=input_data_format)
for image in images
]
if do_normalize:
images = [
self.normalize(image=image, mean=image_mean, std=image_std, input_data_format=input_data_format)
for image in images
]
if do_pad:
images = [
self.pad_image(image=image, size_divisor=size_divisor, input_data_format=input_data_format)
for image in images
]
images = [
to_channel_dimension_format(image, data_format, input_channel_dim=input_data_format) for image in images
]
data = {"pixel_values": images}
return BatchFeature(data=data, tensor_type=return_tensors)
# Copied from transformers.models.beit.image_processing_beit.BeitImageProcessor.post_process_semantic_segmentation with Beit->DPT
def post_process_semantic_segmentation(self, outputs, target_sizes: List[Tuple] = None):
"""
Converts the output of [`DPTForSemanticSegmentation`] into semantic segmentation maps. Only supports PyTorch.
Args:
outputs ([`DPTForSemanticSegmentation`]):
Raw outputs of the model.
target_sizes (`List[Tuple]` of length `batch_size`, *optional*):
List of tuples corresponding to the requested final size (height, width) of each prediction. If unset,
predictions will not be resized.
Returns:
semantic_segmentation: `List[torch.Tensor]` of length `batch_size`, where each item is a semantic
segmentation map of shape (height, width) corresponding to the target_sizes entry (if `target_sizes` is
specified). Each entry of each `torch.Tensor` correspond to a semantic class id.
"""
# TODO: add support for other frameworks
logits = outputs.logits
# Resize logits and compute semantic segmentation maps
if target_sizes is not None:
if len(logits) != len(target_sizes):
raise ValueError(
"Make sure that you pass in as many target sizes as the batch dimension of the logits"
)
if is_torch_tensor(target_sizes):
target_sizes = target_sizes.numpy()
semantic_segmentation = []
for idx in range(len(logits)):
resized_logits = torch.nn.functional.interpolate(
logits[idx].unsqueeze(dim=0), size=target_sizes[idx], mode="bilinear", align_corners=False
)
semantic_map = resized_logits[0].argmax(dim=0)
semantic_segmentation.append(semantic_map)
else:
semantic_segmentation = logits.argmax(dim=1)
semantic_segmentation = [semantic_segmentation[i] for i in range(semantic_segmentation.shape[0])]
return semantic_segmentation
| transformers/src/transformers/models/dpt/image_processing_dpt.py/0 | {
"file_path": "transformers/src/transformers/models/dpt/image_processing_dpt.py",
"repo_id": "transformers",
"token_count": 9556
} | 326 |
# coding=utf-8
# Copyright 2019 The Google AI Language Team Authors and The HuggingFace Inc. team.
#
# Licensed under the Apache License, Version 2.0 (the "License");
# you may not use this file except in compliance with the License.
# You may obtain a copy of the License at
#
# http://www.apache.org/licenses/LICENSE-2.0
#
# Unless required by applicable law or agreed to in writing, software
# distributed under the License is distributed on an "AS IS" BASIS,
# WITHOUT WARRANTIES OR CONDITIONS OF ANY KIND, either express or implied.
# See the License for the specific language governing permissions and
# limitations under the License.
"""PyTorch ELECTRA model."""
import math
import os
from dataclasses import dataclass
from typing import List, Optional, Tuple, Union
import torch
import torch.utils.checkpoint
from torch import nn
from torch.nn import BCEWithLogitsLoss, CrossEntropyLoss, MSELoss
from ...activations import ACT2FN, get_activation
from ...modeling_outputs import (
BaseModelOutputWithCrossAttentions,
BaseModelOutputWithPastAndCrossAttentions,
CausalLMOutputWithCrossAttentions,
MaskedLMOutput,
MultipleChoiceModelOutput,
QuestionAnsweringModelOutput,
SequenceClassifierOutput,
TokenClassifierOutput,
)
from ...modeling_utils import PreTrainedModel, SequenceSummary
from ...pytorch_utils import apply_chunking_to_forward, find_pruneable_heads_and_indices, prune_linear_layer
from ...utils import (
ModelOutput,
add_code_sample_docstrings,
add_start_docstrings,
add_start_docstrings_to_model_forward,
logging,
replace_return_docstrings,
)
from .configuration_electra import ElectraConfig
logger = logging.get_logger(__name__)
_CHECKPOINT_FOR_DOC = "google/electra-small-discriminator"
_CONFIG_FOR_DOC = "ElectraConfig"
ELECTRA_PRETRAINED_MODEL_ARCHIVE_LIST = [
"google/electra-small-generator",
"google/electra-base-generator",
"google/electra-large-generator",
"google/electra-small-discriminator",
"google/electra-base-discriminator",
"google/electra-large-discriminator",
# See all ELECTRA models at https://huggingface.co/models?filter=electra
]
def load_tf_weights_in_electra(model, config, tf_checkpoint_path, discriminator_or_generator="discriminator"):
"""Load tf checkpoints in a pytorch model."""
try:
import re
import numpy as np
import tensorflow as tf
except ImportError:
logger.error(
"Loading a TensorFlow model in PyTorch, requires TensorFlow to be installed. Please see "
"https://www.tensorflow.org/install/ for installation instructions."
)
raise
tf_path = os.path.abspath(tf_checkpoint_path)
logger.info(f"Converting TensorFlow checkpoint from {tf_path}")
# Load weights from TF model
init_vars = tf.train.list_variables(tf_path)
names = []
arrays = []
for name, shape in init_vars:
logger.info(f"Loading TF weight {name} with shape {shape}")
array = tf.train.load_variable(tf_path, name)
names.append(name)
arrays.append(array)
for name, array in zip(names, arrays):
original_name: str = name
try:
if isinstance(model, ElectraForMaskedLM):
name = name.replace("electra/embeddings/", "generator/embeddings/")
if discriminator_or_generator == "generator":
name = name.replace("electra/", "discriminator/")
name = name.replace("generator/", "electra/")
name = name.replace("dense_1", "dense_prediction")
name = name.replace("generator_predictions/output_bias", "generator_lm_head/bias")
name = name.split("/")
# print(original_name, name)
# adam_v and adam_m are variables used in AdamWeightDecayOptimizer to calculated m and v
# which are not required for using pretrained model
if any(n in ["global_step", "temperature"] for n in name):
logger.info(f"Skipping {original_name}")
continue
pointer = model
for m_name in name:
if re.fullmatch(r"[A-Za-z]+_\d+", m_name):
scope_names = re.split(r"_(\d+)", m_name)
else:
scope_names = [m_name]
if scope_names[0] == "kernel" or scope_names[0] == "gamma":
pointer = getattr(pointer, "weight")
elif scope_names[0] == "output_bias" or scope_names[0] == "beta":
pointer = getattr(pointer, "bias")
elif scope_names[0] == "output_weights":
pointer = getattr(pointer, "weight")
elif scope_names[0] == "squad":
pointer = getattr(pointer, "classifier")
else:
pointer = getattr(pointer, scope_names[0])
if len(scope_names) >= 2:
num = int(scope_names[1])
pointer = pointer[num]
if m_name.endswith("_embeddings"):
pointer = getattr(pointer, "weight")
elif m_name == "kernel":
array = np.transpose(array)
try:
if pointer.shape != array.shape:
raise ValueError(f"Pointer shape {pointer.shape} and array shape {array.shape} mismatched")
except ValueError as e:
e.args += (pointer.shape, array.shape)
raise
print(f"Initialize PyTorch weight {name}", original_name)
pointer.data = torch.from_numpy(array)
except AttributeError as e:
print(f"Skipping {original_name}", name, e)
continue
return model
class ElectraEmbeddings(nn.Module):
"""Construct the embeddings from word, position and token_type embeddings."""
def __init__(self, config):
super().__init__()
self.word_embeddings = nn.Embedding(config.vocab_size, config.embedding_size, padding_idx=config.pad_token_id)
self.position_embeddings = nn.Embedding(config.max_position_embeddings, config.embedding_size)
self.token_type_embeddings = nn.Embedding(config.type_vocab_size, config.embedding_size)
# self.LayerNorm is not snake-cased to stick with TensorFlow model variable name and be able to load
# any TensorFlow checkpoint file
self.LayerNorm = nn.LayerNorm(config.embedding_size, eps=config.layer_norm_eps)
self.dropout = nn.Dropout(config.hidden_dropout_prob)
# position_ids (1, len position emb) is contiguous in memory and exported when serialized
self.register_buffer(
"position_ids", torch.arange(config.max_position_embeddings).expand((1, -1)), persistent=False
)
self.position_embedding_type = getattr(config, "position_embedding_type", "absolute")
self.register_buffer(
"token_type_ids", torch.zeros(self.position_ids.size(), dtype=torch.long), persistent=False
)
# Copied from transformers.models.bert.modeling_bert.BertEmbeddings.forward
def forward(
self,
input_ids: Optional[torch.LongTensor] = None,
token_type_ids: Optional[torch.LongTensor] = None,
position_ids: Optional[torch.LongTensor] = None,
inputs_embeds: Optional[torch.FloatTensor] = None,
past_key_values_length: int = 0,
) -> torch.Tensor:
if input_ids is not None:
input_shape = input_ids.size()
else:
input_shape = inputs_embeds.size()[:-1]
seq_length = input_shape[1]
if position_ids is None:
position_ids = self.position_ids[:, past_key_values_length : seq_length + past_key_values_length]
# Setting the token_type_ids to the registered buffer in constructor where it is all zeros, which usually occurs
# when its auto-generated, registered buffer helps users when tracing the model without passing token_type_ids, solves
# issue #5664
if token_type_ids is None:
if hasattr(self, "token_type_ids"):
buffered_token_type_ids = self.token_type_ids[:, :seq_length]
buffered_token_type_ids_expanded = buffered_token_type_ids.expand(input_shape[0], seq_length)
token_type_ids = buffered_token_type_ids_expanded
else:
token_type_ids = torch.zeros(input_shape, dtype=torch.long, device=self.position_ids.device)
if inputs_embeds is None:
inputs_embeds = self.word_embeddings(input_ids)
token_type_embeddings = self.token_type_embeddings(token_type_ids)
embeddings = inputs_embeds + token_type_embeddings
if self.position_embedding_type == "absolute":
position_embeddings = self.position_embeddings(position_ids)
embeddings += position_embeddings
embeddings = self.LayerNorm(embeddings)
embeddings = self.dropout(embeddings)
return embeddings
# Copied from transformers.models.bert.modeling_bert.BertSelfAttention with Bert->Electra
class ElectraSelfAttention(nn.Module):
def __init__(self, config, position_embedding_type=None):
super().__init__()
if config.hidden_size % config.num_attention_heads != 0 and not hasattr(config, "embedding_size"):
raise ValueError(
f"The hidden size ({config.hidden_size}) is not a multiple of the number of attention "
f"heads ({config.num_attention_heads})"
)
self.num_attention_heads = config.num_attention_heads
self.attention_head_size = int(config.hidden_size / config.num_attention_heads)
self.all_head_size = self.num_attention_heads * self.attention_head_size
self.query = nn.Linear(config.hidden_size, self.all_head_size)
self.key = nn.Linear(config.hidden_size, self.all_head_size)
self.value = nn.Linear(config.hidden_size, self.all_head_size)
self.dropout = nn.Dropout(config.attention_probs_dropout_prob)
self.position_embedding_type = position_embedding_type or getattr(
config, "position_embedding_type", "absolute"
)
if self.position_embedding_type == "relative_key" or self.position_embedding_type == "relative_key_query":
self.max_position_embeddings = config.max_position_embeddings
self.distance_embedding = nn.Embedding(2 * config.max_position_embeddings - 1, self.attention_head_size)
self.is_decoder = config.is_decoder
def transpose_for_scores(self, x: torch.Tensor) -> torch.Tensor:
new_x_shape = x.size()[:-1] + (self.num_attention_heads, self.attention_head_size)
x = x.view(new_x_shape)
return x.permute(0, 2, 1, 3)
def forward(
self,
hidden_states: torch.Tensor,
attention_mask: Optional[torch.FloatTensor] = None,
head_mask: Optional[torch.FloatTensor] = None,
encoder_hidden_states: Optional[torch.FloatTensor] = None,
encoder_attention_mask: Optional[torch.FloatTensor] = None,
past_key_value: Optional[Tuple[Tuple[torch.FloatTensor]]] = None,
output_attentions: Optional[bool] = False,
) -> Tuple[torch.Tensor]:
mixed_query_layer = self.query(hidden_states)
# If this is instantiated as a cross-attention module, the keys
# and values come from an encoder; the attention mask needs to be
# such that the encoder's padding tokens are not attended to.
is_cross_attention = encoder_hidden_states is not None
if is_cross_attention and past_key_value is not None:
# reuse k,v, cross_attentions
key_layer = past_key_value[0]
value_layer = past_key_value[1]
attention_mask = encoder_attention_mask
elif is_cross_attention:
key_layer = self.transpose_for_scores(self.key(encoder_hidden_states))
value_layer = self.transpose_for_scores(self.value(encoder_hidden_states))
attention_mask = encoder_attention_mask
elif past_key_value is not None:
key_layer = self.transpose_for_scores(self.key(hidden_states))
value_layer = self.transpose_for_scores(self.value(hidden_states))
key_layer = torch.cat([past_key_value[0], key_layer], dim=2)
value_layer = torch.cat([past_key_value[1], value_layer], dim=2)
else:
key_layer = self.transpose_for_scores(self.key(hidden_states))
value_layer = self.transpose_for_scores(self.value(hidden_states))
query_layer = self.transpose_for_scores(mixed_query_layer)
use_cache = past_key_value is not None
if self.is_decoder:
# if cross_attention save Tuple(torch.Tensor, torch.Tensor) of all cross attention key/value_states.
# Further calls to cross_attention layer can then reuse all cross-attention
# key/value_states (first "if" case)
# if uni-directional self-attention (decoder) save Tuple(torch.Tensor, torch.Tensor) of
# all previous decoder key/value_states. Further calls to uni-directional self-attention
# can concat previous decoder key/value_states to current projected key/value_states (third "elif" case)
# if encoder bi-directional self-attention `past_key_value` is always `None`
past_key_value = (key_layer, value_layer)
# Take the dot product between "query" and "key" to get the raw attention scores.
attention_scores = torch.matmul(query_layer, key_layer.transpose(-1, -2))
if self.position_embedding_type == "relative_key" or self.position_embedding_type == "relative_key_query":
query_length, key_length = query_layer.shape[2], key_layer.shape[2]
if use_cache:
position_ids_l = torch.tensor(key_length - 1, dtype=torch.long, device=hidden_states.device).view(
-1, 1
)
else:
position_ids_l = torch.arange(query_length, dtype=torch.long, device=hidden_states.device).view(-1, 1)
position_ids_r = torch.arange(key_length, dtype=torch.long, device=hidden_states.device).view(1, -1)
distance = position_ids_l - position_ids_r
positional_embedding = self.distance_embedding(distance + self.max_position_embeddings - 1)
positional_embedding = positional_embedding.to(dtype=query_layer.dtype) # fp16 compatibility
if self.position_embedding_type == "relative_key":
relative_position_scores = torch.einsum("bhld,lrd->bhlr", query_layer, positional_embedding)
attention_scores = attention_scores + relative_position_scores
elif self.position_embedding_type == "relative_key_query":
relative_position_scores_query = torch.einsum("bhld,lrd->bhlr", query_layer, positional_embedding)
relative_position_scores_key = torch.einsum("bhrd,lrd->bhlr", key_layer, positional_embedding)
attention_scores = attention_scores + relative_position_scores_query + relative_position_scores_key
attention_scores = attention_scores / math.sqrt(self.attention_head_size)
if attention_mask is not None:
# Apply the attention mask is (precomputed for all layers in ElectraModel forward() function)
attention_scores = attention_scores + attention_mask
# Normalize the attention scores to probabilities.
attention_probs = nn.functional.softmax(attention_scores, dim=-1)
# This is actually dropping out entire tokens to attend to, which might
# seem a bit unusual, but is taken from the original Transformer paper.
attention_probs = self.dropout(attention_probs)
# Mask heads if we want to
if head_mask is not None:
attention_probs = attention_probs * head_mask
context_layer = torch.matmul(attention_probs, value_layer)
context_layer = context_layer.permute(0, 2, 1, 3).contiguous()
new_context_layer_shape = context_layer.size()[:-2] + (self.all_head_size,)
context_layer = context_layer.view(new_context_layer_shape)
outputs = (context_layer, attention_probs) if output_attentions else (context_layer,)
if self.is_decoder:
outputs = outputs + (past_key_value,)
return outputs
# Copied from transformers.models.bert.modeling_bert.BertSelfOutput
class ElectraSelfOutput(nn.Module):
def __init__(self, config):
super().__init__()
self.dense = nn.Linear(config.hidden_size, config.hidden_size)
self.LayerNorm = nn.LayerNorm(config.hidden_size, eps=config.layer_norm_eps)
self.dropout = nn.Dropout(config.hidden_dropout_prob)
def forward(self, hidden_states: torch.Tensor, input_tensor: torch.Tensor) -> torch.Tensor:
hidden_states = self.dense(hidden_states)
hidden_states = self.dropout(hidden_states)
hidden_states = self.LayerNorm(hidden_states + input_tensor)
return hidden_states
# Copied from transformers.models.bert.modeling_bert.BertAttention with Bert->Electra
class ElectraAttention(nn.Module):
def __init__(self, config, position_embedding_type=None):
super().__init__()
self.self = ElectraSelfAttention(config, position_embedding_type=position_embedding_type)
self.output = ElectraSelfOutput(config)
self.pruned_heads = set()
def prune_heads(self, heads):
if len(heads) == 0:
return
heads, index = find_pruneable_heads_and_indices(
heads, self.self.num_attention_heads, self.self.attention_head_size, self.pruned_heads
)
# Prune linear layers
self.self.query = prune_linear_layer(self.self.query, index)
self.self.key = prune_linear_layer(self.self.key, index)
self.self.value = prune_linear_layer(self.self.value, index)
self.output.dense = prune_linear_layer(self.output.dense, index, dim=1)
# Update hyper params and store pruned heads
self.self.num_attention_heads = self.self.num_attention_heads - len(heads)
self.self.all_head_size = self.self.attention_head_size * self.self.num_attention_heads
self.pruned_heads = self.pruned_heads.union(heads)
def forward(
self,
hidden_states: torch.Tensor,
attention_mask: Optional[torch.FloatTensor] = None,
head_mask: Optional[torch.FloatTensor] = None,
encoder_hidden_states: Optional[torch.FloatTensor] = None,
encoder_attention_mask: Optional[torch.FloatTensor] = None,
past_key_value: Optional[Tuple[Tuple[torch.FloatTensor]]] = None,
output_attentions: Optional[bool] = False,
) -> Tuple[torch.Tensor]:
self_outputs = self.self(
hidden_states,
attention_mask,
head_mask,
encoder_hidden_states,
encoder_attention_mask,
past_key_value,
output_attentions,
)
attention_output = self.output(self_outputs[0], hidden_states)
outputs = (attention_output,) + self_outputs[1:] # add attentions if we output them
return outputs
# Copied from transformers.models.bert.modeling_bert.BertIntermediate
class ElectraIntermediate(nn.Module):
def __init__(self, config):
super().__init__()
self.dense = nn.Linear(config.hidden_size, config.intermediate_size)
if isinstance(config.hidden_act, str):
self.intermediate_act_fn = ACT2FN[config.hidden_act]
else:
self.intermediate_act_fn = config.hidden_act
def forward(self, hidden_states: torch.Tensor) -> torch.Tensor:
hidden_states = self.dense(hidden_states)
hidden_states = self.intermediate_act_fn(hidden_states)
return hidden_states
# Copied from transformers.models.bert.modeling_bert.BertOutput
class ElectraOutput(nn.Module):
def __init__(self, config):
super().__init__()
self.dense = nn.Linear(config.intermediate_size, config.hidden_size)
self.LayerNorm = nn.LayerNorm(config.hidden_size, eps=config.layer_norm_eps)
self.dropout = nn.Dropout(config.hidden_dropout_prob)
def forward(self, hidden_states: torch.Tensor, input_tensor: torch.Tensor) -> torch.Tensor:
hidden_states = self.dense(hidden_states)
hidden_states = self.dropout(hidden_states)
hidden_states = self.LayerNorm(hidden_states + input_tensor)
return hidden_states
# Copied from transformers.models.bert.modeling_bert.BertLayer with Bert->Electra
class ElectraLayer(nn.Module):
def __init__(self, config):
super().__init__()
self.chunk_size_feed_forward = config.chunk_size_feed_forward
self.seq_len_dim = 1
self.attention = ElectraAttention(config)
self.is_decoder = config.is_decoder
self.add_cross_attention = config.add_cross_attention
if self.add_cross_attention:
if not self.is_decoder:
raise ValueError(f"{self} should be used as a decoder model if cross attention is added")
self.crossattention = ElectraAttention(config, position_embedding_type="absolute")
self.intermediate = ElectraIntermediate(config)
self.output = ElectraOutput(config)
def forward(
self,
hidden_states: torch.Tensor,
attention_mask: Optional[torch.FloatTensor] = None,
head_mask: Optional[torch.FloatTensor] = None,
encoder_hidden_states: Optional[torch.FloatTensor] = None,
encoder_attention_mask: Optional[torch.FloatTensor] = None,
past_key_value: Optional[Tuple[Tuple[torch.FloatTensor]]] = None,
output_attentions: Optional[bool] = False,
) -> Tuple[torch.Tensor]:
# decoder uni-directional self-attention cached key/values tuple is at positions 1,2
self_attn_past_key_value = past_key_value[:2] if past_key_value is not None else None
self_attention_outputs = self.attention(
hidden_states,
attention_mask,
head_mask,
output_attentions=output_attentions,
past_key_value=self_attn_past_key_value,
)
attention_output = self_attention_outputs[0]
# if decoder, the last output is tuple of self-attn cache
if self.is_decoder:
outputs = self_attention_outputs[1:-1]
present_key_value = self_attention_outputs[-1]
else:
outputs = self_attention_outputs[1:] # add self attentions if we output attention weights
cross_attn_present_key_value = None
if self.is_decoder and encoder_hidden_states is not None:
if not hasattr(self, "crossattention"):
raise ValueError(
f"If `encoder_hidden_states` are passed, {self} has to be instantiated with cross-attention layers"
" by setting `config.add_cross_attention=True`"
)
# cross_attn cached key/values tuple is at positions 3,4 of past_key_value tuple
cross_attn_past_key_value = past_key_value[-2:] if past_key_value is not None else None
cross_attention_outputs = self.crossattention(
attention_output,
attention_mask,
head_mask,
encoder_hidden_states,
encoder_attention_mask,
cross_attn_past_key_value,
output_attentions,
)
attention_output = cross_attention_outputs[0]
outputs = outputs + cross_attention_outputs[1:-1] # add cross attentions if we output attention weights
# add cross-attn cache to positions 3,4 of present_key_value tuple
cross_attn_present_key_value = cross_attention_outputs[-1]
present_key_value = present_key_value + cross_attn_present_key_value
layer_output = apply_chunking_to_forward(
self.feed_forward_chunk, self.chunk_size_feed_forward, self.seq_len_dim, attention_output
)
outputs = (layer_output,) + outputs
# if decoder, return the attn key/values as the last output
if self.is_decoder:
outputs = outputs + (present_key_value,)
return outputs
def feed_forward_chunk(self, attention_output):
intermediate_output = self.intermediate(attention_output)
layer_output = self.output(intermediate_output, attention_output)
return layer_output
# Copied from transformers.models.bert.modeling_bert.BertEncoder with Bert->Electra
class ElectraEncoder(nn.Module):
def __init__(self, config):
super().__init__()
self.config = config
self.layer = nn.ModuleList([ElectraLayer(config) for _ in range(config.num_hidden_layers)])
self.gradient_checkpointing = False
def forward(
self,
hidden_states: torch.Tensor,
attention_mask: Optional[torch.FloatTensor] = None,
head_mask: Optional[torch.FloatTensor] = None,
encoder_hidden_states: Optional[torch.FloatTensor] = None,
encoder_attention_mask: Optional[torch.FloatTensor] = None,
past_key_values: Optional[Tuple[Tuple[torch.FloatTensor]]] = None,
use_cache: Optional[bool] = None,
output_attentions: Optional[bool] = False,
output_hidden_states: Optional[bool] = False,
return_dict: Optional[bool] = True,
) -> Union[Tuple[torch.Tensor], BaseModelOutputWithPastAndCrossAttentions]:
all_hidden_states = () if output_hidden_states else None
all_self_attentions = () if output_attentions else None
all_cross_attentions = () if output_attentions and self.config.add_cross_attention else None
if self.gradient_checkpointing and self.training:
if use_cache:
logger.warning_once(
"`use_cache=True` is incompatible with gradient checkpointing. Setting `use_cache=False`..."
)
use_cache = False
next_decoder_cache = () if use_cache else None
for i, layer_module in enumerate(self.layer):
if output_hidden_states:
all_hidden_states = all_hidden_states + (hidden_states,)
layer_head_mask = head_mask[i] if head_mask is not None else None
past_key_value = past_key_values[i] if past_key_values is not None else None
if self.gradient_checkpointing and self.training:
layer_outputs = self._gradient_checkpointing_func(
layer_module.__call__,
hidden_states,
attention_mask,
layer_head_mask,
encoder_hidden_states,
encoder_attention_mask,
past_key_value,
output_attentions,
)
else:
layer_outputs = layer_module(
hidden_states,
attention_mask,
layer_head_mask,
encoder_hidden_states,
encoder_attention_mask,
past_key_value,
output_attentions,
)
hidden_states = layer_outputs[0]
if use_cache:
next_decoder_cache += (layer_outputs[-1],)
if output_attentions:
all_self_attentions = all_self_attentions + (layer_outputs[1],)
if self.config.add_cross_attention:
all_cross_attentions = all_cross_attentions + (layer_outputs[2],)
if output_hidden_states:
all_hidden_states = all_hidden_states + (hidden_states,)
if not return_dict:
return tuple(
v
for v in [
hidden_states,
next_decoder_cache,
all_hidden_states,
all_self_attentions,
all_cross_attentions,
]
if v is not None
)
return BaseModelOutputWithPastAndCrossAttentions(
last_hidden_state=hidden_states,
past_key_values=next_decoder_cache,
hidden_states=all_hidden_states,
attentions=all_self_attentions,
cross_attentions=all_cross_attentions,
)
class ElectraDiscriminatorPredictions(nn.Module):
"""Prediction module for the discriminator, made up of two dense layers."""
def __init__(self, config):
super().__init__()
self.dense = nn.Linear(config.hidden_size, config.hidden_size)
self.activation = get_activation(config.hidden_act)
self.dense_prediction = nn.Linear(config.hidden_size, 1)
self.config = config
def forward(self, discriminator_hidden_states):
hidden_states = self.dense(discriminator_hidden_states)
hidden_states = self.activation(hidden_states)
logits = self.dense_prediction(hidden_states).squeeze(-1)
return logits
class ElectraGeneratorPredictions(nn.Module):
"""Prediction module for the generator, made up of two dense layers."""
def __init__(self, config):
super().__init__()
self.activation = get_activation("gelu")
self.LayerNorm = nn.LayerNorm(config.embedding_size, eps=config.layer_norm_eps)
self.dense = nn.Linear(config.hidden_size, config.embedding_size)
def forward(self, generator_hidden_states):
hidden_states = self.dense(generator_hidden_states)
hidden_states = self.activation(hidden_states)
hidden_states = self.LayerNorm(hidden_states)
return hidden_states
class ElectraPreTrainedModel(PreTrainedModel):
"""
An abstract class to handle weights initialization and a simple interface for downloading and loading pretrained
models.
"""
config_class = ElectraConfig
load_tf_weights = load_tf_weights_in_electra
base_model_prefix = "electra"
supports_gradient_checkpointing = True
# Copied from transformers.models.bert.modeling_bert.BertPreTrainedModel._init_weights
def _init_weights(self, module):
"""Initialize the weights"""
if isinstance(module, nn.Linear):
# Slightly different from the TF version which uses truncated_normal for initialization
# cf https://github.com/pytorch/pytorch/pull/5617
module.weight.data.normal_(mean=0.0, std=self.config.initializer_range)
if module.bias is not None:
module.bias.data.zero_()
elif isinstance(module, nn.Embedding):
module.weight.data.normal_(mean=0.0, std=self.config.initializer_range)
if module.padding_idx is not None:
module.weight.data[module.padding_idx].zero_()
elif isinstance(module, nn.LayerNorm):
module.bias.data.zero_()
module.weight.data.fill_(1.0)
@dataclass
class ElectraForPreTrainingOutput(ModelOutput):
"""
Output type of [`ElectraForPreTraining`].
Args:
loss (*optional*, returned when `labels` is provided, `torch.FloatTensor` of shape `(1,)`):
Total loss of the ELECTRA objective.
logits (`torch.FloatTensor` of shape `(batch_size, sequence_length)`):
Prediction scores of the head (scores for each token before SoftMax).
hidden_states (`tuple(torch.FloatTensor)`, *optional*, returned when `output_hidden_states=True` is passed or when `config.output_hidden_states=True`):
Tuple of `torch.FloatTensor` (one for the output of the embeddings + one for the output of each layer) of
shape `(batch_size, sequence_length, hidden_size)`.
Hidden-states of the model at the output of each layer plus the initial embedding outputs.
attentions (`tuple(torch.FloatTensor)`, *optional*, returned when `output_attentions=True` is passed or when `config.output_attentions=True`):
Tuple of `torch.FloatTensor` (one for each layer) of shape `(batch_size, num_heads, sequence_length,
sequence_length)`.
Attentions weights after the attention softmax, used to compute the weighted average in the self-attention
heads.
"""
loss: Optional[torch.FloatTensor] = None
logits: torch.FloatTensor = None
hidden_states: Optional[Tuple[torch.FloatTensor]] = None
attentions: Optional[Tuple[torch.FloatTensor]] = None
ELECTRA_START_DOCSTRING = r"""
This model inherits from [`PreTrainedModel`]. Check the superclass documentation for the generic methods the
library implements for all its model (such as downloading or saving, resizing the input embeddings, pruning heads
etc.)
This model is also a PyTorch [torch.nn.Module](https://pytorch.org/docs/stable/nn.html#torch.nn.Module) subclass.
Use it as a regular PyTorch Module and refer to the PyTorch documentation for all matter related to general usage
and behavior.
Parameters:
config ([`ElectraConfig`]): Model configuration class with all the parameters of the model.
Initializing with a config file does not load the weights associated with the model, only the
configuration. Check out the [`~PreTrainedModel.from_pretrained`] method to load the model weights.
"""
ELECTRA_INPUTS_DOCSTRING = r"""
Args:
input_ids (`torch.LongTensor` of shape `({0})`):
Indices of input sequence tokens in the vocabulary.
Indices can be obtained using [`AutoTokenizer`]. See [`PreTrainedTokenizer.encode`] and
[`PreTrainedTokenizer.__call__`] for details.
[What are input IDs?](../glossary#input-ids)
attention_mask (`torch.FloatTensor` of shape `({0})`, *optional*):
Mask to avoid performing attention on padding token indices. Mask values selected in `[0, 1]`:
- 1 for tokens that are **not masked**,
- 0 for tokens that are **masked**.
[What are attention masks?](../glossary#attention-mask)
token_type_ids (`torch.LongTensor` of shape `({0})`, *optional*):
Segment token indices to indicate first and second portions of the inputs. Indices are selected in `[0,
1]`:
- 0 corresponds to a *sentence A* token,
- 1 corresponds to a *sentence B* token.
[What are token type IDs?](../glossary#token-type-ids)
position_ids (`torch.LongTensor` of shape `({0})`, *optional*):
Indices of positions of each input sequence tokens in the position embeddings. Selected in the range `[0,
config.max_position_embeddings - 1]`.
[What are position IDs?](../glossary#position-ids)
head_mask (`torch.FloatTensor` of shape `(num_heads,)` or `(num_layers, num_heads)`, *optional*):
Mask to nullify selected heads of the self-attention modules. Mask values selected in `[0, 1]`:
- 1 indicates the head is **not masked**,
- 0 indicates the head is **masked**.
inputs_embeds (`torch.FloatTensor` of shape `({0}, hidden_size)`, *optional*):
Optionally, instead of passing `input_ids` you can choose to directly pass an embedded representation. This
is useful if you want more control over how to convert `input_ids` indices into associated vectors than the
model's internal embedding lookup matrix.
encoder_hidden_states (`torch.FloatTensor` of shape `({0}, hidden_size)`, *optional*):
Sequence of hidden-states at the output of the last layer of the encoder. Used in the cross-attention if
the model is configured as a decoder.
encoder_attention_mask (`torch.FloatTensor` of shape `({0})`, *optional*):
Mask to avoid performing attention on the padding token indices of the encoder input. This mask is used in
the cross-attention if the model is configured as a decoder. Mask values selected in `[0, 1]`:
- 1 indicates the head is **not masked**,
- 0 indicates the head is **masked**.
output_attentions (`bool`, *optional*):
Whether or not to return the attentions tensors of all attention layers. See `attentions` under returned
tensors for more detail.
output_hidden_states (`bool`, *optional*):
Whether or not to return the hidden states of all layers. See `hidden_states` under returned tensors for
more detail.
return_dict (`bool`, *optional*):
Whether or not to return a [`~utils.ModelOutput`] instead of a plain tuple.
"""
@add_start_docstrings(
"The bare Electra Model transformer outputting raw hidden-states without any specific head on top. Identical to "
"the BERT model except that it uses an additional linear layer between the embedding layer and the encoder if the "
"hidden size and embedding size are different. "
""
"Both the generator and discriminator checkpoints may be loaded into this model.",
ELECTRA_START_DOCSTRING,
)
class ElectraModel(ElectraPreTrainedModel):
def __init__(self, config):
super().__init__(config)
self.embeddings = ElectraEmbeddings(config)
if config.embedding_size != config.hidden_size:
self.embeddings_project = nn.Linear(config.embedding_size, config.hidden_size)
self.encoder = ElectraEncoder(config)
self.config = config
# Initialize weights and apply final processing
self.post_init()
def get_input_embeddings(self):
return self.embeddings.word_embeddings
def set_input_embeddings(self, value):
self.embeddings.word_embeddings = value
def _prune_heads(self, heads_to_prune):
"""
Prunes heads of the model. heads_to_prune: dict of {layer_num: list of heads to prune in this layer} See base
class PreTrainedModel
"""
for layer, heads in heads_to_prune.items():
self.encoder.layer[layer].attention.prune_heads(heads)
@add_start_docstrings_to_model_forward(ELECTRA_INPUTS_DOCSTRING.format("batch_size, sequence_length"))
@add_code_sample_docstrings(
checkpoint=_CHECKPOINT_FOR_DOC,
output_type=BaseModelOutputWithCrossAttentions,
config_class=_CONFIG_FOR_DOC,
)
def forward(
self,
input_ids: Optional[torch.Tensor] = None,
attention_mask: Optional[torch.Tensor] = None,
token_type_ids: Optional[torch.Tensor] = None,
position_ids: Optional[torch.Tensor] = None,
head_mask: Optional[torch.Tensor] = None,
inputs_embeds: Optional[torch.Tensor] = None,
encoder_hidden_states: Optional[torch.Tensor] = None,
encoder_attention_mask: Optional[torch.Tensor] = None,
past_key_values: Optional[List[torch.FloatTensor]] = None,
use_cache: Optional[bool] = None,
output_attentions: Optional[bool] = None,
output_hidden_states: Optional[bool] = None,
return_dict: Optional[bool] = None,
) -> Union[Tuple[torch.Tensor], BaseModelOutputWithCrossAttentions]:
output_attentions = output_attentions if output_attentions is not None else self.config.output_attentions
output_hidden_states = (
output_hidden_states if output_hidden_states is not None else self.config.output_hidden_states
)
return_dict = return_dict if return_dict is not None else self.config.use_return_dict
if input_ids is not None and inputs_embeds is not None:
raise ValueError("You cannot specify both input_ids and inputs_embeds at the same time")
elif input_ids is not None:
self.warn_if_padding_and_no_attention_mask(input_ids, attention_mask)
input_shape = input_ids.size()
elif inputs_embeds is not None:
input_shape = inputs_embeds.size()[:-1]
else:
raise ValueError("You have to specify either input_ids or inputs_embeds")
batch_size, seq_length = input_shape
device = input_ids.device if input_ids is not None else inputs_embeds.device
# past_key_values_length
past_key_values_length = past_key_values[0][0].shape[2] if past_key_values is not None else 0
if attention_mask is None:
attention_mask = torch.ones(input_shape, device=device)
if token_type_ids is None:
if hasattr(self.embeddings, "token_type_ids"):
buffered_token_type_ids = self.embeddings.token_type_ids[:, :seq_length]
buffered_token_type_ids_expanded = buffered_token_type_ids.expand(batch_size, seq_length)
token_type_ids = buffered_token_type_ids_expanded
else:
token_type_ids = torch.zeros(input_shape, dtype=torch.long, device=device)
extended_attention_mask = self.get_extended_attention_mask(attention_mask, input_shape)
# If a 2D or 3D attention mask is provided for the cross-attention
# we need to make broadcastable to [batch_size, num_heads, seq_length, seq_length]
if self.config.is_decoder and encoder_hidden_states is not None:
encoder_batch_size, encoder_sequence_length, _ = encoder_hidden_states.size()
encoder_hidden_shape = (encoder_batch_size, encoder_sequence_length)
if encoder_attention_mask is None:
encoder_attention_mask = torch.ones(encoder_hidden_shape, device=device)
encoder_extended_attention_mask = self.invert_attention_mask(encoder_attention_mask)
else:
encoder_extended_attention_mask = None
head_mask = self.get_head_mask(head_mask, self.config.num_hidden_layers)
hidden_states = self.embeddings(
input_ids=input_ids,
position_ids=position_ids,
token_type_ids=token_type_ids,
inputs_embeds=inputs_embeds,
past_key_values_length=past_key_values_length,
)
if hasattr(self, "embeddings_project"):
hidden_states = self.embeddings_project(hidden_states)
hidden_states = self.encoder(
hidden_states,
attention_mask=extended_attention_mask,
head_mask=head_mask,
encoder_hidden_states=encoder_hidden_states,
encoder_attention_mask=encoder_extended_attention_mask,
past_key_values=past_key_values,
use_cache=use_cache,
output_attentions=output_attentions,
output_hidden_states=output_hidden_states,
return_dict=return_dict,
)
return hidden_states
class ElectraClassificationHead(nn.Module):
"""Head for sentence-level classification tasks."""
def __init__(self, config):
super().__init__()
self.dense = nn.Linear(config.hidden_size, config.hidden_size)
classifier_dropout = (
config.classifier_dropout if config.classifier_dropout is not None else config.hidden_dropout_prob
)
self.activation = get_activation("gelu")
self.dropout = nn.Dropout(classifier_dropout)
self.out_proj = nn.Linear(config.hidden_size, config.num_labels)
def forward(self, features, **kwargs):
x = features[:, 0, :] # take <s> token (equiv. to [CLS])
x = self.dropout(x)
x = self.dense(x)
x = self.activation(x) # although BERT uses tanh here, it seems Electra authors used gelu here
x = self.dropout(x)
x = self.out_proj(x)
return x
@add_start_docstrings(
"""
ELECTRA Model transformer with a sequence classification/regression head on top (a linear layer on top of the
pooled output) e.g. for GLUE tasks.
""",
ELECTRA_START_DOCSTRING,
)
class ElectraForSequenceClassification(ElectraPreTrainedModel):
def __init__(self, config):
super().__init__(config)
self.num_labels = config.num_labels
self.config = config
self.electra = ElectraModel(config)
self.classifier = ElectraClassificationHead(config)
# Initialize weights and apply final processing
self.post_init()
@add_start_docstrings_to_model_forward(ELECTRA_INPUTS_DOCSTRING.format("batch_size, sequence_length"))
@add_code_sample_docstrings(
checkpoint="bhadresh-savani/electra-base-emotion",
output_type=SequenceClassifierOutput,
config_class=_CONFIG_FOR_DOC,
expected_output="'joy'",
expected_loss=0.06,
)
def forward(
self,
input_ids: Optional[torch.Tensor] = None,
attention_mask: Optional[torch.Tensor] = None,
token_type_ids: Optional[torch.Tensor] = None,
position_ids: Optional[torch.Tensor] = None,
head_mask: Optional[torch.Tensor] = None,
inputs_embeds: Optional[torch.Tensor] = None,
labels: Optional[torch.Tensor] = None,
output_attentions: Optional[bool] = None,
output_hidden_states: Optional[bool] = None,
return_dict: Optional[bool] = None,
) -> Union[Tuple[torch.Tensor], SequenceClassifierOutput]:
r"""
labels (`torch.LongTensor` of shape `(batch_size,)`, *optional*):
Labels for computing the sequence classification/regression loss. Indices should be in `[0, ...,
config.num_labels - 1]`. If `config.num_labels == 1` a regression loss is computed (Mean-Square loss), If
`config.num_labels > 1` a classification loss is computed (Cross-Entropy).
"""
return_dict = return_dict if return_dict is not None else self.config.use_return_dict
discriminator_hidden_states = self.electra(
input_ids,
attention_mask=attention_mask,
token_type_ids=token_type_ids,
position_ids=position_ids,
head_mask=head_mask,
inputs_embeds=inputs_embeds,
output_attentions=output_attentions,
output_hidden_states=output_hidden_states,
return_dict=return_dict,
)
sequence_output = discriminator_hidden_states[0]
logits = self.classifier(sequence_output)
loss = None
if labels is not None:
if self.config.problem_type is None:
if self.num_labels == 1:
self.config.problem_type = "regression"
elif self.num_labels > 1 and (labels.dtype == torch.long or labels.dtype == torch.int):
self.config.problem_type = "single_label_classification"
else:
self.config.problem_type = "multi_label_classification"
if self.config.problem_type == "regression":
loss_fct = MSELoss()
if self.num_labels == 1:
loss = loss_fct(logits.squeeze(), labels.squeeze())
else:
loss = loss_fct(logits, labels)
elif self.config.problem_type == "single_label_classification":
loss_fct = CrossEntropyLoss()
loss = loss_fct(logits.view(-1, self.num_labels), labels.view(-1))
elif self.config.problem_type == "multi_label_classification":
loss_fct = BCEWithLogitsLoss()
loss = loss_fct(logits, labels)
if not return_dict:
output = (logits,) + discriminator_hidden_states[1:]
return ((loss,) + output) if loss is not None else output
return SequenceClassifierOutput(
loss=loss,
logits=logits,
hidden_states=discriminator_hidden_states.hidden_states,
attentions=discriminator_hidden_states.attentions,
)
@add_start_docstrings(
"""
Electra model with a binary classification head on top as used during pretraining for identifying generated tokens.
It is recommended to load the discriminator checkpoint into that model.
""",
ELECTRA_START_DOCSTRING,
)
class ElectraForPreTraining(ElectraPreTrainedModel):
def __init__(self, config):
super().__init__(config)
self.electra = ElectraModel(config)
self.discriminator_predictions = ElectraDiscriminatorPredictions(config)
# Initialize weights and apply final processing
self.post_init()
@add_start_docstrings_to_model_forward(ELECTRA_INPUTS_DOCSTRING.format("batch_size, sequence_length"))
@replace_return_docstrings(output_type=ElectraForPreTrainingOutput, config_class=_CONFIG_FOR_DOC)
def forward(
self,
input_ids: Optional[torch.Tensor] = None,
attention_mask: Optional[torch.Tensor] = None,
token_type_ids: Optional[torch.Tensor] = None,
position_ids: Optional[torch.Tensor] = None,
head_mask: Optional[torch.Tensor] = None,
inputs_embeds: Optional[torch.Tensor] = None,
labels: Optional[torch.Tensor] = None,
output_attentions: Optional[bool] = None,
output_hidden_states: Optional[bool] = None,
return_dict: Optional[bool] = None,
) -> Union[Tuple[torch.Tensor], ElectraForPreTrainingOutput]:
r"""
labels (`torch.LongTensor` of shape `(batch_size, sequence_length)`, *optional*):
Labels for computing the ELECTRA loss. Input should be a sequence of tokens (see `input_ids` docstring)
Indices should be in `[0, 1]`:
- 0 indicates the token is an original token,
- 1 indicates the token was replaced.
Returns:
Examples:
```python
>>> from transformers import ElectraForPreTraining, AutoTokenizer
>>> import torch
>>> discriminator = ElectraForPreTraining.from_pretrained("google/electra-base-discriminator")
>>> tokenizer = AutoTokenizer.from_pretrained("google/electra-base-discriminator")
>>> sentence = "The quick brown fox jumps over the lazy dog"
>>> fake_sentence = "The quick brown fox fake over the lazy dog"
>>> fake_tokens = tokenizer.tokenize(fake_sentence, add_special_tokens=True)
>>> fake_inputs = tokenizer.encode(fake_sentence, return_tensors="pt")
>>> discriminator_outputs = discriminator(fake_inputs)
>>> predictions = torch.round((torch.sign(discriminator_outputs[0]) + 1) / 2)
>>> fake_tokens
['[CLS]', 'the', 'quick', 'brown', 'fox', 'fake', 'over', 'the', 'lazy', 'dog', '[SEP]']
>>> predictions.squeeze().tolist()
[0.0, 0.0, 0.0, 0.0, 0.0, 1.0, 0.0, 0.0, 0.0, 0.0, 0.0]
```"""
return_dict = return_dict if return_dict is not None else self.config.use_return_dict
discriminator_hidden_states = self.electra(
input_ids,
attention_mask=attention_mask,
token_type_ids=token_type_ids,
position_ids=position_ids,
head_mask=head_mask,
inputs_embeds=inputs_embeds,
output_attentions=output_attentions,
output_hidden_states=output_hidden_states,
return_dict=return_dict,
)
discriminator_sequence_output = discriminator_hidden_states[0]
logits = self.discriminator_predictions(discriminator_sequence_output)
loss = None
if labels is not None:
loss_fct = nn.BCEWithLogitsLoss()
if attention_mask is not None:
active_loss = attention_mask.view(-1, discriminator_sequence_output.shape[1]) == 1
active_logits = logits.view(-1, discriminator_sequence_output.shape[1])[active_loss]
active_labels = labels[active_loss]
loss = loss_fct(active_logits, active_labels.float())
else:
loss = loss_fct(logits.view(-1, discriminator_sequence_output.shape[1]), labels.float())
if not return_dict:
output = (logits,) + discriminator_hidden_states[1:]
return ((loss,) + output) if loss is not None else output
return ElectraForPreTrainingOutput(
loss=loss,
logits=logits,
hidden_states=discriminator_hidden_states.hidden_states,
attentions=discriminator_hidden_states.attentions,
)
@add_start_docstrings(
"""
Electra model with a language modeling head on top.
Even though both the discriminator and generator may be loaded into this model, the generator is the only model of
the two to have been trained for the masked language modeling task.
""",
ELECTRA_START_DOCSTRING,
)
class ElectraForMaskedLM(ElectraPreTrainedModel):
_tied_weights_keys = ["generator_lm_head.weight"]
def __init__(self, config):
super().__init__(config)
self.electra = ElectraModel(config)
self.generator_predictions = ElectraGeneratorPredictions(config)
self.generator_lm_head = nn.Linear(config.embedding_size, config.vocab_size)
# Initialize weights and apply final processing
self.post_init()
def get_output_embeddings(self):
return self.generator_lm_head
def set_output_embeddings(self, word_embeddings):
self.generator_lm_head = word_embeddings
@add_start_docstrings_to_model_forward(ELECTRA_INPUTS_DOCSTRING.format("batch_size, sequence_length"))
@add_code_sample_docstrings(
checkpoint="google/electra-small-generator",
output_type=MaskedLMOutput,
config_class=_CONFIG_FOR_DOC,
mask="[MASK]",
expected_output="'paris'",
expected_loss=1.22,
)
def forward(
self,
input_ids: Optional[torch.Tensor] = None,
attention_mask: Optional[torch.Tensor] = None,
token_type_ids: Optional[torch.Tensor] = None,
position_ids: Optional[torch.Tensor] = None,
head_mask: Optional[torch.Tensor] = None,
inputs_embeds: Optional[torch.Tensor] = None,
labels: Optional[torch.Tensor] = None,
output_attentions: Optional[bool] = None,
output_hidden_states: Optional[bool] = None,
return_dict: Optional[bool] = None,
) -> Union[Tuple[torch.Tensor], MaskedLMOutput]:
r"""
labels (`torch.LongTensor` of shape `(batch_size, sequence_length)`, *optional*):
Labels for computing the masked language modeling loss. Indices should be in `[-100, 0, ...,
config.vocab_size]` (see `input_ids` docstring) Tokens with indices set to `-100` are ignored (masked), the
loss is only computed for the tokens with labels in `[0, ..., config.vocab_size]`
"""
return_dict = return_dict if return_dict is not None else self.config.use_return_dict
generator_hidden_states = self.electra(
input_ids,
attention_mask=attention_mask,
token_type_ids=token_type_ids,
position_ids=position_ids,
head_mask=head_mask,
inputs_embeds=inputs_embeds,
output_attentions=output_attentions,
output_hidden_states=output_hidden_states,
return_dict=return_dict,
)
generator_sequence_output = generator_hidden_states[0]
prediction_scores = self.generator_predictions(generator_sequence_output)
prediction_scores = self.generator_lm_head(prediction_scores)
loss = None
# Masked language modeling softmax layer
if labels is not None:
loss_fct = nn.CrossEntropyLoss() # -100 index = padding token
loss = loss_fct(prediction_scores.view(-1, self.config.vocab_size), labels.view(-1))
if not return_dict:
output = (prediction_scores,) + generator_hidden_states[1:]
return ((loss,) + output) if loss is not None else output
return MaskedLMOutput(
loss=loss,
logits=prediction_scores,
hidden_states=generator_hidden_states.hidden_states,
attentions=generator_hidden_states.attentions,
)
@add_start_docstrings(
"""
Electra model with a token classification head on top.
Both the discriminator and generator may be loaded into this model.
""",
ELECTRA_START_DOCSTRING,
)
class ElectraForTokenClassification(ElectraPreTrainedModel):
def __init__(self, config):
super().__init__(config)
self.num_labels = config.num_labels
self.electra = ElectraModel(config)
classifier_dropout = (
config.classifier_dropout if config.classifier_dropout is not None else config.hidden_dropout_prob
)
self.dropout = nn.Dropout(classifier_dropout)
self.classifier = nn.Linear(config.hidden_size, config.num_labels)
# Initialize weights and apply final processing
self.post_init()
@add_start_docstrings_to_model_forward(ELECTRA_INPUTS_DOCSTRING.format("batch_size, sequence_length"))
@add_code_sample_docstrings(
checkpoint="bhadresh-savani/electra-base-discriminator-finetuned-conll03-english",
output_type=TokenClassifierOutput,
config_class=_CONFIG_FOR_DOC,
expected_output="['B-LOC', 'B-ORG', 'O', 'O', 'O', 'O', 'O', 'B-LOC', 'O', 'B-LOC', 'I-LOC']",
expected_loss=0.11,
)
def forward(
self,
input_ids: Optional[torch.Tensor] = None,
attention_mask: Optional[torch.Tensor] = None,
token_type_ids: Optional[torch.Tensor] = None,
position_ids: Optional[torch.Tensor] = None,
head_mask: Optional[torch.Tensor] = None,
inputs_embeds: Optional[torch.Tensor] = None,
labels: Optional[torch.Tensor] = None,
output_attentions: Optional[bool] = None,
output_hidden_states: Optional[bool] = None,
return_dict: Optional[bool] = None,
) -> Union[Tuple[torch.Tensor], TokenClassifierOutput]:
r"""
labels (`torch.LongTensor` of shape `(batch_size, sequence_length)`, *optional*):
Labels for computing the token classification loss. Indices should be in `[0, ..., config.num_labels - 1]`.
"""
return_dict = return_dict if return_dict is not None else self.config.use_return_dict
discriminator_hidden_states = self.electra(
input_ids,
attention_mask=attention_mask,
token_type_ids=token_type_ids,
position_ids=position_ids,
head_mask=head_mask,
inputs_embeds=inputs_embeds,
output_attentions=output_attentions,
output_hidden_states=output_hidden_states,
return_dict=return_dict,
)
discriminator_sequence_output = discriminator_hidden_states[0]
discriminator_sequence_output = self.dropout(discriminator_sequence_output)
logits = self.classifier(discriminator_sequence_output)
loss = None
if labels is not None:
loss_fct = CrossEntropyLoss()
loss = loss_fct(logits.view(-1, self.num_labels), labels.view(-1))
if not return_dict:
output = (logits,) + discriminator_hidden_states[1:]
return ((loss,) + output) if loss is not None else output
return TokenClassifierOutput(
loss=loss,
logits=logits,
hidden_states=discriminator_hidden_states.hidden_states,
attentions=discriminator_hidden_states.attentions,
)
@add_start_docstrings(
"""
ELECTRA Model with a span classification head on top for extractive question-answering tasks like SQuAD (a linear
layers on top of the hidden-states output to compute `span start logits` and `span end logits`).
""",
ELECTRA_START_DOCSTRING,
)
class ElectraForQuestionAnswering(ElectraPreTrainedModel):
config_class = ElectraConfig
base_model_prefix = "electra"
def __init__(self, config):
super().__init__(config)
self.num_labels = config.num_labels
self.electra = ElectraModel(config)
self.qa_outputs = nn.Linear(config.hidden_size, config.num_labels)
# Initialize weights and apply final processing
self.post_init()
@add_start_docstrings_to_model_forward(ELECTRA_INPUTS_DOCSTRING.format("batch_size, sequence_length"))
@add_code_sample_docstrings(
checkpoint="bhadresh-savani/electra-base-squad2",
output_type=QuestionAnsweringModelOutput,
config_class=_CONFIG_FOR_DOC,
qa_target_start_index=11,
qa_target_end_index=12,
expected_output="'a nice puppet'",
expected_loss=2.64,
)
def forward(
self,
input_ids: Optional[torch.Tensor] = None,
attention_mask: Optional[torch.Tensor] = None,
token_type_ids: Optional[torch.Tensor] = None,
position_ids: Optional[torch.Tensor] = None,
head_mask: Optional[torch.Tensor] = None,
inputs_embeds: Optional[torch.Tensor] = None,
start_positions: Optional[torch.Tensor] = None,
end_positions: Optional[torch.Tensor] = None,
output_attentions: Optional[bool] = None,
output_hidden_states: Optional[bool] = None,
return_dict: Optional[bool] = None,
) -> Union[Tuple[torch.Tensor], QuestionAnsweringModelOutput]:
r"""
start_positions (`torch.LongTensor` of shape `(batch_size,)`, *optional*):
Labels for position (index) of the start of the labelled span for computing the token classification loss.
Positions are clamped to the length of the sequence (`sequence_length`). Position outside of the sequence
are not taken into account for computing the loss.
end_positions (`torch.LongTensor` of shape `(batch_size,)`, *optional*):
Labels for position (index) of the end of the labelled span for computing the token classification loss.
Positions are clamped to the length of the sequence (`sequence_length`). Position outside of the sequence
are not taken into account for computing the loss.
"""
return_dict = return_dict if return_dict is not None else self.config.use_return_dict
discriminator_hidden_states = self.electra(
input_ids,
attention_mask=attention_mask,
token_type_ids=token_type_ids,
position_ids=position_ids,
head_mask=head_mask,
inputs_embeds=inputs_embeds,
output_attentions=output_attentions,
output_hidden_states=output_hidden_states,
)
sequence_output = discriminator_hidden_states[0]
logits = self.qa_outputs(sequence_output)
start_logits, end_logits = logits.split(1, dim=-1)
start_logits = start_logits.squeeze(-1).contiguous()
end_logits = end_logits.squeeze(-1).contiguous()
total_loss = None
if start_positions is not None and end_positions is not None:
# If we are on multi-GPU, split add a dimension
if len(start_positions.size()) > 1:
start_positions = start_positions.squeeze(-1)
if len(end_positions.size()) > 1:
end_positions = end_positions.squeeze(-1)
# sometimes the start/end positions are outside our model inputs, we ignore these terms
ignored_index = start_logits.size(1)
start_positions = start_positions.clamp(0, ignored_index)
end_positions = end_positions.clamp(0, ignored_index)
loss_fct = CrossEntropyLoss(ignore_index=ignored_index)
start_loss = loss_fct(start_logits, start_positions)
end_loss = loss_fct(end_logits, end_positions)
total_loss = (start_loss + end_loss) / 2
if not return_dict:
output = (
start_logits,
end_logits,
) + discriminator_hidden_states[1:]
return ((total_loss,) + output) if total_loss is not None else output
return QuestionAnsweringModelOutput(
loss=total_loss,
start_logits=start_logits,
end_logits=end_logits,
hidden_states=discriminator_hidden_states.hidden_states,
attentions=discriminator_hidden_states.attentions,
)
@add_start_docstrings(
"""
ELECTRA Model with a multiple choice classification head on top (a linear layer on top of the pooled output and a
softmax) e.g. for RocStories/SWAG tasks.
""",
ELECTRA_START_DOCSTRING,
)
class ElectraForMultipleChoice(ElectraPreTrainedModel):
def __init__(self, config):
super().__init__(config)
self.electra = ElectraModel(config)
self.sequence_summary = SequenceSummary(config)
self.classifier = nn.Linear(config.hidden_size, 1)
# Initialize weights and apply final processing
self.post_init()
@add_start_docstrings_to_model_forward(ELECTRA_INPUTS_DOCSTRING.format("batch_size, num_choices, sequence_length"))
@add_code_sample_docstrings(
checkpoint=_CHECKPOINT_FOR_DOC,
output_type=MultipleChoiceModelOutput,
config_class=_CONFIG_FOR_DOC,
)
def forward(
self,
input_ids: Optional[torch.Tensor] = None,
attention_mask: Optional[torch.Tensor] = None,
token_type_ids: Optional[torch.Tensor] = None,
position_ids: Optional[torch.Tensor] = None,
head_mask: Optional[torch.Tensor] = None,
inputs_embeds: Optional[torch.Tensor] = None,
labels: Optional[torch.Tensor] = None,
output_attentions: Optional[bool] = None,
output_hidden_states: Optional[bool] = None,
return_dict: Optional[bool] = None,
) -> Union[Tuple[torch.Tensor], MultipleChoiceModelOutput]:
r"""
labels (`torch.LongTensor` of shape `(batch_size,)`, *optional*):
Labels for computing the multiple choice classification loss. Indices should be in `[0, ...,
num_choices-1]` where `num_choices` is the size of the second dimension of the input tensors. (See
`input_ids` above)
"""
return_dict = return_dict if return_dict is not None else self.config.use_return_dict
num_choices = input_ids.shape[1] if input_ids is not None else inputs_embeds.shape[1]
input_ids = input_ids.view(-1, input_ids.size(-1)) if input_ids is not None else None
attention_mask = attention_mask.view(-1, attention_mask.size(-1)) if attention_mask is not None else None
token_type_ids = token_type_ids.view(-1, token_type_ids.size(-1)) if token_type_ids is not None else None
position_ids = position_ids.view(-1, position_ids.size(-1)) if position_ids is not None else None
inputs_embeds = (
inputs_embeds.view(-1, inputs_embeds.size(-2), inputs_embeds.size(-1))
if inputs_embeds is not None
else None
)
discriminator_hidden_states = self.electra(
input_ids,
attention_mask=attention_mask,
token_type_ids=token_type_ids,
position_ids=position_ids,
head_mask=head_mask,
inputs_embeds=inputs_embeds,
output_attentions=output_attentions,
output_hidden_states=output_hidden_states,
return_dict=return_dict,
)
sequence_output = discriminator_hidden_states[0]
pooled_output = self.sequence_summary(sequence_output)
logits = self.classifier(pooled_output)
reshaped_logits = logits.view(-1, num_choices)
loss = None
if labels is not None:
loss_fct = CrossEntropyLoss()
loss = loss_fct(reshaped_logits, labels)
if not return_dict:
output = (reshaped_logits,) + discriminator_hidden_states[1:]
return ((loss,) + output) if loss is not None else output
return MultipleChoiceModelOutput(
loss=loss,
logits=reshaped_logits,
hidden_states=discriminator_hidden_states.hidden_states,
attentions=discriminator_hidden_states.attentions,
)
@add_start_docstrings(
"""ELECTRA Model with a `language modeling` head on top for CLM fine-tuning.""", ELECTRA_START_DOCSTRING
)
class ElectraForCausalLM(ElectraPreTrainedModel):
_tied_weights_keys = ["generator_lm_head.weight"]
def __init__(self, config):
super().__init__(config)
if not config.is_decoder:
logger.warning("If you want to use `ElectraForCausalLM` as a standalone, add `is_decoder=True.`")
self.electra = ElectraModel(config)
self.generator_predictions = ElectraGeneratorPredictions(config)
self.generator_lm_head = nn.Linear(config.embedding_size, config.vocab_size)
self.init_weights()
def get_output_embeddings(self):
return self.generator_lm_head
def set_output_embeddings(self, new_embeddings):
self.generator_lm_head = new_embeddings
@add_start_docstrings_to_model_forward(ELECTRA_INPUTS_DOCSTRING.format("batch_size, sequence_length"))
@replace_return_docstrings(output_type=CausalLMOutputWithCrossAttentions, config_class=_CONFIG_FOR_DOC)
def forward(
self,
input_ids: Optional[torch.Tensor] = None,
attention_mask: Optional[torch.Tensor] = None,
token_type_ids: Optional[torch.Tensor] = None,
position_ids: Optional[torch.Tensor] = None,
head_mask: Optional[torch.Tensor] = None,
inputs_embeds: Optional[torch.Tensor] = None,
encoder_hidden_states: Optional[torch.Tensor] = None,
encoder_attention_mask: Optional[torch.Tensor] = None,
labels: Optional[torch.Tensor] = None,
past_key_values: Optional[List[torch.Tensor]] = None,
use_cache: Optional[bool] = None,
output_attentions: Optional[bool] = None,
output_hidden_states: Optional[bool] = None,
return_dict: Optional[bool] = None,
) -> Union[Tuple[torch.Tensor], CausalLMOutputWithCrossAttentions]:
r"""
encoder_hidden_states (`torch.FloatTensor` of shape `(batch_size, sequence_length, hidden_size)`, *optional*):
Sequence of hidden-states at the output of the last layer of the encoder. Used in the cross-attention if
the model is configured as a decoder.
encoder_attention_mask (`torch.FloatTensor` of shape `(batch_size, sequence_length)`, *optional*):
Mask to avoid performing attention on the padding token indices of the encoder input. This mask is used in
the cross-attention if the model is configured as a decoder. Mask values selected in `[0, 1]`:
- 1 for tokens that are **not masked**,
- 0 for tokens that are **masked**.
labels (`torch.LongTensor` of shape `(batch_size, sequence_length)`, *optional*):
Labels for computing the left-to-right language modeling loss (next word prediction). Indices should be in
`[-100, 0, ..., config.vocab_size]` (see `input_ids` docstring) Tokens with indices set to `-100` are
ignored (masked), the loss is only computed for the tokens with labels in `[0, ..., config.vocab_size]`
past_key_values (`tuple(tuple(torch.FloatTensor))` of length `config.n_layers` with each tuple having 4 tensors of shape `(batch_size, num_heads, sequence_length - 1, embed_size_per_head)`):
Contains precomputed key and value hidden states of the attention blocks. Can be used to speed up decoding.
If `past_key_values` are used, the user can optionally input only the last `decoder_input_ids` (those that
don't have their past key value states given to this model) of shape `(batch_size, 1)` instead of all
`decoder_input_ids` of shape `(batch_size, sequence_length)`.
use_cache (`bool`, *optional*):
If set to `True`, `past_key_values` key value states are returned and can be used to speed up decoding (see
`past_key_values`).
Returns:
Example:
```python
>>> from transformers import AutoTokenizer, ElectraForCausalLM, ElectraConfig
>>> import torch
>>> tokenizer = AutoTokenizer.from_pretrained("google/electra-base-generator")
>>> config = ElectraConfig.from_pretrained("google/electra-base-generator")
>>> config.is_decoder = True
>>> model = ElectraForCausalLM.from_pretrained("google/electra-base-generator", config=config)
>>> inputs = tokenizer("Hello, my dog is cute", return_tensors="pt")
>>> outputs = model(**inputs)
>>> prediction_logits = outputs.logits
```"""
return_dict = return_dict if return_dict is not None else self.config.use_return_dict
if labels is not None:
use_cache = False
outputs = self.electra(
input_ids,
attention_mask=attention_mask,
token_type_ids=token_type_ids,
position_ids=position_ids,
head_mask=head_mask,
inputs_embeds=inputs_embeds,
encoder_hidden_states=encoder_hidden_states,
encoder_attention_mask=encoder_attention_mask,
past_key_values=past_key_values,
use_cache=use_cache,
output_attentions=output_attentions,
output_hidden_states=output_hidden_states,
return_dict=return_dict,
)
sequence_output = outputs[0]
prediction_scores = self.generator_lm_head(self.generator_predictions(sequence_output))
lm_loss = None
if labels is not None:
# we are doing next-token prediction; shift prediction scores and input ids by one
shifted_prediction_scores = prediction_scores[:, :-1, :].contiguous()
labels = labels[:, 1:].contiguous()
loss_fct = CrossEntropyLoss()
lm_loss = loss_fct(shifted_prediction_scores.view(-1, self.config.vocab_size), labels.view(-1))
if not return_dict:
output = (prediction_scores,) + outputs[1:]
return ((lm_loss,) + output) if lm_loss is not None else output
return CausalLMOutputWithCrossAttentions(
loss=lm_loss,
logits=prediction_scores,
past_key_values=outputs.past_key_values,
hidden_states=outputs.hidden_states,
attentions=outputs.attentions,
cross_attentions=outputs.cross_attentions,
)
# Copied from transformers.models.roberta.modeling_roberta.RobertaForCausalLM.prepare_inputs_for_generation
def prepare_inputs_for_generation(self, input_ids, past_key_values=None, attention_mask=None, **model_kwargs):
input_shape = input_ids.shape
# if model is used as a decoder in encoder-decoder model, the decoder attention mask is created on the fly
if attention_mask is None:
attention_mask = input_ids.new_ones(input_shape)
# cut decoder_input_ids if past_key_values is used
if past_key_values is not None:
past_length = past_key_values[0][0].shape[2]
# Some generation methods already pass only the last input ID
if input_ids.shape[1] > past_length:
remove_prefix_length = past_length
else:
# Default to old behavior: keep only final ID
remove_prefix_length = input_ids.shape[1] - 1
input_ids = input_ids[:, remove_prefix_length:]
return {"input_ids": input_ids, "attention_mask": attention_mask, "past_key_values": past_key_values}
# Copied from transformers.models.roberta.modeling_roberta.RobertaForCausalLM._reorder_cache
def _reorder_cache(self, past_key_values, beam_idx):
reordered_past = ()
for layer_past in past_key_values:
reordered_past += (
tuple(past_state.index_select(0, beam_idx.to(past_state.device)) for past_state in layer_past),
)
return reordered_past
| transformers/src/transformers/models/electra/modeling_electra.py/0 | {
"file_path": "transformers/src/transformers/models/electra/modeling_electra.py",
"repo_id": "transformers",
"token_count": 32259
} | 327 |
# coding=utf-8
# Copyright 2022 The Google AI Language Team Authors and The HuggingFace Inc. team.
# Copyright (c) 2018, NVIDIA CORPORATION. All rights reserved.
#
# Licensed under the Apache License, Version 2.0 (the "License");
# you may not use this file except in compliance with the License.
# You may obtain a copy of the License at
#
# http://www.apache.org/licenses/LICENSE-2.0
#
# Unless required by applicable law or agreed to in writing, software
# distributed under the License is distributed on an "AS IS" BASIS,
# WITHOUT WARRANTIES OR CONDITIONS OF ANY KIND, either express or implied.
# See the License for the specific language governing permissions and
# limitations under the License.
""" ERNIE model configuration"""
from collections import OrderedDict
from typing import Mapping
from ...configuration_utils import PretrainedConfig
from ...onnx import OnnxConfig
from ...utils import logging
logger = logging.get_logger(__name__)
ERNIE_PRETRAINED_CONFIG_ARCHIVE_MAP = {
"nghuyong/ernie-1.0-base-zh": "https://huggingface.co/nghuyong/ernie-1.0-base-zh/resolve/main/config.json",
"nghuyong/ernie-2.0-base-en": "https://huggingface.co/nghuyong/ernie-2.0-base-en/resolve/main/config.json",
"nghuyong/ernie-2.0-large-en": "https://huggingface.co/nghuyong/ernie-2.0-large-en/resolve/main/config.json",
"nghuyong/ernie-3.0-base-zh": "https://huggingface.co/nghuyong/ernie-3.0-base-zh/resolve/main/config.json",
"nghuyong/ernie-3.0-medium-zh": "https://huggingface.co/nghuyong/ernie-3.0-medium-zh/resolve/main/config.json",
"nghuyong/ernie-3.0-mini-zh": "https://huggingface.co/nghuyong/ernie-3.0-mini-zh/resolve/main/config.json",
"nghuyong/ernie-3.0-micro-zh": "https://huggingface.co/nghuyong/ernie-3.0-micro-zh/resolve/main/config.json",
"nghuyong/ernie-3.0-nano-zh": "https://huggingface.co/nghuyong/ernie-3.0-nano-zh/resolve/main/config.json",
"nghuyong/ernie-gram-zh": "https://huggingface.co/nghuyong/ernie-gram-zh/resolve/main/config.json",
"nghuyong/ernie-health-zh": "https://huggingface.co/nghuyong/ernie-health-zh/resolve/main/config.json",
}
class ErnieConfig(PretrainedConfig):
r"""
This is the configuration class to store the configuration of a [`ErnieModel`] or a [`TFErnieModel`]. It is used to
instantiate a ERNIE model according to the specified arguments, defining the model architecture. Instantiating a
configuration with the defaults will yield a similar configuration to that of the ERNIE
[nghuyong/ernie-3.0-base-zh](https://huggingface.co/nghuyong/ernie-3.0-base-zh) architecture.
Configuration objects inherit from [`PretrainedConfig`] and can be used to control the model outputs. Read the
documentation from [`PretrainedConfig`] for more information.
Args:
vocab_size (`int`, *optional*, defaults to 30522):
Vocabulary size of the ERNIE model. Defines the number of different tokens that can be represented by the
`inputs_ids` passed when calling [`ErnieModel`] or [`TFErnieModel`].
hidden_size (`int`, *optional*, defaults to 768):
Dimensionality of the encoder layers and the pooler layer.
num_hidden_layers (`int`, *optional*, defaults to 12):
Number of hidden layers in the Transformer encoder.
num_attention_heads (`int`, *optional*, defaults to 12):
Number of attention heads for each attention layer in the Transformer encoder.
intermediate_size (`int`, *optional*, defaults to 3072):
Dimensionality of the "intermediate" (often named feed-forward) layer in the Transformer encoder.
hidden_act (`str` or `Callable`, *optional*, defaults to `"gelu"`):
The non-linear activation function (function or string) in the encoder and pooler. If string, `"gelu"`,
`"relu"`, `"silu"` and `"gelu_new"` are supported.
hidden_dropout_prob (`float`, *optional*, defaults to 0.1):
The dropout probability for all fully connected layers in the embeddings, encoder, and pooler.
attention_probs_dropout_prob (`float`, *optional*, defaults to 0.1):
The dropout ratio for the attention probabilities.
max_position_embeddings (`int`, *optional*, defaults to 512):
The maximum sequence length that this model might ever be used with. Typically set this to something large
just in case (e.g., 512 or 1024 or 2048).
type_vocab_size (`int`, *optional*, defaults to 2):
The vocabulary size of the `token_type_ids` passed when calling [`ErnieModel`] or [`TFErnieModel`].
task_type_vocab_size (`int`, *optional*, defaults to 3):
The vocabulary size of the `task_type_ids` for ERNIE2.0/ERNIE3.0 model
use_task_id (`bool`, *optional*, defaults to `False`):
Whether or not the model support `task_type_ids`
initializer_range (`float`, *optional*, defaults to 0.02):
The standard deviation of the truncated_normal_initializer for initializing all weight matrices.
layer_norm_eps (`float`, *optional*, defaults to 1e-12):
The epsilon used by the layer normalization layers.
pad_token_id (`int`, *optional*, defaults to 0):
Padding token id.
position_embedding_type (`str`, *optional*, defaults to `"absolute"`):
Type of position embedding. Choose one of `"absolute"`, `"relative_key"`, `"relative_key_query"`. For
positional embeddings use `"absolute"`. For more information on `"relative_key"`, please refer to
[Self-Attention with Relative Position Representations (Shaw et al.)](https://arxiv.org/abs/1803.02155).
For more information on `"relative_key_query"`, please refer to *Method 4* in [Improve Transformer Models
with Better Relative Position Embeddings (Huang et al.)](https://arxiv.org/abs/2009.13658).
use_cache (`bool`, *optional*, defaults to `True`):
Whether or not the model should return the last key/values attentions (not used by all models). Only
relevant if `config.is_decoder=True`.
classifier_dropout (`float`, *optional*):
The dropout ratio for the classification head.
Examples:
```python
>>> from transformers import ErnieConfig, ErnieModel
>>> # Initializing a ERNIE nghuyong/ernie-3.0-base-zh style configuration
>>> configuration = ErnieConfig()
>>> # Initializing a model (with random weights) from the nghuyong/ernie-3.0-base-zh style configuration
>>> model = ErnieModel(configuration)
>>> # Accessing the model configuration
>>> configuration = model.config
```"""
model_type = "ernie"
def __init__(
self,
vocab_size=30522,
hidden_size=768,
num_hidden_layers=12,
num_attention_heads=12,
intermediate_size=3072,
hidden_act="gelu",
hidden_dropout_prob=0.1,
attention_probs_dropout_prob=0.1,
max_position_embeddings=512,
type_vocab_size=2,
task_type_vocab_size=3,
use_task_id=False,
initializer_range=0.02,
layer_norm_eps=1e-12,
pad_token_id=0,
position_embedding_type="absolute",
use_cache=True,
classifier_dropout=None,
**kwargs,
):
super().__init__(pad_token_id=pad_token_id, **kwargs)
self.vocab_size = vocab_size
self.hidden_size = hidden_size
self.num_hidden_layers = num_hidden_layers
self.num_attention_heads = num_attention_heads
self.hidden_act = hidden_act
self.intermediate_size = intermediate_size
self.hidden_dropout_prob = hidden_dropout_prob
self.attention_probs_dropout_prob = attention_probs_dropout_prob
self.max_position_embeddings = max_position_embeddings
self.type_vocab_size = type_vocab_size
self.task_type_vocab_size = task_type_vocab_size
self.use_task_id = use_task_id
self.initializer_range = initializer_range
self.layer_norm_eps = layer_norm_eps
self.position_embedding_type = position_embedding_type
self.use_cache = use_cache
self.classifier_dropout = classifier_dropout
class ErnieOnnxConfig(OnnxConfig):
@property
def inputs(self) -> Mapping[str, Mapping[int, str]]:
if self.task == "multiple-choice":
dynamic_axis = {0: "batch", 1: "choice", 2: "sequence"}
else:
dynamic_axis = {0: "batch", 1: "sequence"}
return OrderedDict(
[
("input_ids", dynamic_axis),
("attention_mask", dynamic_axis),
("token_type_ids", dynamic_axis),
("task_type_ids", dynamic_axis),
]
)
| transformers/src/transformers/models/ernie/configuration_ernie.py/0 | {
"file_path": "transformers/src/transformers/models/ernie/configuration_ernie.py",
"repo_id": "transformers",
"token_count": 3503
} | 328 |
# Copyright 2021 AlQuraishi Laboratory
# Copyright 2021 DeepMind Technologies Limited
#
# Licensed under the Apache License, Version 2.0 (the "License");
# you may not use this file except in compliance with the License.
# You may obtain a copy of the License at
#
# http://www.apache.org/licenses/LICENSE-2.0
#
# Unless required by applicable law or agreed to in writing, software
# distributed under the License is distributed on an "AS IS" BASIS,
# WITHOUT WARRANTIES OR CONDITIONS OF ANY KIND, either express or implied.
# See the License for the specific language governing permissions and
# limitations under the License.
from typing import Dict, Optional, Tuple
import torch
def _calculate_bin_centers(boundaries: torch.Tensor) -> torch.Tensor:
step = boundaries[1] - boundaries[0]
bin_centers = boundaries + step / 2
bin_centers = torch.cat([bin_centers, (bin_centers[-1] + step).unsqueeze(-1)], dim=0)
return bin_centers
def _calculate_expected_aligned_error(
alignment_confidence_breaks: torch.Tensor,
aligned_distance_error_probs: torch.Tensor,
) -> Tuple[torch.Tensor, torch.Tensor]:
bin_centers = _calculate_bin_centers(alignment_confidence_breaks)
return (
torch.sum(aligned_distance_error_probs * bin_centers, dim=-1),
bin_centers[-1],
)
def compute_predicted_aligned_error(
logits: torch.Tensor,
max_bin: int = 31,
no_bins: int = 64,
**kwargs,
) -> Dict[str, torch.Tensor]:
"""Computes aligned confidence metrics from logits.
Args:
logits: [*, num_res, num_res, num_bins] the logits output from
PredictedAlignedErrorHead.
max_bin: Maximum bin value
no_bins: Number of bins
Returns:
aligned_confidence_probs: [*, num_res, num_res, num_bins] the predicted
aligned error probabilities over bins for each residue pair.
predicted_aligned_error: [*, num_res, num_res] the expected aligned distance
error for each pair of residues.
max_predicted_aligned_error: [*] the maximum predicted error possible.
"""
boundaries = torch.linspace(0, max_bin, steps=(no_bins - 1), device=logits.device)
aligned_confidence_probs = torch.nn.functional.softmax(logits, dim=-1)
predicted_aligned_error, max_predicted_aligned_error = _calculate_expected_aligned_error(
alignment_confidence_breaks=boundaries,
aligned_distance_error_probs=aligned_confidence_probs,
)
return {
"aligned_confidence_probs": aligned_confidence_probs,
"predicted_aligned_error": predicted_aligned_error,
"max_predicted_aligned_error": max_predicted_aligned_error,
}
def compute_tm(
logits: torch.Tensor,
residue_weights: Optional[torch.Tensor] = None,
max_bin: int = 31,
no_bins: int = 64,
eps: float = 1e-8,
**kwargs,
) -> torch.Tensor:
if residue_weights is None:
residue_weights = logits.new_ones(logits.shape[-2])
boundaries = torch.linspace(0, max_bin, steps=(no_bins - 1), device=logits.device)
bin_centers = _calculate_bin_centers(boundaries)
torch.sum(residue_weights)
n = logits.shape[-2]
clipped_n = max(n, 19)
d0 = 1.24 * (clipped_n - 15) ** (1.0 / 3) - 1.8
probs = torch.nn.functional.softmax(logits, dim=-1)
tm_per_bin = 1.0 / (1 + (bin_centers**2) / (d0**2))
predicted_tm_term = torch.sum(probs * tm_per_bin, dim=-1)
normed_residue_mask = residue_weights / (eps + residue_weights.sum())
per_alignment = torch.sum(predicted_tm_term * normed_residue_mask, dim=-1)
weighted = per_alignment * residue_weights
argmax = (weighted == torch.max(weighted)).nonzero()[0]
return per_alignment[tuple(argmax)]
| transformers/src/transformers/models/esm/openfold_utils/loss.py/0 | {
"file_path": "transformers/src/transformers/models/esm/openfold_utils/loss.py",
"repo_id": "transformers",
"token_count": 1389
} | 329 |
# coding=utf-8
# Copyright 2023 The HuggingFace Team and The HuggingFace Inc. team. All rights reserved.
#
# Licensed under the Apache License, Version 2.0 (the "License");
# you may not use this file except in compliance with the License.
# You may obtain a copy of the License at
#
# http://www.apache.org/licenses/LICENSE-2.0
#
# Unless required by applicable law or agreed to in writing, software
# distributed under the License is distributed on an "AS IS" BASIS,
# WITHOUT WARRANTIES OR CONDITIONS OF ANY KIND, either express or implied.
# See the License for the specific language governing permissions and
# limitations under the License.
"""Tokenization classes for FastSpeech2Conformer."""
import json
import os
from typing import Optional, Tuple
import regex
from ...tokenization_utils import PreTrainedTokenizer
from ...utils import logging, requires_backends
logger = logging.get_logger(__name__)
VOCAB_FILES_NAMES = {"vocab_file": "vocab.json"}
PRETRAINED_VOCAB_FILES_MAP = {
"vocab_file": {
"espnet/fastspeech2_conformer": "https://huggingface.co/espnet/fastspeech2_conformer/raw/main/vocab.json",
},
}
PRETRAINED_POSITIONAL_EMBEDDINGS_SIZES = {
# Set to somewhat arbitrary large number as the model input
# isn't constrained by the relative positional encoding
"espnet/fastspeech2_conformer": 4096,
}
class FastSpeech2ConformerTokenizer(PreTrainedTokenizer):
"""
Construct a FastSpeech2Conformer tokenizer.
Args:
vocab_file (`str`):
Path to the vocabulary file.
bos_token (`str`, *optional*, defaults to `"<sos/eos>"`):
The begin of sequence token. Note that for FastSpeech2, it is the same as the `eos_token`.
eos_token (`str`, *optional*, defaults to `"<sos/eos>"`):
The end of sequence token. Note that for FastSpeech2, it is the same as the `bos_token`.
pad_token (`str`, *optional*, defaults to `"<blank>"`):
The token used for padding, for example when batching sequences of different lengths.
unk_token (`str`, *optional*, defaults to `"<unk>"`):
The unknown token. A token that is not in the vocabulary cannot be converted to an ID and is set to be this
token instead.
should_strip_spaces (`bool`, *optional*, defaults to `False`):
Whether or not to strip the spaces from the list of tokens.
"""
vocab_files_names = VOCAB_FILES_NAMES
pretrained_vocab_files_map = PRETRAINED_VOCAB_FILES_MAP
model_input_names = ["input_ids", "attention_mask"]
max_model_input_sizes = PRETRAINED_POSITIONAL_EMBEDDINGS_SIZES
def __init__(
self,
vocab_file,
bos_token="<sos/eos>",
eos_token="<sos/eos>",
pad_token="<blank>",
unk_token="<unk>",
should_strip_spaces=False,
**kwargs,
):
requires_backends(self, "g2p_en")
with open(vocab_file, encoding="utf-8") as vocab_handle:
self.encoder = json.load(vocab_handle)
import g2p_en
self.g2p = g2p_en.G2p()
self.decoder = {v: k for k, v in self.encoder.items()}
super().__init__(
bos_token=bos_token,
eos_token=eos_token,
unk_token=unk_token,
pad_token=pad_token,
should_strip_spaces=should_strip_spaces,
**kwargs,
)
self.should_strip_spaces = should_strip_spaces
@property
def vocab_size(self):
return len(self.decoder)
def get_vocab(self):
"Returns vocab as a dict"
return dict(self.encoder, **self.added_tokens_encoder)
def prepare_for_tokenization(self, text, is_split_into_words=False, **kwargs):
# expand symbols
text = regex.sub(";", ",", text)
text = regex.sub(":", ",", text)
text = regex.sub("-", " ", text)
text = regex.sub("&", "and", text)
# strip unnecessary symbols
text = regex.sub(r"[\(\)\[\]\<\>\"]+", "", text)
# strip whitespaces
text = regex.sub(r"\s+", " ", text)
text = text.upper()
return text, kwargs
def _tokenize(self, text):
"""Returns a tokenized string."""
# phonemize
tokens = self.g2p(text)
if self.should_strip_spaces:
tokens = list(filter(lambda s: s != " ", tokens))
tokens.append(self.eos_token)
return tokens
def _convert_token_to_id(self, token):
"""Converts a token (str) in an id using the vocab."""
return self.encoder.get(token, self.encoder.get(self.unk_token))
def _convert_id_to_token(self, index):
"""Converts an index (integer) in a token (str) using the vocab."""
return self.decoder.get(index, self.unk_token)
# Override since phonemes cannot be converted back to strings
def decode(self, token_ids, **kwargs):
logger.warn(
"Phonemes cannot be reliably converted to a string due to the one-many mapping, converting to tokens instead."
)
return self.convert_ids_to_tokens(token_ids)
# Override since phonemes cannot be converted back to strings
def convert_tokens_to_string(self, tokens, **kwargs):
logger.warn(
"Phonemes cannot be reliably converted to a string due to the one-many mapping, returning the tokens."
)
return tokens
def save_vocabulary(self, save_directory: str, filename_prefix: Optional[str] = None) -> Tuple[str]:
"""
Save the vocabulary and special tokens file to a directory.
Args:
save_directory (`str`):
The directory in which to save the vocabulary.
Returns:
`Tuple(str)`: Paths to the files saved.
"""
if not os.path.isdir(save_directory):
logger.error(f"Vocabulary path ({save_directory}) should be a directory")
return
vocab_file = os.path.join(
save_directory, (filename_prefix + "-" if filename_prefix else "") + VOCAB_FILES_NAMES["vocab_file"]
)
with open(vocab_file, "w", encoding="utf-8") as f:
f.write(json.dumps(self.get_vocab(), ensure_ascii=False))
return (vocab_file,)
def __getstate__(self):
state = self.__dict__.copy()
state["g2p"] = None
return state
def __setstate__(self, d):
self.__dict__ = d
try:
import g2p_en
self.g2p = g2p_en.G2p()
except ImportError:
raise ImportError(
"You need to install g2p-en to use FastSpeech2ConformerTokenizer. "
"See https://pypi.org/project/g2p-en/ for installation."
)
| transformers/src/transformers/models/fastspeech2_conformer/tokenization_fastspeech2_conformer.py/0 | {
"file_path": "transformers/src/transformers/models/fastspeech2_conformer/tokenization_fastspeech2_conformer.py",
"repo_id": "transformers",
"token_count": 2855
} | 330 |
# coding=utf-8
# Copyright 2021 The HuggingFace Inc. team.
#
# Licensed under the Apache License, Version 2.0 (the "License");
# you may not use this file except in compliance with the License.
# You may obtain a copy of the License at
#
# http://www.apache.org/licenses/LICENSE-2.0
#
# Unless required by applicable law or agreed to in writing, software
# distributed under the License is distributed on an "AS IS" BASIS,
# WITHOUT WARRANTIES OR CONDITIONS OF ANY KIND, either express or implied.
# See the License for the specific language governing permissions and
# limitations under the License.
"""Convert FNet checkpoint."""
import argparse
import torch
from flax.training.checkpoints import restore_checkpoint
from transformers import FNetConfig, FNetForPreTraining
from transformers.utils import logging
logging.set_verbosity_info()
def convert_flax_checkpoint_to_pytorch(flax_checkpoint_path, fnet_config_file, save_path):
# Initialise PyTorch model
config = FNetConfig.from_json_file(fnet_config_file)
print(f"Building PyTorch model from configuration: {config}")
fnet_pretraining_model = FNetForPreTraining(config)
checkpoint_dict = restore_checkpoint(flax_checkpoint_path, None)
pretrained_model_params = checkpoint_dict["target"]
# Embeddings
# Position IDs
state_dict = fnet_pretraining_model.state_dict()
position_ids = state_dict["fnet.embeddings.position_ids"]
new_state_dict = {"fnet.embeddings.position_ids": position_ids}
# Embedding Layers
new_state_dict["fnet.embeddings.word_embeddings.weight"] = torch.tensor(
pretrained_model_params["encoder"]["embedder"]["word"]["embedding"]
)
new_state_dict["fnet.embeddings.position_embeddings.weight"] = torch.tensor(
pretrained_model_params["encoder"]["embedder"]["position"]["embedding"][0]
)
new_state_dict["fnet.embeddings.token_type_embeddings.weight"] = torch.tensor(
pretrained_model_params["encoder"]["embedder"]["type"]["embedding"]
)
new_state_dict["fnet.embeddings.projection.weight"] = torch.tensor(
pretrained_model_params["encoder"]["embedder"]["hidden_mapping_in"]["kernel"]
).T
new_state_dict["fnet.embeddings.projection.bias"] = torch.tensor(
pretrained_model_params["encoder"]["embedder"]["hidden_mapping_in"]["bias"]
)
new_state_dict["fnet.embeddings.LayerNorm.weight"] = torch.tensor(
pretrained_model_params["encoder"]["embedder"]["layer_norm"]["scale"]
)
new_state_dict["fnet.embeddings.LayerNorm.bias"] = torch.tensor(
pretrained_model_params["encoder"]["embedder"]["layer_norm"]["bias"]
)
# Encoder Layers
for layer in range(config.num_hidden_layers):
new_state_dict[f"fnet.encoder.layer.{layer}.fourier.output.LayerNorm.weight"] = torch.tensor(
pretrained_model_params["encoder"][f"encoder_{layer}"]["mixing_layer_norm"]["scale"]
)
new_state_dict[f"fnet.encoder.layer.{layer}.fourier.output.LayerNorm.bias"] = torch.tensor(
pretrained_model_params["encoder"][f"encoder_{layer}"]["mixing_layer_norm"]["bias"]
)
new_state_dict[f"fnet.encoder.layer.{layer}.intermediate.dense.weight"] = torch.tensor(
pretrained_model_params["encoder"][f"feed_forward_{layer}"]["intermediate"]["kernel"]
).T
new_state_dict[f"fnet.encoder.layer.{layer}.intermediate.dense.bias"] = torch.tensor(
pretrained_model_params["encoder"][f"feed_forward_{layer}"]["intermediate"]["bias"]
)
new_state_dict[f"fnet.encoder.layer.{layer}.output.dense.weight"] = torch.tensor(
pretrained_model_params["encoder"][f"feed_forward_{layer}"]["output"]["kernel"]
).T
new_state_dict[f"fnet.encoder.layer.{layer}.output.dense.bias"] = torch.tensor(
pretrained_model_params["encoder"][f"feed_forward_{layer}"]["output"]["bias"]
)
new_state_dict[f"fnet.encoder.layer.{layer}.output.LayerNorm.weight"] = torch.tensor(
pretrained_model_params["encoder"][f"encoder_{layer}"]["output_layer_norm"]["scale"]
)
new_state_dict[f"fnet.encoder.layer.{layer}.output.LayerNorm.bias"] = torch.tensor(
pretrained_model_params["encoder"][f"encoder_{layer}"]["output_layer_norm"]["bias"]
)
# Pooler Layers
new_state_dict["fnet.pooler.dense.weight"] = torch.tensor(pretrained_model_params["encoder"]["pooler"]["kernel"]).T
new_state_dict["fnet.pooler.dense.bias"] = torch.tensor(pretrained_model_params["encoder"]["pooler"]["bias"])
# Masked LM Layers
new_state_dict["cls.predictions.transform.dense.weight"] = torch.tensor(
pretrained_model_params["predictions_dense"]["kernel"]
).T
new_state_dict["cls.predictions.transform.dense.bias"] = torch.tensor(
pretrained_model_params["predictions_dense"]["bias"]
)
new_state_dict["cls.predictions.transform.LayerNorm.weight"] = torch.tensor(
pretrained_model_params["predictions_layer_norm"]["scale"]
)
new_state_dict["cls.predictions.transform.LayerNorm.bias"] = torch.tensor(
pretrained_model_params["predictions_layer_norm"]["bias"]
)
new_state_dict["cls.predictions.decoder.weight"] = torch.tensor(
pretrained_model_params["encoder"]["embedder"]["word"]["embedding"]
)
new_state_dict["cls.predictions.decoder.bias"] = torch.tensor(
pretrained_model_params["predictions_output"]["output_bias"]
)
new_state_dict["cls.predictions.bias"] = torch.tensor(pretrained_model_params["predictions_output"]["output_bias"])
# Seq Relationship Layers
new_state_dict["cls.seq_relationship.weight"] = torch.tensor(
pretrained_model_params["classification"]["output_kernel"]
)
new_state_dict["cls.seq_relationship.bias"] = torch.tensor(
pretrained_model_params["classification"]["output_bias"]
)
# Load State Dict
fnet_pretraining_model.load_state_dict(new_state_dict)
# Save PreTrained
print(f"Saving pretrained model to {save_path}")
fnet_pretraining_model.save_pretrained(save_path)
if __name__ == "__main__":
parser = argparse.ArgumentParser()
# Required parameters
parser.add_argument(
"--flax_checkpoint_path", default=None, type=str, required=True, help="Path to the TensorFlow checkpoint path."
)
parser.add_argument(
"--fnet_config_file",
default=None,
type=str,
required=True,
help=(
"The config json file corresponding to the pre-trained FNet model. \n"
"This specifies the model architecture."
),
)
parser.add_argument("--save_path", default=None, type=str, required=True, help="Path to the output model.")
args = parser.parse_args()
convert_flax_checkpoint_to_pytorch(args.flax_checkpoint_path, args.fnet_config_file, args.save_path)
| transformers/src/transformers/models/fnet/convert_fnet_original_flax_checkpoint_to_pytorch.py/0 | {
"file_path": "transformers/src/transformers/models/fnet/convert_fnet_original_flax_checkpoint_to_pytorch.py",
"repo_id": "transformers",
"token_count": 2770
} | 331 |
# coding=utf-8
# Copyright 2020-present Google Brain and Carnegie Mellon University Authors and the HuggingFace Inc. team.
#
# Licensed under the Apache License, Version 2.0 (the "License");
# you may not use this file except in compliance with the License.
# You may obtain a copy of the License at
#
# http://www.apache.org/licenses/LICENSE-2.0
#
# Unless required by applicable law or agreed to in writing, software
# distributed under the License is distributed on an "AS IS" BASIS,
# WITHOUT WARRANTIES OR CONDITIONS OF ANY KIND, either express or implied.
# See the License for the specific language governing permissions and
# limitations under the License.
""" PyTorch Funnel Transformer model."""
import os
from dataclasses import dataclass
from typing import List, Optional, Tuple, Union
import numpy as np
import torch
from torch import nn
from torch.nn import BCEWithLogitsLoss, CrossEntropyLoss, MSELoss
from ...activations import ACT2FN
from ...modeling_outputs import (
BaseModelOutput,
MaskedLMOutput,
MultipleChoiceModelOutput,
QuestionAnsweringModelOutput,
SequenceClassifierOutput,
TokenClassifierOutput,
)
from ...modeling_utils import PreTrainedModel
from ...utils import (
ModelOutput,
add_code_sample_docstrings,
add_start_docstrings,
add_start_docstrings_to_model_forward,
logging,
replace_return_docstrings,
)
from .configuration_funnel import FunnelConfig
logger = logging.get_logger(__name__)
_CONFIG_FOR_DOC = "FunnelConfig"
_CHECKPOINT_FOR_DOC = "funnel-transformer/small"
FUNNEL_PRETRAINED_MODEL_ARCHIVE_LIST = [
"funnel-transformer/small", # B4-4-4H768
"funnel-transformer/small-base", # B4-4-4H768, no decoder
"funnel-transformer/medium", # B6-3x2-3x2H768
"funnel-transformer/medium-base", # B6-3x2-3x2H768, no decoder
"funnel-transformer/intermediate", # B6-6-6H768
"funnel-transformer/intermediate-base", # B6-6-6H768, no decoder
"funnel-transformer/large", # B8-8-8H1024
"funnel-transformer/large-base", # B8-8-8H1024, no decoder
"funnel-transformer/xlarge-base", # B10-10-10H1024
"funnel-transformer/xlarge", # B10-10-10H1024, no decoder
]
INF = 1e6
def load_tf_weights_in_funnel(model, config, tf_checkpoint_path):
"""Load tf checkpoints in a pytorch model."""
try:
import re
import numpy as np
import tensorflow as tf
except ImportError:
logger.error(
"Loading a TensorFlow model in PyTorch, requires TensorFlow to be installed. Please see "
"https://www.tensorflow.org/install/ for installation instructions."
)
raise
tf_path = os.path.abspath(tf_checkpoint_path)
logger.info(f"Converting TensorFlow checkpoint from {tf_path}")
# Load weights from TF model
init_vars = tf.train.list_variables(tf_path)
names = []
arrays = []
for name, shape in init_vars:
logger.info(f"Loading TF weight {name} with shape {shape}")
array = tf.train.load_variable(tf_path, name)
names.append(name)
arrays.append(array)
_layer_map = {
"k": "k_head",
"q": "q_head",
"v": "v_head",
"o": "post_proj",
"layer_1": "linear_1",
"layer_2": "linear_2",
"rel_attn": "attention",
"ff": "ffn",
"kernel": "weight",
"gamma": "weight",
"beta": "bias",
"lookup_table": "weight",
"word_embedding": "word_embeddings",
"input": "embeddings",
}
for name, array in zip(names, arrays):
name = name.split("/")
# adam_v and adam_m are variables used in AdamWeightDecayOptimizer to calculated m and v
# which are not required for using pretrained model
if any(
n in ["adam_v", "adam_m", "AdamWeightDecayOptimizer", "AdamWeightDecayOptimizer_1", "global_step"]
for n in name
):
logger.info(f"Skipping {'/'.join(name)}")
continue
if name[0] == "generator":
continue
pointer = model
skipped = False
for m_name in name[1:]:
if not isinstance(pointer, FunnelPositionwiseFFN) and re.fullmatch(r"layer_\d+", m_name):
layer_index = int(re.search(r"layer_(\d+)", m_name).groups()[0])
if layer_index < config.num_hidden_layers:
block_idx = 0
while layer_index >= config.block_sizes[block_idx]:
layer_index -= config.block_sizes[block_idx]
block_idx += 1
pointer = pointer.blocks[block_idx][layer_index]
else:
layer_index -= config.num_hidden_layers
pointer = pointer.layers[layer_index]
elif m_name == "r" and isinstance(pointer, FunnelRelMultiheadAttention):
pointer = pointer.r_kernel
break
elif m_name in _layer_map:
pointer = getattr(pointer, _layer_map[m_name])
else:
try:
pointer = getattr(pointer, m_name)
except AttributeError:
print(f"Skipping {'/'.join(name)}", array.shape)
skipped = True
break
if not skipped:
if len(pointer.shape) != len(array.shape):
array = array.reshape(pointer.shape)
if m_name == "kernel":
array = np.transpose(array)
pointer.data = torch.from_numpy(array)
return model
class FunnelEmbeddings(nn.Module):
def __init__(self, config: FunnelConfig) -> None:
super().__init__()
self.word_embeddings = nn.Embedding(config.vocab_size, config.hidden_size, padding_idx=config.pad_token_id)
self.layer_norm = nn.LayerNorm(config.d_model, eps=config.layer_norm_eps)
self.dropout = nn.Dropout(config.hidden_dropout)
def forward(
self, input_ids: Optional[torch.Tensor] = None, inputs_embeds: Optional[torch.Tensor] = None
) -> torch.Tensor:
if inputs_embeds is None:
inputs_embeds = self.word_embeddings(input_ids)
embeddings = self.layer_norm(inputs_embeds)
embeddings = self.dropout(embeddings)
return embeddings
class FunnelAttentionStructure(nn.Module):
"""
Contains helpers for `FunnelRelMultiheadAttention `.
"""
cls_token_type_id: int = 2
def __init__(self, config: FunnelConfig) -> None:
super().__init__()
self.config = config
self.sin_dropout = nn.Dropout(config.hidden_dropout)
self.cos_dropout = nn.Dropout(config.hidden_dropout)
# Track where we are at in terms of pooling from the original input, e.g., by how much the sequence length was
# divided.
self.pooling_mult = None
def init_attention_inputs(
self,
inputs_embeds: torch.Tensor,
attention_mask: Optional[torch.Tensor] = None,
token_type_ids: Optional[torch.Tensor] = None,
) -> Tuple[torch.Tensor]:
"""Returns the attention inputs associated to the inputs of the model."""
# inputs_embeds has shape batch_size x seq_len x d_model
# attention_mask and token_type_ids have shape batch_size x seq_len
self.pooling_mult = 1
self.seq_len = seq_len = inputs_embeds.size(1)
position_embeds = self.get_position_embeds(seq_len, inputs_embeds.dtype, inputs_embeds.device)
token_type_mat = self.token_type_ids_to_mat(token_type_ids) if token_type_ids is not None else None
cls_mask = (
nn.functional.pad(inputs_embeds.new_ones([seq_len - 1, seq_len - 1]), (1, 0, 1, 0))
if self.config.separate_cls
else None
)
return (position_embeds, token_type_mat, attention_mask, cls_mask)
def token_type_ids_to_mat(self, token_type_ids: torch.Tensor) -> torch.Tensor:
"""Convert `token_type_ids` to `token_type_mat`."""
token_type_mat = token_type_ids[:, :, None] == token_type_ids[:, None]
# Treat <cls> as in the same segment as both A & B
cls_ids = token_type_ids == self.cls_token_type_id
cls_mat = cls_ids[:, :, None] | cls_ids[:, None]
return cls_mat | token_type_mat
def get_position_embeds(
self, seq_len: int, dtype: torch.dtype, device: torch.device
) -> Union[Tuple[torch.Tensor], List[List[torch.Tensor]]]:
"""
Create and cache inputs related to relative position encoding. Those are very different depending on whether we
are using the factorized or the relative shift attention:
For the factorized attention, it returns the matrices (phi, pi, psi, omega) used in the paper, appendix A.2.2,
final formula.
For the relative shift attention, it returns all possible vectors R used in the paper, appendix A.2.1, final
formula.
Paper link: https://arxiv.org/abs/2006.03236
"""
d_model = self.config.d_model
if self.config.attention_type == "factorized":
# Notations from the paper, appending A.2.2, final formula.
# We need to create and return the matrices phi, psi, pi and omega.
pos_seq = torch.arange(0, seq_len, 1.0, dtype=torch.int64, device=device).to(dtype)
freq_seq = torch.arange(0, d_model // 2, 1.0, dtype=torch.int64, device=device).to(dtype)
inv_freq = 1 / (10000 ** (freq_seq / (d_model // 2)))
sinusoid = pos_seq[:, None] * inv_freq[None]
sin_embed = torch.sin(sinusoid)
sin_embed_d = self.sin_dropout(sin_embed)
cos_embed = torch.cos(sinusoid)
cos_embed_d = self.cos_dropout(cos_embed)
# This is different from the formula on the paper...
phi = torch.cat([sin_embed_d, sin_embed_d], dim=-1)
psi = torch.cat([cos_embed, sin_embed], dim=-1)
pi = torch.cat([cos_embed_d, cos_embed_d], dim=-1)
omega = torch.cat([-sin_embed, cos_embed], dim=-1)
return (phi, pi, psi, omega)
else:
# Notations from the paper, appending A.2.1, final formula.
# We need to create and return all the possible vectors R for all blocks and shifts.
freq_seq = torch.arange(0, d_model // 2, 1.0, dtype=torch.int64, device=device).to(dtype)
inv_freq = 1 / (10000 ** (freq_seq / (d_model // 2)))
# Maximum relative positions for the first input
rel_pos_id = torch.arange(-seq_len * 2, seq_len * 2, 1.0, dtype=torch.int64, device=device).to(dtype)
zero_offset = seq_len * 2
sinusoid = rel_pos_id[:, None] * inv_freq[None]
sin_embed = self.sin_dropout(torch.sin(sinusoid))
cos_embed = self.cos_dropout(torch.cos(sinusoid))
pos_embed = torch.cat([sin_embed, cos_embed], dim=-1)
pos = torch.arange(0, seq_len, dtype=torch.int64, device=device).to(dtype)
pooled_pos = pos
position_embeds_list = []
for block_index in range(0, self.config.num_blocks):
# For each block with block_index > 0, we need two types position embeddings:
# - Attention(pooled-q, unpooled-kv)
# - Attention(pooled-q, pooled-kv)
# For block_index = 0 we only need the second one and leave the first one as None.
# First type
if block_index == 0:
position_embeds_pooling = None
else:
pooled_pos = self.stride_pool_pos(pos, block_index)
# construct rel_pos_id
stride = 2 ** (block_index - 1)
rel_pos = self.relative_pos(pos, stride, pooled_pos, shift=2)
rel_pos = rel_pos[:, None] + zero_offset
rel_pos = rel_pos.expand(rel_pos.size(0), d_model)
position_embeds_pooling = torch.gather(pos_embed, 0, rel_pos)
# Second type
pos = pooled_pos
stride = 2**block_index
rel_pos = self.relative_pos(pos, stride)
rel_pos = rel_pos[:, None] + zero_offset
rel_pos = rel_pos.expand(rel_pos.size(0), d_model)
position_embeds_no_pooling = torch.gather(pos_embed, 0, rel_pos)
position_embeds_list.append([position_embeds_no_pooling, position_embeds_pooling])
return position_embeds_list
def stride_pool_pos(self, pos_id: torch.Tensor, block_index: int):
"""
Pool `pos_id` while keeping the cls token separate (if `config.separate_cls=True`).
"""
if self.config.separate_cls:
# Under separate <cls>, we treat the <cls> as the first token in
# the previous block of the 1st real block. Since the 1st real
# block always has position 1, the position of the previous block
# will be at `1 - 2 ** block_index`.
cls_pos = pos_id.new_tensor([-(2**block_index) + 1])
pooled_pos_id = pos_id[1:-1] if self.config.truncate_seq else pos_id[1:]
return torch.cat([cls_pos, pooled_pos_id[::2]], 0)
else:
return pos_id[::2]
def relative_pos(self, pos: torch.Tensor, stride: int, pooled_pos=None, shift: int = 1) -> torch.Tensor:
"""
Build the relative positional vector between `pos` and `pooled_pos`.
"""
if pooled_pos is None:
pooled_pos = pos
ref_point = pooled_pos[0] - pos[0]
num_remove = shift * len(pooled_pos)
max_dist = ref_point + num_remove * stride
min_dist = pooled_pos[0] - pos[-1]
return torch.arange(max_dist, min_dist - 1, -stride, dtype=torch.long, device=pos.device)
def stride_pool(
self,
tensor: Union[torch.Tensor, Tuple[torch.Tensor], List[torch.Tensor]],
axis: Union[int, Tuple[int], List[int]],
) -> torch.Tensor:
"""
Perform pooling by stride slicing the tensor along the given axis.
"""
if tensor is None:
return None
# Do the stride pool recursively if axis is a list or a tuple of ints.
if isinstance(axis, (list, tuple)):
for ax in axis:
tensor = self.stride_pool(tensor, ax)
return tensor
# Do the stride pool recursively if tensor is a list or tuple of tensors.
if isinstance(tensor, (tuple, list)):
return type(tensor)(self.stride_pool(x, axis) for x in tensor)
# Deal with negative axis
axis %= tensor.ndim
axis_slice = (
slice(None, -1, 2) if self.config.separate_cls and self.config.truncate_seq else slice(None, None, 2)
)
enc_slice = [slice(None)] * axis + [axis_slice]
if self.config.separate_cls:
cls_slice = [slice(None)] * axis + [slice(None, 1)]
tensor = torch.cat([tensor[cls_slice], tensor], axis=axis)
return tensor[enc_slice]
def pool_tensor(
self, tensor: Union[torch.Tensor, Tuple[torch.Tensor], List[torch.Tensor]], mode: str = "mean", stride: int = 2
) -> torch.Tensor:
"""Apply 1D pooling to a tensor of size [B x T (x H)]."""
if tensor is None:
return None
# Do the pool recursively if tensor is a list or tuple of tensors.
if isinstance(tensor, (tuple, list)):
return type(tensor)(self.pool_tensor(tensor, mode=mode, stride=stride) for x in tensor)
if self.config.separate_cls:
suffix = tensor[:, :-1] if self.config.truncate_seq else tensor
tensor = torch.cat([tensor[:, :1], suffix], dim=1)
ndim = tensor.ndim
if ndim == 2:
tensor = tensor[:, None, :, None]
elif ndim == 3:
tensor = tensor[:, None, :, :]
# Stride is applied on the second-to-last dimension.
stride = (stride, 1)
if mode == "mean":
tensor = nn.functional.avg_pool2d(tensor, stride, stride=stride, ceil_mode=True)
elif mode == "max":
tensor = nn.functional.max_pool2d(tensor, stride, stride=stride, ceil_mode=True)
elif mode == "min":
tensor = -nn.functional.max_pool2d(-tensor, stride, stride=stride, ceil_mode=True)
else:
raise NotImplementedError("The supported modes are 'mean', 'max' and 'min'.")
if ndim == 2:
return tensor[:, 0, :, 0]
elif ndim == 3:
return tensor[:, 0]
return tensor
def pre_attention_pooling(
self, output, attention_inputs: Tuple[torch.Tensor]
) -> Tuple[torch.Tensor, Tuple[torch.Tensor]]:
"""Pool `output` and the proper parts of `attention_inputs` before the attention layer."""
position_embeds, token_type_mat, attention_mask, cls_mask = attention_inputs
if self.config.pool_q_only:
if self.config.attention_type == "factorized":
position_embeds = self.stride_pool(position_embeds[:2], 0) + position_embeds[2:]
token_type_mat = self.stride_pool(token_type_mat, 1)
cls_mask = self.stride_pool(cls_mask, 0)
output = self.pool_tensor(output, mode=self.config.pooling_type)
else:
self.pooling_mult *= 2
if self.config.attention_type == "factorized":
position_embeds = self.stride_pool(position_embeds, 0)
token_type_mat = self.stride_pool(token_type_mat, [1, 2])
cls_mask = self.stride_pool(cls_mask, [1, 2])
attention_mask = self.pool_tensor(attention_mask, mode="min")
output = self.pool_tensor(output, mode=self.config.pooling_type)
attention_inputs = (position_embeds, token_type_mat, attention_mask, cls_mask)
return output, attention_inputs
def post_attention_pooling(self, attention_inputs: Tuple[torch.Tensor]) -> Tuple[torch.Tensor]:
"""Pool the proper parts of `attention_inputs` after the attention layer."""
position_embeds, token_type_mat, attention_mask, cls_mask = attention_inputs
if self.config.pool_q_only:
self.pooling_mult *= 2
if self.config.attention_type == "factorized":
position_embeds = position_embeds[:2] + self.stride_pool(position_embeds[2:], 0)
token_type_mat = self.stride_pool(token_type_mat, 2)
cls_mask = self.stride_pool(cls_mask, 1)
attention_mask = self.pool_tensor(attention_mask, mode="min")
attention_inputs = (position_embeds, token_type_mat, attention_mask, cls_mask)
return attention_inputs
def _relative_shift_gather(positional_attn: torch.Tensor, context_len: int, shift: int) -> torch.Tensor:
batch_size, n_head, seq_len, max_rel_len = positional_attn.shape
# max_rel_len = 2 * context_len + shift -1 is the numbers of possible relative positions i-j
# What's next is the same as doing the following gather, which might be clearer code but less efficient.
# idxs = context_len + torch.arange(0, context_len).unsqueeze(0) - torch.arange(0, seq_len).unsqueeze(1)
# # matrix of context_len + i-j
# return positional_attn.gather(3, idxs.expand([batch_size, n_head, context_len, context_len]))
positional_attn = torch.reshape(positional_attn, [batch_size, n_head, max_rel_len, seq_len])
positional_attn = positional_attn[:, :, shift:, :]
positional_attn = torch.reshape(positional_attn, [batch_size, n_head, seq_len, max_rel_len - shift])
positional_attn = positional_attn[..., :context_len]
return positional_attn
class FunnelRelMultiheadAttention(nn.Module):
def __init__(self, config: FunnelConfig, block_index: int) -> None:
super().__init__()
self.config = config
self.block_index = block_index
d_model, n_head, d_head = config.d_model, config.n_head, config.d_head
self.hidden_dropout = nn.Dropout(config.hidden_dropout)
self.attention_dropout = nn.Dropout(config.attention_dropout)
self.q_head = nn.Linear(d_model, n_head * d_head, bias=False)
self.k_head = nn.Linear(d_model, n_head * d_head)
self.v_head = nn.Linear(d_model, n_head * d_head)
self.r_w_bias = nn.Parameter(torch.zeros([n_head, d_head]))
self.r_r_bias = nn.Parameter(torch.zeros([n_head, d_head]))
self.r_kernel = nn.Parameter(torch.zeros([d_model, n_head, d_head]))
self.r_s_bias = nn.Parameter(torch.zeros([n_head, d_head]))
self.seg_embed = nn.Parameter(torch.zeros([2, n_head, d_head]))
self.post_proj = nn.Linear(n_head * d_head, d_model)
self.layer_norm = nn.LayerNorm(d_model, eps=config.layer_norm_eps)
self.scale = 1.0 / (d_head**0.5)
def relative_positional_attention(self, position_embeds, q_head, context_len, cls_mask=None):
"""Relative attention score for the positional encodings"""
# q_head has shape batch_size x sea_len x n_head x d_head
if self.config.attention_type == "factorized":
# Notations from the paper, appending A.2.2, final formula (https://arxiv.org/abs/2006.03236)
# phi and pi have shape seq_len x d_model, psi and omega have shape context_len x d_model
phi, pi, psi, omega = position_embeds
# Shape n_head x d_head
u = self.r_r_bias * self.scale
# Shape d_model x n_head x d_head
w_r = self.r_kernel
# Shape batch_size x sea_len x n_head x d_model
q_r_attention = torch.einsum("binh,dnh->bind", q_head + u, w_r)
q_r_attention_1 = q_r_attention * phi[:, None]
q_r_attention_2 = q_r_attention * pi[:, None]
# Shape batch_size x n_head x seq_len x context_len
positional_attn = torch.einsum("bind,jd->bnij", q_r_attention_1, psi) + torch.einsum(
"bind,jd->bnij", q_r_attention_2, omega
)
else:
shift = 2 if q_head.shape[1] != context_len else 1
# Notations from the paper, appending A.2.1, final formula (https://arxiv.org/abs/2006.03236)
# Grab the proper positional encoding, shape max_rel_len x d_model
r = position_embeds[self.block_index][shift - 1]
# Shape n_head x d_head
v = self.r_r_bias * self.scale
# Shape d_model x n_head x d_head
w_r = self.r_kernel
# Shape max_rel_len x n_head x d_model
r_head = torch.einsum("td,dnh->tnh", r, w_r)
# Shape batch_size x n_head x seq_len x max_rel_len
positional_attn = torch.einsum("binh,tnh->bnit", q_head + v, r_head)
# Shape batch_size x n_head x seq_len x context_len
positional_attn = _relative_shift_gather(positional_attn, context_len, shift)
if cls_mask is not None:
positional_attn *= cls_mask
return positional_attn
def relative_token_type_attention(self, token_type_mat, q_head, cls_mask=None):
"""Relative attention score for the token_type_ids"""
if token_type_mat is None:
return 0
batch_size, seq_len, context_len = token_type_mat.shape
# q_head has shape batch_size x seq_len x n_head x d_head
# Shape n_head x d_head
r_s_bias = self.r_s_bias * self.scale
# Shape batch_size x n_head x seq_len x 2
token_type_bias = torch.einsum("bind,snd->bnis", q_head + r_s_bias, self.seg_embed)
# Shape batch_size x n_head x seq_len x context_len
token_type_mat = token_type_mat[:, None].expand([batch_size, q_head.shape[2], seq_len, context_len])
# Shapes batch_size x n_head x seq_len
diff_token_type, same_token_type = torch.split(token_type_bias, 1, dim=-1)
# Shape batch_size x n_head x seq_len x context_len
token_type_attn = torch.where(
token_type_mat, same_token_type.expand(token_type_mat.shape), diff_token_type.expand(token_type_mat.shape)
)
if cls_mask is not None:
token_type_attn *= cls_mask
return token_type_attn
def forward(
self,
query: torch.Tensor,
key: torch.Tensor,
value: torch.Tensor,
attention_inputs: Tuple[torch.Tensor],
output_attentions: bool = False,
) -> Tuple[torch.Tensor, ...]:
# query has shape batch_size x seq_len x d_model
# key and value have shapes batch_size x context_len x d_model
position_embeds, token_type_mat, attention_mask, cls_mask = attention_inputs
batch_size, seq_len, _ = query.shape
context_len = key.shape[1]
n_head, d_head = self.config.n_head, self.config.d_head
# Shape batch_size x seq_len x n_head x d_head
q_head = self.q_head(query).view(batch_size, seq_len, n_head, d_head)
# Shapes batch_size x context_len x n_head x d_head
k_head = self.k_head(key).view(batch_size, context_len, n_head, d_head)
v_head = self.v_head(value).view(batch_size, context_len, n_head, d_head)
q_head = q_head * self.scale
# Shape n_head x d_head
r_w_bias = self.r_w_bias * self.scale
# Shapes batch_size x n_head x seq_len x context_len
content_score = torch.einsum("bind,bjnd->bnij", q_head + r_w_bias, k_head)
positional_attn = self.relative_positional_attention(position_embeds, q_head, context_len, cls_mask)
token_type_attn = self.relative_token_type_attention(token_type_mat, q_head, cls_mask)
# merge attention scores
attn_score = content_score + positional_attn + token_type_attn
# precision safe in case of mixed precision training
dtype = attn_score.dtype
attn_score = attn_score.float()
# perform masking
if attention_mask is not None:
attn_score = attn_score - INF * (1 - attention_mask[:, None, None].float())
# attention probability
attn_prob = torch.softmax(attn_score, dim=-1, dtype=dtype)
attn_prob = self.attention_dropout(attn_prob)
# attention output, shape batch_size x seq_len x n_head x d_head
attn_vec = torch.einsum("bnij,bjnd->bind", attn_prob, v_head)
# Shape shape batch_size x seq_len x d_model
attn_out = self.post_proj(attn_vec.reshape(batch_size, seq_len, n_head * d_head))
attn_out = self.hidden_dropout(attn_out)
output = self.layer_norm(query + attn_out)
return (output, attn_prob) if output_attentions else (output,)
class FunnelPositionwiseFFN(nn.Module):
def __init__(self, config: FunnelConfig) -> None:
super().__init__()
self.linear_1 = nn.Linear(config.d_model, config.d_inner)
self.activation_function = ACT2FN[config.hidden_act]
self.activation_dropout = nn.Dropout(config.activation_dropout)
self.linear_2 = nn.Linear(config.d_inner, config.d_model)
self.dropout = nn.Dropout(config.hidden_dropout)
self.layer_norm = nn.LayerNorm(config.d_model, config.layer_norm_eps)
def forward(self, hidden: torch.Tensor) -> torch.Tensor:
h = self.linear_1(hidden)
h = self.activation_function(h)
h = self.activation_dropout(h)
h = self.linear_2(h)
h = self.dropout(h)
return self.layer_norm(hidden + h)
class FunnelLayer(nn.Module):
def __init__(self, config: FunnelConfig, block_index: int) -> None:
super().__init__()
self.attention = FunnelRelMultiheadAttention(config, block_index)
self.ffn = FunnelPositionwiseFFN(config)
def forward(
self,
query: torch.Tensor,
key: torch.Tensor,
value: torch.Tensor,
attention_inputs,
output_attentions: bool = False,
) -> Tuple:
attn = self.attention(query, key, value, attention_inputs, output_attentions=output_attentions)
output = self.ffn(attn[0])
return (output, attn[1]) if output_attentions else (output,)
class FunnelEncoder(nn.Module):
def __init__(self, config: FunnelConfig) -> None:
super().__init__()
self.config = config
self.attention_structure = FunnelAttentionStructure(config)
self.blocks = nn.ModuleList(
[
nn.ModuleList([FunnelLayer(config, block_index) for _ in range(block_size)])
for block_index, block_size in enumerate(config.block_sizes)
]
)
def forward(
self,
inputs_embeds: torch.Tensor,
attention_mask: Optional[torch.Tensor] = None,
token_type_ids: Optional[torch.Tensor] = None,
output_attentions: bool = False,
output_hidden_states: bool = False,
return_dict: bool = True,
) -> Union[Tuple, BaseModelOutput]:
# The pooling is not implemented on long tensors, so we convert this mask.
attention_mask = attention_mask.type_as(inputs_embeds)
attention_inputs = self.attention_structure.init_attention_inputs(
inputs_embeds,
attention_mask=attention_mask,
token_type_ids=token_type_ids,
)
hidden = inputs_embeds
all_hidden_states = (inputs_embeds,) if output_hidden_states else None
all_attentions = () if output_attentions else None
for block_index, block in enumerate(self.blocks):
pooling_flag = hidden.size(1) > (2 if self.config.separate_cls else 1)
pooling_flag = pooling_flag and block_index > 0
if pooling_flag:
pooled_hidden, attention_inputs = self.attention_structure.pre_attention_pooling(
hidden, attention_inputs
)
for layer_index, layer in enumerate(block):
for repeat_index in range(self.config.block_repeats[block_index]):
do_pooling = (repeat_index == 0) and (layer_index == 0) and pooling_flag
if do_pooling:
query = pooled_hidden
key = value = hidden if self.config.pool_q_only else pooled_hidden
else:
query = key = value = hidden
layer_output = layer(query, key, value, attention_inputs, output_attentions=output_attentions)
hidden = layer_output[0]
if do_pooling:
attention_inputs = self.attention_structure.post_attention_pooling(attention_inputs)
if output_attentions:
all_attentions = all_attentions + layer_output[1:]
if output_hidden_states:
all_hidden_states = all_hidden_states + (hidden,)
if not return_dict:
return tuple(v for v in [hidden, all_hidden_states, all_attentions] if v is not None)
return BaseModelOutput(last_hidden_state=hidden, hidden_states=all_hidden_states, attentions=all_attentions)
def upsample(
x: torch.Tensor, stride: int, target_len: int, separate_cls: bool = True, truncate_seq: bool = False
) -> torch.Tensor:
"""
Upsample tensor `x` to match `target_len` by repeating the tokens `stride` time on the sequence length dimension.
"""
if stride == 1:
return x
if separate_cls:
cls = x[:, :1]
x = x[:, 1:]
output = torch.repeat_interleave(x, repeats=stride, dim=1)
if separate_cls:
if truncate_seq:
output = nn.functional.pad(output, (0, 0, 0, stride - 1, 0, 0))
output = output[:, : target_len - 1]
output = torch.cat([cls, output], dim=1)
else:
output = output[:, :target_len]
return output
class FunnelDecoder(nn.Module):
def __init__(self, config: FunnelConfig) -> None:
super().__init__()
self.config = config
self.attention_structure = FunnelAttentionStructure(config)
self.layers = nn.ModuleList([FunnelLayer(config, 0) for _ in range(config.num_decoder_layers)])
def forward(
self,
final_hidden: torch.Tensor,
first_block_hidden: torch.Tensor,
attention_mask: Optional[torch.Tensor] = None,
token_type_ids: Optional[torch.Tensor] = None,
output_attentions: bool = False,
output_hidden_states: bool = False,
return_dict: bool = True,
) -> Union[Tuple, BaseModelOutput]:
upsampled_hidden = upsample(
final_hidden,
stride=2 ** (len(self.config.block_sizes) - 1),
target_len=first_block_hidden.shape[1],
separate_cls=self.config.separate_cls,
truncate_seq=self.config.truncate_seq,
)
hidden = upsampled_hidden + first_block_hidden
all_hidden_states = (hidden,) if output_hidden_states else None
all_attentions = () if output_attentions else None
attention_inputs = self.attention_structure.init_attention_inputs(
hidden,
attention_mask=attention_mask,
token_type_ids=token_type_ids,
)
for layer in self.layers:
layer_output = layer(hidden, hidden, hidden, attention_inputs, output_attentions=output_attentions)
hidden = layer_output[0]
if output_attentions:
all_attentions = all_attentions + layer_output[1:]
if output_hidden_states:
all_hidden_states = all_hidden_states + (hidden,)
if not return_dict:
return tuple(v for v in [hidden, all_hidden_states, all_attentions] if v is not None)
return BaseModelOutput(last_hidden_state=hidden, hidden_states=all_hidden_states, attentions=all_attentions)
class FunnelDiscriminatorPredictions(nn.Module):
"""Prediction module for the discriminator, made up of two dense layers."""
def __init__(self, config: FunnelConfig) -> None:
super().__init__()
self.config = config
self.dense = nn.Linear(config.d_model, config.d_model)
self.dense_prediction = nn.Linear(config.d_model, 1)
def forward(self, discriminator_hidden_states: torch.Tensor) -> torch.Tensor:
hidden_states = self.dense(discriminator_hidden_states)
hidden_states = ACT2FN[self.config.hidden_act](hidden_states)
logits = self.dense_prediction(hidden_states).squeeze()
return logits
class FunnelPreTrainedModel(PreTrainedModel):
"""
An abstract class to handle weights initialization and a simple interface for downloading and loading pretrained
models.
"""
config_class = FunnelConfig
load_tf_weights = load_tf_weights_in_funnel
base_model_prefix = "funnel"
def _init_weights(self, module):
classname = module.__class__.__name__
if classname.find("Linear") != -1:
if getattr(module, "weight", None) is not None:
if self.config.initializer_std is None:
fan_out, fan_in = module.weight.shape
std = np.sqrt(1.0 / float(fan_in + fan_out))
else:
std = self.config.initializer_std
nn.init.normal_(module.weight, std=std)
if getattr(module, "bias", None) is not None:
nn.init.constant_(module.bias, 0.0)
elif classname == "FunnelRelMultiheadAttention":
nn.init.uniform_(module.r_w_bias, b=self.config.initializer_range)
nn.init.uniform_(module.r_r_bias, b=self.config.initializer_range)
nn.init.uniform_(module.r_kernel, b=self.config.initializer_range)
nn.init.uniform_(module.r_s_bias, b=self.config.initializer_range)
nn.init.uniform_(module.seg_embed, b=self.config.initializer_range)
elif classname == "FunnelEmbeddings":
std = 1.0 if self.config.initializer_std is None else self.config.initializer_std
nn.init.normal_(module.word_embeddings.weight, std=std)
if module.word_embeddings.padding_idx is not None:
module.word_embeddings.weight.data[module.padding_idx].zero_()
class FunnelClassificationHead(nn.Module):
def __init__(self, config: FunnelConfig, n_labels: int) -> None:
super().__init__()
self.linear_hidden = nn.Linear(config.d_model, config.d_model)
self.dropout = nn.Dropout(config.hidden_dropout)
self.linear_out = nn.Linear(config.d_model, n_labels)
def forward(self, hidden: torch.Tensor) -> torch.Tensor:
hidden = self.linear_hidden(hidden)
hidden = torch.tanh(hidden)
hidden = self.dropout(hidden)
return self.linear_out(hidden)
@dataclass
class FunnelForPreTrainingOutput(ModelOutput):
"""
Output type of [`FunnelForPreTraining`].
Args:
loss (*optional*, returned when `labels` is provided, `torch.FloatTensor` of shape `(1,)`):
Total loss of the ELECTRA-style objective.
logits (`torch.FloatTensor` of shape `(batch_size, sequence_length)`):
Prediction scores of the head (scores for each token before SoftMax).
hidden_states (`tuple(torch.FloatTensor)`, *optional*, returned when `output_hidden_states=True` is passed or when `config.output_hidden_states=True`):
Tuple of `torch.FloatTensor` (one for the output of the embeddings + one for the output of each layer) of
shape `(batch_size, sequence_length, hidden_size)`.
Hidden-states of the model at the output of each layer plus the initial embedding outputs.
attentions (`tuple(torch.FloatTensor)`, *optional*, returned when `output_attentions=True` is passed or when `config.output_attentions=True`):
Tuple of `torch.FloatTensor` (one for each layer) of shape `(batch_size, num_heads, sequence_length,
sequence_length)`.
Attentions weights after the attention softmax, used to compute the weighted average in the self-attention
heads.
"""
loss: Optional[torch.FloatTensor] = None
logits: torch.FloatTensor = None
hidden_states: Optional[Tuple[torch.FloatTensor]] = None
attentions: Optional[Tuple[torch.FloatTensor]] = None
FUNNEL_START_DOCSTRING = r"""
The Funnel Transformer model was proposed in [Funnel-Transformer: Filtering out Sequential Redundancy for Efficient
Language Processing](https://arxiv.org/abs/2006.03236) by Zihang Dai, Guokun Lai, Yiming Yang, Quoc V. Le.
This model inherits from [`PreTrainedModel`]. Check the superclass documentation for the generic methods the
library implements for all its model (such as downloading or saving, resizing the input embeddings, pruning heads
etc.)
This model is also a PyTorch [torch.nn.Module](https://pytorch.org/docs/stable/nn.html#torch.nn.Module) subclass.
Use it as a regular PyTorch Module and refer to the PyTorch documentation for all matter related to general usage
and behavior.
Parameters:
config ([`FunnelConfig`]): Model configuration class with all the parameters of the model.
Initializing with a config file does not load the weights associated with the model, only the
configuration. Check out the [`~PreTrainedModel.from_pretrained`] method to load the model weights.
"""
FUNNEL_INPUTS_DOCSTRING = r"""
Args:
input_ids (`torch.LongTensor` of shape `({0})`):
Indices of input sequence tokens in the vocabulary.
Indices can be obtained using [`AutoTokenizer`]. See [`PreTrainedTokenizer.encode`] and
[`PreTrainedTokenizer.__call__`] for details.
[What are input IDs?](../glossary#input-ids)
attention_mask (`torch.FloatTensor` of shape `({0})`, *optional*):
Mask to avoid performing attention on padding token indices. Mask values selected in `[0, 1]`:
- 1 for tokens that are **not masked**,
- 0 for tokens that are **masked**.
[What are attention masks?](../glossary#attention-mask)
token_type_ids (`torch.LongTensor` of shape `({0})`, *optional*):
Segment token indices to indicate first and second portions of the inputs. Indices are selected in `[0,
1]`:
- 0 corresponds to a *sentence A* token,
- 1 corresponds to a *sentence B* token.
[What are token type IDs?](../glossary#token-type-ids)
inputs_embeds (`torch.FloatTensor` of shape `({0}, hidden_size)`, *optional*):
Optionally, instead of passing `input_ids` you can choose to directly pass an embedded representation. This
is useful if you want more control over how to convert `input_ids` indices into associated vectors than the
model's internal embedding lookup matrix.
output_attentions (`bool`, *optional*):
Whether or not to return the attentions tensors of all attention layers. See `attentions` under returned
tensors for more detail.
output_hidden_states (`bool`, *optional*):
Whether or not to return the hidden states of all layers. See `hidden_states` under returned tensors for
more detail.
return_dict (`bool`, *optional*):
Whether or not to return a [`~utils.ModelOutput`] instead of a plain tuple.
"""
@add_start_docstrings(
"""
The base Funnel Transformer Model transformer outputting raw hidden-states without upsampling head (also called
decoder) or any task-specific head on top.
""",
FUNNEL_START_DOCSTRING,
)
class FunnelBaseModel(FunnelPreTrainedModel):
def __init__(self, config: FunnelConfig) -> None:
super().__init__(config)
self.embeddings = FunnelEmbeddings(config)
self.encoder = FunnelEncoder(config)
# Initialize weights and apply final processing
self.post_init()
def get_input_embeddings(self) -> nn.Embedding:
return self.embeddings.word_embeddings
def set_input_embeddings(self, new_embeddings: nn.Embedding) -> None:
self.embeddings.word_embeddings = new_embeddings
@add_start_docstrings_to_model_forward(FUNNEL_INPUTS_DOCSTRING.format("batch_size, sequence_length"))
@add_code_sample_docstrings(
checkpoint="funnel-transformer/small-base",
output_type=BaseModelOutput,
config_class=_CONFIG_FOR_DOC,
)
def forward(
self,
input_ids: Optional[torch.Tensor] = None,
attention_mask: Optional[torch.Tensor] = None,
token_type_ids: Optional[torch.Tensor] = None,
position_ids: Optional[torch.Tensor] = None,
head_mask: Optional[torch.Tensor] = None,
inputs_embeds: Optional[torch.Tensor] = None,
output_attentions: Optional[bool] = None,
output_hidden_states: Optional[bool] = None,
return_dict: Optional[bool] = None,
) -> Union[Tuple, BaseModelOutput]:
output_attentions = output_attentions if output_attentions is not None else self.config.output_attentions
output_hidden_states = (
output_hidden_states if output_hidden_states is not None else self.config.output_hidden_states
)
return_dict = return_dict if return_dict is not None else self.config.use_return_dict
if input_ids is not None and inputs_embeds is not None:
raise ValueError("You cannot specify both input_ids and inputs_embeds at the same time")
elif input_ids is not None:
self.warn_if_padding_and_no_attention_mask(input_ids, attention_mask)
input_shape = input_ids.size()
elif inputs_embeds is not None:
input_shape = inputs_embeds.size()[:-1]
else:
raise ValueError("You have to specify either input_ids or inputs_embeds")
device = input_ids.device if input_ids is not None else inputs_embeds.device
if attention_mask is None:
attention_mask = torch.ones(input_shape, device=device)
if token_type_ids is None:
token_type_ids = torch.zeros(input_shape, dtype=torch.long, device=device)
# TODO: deal with head_mask
if inputs_embeds is None:
inputs_embeds = self.embeddings(input_ids)
encoder_outputs = self.encoder(
inputs_embeds,
attention_mask=attention_mask,
token_type_ids=token_type_ids,
output_attentions=output_attentions,
output_hidden_states=output_hidden_states,
return_dict=return_dict,
)
return encoder_outputs
@add_start_docstrings(
"The bare Funnel Transformer Model transformer outputting raw hidden-states without any specific head on top.",
FUNNEL_START_DOCSTRING,
)
class FunnelModel(FunnelPreTrainedModel):
def __init__(self, config: FunnelConfig) -> None:
super().__init__(config)
self.config = config
self.embeddings = FunnelEmbeddings(config)
self.encoder = FunnelEncoder(config)
self.decoder = FunnelDecoder(config)
# Initialize weights and apply final processing
self.post_init()
def get_input_embeddings(self) -> nn.Embedding:
return self.embeddings.word_embeddings
def set_input_embeddings(self, new_embeddings: nn.Embedding) -> None:
self.embeddings.word_embeddings = new_embeddings
@add_start_docstrings_to_model_forward(FUNNEL_INPUTS_DOCSTRING.format("batch_size, sequence_length"))
@add_code_sample_docstrings(
checkpoint=_CHECKPOINT_FOR_DOC,
output_type=BaseModelOutput,
config_class=_CONFIG_FOR_DOC,
)
def forward(
self,
input_ids: Optional[torch.Tensor] = None,
attention_mask: Optional[torch.Tensor] = None,
token_type_ids: Optional[torch.Tensor] = None,
inputs_embeds: Optional[torch.Tensor] = None,
output_attentions: Optional[bool] = None,
output_hidden_states: Optional[bool] = None,
return_dict: Optional[bool] = None,
) -> Union[Tuple, BaseModelOutput]:
output_attentions = output_attentions if output_attentions is not None else self.config.output_attentions
output_hidden_states = (
output_hidden_states if output_hidden_states is not None else self.config.output_hidden_states
)
return_dict = return_dict if return_dict is not None else self.config.use_return_dict
if input_ids is not None and inputs_embeds is not None:
raise ValueError("You cannot specify both input_ids and inputs_embeds at the same time")
elif input_ids is not None:
self.warn_if_padding_and_no_attention_mask(input_ids, attention_mask)
input_shape = input_ids.size()
elif inputs_embeds is not None:
input_shape = inputs_embeds.size()[:-1]
else:
raise ValueError("You have to specify either input_ids or inputs_embeds")
device = input_ids.device if input_ids is not None else inputs_embeds.device
if attention_mask is None:
attention_mask = torch.ones(input_shape, device=device)
if token_type_ids is None:
token_type_ids = torch.zeros(input_shape, dtype=torch.long, device=device)
# TODO: deal with head_mask
if inputs_embeds is None:
inputs_embeds = self.embeddings(input_ids)
encoder_outputs = self.encoder(
inputs_embeds,
attention_mask=attention_mask,
token_type_ids=token_type_ids,
output_attentions=output_attentions,
output_hidden_states=True,
return_dict=return_dict,
)
decoder_outputs = self.decoder(
final_hidden=encoder_outputs[0],
first_block_hidden=encoder_outputs[1][self.config.block_sizes[0]],
attention_mask=attention_mask,
token_type_ids=token_type_ids,
output_attentions=output_attentions,
output_hidden_states=output_hidden_states,
return_dict=return_dict,
)
if not return_dict:
idx = 0
outputs = (decoder_outputs[0],)
if output_hidden_states:
idx += 1
outputs = outputs + (encoder_outputs[1] + decoder_outputs[idx],)
if output_attentions:
idx += 1
outputs = outputs + (encoder_outputs[2] + decoder_outputs[idx],)
return outputs
return BaseModelOutput(
last_hidden_state=decoder_outputs[0],
hidden_states=(encoder_outputs.hidden_states + decoder_outputs.hidden_states)
if output_hidden_states
else None,
attentions=(encoder_outputs.attentions + decoder_outputs.attentions) if output_attentions else None,
)
add_start_docstrings(
"""
Funnel Transformer model with a binary classification head on top as used during pretraining for identifying
generated tokens.
""",
FUNNEL_START_DOCSTRING,
)
class FunnelForPreTraining(FunnelPreTrainedModel):
def __init__(self, config: FunnelConfig) -> None:
super().__init__(config)
self.funnel = FunnelModel(config)
self.discriminator_predictions = FunnelDiscriminatorPredictions(config)
# Initialize weights and apply final processing
self.post_init()
@add_start_docstrings_to_model_forward(FUNNEL_INPUTS_DOCSTRING.format("batch_size, sequence_length"))
@replace_return_docstrings(output_type=FunnelForPreTrainingOutput, config_class=_CONFIG_FOR_DOC)
def forward(
self,
input_ids: Optional[torch.Tensor] = None,
attention_mask: Optional[torch.Tensor] = None,
token_type_ids: Optional[torch.Tensor] = None,
inputs_embeds: Optional[torch.Tensor] = None,
labels: Optional[torch.Tensor] = None,
output_attentions: Optional[bool] = None,
output_hidden_states: Optional[bool] = None,
return_dict: Optional[bool] = None,
) -> Union[Tuple, FunnelForPreTrainingOutput]:
r"""
labels (`torch.LongTensor` of shape `(batch_size, sequence_length)`, *optional*):
Labels for computing the ELECTRA-style loss. Input should be a sequence of tokens (see `input_ids`
docstring) Indices should be in `[0, 1]`:
- 0 indicates the token is an original token,
- 1 indicates the token was replaced.
Returns:
Examples:
```python
>>> from transformers import AutoTokenizer, FunnelForPreTraining
>>> import torch
>>> tokenizer = AutoTokenizer.from_pretrained("funnel-transformer/small")
>>> model = FunnelForPreTraining.from_pretrained("funnel-transformer/small")
>>> inputs = tokenizer("Hello, my dog is cute", return_tensors="pt")
>>> logits = model(**inputs).logits
```"""
return_dict = return_dict if return_dict is not None else self.config.use_return_dict
discriminator_hidden_states = self.funnel(
input_ids,
attention_mask=attention_mask,
token_type_ids=token_type_ids,
inputs_embeds=inputs_embeds,
output_attentions=output_attentions,
output_hidden_states=output_hidden_states,
return_dict=return_dict,
)
discriminator_sequence_output = discriminator_hidden_states[0]
logits = self.discriminator_predictions(discriminator_sequence_output)
loss = None
if labels is not None:
loss_fct = nn.BCEWithLogitsLoss()
if attention_mask is not None:
active_loss = attention_mask.view(-1, discriminator_sequence_output.shape[1]) == 1
active_logits = logits.view(-1, discriminator_sequence_output.shape[1])[active_loss]
active_labels = labels[active_loss]
loss = loss_fct(active_logits, active_labels.float())
else:
loss = loss_fct(logits.view(-1, discriminator_sequence_output.shape[1]), labels.float())
if not return_dict:
output = (logits,) + discriminator_hidden_states[1:]
return ((loss,) + output) if loss is not None else output
return FunnelForPreTrainingOutput(
loss=loss,
logits=logits,
hidden_states=discriminator_hidden_states.hidden_states,
attentions=discriminator_hidden_states.attentions,
)
@add_start_docstrings("""Funnel Transformer Model with a `language modeling` head on top.""", FUNNEL_START_DOCSTRING)
class FunnelForMaskedLM(FunnelPreTrainedModel):
_tied_weights_keys = ["lm_head.weight"]
def __init__(self, config: FunnelConfig) -> None:
super().__init__(config)
self.funnel = FunnelModel(config)
self.lm_head = nn.Linear(config.d_model, config.vocab_size)
# Initialize weights and apply final processing
self.post_init()
def get_output_embeddings(self) -> nn.Linear:
return self.lm_head
def set_output_embeddings(self, new_embeddings: nn.Embedding) -> None:
self.lm_head = new_embeddings
@add_start_docstrings_to_model_forward(FUNNEL_INPUTS_DOCSTRING.format("batch_size, sequence_length"))
@add_code_sample_docstrings(
checkpoint=_CHECKPOINT_FOR_DOC,
output_type=MaskedLMOutput,
config_class=_CONFIG_FOR_DOC,
mask="<mask>",
)
def forward(
self,
input_ids: Optional[torch.Tensor] = None,
attention_mask: Optional[torch.Tensor] = None,
token_type_ids: Optional[torch.Tensor] = None,
inputs_embeds: Optional[torch.Tensor] = None,
labels: Optional[torch.Tensor] = None,
output_attentions: Optional[bool] = None,
output_hidden_states: Optional[bool] = None,
return_dict: Optional[bool] = None,
) -> Union[Tuple, MaskedLMOutput]:
r"""
labels (`torch.LongTensor` of shape `(batch_size, sequence_length)`, *optional*):
Labels for computing the masked language modeling loss. Indices should be in `[-100, 0, ...,
config.vocab_size]` (see `input_ids` docstring) Tokens with indices set to `-100` are ignored (masked), the
loss is only computed for the tokens with labels in `[0, ..., config.vocab_size]`
"""
return_dict = return_dict if return_dict is not None else self.config.use_return_dict
outputs = self.funnel(
input_ids,
attention_mask=attention_mask,
token_type_ids=token_type_ids,
inputs_embeds=inputs_embeds,
output_attentions=output_attentions,
output_hidden_states=output_hidden_states,
return_dict=return_dict,
)
last_hidden_state = outputs[0]
prediction_logits = self.lm_head(last_hidden_state)
masked_lm_loss = None
if labels is not None:
loss_fct = CrossEntropyLoss() # -100 index = padding token
masked_lm_loss = loss_fct(prediction_logits.view(-1, self.config.vocab_size), labels.view(-1))
if not return_dict:
output = (prediction_logits,) + outputs[1:]
return ((masked_lm_loss,) + output) if masked_lm_loss is not None else output
return MaskedLMOutput(
loss=masked_lm_loss,
logits=prediction_logits,
hidden_states=outputs.hidden_states,
attentions=outputs.attentions,
)
@add_start_docstrings(
"""
Funnel Transformer Model with a sequence classification/regression head on top (two linear layer on top of the
first timestep of the last hidden state) e.g. for GLUE tasks.
""",
FUNNEL_START_DOCSTRING,
)
class FunnelForSequenceClassification(FunnelPreTrainedModel):
def __init__(self, config: FunnelConfig) -> None:
super().__init__(config)
self.num_labels = config.num_labels
self.config = config
self.funnel = FunnelBaseModel(config)
self.classifier = FunnelClassificationHead(config, config.num_labels)
# Initialize weights and apply final processing
self.post_init()
@add_start_docstrings_to_model_forward(FUNNEL_INPUTS_DOCSTRING.format("batch_size, sequence_length"))
@add_code_sample_docstrings(
checkpoint="funnel-transformer/small-base",
output_type=SequenceClassifierOutput,
config_class=_CONFIG_FOR_DOC,
)
def forward(
self,
input_ids: Optional[torch.Tensor] = None,
attention_mask: Optional[torch.Tensor] = None,
token_type_ids: Optional[torch.Tensor] = None,
inputs_embeds: Optional[torch.Tensor] = None,
labels: Optional[torch.Tensor] = None,
output_attentions: Optional[bool] = None,
output_hidden_states: Optional[bool] = None,
return_dict: Optional[bool] = None,
) -> Union[Tuple, SequenceClassifierOutput]:
r"""
labels (`torch.LongTensor` of shape `(batch_size,)`, *optional*):
Labels for computing the sequence classification/regression loss. Indices should be in `[0, ...,
config.num_labels - 1]`. If `config.num_labels == 1` a regression loss is computed (Mean-Square loss), If
`config.num_labels > 1` a classification loss is computed (Cross-Entropy).
"""
return_dict = return_dict if return_dict is not None else self.config.use_return_dict
outputs = self.funnel(
input_ids,
attention_mask=attention_mask,
token_type_ids=token_type_ids,
inputs_embeds=inputs_embeds,
output_attentions=output_attentions,
output_hidden_states=output_hidden_states,
return_dict=return_dict,
)
last_hidden_state = outputs[0]
pooled_output = last_hidden_state[:, 0]
logits = self.classifier(pooled_output)
loss = None
if labels is not None:
if self.config.problem_type is None:
if self.num_labels == 1:
self.config.problem_type = "regression"
elif self.num_labels > 1 and (labels.dtype == torch.long or labels.dtype == torch.int):
self.config.problem_type = "single_label_classification"
else:
self.config.problem_type = "multi_label_classification"
if self.config.problem_type == "regression":
loss_fct = MSELoss()
if self.num_labels == 1:
loss = loss_fct(logits.squeeze(), labels.squeeze())
else:
loss = loss_fct(logits, labels)
elif self.config.problem_type == "single_label_classification":
loss_fct = CrossEntropyLoss()
loss = loss_fct(logits.view(-1, self.num_labels), labels.view(-1))
elif self.config.problem_type == "multi_label_classification":
loss_fct = BCEWithLogitsLoss()
loss = loss_fct(logits, labels)
if not return_dict:
output = (logits,) + outputs[1:]
return ((loss,) + output) if loss is not None else output
return SequenceClassifierOutput(
loss=loss,
logits=logits,
hidden_states=outputs.hidden_states,
attentions=outputs.attentions,
)
@add_start_docstrings(
"""
Funnel Transformer Model with a multiple choice classification head on top (two linear layer on top of the first
timestep of the last hidden state, and a softmax) e.g. for RocStories/SWAG tasks.
""",
FUNNEL_START_DOCSTRING,
)
class FunnelForMultipleChoice(FunnelPreTrainedModel):
def __init__(self, config: FunnelConfig) -> None:
super().__init__(config)
self.funnel = FunnelBaseModel(config)
self.classifier = FunnelClassificationHead(config, 1)
# Initialize weights and apply final processing
self.post_init()
@add_start_docstrings_to_model_forward(FUNNEL_INPUTS_DOCSTRING.format("batch_size, num_choices, sequence_length"))
@add_code_sample_docstrings(
checkpoint="funnel-transformer/small-base",
output_type=MultipleChoiceModelOutput,
config_class=_CONFIG_FOR_DOC,
)
def forward(
self,
input_ids: Optional[torch.Tensor] = None,
attention_mask: Optional[torch.Tensor] = None,
token_type_ids: Optional[torch.Tensor] = None,
inputs_embeds: Optional[torch.Tensor] = None,
labels: Optional[torch.Tensor] = None,
output_attentions: Optional[bool] = None,
output_hidden_states: Optional[bool] = None,
return_dict: Optional[bool] = None,
) -> Union[Tuple, MultipleChoiceModelOutput]:
r"""
labels (`torch.LongTensor` of shape `(batch_size,)`, *optional*):
Labels for computing the multiple choice classification loss. Indices should be in `[0, ...,
num_choices-1]` where `num_choices` is the size of the second dimension of the input tensors. (See
`input_ids` above)
"""
return_dict = return_dict if return_dict is not None else self.config.use_return_dict
num_choices = input_ids.shape[1] if input_ids is not None else inputs_embeds.shape[1]
input_ids = input_ids.view(-1, input_ids.size(-1)) if input_ids is not None else None
attention_mask = attention_mask.view(-1, attention_mask.size(-1)) if attention_mask is not None else None
token_type_ids = token_type_ids.view(-1, token_type_ids.size(-1)) if token_type_ids is not None else None
inputs_embeds = (
inputs_embeds.view(-1, inputs_embeds.size(-2), inputs_embeds.size(-1))
if inputs_embeds is not None
else None
)
outputs = self.funnel(
input_ids,
attention_mask=attention_mask,
token_type_ids=token_type_ids,
inputs_embeds=inputs_embeds,
output_attentions=output_attentions,
output_hidden_states=output_hidden_states,
return_dict=return_dict,
)
last_hidden_state = outputs[0]
pooled_output = last_hidden_state[:, 0]
logits = self.classifier(pooled_output)
reshaped_logits = logits.view(-1, num_choices)
loss = None
if labels is not None:
loss_fct = CrossEntropyLoss()
loss = loss_fct(reshaped_logits, labels)
if not return_dict:
output = (reshaped_logits,) + outputs[1:]
return ((loss,) + output) if loss is not None else output
return MultipleChoiceModelOutput(
loss=loss,
logits=reshaped_logits,
hidden_states=outputs.hidden_states,
attentions=outputs.attentions,
)
@add_start_docstrings(
"""
Funnel Transformer Model with a token classification head on top (a linear layer on top of the hidden-states
output) e.g. for Named-Entity-Recognition (NER) tasks.
""",
FUNNEL_START_DOCSTRING,
)
class FunnelForTokenClassification(FunnelPreTrainedModel):
def __init__(self, config: FunnelConfig) -> None:
super().__init__(config)
self.num_labels = config.num_labels
self.funnel = FunnelModel(config)
self.dropout = nn.Dropout(config.hidden_dropout)
self.classifier = nn.Linear(config.hidden_size, config.num_labels)
# Initialize weights and apply final processing
self.post_init()
@add_start_docstrings_to_model_forward(FUNNEL_INPUTS_DOCSTRING.format("batch_size, sequence_length"))
@add_code_sample_docstrings(
checkpoint=_CHECKPOINT_FOR_DOC,
output_type=TokenClassifierOutput,
config_class=_CONFIG_FOR_DOC,
)
def forward(
self,
input_ids: Optional[torch.Tensor] = None,
attention_mask: Optional[torch.Tensor] = None,
token_type_ids: Optional[torch.Tensor] = None,
inputs_embeds: Optional[torch.Tensor] = None,
labels: Optional[torch.Tensor] = None,
output_attentions: Optional[bool] = None,
output_hidden_states: Optional[bool] = None,
return_dict: Optional[bool] = None,
) -> Union[Tuple, TokenClassifierOutput]:
r"""
labels (`torch.LongTensor` of shape `(batch_size, sequence_length)`, *optional*):
Labels for computing the token classification loss. Indices should be in `[0, ..., config.num_labels - 1]`.
"""
return_dict = return_dict if return_dict is not None else self.config.use_return_dict
outputs = self.funnel(
input_ids,
attention_mask=attention_mask,
token_type_ids=token_type_ids,
inputs_embeds=inputs_embeds,
output_attentions=output_attentions,
output_hidden_states=output_hidden_states,
return_dict=return_dict,
)
last_hidden_state = outputs[0]
last_hidden_state = self.dropout(last_hidden_state)
logits = self.classifier(last_hidden_state)
loss = None
if labels is not None:
loss_fct = CrossEntropyLoss()
loss = loss_fct(logits.view(-1, self.num_labels), labels.view(-1))
if not return_dict:
output = (logits,) + outputs[1:]
return ((loss,) + output) if loss is not None else output
return TokenClassifierOutput(
loss=loss,
logits=logits,
hidden_states=outputs.hidden_states,
attentions=outputs.attentions,
)
@add_start_docstrings(
"""
Funnel Transformer Model with a span classification head on top for extractive question-answering tasks like SQuAD
(a linear layer on top of the hidden-states output to compute `span start logits` and `span end logits`).
""",
FUNNEL_START_DOCSTRING,
)
class FunnelForQuestionAnswering(FunnelPreTrainedModel):
def __init__(self, config: FunnelConfig) -> None:
super().__init__(config)
self.num_labels = config.num_labels
self.funnel = FunnelModel(config)
self.qa_outputs = nn.Linear(config.hidden_size, config.num_labels)
# Initialize weights and apply final processing
self.post_init()
@add_start_docstrings_to_model_forward(FUNNEL_INPUTS_DOCSTRING.format("batch_size, sequence_length"))
@add_code_sample_docstrings(
checkpoint=_CHECKPOINT_FOR_DOC,
output_type=QuestionAnsweringModelOutput,
config_class=_CONFIG_FOR_DOC,
)
def forward(
self,
input_ids: Optional[torch.Tensor] = None,
attention_mask: Optional[torch.Tensor] = None,
token_type_ids: Optional[torch.Tensor] = None,
inputs_embeds: Optional[torch.Tensor] = None,
start_positions: Optional[torch.Tensor] = None,
end_positions: Optional[torch.Tensor] = None,
output_attentions: Optional[bool] = None,
output_hidden_states: Optional[bool] = None,
return_dict: Optional[bool] = None,
) -> Union[Tuple, QuestionAnsweringModelOutput]:
r"""
start_positions (`torch.LongTensor` of shape `(batch_size,)`, *optional*):
Labels for position (index) of the start of the labelled span for computing the token classification loss.
Positions are clamped to the length of the sequence (`sequence_length`). Position outside of the sequence
are not taken into account for computing the loss.
end_positions (`torch.LongTensor` of shape `(batch_size,)`, *optional*):
Labels for position (index) of the end of the labelled span for computing the token classification loss.
Positions are clamped to the length of the sequence (`sequence_length`). Position outside of the sequence
are not taken into account for computing the loss.
"""
return_dict = return_dict if return_dict is not None else self.config.use_return_dict
outputs = self.funnel(
input_ids,
attention_mask=attention_mask,
token_type_ids=token_type_ids,
inputs_embeds=inputs_embeds,
output_attentions=output_attentions,
output_hidden_states=output_hidden_states,
return_dict=return_dict,
)
last_hidden_state = outputs[0]
logits = self.qa_outputs(last_hidden_state)
start_logits, end_logits = logits.split(1, dim=-1)
start_logits = start_logits.squeeze(-1).contiguous()
end_logits = end_logits.squeeze(-1).contiguous()
total_loss = None
if start_positions is not None and end_positions is not None:
# If we are on multi-GPU, split add a dimension
if len(start_positions.size()) > 1:
start_positions = start_positions.squeze(-1)
if len(end_positions.size()) > 1:
end_positions = end_positions.squeeze(-1)
# sometimes the start/end positions are outside our model inputs, we ignore these terms
ignored_index = start_logits.size(1)
start_positions = start_positions.clamp(0, ignored_index)
end_positions = end_positions.clamp(0, ignored_index)
loss_fct = CrossEntropyLoss(ignore_index=ignored_index)
start_loss = loss_fct(start_logits, start_positions)
end_loss = loss_fct(end_logits, end_positions)
total_loss = (start_loss + end_loss) / 2
if not return_dict:
output = (start_logits, end_logits) + outputs[1:]
return ((total_loss,) + output) if total_loss is not None else output
return QuestionAnsweringModelOutput(
loss=total_loss,
start_logits=start_logits,
end_logits=end_logits,
hidden_states=outputs.hidden_states,
attentions=outputs.attentions,
)
| transformers/src/transformers/models/funnel/modeling_funnel.py/0 | {
"file_path": "transformers/src/transformers/models/funnel/modeling_funnel.py",
"repo_id": "transformers",
"token_count": 30866
} | 332 |
# coding=utf-8
# Copyright 2022 KAIST and The HuggingFace Inc. team. All rights reserved.
#
# Licensed under the Apache License, Version 2.0 (the "License");
# you may not use this file except in compliance with the License.
# You may obtain a copy of the License at
#
# http://www.apache.org/licenses/LICENSE-2.0
#
# Unless required by applicable law or agreed to in writing, software
# distributed under the License is distributed on an "AS IS" BASIS,
# WITHOUT WARRANTIES OR CONDITIONS OF ANY KIND, either express or implied.
# See the License for the specific language governing permissions and
# limitations under the License.
""" GLPN model configuration"""
from ...configuration_utils import PretrainedConfig
from ...utils import logging
logger = logging.get_logger(__name__)
GLPN_PRETRAINED_CONFIG_ARCHIVE_MAP = {
"vinvino02/glpn-kitti": "https://huggingface.co/vinvino02/glpn-kitti/resolve/main/config.json",
# See all GLPN models at https://huggingface.co/models?filter=glpn
}
class GLPNConfig(PretrainedConfig):
r"""
This is the configuration class to store the configuration of a [`GLPNModel`]. It is used to instantiate an GLPN
model according to the specified arguments, defining the model architecture. Instantiating a configuration with the
defaults will yield a similar configuration to that of the GLPN
[vinvino02/glpn-kitti](https://huggingface.co/vinvino02/glpn-kitti) architecture.
Configuration objects inherit from [`PretrainedConfig`] and can be used to control the model outputs. Read the
documentation from [`PretrainedConfig`] for more information.
Args:
num_channels (`int`, *optional*, defaults to 3):
The number of input channels.
num_encoder_blocks (`int`, *optional*, defaults to 4):
The number of encoder blocks (i.e. stages in the Mix Transformer encoder).
depths (`List[int]`, *optional*, defaults to `[2, 2, 2, 2]`):
The number of layers in each encoder block.
sr_ratios (`List[int]`, *optional*, defaults to `[8, 4, 2, 1]`):
Sequence reduction ratios in each encoder block.
hidden_sizes (`List[int]`, *optional*, defaults to `[32, 64, 160, 256]`):
Dimension of each of the encoder blocks.
patch_sizes (`List[int]`, *optional*, defaults to `[7, 3, 3, 3]`):
Patch size before each encoder block.
strides (`List[int]`, *optional*, defaults to `[4, 2, 2, 2]`):
Stride before each encoder block.
num_attention_heads (`List[int]`, *optional*, defaults to `[1, 2, 5, 8]`):
Number of attention heads for each attention layer in each block of the Transformer encoder.
mlp_ratios (`List[int]`, *optional*, defaults to `[4, 4, 4, 4]`):
Ratio of the size of the hidden layer compared to the size of the input layer of the Mix FFNs in the
encoder blocks.
hidden_act (`str` or `function`, *optional*, defaults to `"gelu"`):
The non-linear activation function (function or string) in the encoder and pooler. If string, `"gelu"`,
`"relu"`, `"selu"` and `"gelu_new"` are supported.
hidden_dropout_prob (`float`, *optional*, defaults to 0.0):
The dropout probability for all fully connected layers in the embeddings, encoder, and pooler.
attention_probs_dropout_prob (`float`, *optional*, defaults to 0.0):
The dropout ratio for the attention probabilities.
initializer_range (`float`, *optional*, defaults to 0.02):
The standard deviation of the truncated_normal_initializer for initializing all weight matrices.
drop_path_rate (`float`, *optional*, defaults to 0.1):
The dropout probability for stochastic depth, used in the blocks of the Transformer encoder.
layer_norm_eps (`float`, *optional*, defaults to 1e-06):
The epsilon used by the layer normalization layers.
decoder_hidden_size (`int`, *optional*, defaults to 64):
The dimension of the decoder.
max_depth (`int`, *optional*, defaults to 10):
The maximum depth of the decoder.
head_in_index (`int`, *optional*, defaults to -1):
The index of the features to use in the head.
Example:
```python
>>> from transformers import GLPNModel, GLPNConfig
>>> # Initializing a GLPN vinvino02/glpn-kitti style configuration
>>> configuration = GLPNConfig()
>>> # Initializing a model from the vinvino02/glpn-kitti style configuration
>>> model = GLPNModel(configuration)
>>> # Accessing the model configuration
>>> configuration = model.config
```"""
model_type = "glpn"
def __init__(
self,
num_channels=3,
num_encoder_blocks=4,
depths=[2, 2, 2, 2],
sr_ratios=[8, 4, 2, 1],
hidden_sizes=[32, 64, 160, 256],
patch_sizes=[7, 3, 3, 3],
strides=[4, 2, 2, 2],
num_attention_heads=[1, 2, 5, 8],
mlp_ratios=[4, 4, 4, 4],
hidden_act="gelu",
hidden_dropout_prob=0.0,
attention_probs_dropout_prob=0.0,
initializer_range=0.02,
drop_path_rate=0.1,
layer_norm_eps=1e-6,
decoder_hidden_size=64,
max_depth=10,
head_in_index=-1,
**kwargs,
):
super().__init__(**kwargs)
self.num_channels = num_channels
self.num_encoder_blocks = num_encoder_blocks
self.depths = depths
self.sr_ratios = sr_ratios
self.hidden_sizes = hidden_sizes
self.patch_sizes = patch_sizes
self.strides = strides
self.mlp_ratios = mlp_ratios
self.num_attention_heads = num_attention_heads
self.hidden_act = hidden_act
self.hidden_dropout_prob = hidden_dropout_prob
self.attention_probs_dropout_prob = attention_probs_dropout_prob
self.initializer_range = initializer_range
self.drop_path_rate = drop_path_rate
self.layer_norm_eps = layer_norm_eps
self.decoder_hidden_size = decoder_hidden_size
self.max_depth = max_depth
self.head_in_index = head_in_index
| transformers/src/transformers/models/glpn/configuration_glpn.py/0 | {
"file_path": "transformers/src/transformers/models/glpn/configuration_glpn.py",
"repo_id": "transformers",
"token_count": 2440
} | 333 |
# coding=utf-8
# Copyright 2023 The BigCode team and HuggingFace Inc. team.
#
# Licensed under the Apache License, Version 2.0 (the "License");
# you may not use this file except in compliance with the License.
# You may obtain a copy of the License at
#
# http://www.apache.org/licenses/LICENSE-2.0
#
# Unless required by applicable law or agreed to in writing, software
# distributed under the License is distributed on an "AS IS" BASIS,
# WITHOUT WARRANTIES OR CONDITIONS OF ANY KIND, either express or implied.
# See the License for the specific language governing permissions and
# limitations under the License.
""" GPTBigCode configuration"""
from ...configuration_utils import PretrainedConfig
from ...utils import logging
logger = logging.get_logger(__name__)
GPT_BIGCODE_PRETRAINED_CONFIG_ARCHIVE_MAP = {
"bigcode/gpt_bigcode-santacoder": "https://huggingface.co/bigcode/gpt_bigcode-santacoder/resolve/main/config.json",
}
class GPTBigCodeConfig(PretrainedConfig):
"""
This is the configuration class to store the configuration of a [`GPTBigCodeModel`]. It is used to instantiate a
GPTBigCode model according to the specified arguments, defining the model architecture. Instantiating a
configuration with the defaults will yield a similar configuration to that of the GPTBigCode
[gpt_bigcode](https://huggingface.co/gpt_bigcode) architecture.
Configuration objects inherit from [`PretrainedConfig`] and can be used to control the model outputs. Read the
documentation from [`PretrainedConfig`] for more information.
Args:
vocab_size (`int`, *optional*, defaults to 50257):
Vocabulary size of the GPT-2 model. Defines the number of different tokens that can be represented by the
`inputs_ids` passed when calling [`GPTBigCodeModel`].
n_positions (`int`, *optional*, defaults to 1024):
The maximum sequence length that this model might ever be used with. Typically set this to something large
just in case (e.g., 512 or 1024 or 2048).
n_embd (`int`, *optional*, defaults to 768):
Dimensionality of the embeddings and hidden states.
n_layer (`int`, *optional*, defaults to 12):
Number of hidden layers in the Transformer encoder.
n_head (`int`, *optional*, defaults to 12):
Number of attention heads for each attention layer in the Transformer encoder.
n_inner (`int`, *optional*, defaults to None):
Dimensionality of the inner feed-forward layers. `None` will set it to 4 times n_embd
activation_function (`str`, *optional*, defaults to `"gelu_pytorch_tanh"`):
Activation function, to be selected in the list `["relu", "silu", "gelu", "tanh", "gelu_new",
"gelu_pytorch_tanh"]`.
resid_pdrop (`float`, *optional*, defaults to 0.1):
The dropout probability for all fully connected layers in the embeddings, encoder, and pooler.
embd_pdrop (`float`, *optional*, defaults to 0.1):
The dropout ratio for the embeddings.
attn_pdrop (`float`, *optional*, defaults to 0.1):
The dropout ratio for the attention.
layer_norm_epsilon (`float`, *optional*, defaults to 1e-5):
The epsilon to use in the layer normalization layers.
initializer_range (`float`, *optional*, defaults to 0.02):
The standard deviation of the truncated_normal_initializer for initializing all weight matrices.
scale_attn_weights (`bool`, *optional*, defaults to `True`):
Scale attention weights by dividing by sqrt(hidden_size)..
use_cache (`bool`, *optional*, defaults to `True`):
Whether or not the model should return the last key/values attentions (not used by all models).
attention_softmax_in_fp32 (`bool`, *optional*, defaults to `True`):
Whether to call the fused softmax in float32.
scale_attention_softmax_in_fp32 (`bool`, *optional*, defaults to `True`):
Whether to scale the attention softmax in float32.
attention_type (`bool`, *optional*, defaults to `True`):
Whether to use Multi-Query Attion (`True`) or Multi-Head Attention (`False`).
Example:
```python
>>> from transformers import GPTBigCodeConfig, GPTBigCodeModel
>>> # Initializing a GPTBigCode configuration
>>> configuration = GPTBigCodeConfig()
>>> # Initializing a model (with random weights) from the configuration
>>> model = GPTBigCodeModel(configuration)
>>> # Accessing the model configuration
>>> configuration = model.config
```"""
model_type = "gpt_bigcode"
keys_to_ignore_at_inference = ["past_key_values"]
attribute_map = {
"hidden_size": "n_embd",
"max_position_embeddings": "n_positions",
"num_attention_heads": "n_head",
"num_hidden_layers": "n_layer",
}
def __init__(
self,
vocab_size=50257,
n_positions=1024,
n_embd=768,
n_layer=12,
n_head=12,
n_inner=None,
activation_function="gelu_pytorch_tanh",
resid_pdrop=0.1,
embd_pdrop=0.1,
attn_pdrop=0.1,
layer_norm_epsilon=1e-5,
initializer_range=0.02,
scale_attn_weights=True,
use_cache=True,
bos_token_id=50256,
eos_token_id=50256,
attention_softmax_in_fp32=True,
scale_attention_softmax_in_fp32=True,
multi_query=True,
**kwargs,
):
self.vocab_size = vocab_size
self.n_positions = n_positions
self.n_embd = n_embd
self.n_layer = n_layer
self.n_head = n_head
self.n_inner = n_inner
self.activation_function = activation_function
self.resid_pdrop = resid_pdrop
self.embd_pdrop = embd_pdrop
self.attn_pdrop = attn_pdrop
self.layer_norm_epsilon = layer_norm_epsilon
self.initializer_range = initializer_range
self.scale_attn_weights = scale_attn_weights
self.use_cache = use_cache
self.attention_softmax_in_fp32 = attention_softmax_in_fp32
self.scale_attention_softmax_in_fp32 = scale_attention_softmax_in_fp32
self.multi_query = multi_query
self.bos_token_id = bos_token_id
self.eos_token_id = eos_token_id
super().__init__(bos_token_id=bos_token_id, eos_token_id=eos_token_id, **kwargs)
| transformers/src/transformers/models/gpt_bigcode/configuration_gpt_bigcode.py/0 | {
"file_path": "transformers/src/transformers/models/gpt_bigcode/configuration_gpt_bigcode.py",
"repo_id": "transformers",
"token_count": 2533
} | 334 |
# Copyright 2022 The HuggingFace Inc. team and the AI-Sweden team. All rights reserved.
#
# Licensed under the Apache License, Version 2.0 (the "License");
# you may not use this file except in compliance with the License.
# You may obtain a copy of the License at
#
# http://www.apache.org/licenses/LICENSE-2.0
#
# Unless required by applicable law or agreed to in writing, software
# distributed under the License is distributed on an "AS IS" BASIS,
# WITHOUT WARRANTIES OR CONDITIONS OF ANY KIND, either express or implied.
# See the License for the specific language governing permissions and
# limitations under the License.
""" Convert GPT-SW3 megatron checkpoints to pytorch"""
import argparse
import os
from os.path import isfile
import torch
from transformers import GPT2Config
def recursive_print(name, val, spaces=0):
# Format the message.
if name is None:
msg = None
else:
fmt = "." * max(0, spaces - 2) + "# {:" + str(50 - spaces) + "s}"
msg = fmt.format(name)
# Print and recurse (if needed).
if isinstance(val, dict):
if msg is not None:
print(msg)
for k in val.keys():
recursive_print(k, val[k], spaces + 2)
elif isinstance(val, torch.Tensor):
print(msg, ":", val.size())
else:
print(msg, ":", val)
def fix_query_key_value_ordering(param, num_splits, num_heads, hidden_size):
# Permutes layout of param tensor to [num_splits * num_heads * hidden_size, :]
# for compatibility with later versions of NVIDIA Megatron-LM.
# The inverse operation is performed inside Megatron-LM to read checkpoints:
# https://github.com/NVIDIA/Megatron-LM/blob/v2.4/megatron/checkpointing.py#L209
# If param is the weight tensor of the self-attention block, the returned tensor
# will have to be transposed one more time to be read by HuggingFace GPT2.
input_shape = param.size()
# other versions store [num_heads * num_splits * hidden_size, :]
saved_shape = (num_heads, num_splits, hidden_size) + input_shape[1:]
param = param.view(*saved_shape)
param = param.transpose(0, 1).contiguous()
param = param.view(*input_shape)
return param
def convert_megatron_checkpoint(sd_megatron, config):
"""
Converts a Megatron checkpoint to a HuggingFace GPT-SW3 checkpoint.
"""
n_positions = config.n_positions
layers = config.n_layer
vocab_size = config.vocab_size
heads = config.n_head
hidden_size_per_head = config.n_embd // config.n_head
word_embeddings = sd_megatron["model.language_model.embedding.word_embeddings.weight"][:vocab_size, :]
sd_hf = {
"transformer.wte.weight": word_embeddings,
"transformer.wpe.weight": sd_megatron["model.language_model.embedding.position_embeddings.weight"],
"transformer.ln_f.weight": sd_megatron["model.language_model.encoder.final_layernorm.weight"],
"transformer.ln_f.bias": sd_megatron["model.language_model.encoder.final_layernorm.bias"],
}
pf = "model.language_model.encoder.layers."
for i in range(layers):
causal_mask = torch.tril(torch.ones((n_positions, n_positions), dtype=torch.bool))
causal_mask = causal_mask.view(1, 1, n_positions, n_positions)
sd_hf[f"transformer.h.{i}.attn.bias"] = causal_mask
sd_hf[f"transformer.h.{i}.attn.masked_bias"] = torch.tensor(-1e4, dtype=torch.bfloat16)
sd_hf[f"transformer.h.{i}.ln_1.weight"] = sd_megatron[f"{pf}{i}.input_layernorm.weight"]
sd_hf[f"transformer.h.{i}.ln_1.bias"] = sd_megatron[f"{pf}{i}.input_layernorm.bias"]
val1 = sd_megatron[f"{pf}{i}.self_attention.query_key_value.weight"]
val1 = fix_query_key_value_ordering(val1, 3, heads, hidden_size_per_head)
sd_hf[f"transformer.h.{i}.attn.c_attn.weight"] = val1.transpose(0, 1).contiguous()
val2 = sd_megatron[f"{pf}{i}.self_attention.query_key_value.bias"]
val2 = fix_query_key_value_ordering(val2, 3, heads, hidden_size_per_head)
sd_hf[f"transformer.h.{i}.attn.c_attn.bias"] = val2
sd_hf[f"transformer.h.{i}.attn.c_proj.weight"] = sd_megatron[f"{pf}{i}.self_attention.dense.weight"].transpose(
0, 1
)
sd_hf[f"transformer.h.{i}.attn.c_proj.bias"] = sd_megatron[f"{pf}{i}.self_attention.dense.bias"]
sd_hf[f"transformer.h.{i}.ln_2.weight"] = sd_megatron[f"{pf}{i}.post_attention_layernorm.weight"]
sd_hf[f"transformer.h.{i}.ln_2.bias"] = sd_megatron[f"{pf}{i}.post_attention_layernorm.bias"]
sd_hf[f"transformer.h.{i}.mlp.c_fc.weight"] = sd_megatron[f"{pf}{i}.mlp.dense_h_to_4h.weight"].transpose(0, 1)
sd_hf[f"transformer.h.{i}.mlp.c_fc.bias"] = sd_megatron[f"{pf}{i}.mlp.dense_h_to_4h.bias"]
sd_hf[f"transformer.h.{i}.mlp.c_proj.weight"] = sd_megatron[f"{pf}{i}.mlp.dense_4h_to_h.weight"].transpose(
0, 1
)
sd_hf[f"transformer.h.{i}.mlp.c_proj.bias"] = sd_megatron[f"{pf}{i}.mlp.dense_4h_to_h.bias"]
# For LM head, transformers' wants the matrix to weight embeddings.
sd_hf["lm_head.weight"] = word_embeddings
return sd_hf
def copy_config(config_hf, config_megatron):
"""Copy the config from Megatron to hf."""
config_hf.vocab_size = 64000
config_hf.n_positions = config_megatron["encoder_seq_length"]
config_hf.n_embd = config_megatron["hidden_size"]
config_hf.n_layer = config_megatron["num_layers"]
config_hf.n_head = config_megatron["num_attention_heads"]
config_hf.n_inner = config_megatron["ffn_hidden_size"]
config_hf.activation_function = "gelu"
config_hf.resid_pdrop = 0.1
config_hf.embd_pdrop = 0.1
config_hf.attn_pdrop = 0.1
config_hf.layer_norm_epsilon = config_megatron["layernorm_epsilon"] # 1e-5
config_hf.initializer_range = config_megatron["init_method_std"] # 0.02
config_hf.apply_query_key_layer_scaling = config_megatron["apply_query_key_layer_scaling"] # True
config_hf.normalize_attention_scores = True
config_hf.use_cache = True
# This identifies the 6.7B (7B) model which uses a different tokenizer
if config_megatron["hidden_size"] == 4096:
config_hf.bos_token_id = 1 # <|endoftext|>
config_hf.eos_token_id = 1 # <|endoftext|>
config_hf.pad_token_id = 0 # <unk>
else:
config_hf.bos_token_id = 2 # <s>
config_hf.eos_token_id = 3 # <|endoftext|>
config_hf.pad_token_id = 0 # <pad>
return config_hf
def main(args):
print(args)
checkpoint_path = args.checkpoint_path
save_path = args.save_path
if isfile(checkpoint_path):
raise FileNotFoundError(f"ERROR! could not find file {checkpoint_path}")
# Load the model.
checkpoint = torch.load(checkpoint_path, map_location="cpu")
# Load the config.
config_megatron = checkpoint["hyper_parameters"]["cfg"]
config_hf = GPT2Config()
config_hf = copy_config(config_hf=config_hf, config_megatron=config_megatron)
config_hf.architectures = ["GPT2LMHeadModel"]
sd_megatron = checkpoint["state_dict"]
# Convert.
print("Converting")
sd_hf = convert_megatron_checkpoint(sd_megatron, config_hf)
# Print the structure of converted state dict.
if args.print_checkpoint_structure:
recursive_print(None, sd_hf)
config_hf.tokenizer_class = "GPTSw3Tokenizer"
# Store the config to file.
print("Saving config")
config_hf.save_pretrained(save_path)
# Store the state_dict to file.
output_checkpoint_file = os.path.join(save_path, "pytorch_model.bin")
print(f'Saving checkpoint to "{output_checkpoint_file}"')
torch.save(sd_hf, output_checkpoint_file)
if __name__ == "__main__":
parser = argparse.ArgumentParser()
parser.add_argument(
"--checkpoint_path",
type=str,
required=True,
help="e.g. megatron_gpt--val_loss=2.42-step=38000-consumed_samples=54720000",
)
parser.add_argument("--save_path", type=str, required=True, help="e.g. /home/user/gpt-sw3/hf")
parser.add_argument("--print-checkpoint-structure", action="store_true")
_args = parser.parse_args()
main(_args)
| transformers/src/transformers/models/gpt_sw3/convert_megatron_to_pytorch.py/0 | {
"file_path": "transformers/src/transformers/models/gpt_sw3/convert_megatron_to_pytorch.py",
"repo_id": "transformers",
"token_count": 3464
} | 335 |
# coding=utf-8
# Copyright 2022 Microsoft, clefourrier The HuggingFace Inc. team. All rights reserved.
#
# Licensed under the Apache License, Version 2.0 (the "License");
# you may not use this file except in compliance with the License.
# You may obtain a copy of the License at
#
# http://www.apache.org/licenses/LICENSE-2.0
#
# Unless required by applicable law or agreed to in writing, software
# distributed under the License is distributed on an "AS IS" BASIS,
# WITHOUT WARRANTIES OR CONDITIONS OF ANY KIND, either express or implied.
# See the License for the specific language governing permissions and
# limitations under the License.
""" PyTorch Graphormer model."""
import math
from typing import Iterable, Iterator, List, Optional, Tuple, Union
import torch
import torch.nn as nn
from torch.nn import BCEWithLogitsLoss, CrossEntropyLoss, MSELoss
from ...activations import ACT2FN
from ...modeling_outputs import (
BaseModelOutputWithNoAttention,
SequenceClassifierOutput,
)
from ...modeling_utils import PreTrainedModel
from ...utils import logging
from .configuration_graphormer import GraphormerConfig
logger = logging.get_logger(__name__)
_CHECKPOINT_FOR_DOC = "graphormer-base-pcqm4mv1"
_CONFIG_FOR_DOC = "GraphormerConfig"
GRAPHORMER_PRETRAINED_MODEL_ARCHIVE_LIST = [
"clefourrier/graphormer-base-pcqm4mv1",
"clefourrier/graphormer-base-pcqm4mv2",
# See all Graphormer models at https://huggingface.co/models?filter=graphormer
]
def quant_noise(module: nn.Module, p: float, block_size: int):
"""
From:
https://github.com/facebookresearch/fairseq/blob/dd0079bde7f678b0cd0715cbd0ae68d661b7226d/fairseq/modules/quant_noise.py
Wraps modules and applies quantization noise to the weights for subsequent quantization with Iterative Product
Quantization as described in "Training with Quantization Noise for Extreme Model Compression"
Args:
- module: nn.Module
- p: amount of Quantization Noise
- block_size: size of the blocks for subsequent quantization with iPQ
Remarks:
- Module weights must have the right sizes wrt the block size
- Only Linear, Embedding and Conv2d modules are supported for the moment
- For more detail on how to quantize by blocks with convolutional weights, see "And the Bit Goes Down:
Revisiting the Quantization of Neural Networks"
- We implement the simplest form of noise here as stated in the paper which consists in randomly dropping
blocks
"""
# if no quantization noise, don't register hook
if p <= 0:
return module
# supported modules
if not isinstance(module, (nn.Linear, nn.Embedding, nn.Conv2d)):
raise NotImplementedError("Module unsupported for quant_noise.")
# test whether module.weight has the right sizes wrt block_size
is_conv = module.weight.ndim == 4
# 2D matrix
if not is_conv:
if module.weight.size(1) % block_size != 0:
raise AssertionError("Input features must be a multiple of block sizes")
# 4D matrix
else:
# 1x1 convolutions
if module.kernel_size == (1, 1):
if module.in_channels % block_size != 0:
raise AssertionError("Input channels must be a multiple of block sizes")
# regular convolutions
else:
k = module.kernel_size[0] * module.kernel_size[1]
if k % block_size != 0:
raise AssertionError("Kernel size must be a multiple of block size")
def _forward_pre_hook(mod, input):
# no noise for evaluation
if mod.training:
if not is_conv:
# gather weight and sizes
weight = mod.weight
in_features = weight.size(1)
out_features = weight.size(0)
# split weight matrix into blocks and randomly drop selected blocks
mask = torch.zeros(in_features // block_size * out_features, device=weight.device)
mask.bernoulli_(p)
mask = mask.repeat_interleave(block_size, -1).view(-1, in_features)
else:
# gather weight and sizes
weight = mod.weight
in_channels = mod.in_channels
out_channels = mod.out_channels
# split weight matrix into blocks and randomly drop selected blocks
if mod.kernel_size == (1, 1):
mask = torch.zeros(
int(in_channels // block_size * out_channels),
device=weight.device,
)
mask.bernoulli_(p)
mask = mask.repeat_interleave(block_size, -1).view(-1, in_channels)
else:
mask = torch.zeros(weight.size(0), weight.size(1), device=weight.device)
mask.bernoulli_(p)
mask = mask.unsqueeze(2).unsqueeze(3).repeat(1, 1, mod.kernel_size[0], mod.kernel_size[1])
# scale weights and apply mask
mask = mask.to(torch.bool) # x.bool() is not currently supported in TorchScript
s = 1 / (1 - p)
mod.weight.data = s * weight.masked_fill(mask, 0)
module.register_forward_pre_hook(_forward_pre_hook)
return module
class LayerDropModuleList(nn.ModuleList):
"""
From:
https://github.com/facebookresearch/fairseq/blob/dd0079bde7f678b0cd0715cbd0ae68d661b7226d/fairseq/modules/layer_drop.py
A LayerDrop implementation based on [`torch.nn.ModuleList`]. LayerDrop as described in
https://arxiv.org/abs/1909.11556.
We refresh the choice of which layers to drop every time we iterate over the LayerDropModuleList instance. During
evaluation we always iterate over all layers.
Usage:
```python
layers = LayerDropList(p=0.5, modules=[layer1, layer2, layer3])
for layer in layers: # this might iterate over layers 1 and 3
x = layer(x)
for layer in layers: # this might iterate over all layers
x = layer(x)
for layer in layers: # this might not iterate over any layers
x = layer(x)
```
Args:
p (float): probability of dropping out each layer
modules (iterable, optional): an iterable of modules to add
"""
def __init__(self, p: float, modules: Optional[Iterable[nn.Module]] = None):
super().__init__(modules)
self.p = p
def __iter__(self) -> Iterator[nn.Module]:
dropout_probs = torch.empty(len(self)).uniform_()
for i, m in enumerate(super().__iter__()):
if not self.training or (dropout_probs[i] > self.p):
yield m
class GraphormerGraphNodeFeature(nn.Module):
"""
Compute node features for each node in the graph.
"""
def __init__(self, config: GraphormerConfig):
super().__init__()
self.num_heads = config.num_attention_heads
self.num_atoms = config.num_atoms
self.atom_encoder = nn.Embedding(config.num_atoms + 1, config.hidden_size, padding_idx=config.pad_token_id)
self.in_degree_encoder = nn.Embedding(
config.num_in_degree, config.hidden_size, padding_idx=config.pad_token_id
)
self.out_degree_encoder = nn.Embedding(
config.num_out_degree, config.hidden_size, padding_idx=config.pad_token_id
)
self.graph_token = nn.Embedding(1, config.hidden_size)
def forward(
self,
input_nodes: torch.LongTensor,
in_degree: torch.LongTensor,
out_degree: torch.LongTensor,
) -> torch.Tensor:
n_graph, n_node = input_nodes.size()[:2]
node_feature = ( # node feature + graph token
self.atom_encoder(input_nodes).sum(dim=-2) # [n_graph, n_node, n_hidden]
+ self.in_degree_encoder(in_degree)
+ self.out_degree_encoder(out_degree)
)
graph_token_feature = self.graph_token.weight.unsqueeze(0).repeat(n_graph, 1, 1)
graph_node_feature = torch.cat([graph_token_feature, node_feature], dim=1)
return graph_node_feature
class GraphormerGraphAttnBias(nn.Module):
"""
Compute attention bias for each head.
"""
def __init__(self, config: GraphormerConfig):
super().__init__()
self.num_heads = config.num_attention_heads
self.multi_hop_max_dist = config.multi_hop_max_dist
# We do not change edge feature embedding learning, as edge embeddings are represented as a combination of the original features
# + shortest path
self.edge_encoder = nn.Embedding(config.num_edges + 1, config.num_attention_heads, padding_idx=0)
self.edge_type = config.edge_type
if self.edge_type == "multi_hop":
self.edge_dis_encoder = nn.Embedding(
config.num_edge_dis * config.num_attention_heads * config.num_attention_heads,
1,
)
self.spatial_pos_encoder = nn.Embedding(config.num_spatial, config.num_attention_heads, padding_idx=0)
self.graph_token_virtual_distance = nn.Embedding(1, config.num_attention_heads)
def forward(
self,
input_nodes: torch.LongTensor,
attn_bias: torch.Tensor,
spatial_pos: torch.LongTensor,
input_edges: torch.LongTensor,
attn_edge_type: torch.LongTensor,
) -> torch.Tensor:
n_graph, n_node = input_nodes.size()[:2]
graph_attn_bias = attn_bias.clone()
graph_attn_bias = graph_attn_bias.unsqueeze(1).repeat(
1, self.num_heads, 1, 1
) # [n_graph, n_head, n_node+1, n_node+1]
# spatial pos
# [n_graph, n_node, n_node, n_head] -> [n_graph, n_head, n_node, n_node]
spatial_pos_bias = self.spatial_pos_encoder(spatial_pos).permute(0, 3, 1, 2)
graph_attn_bias[:, :, 1:, 1:] = graph_attn_bias[:, :, 1:, 1:] + spatial_pos_bias
# reset spatial pos here
t = self.graph_token_virtual_distance.weight.view(1, self.num_heads, 1)
graph_attn_bias[:, :, 1:, 0] = graph_attn_bias[:, :, 1:, 0] + t
graph_attn_bias[:, :, 0, :] = graph_attn_bias[:, :, 0, :] + t
# edge feature
if self.edge_type == "multi_hop":
spatial_pos_ = spatial_pos.clone()
spatial_pos_[spatial_pos_ == 0] = 1 # set pad to 1
# set 1 to 1, input_nodes > 1 to input_nodes - 1
spatial_pos_ = torch.where(spatial_pos_ > 1, spatial_pos_ - 1, spatial_pos_)
if self.multi_hop_max_dist > 0:
spatial_pos_ = spatial_pos_.clamp(0, self.multi_hop_max_dist)
input_edges = input_edges[:, :, :, : self.multi_hop_max_dist, :]
# [n_graph, n_node, n_node, max_dist, n_head]
input_edges = self.edge_encoder(input_edges).mean(-2)
max_dist = input_edges.size(-2)
edge_input_flat = input_edges.permute(3, 0, 1, 2, 4).reshape(max_dist, -1, self.num_heads)
edge_input_flat = torch.bmm(
edge_input_flat,
self.edge_dis_encoder.weight.reshape(-1, self.num_heads, self.num_heads)[:max_dist, :, :],
)
input_edges = edge_input_flat.reshape(max_dist, n_graph, n_node, n_node, self.num_heads).permute(
1, 2, 3, 0, 4
)
input_edges = (input_edges.sum(-2) / (spatial_pos_.float().unsqueeze(-1))).permute(0, 3, 1, 2)
else:
# [n_graph, n_node, n_node, n_head] -> [n_graph, n_head, n_node, n_node]
input_edges = self.edge_encoder(attn_edge_type).mean(-2).permute(0, 3, 1, 2)
graph_attn_bias[:, :, 1:, 1:] = graph_attn_bias[:, :, 1:, 1:] + input_edges
graph_attn_bias = graph_attn_bias + attn_bias.unsqueeze(1) # reset
return graph_attn_bias
class GraphormerMultiheadAttention(nn.Module):
"""Multi-headed attention.
See "Attention Is All You Need" for more details.
"""
def __init__(self, config: GraphormerConfig):
super().__init__()
self.embedding_dim = config.embedding_dim
self.kdim = config.kdim if config.kdim is not None else config.embedding_dim
self.vdim = config.vdim if config.vdim is not None else config.embedding_dim
self.qkv_same_dim = self.kdim == config.embedding_dim and self.vdim == config.embedding_dim
self.num_heads = config.num_attention_heads
self.attention_dropout_module = torch.nn.Dropout(p=config.attention_dropout, inplace=False)
self.head_dim = config.embedding_dim // config.num_attention_heads
if not (self.head_dim * config.num_attention_heads == self.embedding_dim):
raise AssertionError("The embedding_dim must be divisible by num_heads.")
self.scaling = self.head_dim**-0.5
self.self_attention = True # config.self_attention
if not (self.self_attention):
raise NotImplementedError("The Graphormer model only supports self attention for now.")
if self.self_attention and not self.qkv_same_dim:
raise AssertionError("Self-attention requires query, key and value to be of the same size.")
self.k_proj = quant_noise(
nn.Linear(self.kdim, config.embedding_dim, bias=config.bias),
config.q_noise,
config.qn_block_size,
)
self.v_proj = quant_noise(
nn.Linear(self.vdim, config.embedding_dim, bias=config.bias),
config.q_noise,
config.qn_block_size,
)
self.q_proj = quant_noise(
nn.Linear(config.embedding_dim, config.embedding_dim, bias=config.bias),
config.q_noise,
config.qn_block_size,
)
self.out_proj = quant_noise(
nn.Linear(config.embedding_dim, config.embedding_dim, bias=config.bias),
config.q_noise,
config.qn_block_size,
)
self.onnx_trace = False
def reset_parameters(self):
if self.qkv_same_dim:
# Empirically observed the convergence to be much better with
# the scaled initialization
nn.init.xavier_uniform_(self.k_proj.weight, gain=1 / math.sqrt(2))
nn.init.xavier_uniform_(self.v_proj.weight, gain=1 / math.sqrt(2))
nn.init.xavier_uniform_(self.q_proj.weight, gain=1 / math.sqrt(2))
else:
nn.init.xavier_uniform_(self.k_proj.weight)
nn.init.xavier_uniform_(self.v_proj.weight)
nn.init.xavier_uniform_(self.q_proj.weight)
nn.init.xavier_uniform_(self.out_proj.weight)
if self.out_proj.bias is not None:
nn.init.constant_(self.out_proj.bias, 0.0)
def forward(
self,
query: torch.LongTensor,
key: Optional[torch.Tensor],
value: Optional[torch.Tensor],
attn_bias: Optional[torch.Tensor],
key_padding_mask: Optional[torch.Tensor] = None,
need_weights: bool = True,
attn_mask: Optional[torch.Tensor] = None,
before_softmax: bool = False,
need_head_weights: bool = False,
) -> Tuple[torch.Tensor, Optional[torch.Tensor]]:
"""
Args:
key_padding_mask (Bytetorch.Tensor, optional): mask to exclude
keys that are pads, of shape `(batch, src_len)`, where padding elements are indicated by 1s.
need_weights (bool, optional): return the attention weights,
averaged over heads (default: False).
attn_mask (Bytetorch.Tensor, optional): typically used to
implement causal attention, where the mask prevents the attention from looking forward in time
(default: None).
before_softmax (bool, optional): return the raw attention
weights and values before the attention softmax.
need_head_weights (bool, optional): return the attention
weights for each head. Implies *need_weights*. Default: return the average attention weights over all
heads.
"""
if need_head_weights:
need_weights = True
tgt_len, bsz, embedding_dim = query.size()
src_len = tgt_len
if not (embedding_dim == self.embedding_dim):
raise AssertionError(
f"The query embedding dimension {embedding_dim} is not equal to the expected embedding_dim"
f" {self.embedding_dim}."
)
if not (list(query.size()) == [tgt_len, bsz, embedding_dim]):
raise AssertionError("Query size incorrect in Graphormer, compared to model dimensions.")
if key is not None:
src_len, key_bsz, _ = key.size()
if not torch.jit.is_scripting():
if (key_bsz != bsz) or (value is None) or not (src_len, bsz == value.shape[:2]):
raise AssertionError(
"The batch shape does not match the key or value shapes provided to the attention."
)
q = self.q_proj(query)
k = self.k_proj(query)
v = self.v_proj(query)
q *= self.scaling
q = q.contiguous().view(tgt_len, bsz * self.num_heads, self.head_dim).transpose(0, 1)
if k is not None:
k = k.contiguous().view(-1, bsz * self.num_heads, self.head_dim).transpose(0, 1)
if v is not None:
v = v.contiguous().view(-1, bsz * self.num_heads, self.head_dim).transpose(0, 1)
if (k is None) or not (k.size(1) == src_len):
raise AssertionError("The shape of the key generated in the attention is incorrect")
# This is part of a workaround to get around fork/join parallelism
# not supporting Optional types.
if key_padding_mask is not None and key_padding_mask.dim() == 0:
key_padding_mask = None
if key_padding_mask is not None:
if key_padding_mask.size(0) != bsz or key_padding_mask.size(1) != src_len:
raise AssertionError(
"The shape of the generated padding mask for the key does not match expected dimensions."
)
attn_weights = torch.bmm(q, k.transpose(1, 2))
attn_weights = self.apply_sparse_mask(attn_weights, tgt_len, src_len, bsz)
if list(attn_weights.size()) != [bsz * self.num_heads, tgt_len, src_len]:
raise AssertionError("The attention weights generated do not match the expected dimensions.")
if attn_bias is not None:
attn_weights += attn_bias.view(bsz * self.num_heads, tgt_len, src_len)
if attn_mask is not None:
attn_mask = attn_mask.unsqueeze(0)
attn_weights += attn_mask
if key_padding_mask is not None:
# don't attend to padding symbols
attn_weights = attn_weights.view(bsz, self.num_heads, tgt_len, src_len)
attn_weights = attn_weights.masked_fill(
key_padding_mask.unsqueeze(1).unsqueeze(2).to(torch.bool), float("-inf")
)
attn_weights = attn_weights.view(bsz * self.num_heads, tgt_len, src_len)
if before_softmax:
return attn_weights, v
attn_weights_float = torch.nn.functional.softmax(attn_weights, dim=-1)
attn_weights = attn_weights_float.type_as(attn_weights)
attn_probs = self.attention_dropout_module(attn_weights)
if v is None:
raise AssertionError("No value generated")
attn = torch.bmm(attn_probs, v)
if list(attn.size()) != [bsz * self.num_heads, tgt_len, self.head_dim]:
raise AssertionError("The attention generated do not match the expected dimensions.")
attn = attn.transpose(0, 1).contiguous().view(tgt_len, bsz, embedding_dim)
attn: torch.Tensor = self.out_proj(attn)
attn_weights = None
if need_weights:
attn_weights = attn_weights_float.contiguous().view(bsz, self.num_heads, tgt_len, src_len).transpose(1, 0)
if not need_head_weights:
# average attention weights over heads
attn_weights = attn_weights.mean(dim=0)
return attn, attn_weights
def apply_sparse_mask(self, attn_weights: torch.Tensor, tgt_len: int, src_len: int, bsz: int) -> torch.Tensor:
return attn_weights
class GraphormerGraphEncoderLayer(nn.Module):
def __init__(self, config: GraphormerConfig) -> None:
super().__init__()
# Initialize parameters
self.embedding_dim = config.embedding_dim
self.num_attention_heads = config.num_attention_heads
self.q_noise = config.q_noise
self.qn_block_size = config.qn_block_size
self.pre_layernorm = config.pre_layernorm
self.dropout_module = torch.nn.Dropout(p=config.dropout, inplace=False)
self.activation_dropout_module = torch.nn.Dropout(p=config.activation_dropout, inplace=False)
# Initialize blocks
self.activation_fn = ACT2FN[config.activation_fn]
self.self_attn = GraphormerMultiheadAttention(config)
# layer norm associated with the self attention layer
self.self_attn_layer_norm = nn.LayerNorm(self.embedding_dim)
self.fc1 = self.build_fc(
self.embedding_dim,
config.ffn_embedding_dim,
q_noise=config.q_noise,
qn_block_size=config.qn_block_size,
)
self.fc2 = self.build_fc(
config.ffn_embedding_dim,
self.embedding_dim,
q_noise=config.q_noise,
qn_block_size=config.qn_block_size,
)
# layer norm associated with the position wise feed-forward NN
self.final_layer_norm = nn.LayerNorm(self.embedding_dim)
def build_fc(
self, input_dim: int, output_dim: int, q_noise: float, qn_block_size: int
) -> Union[nn.Module, nn.Linear, nn.Embedding, nn.Conv2d]:
return quant_noise(nn.Linear(input_dim, output_dim), q_noise, qn_block_size)
def forward(
self,
input_nodes: torch.Tensor,
self_attn_bias: Optional[torch.Tensor] = None,
self_attn_mask: Optional[torch.Tensor] = None,
self_attn_padding_mask: Optional[torch.Tensor] = None,
) -> Tuple[torch.Tensor, Optional[torch.Tensor]]:
"""
nn.LayerNorm is applied either before or after the self-attention/ffn modules similar to the original
Transformer implementation.
"""
residual = input_nodes
if self.pre_layernorm:
input_nodes = self.self_attn_layer_norm(input_nodes)
input_nodes, attn = self.self_attn(
query=input_nodes,
key=input_nodes,
value=input_nodes,
attn_bias=self_attn_bias,
key_padding_mask=self_attn_padding_mask,
need_weights=False,
attn_mask=self_attn_mask,
)
input_nodes = self.dropout_module(input_nodes)
input_nodes = residual + input_nodes
if not self.pre_layernorm:
input_nodes = self.self_attn_layer_norm(input_nodes)
residual = input_nodes
if self.pre_layernorm:
input_nodes = self.final_layer_norm(input_nodes)
input_nodes = self.activation_fn(self.fc1(input_nodes))
input_nodes = self.activation_dropout_module(input_nodes)
input_nodes = self.fc2(input_nodes)
input_nodes = self.dropout_module(input_nodes)
input_nodes = residual + input_nodes
if not self.pre_layernorm:
input_nodes = self.final_layer_norm(input_nodes)
return input_nodes, attn
class GraphormerGraphEncoder(nn.Module):
def __init__(self, config: GraphormerConfig):
super().__init__()
self.dropout_module = torch.nn.Dropout(p=config.dropout, inplace=False)
self.layerdrop = config.layerdrop
self.embedding_dim = config.embedding_dim
self.apply_graphormer_init = config.apply_graphormer_init
self.traceable = config.traceable
self.graph_node_feature = GraphormerGraphNodeFeature(config)
self.graph_attn_bias = GraphormerGraphAttnBias(config)
self.embed_scale = config.embed_scale
if config.q_noise > 0:
self.quant_noise = quant_noise(
nn.Linear(self.embedding_dim, self.embedding_dim, bias=False),
config.q_noise,
config.qn_block_size,
)
else:
self.quant_noise = None
if config.encoder_normalize_before:
self.emb_layer_norm = nn.LayerNorm(self.embedding_dim)
else:
self.emb_layer_norm = None
if config.pre_layernorm:
self.final_layer_norm = nn.LayerNorm(self.embedding_dim)
if self.layerdrop > 0.0:
self.layers = LayerDropModuleList(p=self.layerdrop)
else:
self.layers = nn.ModuleList([])
self.layers.extend([GraphormerGraphEncoderLayer(config) for _ in range(config.num_hidden_layers)])
# Apply initialization of model params after building the model
if config.freeze_embeddings:
raise NotImplementedError("Freezing embeddings is not implemented yet.")
for layer in range(config.num_trans_layers_to_freeze):
m = self.layers[layer]
if m is not None:
for p in m.parameters():
p.requires_grad = False
def forward(
self,
input_nodes: torch.LongTensor,
input_edges: torch.LongTensor,
attn_bias: torch.Tensor,
in_degree: torch.LongTensor,
out_degree: torch.LongTensor,
spatial_pos: torch.LongTensor,
attn_edge_type: torch.LongTensor,
perturb=None,
last_state_only: bool = False,
token_embeddings: Optional[torch.Tensor] = None,
attn_mask: Optional[torch.Tensor] = None,
) -> Tuple[Union[torch.Tensor, List[torch.LongTensor]], torch.Tensor]:
# compute padding mask. This is needed for multi-head attention
data_x = input_nodes
n_graph, n_node = data_x.size()[:2]
padding_mask = (data_x[:, :, 0]).eq(0)
padding_mask_cls = torch.zeros(n_graph, 1, device=padding_mask.device, dtype=padding_mask.dtype)
padding_mask = torch.cat((padding_mask_cls, padding_mask), dim=1)
attn_bias = self.graph_attn_bias(input_nodes, attn_bias, spatial_pos, input_edges, attn_edge_type)
if token_embeddings is not None:
input_nodes = token_embeddings
else:
input_nodes = self.graph_node_feature(input_nodes, in_degree, out_degree)
if perturb is not None:
input_nodes[:, 1:, :] += perturb
if self.embed_scale is not None:
input_nodes = input_nodes * self.embed_scale
if self.quant_noise is not None:
input_nodes = self.quant_noise(input_nodes)
if self.emb_layer_norm is not None:
input_nodes = self.emb_layer_norm(input_nodes)
input_nodes = self.dropout_module(input_nodes)
input_nodes = input_nodes.transpose(0, 1)
inner_states = []
if not last_state_only:
inner_states.append(input_nodes)
for layer in self.layers:
input_nodes, _ = layer(
input_nodes,
self_attn_padding_mask=padding_mask,
self_attn_mask=attn_mask,
self_attn_bias=attn_bias,
)
if not last_state_only:
inner_states.append(input_nodes)
graph_rep = input_nodes[0, :, :]
if last_state_only:
inner_states = [input_nodes]
if self.traceable:
return torch.stack(inner_states), graph_rep
else:
return inner_states, graph_rep
class GraphormerDecoderHead(nn.Module):
def __init__(self, embedding_dim: int, num_classes: int):
super().__init__()
"""num_classes should be 1 for regression, or the number of classes for classification"""
self.lm_output_learned_bias = nn.Parameter(torch.zeros(1))
self.classifier = nn.Linear(embedding_dim, num_classes, bias=False)
self.num_classes = num_classes
def forward(self, input_nodes: torch.Tensor, **unused) -> torch.Tensor:
input_nodes = self.classifier(input_nodes)
input_nodes = input_nodes + self.lm_output_learned_bias
return input_nodes
class GraphormerPreTrainedModel(PreTrainedModel):
"""
An abstract class to handle weights initialization and a simple interface for downloading and loading pretrained
models.
"""
config_class = GraphormerConfig
base_model_prefix = "graphormer"
main_input_name_nodes = "input_nodes"
main_input_name_edges = "input_edges"
def normal_(self, data: torch.Tensor):
# with FSDP, module params will be on CUDA, so we cast them back to CPU
# so that the RNG is consistent with and without FSDP
data.copy_(data.cpu().normal_(mean=0.0, std=0.02).to(data.device))
def init_graphormer_params(self, module: Union[nn.Linear, nn.Embedding, GraphormerMultiheadAttention]):
"""
Initialize the weights specific to the Graphormer Model.
"""
if isinstance(module, nn.Linear):
self.normal_(module.weight.data)
if module.bias is not None:
module.bias.data.zero_()
if isinstance(module, nn.Embedding):
self.normal_(module.weight.data)
if module.padding_idx is not None:
module.weight.data[module.padding_idx].zero_()
if isinstance(module, GraphormerMultiheadAttention):
self.normal_(module.q_proj.weight.data)
self.normal_(module.k_proj.weight.data)
self.normal_(module.v_proj.weight.data)
def _init_weights(
self,
module: Union[
nn.Linear, nn.Conv2d, nn.Embedding, nn.LayerNorm, GraphormerMultiheadAttention, GraphormerGraphEncoder
],
):
"""
Initialize the weights
"""
if isinstance(module, (nn.Linear, nn.Conv2d)):
# We might be missing part of the Linear init, dependant on the layer num
module.weight.data.normal_(mean=0.0, std=0.02)
if module.bias is not None:
module.bias.data.zero_()
elif isinstance(module, nn.Embedding):
module.weight.data.normal_(mean=0.0, std=0.02)
if module.padding_idx is not None:
module.weight.data[module.padding_idx].zero_()
elif isinstance(module, GraphormerMultiheadAttention):
module.q_proj.weight.data.normal_(mean=0.0, std=0.02)
module.k_proj.weight.data.normal_(mean=0.0, std=0.02)
module.v_proj.weight.data.normal_(mean=0.0, std=0.02)
module.reset_parameters()
elif isinstance(module, nn.LayerNorm):
module.bias.data.zero_()
module.weight.data.fill_(1.0)
elif isinstance(module, GraphormerGraphEncoder):
if module.apply_graphormer_init:
module.apply(self.init_graphormer_params)
elif isinstance(module, nn.LayerNorm):
module.bias.data.zero_()
module.weight.data.fill_(1.0)
class GraphormerModel(GraphormerPreTrainedModel):
"""The Graphormer model is a graph-encoder model.
It goes from a graph to its representation. If you want to use the model for a downstream classification task, use
GraphormerForGraphClassification instead. For any other downstream task, feel free to add a new class, or combine
this model with a downstream model of your choice, following the example in GraphormerForGraphClassification.
"""
def __init__(self, config: GraphormerConfig):
super().__init__(config)
self.max_nodes = config.max_nodes
self.graph_encoder = GraphormerGraphEncoder(config)
self.share_input_output_embed = config.share_input_output_embed
self.lm_output_learned_bias = None
# Remove head is set to true during fine-tuning
self.load_softmax = not getattr(config, "remove_head", False)
self.lm_head_transform_weight = nn.Linear(config.embedding_dim, config.embedding_dim)
self.activation_fn = ACT2FN[config.activation_fn]
self.layer_norm = nn.LayerNorm(config.embedding_dim)
self.post_init()
def reset_output_layer_parameters(self):
self.lm_output_learned_bias = nn.Parameter(torch.zeros(1))
def forward(
self,
input_nodes: torch.LongTensor,
input_edges: torch.LongTensor,
attn_bias: torch.Tensor,
in_degree: torch.LongTensor,
out_degree: torch.LongTensor,
spatial_pos: torch.LongTensor,
attn_edge_type: torch.LongTensor,
perturb: Optional[torch.FloatTensor] = None,
masked_tokens: None = None,
return_dict: Optional[bool] = None,
**unused,
) -> Union[Tuple[torch.LongTensor], BaseModelOutputWithNoAttention]:
return_dict = return_dict if return_dict is not None else self.config.use_return_dict
inner_states, graph_rep = self.graph_encoder(
input_nodes, input_edges, attn_bias, in_degree, out_degree, spatial_pos, attn_edge_type, perturb=perturb
)
# last inner state, then revert Batch and Graph len
input_nodes = inner_states[-1].transpose(0, 1)
# project masked tokens only
if masked_tokens is not None:
raise NotImplementedError
input_nodes = self.layer_norm(self.activation_fn(self.lm_head_transform_weight(input_nodes)))
# project back to size of vocabulary
if self.share_input_output_embed and hasattr(self.graph_encoder.embed_tokens, "weight"):
input_nodes = torch.nn.functional.linear(input_nodes, self.graph_encoder.embed_tokens.weight)
if not return_dict:
return tuple(x for x in [input_nodes, inner_states] if x is not None)
return BaseModelOutputWithNoAttention(last_hidden_state=input_nodes, hidden_states=inner_states)
def max_nodes(self):
"""Maximum output length supported by the encoder."""
return self.max_nodes
class GraphormerForGraphClassification(GraphormerPreTrainedModel):
"""
This model can be used for graph-level classification or regression tasks.
It can be trained on
- regression (by setting config.num_classes to 1); there should be one float-type label per graph
- one task classification (by setting config.num_classes to the number of classes); there should be one integer
label per graph
- binary multi-task classification (by setting config.num_classes to the number of labels); there should be a list
of integer labels for each graph.
"""
def __init__(self, config: GraphormerConfig):
super().__init__(config)
self.encoder = GraphormerModel(config)
self.embedding_dim = config.embedding_dim
self.num_classes = config.num_classes
self.classifier = GraphormerDecoderHead(self.embedding_dim, self.num_classes)
self.is_encoder_decoder = True
# Initialize weights and apply final processing
self.post_init()
def forward(
self,
input_nodes: torch.LongTensor,
input_edges: torch.LongTensor,
attn_bias: torch.Tensor,
in_degree: torch.LongTensor,
out_degree: torch.LongTensor,
spatial_pos: torch.LongTensor,
attn_edge_type: torch.LongTensor,
labels: Optional[torch.LongTensor] = None,
return_dict: Optional[bool] = None,
**unused,
) -> Union[Tuple[torch.Tensor], SequenceClassifierOutput]:
return_dict = return_dict if return_dict is not None else self.config.use_return_dict
encoder_outputs = self.encoder(
input_nodes,
input_edges,
attn_bias,
in_degree,
out_degree,
spatial_pos,
attn_edge_type,
return_dict=True,
)
outputs, hidden_states = encoder_outputs["last_hidden_state"], encoder_outputs["hidden_states"]
head_outputs = self.classifier(outputs)
logits = head_outputs[:, 0, :].contiguous()
loss = None
if labels is not None:
mask = ~torch.isnan(labels)
if self.num_classes == 1: # regression
loss_fct = MSELoss()
loss = loss_fct(logits[mask].squeeze(), labels[mask].squeeze().float())
elif self.num_classes > 1 and len(labels.shape) == 1: # One task classification
loss_fct = CrossEntropyLoss()
loss = loss_fct(logits[mask].view(-1, self.num_classes), labels[mask].view(-1))
else: # Binary multi-task classification
loss_fct = BCEWithLogitsLoss(reduction="sum")
loss = loss_fct(logits[mask], labels[mask])
if not return_dict:
return tuple(x for x in [loss, logits, hidden_states] if x is not None)
return SequenceClassifierOutput(loss=loss, logits=logits, hidden_states=hidden_states, attentions=None)
| transformers/src/transformers/models/graphormer/modeling_graphormer.py/0 | {
"file_path": "transformers/src/transformers/models/graphormer/modeling_graphormer.py",
"repo_id": "transformers",
"token_count": 16728
} | 336 |
# coding=utf-8
# Copyright 2021 The OpenAI Team Authors and HuggingFace Inc. team.
#
# Licensed under the Apache License, Version 2.0 (the "License");
# you may not use this file except in compliance with the License.
# You may obtain a copy of the License at
#
# http://www.apache.org/licenses/LICENSE-2.0
#
# Unless required by applicable law or agreed to in writing, software
# distributed under the License is distributed on an "AS IS" BASIS,
# WITHOUT WARRANTIES OR CONDITIONS OF ANY KIND, either express or implied.
# See the License for the specific language governing permissions and
# limitations under the License.
"""PyTorch OpenAI ImageGPT model."""
import math
import os
import warnings
from typing import Any, Optional, Tuple, Union
import torch
import torch.utils.checkpoint
from torch import nn
from torch.cuda.amp import autocast
from torch.nn import BCEWithLogitsLoss, CrossEntropyLoss, MSELoss
from ...activations import ACT2FN
from ...modeling_outputs import (
BaseModelOutputWithPastAndCrossAttentions,
CausalLMOutputWithCrossAttentions,
SequenceClassifierOutputWithPast,
)
from ...modeling_utils import PreTrainedModel
from ...pytorch_utils import Conv1D, find_pruneable_heads_and_indices, prune_conv1d_layer
from ...utils import add_start_docstrings, add_start_docstrings_to_model_forward, logging, replace_return_docstrings
from .configuration_imagegpt import ImageGPTConfig
logger = logging.get_logger(__name__)
_CHECKPOINT_FOR_DOC = "openai/imagegpt-small"
_CONFIG_FOR_DOC = "ImageGPTConfig"
IMAGEGPT_PRETRAINED_MODEL_ARCHIVE_LIST = [
"openai/imagegpt-small",
"openai/imagegpt-medium",
"openai/imagegpt-large",
# See all Image GPT models at https://huggingface.co/models?filter=imagegpt
]
def load_tf_weights_in_imagegpt(model, config, imagegpt_checkpoint_path):
"""
Load tf checkpoints in a pytorch model
"""
try:
import re
import tensorflow as tf
except ImportError:
logger.error(
"Loading a TensorFlow model in PyTorch, requires TensorFlow to be installed. Please see "
"https://www.tensorflow.org/install/ for installation instructions."
)
raise
tf_path = os.path.abspath(imagegpt_checkpoint_path)
logger.info("Converting TensorFlow checkpoint from {}".format(tf_path))
# Load weights from TF model
init_vars = tf.train.list_variables(tf_path)
names = []
arrays = []
for name, shape in init_vars:
logger.info("Loading TF weight {} with shape {}".format(name, shape))
array = tf.train.load_variable(tf_path, name)
names.append(name)
arrays.append(array.squeeze())
for name, array in zip(names, arrays):
name = name[6:] # skip "model/"
name = name.split("/")
# adam_v and adam_m are variables used in AdamWeightDecayOptimizer to calculated m and v
# which are not required for using pretrained model
if any(
n in ["adam_v", "adam_m", "AdamWeightDecayOptimizer", "AdamWeightDecayOptimizer_1", "global_step"]
for n in name
) or name[-1] in ["_step"]:
logger.info("Skipping {}".format("/".join(name)))
continue
pointer = model
if name[-1] not in ["wtet"]:
pointer = getattr(pointer, "transformer")
for m_name in name:
if re.fullmatch(r"[A-Za-z]+\d+", m_name):
scope_names = re.split(r"(\d+)", m_name)
else:
scope_names = [m_name]
if scope_names[0] == "w" or scope_names[0] == "g":
pointer = getattr(pointer, "weight")
elif scope_names[0] == "b":
pointer = getattr(pointer, "bias")
elif scope_names[0] == "wpe" or scope_names[0] == "wte":
pointer = getattr(pointer, scope_names[0])
pointer = getattr(pointer, "weight")
elif scope_names[0] in ["q_proj", "k_proj", "v_proj"]:
pointer = getattr(pointer, "c_attn")
pointer = getattr(pointer, "weight")
elif len(name) == 3 and name[1] == "attn" and scope_names[0] == "c_proj":
pointer = getattr(pointer, scope_names[0])
pointer = getattr(pointer, "weight")
elif scope_names[0] == "wtet":
pointer = getattr(pointer, "lm_head")
pointer = getattr(pointer, "weight")
elif scope_names[0] == "sos":
pointer = getattr(pointer, "wte")
pointer = getattr(pointer, "weight")
else:
pointer = getattr(pointer, scope_names[0])
if len(scope_names) >= 2:
num = int(scope_names[1])
pointer = pointer[num]
if len(name) > 1 and name[1] == "attn" or name[-1] == "wtet" or name[-1] == "sos" or name[-1] == "wte":
pass # array is used to initialize only part of the pointer so sizes won't match
else:
try:
assert pointer.shape == array.shape
except AssertionError as e:
e.args += (pointer.shape, array.shape)
raise
logger.info("Initialize PyTorch weight {}".format(name))
if name[-1] == "q_proj":
pointer.data[:, : config.n_embd] = torch.from_numpy(array.reshape(config.n_embd, config.n_embd)).T
elif name[-1] == "k_proj":
pointer.data[:, config.n_embd : 2 * config.n_embd] = torch.from_numpy(
array.reshape(config.n_embd, config.n_embd)
).T
elif name[-1] == "v_proj":
pointer.data[:, 2 * config.n_embd :] = torch.from_numpy(array.reshape(config.n_embd, config.n_embd)).T
elif len(name) == 3 and name[1] == "attn" and name[2] == "c_proj":
pointer.data = torch.from_numpy(array.reshape(config.n_embd, config.n_embd))
elif name[-1] == "wtet":
pointer.data = torch.from_numpy(array)
elif name[-1] == "wte":
pointer.data[: config.vocab_size - 1, :] = torch.from_numpy(array)
elif name[-1] == "sos":
pointer.data[-1] = torch.from_numpy(array)
else:
pointer.data = torch.from_numpy(array)
return model
class ImageGPTLayerNorm(nn.Module):
def __init__(self, hidden_size: Tuple[int], eps: float = 1e-5):
super().__init__()
self.eps = eps
self.weight = nn.Parameter(torch.Tensor(hidden_size))
def forward(self, tensor: torch.Tensor) -> tuple:
# input is not mean centered
return (
tensor
/ torch.sqrt(torch.mean(torch.square(tensor), axis=-1, keepdim=True) + self.eps)
* self.weight.data[..., :]
)
class ImageGPTAttention(nn.Module):
def __init__(self, config, is_cross_attention: Optional[bool] = False, layer_idx: Optional[int] = None):
super().__init__()
max_positions = config.max_position_embeddings
self.register_buffer(
"bias",
torch.tril(torch.ones((max_positions, max_positions), dtype=torch.bool)).view(
1, 1, max_positions, max_positions
),
persistent=False,
)
self.register_buffer("masked_bias", torch.tensor(-1e4), persistent=False)
self.embed_dim = config.hidden_size
self.num_heads = config.num_attention_heads
self.head_dim = self.embed_dim // self.num_heads
self.split_size = self.embed_dim
if self.head_dim * self.num_heads != self.embed_dim:
raise ValueError(
f"`embed_dim` must be divisible by num_heads (got `embed_dim`: {self.embed_dim} and `num_heads`:"
f" {self.num_heads})."
)
self.scale_attn_weights = config.scale_attn_weights
self.is_cross_attention = is_cross_attention
# Layer-wise attention scaling, reordering, and upcasting
self.scale_attn_by_inverse_layer_idx = config.scale_attn_by_inverse_layer_idx
self.layer_idx = layer_idx
self.reorder_and_upcast_attn = config.reorder_and_upcast_attn
if self.is_cross_attention:
self.c_attn = Conv1D(2 * self.embed_dim, self.embed_dim)
self.q_attn = Conv1D(self.embed_dim, self.embed_dim)
else:
self.c_attn = Conv1D(3 * self.embed_dim, self.embed_dim)
self.c_proj = Conv1D(self.embed_dim, self.embed_dim)
self.attn_dropout = nn.Dropout(config.attn_pdrop)
self.resid_dropout = nn.Dropout(config.resid_pdrop)
self.pruned_heads = set()
def prune_heads(self, heads):
if len(heads) == 0:
return
heads, index = find_pruneable_heads_and_indices(heads, self.num_heads, self.head_dim, self.pruned_heads)
index_attn = torch.cat([index, index + self.split_size, index + (2 * self.split_size)])
# Prune conv1d layers
self.c_attn = prune_conv1d_layer(self.c_attn, index_attn, dim=1)
self.c_proj = prune_conv1d_layer(self.c_proj, index, dim=0)
# Update hyper params
self.split_size = (self.split_size // self.num_heads) * (self.num_heads - len(heads))
self.num_heads = self.num_heads - len(heads)
self.pruned_heads = self.pruned_heads.union(heads)
def _attn(self, query, key, value, attention_mask=None, head_mask=None):
attn_weights = torch.matmul(query, key.transpose(-1, -2))
if self.scale_attn_weights:
attn_weights = attn_weights / (float(value.size(-1)) ** 0.5)
# Layer-wise attention scaling
if self.scale_attn_by_inverse_layer_idx:
attn_weights = attn_weights / float(self.layer_idx + 1)
if not self.is_cross_attention:
# if only "normal" attention layer implements causal mask
query_length, key_length = query.size(-2), key.size(-2)
causal_mask = self.bias[:, :, key_length - query_length : key_length, :key_length]
mask_value = torch.finfo(attn_weights.dtype).min
# Need to be a tensor, otherwise we get error: `RuntimeError: expected scalar type float but found double`.
# Need to be on the same device, otherwise `RuntimeError: ..., x and y to be on the same device`
mask_value = torch.tensor(mask_value, dtype=attn_weights.dtype).to(attn_weights.device)
attn_weights = torch.where(causal_mask, attn_weights, mask_value)
if attention_mask is not None:
# Apply the attention mask
attn_weights = attn_weights + attention_mask
attn_weights = nn.Softmax(dim=-1)(attn_weights)
# Downcast (if necessary) back to V's dtype (if in mixed-precision) -- No-Op otherwise
attn_weights = attn_weights.type(value.dtype)
attn_weights = self.attn_dropout(attn_weights)
# Mask heads if we want to
if head_mask is not None:
attn_weights = attn_weights * head_mask
attn_output = torch.matmul(attn_weights, value)
return attn_output, attn_weights
def _upcast_and_reordered_attn(self, query, key, value, attention_mask=None, head_mask=None):
# Use `torch.baddbmm` (a bit more efficient w/ alpha param for scaling -- from Megatron-LM)
bsz, num_heads, q_seq_len, dk = query.size()
_, _, k_seq_len, _ = key.size()
# Preallocate attn_weights for `baddbmm`
attn_weights = torch.empty(bsz * num_heads, q_seq_len, k_seq_len, dtype=torch.float32, device=query.device)
# Compute Scale Factor
scale_factor = 1.0
if self.scale_attn_weights:
scale_factor /= float(value.size(-1)) ** 0.5
if self.scale_attn_by_inverse_layer_idx:
scale_factor /= float(self.layer_idx + 1)
# Upcast (turn off autocast) and reorder (Scale K by 1 / root(dk))
with autocast(enabled=False):
q, k = query.reshape(-1, q_seq_len, dk), key.transpose(-1, -2).reshape(-1, dk, k_seq_len)
attn_weights = torch.baddbmm(attn_weights, q.float(), k.float(), beta=0, alpha=scale_factor)
attn_weights = attn_weights.reshape(bsz, num_heads, q_seq_len, k_seq_len)
if not self.is_cross_attention:
# if only "normal" attention layer implements causal mask
query_length, key_length = query.size(-2), key.size(-2)
causal_mask = self.bias[:, :, key_length - query_length : key_length, :key_length]
mask_value = torch.finfo(attn_weights.dtype).min
# Need to be a tensor, otherwise we get error: `RuntimeError: expected scalar type float but found double`.
# Need to be on the same device, otherwise `RuntimeError: ..., x and y to be on the same device`
mask_value = torch.tensor(mask_value, dtype=attn_weights.dtype).to(attn_weights.device)
attn_weights = torch.where(causal_mask, attn_weights, mask_value)
if attention_mask is not None:
# Apply the attention mask
attn_weights = attn_weights + attention_mask
attn_weights = nn.Softmax(dim=-1)(attn_weights)
# Downcast (if necessary) back to V's dtype (if in mixed-precision) -- No-Op if otherwise
if attn_weights.dtype != torch.float32:
raise RuntimeError("Error with upcasting, attn_weights does not have dtype torch.float32")
attn_weights = attn_weights.type(value.dtype)
attn_weights = self.attn_dropout(attn_weights)
# Mask heads if we want to
if head_mask is not None:
attn_weights = attn_weights * head_mask
attn_output = torch.matmul(attn_weights, value)
return attn_output, attn_weights
def _split_heads(self, tensor, num_heads, attn_head_size):
"""
Splits hidden_size dim into attn_head_size and num_heads
"""
new_shape = tensor.size()[:-1] + (num_heads, attn_head_size)
tensor = tensor.view(*new_shape)
return tensor.permute(0, 2, 1, 3) # (batch, head, seq_length, head_features)
def _merge_heads(self, tensor, num_heads, attn_head_size):
"""
Merges attn_head_size dim and num_attn_heads dim into hidden_size
"""
tensor = tensor.permute(0, 2, 1, 3).contiguous()
new_shape = tensor.size()[:-2] + (num_heads * attn_head_size,)
return tensor.view(new_shape)
def forward(
self,
hidden_states: torch.Tensor,
layer_past: Optional[bool] = None,
attention_mask: Optional[torch.Tensor] = None,
head_mask: Optional[torch.Tensor] = None,
encoder_hidden_states: Optional[torch.Tensor] = None,
encoder_attention_mask: Optional[torch.Tensor] = None,
use_cache: Optional[bool] = False,
output_attentions: Optional[bool] = False,
) -> tuple:
if encoder_hidden_states is not None:
if not hasattr(self, "q_attn"):
raise ValueError(
"If class is used as cross attention, the weights `q_attn` have to be defined. "
"Please make sure to instantiate class with `ImageGPTAttention(..., is_cross_attention=True)`."
)
query = self.q_attn(hidden_states)
key, value = self.c_attn(encoder_hidden_states).split(self.split_size, dim=2)
attention_mask = encoder_attention_mask
else:
query, key, value = self.c_attn(hidden_states).split(self.split_size, dim=2)
query = self._split_heads(query, self.num_heads, self.head_dim)
key = self._split_heads(key, self.num_heads, self.head_dim)
value = self._split_heads(value, self.num_heads, self.head_dim)
if layer_past is not None:
past_key, past_value = layer_past
key = torch.cat((past_key, key), dim=-2)
value = torch.cat((past_value, value), dim=-2)
if use_cache is True:
present = (key, value)
else:
present = None
if self.reorder_and_upcast_attn:
attn_output, attn_weights = self._upcast_and_reordered_attn(query, key, value, attention_mask, head_mask)
else:
attn_output, attn_weights = self._attn(query, key, value, attention_mask, head_mask)
attn_output = self._merge_heads(attn_output, self.num_heads, self.head_dim)
attn_output = self.c_proj(attn_output)
attn_output = self.resid_dropout(attn_output)
outputs = (attn_output, present)
if output_attentions:
outputs += (attn_weights,)
return outputs # a, present, (attentions)
class ImageGPTMLP(nn.Module):
def __init__(self, intermediate_size, config):
super().__init__()
embed_dim = config.hidden_size
self.c_fc = Conv1D(intermediate_size, embed_dim)
self.c_proj = Conv1D(embed_dim, intermediate_size)
self.act = ACT2FN[config.activation_function]
self.dropout = nn.Dropout(config.resid_pdrop)
def forward(self, hidden_states: torch.Tensor) -> torch.Tensor:
hidden_states = self.c_fc(hidden_states)
hidden_states = self.act(hidden_states)
hidden_states = self.c_proj(hidden_states)
hidden_states = self.dropout(hidden_states)
return hidden_states
class ImageGPTBlock(nn.Module):
def __init__(self, config, layer_idx=None):
super().__init__()
hidden_size = config.hidden_size
inner_dim = config.n_inner if config.n_inner is not None else 4 * hidden_size
self.ln_1 = ImageGPTLayerNorm(hidden_size, eps=config.layer_norm_epsilon)
self.attn = ImageGPTAttention(config, layer_idx=layer_idx)
self.ln_2 = ImageGPTLayerNorm(hidden_size, eps=config.layer_norm_epsilon)
if config.add_cross_attention:
self.crossattention = ImageGPTAttention(config, is_cross_attention=True, layer_idx=layer_idx)
self.ln_cross_attn = ImageGPTLayerNorm(hidden_size, eps=config.layer_norm_epsilon)
self.mlp = ImageGPTMLP(inner_dim, config)
def forward(
self,
hidden_states: torch.Tensor,
layer_past: Optional[bool] = None,
attention_mask: Optional[torch.Tensor] = None,
head_mask: Optional[torch.Tensor] = None,
encoder_hidden_states: Optional[torch.Tensor] = None,
encoder_attention_mask: Optional[torch.Tensor] = None,
use_cache: Optional[bool] = False,
output_attentions: Optional[bool] = False,
) -> tuple:
residual = hidden_states
hidden_states = self.ln_1(hidden_states)
attn_outputs = self.attn(
hidden_states,
layer_past=layer_past,
attention_mask=attention_mask,
head_mask=head_mask,
use_cache=use_cache,
output_attentions=output_attentions,
)
attn_output = attn_outputs[0] # output_attn: a, present, (attentions)
outputs = attn_outputs[1:]
# residual connection
hidden_states = attn_output + residual
if encoder_hidden_states is not None:
# add one self-attention block for cross-attention
if not hasattr(self, "crossattention"):
raise ValueError(
f"If `encoder_hidden_states` are passed, {self} has to be instantiated with "
"cross-attention layers by setting `config.add_cross_attention=True`"
)
residual = hidden_states
hidden_states = self.ln_cross_attn(hidden_states)
cross_attn_outputs = self.crossattention(
hidden_states,
attention_mask=attention_mask,
head_mask=head_mask,
encoder_hidden_states=encoder_hidden_states,
encoder_attention_mask=encoder_attention_mask,
output_attentions=output_attentions,
)
attn_output = cross_attn_outputs[0]
# residual connection
hidden_states = residual + attn_output
outputs = outputs + cross_attn_outputs[2:] # add cross attentions if we output attention weights
residual = hidden_states
hidden_states = self.ln_2(hidden_states)
feed_forward_hidden_states = self.mlp(hidden_states)
# residual connection
hidden_states = residual + feed_forward_hidden_states
outputs = (hidden_states,) + (outputs if use_cache else outputs[1:])
return outputs # hidden_states, present, (attentions, cross_attentions)
class ImageGPTPreTrainedModel(PreTrainedModel):
"""
An abstract class to handle weights initialization and a simple interface for downloading and loading pretrained
models.
"""
config_class = ImageGPTConfig
load_tf_weights = load_tf_weights_in_imagegpt
base_model_prefix = "transformer"
main_input_name = "input_ids"
supports_gradient_checkpointing = True
def __init__(self, *inputs, **kwargs):
super().__init__(*inputs, **kwargs)
def _init_weights(self, module):
"""Initialize the weights."""
if isinstance(module, (nn.Linear, Conv1D)):
# Slightly different from the TF version which uses truncated_normal for initialization
# cf https://github.com/pytorch/pytorch/pull/5617
module.weight.data.normal_(mean=0.0, std=self.config.initializer_range)
if module.bias is not None:
module.bias.data.zero_()
elif isinstance(module, nn.Embedding):
module.weight.data.normal_(mean=0.0, std=self.config.initializer_range)
if module.padding_idx is not None:
module.weight.data[module.padding_idx].zero_()
elif isinstance(module, ImageGPTLayerNorm):
module.weight.data.fill_(1.0)
# Reinitialize selected weights subject to the OpenAI GPT-2 Paper Scheme:
# > A modified initialization which accounts for the accumulation on the residual path with model depth. Scale
# > the weights of residual layers at initialization by a factor of 1/โN where N is the # of residual layers.
# > -- GPT-2 :: https://openai.com/blog/better-language-models/
#
# Reference (Megatron-LM): https://github.com/NVIDIA/Megatron-LM/blob/main/megatron/model/gpt_model.py
for name, p in module.named_parameters():
if "c_proj" in name and "weight" in name:
# Special Scaled Initialization --> There are 2 Layer Norms per Transformer Block
p.data.normal_(mean=0.0, std=(self.config.initializer_range / math.sqrt(2 * self.config.n_layer)))
IMAGEGPT_START_DOCSTRING = r"""
This model inherits from [`PreTrainedModel`]. Check the superclass documentation for the generic methods the
library implements for all its model (such as downloading or saving, resizing the input embeddings, pruning heads
etc.)
This model is also a PyTorch [torch.nn.Module](https://pytorch.org/docs/stable/nn.html#torch.nn.Module) subclass.
Use it as a regular PyTorch Module and refer to the PyTorch documentation for all matter related to general usage
and behavior.
Parameters:
config ([`ImageGPTConfig`]): Model configuration class with all the parameters of the model.
Initializing with a config file does not load the weights associated with the model, only the
configuration. Check out the [`~PreTrainedModel.from_pretrained`] method to load the model weights.
"""
IMAGEGPT_INPUTS_DOCSTRING = r"""
Args:
input_ids (`torch.LongTensor` of shape `(batch_size, sequence_length)`):
`input_ids_length` = `sequence_length` if `past_key_values` is `None` else
`past_key_values[0][0].shape[-2]` (`sequence_length` of input past key value states). Indices of input
sequence tokens in the vocabulary.
If `past_key_values` is used, only `input_ids` that do not have their past calculated should be passed as
`input_ids`.
Indices can be obtained using [`AutoImageProcessor`]. See [`ImageGPTImageProcessor.__call__`] for details.
past_key_values (`Tuple[Tuple[torch.Tensor]]` of length `config.n_layers`):
Contains precomputed hidden-states (key and values in the attention blocks) as computed by the model (see
`past_key_values` output below). Can be used to speed up sequential decoding. The `input_ids` which have
their past given to this model should not be passed as `input_ids` as they have already been computed.
attention_mask (`torch.FloatTensor` of shape `(batch_size, sequence_length)`, *optional*):
Mask to avoid performing attention on padding token indices. Mask values selected in `[0, 1]`:
- 1 for tokens that are **not masked**,
- 0 for tokens that are **masked**.
[What are attention masks?](../glossary#attention-mask)
token_type_ids (`torch.LongTensor` of shape `(batch_size, sequence_length)`, *optional*):
Segment token indices to indicate first and second portions of the inputs. Indices are selected in `[0,
1]`:
- 0 corresponds to a *sentence A* token,
- 1 corresponds to a *sentence B* token.
[What are token type IDs?](../glossary#token-type-ids)
position_ids (`torch.LongTensor` of shape `(batch_size, sequence_length)`, *optional*):
Indices of positions of each input sequence tokens in the position embeddings. Selected in the range `[0,
config.max_position_embeddings - 1]`.
[What are position IDs?](../glossary#position-ids)
head_mask (`torch.FloatTensor` of shape `(num_heads,)` or `(num_layers, num_heads)`, *optional*):
Mask to nullify selected heads of the self-attention modules. Mask values selected in `[0, 1]`:
- 1 indicates the head is **not masked**,
- 0 indicates the head is **masked**.
inputs_embeds (`torch.FloatTensor` of shape `(batch_size, sequence_length, hidden_size)`, *optional*):
Optionally, instead of passing `input_ids` you can choose to directly pass an embedded representation. This
is useful if you want more control over how to convert `input_ids` indices into associated vectors than the
model's internal embedding lookup matrix.
If `past_key_values` is used, optionally only the last `inputs_embeds` have to be input (see
`past_key_values`).
use_cache (`bool`, *optional*):
If set to `True`, `past_key_values` key value states are returned and can be used to speed up decoding (see
`past_key_values`).
output_attentions (`bool`, *optional*):
Whether or not to return the attentions tensors of all attention layers. See `attentions` under returned
tensors for more detail.
output_hidden_states (`bool`, *optional*):
Whether or not to return the hidden states of all layers. See `hidden_states` under returned tensors for
more detail.
return_dict (`bool`, *optional*):
Whether or not to return a [`~utils.ModelOutput`] instead of a plain tuple.
"""
@add_start_docstrings(
"The bare ImageGPT Model transformer outputting raw hidden-states without any specific head on top.",
IMAGEGPT_START_DOCSTRING,
)
class ImageGPTModel(ImageGPTPreTrainedModel):
def __init__(self, config: ImageGPTConfig):
super().__init__(config)
self.embed_dim = config.hidden_size
self.wte = nn.Embedding(config.vocab_size, self.embed_dim)
self.wpe = nn.Embedding(config.max_position_embeddings, self.embed_dim)
self.drop = nn.Dropout(config.embd_pdrop)
self.h = nn.ModuleList([ImageGPTBlock(config, layer_idx=i) for i in range(config.num_hidden_layers)])
self.ln_f = ImageGPTLayerNorm(self.embed_dim, eps=config.layer_norm_epsilon)
# Model parallel
self.model_parallel = False
self.device_map = None
self.gradient_checkpointing = False
# Initialize weights and apply final processing
self.post_init()
def get_input_embeddings(self):
return self.wte
def set_input_embeddings(self, new_embeddings):
self.wte = new_embeddings
def _prune_heads(self, heads_to_prune):
"""
Prunes heads of the model. heads_to_prune: dict of {layer_num: list of heads to prune in this layer}
"""
for layer, heads in heads_to_prune.items():
self.h[layer].attn.prune_heads(heads)
@add_start_docstrings_to_model_forward(IMAGEGPT_INPUTS_DOCSTRING)
@replace_return_docstrings(output_type=BaseModelOutputWithPastAndCrossAttentions, config_class=_CONFIG_FOR_DOC)
def forward(
self,
input_ids: Optional[torch.Tensor] = None,
past_key_values: Optional[Tuple[Tuple[torch.Tensor]]] = None,
attention_mask: Optional[torch.Tensor] = None,
token_type_ids: Optional[torch.Tensor] = None,
position_ids: Optional[torch.Tensor] = None,
head_mask: Optional[torch.Tensor] = None,
inputs_embeds: Optional[torch.Tensor] = None,
encoder_hidden_states: Optional[torch.Tensor] = None,
encoder_attention_mask: Optional[torch.Tensor] = None,
use_cache: Optional[bool] = None,
output_attentions: Optional[bool] = None,
output_hidden_states: Optional[bool] = None,
return_dict: Optional[bool] = None,
**kwargs: Any,
) -> Union[Tuple, BaseModelOutputWithPastAndCrossAttentions]:
r"""
labels (`torch.LongTensor` of shape `(batch_size, sequence_length)`, *optional*):
Labels for language modeling. Note that the labels **are shifted** inside the model, i.e. you can set
`labels = input_ids` Indices are selected in `[-100, 0, ..., config.vocab_size]` All labels set to `-100`
are ignored (masked), the loss is only computed for labels in `[0, ..., config.vocab_size]`
Returns:
Examples:
```python
>>> from transformers import AutoImageProcessor, ImageGPTModel
>>> from PIL import Image
>>> import requests
>>> url = "http://images.cocodataset.org/val2017/000000039769.jpg"
>>> image = Image.open(requests.get(url, stream=True).raw)
>>> image_processor = AutoImageProcessor.from_pretrained("openai/imagegpt-small")
>>> model = ImageGPTModel.from_pretrained("openai/imagegpt-small")
>>> inputs = image_processor(images=image, return_tensors="pt")
>>> outputs = model(**inputs)
>>> last_hidden_states = outputs.last_hidden_state
```"""
if "pixel_values" in kwargs:
warnings.warn(
"The `pixel_values` argument is deprecated and will be removed in a future version, use `input_ids`"
" instead.",
FutureWarning,
)
if input_ids is not None:
raise ValueError(
"You cannot pass both `pixel_values` and `input_ids`. Please make sure to only pass `input_ids`."
)
input_ids = kwargs.pop("pixel_values")
output_attentions = output_attentions if output_attentions is not None else self.config.output_attentions
output_hidden_states = (
output_hidden_states if output_hidden_states is not None else self.config.output_hidden_states
)
use_cache = use_cache if use_cache is not None else self.config.use_cache
return_dict = return_dict if return_dict is not None else self.config.use_return_dict
if input_ids is not None and inputs_embeds is not None:
raise ValueError("You cannot specify both input_ids and inputs_embeds at the same time")
elif input_ids is not None:
self.warn_if_padding_and_no_attention_mask(input_ids, attention_mask)
input_shape = input_ids.size()
input_ids = input_ids.view(-1, input_shape[-1])
batch_size = input_ids.shape[0]
elif inputs_embeds is not None:
input_shape = inputs_embeds.size()[:-1]
batch_size = inputs_embeds.shape[0]
else:
raise ValueError("You have to specify either input_ids or inputs_embeds")
device = input_ids.device if input_ids is not None else inputs_embeds.device
if token_type_ids is not None:
token_type_ids = token_type_ids.view(-1, input_shape[-1])
if past_key_values is None:
past_length = 0
past_key_values = tuple([None] * len(self.h))
else:
past_length = past_key_values[0][0].size(-2)
if position_ids is None:
position_ids = torch.arange(past_length, input_shape[-1] + past_length, dtype=torch.long, device=device)
position_ids = position_ids.unsqueeze(0)
# ImageGPTAttention mask.
if attention_mask is not None:
if batch_size <= 0:
raise ValueError("batch_size has to be defined and > 0")
attention_mask = attention_mask.view(batch_size, -1)
# We create a 3D attention mask from a 2D tensor mask.
# Sizes are [batch_size, 1, 1, to_seq_length]
# So we can broadcast to [batch_size, num_heads, from_seq_length, to_seq_length]
# this attention mask is more simple than the triangular masking of causal attention
# used in OpenAI GPT, we just need to prepare the broadcast dimension here.
attention_mask = attention_mask[:, None, None, :]
# Since attention_mask is 1.0 for positions we want to attend and 0.0 for
# masked positions, this operation will create a tensor which is 0.0 for
# positions we want to attend and the dtype's smallest value for masked positions.
# Since we are adding it to the raw scores before the softmax, this is
# effectively the same as removing these entirely.
attention_mask = attention_mask.to(dtype=self.dtype) # fp16 compatibility
attention_mask = (1.0 - attention_mask) * torch.finfo(self.dtype).min
# If a 2D or 3D attention mask is provided for the cross-attention
# we need to make broadcastable to [batch_size, num_heads, seq_length, seq_length]
if self.config.add_cross_attention and encoder_hidden_states is not None:
encoder_batch_size, encoder_sequence_length, _ = encoder_hidden_states.size()
encoder_hidden_shape = (encoder_batch_size, encoder_sequence_length)
if encoder_attention_mask is None:
encoder_attention_mask = torch.ones(encoder_hidden_shape, device=device)
encoder_attention_mask = self.invert_attention_mask(encoder_attention_mask)
else:
encoder_attention_mask = None
# Prepare head mask if needed
# 1.0 in head_mask indicate we keep the head
# attention_probs has shape bsz x n_heads x N x N
# head_mask has shape n_layer x batch x n_heads x N x N
head_mask = self.get_head_mask(head_mask, self.config.n_layer)
if inputs_embeds is None:
inputs_embeds = self.wte(input_ids)
position_embeds = self.wpe(position_ids)
hidden_states = inputs_embeds + position_embeds
if token_type_ids is not None:
token_type_embeds = self.wte(token_type_ids)
hidden_states = hidden_states + token_type_embeds
hidden_states = self.drop(hidden_states)
output_shape = input_shape + (hidden_states.size(-1),)
if self.gradient_checkpointing and self.training:
if use_cache:
logger.warning_once(
"`use_cache=True` is incompatible with gradient checkpointing. Setting `use_cache=False`..."
)
use_cache = False
presents = () if use_cache else None
all_self_attentions = () if output_attentions else None
all_cross_attentions = () if output_attentions and self.config.add_cross_attention else None
all_hidden_states = () if output_hidden_states else None
for i, (block, layer_past) in enumerate(zip(self.h, past_key_values)):
# Model parallel
if self.model_parallel:
torch.cuda.set_device(hidden_states.device)
# Ensure layer_past is on same device as hidden_states (might not be correct)
if layer_past is not None:
layer_past = tuple(past_state.to(hidden_states.device) for past_state in layer_past)
# Ensure that attention_mask is always on the same device as hidden_states
if attention_mask is not None:
attention_mask = attention_mask.to(hidden_states.device)
if isinstance(head_mask, torch.Tensor):
head_mask = head_mask.to(hidden_states.device)
if output_hidden_states:
all_hidden_states = all_hidden_states + (hidden_states,)
if self.gradient_checkpointing and self.training:
outputs = self._gradient_checkpointing_func(
block.__call__,
hidden_states,
None,
attention_mask,
head_mask[i],
encoder_hidden_states,
encoder_attention_mask,
use_cache,
output_attentions,
)
else:
outputs = block(
hidden_states,
layer_past=layer_past,
attention_mask=attention_mask,
head_mask=head_mask[i],
encoder_hidden_states=encoder_hidden_states,
encoder_attention_mask=encoder_attention_mask,
use_cache=use_cache,
output_attentions=output_attentions,
)
hidden_states = outputs[0]
if use_cache is True:
presents = presents + (outputs[1],)
if output_attentions:
all_self_attentions = all_self_attentions + (outputs[2 if use_cache else 1],)
if self.config.add_cross_attention:
all_cross_attentions = all_cross_attentions + (outputs[3 if use_cache else 2],)
# Model Parallel: If it's the last layer for that device, put things on the next device
if self.model_parallel:
for k, v in self.device_map.items():
if i == v[-1] and "cuda:" + str(k) != self.last_device:
hidden_states = hidden_states.to("cuda:" + str(k + 1))
hidden_states = self.ln_f(hidden_states)
hidden_states = hidden_states.view(*output_shape)
# Add last hidden state
if output_hidden_states:
all_hidden_states = all_hidden_states + (hidden_states,)
if not return_dict:
return tuple(
v
for v in [hidden_states, presents, all_hidden_states, all_self_attentions, all_cross_attentions]
if v is not None
)
return BaseModelOutputWithPastAndCrossAttentions(
last_hidden_state=hidden_states,
past_key_values=presents,
hidden_states=all_hidden_states,
attentions=all_self_attentions,
cross_attentions=all_cross_attentions,
)
@add_start_docstrings(
"""
The ImageGPT Model transformer with a language modeling head on top (linear layer with weights tied to the input
embeddings).
""",
IMAGEGPT_START_DOCSTRING,
)
class ImageGPTForCausalImageModeling(ImageGPTPreTrainedModel):
_tied_weights_keys = ["lm_head.weight"]
def __init__(self, config: ImageGPTConfig):
super().__init__(config)
self.transformer = ImageGPTModel(config)
self.lm_head = nn.Linear(config.n_embd, config.vocab_size - 1, bias=False)
# Model parallel
self.model_parallel = False
self.device_map = None
# Initialize weights and apply final processing
self.post_init()
def get_output_embeddings(self):
return self.lm_head
def set_output_embeddings(self, new_embeddings):
self.lm_head = new_embeddings
def prepare_inputs_for_generation(self, input_ids: torch.Tensor, past_key_values: Optional[bool] = None, **kwargs):
token_type_ids = kwargs.get("token_type_ids", None)
# Omit tokens covered by past_key_values
if past_key_values:
past_length = past_key_values[0][0].shape[2]
# Some generation methods already pass only the last input ID
if input_ids.shape[1] > past_length:
remove_prefix_length = past_length
else:
# Default to old behavior: keep only final ID
remove_prefix_length = input_ids.shape[1] - 1
input_ids = input_ids[:, remove_prefix_length:]
if token_type_ids is not None:
token_type_ids = token_type_ids[:, -input_ids.shape[1] :]
attention_mask = kwargs.get("attention_mask", None)
position_ids = kwargs.get("position_ids", None)
if attention_mask is not None and position_ids is None:
# create position_ids on the fly for batch generation
position_ids = attention_mask.long().cumsum(-1) - 1
position_ids.masked_fill_(attention_mask == 0, 1)
if past_key_values:
position_ids = position_ids[:, -input_ids.shape[1] :]
else:
position_ids = None
return {
"input_ids": input_ids,
"past_key_values": past_key_values,
"use_cache": kwargs.get("use_cache"),
"position_ids": position_ids,
"attention_mask": attention_mask,
"token_type_ids": token_type_ids,
}
@add_start_docstrings_to_model_forward(IMAGEGPT_INPUTS_DOCSTRING)
@replace_return_docstrings(output_type=CausalLMOutputWithCrossAttentions, config_class=_CONFIG_FOR_DOC)
def forward(
self,
input_ids: Optional[torch.Tensor] = None,
past_key_values: Optional[Tuple[Tuple[torch.Tensor]]] = None,
attention_mask: Optional[torch.Tensor] = None,
token_type_ids: Optional[torch.Tensor] = None,
position_ids: Optional[torch.Tensor] = None,
head_mask: Optional[torch.Tensor] = None,
inputs_embeds: Optional[torch.Tensor] = None,
encoder_hidden_states: Optional[torch.Tensor] = None,
encoder_attention_mask: Optional[torch.Tensor] = None,
labels: Optional[torch.Tensor] = None,
use_cache: Optional[bool] = None,
output_attentions: Optional[bool] = None,
output_hidden_states: Optional[bool] = None,
return_dict: Optional[bool] = None,
**kwargs: Any,
) -> Union[Tuple, CausalLMOutputWithCrossAttentions]:
r"""
labels (`torch.LongTensor` of shape `(batch_size, sequence_length)`, *optional*):
Labels for language modeling. Note that the labels **are shifted** inside the model, i.e. you can set
`labels = input_ids` Indices are selected in `[-100, 0, ..., config.vocab_size]` All labels set to `-100`
are ignored (masked), the loss is only computed for labels in `[0, ..., config.vocab_size]`
Returns:
Examples:
```python
>>> from transformers import AutoImageProcessor, ImageGPTForCausalImageModeling
>>> import torch
>>> import matplotlib.pyplot as plt
>>> import numpy as np
>>> image_processor = AutoImageProcessor.from_pretrained("openai/imagegpt-small")
>>> model = ImageGPTForCausalImageModeling.from_pretrained("openai/imagegpt-small")
>>> device = torch.device("cuda" if torch.cuda.is_available() else "cpu")
>>> model.to(device) # doctest: +IGNORE_RESULT
>>> # unconditional generation of 8 images
>>> batch_size = 4
>>> context = torch.full((batch_size, 1), model.config.vocab_size - 1) # initialize with SOS token
>>> context = context.to(device)
>>> output = model.generate(
... input_ids=context, max_length=model.config.n_positions + 1, temperature=1.0, do_sample=True, top_k=40
... )
>>> clusters = image_processor.clusters
>>> height = image_processor.size["height"]
>>> width = image_processor.size["width"]
>>> samples = output[:, 1:].cpu().detach().numpy()
>>> samples_img = [
... np.reshape(np.rint(127.5 * (clusters[s] + 1.0)), [height, width, 3]).astype(np.uint8) for s in samples
... ] # convert color cluster tokens back to pixels
>>> f, axes = plt.subplots(1, batch_size, dpi=300)
>>> for img, ax in zip(samples_img, axes): # doctest: +IGNORE_RESULT
... ax.axis("off")
... ax.imshow(img)
```"""
if "pixel_values" in kwargs:
warnings.warn(
"The `pixel_values` argument is deprecated and will be removed in a future version, use `input_ids`"
" instead.",
FutureWarning,
)
if input_ids is not None:
raise ValueError(
"You cannot pass both `pixel_values` and `input_ids`. Please make sure to only pass `input_ids`."
)
input_ids = kwargs.pop("pixel_values")
return_dict = return_dict if return_dict is not None else self.config.use_return_dict
transformer_outputs = self.transformer(
input_ids,
past_key_values=past_key_values,
attention_mask=attention_mask,
token_type_ids=token_type_ids,
position_ids=position_ids,
head_mask=head_mask,
inputs_embeds=inputs_embeds,
encoder_hidden_states=encoder_hidden_states,
encoder_attention_mask=encoder_attention_mask,
use_cache=use_cache,
output_attentions=output_attentions,
output_hidden_states=output_hidden_states,
return_dict=return_dict,
)
hidden_states = transformer_outputs[0]
lm_logits = self.lm_head(hidden_states)
loss = None
if labels is not None:
# Shift so that tokens < n predict n
shift_logits = lm_logits[..., :-1, :].contiguous()
shift_labels = labels[..., 1:].contiguous()
# Flatten the tokens
loss_fct = CrossEntropyLoss()
loss = loss_fct(shift_logits.view(-1, shift_logits.size(-1)), shift_labels.view(-1))
if not return_dict:
output = (lm_logits,) + transformer_outputs[1:]
return ((loss,) + output) if loss is not None else output
return CausalLMOutputWithCrossAttentions(
loss=loss,
logits=lm_logits,
past_key_values=transformer_outputs.past_key_values,
hidden_states=transformer_outputs.hidden_states,
attentions=transformer_outputs.attentions,
cross_attentions=transformer_outputs.cross_attentions,
)
@staticmethod
def _reorder_cache(
past_key_values: Tuple[Tuple[torch.Tensor]], beam_idx: torch.Tensor
) -> Tuple[Tuple[torch.Tensor]]:
"""
This function is used to re-order the `past_key_values` cache if [`~PreTrainedModel.beam_search`] or
[`~PreTrainedModel.beam_sample`] is called. This is required to match `past_key_values` with the correct
beam_idx at every generation step.
"""
return tuple(
tuple(past_state.index_select(0, beam_idx.to(past_state.device)) for past_state in layer_past)
for layer_past in past_key_values
)
@add_start_docstrings(
"""
The ImageGPT Model transformer with an image classification head on top (linear layer).
[`ImageGPTForImageClassification`] average-pools the hidden states in order to do the classification.
""",
IMAGEGPT_START_DOCSTRING,
)
class ImageGPTForImageClassification(ImageGPTPreTrainedModel):
def __init__(self, config: ImageGPTConfig):
super().__init__(config)
self.num_labels = config.num_labels
self.transformer = ImageGPTModel(config)
self.score = nn.Linear(config.n_embd, self.num_labels, bias=False)
# Initialize weights and apply final processing
self.post_init()
@add_start_docstrings_to_model_forward(IMAGEGPT_INPUTS_DOCSTRING)
@replace_return_docstrings(output_type=SequenceClassifierOutputWithPast, config_class=_CONFIG_FOR_DOC)
def forward(
self,
input_ids: Optional[torch.Tensor] = None,
past_key_values: Optional[Tuple[Tuple[torch.Tensor]]] = None,
attention_mask: Optional[torch.Tensor] = None,
token_type_ids: Optional[torch.Tensor] = None,
position_ids: Optional[torch.Tensor] = None,
head_mask: Optional[torch.Tensor] = None,
inputs_embeds: Optional[torch.Tensor] = None,
labels: Optional[torch.Tensor] = None,
use_cache: Optional[bool] = None,
output_attentions: Optional[bool] = None,
output_hidden_states: Optional[bool] = None,
return_dict: Optional[bool] = None,
**kwargs: Any,
) -> Union[Tuple, SequenceClassifierOutputWithPast]:
r"""
labels (`torch.LongTensor` of shape `(batch_size,)`, *optional*):
Labels for computing the sequence classification/regression loss. Indices should be in `[0, ...,
config.num_labels - 1]`. If `config.num_labels == 1` a regression loss is computed (Mean-Square loss), If
`config.num_labels > 1` a classification loss is computed (Cross-Entropy).
Returns:
Examples:
```python
>>> from transformers import AutoImageProcessor, ImageGPTForImageClassification
>>> from PIL import Image
>>> import requests
>>> url = "http://images.cocodataset.org/val2017/000000039769.jpg"
>>> image = Image.open(requests.get(url, stream=True).raw)
>>> image_processor = AutoImageProcessor.from_pretrained("openai/imagegpt-small")
>>> model = ImageGPTForImageClassification.from_pretrained("openai/imagegpt-small")
>>> inputs = image_processor(images=image, return_tensors="pt")
>>> outputs = model(**inputs)
>>> logits = outputs.logits
```"""
if "pixel_values" in kwargs:
warnings.warn(
"The `pixel_values` argument is deprecated and will be removed in a future version, use `input_ids`"
" instead.",
FutureWarning,
)
if input_ids is not None:
raise ValueError(
"You cannot pass both `pixel_values` and `input_ids`. Please make sure to only pass `input_ids`."
)
input_ids = kwargs.pop("pixel_values")
return_dict = return_dict if return_dict is not None else self.config.use_return_dict
transformer_outputs = self.transformer(
input_ids,
past_key_values=past_key_values,
attention_mask=attention_mask,
token_type_ids=token_type_ids,
position_ids=position_ids,
head_mask=head_mask,
inputs_embeds=inputs_embeds,
use_cache=use_cache,
output_attentions=output_attentions,
output_hidden_states=output_hidden_states,
return_dict=return_dict,
)
hidden_states = transformer_outputs[0]
# average-pool the hidden states along the sequence dimension
pooled_hidden_states = hidden_states.mean(dim=1)
# project from (batch_size, hidden_size) to (batch_size, num_labels)
logits = self.score(pooled_hidden_states)
loss = None
if labels is not None:
if self.config.problem_type is None:
if self.num_labels == 1:
self.config.problem_type = "regression"
elif self.num_labels > 1 and (labels.dtype == torch.long or labels.dtype == torch.int):
self.config.problem_type = "single_label_classification"
else:
self.config.problem_type = "multi_label_classification"
if self.config.problem_type == "regression":
loss_fct = MSELoss()
if self.num_labels == 1:
loss = loss_fct(logits.squeeze(), labels.squeeze())
else:
loss = loss_fct(logits, labels)
elif self.config.problem_type == "single_label_classification":
loss_fct = CrossEntropyLoss()
loss = loss_fct(logits.view(-1, self.num_labels), labels.view(-1))
elif self.config.problem_type == "multi_label_classification":
loss_fct = BCEWithLogitsLoss()
loss = loss_fct(logits, labels)
if not return_dict:
output = (logits,) + transformer_outputs[1:]
return ((loss,) + output) if loss is not None else output
return SequenceClassifierOutputWithPast(
loss=loss,
logits=logits,
past_key_values=transformer_outputs.past_key_values,
hidden_states=transformer_outputs.hidden_states,
attentions=transformer_outputs.attentions,
)
| transformers/src/transformers/models/imagegpt/modeling_imagegpt.py/0 | {
"file_path": "transformers/src/transformers/models/imagegpt/modeling_imagegpt.py",
"repo_id": "transformers",
"token_count": 23704
} | 337 |
import argparse
from fairseq.checkpoint_utils import load_checkpoint_to_cpu
from transformers import Kosmos2Config, Kosmos2ForConditionalGeneration
KEYS_TO_MODIFY_MAPPING = {
"gpt_model.decoder.output_projection": "text_model.lm_head",
"gpt_model.decoder": "text_model.model",
"img_connector": "image_to_text_projection",
"img_model.visual.class_embedding": "vision_model.model.embeddings.class_embedding",
"img_model.visual.positional_embedding": "vision_model.model.embeddings.position_embedding.weight",
"img_model.visual.conv1": "vision_model.model.embeddings.patch_embedding",
"img_model.visual": "vision_model.model",
"ln_pre": "pre_layrnorm",
"ln_post": "post_layernorm",
"transformer.resblocks": "encoder.layers",
"ts_attn": "self_attn",
"ln_1": "layer_norm1",
"ln_2": "layer_norm2",
"c_fc": "fc1",
"c_proj": "fc2",
}
KEYS_TO_IGNORE = [
# this buffer in the original code is only used to send weights to the desired device
"gpt_model.decoder.embed_positions._float_tensor",
# this weight is never used in the forward in the original KOSMOS-2)
"gpt_model.decoder.self_attn_sope.scale",
]
def rename_key(key):
for key_to_modify, new_key in KEYS_TO_MODIFY_MAPPING.items():
if key_to_modify in key:
key = key.replace(key_to_modify, new_key)
return key
def convert_kosmos2_checkpoint_to_pytorch(checkpoint_path, pytorch_dump_folder_path):
state = load_checkpoint_to_cpu(checkpoint_path)
state_dict = state["model"]
state_dict_keys = list(state_dict.keys())
config = Kosmos2Config()
# This is necessary to match the results given by the original demo
config.text_config.no_repeat_ngram_size = 3
model = Kosmos2ForConditionalGeneration(config)
# convert (by renaming keys)
converted_state_dict = {}
for key in state_dict_keys:
if key in KEYS_TO_IGNORE:
continue
renamed_key = rename_key(key)
converted_state_dict[renamed_key] = state_dict[key]
# check weight loading
model.load_state_dict(converted_state_dict, strict=True)
# save the result
model.save_pretrained(pytorch_dump_folder_path)
if __name__ == "__main__":
parser = argparse.ArgumentParser()
# Required parameters
parser.add_argument(
"--kosmos2_checkpoint_path", default=None, type=str, required=True, help="Path the official PyTorch dump."
)
parser.add_argument(
"--pytorch_dump_folder_path", default=None, type=str, required=True, help="Path to the output PyTorch model."
)
args = parser.parse_args()
convert_kosmos2_checkpoint_to_pytorch(args.kosmos2_checkpoint_path, args.pytorch_dump_folder_path)
| transformers/src/transformers/models/kosmos2/convert_kosmos2_original_pytorch_checkpoint_to_pytorch.py/0 | {
"file_path": "transformers/src/transformers/models/kosmos2/convert_kosmos2_original_pytorch_checkpoint_to_pytorch.py",
"repo_id": "transformers",
"token_count": 1082
} | 338 |
# coding=utf-8
# Copyright 2021 The HuggingFace Inc. team.
#
# Licensed under the Apache License, Version 2.0 (the "License");
# you may not use this file except in compliance with the License.
# You may obtain a copy of the License at
#
# http://www.apache.org/licenses/LICENSE-2.0
#
# Unless required by applicable law or agreed to in writing, software
# distributed under the License is distributed on an "AS IS" BASIS,
# WITHOUT WARRANTIES OR CONDITIONS OF ANY KIND, either express or implied.
# See the License for the specific language governing permissions and
# limitations under the License.
"""
Fast tokenization class for LayoutLMv2. It overwrites 2 methods of the slow tokenizer class, namely _batch_encode_plus
and _encode_plus, in which the Rust tokenizer is used.
"""
import json
from typing import Dict, List, Optional, Tuple, Union
from tokenizers import normalizers
from ...tokenization_utils_base import (
BatchEncoding,
EncodedInput,
PaddingStrategy,
PreTokenizedInput,
TensorType,
TextInput,
TextInputPair,
TruncationStrategy,
)
from ...tokenization_utils_fast import PreTrainedTokenizerFast
from ...utils import add_end_docstrings, logging
from .tokenization_layoutlmv2 import (
LAYOUTLMV2_ENCODE_KWARGS_DOCSTRING,
LAYOUTLMV2_ENCODE_PLUS_ADDITIONAL_KWARGS_DOCSTRING,
LayoutLMv2Tokenizer,
)
logger = logging.get_logger(__name__)
VOCAB_FILES_NAMES = {"vocab_file": "vocab.txt", "tokenizer_file": "tokenizer.json"}
PRETRAINED_VOCAB_FILES_MAP = {
"vocab_file": {
"microsoft/layoutlmv2-base-uncased": (
"https://huggingface.co/microsoft/layoutlmv2-base-uncased/resolve/main/vocab.txt"
),
},
"tokenizer_file": {
"microsoft/layoutlmv2-base-uncased": (
"https://huggingface.co/microsoft/layoutlmv2-base-uncased/resolve/main/tokenizer.json"
),
},
}
PRETRAINED_POSITIONAL_EMBEDDINGS_SIZES = {
"microsoft/layoutlmv2-base-uncased": 512,
}
PRETRAINED_INIT_CONFIGURATION = {
"microsoft/layoutlmv2-base-uncased": {"do_lower_case": True},
}
class LayoutLMv2TokenizerFast(PreTrainedTokenizerFast):
r"""
Construct a "fast" LayoutLMv2 tokenizer (backed by HuggingFace's *tokenizers* library). Based on WordPiece.
This tokenizer inherits from [`PreTrainedTokenizerFast`] which contains most of the main methods. Users should
refer to this superclass for more information regarding those methods.
Args:
vocab_file (`str`):
File containing the vocabulary.
do_lower_case (`bool`, *optional*, defaults to `True`):
Whether or not to lowercase the input when tokenizing.
unk_token (`str`, *optional*, defaults to `"[UNK]"`):
The unknown token. A token that is not in the vocabulary cannot be converted to an ID and is set to be this
token instead.
sep_token (`str`, *optional*, defaults to `"[SEP]"`):
The separator token, which is used when building a sequence from multiple sequences, e.g. two sequences for
sequence classification or for a text and a question for question answering. It is also used as the last
token of a sequence built with special tokens.
pad_token (`str`, *optional*, defaults to `"[PAD]"`):
The token used for padding, for example when batching sequences of different lengths.
cls_token (`str`, *optional*, defaults to `"[CLS]"`):
The classifier token which is used when doing sequence classification (classification of the whole sequence
instead of per-token classification). It is the first token of the sequence when built with special tokens.
mask_token (`str`, *optional*, defaults to `"[MASK]"`):
The token used for masking values. This is the token used when training this model with masked language
modeling. This is the token which the model will try to predict.
cls_token_box (`List[int]`, *optional*, defaults to `[0, 0, 0, 0]`):
The bounding box to use for the special [CLS] token.
sep_token_box (`List[int]`, *optional*, defaults to `[1000, 1000, 1000, 1000]`):
The bounding box to use for the special [SEP] token.
pad_token_box (`List[int]`, *optional*, defaults to `[0, 0, 0, 0]`):
The bounding box to use for the special [PAD] token.
pad_token_label (`int`, *optional*, defaults to -100):
The label to use for padding tokens. Defaults to -100, which is the `ignore_index` of PyTorch's
CrossEntropyLoss.
only_label_first_subword (`bool`, *optional*, defaults to `True`):
Whether or not to only label the first subword, in case word labels are provided.
tokenize_chinese_chars (`bool`, *optional*, defaults to `True`):
Whether or not to tokenize Chinese characters. This should likely be deactivated for Japanese (see [this
issue](https://github.com/huggingface/transformers/issues/328)).
strip_accents (`bool`, *optional*):
Whether or not to strip all accents. If this option is not specified, then it will be determined by the
value for `lowercase` (as in the original LayoutLMv2).
"""
vocab_files_names = VOCAB_FILES_NAMES
pretrained_vocab_files_map = PRETRAINED_VOCAB_FILES_MAP
pretrained_init_configuration = PRETRAINED_INIT_CONFIGURATION
max_model_input_sizes = PRETRAINED_POSITIONAL_EMBEDDINGS_SIZES
slow_tokenizer_class = LayoutLMv2Tokenizer
def __init__(
self,
vocab_file=None,
tokenizer_file=None,
do_lower_case=True,
unk_token="[UNK]",
sep_token="[SEP]",
pad_token="[PAD]",
cls_token="[CLS]",
mask_token="[MASK]",
cls_token_box=[0, 0, 0, 0],
sep_token_box=[1000, 1000, 1000, 1000],
pad_token_box=[0, 0, 0, 0],
pad_token_label=-100,
only_label_first_subword=True,
tokenize_chinese_chars=True,
strip_accents=None,
**kwargs,
):
super().__init__(
vocab_file,
tokenizer_file=tokenizer_file,
do_lower_case=do_lower_case,
unk_token=unk_token,
sep_token=sep_token,
pad_token=pad_token,
cls_token=cls_token,
mask_token=mask_token,
cls_token_box=cls_token_box,
sep_token_box=sep_token_box,
pad_token_box=pad_token_box,
pad_token_label=pad_token_label,
only_label_first_subword=only_label_first_subword,
tokenize_chinese_chars=tokenize_chinese_chars,
strip_accents=strip_accents,
**kwargs,
)
pre_tok_state = json.loads(self.backend_tokenizer.normalizer.__getstate__())
if (
pre_tok_state.get("lowercase", do_lower_case) != do_lower_case
or pre_tok_state.get("strip_accents", strip_accents) != strip_accents
):
pre_tok_class = getattr(normalizers, pre_tok_state.pop("type"))
pre_tok_state["lowercase"] = do_lower_case
pre_tok_state["strip_accents"] = strip_accents
self.backend_tokenizer.normalizer = pre_tok_class(**pre_tok_state)
self.do_lower_case = do_lower_case
# additional properties
self.cls_token_box = cls_token_box
self.sep_token_box = sep_token_box
self.pad_token_box = pad_token_box
self.pad_token_label = pad_token_label
self.only_label_first_subword = only_label_first_subword
@add_end_docstrings(LAYOUTLMV2_ENCODE_KWARGS_DOCSTRING, LAYOUTLMV2_ENCODE_PLUS_ADDITIONAL_KWARGS_DOCSTRING)
def __call__(
self,
text: Union[TextInput, PreTokenizedInput, List[TextInput], List[PreTokenizedInput]],
text_pair: Optional[Union[PreTokenizedInput, List[PreTokenizedInput]]] = None,
boxes: Union[List[List[int]], List[List[List[int]]]] = None,
word_labels: Optional[Union[List[int], List[List[int]]]] = None,
add_special_tokens: bool = True,
padding: Union[bool, str, PaddingStrategy] = False,
truncation: Union[bool, str, TruncationStrategy] = None,
max_length: Optional[int] = None,
stride: int = 0,
pad_to_multiple_of: Optional[int] = None,
return_tensors: Optional[Union[str, TensorType]] = None,
return_token_type_ids: Optional[bool] = None,
return_attention_mask: Optional[bool] = None,
return_overflowing_tokens: bool = False,
return_special_tokens_mask: bool = False,
return_offsets_mapping: bool = False,
return_length: bool = False,
verbose: bool = True,
**kwargs,
) -> BatchEncoding:
"""
Main method to tokenize and prepare for the model one or several sequence(s) or one or several pair(s) of
sequences with word-level normalized bounding boxes and optional labels.
Args:
text (`str`, `List[str]`, `List[List[str]]`):
The sequence or batch of sequences to be encoded. Each sequence can be a string, a list of strings
(words of a single example or questions of a batch of examples) or a list of list of strings (batch of
words).
text_pair (`List[str]`, `List[List[str]]`):
The sequence or batch of sequences to be encoded. Each sequence should be a list of strings
(pretokenized string).
boxes (`List[List[int]]`, `List[List[List[int]]]`):
Word-level bounding boxes. Each bounding box should be normalized to be on a 0-1000 scale.
word_labels (`List[int]`, `List[List[int]]`, *optional*):
Word-level integer labels (for token classification tasks such as FUNSD, CORD).
"""
# Input type checking for clearer error
def _is_valid_text_input(t):
if isinstance(t, str):
# Strings are fine
return True
elif isinstance(t, (list, tuple)):
# List are fine as long as they are...
if len(t) == 0:
# ... empty
return True
elif isinstance(t[0], str):
# ... list of strings
return True
elif isinstance(t[0], (list, tuple)):
# ... list with an empty list or with a list of strings
return len(t[0]) == 0 or isinstance(t[0][0], str)
else:
return False
else:
return False
if text_pair is not None:
# in case text + text_pair are provided, text = questions, text_pair = words
if not _is_valid_text_input(text):
raise ValueError("text input must of type `str` (single example) or `List[str]` (batch of examples). ")
if not isinstance(text_pair, (list, tuple)):
raise ValueError(
"Words must be of type `List[str]` (single pretokenized example), "
"or `List[List[str]]` (batch of pretokenized examples)."
)
else:
# in case only text is provided => must be words
if not isinstance(text, (list, tuple)):
raise ValueError(
"Words must be of type `List[str]` (single pretokenized example), "
"or `List[List[str]]` (batch of pretokenized examples)."
)
if text_pair is not None:
is_batched = isinstance(text, (list, tuple))
else:
is_batched = isinstance(text, (list, tuple)) and text and isinstance(text[0], (list, tuple))
words = text if text_pair is None else text_pair
if boxes is None:
raise ValueError("You must provide corresponding bounding boxes")
if is_batched:
if len(words) != len(boxes):
raise ValueError("You must provide words and boxes for an equal amount of examples")
for words_example, boxes_example in zip(words, boxes):
if len(words_example) != len(boxes_example):
raise ValueError("You must provide as many words as there are bounding boxes")
else:
if len(words) != len(boxes):
raise ValueError("You must provide as many words as there are bounding boxes")
if is_batched:
if text_pair is not None and len(text) != len(text_pair):
raise ValueError(
f"batch length of `text`: {len(text)} does not match batch length of `text_pair`:"
f" {len(text_pair)}."
)
batch_text_or_text_pairs = list(zip(text, text_pair)) if text_pair is not None else text
is_pair = bool(text_pair is not None)
return self.batch_encode_plus(
batch_text_or_text_pairs=batch_text_or_text_pairs,
is_pair=is_pair,
boxes=boxes,
word_labels=word_labels,
add_special_tokens=add_special_tokens,
padding=padding,
truncation=truncation,
max_length=max_length,
stride=stride,
pad_to_multiple_of=pad_to_multiple_of,
return_tensors=return_tensors,
return_token_type_ids=return_token_type_ids,
return_attention_mask=return_attention_mask,
return_overflowing_tokens=return_overflowing_tokens,
return_special_tokens_mask=return_special_tokens_mask,
return_offsets_mapping=return_offsets_mapping,
return_length=return_length,
verbose=verbose,
**kwargs,
)
else:
return self.encode_plus(
text=text,
text_pair=text_pair,
boxes=boxes,
word_labels=word_labels,
add_special_tokens=add_special_tokens,
padding=padding,
truncation=truncation,
max_length=max_length,
stride=stride,
pad_to_multiple_of=pad_to_multiple_of,
return_tensors=return_tensors,
return_token_type_ids=return_token_type_ids,
return_attention_mask=return_attention_mask,
return_overflowing_tokens=return_overflowing_tokens,
return_special_tokens_mask=return_special_tokens_mask,
return_offsets_mapping=return_offsets_mapping,
return_length=return_length,
verbose=verbose,
**kwargs,
)
@add_end_docstrings(LAYOUTLMV2_ENCODE_KWARGS_DOCSTRING, LAYOUTLMV2_ENCODE_PLUS_ADDITIONAL_KWARGS_DOCSTRING)
def batch_encode_plus(
self,
batch_text_or_text_pairs: Union[
List[TextInput],
List[TextInputPair],
List[PreTokenizedInput],
],
is_pair: bool = None,
boxes: Optional[List[List[List[int]]]] = None,
word_labels: Optional[Union[List[int], List[List[int]]]] = None,
add_special_tokens: bool = True,
padding: Union[bool, str, PaddingStrategy] = False,
truncation: Union[bool, str, TruncationStrategy] = None,
max_length: Optional[int] = None,
stride: int = 0,
pad_to_multiple_of: Optional[int] = None,
return_tensors: Optional[Union[str, TensorType]] = None,
return_token_type_ids: Optional[bool] = None,
return_attention_mask: Optional[bool] = None,
return_overflowing_tokens: bool = False,
return_special_tokens_mask: bool = False,
return_offsets_mapping: bool = False,
return_length: bool = False,
verbose: bool = True,
**kwargs,
) -> BatchEncoding:
# Backward compatibility for 'truncation_strategy', 'pad_to_max_length'
padding_strategy, truncation_strategy, max_length, kwargs = self._get_padding_truncation_strategies(
padding=padding,
truncation=truncation,
max_length=max_length,
pad_to_multiple_of=pad_to_multiple_of,
verbose=verbose,
**kwargs,
)
return self._batch_encode_plus(
batch_text_or_text_pairs=batch_text_or_text_pairs,
is_pair=is_pair,
boxes=boxes,
word_labels=word_labels,
add_special_tokens=add_special_tokens,
padding_strategy=padding_strategy,
truncation_strategy=truncation_strategy,
max_length=max_length,
stride=stride,
pad_to_multiple_of=pad_to_multiple_of,
return_tensors=return_tensors,
return_token_type_ids=return_token_type_ids,
return_attention_mask=return_attention_mask,
return_overflowing_tokens=return_overflowing_tokens,
return_special_tokens_mask=return_special_tokens_mask,
return_offsets_mapping=return_offsets_mapping,
return_length=return_length,
verbose=verbose,
**kwargs,
)
def tokenize(self, text: str, pair: Optional[str] = None, add_special_tokens: bool = False, **kwargs) -> List[str]:
batched_input = [(text, pair)] if pair else [text]
encodings = self._tokenizer.encode_batch(
batched_input, add_special_tokens=add_special_tokens, is_pretokenized=False, **kwargs
)
return encodings[0].tokens
@add_end_docstrings(LAYOUTLMV2_ENCODE_KWARGS_DOCSTRING, LAYOUTLMV2_ENCODE_PLUS_ADDITIONAL_KWARGS_DOCSTRING)
def encode_plus(
self,
text: Union[TextInput, PreTokenizedInput],
text_pair: Optional[PreTokenizedInput] = None,
boxes: Optional[List[List[int]]] = None,
word_labels: Optional[List[int]] = None,
add_special_tokens: bool = True,
padding: Union[bool, str, PaddingStrategy] = False,
truncation: Union[bool, str, TruncationStrategy] = None,
max_length: Optional[int] = None,
stride: int = 0,
pad_to_multiple_of: Optional[int] = None,
return_tensors: Optional[Union[str, TensorType]] = None,
return_token_type_ids: Optional[bool] = None,
return_attention_mask: Optional[bool] = None,
return_overflowing_tokens: bool = False,
return_special_tokens_mask: bool = False,
return_offsets_mapping: bool = False,
return_length: bool = False,
verbose: bool = True,
**kwargs,
) -> BatchEncoding:
"""
Tokenize and prepare for the model a sequence or a pair of sequences. .. warning:: This method is deprecated,
`__call__` should be used instead.
Args:
text (`str`, `List[str]`, `List[List[str]]`):
The first sequence to be encoded. This can be a string, a list of strings or a list of list of strings.
text_pair (`List[str]` or `List[int]`, *optional*):
Optional second sequence to be encoded. This can be a list of strings (words of a single example) or a
list of list of strings (words of a batch of examples).
"""
# Backward compatibility for 'truncation_strategy', 'pad_to_max_length'
padding_strategy, truncation_strategy, max_length, kwargs = self._get_padding_truncation_strategies(
padding=padding,
truncation=truncation,
max_length=max_length,
pad_to_multiple_of=pad_to_multiple_of,
verbose=verbose,
**kwargs,
)
return self._encode_plus(
text=text,
boxes=boxes,
text_pair=text_pair,
word_labels=word_labels,
add_special_tokens=add_special_tokens,
padding_strategy=padding_strategy,
truncation_strategy=truncation_strategy,
max_length=max_length,
stride=stride,
pad_to_multiple_of=pad_to_multiple_of,
return_tensors=return_tensors,
return_token_type_ids=return_token_type_ids,
return_attention_mask=return_attention_mask,
return_overflowing_tokens=return_overflowing_tokens,
return_special_tokens_mask=return_special_tokens_mask,
return_offsets_mapping=return_offsets_mapping,
return_length=return_length,
verbose=verbose,
**kwargs,
)
def _batch_encode_plus(
self,
batch_text_or_text_pairs: Union[
List[TextInput],
List[TextInputPair],
List[PreTokenizedInput],
],
is_pair: bool = None,
boxes: Optional[List[List[List[int]]]] = None,
word_labels: Optional[List[List[int]]] = None,
add_special_tokens: bool = True,
padding_strategy: PaddingStrategy = PaddingStrategy.DO_NOT_PAD,
truncation_strategy: TruncationStrategy = TruncationStrategy.DO_NOT_TRUNCATE,
max_length: Optional[int] = None,
stride: int = 0,
pad_to_multiple_of: Optional[int] = None,
return_tensors: Optional[str] = None,
return_token_type_ids: Optional[bool] = None,
return_attention_mask: Optional[bool] = None,
return_overflowing_tokens: bool = False,
return_special_tokens_mask: bool = False,
return_offsets_mapping: bool = False,
return_length: bool = False,
verbose: bool = True,
) -> BatchEncoding:
if not isinstance(batch_text_or_text_pairs, list):
raise TypeError(f"batch_text_or_text_pairs has to be a list (got {type(batch_text_or_text_pairs)})")
# Set the truncation and padding strategy and restore the initial configuration
self.set_truncation_and_padding(
padding_strategy=padding_strategy,
truncation_strategy=truncation_strategy,
max_length=max_length,
stride=stride,
pad_to_multiple_of=pad_to_multiple_of,
)
if is_pair:
batch_text_or_text_pairs = [(text.split(), text_pair) for text, text_pair in batch_text_or_text_pairs]
encodings = self._tokenizer.encode_batch(
batch_text_or_text_pairs,
add_special_tokens=add_special_tokens,
is_pretokenized=True, # we set this to True as LayoutLMv2 always expects pretokenized inputs
)
# Convert encoding to dict
# `Tokens` has type: Tuple[
# List[Dict[str, List[List[int]]]] or List[Dict[str, 2D-Tensor]],
# List[EncodingFast]
# ]
# with nested dimensions corresponding to batch, overflows, sequence length
tokens_and_encodings = [
self._convert_encoding(
encoding=encoding,
return_token_type_ids=return_token_type_ids,
return_attention_mask=return_attention_mask,
return_overflowing_tokens=return_overflowing_tokens,
return_special_tokens_mask=return_special_tokens_mask,
return_offsets_mapping=True
if word_labels is not None
else return_offsets_mapping, # we use offsets to create the labels
return_length=return_length,
verbose=verbose,
)
for encoding in encodings
]
# Convert the output to have dict[list] from list[dict] and remove the additional overflows dimension
# From (variable) shape (batch, overflows, sequence length) to ~ (batch * overflows, sequence length)
# (we say ~ because the number of overflow varies with the example in the batch)
#
# To match each overflowing sample with the original sample in the batch
# we add an overflow_to_sample_mapping array (see below)
sanitized_tokens = {}
for key in tokens_and_encodings[0][0].keys():
stack = [e for item, _ in tokens_and_encodings for e in item[key]]
sanitized_tokens[key] = stack
sanitized_encodings = [e for _, item in tokens_and_encodings for e in item]
# If returning overflowing tokens, we need to return a mapping
# from the batch idx to the original sample
if return_overflowing_tokens:
overflow_to_sample_mapping = []
for i, (toks, _) in enumerate(tokens_and_encodings):
overflow_to_sample_mapping += [i] * len(toks["input_ids"])
sanitized_tokens["overflow_to_sample_mapping"] = overflow_to_sample_mapping
for input_ids in sanitized_tokens["input_ids"]:
self._eventual_warn_about_too_long_sequence(input_ids, max_length, verbose)
# create the token boxes
token_boxes = []
for batch_index in range(len(sanitized_tokens["input_ids"])):
if return_overflowing_tokens:
original_index = sanitized_tokens["overflow_to_sample_mapping"][batch_index]
else:
original_index = batch_index
token_boxes_example = []
for id, sequence_id, word_id in zip(
sanitized_tokens["input_ids"][batch_index],
sanitized_encodings[batch_index].sequence_ids,
sanitized_encodings[batch_index].word_ids,
):
if word_id is not None:
if is_pair and sequence_id == 0:
token_boxes_example.append(self.pad_token_box)
else:
token_boxes_example.append(boxes[original_index][word_id])
else:
if id == self.cls_token_id:
token_boxes_example.append(self.cls_token_box)
elif id == self.sep_token_id:
token_boxes_example.append(self.sep_token_box)
elif id == self.pad_token_id:
token_boxes_example.append(self.pad_token_box)
else:
raise ValueError("Id not recognized")
token_boxes.append(token_boxes_example)
sanitized_tokens["bbox"] = token_boxes
# optionally, create the labels
if word_labels is not None:
labels = []
for batch_index in range(len(sanitized_tokens["input_ids"])):
if return_overflowing_tokens:
original_index = sanitized_tokens["overflow_to_sample_mapping"][batch_index]
else:
original_index = batch_index
labels_example = []
for id, offset, word_id in zip(
sanitized_tokens["input_ids"][batch_index],
sanitized_tokens["offset_mapping"][batch_index],
sanitized_encodings[batch_index].word_ids,
):
if word_id is not None:
if self.only_label_first_subword:
if offset[0] == 0:
# Use the real label id for the first token of the word, and padding ids for the remaining tokens
labels_example.append(word_labels[original_index][word_id])
else:
labels_example.append(self.pad_token_label)
else:
labels_example.append(word_labels[original_index][word_id])
else:
labels_example.append(self.pad_token_label)
labels.append(labels_example)
sanitized_tokens["labels"] = labels
# finally, remove offsets if the user didn't want them
if not return_offsets_mapping:
del sanitized_tokens["offset_mapping"]
return BatchEncoding(sanitized_tokens, sanitized_encodings, tensor_type=return_tensors)
def _encode_plus(
self,
text: Union[TextInput, PreTokenizedInput],
text_pair: Optional[PreTokenizedInput] = None,
boxes: Optional[List[List[int]]] = None,
word_labels: Optional[List[int]] = None,
add_special_tokens: bool = True,
padding_strategy: PaddingStrategy = PaddingStrategy.DO_NOT_PAD,
truncation_strategy: TruncationStrategy = TruncationStrategy.DO_NOT_TRUNCATE,
max_length: Optional[int] = None,
stride: int = 0,
pad_to_multiple_of: Optional[int] = None,
return_tensors: Optional[bool] = None,
return_token_type_ids: Optional[bool] = None,
return_attention_mask: Optional[bool] = None,
return_overflowing_tokens: bool = False,
return_special_tokens_mask: bool = False,
return_offsets_mapping: bool = False,
return_length: bool = False,
verbose: bool = True,
**kwargs,
) -> BatchEncoding:
# make it a batched input
# 2 options:
# 1) only text, in case text must be a list of str
# 2) text + text_pair, in which case text = str and text_pair a list of str
batched_input = [(text, text_pair)] if text_pair else [text]
batched_boxes = [boxes]
batched_word_labels = [word_labels] if word_labels is not None else None
batched_output = self._batch_encode_plus(
batched_input,
is_pair=bool(text_pair is not None),
boxes=batched_boxes,
word_labels=batched_word_labels,
add_special_tokens=add_special_tokens,
padding_strategy=padding_strategy,
truncation_strategy=truncation_strategy,
max_length=max_length,
stride=stride,
pad_to_multiple_of=pad_to_multiple_of,
return_tensors=return_tensors,
return_token_type_ids=return_token_type_ids,
return_attention_mask=return_attention_mask,
return_overflowing_tokens=return_overflowing_tokens,
return_special_tokens_mask=return_special_tokens_mask,
return_offsets_mapping=return_offsets_mapping,
return_length=return_length,
verbose=verbose,
**kwargs,
)
# Return tensor is None, then we can remove the leading batch axis
# Overflowing tokens are returned as a batch of output so we keep them in this case
if return_tensors is None and not return_overflowing_tokens:
batched_output = BatchEncoding(
{
key: value[0] if len(value) > 0 and isinstance(value[0], list) else value
for key, value in batched_output.items()
},
batched_output.encodings,
)
self._eventual_warn_about_too_long_sequence(batched_output["input_ids"], max_length, verbose)
return batched_output
def _pad(
self,
encoded_inputs: Union[Dict[str, EncodedInput], BatchEncoding],
max_length: Optional[int] = None,
padding_strategy: PaddingStrategy = PaddingStrategy.DO_NOT_PAD,
pad_to_multiple_of: Optional[int] = None,
return_attention_mask: Optional[bool] = None,
) -> dict:
"""
Pad encoded inputs (on left/right and up to predefined length or max length in the batch)
Args:
encoded_inputs:
Dictionary of tokenized inputs (`List[int]`) or batch of tokenized inputs (`List[List[int]]`).
max_length: maximum length of the returned list and optionally padding length (see below).
Will truncate by taking into account the special tokens.
padding_strategy: PaddingStrategy to use for padding.
- PaddingStrategy.LONGEST Pad to the longest sequence in the batch
- PaddingStrategy.MAX_LENGTH: Pad to the max length (default)
- PaddingStrategy.DO_NOT_PAD: Do not pad
The tokenizer padding sides are defined in self.padding_side:
- 'left': pads on the left of the sequences
- 'right': pads on the right of the sequences
pad_to_multiple_of: (optional) Integer if set will pad the sequence to a multiple of the provided value.
This is especially useful to enable the use of Tensor Core on NVIDIA hardware with compute capability
`>= 7.5` (Volta).
return_attention_mask:
(optional) Set to False to avoid returning attention mask (default: set to model specifics)
"""
# Load from model defaults
if return_attention_mask is None:
return_attention_mask = "attention_mask" in self.model_input_names
required_input = encoded_inputs[self.model_input_names[0]]
if padding_strategy == PaddingStrategy.LONGEST:
max_length = len(required_input)
if max_length is not None and pad_to_multiple_of is not None and (max_length % pad_to_multiple_of != 0):
max_length = ((max_length // pad_to_multiple_of) + 1) * pad_to_multiple_of
needs_to_be_padded = padding_strategy != PaddingStrategy.DO_NOT_PAD and len(required_input) != max_length
# Initialize attention mask if not present.
if return_attention_mask and "attention_mask" not in encoded_inputs:
encoded_inputs["attention_mask"] = [1] * len(required_input)
if needs_to_be_padded:
difference = max_length - len(required_input)
if self.padding_side == "right":
if return_attention_mask:
encoded_inputs["attention_mask"] = encoded_inputs["attention_mask"] + [0] * difference
if "token_type_ids" in encoded_inputs:
encoded_inputs["token_type_ids"] = (
encoded_inputs["token_type_ids"] + [self.pad_token_type_id] * difference
)
if "bbox" in encoded_inputs:
encoded_inputs["bbox"] = encoded_inputs["bbox"] + [self.pad_token_box] * difference
if "labels" in encoded_inputs:
encoded_inputs["labels"] = encoded_inputs["labels"] + [self.pad_token_label] * difference
if "special_tokens_mask" in encoded_inputs:
encoded_inputs["special_tokens_mask"] = encoded_inputs["special_tokens_mask"] + [1] * difference
encoded_inputs[self.model_input_names[0]] = required_input + [self.pad_token_id] * difference
elif self.padding_side == "left":
if return_attention_mask:
encoded_inputs["attention_mask"] = [0] * difference + encoded_inputs["attention_mask"]
if "token_type_ids" in encoded_inputs:
encoded_inputs["token_type_ids"] = [self.pad_token_type_id] * difference + encoded_inputs[
"token_type_ids"
]
if "bbox" in encoded_inputs:
encoded_inputs["bbox"] = [self.pad_token_box] * difference + encoded_inputs["bbox"]
if "labels" in encoded_inputs:
encoded_inputs["labels"] = [self.pad_token_label] * difference + encoded_inputs["labels"]
if "special_tokens_mask" in encoded_inputs:
encoded_inputs["special_tokens_mask"] = [1] * difference + encoded_inputs["special_tokens_mask"]
encoded_inputs[self.model_input_names[0]] = [self.pad_token_id] * difference + required_input
else:
raise ValueError("Invalid padding strategy:" + str(self.padding_side))
return encoded_inputs
def build_inputs_with_special_tokens(self, token_ids_0, token_ids_1=None):
"""
Build model inputs from a sequence or a pair of sequence for sequence classification tasks by concatenating and
adding special tokens. A BERT sequence has the following format:
- single sequence: `[CLS] X [SEP]`
- pair of sequences: `[CLS] A [SEP] B [SEP]`
Args:
token_ids_0 (`List[int]`):
List of IDs to which the special tokens will be added.
token_ids_1 (`List[int]`, *optional*):
Optional second list of IDs for sequence pairs.
Returns:
`List[int]`: List of [input IDs](../glossary#input-ids) with the appropriate special tokens.
"""
output = [self.cls_token_id] + token_ids_0 + [self.sep_token_id]
if token_ids_1:
output += token_ids_1 + [self.sep_token_id]
return output
def create_token_type_ids_from_sequences(
self, token_ids_0: List[int], token_ids_1: Optional[List[int]] = None
) -> List[int]:
"""
Create a mask from the two sequences passed to be used in a sequence-pair classification task. A BERT sequence
pair mask has the following format: :: 0 0 0 0 0 0 0 0 0 0 0 1 1 1 1 1 1 1 1 1 | first sequence | second
sequence | If `token_ids_1` is `None`, this method only returns the first portion of the mask (0s).
Args:
token_ids_0 (`List[int]`):
List of IDs.
token_ids_1 (`List[int]`, *optional*):
Optional second list of IDs for sequence pairs.
Returns:
`List[int]`: List of [token type IDs](../glossary#token-type-ids) according to the given sequence(s).
"""
sep = [self.sep_token_id]
cls = [self.cls_token_id]
if token_ids_1 is None:
return len(cls + token_ids_0 + sep) * [0]
return len(cls + token_ids_0 + sep) * [0] + len(token_ids_1 + sep) * [1]
def save_vocabulary(self, save_directory: str, filename_prefix: Optional[str] = None) -> Tuple[str]:
files = self._tokenizer.model.save(save_directory, name=filename_prefix)
return tuple(files)
| transformers/src/transformers/models/layoutlmv2/tokenization_layoutlmv2_fast.py/0 | {
"file_path": "transformers/src/transformers/models/layoutlmv2/tokenization_layoutlmv2_fast.py",
"repo_id": "transformers",
"token_count": 17706
} | 339 |
# coding=utf-8
# Copyright 2021 Iz Beltagy, Matthew E. Peters, Arman Cohan and The HuggingFace Inc. team. All rights reserved.
#
# Licensed under the Apache License, Version 2.0 (the "License");
# you may not use this file except in compliance with the License.
# You may obtain a copy of the License at
#
# http://www.apache.org/licenses/LICENSE-2.0
#
# Unless required by applicable law or agreed to in writing, software
# distributed under the License is distributed on an "AS IS" BASIS,
# WITHOUT WARRANTIES OR CONDITIONS OF ANY KIND, either express or implied.
# See the License for the specific language governing permissions and
# limitations under the License.
""" PyTorch LED model."""
import math
import warnings
from dataclasses import dataclass
from typing import List, Optional, Tuple, Union
import torch
import torch.utils.checkpoint
from torch import nn
from torch.nn import BCEWithLogitsLoss, CrossEntropyLoss, MSELoss
from ...activations import ACT2FN
from ...modeling_attn_mask_utils import _create_4d_causal_attention_mask
from ...modeling_outputs import (
BaseModelOutputWithPastAndCrossAttentions,
Seq2SeqLMOutput,
Seq2SeqModelOutput,
Seq2SeqQuestionAnsweringModelOutput,
Seq2SeqSequenceClassifierOutput,
)
from ...modeling_utils import PreTrainedModel
from ...utils import (
ModelOutput,
add_code_sample_docstrings,
add_end_docstrings,
add_start_docstrings,
add_start_docstrings_to_model_forward,
logging,
replace_return_docstrings,
)
from .configuration_led import LEDConfig
logger = logging.get_logger(__name__)
_CHECKPOINT_FOR_DOC = "allenai/led-base-16384"
_CONFIG_FOR_DOC = "LEDConfig"
LED_PRETRAINED_MODEL_ARCHIVE_LIST = [
"allenai/led-base-16384",
# See all LED models at https://huggingface.co/models?filter=led
]
def shift_tokens_right(input_ids: torch.Tensor, pad_token_id: int, decoder_start_token_id: int):
"""
Shift input ids one token to the right.
"""
shifted_input_ids = input_ids.new_zeros(input_ids.shape)
shifted_input_ids[:, 1:] = input_ids[:, :-1].clone()
shifted_input_ids[:, 0] = decoder_start_token_id
if pad_token_id is None:
raise ValueError("config.pad_token_id has to be defined.")
# replace possible -100 values in labels by `pad_token_id`
shifted_input_ids.masked_fill_(shifted_input_ids == -100, pad_token_id)
return shifted_input_ids
def _prepare_4d_attention_mask_inverted(mask: torch.Tensor, dtype: torch.dtype, tgt_len: Optional[int] = None):
"""
Expands attention_mask from `[bsz, seq_len]` to `[bsz, 1, tgt_seq_len, src_seq_len]`.
"""
bsz, src_len = mask.size()
tgt_len = tgt_len if tgt_len is not None else src_len
expanded_mask = mask[:, None, None, :].expand(bsz, 1, tgt_len, src_len).to(dtype)
inverted_mask = 1.0 - expanded_mask
expanded_attention_mask = inverted_mask.masked_fill(inverted_mask.bool(), torch.finfo(dtype).min)
# make sure that global_attn_mask is positive
expanded_attention_mask = expanded_attention_mask * inverted_mask
return expanded_attention_mask
class LEDLearnedPositionalEmbedding(nn.Embedding):
"""
This module learns positional embeddings up to a fixed maximum size.
"""
def __init__(self, num_embeddings: int, embedding_dim: int):
super().__init__(num_embeddings, embedding_dim)
def forward(self, input_ids_shape: torch.Size, past_key_values_length: int = 0):
"""`input_ids_shape` is expected to be [bsz x seqlen]."""
bsz, seq_len = input_ids_shape[:2]
positions = torch.arange(
past_key_values_length, past_key_values_length + seq_len, dtype=torch.long, device=self.weight.device
)
return super().forward(positions)
# Copied from transformers.models.longformer.modeling_longformer.LongformerSelfAttention with Longformer->LEDEncoder
class LEDEncoderSelfAttention(nn.Module):
def __init__(self, config, layer_id):
super().__init__()
if config.hidden_size % config.num_attention_heads != 0:
raise ValueError(
f"The hidden size ({config.hidden_size}) is not a multiple of the number of attention "
f"heads ({config.num_attention_heads})"
)
self.num_heads = config.num_attention_heads
self.head_dim = int(config.hidden_size / config.num_attention_heads)
self.embed_dim = config.hidden_size
self.query = nn.Linear(config.hidden_size, self.embed_dim)
self.key = nn.Linear(config.hidden_size, self.embed_dim)
self.value = nn.Linear(config.hidden_size, self.embed_dim)
# separate projection layers for tokens with global attention
self.query_global = nn.Linear(config.hidden_size, self.embed_dim)
self.key_global = nn.Linear(config.hidden_size, self.embed_dim)
self.value_global = nn.Linear(config.hidden_size, self.embed_dim)
self.dropout = config.attention_probs_dropout_prob
self.layer_id = layer_id
attention_window = config.attention_window[self.layer_id]
assert (
attention_window % 2 == 0
), f"`attention_window` for layer {self.layer_id} has to be an even value. Given {attention_window}"
assert (
attention_window > 0
), f"`attention_window` for layer {self.layer_id} has to be positive. Given {attention_window}"
self.one_sided_attn_window_size = attention_window // 2
self.config = config
def forward(
self,
hidden_states,
attention_mask=None,
layer_head_mask=None,
is_index_masked=None,
is_index_global_attn=None,
is_global_attn=None,
output_attentions=False,
):
"""
[`LEDEncoderSelfAttention`] expects *len(hidden_states)* to be multiple of *attention_window*. Padding to
*attention_window* happens in [`LEDEncoderModel.forward`] to avoid redoing the padding on each layer.
The *attention_mask* is changed in [`LEDEncoderModel.forward`] from 0, 1, 2 to:
- -10000: no attention
- 0: local attention
- +10000: global attention
"""
hidden_states = hidden_states.transpose(0, 1)
# project hidden states
query_vectors = self.query(hidden_states)
key_vectors = self.key(hidden_states)
value_vectors = self.value(hidden_states)
seq_len, batch_size, embed_dim = hidden_states.size()
assert (
embed_dim == self.embed_dim
), f"hidden_states should have embed_dim = {self.embed_dim}, but has {embed_dim}"
# normalize query
query_vectors /= math.sqrt(self.head_dim)
query_vectors = query_vectors.view(seq_len, batch_size, self.num_heads, self.head_dim).transpose(0, 1)
key_vectors = key_vectors.view(seq_len, batch_size, self.num_heads, self.head_dim).transpose(0, 1)
attn_scores = self._sliding_chunks_query_key_matmul(
query_vectors, key_vectors, self.one_sided_attn_window_size
)
# values to pad for attention probs
remove_from_windowed_attention_mask = (attention_mask != 0)[:, :, None, None]
# cast to fp32/fp16 then replace 1's with -inf
float_mask = remove_from_windowed_attention_mask.type_as(query_vectors).masked_fill(
remove_from_windowed_attention_mask, torch.finfo(query_vectors.dtype).min
)
# diagonal mask with zeros everywhere and -inf inplace of padding
diagonal_mask = self._sliding_chunks_query_key_matmul(
float_mask.new_ones(size=float_mask.size()), float_mask, self.one_sided_attn_window_size
)
# pad local attention probs
attn_scores += diagonal_mask
assert list(attn_scores.size()) == [
batch_size,
seq_len,
self.num_heads,
self.one_sided_attn_window_size * 2 + 1,
], (
f"local_attn_probs should be of size ({batch_size}, {seq_len}, {self.num_heads},"
f" {self.one_sided_attn_window_size * 2 + 1}), but is of size {attn_scores.size()}"
)
# compute local attention probs from global attention keys and contact over window dim
if is_global_attn:
# compute global attn indices required through out forward fn
(
max_num_global_attn_indices,
is_index_global_attn_nonzero,
is_local_index_global_attn_nonzero,
is_local_index_no_global_attn_nonzero,
) = self._get_global_attn_indices(is_index_global_attn)
# calculate global attn probs from global key
global_key_attn_scores = self._concat_with_global_key_attn_probs(
query_vectors=query_vectors,
key_vectors=key_vectors,
max_num_global_attn_indices=max_num_global_attn_indices,
is_index_global_attn_nonzero=is_index_global_attn_nonzero,
is_local_index_global_attn_nonzero=is_local_index_global_attn_nonzero,
is_local_index_no_global_attn_nonzero=is_local_index_no_global_attn_nonzero,
)
# concat to local_attn_probs
# (batch_size, seq_len, num_heads, extra attention count + 2*window+1)
attn_scores = torch.cat((global_key_attn_scores, attn_scores), dim=-1)
# free memory
del global_key_attn_scores
attn_probs = nn.functional.softmax(
attn_scores, dim=-1, dtype=torch.float32
) # use fp32 for numerical stability
if layer_head_mask is not None:
assert layer_head_mask.size() == (
self.num_heads,
), f"Head mask for a single layer should be of size {(self.num_heads,)}, but is {layer_head_mask.size()}"
attn_probs = layer_head_mask.view(1, 1, -1, 1) * attn_probs
# softmax sometimes inserts NaN if all positions are masked, replace them with 0
attn_probs = torch.masked_fill(attn_probs, is_index_masked[:, :, None, None], 0.0)
attn_probs = attn_probs.type_as(attn_scores)
# free memory
del attn_scores
# apply dropout
attn_probs = nn.functional.dropout(attn_probs, p=self.dropout, training=self.training)
value_vectors = value_vectors.view(seq_len, batch_size, self.num_heads, self.head_dim).transpose(0, 1)
# compute local attention output with global attention value and add
if is_global_attn:
# compute sum of global and local attn
attn_output = self._compute_attn_output_with_global_indices(
value_vectors=value_vectors,
attn_probs=attn_probs,
max_num_global_attn_indices=max_num_global_attn_indices,
is_index_global_attn_nonzero=is_index_global_attn_nonzero,
is_local_index_global_attn_nonzero=is_local_index_global_attn_nonzero,
)
else:
# compute local attn only
attn_output = self._sliding_chunks_matmul_attn_probs_value(
attn_probs, value_vectors, self.one_sided_attn_window_size
)
assert attn_output.size() == (batch_size, seq_len, self.num_heads, self.head_dim), "Unexpected size"
attn_output = attn_output.transpose(0, 1).reshape(seq_len, batch_size, embed_dim).contiguous()
# compute value for global attention and overwrite to attention output
# TODO: remove the redundant computation
if is_global_attn:
global_attn_output, global_attn_probs = self._compute_global_attn_output_from_hidden(
hidden_states=hidden_states,
max_num_global_attn_indices=max_num_global_attn_indices,
layer_head_mask=layer_head_mask,
is_local_index_global_attn_nonzero=is_local_index_global_attn_nonzero,
is_index_global_attn_nonzero=is_index_global_attn_nonzero,
is_local_index_no_global_attn_nonzero=is_local_index_no_global_attn_nonzero,
is_index_masked=is_index_masked,
)
# get only non zero global attn output
nonzero_global_attn_output = global_attn_output[
is_local_index_global_attn_nonzero[0], :, is_local_index_global_attn_nonzero[1]
]
# overwrite values with global attention
attn_output[is_index_global_attn_nonzero[::-1]] = nonzero_global_attn_output.view(
len(is_local_index_global_attn_nonzero[0]), -1
)
# The attention weights for tokens with global attention are
# just filler values, they were never used to compute the output.
# Fill with 0 now, the correct values are in 'global_attn_probs'.
attn_probs[is_index_global_attn_nonzero] = 0
outputs = (attn_output.transpose(0, 1),)
if output_attentions:
outputs += (attn_probs,)
return outputs + (global_attn_probs,) if (is_global_attn and output_attentions) else outputs
@staticmethod
def _pad_and_transpose_last_two_dims(hidden_states_padded, padding):
"""pads rows and then flips rows and columns"""
hidden_states_padded = nn.functional.pad(
hidden_states_padded, padding
) # padding value is not important because it will be overwritten
hidden_states_padded = hidden_states_padded.view(
*hidden_states_padded.size()[:-2], hidden_states_padded.size(-1), hidden_states_padded.size(-2)
)
return hidden_states_padded
@staticmethod
def _pad_and_diagonalize(chunked_hidden_states):
"""
shift every row 1 step right, converting columns into diagonals.
Example:
```python
chunked_hidden_states: [
0.4983,
2.6918,
-0.0071,
1.0492,
-1.8348,
0.7672,
0.2986,
0.0285,
-0.7584,
0.4206,
-0.0405,
0.1599,
2.0514,
-1.1600,
0.5372,
0.2629,
]
window_overlap = num_rows = 4
```
(pad & diagonalize) => [ 0.4983, 2.6918, -0.0071, 1.0492, 0.0000, 0.0000, 0.0000
0.0000, -1.8348, 0.7672, 0.2986, 0.0285, 0.0000, 0.0000 0.0000, 0.0000, -0.7584, 0.4206,
-0.0405, 0.1599, 0.0000 0.0000, 0.0000, 0.0000, 2.0514, -1.1600, 0.5372, 0.2629 ]
"""
total_num_heads, num_chunks, window_overlap, hidden_dim = chunked_hidden_states.size()
chunked_hidden_states = nn.functional.pad(
chunked_hidden_states, (0, window_overlap + 1)
) # total_num_heads x num_chunks x window_overlap x (hidden_dim+window_overlap+1). Padding value is not important because it'll be overwritten
chunked_hidden_states = chunked_hidden_states.view(
total_num_heads, num_chunks, -1
) # total_num_heads x num_chunks x window_overlap*window_overlap+window_overlap
chunked_hidden_states = chunked_hidden_states[
:, :, :-window_overlap
] # total_num_heads x num_chunks x window_overlap*window_overlap
chunked_hidden_states = chunked_hidden_states.view(
total_num_heads, num_chunks, window_overlap, window_overlap + hidden_dim
)
chunked_hidden_states = chunked_hidden_states[:, :, :, :-1]
return chunked_hidden_states
@staticmethod
def _chunk(hidden_states, window_overlap, onnx_export: bool = False):
"""convert into overlapping chunks. Chunk size = 2w, overlap size = w"""
if not onnx_export:
# non-overlapping chunks of size = 2w
hidden_states = hidden_states.view(
hidden_states.size(0),
torch.div(hidden_states.size(1), (window_overlap * 2), rounding_mode="trunc"),
window_overlap * 2,
hidden_states.size(2),
)
# use `as_strided` to make the chunks overlap with an overlap size = window_overlap
chunk_size = list(hidden_states.size())
chunk_size[1] = chunk_size[1] * 2 - 1
chunk_stride = list(hidden_states.stride())
chunk_stride[1] = chunk_stride[1] // 2
return hidden_states.as_strided(size=chunk_size, stride=chunk_stride)
# When exporting to ONNX, use this separate logic
# have to use slow implementation since as_strided, unfold and 2d-tensor indexing aren't supported (yet) in ONNX export
# TODO replace this with
# > return hidden_states.unfold(dimension=1, size=window_overlap * 2, step=window_overlap).transpose(2, 3)
# once `unfold` is supported
# the case hidden_states.size(1) == window_overlap * 2 can also simply return hidden_states.unsqueeze(1), but that's control flow
chunk_size = [
hidden_states.size(0),
torch.div(hidden_states.size(1), window_overlap, rounding_mode="trunc") - 1,
window_overlap * 2,
hidden_states.size(2),
]
overlapping_chunks = torch.empty(chunk_size, device=hidden_states.device)
for chunk in range(chunk_size[1]):
overlapping_chunks[:, chunk, :, :] = hidden_states[
:, chunk * window_overlap : chunk * window_overlap + 2 * window_overlap, :
]
return overlapping_chunks
@staticmethod
def _mask_invalid_locations(input_tensor, affected_seq_len) -> torch.Tensor:
beginning_mask_2d = input_tensor.new_ones(affected_seq_len, affected_seq_len + 1).tril().flip(dims=[0])
beginning_mask = beginning_mask_2d[None, :, None, :]
ending_mask = beginning_mask.flip(dims=(1, 3))
beginning_input = input_tensor[:, :affected_seq_len, :, : affected_seq_len + 1]
beginning_mask = beginning_mask.expand(beginning_input.size())
input_tensor[:, :affected_seq_len, :, : affected_seq_len + 1] = torch.full_like(
beginning_input, -float("inf")
).where(beginning_mask.bool(), beginning_input)
ending_input = input_tensor[:, -affected_seq_len:, :, -(affected_seq_len + 1) :]
ending_mask = ending_mask.expand(ending_input.size())
input_tensor[:, -affected_seq_len:, :, -(affected_seq_len + 1) :] = torch.full_like(
ending_input, -float("inf")
).where(ending_mask.bool(), ending_input)
def _sliding_chunks_query_key_matmul(self, query: torch.Tensor, key: torch.Tensor, window_overlap: int):
"""
Matrix multiplication of query and key tensors using with a sliding window attention pattern. This
implementation splits the input into overlapping chunks of size 2w (e.g. 512 for pretrained LEDEncoder) with an
overlap of size window_overlap
"""
batch_size, seq_len, num_heads, head_dim = query.size()
assert (
seq_len % (window_overlap * 2) == 0
), f"Sequence length should be multiple of {window_overlap * 2}. Given {seq_len}"
assert query.size() == key.size()
chunks_count = torch.div(seq_len, window_overlap, rounding_mode="trunc") - 1
# group batch_size and num_heads dimensions into one, then chunk seq_len into chunks of size window_overlap * 2
query = query.transpose(1, 2).reshape(batch_size * num_heads, seq_len, head_dim)
key = key.transpose(1, 2).reshape(batch_size * num_heads, seq_len, head_dim)
query = self._chunk(query, window_overlap, getattr(self.config, "onnx_export", False))
key = self._chunk(key, window_overlap, getattr(self.config, "onnx_export", False))
# matrix multiplication
# bcxd: batch_size * num_heads x chunks x 2window_overlap x head_dim
# bcyd: batch_size * num_heads x chunks x 2window_overlap x head_dim
# bcxy: batch_size * num_heads x chunks x 2window_overlap x 2window_overlap
diagonal_chunked_attention_scores = torch.einsum("bcxd,bcyd->bcxy", (query, key)) # multiply
# convert diagonals into columns
diagonal_chunked_attention_scores = self._pad_and_transpose_last_two_dims(
diagonal_chunked_attention_scores, padding=(0, 0, 0, 1)
)
# allocate space for the overall attention matrix where the chunks are combined. The last dimension
# has (window_overlap * 2 + 1) columns. The first (window_overlap) columns are the window_overlap lower triangles (attention from a word to
# window_overlap previous words). The following column is attention score from each word to itself, then
# followed by window_overlap columns for the upper triangle.
diagonal_attention_scores = diagonal_chunked_attention_scores.new_zeros(
(batch_size * num_heads, chunks_count + 1, window_overlap, window_overlap * 2 + 1)
)
# copy parts from diagonal_chunked_attention_scores into the combined matrix of attentions
# - copying the main diagonal and the upper triangle
diagonal_attention_scores[:, :-1, :, window_overlap:] = diagonal_chunked_attention_scores[
:, :, :window_overlap, : window_overlap + 1
]
diagonal_attention_scores[:, -1, :, window_overlap:] = diagonal_chunked_attention_scores[
:, -1, window_overlap:, : window_overlap + 1
]
# - copying the lower triangle
diagonal_attention_scores[:, 1:, :, :window_overlap] = diagonal_chunked_attention_scores[
:, :, -(window_overlap + 1) : -1, window_overlap + 1 :
]
diagonal_attention_scores[:, 0, 1:window_overlap, 1:window_overlap] = diagonal_chunked_attention_scores[
:, 0, : window_overlap - 1, 1 - window_overlap :
]
# separate batch_size and num_heads dimensions again
diagonal_attention_scores = diagonal_attention_scores.view(
batch_size, num_heads, seq_len, 2 * window_overlap + 1
).transpose(2, 1)
self._mask_invalid_locations(diagonal_attention_scores, window_overlap)
return diagonal_attention_scores
def _sliding_chunks_matmul_attn_probs_value(
self, attn_probs: torch.Tensor, value: torch.Tensor, window_overlap: int
):
"""
Same as _sliding_chunks_query_key_matmul but for attn_probs and value tensors. Returned tensor will be of the
same shape as `attn_probs`
"""
batch_size, seq_len, num_heads, head_dim = value.size()
assert seq_len % (window_overlap * 2) == 0
assert attn_probs.size()[:3] == value.size()[:3]
assert attn_probs.size(3) == 2 * window_overlap + 1
chunks_count = torch.div(seq_len, window_overlap, rounding_mode="trunc") - 1
# group batch_size and num_heads dimensions into one, then chunk seq_len into chunks of size 2 window overlap
chunked_attn_probs = attn_probs.transpose(1, 2).reshape(
batch_size * num_heads,
torch.div(seq_len, window_overlap, rounding_mode="trunc"),
window_overlap,
2 * window_overlap + 1,
)
# group batch_size and num_heads dimensions into one
value = value.transpose(1, 2).reshape(batch_size * num_heads, seq_len, head_dim)
# pad seq_len with w at the beginning of the sequence and another window overlap at the end
padded_value = nn.functional.pad(value, (0, 0, window_overlap, window_overlap), value=-1)
# chunk padded_value into chunks of size 3 window overlap and an overlap of size window overlap
chunked_value_size = (batch_size * num_heads, chunks_count + 1, 3 * window_overlap, head_dim)
chunked_value_stride = padded_value.stride()
chunked_value_stride = (
chunked_value_stride[0],
window_overlap * chunked_value_stride[1],
chunked_value_stride[1],
chunked_value_stride[2],
)
chunked_value = padded_value.as_strided(size=chunked_value_size, stride=chunked_value_stride)
chunked_attn_probs = self._pad_and_diagonalize(chunked_attn_probs)
context = torch.einsum("bcwd,bcdh->bcwh", (chunked_attn_probs, chunked_value))
return context.view(batch_size, num_heads, seq_len, head_dim).transpose(1, 2)
@staticmethod
def _get_global_attn_indices(is_index_global_attn):
"""compute global attn indices required throughout forward pass"""
# helper variable
num_global_attn_indices = is_index_global_attn.long().sum(dim=1)
# max number of global attn indices in batch
max_num_global_attn_indices = num_global_attn_indices.max()
# indices of global attn
is_index_global_attn_nonzero = is_index_global_attn.nonzero(as_tuple=True)
# helper variable
is_local_index_global_attn = torch.arange(
max_num_global_attn_indices, device=is_index_global_attn.device
) < num_global_attn_indices.unsqueeze(dim=-1)
# location of the non-padding values within global attention indices
is_local_index_global_attn_nonzero = is_local_index_global_attn.nonzero(as_tuple=True)
# location of the padding values within global attention indices
is_local_index_no_global_attn_nonzero = (is_local_index_global_attn == 0).nonzero(as_tuple=True)
return (
max_num_global_attn_indices,
is_index_global_attn_nonzero,
is_local_index_global_attn_nonzero,
is_local_index_no_global_attn_nonzero,
)
def _concat_with_global_key_attn_probs(
self,
key_vectors,
query_vectors,
max_num_global_attn_indices,
is_index_global_attn_nonzero,
is_local_index_global_attn_nonzero,
is_local_index_no_global_attn_nonzero,
):
batch_size = key_vectors.shape[0]
# create only global key vectors
key_vectors_only_global = key_vectors.new_zeros(
batch_size, max_num_global_attn_indices, self.num_heads, self.head_dim
)
key_vectors_only_global[is_local_index_global_attn_nonzero] = key_vectors[is_index_global_attn_nonzero]
# (batch_size, seq_len, num_heads, max_num_global_attn_indices)
attn_probs_from_global_key = torch.einsum("blhd,bshd->blhs", (query_vectors, key_vectors_only_global))
# need to transpose since ONNX export only supports consecutive indexing: https://pytorch.org/docs/stable/onnx.html#writes-sets
attn_probs_from_global_key = attn_probs_from_global_key.transpose(1, 3)
attn_probs_from_global_key[
is_local_index_no_global_attn_nonzero[0], is_local_index_no_global_attn_nonzero[1], :, :
] = torch.finfo(attn_probs_from_global_key.dtype).min
attn_probs_from_global_key = attn_probs_from_global_key.transpose(1, 3)
return attn_probs_from_global_key
def _compute_attn_output_with_global_indices(
self,
value_vectors,
attn_probs,
max_num_global_attn_indices,
is_index_global_attn_nonzero,
is_local_index_global_attn_nonzero,
):
batch_size = attn_probs.shape[0]
# cut local attn probs to global only
attn_probs_only_global = attn_probs.narrow(-1, 0, max_num_global_attn_indices)
# get value vectors for global only
value_vectors_only_global = value_vectors.new_zeros(
batch_size, max_num_global_attn_indices, self.num_heads, self.head_dim
)
value_vectors_only_global[is_local_index_global_attn_nonzero] = value_vectors[is_index_global_attn_nonzero]
# use `matmul` because `einsum` crashes sometimes with fp16
# attn = torch.einsum('blhs,bshd->blhd', (selected_attn_probs, selected_v))
# compute attn output only global
attn_output_only_global = torch.matmul(
attn_probs_only_global.transpose(1, 2).clone(), value_vectors_only_global.transpose(1, 2).clone()
).transpose(1, 2)
# reshape attn probs
attn_probs_without_global = attn_probs.narrow(
-1, max_num_global_attn_indices, attn_probs.size(-1) - max_num_global_attn_indices
).contiguous()
# compute attn output with global
attn_output_without_global = self._sliding_chunks_matmul_attn_probs_value(
attn_probs_without_global, value_vectors, self.one_sided_attn_window_size
)
return attn_output_only_global + attn_output_without_global
def _compute_global_attn_output_from_hidden(
self,
hidden_states,
max_num_global_attn_indices,
layer_head_mask,
is_local_index_global_attn_nonzero,
is_index_global_attn_nonzero,
is_local_index_no_global_attn_nonzero,
is_index_masked,
):
seq_len, batch_size = hidden_states.shape[:2]
# prepare global hidden states
global_attn_hidden_states = hidden_states.new_zeros(max_num_global_attn_indices, batch_size, self.embed_dim)
global_attn_hidden_states[is_local_index_global_attn_nonzero[::-1]] = hidden_states[
is_index_global_attn_nonzero[::-1]
]
# global key, query, value
global_query_vectors_only_global = self.query_global(global_attn_hidden_states)
global_key_vectors = self.key_global(hidden_states)
global_value_vectors = self.value_global(hidden_states)
# normalize
global_query_vectors_only_global /= math.sqrt(self.head_dim)
# reshape
global_query_vectors_only_global = (
global_query_vectors_only_global.contiguous()
.view(max_num_global_attn_indices, batch_size * self.num_heads, self.head_dim)
.transpose(0, 1)
) # (batch_size * self.num_heads, max_num_global_attn_indices, head_dim)
global_key_vectors = (
global_key_vectors.contiguous().view(-1, batch_size * self.num_heads, self.head_dim).transpose(0, 1)
) # batch_size * self.num_heads, seq_len, head_dim)
global_value_vectors = (
global_value_vectors.contiguous().view(-1, batch_size * self.num_heads, self.head_dim).transpose(0, 1)
) # batch_size * self.num_heads, seq_len, head_dim)
# compute attn scores
global_attn_scores = torch.bmm(global_query_vectors_only_global, global_key_vectors.transpose(1, 2))
assert list(global_attn_scores.size()) == [
batch_size * self.num_heads,
max_num_global_attn_indices,
seq_len,
], (
"global_attn_scores have the wrong size. Size should be"
f" {(batch_size * self.num_heads, max_num_global_attn_indices, seq_len)}, but is"
f" {global_attn_scores.size()}."
)
global_attn_scores = global_attn_scores.view(batch_size, self.num_heads, max_num_global_attn_indices, seq_len)
# need to transpose since ONNX export only supports consecutive indexing: https://pytorch.org/docs/stable/onnx.html#writes-sets
global_attn_scores = global_attn_scores.transpose(1, 2)
global_attn_scores[
is_local_index_no_global_attn_nonzero[0], is_local_index_no_global_attn_nonzero[1], :, :
] = torch.finfo(global_attn_scores.dtype).min
global_attn_scores = global_attn_scores.transpose(1, 2)
global_attn_scores = global_attn_scores.masked_fill(
is_index_masked[:, None, None, :],
torch.finfo(global_attn_scores.dtype).min,
)
global_attn_scores = global_attn_scores.view(batch_size * self.num_heads, max_num_global_attn_indices, seq_len)
# compute global attn probs
global_attn_probs_float = nn.functional.softmax(
global_attn_scores, dim=-1, dtype=torch.float32
) # use fp32 for numerical stability
# apply layer head masking
if layer_head_mask is not None:
assert layer_head_mask.size() == (
self.num_heads,
), f"Head mask for a single layer should be of size {(self.num_heads,)}, but is {layer_head_mask.size()}"
global_attn_probs_float = layer_head_mask.view(1, -1, 1, 1) * global_attn_probs_float.view(
batch_size, self.num_heads, max_num_global_attn_indices, seq_len
)
global_attn_probs_float = global_attn_probs_float.view(
batch_size * self.num_heads, max_num_global_attn_indices, seq_len
)
global_attn_probs = nn.functional.dropout(
global_attn_probs_float.type_as(global_attn_scores), p=self.dropout, training=self.training
)
# global attn output
global_attn_output = torch.bmm(global_attn_probs, global_value_vectors)
assert list(global_attn_output.size()) == [
batch_size * self.num_heads,
max_num_global_attn_indices,
self.head_dim,
], (
"global_attn_output tensor has the wrong size. Size should be"
f" {(batch_size * self.num_heads, max_num_global_attn_indices, self.head_dim)}, but is"
f" {global_attn_output.size()}."
)
global_attn_probs = global_attn_probs.view(batch_size, self.num_heads, max_num_global_attn_indices, seq_len)
global_attn_output = global_attn_output.view(
batch_size, self.num_heads, max_num_global_attn_indices, self.head_dim
)
return global_attn_output, global_attn_probs
class LEDEncoderAttention(nn.Module):
def __init__(self, config, layer_id):
super().__init__()
self.longformer_self_attn = LEDEncoderSelfAttention(config, layer_id=layer_id)
self.output = nn.Linear(config.d_model, config.d_model)
def forward(
self,
hidden_states: torch.Tensor,
attention_mask: Optional[torch.Tensor] = None,
layer_head_mask: Optional[torch.Tensor] = None,
is_index_masked: Optional[torch.Tensor] = None,
is_index_global_attn: Optional[torch.Tensor] = None,
is_global_attn: Optional[bool] = None,
output_attentions: bool = False,
) -> Tuple[torch.Tensor, Optional[torch.Tensor], Optional[Tuple[torch.Tensor]]]:
"""Input shape: Batch x Time x Channel"""
self_outputs = self.longformer_self_attn(
hidden_states=hidden_states,
attention_mask=attention_mask,
layer_head_mask=layer_head_mask,
is_index_masked=is_index_masked,
is_index_global_attn=is_index_global_attn,
is_global_attn=is_global_attn,
output_attentions=output_attentions,
)
attn_output = self.output(self_outputs[0])
outputs = (attn_output,) + self_outputs[1:]
return outputs
class LEDDecoderAttention(nn.Module):
"""Multi-headed attention from 'Attention Is All You Need' paper"""
def __init__(
self,
embed_dim: int,
num_heads: int,
dropout: float = 0.0,
is_decoder: bool = False,
bias: bool = True,
):
super().__init__()
self.embed_dim = embed_dim
self.num_heads = num_heads
self.dropout = dropout
self.head_dim = embed_dim // num_heads
if self.head_dim * num_heads != self.embed_dim:
raise ValueError(
f"embed_dim must be divisible by num_heads (got `embed_dim`: {self.embed_dim} and `num_heads`:"
f" {num_heads})."
)
self.scaling = self.head_dim**-0.5
self.is_decoder = is_decoder
self.k_proj = nn.Linear(embed_dim, embed_dim, bias=bias)
self.v_proj = nn.Linear(embed_dim, embed_dim, bias=bias)
self.q_proj = nn.Linear(embed_dim, embed_dim, bias=bias)
self.out_proj = nn.Linear(embed_dim, embed_dim, bias=bias)
def _shape(self, tensor: torch.Tensor, seq_len: int, bsz: int):
return tensor.view(bsz, seq_len, self.num_heads, self.head_dim).transpose(1, 2).contiguous()
def forward(
self,
hidden_states: torch.Tensor,
key_value_states: Optional[torch.Tensor] = None,
past_key_value: Optional[Tuple[torch.Tensor]] = None,
attention_mask: Optional[torch.Tensor] = None,
layer_head_mask: Optional[torch.Tensor] = None,
output_attentions: bool = False,
) -> Tuple[torch.Tensor, Optional[torch.Tensor], Optional[Tuple[torch.Tensor]]]:
"""Input shape: Batch x Time x Channel"""
# if key_value_states are provided this layer is used as a cross-attention layer
# for the decoder
is_cross_attention = key_value_states is not None
bsz, tgt_len, embed_dim = hidden_states.size()
# get query proj
query_states = self.q_proj(hidden_states) * self.scaling
# get key, value proj
if is_cross_attention and past_key_value is not None:
# reuse k,v, cross_attentions
key_states = past_key_value[0]
value_states = past_key_value[1]
elif is_cross_attention:
# cross_attentions
key_states = self._shape(self.k_proj(key_value_states), -1, bsz)
value_states = self._shape(self.v_proj(key_value_states), -1, bsz)
elif past_key_value is not None:
# reuse k, v, self_attention
key_states = self._shape(self.k_proj(hidden_states), -1, bsz)
value_states = self._shape(self.v_proj(hidden_states), -1, bsz)
key_states = torch.cat([past_key_value[0], key_states], dim=2)
value_states = torch.cat([past_key_value[1], value_states], dim=2)
else:
# self_attention
key_states = self._shape(self.k_proj(hidden_states), -1, bsz)
value_states = self._shape(self.v_proj(hidden_states), -1, bsz)
if self.is_decoder:
# if cross_attention save Tuple(torch.Tensor, torch.Tensor) of all cross attention key/value_states.
# Further calls to cross_attention layer can then reuse all cross-attention
# key/value_states (first "if" case)
# if uni-directional self-attention (decoder) save Tuple(torch.Tensor, torch.Tensor) of
# all previous decoder key/value_states. Further calls to uni-directional self-attention
# can concat previous decoder key/value_states to current projected key/value_states (third "elif" case)
# if encoder bi-directional self-attention `past_key_value` is always `None`
past_key_value = (key_states, value_states)
proj_shape = (bsz * self.num_heads, -1, self.head_dim)
query_states = self._shape(query_states, tgt_len, bsz).view(*proj_shape)
key_states = key_states.view(*proj_shape)
value_states = value_states.view(*proj_shape)
src_len = key_states.size(1)
attn_weights = torch.bmm(query_states, key_states.transpose(1, 2))
if attn_weights.size() != (bsz * self.num_heads, tgt_len, src_len):
raise ValueError(
f"Attention weights should be of size {(bsz * self.num_heads, tgt_len, src_len)}, but is"
f" {attn_weights.size()}"
)
if attention_mask is not None:
if attention_mask.size() != (bsz, 1, tgt_len, src_len):
raise ValueError(
f"Attention mask should be of size {(bsz, 1, tgt_len, src_len)}, but is {attention_mask.size()}"
)
attn_weights = attn_weights.view(bsz, self.num_heads, tgt_len, src_len) + attention_mask
attn_weights = attn_weights.view(bsz * self.num_heads, tgt_len, src_len)
attn_weights = nn.functional.softmax(attn_weights, dim=-1)
if layer_head_mask is not None:
if layer_head_mask.size() != (self.num_heads,):
raise ValueError(
f"Head mask for a single layer should be of size {(self.num_heads,)}, but is"
f" {layer_head_mask.size()}"
)
attn_weights = layer_head_mask.view(1, -1, 1, 1) * attn_weights.view(bsz, self.num_heads, tgt_len, src_len)
attn_weights = attn_weights.view(bsz * self.num_heads, tgt_len, src_len)
if output_attentions:
# this operation is a bit awkward, but it's required to
# make sure that attn_weights keeps its gradient.
# In order to do so, attn_weights have to be reshaped
# twice and have to be reused in the following
attn_weights_reshaped = attn_weights.view(bsz, self.num_heads, tgt_len, src_len)
attn_weights = attn_weights_reshaped.view(bsz * self.num_heads, tgt_len, src_len)
else:
attn_weights_reshaped = None
attn_probs = nn.functional.dropout(attn_weights, p=self.dropout, training=self.training)
attn_output = torch.bmm(attn_probs, value_states)
if attn_output.size() != (bsz * self.num_heads, tgt_len, self.head_dim):
raise ValueError(
f"`attn_output` should be of size {(bsz, self.num_heads, tgt_len, self.head_dim)}, but is"
f" {attn_output.size()}"
)
attn_output = (
attn_output.view(bsz, self.num_heads, tgt_len, self.head_dim)
.transpose(1, 2)
.reshape(bsz, tgt_len, embed_dim)
)
attn_output = self.out_proj(attn_output)
return attn_output, attn_weights_reshaped, past_key_value
class LEDEncoderLayer(nn.Module):
def __init__(self, config: LEDConfig, layer_id: int):
super().__init__()
self.embed_dim = config.d_model
self.self_attn = LEDEncoderAttention(config, layer_id)
self.self_attn_layer_norm = nn.LayerNorm(self.embed_dim)
self.dropout = config.dropout
self.activation_fn = ACT2FN[config.activation_function]
self.activation_dropout = config.activation_dropout
self.fc1 = nn.Linear(self.embed_dim, config.encoder_ffn_dim)
self.fc2 = nn.Linear(config.encoder_ffn_dim, self.embed_dim)
self.final_layer_norm = nn.LayerNorm(self.embed_dim)
def forward(
self,
hidden_states: torch.Tensor,
attention_mask: torch.Tensor,
layer_head_mask: torch.Tensor,
is_index_masked=None,
is_index_global_attn=None,
is_global_attn=None,
output_attentions=False,
):
"""
Args:
hidden_states (`torch.FloatTensor`): input to the layer of shape *(batch, seq_len, embed_dim)*
attention_mask (`torch.FloatTensor`): attention mask of size
*(batch, 1, tgt_len, src_len)* where padding elements are indicated by very large negative values.
layer_head_mask (`torch.FloatTensor`): mask for attention heads in a given layer of size
*(encoder_attention_heads,)*.
"""
residual = hidden_states
attn_outputs = self.self_attn(
hidden_states=hidden_states,
attention_mask=attention_mask,
layer_head_mask=layer_head_mask,
is_index_masked=is_index_masked,
is_index_global_attn=is_index_global_attn,
is_global_attn=is_global_attn,
output_attentions=output_attentions,
)
hidden_states = attn_outputs[0]
hidden_states = nn.functional.dropout(hidden_states, p=self.dropout, training=self.training)
hidden_states = residual + hidden_states
hidden_states = self.self_attn_layer_norm(hidden_states)
residual = hidden_states
hidden_states = self.activation_fn(self.fc1(hidden_states))
hidden_states = nn.functional.dropout(hidden_states, p=self.activation_dropout, training=self.training)
hidden_states = self.fc2(hidden_states)
hidden_states = nn.functional.dropout(hidden_states, p=self.dropout, training=self.training)
hidden_states = residual + hidden_states
hidden_states = self.final_layer_norm(hidden_states)
if hidden_states.dtype == torch.float16 and (
torch.isinf(hidden_states).any() or torch.isnan(hidden_states).any()
):
clamp_value = torch.finfo(hidden_states.dtype).max - 1000
hidden_states = torch.clamp(hidden_states, min=-clamp_value, max=clamp_value)
return (hidden_states,) + attn_outputs[1:]
class LEDDecoderLayer(nn.Module):
def __init__(self, config: LEDConfig):
super().__init__()
self.embed_dim = config.d_model
self.self_attn = LEDDecoderAttention(
embed_dim=self.embed_dim,
num_heads=config.decoder_attention_heads,
dropout=config.attention_dropout,
is_decoder=True,
)
self.dropout = config.dropout
self.activation_fn = ACT2FN[config.activation_function]
self.activation_dropout = config.activation_dropout
self.self_attn_layer_norm = nn.LayerNorm(self.embed_dim)
self.encoder_attn = LEDDecoderAttention(
self.embed_dim,
config.decoder_attention_heads,
dropout=config.attention_dropout,
is_decoder=True,
)
self.encoder_attn_layer_norm = nn.LayerNorm(self.embed_dim)
self.fc1 = nn.Linear(self.embed_dim, config.decoder_ffn_dim)
self.fc2 = nn.Linear(config.decoder_ffn_dim, self.embed_dim)
self.final_layer_norm = nn.LayerNorm(self.embed_dim)
def forward(
self,
hidden_states: torch.Tensor,
attention_mask: Optional[torch.Tensor] = None,
encoder_hidden_states: Optional[torch.Tensor] = None,
encoder_attention_mask: Optional[torch.Tensor] = None,
layer_head_mask: Optional[torch.Tensor] = None,
cross_attn_layer_head_mask: Optional[torch.Tensor] = None,
past_key_value: Optional[Tuple[torch.Tensor]] = None,
output_attentions: Optional[bool] = False,
use_cache: Optional[bool] = True,
):
"""
Args:
hidden_states (`torch.FloatTensor`): input to the layer of shape *(batch, seq_len, embed_dim)*
attention_mask (`torch.FloatTensor`): attention mask of size
*(batch, 1, tgt_len, src_len)* where padding elements are indicated by very large negative values.
encoder_hidden_states (`torch.FloatTensor`):
cross attention input to the layer of shape *(batch, seq_len, embed_dim)*
encoder_attention_mask (`torch.FloatTensor`): encoder attention mask of size
*(batch, 1, tgt_len, src_len)* where padding elements are indicated by very large negative values.
layer_head_mask (`torch.FloatTensor`): mask for attention heads in a given layer of size
*(decoder_attention_heads,)*.
cross_attn_layer_head_mask (`torch.FloatTensor`): mask for encoder attention heads in a given layer of
size *(decoder_attention_heads,)*.
past_key_value (`Tuple(torch.FloatTensor)`): cached past key and value projection states
output_attentions (`bool`): Whether the base model outputs attentions.
This requires the attentions tensor to be reshaped in this function.
"""
residual = hidden_states
# Self-Attention
# decoder uni-directional self-attention cached key/values tuple is at positions 1,2
self_attn_past_key_value = past_key_value[:2] if past_key_value is not None else None
# add present self-attn cache to positions 1,2 of present_key_value tuple
hidden_states, self_attn_weights, present_key_value = self.self_attn(
hidden_states=hidden_states,
past_key_value=self_attn_past_key_value,
attention_mask=attention_mask,
layer_head_mask=layer_head_mask,
output_attentions=output_attentions,
)
hidden_states = nn.functional.dropout(hidden_states, p=self.dropout, training=self.training)
hidden_states = residual + hidden_states
hidden_states = self.self_attn_layer_norm(hidden_states)
# Cross-Attention Block
cross_attn_present_key_value = None
cross_attn_weights = None
if encoder_hidden_states is not None:
residual = hidden_states
# cross_attn cached key/values tuple is at positions 3,4 of present_key_value tuple
cross_attn_past_key_value = past_key_value[-2:] if past_key_value is not None else None
hidden_states, cross_attn_weights, cross_attn_present_key_value = self.encoder_attn(
hidden_states=hidden_states,
key_value_states=encoder_hidden_states,
attention_mask=encoder_attention_mask,
layer_head_mask=cross_attn_layer_head_mask,
past_key_value=cross_attn_past_key_value,
output_attentions=output_attentions,
)
hidden_states = nn.functional.dropout(hidden_states, p=self.dropout, training=self.training)
hidden_states = residual + hidden_states
hidden_states = self.encoder_attn_layer_norm(hidden_states)
# add cross-attn to positions 3,4 of present_key_value tuple
present_key_value = present_key_value + cross_attn_present_key_value
# Fully Connected
residual = hidden_states
hidden_states = self.activation_fn(self.fc1(hidden_states))
hidden_states = nn.functional.dropout(hidden_states, p=self.activation_dropout, training=self.training)
hidden_states = self.fc2(hidden_states)
hidden_states = nn.functional.dropout(hidden_states, p=self.dropout, training=self.training)
hidden_states = residual + hidden_states
hidden_states = self.final_layer_norm(hidden_states)
outputs = (hidden_states,)
if output_attentions:
outputs += (self_attn_weights, cross_attn_weights)
if use_cache:
outputs += (present_key_value,)
return outputs
class LEDClassificationHead(nn.Module):
"""Head for sentence-level classification tasks."""
def __init__(
self,
input_dim: int,
inner_dim: int,
num_classes: int,
pooler_dropout: float,
):
super().__init__()
self.dense = nn.Linear(input_dim, inner_dim)
self.dropout = nn.Dropout(p=pooler_dropout)
self.out_proj = nn.Linear(inner_dim, num_classes)
def forward(self, hidden_states: torch.Tensor):
hidden_states = self.dropout(hidden_states)
hidden_states = self.dense(hidden_states)
hidden_states = torch.tanh(hidden_states)
hidden_states = self.dropout(hidden_states)
hidden_states = self.out_proj(hidden_states)
return hidden_states
class LEDPreTrainedModel(PreTrainedModel):
config_class = LEDConfig
base_model_prefix = "led"
supports_gradient_checkpointing = True
def _init_weights(self, module):
std = self.config.init_std
if isinstance(module, nn.Linear):
module.weight.data.normal_(mean=0.0, std=std)
if module.bias is not None:
module.bias.data.zero_()
elif isinstance(module, nn.Embedding):
module.weight.data.normal_(mean=0.0, std=std)
if module.padding_idx is not None:
module.weight.data[module.padding_idx].zero_()
@property
def dummy_inputs(self):
pad_token = self.config.pad_token_id
input_ids = torch.tensor([[0, 6, 10, 4, 2], [0, 8, 12, 2, pad_token]], device=self.device)
dummy_inputs = {
"attention_mask": input_ids.ne(pad_token),
"input_ids": input_ids,
}
return dummy_inputs
@dataclass
# Copied from transformers.models.longformer.modeling_longformer.LongformerBaseModelOutput with Longformer->LEDEncoder
class LEDEncoderBaseModelOutput(ModelOutput):
"""
Base class for LEDEncoder's outputs, with potential hidden states, local and global attentions.
Args:
last_hidden_state (`torch.FloatTensor` of shape `(batch_size, sequence_length, hidden_size)`):
Sequence of hidden-states at the output of the last layer of the model.
hidden_states (`tuple(torch.FloatTensor)`, *optional*, returned when `output_hidden_states=True` is passed or when `config.output_hidden_states=True`):
Tuple of `torch.FloatTensor` (one for the output of the embeddings + one for the output of each layer) of
shape `(batch_size, sequence_length, hidden_size)`.
Hidden-states of the model at the output of each layer plus the initial embedding outputs.
attentions (`tuple(torch.FloatTensor)`, *optional*, returned when `output_attentions=True` is passed or when `config.output_attentions=True`):
Tuple of `torch.FloatTensor` (one for each layer) of shape `(batch_size, num_heads, sequence_length, x +
attention_window + 1)`, where `x` is the number of tokens with global attention mask.
Local attentions weights after the attention softmax, used to compute the weighted average in the
self-attention heads. Those are the attention weights from every token in the sequence to every token with
global attention (first `x` values) and to every token in the attention window (remaining `attention_window
+ 1` values). Note that the first `x` values refer to tokens with fixed positions in the text, but the
remaining `attention_window + 1` values refer to tokens with relative positions: the attention weight of a
token to itself is located at index `x + attention_window / 2` and the `attention_window / 2` preceding
(succeeding) values are the attention weights to the `attention_window / 2` preceding (succeeding) tokens.
If the attention window contains a token with global attention, the attention weight at the corresponding
index is set to 0; the value should be accessed from the first `x` attention weights. If a token has global
attention, the attention weights to all other tokens in `attentions` is set to 0, the values should be
accessed from `global_attentions`.
global_attentions (`tuple(torch.FloatTensor)`, *optional*, returned when `output_attentions=True` is passed or when `config.output_attentions=True`):
Tuple of `torch.FloatTensor` (one for each layer) of shape `(batch_size, num_heads, sequence_length, x)`,
where `x` is the number of tokens with global attention mask.
Global attentions weights after the attention softmax, used to compute the weighted average in the
self-attention heads. Those are the attention weights from every token with global attention to every token
in the sequence.
"""
last_hidden_state: torch.FloatTensor
hidden_states: Optional[Tuple[torch.FloatTensor, ...]] = None
attentions: Optional[Tuple[torch.FloatTensor, ...]] = None
global_attentions: Optional[Tuple[torch.FloatTensor, ...]] = None
@dataclass
class LEDSeq2SeqModelOutput(ModelOutput):
"""
Base class for model encoder's outputs that also contains : pre-computed hidden states that can speed up sequential
decoding.
Args:
last_hidden_state (`torch.FloatTensor` of shape `(batch_size, sequence_length, hidden_size)`):
Sequence of hidden-states at the output of the last layer of the decoder of the model.
If `past_key_values` is used only the last hidden-state of the sequences of shape `(batch_size, 1,
hidden_size)` is output.
past_key_values (`List[torch.FloatTensor]`, *optional*, returned when `use_cache=True` is passed or when `config.use_cache=True`):
List of `torch.FloatTensor` of length `config.n_layers`, with each tensor of shape `(2, batch_size,
num_heads, sequence_length, embed_size_per_head)`).
Contains pre-computed hidden-states (key and values in the attention blocks) of the decoder that can be
used (see `past_key_values` input) to speed up sequential decoding.
decoder_hidden_states (`tuple(torch.FloatTensor)`, *optional*, returned when `output_hidden_states=True` is passed or when `config.output_hidden_states=True`):
Tuple of `torch.FloatTensor` (one for the output of the embeddings + one for the output of each layer) of
shape `(batch_size, sequence_length, hidden_size)`.
Hidden-states of the decoder at the output of each layer plus the initial embedding outputs.
decoder_attentions (`tuple(torch.FloatTensor)`, *optional*, returned when `output_attentions=True` is passed or when `config.output_attentions=True`):
Tuple of `torch.FloatTensor` (one for each layer) of shape `(batch_size, num_heads, sequence_length,
sequence_length)`.
Attentions weights of the decoder, after the attention softmax, used to compute the weighted average in the
self-attention heads.
cross_attentions (`tuple(torch.FloatTensor)`, *optional*, returned when `output_attentions=True` is passed or when `config.output_attentions=True`):
Tuple of `torch.FloatTensor` (one for each layer) of shape `(batch_size, num_heads, sequence_length,
sequence_length)`.
Attentions weights of the decoder's cross-attention layer, after the attention softmax, used to compute the
weighted average in the cross-attention heads.
encoder_last_hidden_state (`torch.FloatTensor` of shape `(batch_size, sequence_length, hidden_size)`, *optional*):
Sequence of hidden-states at the output of the last layer of the encoder of the model.
encoder_hidden_states (`tuple(torch.FloatTensor)`, *optional*, returned when `output_hidden_states=True` is passed or when `config.output_hidden_states=True`):
Tuple of `torch.FloatTensor` (one for the output of the embeddings + one for the output of each layer) of
shape `(batch_size, sequence_length, hidden_size)`.
Hidden-states of the encoder at the output of each layer plus the initial embedding outputs.
encoder_attentions (`tuple(torch.FloatTensor)`, *optional*, returned when `output_attentions=True` is passed or when `config.output_attentions=True`):
Tuple of `torch.FloatTensor` (one for each layer) of shape `(batch_size, num_heads, sequence_length,
sequence_length)`.
Attentions weights of the encoder, after the attention softmax, used to compute the weighted average in the
self-attention heads.
encoder_global_attentions (`tuple(torch.FloatTensor)`, *optional*, returned when `output_attentions=True` is passed or when `config.output_attentions=True`):
Tuple of `torch.FloatTensor` (one for each layer) of shape `(batch_size, num_heads, sequence_length, x)`,
where `x` is the number of tokens with global attention mask.
Global attentions weights after the attention softmax, used to compute the weighted average in the
self-attention heads. Those are the attention weights from every token with global attention to every token
in the sequence.
"""
last_hidden_state: torch.FloatTensor = None
past_key_values: Optional[List[torch.FloatTensor]] = None
decoder_hidden_states: Optional[Tuple[torch.FloatTensor, ...]] = None
decoder_attentions: Optional[Tuple[torch.FloatTensor, ...]] = None
cross_attentions: Optional[Tuple[torch.FloatTensor, ...]] = None
encoder_last_hidden_state: Optional[torch.FloatTensor] = None
encoder_hidden_states: Optional[Tuple[torch.FloatTensor, ...]] = None
encoder_attentions: Optional[Tuple[torch.FloatTensor, ...]] = None
encoder_global_attentions: Optional[Tuple[torch.FloatTensor, ...]] = None
@dataclass
class LEDSeq2SeqLMOutput(ModelOutput):
"""
Base class for sequence-to-sequence language models outputs.
Args:
loss (`torch.FloatTensor` of shape `(1,)`, *optional*, returned when `labels` is provided):
Language modeling loss.
logits (`torch.FloatTensor` of shape `(batch_size, sequence_length, config.vocab_size)`):
Prediction scores of the language modeling head (scores for each vocabulary token before SoftMax).
past_key_values (`List[torch.FloatTensor]`, *optional*, returned when `use_cache=True` is passed or when `config.use_cache=True`):
List of `torch.FloatTensor` of length `config.n_layers`, with each tensor of shape `(2, batch_size,
num_heads, sequence_length, embed_size_per_head)`).
Contains pre-computed hidden-states (key and values in the attention blocks) of the decoder that can be
used (see `past_key_values` input) to speed up sequential decoding.
decoder_hidden_states (`tuple(torch.FloatTensor)`, *optional*, returned when `output_hidden_states=True` is passed or when `config.output_hidden_states=True`):
Tuple of `torch.FloatTensor` (one for the output of the embeddings + one for the output of each layer) of
shape `(batch_size, sequence_length, hidden_size)`.
Hidden-states of the decoder at the output of each layer plus the initial embedding outputs.
decoder_attentions (`tuple(torch.FloatTensor)`, *optional*, returned when `output_attentions=True` is passed or when `config.output_attentions=True`):
Tuple of `torch.FloatTensor` (one for each layer) of shape `(batch_size, num_heads, sequence_length,
sequence_length)`.
Attentions weights of the decoder, after the attention softmax, used to compute the weighted average in the
self-attention heads.
cross_attentions (`tuple(torch.FloatTensor)`, *optional*, returned when `output_attentions=True` is passed or when `config.output_attentions=True`):
Tuple of `torch.FloatTensor` (one for each layer) of shape `(batch_size, num_heads, sequence_length,
sequence_length)`.
Attentions weights of the decoder's cross-attention layer, after the attention softmax, used to compute the
weighted average in the cross-attention heads.
encoder_last_hidden_state (`torch.FloatTensor` of shape `(batch_size, sequence_length, hidden_size)`, *optional*):
Sequence of hidden-states at the output of the last layer of the encoder of the model.
encoder_hidden_states (`tuple(torch.FloatTensor)`, *optional*, returned when `output_hidden_states=True` is passed or when `config.output_hidden_states=True`):
Tuple of `torch.FloatTensor` (one for the output of the embeddings + one for the output of each layer) of
shape `(batch_size, sequence_length, hidden_size)`.
Hidden-states of the encoder at the output of each layer plus the initial embedding outputs.
encoder_attentions (`tuple(torch.FloatTensor)`, *optional*, returned when `output_attentions=True` is passed or when `config.output_attentions=True`):
Tuple of `torch.FloatTensor` (one for each layer) of shape `(batch_size, num_heads, sequence_length,
sequence_length)`.
Attentions weights of the encoder, after the attention softmax, used to compute the weighted average in the
self-attention heads.
encoder_global_attentions (`tuple(torch.FloatTensor)`, *optional*, returned when `output_attentions=True` is passed or when `config.output_attentions=True`):
Tuple of `torch.FloatTensor` (one for each layer) of shape `(batch_size, num_heads, sequence_length, x)`,
where `x` is the number of tokens with global attention mask.
Global attentions weights after the attention softmax, used to compute the weighted average in the
self-attention heads. Those are the attention weights from every token with global attention to every token
in the sequence.
"""
loss: Optional[torch.FloatTensor] = None
logits: torch.FloatTensor = None
past_key_values: Optional[List[torch.FloatTensor]] = None
decoder_hidden_states: Optional[Tuple[torch.FloatTensor, ...]] = None
decoder_attentions: Optional[Tuple[torch.FloatTensor, ...]] = None
cross_attentions: Optional[Tuple[torch.FloatTensor, ...]] = None
encoder_last_hidden_state: Optional[torch.FloatTensor] = None
encoder_hidden_states: Optional[Tuple[torch.FloatTensor, ...]] = None
encoder_attentions: Optional[Tuple[torch.FloatTensor, ...]] = None
encoder_global_attentions: Optional[Tuple[torch.FloatTensor, ...]] = None
@dataclass
class LEDSeq2SeqSequenceClassifierOutput(ModelOutput):
"""
Base class for outputs of sequence-to-sequence sentence classification models.
Args:
loss (`torch.FloatTensor` of shape `(1,)`, *optional*, returned when `label` is provided):
Classification (or regression if config.num_labels==1) loss.
logits (`torch.FloatTensor` of shape `(batch_size, config.num_labels)`):
Classification (or regression if config.num_labels==1) scores (before SoftMax).
past_key_values (`List[torch.FloatTensor]`, *optional*, returned when `use_cache=True` is passed or when `config.use_cache=True`):
List of `torch.FloatTensor` of length `config.n_layers`, with each tensor of shape `(2, batch_size,
num_heads, sequence_length, embed_size_per_head)`).
Contains pre-computed hidden-states (key and values in the attention blocks) of the decoder that can be
used (see `past_key_values` input) to speed up sequential decoding.
decoder_hidden_states (`tuple(torch.FloatTensor)`, *optional*, returned when `output_hidden_states=True` is passed or when `config.output_hidden_states=True`):
Tuple of `torch.FloatTensor` (one for the output of the embeddings + one for the output of each layer) of
shape `(batch_size, sequence_length, hidden_size)`.
Hidden-states of the decoder at the output of each layer plus the initial embedding outputs.
decoder_attentions (`tuple(torch.FloatTensor)`, *optional*, returned when `output_attentions=True` is passed or when `config.output_attentions=True`):
Tuple of `torch.FloatTensor` (one for each layer) of shape `(batch_size, num_heads, sequence_length,
sequence_length)`.
Attentions weights of the decoder, after the attention softmax, used to compute the weighted average in the
self-attention heads.
cross_attentions (`tuple(torch.FloatTensor)`, *optional*, returned when `output_attentions=True` is passed or when `config.output_attentions=True`):
Tuple of `torch.FloatTensor` (one for each layer) of shape `(batch_size, num_heads, sequence_length,
sequence_length)`.
Attentions weights of the decoder's cross-attention layer, after the attention softmax, used to compute the
weighted average in the cross-attention heads.
encoder_last_hidden_state (`torch.FloatTensor` of shape `(batch_size, sequence_length, hidden_size)`, *optional*):
Sequence of hidden-states at the output of the last layer of the encoder of the model.
encoder_hidden_states (`tuple(torch.FloatTensor)`, *optional*, returned when `output_hidden_states=True` is passed or when `config.output_hidden_states=True`):
Tuple of `torch.FloatTensor` (one for the output of the embeddings + one for the output of each layer) of
shape `(batch_size, sequence_length, hidden_size)`.
Hidden-states of the encoder at the output of each layer plus the initial embedding outputs.
encoder_attentions (`tuple(torch.FloatTensor)`, *optional*, returned when `output_attentions=True` is passed or when `config.output_attentions=True`):
Tuple of `torch.FloatTensor` (one for each layer) of shape `(batch_size, num_heads, sequence_length,
sequence_length)`.
Attentions weights of the encoder, after the attention softmax, used to compute the weighted average in the
self-attention heads.
encoder_global_attentions (`tuple(torch.FloatTensor)`, *optional*, returned when `output_attentions=True` is passed or when `config.output_attentions=True`):
Tuple of `torch.FloatTensor` (one for each layer) of shape `(batch_size, num_heads, sequence_length, x)`,
where `x` is the number of tokens with global attention mask.
Global attentions weights after the attention softmax, used to compute the weighted average in the
self-attention heads. Those are the attention weights from every token with global attention to every token
in the sequence.
"""
loss: Optional[torch.FloatTensor] = None
logits: torch.FloatTensor = None
past_key_values: Optional[List[torch.FloatTensor]] = None
decoder_hidden_states: Optional[Tuple[torch.FloatTensor, ...]] = None
decoder_attentions: Optional[Tuple[torch.FloatTensor, ...]] = None
cross_attentions: Optional[Tuple[torch.FloatTensor, ...]] = None
encoder_last_hidden_state: Optional[torch.FloatTensor] = None
encoder_hidden_states: Optional[Tuple[torch.FloatTensor, ...]] = None
encoder_attentions: Optional[Tuple[torch.FloatTensor, ...]] = None
encoder_global_attentions: Optional[Tuple[torch.FloatTensor, ...]] = None
@dataclass
class LEDSeq2SeqQuestionAnsweringModelOutput(ModelOutput):
"""
Base class for outputs of sequence-to-sequence question answering models.
Args:
loss (`torch.FloatTensor` of shape `(1,)`, *optional*, returned when `labels` is provided):
Total span extraction loss is the sum of a Cross-Entropy for the start and end positions.
start_logits (`torch.FloatTensor` of shape `(batch_size, sequence_length)`):
Span-start scores (before SoftMax).
end_logits (`torch.FloatTensor` of shape `(batch_size, sequence_length)`):
Span-end scores (before SoftMax).
past_key_values (`List[torch.FloatTensor]`, *optional*, returned when `use_cache=True` is passed or when `config.use_cache=True`):
List of `torch.FloatTensor` of length `config.n_layers`, with each tensor of shape `(2, batch_size,
num_heads, sequence_length, embed_size_per_head)`).
Contains pre-computed hidden-states (key and values in the attention blocks) of the decoder that can be
used (see `past_key_values` input) to speed up sequential decoding.
decoder_hidden_states (`tuple(torch.FloatTensor)`, *optional*, returned when `output_hidden_states=True` is passed or when `config.output_hidden_states=True`):
Tuple of `torch.FloatTensor` (one for the output of the embeddings + one for the output of each layer) of
shape `(batch_size, sequence_length, hidden_size)`.
Hidden-states of the decoder at the output of each layer plus the initial embedding outputs.
decoder_attentions (`tuple(torch.FloatTensor)`, *optional*, returned when `output_attentions=True` is passed or when `config.output_attentions=True`):
Tuple of `torch.FloatTensor` (one for each layer) of shape `(batch_size, num_heads, sequence_length,
sequence_length)`.
Attentions weights of the decoder, after the attention softmax, used to compute the weighted average in the
self-attention heads.
cross_attentions (`tuple(torch.FloatTensor)`, *optional*, returned when `output_attentions=True` is passed or when `config.output_attentions=True`):
Tuple of `torch.FloatTensor` (one for each layer) of shape `(batch_size, num_heads, sequence_length,
sequence_length)`.
Attentions weights of the decoder's cross-attention layer, after the attention softmax, used to compute the
weighted average in the cross-attention heads.
encoder_last_hidden_state (`torch.FloatTensor` of shape `(batch_size, sequence_length, hidden_size)`, *optional*):
Sequence of hidden-states at the output of the last layer of the encoder of the model.
encoder_hidden_states (`tuple(torch.FloatTensor)`, *optional*, returned when `output_hidden_states=True` is passed or when `config.output_hidden_states=True`):
Tuple of `torch.FloatTensor` (one for the output of the embeddings + one for the output of each layer) of
shape `(batch_size, sequence_length, hidden_size)`.
Hidden-states of the encoder at the output of each layer plus the initial embedding outputs.
encoder_attentions (`tuple(torch.FloatTensor)`, *optional*, returned when `output_attentions=True` is passed or when `config.output_attentions=True`):
Tuple of `torch.FloatTensor` (one for each layer) of shape `(batch_size, num_heads, sequence_length,
sequence_length)`.
Attentions weights of the encoder, after the attention softmax, used to compute the weighted average in the
self-attention heads.
encoder_global_attentions (`tuple(torch.FloatTensor)`, *optional*, returned when `output_attentions=True` is passed or when `config.output_attentions=True`):
Tuple of `torch.FloatTensor` (one for each layer) of shape `(batch_size, num_heads, sequence_length, x)`,
where `x` is the number of tokens with global attention mask.
Global attentions weights after the attention softmax, used to compute the weighted average in the
self-attention heads. Those are the attention weights from every token with global attention to every token
in the sequence.
"""
loss: Optional[torch.FloatTensor] = None
start_logits: torch.FloatTensor = None
end_logits: torch.FloatTensor = None
past_key_values: Optional[List[torch.FloatTensor]] = None
decoder_hidden_states: Optional[Tuple[torch.FloatTensor, ...]] = None
decoder_attentions: Optional[Tuple[torch.FloatTensor, ...]] = None
cross_attentions: Optional[Tuple[torch.FloatTensor, ...]] = None
encoder_last_hidden_state: Optional[torch.FloatTensor] = None
encoder_hidden_states: Optional[Tuple[torch.FloatTensor, ...]] = None
encoder_attentions: Optional[Tuple[torch.FloatTensor, ...]] = None
encoder_global_attentions: Optional[Tuple[torch.FloatTensor, ...]] = None
LED_START_DOCSTRING = r"""
This model inherits from [`PreTrainedModel`]. See the superclass documentation for the generic methods the library
implements for all its models (such as downloading or saving, resizing the input embeddings, pruning heads etc.)
This model is also a PyTorch [torch.nn.Module](https://pytorch.org/docs/stable/nn.html#torch.nn.Module) subclass.
Use it as a regular PyTorch Module and refer to the PyTorch documentation for general usage and behavior.
Parameters:
config ([`LEDConfig`]):
Model configuration class with all the parameters of the model. Initializing with a config file does not
load the weights associated with the model, only the configuration. Check out the
[`~PreTrainedModel.from_pretrained`] method to load the model weights.
"""
LED_GENERATION_EXAMPLE = r"""
Summarization example:
```python
>>> import torch
>>> from transformers import AutoTokenizer, LEDForConditionalGeneration
>>> model = LEDForConditionalGeneration.from_pretrained("allenai/led-large-16384-arxiv")
>>> tokenizer = AutoTokenizer.from_pretrained("allenai/led-large-16384-arxiv")
>>> ARTICLE_TO_SUMMARIZE = '''Transformers (Vaswani et al., 2017) have achieved state-of-the-art
... results in a wide range of natural language tasks including generative language modeling
... (Dai et al., 2019; Radford et al., 2019) and discriminative ... language understanding (Devlin et al., 2019).
... This success is partly due to the self-attention component which enables the network to capture contextual
... information from the entire sequence. While powerful, the memory and computational requirements of
... self-attention grow quadratically with sequence length, making it infeasible (or very expensive) to
... process long sequences. To address this limitation, we present Longformer, a modified Transformer
... architecture with a self-attention operation that scales linearly with the sequence length, making it
... versatile for processing long documents (Fig 1). This is an advantage for natural language tasks such as
... long document classification, question answering (QA), and coreference resolution, where existing approaches
... partition or shorten the long context into smaller sequences that fall within the typical 512 token limit
... of BERT-style pretrained models. Such partitioning could potentially result in loss of important
... cross-partition information, and to mitigate this problem, existing methods often rely on complex
... architectures to address such interactions. On the other hand, our proposed Longformer is able to build
... contextual representations of the entire context using multiple layers of attention, reducing the need for
... task-specific architectures.'''
>>> inputs = tokenizer.encode(ARTICLE_TO_SUMMARIZE, return_tensors="pt")
>>> # Global attention on the first token (cf. Beltagy et al. 2020)
>>> global_attention_mask = torch.zeros_like(inputs)
>>> global_attention_mask[:, 0] = 1
>>> # Generate Summary
>>> summary_ids = model.generate(inputs, global_attention_mask=global_attention_mask, num_beams=3, max_length=32)
>>> print(tokenizer.decode(summary_ids[0], skip_special_tokens=True, clean_up_tokenization_spaces=True))
```
"""
LED_INPUTS_DOCSTRING = r"""
Args:
input_ids (`torch.LongTensor` of shape `(batch_size, sequence_length)`):
Indices of input sequence tokens in the vocabulary. Padding will be ignored by default should you provide
it.
Indices can be obtained using [`AutoTokenizer`]. See [`PreTrainedTokenizer.encode`] and
[`PreTrainedTokenizer.__call__`] for details.
[What are input IDs?](../glossary#input-ids)
attention_mask (`torch.Tensor` of shape `(batch_size, sequence_length)`, *optional*):
Mask to avoid performing attention on padding token indices. Mask values selected in `[0, 1]`:
- 1 for tokens that are **not masked**,
- 0 for tokens that are **masked**.
[What are attention masks?](../glossary#attention-mask)
decoder_input_ids (`torch.LongTensor` of shape `(batch_size, target_sequence_length)`, *optional*):
Indices of decoder input sequence tokens in the vocabulary.
Indices can be obtained using [`LedTokenizer`]. See [`PreTrainedTokenizer.encode`] and
[`PreTrainedTokenizer.__call__`] for details.
[What are input IDs?](../glossary#input-ids)
LED uses the `eos_token_id` as the starting token for `decoder_input_ids` generation. If `past_key_values`
is used, optionally only the last `decoder_input_ids` have to be input (see `past_key_values`).
decoder_attention_mask (`torch.LongTensor` of shape `(batch_size, target_sequence_length)`, *optional*):
Default behavior: generate a tensor that ignores pad tokens in `decoder_input_ids`. Causal mask will also
be used by default.
If you want to change padding behavior, you should read [`modeling_led._prepare_decoder_inputs`] and modify
to your needs. See diagram 1 in [the paper](https://arxiv.org/abs/1910.13461) for more information on the
default strategy.
global_attention_mask (`torch.FloatTensor` of shape `(batch_size, sequence_length)`, *optional*):
Mask to decide the attention given on each token, local attention or global attention for the encoder.
Tokens with global attention attends to all other tokens, and all other tokens attend to them. This is
important for task-specific finetuning because it makes the model more flexible at representing the task.
For example, for classification, the <s> token should be given global attention. For QA, all question
tokens should also have global attention. Please refer to the [Longformer
paper](https://arxiv.org/abs/2004.05150) for more details. Mask values selected in `[0, 1]`:
- 0 for local attention (a sliding window attention),
- 1 for global attention (tokens that attend to all other tokens, and all other tokens attend to them).
head_mask (`torch.Tensor` of shape `(encoder_layers, encoder_attention_heads)`, *optional*):
Mask to nullify selected heads of the attention modules in the encoder. Mask values selected in `[0, 1]`:
- 1 indicates the head is **not masked**,
- 0 indicates the head is **masked**.
decoder_head_mask (`torch.Tensor` of shape `(decoder_layers, decoder_attention_heads)`, *optional*):
Mask to nullify selected heads of the attention modules in the decoder. Mask values selected in `[0, 1]`:
- 1 indicates the head is **not masked**,
- 0 indicates the head is **masked**.
cross_attn_head_mask (`torch.Tensor` of shape `(decoder_layers, decoder_attention_heads)`, *optional*):
Mask to nullify selected heads of the cross-attention modules in the decoder. Mask values selected in `[0,
1]`:
- 1 indicates the head is **not masked**,
- 0 indicates the head is **masked**.
encoder_outputs (`tuple(tuple(torch.FloatTensor)`, *optional*):
Tuple consists of (`last_hidden_state`, *optional*: `hidden_states`, *optional*: `attentions`)
`last_hidden_state` of shape `(batch_size, sequence_length, hidden_size)`, *optional*) is a sequence of
hidden-states at the output of the last layer of the encoder. Used in the cross-attention of the decoder.
past_key_values (`tuple(tuple(torch.FloatTensor))`, *optional*, returned when `use_cache=True` is passed or when `config.use_cache=True`):
Tuple of `tuple(torch.FloatTensor)` of length `config.n_layers`, with each tuple having 2 tensors of shape
`(batch_size, num_heads, sequence_length, embed_size_per_head)`) and 2 additional tensors of shape
`(batch_size, num_heads, encoder_sequence_length, embed_size_per_head)`.
Contains pre-computed hidden-states (key and values in the self-attention blocks and in the cross-attention
blocks) that can be used (see `past_key_values` input) to speed up sequential decoding.
If `past_key_values` are used, the user can optionally input only the last `decoder_input_ids` (those that
don't have their past key value states given to this model) of shape `(batch_size, 1)` instead of all
`decoder_input_ids` of shape `(batch_size, sequence_length)`.
inputs_embeds (`torch.FloatTensor` of shape `(batch_size, sequence_length, hidden_size)`, *optional*):
Optionally, instead of passing `input_ids` you can choose to directly pass an embedded representation. This
is useful if you want more control over how to convert `input_ids` indices into associated vectors than the
model's internal embedding lookup matrix.
decoder_inputs_embeds (`torch.FloatTensor` of shape `(batch_size, target_sequence_length, hidden_size)`, *optional*):
Optionally, instead of passing `decoder_input_ids` you can choose to directly pass an embedded
representation. If `past_key_values` is used, optionally only the last `decoder_inputs_embeds` have to be
input (see `past_key_values`). This is useful if you want more control over how to convert
`decoder_input_ids` indices into associated vectors than the model's internal embedding lookup matrix.
If `decoder_input_ids` and `decoder_inputs_embeds` are both unset, `decoder_inputs_embeds` takes the value
of `inputs_embeds`.
use_cache (`bool`, *optional*):
If set to `True`, `past_key_values` key value states are returned and can be used to speed up decoding (see
`past_key_values`).
output_attentions (`bool`, *optional*):
Whether or not to return the attentions tensors of all attention layers. See `attentions` under returned
tensors for more detail.
output_hidden_states (`bool`, *optional*):
Whether or not to return the hidden states of all layers. See `hidden_states` under returned tensors for
more detail.
return_dict (`bool`, *optional*):
Whether or not to return a [`~utils.ModelOutput`] instead of a plain tuple.
"""
class LEDEncoder(LEDPreTrainedModel):
"""
Transformer encoder consisting of *config.encoder_layers* self-attention layers. Each layer is a
[`LEDEncoderLayer`].
Args:
config: LEDConfig
embed_tokens (nn.Embedding): output embedding
"""
def __init__(self, config: LEDConfig, embed_tokens: Optional[nn.Embedding] = None):
super().__init__(config)
self.dropout = config.dropout
self.layerdrop = config.encoder_layerdrop
embed_dim = config.d_model
self.padding_idx = config.pad_token_id
self.max_source_positions = config.max_encoder_position_embeddings
if isinstance(config.attention_window, int):
if config.attention_window % 2 != 0:
raise ValueError("`config.attention_window` has to be an even value")
if config.attention_window <= 0:
raise ValueError("`config.attention_window` has to be positive")
config.attention_window = [config.attention_window] * config.num_hidden_layers # one value per layer
else:
if len(config.attention_window) != config.num_hidden_layers:
raise ValueError(
"`len(config.attention_window)` should equal `config.num_hidden_layers`. "
f"Expected {config.num_hidden_layers}, given {len(config.attention_window)}"
)
if embed_tokens is not None:
self.embed_tokens = embed_tokens
else:
self.embed_tokens = nn.Embedding(config.vocab_size, embed_dim, self.padding_idx)
self.embed_positions = LEDLearnedPositionalEmbedding(
self.max_source_positions,
embed_dim,
)
self.layers = nn.ModuleList([LEDEncoderLayer(config, i) for i in range(config.encoder_layers)])
self.layernorm_embedding = nn.LayerNorm(embed_dim)
self.gradient_checkpointing = False
# Initialize weights and apply final processing
self.post_init()
def _merge_to_attention_mask(self, attention_mask: torch.Tensor, global_attention_mask: torch.Tensor):
# longformer self-attention expects attention mask to have 0 (no attn), 1 (local attn), 2 (global attn)
# (global_attention_mask + 1) => 1 for local attention, 2 for global attention
# => final attention_mask => 0 for no attention, 1 for local attention 2 for global attention
if attention_mask is not None:
attention_mask = attention_mask * (global_attention_mask + 1)
else:
# simply use `global_attention_mask` as `attention_mask`
# if no `attention_mask` is given
attention_mask = global_attention_mask + 1
return attention_mask
def _pad_to_window_size(
self,
input_ids: torch.Tensor,
attention_mask: torch.Tensor,
inputs_embeds: torch.Tensor,
pad_token_id: int,
):
"""A helper function to pad tokens and mask to work with implementation of Longformer self-attention."""
# padding
attention_window = (
self.config.attention_window
if isinstance(self.config.attention_window, int)
else max(self.config.attention_window)
)
if attention_window % 2 != 0:
raise ValueError(f"`attention_window` should be an even value. Given {attention_window}")
input_shape = input_ids.shape if input_ids is not None else inputs_embeds.shape
batch_size, seq_len = input_shape[:2]
padding_len = (attention_window - seq_len % attention_window) % attention_window
if padding_len > 0:
logger.warning_once(
f"Input ids are automatically padded from {seq_len} to {seq_len + padding_len} to be a multiple of "
f"`config.attention_window`: {attention_window}"
)
if input_ids is not None:
input_ids = nn.functional.pad(input_ids, (0, padding_len), value=pad_token_id)
if inputs_embeds is not None:
input_ids_padding = inputs_embeds.new_full(
(batch_size, padding_len),
self.config.pad_token_id,
dtype=torch.long,
)
inputs_embeds_padding = self.embed_tokens(input_ids_padding)
inputs_embeds = torch.cat([inputs_embeds, inputs_embeds_padding], dim=-2)
attention_mask = nn.functional.pad(
attention_mask, (0, padding_len), value=False
) # no attention on the padding tokens
return padding_len, input_ids, attention_mask, inputs_embeds
def forward(
self,
input_ids=None,
attention_mask=None,
global_attention_mask=None,
head_mask=None,
inputs_embeds=None,
output_attentions=None,
output_hidden_states=None,
return_dict=None,
):
r"""
Args:
input_ids (`torch.LongTensor` of shape `(batch_size, sequence_length)`):
Indices of input sequence tokens in the vocabulary. Padding will be ignored by default should you
provide it.
Indices can be obtained using [`AutoTokenizer`]. See [`PreTrainedTokenizer.encode`] and
[`PreTrainedTokenizer.__call__`] for details.
[What are input IDs?](../glossary#input-ids)
attention_mask (`torch.Tensor` of shape `(batch_size, sequence_length)`, *optional*):
Mask to avoid performing attention on padding token indices. Mask values selected in `[0, 1]`:
- 1 for tokens that are **not masked**,
- 0 for tokens that are **masked**.
[What are attention masks?](../glossary#attention-mask)
global_attention_mask (`torch.FloatTensor` of shape `(batch_size, sequence_length)`, *optional*):
Mask to decide the attention given on each token, local attention or global attention for the encoder.
Tokens with global attention attends to all other tokens, and all other tokens attend to them. This is
important for task-specific finetuning because it makes the model more flexible at representing the
task. For example, for classification, the <s> token should be given global attention. For QA, all
question tokens should also have global attention. Please refer to the [Longformer
paper](https://arxiv.org/abs/2004.05150) for more details. Mask values selected in `[0, 1]`:
- 0 for local attention (a sliding window attention),
- 1 for global attention (tokens that attend to all other tokens, and all other tokens attend to them).
head_mask (`torch.Tensor` of shape `(encoder_layers, encoder_attention_heads)`, *optional*):
Mask to nullify selected heads of the attention modules. Mask values selected in `[0, 1]`:
- 1 indicates the head is **not masked**,
- 0 indicates the head is **masked**.
inputs_embeds (`torch.FloatTensor` of shape `(batch_size, sequence_length, hidden_size)`, *optional*):
Optionally, instead of passing `input_ids` you can choose to directly pass an embedded representation.
This is useful if you want more control over how to convert `input_ids` indices into associated vectors
than the model's internal embedding lookup matrix.
output_attentions (`bool`, *optional*):
Whether or not to return the attentions tensors of all attention layers. See `attentions` under
returned tensors for more detail.
output_hidden_states (`bool`, *optional*):
Whether or not to return the hidden states of all layers. See `hidden_states` under returned tensors
for more detail.
return_dict (`bool`, *optional*):
Whether or not to return a [`~utils.ModelOutput`] instead of a plain tuple.
"""
output_attentions = output_attentions if output_attentions is not None else self.config.output_attentions
output_hidden_states = (
output_hidden_states if output_hidden_states is not None else self.config.output_hidden_states
)
return_dict = return_dict if return_dict is not None else self.config.use_return_dict
# check input_ids and inputs_embeds
if input_ids is not None and inputs_embeds is not None:
raise ValueError("You cannot specify both input_ids and inputs_embeds at the same time")
elif input_ids is None and inputs_embeds is None:
raise ValueError("You have to specify either input_ids or inputs_embeds")
if inputs_embeds is None:
inputs_embeds = self.embed_tokens(input_ids)
# create default attention_mask
if attention_mask is None:
attention_mask = torch.ones(inputs_embeds.size()[:-1], device=inputs_embeds.device, dtype=torch.long)
# merge `global_attention_mask` and `attention_mask`
if global_attention_mask is not None:
attention_mask = self._merge_to_attention_mask(attention_mask, global_attention_mask)
# pad input if necessary
padding_len, input_ids, attention_mask, inputs_embeds = self._pad_to_window_size(
input_ids=input_ids,
attention_mask=attention_mask,
inputs_embeds=inputs_embeds,
pad_token_id=self.config.pad_token_id,
)
# retrieve input_shape
if input_ids is not None:
input_shape = input_ids.size()
input_ids = input_ids.view(-1, input_shape[-1])
elif inputs_embeds is not None:
input_shape = inputs_embeds.size()[:-1]
# convert attention_mask to float
if attention_mask is not None:
# [bsz, seq_len] -> [bsz, seq_len]; 1 -> 0.0; 0 -> "-inf"
attention_mask = _prepare_4d_attention_mask_inverted(attention_mask, inputs_embeds.dtype)[:, 0, 0, :]
# get masking tensors
is_index_masked = attention_mask < 0
is_index_global_attn = attention_mask > 0
is_global_attn = is_index_global_attn.flatten().any().item()
embed_pos = self.embed_positions(input_shape)
hidden_states = inputs_embeds + embed_pos
hidden_states = self.layernorm_embedding(hidden_states)
hidden_states = nn.functional.dropout(hidden_states, p=self.dropout, training=self.training)
encoder_states = () if output_hidden_states else None
all_attentions = () if output_attentions else None
all_global_attentions = () if (output_attentions and is_global_attn) else None
# check if head_mask has a correct number of layers specified if desired
if head_mask is not None:
if head_mask.size()[0] != len(self.layers):
raise ValueError(
f"The head_mask should be specified for {len(self.layers)} layers, but it is for"
f" {head_mask.size()[0]}."
)
for idx, encoder_layer in enumerate(self.layers):
if output_hidden_states:
encoder_states = encoder_states + (hidden_states,)
# add LayerDrop (see https://arxiv.org/abs/1909.11556 for description)
dropout_probability = torch.rand([])
if self.training and (dropout_probability < self.layerdrop): # skip the layer
layer_outputs = (None, None, None)
else:
if self.gradient_checkpointing and self.training:
layer_outputs = self._gradient_checkpointing_func(
encoder_layer.__call__,
hidden_states,
attention_mask,
head_mask[idx] if head_mask is not None else None,
is_index_masked,
is_index_global_attn,
is_global_attn,
output_attentions,
)
else:
layer_outputs = encoder_layer(
hidden_states,
attention_mask=attention_mask,
layer_head_mask=(head_mask[idx] if head_mask is not None else None),
is_index_masked=is_index_masked,
is_index_global_attn=is_index_global_attn,
is_global_attn=is_global_attn,
output_attentions=output_attentions,
)
hidden_states = layer_outputs[0]
if output_attentions:
# bzs x seq_len x num_attn_heads x (num_global_attn + attention_window_len + 1) => bzs x num_attn_heads x seq_len x (num_global_attn + attention_window_len + 1)
all_attentions = all_attentions + (layer_outputs[1].transpose(1, 2),)
if is_global_attn:
# bzs x num_attn_heads x num_global_attn x seq_len => bzs x num_attn_heads x seq_len x num_global_attn
all_global_attentions = all_global_attentions + (layer_outputs[2].transpose(2, 3),)
if output_hidden_states:
encoder_states = encoder_states + (hidden_states,)
# undo padding
if padding_len > 0:
# unpad `hidden_states` because the calling function is expecting a length == input_ids.size(1)
hidden_states = hidden_states[:, :-padding_len]
if output_hidden_states:
encoder_states = tuple([state[:, :-padding_len] for state in encoder_states])
if output_attentions:
all_attentions = tuple([state[:, :, :-padding_len, :] for state in all_attentions])
if not return_dict:
return tuple(
v for v in [hidden_states, encoder_states, all_attentions, all_global_attentions] if v is not None
)
return LEDEncoderBaseModelOutput(
last_hidden_state=hidden_states,
hidden_states=encoder_states,
attentions=all_attentions,
global_attentions=all_global_attentions,
)
class LEDDecoder(LEDPreTrainedModel):
"""
Transformer decoder consisting of *config.decoder_layers* layers. Each layer is a [`LEDDecoderLayer`]
Args:
config: LEDConfig
embed_tokens (nn.Embedding): output embedding
"""
def __init__(self, config: LEDConfig, embed_tokens: Optional[nn.Embedding] = None):
super().__init__(config)
self.dropout = config.dropout
self.layerdrop = config.decoder_layerdrop
self.padding_idx = config.pad_token_id
self.max_target_positions = config.max_decoder_position_embeddings
if embed_tokens is not None:
self.embed_tokens = embed_tokens
else:
self.embed_tokens = nn.Embedding(config.vocab_size, config.d_model, self.padding_idx)
self.embed_positions = LEDLearnedPositionalEmbedding(
self.max_target_positions,
config.d_model,
)
self.layers = nn.ModuleList([LEDDecoderLayer(config) for _ in range(config.decoder_layers)])
self.layernorm_embedding = nn.LayerNorm(config.d_model)
self.gradient_checkpointing = False
# Initialize weights and apply final processing
self.post_init()
def forward(
self,
input_ids=None,
attention_mask=None,
global_attention_mask=None,
encoder_hidden_states=None,
encoder_attention_mask=None,
head_mask=None,
cross_attn_head_mask=None,
past_key_values=None,
inputs_embeds=None,
use_cache=None,
output_attentions=None,
output_hidden_states=None,
return_dict=None,
):
r"""
Args:
input_ids (`torch.LongTensor` of shape `(batch_size, sequence_length)`):
Indices of input sequence tokens in the vocabulary. Padding will be ignored by default should you
provide it.
Indices can be obtained using [`AutoTokenizer`]. See [`PreTrainedTokenizer.encode`] and
[`PreTrainedTokenizer.__call__`] for details.
[What are input IDs?](../glossary#input-ids)
attention_mask (`torch.Tensor` of shape `(batch_size, sequence_length)`, *optional*):
Mask to avoid performing attention on padding token indices. Mask values selected in `[0, 1]`:
- 1 for tokens that are **not masked**,
- 0 for tokens that are **masked**.
[What are attention masks?](../glossary#attention-mask)
global_attention_mask (`torch.FloatTensor` of shape `(batch_size, sequence_length)`, *optional*):
Mask to decide the attention given on each token, local attention or global attention. Tokens with
global attention attends to all other tokens, and all other tokens attend to them. This is important
for task-specific finetuning because it makes the model more flexible at representing the task. For
example, for classification, the <s> token should be given global attention. For QA, all question
tokens should also have global attention. Please refer to the [Longformer
paper](https://arxiv.org/abs/2004.05150) for more details. Mask values selected in `[0, 1]`:
- 0 for local attention (a sliding window attention),
- 1 for global attention (tokens that attend to all other tokens, and all other tokens attend to them).
encoder_hidden_states (`torch.FloatTensor` of shape `(batch_size, encoder_sequence_length, hidden_size)`, *optional*):
Sequence of hidden-states at the output of the last layer of the encoder. Used in the cross-attention
of the decoder.
encoder_attention_mask (`torch.LongTensor` of shape `(batch_size, encoder_sequence_length)`, *optional*):
Mask to avoid performing cross-attention on padding tokens indices of encoder input_ids. Mask values
selected in `[0, 1]`:
- 1 for tokens that are **not masked**,
- 0 for tokens that are **masked**.
[What are attention masks?](../glossary#attention-mask)
head_mask (`torch.Tensor` of shape `(decoder_layers, decoder_attention_heads)`, *optional*):
Mask to nullify selected heads of the attention modules. Mask values selected in `[0, 1]`:
- 1 indicates the head is **not masked**,
- 0 indicates the head is **masked**.
cross_attn_head_mask (`torch.Tensor` of shape `(decoder_layers, decoder_attention_heads)`, *optional*):
Mask to nullify selected heads of the cross-attention modules. Mask values selected in `[0, 1]`:
- 1 indicates the head is **not masked**,
- 0 indicates the head is **masked**.
past_key_values (`tuple(tuple(torch.FloatTensor))`, *optional*, returned when `use_cache=True` is passed or when `config.use_cache=True`):
Tuple of `tuple(torch.FloatTensor)` of length `config.n_layers`, with each tuple having 2 tensors of
shape `(batch_size, num_heads, sequence_length, embed_size_per_head)`) and 2 additional tensors of
shape `(batch_size, num_heads, encoder_sequence_length, embed_size_per_head)`.
Contains pre-computed hidden-states (key and values in the self-attention blocks and in the
cross-attention blocks) that can be used (see `past_key_values` input) to speed up sequential decoding.
If `past_key_values` are used, the user can optionally input only the last `decoder_input_ids` (those
that don't have their past key value states given to this model) of shape `(batch_size, 1)` instead of
all `decoder_input_ids` of shape `(batch_size, sequence_length)`.
inputs_embeds (`torch.FloatTensor` of shape `(batch_size, sequence_length, hidden_size)`, *optional*):
Optionally, instead of passing `input_ids` you can choose to directly pass an embedded representation.
This is useful if you want more control over how to convert `input_ids` indices into associated vectors
than the model's internal embedding lookup matrix.
output_attentions (`bool`, *optional*):
Whether or not to return the attentions tensors of all attention layers. See `attentions` under
returned tensors for more detail.
output_hidden_states (`bool`, *optional*):
Whether or not to return the hidden states of all layers. See `hidden_states` under returned tensors
for more detail.
return_dict (`bool`, *optional*):
Whether or not to return a [`~utils.ModelOutput`] instead of a plain tuple.
"""
output_attentions = output_attentions if output_attentions is not None else self.config.output_attentions
output_hidden_states = (
output_hidden_states if output_hidden_states is not None else self.config.output_hidden_states
)
use_cache = use_cache if use_cache is not None else self.config.use_cache
return_dict = return_dict if return_dict is not None else self.config.use_return_dict
# retrieve input_ids and inputs_embeds
if input_ids is not None and inputs_embeds is not None:
raise ValueError("You cannot specify both decoder_input_ids and decoder_inputs_embeds at the same time")
elif input_ids is not None:
input_shape = input_ids.size()
input_ids = input_ids.view(-1, input_shape[-1])
elif inputs_embeds is not None:
input_shape = inputs_embeds.size()[:-1]
else:
raise ValueError("You have to specify either decoder_input_ids or decoder_inputs_embeds")
# past_key_values_length
past_key_values_length = past_key_values[0][0].shape[2] if past_key_values is not None else 0
if inputs_embeds is None:
inputs_embeds = self.embed_tokens(input_ids)
# create causal mask
# [bsz, seq_len] -> [bsz, 1, tgt_seq_len, src_seq_len]
combined_attention_mask = None
if input_shape[-1] > 1:
combined_attention_mask = _create_4d_causal_attention_mask(
input_shape, inputs_embeds.dtype, inputs_embeds.device, past_key_values_length=past_key_values_length
)
if attention_mask is not None and combined_attention_mask is not None:
# [bsz, seq_len] -> [bsz, 1, tgt_seq_len, src_seq_len]
combined_attention_mask = combined_attention_mask + _prepare_4d_attention_mask_inverted(
attention_mask, inputs_embeds.dtype, tgt_len=input_shape[-1]
)
# expand encoder attention mask
if encoder_hidden_states is not None and encoder_attention_mask is not None:
# [bsz, seq_len] -> [bsz, 1, tgt_seq_len, src_seq_len]
encoder_attention_mask = _prepare_4d_attention_mask_inverted(
encoder_attention_mask, inputs_embeds.dtype, tgt_len=input_shape[-1]
)
# embed positions
positions = self.embed_positions(input_shape, past_key_values_length)
hidden_states = inputs_embeds + positions
hidden_states = self.layernorm_embedding(hidden_states)
hidden_states = nn.functional.dropout(hidden_states, p=self.dropout, training=self.training)
if self.gradient_checkpointing and self.training:
if use_cache:
logger.warning_once(
"`use_cache=True` is incompatible with gradient checkpointing. Setting `use_cache=False`..."
)
use_cache = False
# decoder layers
all_hidden_states = () if output_hidden_states else None
all_self_attns = () if output_attentions else None
all_cross_attentions = () if output_attentions else None
next_decoder_cache = () if use_cache else None
# check if head_mask/cross_attn_head_mask has a correct number of layers specified if desired
for attn_mask, mask_name in zip([head_mask, cross_attn_head_mask], ["head_mask", "cross_attn_head_mask"]):
if attn_mask is not None:
if attn_mask.size()[0] != len(self.layers):
raise ValueError(
f"The `{mask_name}` should be specified for {len(self.layers)} layers, but it is for"
f" {head_mask.size()[0]}."
)
for idx, decoder_layer in enumerate(self.layers):
# add LayerDrop (see https://arxiv.org/abs/1909.11556 for description)
if output_hidden_states:
all_hidden_states += (hidden_states,)
if self.training:
dropout_probability = torch.rand([])
if dropout_probability < self.layerdrop:
continue
past_key_value = past_key_values[idx] if past_key_values is not None else None
if self.gradient_checkpointing and self.training:
layer_outputs = self._gradient_checkpointing_func(
decoder_layer.__call__,
hidden_states,
combined_attention_mask,
encoder_hidden_states,
encoder_attention_mask,
head_mask[idx] if head_mask is not None else None,
cross_attn_head_mask[idx] if cross_attn_head_mask is not None else None,
None,
output_attentions,
use_cache,
)
else:
layer_outputs = decoder_layer(
hidden_states,
attention_mask=combined_attention_mask,
encoder_hidden_states=encoder_hidden_states,
encoder_attention_mask=encoder_attention_mask,
layer_head_mask=(head_mask[idx] if head_mask is not None else None),
cross_attn_layer_head_mask=(
cross_attn_head_mask[idx] if cross_attn_head_mask is not None else None
),
past_key_value=past_key_value,
output_attentions=output_attentions,
use_cache=use_cache,
)
hidden_states = layer_outputs[0]
if use_cache:
next_decoder_cache += (layer_outputs[3 if output_attentions else 1],)
if output_attentions:
all_self_attns += (layer_outputs[1],)
all_cross_attentions += (layer_outputs[2],)
# add hidden states from the last decoder layer
if output_hidden_states:
all_hidden_states += (hidden_states,)
next_cache = next_decoder_cache if use_cache else None
if not return_dict:
return tuple(
v
for v in [hidden_states, next_cache, all_hidden_states, all_self_attns, all_cross_attentions]
if v is not None
)
return BaseModelOutputWithPastAndCrossAttentions(
last_hidden_state=hidden_states,
past_key_values=next_cache,
hidden_states=all_hidden_states,
attentions=all_self_attns,
cross_attentions=all_cross_attentions,
)
@add_start_docstrings(
"The bare LED Model outputting raw hidden-states without any specific head on top.",
LED_START_DOCSTRING,
)
class LEDModel(LEDPreTrainedModel):
_tied_weights_keys = ["decoder.embed_tokens.weight", "encoder.embed_tokens.weight"]
def __init__(self, config: LEDConfig):
super().__init__(config)
padding_idx, vocab_size = config.pad_token_id, config.vocab_size
self.shared = nn.Embedding(vocab_size, config.d_model, padding_idx)
self.encoder = LEDEncoder(config, self.shared)
self.decoder = LEDDecoder(config, self.shared)
# Initialize weights and apply final processing
self.post_init()
def get_input_embeddings(self):
return self.shared
def set_input_embeddings(self, value):
self.shared = value
self.encoder.embed_tokens = self.shared
self.decoder.embed_tokens = self.shared
def get_encoder(self):
return self.encoder
def get_decoder(self):
return self.decoder
@add_start_docstrings_to_model_forward(LED_INPUTS_DOCSTRING)
@add_code_sample_docstrings(
checkpoint=_CHECKPOINT_FOR_DOC,
output_type=Seq2SeqModelOutput,
config_class=_CONFIG_FOR_DOC,
)
def forward(
self,
input_ids: Optional[torch.LongTensor] = None,
attention_mask: Optional[torch.Tensor] = None,
decoder_input_ids: Optional[torch.LongTensor] = None,
decoder_attention_mask: Optional[torch.LongTensor] = None,
head_mask: Optional[torch.Tensor] = None,
decoder_head_mask: Optional[torch.Tensor] = None,
cross_attn_head_mask: Optional[torch.Tensor] = None,
encoder_outputs: Optional[Tuple[Tuple[torch.FloatTensor]]] = None,
global_attention_mask: Optional[torch.FloatTensor] = None,
past_key_values: Optional[Tuple[Tuple[torch.FloatTensor]]] = None,
inputs_embeds: Optional[torch.FloatTensor] = None,
decoder_inputs_embeds: Optional[torch.FloatTensor] = None,
use_cache: Optional[bool] = None,
output_attentions: Optional[bool] = None,
output_hidden_states: Optional[bool] = None,
return_dict: Optional[bool] = None,
) -> Union[Tuple[torch.Tensor], LEDSeq2SeqModelOutput]:
output_attentions = output_attentions if output_attentions is not None else self.config.output_attentions
output_hidden_states = (
output_hidden_states if output_hidden_states is not None else self.config.output_hidden_states
)
use_cache = use_cache if use_cache is not None else self.config.use_cache
return_dict = return_dict if return_dict is not None else self.config.use_return_dict
# Using this like Bart, as LED is derived from it. So far
# No checkpoint on the hub exists that uses that in practice.
# https://github.com/huggingface/transformers/blob/ac3cb660cad283163f7c73cad511124e845ca388/src/transformers/models/bart/modeling_bart.py#L1153
if decoder_input_ids is None and decoder_inputs_embeds is None:
decoder_input_ids = shift_tokens_right(
input_ids, self.config.pad_token_id, self.config.decoder_start_token_id
)
if encoder_outputs is None:
encoder_outputs = self.encoder(
input_ids=input_ids,
attention_mask=attention_mask,
global_attention_mask=global_attention_mask,
head_mask=head_mask,
inputs_embeds=inputs_embeds,
output_attentions=output_attentions,
output_hidden_states=output_hidden_states,
return_dict=return_dict,
)
# If the user passed a tuple for encoder_outputs, we wrap it in a LEDEncoderBaseModelOutput when return_dict=False
elif return_dict and not isinstance(encoder_outputs, LEDEncoderBaseModelOutput):
encoder_outputs = LEDEncoderBaseModelOutput(
last_hidden_state=encoder_outputs[0],
hidden_states=encoder_outputs[1] if len(encoder_outputs) > 1 else None,
attentions=encoder_outputs[2] if len(encoder_outputs) > 2 else None,
global_attentions=encoder_outputs[3] if len(encoder_outputs) > 3 else None,
)
# decoder outputs consists of (dec_features, past_key_value, dec_hidden, dec_attn)
decoder_outputs = self.decoder(
input_ids=decoder_input_ids,
attention_mask=decoder_attention_mask,
encoder_hidden_states=encoder_outputs[0],
encoder_attention_mask=attention_mask,
head_mask=decoder_head_mask,
cross_attn_head_mask=cross_attn_head_mask,
past_key_values=past_key_values,
inputs_embeds=decoder_inputs_embeds,
use_cache=use_cache,
output_attentions=output_attentions,
output_hidden_states=output_hidden_states,
return_dict=return_dict,
)
if not return_dict:
return decoder_outputs + encoder_outputs
return LEDSeq2SeqModelOutput(
last_hidden_state=decoder_outputs.last_hidden_state,
past_key_values=decoder_outputs.past_key_values,
decoder_hidden_states=decoder_outputs.hidden_states,
decoder_attentions=decoder_outputs.attentions,
cross_attentions=decoder_outputs.cross_attentions,
encoder_last_hidden_state=encoder_outputs.last_hidden_state,
encoder_hidden_states=encoder_outputs.hidden_states,
encoder_attentions=encoder_outputs.attentions,
encoder_global_attentions=encoder_outputs.global_attentions,
)
@add_start_docstrings(
"The LED Model with a language modeling head. Can be used for summarization.", LED_START_DOCSTRING
)
class LEDForConditionalGeneration(LEDPreTrainedModel):
base_model_prefix = "led"
_keys_to_ignore_on_load_missing = ["final_logits_bias"]
_tied_weights_keys = ["decoder.embed_tokens.weight", "encoder.embed_tokens.weight", "lm_head.weight"]
def __init__(self, config: LEDConfig):
super().__init__(config)
self.led = LEDModel(config)
self.register_buffer("final_logits_bias", torch.zeros((1, self.led.shared.num_embeddings)))
self.lm_head = nn.Linear(config.d_model, self.led.shared.num_embeddings, bias=False)
# Initialize weights and apply final processing
self.post_init()
def get_encoder(self):
return self.led.get_encoder()
def get_decoder(self):
return self.led.get_decoder()
def resize_token_embeddings(self, new_num_tokens: int, pad_to_multiple_of: Optional[int] = None) -> nn.Embedding:
new_embeddings = super().resize_token_embeddings(new_num_tokens, pad_to_multiple_of)
self._resize_final_logits_bias(new_embeddings.weight.shape[0])
return new_embeddings
def _resize_final_logits_bias(self, new_num_tokens: int) -> None:
old_num_tokens = self.final_logits_bias.shape[-1]
if new_num_tokens <= old_num_tokens:
new_bias = self.final_logits_bias[:, :new_num_tokens]
else:
extra_bias = torch.zeros((1, new_num_tokens - old_num_tokens), device=self.final_logits_bias.device)
new_bias = torch.cat([self.final_logits_bias, extra_bias], dim=1)
self.register_buffer("final_logits_bias", new_bias)
def get_output_embeddings(self):
return self.lm_head
def set_output_embeddings(self, new_embeddings):
self.lm_head = new_embeddings
@add_start_docstrings_to_model_forward(LED_INPUTS_DOCSTRING)
@replace_return_docstrings(output_type=Seq2SeqLMOutput, config_class=_CONFIG_FOR_DOC)
@add_end_docstrings(LED_GENERATION_EXAMPLE)
def forward(
self,
input_ids: Optional[torch.LongTensor] = None,
attention_mask: Optional[torch.Tensor] = None,
decoder_input_ids: Optional[torch.LongTensor] = None,
decoder_attention_mask: Optional[torch.LongTensor] = None,
head_mask: Optional[torch.Tensor] = None,
decoder_head_mask: Optional[torch.Tensor] = None,
cross_attn_head_mask: Optional[torch.Tensor] = None,
encoder_outputs: Optional[Tuple[Tuple[torch.FloatTensor]]] = None,
global_attention_mask: Optional[torch.FloatTensor] = None,
past_key_values: Optional[Tuple[Tuple[torch.FloatTensor]]] = None,
inputs_embeds: Optional[torch.FloatTensor] = None,
decoder_inputs_embeds: Optional[torch.FloatTensor] = None,
labels: Optional[torch.LongTensor] = None,
use_cache: Optional[bool] = None,
output_attentions: Optional[bool] = None,
output_hidden_states: Optional[bool] = None,
return_dict: Optional[bool] = None,
) -> Union[Tuple[torch.Tensor], LEDSeq2SeqLMOutput]:
r"""
labels (`torch.LongTensor` of shape `(batch_size, sequence_length)`, *optional*):
Labels for computing the masked language modeling loss. Indices should either be in `[0, ...,
config.vocab_size]` or -100 (see `input_ids` docstring). Tokens with indices set to `-100` are ignored
(masked), the loss is only computed for the tokens with labels in `[0, ..., config.vocab_size]`.
Returns:
Conditional generation example:
```python
>>> from transformers import AutoTokenizer, LEDForConditionalGeneration
>>> tokenizer = AutoTokenizer.from_pretrained("allenai/led-base-16384")
>>> TXT = "My friends are <mask> but they eat too many carbs."
>>> model = LEDForConditionalGeneration.from_pretrained("allenai/led-base-16384")
>>> input_ids = tokenizer([TXT], return_tensors="pt")["input_ids"]
>>> prediction = model.generate(input_ids)[0]
>>> print(tokenizer.decode(prediction, skip_special_tokens=True))
```"""
return_dict = return_dict if return_dict is not None else self.config.use_return_dict
if labels is not None:
if use_cache:
logger.warning("The `use_cache` argument is changed to `False` since `labels` is provided.")
use_cache = False
if decoder_input_ids is None and decoder_inputs_embeds is None:
decoder_input_ids = shift_tokens_right(
labels, self.config.pad_token_id, self.config.decoder_start_token_id
)
outputs = self.led(
input_ids,
attention_mask=attention_mask,
decoder_input_ids=decoder_input_ids,
decoder_attention_mask=decoder_attention_mask,
encoder_outputs=encoder_outputs,
global_attention_mask=global_attention_mask,
head_mask=head_mask,
decoder_head_mask=decoder_head_mask,
cross_attn_head_mask=cross_attn_head_mask,
past_key_values=past_key_values,
inputs_embeds=inputs_embeds,
decoder_inputs_embeds=decoder_inputs_embeds,
use_cache=use_cache,
output_attentions=output_attentions,
output_hidden_states=output_hidden_states,
return_dict=return_dict,
)
lm_logits = self.lm_head(outputs[0]) + self.final_logits_bias
masked_lm_loss = None
if labels is not None:
loss_fct = CrossEntropyLoss()
masked_lm_loss = loss_fct(lm_logits.view(-1, self.config.vocab_size), labels.view(-1))
if not return_dict:
output = (lm_logits,) + outputs[1:]
return ((masked_lm_loss,) + output) if masked_lm_loss is not None else output
return LEDSeq2SeqLMOutput(
loss=masked_lm_loss,
logits=lm_logits,
past_key_values=outputs.past_key_values,
decoder_hidden_states=outputs.decoder_hidden_states,
decoder_attentions=outputs.decoder_attentions,
cross_attentions=outputs.cross_attentions,
encoder_last_hidden_state=outputs.encoder_last_hidden_state,
encoder_hidden_states=outputs.encoder_hidden_states,
encoder_attentions=outputs.encoder_attentions,
encoder_global_attentions=outputs.encoder_global_attentions,
)
def prepare_inputs_for_generation(
self,
decoder_input_ids,
past_key_values=None,
attention_mask=None,
global_attention_mask=None,
head_mask=None,
decoder_head_mask=None,
cross_attn_head_mask=None,
use_cache=None,
encoder_outputs=None,
**kwargs,
):
# cut decoder_input_ids if past is used
if past_key_values is not None:
decoder_input_ids = decoder_input_ids[:, -1:]
return {
"input_ids": None, # encoder_outputs is defined. input_ids not needed
"encoder_outputs": encoder_outputs,
"past_key_values": past_key_values,
"decoder_input_ids": decoder_input_ids,
"attention_mask": attention_mask,
"global_attention_mask": global_attention_mask,
"head_mask": head_mask,
"decoder_head_mask": decoder_head_mask,
"cross_attn_head_mask": cross_attn_head_mask,
"use_cache": use_cache, # change this to avoid caching (presumably for debugging)
}
def prepare_decoder_input_ids_from_labels(self, labels: torch.Tensor):
return shift_tokens_right(labels, self.config.pad_token_id, self.config.decoder_start_token_id)
@staticmethod
def _reorder_cache(past_key_values, beam_idx):
reordered_past = ()
for layer_past in past_key_values:
# cached cross_attention states don't have to be reordered -> they are always the same
reordered_past += (
tuple(past_state.index_select(0, beam_idx.to(past_state.device)) for past_state in layer_past[:2])
+ layer_past[2:],
)
return reordered_past
@add_start_docstrings(
"""
LED model with a sequence classification/head on top (a linear layer on top of the pooled output) e.g. for GLUE
tasks.
""",
LED_START_DOCSTRING,
)
class LEDForSequenceClassification(LEDPreTrainedModel):
_tied_weights_keys = ["decoder.embed_tokens.weight", "encoder.embed_tokens.weight"]
def __init__(self, config: LEDConfig, **kwargs):
warnings.warn(
"The `transformers.LEDForSequenceClassification` class is deprecated and will be removed in version 5 of"
" Transformers. No actual method were provided in the original paper on how to perfom"
" sequence classification.",
FutureWarning,
)
super().__init__(config, **kwargs)
self.led = LEDModel(config)
self.classification_head = LEDClassificationHead(
config.d_model,
config.d_model,
config.num_labels,
config.classifier_dropout,
)
# Initialize weights and apply final processing
self.post_init()
@add_start_docstrings_to_model_forward(LED_INPUTS_DOCSTRING)
@add_code_sample_docstrings(
checkpoint=_CHECKPOINT_FOR_DOC,
output_type=Seq2SeqSequenceClassifierOutput,
config_class=_CONFIG_FOR_DOC,
)
def forward(
self,
input_ids: Optional[torch.LongTensor] = None,
attention_mask: Optional[torch.Tensor] = None,
decoder_input_ids: Optional[torch.LongTensor] = None,
decoder_attention_mask: Optional[torch.LongTensor] = None,
head_mask: Optional[torch.Tensor] = None,
decoder_head_mask: Optional[torch.Tensor] = None,
cross_attn_head_mask: Optional[torch.Tensor] = None,
encoder_outputs: Optional[Tuple[Tuple[torch.FloatTensor]]] = None,
global_attention_mask: Optional[torch.FloatTensor] = None,
inputs_embeds: Optional[torch.FloatTensor] = None,
decoder_inputs_embeds: Optional[torch.FloatTensor] = None,
labels: Optional[torch.LongTensor] = None,
use_cache: Optional[bool] = None,
output_attentions: Optional[bool] = None,
output_hidden_states: Optional[bool] = None,
return_dict: Optional[bool] = None,
) -> Union[Tuple[torch.Tensor], LEDSeq2SeqSequenceClassifierOutput]:
r"""
labels (`torch.LongTensor` of shape `(batch_size,)`, *optional*):
Labels for computing the sequence classification/regression loss. Indices should be in `[0, ...,
config.num_labels - 1]`. If `config.num_labels > 1` a classification loss is computed (Cross-Entropy).
"""
return_dict = return_dict if return_dict is not None else self.config.use_return_dict
if labels is not None:
use_cache = False
if input_ids is None and inputs_embeds is not None:
raise NotImplementedError(
f"Passing input embeddings is currently not supported for {self.__class__.__name__}"
)
outputs = self.led(
input_ids,
attention_mask=attention_mask,
decoder_input_ids=decoder_input_ids,
decoder_attention_mask=decoder_attention_mask,
global_attention_mask=global_attention_mask,
head_mask=head_mask,
decoder_head_mask=decoder_head_mask,
cross_attn_head_mask=cross_attn_head_mask,
encoder_outputs=encoder_outputs,
inputs_embeds=inputs_embeds,
decoder_inputs_embeds=decoder_inputs_embeds,
use_cache=use_cache,
output_attentions=output_attentions,
output_hidden_states=output_hidden_states,
return_dict=return_dict,
)
hidden_states = outputs[0] # last hidden state
eos_mask = input_ids.eq(self.config.eos_token_id).to(hidden_states.device)
if len(torch.unique_consecutive(eos_mask.sum(1))) > 1:
raise ValueError("All examples must have the same number of <eos> tokens.")
sentence_representation = hidden_states[eos_mask, :].view(hidden_states.size(0), -1, hidden_states.size(-1))[
:, -1, :
]
logits = self.classification_head(sentence_representation)
loss = None
if labels is not None:
if self.config.problem_type is None:
if self.config.num_labels == 1:
self.config.problem_type = "regression"
elif self.config.num_labels > 1 and (labels.dtype == torch.long or labels.dtype == torch.int):
self.config.problem_type = "single_label_classification"
else:
self.config.problem_type = "multi_label_classification"
if self.config.problem_type == "regression":
loss_fct = MSELoss()
if self.config.num_labels == 1:
loss = loss_fct(logits.squeeze(), labels.squeeze())
else:
loss = loss_fct(logits, labels)
elif self.config.problem_type == "single_label_classification":
loss_fct = CrossEntropyLoss()
loss = loss_fct(logits.view(-1, self.config.num_labels), labels.view(-1))
elif self.config.problem_type == "multi_label_classification":
loss_fct = BCEWithLogitsLoss()
loss = loss_fct(logits, labels)
if not return_dict:
output = (logits,) + outputs[1:]
return ((loss,) + output) if loss is not None else output
return LEDSeq2SeqSequenceClassifierOutput(
loss=loss,
logits=logits,
past_key_values=outputs.past_key_values,
decoder_hidden_states=outputs.decoder_hidden_states,
decoder_attentions=outputs.decoder_attentions,
cross_attentions=outputs.cross_attentions,
encoder_last_hidden_state=outputs.encoder_last_hidden_state,
encoder_hidden_states=outputs.encoder_hidden_states,
encoder_attentions=outputs.encoder_attentions,
encoder_global_attentions=outputs.encoder_global_attentions,
)
@add_start_docstrings(
"""
LED Model with a span classification head on top for extractive question-answering tasks like SQuAD (a linear layer
on top of the hidden-states output to compute `span start logits` and `span end logits`).
""",
LED_START_DOCSTRING,
)
class LEDForQuestionAnswering(LEDPreTrainedModel):
_tied_weights_keys = ["decoder.embed_tokens.weight", "encoder.embed_tokens.weight"]
def __init__(self, config):
super().__init__(config)
config.num_labels = 2
self.num_labels = config.num_labels
self.led = LEDModel(config)
self.qa_outputs = nn.Linear(config.hidden_size, config.num_labels)
# Initialize weights and apply final processing
self.post_init()
@add_start_docstrings_to_model_forward(LED_INPUTS_DOCSTRING)
@add_code_sample_docstrings(
checkpoint=_CHECKPOINT_FOR_DOC,
output_type=Seq2SeqQuestionAnsweringModelOutput,
config_class=_CONFIG_FOR_DOC,
)
def forward(
self,
input_ids: Optional[torch.LongTensor] = None,
attention_mask: Optional[torch.Tensor] = None,
decoder_input_ids: Optional[torch.LongTensor] = None,
decoder_attention_mask: Optional[torch.LongTensor] = None,
head_mask: Optional[torch.Tensor] = None,
decoder_head_mask: Optional[torch.Tensor] = None,
cross_attn_head_mask: Optional[torch.Tensor] = None,
encoder_outputs: Optional[Tuple[Tuple[torch.FloatTensor]]] = None,
global_attention_mask: Optional[torch.FloatTensor] = None,
start_positions: Optional[torch.LongTensor] = None,
end_positions: Optional[torch.LongTensor] = None,
inputs_embeds: Optional[torch.FloatTensor] = None,
decoder_inputs_embeds: Optional[torch.FloatTensor] = None,
use_cache: Optional[bool] = None,
output_attentions: Optional[bool] = None,
output_hidden_states: Optional[bool] = None,
return_dict: Optional[bool] = None,
) -> Union[Tuple[torch.Tensor], LEDSeq2SeqQuestionAnsweringModelOutput]:
r"""
start_positions (`torch.LongTensor` of shape `(batch_size,)`, *optional*):
Labels for position (index) of the start of the labelled span for computing the token classification loss.
Positions are clamped to the length of the sequence (*sequence_length*). Position outside of the sequence
are not taken into account for computing the loss.
end_positions (`torch.LongTensor` of shape `(batch_size,)`, *optional*):
Labels for position (index) of the end of the labelled span for computing the token classification loss.
Positions are clamped to the length of the sequence (*sequence_length*). Position outside of the sequence
are not taken into account for computing the loss.
"""
return_dict = return_dict if return_dict is not None else self.config.use_return_dict
if start_positions is not None and end_positions is not None:
use_cache = False
outputs = self.led(
input_ids,
attention_mask=attention_mask,
decoder_input_ids=decoder_input_ids,
decoder_attention_mask=decoder_attention_mask,
global_attention_mask=global_attention_mask,
head_mask=head_mask,
decoder_head_mask=decoder_head_mask,
cross_attn_head_mask=cross_attn_head_mask,
encoder_outputs=encoder_outputs,
inputs_embeds=inputs_embeds,
decoder_inputs_embeds=decoder_inputs_embeds,
use_cache=use_cache,
output_attentions=output_attentions,
output_hidden_states=output_hidden_states,
return_dict=return_dict,
)
sequence_output = outputs[0]
logits = self.qa_outputs(sequence_output)
start_logits, end_logits = logits.split(1, dim=-1)
start_logits = start_logits.squeeze(-1).contiguous()
end_logits = end_logits.squeeze(-1).contiguous()
total_loss = None
if start_positions is not None and end_positions is not None:
# If we are on multi-GPU, split add a dimension
if len(start_positions.size()) > 1:
start_positions = start_positions.squeeze(-1)
if len(end_positions.size()) > 1:
end_positions = end_positions.squeeze(-1)
# sometimes the start/end positions are outside our model inputs, we ignore these terms
ignored_index = start_logits.size(1)
start_positions = start_positions.clamp(0, ignored_index)
end_positions = end_positions.clamp(0, ignored_index)
loss_fct = CrossEntropyLoss(ignore_index=ignored_index)
start_loss = loss_fct(start_logits, start_positions)
end_loss = loss_fct(end_logits, end_positions)
total_loss = (start_loss + end_loss) / 2
if not return_dict:
output = (
start_logits,
end_logits,
) + outputs[1:]
return ((total_loss,) + output) if total_loss is not None else output
return LEDSeq2SeqQuestionAnsweringModelOutput(
loss=total_loss,
start_logits=start_logits,
end_logits=end_logits,
past_key_values=outputs.past_key_values,
decoder_hidden_states=outputs.decoder_hidden_states,
decoder_attentions=outputs.decoder_attentions,
cross_attentions=outputs.cross_attentions,
encoder_last_hidden_state=outputs.encoder_last_hidden_state,
encoder_hidden_states=outputs.encoder_hidden_states,
encoder_attentions=outputs.encoder_attentions,
encoder_global_attentions=outputs.encoder_global_attentions,
)
| transformers/src/transformers/models/led/modeling_led.py/0 | {
"file_path": "transformers/src/transformers/models/led/modeling_led.py",
"repo_id": "transformers",
"token_count": 59459
} | 340 |
# coding=utf-8
# Copyright 2023 Meta AI, EleutherAI and the HuggingFace Inc. team. All rights reserved.
#
# This code is based on EleutherAI's GPT-NeoX library and the GPT-NeoX
# and OPT implementations in this library. It has been modified from its
# original forms to accommodate minor architectural differences compared
# to GPT-NeoX and OPT used by the Meta AI team that trained the model.
#
# Licensed under the Apache License, Version 2.0 (the "License");
# you may not use this file except in compliance with the License.
# You may obtain a copy of the License at
#
# http://www.apache.org/licenses/LICENSE-2.0
#
# Unless required by applicable law or agreed to in writing, software
# distributed under the License is distributed on an "AS IS" BASIS,
# WITHOUT WARRANTIES OR CONDITIONS OF ANY KIND, either express or implied.
# See the License for the specific language governing permissions and
# limitations under the License.
"""Flax LLaMA model."""
from functools import partial
from typing import Optional, Tuple
import flax.linen as nn
import jax
import jax.numpy as jnp
import numpy as np
from flax.core.frozen_dict import FrozenDict, freeze, unfreeze
from flax.linen import combine_masks, make_causal_mask
from flax.linen.attention import dot_product_attention_weights
from flax.traverse_util import flatten_dict, unflatten_dict
from jax import lax
from ...modeling_flax_outputs import FlaxBaseModelOutput, FlaxCausalLMOutput
from ...modeling_flax_utils import ACT2FN, FlaxPreTrainedModel, append_call_sample_docstring
from ...utils import add_start_docstrings, add_start_docstrings_to_model_forward, logging
from .configuration_llama import LlamaConfig
logger = logging.get_logger(__name__)
_CONFIG_FOR_DOC = "LlamaConfig"
_CHECKPOINT_FOR_DOC = "afmck/testing-llama-tiny"
_REAL_CHECKPOINT_FOR_DOC = "openlm-research/open_llama_3b_v2"
LLAMA_START_DOCSTRING = r"""
This model inherits from [`FlaxPreTrainedModel`]. Check the superclass documentation for the generic methods the
library implements for all its model (such as downloading or saving, resizing the input embeddings, pruning heads
etc.)
This model is also a Flax Linen
[flax.nn.Module](https://flax.readthedocs.io/en/latest/_autosummary/flax.nn.module.html) subclass. Use it as a
regular Flax Module and refer to the Flax documentation for all matter related to general usage and behavior.
Finally, this model supports inherent JAX features such as:
- [Just-In-Time (JIT) compilation](https://jax.readthedocs.io/en/latest/jax.html#just-in-time-compilation-jit)
- [Automatic Differentiation](https://jax.readthedocs.io/en/latest/jax.html#automatic-differentiation)
- [Vectorization](https://jax.readthedocs.io/en/latest/jax.html#vectorization-vmap)
- [Parallelization](https://jax.readthedocs.io/en/latest/jax.html#parallelization-pmap)
Parameters:
config ([`LlamaConfig`]): Model configuration class with all the parameters of the model.
Initializing with a config file does not load the weights associated with the model, only the
configuration. Check out the [`~FlaxPreTrainedModel.from_pretrained`] method to load the model weights.
dtype (`jax.numpy.dtype`, *optional*, defaults to `jax.numpy.float32`):
The data type of the computation. Can be one of `jax.numpy.float32`, `jax.numpy.float16`, or
`jax.numpy.bfloat16`.
This can be used to enable mixed-precision training or half-precision inference on GPUs or TPUs. If
specified all the computation will be performed with the given `dtype`.
**Note that this only specifies the dtype of the computation and does not influence the dtype of model
parameters.**
If you wish to change the dtype of the model parameters, see [`~FlaxPreTrainedModel.to_fp16`] and
[`~FlaxPreTrainedModel.to_bf16`].
"""
LLAMA_INPUTS_DOCSTRING = r"""
Args:
input_ids (`numpy.ndarray` of shape `(batch_size, input_ids_length)`):
Indices of input sequence tokens in the vocabulary. Padding will be ignored by default should you provide
it.
Indices can be obtained using [`AutoTokenizer`]. See [`PreTrainedTokenizer.encode`] and
[`PreTrainedTokenizer.__call__`] for details.
[What are input IDs?](../glossary#input-ids)
attention_mask (`numpy.ndarray` of shape `(batch_size, sequence_length)`, *optional*):
Mask to avoid performing attention on padding token indices. Mask values selected in `[0, 1]`:
- 1 for tokens that are **not masked**,
- 0 for tokens that are **masked**.
[What are attention masks?](../glossary#attention-mask)
Indices can be obtained using [`AutoTokenizer`]. See [`PreTrainedTokenizer.encode`] and
[`PreTrainedTokenizer.__call__`] for details.
If `past_key_values` is used, optionally only the last `decoder_input_ids` have to be input (see
`past_key_values`).
If you want to change padding behavior, you should read [`modeling_opt._prepare_decoder_attention_mask`]
and modify to your needs. See diagram 1 in [the paper](https://arxiv.org/abs/1910.13461) for more
information on the default strategy.
- 1 indicates the head is **not masked**,
- 0 indicates the head is **masked**.
position_ids (`numpy.ndarray` of shape `(batch_size, sequence_length)`, *optional*):
Indices of positions of each input sequence tokens in the position embeddings. Selected in the range `[0,
config.n_positions - 1]`.
[What are position IDs?](../glossary#position-ids)
past_key_values (`Dict[str, np.ndarray]`, *optional*, returned by `init_cache` or when passing previous `past_key_values`):
Dictionary of pre-computed hidden-states (key and values in the attention blocks) that can be used for fast
auto-regressive decoding. Pre-computed key and value hidden-states are of shape *[batch_size, max_length]*.
output_attentions (`bool`, *optional*):
Whether or not to return the attentions tensors of all attention layers. See `attentions` under returned
tensors for more detail.
output_hidden_states (`bool`, *optional*):
Whether or not to return the hidden states of all layers. See `hidden_states` under returned tensors for
more detail.
return_dict (`bool`, *optional*):
Whether or not to return a [`~utils.ModelOutput`] instead of a plain tuple.
"""
def create_sinusoidal_positions(num_pos, dim):
inv_freq = 1.0 / (10000 ** (np.arange(0, dim, 2) / dim))
freqs = np.einsum("i , j -> i j", np.arange(num_pos), inv_freq).astype("float32")
emb = np.concatenate((freqs, freqs), axis=-1)
out = np.concatenate((np.sin(emb)[:, None, :], np.cos(emb)[:, None, :]), axis=-1)
return jnp.array(out[:, :, :num_pos])
def rotate_half(tensor):
"""Rotates half the hidden dims of the input."""
rotate_half_tensor = jnp.concatenate(
(-tensor[..., tensor.shape[-1] // 2 :], tensor[..., : tensor.shape[-1] // 2]), axis=-1
)
return rotate_half_tensor
def apply_rotary_pos_emb(tensor, sin_pos, cos_pos):
return (tensor * cos_pos) + (rotate_half(tensor) * sin_pos)
class FlaxLlamaRMSNorm(nn.Module):
config: LlamaConfig
dtype: jnp.dtype = jnp.float32
def setup(self):
self.epsilon = self.config.rms_norm_eps
self.weight = self.param("weight", lambda _, shape: jnp.ones(shape), self.config.hidden_size)
def __call__(self, hidden_states):
variance = jnp.asarray(hidden_states, dtype=jnp.float32)
variance = jnp.power(variance, 2)
variance = variance.mean(-1, keepdims=True)
# use `jax.numpy.sqrt` as `jax.lax.rsqrt` does not match `torch.rsqrt`
hidden_states = hidden_states / jnp.sqrt(variance + self.epsilon)
return self.weight * jnp.asarray(hidden_states, dtype=self.dtype)
class FlaxLlamaRotaryEmbedding(nn.Module):
config: LlamaConfig
dtype: jnp.dtype = jnp.float32
def setup(self):
head_dim = self.config.hidden_size // self.config.num_attention_heads
self.sincos = create_sinusoidal_positions(self.config.max_position_embeddings, head_dim)
def __call__(self, key, query, position_ids):
sincos = self.sincos[position_ids]
sin_pos, cos_pos = jnp.split(sincos, 2, axis=-1)
key = apply_rotary_pos_emb(key, sin_pos, cos_pos)
query = apply_rotary_pos_emb(query, sin_pos, cos_pos)
key = jnp.asarray(key, dtype=self.dtype)
query = jnp.asarray(query, dtype=self.dtype)
return key, query
class FlaxLlamaAttention(nn.Module):
config: LlamaConfig
dtype: jnp.dtype = jnp.float32
causal: bool = True
is_cross_attention: bool = False
def setup(self):
config = self.config
self.embed_dim = config.hidden_size
self.num_heads = config.num_attention_heads
self.head_dim = self.embed_dim // self.num_heads
self.attention_softmax_in_fp32 = self.dtype is not jnp.float32
dense = partial(
nn.Dense,
self.embed_dim,
use_bias=config.attention_bias,
dtype=self.dtype,
kernel_init=jax.nn.initializers.normal(self.config.initializer_range),
)
self.q_proj, self.k_proj, self.v_proj = dense(), dense(), dense()
self.o_proj = dense()
self.causal_mask = make_causal_mask(jnp.ones((1, config.max_position_embeddings), dtype="bool"), dtype="bool")
self.rotary_emb = FlaxLlamaRotaryEmbedding(config, dtype=self.dtype)
def _split_heads(self, hidden_states):
return hidden_states.reshape(hidden_states.shape[:2] + (self.num_heads, self.head_dim))
def _merge_heads(self, hidden_states):
return hidden_states.reshape(hidden_states.shape[:2] + (self.embed_dim,))
@nn.compact
# Copied from transformers.models.gpt_neo.modeling_flax_gpt_neo.FlaxGPTNeoSelfAttention._concatenate_to_cache
def _concatenate_to_cache(self, key, value, query, attention_mask):
"""
This function takes projected key, value states from a single input token and concatenates the states to cached
states from previous steps. This function is slighly adapted from the official Flax repository:
https://github.com/google/flax/blob/491ce18759622506588784b4fca0e4bf05f8c8cd/flax/linen/attention.py#L252
"""
# detect if we're initializing by absence of existing cache data.
is_initialized = self.has_variable("cache", "cached_key")
cached_key = self.variable("cache", "cached_key", jnp.zeros, key.shape, key.dtype)
cached_value = self.variable("cache", "cached_value", jnp.zeros, value.shape, value.dtype)
cache_index = self.variable("cache", "cache_index", lambda: jnp.array(0, dtype=jnp.int32))
if is_initialized:
*batch_dims, max_length, num_heads, depth_per_head = cached_key.value.shape
# update key, value caches with our new 1d spatial slices
cur_index = cache_index.value
indices = (0,) * len(batch_dims) + (cur_index, 0, 0)
key = lax.dynamic_update_slice(cached_key.value, key, indices)
value = lax.dynamic_update_slice(cached_value.value, value, indices)
cached_key.value = key
cached_value.value = value
num_updated_cache_vectors = query.shape[1]
cache_index.value = cache_index.value + num_updated_cache_vectors
# causal mask for cached decoder self-attention: our single query position should only attend to those key positions that have already been generated and cached, not the remaining zero elements.
pad_mask = jnp.broadcast_to(
jnp.arange(max_length) < cur_index + num_updated_cache_vectors,
tuple(batch_dims) + (1, num_updated_cache_vectors, max_length),
)
attention_mask = combine_masks(pad_mask, attention_mask)
return key, value, attention_mask
def __call__(
self,
hidden_states,
attention_mask,
position_ids,
deterministic: bool = True,
init_cache: bool = False,
output_attentions: bool = False,
):
query = self.q_proj(hidden_states)
key = self.k_proj(hidden_states)
value = self.v_proj(hidden_states)
query = self._split_heads(query)
key = self._split_heads(key)
value = self._split_heads(value)
key, query = self.rotary_emb(key, query, position_ids)
query_length, key_length = query.shape[1], key.shape[1]
if self.has_variable("cache", "cached_key"):
mask_shift = self.variables["cache"]["cache_index"]
max_decoder_length = self.variables["cache"]["cached_key"].shape[1]
causal_mask = lax.dynamic_slice(
self.causal_mask, (0, 0, mask_shift, 0), (1, 1, query_length, max_decoder_length)
)
else:
causal_mask = self.causal_mask[:, :, :query_length, :key_length]
batch_size = hidden_states.shape[0]
causal_mask = jnp.broadcast_to(causal_mask, (batch_size,) + causal_mask.shape[1:])
attention_mask = jnp.broadcast_to(jnp.expand_dims(attention_mask, axis=(-3, -2)), causal_mask.shape)
attention_mask = combine_masks(attention_mask, causal_mask)
dropout_rng = None
if not deterministic and self.config.attention_dropout > 0.0:
dropout_rng = self.make_rng("dropout")
# During fast autoregressive decoding, we feed one position at a time,
# and cache the keys and values step by step.
if self.has_variable("cache", "cached_key") or init_cache:
key, value, attention_mask = self._concatenate_to_cache(key, value, query, attention_mask)
# transform boolean mask into float mask
attention_bias = lax.select(
attention_mask > 0,
jnp.full(attention_mask.shape, 0.0).astype(self.dtype),
jnp.full(attention_mask.shape, jnp.finfo(self.dtype).min).astype(self.dtype),
)
# usual dot product attention
attention_dtype = jnp.float32 if self.attention_softmax_in_fp32 else self.dtype
attn_weights = dot_product_attention_weights(
query,
key,
bias=attention_bias,
dropout_rng=dropout_rng,
dropout_rate=self.config.attention_dropout,
deterministic=deterministic,
dtype=attention_dtype,
)
if self.attention_softmax_in_fp32:
attn_weights = attn_weights.astype(self.dtype)
attn_output = jnp.einsum("...hqk,...khd->...qhd", attn_weights, value)
attn_output = self._merge_heads(attn_output)
attn_output = self.o_proj(attn_output)
outputs = (attn_output, attn_weights) if output_attentions else (attn_output,)
return outputs
class FlaxLlamaMLP(nn.Module):
config: LlamaConfig
dtype: jnp.dtype = jnp.float32
def setup(self):
embed_dim = self.config.hidden_size
inner_dim = self.config.intermediate_size if self.config.intermediate_size is not None else 4 * embed_dim
kernel_init = jax.nn.initializers.normal(self.config.initializer_range)
self.act = ACT2FN[self.config.hidden_act]
self.gate_proj = nn.Dense(inner_dim, use_bias=False, dtype=self.dtype, kernel_init=kernel_init)
self.down_proj = nn.Dense(embed_dim, use_bias=False, dtype=self.dtype, kernel_init=kernel_init)
self.up_proj = nn.Dense(inner_dim, use_bias=False, dtype=self.dtype, kernel_init=kernel_init)
def __call__(self, hidden_states):
up_proj_states = self.up_proj(hidden_states)
gate_states = self.act(self.gate_proj(hidden_states))
hidden_states = self.down_proj(up_proj_states * gate_states)
return hidden_states
class FlaxLlamaDecoderLayer(nn.Module):
config: LlamaConfig
dtype: jnp.dtype = jnp.float32
def setup(self):
self.input_layernorm = FlaxLlamaRMSNorm(self.config, dtype=self.dtype)
self.self_attn = FlaxLlamaAttention(self.config, dtype=self.dtype)
self.post_attention_layernorm = FlaxLlamaRMSNorm(self.config, dtype=self.dtype)
self.mlp = FlaxLlamaMLP(self.config, dtype=self.dtype)
def __call__(
self,
hidden_states,
attention_mask=None,
position_ids=None,
deterministic: bool = True,
init_cache: bool = False,
output_attentions: bool = False,
):
residual = hidden_states
hidden_states = self.input_layernorm(hidden_states)
outputs = self.self_attn(
hidden_states,
attention_mask=attention_mask,
position_ids=position_ids,
deterministic=deterministic,
init_cache=init_cache,
output_attentions=output_attentions,
)
# residual connection
attn_output = outputs[0]
hidden_states = residual + attn_output
residual = hidden_states
hidden_states = self.post_attention_layernorm(hidden_states)
hidden_states = self.mlp(hidden_states)
# residual connection
hidden_states = residual + hidden_states
return (hidden_states,) + outputs[1:]
# Copied from transformers.models.gpt_neo.modeling_flax_gpt_neo.FlaxGPTNeoPreTrainedModel with GPTNeo->Llama, GPT_NEO->LLAMA, transformer->model
class FlaxLlamaPreTrainedModel(FlaxPreTrainedModel):
"""
An abstract class to handle weights initialization and a simple interface for downloading and loading pretrained
models.
"""
config_class = LlamaConfig
base_model_prefix = "model"
module_class: nn.Module = None
def __init__(
self,
config: LlamaConfig,
input_shape: Tuple = (1, 1),
seed: int = 0,
dtype: jnp.dtype = jnp.float32,
_do_init: bool = True,
**kwargs,
):
module = self.module_class(config=config, dtype=dtype, **kwargs)
super().__init__(config, module, input_shape=input_shape, seed=seed, dtype=dtype, _do_init=_do_init)
def init_weights(self, rng: jax.random.PRNGKey, input_shape: Tuple, params: FrozenDict = None) -> FrozenDict:
# init input tensors
input_ids = jnp.zeros(input_shape, dtype="i4")
attention_mask = jnp.ones_like(input_ids)
position_ids = jnp.broadcast_to(jnp.arange(jnp.atleast_2d(input_ids).shape[-1]), input_shape)
params_rng, dropout_rng = jax.random.split(rng)
rngs = {"params": params_rng, "dropout": dropout_rng}
random_params = self.module.init(rngs, input_ids, attention_mask, position_ids, return_dict=False)["params"]
if params is not None:
random_params = flatten_dict(unfreeze(random_params))
params = flatten_dict(unfreeze(params))
for missing_key in self._missing_keys:
params[missing_key] = random_params[missing_key]
self._missing_keys = set()
return freeze(unflatten_dict(params))
else:
return random_params
def init_cache(self, batch_size, max_length):
r"""
Args:
batch_size (`int`):
batch_size used for fast auto-regressive decoding. Defines the batch size of the initialized cache.
max_length (`int`):
maximum possible length for auto-regressive decoding. Defines the sequence length of the initialized
cache.
"""
# init input variables to retrieve cache
input_ids = jnp.ones((batch_size, max_length))
attention_mask = jnp.ones_like(input_ids)
position_ids = jnp.broadcast_to(jnp.arange(jnp.atleast_2d(input_ids).shape[-1]), input_ids.shape)
init_variables = self.module.init(
jax.random.PRNGKey(0), input_ids, attention_mask, position_ids, return_dict=False, init_cache=True
)
return unfreeze(init_variables["cache"])
@add_start_docstrings_to_model_forward(LLAMA_INPUTS_DOCSTRING)
def __call__(
self,
input_ids,
attention_mask=None,
position_ids=None,
params: dict = None,
past_key_values: dict = None,
dropout_rng: jax.random.PRNGKey = None,
train: bool = False,
output_attentions: Optional[bool] = None,
output_hidden_states: Optional[bool] = None,
return_dict: Optional[bool] = None,
):
output_attentions = output_attentions if output_attentions is not None else self.config.output_attentions
output_hidden_states = (
output_hidden_states if output_hidden_states is not None else self.config.output_hidden_states
)
return_dict = return_dict if return_dict is not None else self.config.return_dict
batch_size, sequence_length = input_ids.shape
if position_ids is None:
if past_key_values is not None:
raise ValueError("Make sure to provide `position_ids` when passing `past_key_values`.")
position_ids = jnp.broadcast_to(jnp.arange(sequence_length)[None, :], (batch_size, sequence_length))
if attention_mask is None:
attention_mask = jnp.ones((batch_size, sequence_length))
# Handle any PRNG if needed
rngs = {}
if dropout_rng is not None:
rngs["dropout"] = dropout_rng
inputs = {"params": params or self.params}
# if past_key_values are passed then cache is already initialized a private flag init_cache has to be passed down to ensure cache is used. It has to be made sure that cache is marked as mutable so that it can be changed by FlaxLlamaAttention module
if past_key_values:
inputs["cache"] = past_key_values
mutable = ["cache"]
else:
mutable = False
outputs = self.module.apply(
inputs,
jnp.array(input_ids, dtype="i4"),
jnp.array(attention_mask, dtype="i4"),
jnp.array(position_ids, dtype="i4"),
not train,
False,
output_attentions,
output_hidden_states,
return_dict,
rngs=rngs,
mutable=mutable,
)
# add updated cache to model output
if past_key_values is not None and return_dict:
outputs, past_key_values = outputs
outputs["past_key_values"] = unfreeze(past_key_values["cache"])
return outputs
elif past_key_values is not None and not return_dict:
outputs, past_key_values = outputs
outputs = outputs[:1] + (unfreeze(past_key_values["cache"]),) + outputs[1:]
return outputs
class FlaxLlamaLayerCollection(nn.Module):
config: LlamaConfig
dtype: jnp.dtype = jnp.float32
def setup(self):
self.blocks = [
FlaxLlamaDecoderLayer(self.config, dtype=self.dtype, name=str(i))
for i in range(self.config.num_hidden_layers)
]
def __call__(
self,
hidden_states,
attention_mask=None,
position_ids=None,
deterministic: bool = True,
init_cache: bool = False,
output_attentions: bool = False,
output_hidden_states: bool = False,
return_dict: bool = False,
):
all_attentions = () if output_attentions else None
all_hidden_states = () if output_hidden_states else None
for block in self.blocks:
if output_hidden_states:
all_hidden_states += (hidden_states,)
layer_outputs = block(
hidden_states,
attention_mask=attention_mask,
position_ids=position_ids,
deterministic=deterministic,
init_cache=init_cache,
output_attentions=output_attentions,
)
hidden_states = layer_outputs[0]
if output_attentions:
all_attentions += (layer_outputs[1],)
# this contains possible `None` values - `FlaxLlamaModule` will filter them out
outputs = (hidden_states, all_hidden_states, all_attentions)
return outputs
class FlaxLlamaModule(nn.Module):
config: LlamaConfig
dtype: jnp.dtype = jnp.float32
def setup(self):
self.hidden_size = self.config.hidden_size
embedding_init = jax.nn.initializers.normal(stddev=self.config.initializer_range)
self.embed_tokens = nn.Embed(
self.config.vocab_size,
self.hidden_size,
embedding_init=embedding_init,
dtype=self.dtype,
)
self.layers = FlaxLlamaLayerCollection(self.config, dtype=self.dtype)
self.norm = FlaxLlamaRMSNorm(self.config, dtype=self.dtype)
def __call__(
self,
input_ids,
attention_mask=None,
position_ids=None,
deterministic=True,
init_cache: bool = False,
output_attentions: bool = False,
output_hidden_states: bool = False,
return_dict: bool = True,
):
input_embeds = self.embed_tokens(input_ids.astype("i4"))
outputs = self.layers(
input_embeds,
position_ids=position_ids,
attention_mask=attention_mask,
deterministic=deterministic,
init_cache=init_cache,
output_attentions=output_attentions,
output_hidden_states=output_hidden_states,
return_dict=return_dict,
)
hidden_states = outputs[0]
hidden_states = self.norm(hidden_states)
if output_hidden_states:
all_hidden_states = outputs[1] + (hidden_states,)
outputs = (hidden_states, all_hidden_states) + outputs[2:]
else:
outputs = (hidden_states,) + outputs[1:]
if not return_dict:
return tuple(v for v in outputs if v is not None)
return FlaxBaseModelOutput(
last_hidden_state=hidden_states,
hidden_states=outputs[1],
attentions=outputs[-1],
)
@add_start_docstrings(
"The bare Llama Model transformer outputting raw hidden-states without any specific head on top.",
LLAMA_START_DOCSTRING,
)
class FlaxLlamaModel(FlaxLlamaPreTrainedModel):
module_class = FlaxLlamaModule
append_call_sample_docstring(
FlaxLlamaModel,
_CHECKPOINT_FOR_DOC,
FlaxBaseModelOutput,
_CONFIG_FOR_DOC,
real_checkpoint=_REAL_CHECKPOINT_FOR_DOC,
)
class FlaxLlamaForCausalLMModule(nn.Module):
config: LlamaConfig
dtype: jnp.dtype = jnp.float32
def setup(self):
self.model = FlaxLlamaModule(self.config, dtype=self.dtype)
self.lm_head = nn.Dense(
self.config.vocab_size,
use_bias=False,
dtype=self.dtype,
kernel_init=jax.nn.initializers.normal(stddev=self.config.initializer_range),
)
def __call__(
self,
input_ids,
attention_mask=None,
position_ids=None,
deterministic: bool = True,
init_cache: bool = False,
output_attentions: bool = False,
output_hidden_states: bool = False,
return_dict: bool = True,
):
outputs = self.model(
input_ids,
position_ids=position_ids,
attention_mask=attention_mask,
deterministic=deterministic,
init_cache=init_cache,
output_attentions=output_attentions,
output_hidden_states=output_hidden_states,
return_dict=return_dict,
)
hidden_states = outputs[0]
lm_logits = self.lm_head(hidden_states)
if not return_dict:
return (lm_logits,) + outputs[1:]
return FlaxCausalLMOutput(logits=lm_logits, hidden_states=outputs.hidden_states, attentions=outputs.attentions)
@add_start_docstrings(
"""
The Llama Model transformer with a language modeling head (linear layer) on top.
""",
LLAMA_START_DOCSTRING,
)
# Copied from transformers.models.gptj.modeling_flax_gptj.FlaxGPTJForCausalLM with GPTJ->Llama
class FlaxLlamaForCausalLM(FlaxLlamaPreTrainedModel):
module_class = FlaxLlamaForCausalLMModule
def prepare_inputs_for_generation(self, input_ids, max_length, attention_mask: Optional[jax.Array] = None):
# initializing the cache
batch_size, seq_length = input_ids.shape
past_key_values = self.init_cache(batch_size, max_length)
# Note that usually one would have to put 0's in the attention_mask for x > input_ids.shape[-1] and x < cache_length.
# But since Llama uses a causal mask, those positions are masked anyways.
# Thus we can create a single static attention_mask here, which is more efficient for compilation
extended_attention_mask = jnp.ones((batch_size, max_length), dtype="i4")
if attention_mask is not None:
position_ids = attention_mask.cumsum(axis=-1) - 1
extended_attention_mask = lax.dynamic_update_slice(extended_attention_mask, attention_mask, (0, 0))
else:
position_ids = jnp.broadcast_to(jnp.arange(seq_length, dtype="i4")[None, :], (batch_size, seq_length))
return {
"past_key_values": past_key_values,
"attention_mask": extended_attention_mask,
"position_ids": position_ids,
}
def update_inputs_for_generation(self, model_outputs, model_kwargs):
model_kwargs["past_key_values"] = model_outputs.past_key_values
model_kwargs["position_ids"] = model_kwargs["position_ids"][:, -1:] + 1
return model_kwargs
append_call_sample_docstring(
FlaxLlamaForCausalLM,
_CHECKPOINT_FOR_DOC,
FlaxCausalLMOutput,
_CONFIG_FOR_DOC,
real_checkpoint=_REAL_CHECKPOINT_FOR_DOC,
)
| transformers/src/transformers/models/llama/modeling_flax_llama.py/0 | {
"file_path": "transformers/src/transformers/models/llama/modeling_flax_llama.py",
"repo_id": "transformers",
"token_count": 12889
} | 341 |
# coding=utf-8
# Copyright 2022 The HuggingFace Inc. team.
#
# Licensed under the Apache License, Version 2.0 (the "License");
# you may not use this file except in compliance with the License.
# You may obtain a copy of the License at
#
# http://www.apache.org/licenses/LICENSE-2.0
#
# Unless required by applicable law or agreed to in writing, software
# distributed under the License is distributed on an "AS IS" BASIS,
# WITHOUT WARRANTIES OR CONDITIONS OF ANY KIND, either express or implied.
# See the License for the specific language governing permissions and
# limitations under the License.
"""
Feature extractor class for MarkupLM.
"""
import html
from ...feature_extraction_utils import BatchFeature, FeatureExtractionMixin
from ...utils import is_bs4_available, logging, requires_backends
if is_bs4_available():
import bs4
from bs4 import BeautifulSoup
logger = logging.get_logger(__name__)
class MarkupLMFeatureExtractor(FeatureExtractionMixin):
r"""
Constructs a MarkupLM feature extractor. This can be used to get a list of nodes and corresponding xpaths from HTML
strings.
This feature extractor inherits from [`~feature_extraction_utils.PreTrainedFeatureExtractor`] which contains most
of the main methods. Users should refer to this superclass for more information regarding those methods.
"""
def __init__(self, **kwargs):
requires_backends(self, ["bs4"])
super().__init__(**kwargs)
def xpath_soup(self, element):
xpath_tags = []
xpath_subscripts = []
child = element if element.name else element.parent
for parent in child.parents: # type: bs4.element.Tag
siblings = parent.find_all(child.name, recursive=False)
xpath_tags.append(child.name)
xpath_subscripts.append(
0 if 1 == len(siblings) else next(i for i, s in enumerate(siblings, 1) if s is child)
)
child = parent
xpath_tags.reverse()
xpath_subscripts.reverse()
return xpath_tags, xpath_subscripts
def get_three_from_single(self, html_string):
html_code = BeautifulSoup(html_string, "html.parser")
all_doc_strings = []
string2xtag_seq = []
string2xsubs_seq = []
for element in html_code.descendants:
if isinstance(element, bs4.element.NavigableString):
if type(element.parent) != bs4.element.Tag:
continue
text_in_this_tag = html.unescape(element).strip()
if not text_in_this_tag:
continue
all_doc_strings.append(text_in_this_tag)
xpath_tags, xpath_subscripts = self.xpath_soup(element)
string2xtag_seq.append(xpath_tags)
string2xsubs_seq.append(xpath_subscripts)
if len(all_doc_strings) != len(string2xtag_seq):
raise ValueError("Number of doc strings and xtags does not correspond")
if len(all_doc_strings) != len(string2xsubs_seq):
raise ValueError("Number of doc strings and xsubs does not correspond")
return all_doc_strings, string2xtag_seq, string2xsubs_seq
def construct_xpath(self, xpath_tags, xpath_subscripts):
xpath = ""
for tagname, subs in zip(xpath_tags, xpath_subscripts):
xpath += f"/{tagname}"
if subs != 0:
xpath += f"[{subs}]"
return xpath
def __call__(self, html_strings) -> BatchFeature:
"""
Main method to prepare for the model one or several HTML strings.
Args:
html_strings (`str`, `List[str]`):
The HTML string or batch of HTML strings from which to extract nodes and corresponding xpaths.
Returns:
[`BatchFeature`]: A [`BatchFeature`] with the following fields:
- **nodes** -- Nodes.
- **xpaths** -- Corresponding xpaths.
Examples:
```python
>>> from transformers import MarkupLMFeatureExtractor
>>> page_name_1 = "page1.html"
>>> page_name_2 = "page2.html"
>>> page_name_3 = "page3.html"
>>> with open(page_name_1) as f:
... single_html_string = f.read()
>>> feature_extractor = MarkupLMFeatureExtractor()
>>> # single example
>>> encoding = feature_extractor(single_html_string)
>>> print(encoding.keys())
>>> # dict_keys(['nodes', 'xpaths'])
>>> # batched example
>>> multi_html_strings = []
>>> with open(page_name_2) as f:
... multi_html_strings.append(f.read())
>>> with open(page_name_3) as f:
... multi_html_strings.append(f.read())
>>> encoding = feature_extractor(multi_html_strings)
>>> print(encoding.keys())
>>> # dict_keys(['nodes', 'xpaths'])
```"""
# Input type checking for clearer error
valid_strings = False
# Check that strings has a valid type
if isinstance(html_strings, str):
valid_strings = True
elif isinstance(html_strings, (list, tuple)):
if len(html_strings) == 0 or isinstance(html_strings[0], str):
valid_strings = True
if not valid_strings:
raise ValueError(
"HTML strings must of type `str`, `List[str]` (batch of examples), "
f"but is of type {type(html_strings)}."
)
is_batched = bool(isinstance(html_strings, (list, tuple)) and (isinstance(html_strings[0], str)))
if not is_batched:
html_strings = [html_strings]
# Get nodes + xpaths
nodes = []
xpaths = []
for html_string in html_strings:
all_doc_strings, string2xtag_seq, string2xsubs_seq = self.get_three_from_single(html_string)
nodes.append(all_doc_strings)
xpath_strings = []
for node, tag_list, sub_list in zip(all_doc_strings, string2xtag_seq, string2xsubs_seq):
xpath_string = self.construct_xpath(tag_list, sub_list)
xpath_strings.append(xpath_string)
xpaths.append(xpath_strings)
# return as Dict
data = {"nodes": nodes, "xpaths": xpaths}
encoded_inputs = BatchFeature(data=data, tensor_type=None)
return encoded_inputs
| transformers/src/transformers/models/markuplm/feature_extraction_markuplm.py/0 | {
"file_path": "transformers/src/transformers/models/markuplm/feature_extraction_markuplm.py",
"repo_id": "transformers",
"token_count": 2744
} | 342 |
# coding=utf-8
# Copyright 2023 The Mega Authors and The HuggingFace Inc. team.
#
# Licensed under the Apache License, Version 2.0 (the "License");
# you may not use this file except in compliance with the License.
# You may obtain a copy of the License at
#
# http://www.apache.org/licenses/LICENSE-2.0
#
# Unless required by applicable law or agreed to in writing, software
# distributed under the License is distributed on an "AS IS" BASIS,
# WITHOUT WARRANTIES OR CONDITIONS OF ANY KIND, either express or implied.
# See the License for the specific language governing permissions and
# limitations under the License.
""" MEGA configuration"""
from collections import OrderedDict
from typing import Mapping
from ...configuration_utils import PretrainedConfig
from ...onnx import OnnxConfig
from ...utils import logging
logger = logging.get_logger(__name__)
MEGA_PRETRAINED_CONFIG_ARCHIVE_MAP = {
"mnaylor/mega-base-wikitext": "https://huggingface.co/mnaylor/mega-base-wikitext/resolve/main/config.json",
}
class MegaConfig(PretrainedConfig):
r"""
This is the configuration class to store the configuration of a [`MegaModel`]. It is used to instantiate a Mega
model according to the specified arguments, defining the model architecture. Instantiating a configuration with the
defaults will yield a similar configuration to that of the Mega
[mnaylor/mega-base-wikitext](https://huggingface.co/mnaylor/mega-base-wikitext) architecture.
Configuration objects inherit from [`PretrainedConfig`] and can be used to control the model outputs. Read the
documentation from [`PretrainedConfig`] for more information.
Args:
vocab_size (`int`, *optional*, defaults to 30522):
Vocabulary size of the Mega model. Defines the number of different tokens that can be represented by the
`inputs_ids` passed when calling [`MegaModel`].
hidden_size (`int`, *optional*, defaults to 128):
Dimensionality of the encoder layers and the pooler layer.
num_hidden_layers (`int`, *optional*, defaults to 4):
Number of hidden layers in the Mega encoder.
intermediate_size (`int`, *optional*, defaults to 256):
Dimensionality of the hidden size (self-attention value projection) within the Mega encoder
ema_projection_size (`int`, *optional*, defaults to 16):
Dimensionality of the MegaMultiDimensionDampedEma
bidirectional (`bool`, *optional*, defaults to `True`):
Whether the MegaMultiDimensionDampedEma used in Mega's self-attention should work bidirectionally (`True`)
or unidirectionally (`False`). Bidirectional EMA is incompatible with causal decoding, so this should be
False if you intend to use the model as a decoder.
shared_representation_size (`int`, *optional*, defaults to 64):
Dimensionality of the linear projection for shared representation of self-attention queries and keys
use_chunking (`bool`, *optional*, defaults to `False`):
Whether to chunk inputs for linear self-attention complexity (described as Mega-chunk in the paper)
chunk_size (`int`, *optional*, defaults to -1):
If `use_chunking` is set to `True`, determines the size of the chunks to apply to the input sequence. If
chunking is used, input sequences must be padded to a multiple of `chunk_size`
truncation (`int`, *optional*):
If specified, the sequence length for which to truncate MegaMultiDimensionDampedEma
normalize_before_mega (`bool`, *optional*, defaults to `True`):
Whether to normalize before (`True`) or after (`False`) passing through Mega encoder blocks
normalization_type (`str`, *optional*, defaults to `"scalenorm"`):
Type of normalization to use in Mega encoder blocks. Choose one of `"scalenorm"`, `"layernorm"`,
`"rmsnorm"`, `"batchnorm"`, or `"syncbatchnorm"` (GPU required for syncbatchnorm)
norm_affine (`bool`, *optional*, defaults to `True`):
If `True`, applies a parameterized affine transformation to inputs during normalization
activation (`str`, *optional*, defaults to `"silu"`):
Activation function to apply within Mega encoder blocks. Choose one of `"silu"`, `"relu"`, `"linear"`,
`"gelu"`, or `"gelu_accurate"`
attention_activation (`str`, *optional*, defaults to `"softmax"`):
Activation function to apply for single-headed self-attention (a la Transformer). Choose one of
`"softmax"`, `"laplace"`, or `"relu2"`
dropout_prob (`float`, *optional*, defaults to 0.1):
The dropout probability for EMA self-attention
hidden_dropout_prob (`float`, *optional*, defaults to 0.1):
The dropout probability for all fully connected layers in the embeddings, encoder, and pooler.
attention_probs_dropout_prob (`float`, *optional*, defaults to 0.1):
The dropout ratio for the attention probabilities.
use_feature_dropout (`bool`, *optional*, defaults to `False`):
Whether to use feature-based (`True`) or standard dropout (`False`)
use_normalized_ffn (`bool`, *optional*, defaults to `True`):
Whether to use the normalized feed-forward sub-layer in Mega blocks (`True`) or pass Mega encoder output
as-is (`False`)
nffn_hidden_size (`int`, *optional*, defaults to 256):
If using the normalized feed-forward network (NFFN) layer within Mega (`use_normalized_ffn = True`), this
is the hidden size of the NFFN
normalize_before_ffn (`bool`, *optional*, defaults to `True`):
Whether to normalize before (`True`) or after (`False`) the feed-forward portion of NFFN
nffn_activation_dropout_prob (`float`, *optional*, defaults to 0.1):
The dropout ratio for the NFFN component.
max_positions (`int`, *optional*, defaults to 2048):
The maximum sequence length to use for positional representations. For `"simple"` relative positional bias,
this is a hard limit on input length; `"rotary"` relative positional bias will extrapolate to longer
sequences
add_token_type_embeddings (`bool`, *optional*, defaults to `True`):
Whether to account for token types in embeddings. Left as optional to maintain compatibility with original
implementation while adding support for token types.
type_vocab_size (`int`, *optional*, defaults to 2):
The vocabulary size of the `token_type_ids` passed when calling [`MegaModel`]. Only used if
`add_token_type_embeddings = True`
initializer_range (`float`, *optional*, defaults to 0.02):
The standard deviation of the truncated_normal_initializer for initializing all weight matrices.
ema_delta_alpha_range (`float`, *optional*, defaults to 0.2):
The standard deviation for initializing the delta (damping factor) and alpha (decay factor) parameters in
MegaMultiDimensionDampedEma.
ema_beta_range (`float`, *optional*, defaults to 0.02):
The standard deviation for initializing the beta parameter (expansion matrix) in
MegaMultiDimensionDampedEma.
ema_gamma_omega_range (`float`, *optional*, defaults to 1.0):
The standard deviation for initializing the gamma (projection matrix) and omega (residual weight)
parameters in MultiDimensionEMA.
relative_positional_bias (`str`, *optional*, defaults to `"rotary"`):
Type of relative positional encoding. Choose one of `"rotary"` or `"simple"`. If `"simple"` is selected,
`max_positions` is used as a limit on input size, while `"rotary"` extrapolates beyond `max_positions`.
is_decoder (`bool`, *optional*, defaults to `False`):
Whether the model is used as a decoder or not. If `False`, the model is used as an encoder.
use_cache (`bool`, *optional*, defaults to `True`):
Whether or not the model should return the last key/values attentions (not used by all models). Only
relevant if `config.is_decoder=True`.
classifier_dropout (`float`, *optional*):
The dropout ratio for the classification head.
add_lm_hidden_dense_layer (`bool`, *optional*, defaults to `True`):
Whether to include a hidden layer for projection between encoder outputs and LM heads (`True`) or pass
hidden states directly to LM head (`False`). Remains optional for compatibility with original
implementation
Examples:
```python
>>> from transformers import MegaConfig, MegaModel
>>> # Initializing a Mega configuration
>>> configuration = MegaConfig()
>>> # Initializing a model (with random weights) from the configuration
>>> model = MegaModel(configuration)
>>> # Accessing the model configuration
>>> configuration = model.config
```"""
model_type = "mega"
def __init__(
self,
vocab_size=30522,
hidden_size=128,
num_hidden_layers=4,
intermediate_size=256,
ema_projection_size=16,
bidirectional=True,
shared_representation_size=64,
use_chunking=False,
chunk_size=-1,
truncation=None,
normalize_before_mega=True,
normalization_type="scalenorm",
norm_affine=True,
activation="silu",
attention_activation="softmax",
dropout_prob=0.1,
hidden_dropout_prob=0.1,
attention_probs_dropout_prob=0.1,
use_feature_dropout=False,
use_normalized_ffn=True,
nffn_hidden_size=256,
normalize_before_ffn=True,
nffn_activation_dropout_prob=0.1,
max_positions=2048,
add_token_type_embeddings=False,
type_vocab_size=2,
initializer_range=0.02,
ema_delta_alpha_range=0.2,
ema_beta_range=0.02,
ema_gamma_omega_range=1.0,
pad_token_id=1,
bos_token_id=0,
eos_token_id=2,
relative_positional_bias="rotary",
classifier_dropout=None,
use_cache=True,
add_lm_hidden_dense_layer=True,
**kwargs,
):
super().__init__(pad_token_id=pad_token_id, bos_token_id=bos_token_id, eos_token_id=eos_token_id, **kwargs)
self.vocab_size = vocab_size
self.hidden_size = hidden_size
self.num_hidden_layers = num_hidden_layers
self.activation = activation
self.attention_activation = attention_activation
self.intermediate_size = intermediate_size
self.ema_projection_size = ema_projection_size
self.bidirectional = bidirectional
self.shared_representation_size = shared_representation_size
self.use_chunking = use_chunking
self.chunk_size = chunk_size
self.truncation = truncation
self.normalize_before_mega = normalize_before_mega
self.normalization_type = normalization_type
self.norm_affine = norm_affine
self.dropout_prob = dropout_prob
self.hidden_dropout_prob = hidden_dropout_prob
self.attention_probs_dropout_prob = attention_probs_dropout_prob
self.use_feature_dropout = use_feature_dropout
self.use_normalized_ffn = use_normalized_ffn
self.nffn_hidden_size = nffn_hidden_size
self.normalize_before_ffn = normalize_before_ffn
self.nffn_activation_dropout_prob = nffn_activation_dropout_prob
self.max_positions = max_positions
self.add_token_type_embeddings = add_token_type_embeddings
self.type_vocab_size = type_vocab_size
self.initializer_range = initializer_range
self.ema_delta_alpha_range = ema_delta_alpha_range
self.ema_beta_range = ema_beta_range
self.ema_gamma_omega_range = ema_gamma_omega_range
self.relative_positional_bias = relative_positional_bias
self.use_cache = use_cache
self.classifier_dropout = classifier_dropout
self.add_lm_hidden_dense_layer = add_lm_hidden_dense_layer
self.num_attention_heads = 1 # not used but required by Hugging Face
class MegaOnnxConfig(OnnxConfig):
@property
def inputs(self) -> Mapping[str, Mapping[int, str]]:
if self.task == "multiple-choice":
dynamic_axis = {0: "batch", 1: "choice", 2: "sequence"}
else:
dynamic_axis = {0: "batch", 1: "sequence"}
return OrderedDict(
[
("input_ids", dynamic_axis),
("attention_mask", dynamic_axis),
]
)
| transformers/src/transformers/models/mega/configuration_mega.py/0 | {
"file_path": "transformers/src/transformers/models/mega/configuration_mega.py",
"repo_id": "transformers",
"token_count": 4928
} | 343 |
# coding=utf-8
# Copyright 2023 Mistral AI and the HuggingFace Inc. team. All rights reserved.
#
# Licensed under the Apache License, Version 2.0 (the "License");
# you may not use this file except in compliance with the License.
# You may obtain a copy of the License at
#
# http://www.apache.org/licenses/LICENSE-2.0
#
# Unless required by applicable law or agreed to in writing, software
# distributed under the License is distributed on an "AS IS" BASIS,
# WITHOUT WARRANTIES OR CONDITIONS OF ANY KIND, either express or implied.
# See the License for the specific language governing permissions and
# limitations under the License.
""" Mistral model configuration"""
from ...configuration_utils import PretrainedConfig
from ...utils import logging
logger = logging.get_logger(__name__)
MISTRAL_PRETRAINED_CONFIG_ARCHIVE_MAP = {
"mistralai/Mistral-7B-v0.1": "https://huggingface.co/mistralai/Mistral-7B-v0.1/resolve/main/config.json",
"mistralai/Mistral-7B-Instruct-v0.1": "https://huggingface.co/mistralai/Mistral-7B-Instruct-v0.1/resolve/main/config.json",
}
class MistralConfig(PretrainedConfig):
r"""
This is the configuration class to store the configuration of a [`MistralModel`]. It is used to instantiate an
Mistral model according to the specified arguments, defining the model architecture. Instantiating a configuration
with the defaults will yield a similar configuration to that of the Mistral-7B-v0.1 or Mistral-7B-Instruct-v0.1.
[mistralai/Mistral-7B-v0.1](https://huggingface.co/mistralai/Mistral-7B-v0.1)
[mistralai/Mistral-7B-Instruct-v0.1](https://huggingface.co/mistralai/Mistral-7B-Instruct-v0.1)
Configuration objects inherit from [`PretrainedConfig`] and can be used to control the model outputs. Read the
documentation from [`PretrainedConfig`] for more information.
Args:
vocab_size (`int`, *optional*, defaults to 32000):
Vocabulary size of the Mistral model. Defines the number of different tokens that can be represented by the
`inputs_ids` passed when calling [`MistralModel`]
hidden_size (`int`, *optional*, defaults to 4096):
Dimension of the hidden representations.
intermediate_size (`int`, *optional*, defaults to 14336):
Dimension of the MLP representations.
num_hidden_layers (`int`, *optional*, defaults to 32):
Number of hidden layers in the Transformer encoder.
num_attention_heads (`int`, *optional*, defaults to 32):
Number of attention heads for each attention layer in the Transformer encoder.
num_key_value_heads (`int`, *optional*, defaults to 8):
This is the number of key_value heads that should be used to implement Grouped Query Attention. If
`num_key_value_heads=num_attention_heads`, the model will use Multi Head Attention (MHA), if
`num_key_value_heads=1 the model will use Multi Query Attention (MQA) otherwise GQA is used. When
converting a multi-head checkpoint to a GQA checkpoint, each group key and value head should be constructed
by meanpooling all the original heads within that group. For more details checkout [this
paper](https://arxiv.org/pdf/2305.13245.pdf). If it is not specified, will default to `8`.
hidden_act (`str` or `function`, *optional*, defaults to `"silu"`):
The non-linear activation function (function or string) in the decoder.
max_position_embeddings (`int`, *optional*, defaults to `4096*32`):
The maximum sequence length that this model might ever be used with. Mistral's sliding window attention
allows sequence of up to 4096*32 tokens.
initializer_range (`float`, *optional*, defaults to 0.02):
The standard deviation of the truncated_normal_initializer for initializing all weight matrices.
rms_norm_eps (`float`, *optional*, defaults to 1e-06):
The epsilon used by the rms normalization layers.
use_cache (`bool`, *optional*, defaults to `True`):
Whether or not the model should return the last key/values attentions (not used by all models). Only
relevant if `config.is_decoder=True`.
pad_token_id (`int`, *optional*):
The id of the padding token.
bos_token_id (`int`, *optional*, defaults to 1):
The id of the "beginning-of-sequence" token.
eos_token_id (`int`, *optional*, defaults to 2):
The id of the "end-of-sequence" token.
tie_word_embeddings (`bool`, *optional*, defaults to `False`):
Whether the model's input and output word embeddings should be tied.
rope_theta (`float`, *optional*, defaults to 10000.0):
The base period of the RoPE embeddings.
sliding_window (`int`, *optional*, defaults to 4096):
Sliding window attention window size. If not specified, will default to `4096`.
attention_dropout (`float`, *optional*, defaults to 0.0):
The dropout ratio for the attention probabilities.
```python
>>> from transformers import MistralModel, MistralConfig
>>> # Initializing a Mistral 7B style configuration
>>> configuration = MistralConfig()
>>> # Initializing a model from the Mistral 7B style configuration
>>> model = MistralModel(configuration)
>>> # Accessing the model configuration
>>> configuration = model.config
```"""
model_type = "mistral"
keys_to_ignore_at_inference = ["past_key_values"]
def __init__(
self,
vocab_size=32000,
hidden_size=4096,
intermediate_size=14336,
num_hidden_layers=32,
num_attention_heads=32,
num_key_value_heads=8,
hidden_act="silu",
max_position_embeddings=4096 * 32,
initializer_range=0.02,
rms_norm_eps=1e-6,
use_cache=True,
pad_token_id=None,
bos_token_id=1,
eos_token_id=2,
tie_word_embeddings=False,
rope_theta=10000.0,
sliding_window=4096,
attention_dropout=0.0,
**kwargs,
):
self.vocab_size = vocab_size
self.max_position_embeddings = max_position_embeddings
self.hidden_size = hidden_size
self.intermediate_size = intermediate_size
self.num_hidden_layers = num_hidden_layers
self.num_attention_heads = num_attention_heads
self.sliding_window = sliding_window
# for backward compatibility
if num_key_value_heads is None:
num_key_value_heads = num_attention_heads
self.num_key_value_heads = num_key_value_heads
self.hidden_act = hidden_act
self.initializer_range = initializer_range
self.rms_norm_eps = rms_norm_eps
self.use_cache = use_cache
self.rope_theta = rope_theta
self.attention_dropout = attention_dropout
super().__init__(
pad_token_id=pad_token_id,
bos_token_id=bos_token_id,
eos_token_id=eos_token_id,
tie_word_embeddings=tie_word_embeddings,
**kwargs,
)
| transformers/src/transformers/models/mistral/configuration_mistral.py/0 | {
"file_path": "transformers/src/transformers/models/mistral/configuration_mistral.py",
"repo_id": "transformers",
"token_count": 2757
} | 344 |
# coding=utf-8
# Copyright 2022 The HuggingFace Inc. team.
#
# Licensed under the Apache License, Version 2.0 (the "License");
# you may not use this file except in compliance with the License.
# You may obtain a copy of the License at
#
# http://www.apache.org/licenses/LICENSE-2.0
#
# Unless required by applicable law or agreed to in writing, software
# distributed under the License is distributed on an "AS IS" BASIS,
# WITHOUT WARRANTIES OR CONDITIONS OF ANY KIND, either express or implied.
# See the License for the specific language governing permissions and
# limitations under the License.
"""Convert MobileViT checkpoints from the ml-cvnets library."""
import argparse
import json
from pathlib import Path
import requests
import torch
from huggingface_hub import hf_hub_download
from PIL import Image
from transformers import (
MobileViTConfig,
MobileViTForImageClassification,
MobileViTForSemanticSegmentation,
MobileViTImageProcessor,
)
from transformers.utils import logging
logging.set_verbosity_info()
logger = logging.get_logger(__name__)
def get_mobilevit_config(mobilevit_name):
config = MobileViTConfig()
# size of the architecture
if "mobilevit_s" in mobilevit_name:
config.hidden_sizes = [144, 192, 240]
config.neck_hidden_sizes = [16, 32, 64, 96, 128, 160, 640]
elif "mobilevit_xs" in mobilevit_name:
config.hidden_sizes = [96, 120, 144]
config.neck_hidden_sizes = [16, 32, 48, 64, 80, 96, 384]
elif "mobilevit_xxs" in mobilevit_name:
config.hidden_sizes = [64, 80, 96]
config.neck_hidden_sizes = [16, 16, 24, 48, 64, 80, 320]
config.hidden_dropout_prob = 0.05
config.expand_ratio = 2.0
if mobilevit_name.startswith("deeplabv3_"):
config.image_size = 512
config.output_stride = 16
config.num_labels = 21
filename = "pascal-voc-id2label.json"
else:
config.num_labels = 1000
filename = "imagenet-1k-id2label.json"
repo_id = "huggingface/label-files"
id2label = json.load(open(hf_hub_download(repo_id, filename, repo_type="dataset"), "r"))
id2label = {int(k): v for k, v in id2label.items()}
config.id2label = id2label
config.label2id = {v: k for k, v in id2label.items()}
return config
def rename_key(name, base_model=False):
for i in range(1, 6):
if f"layer_{i}." in name:
name = name.replace(f"layer_{i}.", f"encoder.layer.{i - 1}.")
if "conv_1." in name:
name = name.replace("conv_1.", "conv_stem.")
if ".block." in name:
name = name.replace(".block.", ".")
if "exp_1x1" in name:
name = name.replace("exp_1x1", "expand_1x1")
if "red_1x1" in name:
name = name.replace("red_1x1", "reduce_1x1")
if ".local_rep.conv_3x3." in name:
name = name.replace(".local_rep.conv_3x3.", ".conv_kxk.")
if ".local_rep.conv_1x1." in name:
name = name.replace(".local_rep.conv_1x1.", ".conv_1x1.")
if ".norm." in name:
name = name.replace(".norm.", ".normalization.")
if ".conv." in name:
name = name.replace(".conv.", ".convolution.")
if ".conv_proj." in name:
name = name.replace(".conv_proj.", ".conv_projection.")
for i in range(0, 2):
for j in range(0, 4):
if f".{i}.{j}." in name:
name = name.replace(f".{i}.{j}.", f".{i}.layer.{j}.")
for i in range(2, 6):
for j in range(0, 4):
if f".{i}.{j}." in name:
name = name.replace(f".{i}.{j}.", f".{i}.")
if "expand_1x1" in name:
name = name.replace("expand_1x1", "downsampling_layer.expand_1x1")
if "conv_3x3" in name:
name = name.replace("conv_3x3", "downsampling_layer.conv_3x3")
if "reduce_1x1" in name:
name = name.replace("reduce_1x1", "downsampling_layer.reduce_1x1")
for i in range(2, 5):
if f".global_rep.{i}.weight" in name:
name = name.replace(f".global_rep.{i}.weight", ".layernorm.weight")
if f".global_rep.{i}.bias" in name:
name = name.replace(f".global_rep.{i}.bias", ".layernorm.bias")
if ".global_rep." in name:
name = name.replace(".global_rep.", ".transformer.")
if ".pre_norm_mha.0." in name:
name = name.replace(".pre_norm_mha.0.", ".layernorm_before.")
if ".pre_norm_mha.1.out_proj." in name:
name = name.replace(".pre_norm_mha.1.out_proj.", ".attention.output.dense.")
if ".pre_norm_ffn.0." in name:
name = name.replace(".pre_norm_ffn.0.", ".layernorm_after.")
if ".pre_norm_ffn.1." in name:
name = name.replace(".pre_norm_ffn.1.", ".intermediate.dense.")
if ".pre_norm_ffn.4." in name:
name = name.replace(".pre_norm_ffn.4.", ".output.dense.")
if ".transformer." in name:
name = name.replace(".transformer.", ".transformer.layer.")
if ".aspp_layer." in name:
name = name.replace(".aspp_layer.", ".")
if ".aspp_pool." in name:
name = name.replace(".aspp_pool.", ".")
if "seg_head." in name:
name = name.replace("seg_head.", "segmentation_head.")
if "segmentation_head.classifier.classifier." in name:
name = name.replace("segmentation_head.classifier.classifier.", "segmentation_head.classifier.")
if "classifier.fc." in name:
name = name.replace("classifier.fc.", "classifier.")
elif (not base_model) and ("segmentation_head." not in name):
name = "mobilevit." + name
return name
def convert_state_dict(orig_state_dict, model, base_model=False):
if base_model:
model_prefix = ""
else:
model_prefix = "mobilevit."
for key in orig_state_dict.copy().keys():
val = orig_state_dict.pop(key)
if key[:8] == "encoder.":
key = key[8:]
if "qkv" in key:
key_split = key.split(".")
layer_num = int(key_split[0][6:]) - 1
transformer_num = int(key_split[3])
layer = model.get_submodule(f"{model_prefix}encoder.layer.{layer_num}")
dim = layer.transformer.layer[transformer_num].attention.attention.all_head_size
prefix = (
f"{model_prefix}encoder.layer.{layer_num}.transformer.layer.{transformer_num}.attention.attention."
)
if "weight" in key:
orig_state_dict[prefix + "query.weight"] = val[:dim, :]
orig_state_dict[prefix + "key.weight"] = val[dim : dim * 2, :]
orig_state_dict[prefix + "value.weight"] = val[-dim:, :]
else:
orig_state_dict[prefix + "query.bias"] = val[:dim]
orig_state_dict[prefix + "key.bias"] = val[dim : dim * 2]
orig_state_dict[prefix + "value.bias"] = val[-dim:]
else:
orig_state_dict[rename_key(key, base_model)] = val
return orig_state_dict
# We will verify our results on an image of cute cats
def prepare_img():
url = "http://images.cocodataset.org/val2017/000000039769.jpg"
im = Image.open(requests.get(url, stream=True).raw)
return im
@torch.no_grad()
def convert_movilevit_checkpoint(mobilevit_name, checkpoint_path, pytorch_dump_folder_path, push_to_hub=False):
"""
Copy/paste/tweak model's weights to our MobileViT structure.
"""
config = get_mobilevit_config(mobilevit_name)
# load original state_dict
state_dict = torch.load(checkpoint_path, map_location="cpu")
# load ๐ค model
if mobilevit_name.startswith("deeplabv3_"):
model = MobileViTForSemanticSegmentation(config).eval()
else:
model = MobileViTForImageClassification(config).eval()
new_state_dict = convert_state_dict(state_dict, model)
model.load_state_dict(new_state_dict)
# Check outputs on an image, prepared by MobileViTImageProcessor
image_processor = MobileViTImageProcessor(crop_size=config.image_size, size=config.image_size + 32)
encoding = image_processor(images=prepare_img(), return_tensors="pt")
outputs = model(**encoding)
logits = outputs.logits
if mobilevit_name.startswith("deeplabv3_"):
assert logits.shape == (1, 21, 32, 32)
if mobilevit_name == "deeplabv3_mobilevit_s":
expected_logits = torch.tensor(
[
[[6.2065, 6.1292, 6.2070], [6.1079, 6.1254, 6.1747], [6.0042, 6.1071, 6.1034]],
[[-6.9253, -6.8653, -7.0398], [-7.3218, -7.3983, -7.3670], [-7.1961, -7.2482, -7.1569]],
[[-4.4723, -4.4348, -4.3769], [-5.3629, -5.4632, -5.4598], [-5.1587, -5.3402, -5.5059]],
]
)
elif mobilevit_name == "deeplabv3_mobilevit_xs":
expected_logits = torch.tensor(
[
[[5.4449, 5.5733, 5.6314], [5.1815, 5.3930, 5.5963], [5.1656, 5.4333, 5.4853]],
[[-9.4423, -9.7766, -9.6714], [-9.1581, -9.5720, -9.5519], [-9.1006, -9.6458, -9.5703]],
[[-7.7721, -7.3716, -7.1583], [-8.4599, -8.0624, -7.7944], [-8.4172, -7.8366, -7.5025]],
]
)
elif mobilevit_name == "deeplabv3_mobilevit_xxs":
expected_logits = torch.tensor(
[
[[6.9811, 6.9743, 7.3123], [7.1777, 7.1931, 7.3938], [7.5633, 7.8050, 7.8901]],
[[-10.5536, -10.2332, -10.2924], [-10.2336, -9.8624, -9.5964], [-10.8840, -10.8158, -10.6659]],
[[-3.4938, -3.0631, -2.8620], [-3.4205, -2.8135, -2.6875], [-3.4179, -2.7945, -2.8750]],
]
)
else:
raise ValueError(f"Unknown mobilevit_name: {mobilevit_name}")
assert torch.allclose(logits[0, :3, :3, :3], expected_logits, atol=1e-4)
else:
assert logits.shape == (1, 1000)
if mobilevit_name == "mobilevit_s":
expected_logits = torch.tensor([-0.9866, 0.2392, -1.1241])
elif mobilevit_name == "mobilevit_xs":
expected_logits = torch.tensor([-2.4761, -0.9399, -1.9587])
elif mobilevit_name == "mobilevit_xxs":
expected_logits = torch.tensor([-1.9364, -1.2327, -0.4653])
else:
raise ValueError(f"Unknown mobilevit_name: {mobilevit_name}")
assert torch.allclose(logits[0, :3], expected_logits, atol=1e-4)
Path(pytorch_dump_folder_path).mkdir(exist_ok=True)
print(f"Saving model {mobilevit_name} to {pytorch_dump_folder_path}")
model.save_pretrained(pytorch_dump_folder_path)
print(f"Saving image processor to {pytorch_dump_folder_path}")
image_processor.save_pretrained(pytorch_dump_folder_path)
if push_to_hub:
model_mapping = {
"mobilevit_s": "mobilevit-small",
"mobilevit_xs": "mobilevit-x-small",
"mobilevit_xxs": "mobilevit-xx-small",
"deeplabv3_mobilevit_s": "deeplabv3-mobilevit-small",
"deeplabv3_mobilevit_xs": "deeplabv3-mobilevit-x-small",
"deeplabv3_mobilevit_xxs": "deeplabv3-mobilevit-xx-small",
}
print("Pushing to the hub...")
model_name = model_mapping[mobilevit_name]
image_processor.push_to_hub(model_name, organization="apple")
model.push_to_hub(model_name, organization="apple")
if __name__ == "__main__":
parser = argparse.ArgumentParser()
# Required parameters
parser.add_argument(
"--mobilevit_name",
default="mobilevit_s",
type=str,
help=(
"Name of the MobileViT model you'd like to convert. Should be one of 'mobilevit_s', 'mobilevit_xs',"
" 'mobilevit_xxs', 'deeplabv3_mobilevit_s', 'deeplabv3_mobilevit_xs', 'deeplabv3_mobilevit_xxs'."
),
)
parser.add_argument(
"--checkpoint_path", required=True, type=str, help="Path to the original state dict (.pt file)."
)
parser.add_argument(
"--pytorch_dump_folder_path", required=True, type=str, help="Path to the output PyTorch model directory."
)
parser.add_argument(
"--push_to_hub", action="store_true", help="Whether or not to push the converted model to the ๐ค hub."
)
args = parser.parse_args()
convert_movilevit_checkpoint(
args.mobilevit_name, args.checkpoint_path, args.pytorch_dump_folder_path, args.push_to_hub
)
| transformers/src/transformers/models/mobilevit/convert_mlcvnets_to_pytorch.py/0 | {
"file_path": "transformers/src/transformers/models/mobilevit/convert_mlcvnets_to_pytorch.py",
"repo_id": "transformers",
"token_count": 5868
} | 345 |
# coding=utf-8
# Copyright 2023 HuggingFace Inc. team and MosaicML NLP team.
#
# Licensed under the Apache License, Version 2.0 (the "License");
# you may not use this file except in compliance with the License.
# You may obtain a copy of the License at
#
# http://www.apache.org/licenses/LICENSE-2.0
#
# Unless required by applicable law or agreed to in writing, software
# distributed under the License is distributed on an "AS IS" BASIS,
# WITHOUT WARRANTIES OR CONDITIONS OF ANY KIND, either express or implied.
# See the License for the specific language governing permissions and
# limitations under the License.
""" Mpt configuration"""
from typing import TYPE_CHECKING, Optional, Union
if TYPE_CHECKING:
pass
from ...configuration_utils import PretrainedConfig
from ...utils import logging
logger = logging.get_logger(__name__)
MPT_PRETRAINED_CONFIG_ARCHIVE_MAP = {
"mosaicml/mpt-7b": "https://huggingface.co/mosaicml/mpt-7b/resolve/main/config.json",
}
class MptAttentionConfig(PretrainedConfig):
"""
This is the configuration class to store the configuration of a [`MptAttention`] class. It is used to instantiate
attention layers according to the specified arguments, defining the layers architecture. Instantiating a
configuration with the defaults will yield a similar configuration to that of the MPT
[mosaicml/mpt-7b](https://huggingface.co/mosaicml/mpt-7b) architecture. Most of the arguments are kept for backward
compatibility with previous MPT models that are hosted on the Hub (previously with `trust_remote_code=True`).
Configuration objects inherit from [`PretrainedConfig`] and can be used to control the model outputs. Read the
documentation from [`PretrainedConfig`] for more information.
Args:
attn_type (`str`, *optional*, defaults to `"multihead_attention"`):
type of attention to use. Options: `"multihead_attention"`, `"multiquery_attention"`.
attn_pdrop (`float`, *optional*, defaults to 0.0):
The dropout probability for the attention layers.
attn_impl (`str`, *optional*, defaults to `"torch"`):
The attention implementation to use. One of `"torch"`, `"flash"`, or `"triton"`.
clip_qkv (`float`, *optional*):
If not `None`, clip the queries, keys, and values in the attention layer to this value.
softmax_scale (`float`, *optional*, defaults to `None`):
If not `None`, scale the softmax in the attention layer by this value. If `None`, will default to
`1/sqrt(hidden_size)`.
prefix_lm (`bool`, *optional*, defaults to `False`)):
Whether the model should operate as a Prefix LM. This requires passing an extra `prefix_mask` argument
which indicates which tokens belong to the prefix. Tokens in the prefix can attend to one another
bi-directionally. Tokens outside the prefix use causal attention.
qk_ln (`bool`, *optional*, defaults to `False`):
Whether to apply layer normalization to the queries and keys in the attention layer.
attn_uses_sequence_id (`bool`, *optional*, defaults to `False`)):
Whether to restrict attention to tokens that have the same token_type_ids. When the model is in `train`
mode, this requires passing an extra *token_type_ids* argument which indicates which sub-sequence each
token belongs to. Defaults to `False` meaning any provided *token_type_ids* will be ignored.
alibi (`bool`, *optional*, defaults to `True`):
Whether or not to use the alibi bias instead of positional embedding.
alibi_bias_max (`int`, *optional*, defaults to 8):
The maximum value of the alibi bias.
"""
def __init__(
self,
attn_type="multihead_attention",
attn_pdrop=0,
attn_impl="torch",
clip_qkv=None,
softmax_scale=None,
prefix_lm=False,
qk_ln=False,
attn_uses_sequence_id=False,
alibi=True,
alibi_bias_max=8,
**kwargs,
):
super().__init__()
self.attn_type = attn_type
self.attn_pdrop = attn_pdrop
self.attn_impl = attn_impl
self.clip_qkv = clip_qkv
self.softmax_scale = softmax_scale
self.prefix_lm = prefix_lm
self.attn_uses_sequence_id = attn_uses_sequence_id
self.alibi = alibi
self.qk_ln = qk_ln
self.alibi_bias_max = alibi_bias_max
if attn_type not in ["multihead_attention", "multiquery_attention"]:
raise ValueError(
f"`attn_type` has to be either `multihead_attention` or `multiquery_attention`. Received: {attn_type}"
)
@classmethod
def from_pretrained(cls, pretrained_model_name_or_path, **kwargs) -> "PretrainedConfig":
cls._set_token_in_kwargs(kwargs)
config_dict, kwargs = cls.get_config_dict(pretrained_model_name_or_path, **kwargs)
if config_dict.get("model_type") == "mpt":
config_dict = config_dict["attn_config"]
if "model_type" in config_dict and hasattr(cls, "model_type") and config_dict["model_type"] != cls.model_type:
logger.warning(
f"You are using a model of type {config_dict['model_type']} to instantiate a model of type "
f"{cls.model_type}. This is not supported for all configurations of models and can yield errors."
)
return cls.from_dict(config_dict, **kwargs)
class MptConfig(PretrainedConfig):
"""
This is the configuration class to store the configuration of a [`MptModel`]. It is used to instantiate a Mpt model
according to the specified arguments, defining the model architecture. Instantiating a configuration with the
defaults will yield a similar configuration to the Mpt-7b architecture
[mosaicml/mpt-7b](https://huggingface.co/mosaicml/mpt-7b).
Configuration objects inherit from [`PretrainedConfig`] and can be used to control the model outputs. Read the
documentation from [`PretrainedConfig`] for more information.
Args:
d_model (`int`, *optional*, defaults to 2048):
Dimensionality of the embeddings and hidden states.
n_heads (`int`, *optional*, defaults to 16):
Number of attention heads for each attention layer in the Transformer encoder.
n_layers (`int`, *optional*, defaults to 24):
Number of hidden layers in the Transformer encoder.
expansion_ratio (`int`, *optional*, defaults to 4):
The ratio of the up/down scale in the MLP.
max_seq_len (`int`, *optional*, defaults to 2048):
The maximum sequence length of the model.
vocab_size (`int`, *optional*, defaults to 50368):
Vocabulary size of the Mpt model. Defines the maximum number of different tokens that can be represented by
the `inputs_ids` passed when calling [`MptModel`]. Check [this
discussion](https://huggingface.co/bigscience/mpt/discussions/120#633d28389addb8530b406c2a) on how the
`vocab_size` has been defined.
resid_pdrop (`float`, *optional*, defaults to 0.0):
The dropout probability applied to the attention output before combining with residual.
layer_norm_epsilon (`float`, *optional*, defaults to 1e-05):
The epsilon to use in the layer normalization layers.
emb_pdrop (`float`, *optional*, defaults to 0.0):
The dropout probability for the embedding layer.
learned_pos_emb (`bool`, *optional*, defaults to `True`):
Whether to use learned positional embeddings.
attn_config (`dict`, *optional*):
A dictionary used to configure the model's attention module.
init_device (`str`, *optional*, defaults to `"cpu"`):
The device to use for parameter initialization. Defined for backward compatibility
logit_scale (`float`, *optional*):
If not None, scale the logits by this value.
no_bias (`bool`, *optional*, defaults to `True`):
Whether to use bias in all linear layers.
verbose (`int`, *optional*, defaults to 0):
The verbosity level to use for logging. Used in the previous versions of MPT models for logging. This
argument is deprecated.
embedding_fraction (`float`, *optional*, defaults to 1.0):
The fraction to scale the gradients of the embedding layer by.
norm_type (`str`, *optional*, defaults to `"low_precision_layernorm"`):
Type of layer norm to use. All MPT models uses the same layer norm implementation. Defined for backward
compatibility.
use_cache (`bool`, *optional*, defaults to `False`):
Whether or not the model should return the last key/values attentions (not used by all models).
initializer_range (`float`, *optional*, defaults to 0.02):
The standard deviation of the truncated_normal_initializer for initializing all weight matrices.
Example:
```python
>>> from transformers import MptConfig, MptModel
>>> # Initializing a Mpt configuration
>>> configuration = MptConfig()
>>> # Initializing a model (with random weights) from the configuration
>>> model = MptModel(configuration)
>>> # Accessing the model configuration
>>> configuration = model.config
```
"""
model_type = "mpt"
attribute_map = {
"num_attention_heads": "n_heads",
"hidden_size": "d_model",
"num_hidden_layers": "n_layers",
}
def __init__(
self,
d_model: int = 2048,
n_heads: int = 16,
n_layers: int = 24,
expansion_ratio: int = 4,
max_seq_len: int = 2048,
vocab_size: int = 50368,
resid_pdrop: float = 0.0,
layer_norm_epsilon: float = 1e-5,
emb_pdrop: float = 0.0,
learned_pos_emb: bool = True,
attn_config: MptAttentionConfig = None,
init_device: str = "cpu",
logit_scale: Optional[Union[float, str]] = None,
no_bias: bool = True,
verbose: int = 0,
embedding_fraction: float = 1.0,
norm_type: str = "low_precision_layernorm",
use_cache: bool = False,
initializer_range=0.02,
**kwargs,
):
if attn_config is None:
self.attn_config = MptAttentionConfig()
elif isinstance(attn_config, dict):
self.attn_config = MptAttentionConfig(**attn_config)
else:
self.attn_config = attn_config
self.d_model = d_model
self.n_heads = n_heads
self.n_layers = n_layers
self.expansion_ratio = expansion_ratio
self.max_seq_len = max_seq_len
self.vocab_size = vocab_size
self.resid_pdrop = resid_pdrop
self.emb_pdrop = emb_pdrop
self.learned_pos_emb = learned_pos_emb
self.init_device = init_device
self.logit_scale = logit_scale
self.no_bias = no_bias
self.verbose = verbose
self.embedding_fraction = embedding_fraction
self.norm_type = norm_type
self.layer_norm_epsilon = layer_norm_epsilon
self.use_cache = use_cache
self.initializer_range = initializer_range
super().__init__(**kwargs)
| transformers/src/transformers/models/mpt/configuration_mpt.py/0 | {
"file_path": "transformers/src/transformers/models/mpt/configuration_mpt.py",
"repo_id": "transformers",
"token_count": 4467
} | 346 |
# Copyright 2022 The HuggingFace Team. All rights reserved.
#
# Licensed under the Apache License, Version 2.0 (the "License");
# you may not use this file except in compliance with the License.
# You may obtain a copy of the License at
#
# http://www.apache.org/licenses/LICENSE-2.0
#
# Unless required by applicable law or agreed to in writing, software
# distributed under the License is distributed on an "AS IS" BASIS,
# WITHOUT WARRANTIES OR CONDITIONS OF ANY KIND, either express or implied.
# See the License for the specific language governing permissions and
# limitations under the License.
from typing import TYPE_CHECKING
from ...utils import OptionalDependencyNotAvailable, _LazyModule, is_tokenizers_available, is_torch_available
_import_structure = {
"configuration_mvp": ["MVP_PRETRAINED_CONFIG_ARCHIVE_MAP", "MvpConfig", "MvpOnnxConfig"],
"tokenization_mvp": ["MvpTokenizer"],
}
try:
if not is_tokenizers_available():
raise OptionalDependencyNotAvailable()
except OptionalDependencyNotAvailable:
pass
else:
_import_structure["tokenization_mvp_fast"] = ["MvpTokenizerFast"]
try:
if not is_torch_available():
raise OptionalDependencyNotAvailable()
except OptionalDependencyNotAvailable:
pass
else:
_import_structure["modeling_mvp"] = [
"MVP_PRETRAINED_MODEL_ARCHIVE_LIST",
"MvpForCausalLM",
"MvpForConditionalGeneration",
"MvpForQuestionAnswering",
"MvpForSequenceClassification",
"MvpModel",
"MvpPreTrainedModel",
]
if TYPE_CHECKING:
from .configuration_mvp import MVP_PRETRAINED_CONFIG_ARCHIVE_MAP, MvpConfig, MvpOnnxConfig
from .tokenization_mvp import MvpTokenizer
try:
if not is_tokenizers_available():
raise OptionalDependencyNotAvailable()
except OptionalDependencyNotAvailable:
pass
else:
from .tokenization_mvp_fast import MvpTokenizerFast
try:
if not is_torch_available():
raise OptionalDependencyNotAvailable()
except OptionalDependencyNotAvailable:
pass
else:
from .modeling_mvp import (
MVP_PRETRAINED_MODEL_ARCHIVE_LIST,
MvpForCausalLM,
MvpForConditionalGeneration,
MvpForQuestionAnswering,
MvpForSequenceClassification,
MvpModel,
MvpPreTrainedModel,
)
else:
import sys
sys.modules[__name__] = _LazyModule(__name__, globals()["__file__"], _import_structure, module_spec=__spec__)
| transformers/src/transformers/models/mvp/__init__.py/0 | {
"file_path": "transformers/src/transformers/models/mvp/__init__.py",
"repo_id": "transformers",
"token_count": 983
} | 347 |
# Copyright 2023 The HuggingFace Inc. team. All rights reserved.
#
# Licensed under the Apache License, Version 2.0 (the "License");
# you may not use this file except in compliance with the License.
# You may obtain a copy of the License at
#
# http://www.apache.org/licenses/LICENSE-2.0
#
# Unless required by applicable law or agreed to in writing, software
# distributed under the License is distributed on an "AS IS" BASIS,
# WITHOUT WARRANTIES OR CONDITIONS OF ANY KIND, either express or implied.
# See the License for the specific language governing permissions and
# limitations under the License.
import argparse
import json
import os
import torch
from torch import nn
from transformers import NllbMoeConfig, NllbMoeModel
from transformers.modeling_utils import dtype_byte_size
from transformers.utils import WEIGHTS_INDEX_NAME, WEIGHTS_NAME
def remove_ignore_keys_(state_dict):
ignore_keys = [
"encoder.version",
"decoder.version",
"model.encoder.version",
"model.decoder.version",
"decoder.output_projection.weight",
"_float_tensor",
"encoder.embed_positions._float_tensor",
"decoder.embed_positions._float_tensor",
]
for k in ignore_keys:
state_dict.pop(k, None)
def make_linear_from_emb(emb):
vocab_size, emb_size = emb.weight.shape
lin_layer = nn.Linear(vocab_size, emb_size, bias=False)
lin_layer.weight.data = emb.weight.data
return lin_layer
def rename_fairseq_keys(state_dict, expert_idx=None):
new_dict = {}
for old_key in state_dict.keys():
key = old_key
if "moe_layer.experts." in key:
if expert_idx is not None:
key = key.replace("moe_layer.experts.0", f"ffn.experts.expert_{expert_idx}")
else:
key = key.replace("moe_layer.experts.", "ffn.experts.expert_")
if "gate" in key:
key = key.replace(".moe_layer.gate.wg", ".ffn.router.classifier")
if "fc2" and "experts" not in key:
key = key.replace(".fc2.", ".ffn.fc2.")
if "fc1" and "experts" not in key:
key = key.replace(".fc1.", ".ffn.fc1.")
if ".encoder_attn." in key:
key = key.replace(".encoder_attn.", ".cross_attention.")
if "encoder_attn_layer_norm" in key:
key = key.replace("encoder_attn_layer_norm", "cross_attention_layer_norm")
if "final_layer_norm" in key:
key = key.replace("final_layer_norm", "ff_layer_norm")
new_dict[key] = state_dict[old_key]
return new_dict
def shard_on_the_fly(switch_checkpoint_path, dump_path, num_experts, dtype, weights_name: str = WEIGHTS_NAME):
sharded_state_dicts = []
total_size = 0
os.makedirs(dump_path, exist_ok=True)
for expert in range(num_experts):
expert_path = switch_checkpoint_path + f"-rank-{expert}.pt"
if os.path.isfile(expert_path):
expert_state = torch.load(expert_path)["model"]
remove_ignore_keys_(expert_state)
expert_state = rename_fairseq_keys(expert_state, expert)
save_path = os.path.join(
dump_path, weights_name.replace(".bin", f"-{len(sharded_state_dicts)+1:05d}-of-???.bin")
)
torch.save(expert_state, save_path)
sharded_state_dicts.append(expert_state.keys())
total_size += sum([value.numel() for key, value in expert_state.items()]) * dtype_byte_size(
expert_state[list(expert_state)[0]].dtype
)
# Add the last block
save_path = os.path.join(dump_path, weights_name.replace(".bin", f"-{len(sharded_state_dicts)+1:05d}-of-???.bin"))
shared_weights = torch.load(switch_checkpoint_path + "-shared.pt")["model"]
remove_ignore_keys_(shared_weights)
shared_weights = rename_fairseq_keys(shared_weights, None)
shared_weights["shared.weight"] = shared_weights["decoder.embed_tokens.weight"]
sharded_state_dicts.append(shared_weights.keys())
# If we only have the shared weights (dummy model/experts saved on the same file)
if len(sharded_state_dicts) == 1:
save_path = os.path.join(dump_path, weights_name)
torch.save(shared_weights, save_path)
return {weights_name: sharded_state_dicts[0]}, None
else:
torch.save(shared_weights, save_path)
# Otherwise, let's build the index
weight_map = {}
for idx, shard in enumerate(sharded_state_dicts):
shard_file = weights_name.replace(".bin", f"-{idx+1:05d}-of-{len(sharded_state_dicts):05d}.bin")
temp_filename = os.path.join(dump_path, weights_name.replace(".bin", f"-{idx+1:05d}-of-???.bin"))
os.rename(temp_filename, os.path.join(dump_path, shard_file))
for key in shard:
weight_map[key] = shard_file
# Add the metadata
metadata = {"total_size": total_size}
index = {"metadata": metadata, "weight_map": weight_map}
with open(os.path.join(dump_path, WEIGHTS_INDEX_NAME), "w", encoding="utf-8") as f:
content = json.dumps(index, indent=2, sort_keys=True) + "\n"
f.write(content)
return metadata, index
if __name__ == "__main__":
parser = argparse.ArgumentParser()
# Required parameters
parser.add_argument(
"--nllb_moe_checkpoint_path",
default="/home/arthur_huggingface_co/fairseq/weights/checkpoints/model_moe_54b/checkpoint_2_300000",
type=str,
required=False,
help="Path to a directory containing a folder per layer. Follows the original Google format.",
)
parser.add_argument("--dtype", default="float32", type=str, required=False, help="dtype of the saved model")
parser.add_argument(
"--pytorch_dump_folder_path",
default="/home/arthur_huggingface_co/fairseq/weights/checkpoints/hf-converted-moe-54b",
type=str,
required=False,
help="Path to the output pytorch model.",
)
args = parser.parse_args()
metadata, index = shard_on_the_fly(
args.nllb_moe_checkpoint_path,
args.pytorch_dump_folder_path,
128,
args.dtype,
)
config = NllbMoeConfig.from_pretrained(
"facebook/nllb-200-3.3B", encoder_sparse_step=4, decoder_sparse_step=4, num_experts=128
)
config.save_pretrained(args.pytorch_dump_folder_path)
model = NllbMoeModel.from_pretrained(args.pytorch_dump_folder_path)
print("Done")
model.save_pretrained(args.pytorch_dump_folder_path)
| transformers/src/transformers/models/nllb_moe/convert_nllb_moe_sharded_original_checkpoint_to_pytorch.py/0 | {
"file_path": "transformers/src/transformers/models/nllb_moe/convert_nllb_moe_sharded_original_checkpoint_to_pytorch.py",
"repo_id": "transformers",
"token_count": 2756
} | 348 |
# coding=utf-8
# Copyright 2022 SHI Labs and The HuggingFace Inc. team.
#
# Licensed under the Apache License, Version 2.0 (the "License");
# you may not use this file except in compliance with the License.
# You may obtain a copy of the License at
#
# http://www.apache.org/licenses/LICENSE-2.0
#
# Unless required by applicable law or agreed to in writing, software
# distributed under the License is distributed on an "AS IS" BASIS,
# WITHOUT WARRANTIES OR CONDITIONS OF ANY KIND, either express or implied.
# See the License for the specific language governing permissions and
# limitations under the License.
"""
Image/Text processor class for OneFormer
"""
from typing import List
from ...processing_utils import ProcessorMixin
from ...utils import is_torch_available
if is_torch_available():
import torch
class OneFormerProcessor(ProcessorMixin):
r"""
Constructs an OneFormer processor which wraps [`OneFormerImageProcessor`] and
[`CLIPTokenizer`]/[`CLIPTokenizerFast`] into a single processor that inherits both the image processor and
tokenizer functionalities.
Args:
image_processor ([`OneFormerImageProcessor`]):
The image processor is a required input.
tokenizer ([`CLIPTokenizer`, `CLIPTokenizerFast`]):
The tokenizer is a required input.
max_seq_len (`int`, *optional*, defaults to 77)):
Sequence length for input text list.
task_seq_len (`int`, *optional*, defaults to 77):
Sequence length for input task token.
"""
attributes = ["image_processor", "tokenizer"]
image_processor_class = "OneFormerImageProcessor"
tokenizer_class = ("CLIPTokenizer", "CLIPTokenizerFast")
def __init__(
self, image_processor=None, tokenizer=None, max_seq_length: int = 77, task_seq_length: int = 77, **kwargs
):
if image_processor is None:
raise ValueError("You need to specify an `image_processor`.")
if tokenizer is None:
raise ValueError("You need to specify a `tokenizer`.")
self.max_seq_length = max_seq_length
self.task_seq_length = task_seq_length
super().__init__(image_processor, tokenizer)
def _preprocess_text(self, text_list=None, max_length=77):
if text_list is None:
raise ValueError("tokens cannot be None.")
tokens = self.tokenizer(text_list, padding="max_length", max_length=max_length, truncation=True)
attention_masks, input_ids = tokens["attention_mask"], tokens["input_ids"]
token_inputs = []
for attn_mask, input_id in zip(attention_masks, input_ids):
token = torch.tensor(attn_mask) * torch.tensor(input_id)
token_inputs.append(token.unsqueeze(0))
token_inputs = torch.cat(token_inputs, dim=0)
return token_inputs
def __call__(self, images=None, task_inputs=None, segmentation_maps=None, **kwargs):
"""
Main method to prepare for the model one or several task input(s) and image(s). This method forwards the
`task_inputs` and `kwargs` arguments to CLIPTokenizer's [`~CLIPTokenizer.__call__`] if `task_inputs` is not
`None` to encode. To prepare the image(s), this method forwards the `images` and `kwargs` arguments to
OneFormerImageProcessor's [`~OneFormerImageProcessor.__call__`] if `images` is not `None`. Please refer to the
doctsring of the above two methods for more information.
Args:
task_inputs (`str`, `List[str]`):
The sequence or batch of task_inputs sequences to be encoded. Each sequence can be a string or a list
of strings of the template "the task is {task}".
images (`PIL.Image.Image`, `np.ndarray`, `torch.Tensor`, `List[PIL.Image.Image]`, `List[np.ndarray]`,
`List[torch.Tensor]`):
The image or batch of images to be prepared. Each image can be a PIL image, NumPy array or PyTorch
tensor. In case of a NumPy array/PyTorch tensor, each image should be of shape (C, H, W), where C is a
number of channels, H and W are image height and width.
segmentation_maps (`ImageInput`, *optional*):
The corresponding semantic segmentation maps with the pixel-wise annotations.
(`bool`, *optional*, defaults to `True`):
Whether or not to pad images up to the largest image in a batch and create a pixel mask.
If left to the default, will return a pixel mask that is:
- 1 for pixels that are real (i.e. **not masked**),
- 0 for pixels that are padding (i.e. **masked**).
Returns:
[`BatchFeature`]: A [`BatchFeature`] with the following fields:
- **task_inputs** -- List of token ids to be fed to a model. Returned when `text` is not `None`.
- **pixel_values** -- Pixel values to be fed to a model. Returned when `images` is not `None`.
"""
if task_inputs is None:
raise ValueError("You have to specify the task_input. Found None.")
elif images is None:
raise ValueError("You have to specify the image. Found None.")
if not all(task in ["semantic", "instance", "panoptic"] for task in task_inputs):
raise ValueError("task_inputs must be semantic, instance, or panoptic.")
encoded_inputs = self.image_processor(images, task_inputs, segmentation_maps, **kwargs)
if isinstance(task_inputs, str):
task_inputs = [task_inputs]
if isinstance(task_inputs, List) and all(isinstance(task_input, str) for task_input in task_inputs):
task_token_inputs = []
for task in task_inputs:
task_input = f"the task is {task}"
task_token_inputs.append(task_input)
encoded_inputs["task_inputs"] = self._preprocess_text(task_token_inputs, max_length=self.task_seq_length)
else:
raise TypeError("Task Inputs should be a string or a list of strings.")
if hasattr(encoded_inputs, "text_inputs"):
texts_list = encoded_inputs.text_inputs
text_inputs = []
for texts in texts_list:
text_input_list = self._preprocess_text(texts, max_length=self.max_seq_length)
text_inputs.append(text_input_list.unsqueeze(0))
encoded_inputs["text_inputs"] = torch.cat(text_inputs, dim=0)
return encoded_inputs
def encode_inputs(self, images=None, task_inputs=None, segmentation_maps=None, **kwargs):
"""
This method forwards all its arguments to [`OneFormerImageProcessor.encode_inputs`] and then tokenizes the
task_inputs. Please refer to the docstring of this method for more information.
"""
if task_inputs is None:
raise ValueError("You have to specify the task_input. Found None.")
elif images is None:
raise ValueError("You have to specify the image. Found None.")
if not all(task in ["semantic", "instance", "panoptic"] for task in task_inputs):
raise ValueError("task_inputs must be semantic, instance, or panoptic.")
encoded_inputs = self.image_processor.encode_inputs(images, task_inputs, segmentation_maps, **kwargs)
if isinstance(task_inputs, str):
task_inputs = [task_inputs]
if isinstance(task_inputs, List) and all(isinstance(task_input, str) for task_input in task_inputs):
task_token_inputs = []
for task in task_inputs:
task_input = f"the task is {task}"
task_token_inputs.append(task_input)
encoded_inputs["task_inputs"] = self._preprocess_text(task_token_inputs, max_length=self.task_seq_length)
else:
raise TypeError("Task Inputs should be a string or a list of strings.")
if hasattr(encoded_inputs, "text_inputs"):
texts_list = encoded_inputs.text_inputs
text_inputs = []
for texts in texts_list:
text_input_list = self._preprocess_text(texts, max_length=self.max_seq_length)
text_inputs.append(text_input_list.unsqueeze(0))
encoded_inputs["text_inputs"] = torch.cat(text_inputs, dim=0)
return encoded_inputs
def post_process_semantic_segmentation(self, *args, **kwargs):
"""
This method forwards all its arguments to [`OneFormerImageProcessor.post_process_semantic_segmentation`].
Please refer to the docstring of this method for more information.
"""
return self.image_processor.post_process_semantic_segmentation(*args, **kwargs)
def post_process_instance_segmentation(self, *args, **kwargs):
"""
This method forwards all its arguments to [`OneFormerImageProcessor.post_process_instance_segmentation`].
Please refer to the docstring of this method for more information.
"""
return self.image_processor.post_process_instance_segmentation(*args, **kwargs)
def post_process_panoptic_segmentation(self, *args, **kwargs):
"""
This method forwards all its arguments to [`OneFormerImageProcessor.post_process_panoptic_segmentation`].
Please refer to the docstring of this method for more information.
"""
return self.image_processor.post_process_panoptic_segmentation(*args, **kwargs)
| transformers/src/transformers/models/oneformer/processing_oneformer.py/0 | {
"file_path": "transformers/src/transformers/models/oneformer/processing_oneformer.py",
"repo_id": "transformers",
"token_count": 3741
} | 349 |
# coding=utf-8
# Copyright 2023 The HuggingFace Inc. team.
#
# Licensed under the Apache License, Version 2.0 (the "License");
# you may not use this file except in compliance with the License.
# You may obtain a copy of the License at
#
# http://www.apache.org/licenses/LICENSE-2.0
#
# Unless required by applicable law or agreed to in writing, software
# distributed under the License is distributed on an "AS IS" BASIS,
# WITHOUT WARRANTIES OR CONDITIONS OF ANY KIND, either express or implied.
# See the License for the specific language governing permissions and
# limitations under the License.
"""Convert OWLv2 checkpoints from the original repository.
URL: https://github.com/google-research/scenic/tree/main/scenic/projects/owl_vit"""
import argparse
import collections
import os
import jax
import jax.numpy as jnp
import numpy as np
import torch
from flax.training import checkpoints
from huggingface_hub import hf_hub_download
from PIL import Image
from transformers import (
CLIPTokenizer,
Owlv2Config,
Owlv2ForObjectDetection,
Owlv2ImageProcessor,
Owlv2Processor,
Owlv2TextConfig,
Owlv2VisionConfig,
)
from transformers.utils import logging
logging.set_verbosity_info()
logger = logging.get_logger(__name__)
def get_owlv2_config(model_name):
if "large" in model_name:
image_size = 1008
patch_size = 14
vision_hidden_size = 1024
vision_intermediate_size = 4096
vision_num_hidden_layers = 24
vision_num_attention_heads = 16
projection_dim = 768
text_hidden_size = 768
text_intermediate_size = 3072
text_num_attention_heads = 12
text_num_hidden_layers = 12
else:
image_size = 960
patch_size = 16
vision_hidden_size = 768
vision_intermediate_size = 3072
vision_num_hidden_layers = 12
vision_num_attention_heads = 12
projection_dim = 512
text_hidden_size = 512
text_intermediate_size = 2048
text_num_attention_heads = 8
text_num_hidden_layers = 12
vision_config = Owlv2VisionConfig(
patch_size=patch_size,
image_size=image_size,
hidden_size=vision_hidden_size,
num_hidden_layers=vision_num_hidden_layers,
intermediate_size=vision_intermediate_size,
num_attention_heads=vision_num_attention_heads,
)
text_config = Owlv2TextConfig(
hidden_size=text_hidden_size,
intermediate_size=text_intermediate_size,
num_attention_heads=text_num_attention_heads,
num_hidden_layers=text_num_hidden_layers,
)
config = Owlv2Config(
text_config=text_config.to_dict(),
vision_config=vision_config.to_dict(),
projection_dim=projection_dim,
)
return config
def flatten_nested_dict(params, parent_key="", sep="/"):
items = []
for k, v in params.items():
new_key = parent_key + sep + k if parent_key else k
if isinstance(v, collections.MutableMapping):
items.extend(flatten_nested_dict(v, new_key, sep=sep).items())
else:
items.append((new_key, v))
return dict(items)
# here we list all keys to be renamed (original name on the left, our name on the right)
def create_rename_keys(config, model_name):
rename_keys = []
# fmt: off
# CLIP vision encoder
rename_keys.append(("backbone/clip/visual/class_embedding", "owlv2.vision_model.embeddings.class_embedding"))
rename_keys.append(("backbone/clip/visual/conv1/kernel", "owlv2.vision_model.embeddings.patch_embedding.weight"))
rename_keys.append(("backbone/clip/visual/positional_embedding", "owlv2.vision_model.embeddings.position_embedding.weight"))
rename_keys.append(("backbone/clip/visual/ln_pre/scale", "owlv2.vision_model.pre_layernorm.weight"))
rename_keys.append(("backbone/clip/visual/ln_pre/bias", "owlv2.vision_model.pre_layernorm.bias"))
for i in range(config.vision_config.num_hidden_layers):
if "v2" in model_name:
rename_keys.append((f"backbone/clip/visual/transformer/resblocks.{i}/ln_0/scale", f"owlv2.vision_model.encoder.layers.{i}.layer_norm1.weight"))
rename_keys.append((f"backbone/clip/visual/transformer/resblocks.{i}/ln_0/bias", f"owlv2.vision_model.encoder.layers.{i}.layer_norm1.bias"))
rename_keys.append((f"backbone/clip/visual/transformer/resblocks.{i}/ln_1/scale", f"owlv2.vision_model.encoder.layers.{i}.layer_norm2.weight"))
rename_keys.append((f"backbone/clip/visual/transformer/resblocks.{i}/ln_1/bias", f"owlv2.vision_model.encoder.layers.{i}.layer_norm2.bias"))
else:
rename_keys.append((f"backbone/clip/visual/transformer/resblocks.{i}/ln_1/scale", f"owlv2.vision_model.encoder.layers.{i}.layer_norm1.weight"))
rename_keys.append((f"backbone/clip/visual/transformer/resblocks.{i}/ln_1/bias", f"owlv2.vision_model.encoder.layers.{i}.layer_norm1.bias"))
rename_keys.append((f"backbone/clip/visual/transformer/resblocks.{i}/ln_2/scale", f"owlv2.vision_model.encoder.layers.{i}.layer_norm2.weight"))
rename_keys.append((f"backbone/clip/visual/transformer/resblocks.{i}/ln_2/bias", f"owlv2.vision_model.encoder.layers.{i}.layer_norm2.bias"))
rename_keys.append((f"backbone/clip/visual/transformer/resblocks.{i}/mlp/c_fc/kernel", f"owlv2.vision_model.encoder.layers.{i}.mlp.fc1.weight"))
rename_keys.append((f"backbone/clip/visual/transformer/resblocks.{i}/mlp/c_fc/bias", f"owlv2.vision_model.encoder.layers.{i}.mlp.fc1.bias"))
rename_keys.append((f"backbone/clip/visual/transformer/resblocks.{i}/mlp/c_proj/kernel", f"owlv2.vision_model.encoder.layers.{i}.mlp.fc2.weight"))
rename_keys.append((f"backbone/clip/visual/transformer/resblocks.{i}/mlp/c_proj/bias", f"owlv2.vision_model.encoder.layers.{i}.mlp.fc2.bias"))
rename_keys.append((f"backbone/clip/visual/transformer/resblocks.{i}/attn/query/kernel", f"owlv2.vision_model.encoder.layers.{i}.self_attn.q_proj.weight"))
rename_keys.append((f"backbone/clip/visual/transformer/resblocks.{i}/attn/query/bias", f"owlv2.vision_model.encoder.layers.{i}.self_attn.q_proj.bias"))
rename_keys.append((f"backbone/clip/visual/transformer/resblocks.{i}/attn/key/kernel", f"owlv2.vision_model.encoder.layers.{i}.self_attn.k_proj.weight"))
rename_keys.append((f"backbone/clip/visual/transformer/resblocks.{i}/attn/key/bias", f"owlv2.vision_model.encoder.layers.{i}.self_attn.k_proj.bias"))
rename_keys.append((f"backbone/clip/visual/transformer/resblocks.{i}/attn/value/kernel", f"owlv2.vision_model.encoder.layers.{i}.self_attn.v_proj.weight"))
rename_keys.append((f"backbone/clip/visual/transformer/resblocks.{i}/attn/value/bias", f"owlv2.vision_model.encoder.layers.{i}.self_attn.v_proj.bias"))
rename_keys.append((f"backbone/clip/visual/transformer/resblocks.{i}/attn/out/kernel", f"owlv2.vision_model.encoder.layers.{i}.self_attn.out_proj.weight"))
rename_keys.append((f"backbone/clip/visual/transformer/resblocks.{i}/attn/out/bias", f"owlv2.vision_model.encoder.layers.{i}.self_attn.out_proj.bias"))
rename_keys.append(("backbone/clip/visual/ln_post/scale", "owlv2.vision_model.post_layernorm.weight"))
rename_keys.append(("backbone/clip/visual/ln_post/bias", "owlv2.vision_model.post_layernorm.bias"))
# CLIP text encoder
rename_keys.append(("backbone/clip/text/token_embedding/embedding", "owlv2.text_model.embeddings.token_embedding.weight"))
rename_keys.append(("backbone/clip/text/positional_embedding", "owlv2.text_model.embeddings.position_embedding.weight"))
for i in range(config.text_config.num_hidden_layers):
if "v2" in model_name:
rename_keys.append((f"backbone/clip/text/transformer/resblocks.{i}/ln_0/scale", f"owlv2.text_model.encoder.layers.{i}.layer_norm1.weight"))
rename_keys.append((f"backbone/clip/text/transformer/resblocks.{i}/ln_0/bias", f"owlv2.text_model.encoder.layers.{i}.layer_norm1.bias"))
rename_keys.append((f"backbone/clip/text/transformer/resblocks.{i}/ln_1/scale", f"owlv2.text_model.encoder.layers.{i}.layer_norm2.weight"))
rename_keys.append((f"backbone/clip/text/transformer/resblocks.{i}/ln_1/bias", f"owlv2.text_model.encoder.layers.{i}.layer_norm2.bias"))
else:
rename_keys.append((f"backbone/clip/text/transformer/resblocks.{i}/ln_1/scale", f"owlv2.text_model.encoder.layers.{i}.layer_norm1.weight"))
rename_keys.append((f"backbone/clip/text/transformer/resblocks.{i}/ln_1/bias", f"owlv2.text_model.encoder.layers.{i}.layer_norm1.bias"))
rename_keys.append((f"backbone/clip/text/transformer/resblocks.{i}/ln_2/scale", f"owlv2.text_model.encoder.layers.{i}.layer_norm2.weight"))
rename_keys.append((f"backbone/clip/text/transformer/resblocks.{i}/ln_2/bias", f"owlv2.text_model.encoder.layers.{i}.layer_norm2.bias"))
rename_keys.append((f"backbone/clip/text/transformer/resblocks.{i}/mlp/c_fc/kernel", f"owlv2.text_model.encoder.layers.{i}.mlp.fc1.weight"))
rename_keys.append((f"backbone/clip/text/transformer/resblocks.{i}/mlp/c_fc/bias", f"owlv2.text_model.encoder.layers.{i}.mlp.fc1.bias"))
rename_keys.append((f"backbone/clip/text/transformer/resblocks.{i}/mlp/c_proj/kernel", f"owlv2.text_model.encoder.layers.{i}.mlp.fc2.weight"))
rename_keys.append((f"backbone/clip/text/transformer/resblocks.{i}/mlp/c_proj/bias", f"owlv2.text_model.encoder.layers.{i}.mlp.fc2.bias"))
rename_keys.append((f"backbone/clip/text/transformer/resblocks.{i}/attn/query/kernel", f"owlv2.text_model.encoder.layers.{i}.self_attn.q_proj.weight"))
rename_keys.append((f"backbone/clip/text/transformer/resblocks.{i}/attn/query/bias", f"owlv2.text_model.encoder.layers.{i}.self_attn.q_proj.bias"))
rename_keys.append((f"backbone/clip/text/transformer/resblocks.{i}/attn/key/kernel", f"owlv2.text_model.encoder.layers.{i}.self_attn.k_proj.weight"))
rename_keys.append((f"backbone/clip/text/transformer/resblocks.{i}/attn/key/bias", f"owlv2.text_model.encoder.layers.{i}.self_attn.k_proj.bias"))
rename_keys.append((f"backbone/clip/text/transformer/resblocks.{i}/attn/value/kernel", f"owlv2.text_model.encoder.layers.{i}.self_attn.v_proj.weight"))
rename_keys.append((f"backbone/clip/text/transformer/resblocks.{i}/attn/value/bias", f"owlv2.text_model.encoder.layers.{i}.self_attn.v_proj.bias"))
rename_keys.append((f"backbone/clip/text/transformer/resblocks.{i}/attn/out/kernel", f"owlv2.text_model.encoder.layers.{i}.self_attn.out_proj.weight"))
rename_keys.append((f"backbone/clip/text/transformer/resblocks.{i}/attn/out/bias", f"owlv2.text_model.encoder.layers.{i}.self_attn.out_proj.bias"))
rename_keys.append(("backbone/clip/text/ln_final/scale", "owlv2.text_model.final_layer_norm.weight"))
rename_keys.append(("backbone/clip/text/ln_final/bias", "owlv2.text_model.final_layer_norm.bias"))
# logit scale
rename_keys.append(("backbone/clip/logit_scale", "owlv2.logit_scale"))
# projection heads
rename_keys.append(("backbone/clip/text/text_projection/kernel", "owlv2.text_projection.weight"))
# class and box heads
rename_keys.append(("backbone/merged_class_token/scale", "layer_norm.weight"))
rename_keys.append(("backbone/merged_class_token/bias", "layer_norm.bias"))
rename_keys.append(("class_head/Dense_0/kernel", "class_head.dense0.weight"))
rename_keys.append(("class_head/Dense_0/bias", "class_head.dense0.bias"))
rename_keys.append(("class_head/logit_shift/kernel", "class_head.logit_shift.weight"))
rename_keys.append(("class_head/logit_scale/kernel", "class_head.logit_scale.weight"))
rename_keys.append(("class_head/logit_scale/bias", "class_head.logit_scale.bias"))
rename_keys.append(("class_head/logit_shift/bias", "class_head.logit_shift.bias"))
rename_keys.append(("obj_box_head/Dense_0/kernel", "box_head.dense0.weight"))
rename_keys.append(("obj_box_head/Dense_0/bias", "box_head.dense0.bias"))
rename_keys.append(("obj_box_head/Dense_1/kernel", "box_head.dense1.weight"))
rename_keys.append(("obj_box_head/Dense_1/bias", "box_head.dense1.bias"))
rename_keys.append(("obj_box_head/Dense_2/kernel", "box_head.dense2.weight"))
rename_keys.append(("obj_box_head/Dense_2/bias", "box_head.dense2.bias"))
# objectness head (only for v2)
if "v2" in model_name:
rename_keys.append(("objectness_head/Dense_0/kernel", "objectness_head.dense0.weight"))
rename_keys.append(("objectness_head/Dense_0/bias", "objectness_head.dense0.bias"))
rename_keys.append(("objectness_head/Dense_1/kernel", "objectness_head.dense1.weight"))
rename_keys.append(("objectness_head/Dense_1/bias", "objectness_head.dense1.bias"))
rename_keys.append(("objectness_head/Dense_2/kernel", "objectness_head.dense2.weight"))
rename_keys.append(("objectness_head/Dense_2/bias", "objectness_head.dense2.bias"))
# fmt: on
return rename_keys
def rename_and_reshape_key(dct, old, new, config):
val = dct.pop(old)
if ("out_proj" in new or "v_proj" in new or "k_proj" in new or "q_proj" in new) and "vision" in new:
val = val.reshape(-1, config.vision_config.hidden_size)
if ("out_proj" in new or "v_proj" in new or "k_proj" in new or "q_proj" in new) and "text" in new:
val = val.reshape(-1, config.text_config.hidden_size)
if "patch_embedding" in new:
print("Reshaping patch embedding... for", new)
val = val.transpose(3, 2, 0, 1)
elif new.endswith("weight") and "position_embedding" not in new and "token_embedding" not in new:
val = val.T
if new.endswith("bias"):
val = val.reshape(-1)
dct[new] = torch.from_numpy(np.array(val))
@torch.no_grad()
def convert_owlv2_checkpoint(model_name, checkpoint_path, pytorch_dump_folder_path, push_to_hub, verify_logits):
"""
Copy/paste/tweak model's weights to our OWL-ViT structure.
"""
config = get_owlv2_config(model_name)
# see available checkpoints at https://github.com/google-research/scenic/tree/main/scenic/projects/owl_vit#pretrained-checkpoints
variables = checkpoints.restore_checkpoint(checkpoint_path, target=None)
variables = variables["params"] if "v2" in model_name else variables["optimizer"]["target"]
flax_params = jax.tree_util.tree_map(lambda x: x.astype(jnp.float32) if x.dtype == jnp.bfloat16 else x, variables)
state_dict = flatten_nested_dict(flax_params)
# Rename keys
rename_keys = create_rename_keys(config, model_name)
for src, dest in rename_keys:
rename_and_reshape_key(state_dict, src, dest, config)
# load HuggingFace model
model = Owlv2ForObjectDetection(config)
missing_keys, unexpected_keys = model.load_state_dict(state_dict, strict=False)
assert missing_keys == ["owlv2.visual_projection.weight"]
assert unexpected_keys == []
model.eval()
# Initialize image processor
size = {"height": config.vision_config.image_size, "width": config.vision_config.image_size}
image_processor = Owlv2ImageProcessor(size=size)
# Initialize tokenizer
tokenizer = CLIPTokenizer.from_pretrained("openai/clip-vit-base-patch32", pad_token="!", model_max_length=16)
# Initialize processor
processor = Owlv2Processor(image_processor=image_processor, tokenizer=tokenizer)
# Verify pixel_values and input_ids
filepath = hf_hub_download(repo_id="nielsr/test-image", filename="owlvit_pixel_values_960.pt", repo_type="dataset")
original_pixel_values = torch.load(filepath).permute(0, 3, 1, 2)
filepath = hf_hub_download(repo_id="nielsr/test-image", filename="owlv2_input_ids.pt", repo_type="dataset")
original_input_ids = torch.load(filepath).squeeze()
filepath = hf_hub_download(repo_id="adirik/OWL-ViT", repo_type="space", filename="assets/astronaut.png")
image = Image.open(filepath)
texts = [["face", "rocket", "nasa badge", "star-spangled banner"]]
inputs = processor(text=texts, images=image, return_tensors="pt")
if "large" not in model_name:
assert torch.allclose(inputs.pixel_values, original_pixel_values.float(), atol=1e-6)
assert torch.allclose(inputs.input_ids[:4, :], original_input_ids[:4, :], atol=1e-6)
with torch.no_grad():
outputs = model(**inputs)
logits = outputs.logits
pred_boxes = outputs.pred_boxes
objectness_logits = outputs.objectness_logits
if verify_logits:
if model_name == "owlv2-base-patch16":
expected_logits = torch.tensor(
[[-10.0043, -9.0226, -8.0433], [-12.4569, -14.0380, -12.6153], [-21.0731, -22.2705, -21.8850]]
)
expected_boxes = torch.tensor(
[[0.0136, 0.0223, 0.0269], [0.0406, 0.0327, 0.0797], [0.0638, 0.1539, 0.1255]]
)
expected_objectness_logits = torch.tensor(
[[-5.6589, -7.7702, -16.3965]],
)
elif model_name == "owlv2-base-patch16-finetuned":
expected_logits = torch.tensor(
[[-9.2391, -9.2313, -8.0295], [-14.5498, -16.8450, -14.7166], [-15.1278, -17.3060, -15.7169]],
)
expected_boxes = torch.tensor(
[[0.0103, 0.0094, 0.0207], [0.0483, 0.0729, 0.1013], [0.0629, 0.1396, 0.1313]]
)
expected_objectness_logits = torch.tensor(
[[-6.5234, -13.3788, -14.6627]],
)
elif model_name == "owlv2-base-patch16-ensemble":
expected_logits = torch.tensor(
[[-8.6353, -9.5409, -6.6154], [-7.9442, -9.6151, -6.7117], [-12.4593, -15.3332, -12.1048]]
)
expected_boxes = torch.tensor(
[[0.0126, 0.0090, 0.0238], [0.0387, 0.0227, 0.0754], [0.0582, 0.1058, 0.1139]]
)
expected_objectness_logits = torch.tensor(
[[-6.0628, -5.9507, -10.4486]],
)
elif model_name == "owlv2-large-patch14":
expected_logits = torch.tensor(
[[-12.6662, -11.8384, -12.1880], [-16.0599, -16.5835, -16.9364], [-21.4957, -26.7038, -25.1313]],
)
expected_boxes = torch.tensor(
[[0.0136, 0.0161, 0.0256], [0.0126, 0.0135, 0.0202], [0.0498, 0.0948, 0.0915]],
)
expected_objectness_logits = torch.tensor(
[[-6.7196, -9.4590, -13.9472]],
)
elif model_name == "owlv2-large-patch14-finetuned":
expected_logits = torch.tensor(
[[-9.5413, -9.7130, -7.9762], [-9.5731, -9.7277, -8.2252], [-15.4434, -19.3084, -16.5490]],
)
expected_boxes = torch.tensor(
[[0.0089, 0.0080, 0.0175], [0.0112, 0.0098, 0.0179], [0.0375, 0.0821, 0.0528]],
)
expected_objectness_logits = torch.tensor(
[[-6.2655, -6.5845, -11.3105]],
)
elif model_name == "owlv2-large-patch14-ensemble":
expected_logits = torch.tensor(
[[-12.2037, -12.2070, -11.5371], [-13.4875, -13.8235, -13.1586], [-18.2007, -22.9834, -20.6816]],
)
expected_boxes = torch.tensor(
[[0.0126, 0.0127, 0.0222], [0.0107, 0.0113, 0.0164], [0.0482, 0.1162, 0.0885]],
)
expected_objectness_logits = torch.tensor(
[[-7.7572, -8.3637, -13.0334]],
)
print("Objectness logits:", objectness_logits[:3, :3])
print("Logits:", logits[0, :3, :3])
print("Pred boxes:", pred_boxes[0, :3, :3])
assert torch.allclose(logits[0, :3, :3], expected_logits, atol=1e-3)
assert torch.allclose(pred_boxes[0, :3, :3], expected_boxes, atol=1e-3)
assert torch.allclose(objectness_logits[:3, :3], expected_objectness_logits, atol=1e-3)
print("Looks ok!")
else:
print("Model converted without verifying logits")
if pytorch_dump_folder_path is not None:
print("Saving model and processor locally...")
# Create folder to save model
if not os.path.isdir(pytorch_dump_folder_path):
os.mkdir(pytorch_dump_folder_path)
model.save_pretrained(pytorch_dump_folder_path)
processor.save_pretrained(pytorch_dump_folder_path)
if push_to_hub:
print(f"Pushing {model_name} to the hub...")
model.push_to_hub(f"google/{model_name}")
processor.push_to_hub(f"google/{model_name}")
if __name__ == "__main__":
parser = argparse.ArgumentParser()
# Required parameters
parser.add_argument(
"--model_name",
default="owlv2-base-patch16",
choices=[
"owlv2-base-patch16",
"owlv2-base-patch16-finetuned",
"owlv2-base-patch16-ensemble",
"owlv2-large-patch14",
"owlv2-large-patch14-finetuned",
"owlv2-large-patch14-ensemble",
],
type=str,
help="Name of the Owlv2 model you'd like to convert from FLAX to PyTorch.",
)
parser.add_argument(
"--checkpoint_path",
default=None,
type=str,
required=True,
help="Path to the original Flax checkpoint.",
)
parser.add_argument(
"--pytorch_dump_folder_path",
default=None,
type=str,
required=False,
help="Path to the output PyTorch model directory.",
)
parser.add_argument(
"--verify_logits",
action="store_false",
required=False,
help="Path to the output PyTorch model directory.",
)
parser.add_argument("--push_to_hub", action="store_true", help="Push model and image preprocessor to the hub")
args = parser.parse_args()
convert_owlv2_checkpoint(
args.model_name, args.checkpoint_path, args.pytorch_dump_folder_path, args.push_to_hub, args.verify_logits
)
| transformers/src/transformers/models/owlv2/convert_owlv2_to_hf.py/0 | {
"file_path": "transformers/src/transformers/models/owlv2/convert_owlv2_to_hf.py",
"repo_id": "transformers",
"token_count": 9921
} | 350 |
# coding=utf-8
# Copyright 2023 IBM & Hugging Face. All rights reserved.
#
# Licensed under the Apache License, Version 2.0 (the "License");
# you may not use this file except in compliance with the License.
# You may obtain a copy of the License at
#
# http://www.apache.org/licenses/LICENSE-2.0
#
# Unless required by applicable law or agreed to in writing, software
# distributed under the License is distributed on an "AS IS" BASIS,
# WITHOUT WARRANTIES OR CONDITIONS OF ANY KIND, either express or implied.
# See the License for the specific language governing permissions and
# limitations under the License.
""" PyTorch PatchTST model."""
import math
from dataclasses import dataclass
from typing import Optional, Tuple, Union
import torch
from torch import nn
from ...activations import ACT2CLS
from ...modeling_outputs import BaseModelOutput
from ...modeling_utils import PreTrainedModel
from ...time_series_utils import NegativeBinomialOutput, NormalOutput, StudentTOutput
from ...utils import ModelOutput, add_start_docstrings, logging
from .configuration_patchtst import PatchTSTConfig
logger = logging.get_logger(__name__)
_CONFIG_FOR_DOC = "PatchTSTConfig"
PATCHTST_PRETRAINED_MODEL_ARCHIVE_LIST = [
"ibm/patchtst-etth1-pretrain",
# See all PatchTST models at https://huggingface.co/models?filter=patchtst
]
# Copied from transformers.models.bart.modeling_bart.BartAttention with Bart->PatchTST
class PatchTSTAttention(nn.Module):
"""Multi-headed attention from 'Attention Is All You Need' paper"""
def __init__(
self,
embed_dim: int,
num_heads: int,
dropout: float = 0.0,
is_decoder: bool = False,
bias: bool = True,
is_causal: bool = False,
config: Optional[PatchTSTConfig] = None,
):
super().__init__()
self.embed_dim = embed_dim
self.num_heads = num_heads
self.dropout = dropout
self.head_dim = embed_dim // num_heads
self.config = config
if (self.head_dim * num_heads) != self.embed_dim:
raise ValueError(
f"embed_dim must be divisible by num_heads (got `embed_dim`: {self.embed_dim}"
f" and `num_heads`: {num_heads})."
)
self.scaling = self.head_dim**-0.5
self.is_decoder = is_decoder
self.is_causal = is_causal
self.k_proj = nn.Linear(embed_dim, embed_dim, bias=bias)
self.v_proj = nn.Linear(embed_dim, embed_dim, bias=bias)
self.q_proj = nn.Linear(embed_dim, embed_dim, bias=bias)
self.out_proj = nn.Linear(embed_dim, embed_dim, bias=bias)
def _shape(self, tensor: torch.Tensor, seq_len: int, bsz: int):
return tensor.view(bsz, seq_len, self.num_heads, self.head_dim).transpose(1, 2).contiguous()
def forward(
self,
hidden_states: torch.Tensor,
key_value_states: Optional[torch.Tensor] = None,
past_key_value: Optional[Tuple[torch.Tensor]] = None,
attention_mask: Optional[torch.Tensor] = None,
layer_head_mask: Optional[torch.Tensor] = None,
output_attentions: bool = False,
) -> Tuple[torch.Tensor, Optional[torch.Tensor], Optional[Tuple[torch.Tensor]]]:
"""Input shape: Batch x Time x Channel"""
# if key_value_states are provided this layer is used as a cross-attention layer
# for the decoder
is_cross_attention = key_value_states is not None
bsz, tgt_len, _ = hidden_states.size()
# get query proj
query_states = self.q_proj(hidden_states) * self.scaling
# get key, value proj
# `past_key_value[0].shape[2] == key_value_states.shape[1]`
# is checking that the `sequence_length` of the `past_key_value` is the same as
# the provided `key_value_states` to support prefix tuning
if (
is_cross_attention
and past_key_value is not None
and past_key_value[0].shape[2] == key_value_states.shape[1]
):
# reuse k,v, cross_attentions
key_states = past_key_value[0]
value_states = past_key_value[1]
elif is_cross_attention:
# cross_attentions
key_states = self._shape(self.k_proj(key_value_states), -1, bsz)
value_states = self._shape(self.v_proj(key_value_states), -1, bsz)
elif past_key_value is not None:
# reuse k, v, self_attention
key_states = self._shape(self.k_proj(hidden_states), -1, bsz)
value_states = self._shape(self.v_proj(hidden_states), -1, bsz)
key_states = torch.cat([past_key_value[0], key_states], dim=2)
value_states = torch.cat([past_key_value[1], value_states], dim=2)
else:
# self_attention
key_states = self._shape(self.k_proj(hidden_states), -1, bsz)
value_states = self._shape(self.v_proj(hidden_states), -1, bsz)
if self.is_decoder:
# if cross_attention save Tuple(torch.Tensor, torch.Tensor) of all cross attention key/value_states.
# Further calls to cross_attention layer can then reuse all cross-attention
# key/value_states (first "if" case)
# if uni-directional self-attention (decoder) save Tuple(torch.Tensor, torch.Tensor) of
# all previous decoder key/value_states. Further calls to uni-directional self-attention
# can concat previous decoder key/value_states to current projected key/value_states (third "elif" case)
# if encoder bi-directional self-attention `past_key_value` is always `None`
past_key_value = (key_states, value_states)
proj_shape = (bsz * self.num_heads, -1, self.head_dim)
query_states = self._shape(query_states, tgt_len, bsz).view(*proj_shape)
key_states = key_states.reshape(*proj_shape)
value_states = value_states.reshape(*proj_shape)
src_len = key_states.size(1)
attn_weights = torch.bmm(query_states, key_states.transpose(1, 2))
if attn_weights.size() != (bsz * self.num_heads, tgt_len, src_len):
raise ValueError(
f"Attention weights should be of size {(bsz * self.num_heads, tgt_len, src_len)}, but is"
f" {attn_weights.size()}"
)
if attention_mask is not None:
if attention_mask.size() != (bsz, 1, tgt_len, src_len):
raise ValueError(
f"Attention mask should be of size {(bsz, 1, tgt_len, src_len)}, but is {attention_mask.size()}"
)
attn_weights = attn_weights.view(bsz, self.num_heads, tgt_len, src_len) + attention_mask
attn_weights = attn_weights.view(bsz * self.num_heads, tgt_len, src_len)
attn_weights = nn.functional.softmax(attn_weights, dim=-1)
if layer_head_mask is not None:
if layer_head_mask.size() != (self.num_heads,):
raise ValueError(
f"Head mask for a single layer should be of size {(self.num_heads,)}, but is"
f" {layer_head_mask.size()}"
)
attn_weights = layer_head_mask.view(1, -1, 1, 1) * attn_weights.view(bsz, self.num_heads, tgt_len, src_len)
attn_weights = attn_weights.view(bsz * self.num_heads, tgt_len, src_len)
if output_attentions:
# this operation is a bit awkward, but it's required to
# make sure that attn_weights keeps its gradient.
# In order to do so, attn_weights have to be reshaped
# twice and have to be reused in the following
attn_weights_reshaped = attn_weights.view(bsz, self.num_heads, tgt_len, src_len)
attn_weights = attn_weights_reshaped.view(bsz * self.num_heads, tgt_len, src_len)
else:
attn_weights_reshaped = None
attn_probs = nn.functional.dropout(attn_weights, p=self.dropout, training=self.training)
attn_output = torch.bmm(attn_probs, value_states)
if attn_output.size() != (bsz * self.num_heads, tgt_len, self.head_dim):
raise ValueError(
f"`attn_output` should be of size {(bsz * self.num_heads, tgt_len, self.head_dim)}, but is"
f" {attn_output.size()}"
)
attn_output = attn_output.view(bsz, self.num_heads, tgt_len, self.head_dim)
attn_output = attn_output.transpose(1, 2)
# Use the `embed_dim` from the config (stored in the class) rather than `hidden_state` because `attn_output` can be
# partitioned across GPUs when using tensor-parallelism.
attn_output = attn_output.reshape(bsz, tgt_len, self.embed_dim)
attn_output = self.out_proj(attn_output)
return attn_output, attn_weights_reshaped, past_key_value
class PatchTSTBatchNorm(nn.Module):
"""
Compute batch normalization over the sequence length (time) dimension.
"""
def __init__(self, config: PatchTSTConfig):
super().__init__()
self.batchnorm = nn.BatchNorm1d(config.d_model, eps=config.norm_eps)
def forward(self, inputs: torch.Tensor):
"""
Parameters:
inputs (`torch.Tensor` of shape `(batch_size, sequence_length, d_model)`):
input for Batch norm calculation
Returns:
`torch.Tensor` of shape `(batch_size, sequence_length, d_model)`
"""
output = inputs.transpose(1, 2) # output: (batch_size, d_model, sequence_length)
output = self.batchnorm(output)
return output.transpose(1, 2)
def random_masking(
inputs: torch.Tensor,
mask_ratio: float,
unmasked_channel_indices: list = None,
channel_consistent_masking: bool = False,
mask_value: int = 0,
):
"""random_masking: Mask the input considering the control variables.
Args:
inputs (`torch.Tensor` of shape `(batch_size, num_channels, sequence_length, num_features)`):
The input tensor to mask.
mask_ratio (`float`):
Masking ratio applied to mask the input data during random pretraining. It is the number between 0 and 1.
unmasked_channel_indices (list, *optional*):
Indices of channels that will not be masked.
channel_consistent_masking (bool, *optional*, defaults to `False`):
When true, masking will be same across all channels of a timeseries. Otherwise, masking positions will vary
across channels.
mask_value (int, *optional*, defaults to 0):
Define the value of masked patches for pretraining.
Returns:
`tuple(torch.Tensor)`: inputs_mask, masked input, same shape as input Tensor and mask tensor of shape [bs x c x
n]
"""
if mask_ratio < 0 or mask_ratio >= 1:
raise ValueError(f"Mask ratio {mask_ratio} has to be between 0 and 1.")
batch_size, num_channels, sequence_length, num_features = inputs.shape
device = inputs.device
len_keep = int(sequence_length * (1 - mask_ratio))
if channel_consistent_masking:
noise = torch.rand(batch_size, 1, sequence_length, device=device) # noise in [0, 1], bs x 1 x L
noise = noise.repeat(1, num_channels, 1) # bs x num_channels x time
else:
# noise in [0, 1], bs x num_channels x L
noise = torch.rand(batch_size, num_channels, sequence_length, device=device)
# mask: [bs x num_channels x num_patch]
mask = torch.ones(batch_size, num_channels, sequence_length, device=device)
mask[:, :, :len_keep] = 0
# sort noise for each sample
ids_shuffle = torch.argsort(noise, dim=-1) # ascend: small is keep, large is remove
ids_restore = torch.argsort(ids_shuffle, dim=-1) # ids_restore: [bs x num_channels x L]
mask = torch.gather(mask, dim=-1, index=ids_restore)
mask = mask.unsqueeze(-1).repeat(1, 1, 1, num_features) # mask: [bs x num_channels x num_patches x patch_length]
if unmasked_channel_indices is not None:
mask[:, unmasked_channel_indices, :, :] = 0
inputs_mask = inputs.masked_fill(mask.bool(), mask_value)
return inputs_mask, mask[..., 0]
def forecast_masking(
inputs: torch.Tensor,
num_forecast_mask_patches: Union[list, int],
unmasked_channel_indices: list = None,
mask_value: int = 0,
):
"""Forecast masking that masks the last K patches where K is from the num_forecast_mask_patches.
If num_forecast_mask_patches is a list, samples in the batch will be randomly masked by numbers defined in the list.
Parameters:
inputs (`torch.Tensor`):
Input of shape `(bs, num_channels, num_patch, patch_length)`
num_forecast_mask_patches (`list`):
Number of patches to be masked at the end of each batch sample. e.g. 4 or [3, 5].
unmasked_channel_indices (`list`, *optional*):
Indices of channels that are not masked.
mask_value (`int`, *optional*, defaults to 0):
Values in the masked patches will be filled by `mask_value`.
Returns:
`tuple(torch.Tensor)`: inputs_mask, masked input, same shape as inputs Tensor and Mask tensor of shape `(bs,
num_channels , num_patch)` or `(bs, tsg1, tsg2, num_channels, num_patch)`
"""
if isinstance(num_forecast_mask_patches, int):
num_forecast_mask_patches = [num_forecast_mask_patches]
forecast_mask_ratios = [1 for _ in num_forecast_mask_patches]
batch_size, num_channels, sequence_length, num_features = inputs.shape
mask = torch.zeros(batch_size, num_channels, sequence_length, device=inputs.device)
t_list = []
total_length = 0
total_ratio = sum(forecast_mask_ratios)
for patch_length, ratio in zip(num_forecast_mask_patches, forecast_mask_ratios):
if patch_length <= 0 or patch_length >= sequence_length:
raise ValueError(
f"num_forecast_mask_patches {patch_length} should be greater than 0 and less than total patches."
)
temp_len = int(batch_size * ratio / total_ratio)
t_list.append([patch_length, ratio, temp_len])
total_length += temp_len
t_list = sorted(t_list, key=lambda x: x[2])
if total_length < batch_size:
t_list[0][2] = t_list[0][2] + (batch_size - total_length)
elif total_length > batch_size:
t_list[-1][2] = t_list[-1][2] + (total_length - batch_size)
batch1 = 0
for patch_len, _, temp_len in t_list:
batch2 = batch1 + temp_len
mask[batch1:batch2, :, -patch_len:] = 1
batch1 = batch2
perm = torch.randperm(mask.shape[0])
mask = mask[perm]
mask = mask.unsqueeze(-1).repeat(1, 1, 1, num_features) # mask: [bs x num_channels x num_patch x patch_len]
if unmasked_channel_indices is not None:
mask[:, unmasked_channel_indices, :, :] = 0
inputs_mask = inputs.masked_fill(mask.bool(), mask_value)
return inputs_mask, mask[..., 0]
class PatchTSTPatchify(nn.Module):
"""
A class to patchify the time series sequence into different patches
Returns:
`torch.Tensor` of shape `(batch_size, num_channels, num_patches, patch_length)`
"""
def __init__(self, config: PatchTSTConfig):
super().__init__()
self.sequence_length = config.context_length
self.patch_length = config.patch_length
self.patch_stride = config.patch_stride
if self.sequence_length <= self.patch_length:
raise ValueError(
f"Sequence length ({self.sequence_length}) has to be greater than the patch length ({self.patch_length})"
)
# get the number of patches
self.num_patches = (max(self.sequence_length, self.patch_length) - self.patch_length) // self.patch_stride + 1
new_sequence_length = self.patch_length + self.patch_stride * (self.num_patches - 1)
self.sequence_start = self.sequence_length - new_sequence_length
def forward(self, past_values: torch.Tensor):
"""
Parameters:
past_values (`torch.Tensor` of shape `(batch_size, sequence_length, num_channels)`, *required*):
Input for patchification
Returns:
`torch.Tensor` of shape `(batch_size, num_channels, num_patches, patch_length)`
"""
sequence_length = past_values.shape[-2]
if sequence_length != self.sequence_length:
raise ValueError(
f"Input sequence length ({sequence_length}) doesn't match model configuration ({self.sequence_length})."
)
# output: [bs x new_sequence_length x num_channels]
output = past_values[:, self.sequence_start :, :]
# output: [bs x num_patches x num_input_channels x patch_length]
output = output.unfold(dimension=-2, size=self.patch_length, step=self.patch_stride)
# output: [bs x num_input_channels x num_patches x patch_length]
output = output.transpose(-2, -3).contiguous()
return output
class PatchTSTMasking(nn.Module):
"""
Class to perform random or forecast masking.
Parameters:
config (`PatchTSTConfig`): model config
Returns:
x_mask (`torch.Tensor` of shape `(batch_size, num_channels, num_patches, patch_length)`)
Masked patched input
mask (`torch.Tensor` of shape `(batch_size, num_channels, num_patches)`)
Bool tensor indicating True on masked points
"""
def __init__(self, config: PatchTSTConfig):
super().__init__()
self.random_mask_ratio = config.random_mask_ratio
self.channel_consistent_masking = config.channel_consistent_masking
self.mask_type = config.mask_type
self.num_forecast_mask_patches = config.num_forecast_mask_patches
self.unmasked_channel_indices = config.unmasked_channel_indices
self.mask_value = config.mask_value
if self.unmasked_channel_indices is not None:
self.unmasked_channel_indices = sorted(self.unmasked_channel_indices)
def forward(self, patch_input: torch.Tensor):
"""
Parameters:
patch_input (`torch.Tensor` of shape `(batch_size, num_channels, num_patches, patch_length)`, *required*):
Patch input
Return:
masked_input (`torch.Tensor` of shape `(batch_size, num_channels, num_patches, patch_length)`)
Masked patched input
mask (`torch.Tensor` of shape `(batch_size, num_channels, num_patches)`)
Bool tensor indicating True on masked points
"""
if self.mask_type == "random":
masked_input, mask = random_masking(
inputs=patch_input,
mask_ratio=self.random_mask_ratio,
unmasked_channel_indices=self.unmasked_channel_indices,
channel_consistent_masking=self.channel_consistent_masking,
mask_value=self.mask_value,
)
elif self.mask_type == "forecast":
masked_input, mask = forecast_masking(
inputs=patch_input,
num_forecast_mask_patches=self.num_forecast_mask_patches,
unmasked_channel_indices=self.unmasked_channel_indices,
mask_value=self.mask_value,
)
else:
raise ValueError(f"Invalid mask type {self.mask_type}.")
# mask: [bs x num_input_channels x num_patch]
mask = mask.bool()
return masked_input, mask
class PatchTSTEncoderLayer(nn.Module):
"""
PatchTST encoder layer
"""
def __init__(self, config: PatchTSTConfig):
super().__init__()
self.channel_attention = config.channel_attention
# Multi-Head attention
self.self_attn = PatchTSTAttention(
embed_dim=config.d_model,
num_heads=config.num_attention_heads,
dropout=config.attention_dropout,
)
# Add & Norm of the sublayer 1
self.dropout_path1 = nn.Dropout(config.path_dropout) if config.path_dropout > 0 else nn.Identity()
if config.norm_type == "batchnorm":
self.norm_sublayer1 = PatchTSTBatchNorm(config)
elif config.norm_type == "layernorm":
self.norm_sublayer1 = nn.LayerNorm(config.d_model, eps=config.norm_eps)
else:
raise ValueError(f"{config.norm_type} is not a supported norm layer type.")
# Add & Norm of the sublayer 2
if self.channel_attention:
self.dropout_path2 = nn.Dropout(config.path_dropout) if config.path_dropout > 0 else nn.Identity()
if config.norm_type == "batchnorm":
self.norm_sublayer2 = PatchTSTBatchNorm(config)
elif config.norm_type == "layernorm":
self.norm_sublayer2 = nn.LayerNorm(config.d_model, eps=config.norm_eps)
else:
raise ValueError(f"{config.norm_type} is not a supported norm layer type.")
# Position-wise Feed-Forward
self.ff = nn.Sequential(
nn.Linear(config.d_model, config.ffn_dim, bias=config.bias),
ACT2CLS[config.activation_function](),
nn.Dropout(config.ff_dropout) if config.ff_dropout > 0 else nn.Identity(),
nn.Linear(config.ffn_dim, config.d_model, bias=config.bias),
)
# Add & Norm of sublayer 3
self.dropout_path3 = nn.Dropout(config.path_dropout) if config.path_dropout > 0 else nn.Identity()
if config.norm_type == "batchnorm":
self.norm_sublayer3 = PatchTSTBatchNorm(config)
elif config.norm_type == "layernorm":
self.norm_sublayer3 = nn.LayerNorm(config.d_model, eps=config.norm_eps)
else:
raise ValueError(f"{config.norm_type} is not a supported norm layer type.")
self.pre_norm = config.pre_norm
def forward(self, hidden_state: torch.Tensor, output_attentions: Optional[bool] = None):
"""
Parameters:
hidden_state (`torch.Tensor` of shape `(batch_size, num_channels, sequence_length, d_model)`, *required*):
Past values of the time series
output_attentions (`bool`, *optional*):
Whether or not to return the output attention of all layers
Return:
`torch.Tensor` of shape `(batch_size, num_channels, sequence_length, d_model)`
"""
batch_size, num_input_channels, sequence_length, d_model = hidden_state.shape
# First sublayer: attention across time
# hidden_states: [(bs*num_channels) x sequence_length x d_model]
hidden_state = hidden_state.view(batch_size * num_input_channels, sequence_length, d_model)
if self.pre_norm:
## Norm and Multi-Head attention and Add residual connection
attn_output, attn_weights, _ = self.self_attn(
hidden_states=self.norm_sublayer1(hidden_state), output_attentions=output_attentions
)
# Add: residual connection with residual dropout
hidden_state = hidden_state + self.dropout_path1(attn_output)
else:
## Multi-Head attention and Add residual connection and Norm - Standard Transformer from BERT
attn_output, attn_weights, _ = self.self_attn(
hidden_states=hidden_state, output_attentions=output_attentions
)
# hidden_states: [(bs*num_channels) x sequence_length x d_model]
hidden_state = self.norm_sublayer1(hidden_state + self.dropout_path1(attn_output))
# hidden_state: [bs x num_channels x sequence_length x d_model]
hidden_state = hidden_state.reshape(batch_size, num_input_channels, sequence_length, d_model)
# second sublayer: attention across variable at any given time
if self.channel_attention:
# hidden_state: [bs x sequence_length x num_channels x d_model]
hidden_state = hidden_state.transpose(2, 1).contiguous()
# hidden_state: [(bs*sequence_length) x num_channels x d_model]
hidden_state = hidden_state.view(batch_size * sequence_length, num_input_channels, d_model)
if self.pre_norm:
## Norm and Multi-Head attention and Add residual connection
attn_output, channel_attn_weights, _ = self.self_attn(
hidden_states=self.norm_sublayer2(hidden_state), output_attentions=output_attentions
)
# Add: residual connection with residual dropout
hidden_state = hidden_state + self.dropout_path2(attn_output)
else:
## Multi-Head attention and Add residual connection and Norm
attn_output, channel_attn_weights, _ = self.self_attn(
hidden_states=hidden_state, output_attentions=output_attentions
)
# hidden_states: [(bs*sequence_length) x num_channels x d_model]
hidden_state = self.norm_sublayer2(hidden_state + self.dropout_path2(attn_output))
# Reshape hidden state
# hidden_state: [bs x sequence_length x num_channels x d_model]
hidden_state = hidden_state.reshape(batch_size, sequence_length, num_input_channels, d_model)
# hidden_state: [bs x num_channels x sequence_length x d_model]
hidden_state = hidden_state.transpose(1, 2).contiguous()
# Third sublayer: mixing across hidden
# hidden_state: [(batch_size*num_channels) x sequence_length x d_model]
hidden_state = hidden_state.view(batch_size * num_input_channels, sequence_length, d_model)
if self.pre_norm:
## Norm and Position-wise Feed-Forward and Add residual connection
# Add: residual connection with residual dropout
hidden_state = hidden_state + self.dropout_path3(self.ff(self.norm_sublayer3(hidden_state)))
else:
## Position-wise Feed-Forward and Add residual connection and Norm
# Add: residual connection with residual dropout
hidden_state = self.norm_sublayer3(hidden_state + self.dropout_path3(self.ff(hidden_state)))
# [bs x num_channels x sequence_length x d_model]
hidden_state = hidden_state.reshape(batch_size, num_input_channels, sequence_length, d_model)
outputs = (hidden_state,)
if output_attentions:
outputs += (attn_weights, channel_attn_weights) if self.channel_attention else (attn_weights,)
return outputs
class PatchTSTPreTrainedModel(PreTrainedModel):
config_class = PatchTSTConfig
base_model_prefix = "model"
main_input_name = "past_values"
supports_gradient_checkpointing = False
def _init_weights(self, module):
"""
Initialize weights
"""
if isinstance(module, PatchTSTPositionalEncoding):
# initialize cls_token
if self.config.use_cls_token:
nn.init.normal_(module.cls_token, std=0.02)
# initialize positional encoding
if self.config.positional_encoding_type == "random":
nn.init.normal_(module.position_enc, mean=0.0, std=0.1)
elif isinstance(module, nn.LayerNorm):
module.bias.data.zero_()
module.weight.data.fill_(1.0)
elif isinstance(module, PatchTSTBatchNorm):
module.batchnorm.bias.data.zero_()
module.batchnorm.weight.data.fill_(1.0)
elif isinstance(module, (nn.Linear, nn.Conv1d)):
module.weight.data.normal_(mean=0.0, std=self.config.init_std)
if module.bias is not None:
module.bias.data.zero_()
def _set_gradient_checkpointing(self, module, value=False):
if isinstance(module, (PatchTSTEncoder)):
module.gradient_checkpointing = value
class PatchTSTEmbedding(nn.Module):
def __init__(self, config: PatchTSTConfig):
super().__init__()
self.num_input_channels = config.num_input_channels
self.share_embedding = config.share_embedding
# Input encoding: projection of feature vectors onto a d-dim vector space
if self.share_embedding:
self.input_embedding = nn.Linear(config.patch_length, config.d_model)
else:
self.input_embedding = nn.ModuleList()
for _ in range(config.num_input_channels):
self.input_embedding.append(nn.Linear(config.patch_length, config.d_model))
def forward(self, patch_input: torch.Tensor):
"""
Parameters:
patch_input (`torch.Tensor` of shape `(batch_size, num_channels, num_patches, patch_length)`, *required*):
Patch input for embedding
return:
`torch.Tensor` of shape `(batch_size, num_channels, num_patches, d_model)`
"""
# Input encoding
num_input_channels = patch_input.shape[1]
if num_input_channels != self.num_input_channels:
raise ValueError(
f"The defined number of input channels ({self.num_input_channels}) in the config "
f"has to be the same as the number of channels in the batch input ({num_input_channels})"
)
if self.share_embedding:
embeddings = self.input_embedding(patch_input) # x: [bs x num_channels x num_patches x d_model]
else:
embeddings = [self.input_embedding[i](patch_input[:, i, :, :]) for i in range(num_input_channels)]
embeddings = torch.stack(embeddings, dim=1)
return embeddings
class PatchTSTPositionalEncoding(nn.Module):
"""
Class for positional encoding
"""
def __init__(self, config: PatchTSTConfig, num_patches: int):
super().__init__()
self.use_cls_token = config.use_cls_token
self.num_input_channels = config.num_input_channels
if config.use_cls_token:
# cls_token: [1 x num_input_channels x 1 x d_model]
self.cls_token = nn.Parameter(torch.zeros(1, 1, 1, config.d_model))
num_patches += 1
# postional encoding: [num_patches x d_model]
self.position_enc = self._init_pe(config, num_patches)
# Positional dropout
self.positional_dropout = (
nn.Dropout(config.positional_dropout) if config.positional_dropout > 0 else nn.Identity()
)
@staticmethod
def _init_pe(config: PatchTSTConfig, num_patches: int) -> nn.Parameter:
# Positional encoding
if config.positional_encoding_type == "random":
position_enc = nn.Parameter(torch.randn(num_patches, config.d_model), requires_grad=True)
elif config.positional_encoding_type == "sincos":
position_enc = torch.zeros(num_patches, config.d_model)
position = torch.arange(0, num_patches).unsqueeze(1)
div_term = torch.exp(torch.arange(0, config.d_model, 2) * -(math.log(10000.0) / config.d_model))
position_enc[:, 0::2] = torch.sin(position * div_term)
position_enc[:, 1::2] = torch.cos(position * div_term)
position_enc = position_enc - position_enc.mean()
position_enc = position_enc / (position_enc.std() * 10)
position_enc = nn.Parameter(position_enc, requires_grad=False)
else:
raise ValueError(
f"{config.positional_encoding_type} is not a valid positional encoder. Available types are 'random' and 'sincos'."
)
return position_enc
def forward(self, patch_input: torch.Tensor):
if self.use_cls_token:
# patch_input: [bs x num_channels x num_patches x d_model]
patch_input = self.positional_dropout(patch_input + self.position_enc[1:, :])
# append cls token where cls_token: [1 x num_channels x 1 x d_model]
cls_token = self.cls_token + self.position_enc[:1, :]
# get the same copy of cls_token for all the samples in batch: [bs x num_channels x 1 x d_model]
cls_tokens = cls_token.expand(patch_input.shape[0], self.num_input_channels, -1, -1)
# hidden_state: [bs x num_channels x (num_patches+1) x d_model]
hidden_state = torch.cat((cls_tokens, patch_input), dim=2)
else:
# hidden_state: [bs x num_channels x num_patches x d_model]
hidden_state = self.positional_dropout(patch_input + self.position_enc)
return hidden_state
class PatchTSTEncoder(PatchTSTPreTrainedModel):
"""
PatchTST Encoder
"""
def __init__(self, config: PatchTSTConfig, num_patches: int):
super().__init__(config)
self.gradient_checkpointing = False
# Input embedding: projection of feature vectors onto a d-dim vector space
self.embedder = PatchTSTEmbedding(config)
# Positional encoding
self.positional_encoder = PatchTSTPositionalEncoding(config, num_patches)
# Encoder
self.layers = nn.ModuleList([PatchTSTEncoderLayer(config) for i in range(config.num_hidden_layers)])
# Initialize weights and apply final processing
self.post_init()
def forward(
self,
patch_input: torch.Tensor,
output_hidden_states: Optional[bool] = None,
output_attentions: Optional[bool] = None,
) -> BaseModelOutput:
"""
Parameters:
patch_input (`torch.Tensor` of shape `(batch_size, num_channels, num_patches, patch_length)`, *required*):
Past values of the time series
output_hidden_states (bool, optional): Indicates if hidden states should be outputted.
output_attentions (bool, optional): Indicates if attentions should be outputted.
return:
`BaseModelOutput`
"""
output_attentions = output_attentions if output_attentions is not None else self.config.output_attentions
output_hidden_states = (
output_hidden_states if output_hidden_states is not None else self.config.output_hidden_states
)
# Input embedding
patch_input = self.embedder(patch_input)
# Positional encoding
hidden_state = self.positional_encoder(patch_input)
encoder_states = () if output_hidden_states else None
all_attentions = () if output_attentions else None
for encoder_layer in self.layers:
if output_hidden_states:
encoder_states = encoder_states + (hidden_state,)
layer_outputs = encoder_layer(hidden_state=hidden_state, output_attentions=output_attentions)
# get hidden state. hidden_state shape is [bs x num_channels x num_patches x d_model]
# or [bs x num_channels x (num_patches+1) x d_model] if use cls_token
hidden_state = layer_outputs[0]
# append attention matrix at each layer
if output_attentions:
all_attentions = all_attentions + (layer_outputs[1],)
# return past_values, hidden_states
return BaseModelOutput(last_hidden_state=hidden_state, hidden_states=encoder_states, attentions=all_attentions)
PATCHTST_START_DOCSTRING = r"""
This model inherits from [`PreTrainedModel`]. Check the superclass documentation for the generic methods the
library implements for all its model (such as downloading or saving, resizing the input embeddings, pruning heads
etc.)
This model is also a PyTorch [torch.nn.Module](https://pytorch.org/docs/stable/nn.html#torch.nn.Module) subclass.
Use it as a regular PyTorch Module and refer to the PyTorch documentation for all matter related to general usage
and behavior.
Parameters:
config ([`PatchTSTConfig`]):
Model configuration class with all the parameters of the model. Initializing with a config file does not
load the weights associated with the model, only the configuration. Check out the
[`~PreTrainedModel.from_pretrained`] method to load the model weights.
"""
@dataclass
class PatchTSTModelOutput(ModelOutput):
"""
Base class for model's outputs, with potential hidden states.
Parameters:
last_hidden_state (`torch.FloatTensor` of shape `(batch_size, num_channels, num_patches, patch_length)`):
Sequence of hidden-states at the output of the last layer of the model.
hidden_states (`tuple(torch.FloatTensor)`, *optional*, returned when `output_hidden_states=True` is passed or when `config.output_hidden_states=True`):
Tuple of `torch.FloatTensor` (one for the output of the embeddings, if the model has an embedding layer, +
one for the output of each layer) of shape `(batch_size, num_channels, height, width)`. Hidden-states of
the model at the output of each layer plus the optional initial embedding outputs.
mask: (`torch.FloatTensor` of shape `(batch_size, num_channels, num_patches)`, *optional*)
Bool masked tensor indicating which patches are masked
loc: (`torch.FloatTensor` of shape `(batch_size, 1, num_channels)`, *optional*)
Mean of the input data (batch_size, sequence_length, num_channels) over the sequence_length
scale: (`torch.FloatTensor` of shape `(batch_size, 1, num_channels)`, *optional*)
Std of the input data (batch_size, sequence_length, num_channels) over the sequence_length
patch_input (`torch.FloatTensor` of shape `(batch_size, num_channels, num_patches, patch_length)`):
Patched input to the Transformer
"""
last_hidden_state: torch.FloatTensor = None
hidden_states: Optional[Tuple[torch.FloatTensor]] = None
attentions: Optional[Tuple[torch.FloatTensor]] = None
mask: torch.FloatTensor = None
loc: torch.FloatTensor = None
scale: torch.FloatTensor = None
patch_input: torch.FloatTensor = None
@dataclass
class PatchTSTForPretrainingOutput(ModelOutput):
"""
Output type of [`PatchTSTForPretraining`].
Parameters:
loss (*optional*, returned when `labels` is provided, `torch.FloatTensor` of shape `(1,)`):
MSE loss.
prediction_outputs (`torch.FloatTensor` of shape `(batch_size, sequence_length, config.vocab_size)`):
Prediction outputs of the time series modeling heads.
hidden_states (`tuple(torch.FloatTensor)`, *optional*, returned when `output_hidden_states=True` is passed or when `config.output_hidden_states=True`):
Tuple of `torch.FloatTensor` (one for the output of the embeddings + one for the output of each layer) of
shape `(batch_size, sequence_length, hidden_size)`.
Hidden-states of the model at the output of each layer plus the initial embedding outputs.
attentions (`tuple(torch.FloatTensor)`, *optional*, returned when `output_attentions=True` is passed or when `config.output_attentions=True`):
Tuple of `torch.FloatTensor` (one for each layer) of shape `(batch_size, num_heads, sequence_length,
sequence_length)`.
Attentions weights after the attention softmax, used to compute the weighted average in the self-attention
heads.
"""
loss: Optional[torch.FloatTensor] = None
prediction_output: torch.FloatTensor = None
hidden_states: Optional[Tuple[torch.FloatTensor]] = None
attentions: Optional[Tuple[torch.FloatTensor]] = None
@dataclass
class PatchTSTForRegressionOutput(ModelOutput):
"""
Output type of [`PatchTSTForRegression`].
Parameters:
loss (*optional*, returned when `labels` is provided, `torch.FloatTensor` of shape `(1,)`):
MSE loss.
regression_outputs (`torch.FloatTensor` of shape `(batch_size, num_targets)`):
Regression outputs of the time series modeling heads.
hidden_states (`tuple(torch.FloatTensor)`, *optional*, returned when `output_hidden_states=True` is passed or when `config.output_hidden_states=True`):
Tuple of `torch.FloatTensor` (one for the output of the embeddings + one for the output of each layer) of
shape `(batch_size, sequence_length, hidden_size)`.
Hidden-states of the model at the output of each layer plus the initial embedding outputs.
attentions (`tuple(torch.FloatTensor)`, *optional*, returned when `output_attentions=True` is passed or when `config.output_attentions=True`):
Tuple of `torch.FloatTensor` (one for each layer) of shape `(batch_size, num_heads, sequence_length,
sequence_length)`.
Attentions weights after the attention softmax, used to compute the weighted average in the self-attention
heads.
"""
loss: Optional[torch.FloatTensor] = None
regression_outputs: torch.FloatTensor = None
hidden_states: Optional[Tuple[torch.FloatTensor]] = None
attentions: Optional[Tuple[torch.FloatTensor]] = None
@dataclass
class PatchTSTForPredictionOutput(ModelOutput):
"""
Output type of [`PatchTSTForPrediction`].
Parameters:
loss (*optional*, returned when `labels` is provided, `torch.FloatTensor` of shape `(1,)`):
MSE loss.
prediction_outputs (`torch.FloatTensor` of shape `(batch_size, prediction_length, -1)`):
Prediction outputs of the time series modeling heads.
hidden_states (`tuple(torch.FloatTensor)`, *optional*, returned when `output_hidden_states=True` is passed or when `config.output_hidden_states=True`):
Tuple of `torch.FloatTensor` (one for the output of the embeddings + one for the output of each layer) of
shape `(batch_size, sequence_length, hidden_size)`.
Hidden-states of the model at the output of each layer plus the initial embedding outputs.
attentions (`tuple(torch.FloatTensor)`, *optional*, returned when `output_attentions=True` is passed or when `config.output_attentions=True`):
Tuple of `torch.FloatTensor` (one for each layer) of shape `(batch_size, num_heads, sequence_length,
sequence_length)`.
Attentions weights after the attention softmax, used to compute the weighted average in the self-attention
heads.
loc: (`torch.FloatTensor` of shape `(batch_size, 1, num_channels)`, *optional*)
Mean of the input data (batch_size, sequence_length, num_channels) over the sequence_length
scale: (`torch.FloatTensor` of shape `(batch_size, 1, num_channels)`, *optional*)
Std of the input data (batch_size, sequence_length, num_channels) over the sequence_length
"""
loss: Optional[torch.FloatTensor] = None
prediction_outputs: torch.FloatTensor = None
hidden_states: Optional[Tuple[torch.FloatTensor]] = None
attentions: Optional[Tuple[torch.FloatTensor]] = None
loc: torch.FloatTensor = None
scale: torch.FloatTensor = None
@dataclass
class PatchTSTForClassificationOutput(ModelOutput):
"""
Output type of [`PatchTSTForClassification`].
Parameters:
loss (*optional*, returned when `labels` is provided, `torch.FloatTensor` of shape `(1,)`):
Total loss as the sum of the masked language modeling loss and the next sequence prediction
(classification) loss.
prediction_logits (`torch.FloatTensor` of shape `(batch_size, num_targets)`):
Prediction scores of the PatchTST modeling head (scores before SoftMax).
hidden_states (`tuple(torch.FloatTensor)`, *optional*, returned when `output_hidden_states=True` is passed or when `config.output_hidden_states=True`):
Tuple of `torch.FloatTensor` (one for the output of the embeddings + one for the output of each layer) of
shape `(batch_size, sequence_length, hidden_size)`.
Hidden-states of the model at the output of each layer plus the initial embedding outputs.
attentions (`tuple(torch.FloatTensor)`, *optional*, returned when `output_attentions=True` is passed or when `config.output_attentions=True`):
Tuple of `torch.FloatTensor` (one for each layer) of shape `(batch_size, num_heads, sequence_length,
sequence_length)`.
Attentions weights after the attention softmax, used to compute the weighted average in the self-attention
heads.
"""
loss: Optional[torch.FloatTensor] = None
prediction_logits: torch.FloatTensor = None
hidden_states: Optional[Tuple[torch.FloatTensor]] = None
attentions: Optional[Tuple[torch.FloatTensor]] = None
@dataclass
class SamplePatchTSTOutput(ModelOutput):
"""
Base class for time series model's predictions outputs that contains the sampled values from the chosen
distribution.
Parameters:
sequences `(batch_size, num_samples, prediction_length, num_targets)`):
Sampled values from the chosen distribution.
"""
sequences: torch.FloatTensor = None
# Copied from transformers.models.time_series_transformer.modeling_time_series_transformer.nll
def nll(input: torch.distributions.Distribution, target: torch.Tensor) -> torch.Tensor:
"""
Computes the negative log likelihood loss from input distribution with respect to target.
"""
return -input.log_prob(target)
# Copied from transformers.models.time_series_transformer.modeling_time_series_transformer.weighted_average
def weighted_average(input_tensor: torch.Tensor, weights: Optional[torch.Tensor] = None, dim=None) -> torch.Tensor:
"""
Computes the weighted average of a given tensor across a given `dim`, masking values associated with weight zero,
meaning instead of `nan * 0 = nan` you will get `0 * 0 = 0`.
Args:
input_tensor (`torch.FloatTensor`):
Input tensor, of which the average must be computed.
weights (`torch.FloatTensor`, *optional*):
Weights tensor, of the same shape as `input_tensor`.
dim (`int`, *optional*):
The dim along which to average `input_tensor`.
Returns:
`torch.FloatTensor`: The tensor with values averaged along the specified `dim`.
"""
if weights is not None:
weighted_tensor = torch.where(weights != 0, input_tensor * weights, torch.zeros_like(input_tensor))
sum_weights = torch.clamp(weights.sum(dim=dim) if dim else weights.sum(), min=1.0)
return (weighted_tensor.sum(dim=dim) if dim else weighted_tensor.sum()) / sum_weights
else:
return input_tensor.mean(dim=dim)
# Copied from transformers.models.time_series_transformer.modeling_time_series_transformer.TimeSeriesStdScaler with TimeSeriesTransformer->PatchTST,TimeSeries->PatchTST
class PatchTSTStdScaler(nn.Module):
"""
Standardize features by calculating the mean and scaling along the first dimension, and then normalizes it by
subtracting from the mean and dividing by the standard deviation.
"""
def __init__(self, config: PatchTSTConfig):
super().__init__()
self.dim = config.scaling_dim if hasattr(config, "scaling_dim") else 1
self.keepdim = config.keepdim if hasattr(config, "keepdim") else True
self.minimum_scale = config.minimum_scale if hasattr(config, "minimum_scale") else 1e-5
def forward(
self, data: torch.Tensor, observed_indicator: torch.Tensor
) -> Tuple[torch.Tensor, torch.Tensor, torch.Tensor]:
"""
Parameters:
data (`torch.Tensor` of shape `(batch_size, sequence_length, num_input_channels)`):
input for Batch norm calculation
observed_indicator (`torch.BoolTensor` of shape `(batch_size, sequence_length, num_input_channels)`):
Calculating the scale on the observed indicator.
Returns:
tuple of `torch.Tensor` of shapes
(`(batch_size, sequence_length, num_input_channels)`,`(batch_size, 1, num_input_channels)`,
`(batch_size, 1, num_input_channels)`)
"""
denominator = observed_indicator.sum(self.dim, keepdim=self.keepdim)
denominator = denominator.clamp_min(1.0)
loc = (data * observed_indicator).sum(self.dim, keepdim=self.keepdim) / denominator
variance = (((data - loc) * observed_indicator) ** 2).sum(self.dim, keepdim=self.keepdim) / denominator
scale = torch.sqrt(variance + self.minimum_scale)
return (data - loc) / scale, loc, scale
# Copied from transformers.models.time_series_transformer.modeling_time_series_transformer.TimeSeriesMeanScaler with TimeSeriesTransformer->PatchTST,TimeSeries->PatchTST
class PatchTSTMeanScaler(nn.Module):
"""
Computes a scaling factor as the weighted average absolute value along the first dimension, and scales the data
accordingly.
"""
def __init__(self, config: PatchTSTConfig):
super().__init__()
self.dim = config.scaling_dim if hasattr(config, "scaling_dim") else 1
self.keepdim = config.keepdim if hasattr(config, "keepdim") else True
self.minimum_scale = config.minimum_scale if hasattr(config, "minimum_scale") else 1e-10
self.default_scale = config.default_scale if hasattr(config, "default_scale") else None
def forward(
self, data: torch.Tensor, observed_indicator: torch.Tensor
) -> Tuple[torch.Tensor, torch.Tensor, torch.Tensor]:
"""
Parameters:
data (`torch.Tensor` of shape `(batch_size, sequence_length, num_input_channels)`):
input for Batch norm calculation
observed_indicator (`torch.BoolTensor` of shape `(batch_size, sequence_length, num_input_channels)`):
Calculating the scale on the observed indicator.
Returns:
tuple of `torch.Tensor` of shapes
(`(batch_size, sequence_length, num_input_channels)`,`(batch_size, 1, num_input_channels)`,
`(batch_size, 1, num_input_channels)`)
"""
ts_sum = (data * observed_indicator).abs().sum(self.dim, keepdim=True)
num_observed = observed_indicator.sum(self.dim, keepdim=True)
scale = ts_sum / torch.clamp(num_observed, min=1)
# If `default_scale` is provided, we use it, otherwise we use the scale
# of the batch.
if self.default_scale is None:
batch_sum = ts_sum.sum(dim=0)
batch_observations = torch.clamp(num_observed.sum(0), min=1)
default_scale = torch.squeeze(batch_sum / batch_observations)
else:
default_scale = self.default_scale * torch.ones_like(scale)
# apply default scale where there are no observations
scale = torch.where(num_observed > 0, scale, default_scale)
# ensure the scale is at least `self.minimum_scale`
scale = torch.clamp(scale, min=self.minimum_scale)
scaled_data = data / scale
if not self.keepdim:
scale = scale.squeeze(dim=self.dim)
return scaled_data, torch.zeros_like(scale), scale
# Copied from transformers.models.time_series_transformer.modeling_time_series_transformer.TimeSeriesNOPScaler with TimeSeriesTransformer->PatchTST,TimeSeries->PatchTST
class PatchTSTNOPScaler(nn.Module):
"""
Assigns a scaling factor equal to 1 along the first dimension, and therefore applies no scaling to the input data.
"""
def __init__(self, config: PatchTSTConfig):
super().__init__()
self.dim = config.scaling_dim if hasattr(config, "scaling_dim") else 1
self.keepdim = config.keepdim if hasattr(config, "keepdim") else True
def forward(
self, data: torch.Tensor, observed_indicator: torch.Tensor = None
) -> Tuple[torch.Tensor, torch.Tensor, torch.Tensor]:
"""
Parameters:
data (`torch.Tensor` of shape `(batch_size, sequence_length, num_input_channels)`):
input for Batch norm calculation
Returns:
tuple of `torch.Tensor` of shapes
(`(batch_size, sequence_length, num_input_channels)`,`(batch_size, 1, num_input_channels)`,
`(batch_size, 1, num_input_channels)`)
"""
scale = torch.ones_like(data, requires_grad=False).mean(dim=self.dim, keepdim=self.keepdim)
loc = torch.zeros_like(data, requires_grad=False).mean(dim=self.dim, keepdim=self.keepdim)
return data, loc, scale
class PatchTSTScaler(nn.Module):
def __init__(self, config: PatchTSTConfig):
super().__init__()
if config.scaling == "mean" or config.scaling is True:
self.scaler = PatchTSTMeanScaler(config)
elif config.scaling == "std":
self.scaler = PatchTSTStdScaler(config)
else:
self.scaler = PatchTSTNOPScaler(config)
def forward(
self, data: torch.Tensor, observed_indicator: torch.Tensor
) -> Tuple[torch.Tensor, torch.Tensor, torch.Tensor]:
"""
Parameters:
data (`torch.Tensor` of shape `(batch_size, sequence_length, num_input_channels)`):
Input for scaler calculation
observed_indicator (`torch.BoolTensor` of shape `(batch_size, sequence_length, num_input_channels)`):
Calculating the scale on the observed indicator.
Returns:
tuple of `torch.Tensor` of shapes
(`(batch_size, sequence_length, num_input_channels)`,`(batch_size, 1, num_input_channels)`,
`(batch_size, 1, um_input_channels)`)
"""
data, loc, scale = self.scaler(data, observed_indicator)
return data, loc, scale
@add_start_docstrings(
"The bare PatchTST Model outputting raw hidden-states without any specific head.",
PATCHTST_START_DOCSTRING,
)
class PatchTSTModel(PatchTSTPreTrainedModel):
def __init__(self, config: PatchTSTConfig):
super().__init__(config)
self.scaler = PatchTSTScaler(config)
self.patchifier = PatchTSTPatchify(config)
self.do_mask_input = config.do_mask_input
# get num_patches information from PatchTSTPatchify
num_patches = self.patchifier.num_patches
if self.do_mask_input:
self.masking = PatchTSTMasking(config)
else:
self.masking = nn.Identity()
self.encoder = PatchTSTEncoder(config, num_patches=num_patches)
# Initialize weights and apply final processing
self.post_init()
def forward(
self,
past_values: torch.Tensor,
past_observed_mask: Optional[torch.Tensor] = None,
future_values: Optional[torch.Tensor] = None,
output_hidden_states: Optional[bool] = None,
output_attentions: Optional[bool] = None,
return_dict: Optional[bool] = None,
) -> Union[Tuple, PatchTSTModelOutput]:
r"""
Parameters:
past_values (`torch.Tensor` of shape `(bs, sequence_length, num_input_channels)`, *required*):
Input sequence to the model
past_observed_mask (`torch.BoolTensor` of shape `(batch_size, sequence_length, num_input_channels)`, *optional*):
Boolean mask to indicate which `past_values` were observed and which were missing. Mask values selected
in `[0, 1]`:
- 1 for values that are **observed**,
- 0 for values that are **missing** (i.e. NaNs that were replaced by zeros).
future_values (`torch.BoolTensor` of shape `(batch_size, prediction_length, num_input_channels)`, *optional*):
Future target values associated with the `past_values`
output_hidden_states (`bool`, *optional*):
Whether or not to return the hidden states of all layers
output_attentions (`bool`, *optional*):
Whether or not to return the output attention of all layers
return_dict (`bool`, *optional*):
Whether or not to return a `ModelOutput` instead of a plain tuple.
Returns:
`PatchTSTModelOutput` or tuple of `torch.Tensor` (if `return_dict`=False or `config.return_dict`=False)
Examples:
```python
>>> from huggingface_hub import hf_hub_download
>>> import torch
>>> from transformers import PatchTSTModel
>>> file = hf_hub_download(
... repo_id="hf-internal-testing/etth1-hourly-batch", filename="train-batch.pt", repo_type="dataset"
... )
>>> batch = torch.load(file)
>>> model = PatchTSTModel.from_pretrained("namctin/patchtst_etth1_pretrain")
>>> # during training, one provides both past and future values
>>> outputs = model(
... past_values=batch["past_values"],
... future_values=batch["future_values"],
... )
>>> last_hidden_state = outputs.last_hidden_state
```"""
return_dict = return_dict if return_dict is not None else self.config.use_return_dict
output_attentions = output_attentions if output_attentions is not None else self.config.output_attentions
output_hidden_states = (
output_hidden_states if output_hidden_states is not None else self.config.output_hidden_states
)
if past_observed_mask is None:
past_observed_mask = torch.ones_like(past_values)
# x: tensor [bs x sequence_length x num_input_channels]
scaled_past_values, loc, scale = self.scaler(past_values, past_observed_mask)
# patched_values: [bs x num_input_channels x num_patches x patch_length] for pretrain
patched_values = self.patchifier(scaled_past_values)
if self.do_mask_input:
masked_values, mask = self.masking(patched_values)
else:
masked_values, mask = self.masking(patched_values), None
encoder_output = self.encoder(
patch_input=masked_values, output_hidden_states=output_hidden_states, output_attentions=output_attentions
)
if not return_dict:
outputs = (encoder_output.last_hidden_state, encoder_output.hidden_states, encoder_output.attentions)
outputs = outputs + (mask, loc, scale, patched_values)
return tuple(v for v in outputs if v is not None)
return PatchTSTModelOutput(
last_hidden_state=encoder_output.last_hidden_state,
hidden_states=encoder_output.hidden_states,
attentions=encoder_output.attentions,
mask=mask,
loc=loc,
scale=scale,
patch_input=patched_values,
)
class PatchTSTMaskPretrainHead(nn.Module):
"""
Pretraining head for mask modelling
"""
def __init__(self, config: PatchTSTConfig):
super().__init__()
self.dropout = nn.Dropout(config.dropout)
self.linear = nn.Linear(config.d_model, config.patch_length)
self.use_cls_token = config.use_cls_token
def forward(self, embedding: torch.Tensor) -> torch.Tensor:
"""
Parameters:
embedding (`torch.Tensor` of shape `(bs, num_channels, num_patches, d_model)` or
`(bs, num_channels, num_patches+1, d_model)` if `cls_token` is set to True, *required*):
Embedding from the model
Returns:
`torch.Tensor` of shape `(bs, num_channels, num_patches, d_model)` or
`(bs, num_channels, num_patches+1, d_model)` if `cls_token` is set to True
"""
embedding = self.linear(self.dropout(embedding)) # [bs x num_channels x num_patches x patch_length]
if self.use_cls_token:
embedding = embedding[:, :, 1:, :] # remove the first cls token
return embedding
@add_start_docstrings(
"The PatchTST for pretrain model.",
PATCHTST_START_DOCSTRING,
)
class PatchTSTForPretraining(PatchTSTPreTrainedModel):
def __init__(self, config: PatchTSTConfig):
super().__init__(config)
config.do_mask_input = True
self.model = PatchTSTModel(config=config)
self.head = PatchTSTMaskPretrainHead(config)
# Initialize weights and apply final processing
self.post_init()
def forward(
self,
past_values: torch.Tensor,
past_observed_mask: Optional[torch.Tensor] = None,
output_hidden_states: Optional[bool] = None,
output_attentions: Optional[bool] = None,
return_dict: Optional[bool] = None,
) -> Union[Tuple, PatchTSTForPretrainingOutput]:
r"""
Parameters:
past_values (`torch.Tensor` of shape `(bs, sequence_length, num_input_channels)`, *required*):
Input sequence to the model
past_observed_mask (`torch.BoolTensor` of shape `(batch_size, sequence_length, num_input_channels)`, *optional*):
Boolean mask to indicate which `past_values` were observed and which were missing. Mask values selected
in `[0, 1]`:
- 1 for values that are **observed**,
- 0 for values that are **missing** (i.e. NaNs that were replaced by zeros).
output_hidden_states (`bool`, *optional*):
Whether or not to return the hidden states of all layers
output_attentions (`bool`, *optional*):
Whether or not to return the output attention of all layers
return_dict (`bool`, *optional*): Whether or not to return a `ModelOutput` instead of a plain tuple.
Returns:
`PatchTSTForPretrainingOutput` or tuple of `torch.Tensor` (if `return_dict`=False or
`config.return_dict`=False)
Examples:
```python
>>> from huggingface_hub import hf_hub_download
>>> import torch
>>> from transformers import PatchTSTConfig, PatchTSTForPretraining
>>> file = hf_hub_download(
... repo_id="hf-internal-testing/etth1-hourly-batch", filename="train-batch.pt", repo_type="dataset"
... )
>>> batch = torch.load(file)
>>> # Config for random mask pretraining
>>> config = PatchTSTConfig(
... num_input_channels=7,
... context_length=512,
... patch_length=12,
... stride=12,
... mask_type='random',
... random_mask_ratio=0.4,
... use_cls_token=True,
... )
>>> # Config for forecast mask pretraining
>>> config = PatchTSTConfig(
... num_input_channels=7,
... context_length=512,
... patch_length=12,
... stride=12,
... mask_type='forecast',
... num_forecast_mask_patches=5,
... use_cls_token=True,
... )
>>> model = PatchTSTForPretraining(config)
>>> # during training, one provides both past and future values
>>> outputs = model(past_values=batch["past_values"])
>>> loss = outputs.loss
>>> loss.backward()
```"""
return_dict = return_dict if return_dict is not None else self.config.use_return_dict
# past_values: [bs x num_channels x num_patches x d_model] or
# [bs x num_channels x (num_patches+1) x d_model] if use cls_token
model_output = self.model(
past_values=past_values,
past_observed_mask=past_observed_mask,
output_hidden_states=output_hidden_states,
output_attentions=output_attentions,
return_dict=True,
)
# last_hidden_state: [bs x num_channels x num_patches x patch_length] or
# [bs x num_channels x (num_patches+1) x patch_length] if use cls_token
x_hat = self.head(model_output.last_hidden_state)
# calculate masked_loss
loss = nn.MSELoss(reduction="none")
loss_val = loss(x_hat, model_output.patch_input)
masked_loss = (loss_val.mean(dim=-1) * model_output.mask).sum() / (model_output.mask.sum() + 1e-10)
encoder_states = model_output.hidden_states
if not return_dict:
outputs = (x_hat,) + model_output[1:-4]
outputs = (masked_loss,) + outputs if masked_loss is not None else outputs
return outputs
return PatchTSTForPretrainingOutput(
loss=masked_loss, prediction_output=x_hat, hidden_states=encoder_states, attentions=model_output.attentions
)
class PatchTSTClassificationHead(nn.Module):
def __init__(self, config: PatchTSTConfig):
super().__init__()
self.use_cls_token = config.use_cls_token
self.pooling_type = config.pooling_type
self.flatten = nn.Flatten(start_dim=1)
self.dropout = nn.Dropout(config.head_dropout) if config.head_dropout > 0 else nn.Identity()
self.linear = nn.Linear(config.num_input_channels * config.d_model, config.num_targets)
def forward(self, embedding: torch.Tensor):
"""
Parameters:
embedding (`torch.Tensor` of shape `(bs, num_channels, num_patches, d_model)` or
`(bs, num_channels, num_patches+1, d_model)` if `cls_token` is set to True, *required*):
Embedding from the model
Returns:
`torch.Tensor` of shape `(bs, num_targets)`
"""
if self.use_cls_token:
# use the first output token, pooled_embedding: bs x num_channels x d_model
pooled_embedding = embedding[:, :, 0, :]
elif self.pooling_type == "mean":
# pooled_embedding: [bs x num_channels x d_model]
pooled_embedding = embedding.mean(dim=2)
elif self.pooling_type == "max":
# pooled_embedding: [bs x num_channels x d_model]
pooled_embedding = embedding.max(dim=2).values
else:
raise ValueError(f"pooling operator {self.pooling_type} is not implemented yet")
# pooled_embedding: bs x num_channels * d_model
pooled_embedding = self.flatten(pooled_embedding)
# output: bs x n_classes
output = self.linear(self.dropout(pooled_embedding))
return output
@add_start_docstrings(
"The PatchTST for classification model.",
PATCHTST_START_DOCSTRING,
)
class PatchTSTForClassification(PatchTSTPreTrainedModel):
def __init__(self, config: PatchTSTConfig):
super().__init__(config)
# Turn off masking
if config.do_mask_input:
logger.warning("Setting `do_mask_input` parameter to False.")
config.do_mask_input = False
self.model = PatchTSTModel(config)
self.head = PatchTSTClassificationHead(config)
# Initialize weights and apply final processing
self.post_init()
def forward(
self,
past_values: torch.Tensor,
target_values: torch.Tensor = None,
past_observed_mask: Optional[bool] = None,
output_hidden_states: Optional[bool] = None,
output_attentions: Optional[bool] = None,
return_dict: Optional[bool] = None,
) -> Union[tuple, PatchTSTForClassificationOutput]:
r"""
Parameters:
past_values (`torch.Tensor` of shape `(bs, sequence_length, num_input_channels)`, *required*):
Input sequence to the model
target_values (`torch.Tensor`, *optional*):
Labels associates with the `past_values`
past_observed_mask (`torch.BoolTensor` of shape `(batch_size, sequence_length, num_input_channels)`, *optional*):
Boolean mask to indicate which `past_values` were observed and which were missing. Mask values selected
in `[0, 1]`:
- 1 for values that are **observed**,
- 0 for values that are **missing** (i.e. NaNs that were replaced by zeros).
output_hidden_states (`bool`, *optional*):
Whether or not to return the hidden states of all layers
output_attentions (`bool`, *optional*):
Whether or not to return the output attention of all layers
return_dict (`bool`, *optional*):
Whether or not to return a `ModelOutput` instead of a plain tuple.
Returns:
`PatchTSTForClassificationOutput` or tuple of `torch.Tensor` (if `return_dict`=False or
`config.return_dict`=False)
Examples:
```python
>>> from transformers import PatchTSTConfig, PatchTSTForClassification
>>> # classification task with two input channel2 and 3 classes
>>> config = PatchTSTConfig(
... num_input_channels=2,
... num_targets=3,
... context_length=512,
... patch_length=12,
... stride=12,
... use_cls_token=True,
... )
>>> model = PatchTSTForClassification(config=config)
>>> # during inference, one only provides past values
>>> past_values = torch.randn(20, 512, 2)
>>> outputs = model(past_values=past_values)
>>> labels = outputs.prediction_logits
```"""
return_dict = return_dict if return_dict is not None else self.config.use_return_dict
model_output = self.model(
past_values=past_values,
past_observed_mask=past_observed_mask,
output_hidden_states=output_hidden_states,
output_attentions=output_attentions,
return_dict=True,
)
y_hat = self.head(model_output.last_hidden_state)
loss_val = None
if target_values is not None:
loss = nn.CrossEntropyLoss()
loss_val = loss(y_hat, target_values)
if not return_dict:
outputs = (y_hat,) + model_output[1:-3]
outputs = (loss_val,) + outputs if loss_val is not None else outputs
return outputs
return PatchTSTForClassificationOutput(
loss=loss_val,
prediction_logits=y_hat,
hidden_states=model_output.hidden_states,
attentions=model_output.attentions,
)
@add_start_docstrings(
"The PatchTST for regression Model.",
PATCHTST_START_DOCSTRING,
)
class PatchTSTPredictionHead(nn.Module):
def __init__(self, config: PatchTSTConfig, num_patches, distribution_output=None):
super().__init__()
self.share_projection = config.share_projection
self.num_input_channels = config.num_input_channels
self.use_cls_token = config.use_cls_token
self.pooling_type = config.pooling_type
if self.pooling_type or self.use_cls_token:
head_dim = config.d_model
else:
head_dim = config.d_model * num_patches
if not self.share_projection:
# if each channel has its own head
self.projections = nn.ModuleList()
self.dropouts = nn.ModuleList()
self.flattens = nn.ModuleList()
for i in range(self.num_input_channels):
self.flattens.append(nn.Flatten(start_dim=2))
if distribution_output is None:
# use linear head
self.projections.append(nn.Linear(head_dim, config.prediction_length))
else:
# use distribution head
self.projections.append(distribution_output.get_parameter_projection(head_dim))
self.dropouts.append(nn.Dropout(config.head_dropout) if config.head_dropout > 0 else nn.Identity())
else:
# all the channels share the same head
self.flatten = nn.Flatten(start_dim=2)
if distribution_output is None:
# use linear head
self.projection = nn.Linear(head_dim, config.prediction_length)
else:
# use distribution head
self.projection = distribution_output.get_parameter_projection(head_dim)
self.dropout = nn.Dropout(config.head_dropout) if config.head_dropout > 0 else nn.Identity()
def forward(self, embedding: torch.Tensor):
"""
Parameters:
embedding (`torch.Tensor` of shape `(bs, num_channels, num_patches, d_model)` or
`(bs, num_channels, num_patches+1, d_model)` if `cls_token` is set to True, *required*):
Embedding from the model
Returns:
`torch.Tensor` of shape `(bs, forecast_len, num_channels)`
"""
if self.use_cls_token:
# pooled_embedding: [bs x num_channels x d_model]
pooled_embedding = embedding[:, :, 0, :]
else:
if self.pooling_type == "mean":
# pooled_embedding: [bs x num_channels x d_model]
pooled_embedding = embedding.mean(dim=2)
elif self.pooling_type == "max":
# pooled_embedding: [bs x num_channels x d_model]
pooled_embedding = embedding.max(dim=2).values
else:
# pooled_embedding: [bs x num_channels x num_patches x d_model]
pooled_embedding = embedding
if not self.share_projection:
output = []
for i in range(self.num_input_channels):
# pooled_embedding: [bs x (d_model * num_patches)] or [bs x d_model)]
pooled_embedding = self.flattens[i](pooled_embedding[:, i, :])
pooled_embedding = self.dropouts[i](pooled_embedding)
# pooled_embedding: [bs x forecast_len]
# or tuple ([bs x forecast_len], [bs x forecast_len]) if using distribution head
pooled_embedding = self.projections[i](pooled_embedding)
output.append(pooled_embedding)
# output: [bs x num_channels x forecast_len]
output = torch.stack(output, dim=1)
else:
# pooled_embedding: [bs x num_channels x (d_model * num_patches)] or [bs x num_channels x d_model)]
pooled_embedding = self.flatten(pooled_embedding)
pooled_embedding = self.dropout(pooled_embedding)
# output: [bs x num_channels x forecast_len] or
# tuple ([bs x num_channels x forecast_len], [bs x num_channels x forecast_len]) if using distribution head
output = self.projection(pooled_embedding)
if isinstance(output, tuple):
# output: ([bs x forecast_len x num_channels], [bs x forecast_len x num_channels])
output = tuple(z.transpose(2, 1) for z in output)
else:
output = output.transpose(2, 1) # [bs x forecast_len x num_channels]
return output
@add_start_docstrings(
"The PatchTST for prediction model.",
PATCHTST_START_DOCSTRING,
)
class PatchTSTForPrediction(PatchTSTPreTrainedModel):
def __init__(self, config: PatchTSTConfig):
super().__init__(config)
# Turn off masking
if config.do_mask_input:
logger.warning("Setting `do_mask_input` parameter to False.")
config.do_mask_input = False
self.model = PatchTSTModel(config)
if config.loss == "mse":
self.distribution_output = None
else:
if config.distribution_output == "student_t":
self.distribution_output = StudentTOutput(dim=config.prediction_length)
elif config.distribution_output == "normal":
self.distribution_output = NormalOutput(dim=config.prediction_length)
elif config.distribution_output == "negative_binomial":
self.distribution_output = NegativeBinomialOutput(dim=config.prediction_length)
else:
raise ValueError(f"Unknown distribution output {config.distribution_output}")
self.head = PatchTSTPredictionHead(
config, self.model.patchifier.num_patches, distribution_output=self.distribution_output
)
# Initialize weights and apply final processing
self.post_init()
def forward(
self,
past_values: torch.Tensor,
past_observed_mask: Optional[torch.Tensor] = None,
future_values: Optional[torch.Tensor] = None,
output_hidden_states: Optional[bool] = None,
output_attentions: Optional[bool] = None,
return_dict: Optional[bool] = None,
) -> Union[Tuple, PatchTSTForPredictionOutput]:
r"""
Parameters:
past_values (`torch.Tensor` of shape `(bs, sequence_length, num_input_channels)`, *required*):
Input sequence to the model
past_observed_mask (`torch.BoolTensor` of shape `(batch_size, sequence_length, num_input_channels)`, *optional*):
Boolean mask to indicate which `past_values` were observed and which were missing. Mask values selected
in `[0, 1]`:
- 1 for values that are **observed**,
- 0 for values that are **missing** (i.e. NaNs that were replaced by zeros).
future_values (`torch.Tensor` of shape `(bs, forecast_len, num_input_channels)`, *optional*):
Future target values associated with the `past_values`
output_hidden_states (`bool`, *optional*):
Whether or not to return the hidden states of all layers
output_attentions (`bool`, *optional*):
Whether or not to return the output attention of all layers
return_dict (`bool`, *optional*):
Whether or not to return a `ModelOutput` instead of a plain tuple.
Returns:
`PatchTSTForPredictionOutput` or tuple of `torch.Tensor` (if `return_dict`=False or
`config.return_dict`=False)
Examples:
```python
>>> from huggingface_hub import hf_hub_download
>>> import torch
>>> from transformers import PatchTSTConfig, PatchTSTForPrediction
>>> file = hf_hub_download(
... repo_id="hf-internal-testing/etth1-hourly-batch", filename="train-batch.pt", repo_type="dataset"
... )
>>> batch = torch.load(file)
>>> # Prediction task with 7 input channels and prediction length is 96
>>> model = PatchTSTForPrediction.from_pretrained("namctin/patchtst_etth1_forecast")
>>> # during training, one provides both past and future values
>>> outputs = model(
... past_values=batch["past_values"],
... future_values=batch["future_values"],
... )
>>> loss = outputs.loss
>>> loss.backward()
>>> # during inference, one only provides past values, the model outputs future values
>>> outputs = model(past_values=batch["past_values"])
>>> prediction_outputs = outputs.prediction_outputs
```"""
return_dict = return_dict if return_dict is not None else self.config.use_return_dict
# get model output
model_output = self.model(
past_values=past_values,
past_observed_mask=past_observed_mask,
output_hidden_states=output_hidden_states,
output_attentions=output_attentions,
return_dict=True,
)
# get output head
y_hat = self.head(model_output.last_hidden_state)
loss_val = None
if self.distribution_output:
y_hat_out = y_hat
else:
y_hat_out = y_hat * model_output.scale + model_output.loc
if future_values is not None:
if self.distribution_output:
distribution = self.distribution_output.distribution(
y_hat, loc=model_output.loc, scale=model_output.scale
)
loss_val = nll(distribution, future_values)
# take average of the loss
loss_val = weighted_average(loss_val)
else:
loss = nn.MSELoss(reduction="mean")
loss_val = loss(y_hat_out, future_values)
loc = model_output.loc
scale = model_output.scale
if not return_dict:
outputs = (y_hat_out,) + model_output[1:-1]
outputs = (loss_val,) + outputs if loss_val is not None else outputs
return outputs
return PatchTSTForPredictionOutput(
loss=loss_val,
prediction_outputs=y_hat_out,
hidden_states=model_output.hidden_states,
attentions=model_output.attentions,
loc=loc,
scale=scale,
)
def generate(
self,
past_values: torch.Tensor,
past_observed_mask: Optional[torch.Tensor] = None,
) -> SamplePatchTSTOutput:
"""
Generate sequences of sample predictions from a model with a probability distribution head.
Parameters:
past_values (`torch.FloatTensor` of shape `(batch_size, sequence_length, num_input_channels)`):
Past values of the time series that serves as context in order to predict the future.
past_observed_mask (`torch.BoolTensor` of shape `(batch_size, sequence_length, num_input_channels)`, *optional*):
Boolean mask to indicate which `past_values` were observed and which were missing. Mask values selected
in `[0, 1]`:
- 1 for values that are **observed**,
- 0 for values that are **missing** (i.e. NaNs that were replaced by zeros).
Return:
[`SamplePatchTSTOutput`] where the outputs `sequences` tensor will have shape `(batch_size, number of
samples, prediction_length, 1)` or `(batch_size, number of samples, prediction_length, num_input_channels)`
for multivariate predictions.
"""
# get number of samples
num_parallel_samples = self.config.num_parallel_samples
# get model output
outputs = self(
past_values=past_values,
future_values=None,
past_observed_mask=past_observed_mask,
output_hidden_states=False,
)
if self.distribution_output:
# get distribution
distribution = self.distribution_output.distribution(
outputs.prediction_outputs, loc=outputs.loc, scale=outputs.scale
)
# get samples: list of [bs x forecast_len x num_channels]
samples = [distribution.sample() for _ in range(num_parallel_samples)]
# samples: [bs x num_samples x forecast_len x num_channels]
samples = torch.stack(samples, dim=1)
else:
samples = outputs.prediction_outputs.unsqueeze(1)
return SamplePatchTSTOutput(sequences=samples)
class PatchTSTRegressionHead(nn.Module):
"""
Regression head
"""
def __init__(self, config: PatchTSTConfig, distribution_output=None):
super().__init__()
self.y_range = config.output_range
self.use_cls_token = config.use_cls_token
self.pooling_type = config.pooling_type
self.distribution_output = distribution_output
head_dim = config.num_input_channels * config.d_model
self.flatten = nn.Flatten(start_dim=1)
self.dropout = nn.Dropout(config.head_dropout) if config.head_dropout > 0 else nn.Identity()
if distribution_output is None:
self.projection = nn.Linear(head_dim, config.num_targets)
else:
self.projection = distribution_output.get_parameter_projection(head_dim)
def forward(self, embedding: torch.Tensor):
"""
Parameters:
embedding (`torch.Tensor` of shape `(bs, num_channels, num_patches, d_model)` or
`(bs, num_channels, num_patches+1, d_model)` if `cls_token` is set to True, *required*):
Embedding from the model
Returns:
`torch.Tensor` of shape `(bs, output_dim)`
"""
if self.use_cls_token:
# use the first output token, pooled_embedding: [bs x num_channels x d_model]
pooled_embedding = embedding[:, :, 0, :]
elif self.pooling_type == "mean":
# pooled_embedding: [bs x num_channels x d_model]
pooled_embedding = embedding.mean(dim=2)
elif self.pooling_type == "max":
# pooled_embedding: [bs x num_channels x d_model]
pooled_embedding = embedding.max(dim=2).values
else:
raise ValueError(f"pooling operator {self.pooling_type} is not implemented yet")
# flatten the input
# pooled_embedding: bs x (num_channels * d_model)
pooled_embedding = self.dropout(self.flatten(pooled_embedding))
# projection
# output: bs x output_dim or a tuple of this shape for distribution head
output = self.projection(pooled_embedding)
# apply sigmoid to bound the output if required
if (self.distribution_output is None) & (self.y_range is not None): # linear head
output = torch.sigmoid(output) * (self.y_range[1] - self.y_range[0]) + self.y_range[0]
return output
@add_start_docstrings(
"The PatchTST for regression model.",
PATCHTST_START_DOCSTRING,
)
class PatchTSTForRegression(PatchTSTPreTrainedModel):
def __init__(self, config: PatchTSTConfig):
super().__init__(config)
# Turn off masking
if config.do_mask_input:
logger.warning("Setting `do_mask_input` parameter to False.")
config.do_mask_input = False
self.model = PatchTSTModel(config)
if config.loss == "mse":
self.distribution_output = None
else:
if config.distribution_output == "student_t":
self.distribution_output = StudentTOutput(dim=config.num_targets)
elif config.distribution_output == "normal":
self.distribution_output = NormalOutput(dim=config.num_targets)
elif config.distribution_output == "negative_binomial":
self.distribution_output = NegativeBinomialOutput(dim=config.num_targets)
else:
raise ValueError(f"Unknown distribution output {config.distribution_output}")
self.head = PatchTSTRegressionHead(config, self.distribution_output)
# Initialize weights and apply final processing
self.post_init()
def forward(
self,
past_values: torch.Tensor,
target_values: torch.Tensor = None,
past_observed_mask: Optional[torch.Tensor] = None,
output_hidden_states: Optional[bool] = None,
output_attentions: Optional[bool] = None,
return_dict: Optional[bool] = None,
) -> Union[tuple, PatchTSTForRegressionOutput]:
r"""
Parameters:
past_values (`torch.Tensor` of shape `(bs, sequence_length, num_input_channels)`, *required*):
Input sequence to the model
target_values (`torch.Tensor` of shape `(bs, num_input_channels)`):
Target values associates with the `past_values`
past_observed_mask (`torch.BoolTensor` of shape `(batch_size, sequence_length, num_input_channels)`, *optional*):
Boolean mask to indicate which `past_values` were observed and which were missing. Mask values selected
in `[0, 1]`:
- 1 for values that are **observed**,
- 0 for values that are **missing** (i.e. NaNs that were replaced by zeros).
output_hidden_states (`bool`, *optional*):
Whether or not to return the hidden states of all layers
output_attentions (`bool`, *optional*):
Whether or not to return the output attention of all layers
return_dict (`bool`, *optional*):
Whether or not to return a `ModelOutput` instead of a plain tuple.
Returns:
`PatchTSTForRegressionOutput` or tuple of `torch.Tensor` (if `return_dict`=False or
`config.return_dict`=False)
Examples:
```python
>>> from transformers import PatchTSTConfig, PatchTSTForRegression
>>> # Regression task with 6 input channels and regress 2 targets
>>> model = PatchTSTForRegression.from_pretrained("namctin/patchtst_etth1_regression")
>>> # during inference, one only provides past values, the model outputs future values
>>> past_values = torch.randn(20, 512, 6)
>>> outputs = model(past_values=past_values)
>>> regression_outputs = outputs.regression_outputs
```"""
return_dict = return_dict if return_dict is not None else self.config.use_return_dict
model_output = self.model(
past_values=past_values,
past_observed_mask=past_observed_mask,
output_hidden_states=output_hidden_states,
output_attentions=output_attentions,
return_dict=True,
)
# get output head. y_hat is of shape [bs x num_targets] or tuple of this shape
y_hat = self.head(model_output.last_hidden_state)
loss = None
if target_values is not None:
if self.distribution_output:
distribution = self.distribution_output.distribution(y_hat)
# y_hat should be a 2-tuple, each with dimension [bs, num_targets]
y_hat = tuple([item.view(-1, self.config.num_targets) for item in y_hat])
loss = nll(distribution, target_values)
# take average of the loss
loss = weighted_average(loss)
else:
loss = nn.MSELoss(reduction="mean")
loss = loss(y_hat, target_values)
if not return_dict:
# hidden_states, attentions, mask
outputs = (y_hat,) + model_output[1:-3]
outputs = (loss,) + outputs if loss is not None else outputs
return outputs
return PatchTSTForRegressionOutput(
loss=loss,
regression_outputs=y_hat,
hidden_states=model_output.hidden_states,
attentions=model_output.attentions,
)
def generate(
self,
past_values: torch.Tensor,
past_observed_mask: Optional[torch.Tensor] = None,
) -> SamplePatchTSTOutput:
"""
Generate sequences of sample predictions from a model with a probability distribution head.
Parameters:
past_values (`torch.FloatTensor` of shape `(batch_size, sequence_length, num_input_channels)`):
Past values of the time series that serves as context in order to predict the future.
past_observed_mask (`torch.BoolTensor` of shape `(batch_size, sequence_length, num_input_channels)`, *optional*):
Boolean mask to indicate which `past_values` were observed and which were missing. Mask values selected
in `[0, 1]`:
- 1 for values that are **observed**,
- 0 for values that are **missing** (i.e. NaNs that were replaced by zeros).
Return:
[`SamplePatchTSTOutput`] where the outputs `sequences` tensor will have shape `(batch_size, number of
samples, num_targets)`.
"""
# get number of samples
num_parallel_samples = self.config.num_parallel_samples
# get model output
outputs = self(
past_values=past_values,
target_values=None,
past_observed_mask=past_observed_mask,
output_hidden_states=False,
)
# get distribution
distribution = self.distribution_output.distribution(outputs.regression_outputs)
# get samples: list of [bs x num_targets]
samples = [distribution.sample() for _ in range(num_parallel_samples)]
# samples: [bs x num_samples x num_targets]
samples = torch.stack(samples, dim=1).view(-1, num_parallel_samples, self.config.num_targets)
return SamplePatchTSTOutput(sequences=samples)
| transformers/src/transformers/models/patchtst/modeling_patchtst.py/0 | {
"file_path": "transformers/src/transformers/models/patchtst/modeling_patchtst.py",
"repo_id": "transformers",
"token_count": 39278
} | 351 |
# coding=utf-8
# Copyright 2022 The HuggingFace Inc. team. All rights reserved.
#
# Licensed under the Apache License, Version 2.0 (the "License");
# you may not use this file except in compliance with the License.
# You may obtain a copy of the License at
#
# http://www.apache.org/licenses/LICENSE-2.0
#
# Unless required by applicable law or agreed to in writing, software
# distributed under the License is distributed on an "AS IS" BASIS,
# WITHOUT WARRANTIES OR CONDITIONS OF ANY KIND, either express or implied.
# See the License for the specific language governing permissions and
# limitations under the License.
"""Image processor class for Perceiver."""
from typing import Dict, List, Optional, Union
import numpy as np
from ...image_processing_utils import BaseImageProcessor, BatchFeature, get_size_dict
from ...image_transforms import center_crop, resize, to_channel_dimension_format
from ...image_utils import (
IMAGENET_DEFAULT_MEAN,
IMAGENET_DEFAULT_STD,
ChannelDimension,
ImageInput,
PILImageResampling,
get_image_size,
infer_channel_dimension_format,
is_scaled_image,
make_list_of_images,
to_numpy_array,
valid_images,
)
from ...utils import TensorType, is_vision_available, logging
if is_vision_available():
import PIL
logger = logging.get_logger(__name__)
class PerceiverImageProcessor(BaseImageProcessor):
r"""
Constructs a Perceiver image processor.
Args:
do_center_crop (`bool`, `optional`, defaults to `True`):
Whether or not to center crop the image. If the input size if smaller than `crop_size` along any edge, the
image will be padded with zeros and then center cropped. Can be overridden by the `do_center_crop`
parameter in the `preprocess` method.
crop_size (`Dict[str, int]`, *optional*, defaults to `{"height": 256, "width": 256}`):
Desired output size when applying center-cropping. Can be overridden by the `crop_size` parameter in the
`preprocess` method.
do_resize (`bool`, *optional*, defaults to `True`):
Whether to resize the image to `(size["height"], size["width"])`. Can be overridden by the `do_resize`
parameter in the `preprocess` method.
size (`Dict[str, int]` *optional*, defaults to `{"height": 224, "width": 224}`):
Size of the image after resizing. Can be overridden by the `size` parameter in the `preprocess` method.
resample (`PILImageResampling`, *optional*, defaults to `PILImageResampling.BICUBIC`):
Defines the resampling filter to use if resizing the image. Can be overridden by the `resample` parameter
in the `preprocess` method.
do_rescale (`bool`, *optional*, defaults to `True`):
Whether to rescale the image by the specified scale `rescale_factor`. Can be overridden by the `do_rescale`
parameter in the `preprocess` method.
rescale_factor (`int` or `float`, *optional*, defaults to `1/255`):
Defines the scale factor to use if rescaling the image. Can be overridden by the `rescale_factor` parameter
in the `preprocess` method.
do_normalize:
Whether to normalize the image. Can be overridden by the `do_normalize` parameter in the `preprocess`
method.
image_mean (`float` or `List[float]`, *optional*, defaults to `IMAGENET_STANDARD_MEAN`):
Mean to use if normalizing the image. This is a float or list of floats the length of the number of
channels in the image. Can be overridden by the `image_mean` parameter in the `preprocess` method.
image_std (`float` or `List[float]`, *optional*, defaults to `IMAGENET_STANDARD_STD`):
Standard deviation to use if normalizing the image. This is a float or list of floats the length of the
number of channels in the image. Can be overridden by the `image_std` parameter in the `preprocess` method.
"""
model_input_names = ["pixel_values"]
def __init__(
self,
do_center_crop: bool = True,
crop_size: Dict[str, int] = None,
do_resize: bool = True,
size: Dict[str, int] = None,
resample: PILImageResampling = PILImageResampling.BICUBIC,
do_rescale: bool = True,
rescale_factor: Union[int, float] = 1 / 255,
do_normalize: bool = True,
image_mean: Optional[Union[float, List[float]]] = None,
image_std: Optional[Union[float, List[float]]] = None,
**kwargs,
) -> None:
super().__init__(**kwargs)
crop_size = crop_size if crop_size is not None else {"height": 256, "width": 256}
crop_size = get_size_dict(crop_size, param_name="crop_size")
size = size if size is not None else {"height": 224, "width": 224}
size = get_size_dict(size)
self.do_center_crop = do_center_crop
self.crop_size = crop_size
self.do_resize = do_resize
self.size = size
self.resample = resample
self.do_rescale = do_rescale
self.rescale_factor = rescale_factor
self.do_normalize = do_normalize
self.image_mean = image_mean if image_mean is not None else IMAGENET_DEFAULT_MEAN
self.image_std = image_std if image_std is not None else IMAGENET_DEFAULT_STD
def center_crop(
self,
image: np.ndarray,
crop_size: Dict[str, int],
size: Optional[int] = None,
data_format: Optional[Union[str, ChannelDimension]] = None,
input_data_format: Optional[Union[str, ChannelDimension]] = None,
**kwargs,
) -> np.ndarray:
"""
Center crop an image to `(size["height"] / crop_size["height"] * min_dim, size["width"] / crop_size["width"] *
min_dim)`. Where `min_dim = min(size["height"], size["width"])`.
If the input size is smaller than `crop_size` along any edge, the image will be padded with zeros and then
center cropped.
Args:
image (`np.ndarray`):
Image to center crop.
crop_size (`Dict[str, int]`):
Desired output size after applying the center crop.
size (`Dict[str, int]`, *optional*):
Size of the image after resizing. If not provided, the self.size attribute will be used.
data_format (`str` or `ChannelDimension`, *optional*):
The channel dimension format of the image. If not provided, it will be the same as the input image.
input_data_format (`str` or `ChannelDimension`, *optional*):
The channel dimension format of the input image. If not provided, it will be inferred.
"""
size = self.size if size is None else size
size = get_size_dict(size)
crop_size = get_size_dict(crop_size, param_name="crop_size")
height, width = get_image_size(image, channel_dim=input_data_format)
min_dim = min(height, width)
cropped_height = (size["height"] / crop_size["height"]) * min_dim
cropped_width = (size["width"] / crop_size["width"]) * min_dim
return center_crop(
image,
size=(cropped_height, cropped_width),
data_format=data_format,
input_data_format=input_data_format,
**kwargs,
)
# Copied from transformers.models.vit.image_processing_vit.ViTImageProcessor.resize with PILImageResampling.BILINEAR->PILImageResampling.BICUBIC
def resize(
self,
image: np.ndarray,
size: Dict[str, int],
resample: PILImageResampling = PILImageResampling.BICUBIC,
data_format: Optional[Union[str, ChannelDimension]] = None,
input_data_format: Optional[Union[str, ChannelDimension]] = None,
**kwargs,
) -> np.ndarray:
"""
Resize an image to `(size["height"], size["width"])`.
Args:
image (`np.ndarray`):
Image to resize.
size (`Dict[str, int]`):
Dictionary in the format `{"height": int, "width": int}` specifying the size of the output image.
resample (`PILImageResampling`, *optional*, defaults to `PILImageResampling.BICUBIC`):
`PILImageResampling` filter to use when resizing the image e.g. `PILImageResampling.BICUBIC`.
data_format (`ChannelDimension` or `str`, *optional*):
The channel dimension format for the output image. If unset, the channel dimension format of the input
image is used. Can be one of:
- `"channels_first"` or `ChannelDimension.FIRST`: image in (num_channels, height, width) format.
- `"channels_last"` or `ChannelDimension.LAST`: image in (height, width, num_channels) format.
- `"none"` or `ChannelDimension.NONE`: image in (height, width) format.
input_data_format (`ChannelDimension` or `str`, *optional*):
The channel dimension format for the input image. If unset, the channel dimension format is inferred
from the input image. Can be one of:
- `"channels_first"` or `ChannelDimension.FIRST`: image in (num_channels, height, width) format.
- `"channels_last"` or `ChannelDimension.LAST`: image in (height, width, num_channels) format.
- `"none"` or `ChannelDimension.NONE`: image in (height, width) format.
Returns:
`np.ndarray`: The resized image.
"""
size = get_size_dict(size)
if "height" not in size or "width" not in size:
raise ValueError(f"The `size` dictionary must contain the keys `height` and `width`. Got {size.keys()}")
output_size = (size["height"], size["width"])
return resize(
image,
size=output_size,
resample=resample,
data_format=data_format,
input_data_format=input_data_format,
**kwargs,
)
def preprocess(
self,
images: ImageInput,
do_center_crop: Optional[bool] = None,
crop_size: Optional[Dict[str, int]] = None,
do_resize: Optional[bool] = None,
size: Optional[Dict[str, int]] = None,
resample: PILImageResampling = None,
do_rescale: Optional[bool] = None,
rescale_factor: Optional[float] = None,
do_normalize: Optional[bool] = None,
image_mean: Optional[Union[float, List[float]]] = None,
image_std: Optional[Union[float, List[float]]] = None,
return_tensors: Optional[Union[str, TensorType]] = None,
data_format: ChannelDimension = ChannelDimension.FIRST,
input_data_format: Optional[Union[str, ChannelDimension]] = None,
**kwargs,
) -> PIL.Image.Image:
"""
Preprocess an image or batch of images.
Args:
images (`ImageInput`):
Image to preprocess. Expects a single or batch of images with pixel values ranging from 0 to 255. If
passing in images with pixel values between 0 and 1, set `do_rescale=False`.
do_center_crop (`bool`, *optional*, defaults to `self.do_center_crop`):
Whether to center crop the image to `crop_size`.
crop_size (`Dict[str, int]`, *optional*, defaults to `self.crop_size`):
Desired output size after applying the center crop.
do_resize (`bool`, *optional*, defaults to `self.do_resize`):
Whether to resize the image.
size (`Dict[str, int]`, *optional*, defaults to `self.size`):
Size of the image after resizing.
resample (`int`, *optional*, defaults to `self.resample`):
Resampling filter to use if resizing the image. This can be one of the enum `PILImageResampling`, Only
has an effect if `do_resize` is set to `True`.
do_rescale (`bool`, *optional*, defaults to `self.do_rescale`):
Whether to rescale the image.
rescale_factor (`float`, *optional*, defaults to `self.rescale_factor`):
Rescale factor to rescale the image by if `do_rescale` is set to `True`.
do_normalize (`bool`, *optional*, defaults to `self.do_normalize`):
Whether to normalize the image.
image_mean (`float` or `List[float]`, *optional*, defaults to `self.image_mean`):
Image mean.
image_std (`float` or `List[float]`, *optional*, defaults to `self.image_std`):
Image standard deviation.
return_tensors (`str` or `TensorType`, *optional*):
The type of tensors to return. Can be one of:
- Unset: Return a list of `np.ndarray`.
- `TensorType.TENSORFLOW` or `'tf'`: Return a batch of type `tf.Tensor`.
- `TensorType.PYTORCH` or `'pt'`: Return a batch of type `torch.Tensor`.
- `TensorType.NUMPY` or `'np'`: Return a batch of type `np.ndarray`.
- `TensorType.JAX` or `'jax'`: Return a batch of type `jax.numpy.ndarray`.
data_format (`ChannelDimension` or `str`, *optional*, defaults to `ChannelDimension.FIRST`):
The channel dimension format for the output image. Can be one of:
- `ChannelDimension.FIRST`: image in (num_channels, height, width) format.
- `ChannelDimension.LAST`: image in (height, width, num_channels) format.
input_data_format (`ChannelDimension` or `str`, *optional*):
The channel dimension format for the input image. If unset, the channel dimension format is inferred
from the input image. Can be one of:
- `"channels_first"` or `ChannelDimension.FIRST`: image in (num_channels, height, width) format.
- `"channels_last"` or `ChannelDimension.LAST`: image in (height, width, num_channels) format.
- `"none"` or `ChannelDimension.NONE`: image in (height, width) format.
"""
do_center_crop = do_center_crop if do_center_crop is not None else self.do_center_crop
crop_size = crop_size if crop_size is not None else self.crop_size
crop_size = get_size_dict(crop_size, param_name="crop_size")
do_resize = do_resize if do_resize is not None else self.do_resize
size = size if size is not None else self.size
size = get_size_dict(size)
resample = resample if resample is not None else self.resample
do_rescale = do_rescale if do_rescale is not None else self.do_rescale
rescale_factor = rescale_factor if rescale_factor is not None else self.rescale_factor
do_normalize = do_normalize if do_normalize is not None else self.do_normalize
image_mean = image_mean if image_mean is not None else self.image_mean
image_std = image_std if image_std is not None else self.image_std
images = make_list_of_images(images)
if not valid_images(images):
raise ValueError(
"Invalid image type. Must be of type PIL.Image.Image, numpy.ndarray, "
"torch.Tensor, tf.Tensor or jax.ndarray."
)
if do_center_crop and crop_size is None:
raise ValueError("If `do_center_crop` is set to `True`, `crop_size` must be provided.")
if do_resize and size is None:
raise ValueError("Size must be specified if do_resize is True.")
if do_rescale and rescale_factor is None:
raise ValueError("Rescale factor must be specified if do_rescale is True.")
if do_normalize and (image_mean is None or image_std is None):
raise ValueError("Image mean and image standard deviation must be specified if do_normalize is True.")
# All transformations expect numpy arrays.
images = [to_numpy_array(image) for image in images]
if is_scaled_image(images[0]) and do_rescale:
logger.warning_once(
"It looks like you are trying to rescale already rescaled images. If the input"
" images have pixel values between 0 and 1, set `do_rescale=False` to avoid rescaling them again."
)
if input_data_format is None:
# We assume that all images have the same channel dimension format.
input_data_format = infer_channel_dimension_format(images[0])
if do_center_crop:
images = [
self.center_crop(image, crop_size, size=size, input_data_format=input_data_format) for image in images
]
if do_resize:
images = [
self.resize(image=image, size=size, resample=resample, input_data_format=input_data_format)
for image in images
]
if do_rescale:
images = [
self.rescale(image=image, scale=rescale_factor, input_data_format=input_data_format)
for image in images
]
if do_normalize:
images = [
self.normalize(image=image, mean=image_mean, std=image_std, input_data_format=input_data_format)
for image in images
]
images = [
to_channel_dimension_format(image, data_format, input_channel_dim=input_data_format) for image in images
]
data = {"pixel_values": images}
return BatchFeature(data=data, tensor_type=return_tensors)
| transformers/src/transformers/models/perceiver/image_processing_perceiver.py/0 | {
"file_path": "transformers/src/transformers/models/perceiver/image_processing_perceiver.py",
"repo_id": "transformers",
"token_count": 7364
} | 352 |
# coding=utf-8
# Copyright 2023 The HuggingFace Inc. team. All rights reserved.
#
# Licensed under the Apache License, Version 2.0 (the "License");
# you may not use this file except in compliance with the License.
# You may obtain a copy of the License at
#
# http://www.apache.org/licenses/LICENSE-2.0
#
# Unless required by applicable law or agreed to in writing, software
# distributed under the License is distributed on an "AS IS" BASIS,
# WITHOUT WARRANTIES OR CONDITIONS OF ANY KIND, either express or implied.
# See the License for the specific language governing permissions and
# limitations under the License.
"""Image processor class for Pix2Struct."""
import io
import math
from typing import Dict, Optional, Union
import numpy as np
from huggingface_hub import hf_hub_download
from ...image_processing_utils import BaseImageProcessor, BatchFeature
from ...image_transforms import convert_to_rgb, normalize, to_channel_dimension_format, to_pil_image
from ...image_utils import (
ChannelDimension,
ImageInput,
get_image_size,
infer_channel_dimension_format,
make_list_of_images,
to_numpy_array,
valid_images,
)
from ...utils import TensorType, is_torch_available, is_vision_available, logging
from ...utils.import_utils import requires_backends
if is_vision_available():
import textwrap
from PIL import Image, ImageDraw, ImageFont
if is_torch_available():
import torch
logger = logging.get_logger(__name__)
DEFAULT_FONT_PATH = "ybelkada/fonts"
# adapted from: https://discuss.pytorch.org/t/tf-image-extract-patches-in-pytorch/171409/2
def torch_extract_patches(image_tensor, patch_height, patch_width):
"""
Utiliy function to extract patches from a given image tensor. Returns a tensor of shape (1, `patch_height`,
`patch_width`, `num_channels`x `patch_height` x `patch_width`)
Args:
image_tensor (torch.Tensor):
The image tensor to extract patches from.
patch_height (int):
The height of the patches to extract.
patch_width (int):
The width of the patches to extract.
"""
requires_backends(torch_extract_patches, ["torch"])
image_tensor = image_tensor.unsqueeze(0)
patches = torch.nn.functional.unfold(image_tensor, (patch_height, patch_width), stride=(patch_height, patch_width))
patches = patches.reshape(image_tensor.size(0), image_tensor.size(1), patch_height, patch_width, -1)
patches = patches.permute(0, 4, 2, 3, 1).reshape(
image_tensor.size(2) // patch_height,
image_tensor.size(3) // patch_width,
image_tensor.size(1) * patch_height * patch_width,
)
return patches.unsqueeze(0)
# Adapted from https://github.com/google-research/pix2struct/blob/0e1779af0f4db4b652c1d92b3bbd2550a7399123/pix2struct/preprocessing/preprocessing_utils.py#L106
def render_text(
text: str,
text_size: int = 36,
text_color: str = "black",
background_color: str = "white",
left_padding: int = 5,
right_padding: int = 5,
top_padding: int = 5,
bottom_padding: int = 5,
font_bytes: Optional[bytes] = None,
font_path: Optional[str] = None,
) -> Image.Image:
"""
Render text. This script is entirely adapted from the original script that can be found here:
https://github.com/google-research/pix2struct/blob/main/pix2struct/preprocessing/preprocessing_utils.py
Args:
text (`str`, *optional*, defaults to ):
Text to render.
text_size (`int`, *optional*, defaults to 36):
Size of the text.
text_color (`str`, *optional*, defaults to `"black"`):
Color of the text.
background_color (`str`, *optional*, defaults to `"white"`):
Color of the background.
left_padding (`int`, *optional*, defaults to 5):
Padding on the left.
right_padding (`int`, *optional*, defaults to 5):
Padding on the right.
top_padding (`int`, *optional*, defaults to 5):
Padding on the top.
bottom_padding (`int`, *optional*, defaults to 5):
Padding on the bottom.
font_bytes (`bytes`, *optional*):
Bytes of the font to use. If `None`, the default font will be used.
font_path (`str`, *optional*):
Path to the font to use. If `None`, the default font will be used.
"""
requires_backends(render_text, "vision")
# Add new lines so that each line is no more than 80 characters.
wrapper = textwrap.TextWrapper(width=80)
lines = wrapper.wrap(text=text)
wrapped_text = "\n".join(lines)
if font_bytes is not None and font_path is None:
font = io.BytesIO(font_bytes)
elif font_path is not None:
font = font_path
else:
font = hf_hub_download(DEFAULT_FONT_PATH, "Arial.TTF")
font = ImageFont.truetype(font, encoding="UTF-8", size=text_size)
# Use a temporary canvas to determine the width and height in pixels when
# rendering the text.
temp_draw = ImageDraw.Draw(Image.new("RGB", (1, 1), background_color))
_, _, text_width, text_height = temp_draw.textbbox((0, 0), wrapped_text, font)
# Create the actual image with a bit of padding around the text.
image_width = text_width + left_padding + right_padding
image_height = text_height + top_padding + bottom_padding
image = Image.new("RGB", (image_width, image_height), background_color)
draw = ImageDraw.Draw(image)
draw.text(xy=(left_padding, top_padding), text=wrapped_text, fill=text_color, font=font)
return image
# Adapted from https://github.com/google-research/pix2struct/blob/0e1779af0f4db4b652c1d92b3bbd2550a7399123/pix2struct/preprocessing/preprocessing_utils.py#L87
def render_header(
image: np.ndarray, header: str, input_data_format: Optional[Union[str, ChildProcessError]] = None, **kwargs
):
"""
Renders the input text as a header on the input image.
Args:
image (`np.ndarray`):
The image to render the header on.
header (`str`):
The header text.
data_format (`Union[ChannelDimension, str]`, *optional*):
The data format of the image. Can be either "ChannelDimension.channels_first" or
"ChannelDimension.channels_last".
Returns:
`np.ndarray`: The image with the header rendered.
"""
requires_backends(render_header, "vision")
# Convert to PIL image if necessary
image = to_pil_image(image, input_data_format=input_data_format)
header_image = render_text(header, **kwargs)
new_width = max(header_image.width, image.width)
new_height = int(image.height * (new_width / image.width))
new_header_height = int(header_image.height * (new_width / header_image.width))
new_image = Image.new("RGB", (new_width, new_height + new_header_height), "white")
new_image.paste(header_image.resize((new_width, new_header_height)), (0, 0))
new_image.paste(image.resize((new_width, new_height)), (0, new_header_height))
# Convert back to the original framework if necessary
new_image = to_numpy_array(new_image)
if infer_channel_dimension_format(new_image) == ChannelDimension.LAST:
new_image = to_channel_dimension_format(new_image, ChannelDimension.LAST)
return new_image
class Pix2StructImageProcessor(BaseImageProcessor):
r"""
Constructs a Pix2Struct image processor.
Args:
do_convert_rgb (`bool`, *optional*, defaults to `True`):
Whether to convert the image to RGB.
do_normalize (`bool`, *optional*, defaults to `True`):
Whether to normalize the image. Can be overridden by the `do_normalize` parameter in the `preprocess`
method. According to Pix2Struct paper and code, the image is normalized with its own mean and standard
deviation.
patch_size (`Dict[str, int]`, *optional*, defaults to `{"height": 16, "width": 16}`):
The patch size to use for the image. According to Pix2Struct paper and code, the patch size is 16x16.
max_patches (`int`, *optional*, defaults to 2048):
The maximum number of patches to extract from the image as per the [Pix2Struct
paper](https://arxiv.org/pdf/2210.03347.pdf).
is_vqa (`bool`, *optional*, defaults to `False`):
Whether or not the image processor is for the VQA task. If `True` and `header_text` is passed in, text is
rendered onto the input images.
"""
model_input_names = ["flattened_patches"]
def __init__(
self,
do_convert_rgb: bool = True,
do_normalize: bool = True,
patch_size: Dict[str, int] = None,
max_patches: int = 2048,
is_vqa: bool = False,
**kwargs,
) -> None:
super().__init__(**kwargs)
self.patch_size = patch_size if patch_size is not None else {"height": 16, "width": 16}
self.do_normalize = do_normalize
self.do_convert_rgb = do_convert_rgb
self.max_patches = max_patches
self.is_vqa = is_vqa
def extract_flattened_patches(
self,
image: np.ndarray,
max_patches: int,
patch_size: dict,
input_data_format: Optional[Union[str, ChannelDimension]] = None,
**kwargs,
) -> np.ndarray:
"""
Extract flattened patches from an image.
Args:
image (`np.ndarray`):
Image to extract flattened patches from.
max_patches (`int`):
Maximum number of patches to extract.
patch_size (`dict`):
Dictionary containing the patch height and width.
Returns:
result (`np.ndarray`):
A sequence of `max_patches` flattened patches.
"""
requires_backends(self.extract_flattened_patches, "torch")
# convert to torch
image = to_channel_dimension_format(image, ChannelDimension.FIRST, input_data_format)
image = torch.from_numpy(image)
patch_height, patch_width = patch_size["height"], patch_size["width"]
image_height, image_width = get_image_size(image, ChannelDimension.FIRST)
# maximize scale s.t.
scale = math.sqrt(max_patches * (patch_height / image_height) * (patch_width / image_width))
num_feasible_rows = max(min(math.floor(scale * image_height / patch_height), max_patches), 1)
num_feasible_cols = max(min(math.floor(scale * image_width / patch_width), max_patches), 1)
resized_height = max(num_feasible_rows * patch_height, 1)
resized_width = max(num_feasible_cols * patch_width, 1)
image = torch.nn.functional.interpolate(
image.unsqueeze(0),
size=(resized_height, resized_width),
mode="bilinear",
align_corners=False,
antialias=True,
).squeeze(0)
# [1, rows, columns, patch_height * patch_width * image_channels]
patches = torch_extract_patches(image, patch_height, patch_width)
patches_shape = patches.shape
rows = patches_shape[1]
columns = patches_shape[2]
depth = patches_shape[3]
# [rows * columns, patch_height * patch_width * image_channels]
patches = patches.reshape([rows * columns, depth])
# [rows * columns, 1]
row_ids = torch.arange(rows).reshape([rows, 1]).repeat(1, columns).reshape([rows * columns, 1])
col_ids = torch.arange(columns).reshape([1, columns]).repeat(rows, 1).reshape([rows * columns, 1])
# Offset by 1 so the ids do not contain zeros, which represent padding.
row_ids += 1
col_ids += 1
# Prepare additional patch features.
# [rows * columns, 1]
row_ids = row_ids.to(torch.float32)
col_ids = col_ids.to(torch.float32)
# [rows * columns, 2 + patch_height * patch_width * image_channels]
result = torch.cat([row_ids, col_ids, patches], -1)
# [max_patches, 2 + patch_height * patch_width * image_channels]
result = torch.nn.functional.pad(result, [0, 0, 0, max_patches - (rows * columns)]).float()
result = to_numpy_array(result)
return result
def normalize(
self,
image: np.ndarray,
data_format: Optional[Union[str, ChannelDimension]] = None,
input_data_format: Optional[Union[str, ChannelDimension]] = None,
**kwargs,
) -> np.ndarray:
"""
Normalize an image. image = (image - image_mean) / image_std.
The image std is to mimic the tensorflow implementation of the `per_image_standardization`:
https://www.tensorflow.org/api_docs/python/tf/image/per_image_standardization
Args:
image (`np.ndarray`):
Image to normalize.
data_format (`str` or `ChannelDimension`, *optional*):
The channel dimension format for the output image. If unset, the channel dimension format of the input
image is used.
input_data_format (`str` or `ChannelDimension`, *optional*):
The channel dimension format of the input image. If not provided, it will be inferred.
"""
if image.dtype == np.uint8:
image = image.astype(np.float32)
# take mean across the whole `image`
mean = np.mean(image)
std = np.std(image)
adjusted_stddev = max(std, 1.0 / math.sqrt(np.prod(image.shape)))
return normalize(
image,
mean=mean,
std=adjusted_stddev,
data_format=data_format,
input_data_format=input_data_format,
**kwargs,
)
def preprocess(
self,
images: ImageInput,
header_text: Optional[str] = None,
do_convert_rgb: bool = None,
do_normalize: Optional[bool] = None,
max_patches: Optional[int] = None,
patch_size: Optional[Dict[str, int]] = None,
return_tensors: Optional[Union[str, TensorType]] = None,
data_format: ChannelDimension = ChannelDimension.FIRST,
input_data_format: Optional[Union[str, ChannelDimension]] = None,
**kwargs,
) -> ImageInput:
"""
Preprocess an image or batch of images. The processor first computes the maximum possible number of
aspect-ratio preserving patches of size `patch_size` that can be extracted from the image. It then pads the
image with zeros to make the image respect the constraint of `max_patches`. Before extracting the patches the
images are standardized following the tensorflow implementation of `per_image_standardization`
(https://www.tensorflow.org/api_docs/python/tf/image/per_image_standardization).
Args:
images (`ImageInput`):
Image to preprocess. Expects a single or batch of images.
header_text (`Union[List[str], str]`, *optional*):
Text to render as a header. Only has an effect if `image_processor.is_vqa` is `True`.
do_convert_rgb (`bool`, *optional*, defaults to `self.do_convert_rgb`):
Whether to convert the image to RGB.
do_normalize (`bool`, *optional*, defaults to `self.do_normalize`):
Whether to normalize the image.
max_patches (`int`, *optional*, defaults to `self.max_patches`):
Maximum number of patches to extract.
patch_size (`dict`, *optional*, defaults to `self.patch_size`):
Dictionary containing the patch height and width.
return_tensors (`str` or `TensorType`, *optional*):
The type of tensors to return. Can be one of:
- Unset: Return a list of `np.ndarray`.
- `TensorType.TENSORFLOW` or `'tf'`: Return a batch of type `tf.Tensor`.
- `TensorType.PYTORCH` or `'pt'`: Return a batch of type `torch.Tensor`.
- `TensorType.NUMPY` or `'np'`: Return a batch of type `np.ndarray`.
- `TensorType.JAX` or `'jax'`: Return a batch of type `jax.numpy.ndarray`.
data_format (`ChannelDimension` or `str`, *optional*, defaults to `ChannelDimension.FIRST`):
The channel dimension format for the output image. Can be one of:
- `"channels_first"` or `ChannelDimension.FIRST`: image in (num_channels, height, width) format.
- `"channels_last"` or `ChannelDimension.LAST`: image in (height, width, num_channels) format.
- Unset: Use the channel dimension format of the input image.
input_data_format (`ChannelDimension` or `str`, *optional*):
The channel dimension format for the input image. If unset, the channel dimension format is inferred
from the input image. Can be one of:
- `"channels_first"` or `ChannelDimension.FIRST`: image in (num_channels, height, width) format.
- `"channels_last"` or `ChannelDimension.LAST`: image in (height, width, num_channels) format.
- `"none"` or `ChannelDimension.NONE`: image in (height, width) format.
"""
do_normalize = do_normalize if do_normalize is not None else self.do_normalize
do_convert_rgb = do_convert_rgb if do_convert_rgb is not None else self.do_convert_rgb
patch_size = patch_size if patch_size is not None else self.patch_size
max_patches = max_patches if max_patches is not None else self.max_patches
is_vqa = self.is_vqa
if kwargs.get("data_format", None) is not None:
raise ValueError("data_format is not an accepted input as the outputs are ")
images = make_list_of_images(images)
if not valid_images(images):
raise ValueError(
"Invalid image type. Must be of type PIL.Image.Image, numpy.ndarray, "
"torch.Tensor, tf.Tensor or jax.ndarray."
)
# PIL RGBA images are converted to RGB
if do_convert_rgb:
images = [convert_to_rgb(image) for image in images]
# All transformations expect numpy arrays.
images = [to_numpy_array(image) for image in images]
if input_data_format is None:
# We assume that all images have the same channel dimension format.
input_data_format = infer_channel_dimension_format(images[0])
if is_vqa:
if header_text is None:
raise ValueError("A header text must be provided for VQA models.")
font_bytes = kwargs.pop("font_bytes", None)
font_path = kwargs.pop("font_path", None)
if isinstance(header_text, str):
header_text = [header_text] * len(images)
images = [
render_header(image, header_text[i], font_bytes=font_bytes, font_path=font_path)
for i, image in enumerate(images)
]
if do_normalize:
images = [self.normalize(image=image, input_data_format=input_data_format) for image in images]
# convert to torch tensor and permute
images = [
self.extract_flattened_patches(
image=image, max_patches=max_patches, patch_size=patch_size, input_data_format=input_data_format
)
for image in images
]
# create attention mask in numpy
attention_masks = [(image.sum(axis=-1) != 0).astype(np.float32) for image in images]
encoded_outputs = BatchFeature(
data={"flattened_patches": images, "attention_mask": attention_masks}, tensor_type=return_tensors
)
return encoded_outputs
| transformers/src/transformers/models/pix2struct/image_processing_pix2struct.py/0 | {
"file_path": "transformers/src/transformers/models/pix2struct/image_processing_pix2struct.py",
"repo_id": "transformers",
"token_count": 8161
} | 353 |
# Copyright 2023 The HuggingFace Inc. team. All rights reserved.
#
# Licensed under the Apache License, Version 2.0 (the "License");
# you may not use this file except in compliance with the License.
# You may obtain a copy of the License at
#
# http://www.apache.org/licenses/LICENSE-2.0
#
# Unless required by applicable law or agreed to in writing, software
# distributed under the License is distributed on an "AS IS" BASIS,
# WITHOUT WARRANTIES OR CONDITIONS OF ANY KIND, either express or implied.
# See the License for the specific language governing permissions and
# limitations under the License.
""" File for loading the Pop2Piano model weights from the official repository and to show how tokenizer vocab was
constructed"""
import json
import torch
from transformers import Pop2PianoConfig, Pop2PianoForConditionalGeneration
########################## MODEL WEIGHTS ##########################
# This weights were downloaded from the official pop2piano repository
# https://huggingface.co/sweetcocoa/pop2piano/blob/main/model-1999-val_0.67311615.ckpt
official_weights = torch.load("./model-1999-val_0.67311615.ckpt")
state_dict = {}
# load the config and init the model
cfg = Pop2PianoConfig.from_pretrained("sweetcocoa/pop2piano")
model = Pop2PianoForConditionalGeneration(cfg)
# load relative attention bias
state_dict["encoder.block.0.layer.0.SelfAttention.relative_attention_bias.weight"] = official_weights["state_dict"][
"transformer.encoder.block.0.layer.0.SelfAttention.relative_attention_bias.weight"
]
state_dict["decoder.block.0.layer.0.SelfAttention.relative_attention_bias.weight"] = official_weights["state_dict"][
"transformer.decoder.block.0.layer.0.SelfAttention.relative_attention_bias.weight"
]
# load embed tokens and final layer norm for both encoder and decoder
state_dict["encoder.embed_tokens.weight"] = official_weights["state_dict"]["transformer.encoder.embed_tokens.weight"]
state_dict["decoder.embed_tokens.weight"] = official_weights["state_dict"]["transformer.decoder.embed_tokens.weight"]
state_dict["encoder.final_layer_norm.weight"] = official_weights["state_dict"][
"transformer.encoder.final_layer_norm.weight"
]
state_dict["decoder.final_layer_norm.weight"] = official_weights["state_dict"][
"transformer.decoder.final_layer_norm.weight"
]
# load lm_head, mel_conditioner.emb and shared
state_dict["lm_head.weight"] = official_weights["state_dict"]["transformer.lm_head.weight"]
state_dict["mel_conditioner.embedding.weight"] = official_weights["state_dict"]["mel_conditioner.embedding.weight"]
state_dict["shared.weight"] = official_weights["state_dict"]["transformer.shared.weight"]
# load each encoder blocks
for i in range(cfg.num_layers):
# layer 0
state_dict[f"encoder.block.{i}.layer.0.SelfAttention.q.weight"] = official_weights["state_dict"][
f"transformer.encoder.block.{i}.layer.0.SelfAttention.q.weight"
]
state_dict[f"encoder.block.{i}.layer.0.SelfAttention.k.weight"] = official_weights["state_dict"][
f"transformer.encoder.block.{i}.layer.0.SelfAttention.k.weight"
]
state_dict[f"encoder.block.{i}.layer.0.SelfAttention.v.weight"] = official_weights["state_dict"][
f"transformer.encoder.block.{i}.layer.0.SelfAttention.v.weight"
]
state_dict[f"encoder.block.{i}.layer.0.SelfAttention.o.weight"] = official_weights["state_dict"][
f"transformer.encoder.block.{i}.layer.0.SelfAttention.o.weight"
]
state_dict[f"encoder.block.{i}.layer.0.layer_norm.weight"] = official_weights["state_dict"][
f"transformer.encoder.block.{i}.layer.0.layer_norm.weight"
]
# layer 1
state_dict[f"encoder.block.{i}.layer.1.DenseReluDense.wi_0.weight"] = official_weights["state_dict"][
f"transformer.encoder.block.{i}.layer.1.DenseReluDense.wi_0.weight"
]
state_dict[f"encoder.block.{i}.layer.1.DenseReluDense.wi_1.weight"] = official_weights["state_dict"][
f"transformer.encoder.block.{i}.layer.1.DenseReluDense.wi_1.weight"
]
state_dict[f"encoder.block.{i}.layer.1.DenseReluDense.wo.weight"] = official_weights["state_dict"][
f"transformer.encoder.block.{i}.layer.1.DenseReluDense.wo.weight"
]
state_dict[f"encoder.block.{i}.layer.1.layer_norm.weight"] = official_weights["state_dict"][
f"transformer.encoder.block.{i}.layer.1.layer_norm.weight"
]
# load each decoder blocks
for i in range(6):
# layer 0
state_dict[f"decoder.block.{i}.layer.0.SelfAttention.q.weight"] = official_weights["state_dict"][
f"transformer.decoder.block.{i}.layer.0.SelfAttention.q.weight"
]
state_dict[f"decoder.block.{i}.layer.0.SelfAttention.k.weight"] = official_weights["state_dict"][
f"transformer.decoder.block.{i}.layer.0.SelfAttention.k.weight"
]
state_dict[f"decoder.block.{i}.layer.0.SelfAttention.v.weight"] = official_weights["state_dict"][
f"transformer.decoder.block.{i}.layer.0.SelfAttention.v.weight"
]
state_dict[f"decoder.block.{i}.layer.0.SelfAttention.o.weight"] = official_weights["state_dict"][
f"transformer.decoder.block.{i}.layer.0.SelfAttention.o.weight"
]
state_dict[f"decoder.block.{i}.layer.0.layer_norm.weight"] = official_weights["state_dict"][
f"transformer.decoder.block.{i}.layer.0.layer_norm.weight"
]
# layer 1
state_dict[f"decoder.block.{i}.layer.1.EncDecAttention.q.weight"] = official_weights["state_dict"][
f"transformer.decoder.block.{i}.layer.1.EncDecAttention.q.weight"
]
state_dict[f"decoder.block.{i}.layer.1.EncDecAttention.k.weight"] = official_weights["state_dict"][
f"transformer.decoder.block.{i}.layer.1.EncDecAttention.k.weight"
]
state_dict[f"decoder.block.{i}.layer.1.EncDecAttention.v.weight"] = official_weights["state_dict"][
f"transformer.decoder.block.{i}.layer.1.EncDecAttention.v.weight"
]
state_dict[f"decoder.block.{i}.layer.1.EncDecAttention.o.weight"] = official_weights["state_dict"][
f"transformer.decoder.block.{i}.layer.1.EncDecAttention.o.weight"
]
state_dict[f"decoder.block.{i}.layer.1.layer_norm.weight"] = official_weights["state_dict"][
f"transformer.decoder.block.{i}.layer.1.layer_norm.weight"
]
# layer 2
state_dict[f"decoder.block.{i}.layer.2.DenseReluDense.wi_0.weight"] = official_weights["state_dict"][
f"transformer.decoder.block.{i}.layer.2.DenseReluDense.wi_0.weight"
]
state_dict[f"decoder.block.{i}.layer.2.DenseReluDense.wi_1.weight"] = official_weights["state_dict"][
f"transformer.decoder.block.{i}.layer.2.DenseReluDense.wi_1.weight"
]
state_dict[f"decoder.block.{i}.layer.2.DenseReluDense.wo.weight"] = official_weights["state_dict"][
f"transformer.decoder.block.{i}.layer.2.DenseReluDense.wo.weight"
]
state_dict[f"decoder.block.{i}.layer.2.layer_norm.weight"] = official_weights["state_dict"][
f"transformer.decoder.block.{i}.layer.2.layer_norm.weight"
]
model.load_state_dict(state_dict, strict=True)
# save the weights
torch.save(state_dict, "./pytorch_model.bin")
########################## TOKENIZER ##########################
# the tokenize and detokenize methods are taken from the official implementation
# link : https://github.com/sweetcocoa/pop2piano/blob/fac11e8dcfc73487513f4588e8d0c22a22f2fdc5/midi_tokenizer.py#L34
def tokenize(idx, token_type, n_special=4, n_note=128, n_velocity=2):
if token_type == "TOKEN_TIME":
return n_special + n_note + n_velocity + idx
elif token_type == "TOKEN_VELOCITY":
return n_special + n_note + idx
elif token_type == "TOKEN_NOTE":
return n_special + idx
elif token_type == "TOKEN_SPECIAL":
return idx
else:
return -1
# link : https://github.com/sweetcocoa/pop2piano/blob/fac11e8dcfc73487513f4588e8d0c22a22f2fdc5/midi_tokenizer.py#L48
def detokenize(idx, n_special=4, n_note=128, n_velocity=2, time_idx_offset=0):
if idx >= n_special + n_note + n_velocity:
return "TOKEN_TIME", (idx - (n_special + n_note + n_velocity)) + time_idx_offset
elif idx >= n_special + n_note:
return "TOKEN_VELOCITY", idx - (n_special + n_note)
elif idx >= n_special:
return "TOKEN_NOTE", idx - n_special
else:
return "TOKEN_SPECIAL", idx
# crate the decoder and then the encoder of the tokenizer
decoder = {}
for i in range(cfg.vocab_size):
decoder.update({i: f"{detokenize(i)[1]}_{detokenize(i)[0]}"})
encoder = {v: k for k, v in decoder.items()}
# save the vocab
with open("./vocab.json", "w") as file:
file.write(json.dumps(encoder))
| transformers/src/transformers/models/pop2piano/convert_pop2piano_weights_to_hf.py/0 | {
"file_path": "transformers/src/transformers/models/pop2piano/convert_pop2piano_weights_to_hf.py",
"repo_id": "transformers",
"token_count": 3446
} | 354 |
# coding=utf-8
# Copyright 2022 The REALM authors and The HuggingFace Inc. team.
#
# Licensed under the Apache License, Version 2.0 (the "License");
# you may not use this file except in compliance with the License.
# You may obtain a copy of the License at
#
# http://www.apache.org/licenses/LICENSE-2.0
#
# Unless required by applicable law or agreed to in writing, software
# distributed under the License is distributed on an "AS IS" BASIS,
# WITHOUT WARRANTIES OR CONDITIONS OF ANY KIND, either express or implied.
# See the License for the specific language governing permissions and
# limitations under the License.
"""REALM Retriever model implementation."""
import os
from typing import Optional, Union
import numpy as np
from huggingface_hub import hf_hub_download
from ... import AutoTokenizer
from ...utils import logging
_REALM_BLOCK_RECORDS_FILENAME = "block_records.npy"
logger = logging.get_logger(__name__)
def convert_tfrecord_to_np(block_records_path: str, num_block_records: int) -> np.ndarray:
import tensorflow.compat.v1 as tf
blocks_dataset = tf.data.TFRecordDataset(block_records_path, buffer_size=512 * 1024 * 1024)
blocks_dataset = blocks_dataset.batch(num_block_records, drop_remainder=True)
np_record = next(blocks_dataset.take(1).as_numpy_iterator())
return np_record
class ScaNNSearcher:
"""Note that ScaNNSearcher cannot currently be used within the model. In future versions, it might however be included."""
def __init__(
self,
db,
num_neighbors,
dimensions_per_block=2,
num_leaves=1000,
num_leaves_to_search=100,
training_sample_size=100000,
):
"""Build scann searcher."""
from scann.scann_ops.py.scann_ops_pybind import builder as Builder
builder = Builder(db=db, num_neighbors=num_neighbors, distance_measure="dot_product")
builder = builder.tree(
num_leaves=num_leaves, num_leaves_to_search=num_leaves_to_search, training_sample_size=training_sample_size
)
builder = builder.score_ah(dimensions_per_block=dimensions_per_block)
self.searcher = builder.build()
def search_batched(self, question_projection):
retrieved_block_ids, _ = self.searcher.search_batched(question_projection.detach().cpu())
return retrieved_block_ids.astype("int64")
class RealmRetriever:
"""The retriever of REALM outputting the retrieved evidence block and whether the block has answers as well as answer
positions."
Parameters:
block_records (`np.ndarray`):
A numpy array which cantains evidence texts.
tokenizer ([`RealmTokenizer`]):
The tokenizer to encode retrieved texts.
"""
def __init__(self, block_records, tokenizer):
super().__init__()
self.block_records = block_records
self.tokenizer = tokenizer
def __call__(self, retrieved_block_ids, question_input_ids, answer_ids, max_length=None, return_tensors="pt"):
retrieved_blocks = np.take(self.block_records, indices=retrieved_block_ids, axis=0)
question = self.tokenizer.decode(question_input_ids[0], skip_special_tokens=True)
text = []
text_pair = []
for retrieved_block in retrieved_blocks:
text.append(question)
text_pair.append(retrieved_block.decode())
concat_inputs = self.tokenizer(
text, text_pair, padding=True, truncation=True, return_special_tokens_mask=True, max_length=max_length
)
concat_inputs_tensors = concat_inputs.convert_to_tensors(return_tensors)
if answer_ids is not None:
return self.block_has_answer(concat_inputs, answer_ids) + (concat_inputs_tensors,)
else:
return (None, None, None, concat_inputs_tensors)
@classmethod
def from_pretrained(cls, pretrained_model_name_or_path: Optional[Union[str, os.PathLike]], *init_inputs, **kwargs):
if os.path.isdir(pretrained_model_name_or_path):
block_records_path = os.path.join(pretrained_model_name_or_path, _REALM_BLOCK_RECORDS_FILENAME)
else:
block_records_path = hf_hub_download(
repo_id=pretrained_model_name_or_path, filename=_REALM_BLOCK_RECORDS_FILENAME, **kwargs
)
block_records = np.load(block_records_path, allow_pickle=True)
tokenizer = AutoTokenizer.from_pretrained(pretrained_model_name_or_path, *init_inputs, **kwargs)
return cls(block_records, tokenizer)
def save_pretrained(self, save_directory):
# save block records
np.save(os.path.join(save_directory, _REALM_BLOCK_RECORDS_FILENAME), self.block_records)
# save tokenizer
self.tokenizer.save_pretrained(save_directory)
def block_has_answer(self, concat_inputs, answer_ids):
"""check if retrieved_blocks has answers."""
has_answers = []
start_pos = []
end_pos = []
max_answers = 0
for input_id in concat_inputs.input_ids:
input_id_list = input_id.tolist()
# Check answers between two [SEP] tokens
first_sep_idx = input_id_list.index(self.tokenizer.sep_token_id)
second_sep_idx = first_sep_idx + 1 + input_id_list[first_sep_idx + 1 :].index(self.tokenizer.sep_token_id)
start_pos.append([])
end_pos.append([])
for answer in answer_ids:
for idx in range(first_sep_idx + 1, second_sep_idx):
if answer[0] == input_id_list[idx]:
if input_id_list[idx : idx + len(answer)] == answer:
start_pos[-1].append(idx)
end_pos[-1].append(idx + len(answer) - 1)
if len(start_pos[-1]) == 0:
has_answers.append(False)
else:
has_answers.append(True)
if len(start_pos[-1]) > max_answers:
max_answers = len(start_pos[-1])
# Pad -1 to max_answers
for start_pos_, end_pos_ in zip(start_pos, end_pos):
if len(start_pos_) < max_answers:
padded = [-1] * (max_answers - len(start_pos_))
start_pos_ += padded
end_pos_ += padded
return has_answers, start_pos, end_pos
| transformers/src/transformers/models/realm/retrieval_realm.py/0 | {
"file_path": "transformers/src/transformers/models/realm/retrieval_realm.py",
"repo_id": "transformers",
"token_count": 2788
} | 355 |
# Copyright 2020 The HuggingFace Team. All rights reserved.
#
# Licensed under the Apache License, Version 2.0 (the "License");
# you may not use this file except in compliance with the License.
# You may obtain a copy of the License at
#
# http://www.apache.org/licenses/LICENSE-2.0
#
# Unless required by applicable law or agreed to in writing, software
# distributed under the License is distributed on an "AS IS" BASIS,
# WITHOUT WARRANTIES OR CONDITIONS OF ANY KIND, either express or implied.
# See the License for the specific language governing permissions and
# limitations under the License.
from typing import TYPE_CHECKING
from ...utils import (
OptionalDependencyNotAvailable,
_LazyModule,
is_sentencepiece_available,
is_tf_available,
is_tokenizers_available,
is_torch_available,
)
_import_structure = {
"configuration_rembert": ["REMBERT_PRETRAINED_CONFIG_ARCHIVE_MAP", "RemBertConfig", "RemBertOnnxConfig"]
}
try:
if not is_sentencepiece_available():
raise OptionalDependencyNotAvailable()
except OptionalDependencyNotAvailable:
pass
else:
_import_structure["tokenization_rembert"] = ["RemBertTokenizer"]
try:
if not is_tokenizers_available():
raise OptionalDependencyNotAvailable()
except OptionalDependencyNotAvailable:
pass
else:
_import_structure["tokenization_rembert_fast"] = ["RemBertTokenizerFast"]
try:
if not is_torch_available():
raise OptionalDependencyNotAvailable()
except OptionalDependencyNotAvailable:
pass
else:
_import_structure["modeling_rembert"] = [
"REMBERT_PRETRAINED_MODEL_ARCHIVE_LIST",
"RemBertForCausalLM",
"RemBertForMaskedLM",
"RemBertForMultipleChoice",
"RemBertForQuestionAnswering",
"RemBertForSequenceClassification",
"RemBertForTokenClassification",
"RemBertLayer",
"RemBertModel",
"RemBertPreTrainedModel",
"load_tf_weights_in_rembert",
]
try:
if not is_tf_available():
raise OptionalDependencyNotAvailable()
except OptionalDependencyNotAvailable:
pass
else:
_import_structure["modeling_tf_rembert"] = [
"TF_REMBERT_PRETRAINED_MODEL_ARCHIVE_LIST",
"TFRemBertForCausalLM",
"TFRemBertForMaskedLM",
"TFRemBertForMultipleChoice",
"TFRemBertForQuestionAnswering",
"TFRemBertForSequenceClassification",
"TFRemBertForTokenClassification",
"TFRemBertLayer",
"TFRemBertModel",
"TFRemBertPreTrainedModel",
]
if TYPE_CHECKING:
from .configuration_rembert import REMBERT_PRETRAINED_CONFIG_ARCHIVE_MAP, RemBertConfig, RemBertOnnxConfig
try:
if not is_sentencepiece_available():
raise OptionalDependencyNotAvailable()
except OptionalDependencyNotAvailable:
pass
else:
from .tokenization_rembert import RemBertTokenizer
try:
if not is_tokenizers_available():
raise OptionalDependencyNotAvailable()
except OptionalDependencyNotAvailable:
pass
else:
from .tokenization_rembert_fast import RemBertTokenizerFast
try:
if not is_torch_available():
raise OptionalDependencyNotAvailable()
except OptionalDependencyNotAvailable:
pass
else:
from .modeling_rembert import (
REMBERT_PRETRAINED_MODEL_ARCHIVE_LIST,
RemBertForCausalLM,
RemBertForMaskedLM,
RemBertForMultipleChoice,
RemBertForQuestionAnswering,
RemBertForSequenceClassification,
RemBertForTokenClassification,
RemBertLayer,
RemBertModel,
RemBertPreTrainedModel,
load_tf_weights_in_rembert,
)
try:
if not is_tf_available():
raise OptionalDependencyNotAvailable()
except OptionalDependencyNotAvailable:
pass
else:
from .modeling_tf_rembert import (
TF_REMBERT_PRETRAINED_MODEL_ARCHIVE_LIST,
TFRemBertForCausalLM,
TFRemBertForMaskedLM,
TFRemBertForMultipleChoice,
TFRemBertForQuestionAnswering,
TFRemBertForSequenceClassification,
TFRemBertForTokenClassification,
TFRemBertLayer,
TFRemBertModel,
TFRemBertPreTrainedModel,
)
else:
import sys
sys.modules[__name__] = _LazyModule(__name__, globals()["__file__"], _import_structure, module_spec=__spec__)
| transformers/src/transformers/models/rembert/__init__.py/0 | {
"file_path": "transformers/src/transformers/models/rembert/__init__.py",
"repo_id": "transformers",
"token_count": 1913
} | 356 |
# coding=utf-8
# Copyright 2021 The Google Flax Team Authors and The HuggingFace Inc. team.
#
# Licensed under the Apache License, Version 2.0 (the "License");
# you may not use this file except in compliance with the License.
# You may obtain a copy of the License at
#
# http://www.apache.org/licenses/LICENSE-2.0
#
# Unless required by applicable law or agreed to in writing, software
# distributed under the License is distributed on an "AS IS" BASIS,
# WITHOUT WARRANTIES OR CONDITIONS OF ANY KIND, either express or implied.
# See the License for the specific language governing permissions and
# limitations under the License.
from typing import Callable, Optional, Tuple
import flax.linen as nn
import jax
import jax.numpy as jnp
import numpy as np
from flax.core.frozen_dict import FrozenDict, freeze, unfreeze
from flax.linen import combine_masks, make_causal_mask
from flax.linen import partitioning as nn_partitioning
from flax.linen.attention import dot_product_attention_weights
from flax.traverse_util import flatten_dict, unflatten_dict
from jax import lax
from ...modeling_flax_outputs import (
FlaxBaseModelOutputWithPastAndCrossAttentions,
FlaxBaseModelOutputWithPooling,
FlaxBaseModelOutputWithPoolingAndCrossAttentions,
FlaxCausalLMOutputWithCrossAttentions,
FlaxMaskedLMOutput,
FlaxMultipleChoiceModelOutput,
FlaxQuestionAnsweringModelOutput,
FlaxSequenceClassifierOutput,
FlaxTokenClassifierOutput,
)
from ...modeling_flax_utils import ACT2FN, FlaxPreTrainedModel, append_call_sample_docstring, overwrite_call_docstring
from ...utils import add_start_docstrings, add_start_docstrings_to_model_forward, logging
from .configuration_roberta import RobertaConfig
logger = logging.get_logger(__name__)
_CHECKPOINT_FOR_DOC = "roberta-base"
_CONFIG_FOR_DOC = "RobertaConfig"
remat = nn_partitioning.remat
def create_position_ids_from_input_ids(input_ids, padding_idx):
"""
Replace non-padding symbols with their position numbers. Position numbers begin at padding_idx+1. Padding symbols
are ignored. This is modified from fairseq's `utils.make_positions`.
Args:
input_ids: jnp.ndarray
padding_idx: int
Returns: jnp.ndarray
"""
# The series of casts and type-conversions here are carefully balanced to both work with ONNX export and XLA.
mask = (input_ids != padding_idx).astype("i4")
if mask.ndim > 2:
mask = mask.reshape((-1, mask.shape[-1]))
incremental_indices = jnp.cumsum(mask, axis=1).astype("i4") * mask
incremental_indices = incremental_indices.reshape(input_ids.shape)
else:
incremental_indices = jnp.cumsum(mask, axis=1).astype("i4") * mask
return incremental_indices.astype("i4") + padding_idx
ROBERTA_START_DOCSTRING = r"""
This model inherits from [`FlaxPreTrainedModel`]. Check the superclass documentation for the generic methods the
library implements for all its model (such as downloading, saving and converting weights from PyTorch models)
This model is also a
[flax.linen.Module](https://flax.readthedocs.io/en/latest/api_reference/flax.linen/module.html) subclass. Use it as
a regular Flax linen Module and refer to the Flax documentation for all matter related to general usage and
behavior.
Finally, this model supports inherent JAX features such as:
- [Just-In-Time (JIT) compilation](https://jax.readthedocs.io/en/latest/jax.html#just-in-time-compilation-jit)
- [Automatic Differentiation](https://jax.readthedocs.io/en/latest/jax.html#automatic-differentiation)
- [Vectorization](https://jax.readthedocs.io/en/latest/jax.html#vectorization-vmap)
- [Parallelization](https://jax.readthedocs.io/en/latest/jax.html#parallelization-pmap)
Parameters:
config ([`RobertaConfig`]): Model configuration class with all the parameters of the
model. Initializing with a config file does not load the weights associated with the model, only the
configuration. Check out the [`~FlaxPreTrainedModel.from_pretrained`] method to load the model weights.
"""
ROBERTA_INPUTS_DOCSTRING = r"""
Args:
input_ids (`numpy.ndarray` of shape `({0})`):
Indices of input sequence tokens in the vocabulary.
Indices can be obtained using [`AutoTokenizer`]. See [`PreTrainedTokenizer.encode`] and
[`PreTrainedTokenizer.__call__`] for details.
[What are input IDs?](../glossary#input-ids)
attention_mask (`numpy.ndarray` of shape `({0})`, *optional*):
Mask to avoid performing attention on padding token indices. Mask values selected in `[0, 1]`:
- 1 for tokens that are **not masked**,
- 0 for tokens that are **masked**.
[What are attention masks?](../glossary#attention-mask)
token_type_ids (`numpy.ndarray` of shape `({0})`, *optional*):
Segment token indices to indicate first and second portions of the inputs. Indices are selected in `[0,
1]`:
- 0 corresponds to a *sentence A* token,
- 1 corresponds to a *sentence B* token.
[What are token type IDs?](../glossary#token-type-ids)
position_ids (`numpy.ndarray` of shape `({0})`, *optional*):
Indices of positions of each input sequence tokens in the position embeddings. Selected in the range `[0,
config.max_position_embeddings - 1]`.
head_mask (`numpy.ndarray` of shape `({0})`, `optional):
Mask to nullify selected heads of the attention modules. Mask values selected in `[0, 1]`:
- 1 indicates the head is **not masked**,
- 0 indicates the head is **masked**.
return_dict (`bool`, *optional*):
Whether or not to return a [`~utils.ModelOutput`] instead of a plain tuple.
"""
# Copied from transformers.models.bert.modeling_flax_bert.FlaxBertEmbeddings with Bert->Roberta
class FlaxRobertaEmbeddings(nn.Module):
"""Construct the embeddings from word, position and token_type embeddings."""
config: RobertaConfig
dtype: jnp.dtype = jnp.float32 # the dtype of the computation
def setup(self):
self.word_embeddings = nn.Embed(
self.config.vocab_size,
self.config.hidden_size,
embedding_init=jax.nn.initializers.normal(stddev=self.config.initializer_range),
dtype=self.dtype,
)
self.position_embeddings = nn.Embed(
self.config.max_position_embeddings,
self.config.hidden_size,
embedding_init=jax.nn.initializers.normal(stddev=self.config.initializer_range),
dtype=self.dtype,
)
self.token_type_embeddings = nn.Embed(
self.config.type_vocab_size,
self.config.hidden_size,
embedding_init=jax.nn.initializers.normal(stddev=self.config.initializer_range),
dtype=self.dtype,
)
self.LayerNorm = nn.LayerNorm(epsilon=self.config.layer_norm_eps, dtype=self.dtype)
self.dropout = nn.Dropout(rate=self.config.hidden_dropout_prob)
def __call__(self, input_ids, token_type_ids, position_ids, attention_mask, deterministic: bool = True):
# Embed
inputs_embeds = self.word_embeddings(input_ids.astype("i4"))
position_embeds = self.position_embeddings(position_ids.astype("i4"))
token_type_embeddings = self.token_type_embeddings(token_type_ids.astype("i4"))
# Sum all embeddings
hidden_states = inputs_embeds + token_type_embeddings + position_embeds
# Layer Norm
hidden_states = self.LayerNorm(hidden_states)
hidden_states = self.dropout(hidden_states, deterministic=deterministic)
return hidden_states
# Copied from transformers.models.bert.modeling_flax_bert.FlaxBertSelfAttention with Bert->Roberta
class FlaxRobertaSelfAttention(nn.Module):
config: RobertaConfig
causal: bool = False
dtype: jnp.dtype = jnp.float32 # the dtype of the computation
def setup(self):
self.head_dim = self.config.hidden_size // self.config.num_attention_heads
if self.config.hidden_size % self.config.num_attention_heads != 0:
raise ValueError(
"`config.hidden_size`: {self.config.hidden_size} has to be a multiple of `config.num_attention_heads` "
" : {self.config.num_attention_heads}"
)
self.query = nn.Dense(
self.config.hidden_size,
dtype=self.dtype,
kernel_init=jax.nn.initializers.normal(self.config.initializer_range),
)
self.key = nn.Dense(
self.config.hidden_size,
dtype=self.dtype,
kernel_init=jax.nn.initializers.normal(self.config.initializer_range),
)
self.value = nn.Dense(
self.config.hidden_size,
dtype=self.dtype,
kernel_init=jax.nn.initializers.normal(self.config.initializer_range),
)
if self.causal:
self.causal_mask = make_causal_mask(
jnp.ones((1, self.config.max_position_embeddings), dtype="bool"), dtype="bool"
)
def _split_heads(self, hidden_states):
return hidden_states.reshape(hidden_states.shape[:2] + (self.config.num_attention_heads, self.head_dim))
def _merge_heads(self, hidden_states):
return hidden_states.reshape(hidden_states.shape[:2] + (self.config.hidden_size,))
@nn.compact
# Copied from transformers.models.bart.modeling_flax_bart.FlaxBartAttention._concatenate_to_cache
def _concatenate_to_cache(self, key, value, query, attention_mask):
"""
This function takes projected key, value states from a single input token and concatenates the states to cached
states from previous steps. This function is slighly adapted from the official Flax repository:
https://github.com/google/flax/blob/491ce18759622506588784b4fca0e4bf05f8c8cd/flax/linen/attention.py#L252
"""
# detect if we're initializing by absence of existing cache data.
is_initialized = self.has_variable("cache", "cached_key")
cached_key = self.variable("cache", "cached_key", jnp.zeros, key.shape, key.dtype)
cached_value = self.variable("cache", "cached_value", jnp.zeros, value.shape, value.dtype)
cache_index = self.variable("cache", "cache_index", lambda: jnp.array(0, dtype=jnp.int32))
if is_initialized:
*batch_dims, max_length, num_heads, depth_per_head = cached_key.value.shape
# update key, value caches with our new 1d spatial slices
cur_index = cache_index.value
indices = (0,) * len(batch_dims) + (cur_index, 0, 0)
key = lax.dynamic_update_slice(cached_key.value, key, indices)
value = lax.dynamic_update_slice(cached_value.value, value, indices)
cached_key.value = key
cached_value.value = value
num_updated_cache_vectors = query.shape[1]
cache_index.value = cache_index.value + num_updated_cache_vectors
# causal mask for cached decoder self-attention: our single query position should only attend to those key positions that have already been generated and cached, not the remaining zero elements.
pad_mask = jnp.broadcast_to(
jnp.arange(max_length) < cur_index + num_updated_cache_vectors,
tuple(batch_dims) + (1, num_updated_cache_vectors, max_length),
)
attention_mask = combine_masks(pad_mask, attention_mask)
return key, value, attention_mask
def __call__(
self,
hidden_states,
attention_mask,
layer_head_mask,
key_value_states: Optional[jnp.ndarray] = None,
init_cache: bool = False,
deterministic=True,
output_attentions: bool = False,
):
# if key_value_states are provided this layer is used as a cross-attention layer
# for the decoder
is_cross_attention = key_value_states is not None
batch_size = hidden_states.shape[0]
# get query proj
query_states = self.query(hidden_states)
# get key, value proj
if is_cross_attention:
# cross_attentions
key_states = self.key(key_value_states)
value_states = self.value(key_value_states)
else:
# self_attention
key_states = self.key(hidden_states)
value_states = self.value(hidden_states)
query_states = self._split_heads(query_states)
key_states = self._split_heads(key_states)
value_states = self._split_heads(value_states)
# handle cache prepare causal attention mask
if self.causal:
query_length, key_length = query_states.shape[1], key_states.shape[1]
if self.has_variable("cache", "cached_key"):
mask_shift = self.variables["cache"]["cache_index"]
max_decoder_length = self.variables["cache"]["cached_key"].shape[1]
causal_mask = lax.dynamic_slice(
self.causal_mask, (0, 0, mask_shift, 0), (1, 1, query_length, max_decoder_length)
)
else:
causal_mask = self.causal_mask[:, :, :query_length, :key_length]
causal_mask = jnp.broadcast_to(causal_mask, (batch_size,) + causal_mask.shape[1:])
# combine masks if needed
if attention_mask is not None and self.causal:
attention_mask = jnp.broadcast_to(jnp.expand_dims(attention_mask, axis=(-3, -2)), causal_mask.shape)
attention_mask = combine_masks(attention_mask, causal_mask)
elif self.causal:
attention_mask = causal_mask
elif attention_mask is not None:
attention_mask = jnp.expand_dims(attention_mask, axis=(-3, -2))
# During fast autoregressive decoding, we feed one position at a time,
# and cache the keys and values step by step.
if self.causal and (self.has_variable("cache", "cached_key") or init_cache):
key_states, value_states, attention_mask = self._concatenate_to_cache(
key_states, value_states, query_states, attention_mask
)
# Convert the boolean attention mask to an attention bias.
if attention_mask is not None:
# attention mask in the form of attention bias
attention_bias = lax.select(
attention_mask > 0,
jnp.full(attention_mask.shape, 0.0).astype(self.dtype),
jnp.full(attention_mask.shape, jnp.finfo(self.dtype).min).astype(self.dtype),
)
else:
attention_bias = None
dropout_rng = None
if not deterministic and self.config.attention_probs_dropout_prob > 0.0:
dropout_rng = self.make_rng("dropout")
attn_weights = dot_product_attention_weights(
query_states,
key_states,
bias=attention_bias,
dropout_rng=dropout_rng,
dropout_rate=self.config.attention_probs_dropout_prob,
broadcast_dropout=True,
deterministic=deterministic,
dtype=self.dtype,
precision=None,
)
# Mask heads if we want to
if layer_head_mask is not None:
attn_weights = jnp.einsum("...hqk,h->...hqk", attn_weights, layer_head_mask)
attn_output = jnp.einsum("...hqk,...khd->...qhd", attn_weights, value_states)
attn_output = attn_output.reshape(attn_output.shape[:2] + (-1,))
outputs = (attn_output, attn_weights) if output_attentions else (attn_output,)
return outputs
# Copied from transformers.models.bert.modeling_flax_bert.FlaxBertSelfOutput with Bert->Roberta
class FlaxRobertaSelfOutput(nn.Module):
config: RobertaConfig
dtype: jnp.dtype = jnp.float32 # the dtype of the computation
def setup(self):
self.dense = nn.Dense(
self.config.hidden_size,
kernel_init=jax.nn.initializers.normal(self.config.initializer_range),
dtype=self.dtype,
)
self.LayerNorm = nn.LayerNorm(epsilon=self.config.layer_norm_eps, dtype=self.dtype)
self.dropout = nn.Dropout(rate=self.config.hidden_dropout_prob)
def __call__(self, hidden_states, input_tensor, deterministic: bool = True):
hidden_states = self.dense(hidden_states)
hidden_states = self.dropout(hidden_states, deterministic=deterministic)
hidden_states = self.LayerNorm(hidden_states + input_tensor)
return hidden_states
# Copied from transformers.models.bert.modeling_flax_bert.FlaxBertAttention with Bert->Roberta
class FlaxRobertaAttention(nn.Module):
config: RobertaConfig
causal: bool = False
dtype: jnp.dtype = jnp.float32
def setup(self):
self.self = FlaxRobertaSelfAttention(self.config, causal=self.causal, dtype=self.dtype)
self.output = FlaxRobertaSelfOutput(self.config, dtype=self.dtype)
def __call__(
self,
hidden_states,
attention_mask,
layer_head_mask,
key_value_states=None,
init_cache=False,
deterministic=True,
output_attentions: bool = False,
):
# Attention mask comes in as attention_mask.shape == (*batch_sizes, kv_length)
# FLAX expects: attention_mask.shape == (*batch_sizes, 1, 1, kv_length) such that it is broadcastable
# with attn_weights.shape == (*batch_sizes, num_heads, q_length, kv_length)
attn_outputs = self.self(
hidden_states,
attention_mask,
layer_head_mask=layer_head_mask,
key_value_states=key_value_states,
init_cache=init_cache,
deterministic=deterministic,
output_attentions=output_attentions,
)
attn_output = attn_outputs[0]
hidden_states = self.output(attn_output, hidden_states, deterministic=deterministic)
outputs = (hidden_states,)
if output_attentions:
outputs += (attn_outputs[1],)
return outputs
# Copied from transformers.models.bert.modeling_flax_bert.FlaxBertIntermediate with Bert->Roberta
class FlaxRobertaIntermediate(nn.Module):
config: RobertaConfig
dtype: jnp.dtype = jnp.float32 # the dtype of the computation
def setup(self):
self.dense = nn.Dense(
self.config.intermediate_size,
kernel_init=jax.nn.initializers.normal(self.config.initializer_range),
dtype=self.dtype,
)
self.activation = ACT2FN[self.config.hidden_act]
def __call__(self, hidden_states):
hidden_states = self.dense(hidden_states)
hidden_states = self.activation(hidden_states)
return hidden_states
# Copied from transformers.models.bert.modeling_flax_bert.FlaxBertOutput with Bert->Roberta
class FlaxRobertaOutput(nn.Module):
config: RobertaConfig
dtype: jnp.dtype = jnp.float32 # the dtype of the computation
def setup(self):
self.dense = nn.Dense(
self.config.hidden_size,
kernel_init=jax.nn.initializers.normal(self.config.initializer_range),
dtype=self.dtype,
)
self.dropout = nn.Dropout(rate=self.config.hidden_dropout_prob)
self.LayerNorm = nn.LayerNorm(epsilon=self.config.layer_norm_eps, dtype=self.dtype)
def __call__(self, hidden_states, attention_output, deterministic: bool = True):
hidden_states = self.dense(hidden_states)
hidden_states = self.dropout(hidden_states, deterministic=deterministic)
hidden_states = self.LayerNorm(hidden_states + attention_output)
return hidden_states
# Copied from transformers.models.bert.modeling_flax_bert.FlaxBertLayer with Bert->Roberta
class FlaxRobertaLayer(nn.Module):
config: RobertaConfig
dtype: jnp.dtype = jnp.float32 # the dtype of the computation
def setup(self):
self.attention = FlaxRobertaAttention(self.config, causal=self.config.is_decoder, dtype=self.dtype)
self.intermediate = FlaxRobertaIntermediate(self.config, dtype=self.dtype)
self.output = FlaxRobertaOutput(self.config, dtype=self.dtype)
if self.config.add_cross_attention:
self.crossattention = FlaxRobertaAttention(self.config, causal=False, dtype=self.dtype)
def __call__(
self,
hidden_states,
attention_mask,
layer_head_mask,
encoder_hidden_states: Optional[jnp.ndarray] = None,
encoder_attention_mask: Optional[jnp.ndarray] = None,
init_cache: bool = False,
deterministic: bool = True,
output_attentions: bool = False,
):
# Self Attention
attention_outputs = self.attention(
hidden_states,
attention_mask,
layer_head_mask=layer_head_mask,
init_cache=init_cache,
deterministic=deterministic,
output_attentions=output_attentions,
)
attention_output = attention_outputs[0]
# Cross-Attention Block
if encoder_hidden_states is not None:
cross_attention_outputs = self.crossattention(
attention_output,
attention_mask=encoder_attention_mask,
layer_head_mask=layer_head_mask,
key_value_states=encoder_hidden_states,
deterministic=deterministic,
output_attentions=output_attentions,
)
attention_output = cross_attention_outputs[0]
hidden_states = self.intermediate(attention_output)
hidden_states = self.output(hidden_states, attention_output, deterministic=deterministic)
outputs = (hidden_states,)
if output_attentions:
outputs += (attention_outputs[1],)
if encoder_hidden_states is not None:
outputs += (cross_attention_outputs[1],)
return outputs
# Copied from transformers.models.bert.modeling_flax_bert.FlaxBertLayerCollection with Bert->Roberta
class FlaxRobertaLayerCollection(nn.Module):
config: RobertaConfig
dtype: jnp.dtype = jnp.float32 # the dtype of the computation
gradient_checkpointing: bool = False
def setup(self):
if self.gradient_checkpointing:
FlaxRobertaCheckpointLayer = remat(FlaxRobertaLayer, static_argnums=(5, 6, 7))
self.layers = [
FlaxRobertaCheckpointLayer(self.config, name=str(i), dtype=self.dtype)
for i in range(self.config.num_hidden_layers)
]
else:
self.layers = [
FlaxRobertaLayer(self.config, name=str(i), dtype=self.dtype)
for i in range(self.config.num_hidden_layers)
]
def __call__(
self,
hidden_states,
attention_mask,
head_mask,
encoder_hidden_states: Optional[jnp.ndarray] = None,
encoder_attention_mask: Optional[jnp.ndarray] = None,
init_cache: bool = False,
deterministic: bool = True,
output_attentions: bool = False,
output_hidden_states: bool = False,
return_dict: bool = True,
):
all_attentions = () if output_attentions else None
all_hidden_states = () if output_hidden_states else None
all_cross_attentions = () if (output_attentions and encoder_hidden_states is not None) else None
# Check if head_mask has a correct number of layers specified if desired
if head_mask is not None:
if head_mask.shape[0] != (len(self.layers)):
raise ValueError(
f"The head_mask should be specified for {len(self.layers)} layers, but it is for "
f" {head_mask.shape[0]}."
)
for i, layer in enumerate(self.layers):
if output_hidden_states:
all_hidden_states += (hidden_states,)
layer_outputs = layer(
hidden_states,
attention_mask,
head_mask[i] if head_mask is not None else None,
encoder_hidden_states,
encoder_attention_mask,
init_cache,
deterministic,
output_attentions,
)
hidden_states = layer_outputs[0]
if output_attentions:
all_attentions += (layer_outputs[1],)
if encoder_hidden_states is not None:
all_cross_attentions += (layer_outputs[2],)
if output_hidden_states:
all_hidden_states += (hidden_states,)
outputs = (hidden_states, all_hidden_states, all_attentions, all_cross_attentions)
if not return_dict:
return tuple(v for v in outputs if v is not None)
return FlaxBaseModelOutputWithPastAndCrossAttentions(
last_hidden_state=hidden_states,
hidden_states=all_hidden_states,
attentions=all_attentions,
cross_attentions=all_cross_attentions,
)
# Copied from transformers.models.bert.modeling_flax_bert.FlaxBertEncoder with Bert->Roberta
class FlaxRobertaEncoder(nn.Module):
config: RobertaConfig
dtype: jnp.dtype = jnp.float32 # the dtype of the computation
gradient_checkpointing: bool = False
def setup(self):
self.layer = FlaxRobertaLayerCollection(
self.config,
dtype=self.dtype,
gradient_checkpointing=self.gradient_checkpointing,
)
def __call__(
self,
hidden_states,
attention_mask,
head_mask,
encoder_hidden_states: Optional[jnp.ndarray] = None,
encoder_attention_mask: Optional[jnp.ndarray] = None,
init_cache: bool = False,
deterministic: bool = True,
output_attentions: bool = False,
output_hidden_states: bool = False,
return_dict: bool = True,
):
return self.layer(
hidden_states,
attention_mask,
head_mask=head_mask,
encoder_hidden_states=encoder_hidden_states,
encoder_attention_mask=encoder_attention_mask,
init_cache=init_cache,
deterministic=deterministic,
output_attentions=output_attentions,
output_hidden_states=output_hidden_states,
return_dict=return_dict,
)
# Copied from transformers.models.bert.modeling_flax_bert.FlaxBertPooler with Bert->Roberta
class FlaxRobertaPooler(nn.Module):
config: RobertaConfig
dtype: jnp.dtype = jnp.float32 # the dtype of the computation
def setup(self):
self.dense = nn.Dense(
self.config.hidden_size,
kernel_init=jax.nn.initializers.normal(self.config.initializer_range),
dtype=self.dtype,
)
def __call__(self, hidden_states):
cls_hidden_state = hidden_states[:, 0]
cls_hidden_state = self.dense(cls_hidden_state)
return nn.tanh(cls_hidden_state)
class FlaxRobertaLMHead(nn.Module):
config: RobertaConfig
dtype: jnp.dtype = jnp.float32
bias_init: Callable[..., np.ndarray] = jax.nn.initializers.zeros
def setup(self):
self.dense = nn.Dense(
self.config.hidden_size,
dtype=self.dtype,
kernel_init=jax.nn.initializers.normal(self.config.initializer_range),
)
self.layer_norm = nn.LayerNorm(epsilon=self.config.layer_norm_eps, dtype=self.dtype)
self.decoder = nn.Dense(
self.config.vocab_size,
dtype=self.dtype,
use_bias=False,
kernel_init=jax.nn.initializers.normal(self.config.initializer_range),
)
self.bias = self.param("bias", self.bias_init, (self.config.vocab_size,))
def __call__(self, hidden_states, shared_embedding=None):
hidden_states = self.dense(hidden_states)
hidden_states = ACT2FN["gelu"](hidden_states)
hidden_states = self.layer_norm(hidden_states)
if shared_embedding is not None:
hidden_states = self.decoder.apply({"params": {"kernel": shared_embedding.T}}, hidden_states)
else:
hidden_states = self.decoder(hidden_states)
bias = jnp.asarray(self.bias, self.dtype)
hidden_states += bias
return hidden_states
class FlaxRobertaClassificationHead(nn.Module):
config: RobertaConfig
dtype: jnp.dtype = jnp.float32
def setup(self):
self.dense = nn.Dense(
self.config.hidden_size,
dtype=self.dtype,
kernel_init=jax.nn.initializers.normal(self.config.initializer_range),
)
classifier_dropout = (
self.config.classifier_dropout
if self.config.classifier_dropout is not None
else self.config.hidden_dropout_prob
)
self.dropout = nn.Dropout(rate=classifier_dropout)
self.out_proj = nn.Dense(
self.config.num_labels,
dtype=self.dtype,
kernel_init=jax.nn.initializers.normal(self.config.initializer_range),
)
def __call__(self, hidden_states, deterministic=True):
hidden_states = hidden_states[:, 0, :] # take <s> token (equiv. to [CLS])
hidden_states = self.dropout(hidden_states, deterministic=deterministic)
hidden_states = self.dense(hidden_states)
hidden_states = nn.tanh(hidden_states)
hidden_states = self.dropout(hidden_states, deterministic=deterministic)
hidden_states = self.out_proj(hidden_states)
return hidden_states
class FlaxRobertaPreTrainedModel(FlaxPreTrainedModel):
"""
An abstract class to handle weights initialization and a simple interface for downloading and loading pretrained
models.
"""
config_class = RobertaConfig
base_model_prefix = "roberta"
module_class: nn.Module = None
def __init__(
self,
config: RobertaConfig,
input_shape: Tuple = (1, 1),
seed: int = 0,
dtype: jnp.dtype = jnp.float32,
_do_init: bool = True,
gradient_checkpointing: bool = False,
**kwargs,
):
module = self.module_class(config=config, dtype=dtype, gradient_checkpointing=gradient_checkpointing, **kwargs)
super().__init__(config, module, input_shape=input_shape, seed=seed, dtype=dtype, _do_init=_do_init)
# Copied from transformers.models.bert.modeling_flax_bert.FlaxBertPreTrainedModel.enable_gradient_checkpointing
def enable_gradient_checkpointing(self):
self._module = self.module_class(
config=self.config,
dtype=self.dtype,
gradient_checkpointing=True,
)
def init_weights(self, rng: jax.random.PRNGKey, input_shape: Tuple, params: FrozenDict = None) -> FrozenDict:
# init input tensors
input_ids = jnp.zeros(input_shape, dtype="i4")
token_type_ids = jnp.ones_like(input_ids)
position_ids = create_position_ids_from_input_ids(input_ids, self.config.pad_token_id)
attention_mask = jnp.ones_like(input_ids)
head_mask = jnp.ones((self.config.num_hidden_layers, self.config.num_attention_heads))
params_rng, dropout_rng = jax.random.split(rng)
rngs = {"params": params_rng, "dropout": dropout_rng}
if self.config.add_cross_attention:
encoder_hidden_states = jnp.zeros(input_shape + (self.config.hidden_size,))
encoder_attention_mask = attention_mask
module_init_outputs = self.module.init(
rngs,
input_ids,
attention_mask,
token_type_ids,
position_ids,
head_mask,
encoder_hidden_states,
encoder_attention_mask,
return_dict=False,
)
else:
module_init_outputs = self.module.init(
rngs, input_ids, attention_mask, token_type_ids, position_ids, head_mask, return_dict=False
)
random_params = module_init_outputs["params"]
if params is not None:
random_params = flatten_dict(unfreeze(random_params))
params = flatten_dict(unfreeze(params))
for missing_key in self._missing_keys:
params[missing_key] = random_params[missing_key]
self._missing_keys = set()
return freeze(unflatten_dict(params))
else:
return random_params
# Copied from transformers.models.bart.modeling_flax_bart.FlaxBartDecoderPreTrainedModel.init_cache
def init_cache(self, batch_size, max_length):
r"""
Args:
batch_size (`int`):
batch_size used for fast auto-regressive decoding. Defines the batch size of the initialized cache.
max_length (`int`):
maximum possible length for auto-regressive decoding. Defines the sequence length of the initialized
cache.
"""
# init input variables to retrieve cache
input_ids = jnp.ones((batch_size, max_length), dtype="i4")
attention_mask = jnp.ones_like(input_ids, dtype="i4")
position_ids = jnp.broadcast_to(jnp.arange(jnp.atleast_2d(input_ids).shape[-1]), input_ids.shape)
init_variables = self.module.init(
jax.random.PRNGKey(0), input_ids, attention_mask, position_ids, return_dict=False, init_cache=True
)
return unfreeze(init_variables["cache"])
@add_start_docstrings_to_model_forward(ROBERTA_INPUTS_DOCSTRING.format("batch_size, sequence_length"))
def __call__(
self,
input_ids,
attention_mask=None,
token_type_ids=None,
position_ids=None,
head_mask=None,
encoder_hidden_states=None,
encoder_attention_mask=None,
params: dict = None,
dropout_rng: jax.random.PRNGKey = None,
train: bool = False,
output_attentions: Optional[bool] = None,
output_hidden_states: Optional[bool] = None,
return_dict: Optional[bool] = None,
past_key_values: dict = None,
):
output_attentions = output_attentions if output_attentions is not None else self.config.output_attentions
output_hidden_states = (
output_hidden_states if output_hidden_states is not None else self.config.output_hidden_states
)
return_dict = return_dict if return_dict is not None else self.config.return_dict
# init input tensors if not passed
if token_type_ids is None:
token_type_ids = jnp.zeros_like(input_ids)
if position_ids is None:
position_ids = create_position_ids_from_input_ids(input_ids, self.config.pad_token_id)
if attention_mask is None:
attention_mask = jnp.ones_like(input_ids)
if head_mask is None:
head_mask = jnp.ones((self.config.num_hidden_layers, self.config.num_attention_heads))
# Handle any PRNG if needed
rngs = {}
if dropout_rng is not None:
rngs["dropout"] = dropout_rng
inputs = {"params": params or self.params}
if self.config.add_cross_attention:
# if past_key_values are passed then cache is already initialized a private flag init_cache has to be passed
# down to ensure cache is used. It has to be made sure that cache is marked as mutable so that it can be
# changed by FlaxRobertaAttention module
if past_key_values:
inputs["cache"] = past_key_values
mutable = ["cache"]
else:
mutable = False
outputs = self.module.apply(
inputs,
jnp.array(input_ids, dtype="i4"),
jnp.array(attention_mask, dtype="i4"),
token_type_ids=jnp.array(token_type_ids, dtype="i4"),
position_ids=jnp.array(position_ids, dtype="i4"),
head_mask=jnp.array(head_mask, dtype="i4"),
encoder_hidden_states=encoder_hidden_states,
encoder_attention_mask=encoder_attention_mask,
deterministic=not train,
output_attentions=output_attentions,
output_hidden_states=output_hidden_states,
return_dict=return_dict,
rngs=rngs,
mutable=mutable,
)
# add updated cache to model output
if past_key_values is not None and return_dict:
outputs, past_key_values = outputs
outputs["past_key_values"] = unfreeze(past_key_values["cache"])
return outputs
elif past_key_values is not None and not return_dict:
outputs, past_key_values = outputs
outputs = outputs[:1] + (unfreeze(past_key_values["cache"]),) + outputs[1:]
else:
outputs = self.module.apply(
inputs,
jnp.array(input_ids, dtype="i4"),
jnp.array(attention_mask, dtype="i4"),
token_type_ids=jnp.array(token_type_ids, dtype="i4"),
position_ids=jnp.array(position_ids, dtype="i4"),
head_mask=jnp.array(head_mask, dtype="i4"),
deterministic=not train,
output_attentions=output_attentions,
output_hidden_states=output_hidden_states,
return_dict=return_dict,
rngs=rngs,
)
return outputs
# Copied from transformers.models.bert.modeling_flax_bert.FlaxBertModule with Bert->Roberta
class FlaxRobertaModule(nn.Module):
config: RobertaConfig
dtype: jnp.dtype = jnp.float32 # the dtype of the computation
add_pooling_layer: bool = True
gradient_checkpointing: bool = False
def setup(self):
self.embeddings = FlaxRobertaEmbeddings(self.config, dtype=self.dtype)
self.encoder = FlaxRobertaEncoder(
self.config,
dtype=self.dtype,
gradient_checkpointing=self.gradient_checkpointing,
)
self.pooler = FlaxRobertaPooler(self.config, dtype=self.dtype)
def __call__(
self,
input_ids,
attention_mask,
token_type_ids: Optional[jnp.ndarray] = None,
position_ids: Optional[jnp.ndarray] = None,
head_mask: Optional[jnp.ndarray] = None,
encoder_hidden_states: Optional[jnp.ndarray] = None,
encoder_attention_mask: Optional[jnp.ndarray] = None,
init_cache: bool = False,
deterministic: bool = True,
output_attentions: bool = False,
output_hidden_states: bool = False,
return_dict: bool = True,
):
# make sure `token_type_ids` is correctly initialized when not passed
if token_type_ids is None:
token_type_ids = jnp.zeros_like(input_ids)
# make sure `position_ids` is correctly initialized when not passed
if position_ids is None:
position_ids = jnp.broadcast_to(jnp.arange(jnp.atleast_2d(input_ids).shape[-1]), input_ids.shape)
hidden_states = self.embeddings(
input_ids, token_type_ids, position_ids, attention_mask, deterministic=deterministic
)
outputs = self.encoder(
hidden_states,
attention_mask,
head_mask=head_mask,
deterministic=deterministic,
encoder_hidden_states=encoder_hidden_states,
encoder_attention_mask=encoder_attention_mask,
init_cache=init_cache,
output_attentions=output_attentions,
output_hidden_states=output_hidden_states,
return_dict=return_dict,
)
hidden_states = outputs[0]
pooled = self.pooler(hidden_states) if self.add_pooling_layer else None
if not return_dict:
# if pooled is None, don't return it
if pooled is None:
return (hidden_states,) + outputs[1:]
return (hidden_states, pooled) + outputs[1:]
return FlaxBaseModelOutputWithPoolingAndCrossAttentions(
last_hidden_state=hidden_states,
pooler_output=pooled,
hidden_states=outputs.hidden_states,
attentions=outputs.attentions,
cross_attentions=outputs.cross_attentions,
)
@add_start_docstrings(
"The bare RoBERTa Model transformer outputting raw hidden-states without any specific head on top.",
ROBERTA_START_DOCSTRING,
)
class FlaxRobertaModel(FlaxRobertaPreTrainedModel):
module_class = FlaxRobertaModule
append_call_sample_docstring(FlaxRobertaModel, _CHECKPOINT_FOR_DOC, FlaxBaseModelOutputWithPooling, _CONFIG_FOR_DOC)
class FlaxRobertaForMaskedLMModule(nn.Module):
config: RobertaConfig
dtype: jnp.dtype = jnp.float32
gradient_checkpointing: bool = False
def setup(self):
self.roberta = FlaxRobertaModule(
config=self.config,
add_pooling_layer=False,
dtype=self.dtype,
gradient_checkpointing=self.gradient_checkpointing,
)
self.lm_head = FlaxRobertaLMHead(config=self.config, dtype=self.dtype)
def __call__(
self,
input_ids,
attention_mask,
token_type_ids,
position_ids,
head_mask,
deterministic: bool = True,
output_attentions: bool = False,
output_hidden_states: bool = False,
return_dict: bool = True,
):
# Model
outputs = self.roberta(
input_ids,
attention_mask,
token_type_ids,
position_ids,
head_mask,
deterministic=deterministic,
output_attentions=output_attentions,
output_hidden_states=output_hidden_states,
return_dict=return_dict,
)
hidden_states = outputs[0]
if self.config.tie_word_embeddings:
shared_embedding = self.roberta.variables["params"]["embeddings"]["word_embeddings"]["embedding"]
else:
shared_embedding = None
# Compute the prediction scores
logits = self.lm_head(hidden_states, shared_embedding=shared_embedding)
if not return_dict:
return (logits,) + outputs[1:]
return FlaxMaskedLMOutput(
logits=logits,
hidden_states=outputs.hidden_states,
attentions=outputs.attentions,
)
@add_start_docstrings("""RoBERTa Model with a `language modeling` head on top.""", ROBERTA_START_DOCSTRING)
class FlaxRobertaForMaskedLM(FlaxRobertaPreTrainedModel):
module_class = FlaxRobertaForMaskedLMModule
append_call_sample_docstring(
FlaxRobertaForMaskedLM,
_CHECKPOINT_FOR_DOC,
FlaxBaseModelOutputWithPooling,
_CONFIG_FOR_DOC,
mask="<mask>",
)
class FlaxRobertaForSequenceClassificationModule(nn.Module):
config: RobertaConfig
dtype: jnp.dtype = jnp.float32
gradient_checkpointing: bool = False
def setup(self):
self.roberta = FlaxRobertaModule(
config=self.config,
dtype=self.dtype,
add_pooling_layer=False,
gradient_checkpointing=self.gradient_checkpointing,
)
self.classifier = FlaxRobertaClassificationHead(config=self.config, dtype=self.dtype)
def __call__(
self,
input_ids,
attention_mask,
token_type_ids,
position_ids,
head_mask,
deterministic: bool = True,
output_attentions: bool = False,
output_hidden_states: bool = False,
return_dict: bool = True,
):
# Model
outputs = self.roberta(
input_ids,
attention_mask,
token_type_ids,
position_ids,
head_mask,
deterministic=deterministic,
output_attentions=output_attentions,
output_hidden_states=output_hidden_states,
return_dict=return_dict,
)
sequence_output = outputs[0]
logits = self.classifier(sequence_output, deterministic=deterministic)
if not return_dict:
return (logits,) + outputs[1:]
return FlaxSequenceClassifierOutput(
logits=logits,
hidden_states=outputs.hidden_states,
attentions=outputs.attentions,
)
@add_start_docstrings(
"""
Roberta Model transformer with a sequence classification/regression head on top (a linear layer on top of the
pooled output) e.g. for GLUE tasks.
""",
ROBERTA_START_DOCSTRING,
)
class FlaxRobertaForSequenceClassification(FlaxRobertaPreTrainedModel):
module_class = FlaxRobertaForSequenceClassificationModule
append_call_sample_docstring(
FlaxRobertaForSequenceClassification,
_CHECKPOINT_FOR_DOC,
FlaxSequenceClassifierOutput,
_CONFIG_FOR_DOC,
)
# Copied from transformers.models.bert.modeling_flax_bert.FlaxBertForMultipleChoiceModule with Bert->Roberta, with self.bert->self.roberta
class FlaxRobertaForMultipleChoiceModule(nn.Module):
config: RobertaConfig
dtype: jnp.dtype = jnp.float32
gradient_checkpointing: bool = False
def setup(self):
self.roberta = FlaxRobertaModule(
config=self.config,
dtype=self.dtype,
gradient_checkpointing=self.gradient_checkpointing,
)
self.dropout = nn.Dropout(rate=self.config.hidden_dropout_prob)
self.classifier = nn.Dense(1, dtype=self.dtype)
def __call__(
self,
input_ids,
attention_mask,
token_type_ids,
position_ids,
head_mask,
deterministic: bool = True,
output_attentions: bool = False,
output_hidden_states: bool = False,
return_dict: bool = True,
):
num_choices = input_ids.shape[1]
input_ids = input_ids.reshape(-1, input_ids.shape[-1]) if input_ids is not None else None
attention_mask = attention_mask.reshape(-1, attention_mask.shape[-1]) if attention_mask is not None else None
token_type_ids = token_type_ids.reshape(-1, token_type_ids.shape[-1]) if token_type_ids is not None else None
position_ids = position_ids.reshape(-1, position_ids.shape[-1]) if position_ids is not None else None
# Model
outputs = self.roberta(
input_ids,
attention_mask,
token_type_ids,
position_ids,
head_mask,
deterministic=deterministic,
output_attentions=output_attentions,
output_hidden_states=output_hidden_states,
return_dict=return_dict,
)
pooled_output = outputs[1]
pooled_output = self.dropout(pooled_output, deterministic=deterministic)
logits = self.classifier(pooled_output)
reshaped_logits = logits.reshape(-1, num_choices)
if not return_dict:
return (reshaped_logits,) + outputs[2:]
return FlaxMultipleChoiceModelOutput(
logits=reshaped_logits,
hidden_states=outputs.hidden_states,
attentions=outputs.attentions,
)
@add_start_docstrings(
"""
Roberta Model with a multiple choice classification head on top (a linear layer on top of the pooled output and a
softmax) e.g. for RocStories/SWAG tasks.
""",
ROBERTA_START_DOCSTRING,
)
class FlaxRobertaForMultipleChoice(FlaxRobertaPreTrainedModel):
module_class = FlaxRobertaForMultipleChoiceModule
overwrite_call_docstring(
FlaxRobertaForMultipleChoice, ROBERTA_INPUTS_DOCSTRING.format("batch_size, num_choices, sequence_length")
)
append_call_sample_docstring(
FlaxRobertaForMultipleChoice,
_CHECKPOINT_FOR_DOC,
FlaxMultipleChoiceModelOutput,
_CONFIG_FOR_DOC,
)
# Copied from transformers.models.bert.modeling_flax_bert.FlaxBertForTokenClassificationModule with Bert->Roberta, with self.bert->self.roberta
class FlaxRobertaForTokenClassificationModule(nn.Module):
config: RobertaConfig
dtype: jnp.dtype = jnp.float32
gradient_checkpointing: bool = False
def setup(self):
self.roberta = FlaxRobertaModule(
config=self.config,
dtype=self.dtype,
add_pooling_layer=False,
gradient_checkpointing=self.gradient_checkpointing,
)
classifier_dropout = (
self.config.classifier_dropout
if self.config.classifier_dropout is not None
else self.config.hidden_dropout_prob
)
self.dropout = nn.Dropout(rate=classifier_dropout)
self.classifier = nn.Dense(self.config.num_labels, dtype=self.dtype)
def __call__(
self,
input_ids,
attention_mask,
token_type_ids,
position_ids,
head_mask,
deterministic: bool = True,
output_attentions: bool = False,
output_hidden_states: bool = False,
return_dict: bool = True,
):
# Model
outputs = self.roberta(
input_ids,
attention_mask,
token_type_ids,
position_ids,
head_mask,
deterministic=deterministic,
output_attentions=output_attentions,
output_hidden_states=output_hidden_states,
return_dict=return_dict,
)
hidden_states = outputs[0]
hidden_states = self.dropout(hidden_states, deterministic=deterministic)
logits = self.classifier(hidden_states)
if not return_dict:
return (logits,) + outputs[1:]
return FlaxTokenClassifierOutput(
logits=logits,
hidden_states=outputs.hidden_states,
attentions=outputs.attentions,
)
@add_start_docstrings(
"""
Roberta Model with a token classification head on top (a linear layer on top of the hidden-states output) e.g. for
Named-Entity-Recognition (NER) tasks.
""",
ROBERTA_START_DOCSTRING,
)
class FlaxRobertaForTokenClassification(FlaxRobertaPreTrainedModel):
module_class = FlaxRobertaForTokenClassificationModule
append_call_sample_docstring(
FlaxRobertaForTokenClassification,
_CHECKPOINT_FOR_DOC,
FlaxTokenClassifierOutput,
_CONFIG_FOR_DOC,
)
# Copied from transformers.models.bert.modeling_flax_bert.FlaxBertForQuestionAnsweringModule with Bert->Roberta, with self.bert->self.roberta
class FlaxRobertaForQuestionAnsweringModule(nn.Module):
config: RobertaConfig
dtype: jnp.dtype = jnp.float32
gradient_checkpointing: bool = False
def setup(self):
self.roberta = FlaxRobertaModule(
config=self.config,
dtype=self.dtype,
add_pooling_layer=False,
gradient_checkpointing=self.gradient_checkpointing,
)
self.qa_outputs = nn.Dense(self.config.num_labels, dtype=self.dtype)
def __call__(
self,
input_ids,
attention_mask,
token_type_ids,
position_ids,
head_mask,
deterministic: bool = True,
output_attentions: bool = False,
output_hidden_states: bool = False,
return_dict: bool = True,
):
# Model
outputs = self.roberta(
input_ids,
attention_mask,
token_type_ids,
position_ids,
head_mask,
deterministic=deterministic,
output_attentions=output_attentions,
output_hidden_states=output_hidden_states,
return_dict=return_dict,
)
hidden_states = outputs[0]
logits = self.qa_outputs(hidden_states)
start_logits, end_logits = jnp.split(logits, self.config.num_labels, axis=-1)
start_logits = start_logits.squeeze(-1)
end_logits = end_logits.squeeze(-1)
if not return_dict:
return (start_logits, end_logits) + outputs[1:]
return FlaxQuestionAnsweringModelOutput(
start_logits=start_logits,
end_logits=end_logits,
hidden_states=outputs.hidden_states,
attentions=outputs.attentions,
)
@add_start_docstrings(
"""
Roberta Model with a span classification head on top for extractive question-answering tasks like SQuAD (a linear
layers on top of the hidden-states output to compute `span start logits` and `span end logits`).
""",
ROBERTA_START_DOCSTRING,
)
class FlaxRobertaForQuestionAnswering(FlaxRobertaPreTrainedModel):
module_class = FlaxRobertaForQuestionAnsweringModule
append_call_sample_docstring(
FlaxRobertaForQuestionAnswering,
_CHECKPOINT_FOR_DOC,
FlaxQuestionAnsweringModelOutput,
_CONFIG_FOR_DOC,
)
class FlaxRobertaForCausalLMModule(nn.Module):
config: RobertaConfig
dtype: jnp.dtype = jnp.float32
gradient_checkpointing: bool = False
def setup(self):
self.roberta = FlaxRobertaModule(
config=self.config,
add_pooling_layer=False,
dtype=self.dtype,
gradient_checkpointing=self.gradient_checkpointing,
)
self.lm_head = FlaxRobertaLMHead(config=self.config, dtype=self.dtype)
def __call__(
self,
input_ids,
attention_mask,
position_ids,
token_type_ids: Optional[jnp.ndarray] = None,
head_mask: Optional[jnp.ndarray] = None,
encoder_hidden_states: Optional[jnp.ndarray] = None,
encoder_attention_mask: Optional[jnp.ndarray] = None,
init_cache: bool = False,
deterministic: bool = True,
output_attentions: bool = False,
output_hidden_states: bool = False,
return_dict: bool = True,
):
# Model
outputs = self.roberta(
input_ids,
attention_mask,
token_type_ids,
position_ids,
head_mask,
encoder_hidden_states=encoder_hidden_states,
encoder_attention_mask=encoder_attention_mask,
init_cache=init_cache,
deterministic=deterministic,
output_attentions=output_attentions,
output_hidden_states=output_hidden_states,
return_dict=return_dict,
)
hidden_states = outputs[0]
if self.config.tie_word_embeddings:
shared_embedding = self.roberta.variables["params"]["embeddings"]["word_embeddings"]["embedding"]
else:
shared_embedding = None
# Compute the prediction scores
logits = self.lm_head(hidden_states, shared_embedding=shared_embedding)
if not return_dict:
return (logits,) + outputs[1:]
return FlaxCausalLMOutputWithCrossAttentions(
logits=logits,
hidden_states=outputs.hidden_states,
attentions=outputs.attentions,
cross_attentions=outputs.cross_attentions,
)
@add_start_docstrings(
"""
Roberta Model with a language modeling head on top (a linear layer on top of the hidden-states output) e.g for
autoregressive tasks.
""",
ROBERTA_START_DOCSTRING,
)
class FlaxRobertaForCausalLM(FlaxRobertaPreTrainedModel):
module_class = FlaxRobertaForCausalLMModule
def prepare_inputs_for_generation(self, input_ids, max_length, attention_mask: Optional[jax.Array] = None):
# initializing the cache
batch_size, seq_length = input_ids.shape
past_key_values = self.init_cache(batch_size, max_length)
# Note that usually one would have to put 0's in the attention_mask for x > input_ids.shape[-1] and x < cache_length.
# But since the decoder uses a causal mask, those positions are masked anyway.
# Thus, we can create a single static attention_mask here, which is more efficient for compilation
extended_attention_mask = jnp.ones((batch_size, max_length), dtype="i4")
if attention_mask is not None:
position_ids = attention_mask.cumsum(axis=-1) - 1
extended_attention_mask = lax.dynamic_update_slice(extended_attention_mask, attention_mask, (0, 0))
else:
position_ids = jnp.broadcast_to(jnp.arange(seq_length, dtype="i4")[None, :], (batch_size, seq_length))
return {
"past_key_values": past_key_values,
"attention_mask": extended_attention_mask,
"position_ids": position_ids,
}
def update_inputs_for_generation(self, model_outputs, model_kwargs):
model_kwargs["past_key_values"] = model_outputs.past_key_values
model_kwargs["position_ids"] = model_kwargs["position_ids"][:, -1:] + 1
return model_kwargs
append_call_sample_docstring(
FlaxRobertaForCausalLM,
_CHECKPOINT_FOR_DOC,
FlaxCausalLMOutputWithCrossAttentions,
_CONFIG_FOR_DOC,
)
| transformers/src/transformers/models/roberta/modeling_flax_roberta.py/0 | {
"file_path": "transformers/src/transformers/models/roberta/modeling_flax_roberta.py",
"repo_id": "transformers",
"token_count": 25323
} | 357 |
# coding=utf-8
# Copyright 2021 The HuggingFace Inc. team. All rights reserved.
#
# Licensed under the Apache License, Version 2.0 (the "License");
# you may not use this file except in compliance with the License.
# You may obtain a copy of the License at
#
# http://www.apache.org/licenses/LICENSE-2.0
#
# Unless required by applicable law or agreed to in writing, software
# distributed under the License is distributed on an "AS IS" BASIS,
# WITHOUT WARRANTIES OR CONDITIONS OF ANY KIND, either express or implied.
# See the License for the specific language governing permissions and
# limitations under the License.
""" RoFormer model configuration"""
from collections import OrderedDict
from typing import Mapping
from ...configuration_utils import PretrainedConfig
from ...onnx import OnnxConfig
from ...utils import logging
logger = logging.get_logger(__name__)
ROFORMER_PRETRAINED_CONFIG_ARCHIVE_MAP = {
"junnyu/roformer_chinese_small": "https://huggingface.co/junnyu/roformer_chinese_small/resolve/main/config.json",
"junnyu/roformer_chinese_base": "https://huggingface.co/junnyu/roformer_chinese_base/resolve/main/config.json",
"junnyu/roformer_chinese_char_small": (
"https://huggingface.co/junnyu/roformer_chinese_char_small/resolve/main/config.json"
),
"junnyu/roformer_chinese_char_base": (
"https://huggingface.co/junnyu/roformer_chinese_char_base/resolve/main/config.json"
),
"junnyu/roformer_small_discriminator": (
"https://huggingface.co/junnyu/roformer_small_discriminator/resolve/main/config.json"
),
"junnyu/roformer_small_generator": (
"https://huggingface.co/junnyu/roformer_small_generator/resolve/main/config.json"
),
# See all RoFormer models at https://huggingface.co/models?filter=roformer
}
class RoFormerConfig(PretrainedConfig):
r"""
This is the configuration class to store the configuration of a [`RoFormerModel`]. It is used to instantiate an
RoFormer model according to the specified arguments, defining the model architecture. Instantiating a configuration
with the defaults will yield a similar configuration to that of the RoFormer
[junnyu/roformer_chinese_base](https://huggingface.co/junnyu/roformer_chinese_base) architecture.
Configuration objects inherit from [`PretrainedConfig`] and can be used to control the model outputs. Read the
documentation from [`PretrainedConfig`] for more information.
Args:
vocab_size (`int`, *optional*, defaults to 50000):
Vocabulary size of the RoFormer model. Defines the number of different tokens that can be represented by
the `inputs_ids` passed when calling [`RoFormerModel`] or [`TFRoFormerModel`].
embedding_size (`int`, *optional*, defaults to None):
Dimensionality of the encoder layers and the pooler layer. Defaults to the `hidden_size` if not provided.
hidden_size (`int`, *optional*, defaults to 768):
Dimension of the encoder layers and the pooler layer.
num_hidden_layers (`int`, *optional*, defaults to 12):
Number of hidden layers in the Transformer encoder.
num_attention_heads (`int`, *optional*, defaults to 12):
Number of attention heads for each attention layer in the Transformer encoder.
intermediate_size (`int`, *optional*, defaults to 3072):
Dimension of the "intermediate" (i.e., feed-forward) layer in the Transformer encoder.
hidden_act (`str` or `function`, *optional*, defaults to `"gelu"`):
The non-linear activation function (function or string) in the encoder and pooler. If string, `"gelu"`,
`"relu"`, `"selu"` and `"gelu_new"` are supported.
hidden_dropout_prob (`float`, *optional*, defaults to 0.1):
The dropout probability for all fully connected layers in the embeddings, encoder, and pooler.
attention_probs_dropout_prob (`float`, *optional*, defaults to 0.1):
The dropout ratio for the attention probabilities.
max_position_embeddings (`int`, *optional*, defaults to 1536):
The maximum sequence length that this model might ever be used with. Typically set this to something large
just in case (e.g., 512 or 1024 or 1536).
type_vocab_size (`int`, *optional*, defaults to 2):
The vocabulary size of the `token_type_ids` passed when calling [`RoFormerModel`] or [`TFRoFormerModel`].
initializer_range (`float`, *optional*, defaults to 0.02):
The standard deviation of the truncated_normal_initializer for initializing all weight matrices.
layer_norm_eps (`float`, *optional*, defaults to 1e-12):
The epsilon used by the layer normalization layers.
is_decoder (`bool`, *optional*, defaults to `False`):
Whether the model is used as a decoder or not. If `False`, the model is used as an encoder.
use_cache (`bool`, *optional*, defaults to `True`):
Whether or not the model should return the last key/values attentions (not used by all models). Only
relevant if `config.is_decoder=True`.
rotary_value (`bool`, *optional*, defaults to `False`):
Whether or not apply rotary position embeddings on value layer.
Example:
```python
>>> from transformers import RoFormerModel, RoFormerConfig
>>> # Initializing a RoFormer junnyu/roformer_chinese_base style configuration
>>> configuration = RoFormerConfig()
>>> # Initializing a model (with random weights) from the junnyu/roformer_chinese_base style configuration
>>> model = RoFormerModel(configuration)
>>> # Accessing the model configuration
>>> configuration = model.config
```"""
model_type = "roformer"
def __init__(
self,
vocab_size=50000,
embedding_size=None,
hidden_size=768,
num_hidden_layers=12,
num_attention_heads=12,
intermediate_size=3072,
hidden_act="gelu",
hidden_dropout_prob=0.1,
attention_probs_dropout_prob=0.1,
max_position_embeddings=1536,
type_vocab_size=2,
initializer_range=0.02,
layer_norm_eps=1e-12,
pad_token_id=0,
rotary_value=False,
use_cache=True,
**kwargs,
):
super().__init__(pad_token_id=pad_token_id, **kwargs)
self.vocab_size = vocab_size
self.embedding_size = hidden_size if embedding_size is None else embedding_size
self.hidden_size = hidden_size
self.num_hidden_layers = num_hidden_layers
self.num_attention_heads = num_attention_heads
self.hidden_act = hidden_act
self.intermediate_size = intermediate_size
self.hidden_dropout_prob = hidden_dropout_prob
self.attention_probs_dropout_prob = attention_probs_dropout_prob
self.max_position_embeddings = max_position_embeddings
self.type_vocab_size = type_vocab_size
self.initializer_range = initializer_range
self.layer_norm_eps = layer_norm_eps
self.rotary_value = rotary_value
self.use_cache = use_cache
class RoFormerOnnxConfig(OnnxConfig):
@property
def inputs(self) -> Mapping[str, Mapping[int, str]]:
if self.task == "multiple-choice":
dynamic_axis = {0: "batch", 1: "choice", 2: "sequence"}
else:
dynamic_axis = {0: "batch", 1: "sequence"}
dynamic_axis = {0: "batch", 1: "sequence"}
return OrderedDict(
[
("input_ids", dynamic_axis),
("attention_mask", dynamic_axis),
("token_type_ids", dynamic_axis),
]
)
| transformers/src/transformers/models/roformer/configuration_roformer.py/0 | {
"file_path": "transformers/src/transformers/models/roformer/configuration_roformer.py",
"repo_id": "transformers",
"token_count": 2947
} | 358 |
# coding=utf-8
# Copyright 2023 The Meta AI Authors and The HuggingFace Team. All rights reserved.
#
# Licensed under the Apache License, Version 2.0 (the "License");
# you may not use this file except in compliance with the License.
# You may obtain a copy of the License at
#
# http://www.apache.org/licenses/LICENSE-2.0
#
# Unless required by applicable law or agreed to in writing, software
# distributed under the License is distributed on an "AS IS" BASIS,
# WITHOUT WARRANTIES OR CONDITIONS OF ANY KIND, either express or implied.
# See the License for the specific language governing permissions and
# limitations under the License.
""" PyTorch SAM model."""
import collections
import math
from dataclasses import dataclass
from typing import Dict, List, Optional, Tuple, Union
import numpy as np
import torch
import torch.nn.functional as F
import torch.utils.checkpoint
from torch import Tensor, nn
from ...activations import ACT2FN
from ...modeling_outputs import BaseModelOutput
from ...modeling_utils import PreTrainedModel
from ...utils import ModelOutput, add_start_docstrings, add_start_docstrings_to_model_forward, logging
from .configuration_sam import SamConfig, SamMaskDecoderConfig, SamPromptEncoderConfig, SamVisionConfig
logger = logging.get_logger(__name__)
_CONFIG_FOR_DOC = "SamConfig"
_CHECKPOINT_FOR_DOC = "facebook/sam-vit-huge"
SAM_PRETRAINED_MODEL_ARCHIVE_LIST = [
"facebook/sam-vit-huge",
"facebook/sam-vit-large",
"facebook/sam-vit-base",
# See all SAM models at https://huggingface.co/models?filter=sam
]
@dataclass
class SamVisionEncoderOutput(ModelOutput):
"""
Base class for sam vision model's outputs that also contains image embeddings obtained by applying the projection
layer to the pooler_output.
Args:
image_embeds (`torch.FloatTensor` of shape `(batch_size, output_dim)` *optional* returned when model is initialized with `with_projection=True`):
The image embeddings obtained by applying the projection layer to the pooler_output.
last_hidden_state (`torch.FloatTensor` of shape `(batch_size, sequence_length, hidden_size)`):
Sequence of hidden-states at the output of the last layer of the model.
hidden_states (`tuple(torch.FloatTensor)`, *optional*, returned when `output_hidden_states=True` is passed or when `config.output_hidden_states=True`):
Tuple of `torch.FloatTensor` (one for the output of the embeddings, if the model has an embedding layer, +
one for the output of each layer) of shape `(batch_size, sequence_length, hidden_size)`.
Hidden-states of the model at the output of each layer plus the optional initial embedding outputs.
attentions (`tuple(torch.FloatTensor)`, *optional*, returned when `output_attentions=True` is passed or when `config.output_attentions=True`):
Tuple of `torch.FloatTensor` (one for each layer) of shape `(batch_size, num_heads, sequence_length,
sequence_length)`.
Attentions weights after the attention softmax, used to compute the weighted average in the self-attention
heads.
"""
image_embeds: Optional[torch.FloatTensor] = None
last_hidden_state: torch.FloatTensor = None
hidden_states: Optional[Tuple[torch.FloatTensor, ...]] = None
attentions: Optional[Tuple[torch.FloatTensor, ...]] = None
@dataclass
class SamImageSegmentationOutput(ModelOutput):
"""
Base class for Segment-Anything model's output
Args:
iou_scores (`torch.FloatTensor` of shape `(batch_size, num_masks)`):
The iou scores of the predicted masks.
pred_masks (`torch.FloatTensor` of shape `(batch_size, num_masks, height, width)`):
The predicted low resolutions masks. Needs to be post-processed by the processor
vision_hidden_states (`tuple(torch.FloatTensor)`, *optional*, returned when `output_hidden_states=True` is passed or when `config.output_hidden_states=True`):
Tuple of `torch.FloatTensor` (one for the output of the embeddings, if the model has an embedding layer, +
one for the output of each layer) of shape `(batch_size, sequence_length, hidden_size)`.
Hidden-states of the vision model at the output of each layer plus the optional initial embedding outputs.
vision_attentions (`tuple(torch.FloatTensor)`, *optional*, returned when `output_attentions=True` is passed or when `config.output_attentions=True`):
Tuple of `torch.FloatTensor` (one for each layer) of shape `(batch_size, num_heads, sequence_length,
sequence_length)`.
Attentions weights after the attention softmax, used to compute the weighted average in the self-attention
heads.
mask_decoder_attentions (`tuple(torch.FloatTensor)`, *optional*, returned when `output_attentions=True` is passed or when `config.output_attentions=True`):
Tuple of `torch.FloatTensor` (one for each layer) of shape `(batch_size, num_heads, sequence_length,
sequence_length)`.
Attentions weights after the attention softmax, used to compute the weighted average in the self-attention
heads.
"""
iou_scores: torch.FloatTensor = None
pred_masks: torch.FloatTensor = None
vision_hidden_states: Optional[Tuple[torch.FloatTensor, ...]] = None
vision_attentions: Optional[Tuple[torch.FloatTensor, ...]] = None
mask_decoder_attentions: Optional[Tuple[torch.FloatTensor, ...]] = None
class SamPatchEmbeddings(nn.Module):
"""
This class turns `pixel_values` of shape `(batch_size, num_channels, height, width)` into the initial
`hidden_states` (patch embeddings) of shape `(batch_size, seq_length, hidden_size)` to be consumed by a
Transformer.
"""
def __init__(self, config):
super().__init__()
image_size, patch_size = config.image_size, config.patch_size
num_channels, hidden_size = config.num_channels, config.hidden_size
image_size = image_size if isinstance(image_size, collections.abc.Iterable) else (image_size, image_size)
patch_size = patch_size if isinstance(patch_size, collections.abc.Iterable) else (patch_size, patch_size)
num_patches = (image_size[1] // patch_size[1]) * (image_size[0] // patch_size[0])
self.image_size = image_size
self.patch_size = patch_size
self.num_channels = num_channels
self.num_patches = num_patches
self.projection = nn.Conv2d(num_channels, hidden_size, kernel_size=patch_size, stride=patch_size)
def forward(self, pixel_values):
batch_size, num_channels, height, width = pixel_values.shape
if num_channels != self.num_channels:
raise ValueError(
"Make sure that the channel dimension of the pixel values match with the one set in the configuration."
)
if height != self.image_size[0] or width != self.image_size[1]:
raise ValueError(
f"Input image size ({height}*{width}) doesn't match model ({self.image_size[0]}*{self.image_size[1]})."
)
embeddings = self.projection(pixel_values).permute(0, 2, 3, 1)
return embeddings
class SamMLPBlock(nn.Module):
def __init__(self, config):
super().__init__()
self.lin1 = nn.Linear(config.hidden_size, config.mlp_dim)
self.lin2 = nn.Linear(config.mlp_dim, config.hidden_size)
self.act = ACT2FN[config.hidden_act]
def forward(self, hidden_states: torch.Tensor) -> torch.Tensor:
hidden_states = self.lin1(hidden_states)
hidden_states = self.act(hidden_states)
hidden_states = self.lin2(hidden_states)
return hidden_states
# Copied from transformers.models.convnext.modeling_convnext.ConvNextLayerNorm with ConvNext->Sam
class SamLayerNorm(nn.Module):
r"""LayerNorm that supports two data formats: channels_last (default) or channels_first.
The ordering of the dimensions in the inputs. channels_last corresponds to inputs with shape (batch_size, height,
width, channels) while channels_first corresponds to inputs with shape (batch_size, channels, height, width).
"""
def __init__(self, normalized_shape, eps=1e-6, data_format="channels_last"):
super().__init__()
self.weight = nn.Parameter(torch.ones(normalized_shape))
self.bias = nn.Parameter(torch.zeros(normalized_shape))
self.eps = eps
self.data_format = data_format
if self.data_format not in ["channels_last", "channels_first"]:
raise NotImplementedError(f"Unsupported data format: {self.data_format}")
self.normalized_shape = (normalized_shape,)
def forward(self, x: torch.Tensor) -> torch.Tensor:
if self.data_format == "channels_last":
x = torch.nn.functional.layer_norm(x, self.normalized_shape, self.weight, self.bias, self.eps)
elif self.data_format == "channels_first":
input_dtype = x.dtype
x = x.float()
u = x.mean(1, keepdim=True)
s = (x - u).pow(2).mean(1, keepdim=True)
x = (x - u) / torch.sqrt(s + self.eps)
x = x.to(dtype=input_dtype)
x = self.weight[:, None, None] * x + self.bias[:, None, None]
return x
class SamAttention(nn.Module):
"""
SAM's attention layer that allows for downscaling the size of the embedding after projection to queries, keys, and
values.
"""
def __init__(self, config, downsample_rate=None):
super().__init__()
self.hidden_size = config.hidden_size
downsample_rate = config.attention_downsample_rate if downsample_rate is None else downsample_rate
self.internal_dim = config.hidden_size // downsample_rate
self.num_attention_heads = config.num_attention_heads
if self.internal_dim % config.num_attention_heads != 0:
raise ValueError("num_attention_heads must divide hidden_size.")
self.q_proj = nn.Linear(self.hidden_size, self.internal_dim)
self.k_proj = nn.Linear(self.hidden_size, self.internal_dim)
self.v_proj = nn.Linear(self.hidden_size, self.internal_dim)
self.out_proj = nn.Linear(self.internal_dim, self.hidden_size)
def _separate_heads(self, hidden_states: Tensor, num_attention_heads: int) -> Tensor:
batch, point_batch_size, n_tokens, channel = hidden_states.shape
c_per_head = channel // num_attention_heads
hidden_states = hidden_states.reshape(batch * point_batch_size, n_tokens, num_attention_heads, c_per_head)
return hidden_states.transpose(1, 2)
def _recombine_heads(self, hidden_states: Tensor, point_batch_size: int) -> Tensor:
batch, n_heads, n_tokens, c_per_head = hidden_states.shape
hidden_states = hidden_states.transpose(1, 2)
return hidden_states.reshape(batch // point_batch_size, point_batch_size, n_tokens, n_heads * c_per_head)
def forward(self, query: Tensor, key: Tensor, value: Tensor, attention_similarity: Tensor = None) -> Tensor:
# Input projections
query = self.q_proj(query)
key = self.k_proj(key)
value = self.v_proj(value)
point_batch_size = query.shape[1]
# Separate into heads
query = self._separate_heads(query, self.num_attention_heads)
key = self._separate_heads(key, self.num_attention_heads)
value = self._separate_heads(value, self.num_attention_heads)
# SamAttention
_, _, _, c_per_head = query.shape
attn = query @ key.permute(0, 1, 3, 2) # batch_size * point_batch_size x N_heads x N_tokens x N_tokens
attn = attn / math.sqrt(c_per_head)
attn = torch.softmax(attn, dim=-1)
if attention_similarity is not None:
attn = attn + attention_similarity
attn = torch.softmax(attn, dim=-1)
# Get output
out = attn @ value
out = self._recombine_heads(out, point_batch_size)
out = self.out_proj(out)
return out
class SamTwoWayAttentionBlock(nn.Module):
def __init__(self, config, attention_downsample_rate: int = 2, skip_first_layer_pe: bool = False):
"""
A transformer block with four layers:
(1) self-attention of sparse inputs (2) cross attention of sparse inputs -> dense inputs (3) mlp block on
sparse inputs (4) cross attention of dense inputs -> sparse inputs
Arguments:
config (`SamMaskDecoderConfig`):
The configuration file used to instantiate the block
attention_downsample_rate (*optionalk*, int, defaults to 2):
The downsample ratio of the block used to reduce the inner dim of the attention.
skip_first_layer_pe (*optional*, bool, defaults to `False`):
Whether or not to skip the addition of the query_point_embedding on the first layer.
"""
super().__init__()
self.hidden_size = config.hidden_size
self.layer_norm_eps = config.layer_norm_eps
self.self_attn = SamAttention(config, downsample_rate=1)
self.layer_norm1 = nn.LayerNorm(self.hidden_size, eps=self.layer_norm_eps)
self.cross_attn_token_to_image = SamAttention(config, downsample_rate=attention_downsample_rate)
self.layer_norm2 = nn.LayerNorm(self.hidden_size, eps=self.layer_norm_eps)
self.mlp = SamMLPBlock(config)
self.layer_norm3 = nn.LayerNorm(self.hidden_size, eps=self.layer_norm_eps)
self.layer_norm4 = nn.LayerNorm(self.hidden_size, eps=self.layer_norm_eps)
self.cross_attn_image_to_token = SamAttention(config, downsample_rate=attention_downsample_rate)
self.skip_first_layer_pe = skip_first_layer_pe
def forward(
self,
queries: Tensor,
keys: Tensor,
query_point_embedding: Tensor,
key_point_embedding: Tensor,
attention_similarity: Tensor,
output_attentions: bool = False,
):
# Self attention block
if self.skip_first_layer_pe:
queries = self.self_attn(query=queries, key=queries, value=queries)
else:
query = queries + query_point_embedding
attn_out = self.self_attn(query=query, key=query, value=queries)
queries = queries + attn_out
queries = self.layer_norm1(queries)
# Cross attention block, tokens attending to image embedding
query = queries + query_point_embedding
key = keys + key_point_embedding
attn_out = self.cross_attn_token_to_image(
query=query, key=key, value=keys, attention_similarity=attention_similarity
)
queries = queries + attn_out
queries = self.layer_norm2(queries)
# MLP block
mlp_out = self.mlp(queries)
queries = queries + mlp_out
queries = self.layer_norm3(queries)
# Cross attention block, image embedding attending to tokens
query = queries + query_point_embedding
key = keys + key_point_embedding
attn_out = self.cross_attn_image_to_token(query=key, key=query, value=queries)
keys = keys + attn_out
keys = self.layer_norm4(keys)
outputs = (queries, keys)
if output_attentions:
outputs = outputs + (attn_out,)
else:
outputs = outputs + (None,)
return outputs
class SamTwoWayTransformer(nn.Module):
def __init__(self, config: SamMaskDecoderConfig):
super().__init__()
self.config = config
self.num_hidden_layers = config.num_hidden_layers
self.layers = nn.ModuleList()
for i in range(self.num_hidden_layers):
self.layers.append(SamTwoWayAttentionBlock(config, skip_first_layer_pe=(i == 0)))
self.final_attn_token_to_image = SamAttention(config)
self.layer_norm_final_attn = nn.LayerNorm(config.hidden_size)
def forward(
self,
point_embeddings: Tensor,
image_embeddings: Tensor,
image_positional_embeddings: Tensor,
attention_similarity: Tensor,
target_embedding=None,
output_attentions: Optional[bool] = None,
output_hidden_states: Optional[bool] = None,
return_dict: Optional[bool] = None,
) -> Union[Tuple, BaseModelOutput]:
output_attentions = output_attentions if output_attentions is not None else self.config.output_attentions
output_hidden_states = (
output_hidden_states if output_hidden_states is not None else self.config.output_hidden_states
)
return_dict = return_dict if return_dict is not None else self.config.use_return_dict
all_attentions = ()
if image_embeddings is None:
raise ValueError("You have to specify an image_embedding")
image_embeddings = image_embeddings.flatten(2).permute(0, 2, 1).unsqueeze(1)
image_positional_embeddings = image_positional_embeddings.flatten(2).permute(0, 2, 1).unsqueeze(1)
# Prepare queries
queries = point_embeddings
keys = image_embeddings
# Apply transformer blocks and final layernorm
for layer in self.layers:
if target_embedding is not None:
queries += target_embedding
queries, keys, attention_outputs = layer(
queries=queries,
keys=keys,
query_point_embedding=point_embeddings,
key_point_embedding=image_positional_embeddings,
attention_similarity=attention_similarity,
output_attentions=output_attentions,
)
if output_attentions:
all_attentions = all_attentions + (attention_outputs,)
# Apply the final attenion layer from the points to the image
query = queries + point_embeddings
key = keys + image_positional_embeddings
attn_out = self.final_attn_token_to_image(query=query, key=key, value=keys)
queries = queries + attn_out
queries = self.layer_norm_final_attn(queries)
return queries, keys, all_attentions
class SamFeedForward(nn.Module):
def __init__(
self, input_dim: int, hidden_dim: int, output_dim: int, num_layers: int, sigmoid_output: bool = False
):
super().__init__()
self.num_layers = num_layers
self.activation = nn.ReLU()
self.proj_in = nn.Linear(input_dim, hidden_dim)
self.proj_out = nn.Linear(hidden_dim, output_dim)
self.layers = nn.ModuleList([nn.Linear(hidden_dim, hidden_dim) for _ in range(num_layers - 2)])
self.sigmoid_output = sigmoid_output
def forward(self, hidden_states):
hidden_states = self.proj_in(hidden_states)
hidden_states = self.activation(hidden_states)
for layer in self.layers:
hidden_states = self.activation(layer(hidden_states))
hidden_states = self.proj_out(hidden_states)
if self.sigmoid_output:
hidden_states = F.sigmoid(hidden_states)
return hidden_states
class SamMaskDecoder(nn.Module):
def __init__(self, config: SamMaskDecoderConfig):
super().__init__()
self.hidden_size = config.hidden_size
self.num_multimask_outputs = config.num_multimask_outputs
self.num_mask_tokens = config.num_multimask_outputs + 1
self.iou_token = nn.Embedding(1, self.hidden_size)
self.mask_tokens = nn.Embedding(self.num_mask_tokens, self.hidden_size)
self.transformer = SamTwoWayTransformer(config)
# should we create a new class for this?
self.upscale_conv1 = nn.ConvTranspose2d(self.hidden_size, self.hidden_size // 4, kernel_size=2, stride=2)
self.upscale_conv2 = nn.ConvTranspose2d(self.hidden_size // 4, self.hidden_size // 8, kernel_size=2, stride=2)
self.upscale_layer_norm = SamLayerNorm(self.hidden_size // 4, data_format="channels_first")
self.activation = nn.GELU()
mlps_list = []
for _ in range(self.num_mask_tokens):
mlps_list += [SamFeedForward(self.hidden_size, self.hidden_size, self.hidden_size // 8, 3)]
self.output_hypernetworks_mlps = nn.ModuleList(mlps_list)
self.iou_prediction_head = SamFeedForward(
self.hidden_size, config.iou_head_hidden_dim, self.num_mask_tokens, config.iou_head_depth
)
def forward(
self,
image_embeddings: torch.Tensor,
image_positional_embeddings: torch.Tensor,
sparse_prompt_embeddings: torch.Tensor,
dense_prompt_embeddings: torch.Tensor,
multimask_output: bool,
output_attentions: Optional[bool] = None,
attention_similarity: torch.Tensor = None,
target_embedding: torch.Tensor = None,
) -> Tuple[torch.Tensor, torch.Tensor]:
"""
Predict masks given image and prompt embeddings.
Args:
image_embeddings (`torch.Tensor`):
the embeddings from the image encoder
image_positional_embedding (`torch.Tensor`):
positional encoding with the shape of image_embeddings
sparse_prompt_embeddings (`torch.Tensor`):
The embeddings of the points and boxes
dense_prompt_embeddings (`torch.Tensor`):
the embeddings of the mask inputs
multimask_output (bool):
Whether to return multiple masks or a single mask.
output_attentions (bool, *optional*):
Whether or not to return the attentions tensors of all attention layers.
"""
batch_size, num_channels, height, width = image_embeddings.shape
point_batch_size = sparse_prompt_embeddings.shape[1]
# Concatenate output tokens
output_tokens = torch.cat([self.iou_token.weight, self.mask_tokens.weight], dim=0)
output_tokens = output_tokens.repeat(batch_size, point_batch_size, 1, 1)
if sparse_prompt_embeddings.sum().item() != 0:
tokens = torch.cat((output_tokens, sparse_prompt_embeddings), dim=2)
else:
tokens = output_tokens
point_embeddings = tokens.to(self.iou_token.weight.dtype)
# Expand per-image data in batch direction to be per-point
image_embeddings = image_embeddings + dense_prompt_embeddings
image_embeddings = image_embeddings.repeat_interleave(point_batch_size, 0)
image_positional_embeddings = image_positional_embeddings.repeat_interleave(point_batch_size, 0)
# Run the transformer, image_positional_embedding are consumed
point_embedding, image_embeddings, attentions = self.transformer(
point_embeddings=point_embeddings,
image_embeddings=image_embeddings,
image_positional_embeddings=image_positional_embeddings,
attention_similarity=attention_similarity,
target_embedding=target_embedding,
output_attentions=output_attentions,
)
iou_token_out = point_embedding[:, :, 0, :]
mask_tokens_out = point_embedding[:, :, 1 : (1 + self.num_mask_tokens), :]
# Upscale mask embeddings and predict masks using the mask tokens
image_embeddings = image_embeddings.transpose(2, 3).reshape(
batch_size * point_batch_size, num_channels, height, width
)
upscaled_embedding = self.upscale_conv1(image_embeddings)
upscaled_embedding = self.activation(self.upscale_layer_norm(upscaled_embedding))
upscaled_embedding = self.activation(self.upscale_conv2(upscaled_embedding))
hyper_in_list = []
for i in range(self.num_mask_tokens):
current_mlp = self.output_hypernetworks_mlps[i]
hyper_in_list += [current_mlp(mask_tokens_out[:, :, i, :])]
hyper_in = torch.stack(hyper_in_list, dim=2)
_, num_channels, height, width = upscaled_embedding.shape
upscaled_embedding = upscaled_embedding.reshape(batch_size, point_batch_size, num_channels, height * width)
masks = (hyper_in @ upscaled_embedding).reshape(batch_size, point_batch_size, -1, height, width)
# Generate mask quality predictions
iou_pred = self.iou_prediction_head(iou_token_out)
# Select the correct mask or masks for output
if multimask_output:
mask_slice = slice(1, None)
else:
mask_slice = slice(0, 1)
masks = masks[:, :, mask_slice, :, :]
iou_pred = iou_pred[:, :, mask_slice]
outputs = (masks, iou_pred)
if output_attentions:
outputs = outputs + (attentions,)
else:
outputs = outputs + (None,)
return outputs
class SamPositionalEmbedding(nn.Module):
def __init__(self, config):
super().__init__()
self.scale = config.hidden_size // 2
self.register_buffer("positional_embedding", self.scale * torch.randn((2, config.num_pos_feats)))
def forward(self, input_coords, input_shape=None):
"""Positionally encode points that are normalized to [0,1]."""
coordinates = input_coords.clone()
if input_shape is not None:
coordinates[:, :, :, 0] = coordinates[:, :, :, 0] / input_shape[1]
coordinates[:, :, :, 1] = coordinates[:, :, :, 1] / input_shape[0]
# assuming coords are in [0, 1]^2 square and have d_1 x ... x d_n x 2 shape
coordinates = 2 * coordinates - 1
coordinates = coordinates.to(self.positional_embedding.dtype)
coordinates = coordinates @ self.positional_embedding
coordinates = 2 * np.pi * coordinates
# outputs d_1 x ... x d_n x channel shape
return torch.cat([torch.sin(coordinates), torch.cos(coordinates)], dim=-1)
class SamMaskEmbedding(nn.Module):
def __init__(self, config: SamPromptEncoderConfig):
super().__init__()
self.mask_input_channels = config.mask_input_channels // 4
self.activation = ACT2FN[config.hidden_act]
self.conv1 = nn.Conv2d(1, self.mask_input_channels, kernel_size=2, stride=2)
self.conv2 = nn.Conv2d(self.mask_input_channels, config.mask_input_channels, kernel_size=2, stride=2)
self.conv3 = nn.Conv2d(config.mask_input_channels, config.hidden_size, kernel_size=1)
self.layer_norm1 = SamLayerNorm(
self.mask_input_channels, eps=config.layer_norm_eps, data_format="channels_first"
)
self.layer_norm2 = SamLayerNorm(
self.mask_input_channels * 4, eps=config.layer_norm_eps, data_format="channels_first"
)
def forward(self, masks):
hidden_states = self.conv1(masks)
hidden_states = self.layer_norm1(hidden_states)
hidden_states = self.activation(hidden_states)
hidden_states = self.conv2(hidden_states)
hidden_states = self.layer_norm2(hidden_states)
hidden_states = self.activation(hidden_states)
dense_embeddings = self.conv3(hidden_states)
return dense_embeddings
class SamPromptEncoder(nn.Module):
def __init__(self, config: SamPromptEncoderConfig, shared_patch_embedding):
super().__init__()
self.shared_embedding = shared_patch_embedding
self.mask_embed = SamMaskEmbedding(config)
self.no_mask_embed = nn.Embedding(1, config.hidden_size)
self.image_embedding_size = (config.image_embedding_size, config.image_embedding_size)
self.input_image_size = config.image_size
self.point_embed = nn.ModuleList(
[nn.Embedding(1, config.hidden_size) for i in range(config.num_point_embeddings)]
)
self.hidden_size = config.hidden_size
self.not_a_point_embed = nn.Embedding(1, config.hidden_size)
def _embed_points(self, points: torch.Tensor, labels: torch.Tensor, pad: bool) -> torch.Tensor:
"""Embeds point prompts."""
points = points + 0.5 # Shift to center of pixel
if pad:
target_point_shape = (points.shape[0], points.shape[1], 1, points.shape[-1])
target_labels_shape = (points.shape[0], points.shape[1], 1)
padding_point = torch.zeros(target_point_shape, device=points.device)
padding_label = -torch.ones(target_labels_shape, device=labels.device)
points = torch.cat([points, padding_point], dim=2)
labels = torch.cat([labels, padding_label], dim=2)
input_shape = (self.input_image_size, self.input_image_size)
point_embedding = self.shared_embedding(points, input_shape)
# torch.where and expanding the labels tensor is required by the ONNX export
point_embedding = torch.where(labels[..., None] == -1, self.not_a_point_embed.weight, point_embedding)
# This is required for the ONNX export. The dtype, device need to be explicitely
# specificed as otherwise torch.onnx.export interprets as double
point_embedding = torch.where(
labels[..., None] != -10,
point_embedding,
torch.tensor(0.0, dtype=point_embedding.dtype, device=point_embedding.device),
)
point_embedding = torch.where(
(labels == 0)[:, :, :, None],
point_embedding + self.point_embed[0].weight[None, None, :, :],
point_embedding,
)
point_embedding = torch.where(
(labels == 1)[:, :, :, None],
point_embedding + self.point_embed[1].weight[None, None, :, :],
point_embedding,
)
return point_embedding
def _embed_boxes(self, boxes: torch.Tensor) -> torch.Tensor:
"""Embeds box prompts."""
boxes = boxes + 0.5 # Shift to center of pixel
batch_size, nb_boxes = boxes.shape[:2]
coords = boxes.reshape(batch_size, nb_boxes, 2, 2)
input_shape = (self.input_image_size, self.input_image_size)
corner_embedding = self.shared_embedding(coords, input_shape)
corner_embedding[:, :, 0, :] += self.point_embed[2].weight
corner_embedding[:, :, 1, :] += self.point_embed[3].weight
return corner_embedding
def forward(
self,
input_points: Optional[Tuple[torch.Tensor, torch.Tensor]],
input_labels: Optional[torch.Tensor],
input_boxes: Optional[torch.Tensor],
input_masks: Optional[torch.Tensor],
) -> Tuple[torch.Tensor, torch.Tensor]:
"""
Embeds different types of prompts, returning both sparse and dense embeddings.
Args:
points (`torch.Tensor`, *optional*):
point coordinates and labels to embed.
boxes (`torch.Tensor`, *optional*):
boxes to embed
masks (`torch.Tensor`, *optional*):
masks to embed
"""
sparse_embeddings = None
batch_size = 1
target_device = self.shared_embedding.positional_embedding.device
if input_points is not None:
batch_size, point_batch_size = input_points.shape[:2]
if input_labels is None:
raise ValueError("If points are provided, labels must also be provided.")
point_embeddings = self._embed_points(input_points, input_labels, pad=(input_boxes is None))
sparse_embeddings = point_embeddings
if input_boxes is not None:
batch_size = input_boxes.shape[0]
box_embeddings = self._embed_boxes(input_boxes)
if sparse_embeddings is None:
sparse_embeddings = box_embeddings
else:
sparse_embeddings = torch.cat([sparse_embeddings, box_embeddings], dim=2)
if input_masks is not None:
dense_embeddings = self.mask_embed(input_masks)
else:
dense_embeddings = self.no_mask_embed.weight.reshape(1, -1, 1, 1).expand(
batch_size, -1, self.image_embedding_size[0], self.image_embedding_size[1]
)
if sparse_embeddings is None:
sparse_embeddings = torch.zeros((batch_size, 1, 1, self.hidden_size), device=target_device)
return sparse_embeddings, dense_embeddings
class SamVisionAttention(nn.Module):
"""Multi-head Attention block with relative position embeddings."""
def __init__(self, config, window_size):
super().__init__()
input_size = (
(config.image_size // config.patch_size, config.image_size // config.patch_size)
if window_size == 0
else (window_size, window_size)
)
self.num_attention_heads = config.num_attention_heads
head_dim = config.hidden_size // config.num_attention_heads
self.scale = head_dim**-0.5
self.dropout = config.attention_dropout
self.qkv = nn.Linear(config.hidden_size, config.hidden_size * 3, bias=config.qkv_bias)
self.proj = nn.Linear(config.hidden_size, config.hidden_size)
self.use_rel_pos = config.use_rel_pos
if self.use_rel_pos:
if input_size is None:
raise ValueError("Input size must be provided if using relative positional encoding.")
# initialize relative positional embeddings
self.rel_pos_h = nn.Parameter(torch.zeros(2 * input_size[0] - 1, head_dim))
self.rel_pos_w = nn.Parameter(torch.zeros(2 * input_size[1] - 1, head_dim))
def get_rel_pos(self, q_size: int, k_size: int, rel_pos: torch.Tensor) -> torch.Tensor:
"""
Get relative positional embeddings according to the relative positions of
query and key sizes.
Args:
q_size (int):
size of the query.
k_size (int):
size of key k.
rel_pos (`torch.Tensor`):
relative position embeddings (L, channel).
Returns:
Extracted positional embeddings according to relative positions.
"""
max_rel_dist = int(2 * max(q_size, k_size) - 1)
# Interpolate rel pos.
rel_pos_resized = F.interpolate(
rel_pos.reshape(1, rel_pos.shape[0], -1).permute(0, 2, 1),
size=max_rel_dist,
mode="linear",
)
rel_pos_resized = rel_pos_resized.reshape(-1, max_rel_dist).permute(1, 0)
# Scale the coords with short length if shapes for q and k are different.
q_coords = torch.arange(q_size)[:, None] * max(k_size / q_size, 1.0)
k_coords = torch.arange(k_size)[None, :] * max(q_size / k_size, 1.0)
relative_coords = (q_coords - k_coords) + (k_size - 1) * max(q_size / k_size, 1.0)
return rel_pos_resized[relative_coords.long()]
def add_decomposed_rel_pos(
self,
attn: torch.Tensor,
query: torch.Tensor,
rel_pos_h: torch.Tensor,
rel_pos_w: torch.Tensor,
q_size: Tuple[int, int],
k_size: Tuple[int, int],
) -> torch.Tensor:
"""
Calculate decomposed Relative Positional Embeddings from :paper:`mvitv2`.
https://github.com/facebookresearch/mvit/blob/19786631e330df9f3622e5402b4a419a263a2c80/mvit/models/attention.py
Args:
attn (`torch.Tensor`):
attention map.
query (`torch.Tensor`):
query q in the attention layer with shape (batch_size, query_height * query_width, channel).
rel_pos_h (`torch.Tensor`):
relative position embeddings (Lh, channel) for height axis.
rel_pos_w (`torch.Tensor`):
relative position embeddings (Lw, channel) for width axis.
q_size (tuple):
spatial sequence size of query q with (query_height, query_width).
k_size (tuple):
spatial sequence size of key k with (key_height, key_width).
Returns:
attn (`torch.Tensor`):
attention map with added relative positional embeddings.
"""
query_height, query_width = q_size
key_height, key_width = k_size
relative_position_height = self.get_rel_pos(query_height, key_height, rel_pos_h)
relative_position_width = self.get_rel_pos(query_width, key_width, rel_pos_w)
batch_size, _, dim = query.shape
reshaped_query = query.reshape(batch_size, query_height, query_width, dim)
rel_h = torch.einsum("bhwc,hkc->bhwk", reshaped_query, relative_position_height)
rel_w = torch.einsum("bhwc,wkc->bhwk", reshaped_query, relative_position_width)
attn = attn.reshape(batch_size, query_height, query_width, key_height, key_width)
attn = attn + rel_h[:, :, :, :, None] + rel_w[:, :, :, None, :]
attn = attn.reshape(batch_size, query_height * query_width, key_height * key_width)
return attn
def forward(self, hidden_states: torch.Tensor, output_attentions=False) -> torch.Tensor:
batch_size, height, width, _ = hidden_states.shape
# qkv with shape (3, batch_size, nHead, height * width, channel)
qkv = (
self.qkv(hidden_states)
.reshape(batch_size, height * width, 3, self.num_attention_heads, -1)
.permute(2, 0, 3, 1, 4)
)
# q, k, v with shape (batch_size * nHead, height * width, channel)
query, key, value = qkv.reshape(3, batch_size * self.num_attention_heads, height * width, -1).unbind(0)
attn_weights = (query * self.scale) @ key.transpose(-2, -1)
if self.use_rel_pos:
attn_weights = self.add_decomposed_rel_pos(
attn_weights, query, self.rel_pos_h, self.rel_pos_w, (height, width), (height, width)
)
attn_weights = torch.nn.functional.softmax(attn_weights, dtype=torch.float32, dim=-1).to(query.dtype)
attn_probs = nn.functional.dropout(attn_weights, p=self.dropout, training=self.training)
attn_output = (attn_probs @ value).reshape(batch_size, self.num_attention_heads, height, width, -1)
attn_output = attn_output.permute(0, 2, 3, 1, 4).reshape(batch_size, height, width, -1)
attn_output = self.proj(attn_output)
if output_attentions:
outputs = (attn_output, attn_weights)
else:
outputs = (attn_output, None)
return outputs
class SamVisionLayer(nn.Module):
def __init__(self, config, window_size):
super().__init__()
self.layer_norm1 = nn.LayerNorm(config.hidden_size, eps=config.layer_norm_eps)
self.attn = SamVisionAttention(config, window_size)
self.layer_norm2 = nn.LayerNorm(config.hidden_size, eps=config.layer_norm_eps)
self.mlp = SamMLPBlock(config)
self.window_size = window_size
def window_partition(self, hidden_states: torch.Tensor, window_size: int) -> Tuple[torch.Tensor, Tuple[int, int]]:
"""
Args:
Partition into non-overlapping windows with padding if needed.
hidden_states (tensor): input tokens with [batch_size, height, width, channel]. window_size (int): window
size.
Returns:
windows: windows after partition with [batch_size * num_windows, window_size, window_size, channel].
(pad_height, pad_width): padded height and width before partition
"""
batch_size, height, width, channel = hidden_states.shape
pad_h = (window_size - height % window_size) % window_size
pad_w = (window_size - width % window_size) % window_size
hidden_states = F.pad(hidden_states, (0, 0, 0, pad_w, 0, pad_h))
pad_height, pad_width = height + pad_h, width + pad_w
hidden_states = hidden_states.reshape(
batch_size, pad_height // window_size, window_size, pad_width // window_size, window_size, channel
)
windows = hidden_states.permute(0, 1, 3, 2, 4, 5).contiguous().reshape(-1, window_size, window_size, channel)
return windows, (pad_height, pad_width)
def window_unpartition(
self, windows: torch.Tensor, window_size: int, padding_shape: Tuple[int, int], original_shape: Tuple[int, int]
) -> torch.Tensor:
"""
Args:
Window unpartition into original sequences and removing padding.
hidden_states (tensor):
input tokens with [batch_size * num_windows, window_size, window_size, channel].
window_size (int):
window size.
padding_shape (Tuple):
padded height and width (pad_height, pad_width).
original_shape (Tuple): original height and width (height, width) before padding.
Returns:
hidden_states: unpartitioned sequences with [batch_size, height, width, channel].
"""
pad_height, pad_width = padding_shape
height, width = original_shape
batch_size = windows.shape[0] // (pad_height * pad_width // window_size // window_size)
hidden_states = windows.reshape(
batch_size, pad_height // window_size, pad_width // window_size, window_size, window_size, -1
)
hidden_states = (
hidden_states.permute(0, 1, 3, 2, 4, 5).contiguous().reshape(batch_size, pad_height, pad_width, -1)
)
hidden_states = hidden_states[:, :height, :width, :].contiguous()
return hidden_states
def forward(
self,
hidden_states: torch.Tensor,
output_attentions: Optional[bool] = False,
) -> Tuple[torch.FloatTensor]:
residual = hidden_states
hidden_states = self.layer_norm1(hidden_states)
# Window partition
if self.window_size > 0:
height, width = hidden_states.shape[1], hidden_states.shape[2]
hidden_states, padding_shape = self.window_partition(hidden_states, self.window_size)
hidden_states, attn_weights = self.attn(
hidden_states=hidden_states,
output_attentions=output_attentions,
)
# Reverse window partition
if self.window_size > 0:
hidden_states = self.window_unpartition(hidden_states, self.window_size, padding_shape, (height, width))
hidden_states = residual + hidden_states
layernorm_output = self.layer_norm2(hidden_states)
hidden_states = hidden_states + self.mlp(layernorm_output)
outputs = (hidden_states,)
if output_attentions:
outputs += (attn_weights,)
return outputs
class SamVisionNeck(nn.Module):
def __init__(self, config: SamVisionConfig):
super().__init__()
self.config = config
self.conv1 = nn.Conv2d(config.hidden_size, config.output_channels, kernel_size=1, bias=False)
self.layer_norm1 = SamLayerNorm(config.output_channels, data_format="channels_first")
self.conv2 = nn.Conv2d(config.output_channels, config.output_channels, kernel_size=3, padding=1, bias=False)
self.layer_norm2 = SamLayerNorm(config.output_channels, data_format="channels_first")
def forward(self, hidden_states):
hidden_states = hidden_states.permute(0, 3, 1, 2)
hidden_states = self.conv1(hidden_states)
hidden_states = self.layer_norm1(hidden_states)
hidden_states = self.conv2(hidden_states)
hidden_states = self.layer_norm2(hidden_states)
return hidden_states
class SamVisionEncoder(nn.Module):
def __init__(self, config: SamVisionConfig):
super().__init__()
self.config = config
self.image_size = config.image_size
self.patch_embed = SamPatchEmbeddings(config)
self.pos_embed = None
if config.use_abs_pos:
# Initialize absolute positional embedding with pretrain image size.
self.pos_embed = nn.Parameter(
torch.zeros(
1,
config.image_size // config.patch_size,
config.image_size // config.patch_size,
config.hidden_size,
)
)
self.layers = nn.ModuleList()
for i in range(config.num_hidden_layers):
layer = SamVisionLayer(
config,
window_size=config.window_size if i not in config.global_attn_indexes else 0,
)
self.layers.append(layer)
self.neck = SamVisionNeck(config)
self.gradient_checkpointing = False
def get_input_embeddings(self):
return self.patch_embed
def forward(
self,
pixel_values: Optional[torch.FloatTensor] = None,
output_attentions: Optional[bool] = None,
output_hidden_states: Optional[bool] = None,
return_dict: Optional[bool] = None,
) -> Union[Tuple, SamVisionEncoderOutput]:
output_attentions = output_attentions if output_attentions is not None else self.config.output_attentions
output_hidden_states = (
output_hidden_states if output_hidden_states is not None else self.config.output_hidden_states
)
return_dict = return_dict if return_dict is not None else self.config.use_return_dict
if pixel_values is None:
raise ValueError("You have to specify pixel_values")
hidden_states = self.patch_embed(pixel_values)
if self.pos_embed is not None:
hidden_states = hidden_states + self.pos_embed
all_hidden_states = () if output_hidden_states else None
all_self_attentions = () if output_attentions else None
for i, layer_module in enumerate(self.layers):
if output_hidden_states:
all_hidden_states = all_hidden_states + (hidden_states,)
if self.gradient_checkpointing and self.training:
layer_outputs = self._gradient_checkpointing_func(
layer_module.__call__,
hidden_states,
)
else:
layer_outputs = layer_module(hidden_states, output_attentions=output_attentions)
hidden_states = layer_outputs[0]
if output_attentions:
all_self_attentions = all_self_attentions + (layer_outputs[1],)
if output_hidden_states:
all_hidden_states = all_hidden_states + (hidden_states,)
hidden_states = self.neck(hidden_states)
if not return_dict:
outputs = (hidden_states,)
if output_hidden_states:
outputs = outputs + (all_hidden_states,)
if output_attentions:
outputs = outputs + (all_self_attentions,)
return outputs
return SamVisionEncoderOutput(
last_hidden_state=hidden_states,
hidden_states=all_hidden_states,
attentions=all_self_attentions,
)
class SamPreTrainedModel(PreTrainedModel):
config_class = SamConfig
base_model_prefix = "sam"
main_input_name = "pixel_values"
def _init_weights(self, module):
std = self.config.initializer_range
if isinstance(module, (nn.Linear, nn.Conv2d, nn.ConvTranspose2d)):
module.weight.data.normal_(mean=0.0, std=std)
if module.bias is not None:
module.bias.data.zero_()
elif isinstance(module, nn.Embedding):
module.weight.data.normal_(mean=0.0, std=std)
if module.padding_idx is not None:
module.weight.data[module.padding_idx].zero_()
SAM_START_DOCSTRING = r"""
This model inherits from [`PreTrainedModel`]. Check the superclass documentation for the generic methods the
library implements for all its model (such as downloading or saving, resizing the input embeddings, pruning heads
etc.)
This model is also a PyTorch [torch.nn.Module](https://pytorch.org/docs/stable/nn.html#torch.nn.Module) subclass.
Use it as a regular PyTorch Module and refer to the PyTorch documentation for all matter related to general usage
and behavior.
Parameters:
config ([`SamConfig`]): Model configuration class with all the parameters of the model.
Initializing with a config file does not load the weights associated with the model, only the
configuration. Check out the [`~PreTrainedModel.from_pretrained`] method to load the model weights.
"""
SAM_INPUTS_DOCSTRING = r"""
Args:
pixel_values (`torch.FloatTensor` of shape `(batch_size, num_channels, height, width)`):
Pixel values. Pixel values can be obtained using [`SamProcessor`]. See [`SamProcessor.__call__`] for
details.
input_points (`torch.FloatTensor` of shape `(batch_size, num_points, 2)`):
Input 2D spatial points, this is used by the prompt encoder to encode the prompt. Generally yields to much
better results. The points can be obtained by passing a list of list of list to the processor that will
create corresponding `torch` tensors of dimension 4. The first dimension is the image batch size, the
second dimension is the point batch size (i.e. how many segmentation masks do we want the model to predict
per input point), the third dimension is the number of points per segmentation mask (it is possible to pass
multiple points for a single mask), and the last dimension is the x (vertical) and y (horizontal)
coordinates of the point. If a different number of points is passed either for each image, or for each
mask, the processor will create "PAD" points that will correspond to the (0, 0) coordinate, and the
computation of the embedding will be skipped for these points using the labels.
input_labels (`torch.LongTensor` of shape `(batch_size, point_batch_size, num_points)`):
Input labels for the points, this is used by the prompt encoder to encode the prompt. According to the
official implementation, there are 3 types of labels
- `1`: the point is a point that contains the object of interest
- `0`: the point is a point that does not contain the object of interest
- `-1`: the point corresponds to the background
We added the label:
- `-10`: the point is a padding point, thus should be ignored by the prompt encoder
The padding labels should be automatically done by the processor.
input_boxes (`torch.FloatTensor` of shape `(batch_size, num_boxes, 4)`):
Input boxes for the points, this is used by the prompt encoder to encode the prompt. Generally yields to
much better generated masks. The boxes can be obtained by passing a list of list of list to the processor,
that will generate a `torch` tensor, with each dimension corresponding respectively to the image batch
size, the number of boxes per image and the coordinates of the top left and botton right point of the box.
In the order (`x1`, `y1`, `x2`, `y2`):
- `x1`: the x coordinate of the top left point of the input box
- `y1`: the y coordinate of the top left point of the input box
- `x2`: the x coordinate of the bottom right point of the input box
- `y2`: the y coordinate of the bottom right point of the input box
input_masks (`torch.FloatTensor` of shape `(batch_size, image_size, image_size)`):
SAM model also accepts segmentation masks as input. The mask will be embedded by the prompt encoder to
generate a corresponding embedding, that will be fed later on to the mask decoder. These masks needs to be
manually fed by the user, and they need to be of shape (`batch_size`, `image_size`, `image_size`).
image_embeddings (`torch.FloatTensor` of shape `(batch_size, output_channels, window_size, window_size)`):
Image embeddings, this is used by the mask decder to generate masks and iou scores. For more memory
efficient computation, users can first retrieve the image embeddings using the `get_image_embeddings`
method, and then feed them to the `forward` method instead of feeding the `pixel_values`.
multimask_output (`bool`, *optional*):
In the original implementation and paper, the model always outputs 3 masks per image (or per point / per
bounding box if relevant). However, it is possible to just output a single mask, that corresponds to the
"best" mask, by specifying `multimask_output=False`.
attention_similarity (`torch.FloatTensor`, *optional*):
Attention similarity tensor, to be provided to the mask decoder for target-guided attention in case the
model is used for personalization as introduced in [PerSAM](https://arxiv.org/abs/2305.03048).
target_embedding (`torch.FloatTensor`, *optional*):
Embedding of the target concept, to be provided to the mask decoder for target-semantic prompting in case
the model is used for personalization as introduced in [PerSAM](https://arxiv.org/abs/2305.03048).
output_attentions (`bool`, *optional*):
Whether or not to return the attentions tensors of all attention layers. See `attentions` under returned
tensors for more detail.
output_hidden_states (`bool`, *optional*):
Whether or not to return the hidden states of all layers. See `hidden_states` under returned tensors for
more detail.
return_dict (`bool`, *optional*):
Whether or not to return a [`~utils.ModelOutput`] instead of a plain tuple.
"""
@add_start_docstrings(
"Segment Anything Model (SAM) for generating segmentation masks, given an input image and ",
" optional 2D location and bounding boxes.",
SAM_START_DOCSTRING,
)
class SamModel(SamPreTrainedModel):
_tied_weights_keys = ["prompt_encoder.shared_embedding.positional_embedding"]
def __init__(self, config):
super().__init__(config)
self.shared_image_embedding = SamPositionalEmbedding(config.vision_config)
self.vision_encoder = SamVisionEncoder(config.vision_config)
self.prompt_encoder = SamPromptEncoder(config.prompt_encoder_config, self.shared_image_embedding)
self.mask_decoder = SamMaskDecoder(config.mask_decoder_config)
self.post_init()
def get_input_embeddings(self):
return self.vision_encoder.get_input_embeddings()
def get_image_wide_positional_embeddings(self):
size = self.config.prompt_encoder_config.image_embedding_size
target_device = self.shared_image_embedding.positional_embedding.device
target_dtype = self.shared_image_embedding.positional_embedding.dtype
grid = torch.ones((size, size), device=target_device, dtype=target_dtype)
y_embed = grid.cumsum(dim=0) - 0.5
x_embed = grid.cumsum(dim=1) - 0.5
y_embed = y_embed / size
x_embed = x_embed / size
positional_embedding = self.shared_image_embedding(torch.stack([x_embed, y_embed], dim=-1))
return positional_embedding.permute(2, 0, 1).unsqueeze(0) # channel x height x width
@torch.no_grad()
def get_image_embeddings(
self,
pixel_values,
output_attentions: Optional[bool] = None,
output_hidden_states: Optional[bool] = None,
return_dict: Optional[bool] = None,
):
r"""
Returns the image embeddings by passing the pixel values through the vision encoder.
Args:
pixel_values (`torch.FloatTensor` of shape `(batch_size, num_channels, height, width)`):
Input pixel values
output_attentions (`bool`, *optional*):
Whether or not to return the attentions tensors of all attention layers.
output_hidden_states (`bool`, *optional*):
Whether or not to return the hidden states of all layers.
return_dict (`bool`, *optional*):
Whether or not to return a [`~utils.ModelOutput`] instead of a plain tuple.
"""
vision_output = self.vision_encoder(
pixel_values,
output_attentions=output_attentions,
output_hidden_states=output_hidden_states,
return_dict=return_dict,
)
image_embeddings = vision_output[0]
return image_embeddings
@torch.no_grad()
def get_prompt_embeddings(
self,
input_points: Optional[torch.FloatTensor] = None,
input_labels: Optional[torch.LongTensor] = None,
input_boxes: Optional[torch.FloatTensor] = None,
input_masks: Optional[torch.LongTensor] = None,
):
r"""
Returns the prompt embeddings by passing the input points, labels, boxes and masks through the prompt encoder.
Args:
input_points (`torch.FloatTensor` of shape `(batch_size, point_batch_size, num_points_per_image, 2)`):
Optional input points for the prompt encoder. The padding of the point is automatically done by the
processor. `point_batch_size` refers to the number of masks that we want the model to predict per
point. The model will output `point_batch_size` times 3 masks in total.
input_labels (`torch.LongTensor` of shape `(batch_size, point_batch_size, num_points_per_image)`):
Optional input labels for the prompt encoder. The padding of the labels is automatically done by the
processor, or can be fed by the user.
input_boxes (`torch.FloatTensor` of shape `(batch_size, num_boxes_per_image, 4)`):
Optional input boxes for the prompt encoder. The padding of the boxes is automatically done by the
processor. users can also pass manually the input boxes.
input_masks (`torch.LongTensor` of shape `(batch_size, image_size, image_size)`):
Optional input masks for the prompt encoder.
"""
prompt_output = self.prompt_encoder(
input_points=input_points,
input_labels=input_labels,
input_boxes=input_boxes,
input_masks=input_masks,
)
return prompt_output
@add_start_docstrings_to_model_forward(SAM_INPUTS_DOCSTRING)
def forward(
self,
pixel_values: Optional[torch.FloatTensor] = None,
input_points: Optional[torch.FloatTensor] = None,
input_labels: Optional[torch.LongTensor] = None,
input_boxes: Optional[torch.FloatTensor] = None,
input_masks: Optional[torch.LongTensor] = None,
image_embeddings: Optional[torch.FloatTensor] = None,
multimask_output: bool = True,
attention_similarity: Optional[torch.FloatTensor] = None,
target_embedding: Optional[torch.FloatTensor] = None,
output_attentions: Optional[bool] = None,
output_hidden_states: Optional[bool] = None,
return_dict: Optional[bool] = None,
**kwargs,
) -> List[Dict[str, torch.Tensor]]:
r"""
Example:
```python
>>> from PIL import Image
>>> import requests
>>> from transformers import AutoModel, AutoProcessor
>>> model = AutoModel.from_pretrained("facebook/sam-vit-base")
>>> processor = AutoProcessor.from_pretrained("facebook/sam-vit-base")
>>> img_url = "https://huggingface.co/datasets/huggingface/documentation-images/resolve/main/transformers/model_doc/sam-car.png"
>>> raw_image = Image.open(requests.get(img_url, stream=True).raw).convert("RGB")
>>> input_points = [[[400, 650]]] # 2D location of a window on the car
>>> inputs = processor(images=raw_image, input_points=input_points, return_tensors="pt")
>>> # Get segmentation mask
>>> outputs = model(**inputs)
>>> # Postprocess masks
>>> masks = processor.post_process_masks(
... outputs.pred_masks, inputs["original_sizes"], inputs["reshaped_input_sizes"]
... )
```
"""
output_attentions = output_attentions if output_attentions is not None else self.config.output_attentions
output_hidden_states = (
output_hidden_states if output_hidden_states is not None else self.config.output_hidden_states
)
return_dict = return_dict if return_dict is not None else self.config.use_return_dict
if pixel_values is None and image_embeddings is None:
raise ValueError("Either pixel_values or image_embeddings must be provided.")
if pixel_values is not None and image_embeddings is not None:
raise ValueError("Only one of pixel_values and image_embeddings can be provided.")
if input_points is not None and len(input_points.shape) != 4:
raise ValueError(
"The input_points must be a 4D tensor. Of shape `batch_size`, `point_batch_size`, `nb_points_per_image`, `2`.",
" got {}.".format(input_points.shape),
)
if input_boxes is not None and len(input_boxes.shape) != 3:
raise ValueError(
"The input_points must be a 3D tensor. Of shape `batch_size`, `nb_boxes`, `4`.",
" got {}.".format(input_boxes.shape),
)
if input_points is not None and input_boxes is not None:
point_batch_size = input_points.shape[1]
box_batch_size = input_boxes.shape[1]
if point_batch_size != box_batch_size:
raise ValueError(
"You should provide as many bounding boxes as input points per box. Got {} and {}.".format(
point_batch_size, box_batch_size
)
)
image_positional_embeddings = self.get_image_wide_positional_embeddings()
# repeat with batch size
batch_size = pixel_values.shape[0] if pixel_values is not None else image_embeddings.shape[0]
image_positional_embeddings = image_positional_embeddings.repeat(batch_size, 1, 1, 1)
vision_attentions = None
vision_hidden_states = None
if pixel_values is not None:
vision_outputs = self.vision_encoder(
pixel_values,
output_attentions=output_attentions,
output_hidden_states=output_hidden_states,
return_dict=return_dict,
)
image_embeddings = vision_outputs[0]
if output_hidden_states:
vision_hidden_states = vision_outputs[1]
if output_attentions:
vision_attentions = vision_outputs[-1]
if input_points is not None and input_labels is None:
input_labels = torch.ones_like(input_points[:, :, :, 0], dtype=torch.int, device=input_points.device)
if input_points is not None and image_embeddings.shape[0] != input_points.shape[0]:
raise ValueError(
"The batch size of the image embeddings and the input points must be the same. ",
"Got {} and {} respectively.".format(image_embeddings.shape[0], input_points.shape[0]),
" if you want to pass multiple points for the same image, make sure that you passed ",
" input_points of shape (batch_size, point_batch_size, num_points_per_image, 3) and ",
" input_labels of shape (batch_size, point_batch_size, num_points_per_image)",
)
sparse_embeddings, dense_embeddings = self.prompt_encoder(
input_points=input_points,
input_labels=input_labels,
input_boxes=input_boxes,
input_masks=input_masks,
)
low_res_masks, iou_predictions, mask_decoder_attentions = self.mask_decoder(
image_embeddings=image_embeddings,
image_positional_embeddings=image_positional_embeddings,
sparse_prompt_embeddings=sparse_embeddings,
dense_prompt_embeddings=dense_embeddings,
multimask_output=multimask_output,
attention_similarity=attention_similarity,
target_embedding=target_embedding,
output_attentions=output_attentions,
)
if not return_dict:
output = (iou_predictions, low_res_masks)
if output_hidden_states:
output = output + (vision_hidden_states,)
if output_attentions:
output = output + (vision_attentions, mask_decoder_attentions)
return output
return SamImageSegmentationOutput(
iou_scores=iou_predictions,
pred_masks=low_res_masks,
vision_hidden_states=vision_hidden_states,
vision_attentions=vision_attentions,
mask_decoder_attentions=mask_decoder_attentions,
)
| transformers/src/transformers/models/sam/modeling_sam.py/0 | {
"file_path": "transformers/src/transformers/models/sam/modeling_sam.py",
"repo_id": "transformers",
"token_count": 27304
} | 359 |
# coding=utf-8
# Copyright 2021 NVIDIA and The HuggingFace Inc. team. All rights reserved.
#
# Licensed under the Apache License, Version 2.0 (the "License");
# you may not use this file except in compliance with the License.
# You may obtain a copy of the License at
#
# http://www.apache.org/licenses/LICENSE-2.0
#
# Unless required by applicable law or agreed to in writing, software
# distributed under the License is distributed on an "AS IS" BASIS,
# WITHOUT WARRANTIES OR CONDITIONS OF ANY KIND, either express or implied.
# See the License for the specific language governing permissions and
# limitations under the License.
""" SegFormer model configuration"""
import warnings
from collections import OrderedDict
from typing import Mapping
from packaging import version
from ...configuration_utils import PretrainedConfig
from ...onnx import OnnxConfig
from ...utils import logging
logger = logging.get_logger(__name__)
SEGFORMER_PRETRAINED_CONFIG_ARCHIVE_MAP = {
"nvidia/segformer-b0-finetuned-ade-512-512": (
"https://huggingface.co/nvidia/segformer-b0-finetuned-ade-512-512/resolve/main/config.json"
),
# See all SegFormer models at https://huggingface.co/models?filter=segformer
}
class SegformerConfig(PretrainedConfig):
r"""
This is the configuration class to store the configuration of a [`SegformerModel`]. It is used to instantiate an
SegFormer model according to the specified arguments, defining the model architecture. Instantiating a
configuration with the defaults will yield a similar configuration to that of the SegFormer
[nvidia/segformer-b0-finetuned-ade-512-512](https://huggingface.co/nvidia/segformer-b0-finetuned-ade-512-512)
architecture.
Configuration objects inherit from [`PretrainedConfig`] and can be used to control the model outputs. Read the
documentation from [`PretrainedConfig`] for more information.
Args:
num_channels (`int`, *optional*, defaults to 3):
The number of input channels.
num_encoder_blocks (`int`, *optional*, defaults to 4):
The number of encoder blocks (i.e. stages in the Mix Transformer encoder).
depths (`List[int]`, *optional*, defaults to `[2, 2, 2, 2]`):
The number of layers in each encoder block.
sr_ratios (`List[int]`, *optional*, defaults to `[8, 4, 2, 1]`):
Sequence reduction ratios in each encoder block.
hidden_sizes (`List[int]`, *optional*, defaults to `[32, 64, 160, 256]`):
Dimension of each of the encoder blocks.
patch_sizes (`List[int]`, *optional*, defaults to `[7, 3, 3, 3]`):
Patch size before each encoder block.
strides (`List[int]`, *optional*, defaults to `[4, 2, 2, 2]`):
Stride before each encoder block.
num_attention_heads (`List[int]`, *optional*, defaults to `[1, 2, 5, 8]`):
Number of attention heads for each attention layer in each block of the Transformer encoder.
mlp_ratios (`List[int]`, *optional*, defaults to `[4, 4, 4, 4]`):
Ratio of the size of the hidden layer compared to the size of the input layer of the Mix FFNs in the
encoder blocks.
hidden_act (`str` or `function`, *optional*, defaults to `"gelu"`):
The non-linear activation function (function or string) in the encoder and pooler. If string, `"gelu"`,
`"relu"`, `"selu"` and `"gelu_new"` are supported.
hidden_dropout_prob (`float`, *optional*, defaults to 0.0):
The dropout probability for all fully connected layers in the embeddings, encoder, and pooler.
attention_probs_dropout_prob (`float`, *optional*, defaults to 0.0):
The dropout ratio for the attention probabilities.
classifier_dropout_prob (`float`, *optional*, defaults to 0.1):
The dropout probability before the classification head.
initializer_range (`float`, *optional*, defaults to 0.02):
The standard deviation of the truncated_normal_initializer for initializing all weight matrices.
drop_path_rate (`float`, *optional*, defaults to 0.1):
The dropout probability for stochastic depth, used in the blocks of the Transformer encoder.
layer_norm_eps (`float`, *optional*, defaults to 1e-06):
The epsilon used by the layer normalization layers.
decoder_hidden_size (`int`, *optional*, defaults to 256):
The dimension of the all-MLP decode head.
semantic_loss_ignore_index (`int`, *optional*, defaults to 255):
The index that is ignored by the loss function of the semantic segmentation model.
Example:
```python
>>> from transformers import SegformerModel, SegformerConfig
>>> # Initializing a SegFormer nvidia/segformer-b0-finetuned-ade-512-512 style configuration
>>> configuration = SegformerConfig()
>>> # Initializing a model from the nvidia/segformer-b0-finetuned-ade-512-512 style configuration
>>> model = SegformerModel(configuration)
>>> # Accessing the model configuration
>>> configuration = model.config
```"""
model_type = "segformer"
def __init__(
self,
num_channels=3,
num_encoder_blocks=4,
depths=[2, 2, 2, 2],
sr_ratios=[8, 4, 2, 1],
hidden_sizes=[32, 64, 160, 256],
patch_sizes=[7, 3, 3, 3],
strides=[4, 2, 2, 2],
num_attention_heads=[1, 2, 5, 8],
mlp_ratios=[4, 4, 4, 4],
hidden_act="gelu",
hidden_dropout_prob=0.0,
attention_probs_dropout_prob=0.0,
classifier_dropout_prob=0.1,
initializer_range=0.02,
drop_path_rate=0.1,
layer_norm_eps=1e-6,
decoder_hidden_size=256,
semantic_loss_ignore_index=255,
**kwargs,
):
super().__init__(**kwargs)
if "reshape_last_stage" in kwargs and kwargs["reshape_last_stage"] is False:
warnings.warn(
"Reshape_last_stage is set to False in this config. This argument is deprecated and will soon be"
" removed, as the behaviour will default to that of reshape_last_stage = True.",
FutureWarning,
)
self.num_channels = num_channels
self.num_encoder_blocks = num_encoder_blocks
self.depths = depths
self.sr_ratios = sr_ratios
self.hidden_sizes = hidden_sizes
self.patch_sizes = patch_sizes
self.strides = strides
self.mlp_ratios = mlp_ratios
self.num_attention_heads = num_attention_heads
self.hidden_act = hidden_act
self.hidden_dropout_prob = hidden_dropout_prob
self.attention_probs_dropout_prob = attention_probs_dropout_prob
self.classifier_dropout_prob = classifier_dropout_prob
self.initializer_range = initializer_range
self.drop_path_rate = drop_path_rate
self.layer_norm_eps = layer_norm_eps
self.decoder_hidden_size = decoder_hidden_size
self.reshape_last_stage = kwargs.get("reshape_last_stage", True)
self.semantic_loss_ignore_index = semantic_loss_ignore_index
class SegformerOnnxConfig(OnnxConfig):
torch_onnx_minimum_version = version.parse("1.11")
@property
def inputs(self) -> Mapping[str, Mapping[int, str]]:
return OrderedDict(
[
("pixel_values", {0: "batch", 1: "num_channels", 2: "height", 3: "width"}),
]
)
@property
def atol_for_validation(self) -> float:
return 1e-4
@property
def default_onnx_opset(self) -> int:
return 12
| transformers/src/transformers/models/segformer/configuration_segformer.py/0 | {
"file_path": "transformers/src/transformers/models/segformer/configuration_segformer.py",
"repo_id": "transformers",
"token_count": 3011
} | 360 |
# coding=utf-8
# Copyright 2024 The HuggingFace Inc. team.
#
# Licensed under the Apache License, Version 2.0 (the "License");
# you may not use this file except in compliance with the License.
# You may obtain a copy of the License at
#
# http://www.apache.org/licenses/LICENSE-2.0
#
# Unless required by applicable law or agreed to in writing, software
# distributed under the License is distributed on an "AS IS" BASIS,
# WITHOUT WARRANTIES OR CONDITIONS OF ANY KIND, either express or implied.
# See the License for the specific language governing permissions and
# limitations under the License.
"""Convert SigLIP checkpoints from the original repository.
URL: https://github.com/google-research/big_vision/tree/main
"""
import argparse
import collections
from pathlib import Path
import numpy as np
import requests
import torch
from huggingface_hub import hf_hub_download
from numpy import load
from PIL import Image
from transformers import SiglipConfig, SiglipImageProcessor, SiglipModel, SiglipProcessor, SiglipTokenizer
from transformers.utils import logging
logging.set_verbosity_info()
logger = logging.get_logger(__name__)
model_name_to_checkpoint = {
# base checkpoints
"siglip-base-patch16-224": "/Users/nielsrogge/Documents/SigLIP/webli_en_b16_224_63724782.npz",
"siglip-base-patch16-256": "/Users/nielsrogge/Documents/SigLIP/webli_en_b16_256_60500360.npz",
"siglip-base-patch16-384": "/Users/nielsrogge/Documents/SigLIP/webli_en_b16_384_68578854.npz",
"siglip-base-patch16-512": "/Users/nielsrogge/Documents/SigLIP/webli_en_b16_512_68580893.npz",
# large checkpoints
"siglip-large-patch16-256": "/Users/nielsrogge/Documents/SigLIP/webli_en_l16_256_60552751.npz",
"siglip-large-patch16-384": "/Users/nielsrogge/Documents/SigLIP/webli_en_l16_384_63634585.npz",
# multilingual checkpoint
"siglip-base-patch16-256-i18n": "/Users/nielsrogge/Documents/SigLIP/webli_i18n_b16_256_66117334.npz",
# so400m checkpoints
"siglip-so400m-patch14-384": "/Users/nielsrogge/Documents/SigLIP/webli_en_so400m_384_58765454.npz",
}
model_name_to_image_size = {
"siglip-base-patch16-224": 224,
"siglip-base-patch16-256": 256,
"siglip-base-patch16-384": 384,
"siglip-base-patch16-512": 512,
"siglip-large-patch16-256": 256,
"siglip-large-patch16-384": 384,
"siglip-base-patch16-256-i18n": 256,
"siglip-so400m-patch14-384": 384,
}
def get_siglip_config(model_name):
config = SiglipConfig()
vocab_size = 250000 if "i18n" in model_name else 32000
image_size = model_name_to_image_size[model_name]
patch_size = 16 if "patch16" in model_name else 14
# size of the architecture
config.vision_config.image_size = image_size
config.vision_config.patch_size = patch_size
config.text_config.vocab_size = vocab_size
if "base" in model_name:
pass
elif "large" in model_name:
config.text_config.hidden_size = 1024
config.text_config.intermediate_size = 4096
config.text_config.num_hidden_layers = 24
config.text_config.num_attention_heads = 16
config.vision_config.hidden_size = 1024
config.vision_config.intermediate_size = 4096
config.vision_config.num_hidden_layers = 24
config.vision_config.num_attention_heads = 16
elif "so400m" in model_name:
config.text_config.hidden_size = 1152
config.text_config.intermediate_size = 4304
config.text_config.num_hidden_layers = 27
config.text_config.num_attention_heads = 16
config.vision_config.hidden_size = 1152
config.vision_config.intermediate_size = 4304
config.vision_config.num_hidden_layers = 27
config.vision_config.num_attention_heads = 16
else:
raise ValueError("Model not supported")
return config
def create_rename_keys(config):
rename_keys = []
# fmt: off
# vision encoder
rename_keys.append(("params/img/embedding/kernel", "vision_model.embeddings.patch_embedding.weight"))
rename_keys.append(("params/img/embedding/bias", "vision_model.embeddings.patch_embedding.bias"))
rename_keys.append(("params/img/pos_embedding", "vision_model.embeddings.position_embedding.weight"))
for i in range(config.vision_config.num_hidden_layers):
rename_keys.append((f"params/img/Transformer/encoderblock_{i}/LayerNorm_0/scale", f"vision_model.encoder.layers.{i}.layer_norm1.weight"))
rename_keys.append((f"params/img/Transformer/encoderblock_{i}/LayerNorm_0/bias", f"vision_model.encoder.layers.{i}.layer_norm1.bias"))
rename_keys.append((f"params/img/Transformer/encoderblock_{i}/LayerNorm_1/scale", f"vision_model.encoder.layers.{i}.layer_norm2.weight"))
rename_keys.append((f"params/img/Transformer/encoderblock_{i}/LayerNorm_1/bias", f"vision_model.encoder.layers.{i}.layer_norm2.bias"))
rename_keys.append((f"params/img/Transformer/encoderblock_{i}/MlpBlock_0/Dense_0/kernel", f"vision_model.encoder.layers.{i}.mlp.fc1.weight"))
rename_keys.append((f"params/img/Transformer/encoderblock_{i}/MlpBlock_0/Dense_0/bias", f"vision_model.encoder.layers.{i}.mlp.fc1.bias"))
rename_keys.append((f"params/img/Transformer/encoderblock_{i}/MlpBlock_0/Dense_1/kernel", f"vision_model.encoder.layers.{i}.mlp.fc2.weight"))
rename_keys.append((f"params/img/Transformer/encoderblock_{i}/MlpBlock_0/Dense_1/bias", f"vision_model.encoder.layers.{i}.mlp.fc2.bias"))
rename_keys.append((f"params/img/Transformer/encoderblock_{i}/MultiHeadDotProductAttention_0/key/kernel", f"vision_model.encoder.layers.{i}.self_attn.k_proj.weight"))
rename_keys.append((f"params/img/Transformer/encoderblock_{i}/MultiHeadDotProductAttention_0/key/bias", f"vision_model.encoder.layers.{i}.self_attn.k_proj.bias"))
rename_keys.append((f"params/img/Transformer/encoderblock_{i}/MultiHeadDotProductAttention_0/value/kernel", f"vision_model.encoder.layers.{i}.self_attn.v_proj.weight"))
rename_keys.append((f"params/img/Transformer/encoderblock_{i}/MultiHeadDotProductAttention_0/value/bias", f"vision_model.encoder.layers.{i}.self_attn.v_proj.bias"))
rename_keys.append((f"params/img/Transformer/encoderblock_{i}/MultiHeadDotProductAttention_0/query/kernel", f"vision_model.encoder.layers.{i}.self_attn.q_proj.weight"))
rename_keys.append((f"params/img/Transformer/encoderblock_{i}/MultiHeadDotProductAttention_0/query/bias", f"vision_model.encoder.layers.{i}.self_attn.q_proj.bias"))
rename_keys.append((f"params/img/Transformer/encoderblock_{i}/MultiHeadDotProductAttention_0/out/kernel", f"vision_model.encoder.layers.{i}.self_attn.out_proj.weight"))
rename_keys.append((f"params/img/Transformer/encoderblock_{i}/MultiHeadDotProductAttention_0/out/bias", f"vision_model.encoder.layers.{i}.self_attn.out_proj.bias"))
rename_keys.append(("params/img/Transformer/encoder_norm/scale", "vision_model.post_layernorm.weight"))
rename_keys.append(("params/img/Transformer/encoder_norm/bias", "vision_model.post_layernorm.bias"))
rename_keys.append(("params/img/MAPHead_0/probe", "vision_model.head.probe"))
rename_keys.append(("params/img/MAPHead_0/LayerNorm_0/scale", "vision_model.head.layernorm.weight"))
rename_keys.append(("params/img/MAPHead_0/LayerNorm_0/bias", "vision_model.head.layernorm.bias"))
rename_keys.append(("params/img/MAPHead_0/MlpBlock_0/Dense_0/kernel", "vision_model.head.mlp.fc1.weight"))
rename_keys.append(("params/img/MAPHead_0/MlpBlock_0/Dense_0/bias", "vision_model.head.mlp.fc1.bias"))
rename_keys.append(("params/img/MAPHead_0/MlpBlock_0/Dense_1/kernel", "vision_model.head.mlp.fc2.weight"))
rename_keys.append(("params/img/MAPHead_0/MlpBlock_0/Dense_1/bias", "vision_model.head.mlp.fc2.bias"))
rename_keys.append(("params/img/MAPHead_0/MultiHeadDotProductAttention_0/out/kernel", "vision_model.head.attention.out_proj.weight"))
rename_keys.append(("params/img/MAPHead_0/MultiHeadDotProductAttention_0/out/bias", "vision_model.head.attention.out_proj.bias"))
# text encoder
rename_keys.append(("params/txt/Embed_0/embedding", "text_model.embeddings.token_embedding.weight"))
rename_keys.append(("params/txt/pos_embedding", "text_model.embeddings.position_embedding.weight"))
for i in range(config.text_config.num_hidden_layers):
rename_keys.append((f"params/txt/Encoder_0/encoderblock_{i}/LayerNorm_0/scale", f"text_model.encoder.layers.{i}.layer_norm1.weight"))
rename_keys.append((f"params/txt/Encoder_0/encoderblock_{i}/LayerNorm_0/bias", f"text_model.encoder.layers.{i}.layer_norm1.bias"))
rename_keys.append((f"params/txt/Encoder_0/encoderblock_{i}/LayerNorm_1/scale", f"text_model.encoder.layers.{i}.layer_norm2.weight"))
rename_keys.append((f"params/txt/Encoder_0/encoderblock_{i}/LayerNorm_1/bias", f"text_model.encoder.layers.{i}.layer_norm2.bias"))
rename_keys.append((f"params/txt/Encoder_0/encoderblock_{i}/MlpBlock_0/Dense_0/kernel", f"text_model.encoder.layers.{i}.mlp.fc1.weight"))
rename_keys.append((f"params/txt/Encoder_0/encoderblock_{i}/MlpBlock_0/Dense_0/bias", f"text_model.encoder.layers.{i}.mlp.fc1.bias"))
rename_keys.append((f"params/txt/Encoder_0/encoderblock_{i}/MlpBlock_0/Dense_1/kernel", f"text_model.encoder.layers.{i}.mlp.fc2.weight"))
rename_keys.append((f"params/txt/Encoder_0/encoderblock_{i}/MlpBlock_0/Dense_1/bias", f"text_model.encoder.layers.{i}.mlp.fc2.bias"))
rename_keys.append((f"params/txt/Encoder_0/encoderblock_{i}/MultiHeadDotProductAttention_0/key/kernel", f"text_model.encoder.layers.{i}.self_attn.k_proj.weight"))
rename_keys.append((f"params/txt/Encoder_0/encoderblock_{i}/MultiHeadDotProductAttention_0/key/bias", f"text_model.encoder.layers.{i}.self_attn.k_proj.bias"))
rename_keys.append((f"params/txt/Encoder_0/encoderblock_{i}/MultiHeadDotProductAttention_0/value/kernel", f"text_model.encoder.layers.{i}.self_attn.v_proj.weight"))
rename_keys.append((f"params/txt/Encoder_0/encoderblock_{i}/MultiHeadDotProductAttention_0/value/bias", f"text_model.encoder.layers.{i}.self_attn.v_proj.bias"))
rename_keys.append((f"params/txt/Encoder_0/encoderblock_{i}/MultiHeadDotProductAttention_0/query/kernel", f"text_model.encoder.layers.{i}.self_attn.q_proj.weight"))
rename_keys.append((f"params/txt/Encoder_0/encoderblock_{i}/MultiHeadDotProductAttention_0/query/bias", f"text_model.encoder.layers.{i}.self_attn.q_proj.bias"))
rename_keys.append((f"params/txt/Encoder_0/encoderblock_{i}/MultiHeadDotProductAttention_0/out/kernel", f"text_model.encoder.layers.{i}.self_attn.out_proj.weight"))
rename_keys.append((f"params/txt/Encoder_0/encoderblock_{i}/MultiHeadDotProductAttention_0/out/bias", f"text_model.encoder.layers.{i}.self_attn.out_proj.bias"))
rename_keys.append(("params/txt/Encoder_0/encoder_norm/scale", "text_model.final_layer_norm.weight"))
rename_keys.append(("params/txt/Encoder_0/encoder_norm/bias", "text_model.final_layer_norm.bias"))
rename_keys.append(("params/txt/head/kernel", "text_model.head.weight"))
rename_keys.append(("params/txt/head/bias", "text_model.head.bias"))
# learned temperature and bias
rename_keys.append(("params/t", "logit_scale"))
rename_keys.append(("params/b", "logit_bias"))
# fmt: on
return rename_keys
def rename_key(dct, old, new, config):
val = dct.pop(old)
if ("out_proj" in new or "v_proj" in new or "k_proj" in new or "q_proj" in new) and "vision" in new:
val = val.reshape(-1, config.vision_config.hidden_size)
if ("out_proj" in new or "v_proj" in new or "k_proj" in new or "q_proj" in new) and "text" in new:
val = val.reshape(-1, config.text_config.hidden_size)
if "patch_embedding.weight" in new:
val = val.transpose(3, 2, 0, 1)
elif new.endswith("weight") and "position_embedding" not in new and "token_embedding" not in new:
val = val.T
if "position_embedding" in new and "vision" in new:
val = val.reshape(-1, config.vision_config.hidden_size)
if "position_embedding" in new and "text" in new:
val = val.reshape(-1, config.text_config.hidden_size)
if new.endswith("bias"):
val = val.reshape(-1)
dct[new] = torch.from_numpy(val)
def read_in_q_k_v_head(state_dict, config):
# read in individual input projection layers
key_proj_weight = (
state_dict.pop("params/img/MAPHead_0/MultiHeadDotProductAttention_0/key/kernel")
.reshape(-1, config.vision_config.hidden_size)
.T
)
key_proj_bias = state_dict.pop("params/img/MAPHead_0/MultiHeadDotProductAttention_0/key/bias").reshape(-1)
value_proj_weight = (
state_dict.pop("params/img/MAPHead_0/MultiHeadDotProductAttention_0/value/kernel")
.reshape(-1, config.vision_config.hidden_size)
.T
)
value_proj_bias = state_dict.pop("params/img/MAPHead_0/MultiHeadDotProductAttention_0/value/bias").reshape(-1)
query_proj_weight = (
state_dict.pop("params/img/MAPHead_0/MultiHeadDotProductAttention_0/query/kernel")
.reshape(-1, config.vision_config.hidden_size)
.T
)
query_proj_bias = state_dict.pop("params/img/MAPHead_0/MultiHeadDotProductAttention_0/query/bias").reshape(-1)
# next, add them to the state dict as a single matrix + vector
state_dict["vision_model.head.attention.in_proj_weight"] = torch.from_numpy(
np.concatenate([query_proj_weight, key_proj_weight, value_proj_weight], axis=0)
)
state_dict["vision_model.head.attention.in_proj_bias"] = torch.from_numpy(
np.concatenate([query_proj_bias, key_proj_bias, value_proj_bias], axis=0)
)
# We will verify our results on an image of cute cats
def prepare_img():
url = "http://images.cocodataset.org/val2017/000000039769.jpg"
image = Image.open(requests.get(url, stream=True).raw)
return image
def flatten_nested_dict(params, parent_key="", sep="/"):
items = []
for k, v in params.items():
new_key = parent_key + sep + k if parent_key else k
if isinstance(v, collections.abc.MutableMapping):
items.extend(flatten_nested_dict(v, new_key, sep=sep).items())
else:
items.append((new_key, v))
return dict(items)
@torch.no_grad()
def convert_siglip_checkpoint(model_name, pytorch_dump_folder_path, verify_logits=True, push_to_hub=False):
"""
Copy/paste/tweak model's weights to our SigLIP structure.
"""
# define default SigLIP configuration
config = get_siglip_config(model_name)
# get checkpoint
checkpoint = model_name_to_checkpoint[model_name]
# get vocab file
if "i18n" in model_name:
vocab_file = "/Users/nielsrogge/Documents/SigLIP/multilingual_vocab/sentencepiece.model"
else:
vocab_file = "/Users/nielsrogge/Documents/SigLIP/english_vocab/sentencepiece.model"
# load original state dict
data = load(checkpoint)
state_dict = flatten_nested_dict(data)
# remove and rename some keys
rename_keys = create_rename_keys(config)
for src, dest in rename_keys:
rename_key(state_dict, src, dest, config)
# qkv matrices of attention pooling head need special treatment
read_in_q_k_v_head(state_dict, config)
# load HuggingFace model
model = SiglipModel(config).eval()
model.load_state_dict(state_dict)
# create processor
# important: make tokenizer not return attention_mask since original one doesn't require it
image_size = config.vision_config.image_size
size = {"height": image_size, "width": image_size}
image_processor = SiglipImageProcessor(size=size)
tokenizer = SiglipTokenizer(vocab_file=vocab_file, model_input_names=["input_ids"])
processor = SiglipProcessor(image_processor=image_processor, tokenizer=tokenizer)
# verify on dummy images and texts
url_1 = "https://cdn.openai.com/multimodal-neurons/assets/apple/apple-ipod.jpg"
image_1 = Image.open(requests.get(url_1, stream=True).raw).convert("RGB")
url_2 = "https://cdn.openai.com/multimodal-neurons/assets/apple/apple-blank.jpg"
image_2 = Image.open(requests.get(url_2, stream=True).raw).convert("RGB")
texts = ["an apple", "a picture of an apple"]
inputs = processor(images=[image_1, image_2], text=texts, return_tensors="pt", padding="max_length")
# verify input_ids against original ones
if image_size == 224:
filename = "siglip_pixel_values.pt"
elif image_size == 256:
filename = "siglip_pixel_values_256.pt"
elif image_size == 384:
filename = "siglip_pixel_values_384.pt"
elif image_size == 512:
filename = "siglip_pixel_values_512.pt"
else:
raise ValueError("Image size not supported")
filepath = hf_hub_download(repo_id="nielsr/test-image", filename=filename, repo_type="dataset")
original_pixel_values = torch.load(filepath)
filepath = hf_hub_download(repo_id="nielsr/test-image", filename="siglip_input_ids.pt", repo_type="dataset")
original_input_ids = torch.load(filepath)
if "i18n" not in model_name:
assert inputs.input_ids.tolist() == original_input_ids.tolist()
print("Mean of original pixel values:", original_pixel_values.mean())
print("Mean of new pixel values:", inputs.pixel_values.mean())
# note: we're testing with original pixel values here since we don't have exact pixel values
with torch.no_grad():
outputs = model(input_ids=inputs.input_ids, pixel_values=original_pixel_values)
# with torch.no_grad():
# outputs = model(input_ids=inputs.input_ids, pixel_values=inputs.pixel_values)
print(outputs.logits_per_image[:3, :3])
probs = torch.sigmoid(outputs.logits_per_image) # these are the probabilities
print(f"{probs[0][0]:.1%} that image 0 is '{texts[0]}'")
print(f"{probs[0][1]:.1%} that image 0 is '{texts[1]}'")
if verify_logits:
if model_name == "siglip-base-patch16-224":
expected_slice = torch.tensor(
[[-2.9621, -2.1672], [-0.2713, 0.2910]],
)
elif model_name == "siglip-base-patch16-256":
expected_slice = torch.tensor(
[[-3.1146, -1.9894], [-0.7312, 0.6387]],
)
elif model_name == "siglip-base-patch16-384":
expected_slice = torch.tensor(
[[-2.8098, -2.1891], [-0.4242, 0.4102]],
)
elif model_name == "siglip-base-patch16-512":
expected_slice = torch.tensor(
[[-2.7899, -2.2668], [-0.4295, -0.0735]],
)
elif model_name == "siglip-large-patch16-256":
expected_slice = torch.tensor(
[[-1.5827, -0.5801], [-0.9153, 0.1363]],
)
elif model_name == "siglip-large-patch16-384":
expected_slice = torch.tensor(
[[-2.1523, -0.2899], [-0.2959, 0.7884]],
)
elif model_name == "siglip-so400m-patch14-384":
expected_slice = torch.tensor([[-1.2441, -0.6649], [-0.7060, 0.7374]])
elif model_name == "siglip-base-patch16-256-i18n":
expected_slice = torch.tensor(
[[-0.9064, 0.1073], [-0.0299, 0.5304]],
)
assert torch.allclose(outputs.logits_per_image[:3, :3], expected_slice, atol=1e-4)
print("Looks ok!")
if pytorch_dump_folder_path is not None:
Path(pytorch_dump_folder_path).mkdir(exist_ok=True)
print(f"Saving model {model_name} to {pytorch_dump_folder_path}")
model.save_pretrained(pytorch_dump_folder_path)
print(f"Saving processor to {pytorch_dump_folder_path}")
processor.save_pretrained(pytorch_dump_folder_path)
if push_to_hub:
model.push_to_hub(f"nielsr/{model_name}")
processor.push_to_hub(f"nielsr/{model_name}")
if __name__ == "__main__":
parser = argparse.ArgumentParser()
# Required parameters
parser.add_argument(
"--model_name",
default="siglip-base-patch16-224",
type=str,
choices=model_name_to_checkpoint.keys(),
help="Name of the model you'd like to convert.",
)
parser.add_argument(
"--pytorch_dump_folder_path", default=None, type=str, help="Path to the output PyTorch model directory."
)
parser.add_argument(
"--verify_logits",
action="store_false",
help="Whether to verify logits against the original implementation.",
)
parser.add_argument(
"--push_to_hub", action="store_true", help="Whether or not to push the converted model to the ๐ค hub."
)
args = parser.parse_args()
convert_siglip_checkpoint(args.model_name, args.pytorch_dump_folder_path, args.verify_logits, args.push_to_hub)
| transformers/src/transformers/models/siglip/convert_siglip_to_hf.py/0 | {
"file_path": "transformers/src/transformers/models/siglip/convert_siglip_to_hf.py",
"repo_id": "transformers",
"token_count": 8771
} | 361 |
# coding=utf-8
# Copyright 2021 The Fairseq Authors and The HuggingFace Inc. team. All rights reserved.
#
# Licensed under the Apache License, Version 2.0 (the "License");
# you may not use this file except in compliance with the License.
# You may obtain a copy of the License at
#
# http://www.apache.org/licenses/LICENSE-2.0
#
# Unless required by applicable law or agreed to in writing, software
# distributed under the License is distributed on an "AS IS" BASIS,
# WITHOUT WARRANTIES OR CONDITIONS OF ANY KIND, either express or implied.
# See the License for the specific language governing permissions and
# limitations under the License.
""" TensorFlow Speech2Text model."""
from __future__ import annotations
import random
from typing import Optional, Tuple, Union
import numpy as np
import tensorflow as tf
from ...activations_tf import get_tf_activation, glu
from ...modeling_tf_outputs import (
TFBaseModelOutput,
TFBaseModelOutputWithPastAndCrossAttentions,
TFSeq2SeqLMOutput,
TFSeq2SeqModelOutput,
)
from ...modeling_tf_utils import (
TFCausalLanguageModelingLoss,
TFModelInputType,
TFPreTrainedModel,
TFSharedEmbeddings,
keras,
keras_serializable,
unpack_inputs,
)
from ...tf_utils import check_embeddings_within_bounds, shape_list, stable_softmax
from ...utils import (
add_code_sample_docstrings,
add_start_docstrings,
add_start_docstrings_to_model_forward,
logging,
replace_return_docstrings,
)
from .configuration_speech_to_text import Speech2TextConfig
logger = logging.get_logger(__name__)
_CONFIG_FOR_DOC = "Speech2TextConfig"
_CHECKPOINT_FOR_DOC = "facebook/s2t-small-librispeech-asr"
TF_SPEECH_TO_TEXT_PRETRAINED_MODEL_ARCHIVE_LIST = [
"facebook/s2t-small-librispeech-asr",
# See all Speech2Text models at https://huggingface.co/models?filter=speech_to_text
]
LARGE_NEGATIVE = -1e8
# Copied from transformers.models.bart.modeling_tf_bart.shift_tokens_right
def shift_tokens_right(input_ids: tf.Tensor, pad_token_id: int, decoder_start_token_id: int):
pad_token_id = tf.cast(pad_token_id, input_ids.dtype)
decoder_start_token_id = tf.cast(decoder_start_token_id, input_ids.dtype)
start_tokens = tf.fill(
(shape_list(input_ids)[0], 1), tf.convert_to_tensor(decoder_start_token_id, input_ids.dtype)
)
shifted_input_ids = tf.concat([start_tokens, input_ids[:, :-1]], -1)
# replace possible -100 values in labels by `pad_token_id`
shifted_input_ids = tf.where(
shifted_input_ids == -100,
tf.fill(shape_list(shifted_input_ids), tf.convert_to_tensor(pad_token_id, input_ids.dtype)),
shifted_input_ids,
)
# "Verify that `labels` has only positive values and -100"
assert_gte0 = tf.debugging.assert_greater_equal(shifted_input_ids, tf.constant(0, dtype=input_ids.dtype))
# Make sure the assertion op is called by wrapping the result in an identity no-op
with tf.control_dependencies([assert_gte0]):
shifted_input_ids = tf.identity(shifted_input_ids)
return shifted_input_ids
# Copied from transformers.models.bart.modeling_tf_bart._make_causal_mask
def _make_causal_mask(input_ids_shape: tf.TensorShape, past_key_values_length: int = 0):
"""
Make causal mask used for bi-directional self-attention.
"""
bsz = input_ids_shape[0]
tgt_len = input_ids_shape[1]
mask = tf.ones((tgt_len, tgt_len)) * LARGE_NEGATIVE
mask_cond = tf.range(shape_list(mask)[-1])
mask = tf.where(mask_cond < tf.reshape(mask_cond + 1, (shape_list(mask)[-1], 1)), 0.0, mask)
if past_key_values_length > 0:
mask = tf.concat([tf.zeros((tgt_len, past_key_values_length)), mask], axis=-1)
return tf.tile(mask[None, None, :, :], (bsz, 1, 1, 1))
# Copied from transformers.models.bart.modeling_tf_bart._expand_mask
def _expand_mask(mask: tf.Tensor, tgt_len: Optional[int] = None):
"""
Expands attention_mask from `[bsz, seq_len]` to `[bsz, 1, tgt_seq_len, src_seq_len]`.
"""
src_len = shape_list(mask)[1]
tgt_len = tgt_len if tgt_len is not None else src_len
one_cst = tf.constant(1.0)
mask = tf.cast(mask, dtype=one_cst.dtype)
expanded_mask = tf.tile(mask[:, None, None, :], (1, 1, tgt_len, 1))
return (one_cst - expanded_mask) * LARGE_NEGATIVE
class TFConv1dSubsampler(keras.layers.Layer):
"""
Convolutional subsampler: a stack of 1D convolution (along temporal dimension) followed by non-linear activation
via gated linear units (https://arxiv.org/abs/1911.08460)
"""
def __init__(self, config: Speech2TextConfig, **kwargs):
super().__init__(**kwargs)
self.config = config
self.num_layers = config.num_conv_layers
self.in_channels = config.input_feat_per_channel * config.input_channels
self.mid_channels = config.conv_channels
self.out_channels = config.d_model
self.kernel_sizes = config.conv_kernel_sizes
self.conv_layers = [
keras.layers.Conv1D(
filters=self.mid_channels if i < self.num_layers - 1 else self.out_channels * 2,
kernel_size=k,
strides=2,
name=f"conv_layers.{i}",
)
for i, k in enumerate(self.kernel_sizes)
]
def call(self, input_features: tf.Tensor) -> tf.Tensor:
# TF Conv1D assumes Batch x Time x Channels, same as the input
hidden_states = tf.cast(input_features, tf.float32)
for i, conv in enumerate(self.conv_layers):
# equivalent to `padding=k // 2` on PT's `nn.Conv1d`
pad_len = self.kernel_sizes[i] // 2
hidden_shapes = shape_list(hidden_states)
hidden_states = tf.concat(
(
tf.zeros((hidden_shapes[0], pad_len, hidden_shapes[2])),
hidden_states,
tf.zeros((hidden_shapes[0], pad_len, hidden_shapes[2])),
),
axis=1,
)
hidden_states = conv(hidden_states)
hidden_states = glu(hidden_states, axis=2) # GLU over the Channel dimension
return hidden_states
def build(self, input_shape=None):
if self.built:
return
self.built = True
if getattr(self, "conv_layers", None) is not None:
for i, layer in enumerate(self.conv_layers):
with tf.name_scope(layer.name):
layer.build([None, None, self.in_channels] if i == 0 else [None, None, self.mid_channels // 2])
class TFSpeech2TextSinusoidalPositionalEmbedding(keras.layers.Layer):
"""This module produces sinusoidal positional embeddings of any length."""
def __init__(self, num_positions: int, embedding_dim: int, padding_idx: Optional[int] = None, **kwargs):
super().__init__(**kwargs)
self.offset = 2
self.embedding_dim = embedding_dim
self.padding_idx = padding_idx
self.embedding_weights = self._get_embedding(num_positions + self.offset, embedding_dim, padding_idx)
@staticmethod
def _get_embedding(num_embeddings: int, embedding_dim: int, padding_idx: Optional[int] = None) -> tf.Tensor:
"""
Build sinusoidal embeddings. This matches the implementation in tensor2tensor, but differs slightly from the
description in Section 3.5 of "Attention Is All You Need".
"""
half_dim = embedding_dim // 2
emb = tf.math.log(10000.0) / (half_dim - 1)
emb = tf.math.exp(tf.range(half_dim, dtype=tf.float32) * -emb)
emb = tf.expand_dims(tf.range(num_embeddings, dtype=tf.float32), axis=1) * tf.expand_dims(emb, axis=0)
emb = tf.reshape(tf.concat([tf.math.sin(emb), tf.math.cos(emb)], axis=1), shape=[num_embeddings, -1])
if embedding_dim % 2 == 1:
# zero pad
emb = tf.concat([emb, tf.zeros(num_embeddings, 1)], axis=1)
if padding_idx is not None:
emb = tf.concat([emb[:padding_idx, :], tf.zeros((1, tf.shape(emb)[1])), emb[padding_idx + 1 :, :]], axis=0)
return emb
def call(self, input_ids: tf.Tensor, past_key_values_length: int = 0) -> tf.Tensor:
bsz, seq_len = shape_list(input_ids)
# Create the position ids from the input token ids. Any padded tokens remain padded.
position_ids = self.create_position_ids_from_input_ids(input_ids, self.padding_idx, past_key_values_length)
# Matt: The PyTorch code does a lot of work to cache the embeddings, setting the cached values as a
# model attribute in the forward pass. This is extremely forbidden in TF, which wants forward calls to be
# idempotent. TF doesn't need that caching anyway, since it can just store constants during compilation,
# so we just remove all of that code.
embeddings = self._get_embedding(
self.padding_idx + 1 + seq_len + self.offset + past_key_values_length, self.embedding_dim, self.padding_idx
)
return tf.reshape(tf.gather(embeddings, tf.reshape(position_ids, (-1,)), axis=0), (bsz, seq_len, -1))
@staticmethod
def create_position_ids_from_input_ids(
input_ids: tf.Tensor, padding_idx: int, past_key_values_length: Optional[int] = 0
) -> tf.Tensor:
"""
Replace non-padding symbols with their position numbers. Position numbers begin at padding_idx+1. Padding
symbols are ignored. This is modified from fairseq's `utils.make_positions`.
Args:
x: tf.Tensor x:
Returns: tf.Tensor
"""
mask = tf.cast(tf.math.not_equal(input_ids, padding_idx), dtype=tf.int32)
incremental_indices = (tf.math.cumsum(mask, axis=1) + past_key_values_length) * mask
return tf.cast(incremental_indices, dtype=tf.int64) + padding_idx
# Copied from transformers.models.bart.modeling_tf_bart.TFBartAttention with Bart->Speech2Text
class TFSpeech2TextAttention(keras.layers.Layer):
"""Multi-headed attention from "Attention Is All You Need"""
def __init__(
self,
embed_dim: int,
num_heads: int,
dropout: float = 0.0,
is_decoder: bool = False,
bias: bool = True,
**kwargs,
):
super().__init__(**kwargs)
self.embed_dim = embed_dim
self.num_heads = num_heads
self.dropout = keras.layers.Dropout(dropout)
self.head_dim = embed_dim // num_heads
if (self.head_dim * num_heads) != self.embed_dim:
raise ValueError(
f"embed_dim must be divisible by num_heads (got `embed_dim`: {self.embed_dim}"
f" and `num_heads`: {num_heads})."
)
self.scaling = self.head_dim**-0.5
self.is_decoder = is_decoder
self.k_proj = keras.layers.Dense(embed_dim, use_bias=bias, name="k_proj")
self.q_proj = keras.layers.Dense(embed_dim, use_bias=bias, name="q_proj")
self.v_proj = keras.layers.Dense(embed_dim, use_bias=bias, name="v_proj")
self.out_proj = keras.layers.Dense(embed_dim, use_bias=bias, name="out_proj")
def _shape(self, tensor: tf.Tensor, seq_len: int, bsz: int):
return tf.transpose(tf.reshape(tensor, (bsz, seq_len, self.num_heads, self.head_dim)), (0, 2, 1, 3))
def call(
self,
hidden_states: tf.Tensor,
key_value_states: tf.Tensor | None = None,
past_key_value: Tuple[Tuple[tf.Tensor]] | None = None,
attention_mask: tf.Tensor | None = None,
layer_head_mask: tf.Tensor | None = None,
training: Optional[bool] = False,
) -> Tuple[tf.Tensor, tf.Tensor | None]:
"""Input shape: Batch x Time x Channel"""
# if key_value_states are provided this layer is used as a cross-attention layer
# for the decoder
is_cross_attention = key_value_states is not None
bsz, tgt_len, embed_dim = shape_list(hidden_states)
# get query proj
query_states = self.q_proj(hidden_states) * self.scaling
# get key, value proj
if is_cross_attention and past_key_value is not None:
# reuse k,v, cross_attentions
key_states = past_key_value[0]
value_states = past_key_value[1]
elif is_cross_attention:
# cross_attentions
key_states = self._shape(self.k_proj(key_value_states), -1, bsz)
value_states = self._shape(self.v_proj(key_value_states), -1, bsz)
elif past_key_value is not None:
# reuse k, v, self_attention
key_states = self._shape(self.k_proj(hidden_states), -1, bsz)
value_states = self._shape(self.v_proj(hidden_states), -1, bsz)
key_states = tf.concat([past_key_value[0], key_states], axis=2)
value_states = tf.concat([past_key_value[1], value_states], axis=2)
else:
# self_attention
key_states = self._shape(self.k_proj(hidden_states), -1, bsz)
value_states = self._shape(self.v_proj(hidden_states), -1, bsz)
if self.is_decoder:
# if cross_attention save Tuple(tf.Tensor, tf.Tensor) of all cross attention key/value_states.
# Further calls to cross_attention layer can then reuse all cross-attention
# key/value_states (first "if" case)
# if uni-directional self-attention (decoder) save Tuple(tf.Tensor, tf.Tensor) of
# all previous decoder key/value_states. Further calls to uni-directional self-attention
# can concat previous decoder key/value_states to current projected key/value_states (third "elif" case)
# if encoder bi-directional self-attention `past_key_value` is always `None`
past_key_value = (key_states, value_states)
proj_shape = (bsz * self.num_heads, -1, self.head_dim)
query_states = tf.reshape(self._shape(query_states, tgt_len, bsz), proj_shape)
key_states = tf.reshape(key_states, proj_shape)
value_states = tf.reshape(value_states, proj_shape)
src_len = shape_list(key_states)[1]
attn_weights = tf.matmul(query_states, key_states, transpose_b=True)
tf.debugging.assert_equal(
shape_list(attn_weights),
[bsz * self.num_heads, tgt_len, src_len],
message=(
f"Attention weights should be of size {(bsz * self.num_heads, tgt_len, src_len)}, but is"
f" {shape_list(attn_weights)}"
),
)
if attention_mask is not None:
tf.debugging.assert_equal(
shape_list(attention_mask),
[bsz, 1, tgt_len, src_len],
message=(
f"Attention mask should be of size {(bsz, 1, tgt_len, src_len)}, but is"
f" {shape_list(attention_mask)}"
),
)
attention_mask = tf.cast(attention_mask, dtype=attn_weights.dtype)
attn_weights = tf.reshape(attn_weights, (bsz, self.num_heads, tgt_len, src_len)) + attention_mask
attn_weights = tf.reshape(attn_weights, (bsz * self.num_heads, tgt_len, src_len))
attn_weights = stable_softmax(attn_weights, axis=-1)
if layer_head_mask is not None:
tf.debugging.assert_equal(
shape_list(layer_head_mask),
[self.num_heads],
message=(
f"Head mask for a single layer should be of size {(self.num_heads)}, but is"
f" {shape_list(layer_head_mask)}"
),
)
attn_weights = tf.reshape(layer_head_mask, (1, -1, 1, 1)) * tf.reshape(
attn_weights, (bsz, self.num_heads, tgt_len, src_len)
)
attn_weights = tf.reshape(attn_weights, (bsz * self.num_heads, tgt_len, src_len))
attn_probs = self.dropout(attn_weights, training=training)
attn_output = tf.matmul(attn_probs, value_states)
tf.debugging.assert_equal(
shape_list(attn_output),
[bsz * self.num_heads, tgt_len, self.head_dim],
message=(
f"`attn_output` should be of size {(bsz, self.num_heads, tgt_len, self.head_dim)}, but is"
f" {shape_list(attn_output)}"
),
)
attn_output = tf.transpose(
tf.reshape(attn_output, (bsz, self.num_heads, tgt_len, self.head_dim)), (0, 2, 1, 3)
)
attn_output = tf.reshape(attn_output, (bsz, tgt_len, embed_dim))
attn_output = self.out_proj(attn_output)
attn_weights: tf.Tensor = tf.reshape(attn_weights, (bsz, self.num_heads, tgt_len, src_len))
return attn_output, attn_weights, past_key_value
def build(self, input_shape=None):
if self.built:
return
self.built = True
if getattr(self, "k_proj", None) is not None:
with tf.name_scope(self.k_proj.name):
self.k_proj.build([None, None, self.embed_dim])
if getattr(self, "q_proj", None) is not None:
with tf.name_scope(self.q_proj.name):
self.q_proj.build([None, None, self.embed_dim])
if getattr(self, "v_proj", None) is not None:
with tf.name_scope(self.v_proj.name):
self.v_proj.build([None, None, self.embed_dim])
if getattr(self, "out_proj", None) is not None:
with tf.name_scope(self.out_proj.name):
self.out_proj.build([None, None, self.embed_dim])
class TFSpeech2TextEncoderLayer(keras.layers.Layer):
def __init__(self, config: Speech2TextConfig, **kwargs):
super().__init__(**kwargs)
self.embed_dim = config.d_model
self.self_attn = TFSpeech2TextAttention(
self.embed_dim, config.encoder_attention_heads, dropout=config.attention_dropout, name="self_attn"
)
self.self_attn_layer_norm = keras.layers.LayerNormalization(epsilon=1e-5, name="self_attn_layer_norm")
self.dropout = keras.layers.Dropout(config.dropout)
self.activation_fn = get_tf_activation(config.activation_function)
self.activation_dropout = keras.layers.Dropout(config.activation_dropout)
self.fc1 = keras.layers.Dense(config.encoder_ffn_dim, name="fc1")
self.fc2 = keras.layers.Dense(self.embed_dim, name="fc2")
self.final_layer_norm = keras.layers.LayerNormalization(epsilon=1e-5, name="final_layer_norm")
self.config = config
def call(
self, hidden_states: tf.Tensor, attention_mask: tf.Tensor, layer_head_mask: tf.Tensor, training: bool = False
):
"""
Args:
hidden_states (`tf.Tensor`): input to the layer of shape `(batch, seq_len, embed_dim)`
attention_mask (`tf.Tensor`): attention mask of size
`(batch, 1, tgt_len, src_len)` where padding elements are indicated by very large negative values.
layer_head_mask (`tf.Tensor`): mask for attention heads in a given layer of size
`(encoder_attention_heads,)`
"""
residual = hidden_states
hidden_states = self.self_attn_layer_norm(hidden_states)
hidden_states, self_attn_weights, _ = self.self_attn(
hidden_states=hidden_states,
attention_mask=attention_mask,
layer_head_mask=layer_head_mask,
training=training,
)
tf.debugging.assert_equal(
shape_list(hidden_states),
shape_list(residual),
message=f"Self attn modified the shape of query {shape_list(residual)} to {shape_list(hidden_states)}",
)
hidden_states = self.dropout(hidden_states, training=training)
hidden_states = residual + hidden_states
residual = hidden_states
hidden_states = self.final_layer_norm(hidden_states)
hidden_states = self.activation_fn(self.fc1(hidden_states))
hidden_states = self.activation_dropout(hidden_states, training=training)
hidden_states = self.fc2(hidden_states)
hidden_states = self.dropout(hidden_states, training=training)
hidden_states = residual + hidden_states
return hidden_states, self_attn_weights
def build(self, input_shape=None):
if self.built:
return
self.built = True
if getattr(self, "self_attn", None) is not None:
with tf.name_scope(self.self_attn.name):
self.self_attn.build(None)
if getattr(self, "self_attn_layer_norm", None) is not None:
with tf.name_scope(self.self_attn_layer_norm.name):
self.self_attn_layer_norm.build([None, None, self.embed_dim])
if getattr(self, "fc1", None) is not None:
with tf.name_scope(self.fc1.name):
self.fc1.build([None, None, self.embed_dim])
if getattr(self, "fc2", None) is not None:
with tf.name_scope(self.fc2.name):
self.fc2.build([None, None, self.config.encoder_ffn_dim])
if getattr(self, "final_layer_norm", None) is not None:
with tf.name_scope(self.final_layer_norm.name):
self.final_layer_norm.build([None, None, self.embed_dim])
class TFSpeech2TextDecoderLayer(keras.layers.Layer):
def __init__(self, config: Speech2TextConfig, **kwargs):
super().__init__(**kwargs)
self.embed_dim = config.d_model
self.self_attn = TFSpeech2TextAttention(
embed_dim=self.embed_dim,
num_heads=config.decoder_attention_heads,
dropout=config.attention_dropout,
name="self_attn",
is_decoder=True,
)
self.dropout = keras.layers.Dropout(config.dropout)
self.activation_fn = get_tf_activation(config.activation_function)
self.activation_dropout = keras.layers.Dropout(config.activation_dropout)
self.self_attn_layer_norm = keras.layers.LayerNormalization(epsilon=1e-5, name="self_attn_layer_norm")
self.encoder_attn = TFSpeech2TextAttention(
self.embed_dim,
config.decoder_attention_heads,
dropout=config.attention_dropout,
name="encoder_attn",
is_decoder=True,
)
self.encoder_attn_layer_norm = keras.layers.LayerNormalization(epsilon=1e-5, name="encoder_attn_layer_norm")
self.fc1 = keras.layers.Dense(config.decoder_ffn_dim, name="fc1")
self.fc2 = keras.layers.Dense(self.embed_dim, name="fc2")
self.final_layer_norm = keras.layers.LayerNormalization(epsilon=1e-5, name="final_layer_norm")
self.config = config
def call(
self,
hidden_states,
attention_mask: tf.Tensor | None = None,
encoder_hidden_states: tf.Tensor | None = None,
encoder_attention_mask: tf.Tensor | None = None,
layer_head_mask: tf.Tensor | None = None,
cross_attn_layer_head_mask: tf.Tensor | None = None,
past_key_value: Tuple[tf.Tensor] | None = None,
training=False,
) -> Tuple[tf.Tensor, tf.Tensor, Tuple[Tuple[tf.Tensor]]]:
"""
Args:
hidden_states (`tf.Tensor`): input to the layer of shape `(batch, seq_len, embed_dim)`
attention_mask (`tf.Tensor`): attention mask of size
`(batch, 1, tgt_len, src_len)` where padding elements are indicated by very large negative values.
encoder_hidden_states (`tf.Tensor`):
cross attention input to the layer of shape `(batch, seq_len, embed_dim)`
encoder_attention_mask (`tf.Tensor`): encoder attention mask of size
`(batch, 1, tgt_len, src_len)` where padding elements are indicated by very large negative values.
layer_head_mask (`tf.Tensor`): mask for attention heads in a given layer of size
`(decoder_attention_heads,)`
cross_attn_layer_head_mask (`tf.Tensor`): mask for heads of the cross-attention module.
`(decoder_attention_heads,)`
past_key_value (`Tuple(tf.Tensor)`): cached past key and value projection states
"""
residual = hidden_states
hidden_states = self.self_attn_layer_norm(hidden_states)
# Self Attention
# decoder uni-directional self-attention cached key/values tuple is at positions 1,2
self_attn_past_key_value = past_key_value[:2] if past_key_value is not None else None
# add present self-attn cache to positions 1,2 of present_key_value tuple
hidden_states, self_attn_weights, present_key_value = self.self_attn(
hidden_states=hidden_states,
past_key_value=self_attn_past_key_value,
attention_mask=attention_mask,
layer_head_mask=layer_head_mask,
training=training,
)
hidden_states = self.dropout(hidden_states, training=training)
hidden_states = residual + hidden_states
# Cross-Attention Block
cross_attn_present_key_value = None
cross_attn_weights = None
if encoder_hidden_states is not None:
residual = hidden_states
hidden_states = self.encoder_attn_layer_norm(hidden_states)
# cross_attn cached key/values tuple is at positions 3,4 of present_key_value tuple
cross_attn_past_key_value = past_key_value[-2:] if past_key_value is not None else None
hidden_states, cross_attn_weights, cross_attn_present_key_value = self.encoder_attn(
hidden_states=hidden_states,
key_value_states=encoder_hidden_states,
attention_mask=encoder_attention_mask,
layer_head_mask=cross_attn_layer_head_mask,
past_key_value=cross_attn_past_key_value,
training=training,
)
hidden_states = self.dropout(hidden_states, training=training)
hidden_states = residual + hidden_states
# add cross-attn to positions 3,4 of present_key_value tuple
present_key_value = present_key_value + cross_attn_present_key_value
# Fully Connected
residual = hidden_states
hidden_states = self.final_layer_norm(hidden_states)
hidden_states = self.activation_fn(self.fc1(hidden_states))
hidden_states = self.activation_dropout(hidden_states, training=training)
hidden_states = self.fc2(hidden_states)
hidden_states = self.dropout(hidden_states, training=training)
hidden_states = residual + hidden_states
return (
hidden_states,
self_attn_weights,
cross_attn_weights,
present_key_value,
)
def build(self, input_shape=None):
if self.built:
return
self.built = True
if getattr(self, "self_attn", None) is not None:
with tf.name_scope(self.self_attn.name):
self.self_attn.build(None)
if getattr(self, "self_attn_layer_norm", None) is not None:
with tf.name_scope(self.self_attn_layer_norm.name):
self.self_attn_layer_norm.build([None, None, self.embed_dim])
if getattr(self, "encoder_attn", None) is not None:
with tf.name_scope(self.encoder_attn.name):
self.encoder_attn.build(None)
if getattr(self, "encoder_attn_layer_norm", None) is not None:
with tf.name_scope(self.encoder_attn_layer_norm.name):
self.encoder_attn_layer_norm.build([None, None, self.embed_dim])
if getattr(self, "fc1", None) is not None:
with tf.name_scope(self.fc1.name):
self.fc1.build([None, None, self.embed_dim])
if getattr(self, "fc2", None) is not None:
with tf.name_scope(self.fc2.name):
self.fc2.build([None, None, self.config.decoder_ffn_dim])
if getattr(self, "final_layer_norm", None) is not None:
with tf.name_scope(self.final_layer_norm.name):
self.final_layer_norm.build([None, None, self.embed_dim])
class TFSpeech2TextPreTrainedModel(TFPreTrainedModel):
config_class = Speech2TextConfig
base_model_prefix = "model"
main_input_name = "input_features"
_keys_to_ignore_on_load_unexpected = [r"encoder.embed_positions.weights"]
def _get_feat_extract_output_lengths(self, input_lengths: tf.Tensor):
"""
Computes the output length of the convolutional layers
"""
for _ in range(self.config.num_conv_layers):
input_lengths = (input_lengths - 1) // 2 + 1
return input_lengths
@property
def input_signature(self):
return {
"input_features": tf.TensorSpec(
(None, None, self.config.input_feat_per_channel * self.config.input_channels),
tf.float32,
name="input_features",
),
"attention_mask": tf.TensorSpec((None, None), tf.int32, name="attention_mask"),
"decoder_input_ids": tf.TensorSpec((None, None), tf.int32, name="decoder_input_ids"),
"decoder_attention_mask": tf.TensorSpec((None, None), tf.int32, name="decoder_attention_mask"),
}
SPEECH_TO_TEXT_START_DOCSTRING = r"""
This model inherits from [`TFPreTrainedModel`]. Check the superclass documentation for the generic methods the
library implements for all its model (such as downloading or saving, resizing the input embeddings, pruning heads
etc.)
This model is also a [keras.Model](https://www.tensorflow.org/api_docs/python/tf/keras/Model) subclass. Use it
as a regular TF 2.0 Keras Model and refer to the TF 2.0 documentation for all matter related to general usage and
behavior.
<Tip>
TensorFlow models and layers in `transformers` accept two formats as input:
- having all inputs as keyword arguments (like PyTorch models), or
- having all inputs as a list, tuple or dict in the first positional argument.
The reason the second format is supported is that Keras methods prefer this format when passing inputs to models
and layers. Because of this support, when using methods like `model.fit()` things should "just work" for you - just
pass your inputs and labels in any format that `model.fit()` supports! If, however, you want to use the second
format outside of Keras methods like `fit()` and `predict()`, such as when creating your own layers or models with
the Keras `Functional` API, there are three possibilities you can use to gather all the input Tensors in the first
positional argument:
- a single Tensor with `input_ids` only and nothing else: `model(input_ids)`
- a list of varying length with one or several input Tensors IN THE ORDER given in the docstring:
`model([input_ids, attention_mask])` or `model([input_ids, attention_mask, token_type_ids])`
- a dictionary with one or several input Tensors associated to the input names given in the docstring:
`model({"input_ids": input_ids, "token_type_ids": token_type_ids})`
Note that when creating models and layers with
[subclassing](https://keras.io/guides/making_new_layers_and_models_via_subclassing/) then you don't need to worry
about any of this, as you can just pass inputs like you would to any other Python function!
</Tip>
Parameters:
config ([`Speech2TextConfig`]):
Model configuration class with all the parameters of the model. Initializing with a config file does not
load the weights associated with the model, only the configuration. Check out the
[`~TFPreTrainedModel.from_pretrained`] method to load the model weights.
"""
SPEECH_TO_TEXT_INPUTS_DOCSTRING = r"""
Args:
input_features (`tf.Tensor` of shape `(batch_size, sequence_length, feature_size)`):
Float values of fbank features extracted from the raw speech waveform. Raw speech waveform can be obtained
by loading a `.flac` or `.wav` audio file into an array of type `List[float]` or a `numpy.ndarray`, *e.g.*
via the soundfile library (`pip install soundfile`). To prepare the array into `input_features`, the
[`AutoFeatureExtractor`] should be used for extracting the fbank features, padding and conversion into a
tensor of floats. See [`~Speech2TextFeatureExtractor.__call__`]
attention_mask (`tf.Tensor` of shape `({0})`, *optional*):
Mask to avoid performing attention on padding token indices. Mask values selected in `[0, 1]`:
- 1 for tokens that are **not masked**,
- 0 for tokens that are **masked**.
[What are attention masks?](../glossary#attention-mask)
decoder_input_ids (`tf.Tensor` of shape `(batch_size, target_sequence_length)`, *optional*):
Indices of decoder input sequence tokens in the vocabulary.
Indices can be obtained using [`Speech2TextTokenizer`]. See [`PreTrainedTokenizer.encode`] and
[`PreTrainedTokenizer.__call__`] for details.
[What are decoder input IDs?](../glossary#decoder-input-ids)
SpeechToText uses the `eos_token_id` as the starting token for `decoder_input_ids` generation. If
`past_key_values` is used, optionally only the last `decoder_input_ids` have to be input (see
`past_key_values`).
For translation and summarization training, `decoder_input_ids` should be provided. If no
`decoder_input_ids` is provided, the model will create this tensor by shifting the `input_ids` to the right
for denoising pre-training following the paper.
decoder_attention_mask (`tf.Tensor` of shape `(batch_size, target_sequence_length)`, *optional*):
will be made by default and ignore pad tokens. It is not recommended to set this for most use cases.
head_mask (`tf.Tensor` of shape `(encoder_layers, encoder_attention_heads)`, *optional*):
Mask to nullify selected heads of the attention modules in the encoder. Mask values selected in `[0, 1]`:
- 1 indicates the head is **not masked**,
- 0 indicates the head is **masked**.
decoder_head_mask (`tf.Tensor` of shape `(decoder_layers, decoder_attention_heads)`, *optional*):
Mask to nullify selected heads of the attention modules in the decoder. Mask values selected in `[0, 1]`:
- 1 indicates the head is **not masked**,
- 0 indicates the head is **masked**.
cross_attn_head_mask (`tf.Tensor` of shape `(decoder_layers, decoder_attention_heads)`, *optional*):
Mask to nullify selected heads of the cross-attention modules. Mask values selected in `[0, 1]`:
- 1 indicates the head is **not masked**,
- 0 indicates the head is **masked**.
encoder_outputs (`tf.FloatTensor`, *optional*):
hidden states at the output of the last layer of the encoder. Used in the cross-attention of the decoder.
of shape `(batch_size, sequence_length, hidden_size)` is a sequence of
past_key_values (`Tuple[Tuple[tf.Tensor]]` of length `config.n_layers`)
contains precomputed key and value hidden states of the attention blocks. Can be used to speed up decoding.
If `past_key_values` are used, the user can optionally input only the last `decoder_input_ids` (those that
don't have their past key value states given to this model) of shape `(batch_size, 1)` instead of all
`decoder_input_ids` of shape `(batch_size, sequence_length)`.
decoder_inputs_embeds (`tf.FloatTensor` of shape `(batch_size, target_sequence_length, hidden_size)`, *optional*):
Optionally, instead of passing `decoder_input_ids` you can choose to directly pass an embedded
representation. If `past_key_values` is used, optionally only the last `decoder_inputs_embeds` have to be
input (see `past_key_values`). This is useful if you want more control over how to convert
`decoder_input_ids` indices into associated vectors than the model's internal embedding lookup matrix.
use_cache (`bool`, *optional*):
If set to `True`, `past_key_values` key value states are returned and can be used to speed up decoding (see
`past_key_values`).
output_attentions (`bool`, *optional*):
Whether or not to return the attentions tensors of all attention layers. See `attentions` under returned
tensors for more detail. This argument can be used only in eager mode, in graph mode the value in the
config will be used instead.
output_hidden_states (`bool`, *optional*):
Whether or not to return the hidden states of all layers. See `hidden_states` under returned tensors for
more detail. This argument can be used only in eager mode, in graph mode the value in the config will be
used instead.
return_dict (`bool`, *optional*):
Whether or not to return a [`~utils.ModelOutput`] instead of a plain tuple. This argument can be used in
eager mode, in graph mode the value will always be set to True.
training (`bool`, *optional*, defaults to `False`):
Whether or not to use the model in training mode (some modules like dropout modules have different
behaviors between training and evaluation).
"""
@keras_serializable
class TFSpeech2TextEncoder(keras.layers.Layer):
config_class = Speech2TextConfig
"""
Transformer encoder consisting of *config.encoder_layers* self attention layers. Each layer is a
[`TFSpeech2TextEncoderLayer`].
Args:
config: Speech2TextConfig
"""
def __init__(self, config: Speech2TextConfig, **kwargs):
super().__init__(**kwargs)
self.config = config
self.dropout = keras.layers.Dropout(config.dropout)
self.layerdrop = config.encoder_layerdrop
embed_dim = config.d_model
self.padding_idx = config.pad_token_id
self.max_source_positions = config.max_source_positions
self.embed_scale = tf.math.sqrt(float(embed_dim)) if config.scale_embedding else 1.0
self.conv = TFConv1dSubsampler(config, name="conv")
self.embed_positions = TFSpeech2TextSinusoidalPositionalEmbedding(
num_positions=config.max_source_positions,
embedding_dim=embed_dim,
padding_idx=self.padding_idx,
name="embed_positions",
)
self.layers = [TFSpeech2TextEncoderLayer(config, name=f"layers.{i}") for i in range(config.encoder_layers)]
self.layer_norm = keras.layers.LayerNormalization(epsilon=1e-5, name="layer_norm")
def _get_feat_extract_output_lengths(self, input_lengths: tf.Tensor):
"""
Computes the output length of the convolutional layers
"""
for _ in range(self.config.num_conv_layers):
input_lengths = (input_lengths - 1) // 2 + 1
return input_lengths
def _get_feature_vector_attention_mask(self, feature_vector_length, attention_mask):
# generate creates 3D attention mask, because of the shape of input_features
# convert it to 2D if thats the case
if len(attention_mask.shape) > 2:
attention_mask = attention_mask[:, :, -1]
subsampled_lengths = self._get_feat_extract_output_lengths(tf.math.reduce_sum(attention_mask, -1))
bsz = shape_list(attention_mask)[0]
indices = tf.concat(
(
tf.expand_dims(tf.range(bsz, dtype=attention_mask.dtype), -1),
tf.expand_dims(subsampled_lengths - 1, -1),
),
axis=-1,
)
attention_mask = tf.scatter_nd(indices=indices, updates=tf.ones(bsz), shape=[bsz, feature_vector_length])
attention_mask = tf.cast(tf.reverse(tf.math.cumsum(tf.reverse(attention_mask, [-1]), -1), [-1]), tf.int64)
return attention_mask
@unpack_inputs
def call(
self,
input_features=None,
attention_mask=None,
head_mask=None,
output_attentions=None,
output_hidden_states=None,
return_dict=None,
training=False,
):
"""
Args:
input_features (`tf.Tensor` of shape `(batch_size, sequence_length, feature_size)`):
Float values of fbank features extracted from the raw speech waveform. Raw speech waveform can be
obtained by loading a `.flac` or `.wav` audio file into an array of type `List[float]` or a
`numpy.ndarray`, *e.g.* via the soundfile library (`pip install soundfile`). To prepare the array into
`input_features`, the [`AutoFeatureExtractor`] should be used for extracting the fbank features,
padding and conversion into a tensor of floats. See [`~Speech2TextFeatureExtractor.__call__`]
attention_mask (`tf.Tensor` of shape `(batch_size, sequence_length)`, *optional*):
Mask to avoid performing attention on padding token indices. Mask values selected in `[0, 1]`:
- 1 for tokens that are **not masked**,
- 0 for tokens that are **masked**.
[What are attention masks?](../glossary#attention-mask)
head_mask (`tf.Tensor` of shape `(encoder_layers, encoder_attention_heads)`, `optional):
Mask to nullify selected heads of the attention modules. Mask values selected in `[0, 1]`:
- 1 indicates the head is **not masked**,
- 0 indicates the head is **masked**.
output_attentions (`bool`, *optional*):
Whether or not to return the attentions tensors of all attention layers. See `attentions` under
returned tensors for more detail.
output_hidden_states (`bool`, *optional*):
Whether or not to return the hidden states of all layers. See `hidden_states` under returned tensors
for more detail.
return_dict (`bool`, *optional*):
Whether or not to return a [`~utils.ModelOutput`] instead of a plain tuple.
"""
if input_features is None:
raise ValueError("You have to specify input_features")
inputs_embeds = self.conv(input_features)
inputs_embeds = self.embed_scale * inputs_embeds
# subsample attention mask if necessary
if attention_mask is not None:
attention_mask = self._get_feature_vector_attention_mask(tf.shape(inputs_embeds)[1], attention_mask)
padding_mask = tf.cast(tf.math.not_equal(attention_mask, 1), tf.int64)
else:
padding_mask = tf.zeros(tf.shape(inputs_embeds)[:-1], dtype=tf.int64)
embed_pos = self.embed_positions(padding_mask)
hidden_states = inputs_embeds + embed_pos
hidden_states = self.dropout(hidden_states, training=training)
# check attention mask and invert
if attention_mask is not None:
# [bsz, seq_len] -> [bsz, 1, tgt_seq_len, src_seq_len]
attention_mask = _expand_mask(attention_mask)
encoder_states = () if output_hidden_states else None
all_attentions = () if output_attentions else None
# check if head_mask has a correct number of layers specified if desired
if head_mask is not None:
tf.debugging.assert_equal(
shape_list(head_mask)[0],
len(self.layers),
message=(
f"The head_mask should be specified for {len(self.layers)} layers, but it is for"
f" {shape_list(head_mask)[0]}."
),
)
for idx, encoder_layer in enumerate(self.layers):
if output_hidden_states:
encoder_states = encoder_states + (hidden_states,)
# add LayerDrop (see https://arxiv.org/abs/1909.11556 for description)
dropout_probability = random.uniform(0, 1)
if training and (dropout_probability < self.layerdrop): # skip the layer
continue
hidden_states, attn = encoder_layer(
hidden_states,
attention_mask,
head_mask[idx] if head_mask is not None else None,
training=training,
)
if output_attentions:
all_attentions += (attn,)
hidden_states = self.layer_norm(hidden_states)
if output_hidden_states:
encoder_states = encoder_states + (hidden_states,)
if not return_dict:
return tuple(v for v in [hidden_states, encoder_states, all_attentions] if v is not None)
return TFBaseModelOutput(
last_hidden_state=hidden_states, hidden_states=encoder_states, attentions=all_attentions
)
def build(self, input_shape=None):
if self.built:
return
self.built = True
if getattr(self, "conv", None) is not None:
with tf.name_scope(self.conv.name):
self.conv.build(None)
if getattr(self, "embed_positions", None) is not None:
with tf.name_scope(self.embed_positions.name):
self.embed_positions.build(None)
if getattr(self, "layer_norm", None) is not None:
with tf.name_scope(self.layer_norm.name):
self.layer_norm.build([None, None, self.config.d_model])
if getattr(self, "layers", None) is not None:
for layer in self.layers:
with tf.name_scope(layer.name):
layer.build(None)
@keras_serializable
class TFSpeech2TextDecoder(keras.layers.Layer):
config_class = Speech2TextConfig
"""
Transformer decoder consisting of *config.decoder_layers* layers. Each layer is a [`TFSpeech2TextDecoderLayer`]
Args:
config: Speech2TextConfig
"""
def __init__(self, config: Speech2TextConfig, **kwargs):
super().__init__(**kwargs)
self.config = config
self.layerdrop = config.decoder_layerdrop
self.padding_idx = config.pad_token_id
self.max_target_positions = config.max_target_positions
self.embed_scale = tf.math.sqrt(float(config.d_model)) if config.scale_embedding else 1.0
self.embed_tokens = TFSharedEmbeddings(config.vocab_size, config.d_model, name="embed_tokens")
self.embed_positions = TFSpeech2TextSinusoidalPositionalEmbedding(
num_positions=config.max_target_positions,
embedding_dim=config.d_model,
padding_idx=self.padding_idx,
name="embed_positions",
)
self.layers = [TFSpeech2TextDecoderLayer(config, name=f"layers.{i}") for i in range(config.decoder_layers)]
self.layer_norm = keras.layers.LayerNormalization(epsilon=1e-5, name="layer_norm")
self.dropout = keras.layers.Dropout(config.dropout)
def get_embed_tokens(self):
return self.embed_tokens
def set_embed_tokens(self, embed_tokens):
self.embed_tokens = embed_tokens
@unpack_inputs
def call(
self,
input_ids=None,
inputs_embeds=None,
attention_mask=None,
encoder_hidden_states=None,
encoder_attention_mask=None,
head_mask=None,
cross_attn_head_mask=None,
past_key_values=None,
use_cache=None,
output_attentions=None,
output_hidden_states=None,
return_dict=None,
training=False,
):
r"""
Args:
input_ids (`tf.Tensor` of shape `(batch_size, sequence_length)`):
Indices of input sequence tokens in the vocabulary. Padding will be ignored by default should you
provide it.
Indices can be obtained using [`Speech2TextTokenizer`]. See [`PreTrainedTokenizer.encode`] and
[`PreTrainedTokenizer.__call__`] for details.
[What are input IDs?](../glossary#input-ids)
attention_mask (`tf.Tensor` of shape `(batch_size, sequence_length)`, *optional*):
Mask to avoid performing attention on padding token indices. Mask values selected in `[0, 1]`:
- 1 for tokens that are **not masked**,
- 0 for tokens that are **masked**.
[What are attention masks?](../glossary#attention-mask)
encoder_hidden_states (`tf.Tensor` of shape `(batch_size, encoder_sequence_length, hidden_size)`, *optional*):
Sequence of hidden-states at the output of the last layer of the encoder. Used in the cross-attention
of the decoder.
encoder_attention_mask (`tf.Tensor` of shape `(batch_size, encoder_sequence_length)`, *optional*):
Mask to avoid performing cross-attention on padding tokens indices of encoder input_ids. Mask values
selected in `[0, 1]`:
- 1 for tokens that are **not masked**,
- 0 for tokens that are **masked**.
[What are attention masks?](../glossary#attention-mask)
head_mask (`tf.Tensor` of shape `(decoder_layers, decoder_attention_heads)`, *optional*):
Mask to nullify selected heads of the attention modules. Mask values selected in `[0, 1]`:
- 1 indicates the head is **not masked**,
- 0 indicates the head is **masked**.
cross_attn_head_mask (`tf.Tensor` of shape `(decoder_layers, decoder_attention_heads)`, *optional*):
Mask to nullify selected heads of the cross-attention modules. Mask values selected in `[0, 1]`:
- 1 indicates the head is **not masked**,
- 0 indicates the head is **masked**.
past_key_values (`Tuple[Tuple[tf.Tensor]]` of length `config.n_layers` with each tuple having 2 tuples each of which has 2 tensors of shape `(batch_size, num_heads, sequence_length - 1, embed_size_per_head)`):
Contains precomputed key and value hidden-states of the attention blocks. Can be used to speed up
decoding.
If `past_key_values` are used, the user can optionally input only the last `decoder_input_ids` (those
that don't have their past key value states given to this model) of shape `(batch_size, 1)` instead of
all `decoder_input_ids` of shape `(batch_size, sequence_length)`.
inputs_embeds (`tf.Tensor` of shape `(batch_size, sequence_length, hidden_size)`, *optional*):
Optionally, instead of passing `input_ids` you can choose to directly pass an embedded representation.
This is useful if you want more control over how to convert `input_ids` indices into associated vectors
than the model's internal embedding lookup matrix.
output_attentions (`bool`, *optional*):
Whether or not to return the attentions tensors of all attention layers. See `attentions` under
returned tensors for more detail.
output_hidden_states (`bool`, *optional*):
Whether or not to return the hidden states of all layers. See `hidden_states` under returned tensors
for more detail.
return_dict (`bool`, *optional*):
Whether or not to return a [`~utils.ModelOutput`] instead of a plain tuple.
"""
if input_ids is not None and inputs_embeds is not None:
raise ValueError("You cannot specify both decoder_input_ids and decoder_inputs_embeds at the same time")
elif input_ids is not None:
input_shape = shape_list(input_ids)
elif inputs_embeds is not None:
input_shape = shape_list(inputs_embeds)[:-1]
else:
raise ValueError("You have to specify either decoder_input_ids or decoder_inputs_embeds")
# past_key_values_length
past_key_values_length = shape_list(past_key_values[0][0])[2] if past_key_values is not None else 0
if inputs_embeds is None:
check_embeddings_within_bounds(input_ids, self.embed_tokens.vocab_size)
inputs_embeds = self.embed_tokens(input_ids) * self.embed_scale
else:
inputs_embeds = inputs_embeds
# [bsz, seq_len] -> [bsz, 1, tgt_seq_len, src_seq_len]
if input_shape[-1] > 1:
combined_attention_mask = _make_causal_mask(input_shape, past_key_values_length=past_key_values_length)
else:
combined_attention_mask = _expand_mask(
tf.ones((input_shape[0], input_shape[1] + past_key_values_length)), tgt_len=input_shape[-1]
)
if attention_mask is not None:
combined_attention_mask = combined_attention_mask + _expand_mask(attention_mask, tgt_len=input_shape[-1])
# expand encoder attention mask
if encoder_hidden_states is not None and encoder_attention_mask is not None:
# [bsz, seq_len] -> [bsz, 1, tgt_seq_len, src_seq_len]
encoder_attention_mask = _expand_mask(encoder_attention_mask, tgt_len=input_shape[-1])
# embed positions
positions = self.embed_positions(input_ids, past_key_values_length=past_key_values_length)
hidden_states = inputs_embeds + positions
hidden_states = self.dropout(hidden_states, training=training)
# decoder layers
all_hidden_states = () if output_hidden_states else None
all_self_attns = () if output_attentions else None
all_cross_attns = () if (output_attentions and encoder_hidden_states is not None) else None
next_decoder_cache = () if use_cache else None
# check if head_mask and cross_attn_head_mask have a correct number of layers specified if desired
for attn_mask_name, attn_mask in [("head_mask", head_mask), ("cross_attn_head_mask", cross_attn_head_mask)]:
if attn_mask is not None:
tf.debugging.assert_equal(
shape_list(attn_mask)[0],
len(self.layers),
message=(
f"The {attn_mask_name} should be specified for {len(self.layers)} layers, but it is for"
f" {shape_list(attn_mask)[0]}."
),
)
for idx, decoder_layer in enumerate(self.layers):
# add LayerDrop (see https://arxiv.org/abs/1909.11556 for description)
if output_hidden_states:
all_hidden_states += (hidden_states,)
dropout_probability = random.uniform(0, 1)
if training and (dropout_probability < self.layerdrop):
continue
past_key_value = past_key_values[idx] if past_key_values is not None else None
cross_attn_layer_head_mask = cross_attn_head_mask[idx] if cross_attn_head_mask is not None else None
hidden_states, layer_self_attn, layer_cross_attn, present_key_value = decoder_layer(
hidden_states,
attention_mask=combined_attention_mask,
encoder_hidden_states=encoder_hidden_states,
encoder_attention_mask=encoder_attention_mask,
layer_head_mask=head_mask[idx] if head_mask is not None else None,
cross_attn_layer_head_mask=cross_attn_layer_head_mask,
past_key_value=past_key_value,
)
if use_cache:
next_decoder_cache += (present_key_value,)
if output_attentions:
all_self_attns += (layer_self_attn,)
if encoder_hidden_states is not None:
all_cross_attns += (layer_cross_attn,)
hidden_states = self.layer_norm(hidden_states)
if output_hidden_states:
all_hidden_states += (hidden_states,)
next_cache = next_decoder_cache if use_cache else None
if not return_dict:
return hidden_states, next_cache, all_hidden_states, all_self_attns, all_cross_attns
else:
return TFBaseModelOutputWithPastAndCrossAttentions(
last_hidden_state=hidden_states,
past_key_values=next_cache,
hidden_states=all_hidden_states,
attentions=all_self_attns,
cross_attentions=all_cross_attns,
)
def build(self, input_shape=None):
if self.built:
return
self.built = True
if getattr(self, "embed_tokens", None) is not None:
with tf.name_scope(self.embed_tokens.name):
self.embed_tokens.build(None)
if getattr(self, "embed_positions", None) is not None:
with tf.name_scope(self.embed_positions.name):
self.embed_positions.build(None)
if getattr(self, "layer_norm", None) is not None:
with tf.name_scope(self.layer_norm.name):
self.layer_norm.build([None, None, self.config.d_model])
if getattr(self, "layers", None) is not None:
for layer in self.layers:
with tf.name_scope(layer.name):
layer.build(None)
@keras_serializable
class TFSpeech2TextMainLayer(keras.layers.Layer):
config_class = Speech2TextConfig
def __init__(self, config: Speech2TextConfig, **kwargs):
super().__init__(**kwargs)
self.config = config
self.encoder = TFSpeech2TextEncoder(config, name="encoder")
self.decoder = TFSpeech2TextDecoder(config, name="decoder")
def get_input_embeddings(self):
return self.decoder.embed_tokens
def set_input_embeddings(self, new_embeddings):
self.decoder.embed_tokens = new_embeddings
@unpack_inputs
def call(
self,
input_features=None,
attention_mask=None,
decoder_input_ids=None,
decoder_attention_mask=None,
head_mask=None,
decoder_head_mask=None,
cross_attn_head_mask=None,
encoder_outputs=None,
past_key_values=None,
decoder_inputs_embeds=None,
use_cache=None,
output_attentions=None,
output_hidden_states=None,
return_dict=None,
training=False,
**kwargs,
):
output_attentions = output_attentions if output_attentions is not None else self.config.output_attentions
output_hidden_states = (
output_hidden_states if output_hidden_states is not None else self.config.output_hidden_states
)
use_cache = use_cache if use_cache is not None else self.config.use_cache
return_dict = return_dict if return_dict is not None else self.config.use_return_dict
if encoder_outputs is None:
encoder_outputs = self.encoder(
input_features=input_features,
attention_mask=attention_mask,
head_mask=head_mask,
output_attentions=output_attentions,
output_hidden_states=output_hidden_states,
return_dict=return_dict,
training=training,
)
# If the user passed a tuple for encoder_outputs, we wrap it in a TFBaseModelOutput when return_dict=True
elif return_dict and not isinstance(encoder_outputs, TFBaseModelOutput):
encoder_outputs = TFBaseModelOutput(
last_hidden_state=encoder_outputs[0],
hidden_states=encoder_outputs[1] if len(encoder_outputs) > 1 else None,
attentions=encoder_outputs[2] if len(encoder_outputs) > 2 else None,
)
# If the user passed a TFBaseModelOutput for encoder_outputs, we wrap it in a tuple when return_dict=False
elif not return_dict and not isinstance(encoder_outputs, tuple):
encoder_outputs = encoder_outputs.to_tuple()
# downsample encoder attention mask
if attention_mask is not None:
encoder_attention_mask = self.encoder._get_feature_vector_attention_mask(
tf.shape(encoder_outputs[0])[1], attention_mask
)
else:
encoder_attention_mask = None
# decoder outputs consists of (dec_features, past_key_value, dec_hidden, dec_attn)
decoder_outputs = self.decoder(
input_ids=decoder_input_ids,
attention_mask=decoder_attention_mask,
encoder_hidden_states=encoder_outputs[0],
encoder_attention_mask=encoder_attention_mask,
head_mask=decoder_head_mask,
cross_attn_head_mask=cross_attn_head_mask,
past_key_values=past_key_values,
inputs_embeds=decoder_inputs_embeds,
use_cache=use_cache,
output_attentions=output_attentions,
output_hidden_states=output_hidden_states,
return_dict=return_dict,
training=training,
)
if not return_dict:
return decoder_outputs + encoder_outputs
return TFSeq2SeqModelOutput(
last_hidden_state=decoder_outputs.last_hidden_state,
past_key_values=decoder_outputs.past_key_values,
decoder_hidden_states=decoder_outputs.hidden_states,
decoder_attentions=decoder_outputs.attentions,
cross_attentions=decoder_outputs.cross_attentions,
encoder_last_hidden_state=encoder_outputs.last_hidden_state,
encoder_hidden_states=encoder_outputs.hidden_states,
encoder_attentions=encoder_outputs.attentions,
)
def build(self, input_shape=None):
if self.built:
return
self.built = True
if getattr(self, "encoder", None) is not None:
with tf.name_scope(self.encoder.name):
self.encoder.build(None)
if getattr(self, "decoder", None) is not None:
with tf.name_scope(self.decoder.name):
self.decoder.build(None)
@add_start_docstrings(
"The bare Speech2Text Model outputting raw hidden-states without any specific head on top.",
SPEECH_TO_TEXT_START_DOCSTRING,
)
class TFSpeech2TextModel(TFSpeech2TextPreTrainedModel):
def __init__(self, config: Speech2TextConfig, *inputs, **kwargs):
super().__init__(config, *inputs, **kwargs)
self.model = TFSpeech2TextMainLayer(config, name="model")
def get_encoder(self):
return self.model.encoder
def get_decoder(self):
return self.model.decoder
@unpack_inputs
@add_start_docstrings_to_model_forward(SPEECH_TO_TEXT_INPUTS_DOCSTRING)
@add_code_sample_docstrings(
checkpoint=_CHECKPOINT_FOR_DOC,
output_type=TFSeq2SeqModelOutput,
config_class=_CONFIG_FOR_DOC,
)
def call(
self,
input_features: TFModelInputType | None = None,
attention_mask: np.ndarray | tf.Tensor | None = None,
decoder_input_ids: np.ndarray | tf.Tensor | None = None,
decoder_attention_mask: np.ndarray | tf.Tensor | None = None,
head_mask: np.ndarray | tf.Tensor | None = None,
decoder_head_mask: np.ndarray | tf.Tensor | None = None,
cross_attn_head_mask: np.ndarray | tf.Tensor | None = None,
encoder_outputs: np.ndarray | tf.Tensor | None = None,
past_key_values: Optional[Tuple[Tuple[Union[np.ndarray, tf.Tensor]]]] = None,
decoder_inputs_embeds: np.ndarray | tf.Tensor | None = None,
use_cache: Optional[bool] = None,
output_attentions: Optional[bool] = None,
output_hidden_states: Optional[bool] = None,
return_dict: Optional[bool] = None,
training: bool = False,
**kwargs,
) -> Union[Tuple, TFSeq2SeqModelOutput]:
outputs = self.model(
input_features=input_features,
attention_mask=attention_mask,
decoder_input_ids=decoder_input_ids,
decoder_attention_mask=decoder_attention_mask,
head_mask=head_mask,
decoder_head_mask=decoder_head_mask,
cross_attn_head_mask=cross_attn_head_mask,
encoder_outputs=encoder_outputs,
past_key_values=past_key_values,
decoder_inputs_embeds=decoder_inputs_embeds,
use_cache=use_cache,
output_attentions=output_attentions,
output_hidden_states=output_hidden_states,
return_dict=return_dict,
training=training,
)
return outputs
def serving_output(self, output):
pkv = tf.tuple(output.past_key_values)[1] if self.config.use_cache else None
dec_hs = tf.convert_to_tensor(output.decoder_hidden_states) if self.config.output_hidden_states else None
dec_attns = tf.convert_to_tensor(output.decoder_attentions) if self.config.output_attentions else None
cross_attns = tf.convert_to_tensor(output.cross_attentions) if self.config.output_attentions else None
enc_hs = tf.convert_to_tensor(output.encoder_hidden_states) if self.config.output_hidden_states else None
enc_attns = tf.convert_to_tensor(output.encoder_attentions) if self.config.output_attentions else None
return TFSeq2SeqModelOutput(
last_hidden_state=output.last_hidden_state,
past_key_values=pkv,
decoder_hidden_states=dec_hs,
decoder_attentions=dec_attns,
cross_attentions=cross_attns,
encoder_last_hidden_state=output.encoder_last_hidden_state,
encoder_hidden_states=enc_hs,
encoder_attentions=enc_attns,
)
def build(self, input_shape=None):
if self.built:
return
self.built = True
if getattr(self, "model", None) is not None:
with tf.name_scope(self.model.name):
self.model.build(None)
@add_start_docstrings(
"The Speech2Text Model with a language modeling head. Can be used for summarization.",
SPEECH_TO_TEXT_START_DOCSTRING,
)
class TFSpeech2TextForConditionalGeneration(TFSpeech2TextPreTrainedModel, TFCausalLanguageModelingLoss):
def __init__(self, config: Speech2TextConfig):
super().__init__(config)
self.model = TFSpeech2TextMainLayer(config, name="model")
self.lm_head = keras.layers.Dense(self.config.vocab_size, use_bias=False, name="lm_head")
# TODO (Joao): investigate why Speech2Text has numerical issues in XLA generate
self.supports_xla_generation = False
self.config = config
def get_encoder(self):
return self.model.encoder
def get_decoder(self):
return self.model.decoder
def resize_token_embeddings(self, new_num_tokens: int) -> tf.Variable:
new_embeddings = super().resize_token_embeddings(new_num_tokens)
return new_embeddings
def get_output_embeddings(self):
return self.lm_head
def set_output_embeddings(self, new_embeddings):
self.lm_head = new_embeddings
@unpack_inputs
@add_start_docstrings_to_model_forward(SPEECH_TO_TEXT_INPUTS_DOCSTRING)
@replace_return_docstrings(output_type=TFSeq2SeqLMOutput, config_class=_CONFIG_FOR_DOC)
def call(
self,
input_features: TFModelInputType | None = None,
attention_mask: np.ndarray | tf.Tensor | None = None,
decoder_input_ids: np.ndarray | tf.Tensor | None = None,
decoder_attention_mask: np.ndarray | tf.Tensor | None = None,
head_mask: np.ndarray | tf.Tensor | None = None,
decoder_head_mask: np.ndarray | tf.Tensor | None = None,
cross_attn_head_mask: np.ndarray | tf.Tensor | None = None,
encoder_outputs: np.ndarray | tf.Tensor | None = None,
past_key_values: Optional[Tuple[Tuple[Union[np.ndarray, tf.Tensor]]]] = None,
decoder_inputs_embeds: np.ndarray | tf.Tensor | None = None,
labels: np.ndarray | tf.Tensor | None = None,
use_cache: Optional[bool] = None,
output_attentions: Optional[bool] = None,
output_hidden_states: Optional[bool] = None,
return_dict: Optional[bool] = None,
training: Optional[bool] = False,
**kwargs,
) -> Union[Tuple, TFSeq2SeqLMOutput]:
r"""
labels (`tf.Tensor` of shape `(batch_size, sequence_length)`, *optional*):
Labels for computing the masked language modeling loss. Indices should either be in `[0, ...,
config.vocab_size]` or -100 (see `input_ids` docstring). Tokens with indices set to `-100` are ignored
(masked), the loss is only computed for the tokens with labels in `[0, ..., config.vocab_size]`.
Returns:
Example:
```python
>>> import tensorflow as tf
>>> from transformers import Speech2TextProcessor, TFSpeech2TextForConditionalGeneration
>>> from datasets import load_dataset
>>> import soundfile as sf
>>> model = TFSpeech2TextForConditionalGeneration.from_pretrained(
... "facebook/s2t-small-librispeech-asr", from_pt=True
... )
>>> processor = Speech2TextProcessor.from_pretrained("facebook/s2t-small-librispeech-asr")
>>> def map_to_array(batch):
... speech, _ = sf.read(batch["file"])
... batch["speech"] = speech
... return batch
>>> ds = load_dataset("hf-internal-testing/librispeech_asr_dummy", "clean", split="validation")
>>> ds = ds.map(map_to_array)
>>> ds.set_format(type="tf")
>>> input_features = processor(
... ds["speech"][0], sampling_rate=16000, return_tensors="tf"
... ).input_features # Batch size 1
>>> generated_ids = model.generate(input_features)
>>> transcription = processor.batch_decode(generated_ids)
```"""
return_dict = return_dict if return_dict is not None else self.config.use_return_dict
if labels is not None:
if decoder_input_ids is None and decoder_inputs_embeds is None:
decoder_input_ids = shift_tokens_right(
labels, self.config.pad_token_id, self.config.decoder_start_token_id
)
outputs = self.model(
input_features=input_features,
attention_mask=attention_mask,
decoder_input_ids=decoder_input_ids,
encoder_outputs=encoder_outputs,
decoder_attention_mask=decoder_attention_mask,
head_mask=head_mask,
decoder_head_mask=decoder_head_mask,
cross_attn_head_mask=cross_attn_head_mask,
past_key_values=past_key_values,
decoder_inputs_embeds=decoder_inputs_embeds,
use_cache=use_cache,
output_attentions=output_attentions,
output_hidden_states=output_hidden_states,
return_dict=return_dict,
training=training,
)
lm_logits = self.lm_head(outputs[0])
masked_lm_loss = None if labels is None else self.hf_compute_loss(labels, lm_logits)
if not return_dict:
output = (lm_logits,) + outputs[1:]
return ((masked_lm_loss,) + output) if masked_lm_loss is not None else output
return TFSeq2SeqLMOutput(
loss=masked_lm_loss,
logits=lm_logits,
past_key_values=outputs.past_key_values,
decoder_hidden_states=outputs.decoder_hidden_states,
decoder_attentions=outputs.decoder_attentions,
cross_attentions=outputs.cross_attentions,
encoder_last_hidden_state=outputs.encoder_last_hidden_state,
encoder_hidden_states=outputs.encoder_hidden_states,
encoder_attentions=outputs.encoder_attentions,
)
def serving_output(self, output):
pkv = tf.tuple(output.past_key_values)[1] if self.config.use_cache else None
dec_hs = tf.convert_to_tensor(output.decoder_hidden_states) if self.config.output_hidden_states else None
dec_attns = tf.convert_to_tensor(output.decoder_attentions) if self.config.output_attentions else None
cross_attns = tf.convert_to_tensor(output.cross_attentions) if self.config.output_attentions else None
enc_hs = tf.convert_to_tensor(output.encoder_hidden_states) if self.config.output_hidden_states else None
enc_attns = tf.convert_to_tensor(output.encoder_attentions) if self.config.output_attentions else None
return TFSeq2SeqLMOutput(
logits=output.logits,
past_key_values=pkv,
decoder_hidden_states=dec_hs,
decoder_attentions=dec_attns,
cross_attentions=cross_attns,
encoder_last_hidden_state=output.encoder_last_hidden_state,
encoder_hidden_states=enc_hs,
encoder_attentions=enc_attns,
)
def prepare_inputs_for_generation(
self,
decoder_input_ids,
past_key_values=None,
attention_mask=None,
head_mask=None,
decoder_head_mask=None,
cross_attn_head_mask=None,
use_cache=None,
encoder_outputs=None,
**kwargs,
):
# cut decoder_input_ids if past is used
if past_key_values is not None:
decoder_input_ids = decoder_input_ids[:, -1:]
return {
"input_features": None, # needs to be passed to make Keras.layer.__call__ happy
"encoder_outputs": encoder_outputs,
"past_key_values": past_key_values,
"decoder_input_ids": decoder_input_ids,
"attention_mask": attention_mask,
"head_mask": head_mask,
"decoder_head_mask": decoder_head_mask,
"cross_attn_head_mask": cross_attn_head_mask,
"use_cache": use_cache, # change this to avoid caching (presumably for debugging)
}
def build(self, input_shape=None):
if self.built:
return
self.built = True
if getattr(self, "model", None) is not None:
with tf.name_scope(self.model.name):
self.model.build(None)
if getattr(self, "lm_head", None) is not None:
with tf.name_scope(self.lm_head.name):
self.lm_head.build([None, None, self.config.d_model])
def tf_to_pt_weight_rename(self, tf_weight):
if tf_weight == "lm_head.weight":
return tf_weight, "model.decoder.embed_tokens.weight"
else:
return (tf_weight,)
| transformers/src/transformers/models/speech_to_text/modeling_tf_speech_to_text.py/0 | {
"file_path": "transformers/src/transformers/models/speech_to_text/modeling_tf_speech_to_text.py",
"repo_id": "transformers",
"token_count": 32975
} | 362 |
# coding=utf-8
# Copyright 2023 The Facebook Inc. and The HuggingFace Inc. team. All rights reserved.
#
# Licensed under the Apache License, Version 2.0 (the "License");
# you may not use this file except in compliance with the License.
# You may obtain a copy of the License at
#
# http://www.apache.org/licenses/LICENSE-2.0
#
# Unless required by applicable law or agreed to in writing, software
# distributed under the License is distributed on an "AS IS" BASIS,
# WITHOUT WARRANTIES OR CONDITIONS OF ANY KIND, either express or implied.
# See the License for the specific language governing permissions and
# limitations under the License.
"""Tokenization class for SpeechT5."""
import os
from shutil import copyfile
from typing import Any, Dict, List, Optional, Tuple
import sentencepiece as spm
from ...tokenization_utils import PreTrainedTokenizer
from ...utils import logging
from .number_normalizer import EnglishNumberNormalizer
logger = logging.get_logger(__name__)
VOCAB_FILES_NAMES = {"vocab_file": "spm_char.model"}
PRETRAINED_VOCAB_FILES_MAP = {
"vocab_file": {
"microsoft/speecht5_asr": "https://huggingface.co/microsoft/speecht5_asr/resolve/main/spm_char.model",
"microsoft/speecht5_tts": "https://huggingface.co/microsoft/speecht5_tts/resolve/main/spm_char.model",
"microsoft/speecht5_vc": "https://huggingface.co/microsoft/speecht5_vc/resolve/main/spm_char.model",
}
}
PRETRAINED_POSITIONAL_EMBEDDINGS_SIZES = {
"microsoft/speecht5_asr": 1024,
"microsoft/speecht5_tts": 1024,
"microsoft/speecht5_vc": 1024,
}
class SpeechT5Tokenizer(PreTrainedTokenizer):
"""
Construct a SpeechT5 tokenizer. Based on [SentencePiece](https://github.com/google/sentencepiece).
This tokenizer inherits from [`PreTrainedTokenizer`] which contains most of the main methods. Users should refer to
this superclass for more information regarding those methods.
Args:
vocab_file (`str`):
[SentencePiece](https://github.com/google/sentencepiece) file (generally has a *.spm* extension) that
contains the vocabulary necessary to instantiate a tokenizer.
bos_token (`str`, *optional*, defaults to `"<s>"`):
The begin of sequence token.
eos_token (`str`, *optional*, defaults to `"</s>"`):
The end of sequence token.
unk_token (`str`, *optional*, defaults to `"<unk>"`):
The unknown token. A token that is not in the vocabulary cannot be converted to an ID and is set to be this
token instead.
pad_token (`str`, *optional*, defaults to `"<pad>"`):
The token used for padding, for example when batching sequences of different lengths.
normalize (`bool`, *optional*, defaults to `False`):
Whether to convert numeric quantities in the text to their spelt-out english counterparts.
sp_model_kwargs (`dict`, *optional*):
Will be passed to the `SentencePieceProcessor.__init__()` method. The [Python wrapper for
SentencePiece](https://github.com/google/sentencepiece/tree/master/python) can be used, among other things,
to set:
- `enable_sampling`: Enable subword regularization.
- `nbest_size`: Sampling parameters for unigram. Invalid for BPE-Dropout.
- `nbest_size = {0,1}`: No sampling is performed.
- `nbest_size > 1`: samples from the nbest_size results.
- `nbest_size < 0`: assuming that nbest_size is infinite and samples from the all hypothesis (lattice)
using forward-filtering-and-backward-sampling algorithm.
- `alpha`: Smoothing parameter for unigram sampling, and dropout probability of merge operations for
BPE-dropout.
Attributes:
sp_model (`SentencePieceProcessor`):
The *SentencePiece* processor that is used for every conversion (string, tokens and IDs).
"""
vocab_files_names = VOCAB_FILES_NAMES
pretrained_vocab_files_map = PRETRAINED_VOCAB_FILES_MAP
max_model_input_sizes = PRETRAINED_POSITIONAL_EMBEDDINGS_SIZES
model_input_names = ["input_ids", "attention_mask"]
def __init__(
self,
vocab_file,
bos_token="<s>",
eos_token="</s>",
unk_token="<unk>",
pad_token="<pad>",
normalize=False,
sp_model_kwargs: Optional[Dict[str, Any]] = None,
**kwargs,
) -> None:
self.sp_model_kwargs = {} if sp_model_kwargs is None else sp_model_kwargs
self.vocab_file = vocab_file
self.normalize = normalize
self._normalizer = None
self.sp_model = spm.SentencePieceProcessor(**self.sp_model_kwargs)
self.sp_model.Load(vocab_file)
super().__init__(
bos_token=bos_token,
eos_token=eos_token,
unk_token=unk_token,
pad_token=pad_token,
normalize=normalize,
sp_model_kwargs=self.sp_model_kwargs,
**kwargs,
)
def prepare_for_tokenization(self, text, is_split_into_words=False, **kwargs):
normalize = kwargs.pop("normalize", self.normalize)
if is_split_into_words:
text = " " + text
if normalize:
text = self.normalizer(text)
return (text, kwargs)
@property
def vocab_size(self):
return self.sp_model.get_piece_size()
@property
def normalizer(self):
if self._normalizer is None:
self._normalizer = EnglishNumberNormalizer()
return self._normalizer
@normalizer.setter
def normalizer(self, value):
self._normalizer = value
def get_vocab(self):
vocab = {self.convert_ids_to_tokens(i): i for i in range(self.vocab_size)}
vocab.update(self.added_tokens_encoder)
return vocab
def __getstate__(self):
state = self.__dict__.copy()
state["sp_model"] = None
return state
def __setstate__(self, d):
self.__dict__ = d
# for backward compatibility
if not hasattr(self, "sp_model_kwargs"):
self.sp_model_kwargs = {}
self.sp_model = spm.SentencePieceProcessor(**self.sp_model_kwargs)
self.sp_model.Load(self.vocab_file)
def _tokenize(self, text: str) -> List[str]:
"""Take as input a string and return a list of strings (tokens) for words/sub-words"""
return self.sp_model.encode(text, out_type=str)
def _convert_token_to_id(self, token):
"""Converts a token (str) in an id using the vocab."""
return self.sp_model.piece_to_id(token)
def _convert_id_to_token(self, index):
"""Converts an index (integer) in a token (str) using the vocab."""
token = self.sp_model.IdToPiece(index)
return token
# Copied from transformers.models.albert.tokenization_albert.AlbertTokenizer.convert_tokens_to_string
def convert_tokens_to_string(self, tokens):
"""Converts a sequence of tokens (string) in a single string."""
current_sub_tokens = []
out_string = ""
prev_is_special = False
for token in tokens:
# make sure that special tokens are not decoded using sentencepiece model
if token in self.all_special_tokens:
if not prev_is_special:
out_string += " "
out_string += self.sp_model.decode(current_sub_tokens) + token
prev_is_special = True
current_sub_tokens = []
else:
current_sub_tokens.append(token)
prev_is_special = False
out_string += self.sp_model.decode(current_sub_tokens)
return out_string.strip()
def build_inputs_with_special_tokens(self, token_ids_0, token_ids_1=None) -> List[int]:
"""Build model inputs from a sequence by appending eos_token_id."""
if token_ids_1 is None:
return token_ids_0 + [self.eos_token_id]
# We don't expect to process pairs, but leave the pair logic for API consistency
return token_ids_0 + token_ids_1 + [self.eos_token_id]
def get_special_tokens_mask(
self, token_ids_0: List[int], token_ids_1: Optional[List[int]] = None, already_has_special_tokens: bool = False
) -> List[int]:
if already_has_special_tokens:
return super().get_special_tokens_mask(
token_ids_0=token_ids_0, token_ids_1=token_ids_1, already_has_special_tokens=True
)
suffix_ones = [1]
if token_ids_1 is None:
return ([0] * len(token_ids_0)) + suffix_ones
return ([0] * len(token_ids_0)) + ([0] * len(token_ids_1)) + suffix_ones
def save_vocabulary(self, save_directory: str, filename_prefix: Optional[str] = None) -> Tuple[str]:
if not os.path.isdir(save_directory):
logger.error(f"Vocabulary path ({save_directory}) should be a directory")
return
out_vocab_file = os.path.join(
save_directory, (filename_prefix + "-" if filename_prefix else "") + VOCAB_FILES_NAMES["vocab_file"]
)
if os.path.abspath(self.vocab_file) != os.path.abspath(out_vocab_file) and os.path.isfile(self.vocab_file):
copyfile(self.vocab_file, out_vocab_file)
elif not os.path.isfile(self.vocab_file):
with open(out_vocab_file, "wb") as fi:
content_spiece_model = self.sp_model.serialized_model_proto()
fi.write(content_spiece_model)
return (out_vocab_file,)
| transformers/src/transformers/models/speecht5/tokenization_speecht5.py/0 | {
"file_path": "transformers/src/transformers/models/speecht5/tokenization_speecht5.py",
"repo_id": "transformers",
"token_count": 4079
} | 363 |
# coding=utf-8
# Copyright 2022 The HuggingFace Inc. team. All rights reserved.
#
# Licensed under the Apache License, Version 2.0 (the "License");
# you may not use this file except in compliance with the License.
# You may obtain a copy of the License at
#
# http://www.apache.org/licenses/LICENSE-2.0
#
# Unless required by applicable law or agreed to in writing, software
# distributed under the License is distributed on an "AS IS" BASIS,
# WITHOUT WARRANTIES OR CONDITIONS OF ANY KIND, either express or implied.
# See the License for the specific language governing permissions and
# limitations under the License.
""" Swin Transformer model configuration"""
from collections import OrderedDict
from typing import Mapping
from packaging import version
from ...configuration_utils import PretrainedConfig
from ...onnx import OnnxConfig
from ...utils import logging
from ...utils.backbone_utils import BackboneConfigMixin, get_aligned_output_features_output_indices
logger = logging.get_logger(__name__)
SWIN_PRETRAINED_CONFIG_ARCHIVE_MAP = {
"microsoft/swin-tiny-patch4-window7-224": (
"https://huggingface.co/microsoft/swin-tiny-patch4-window7-224/resolve/main/config.json"
),
# See all Swin models at https://huggingface.co/models?filter=swin
}
class SwinConfig(BackboneConfigMixin, PretrainedConfig):
r"""
This is the configuration class to store the configuration of a [`SwinModel`]. It is used to instantiate a Swin
model according to the specified arguments, defining the model architecture. Instantiating a configuration with the
defaults will yield a similar configuration to that of the Swin
[microsoft/swin-tiny-patch4-window7-224](https://huggingface.co/microsoft/swin-tiny-patch4-window7-224)
architecture.
Configuration objects inherit from [`PretrainedConfig`] and can be used to control the model outputs. Read the
documentation from [`PretrainedConfig`] for more information.
Args:
image_size (`int`, *optional*, defaults to 224):
The size (resolution) of each image.
patch_size (`int`, *optional*, defaults to 4):
The size (resolution) of each patch.
num_channels (`int`, *optional*, defaults to 3):
The number of input channels.
embed_dim (`int`, *optional*, defaults to 96):
Dimensionality of patch embedding.
depths (`list(int)`, *optional*, defaults to `[2, 2, 6, 2]`):
Depth of each layer in the Transformer encoder.
num_heads (`list(int)`, *optional*, defaults to `[3, 6, 12, 24]`):
Number of attention heads in each layer of the Transformer encoder.
window_size (`int`, *optional*, defaults to 7):
Size of windows.
mlp_ratio (`float`, *optional*, defaults to 4.0):
Ratio of MLP hidden dimensionality to embedding dimensionality.
qkv_bias (`bool`, *optional*, defaults to `True`):
Whether or not a learnable bias should be added to the queries, keys and values.
hidden_dropout_prob (`float`, *optional*, defaults to 0.0):
The dropout probability for all fully connected layers in the embeddings and encoder.
attention_probs_dropout_prob (`float`, *optional*, defaults to 0.0):
The dropout ratio for the attention probabilities.
drop_path_rate (`float`, *optional*, defaults to 0.1):
Stochastic depth rate.
hidden_act (`str` or `function`, *optional*, defaults to `"gelu"`):
The non-linear activation function (function or string) in the encoder. If string, `"gelu"`, `"relu"`,
`"selu"` and `"gelu_new"` are supported.
use_absolute_embeddings (`bool`, *optional*, defaults to `False`):
Whether or not to add absolute position embeddings to the patch embeddings.
initializer_range (`float`, *optional*, defaults to 0.02):
The standard deviation of the truncated_normal_initializer for initializing all weight matrices.
layer_norm_eps (`float`, *optional*, defaults to 1e-05):
The epsilon used by the layer normalization layers.
encoder_stride (`int`, *optional*, defaults to 32):
Factor to increase the spatial resolution by in the decoder head for masked image modeling.
out_features (`List[str]`, *optional*):
If used as backbone, list of features to output. Can be any of `"stem"`, `"stage1"`, `"stage2"`, etc.
(depending on how many stages the model has). If unset and `out_indices` is set, will default to the
corresponding stages. If unset and `out_indices` is unset, will default to the last stage. Must be in the
same order as defined in the `stage_names` attribute.
out_indices (`List[int]`, *optional*):
If used as backbone, list of indices of features to output. Can be any of 0, 1, 2, etc. (depending on how
many stages the model has). If unset and `out_features` is set, will default to the corresponding stages.
If unset and `out_features` is unset, will default to the last stage. Must be in the
same order as defined in the `stage_names` attribute.
Example:
```python
>>> from transformers import SwinConfig, SwinModel
>>> # Initializing a Swin microsoft/swin-tiny-patch4-window7-224 style configuration
>>> configuration = SwinConfig()
>>> # Initializing a model (with random weights) from the microsoft/swin-tiny-patch4-window7-224 style configuration
>>> model = SwinModel(configuration)
>>> # Accessing the model configuration
>>> configuration = model.config
```"""
model_type = "swin"
attribute_map = {
"num_attention_heads": "num_heads",
"num_hidden_layers": "num_layers",
}
def __init__(
self,
image_size=224,
patch_size=4,
num_channels=3,
embed_dim=96,
depths=[2, 2, 6, 2],
num_heads=[3, 6, 12, 24],
window_size=7,
mlp_ratio=4.0,
qkv_bias=True,
hidden_dropout_prob=0.0,
attention_probs_dropout_prob=0.0,
drop_path_rate=0.1,
hidden_act="gelu",
use_absolute_embeddings=False,
initializer_range=0.02,
layer_norm_eps=1e-5,
encoder_stride=32,
out_features=None,
out_indices=None,
**kwargs,
):
super().__init__(**kwargs)
self.image_size = image_size
self.patch_size = patch_size
self.num_channels = num_channels
self.embed_dim = embed_dim
self.depths = depths
self.num_layers = len(depths)
self.num_heads = num_heads
self.window_size = window_size
self.mlp_ratio = mlp_ratio
self.qkv_bias = qkv_bias
self.hidden_dropout_prob = hidden_dropout_prob
self.attention_probs_dropout_prob = attention_probs_dropout_prob
self.drop_path_rate = drop_path_rate
self.hidden_act = hidden_act
self.use_absolute_embeddings = use_absolute_embeddings
self.layer_norm_eps = layer_norm_eps
self.initializer_range = initializer_range
self.encoder_stride = encoder_stride
# we set the hidden_size attribute in order to make Swin work with VisionEncoderDecoderModel
# this indicates the channel dimension after the last stage of the model
self.hidden_size = int(embed_dim * 2 ** (len(depths) - 1))
self.stage_names = ["stem"] + [f"stage{idx}" for idx in range(1, len(depths) + 1)]
self._out_features, self._out_indices = get_aligned_output_features_output_indices(
out_features=out_features, out_indices=out_indices, stage_names=self.stage_names
)
class SwinOnnxConfig(OnnxConfig):
torch_onnx_minimum_version = version.parse("1.11")
@property
def inputs(self) -> Mapping[str, Mapping[int, str]]:
return OrderedDict(
[
("pixel_values", {0: "batch", 1: "num_channels", 2: "height", 3: "width"}),
]
)
@property
def atol_for_validation(self) -> float:
return 1e-4
| transformers/src/transformers/models/swin/configuration_swin.py/0 | {
"file_path": "transformers/src/transformers/models/swin/configuration_swin.py",
"repo_id": "transformers",
"token_count": 3174
} | 364 |
import argparse
import json
import os
import tensorstore as ts
import torch
from flax import serialization
from flax.traverse_util import flatten_dict, unflatten_dict
from tensorflow.io import gfile
from transformers.modeling_utils import dtype_byte_size
from transformers.models.switch_transformers.convert_switch_transformers_original_flax_checkpoint_to_pytorch import (
rename_keys,
)
from transformers.utils import WEIGHTS_INDEX_NAME, WEIGHTS_NAME
from transformers.utils.hub import convert_file_size_to_int
def rename_base_flax_keys(flax_key_tuple, flax_tensor):
"""
Post renaming of basic JAX keys to pytorch.
"""
if flax_key_tuple[-1] == "kernel" and flax_tensor.ndim == 3:
# expert layer
flax_key_tuple = flax_key_tuple[:-1] + ("weight",)
flax_tensor = torch.permute(flax_tensor, (0, 2, 1))
elif flax_key_tuple[-1] == "kernel" and ".".join(flax_key_tuple):
# linear layer
flax_key_tuple = flax_key_tuple[:-1] + ("weight",)
flax_tensor = flax_tensor.T
elif flax_key_tuple[-1] in ["scale", "embedding"]:
flax_key_tuple = flax_key_tuple[:-1] + ("weight",)
return flax_key_tuple, flax_tensor
def get_key_and_tensorstore_dict(layer, checkpoint_info, switch_checkpoint_path):
if "metadata" in layer:
split_layer = layer.split("metadata")
curr_real_layer_name = "".join(split_layer[0])[:-1]
split_layer = [tuple(("metadata" + split_layer[1]).split("/"))]
elif "kvstore" in layer:
split_layer = layer.split("kvstore")
curr_real_layer_name = "".join(split_layer[0])[:-1]
split_layer = [tuple(("kvstore" + split_layer[1]).split("/"))]
else:
split_layer = layer.split("/")
curr_real_layer_name = "/".join(split_layer[:-1])
split_layer[-1] = (split_layer[-1],)
if "kvstore/path" in layer:
content = f"{switch_checkpoint_path}/{checkpoint_info[layer]}"
elif "kvstore/driver" in layer:
content = "file"
else:
content = checkpoint_info[layer]
return curr_real_layer_name, split_layer, content
def rename_and_save_block(current_block, save_path):
current_block = rename_keys(current_block)
new_current_block = {}
for k, v in current_block.items():
new_current_block[k.replace("/", ".")] = v
current_block = new_current_block
torch.save(current_block, save_path)
def shard_on_the_fly(switch_checkpoint_path, dump_path, max_shard_size, dtype, weights_name: str = WEIGHTS_NAME):
max_shard_size = convert_file_size_to_int(max_shard_size)
sharded_state_dicts = []
current_block = {}
current_block_size = 0
total_size = 0
os.makedirs(dump_path, exist_ok=True)
with gfile.GFile(switch_checkpoint_path + "/checkpoint", "rb") as fp:
checkpoint_info = serialization.msgpack_restore(fp.read())["optimizer"]["target"]
checkpoint_info = flatten_dict(checkpoint_info, sep="/")
all_layers = {}
for layer in checkpoint_info.keys():
curr_real_layer_name, split_layer, content = get_key_and_tensorstore_dict(
layer, checkpoint_info, switch_checkpoint_path
)
if curr_real_layer_name in all_layers:
all_layers[curr_real_layer_name][split_layer[-1]] = content
else:
all_layers[curr_real_layer_name] = {split_layer[-1]: content}
for key in all_layers.keys():
# open tensorstore file
raw_weights = ts.open(unflatten_dict(all_layers[key])).result().read().result()
raw_weights = torch.tensor(raw_weights)
weight_size = raw_weights.numel() * dtype_byte_size(raw_weights.dtype)
# use the renaming pattern from the small conversion scripts
key, raw_weights = rename_base_flax_keys(tuple(key.split("/")), raw_weights)
key = "/".join(key)
# If this weight is going to tip up over the maximal size, we split.
if current_block_size + weight_size > max_shard_size:
save_path = os.path.join(
dump_path, weights_name.replace(".bin", f"-{len(sharded_state_dicts)+1:05d}-of-???.bin")
)
rename_and_save_block(current_block, save_path)
sharded_state_dicts.append(current_block.keys())
del current_block
current_block = {}
current_block_size = 0
current_block[key] = raw_weights.to(getattr(torch, dtype))
current_block_size += weight_size
total_size += weight_size
# Add the last block
save_path = os.path.join(dump_path, weights_name.replace(".bin", f"-{len(sharded_state_dicts)+1:05d}-of-???.bin"))
rename_and_save_block(current_block, save_path)
sharded_state_dicts.append(current_block.keys())
# If we only have one shard, we return it
if len(sharded_state_dicts) == 1:
return {weights_name: sharded_state_dicts[0]}, None
# Otherwise, let's build the index
weight_map = {}
shards = {}
for idx, shard in enumerate(sharded_state_dicts):
shard_file = weights_name.replace(
".bin", f"-{idx+1:05d}-of-{len(sharded_state_dicts):05d}.bin"
) # len(sharded_state_dicts):05d}
temp_filename = os.path.join(dump_path, weights_name.replace(".bin", f"-{idx+1:05d}-of-???.bin"))
os.rename(temp_filename, os.path.join(dump_path, shard_file))
shards[shard_file] = shard
for key in shard:
weight_map[key] = shard_file
# Add the metadata
metadata = {"total_size": total_size}
index = {"metadata": metadata, "weight_map": weight_map}
with open(os.path.join(dump_path, WEIGHTS_INDEX_NAME), "w", encoding="utf-8") as f:
content = json.dumps(index, indent=2, sort_keys=True) + "\n"
f.write(content)
return metadata, index
if __name__ == "__main__":
parser = argparse.ArgumentParser()
# Required parameters
parser.add_argument(
"--switch_t5x_checkpoint_path",
default="/mnt/disks/disk_switch/original_checkpoints/switch-xxl-128/checkpoint_634600",
type=str,
required=False,
help="Path to a directory containing a folder per layer. Follows the original Google format.",
)
parser.add_argument("--max_shard_size", default="10GB", required=False, help="Max shard size")
parser.add_argument("--dtype", default="bfloat16", type=str, required=False, help="dtype of the saved model")
parser.add_argument(
"--pytorch_dump_folder_path",
default="/mnt/disks/disk_switch/original_checkpoints/switch-xxl-128-converted",
type=str,
required=False,
help="Path to the output pytorch model.",
)
args = parser.parse_args()
shard_on_the_fly(
args.switch_t5x_checkpoint_path,
args.pytorch_dump_folder_path,
args.max_shard_size,
args.dtype,
)
def sanity_check():
from transformers import SwitchTransformersConfig, SwitchTransformersForConditionalGeneration, T5Tokenizer
config = SwitchTransformersConfig.from_pretrained("google/switch-base-8")
config.save_pretrained("/home/arthur_huggingface_co/transformers/switch_converted")
model = SwitchTransformersForConditionalGeneration.from_pretrained(
"/home/arthur_huggingface_co/transformers/switch_converted", device_map="auto"
)
tokenizer = T5Tokenizer.from_pretrained("t5-small")
text = "A <extra_id_0> walks into a bar a orders a <extra_id_1> with <extra_id_2> pinch of <extra_id_3>."
input_ids = tokenizer(text, return_tensors="pt").input_ids
out = model.generate(input_ids, decoder_start_token_id=0)
print(tokenizer.decode(out[0]))
| transformers/src/transformers/models/switch_transformers/convert_big_switch.py/0 | {
"file_path": "transformers/src/transformers/models/switch_transformers/convert_big_switch.py",
"repo_id": "transformers",
"token_count": 3229
} | 365 |
# coding=utf-8
# Copyright 2022 The HuggingFace Inc. team.
#
# Licensed under the Apache License, Version 2.0 (the "License");
# you may not use this file except in compliance with the License.
# You may obtain a copy of the License at
#
# http://www.apache.org/licenses/LICENSE-2.0
#
# Unless required by applicable law or agreed to in writing, software
# distributed under the License is distributed on an "AS IS" BASIS,
# WITHOUT WARRANTIES OR CONDITIONS OF ANY KIND, either express or implied.
# See the License for the specific language governing permissions and
# limitations under the License.
"""Convert Table Transformer checkpoints with timm-backbone.
URL: https://github.com/microsoft/table-transformer
"""
import argparse
from collections import OrderedDict
from pathlib import Path
import torch
from huggingface_hub import hf_hub_download
from PIL import Image
from torchvision.transforms import functional as F
from transformers import DetrImageProcessor, TableTransformerConfig, TableTransformerForObjectDetection
from transformers.utils import logging
logging.set_verbosity_info()
logger = logging.get_logger(__name__)
# here we list all keys to be renamed (original name on the left, our name on the right)
rename_keys = []
for i in range(6):
# encoder layers: output projection, 2 feedforward neural networks and 2 layernorms
rename_keys.append(
(f"transformer.encoder.layers.{i}.self_attn.out_proj.weight", f"encoder.layers.{i}.self_attn.out_proj.weight")
)
rename_keys.append(
(f"transformer.encoder.layers.{i}.self_attn.out_proj.bias", f"encoder.layers.{i}.self_attn.out_proj.bias")
)
rename_keys.append((f"transformer.encoder.layers.{i}.linear1.weight", f"encoder.layers.{i}.fc1.weight"))
rename_keys.append((f"transformer.encoder.layers.{i}.linear1.bias", f"encoder.layers.{i}.fc1.bias"))
rename_keys.append((f"transformer.encoder.layers.{i}.linear2.weight", f"encoder.layers.{i}.fc2.weight"))
rename_keys.append((f"transformer.encoder.layers.{i}.linear2.bias", f"encoder.layers.{i}.fc2.bias"))
rename_keys.append(
(f"transformer.encoder.layers.{i}.norm1.weight", f"encoder.layers.{i}.self_attn_layer_norm.weight")
)
rename_keys.append((f"transformer.encoder.layers.{i}.norm1.bias", f"encoder.layers.{i}.self_attn_layer_norm.bias"))
rename_keys.append((f"transformer.encoder.layers.{i}.norm2.weight", f"encoder.layers.{i}.final_layer_norm.weight"))
rename_keys.append((f"transformer.encoder.layers.{i}.norm2.bias", f"encoder.layers.{i}.final_layer_norm.bias"))
# decoder layers: 2 times output projection, 2 feedforward neural networks and 3 layernorms
rename_keys.append(
(f"transformer.decoder.layers.{i}.self_attn.out_proj.weight", f"decoder.layers.{i}.self_attn.out_proj.weight")
)
rename_keys.append(
(f"transformer.decoder.layers.{i}.self_attn.out_proj.bias", f"decoder.layers.{i}.self_attn.out_proj.bias")
)
rename_keys.append(
(
f"transformer.decoder.layers.{i}.multihead_attn.out_proj.weight",
f"decoder.layers.{i}.encoder_attn.out_proj.weight",
)
)
rename_keys.append(
(
f"transformer.decoder.layers.{i}.multihead_attn.out_proj.bias",
f"decoder.layers.{i}.encoder_attn.out_proj.bias",
)
)
rename_keys.append((f"transformer.decoder.layers.{i}.linear1.weight", f"decoder.layers.{i}.fc1.weight"))
rename_keys.append((f"transformer.decoder.layers.{i}.linear1.bias", f"decoder.layers.{i}.fc1.bias"))
rename_keys.append((f"transformer.decoder.layers.{i}.linear2.weight", f"decoder.layers.{i}.fc2.weight"))
rename_keys.append((f"transformer.decoder.layers.{i}.linear2.bias", f"decoder.layers.{i}.fc2.bias"))
rename_keys.append(
(f"transformer.decoder.layers.{i}.norm1.weight", f"decoder.layers.{i}.self_attn_layer_norm.weight")
)
rename_keys.append((f"transformer.decoder.layers.{i}.norm1.bias", f"decoder.layers.{i}.self_attn_layer_norm.bias"))
rename_keys.append(
(f"transformer.decoder.layers.{i}.norm2.weight", f"decoder.layers.{i}.encoder_attn_layer_norm.weight")
)
rename_keys.append(
(f"transformer.decoder.layers.{i}.norm2.bias", f"decoder.layers.{i}.encoder_attn_layer_norm.bias")
)
rename_keys.append((f"transformer.decoder.layers.{i}.norm3.weight", f"decoder.layers.{i}.final_layer_norm.weight"))
rename_keys.append((f"transformer.decoder.layers.{i}.norm3.bias", f"decoder.layers.{i}.final_layer_norm.bias"))
# convolutional projection + query embeddings + layernorm of encoder + layernorm of decoder + class and bounding box heads
rename_keys.extend(
[
("input_proj.weight", "input_projection.weight"),
("input_proj.bias", "input_projection.bias"),
("query_embed.weight", "query_position_embeddings.weight"),
("transformer.encoder.norm.weight", "encoder.layernorm.weight"),
("transformer.encoder.norm.bias", "encoder.layernorm.bias"),
("transformer.decoder.norm.weight", "decoder.layernorm.weight"),
("transformer.decoder.norm.bias", "decoder.layernorm.bias"),
("class_embed.weight", "class_labels_classifier.weight"),
("class_embed.bias", "class_labels_classifier.bias"),
("bbox_embed.layers.0.weight", "bbox_predictor.layers.0.weight"),
("bbox_embed.layers.0.bias", "bbox_predictor.layers.0.bias"),
("bbox_embed.layers.1.weight", "bbox_predictor.layers.1.weight"),
("bbox_embed.layers.1.bias", "bbox_predictor.layers.1.bias"),
("bbox_embed.layers.2.weight", "bbox_predictor.layers.2.weight"),
("bbox_embed.layers.2.bias", "bbox_predictor.layers.2.bias"),
]
)
def rename_key(state_dict, old, new):
val = state_dict.pop(old)
state_dict[new] = val
def rename_backbone_keys(state_dict):
new_state_dict = OrderedDict()
for key, value in state_dict.items():
if "backbone.0.body" in key:
new_key = key.replace("backbone.0.body", "backbone.conv_encoder.model")
new_state_dict[new_key] = value
else:
new_state_dict[key] = value
return new_state_dict
def read_in_q_k_v(state_dict):
prefix = ""
# first: transformer encoder
for i in range(6):
# read in weights + bias of input projection layer (in PyTorch's MultiHeadAttention, this is a single matrix + bias)
in_proj_weight = state_dict.pop(f"{prefix}transformer.encoder.layers.{i}.self_attn.in_proj_weight")
in_proj_bias = state_dict.pop(f"{prefix}transformer.encoder.layers.{i}.self_attn.in_proj_bias")
# next, add query, keys and values (in that order) to the state dict
state_dict[f"encoder.layers.{i}.self_attn.q_proj.weight"] = in_proj_weight[:256, :]
state_dict[f"encoder.layers.{i}.self_attn.q_proj.bias"] = in_proj_bias[:256]
state_dict[f"encoder.layers.{i}.self_attn.k_proj.weight"] = in_proj_weight[256:512, :]
state_dict[f"encoder.layers.{i}.self_attn.k_proj.bias"] = in_proj_bias[256:512]
state_dict[f"encoder.layers.{i}.self_attn.v_proj.weight"] = in_proj_weight[-256:, :]
state_dict[f"encoder.layers.{i}.self_attn.v_proj.bias"] = in_proj_bias[-256:]
# next: transformer decoder (which is a bit more complex because it also includes cross-attention)
for i in range(6):
# read in weights + bias of input projection layer of self-attention
in_proj_weight = state_dict.pop(f"{prefix}transformer.decoder.layers.{i}.self_attn.in_proj_weight")
in_proj_bias = state_dict.pop(f"{prefix}transformer.decoder.layers.{i}.self_attn.in_proj_bias")
# next, add query, keys and values (in that order) to the state dict
state_dict[f"decoder.layers.{i}.self_attn.q_proj.weight"] = in_proj_weight[:256, :]
state_dict[f"decoder.layers.{i}.self_attn.q_proj.bias"] = in_proj_bias[:256]
state_dict[f"decoder.layers.{i}.self_attn.k_proj.weight"] = in_proj_weight[256:512, :]
state_dict[f"decoder.layers.{i}.self_attn.k_proj.bias"] = in_proj_bias[256:512]
state_dict[f"decoder.layers.{i}.self_attn.v_proj.weight"] = in_proj_weight[-256:, :]
state_dict[f"decoder.layers.{i}.self_attn.v_proj.bias"] = in_proj_bias[-256:]
# read in weights + bias of input projection layer of cross-attention
in_proj_weight_cross_attn = state_dict.pop(
f"{prefix}transformer.decoder.layers.{i}.multihead_attn.in_proj_weight"
)
in_proj_bias_cross_attn = state_dict.pop(f"{prefix}transformer.decoder.layers.{i}.multihead_attn.in_proj_bias")
# next, add query, keys and values (in that order) of cross-attention to the state dict
state_dict[f"decoder.layers.{i}.encoder_attn.q_proj.weight"] = in_proj_weight_cross_attn[:256, :]
state_dict[f"decoder.layers.{i}.encoder_attn.q_proj.bias"] = in_proj_bias_cross_attn[:256]
state_dict[f"decoder.layers.{i}.encoder_attn.k_proj.weight"] = in_proj_weight_cross_attn[256:512, :]
state_dict[f"decoder.layers.{i}.encoder_attn.k_proj.bias"] = in_proj_bias_cross_attn[256:512]
state_dict[f"decoder.layers.{i}.encoder_attn.v_proj.weight"] = in_proj_weight_cross_attn[-256:, :]
state_dict[f"decoder.layers.{i}.encoder_attn.v_proj.bias"] = in_proj_bias_cross_attn[-256:]
def resize(image, checkpoint_url):
width, height = image.size
current_max_size = max(width, height)
target_max_size = 800 if "detection" in checkpoint_url else 1000
scale = target_max_size / current_max_size
resized_image = image.resize((int(round(scale * width)), int(round(scale * height))))
return resized_image
def normalize(image):
image = F.to_tensor(image)
image = F.normalize(image, mean=[0.485, 0.456, 0.406], std=[0.229, 0.224, 0.225])
return image
@torch.no_grad()
def convert_table_transformer_checkpoint(checkpoint_url, pytorch_dump_folder_path, push_to_hub):
"""
Copy/paste/tweak model's weights to our DETR structure.
"""
logger.info("Converting model...")
# load original state dict
state_dict = torch.hub.load_state_dict_from_url(checkpoint_url, map_location="cpu")
# rename keys
for src, dest in rename_keys:
rename_key(state_dict, src, dest)
state_dict = rename_backbone_keys(state_dict)
# query, key and value matrices need special treatment
read_in_q_k_v(state_dict)
# important: we need to prepend a prefix to each of the base model keys as the head models use different attributes for them
prefix = "model."
for key in state_dict.copy().keys():
if not key.startswith("class_labels_classifier") and not key.startswith("bbox_predictor"):
val = state_dict.pop(key)
state_dict[prefix + key] = val
# create HuggingFace model and load state dict
config = TableTransformerConfig(
backbone="resnet18",
mask_loss_coefficient=1,
dice_loss_coefficient=1,
ce_loss_coefficient=1,
bbox_loss_coefficient=5,
giou_loss_coefficient=2,
eos_coefficient=0.4,
class_cost=1,
bbox_cost=5,
giou_cost=2,
)
if "detection" in checkpoint_url:
config.num_queries = 15
config.num_labels = 2
id2label = {0: "table", 1: "table rotated"}
config.id2label = id2label
config.label2id = {v: k for k, v in id2label.items()}
else:
config.num_queries = 125
config.num_labels = 6
id2label = {
0: "table",
1: "table column",
2: "table row",
3: "table column header",
4: "table projected row header",
5: "table spanning cell",
}
config.id2label = id2label
config.label2id = {v: k for k, v in id2label.items()}
image_processor = DetrImageProcessor(
format="coco_detection", max_size=800 if "detection" in checkpoint_url else 1000
)
model = TableTransformerForObjectDetection(config)
model.load_state_dict(state_dict)
model.eval()
# verify our conversion
filename = "example_pdf.png" if "detection" in checkpoint_url else "example_table.png"
file_path = hf_hub_download(repo_id="nielsr/example-pdf", repo_type="dataset", filename=filename)
image = Image.open(file_path).convert("RGB")
pixel_values = normalize(resize(image, checkpoint_url)).unsqueeze(0)
outputs = model(pixel_values)
if "detection" in checkpoint_url:
expected_shape = (1, 15, 3)
expected_logits = torch.tensor(
[[-6.7897, -16.9985, 6.7937], [-8.0186, -22.2192, 6.9677], [-7.3117, -21.0708, 7.4055]]
)
expected_boxes = torch.tensor([[0.4867, 0.1767, 0.6732], [0.6718, 0.4479, 0.3830], [0.4716, 0.1760, 0.6364]])
else:
expected_shape = (1, 125, 7)
expected_logits = torch.tensor(
[[-18.1430, -8.3214, 4.8274], [-18.4685, -7.1361, -4.2667], [-26.3693, -9.3429, -4.9962]]
)
expected_boxes = torch.tensor([[0.4983, 0.5595, 0.9440], [0.4916, 0.6315, 0.5954], [0.6108, 0.8637, 0.1135]])
assert outputs.logits.shape == expected_shape
assert torch.allclose(outputs.logits[0, :3, :3], expected_logits, atol=1e-4)
assert torch.allclose(outputs.pred_boxes[0, :3, :3], expected_boxes, atol=1e-4)
print("Looks ok!")
if pytorch_dump_folder_path is not None:
# Save model and image processor
logger.info(f"Saving PyTorch model and image processor to {pytorch_dump_folder_path}...")
Path(pytorch_dump_folder_path).mkdir(exist_ok=True)
model.save_pretrained(pytorch_dump_folder_path)
image_processor.save_pretrained(pytorch_dump_folder_path)
if push_to_hub:
# Push model to HF hub
logger.info("Pushing model to the hub...")
model_name = (
"microsoft/table-transformer-detection"
if "detection" in checkpoint_url
else "microsoft/table-transformer-structure-recognition"
)
model.push_to_hub(model_name)
image_processor.push_to_hub(model_name)
if __name__ == "__main__":
parser = argparse.ArgumentParser()
parser.add_argument(
"--checkpoint_url",
default="https://pubtables1m.blob.core.windows.net/model/pubtables1m_detection_detr_r18.pth",
type=str,
choices=[
"https://pubtables1m.blob.core.windows.net/model/pubtables1m_detection_detr_r18.pth",
"https://pubtables1m.blob.core.windows.net/model/pubtables1m_structure_detr_r18.pth",
],
help="URL of the Table Transformer checkpoint you'd like to convert.",
)
parser.add_argument(
"--pytorch_dump_folder_path", default=None, type=str, help="Path to the folder to output PyTorch model."
)
parser.add_argument(
"--push_to_hub", action="store_true", help="Whether or not to push the converted model to the ๐ค hub."
)
args = parser.parse_args()
convert_table_transformer_checkpoint(args.checkpoint_url, args.pytorch_dump_folder_path, args.push_to_hub)
| transformers/src/transformers/models/table_transformer/convert_table_transformer_to_hf.py/0 | {
"file_path": "transformers/src/transformers/models/table_transformer/convert_table_transformer_to_hf.py",
"repo_id": "transformers",
"token_count": 6591
} | 366 |
# coding=utf-8
# Copyright 2023 The Intel AIA Team Authors, and HuggingFace Inc. team. All rights reserved.
#
# Licensed under the Apache License=, Version 2.0 (the "License");
# you may not use this file except in compliance with the License.
# You may obtain a copy of the License at
#
# http://www.apache.org/licenses/LICENSE-2.0
#
# Unless required by applicable law or agreed to in writing=, software
# distributed under the License is distributed on an "AS IS" BASIS=,
# WITHOUT WARRANTIES OR CONDITIONS OF ANY KIND=, either express or implied.
# See the License for the specific language governing permissions and
# limitations under the License.
"""Image processor class for TVP."""
from typing import Dict, Iterable, List, Optional, Tuple, Union
import numpy as np
from ...image_processing_utils import BaseImageProcessor, BatchFeature, get_size_dict
from ...image_transforms import (
PaddingMode,
flip_channel_order,
pad,
resize,
to_channel_dimension_format,
)
from ...image_utils import (
IMAGENET_STANDARD_MEAN,
IMAGENET_STANDARD_STD,
ChannelDimension,
ImageInput,
PILImageResampling,
get_image_size,
is_valid_image,
to_numpy_array,
valid_images,
)
from ...utils import TensorType, is_vision_available, logging
if is_vision_available():
import PIL
logger = logging.get_logger(__name__)
# Copied from transformers.models.vivit.image_processing_vivit.make_batched
def make_batched(videos) -> List[List[ImageInput]]:
if isinstance(videos, (list, tuple)) and isinstance(videos[0], (list, tuple)) and is_valid_image(videos[0][0]):
return videos
elif isinstance(videos, (list, tuple)) and is_valid_image(videos[0]):
return [videos]
elif is_valid_image(videos):
return [[videos]]
raise ValueError(f"Could not make batched video from {videos}")
def get_resize_output_image_size(
input_image: np.ndarray,
max_size: int = 448,
input_data_format: Optional[Union[str, ChannelDimension]] = None,
) -> Tuple[int, int]:
height, width = get_image_size(input_image, input_data_format)
if height >= width:
ratio = width * 1.0 / height
new_height = max_size
new_width = new_height * ratio
else:
ratio = height * 1.0 / width
new_width = max_size
new_height = new_width * ratio
size = (int(new_height), int(new_width))
return size
class TvpImageProcessor(BaseImageProcessor):
r"""
Constructs a Tvp image processor.
Args:
do_resize (`bool`, *optional*, defaults to `True`):
Whether to resize the image's (height, width) dimensions to the specified `size`. Can be overridden by the
`do_resize` parameter in the `preprocess` method.
size (`Dict[str, int]` *optional*, defaults to `{"longest_edge": 448}`):
Size of the output image after resizing. The longest edge of the image will be resized to
`size["longest_edge"]` while maintaining the aspect ratio of the original image. Can be overriden by
`size` in the `preprocess` method.
resample (`PILImageResampling`, *optional*, defaults to `Resampling.BILINEAR`):
Resampling filter to use if resizing the image. Can be overridden by the `resample` parameter in the
`preprocess` method.
do_center_crop (`bool`, *optional*, defaults to `True`):
Whether to center crop the image to the specified `crop_size`. Can be overridden by the `do_center_crop`
parameter in the `preprocess` method.
crop_size (`Dict[str, int]`, *optional*, defaults to `{"height": 448, "width": 448}`):
Size of the image after applying the center crop. Can be overridden by the `crop_size` parameter in the
`preprocess` method.
do_rescale (`bool`, *optional*, defaults to `True`):
Whether to rescale the image by the specified scale `rescale_factor`. Can be overridden by the `do_rescale`
parameter in the `preprocess` method.
rescale_factor (`int` or `float`, *optional*, defaults to `1/255`):
Defines the scale factor to use if rescaling the image. Can be overridden by the `rescale_factor` parameter
in the `preprocess` method.
do_pad (`bool`, *optional*, defaults to `True`):
Whether to pad the image. Can be overridden by the `do_pad` parameter in the `preprocess` method.
pad_size (`Dict[str, int]`, *optional*, defaults to `{"height": 448, "width": 448}`):
Size of the image after applying the padding. Can be overridden by the `pad_size` parameter in the
`preprocess` method.
constant_values (`Union[float, Iterable[float]]`, *optional*, defaults to 0):
The fill value to use when padding the image.
pad_mode (`PaddingMode`, *optional*, defaults to `PaddingMode.CONSTANT`):
Use what kind of mode in padding.
do_normalize (`bool`, *optional*, defaults to `True`):
Whether to normalize the image. Can be overridden by the `do_normalize` parameter in the `preprocess`
method.
do_flip_channel_order (`bool`, *optional*, defaults to `True`):
Whether to flip the color channels from RGB to BGR. Can be overridden by the `do_flip_channel_order`
parameter in the `preprocess` method.
image_mean (`float` or `List[float]`, *optional*, defaults to `IMAGENET_STANDARD_MEAN`):
Mean to use if normalizing the image. This is a float or list of floats the length of the number of
channels in the image. Can be overridden by the `image_mean` parameter in the `preprocess` method.
image_std (`float` or `List[float]`, *optional*, defaults to `IMAGENET_STANDARD_STD`):
Standard deviation to use if normalizing the image. This is a float or list of floats the length of the
number of channels in the image. Can be overridden by the `image_std` parameter in the `preprocess` method.
"""
model_input_names = ["pixel_values"]
def __init__(
self,
do_resize: bool = True,
size: Dict[str, int] = None,
resample: PILImageResampling = PILImageResampling.BILINEAR,
do_center_crop: bool = True,
crop_size: Dict[str, int] = None,
do_rescale: bool = True,
rescale_factor: Union[int, float] = 1 / 255,
do_pad: bool = True,
pad_size: Dict[str, int] = None,
constant_values: Union[float, Iterable[float]] = 0,
pad_mode: PaddingMode = PaddingMode.CONSTANT,
do_normalize: bool = True,
do_flip_channel_order: bool = True,
image_mean: Optional[Union[float, List[float]]] = None,
image_std: Optional[Union[float, List[float]]] = None,
**kwargs,
) -> None:
super().__init__(**kwargs)
size = size if size is not None else {"longest_edge": 448}
crop_size = crop_size if crop_size is not None else {"height": 448, "width": 448}
pad_size = pad_size if pad_size is not None else {"height": 448, "width": 448}
self.do_resize = do_resize
self.size = size
self.do_center_crop = do_center_crop
self.crop_size = crop_size
self.resample = resample
self.do_rescale = do_rescale
self.rescale_factor = rescale_factor
self.do_pad = do_pad
self.pad_size = pad_size
self.constant_values = constant_values
self.pad_mode = pad_mode
self.do_normalize = do_normalize
self.do_flip_channel_order = do_flip_channel_order
self.image_mean = image_mean if image_mean is not None else IMAGENET_STANDARD_MEAN
self.image_std = image_std if image_std is not None else IMAGENET_STANDARD_STD
def resize(
self,
image: np.ndarray,
size: Dict[str, int],
resample: PILImageResampling = PILImageResampling.BILINEAR,
data_format: Optional[Union[str, ChannelDimension]] = None,
input_data_format: Optional[Union[str, ChannelDimension]] = None,
**kwargs,
) -> np.ndarray:
"""
Resize an image.
Args:
image (`np.ndarray`):
Image to resize.
size (`Dict[str, int]`):
Size of the output image. If `size` is of the form `{"height": h, "width": w}`, the output image will
have the size `(h, w)`. If `size` is of the form `{"longest_edge": s}`, the output image will have its
longest edge of length `s` while keeping the aspect ratio of the original image.
resample (`PILImageResampling`, *optional*, defaults to `PILImageResampling.BILINEAR`):
Resampling filter to use when resiizing the image.
data_format (`str` or `ChannelDimension`, *optional*):
The channel dimension format of the image. If not provided, it will be the same as the input image.
input_data_format (`str` or `ChannelDimension`, *optional*):
The channel dimension format of the input image. If not provided, it will be inferred.
"""
size = get_size_dict(size, default_to_square=False)
if "height" in size and "width" in size:
output_size = (size["height"], size["width"])
elif "longest_edge" in size:
output_size = get_resize_output_image_size(image, size["longest_edge"], input_data_format)
else:
raise ValueError(f"Size must have 'height' and 'width' or 'longest_edge' as keys. Got {size.keys()}")
return resize(
image,
size=output_size,
resample=resample,
data_format=data_format,
input_data_format=input_data_format,
**kwargs,
)
def pad_image(
self,
image: np.ndarray,
pad_size: Dict[str, int] = None,
constant_values: Union[float, Iterable[float]] = 0,
pad_mode: PaddingMode = PaddingMode.CONSTANT,
data_format: Optional[Union[str, ChannelDimension]] = None,
input_data_format: Optional[Union[str, ChannelDimension]] = None,
**kwargs,
):
"""
Pad an image with zeros to the given size.
Args:
image (`np.ndarray`):
Image to pad.
pad_size (`Dict[str, int]`)
Size of the output image with pad.
constant_values (`Union[float, Iterable[float]]`)
The fill value to use when padding the image.
pad_mode (`PaddingMode`)
The pad mode, default to PaddingMode.CONSTANT
data_format (`ChannelDimension` or `str`, *optional*)
The channel dimension format of the image. If not provided, it will be the same as the input image.
input_data_format (`ChannelDimension` or `str`, *optional*):
The channel dimension format of the input image. If not provided, it will be inferred.
"""
height, width = get_image_size(image, channel_dim=input_data_format)
max_height = pad_size.get("height", height)
max_width = pad_size.get("width", width)
pad_right, pad_bottom = max_width - width, max_height - height
if pad_right < 0 or pad_bottom < 0:
raise ValueError("The padding size must be greater than image size")
padding = ((0, pad_bottom), (0, pad_right))
padded_image = pad(
image,
padding,
mode=pad_mode,
constant_values=constant_values,
data_format=data_format,
input_data_format=input_data_format,
)
return padded_image
def _preprocess_image(
self,
image: ImageInput,
do_resize: bool = None,
size: Dict[str, int] = None,
resample: PILImageResampling = None,
do_center_crop: bool = None,
crop_size: Dict[str, int] = None,
do_rescale: bool = None,
rescale_factor: float = None,
do_pad: bool = True,
pad_size: Dict[str, int] = None,
constant_values: Union[float, Iterable[float]] = None,
pad_mode: PaddingMode = None,
do_normalize: bool = None,
do_flip_channel_order: bool = None,
image_mean: Optional[Union[float, List[float]]] = None,
image_std: Optional[Union[float, List[float]]] = None,
data_format: Optional[ChannelDimension] = ChannelDimension.FIRST,
input_data_format: Optional[Union[str, ChannelDimension]] = None,
**kwargs,
) -> np.ndarray:
"""Preprocesses a single image."""
if do_resize and size is None or resample is None:
raise ValueError("Size and resample must be specified if do_resize is True.")
if do_center_crop and crop_size is None:
raise ValueError("Crop size must be specified if do_center_crop is True.")
if do_rescale and rescale_factor is None:
raise ValueError("Rescale factor must be specified if do_rescale is True.")
if do_pad and pad_size is None:
raise ValueError("Padding size must be specified if do_pad is True.")
if do_normalize and (image_mean is None or image_std is None):
raise ValueError("Image mean and std must be specified if do_normalize is True.")
# All transformations expect numpy arrays.
image = to_numpy_array(image)
if do_resize:
image = self.resize(image=image, size=size, resample=resample, input_data_format=input_data_format)
if do_center_crop:
image = self.center_crop(image, size=crop_size, input_data_format=input_data_format)
if do_rescale:
image = self.rescale(image=image, scale=rescale_factor, input_data_format=input_data_format)
if do_normalize:
image = self.normalize(
image=image.astype(np.float32), mean=image_mean, std=image_std, input_data_format=input_data_format
)
if do_pad:
image = self.pad_image(
image=image,
pad_size=pad_size,
constant_values=constant_values,
pad_mode=pad_mode,
input_data_format=input_data_format,
)
# the pretrained checkpoints assume images are BGR, not RGB
if do_flip_channel_order:
image = flip_channel_order(image=image, input_data_format=input_data_format)
image = to_channel_dimension_format(image, data_format, input_channel_dim=input_data_format)
return image
def preprocess(
self,
videos: Union[ImageInput, List[ImageInput], List[List[ImageInput]]],
do_resize: bool = None,
size: Dict[str, int] = None,
resample: PILImageResampling = None,
do_center_crop: bool = None,
crop_size: Dict[str, int] = None,
do_rescale: bool = None,
rescale_factor: float = None,
do_pad: bool = None,
pad_size: Dict[str, int] = None,
constant_values: Union[float, Iterable[float]] = None,
pad_mode: PaddingMode = None,
do_normalize: bool = None,
do_flip_channel_order: bool = None,
image_mean: Optional[Union[float, List[float]]] = None,
image_std: Optional[Union[float, List[float]]] = None,
return_tensors: Optional[Union[str, TensorType]] = None,
data_format: ChannelDimension = ChannelDimension.FIRST,
input_data_format: Optional[Union[str, ChannelDimension]] = None,
**kwargs,
) -> PIL.Image.Image:
"""
Preprocess an image or batch of images.
Args:
videos (`ImageInput` or `List[ImageInput]` or `List[List[ImageInput]]`):
Frames to preprocess.
do_resize (`bool`, *optional*, defaults to `self.do_resize`):
Whether to resize the image.
size (`Dict[str, int]`, *optional*, defaults to `self.size`):
Size of the image after applying resize.
resample (`PILImageResampling`, *optional*, defaults to `self.resample`):
Resampling filter to use if resizing the image. This can be one of the enum `PILImageResampling`, Only
has an effect if `do_resize` is set to `True`.
do_center_crop (`bool`, *optional*, defaults to `self.do_centre_crop`):
Whether to centre crop the image.
crop_size (`Dict[str, int]`, *optional*, defaults to `self.crop_size`):
Size of the image after applying the centre crop.
do_rescale (`bool`, *optional*, defaults to `self.do_rescale`):
Whether to rescale the image values between [0 - 1].
rescale_factor (`float`, *optional*, defaults to `self.rescale_factor`):
Rescale factor to rescale the image by if `do_rescale` is set to `True`.
do_pad (`bool`, *optional*, defaults to `True`):
Whether to pad the image. Can be overridden by the `do_pad` parameter in the `preprocess` method.
pad_size (`Dict[str, int]`, *optional*, defaults to `{"height": 448, "width": 448}`):
Size of the image after applying the padding. Can be overridden by the `pad_size` parameter in the
`preprocess` method.
constant_values (`Union[float, Iterable[float]]`, *optional*, defaults to 0):
The fill value to use when padding the image.
pad_mode (`PaddingMode`, *optional*, defaults to "PaddingMode.CONSTANT"):
Use what kind of mode in padding.
do_normalize (`bool`, *optional*, defaults to `self.do_normalize`):
Whether to normalize the image.
do_flip_channel_order (`bool`, *optional*, defaults to `self.do_flip_channel_order`):
Whether to flip the channel order of the image.
image_mean (`float` or `List[float]`, *optional*, defaults to `self.image_mean`):
Image mean.
image_std (`float` or `List[float]`, *optional*, defaults to `self.image_std`):
Image standard deviation.
return_tensors (`str` or `TensorType`, *optional*):
The type of tensors to return. Can be one of:
- Unset: Return a list of `np.ndarray`.
- `TensorType.TENSORFLOW` or `'tf'`: Return a batch of type `tf.Tensor`.
- `TensorType.PYTORCH` or `'pt'`: Return a batch of type `torch.Tensor`.
- `TensorType.NUMPY` or `'np'`: Return a batch of type `np.ndarray`.
- `TensorType.JAX` or `'jax'`: Return a batch of type `jax.numpy.ndarray`.
data_format (`ChannelDimension` or `str`, *optional*, defaults to `ChannelDimension.FIRST`):
The channel dimension format for the output image. Can be one of:
- `ChannelDimension.FIRST`: image in (num_channels, height, width) format.
- `ChannelDimension.LAST`: image in (height, width, num_channels) format.
- Unset: Use the inferred channel dimension format of the input image.
input_data_format (`ChannelDimension` or `str`, *optional*):
The channel dimension format for the input image. If unset, the channel dimension format is inferred
from the input image. Can be one of:
- `"channels_first"` or `ChannelDimension.FIRST`: image in (num_channels, height, width) format.
- `"channels_last"` or `ChannelDimension.LAST`: image in (height, width, num_channels) format.
- `"none"` or `ChannelDimension.NONE`: image in (height, width) format.
"""
do_resize = do_resize if do_resize is not None else self.do_resize
resample = resample if resample is not None else self.resample
do_center_crop = do_center_crop if do_center_crop is not None else self.do_center_crop
do_rescale = do_rescale if do_rescale is not None else self.do_rescale
rescale_factor = rescale_factor if rescale_factor is not None else self.rescale_factor
do_pad = do_pad if do_pad is not None else self.do_pad
pad_size = pad_size if pad_size is not None else self.pad_size
constant_values = constant_values if constant_values is not None else self.constant_values
pad_mode = pad_mode if pad_mode else self.pad_mode
do_normalize = do_normalize if do_normalize is not None else self.do_normalize
do_flip_channel_order = (
do_flip_channel_order if do_flip_channel_order is not None else self.do_flip_channel_order
)
image_mean = image_mean if image_mean is not None else self.image_mean
image_std = image_std if image_std is not None else self.image_std
size = size if size is not None else self.size
size = get_size_dict(size, default_to_square=False)
crop_size = crop_size if crop_size is not None else self.crop_size
crop_size = get_size_dict(crop_size, param_name="crop_size")
if not valid_images(videos):
raise ValueError(
"Invalid image type. Must be of type PIL.Image.Image, numpy.ndarray, "
"torch.Tensor, tf.Tensor or jax.ndarray."
)
videos = make_batched(videos)
videos = [
np.array(
[
self._preprocess_image(
image=img,
do_resize=do_resize,
size=size,
resample=resample,
do_center_crop=do_center_crop,
crop_size=crop_size,
do_rescale=do_rescale,
rescale_factor=rescale_factor,
do_pad=do_pad,
pad_size=pad_size,
constant_values=constant_values,
pad_mode=pad_mode,
do_normalize=do_normalize,
do_flip_channel_order=do_flip_channel_order,
image_mean=image_mean,
image_std=image_std,
data_format=data_format,
input_data_format=input_data_format,
)
for img in video
]
)
for video in videos
]
data = {"pixel_values": videos}
return BatchFeature(data=data, tensor_type=return_tensors)
| transformers/src/transformers/models/tvp/image_processing_tvp.py/0 | {
"file_path": "transformers/src/transformers/models/tvp/image_processing_tvp.py",
"repo_id": "transformers",
"token_count": 9831
} | 367 |
# Copyright 2022 The HuggingFace Team. All rights reserved.
#
# Licensed under the Apache License, Version 2.0 (the "License");
# you may not use this file except in compliance with the License.
# You may obtain a copy of the License at
#
# http://www.apache.org/licenses/LICENSE-2.0
#
# Unless required by applicable law or agreed to in writing, software
# distributed under the License is distributed on an "AS IS" BASIS,
# WITHOUT WARRANTIES OR CONDITIONS OF ANY KIND, either express or implied.
# See the License for the specific language governing permissions and
# limitations under the License.
from typing import TYPE_CHECKING
from ...utils import OptionalDependencyNotAvailable, _LazyModule, is_torch_available, is_vision_available
_import_structure = {"configuration_vilt": ["VILT_PRETRAINED_CONFIG_ARCHIVE_MAP", "ViltConfig"]}
try:
if not is_vision_available():
raise OptionalDependencyNotAvailable()
except OptionalDependencyNotAvailable:
pass
else:
_import_structure["feature_extraction_vilt"] = ["ViltFeatureExtractor"]
_import_structure["image_processing_vilt"] = ["ViltImageProcessor"]
_import_structure["processing_vilt"] = ["ViltProcessor"]
try:
if not is_torch_available():
raise OptionalDependencyNotAvailable()
except OptionalDependencyNotAvailable:
pass
else:
_import_structure["modeling_vilt"] = [
"VILT_PRETRAINED_MODEL_ARCHIVE_LIST",
"ViltForImageAndTextRetrieval",
"ViltForImagesAndTextClassification",
"ViltForTokenClassification",
"ViltForMaskedLM",
"ViltForQuestionAnswering",
"ViltLayer",
"ViltModel",
"ViltPreTrainedModel",
]
if TYPE_CHECKING:
from .configuration_vilt import VILT_PRETRAINED_CONFIG_ARCHIVE_MAP, ViltConfig
try:
if not is_vision_available():
raise OptionalDependencyNotAvailable()
except OptionalDependencyNotAvailable:
pass
else:
from .feature_extraction_vilt import ViltFeatureExtractor
from .image_processing_vilt import ViltImageProcessor
from .processing_vilt import ViltProcessor
try:
if not is_torch_available():
raise OptionalDependencyNotAvailable()
except OptionalDependencyNotAvailable:
pass
else:
from .modeling_vilt import (
VILT_PRETRAINED_MODEL_ARCHIVE_LIST,
ViltForImageAndTextRetrieval,
ViltForImagesAndTextClassification,
ViltForMaskedLM,
ViltForQuestionAnswering,
ViltForTokenClassification,
ViltLayer,
ViltModel,
ViltPreTrainedModel,
)
else:
import sys
sys.modules[__name__] = _LazyModule(__name__, globals()["__file__"], _import_structure)
| transformers/src/transformers/models/vilt/__init__.py/0 | {
"file_path": "transformers/src/transformers/models/vilt/__init__.py",
"repo_id": "transformers",
"token_count": 1082
} | 368 |
# Copyright 2021 The HuggingFace Team. All rights reserved.
#
# Licensed under the Apache License, Version 2.0 (the "License");
# you may not use this file except in compliance with the License.
# You may obtain a copy of the License at
#
# http://www.apache.org/licenses/LICENSE-2.0
#
# Unless required by applicable law or agreed to in writing, software
# distributed under the License is distributed on an "AS IS" BASIS,
# WITHOUT WARRANTIES OR CONDITIONS OF ANY KIND, either express or implied.
# See the License for the specific language governing permissions and
# limitations under the License.
from typing import TYPE_CHECKING
from ...utils import (
OptionalDependencyNotAvailable,
_LazyModule,
is_flax_available,
is_tf_available,
is_torch_available,
)
_import_structure = {
"configuration_vision_text_dual_encoder": ["VisionTextDualEncoderConfig"],
"processing_vision_text_dual_encoder": ["VisionTextDualEncoderProcessor"],
}
try:
if not is_torch_available():
raise OptionalDependencyNotAvailable()
except OptionalDependencyNotAvailable:
pass
else:
_import_structure["modeling_vision_text_dual_encoder"] = ["VisionTextDualEncoderModel"]
try:
if not is_flax_available():
raise OptionalDependencyNotAvailable()
except OptionalDependencyNotAvailable:
pass
else:
_import_structure["modeling_flax_vision_text_dual_encoder"] = ["FlaxVisionTextDualEncoderModel"]
try:
if not is_tf_available():
raise OptionalDependencyNotAvailable()
except OptionalDependencyNotAvailable:
pass
else:
_import_structure["modeling_tf_vision_text_dual_encoder"] = ["TFVisionTextDualEncoderModel"]
if TYPE_CHECKING:
from .configuration_vision_text_dual_encoder import VisionTextDualEncoderConfig
from .processing_vision_text_dual_encoder import VisionTextDualEncoderProcessor
try:
if not is_torch_available():
raise OptionalDependencyNotAvailable()
except OptionalDependencyNotAvailable:
pass
else:
from .modeling_vision_text_dual_encoder import VisionTextDualEncoderModel
try:
if not is_flax_available():
raise OptionalDependencyNotAvailable()
except OptionalDependencyNotAvailable:
pass
else:
from .modeling_flax_vision_text_dual_encoder import FlaxVisionTextDualEncoderModel
try:
if not is_tf_available():
raise OptionalDependencyNotAvailable()
except OptionalDependencyNotAvailable:
pass
else:
from .modeling_tf_vision_text_dual_encoder import TFVisionTextDualEncoderModel
else:
import sys
sys.modules[__name__] = _LazyModule(__name__, globals()["__file__"], _import_structure)
| transformers/src/transformers/models/vision_text_dual_encoder/__init__.py/0 | {
"file_path": "transformers/src/transformers/models/vision_text_dual_encoder/__init__.py",
"repo_id": "transformers",
"token_count": 949
} | 369 |
# coding=utf-8
# Copyright 2021 The Google Flax Team Authors and The HuggingFace Inc. team.
#
# Licensed under the Apache License, Version 2.0 (the "License");
# you may not use this file except in compliance with the License.
# You may obtain a copy of the License at
#
# http://www.apache.org/licenses/LICENSE-2.0
#
# Unless required by applicable law or agreed to in writing, software
# distributed under the License is distributed on an "AS IS" BASIS,
# WITHOUT WARRANTIES OR CONDITIONS OF ANY KIND, either express or implied.
# See the License for the specific language governing permissions and
# limitations under the License.
from typing import Optional, Tuple
import flax.linen as nn
import jax
import jax.numpy as jnp
from flax.core.frozen_dict import FrozenDict, freeze, unfreeze
from flax.linen.attention import dot_product_attention_weights
from flax.traverse_util import flatten_dict, unflatten_dict
from ...modeling_flax_outputs import FlaxBaseModelOutput, FlaxBaseModelOutputWithPooling, FlaxSequenceClassifierOutput
from ...modeling_flax_utils import (
ACT2FN,
FlaxPreTrainedModel,
append_replace_return_docstrings,
overwrite_call_docstring,
)
from ...utils import add_start_docstrings, add_start_docstrings_to_model_forward
from .configuration_vit import ViTConfig
VIT_START_DOCSTRING = r"""
This model inherits from [`FlaxPreTrainedModel`]. Check the superclass documentation for the generic methods the
library implements for all its model (such as downloading, saving and converting weights from PyTorch models)
This model is also a
[flax.linen.Module](https://flax.readthedocs.io/en/latest/api_reference/flax.linen/module.html) subclass. Use it as
a regular Flax linen Module and refer to the Flax documentation for all matter related to general usage and
behavior.
Finally, this model supports inherent JAX features such as:
- [Just-In-Time (JIT) compilation](https://jax.readthedocs.io/en/latest/jax.html#just-in-time-compilation-jit)
- [Automatic Differentiation](https://jax.readthedocs.io/en/latest/jax.html#automatic-differentiation)
- [Vectorization](https://jax.readthedocs.io/en/latest/jax.html#vectorization-vmap)
- [Parallelization](https://jax.readthedocs.io/en/latest/jax.html#parallelization-pmap)
Parameters:
config ([`ViTConfig`]): Model configuration class with all the parameters of the model.
Initializing with a config file does not load the weights associated with the model, only the
configuration. Check out the [`~FlaxPreTrainedModel.from_pretrained`] method to load the model weights.
dtype (`jax.numpy.dtype`, *optional*, defaults to `jax.numpy.float32`):
The data type of the computation. Can be one of `jax.numpy.float32`, `jax.numpy.float16` (on GPUs) and
`jax.numpy.bfloat16` (on TPUs).
This can be used to enable mixed-precision training or half-precision inference on GPUs or TPUs. If
specified all the computation will be performed with the given `dtype`.
**Note that this only specifies the dtype of the computation and does not influence the dtype of model
parameters.**
If you wish to change the dtype of the model parameters, see [`~FlaxPreTrainedModel.to_fp16`] and
[`~FlaxPreTrainedModel.to_bf16`].
"""
VIT_INPUTS_DOCSTRING = r"""
Args:
pixel_values (`numpy.ndarray` of shape `(batch_size, num_channels, height, width)`):
Pixel values. Pixel values can be obtained using [`AutoImageProcessor`]. See [`ViTImageProcessor.__call__`]
for details.
output_attentions (`bool`, *optional*):
Whether or not to return the attentions tensors of all attention layers. See `attentions` under returned
tensors for more detail.
output_hidden_states (`bool`, *optional*):
Whether or not to return the hidden states of all layers. See `hidden_states` under returned tensors for
more detail.
return_dict (`bool`, *optional*):
Whether or not to return a [`~utils.ModelOutput`] instead of a plain tuple.
"""
class FlaxViTPatchEmbeddings(nn.Module):
config: ViTConfig
dtype: jnp.dtype = jnp.float32 # the dtype of the computation
def setup(self):
image_size = self.config.image_size
patch_size = self.config.patch_size
num_patches = (image_size // patch_size) * (image_size // patch_size)
self.num_patches = num_patches
self.num_channels = self.config.num_channels
self.projection = nn.Conv(
self.config.hidden_size,
kernel_size=(patch_size, patch_size),
strides=(patch_size, patch_size),
padding="VALID",
dtype=self.dtype,
kernel_init=jax.nn.initializers.variance_scaling(
self.config.initializer_range**2, "fan_in", "truncated_normal"
),
)
def __call__(self, pixel_values):
num_channels = pixel_values.shape[-1]
if num_channels != self.num_channels:
raise ValueError(
"Make sure that the channel dimension of the pixel values match with the one set in the configuration."
)
embeddings = self.projection(pixel_values)
batch_size, _, _, channels = embeddings.shape
return jnp.reshape(embeddings, (batch_size, -1, channels))
class FlaxViTEmbeddings(nn.Module):
"""Construct the CLS token, position and patch embeddings."""
config: ViTConfig
dtype: jnp.dtype = jnp.float32 # the dtype of the computation
def setup(self):
self.cls_token = self.param(
"cls_token",
jax.nn.initializers.variance_scaling(self.config.initializer_range**2, "fan_in", "truncated_normal"),
(1, 1, self.config.hidden_size),
)
self.patch_embeddings = FlaxViTPatchEmbeddings(self.config, dtype=self.dtype)
num_patches = self.patch_embeddings.num_patches
self.position_embeddings = self.param(
"position_embeddings",
jax.nn.initializers.variance_scaling(self.config.initializer_range**2, "fan_in", "truncated_normal"),
(1, num_patches + 1, self.config.hidden_size),
)
self.dropout = nn.Dropout(rate=self.config.hidden_dropout_prob)
def __call__(self, pixel_values, deterministic=True):
batch_size = pixel_values.shape[0]
embeddings = self.patch_embeddings(pixel_values)
cls_tokens = jnp.broadcast_to(self.cls_token, (batch_size, 1, self.config.hidden_size))
embeddings = jnp.concatenate((cls_tokens, embeddings), axis=1)
embeddings = embeddings + self.position_embeddings
embeddings = self.dropout(embeddings, deterministic=deterministic)
return embeddings
class FlaxViTSelfAttention(nn.Module):
config: ViTConfig
dtype: jnp.dtype = jnp.float32 # the dtype of the computation
def setup(self):
if self.config.hidden_size % self.config.num_attention_heads != 0:
raise ValueError(
"`config.hidden_size`: {self.config.hidden_size} has to be a multiple of `config.num_attention_heads`:"
" {self.config.num_attention_heads}"
)
self.query = nn.Dense(
self.config.hidden_size,
dtype=self.dtype,
kernel_init=jax.nn.initializers.variance_scaling(
self.config.initializer_range**2, mode="fan_in", distribution="truncated_normal"
),
use_bias=self.config.qkv_bias,
)
self.key = nn.Dense(
self.config.hidden_size,
dtype=self.dtype,
kernel_init=jax.nn.initializers.variance_scaling(
self.config.initializer_range**2, mode="fan_in", distribution="truncated_normal"
),
use_bias=self.config.qkv_bias,
)
self.value = nn.Dense(
self.config.hidden_size,
dtype=self.dtype,
kernel_init=jax.nn.initializers.variance_scaling(
self.config.initializer_range**2, mode="fan_in", distribution="truncated_normal"
),
use_bias=self.config.qkv_bias,
)
def __call__(self, hidden_states, deterministic: bool = True, output_attentions: bool = False):
head_dim = self.config.hidden_size // self.config.num_attention_heads
query_states = self.query(hidden_states).reshape(
hidden_states.shape[:2] + (self.config.num_attention_heads, head_dim)
)
value_states = self.value(hidden_states).reshape(
hidden_states.shape[:2] + (self.config.num_attention_heads, head_dim)
)
key_states = self.key(hidden_states).reshape(
hidden_states.shape[:2] + (self.config.num_attention_heads, head_dim)
)
dropout_rng = None
if not deterministic and self.config.attention_probs_dropout_prob > 0.0:
dropout_rng = self.make_rng("dropout")
attn_weights = dot_product_attention_weights(
query_states,
key_states,
dropout_rng=dropout_rng,
dropout_rate=self.config.attention_probs_dropout_prob,
broadcast_dropout=True,
deterministic=deterministic,
dtype=self.dtype,
precision=None,
)
attn_output = jnp.einsum("...hqk,...khd->...qhd", attn_weights, value_states)
attn_output = attn_output.reshape(attn_output.shape[:2] + (-1,))
outputs = (attn_output, attn_weights) if output_attentions else (attn_output,)
return outputs
class FlaxViTSelfOutput(nn.Module):
config: ViTConfig
dtype: jnp.dtype = jnp.float32 # the dtype of the computation
def setup(self):
self.dense = nn.Dense(
self.config.hidden_size,
kernel_init=jax.nn.initializers.variance_scaling(
self.config.initializer_range**2, "fan_in", "truncated_normal"
),
dtype=self.dtype,
)
self.dropout = nn.Dropout(rate=self.config.hidden_dropout_prob)
def __call__(self, hidden_states, input_tensor, deterministic: bool = True):
hidden_states = self.dense(hidden_states)
hidden_states = self.dropout(hidden_states, deterministic=deterministic)
return hidden_states
class FlaxViTAttention(nn.Module):
config: ViTConfig
dtype: jnp.dtype = jnp.float32
def setup(self):
self.attention = FlaxViTSelfAttention(self.config, dtype=self.dtype)
self.output = FlaxViTSelfOutput(self.config, dtype=self.dtype)
def __call__(self, hidden_states, deterministic=True, output_attentions: bool = False):
attn_outputs = self.attention(hidden_states, deterministic=deterministic, output_attentions=output_attentions)
attn_output = attn_outputs[0]
hidden_states = self.output(attn_output, hidden_states, deterministic=deterministic)
outputs = (hidden_states,)
if output_attentions:
outputs += (attn_outputs[1],)
return outputs
class FlaxViTIntermediate(nn.Module):
config: ViTConfig
dtype: jnp.dtype = jnp.float32 # the dtype of the computation
def setup(self):
self.dense = nn.Dense(
self.config.intermediate_size,
kernel_init=jax.nn.initializers.variance_scaling(
self.config.initializer_range**2, "fan_in", "truncated_normal"
),
dtype=self.dtype,
)
self.activation = ACT2FN[self.config.hidden_act]
def __call__(self, hidden_states):
hidden_states = self.dense(hidden_states)
hidden_states = self.activation(hidden_states)
return hidden_states
class FlaxViTOutput(nn.Module):
config: ViTConfig
dtype: jnp.dtype = jnp.float32 # the dtype of the computation
def setup(self):
self.dense = nn.Dense(
self.config.hidden_size,
kernel_init=jax.nn.initializers.variance_scaling(
self.config.initializer_range**2, "fan_in", "truncated_normal"
),
dtype=self.dtype,
)
self.dropout = nn.Dropout(rate=self.config.hidden_dropout_prob)
def __call__(self, hidden_states, attention_output, deterministic: bool = True):
hidden_states = self.dense(hidden_states)
hidden_states = self.dropout(hidden_states, deterministic=deterministic)
hidden_states = hidden_states + attention_output
return hidden_states
class FlaxViTLayer(nn.Module):
config: ViTConfig
dtype: jnp.dtype = jnp.float32 # the dtype of the computation
def setup(self):
self.attention = FlaxViTAttention(self.config, dtype=self.dtype)
self.intermediate = FlaxViTIntermediate(self.config, dtype=self.dtype)
self.output = FlaxViTOutput(self.config, dtype=self.dtype)
self.layernorm_before = nn.LayerNorm(epsilon=self.config.layer_norm_eps, dtype=self.dtype)
self.layernorm_after = nn.LayerNorm(epsilon=self.config.layer_norm_eps, dtype=self.dtype)
def __call__(self, hidden_states, deterministic: bool = True, output_attentions: bool = False):
attention_outputs = self.attention(
self.layernorm_before(hidden_states), # in ViT, layernorm is applied before self-attention
deterministic=deterministic,
output_attentions=output_attentions,
)
attention_output = attention_outputs[0]
# first residual connection
attention_output = attention_output + hidden_states
# in ViT, layernorm is also applied after self-attention
layer_output = self.layernorm_after(attention_output)
hidden_states = self.intermediate(layer_output)
hidden_states = self.output(hidden_states, attention_output, deterministic=deterministic)
outputs = (hidden_states,)
if output_attentions:
outputs += (attention_outputs[1],)
return outputs
class FlaxViTLayerCollection(nn.Module):
config: ViTConfig
dtype: jnp.dtype = jnp.float32 # the dtype of the computation
def setup(self):
self.layers = [
FlaxViTLayer(self.config, name=str(i), dtype=self.dtype) for i in range(self.config.num_hidden_layers)
]
def __call__(
self,
hidden_states,
deterministic: bool = True,
output_attentions: bool = False,
output_hidden_states: bool = False,
return_dict: bool = True,
):
all_attentions = () if output_attentions else None
all_hidden_states = () if output_hidden_states else None
for i, layer in enumerate(self.layers):
if output_hidden_states:
all_hidden_states += (hidden_states,)
layer_outputs = layer(hidden_states, deterministic=deterministic, output_attentions=output_attentions)
hidden_states = layer_outputs[0]
if output_attentions:
all_attentions += (layer_outputs[1],)
if output_hidden_states:
all_hidden_states += (hidden_states,)
outputs = (hidden_states,)
if not return_dict:
return tuple(v for v in outputs if v is not None)
return FlaxBaseModelOutput(
last_hidden_state=hidden_states, hidden_states=all_hidden_states, attentions=all_attentions
)
class FlaxViTEncoder(nn.Module):
config: ViTConfig
dtype: jnp.dtype = jnp.float32 # the dtype of the computation
def setup(self):
self.layer = FlaxViTLayerCollection(self.config, dtype=self.dtype)
def __call__(
self,
hidden_states,
deterministic: bool = True,
output_attentions: bool = False,
output_hidden_states: bool = False,
return_dict: bool = True,
):
return self.layer(
hidden_states,
deterministic=deterministic,
output_attentions=output_attentions,
output_hidden_states=output_hidden_states,
return_dict=return_dict,
)
class FlaxViTPooler(nn.Module):
config: ViTConfig
dtype: jnp.dtype = jnp.float32 # the dtype of the computation
def setup(self):
self.dense = nn.Dense(
self.config.hidden_size,
kernel_init=jax.nn.initializers.variance_scaling(
self.config.initializer_range**2, "fan_in", "truncated_normal"
),
dtype=self.dtype,
)
def __call__(self, hidden_states):
cls_hidden_state = hidden_states[:, 0]
cls_hidden_state = self.dense(cls_hidden_state)
return nn.tanh(cls_hidden_state)
class FlaxViTPreTrainedModel(FlaxPreTrainedModel):
"""
An abstract class to handle weights initialization and a simple interface for downloading and loading pretrained
models.
"""
config_class = ViTConfig
base_model_prefix = "vit"
main_input_name = "pixel_values"
module_class: nn.Module = None
def __init__(
self,
config: ViTConfig,
input_shape=None,
seed: int = 0,
dtype: jnp.dtype = jnp.float32,
_do_init: bool = True,
**kwargs,
):
module = self.module_class(config=config, dtype=dtype, **kwargs)
if input_shape is None:
input_shape = (1, config.image_size, config.image_size, config.num_channels)
super().__init__(config, module, input_shape=input_shape, seed=seed, dtype=dtype, _do_init=_do_init)
def init_weights(self, rng: jax.random.PRNGKey, input_shape: Tuple, params: FrozenDict = None) -> FrozenDict:
# init input tensors
pixel_values = jnp.zeros(input_shape, dtype=self.dtype)
params_rng, dropout_rng = jax.random.split(rng)
rngs = {"params": params_rng, "dropout": dropout_rng}
random_params = self.module.init(rngs, pixel_values, return_dict=False)["params"]
if params is not None:
random_params = flatten_dict(unfreeze(random_params))
params = flatten_dict(unfreeze(params))
for missing_key in self._missing_keys:
params[missing_key] = random_params[missing_key]
self._missing_keys = set()
return freeze(unflatten_dict(params))
else:
return random_params
@add_start_docstrings_to_model_forward(VIT_INPUTS_DOCSTRING.format("batch_size, sequence_length"))
def __call__(
self,
pixel_values,
params: dict = None,
dropout_rng: jax.random.PRNGKey = None,
train: bool = False,
output_attentions: Optional[bool] = None,
output_hidden_states: Optional[bool] = None,
return_dict: Optional[bool] = None,
):
output_attentions = output_attentions if output_attentions is not None else self.config.output_attentions
output_hidden_states = (
output_hidden_states if output_hidden_states is not None else self.config.output_hidden_states
)
return_dict = return_dict if return_dict is not None else self.config.return_dict
pixel_values = jnp.transpose(pixel_values, (0, 2, 3, 1))
# Handle any PRNG if needed
rngs = {}
if dropout_rng is not None:
rngs["dropout"] = dropout_rng
return self.module.apply(
{"params": params or self.params},
jnp.array(pixel_values, dtype=jnp.float32),
not train,
output_attentions,
output_hidden_states,
return_dict,
rngs=rngs,
)
class FlaxViTModule(nn.Module):
config: ViTConfig
dtype: jnp.dtype = jnp.float32 # the dtype of the computation
add_pooling_layer: bool = True
def setup(self):
self.embeddings = FlaxViTEmbeddings(self.config, dtype=self.dtype)
self.encoder = FlaxViTEncoder(self.config, dtype=self.dtype)
self.layernorm = nn.LayerNorm(epsilon=self.config.layer_norm_eps, dtype=self.dtype)
self.pooler = FlaxViTPooler(self.config, dtype=self.dtype) if self.add_pooling_layer else None
def __call__(
self,
pixel_values,
deterministic: bool = True,
output_attentions: bool = False,
output_hidden_states: bool = False,
return_dict: bool = True,
):
hidden_states = self.embeddings(pixel_values, deterministic=deterministic)
outputs = self.encoder(
hidden_states,
deterministic=deterministic,
output_attentions=output_attentions,
output_hidden_states=output_hidden_states,
return_dict=return_dict,
)
hidden_states = outputs[0]
hidden_states = self.layernorm(hidden_states)
pooled = self.pooler(hidden_states) if self.add_pooling_layer else None
if not return_dict:
# if pooled is None, don't return it
if pooled is None:
return (hidden_states,) + outputs[1:]
return (hidden_states, pooled) + outputs[1:]
return FlaxBaseModelOutputWithPooling(
last_hidden_state=hidden_states,
pooler_output=pooled,
hidden_states=outputs.hidden_states,
attentions=outputs.attentions,
)
@add_start_docstrings(
"The bare ViT Model transformer outputting raw hidden-states without any specific head on top.",
VIT_START_DOCSTRING,
)
class FlaxViTModel(FlaxViTPreTrainedModel):
module_class = FlaxViTModule
FLAX_VISION_MODEL_DOCSTRING = """
Returns:
Examples:
```python
>>> from transformers import AutoImageProcessor, FlaxViTModel
>>> from PIL import Image
>>> import requests
>>> url = "http://images.cocodataset.org/val2017/000000039769.jpg"
>>> image = Image.open(requests.get(url, stream=True).raw)
>>> image_processor = AutoImageProcessor.from_pretrained("google/vit-base-patch16-224-in21k")
>>> model = FlaxViTModel.from_pretrained("google/vit-base-patch16-224-in21k")
>>> inputs = image_processor(images=image, return_tensors="np")
>>> outputs = model(**inputs)
>>> last_hidden_states = outputs.last_hidden_state
```
"""
overwrite_call_docstring(FlaxViTModel, FLAX_VISION_MODEL_DOCSTRING)
append_replace_return_docstrings(FlaxViTModel, output_type=FlaxBaseModelOutputWithPooling, config_class=ViTConfig)
class FlaxViTForImageClassificationModule(nn.Module):
config: ViTConfig
dtype: jnp.dtype = jnp.float32
def setup(self):
self.vit = FlaxViTModule(config=self.config, dtype=self.dtype, add_pooling_layer=False)
self.classifier = nn.Dense(
self.config.num_labels,
dtype=self.dtype,
kernel_init=jax.nn.initializers.variance_scaling(
self.config.initializer_range**2, "fan_in", "truncated_normal"
),
)
def __call__(
self,
pixel_values=None,
deterministic: bool = True,
output_attentions=None,
output_hidden_states=None,
return_dict=None,
):
return_dict = return_dict if return_dict is not None else self.config.use_return_dict
outputs = self.vit(
pixel_values,
deterministic=deterministic,
output_attentions=output_attentions,
output_hidden_states=output_hidden_states,
return_dict=return_dict,
)
hidden_states = outputs[0]
logits = self.classifier(hidden_states[:, 0, :])
if not return_dict:
output = (logits,) + outputs[2:]
return output
return FlaxSequenceClassifierOutput(
logits=logits,
hidden_states=outputs.hidden_states,
attentions=outputs.attentions,
)
@add_start_docstrings(
"""
ViT Model transformer with an image classification head on top (a linear layer on top of the final hidden state of
the [CLS] token) e.g. for ImageNet.
""",
VIT_START_DOCSTRING,
)
class FlaxViTForImageClassification(FlaxViTPreTrainedModel):
module_class = FlaxViTForImageClassificationModule
FLAX_VISION_CLASSIF_DOCSTRING = """
Returns:
Example:
```python
>>> from transformers import AutoImageProcessor, FlaxViTForImageClassification
>>> from PIL import Image
>>> import jax
>>> import requests
>>> url = "http://images.cocodataset.org/val2017/000000039769.jpg"
>>> image = Image.open(requests.get(url, stream=True).raw)
>>> image_processor = AutoImageProcessor.from_pretrained("google/vit-base-patch16-224")
>>> model = FlaxViTForImageClassification.from_pretrained("google/vit-base-patch16-224")
>>> inputs = image_processor(images=image, return_tensors="np")
>>> outputs = model(**inputs)
>>> logits = outputs.logits
>>> # model predicts one of the 1000 ImageNet classes
>>> predicted_class_idx = jax.numpy.argmax(logits, axis=-1)
>>> print("Predicted class:", model.config.id2label[predicted_class_idx.item()])
```
"""
overwrite_call_docstring(FlaxViTForImageClassification, FLAX_VISION_CLASSIF_DOCSTRING)
append_replace_return_docstrings(
FlaxViTForImageClassification, output_type=FlaxSequenceClassifierOutput, config_class=ViTConfig
)
| transformers/src/transformers/models/vit/modeling_flax_vit.py/0 | {
"file_path": "transformers/src/transformers/models/vit/modeling_flax_vit.py",
"repo_id": "transformers",
"token_count": 10847
} | 370 |
Subsets and Splits
No community queries yet
The top public SQL queries from the community will appear here once available.